- University Libraries
- Research Guides
- Topic Guides
- Research Methods Guide
- Data Analysis

Research Methods Guide: Data Analysis
- Introduction
- Research Design & Method
- Survey Research
- Interview Research
- Resources & Consultation
Tools for Analyzing Survey Data
- R (open source)
- Stata
- DataCracker (free up to 100 responses per survey)
- SurveyMonkey (free up to 100 responses per survey)
Tools for Analyzing Interview Data
- AQUAD (open source)
- NVivo
Data Analysis and Presentation Techniques that Apply to both Survey and Interview Research
- Create a documentation of the data and the process of data collection.
- Analyze the data rather than just describing it - use it to tell a story that focuses on answering the research question.
- Use charts or tables to help the reader understand the data and then highlight the most interesting findings.
- Don’t get bogged down in the detail - tell the reader about the main themes as they relate to the research question, rather than reporting everything that survey respondents or interviewees said.
- State that ‘most people said …’ or ‘few people felt …’ rather than giving the number of people who said a particular thing.
- Use brief quotes where these illustrate a particular point really well.
- Respect confidentiality - you could attribute a quote to 'a faculty member', ‘a student’, or 'a customer' rather than ‘Dr. Nicholls.'
Survey Data Analysis
- If you used an online survey, the software will automatically collate the data – you will just need to download the data, for example as a spreadsheet.
- If you used a paper questionnaire, you will need to manually transfer the responses from the questionnaires into a spreadsheet. Put each question number as a column heading, and use one row for each person’s answers. Then assign each possible answer a number or ‘code’.
- When all the data is present and correct, calculate how many people selected each response.
- Once you have calculated how many people selected each response, you can set up tables and/or graph to display the data. This could take the form of a table or chart.
- In addition to descriptive statistics that characterize findings from your survey, you can use statistical and analytical reporting techniques if needed.
Interview Data Analysis
- Data Reduction and Organization: Try not to feel overwhelmed by quantity of information that has been collected from interviews- a one-hour interview can generate 20 to 25 pages of single-spaced text. Once you start organizing your fieldwork notes around themes, you can easily identify which part of your data to be used for further analysis.
- What were the main issues or themes that struck you in this contact / interviewee?"
- Was there anything else that struck you as salient, interesting, illuminating or important in this contact / interviewee?
- What information did you get (or failed to get) on each of the target questions you had for this contact / interviewee?
- Connection of the data: You can connect data around themes and concepts - then you can show how one concept may influence another.
- Examination of Relationships: Examining relationships is the centerpiece of the analytic process, because it allows you to move from simple description of the people and settings to explanations of why things happened as they did with those people in that setting.
- << Previous: Interview Research
- Next: Resources & Consultation >>
- Last Updated: Aug 21, 2023 10:42 AM
- Skip to main content
- Skip to primary sidebar
- Skip to footer
- QuestionPro

- Solutions Industries Gaming Automotive Sports and events Education Government Travel & Hospitality Financial Services Healthcare Cannabis Technology Use Case NPS+ Communities Audience Contactless surveys Mobile LivePolls Member Experience GDPR Positive People Science 360 Feedback Surveys
- Resources Blog eBooks Survey Templates Case Studies Training Help center

Home Market Research
Data Analysis in Research: Types & Methods
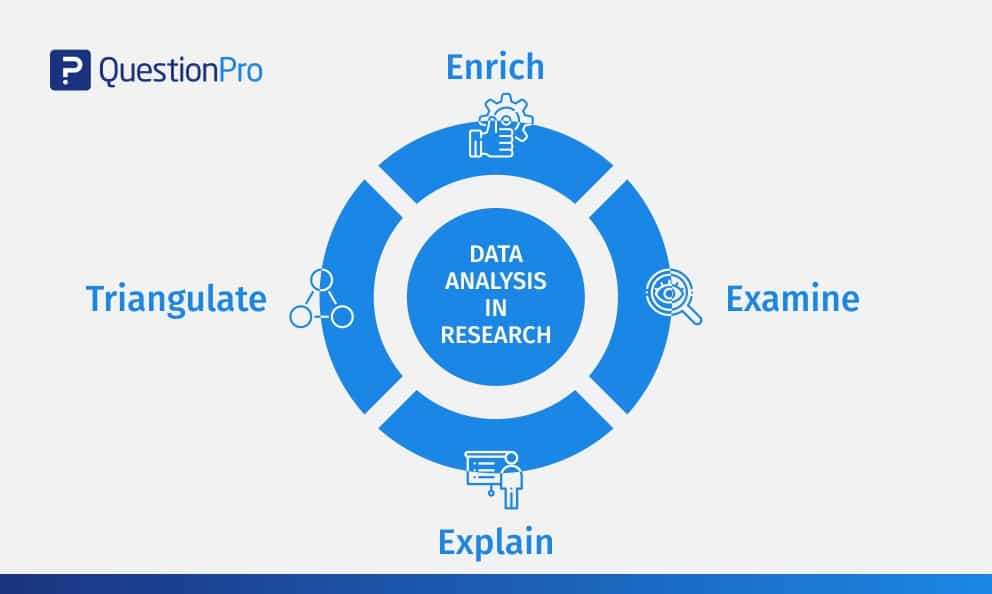
Content Index
Why analyze data in research?
Types of data in research, finding patterns in the qualitative data, methods used for data analysis in qualitative research, preparing data for analysis, methods used for data analysis in quantitative research, considerations in research data analysis, what is data analysis in research.
Definition of research in data analysis: According to LeCompte and Schensul, research data analysis is a process used by researchers to reduce data to a story and interpret it to derive insights. The data analysis process helps reduce a large chunk of data into smaller fragments, which makes sense.
Three essential things occur during the data analysis process — the first is data organization . Summarization and categorization together contribute to becoming the second known method used for data reduction. It helps find patterns and themes in the data for easy identification and linking. The third and last way is data analysis – researchers do it in both top-down and bottom-up fashion.
LEARN ABOUT: Research Process Steps
On the other hand, Marshall and Rossman describe data analysis as a messy, ambiguous, and time-consuming but creative and fascinating process through which a mass of collected data is brought to order, structure and meaning.
We can say that “the data analysis and data interpretation is a process representing the application of deductive and inductive logic to the research and data analysis.”
Researchers rely heavily on data as they have a story to tell or research problems to solve. It starts with a question, and data is nothing but an answer to that question. But, what if there is no question to ask? Well! It is possible to explore data even without a problem – we call it ‘Data Mining’, which often reveals some interesting patterns within the data that are worth exploring.
Irrelevant to the type of data researchers explore, their mission and audiences’ vision guide them to find the patterns to shape the story they want to tell. One of the essential things expected from researchers while analyzing data is to stay open and remain unbiased toward unexpected patterns, expressions, and results. Remember, sometimes, data analysis tells the most unforeseen yet exciting stories that were not expected when initiating data analysis. Therefore, rely on the data you have at hand and enjoy the journey of exploratory research.
Create a Free Account
Every kind of data has a rare quality of describing things after assigning a specific value to it. For analysis, you need to organize these values, processed and presented in a given context, to make it useful. Data can be in different forms; here are the primary data types.
- Qualitative data: When the data presented has words and descriptions, then we call it qualitative data . Although you can observe this data, it is subjective and harder to analyze data in research, especially for comparison. Example: Quality data represents everything describing taste, experience, texture, or an opinion that is considered quality data. This type of data is usually collected through focus groups, personal qualitative interviews , qualitative observation or using open-ended questions in surveys.
- Quantitative data: Any data expressed in numbers of numerical figures are called quantitative data . This type of data can be distinguished into categories, grouped, measured, calculated, or ranked. Example: questions such as age, rank, cost, length, weight, scores, etc. everything comes under this type of data. You can present such data in graphical format, charts, or apply statistical analysis methods to this data. The (Outcomes Measurement Systems) OMS questionnaires in surveys are a significant source of collecting numeric data.
- Categorical data: It is data presented in groups. However, an item included in the categorical data cannot belong to more than one group. Example: A person responding to a survey by telling his living style, marital status, smoking habit, or drinking habit comes under the categorical data. A chi-square test is a standard method used to analyze this data.
Learn More : Examples of Qualitative Data in Education
Data analysis in qualitative research
Data analysis and qualitative data research work a little differently from the numerical data as the quality data is made up of words, descriptions, images, objects, and sometimes symbols. Getting insight from such complicated information is a complicated process. Hence it is typically used for exploratory research and data analysis .
Although there are several ways to find patterns in the textual information, a word-based method is the most relied and widely used global technique for research and data analysis. Notably, the data analysis process in qualitative research is manual. Here the researchers usually read the available data and find repetitive or commonly used words.
For example, while studying data collected from African countries to understand the most pressing issues people face, researchers might find “food” and “hunger” are the most commonly used words and will highlight them for further analysis.
LEARN ABOUT: Level of Analysis
The keyword context is another widely used word-based technique. In this method, the researcher tries to understand the concept by analyzing the context in which the participants use a particular keyword.
For example , researchers conducting research and data analysis for studying the concept of ‘diabetes’ amongst respondents might analyze the context of when and how the respondent has used or referred to the word ‘diabetes.’
The scrutiny-based technique is also one of the highly recommended text analysis methods used to identify a quality data pattern. Compare and contrast is the widely used method under this technique to differentiate how a specific text is similar or different from each other.
For example: To find out the “importance of resident doctor in a company,” the collected data is divided into people who think it is necessary to hire a resident doctor and those who think it is unnecessary. Compare and contrast is the best method that can be used to analyze the polls having single-answer questions types .
Metaphors can be used to reduce the data pile and find patterns in it so that it becomes easier to connect data with theory.
Variable Partitioning is another technique used to split variables so that researchers can find more coherent descriptions and explanations from the enormous data.
LEARN ABOUT: Qualitative Research Questions and Questionnaires
There are several techniques to analyze the data in qualitative research, but here are some commonly used methods,
- Content Analysis: It is widely accepted and the most frequently employed technique for data analysis in research methodology. It can be used to analyze the documented information from text, images, and sometimes from the physical items. It depends on the research questions to predict when and where to use this method.
- Narrative Analysis: This method is used to analyze content gathered from various sources such as personal interviews, field observation, and surveys . The majority of times, stories, or opinions shared by people are focused on finding answers to the research questions.
- Discourse Analysis: Similar to narrative analysis, discourse analysis is used to analyze the interactions with people. Nevertheless, this particular method considers the social context under which or within which the communication between the researcher and respondent takes place. In addition to that, discourse analysis also focuses on the lifestyle and day-to-day environment while deriving any conclusion.
- Grounded Theory: When you want to explain why a particular phenomenon happened, then using grounded theory for analyzing quality data is the best resort. Grounded theory is applied to study data about the host of similar cases occurring in different settings. When researchers are using this method, they might alter explanations or produce new ones until they arrive at some conclusion.
LEARN ABOUT: 12 Best Tools for Researchers
Data analysis in quantitative research
The first stage in research and data analysis is to make it for the analysis so that the nominal data can be converted into something meaningful. Data preparation consists of the below phases.
Phase I: Data Validation
Data validation is done to understand if the collected data sample is per the pre-set standards, or it is a biased data sample again divided into four different stages
- Fraud: To ensure an actual human being records each response to the survey or the questionnaire
- Screening: To make sure each participant or respondent is selected or chosen in compliance with the research criteria
- Procedure: To ensure ethical standards were maintained while collecting the data sample
- Completeness: To ensure that the respondent has answered all the questions in an online survey. Else, the interviewer had asked all the questions devised in the questionnaire.
Phase II: Data Editing
More often, an extensive research data sample comes loaded with errors. Respondents sometimes fill in some fields incorrectly or sometimes skip them accidentally. Data editing is a process wherein the researchers have to confirm that the provided data is free of such errors. They need to conduct necessary checks and outlier checks to edit the raw edit and make it ready for analysis.
Phase III: Data Coding
Out of all three, this is the most critical phase of data preparation associated with grouping and assigning values to the survey responses . If a survey is completed with a 1000 sample size, the researcher will create an age bracket to distinguish the respondents based on their age. Thus, it becomes easier to analyze small data buckets rather than deal with the massive data pile.
LEARN ABOUT: Steps in Qualitative Research
After the data is prepared for analysis, researchers are open to using different research and data analysis methods to derive meaningful insights. For sure, statistical analysis plans are the most favored to analyze numerical data. In statistical analysis, distinguishing between categorical data and numerical data is essential, as categorical data involves distinct categories or labels, while numerical data consists of measurable quantities. The method is again classified into two groups. First, ‘Descriptive Statistics’ used to describe data. Second, ‘Inferential statistics’ that helps in comparing the data .
Descriptive statistics
This method is used to describe the basic features of versatile types of data in research. It presents the data in such a meaningful way that pattern in the data starts making sense. Nevertheless, the descriptive analysis does not go beyond making conclusions. The conclusions are again based on the hypothesis researchers have formulated so far. Here are a few major types of descriptive analysis methods.
Measures of Frequency
- Count, Percent, Frequency
- It is used to denote home often a particular event occurs.
- Researchers use it when they want to showcase how often a response is given.
Measures of Central Tendency
- Mean, Median, Mode
- The method is widely used to demonstrate distribution by various points.
- Researchers use this method when they want to showcase the most commonly or averagely indicated response.
Measures of Dispersion or Variation
- Range, Variance, Standard deviation
- Here the field equals high/low points.
- Variance standard deviation = difference between the observed score and mean
- It is used to identify the spread of scores by stating intervals.
- Researchers use this method to showcase data spread out. It helps them identify the depth until which the data is spread out that it directly affects the mean.
Measures of Position
- Percentile ranks, Quartile ranks
- It relies on standardized scores helping researchers to identify the relationship between different scores.
- It is often used when researchers want to compare scores with the average count.
For quantitative research use of descriptive analysis often give absolute numbers, but the in-depth analysis is never sufficient to demonstrate the rationale behind those numbers. Nevertheless, it is necessary to think of the best method for research and data analysis suiting your survey questionnaire and what story researchers want to tell. For example, the mean is the best way to demonstrate the students’ average scores in schools. It is better to rely on the descriptive statistics when the researchers intend to keep the research or outcome limited to the provided sample without generalizing it. For example, when you want to compare average voting done in two different cities, differential statistics are enough.
Descriptive analysis is also called a ‘univariate analysis’ since it is commonly used to analyze a single variable.
Inferential statistics
Inferential statistics are used to make predictions about a larger population after research and data analysis of the representing population’s collected sample. For example, you can ask some odd 100 audiences at a movie theater if they like the movie they are watching. Researchers then use inferential statistics on the collected sample to reason that about 80-90% of people like the movie.
Here are two significant areas of inferential statistics.
- Estimating parameters: It takes statistics from the sample research data and demonstrates something about the population parameter.
- Hypothesis test: I t’s about sampling research data to answer the survey research questions. For example, researchers might be interested to understand if the new shade of lipstick recently launched is good or not, or if the multivitamin capsules help children to perform better at games.
These are sophisticated analysis methods used to showcase the relationship between different variables instead of describing a single variable. It is often used when researchers want something beyond absolute numbers to understand the relationship between variables.
Here are some of the commonly used methods for data analysis in research.
- Correlation: When researchers are not conducting experimental research or quasi-experimental research wherein the researchers are interested to understand the relationship between two or more variables, they opt for correlational research methods.
- Cross-tabulation: Also called contingency tables, cross-tabulation is used to analyze the relationship between multiple variables. Suppose provided data has age and gender categories presented in rows and columns. A two-dimensional cross-tabulation helps for seamless data analysis and research by showing the number of males and females in each age category.
- Regression analysis: For understanding the strong relationship between two variables, researchers do not look beyond the primary and commonly used regression analysis method, which is also a type of predictive analysis used. In this method, you have an essential factor called the dependent variable. You also have multiple independent variables in regression analysis. You undertake efforts to find out the impact of independent variables on the dependent variable. The values of both independent and dependent variables are assumed as being ascertained in an error-free random manner.
- Frequency tables: The statistical procedure is used for testing the degree to which two or more vary or differ in an experiment. A considerable degree of variation means research findings were significant. In many contexts, ANOVA testing and variance analysis are similar.
- Analysis of variance: The statistical procedure is used for testing the degree to which two or more vary or differ in an experiment. A considerable degree of variation means research findings were significant. In many contexts, ANOVA testing and variance analysis are similar.
- Researchers must have the necessary research skills to analyze and manipulation the data , Getting trained to demonstrate a high standard of research practice. Ideally, researchers must possess more than a basic understanding of the rationale of selecting one statistical method over the other to obtain better data insights.
- Usually, research and data analytics projects differ by scientific discipline; therefore, getting statistical advice at the beginning of analysis helps design a survey questionnaire, select data collection methods , and choose samples.
LEARN ABOUT: Best Data Collection Tools
- The primary aim of data research and analysis is to derive ultimate insights that are unbiased. Any mistake in or keeping a biased mind to collect data, selecting an analysis method, or choosing audience sample il to draw a biased inference.
- Irrelevant to the sophistication used in research data and analysis is enough to rectify the poorly defined objective outcome measurements. It does not matter if the design is at fault or intentions are not clear, but lack of clarity might mislead readers, so avoid the practice.
- The motive behind data analysis in research is to present accurate and reliable data. As far as possible, avoid statistical errors, and find a way to deal with everyday challenges like outliers, missing data, data altering, data mining , or developing graphical representation.
LEARN MORE: Descriptive Research vs Correlational Research The sheer amount of data generated daily is frightening. Especially when data analysis has taken center stage. in 2018. In last year, the total data supply amounted to 2.8 trillion gigabytes. Hence, it is clear that the enterprises willing to survive in the hypercompetitive world must possess an excellent capability to analyze complex research data, derive actionable insights, and adapt to the new market needs.
LEARN ABOUT: Average Order Value
QuestionPro is an online survey platform that empowers organizations in data analysis and research and provides them a medium to collect data by creating appealing surveys.
MORE LIKE THIS
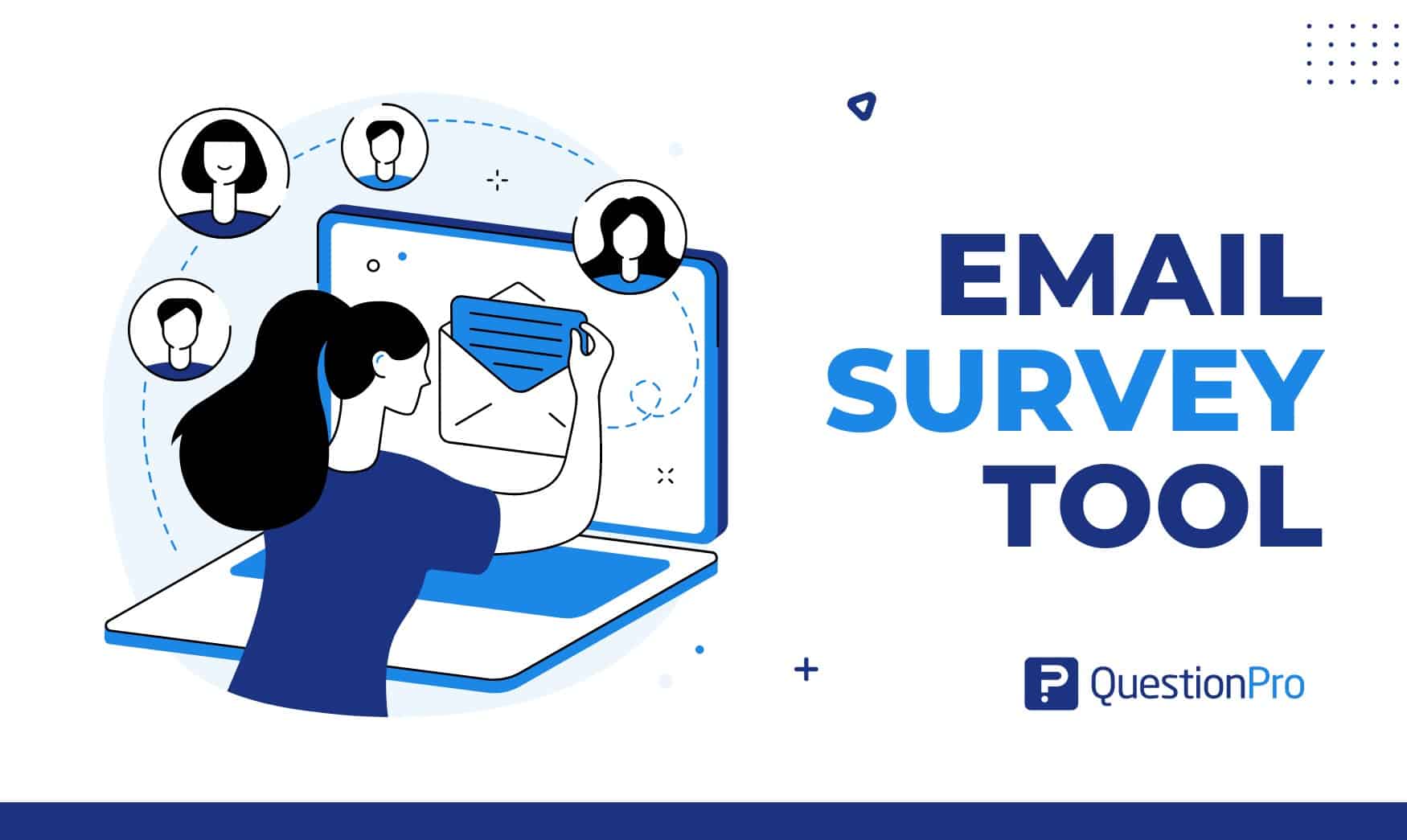
The Best Email Survey Tool to Boost Your Feedback Game
May 7, 2024
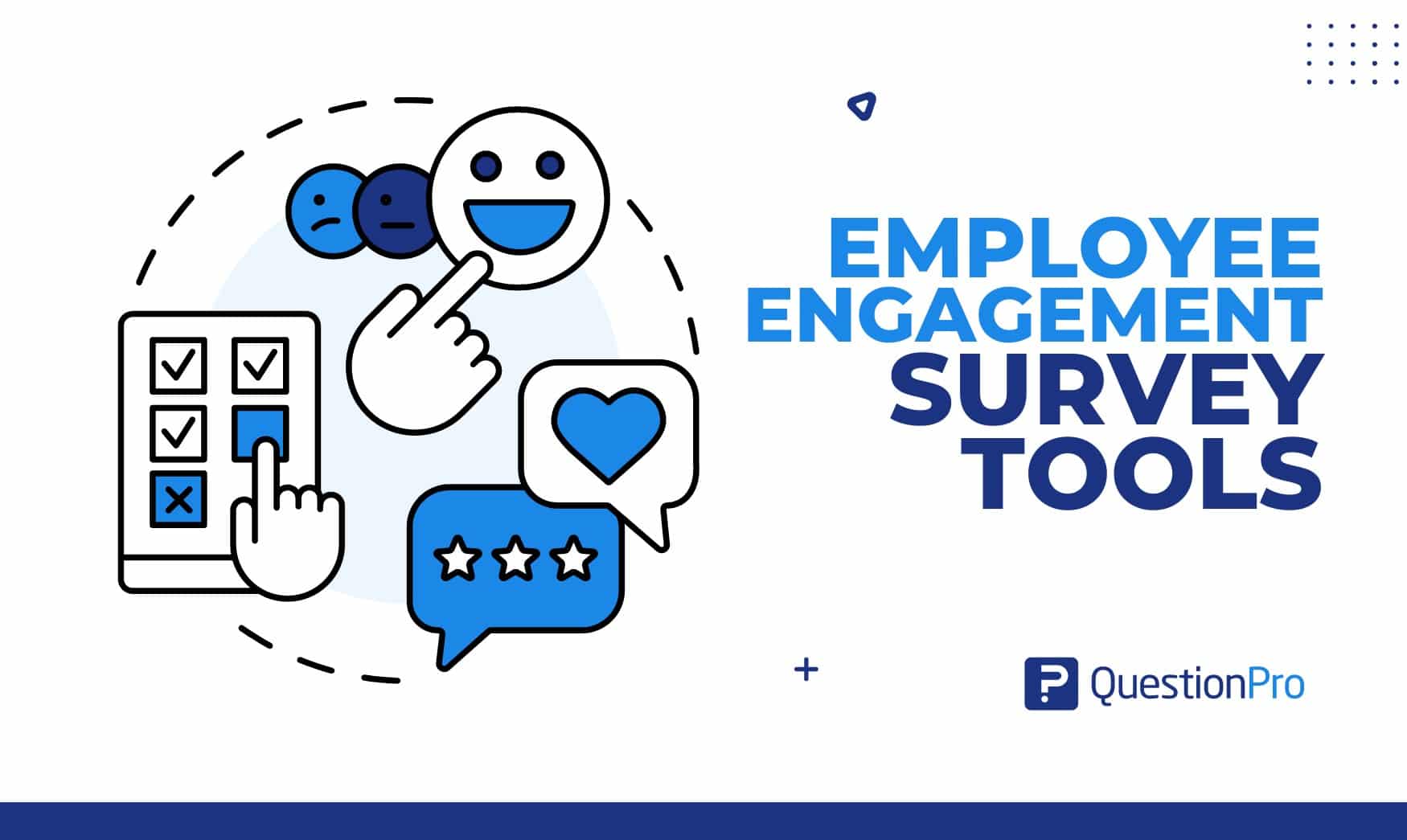
Top 10 Employee Engagement Survey Tools
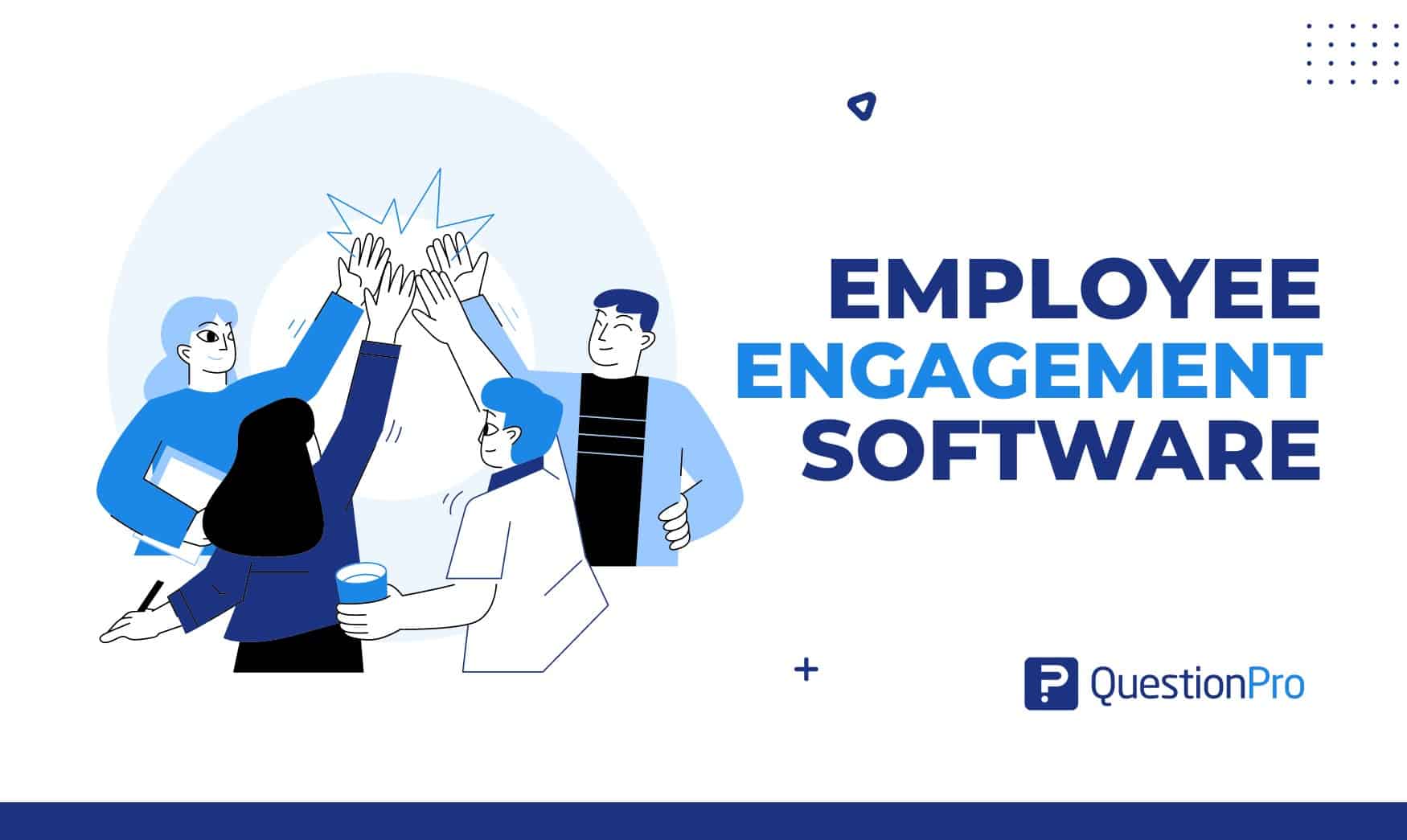
Top 20 Employee Engagement Software Solutions
May 3, 2024
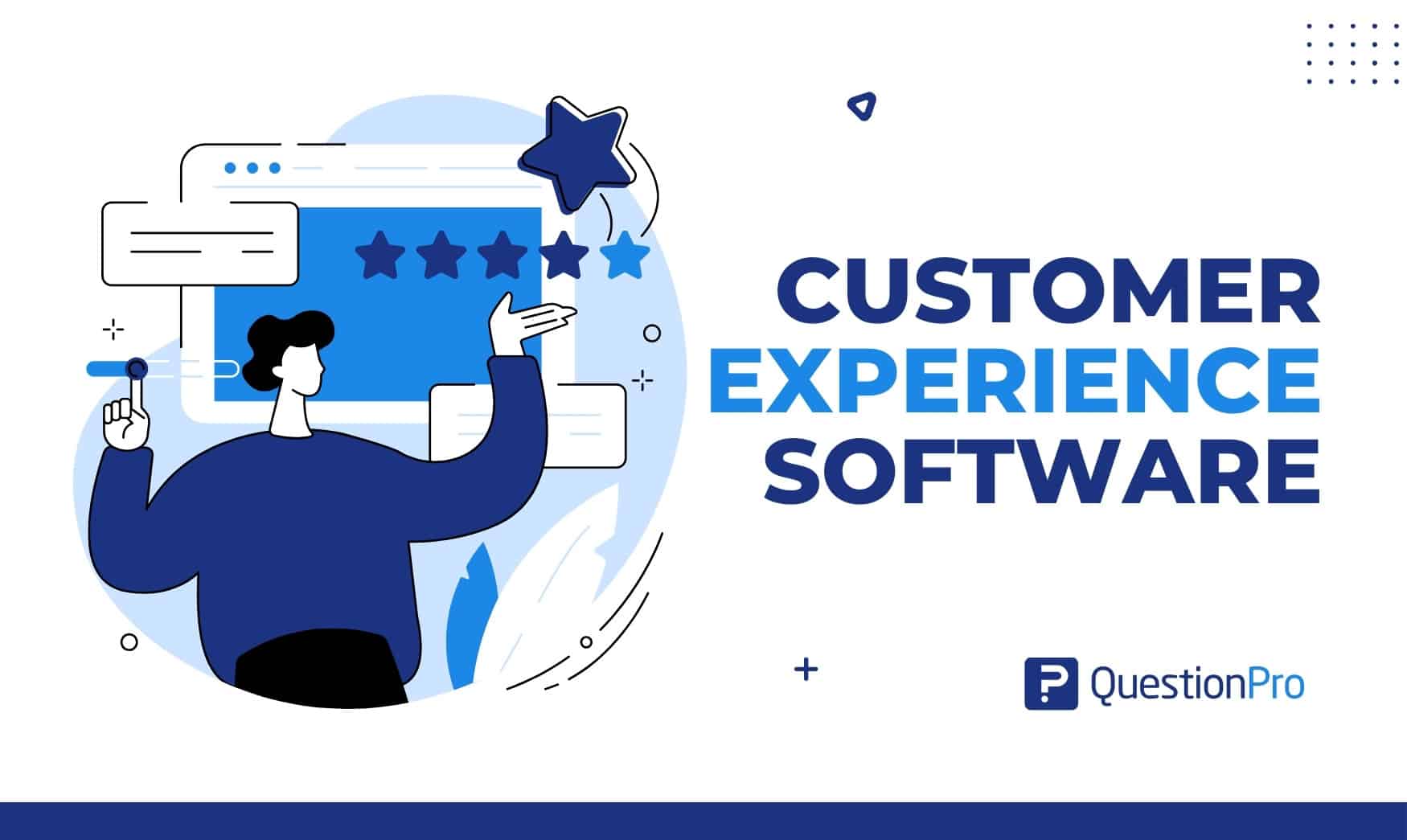
15 Best Customer Experience Software of 2024
May 2, 2024
Other categories
- Academic Research
- Artificial Intelligence
- Assessments
- Brand Awareness
- Case Studies
- Communities
- Consumer Insights
- Customer effort score
- Customer Engagement
- Customer Experience
- Customer Loyalty
- Customer Research
- Customer Satisfaction
- Employee Benefits
- Employee Engagement
- Employee Retention
- Friday Five
- General Data Protection Regulation
- Insights Hub
- Life@QuestionPro
- Market Research
- Mobile diaries
- Mobile Surveys
- New Features
- Online Communities
- Question Types
- Questionnaire
- QuestionPro Products
- Release Notes
- Research Tools and Apps
- Revenue at Risk
- Survey Templates
- Training Tips
- Uncategorized
- Video Learning Series
- What’s Coming Up
- Workforce Intelligence
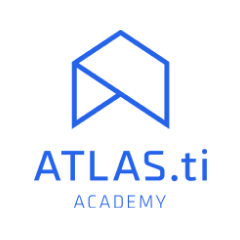
Level up your expertise with our growing collection of guides to qualitative research and analysis.
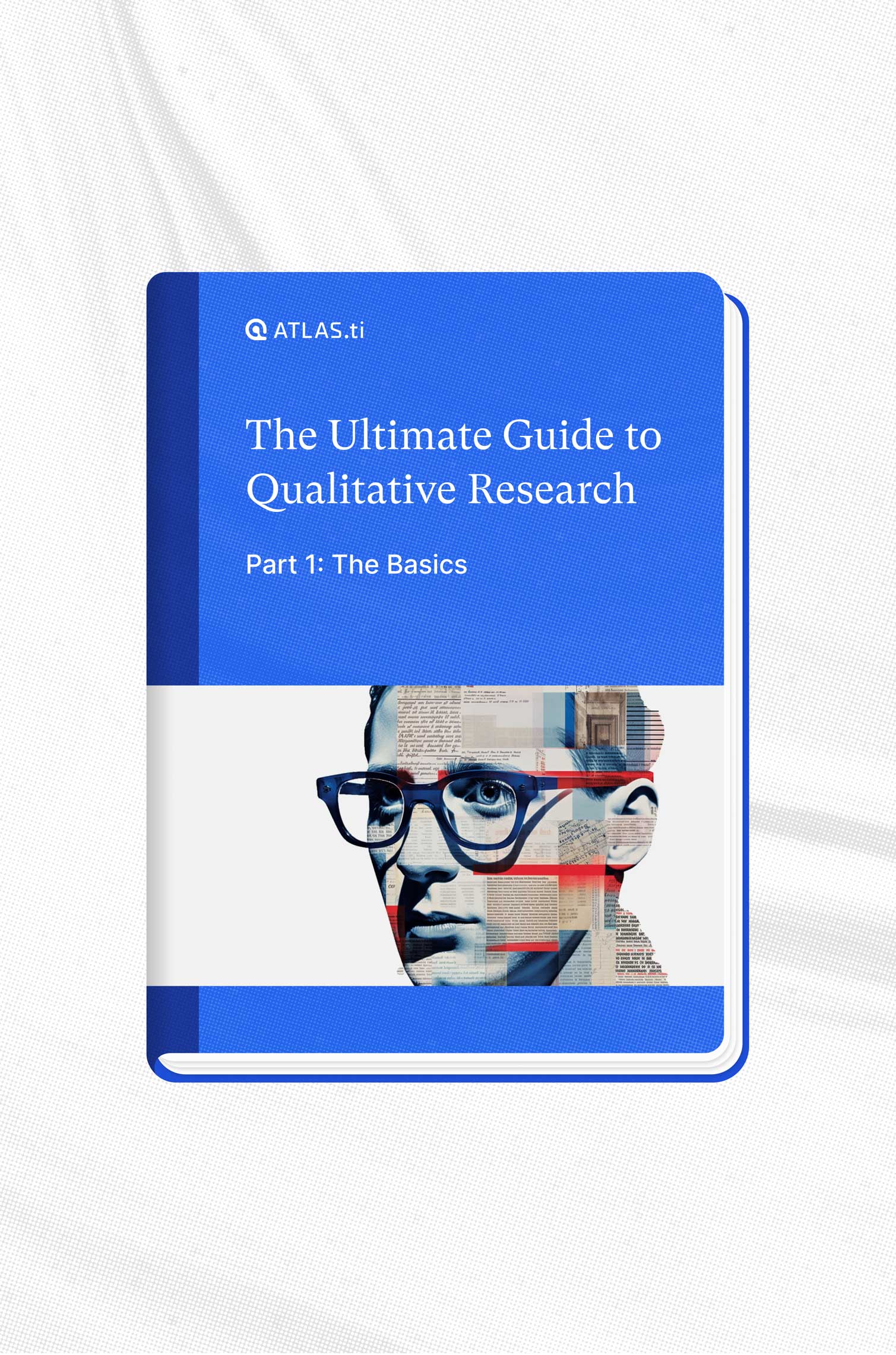
The Ultimate Guide to Qualitative Research - Part 1: The Basics
Learn the essential building blocks of qualitative research. This section covers everything from key definitions to research design, data collection methods, and important ethical considerations.
- Understand what qualitative research is and how it differs from quantitative research
- Get an overview of qualitative data collection methods like interviews, observations, and surveys
- Leverage best practices for conducting ethical qualitative research
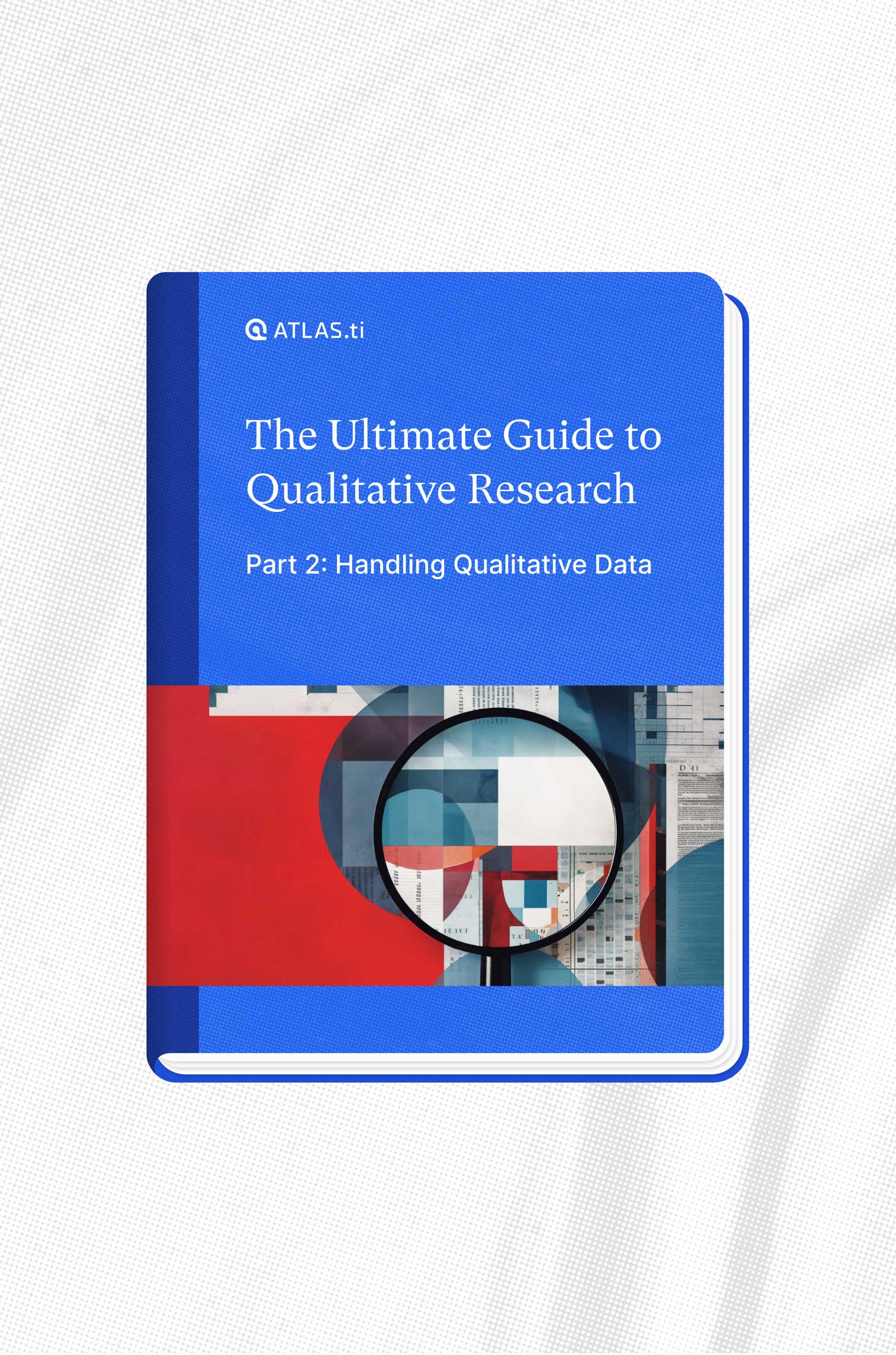
The Ultimate Guide to Qualitative Research - Part 2: Handling Qualitative Data
Get hands-on guidance for working with qualitative data. Discover how to transcribe, organize, code, and analyze textual, verbal, and visual data.
- Transform raw qualitative data into organized, coded sets ready for analysis
- Master qualitative analysis approaches like thematic analysis, content analysis, and grounded theory
- Leverage qualitative data analysis software to streamline the process
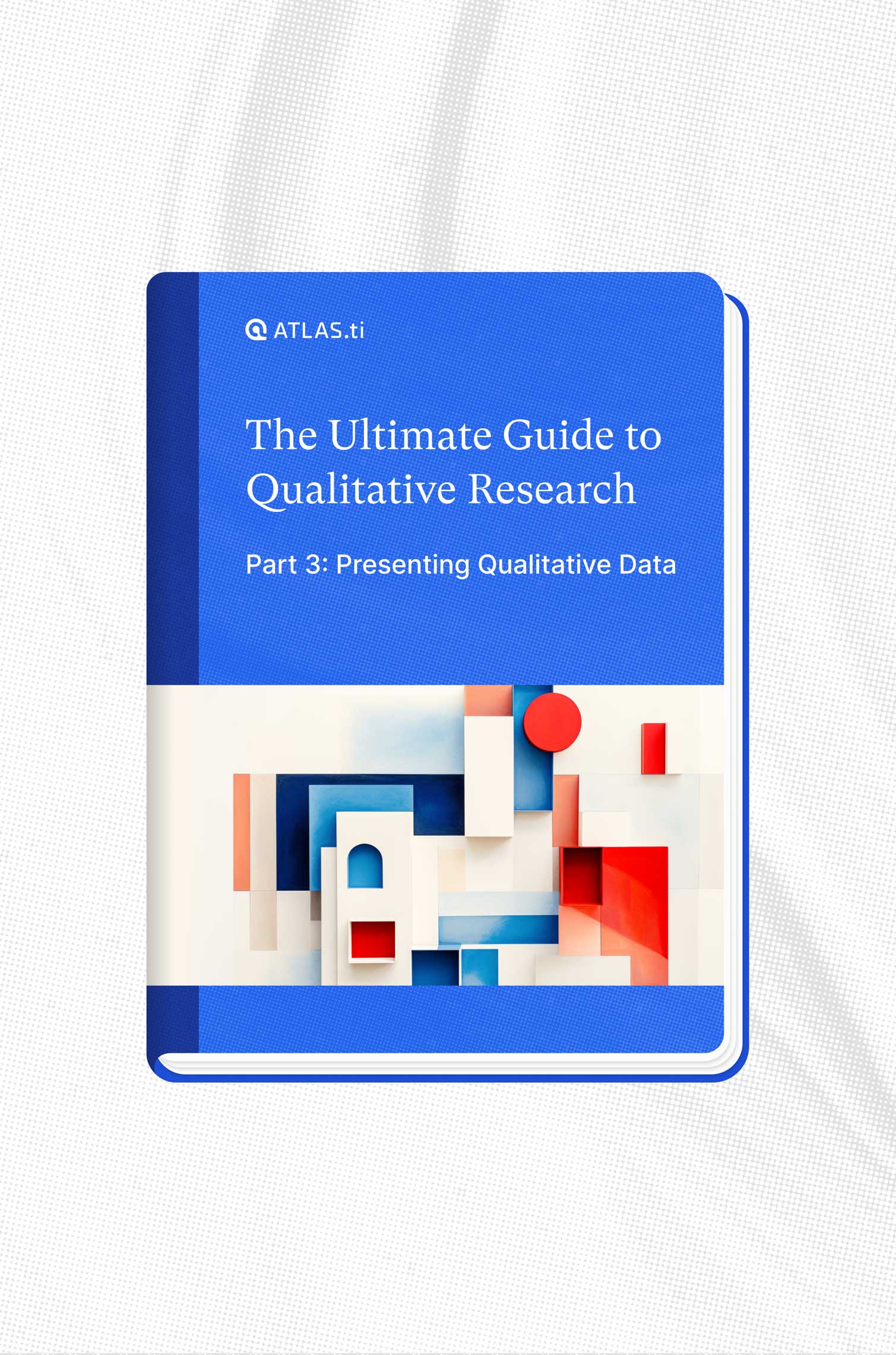
The Ultimate Guide to Qualitative Research - Part 3: Presenting Qualitative Data
Finish strong by effectively presenting qualitative findings. Learn how to visualize data, write up analyses, and meet standards for transparency and rigor.
- Communicate insights through effective qualitative data visualization
- Write a compelling qualitative research paper from start to finish
- Ensure research transparency, quality, and impact through rigorous practices
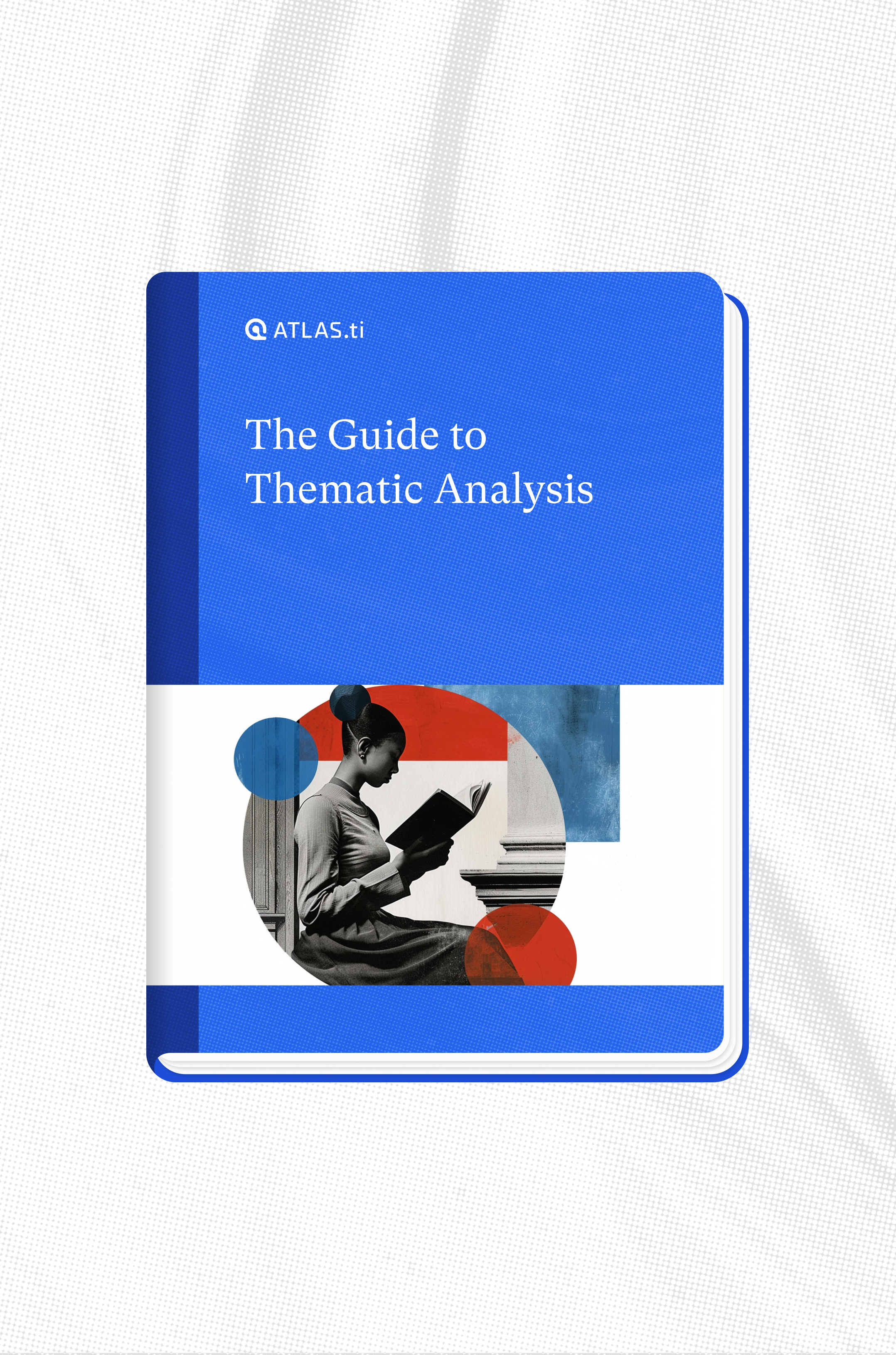
The Guide to Thematic Analysis
Master the art of thematic analysis, a powerful method for identifying patterns and themes in qualitative data. This guide covers everything from the basics to advanced techniques.
- Understand the purpose, advantages, and unique features of thematic analysis
- Learn how to apply thematic analysis to various qualitative data collection methods
- Discover best practices for presenting results and ensuring research rigor
- Utility Menu

User Research Center
User research analysis guide, by meg mcmahon.
Analyzing the notes from a user research study is an essential part of answering the research questions that prompted the study.
During the analysis, you or your team decide what insights are generated from the notes taken about the research studies. The insights should relate to the research questions posed at the beginning of the study or point to future research.
Before Analysis
Become familiar with the study.
Read over the research questions, script, background information on the project, and any notes moderators took during interviews.
Decide on analysis type
There are two types of analysis that the URC team uses frequently, an affinity diagramming session and thematic coding.
- Affinity diagramming: this is a process in which all of the notes are separated into individual sticky notes (virtual or physical) and grouped by question or participant at first. Then the analyzers take an even number of groups and sort them individually and then together to find themes. We at the URC use Miro or Dovetail to virtually facilitate this process.
- Thematic coding: this is a process where the notes or a transcript are marked up using codes. Codes are like tags or hashtags. They are bits of metadata that you use to describe other data. After the codes are created the analyzers go through and draw insights from the coded information. We at the URC use Dovetail for this type of analysis.
Decide on who should help with analysis
Often it is a good idea to include stakeholders, as well as yourself, in the analysis process for two primary reasons. One, the stakeholders are able to be a subject matter expert on the system, process, or workflow you are evaluating and two, by participating in the analysis process they are more likely to feel ownership over the final findings and recommendations.
Analysis Basics
Keep a scratch document.
While analyzing your data, it can be helpful to have a scratch document (a document separate from the main notes document) open to the side. You can use this scratch document to:
- Write down quotes from notes that are especially powerful or meaningful.
- Write down any initial insights you have. An insight informs the research questions or furthers your research goals in some way.
Give insights specific names
Insights are created from the themes or codes created during affinity diagramming or thematic coding. Look at the connections between themes or codes, what are larger insights you can draw from them?
Give each insight a name and be as specific as possible. Insights should help your stakeholders understand their research questions.
- DO: use a strong, clear insight: Participants collaboratively search with a collection specialist as a part of the search process. This was noted to build researchers' special collections research skills.
- DON’T: use a hard to understand, non-specific insight: collaborative search
Review the data with other analyzers
After reviewing the data on your own, discuss what you think of the first themes. Why are they important? What pattern do they surface? How do they relate to the research questions?
Back up insights with quotes
Quote or paraphrase ideas directly from the participants that relate to insights add more impact in the future report.
Look for research gaps
After having insights figured out the next step is to review the insights for any research gaps. Can you answer the research questions in your research plan? If not, what additional research is needed? Or are there other themes that don’t directly relate to the research questions which point to a future follow up study?
Prioritize insights most closely related to research questions
As a user researcher your job is to answer the research questions that started the study. If there is an insight that is only tangentially related to the research questions, it is okay to prioritize insights that are more directly related to the research questions when writing the report.
Affinity diagramming
Take the time to set up session.
Affinity diagramming takes time to set up, but it is important to have every note grouped either by participant or by question.
Create top level themes and sub themes
Start to sort the notes into themes. It is okay to create multiple levels of themes if the data lends itself to this kind of grouping. This is helpful for reports to group like insights together for readability.
Themes are not insights
Themes are not insights, they are building blocks for insights. When you are grouping themes it is okay to keep them more vague than you would an insight.
Collapse themes together if necessary
Often when affinity diagramming you may find similar themes within different questions. It is okay to collapse those themes together under a unifying heading. Themes (and insights) don’t need to be based on only a single question.
Avoid creating a "group" of unrelated notes
There are times in affinity diagramming where there are notes that don’t relate easily to any other notes for the study. It is okay to have orphan notes, notes that don’t relate to any theme. If the finding is important enough, it is okay for the orphan note to be its own theme.
Thematic coding
Codes (tags) are not insights.
Codes (tags) are not insights, they are building blocks for insights. When you are adding codes to the notes or transcripts it is okay to keep them more vague than you would an insight.
Use nested coding
When coding you may need to use nested codes. For example, say you’ve created a tag labeled “Coffee”. Now, if you are talking about how people like their coffee served you may make it a two word code/tag like “Coffee Served”.
If you want to specify that someone likes the coffee “black”, you can add a third word. However, considering “black” is a word that now describes “Coffee Served”, it would be best to create a nested tag which should look something like this: “Coffee Served: Black”.
This makes the tag structure easy to use again. For example, if you have to tag a comment later where someone mentions they like the coffee with cream and sugar, it’s easy to add that as “Coffee Served: Cream & Sugar”.
Repeat codes if necessary
Your codes should inform your research questions and you should repeat codes as necessary. If someone talks about the same thing at various times throughout the interview, code each of those instances with the same code.
Blog posts by month
- October 2023 (1)
- August 2023 (2)
- July 2023 (1)
- June 2023 (1)
- May 2023 (2)
- Privacy Policy

Home » Qualitative Research – Methods, Analysis Types and Guide
Qualitative Research – Methods, Analysis Types and Guide
Table of Contents

Qualitative Research
Qualitative research is a type of research methodology that focuses on exploring and understanding people’s beliefs, attitudes, behaviors, and experiences through the collection and analysis of non-numerical data. It seeks to answer research questions through the examination of subjective data, such as interviews, focus groups, observations, and textual analysis.
Qualitative research aims to uncover the meaning and significance of social phenomena, and it typically involves a more flexible and iterative approach to data collection and analysis compared to quantitative research. Qualitative research is often used in fields such as sociology, anthropology, psychology, and education.
Qualitative Research Methods

Qualitative Research Methods are as follows:
One-to-One Interview
This method involves conducting an interview with a single participant to gain a detailed understanding of their experiences, attitudes, and beliefs. One-to-one interviews can be conducted in-person, over the phone, or through video conferencing. The interviewer typically uses open-ended questions to encourage the participant to share their thoughts and feelings. One-to-one interviews are useful for gaining detailed insights into individual experiences.
Focus Groups
This method involves bringing together a group of people to discuss a specific topic in a structured setting. The focus group is led by a moderator who guides the discussion and encourages participants to share their thoughts and opinions. Focus groups are useful for generating ideas and insights, exploring social norms and attitudes, and understanding group dynamics.
Ethnographic Studies
This method involves immersing oneself in a culture or community to gain a deep understanding of its norms, beliefs, and practices. Ethnographic studies typically involve long-term fieldwork and observation, as well as interviews and document analysis. Ethnographic studies are useful for understanding the cultural context of social phenomena and for gaining a holistic understanding of complex social processes.
Text Analysis
This method involves analyzing written or spoken language to identify patterns and themes. Text analysis can be quantitative or qualitative. Qualitative text analysis involves close reading and interpretation of texts to identify recurring themes, concepts, and patterns. Text analysis is useful for understanding media messages, public discourse, and cultural trends.
This method involves an in-depth examination of a single person, group, or event to gain an understanding of complex phenomena. Case studies typically involve a combination of data collection methods, such as interviews, observations, and document analysis, to provide a comprehensive understanding of the case. Case studies are useful for exploring unique or rare cases, and for generating hypotheses for further research.
Process of Observation
This method involves systematically observing and recording behaviors and interactions in natural settings. The observer may take notes, use audio or video recordings, or use other methods to document what they see. Process of observation is useful for understanding social interactions, cultural practices, and the context in which behaviors occur.
Record Keeping
This method involves keeping detailed records of observations, interviews, and other data collected during the research process. Record keeping is essential for ensuring the accuracy and reliability of the data, and for providing a basis for analysis and interpretation.
This method involves collecting data from a large sample of participants through a structured questionnaire. Surveys can be conducted in person, over the phone, through mail, or online. Surveys are useful for collecting data on attitudes, beliefs, and behaviors, and for identifying patterns and trends in a population.
Qualitative data analysis is a process of turning unstructured data into meaningful insights. It involves extracting and organizing information from sources like interviews, focus groups, and surveys. The goal is to understand people’s attitudes, behaviors, and motivations
Qualitative Research Analysis Methods
Qualitative Research analysis methods involve a systematic approach to interpreting and making sense of the data collected in qualitative research. Here are some common qualitative data analysis methods:
Thematic Analysis
This method involves identifying patterns or themes in the data that are relevant to the research question. The researcher reviews the data, identifies keywords or phrases, and groups them into categories or themes. Thematic analysis is useful for identifying patterns across multiple data sources and for generating new insights into the research topic.
Content Analysis
This method involves analyzing the content of written or spoken language to identify key themes or concepts. Content analysis can be quantitative or qualitative. Qualitative content analysis involves close reading and interpretation of texts to identify recurring themes, concepts, and patterns. Content analysis is useful for identifying patterns in media messages, public discourse, and cultural trends.
Discourse Analysis
This method involves analyzing language to understand how it constructs meaning and shapes social interactions. Discourse analysis can involve a variety of methods, such as conversation analysis, critical discourse analysis, and narrative analysis. Discourse analysis is useful for understanding how language shapes social interactions, cultural norms, and power relationships.
Grounded Theory Analysis
This method involves developing a theory or explanation based on the data collected. Grounded theory analysis starts with the data and uses an iterative process of coding and analysis to identify patterns and themes in the data. The theory or explanation that emerges is grounded in the data, rather than preconceived hypotheses. Grounded theory analysis is useful for understanding complex social phenomena and for generating new theoretical insights.
Narrative Analysis
This method involves analyzing the stories or narratives that participants share to gain insights into their experiences, attitudes, and beliefs. Narrative analysis can involve a variety of methods, such as structural analysis, thematic analysis, and discourse analysis. Narrative analysis is useful for understanding how individuals construct their identities, make sense of their experiences, and communicate their values and beliefs.
Phenomenological Analysis
This method involves analyzing how individuals make sense of their experiences and the meanings they attach to them. Phenomenological analysis typically involves in-depth interviews with participants to explore their experiences in detail. Phenomenological analysis is useful for understanding subjective experiences and for developing a rich understanding of human consciousness.

Comparative Analysis
This method involves comparing and contrasting data across different cases or groups to identify similarities and differences. Comparative analysis can be used to identify patterns or themes that are common across multiple cases, as well as to identify unique or distinctive features of individual cases. Comparative analysis is useful for understanding how social phenomena vary across different contexts and groups.
Applications of Qualitative Research
Qualitative research has many applications across different fields and industries. Here are some examples of how qualitative research is used:
- Market Research: Qualitative research is often used in market research to understand consumer attitudes, behaviors, and preferences. Researchers conduct focus groups and one-on-one interviews with consumers to gather insights into their experiences and perceptions of products and services.
- Health Care: Qualitative research is used in health care to explore patient experiences and perspectives on health and illness. Researchers conduct in-depth interviews with patients and their families to gather information on their experiences with different health care providers and treatments.
- Education: Qualitative research is used in education to understand student experiences and to develop effective teaching strategies. Researchers conduct classroom observations and interviews with students and teachers to gather insights into classroom dynamics and instructional practices.
- Social Work : Qualitative research is used in social work to explore social problems and to develop interventions to address them. Researchers conduct in-depth interviews with individuals and families to understand their experiences with poverty, discrimination, and other social problems.
- Anthropology : Qualitative research is used in anthropology to understand different cultures and societies. Researchers conduct ethnographic studies and observe and interview members of different cultural groups to gain insights into their beliefs, practices, and social structures.
- Psychology : Qualitative research is used in psychology to understand human behavior and mental processes. Researchers conduct in-depth interviews with individuals to explore their thoughts, feelings, and experiences.
- Public Policy : Qualitative research is used in public policy to explore public attitudes and to inform policy decisions. Researchers conduct focus groups and one-on-one interviews with members of the public to gather insights into their perspectives on different policy issues.
How to Conduct Qualitative Research
Here are some general steps for conducting qualitative research:
- Identify your research question: Qualitative research starts with a research question or set of questions that you want to explore. This question should be focused and specific, but also broad enough to allow for exploration and discovery.
- Select your research design: There are different types of qualitative research designs, including ethnography, case study, grounded theory, and phenomenology. You should select a design that aligns with your research question and that will allow you to gather the data you need to answer your research question.
- Recruit participants: Once you have your research question and design, you need to recruit participants. The number of participants you need will depend on your research design and the scope of your research. You can recruit participants through advertisements, social media, or through personal networks.
- Collect data: There are different methods for collecting qualitative data, including interviews, focus groups, observation, and document analysis. You should select the method or methods that align with your research design and that will allow you to gather the data you need to answer your research question.
- Analyze data: Once you have collected your data, you need to analyze it. This involves reviewing your data, identifying patterns and themes, and developing codes to organize your data. You can use different software programs to help you analyze your data, or you can do it manually.
- Interpret data: Once you have analyzed your data, you need to interpret it. This involves making sense of the patterns and themes you have identified, and developing insights and conclusions that answer your research question. You should be guided by your research question and use your data to support your conclusions.
- Communicate results: Once you have interpreted your data, you need to communicate your results. This can be done through academic papers, presentations, or reports. You should be clear and concise in your communication, and use examples and quotes from your data to support your findings.
Examples of Qualitative Research
Here are some real-time examples of qualitative research:
- Customer Feedback: A company may conduct qualitative research to understand the feedback and experiences of its customers. This may involve conducting focus groups or one-on-one interviews with customers to gather insights into their attitudes, behaviors, and preferences.
- Healthcare : A healthcare provider may conduct qualitative research to explore patient experiences and perspectives on health and illness. This may involve conducting in-depth interviews with patients and their families to gather information on their experiences with different health care providers and treatments.
- Education : An educational institution may conduct qualitative research to understand student experiences and to develop effective teaching strategies. This may involve conducting classroom observations and interviews with students and teachers to gather insights into classroom dynamics and instructional practices.
- Social Work: A social worker may conduct qualitative research to explore social problems and to develop interventions to address them. This may involve conducting in-depth interviews with individuals and families to understand their experiences with poverty, discrimination, and other social problems.
- Anthropology : An anthropologist may conduct qualitative research to understand different cultures and societies. This may involve conducting ethnographic studies and observing and interviewing members of different cultural groups to gain insights into their beliefs, practices, and social structures.
- Psychology : A psychologist may conduct qualitative research to understand human behavior and mental processes. This may involve conducting in-depth interviews with individuals to explore their thoughts, feelings, and experiences.
- Public Policy: A government agency or non-profit organization may conduct qualitative research to explore public attitudes and to inform policy decisions. This may involve conducting focus groups and one-on-one interviews with members of the public to gather insights into their perspectives on different policy issues.
Purpose of Qualitative Research
The purpose of qualitative research is to explore and understand the subjective experiences, behaviors, and perspectives of individuals or groups in a particular context. Unlike quantitative research, which focuses on numerical data and statistical analysis, qualitative research aims to provide in-depth, descriptive information that can help researchers develop insights and theories about complex social phenomena.
Qualitative research can serve multiple purposes, including:
- Exploring new or emerging phenomena : Qualitative research can be useful for exploring new or emerging phenomena, such as new technologies or social trends. This type of research can help researchers develop a deeper understanding of these phenomena and identify potential areas for further study.
- Understanding complex social phenomena : Qualitative research can be useful for exploring complex social phenomena, such as cultural beliefs, social norms, or political processes. This type of research can help researchers develop a more nuanced understanding of these phenomena and identify factors that may influence them.
- Generating new theories or hypotheses: Qualitative research can be useful for generating new theories or hypotheses about social phenomena. By gathering rich, detailed data about individuals’ experiences and perspectives, researchers can develop insights that may challenge existing theories or lead to new lines of inquiry.
- Providing context for quantitative data: Qualitative research can be useful for providing context for quantitative data. By gathering qualitative data alongside quantitative data, researchers can develop a more complete understanding of complex social phenomena and identify potential explanations for quantitative findings.
When to use Qualitative Research
Here are some situations where qualitative research may be appropriate:
- Exploring a new area: If little is known about a particular topic, qualitative research can help to identify key issues, generate hypotheses, and develop new theories.
- Understanding complex phenomena: Qualitative research can be used to investigate complex social, cultural, or organizational phenomena that are difficult to measure quantitatively.
- Investigating subjective experiences: Qualitative research is particularly useful for investigating the subjective experiences of individuals or groups, such as their attitudes, beliefs, values, or emotions.
- Conducting formative research: Qualitative research can be used in the early stages of a research project to develop research questions, identify potential research participants, and refine research methods.
- Evaluating interventions or programs: Qualitative research can be used to evaluate the effectiveness of interventions or programs by collecting data on participants’ experiences, attitudes, and behaviors.
Characteristics of Qualitative Research
Qualitative research is characterized by several key features, including:
- Focus on subjective experience: Qualitative research is concerned with understanding the subjective experiences, beliefs, and perspectives of individuals or groups in a particular context. Researchers aim to explore the meanings that people attach to their experiences and to understand the social and cultural factors that shape these meanings.
- Use of open-ended questions: Qualitative research relies on open-ended questions that allow participants to provide detailed, in-depth responses. Researchers seek to elicit rich, descriptive data that can provide insights into participants’ experiences and perspectives.
- Sampling-based on purpose and diversity: Qualitative research often involves purposive sampling, in which participants are selected based on specific criteria related to the research question. Researchers may also seek to include participants with diverse experiences and perspectives to capture a range of viewpoints.
- Data collection through multiple methods: Qualitative research typically involves the use of multiple data collection methods, such as in-depth interviews, focus groups, and observation. This allows researchers to gather rich, detailed data from multiple sources, which can provide a more complete picture of participants’ experiences and perspectives.
- Inductive data analysis: Qualitative research relies on inductive data analysis, in which researchers develop theories and insights based on the data rather than testing pre-existing hypotheses. Researchers use coding and thematic analysis to identify patterns and themes in the data and to develop theories and explanations based on these patterns.
- Emphasis on researcher reflexivity: Qualitative research recognizes the importance of the researcher’s role in shaping the research process and outcomes. Researchers are encouraged to reflect on their own biases and assumptions and to be transparent about their role in the research process.
Advantages of Qualitative Research
Qualitative research offers several advantages over other research methods, including:
- Depth and detail: Qualitative research allows researchers to gather rich, detailed data that provides a deeper understanding of complex social phenomena. Through in-depth interviews, focus groups, and observation, researchers can gather detailed information about participants’ experiences and perspectives that may be missed by other research methods.
- Flexibility : Qualitative research is a flexible approach that allows researchers to adapt their methods to the research question and context. Researchers can adjust their research methods in real-time to gather more information or explore unexpected findings.
- Contextual understanding: Qualitative research is well-suited to exploring the social and cultural context in which individuals or groups are situated. Researchers can gather information about cultural norms, social structures, and historical events that may influence participants’ experiences and perspectives.
- Participant perspective : Qualitative research prioritizes the perspective of participants, allowing researchers to explore subjective experiences and understand the meanings that participants attach to their experiences.
- Theory development: Qualitative research can contribute to the development of new theories and insights about complex social phenomena. By gathering rich, detailed data and using inductive data analysis, researchers can develop new theories and explanations that may challenge existing understandings.
- Validity : Qualitative research can offer high validity by using multiple data collection methods, purposive and diverse sampling, and researcher reflexivity. This can help ensure that findings are credible and trustworthy.
Limitations of Qualitative Research
Qualitative research also has some limitations, including:
- Subjectivity : Qualitative research relies on the subjective interpretation of researchers, which can introduce bias into the research process. The researcher’s perspective, beliefs, and experiences can influence the way data is collected, analyzed, and interpreted.
- Limited generalizability: Qualitative research typically involves small, purposive samples that may not be representative of larger populations. This limits the generalizability of findings to other contexts or populations.
- Time-consuming: Qualitative research can be a time-consuming process, requiring significant resources for data collection, analysis, and interpretation.
- Resource-intensive: Qualitative research may require more resources than other research methods, including specialized training for researchers, specialized software for data analysis, and transcription services.
- Limited reliability: Qualitative research may be less reliable than quantitative research, as it relies on the subjective interpretation of researchers. This can make it difficult to replicate findings or compare results across different studies.
- Ethics and confidentiality: Qualitative research involves collecting sensitive information from participants, which raises ethical concerns about confidentiality and informed consent. Researchers must take care to protect the privacy and confidentiality of participants and obtain informed consent.
Also see Research Methods
About the author
Muhammad Hassan
Researcher, Academic Writer, Web developer
You may also like

Questionnaire – Definition, Types, and Examples

Case Study – Methods, Examples and Guide

Observational Research – Methods and Guide

Quantitative Research – Methods, Types and...

Qualitative Research Methods

Explanatory Research – Types, Methods, Guide
Understanding data analysis: A beginner's guide
Before data can be used to tell a story, it must go through a process that makes it usable. Explore the role of data analysis in decision-making.
What is data analysis?
Data analysis is the process of gathering, cleaning, and modeling data to reveal meaningful insights. This data is then crafted into reports that support the strategic decision-making process.
Types of data analysis
There are many different types of data analysis. Each type can be used to answer a different question.
Descriptive analytics
Descriptive analytics refers to the process of analyzing historical data to understand trends and patterns. For example, success or failure to achieve key performance indicators like return on investment.
An example of descriptive analytics is generating reports to provide an overview of an organization's sales and financial data, offering valuable insights into past activities and outcomes.
Predictive analytics
Predictive analytics uses historical data to help predict what might happen in the future, such as identifying past trends in data to determine if they’re likely to recur.
Methods include a range of statistical and machine learning techniques, including neural networks, decision trees, and regression analysis.
Diagnostic analytics
Diagnostic analytics helps answer questions about what caused certain events by looking at performance indicators. Diagnostic analytics techniques supplement basic descriptive analysis.
Generally, diagnostic analytics involves spotting anomalies in data (like an unexpected shift in a metric), gathering data related to these anomalies, and using statistical techniques to identify potential explanations.
Cognitive analytics
Cognitive analytics is a sophisticated form of data analysis that goes beyond traditional methods. This method uses machine learning and natural language processing to understand, reason, and learn from data in a way that resembles human thought processes.
The goal of cognitive analytics is to simulate human-like thinking to provide deeper insights, recognize patterns, and make predictions.
Prescriptive analytics
Prescriptive analytics helps answer questions about what needs to happen next to achieve a certain goal or target. By using insights from prescriptive analytics, organizations can make data-driven decisions in the face of uncertainty.
Data analysts performing prescriptive analysis often rely on machine learning to find patterns in large semantic models and estimate the likelihood of various outcomes.
analyticsText analytics
Text analytics is a way to teach computers to understand human language. It involves using algorithms and other techniques to extract information from large amounts of text data, such as social media posts or customer previews.
Text analytics helps data analysts make sense of what people are saying, find patterns, and gain insights that can be used to make better decisions in fields like business, marketing, and research.
The data analysis process
Compiling and interpreting data so it can be used in decision making is a detailed process and requires a systematic approach. Here are the steps that data analysts follow:
1. Define your objectives.
Clearly define the purpose of your analysis. What specific question are you trying to answer? What problem do you want to solve? Identify your core objectives. This will guide the entire process.
2. Collect and consolidate your data.
Gather your data from all relevant sources using data analysis software . Ensure that the data is representative and actually covers the variables you want to analyze.
3. Select your analytical methods.
Investigate the various data analysis methods and select the technique that best aligns with your objectives. Many free data analysis software solutions offer built-in algorithms and methods to facilitate this selection process.
4. Clean your data.
Scrutinize your data for errors, missing values, or inconsistencies using the cleansing features already built into your data analysis software. Cleaning the data ensures accuracy and reliability in your analysis and is an important part of data analytics.
5. Uncover valuable insights.
Delve into your data to uncover patterns, trends, and relationships. Use statistical methods, machine learning algorithms, or other analytical techniques that are aligned with your goals. This step transforms raw data into valuable insights.
6. Interpret and visualize the results.
Examine the results of your analyses to understand their implications. Connect these findings with your initial objectives. Then, leverage the visualization tools within free data analysis software to present your insights in a more digestible format.
7. Make an informed decision.
Use the insights gained from your analysis to inform your next steps. Think about how these findings can be utilized to enhance processes, optimize strategies, or improve overall performance.
By following these steps, analysts can systematically approach large sets of data, breaking down the complexities and ensuring the results are actionable for decision makers.
The importance of data analysis
Data analysis is critical because it helps business decision makers make sense of the information they collect in our increasingly data-driven world. Imagine you have a massive pile of puzzle pieces (data), and you want to see the bigger picture (insights). Data analysis is like putting those puzzle pieces together—turning that data into knowledge—to reveal what’s important.
Whether you’re a business decision maker trying to make sense of customer preferences or a scientist studying trends, data analysis is an important tool that helps us understand the world and make informed choices.
Primary data analysis methods
Quantitative analysis
Quantitative analysis deals with numbers and measurements (for example, looking at survey results captured through ratings). When performing quantitative analysis, you’ll use mathematical and statistical methods exclusively and answer questions like ‘how much’ or ‘how many.’
Qualitative analysis
Qualitative analysis is about understanding the subjective meaning behind non-numerical data. For example, analyzing interview responses or looking at pictures to understand emotions. Qualitative analysis looks for patterns, themes, or insights, and is mainly concerned with depth and detail.
Data analysis solutions and resources
Turn your data into actionable insights and visualize the results with ease.
Microsoft 365
Process data and turn ideas into reality with innovative apps, including Excel.
Importance of backing up data
Learn how to back up your data and devices for peace of mind—and added security.
Copilot in Excel
Go deeper with your data using Microsoft Copilot—your AI assistant.
Excel expense template
Organize and track your business expenses using Excel.
Excel templates
Boost your productivity with free, customizable Excel templates for all types of documents.
Chart designs
Enhance presentations, research, and other materials with customizable chart templates.
Follow Microsoft
- Open access
- Published: 17 August 2023
Data visualisation in scoping reviews and evidence maps on health topics: a cross-sectional analysis
- Emily South ORCID: orcid.org/0000-0003-2187-4762 1 &
- Mark Rodgers 1
Systematic Reviews volume 12 , Article number: 142 ( 2023 ) Cite this article
3626 Accesses
13 Altmetric
Metrics details
Scoping reviews and evidence maps are forms of evidence synthesis that aim to map the available literature on a topic and are well-suited to visual presentation of results. A range of data visualisation methods and interactive data visualisation tools exist that may make scoping reviews more useful to knowledge users. The aim of this study was to explore the use of data visualisation in a sample of recent scoping reviews and evidence maps on health topics, with a particular focus on interactive data visualisation.
Ovid MEDLINE ALL was searched for recent scoping reviews and evidence maps (June 2020-May 2021), and a sample of 300 papers that met basic selection criteria was taken. Data were extracted on the aim of each review and the use of data visualisation, including types of data visualisation used, variables presented and the use of interactivity. Descriptive data analysis was undertaken of the 238 reviews that aimed to map evidence.
Of the 238 scoping reviews or evidence maps in our analysis, around one-third (37.8%) included some form of data visualisation. Thirty-five different types of data visualisation were used across this sample, although most data visualisations identified were simple bar charts (standard, stacked or multi-set), pie charts or cross-tabulations (60.8%). Most data visualisations presented a single variable (64.4%) or two variables (26.1%). Almost a third of the reviews that used data visualisation did not use any colour (28.9%). Only two reviews presented interactive data visualisation, and few reported the software used to create visualisations.
Conclusions
Data visualisation is currently underused by scoping review authors. In particular, there is potential for much greater use of more innovative forms of data visualisation and interactive data visualisation. Where more innovative data visualisation is used, scoping reviews have made use of a wide range of different methods. Increased use of these more engaging visualisations may make scoping reviews more useful for a range of stakeholders.
Peer Review reports
Scoping reviews are “a type of evidence synthesis that aims to systematically identify and map the breadth of evidence available on a particular topic, field, concept, or issue” ([ 1 ], p. 950). While they include some of the same steps as a systematic review, such as systematic searches and the use of predetermined eligibility criteria, scoping reviews often address broader research questions and do not typically involve the quality appraisal of studies or synthesis of data [ 2 ]. Reasons for conducting a scoping review include the following: to map types of evidence available, to explore research design and conduct, to clarify concepts or definitions and to map characteristics or factors related to a concept [ 3 ]. Scoping reviews can also be undertaken to inform a future systematic review (e.g. to assure authors there will be adequate studies) or to identify knowledge gaps [ 3 ]. Other evidence synthesis approaches with similar aims have been described as evidence maps, mapping reviews or systematic maps [ 4 ]. While this terminology is used inconsistently, evidence maps can be used to identify evidence gaps and present them in a user-friendly (and often visual) way [ 5 ].
Scoping reviews are often targeted to an audience of healthcare professionals or policy-makers [ 6 ], suggesting that it is important to present results in a user-friendly and informative way. Until recently, there was little guidance on how to present the findings of scoping reviews. In recent literature, there has been some discussion of the importance of clearly presenting data for the intended audience of a scoping review, with creative and innovative use of visual methods if appropriate [ 7 , 8 , 9 ]. Lockwood et al. suggest that innovative visual presentation should be considered over dense sections of text or long tables in many cases [ 8 ]. Khalil et al. suggest that inspiration could be drawn from the field of data visualisation [ 7 ]. JBI guidance on scoping reviews recommends that reviewers carefully consider the best format for presenting data at the protocol development stage and provides a number of examples of possible methods [ 10 ].
Interactive resources are another option for presentation in scoping reviews [ 9 ]. Researchers without the relevant programming skills can now use several online platforms (such as Tableau [ 11 ] and Flourish [ 12 ]) to create interactive data visualisations. The benefits of using interactive visualisation in research include the ability to easily present more than two variables [ 13 ] and increased engagement of users [ 14 ]. Unlike static graphs, interactive visualisations can allow users to view hierarchical data at different levels, exploring both the “big picture” and looking in more detail ([ 15 ], p. 291). Interactive visualizations are often targeted at practitioners and decision-makers [ 13 ], and there is some evidence from qualitative research that they are valued by policy-makers [ 16 , 17 , 18 ].
Given their focus on mapping evidence, we believe that scoping reviews are particularly well-suited to visually presenting data and the use of interactive data visualisation tools. However, it is unknown how many recent scoping reviews visually map data or which types of data visualisation are used. The aim of this study was to explore the use of data visualisation methods in a large sample of recent scoping reviews and evidence maps on health topics. In particular, we were interested in the extent to which these forms of synthesis use any form of interactive data visualisation.
This study was a cross-sectional analysis of studies labelled as scoping reviews or evidence maps (or synonyms of these terms) in the title or abstract.
The search strategy was developed with help from an information specialist. Ovid MEDLINE® ALL was searched in June 2021 for studies added to the database in the previous 12 months. The search was limited to English language studies only.
The search strategy was as follows:
Ovid MEDLINE(R) ALL
(scoping review or evidence map or systematic map or mapping review or scoping study or scoping project or scoping exercise or literature mapping or evidence mapping or systematic mapping or literature scoping or evidence gap map).ab,ti.
limit 1 to english language
(202006* or 202007* or 202008* or 202009* or 202010* or 202011* or 202012* or 202101* or 202102* or 202103* or 202104* or 202105*).dt.
The search returned 3686 records. Records were de-duplicated in EndNote 20 software, leaving 3627 unique records.
A sample of these reviews was taken by screening the search results against basic selection criteria (Table 1 ). These criteria were piloted and refined after discussion between the two researchers. A single researcher (E.S.) screened the records in EPPI-Reviewer Web software using the machine-learning priority screening function. Where a second opinion was needed, decisions were checked by a second researcher (M.R.).
Our initial plan for sampling, informed by pilot searching, was to screen and data extract records in batches of 50 included reviews at a time. We planned to stop screening when a batch of 50 reviews had been extracted that included no new types of data visualisation or after screening time had reached 2 days. However, once data extraction was underway, we found the sample to be richer in terms of data visualisation than anticipated. After the inclusion of 300 reviews, we took the decision to end screening in order to ensure the study was manageable.
Data extraction
A data extraction form was developed in EPPI-Reviewer Web, piloted on 50 reviews and refined. Data were extracted by one researcher (E. S. or M. R.), with a second researcher (M. R. or E. S.) providing a second opinion when needed. The data items extracted were as follows: type of review (term used by authors), aim of review (mapping evidence vs. answering specific question vs. borderline), number of visualisations (if any), types of data visualisation used, variables/domains presented by each visualisation type, interactivity, use of colour and any software requirements.
When categorising review aims, we considered “mapping evidence” to incorporate all of the six purposes for conducting a scoping review proposed by Munn et al. [ 3 ]. Reviews were categorised as “answering a specific question” if they aimed to synthesise study findings to answer a particular question, for example on effectiveness of an intervention. We were inclusive with our definition of “mapping evidence” and included reviews with mixed aims in this category. However, some reviews were difficult to categorise (for example where aims were unclear or the stated aims did not match the actual focus of the paper) and were considered to be “borderline”. It became clear that a proportion of identified records that described themselves as “scoping” or “mapping” reviews were in fact pseudo-systematic reviews that failed to undertake key systematic review processes. Such reviews attempted to integrate the findings of included studies rather than map the evidence, and so reviews categorised as “answering a specific question” were excluded from the main analysis. Data visualisation methods for meta-analyses have been explored previously [ 19 ]. Figure 1 shows the flow of records from search results to final analysis sample.
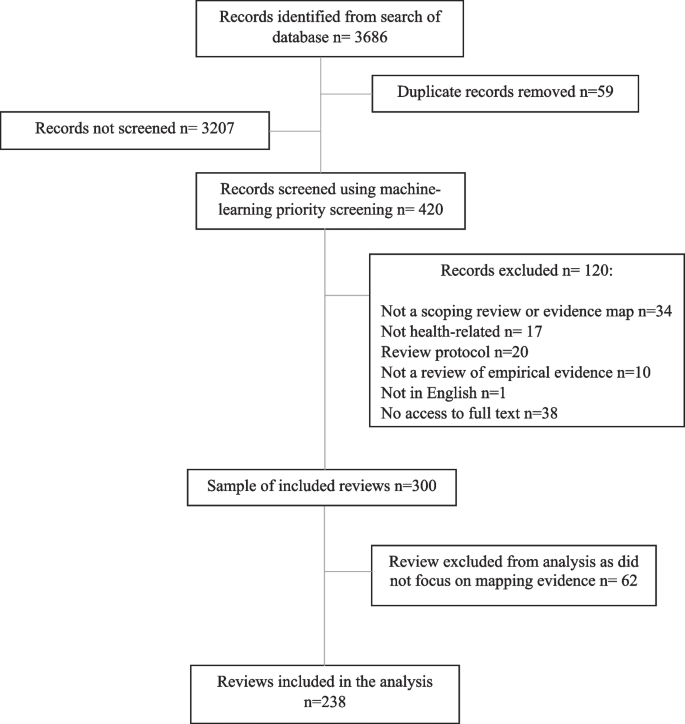
Flow diagram of the sampling process
Data visualisation was defined as any graph or diagram that presented results data, including tables with a visual mapping element, such as cross-tabulations and heat maps. However, tables which displayed data at a study level (e.g. tables summarising key characteristics of each included study) were not included, even if they used symbols, shading or colour. Flow diagrams showing the study selection process were also excluded. Data visualisations in appendices or supplementary information were included, as well as any in publicly available dissemination products (e.g. visualisations hosted online) if mentioned in papers.
The typology used to categorise data visualisation methods was based on an existing online catalogue [ 20 ]. Specific types of data visualisation were categorised in five broad categories: graphs, diagrams, tables, maps/geographical and other. If a data visualisation appeared in our sample that did not feature in the original catalogue, we checked a second online catalogue [ 21 ] for an appropriate term, followed by wider Internet searches. These additional visualisation methods were added to the appropriate section of the typology. The final typology can be found in Additional file 1 .
We conducted descriptive data analysis in Microsoft Excel 2019 and present frequencies and percentages. Where appropriate, data are presented using graphs or other data visualisations created using Flourish. We also link to interactive versions of some of these visualisations.
Almost all of the 300 reviews in the total sample were labelled by review authors as “scoping reviews” ( n = 293, 97.7%). There were also four “mapping reviews”, one “scoping study”, one “evidence mapping” and one that was described as a “scoping review and evidence map”. Included reviews were all published in 2020 or 2021, with the exception of one review published in 2018. Just over one-third of these reviews ( n = 105, 35.0%) included some form of data visualisation. However, we excluded 62 reviews that did not focus on mapping evidence from the following analysis (see “ Methods ” section). Of the 238 remaining reviews (that either clearly aimed to map evidence or were judged to be “borderline”), 90 reviews (37.8%) included at least one data visualisation. The references for these reviews can be found in Additional file 2 .
Number of visualisations
Thirty-six (40.0%) of these 90 reviews included just one example of data visualisation (Fig. 2 ). Less than a third ( n = 28, 31.1%) included three or more visualisations. The greatest number of data visualisations in one review was 17 (all bar or pie charts). In total, 222 individual data visualisations were identified across the sample of 238 reviews.
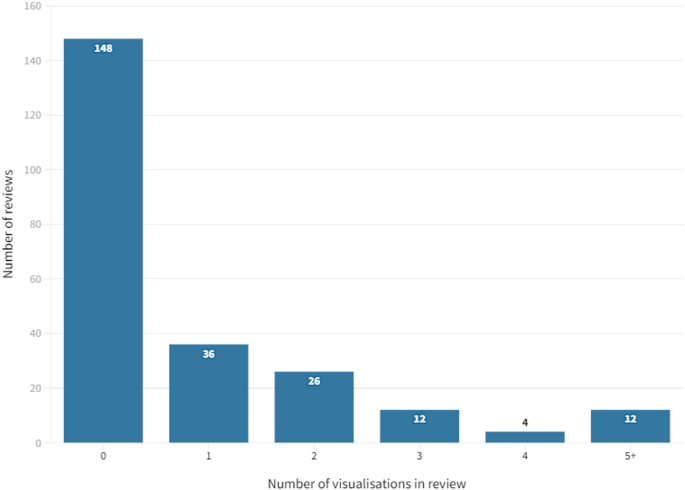
Number of data visualisations per review
Categories of data visualisation
Graphs were the most frequently used category of data visualisation in the sample. Over half of the reviews with data visualisation included at least one graph ( n = 59, 65.6%). The least frequently used category was maps, with 15.6% ( n = 14) of these reviews including a map.
Of the total number of 222 individual data visualisations, 102 were graphs (45.9%), 34 were tables (15.3%), 23 were diagrams (10.4%), 15 were maps (6.8%) and 48 were classified as “other” in the typology (21.6%).
Types of data visualisation
All of the types of data visualisation identified in our sample are reported in Table 2 . In total, 35 different types were used across the sample of reviews.
The most frequently used data visualisation type was a bar chart. Of 222 total data visualisations, 78 (35.1%) were a variation on a bar chart (either standard bar chart, stacked bar chart or multi-set bar chart). There were also 33 pie charts (14.9% of data visualisations) and 24 cross-tabulations (10.8% of data visualisations). In total, these five types of data visualisation accounted for 60.8% ( n = 135) of all data visualisations. Figure 3 shows the frequency of each data visualisation category and type; an interactive online version of this treemap is also available ( https://public.flourish.studio/visualisation/9396133/ ). Figure 4 shows how users can further explore the data using the interactive treemap.
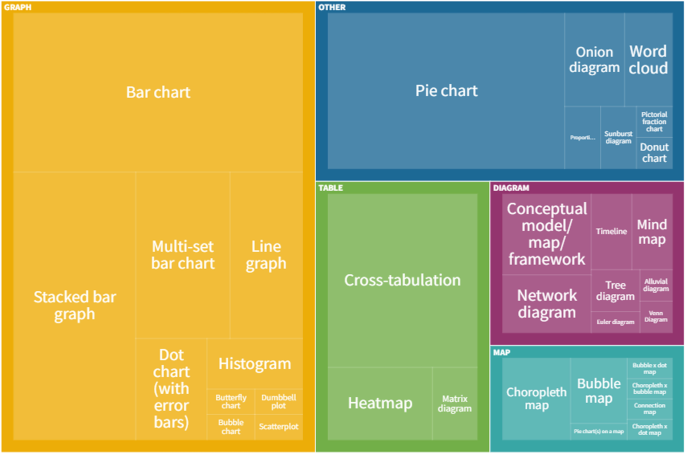
Data visualisation categories and types. An interactive version of this treemap is available online: https://public.flourish.studio/visualisation/9396133/ . Through the interactive version, users can further explore the data (see Fig. 4 ). The unit of this treemap is the individual data visualisation, so multiple data visualisations within the same scoping review are represented in this map. Created with flourish.studio ( https://flourish.studio )
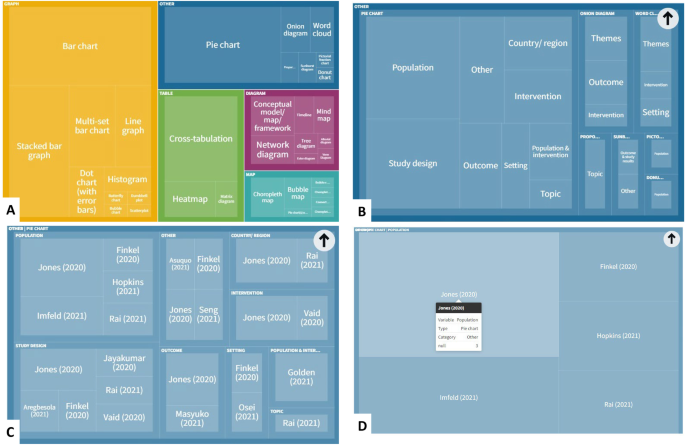
Screenshots showing how users of the interactive treemap can explore the data further. Users can explore each level of the hierarchical treemap ( A Visualisation category > B Visualisation subcategory > C Variables presented in visualisation > D Individual references reporting this category/subcategory/variable permutation). Created with flourish.studio ( https://flourish.studio )
Data presented
Around two-thirds of data visualisations in the sample presented a single variable ( n = 143, 64.4%). The most frequently presented single variables were themes ( n = 22, 9.9% of data visualisations), population ( n = 21, 9.5%), country or region ( n = 21, 9.5%) and year ( n = 20, 9.0%). There were 58 visualisations (26.1%) that presented two different variables. The remaining 21 data visualisations (9.5%) presented three or more variables. Figure 5 shows the variables presented by each different type of data visualisation (an interactive version of this figure is available online).
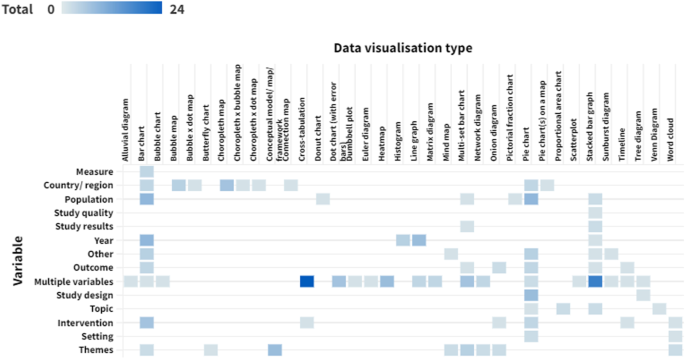
Variables presented by each data visualisation type. Darker cells indicate a larger number of reviews. An interactive version of this heat map is available online: https://public.flourish.studio/visualisation/10632665/ . Users can hover over each cell to see the number of data visualisations for that combination of data visualisation type and variable. The unit of this heat map is the individual data visualisation, so multiple data visualisations within a single scoping review are represented in this map. Created with flourish.studio ( https://flourish.studio )
Most reviews presented at least one data visualisation in colour ( n = 64, 71.1%). However, almost a third ( n = 26, 28.9%) used only black and white or greyscale.
Interactivity
Only two of the reviews included data visualisations with any level of interactivity. One scoping review on music and serious mental illness [ 22 ] linked to an interactive bubble chart hosted online on Tableau. Functionality included the ability to filter the studies displayed by various attributes.
The other review was an example of evidence mapping from the environmental health field [ 23 ]. All four of the data visualisations included in the paper were available in an interactive format hosted either by the review management software or on Tableau. The interactive versions linked to the relevant references so users could directly explore the evidence base. This was the only review that provided this feature.
Software requirements
Nine reviews clearly reported the software used to create data visualisations. Three reviews used Tableau (one of them also used review management software as discussed above) [ 22 , 23 , 24 ]. Two reviews generated maps using ArcGIS [ 25 ] or ArcMap [ 26 ]. One review used Leximancer for a lexical analysis [ 27 ]. One review undertook a bibliometric analysis using VOSviewer [ 28 ], and another explored citation patterns using CitNetExplorer [ 29 ]. Other reviews used Excel [ 30 ] or R [ 26 ].
To our knowledge, this is the first systematic and in-depth exploration of the use of data visualisation techniques in scoping reviews. Our findings suggest that the majority of scoping reviews do not use any data visualisation at all, and, in particular, more innovative examples of data visualisation are rare. Around 60% of data visualisations in our sample were simple bar charts, pie charts or cross-tabulations. There appears to be very limited use of interactive online visualisation, despite the potential this has for communicating results to a range of stakeholders. While it is not always appropriate to use data visualisation (or a simple bar chart may be the most user-friendly way of presenting the data), these findings suggest that data visualisation is being underused in scoping reviews. In a large minority of reviews, visualisations were not published in colour, potentially limiting how user-friendly and attractive papers are to decision-makers and other stakeholders. Also, very few reviews clearly reported the software used to create data visualisations. However, 35 different types of data visualisation were used across the sample, highlighting the wide range of methods that are potentially available to scoping review authors.
Our results build on the limited research that has previously been undertaken in this area. Two previous publications also found limited use of graphs in scoping reviews. Results were “mapped graphically” in 29% of scoping reviews in any field in one 2014 publication [ 31 ] and 17% of healthcare scoping reviews in a 2016 article [ 6 ]. Our results suggest that the use of data visualisation has increased somewhat since these reviews were conducted. Scoping review methods have also evolved in the last 10 years; formal guidance on scoping review conduct was published in 2014 [ 32 ], and an extension of the PRISMA checklist for scoping reviews was published in 2018 [ 33 ]. It is possible that an overall increase in use of data visualisation reflects increased quality of published scoping reviews. There is also some literature supporting our findings on the wide range of data visualisation methods that are used in evidence synthesis. An investigation of methods to identify, prioritise or display health research gaps (25/139 included studies were scoping reviews; 6/139 were evidence maps) identified 14 different methods used to display gaps or priorities, with half being “more advanced” (e.g. treemaps, radial bar plots) ([ 34 ], p. 107). A review of data visualisation methods used in papers reporting meta-analyses found over 200 different ways of displaying data [ 19 ].
Only two reviews in our sample used interactive data visualisation, and one of these was an example of systematic evidence mapping from the environmental health field rather than a scoping review (in environmental health, systematic evidence mapping explicitly involves producing a searchable database [ 35 ]). A scoping review of papers on the use of interactive data visualisation in population health or health services research found a range of examples but still limited use overall [ 13 ]. For example, the authors noted the currently underdeveloped potential for using interactive visualisation in research on health inequalities. It is possible that the use of interactive data visualisation in academic papers is restricted by academic publishing requirements; for example, it is currently difficult to incorporate an interactive figure into a journal article without linking to an external host or platform. However, we believe that there is a lot of potential to add value to future scoping reviews by using interactive data visualisation software. Few reviews in our sample presented three or more variables in a single visualisation, something which can easily be achieved using interactive data visualisation tools. We have previously used EPPI-Mapper [ 36 ] to present results of a scoping review of systematic reviews on behaviour change in disadvantaged groups, with links to the maps provided in the paper [ 37 ]. These interactive maps allowed policy-makers to explore the evidence on different behaviours and disadvantaged groups and access full publications of the included studies directly from the map.
We acknowledge there are barriers to use for some of the data visualisation software available. EPPI-Mapper and some of the software used by reviews in our sample incur a cost. Some software requires a certain level of knowledge and skill in its use. However numerous online free data visualisation tools and resources exist. We have used Flourish to present data for this review, a basic version of which is currently freely available and easy to use. Previous health research has been found to have used a range of different interactive data visualisation software, much of which does not required advanced knowledge or skills to use [ 13 ].
There are likely to be other barriers to the use of data visualisation in scoping reviews. Journal guidelines and policies may present barriers for using innovative data visualisation. For example, some journals charge a fee for publication of figures in colour. As previously mentioned, there are limited options for incorporating interactive data visualisation into journal articles. Authors may also be unaware of the data visualisation methods and tools that are available. Producing data visualisations can be time-consuming, particularly if authors lack experience and skills in this. It is possible that many authors prioritise speed of publication over spending time producing innovative data visualisations, particularly in a context where there is pressure to achieve publications.
Limitations
A limitation of this study was that we did not assess how appropriate the use of data visualisation was in our sample as this would have been highly subjective. Simple descriptive or tabular presentation of results may be the most appropriate approach for some scoping review objectives [ 7 , 8 , 10 ], and the scoping review literature cautions against “over-using” different visual presentation methods [ 7 , 8 ]. It cannot be assumed that all of the reviews that did not include data visualisation should have done so. Likewise, we do not know how many reviews used methods of data visualisation that were not well suited to their data.
We initially relied on authors’ own use of the term “scoping review” (or equivalent) to sample reviews but identified a relatively large number of papers labelled as scoping reviews that did not meet the basic definition, despite the availability of guidance and reporting guidelines [ 10 , 33 ]. It has previously been noted that scoping reviews may be undertaken inappropriately because they are seen as “easier” to conduct than a systematic review ([ 3 ], p.6), and that reviews are often labelled as “scoping reviews” while not appearing to follow any established framework or guidance [ 2 ]. We therefore took the decision to remove these reviews from our main analysis. However, decisions on how to classify review aims were subjective, and we did include some reviews that were of borderline relevance.
A further limitation is that this was a sample of published reviews, rather than a comprehensive systematic scoping review as have previously been undertaken [ 6 , 31 ]. The number of scoping reviews that are published has increased rapidly, and this would now be difficult to undertake. As this was a sample, not all relevant scoping reviews or evidence maps that would have met our criteria were included. We used machine learning to screen our search results for pragmatic reasons (to reduce screening time), but we do not see any reason that our sample would not be broadly reflective of the wider literature.
Data visualisation, and in particular more innovative examples of it, is currently underused in published scoping reviews on health topics. The examples that we have found highlight the wide range of methods that scoping review authors could draw upon to present their data in an engaging way. In particular, we believe that interactive data visualisation has significant potential for mapping the available literature on a topic. Appropriate use of data visualisation may increase the usefulness, and thus uptake, of scoping reviews as a way of identifying existing evidence or research gaps by decision-makers, researchers and commissioners of research. We recommend that scoping review authors explore the extensive free resources and online tools available for data visualisation. However, we also think that it would be useful for publishers to explore allowing easier integration of interactive tools into academic publishing, given the fact that papers are now predominantly accessed online. Future research may be helpful to explore which methods are particularly useful to scoping review users.
Availability of data and materials
The datasets used and/or analysed during the current study are available from the corresponding author on reasonable request.
Abbreviations
Organisation formerly known as Joanna Briggs Institute
Preferred Reporting Items for Systematic Reviews and Meta-Analyses
Munn Z, Pollock D, Khalil H, Alexander L, McLnerney P, Godfrey CM, Peters M, Tricco AC. What are scoping reviews? Providing a formal definition of scoping reviews as a type of evidence synthesis. JBI Evid Synth. 2022;20:950–952.
Peters MDJ, Marnie C, Colquhoun H, Garritty CM, Hempel S, Horsley T, Langlois EV, Lillie E, O’Brien KK, Tunçalp Ӧ, et al. Scoping reviews: reinforcing and advancing the methodology and application. Syst Rev. 2021;10:263.
Article PubMed PubMed Central Google Scholar
Munn Z, Peters MDJ, Stern C, Tufanaru C, McArthur A, Aromataris E. Systematic review or scoping review? Guidance for authors when choosing between a systematic or scoping review approach. BMC Med Res Methodol. 2018;18:143.
Sutton A, Clowes M, Preston L, Booth A. Meeting the review family: exploring review types and associated information retrieval requirements. Health Info Libr J. 2019;36:202–22.
Article PubMed Google Scholar
Miake-Lye IM, Hempel S, Shanman R, Shekelle PG. What is an evidence map? A systematic review of published evidence maps and their definitions, methods, and products. Syst Rev. 2016;5:28.
Tricco AC, Lillie E, Zarin W, O’Brien K, Colquhoun H, Kastner M, Levac D, Ng C, Sharpe JP, Wilson K, et al. A scoping review on the conduct and reporting of scoping reviews. BMC Med Res Methodol. 2016;16:15.
Khalil H, Peters MDJ, Tricco AC, Pollock D, Alexander L, McInerney P, Godfrey CM, Munn Z. Conducting high quality scoping reviews-challenges and solutions. J Clin Epidemiol. 2021;130:156–60.
Lockwood C, dos Santos KB, Pap R. Practical guidance for knowledge synthesis: scoping review methods. Asian Nurs Res. 2019;13:287–94.
Article Google Scholar
Pollock D, Peters MDJ, Khalil H, McInerney P, Alexander L, Tricco AC, Evans C, de Moraes ÉB, Godfrey CM, Pieper D, et al. Recommendations for the extraction, analysis, and presentation of results in scoping reviews. JBI Evidence Synthesis. 2022;10:11124.
Google Scholar
Peters MDJ GC, McInerney P, Munn Z, Tricco AC, Khalil, H. Chapter 11: Scoping reviews (2020 version). In: Aromataris E MZ, editor. JBI Manual for Evidence Synthesis. JBI; 2020. Available from https://synthesismanual.jbi.global . Accessed 1 Feb 2023.
Tableau Public. https://www.tableau.com/en-gb/products/public . Accessed 24 January 2023.
flourish.studio. https://flourish.studio/ . Accessed 24 January 2023.
Chishtie J, Bielska IA, Barrera A, Marchand J-S, Imran M, Tirmizi SFA, Turcotte LA, Munce S, Shepherd J, Senthinathan A, et al. Interactive visualization applications in population health and health services research: systematic scoping review. J Med Internet Res. 2022;24: e27534.
Isett KR, Hicks DM. Providing public servants what they need: revealing the “unseen” through data visualization. Public Adm Rev. 2018;78:479–85.
Carroll LN, Au AP, Detwiler LT, Fu T-c, Painter IS, Abernethy NF. Visualization and analytics tools for infectious disease epidemiology: a systematic review. J Biomed Inform. 2014;51:287–298.
Lundkvist A, El-Khatib Z, Kalra N, Pantoja T, Leach-Kemon K, Gapp C, Kuchenmüller T. Policy-makers’ views on translating burden of disease estimates in health policies: bridging the gap through data visualization. Arch Public Health. 2021;79:17.
Zakkar M, Sedig K. Interactive visualization of public health indicators to support policymaking: an exploratory study. Online J Public Health Inform. 2017;9:e190–e190.
Park S, Bekemeier B, Flaxman AD. Understanding data use and preference of data visualization for public health professionals: a qualitative study. Public Health Nurs. 2021;38:531–41.
Kossmeier M, Tran US, Voracek M. Charting the landscape of graphical displays for meta-analysis and systematic reviews: a comprehensive review, taxonomy, and feature analysis. BMC Med Res Methodol. 2020;20:26.
Ribecca, S. The Data Visualisation Catalogue. https://datavizcatalogue.com/index.html . Accessed 23 November 2021.
Ferdio. Data Viz Project. https://datavizproject.com/ . Accessed 23 November 2021.
Golden TL, Springs S, Kimmel HJ, Gupta S, Tiedemann A, Sandu CC, Magsamen S. The use of music in the treatment and management of serious mental illness: a global scoping review of the literature. Front Psychol. 2021;12: 649840.
Keshava C, Davis JA, Stanek J, Thayer KA, Galizia A, Keshava N, Gift J, Vulimiri SV, Woodall G, Gigot C, et al. Application of systematic evidence mapping to assess the impact of new research when updating health reference values: a case example using acrolein. Environ Int. 2020;143: 105956.
Article CAS PubMed PubMed Central Google Scholar
Jayakumar P, Lin E, Galea V, Mathew AJ, Panda N, Vetter I, Haynes AB. Digital phenotyping and patient-generated health data for outcome measurement in surgical care: a scoping review. J Pers Med. 2020;10:282.
Qu LG, Perera M, Lawrentschuk N, Umbas R, Klotz L. Scoping review: hotspots for COVID-19 urological research: what is being published and from where? World J Urol. 2021;39:3151–60.
Article CAS PubMed Google Scholar
Rossa-Roccor V, Acheson ES, Andrade-Rivas F, Coombe M, Ogura S, Super L, Hong A. Scoping review and bibliometric analysis of the term “planetary health” in the peer-reviewed literature. Front Public Health. 2020;8:343.
Hewitt L, Dahlen HG, Hartz DL, Dadich A. Leadership and management in midwifery-led continuity of care models: a thematic and lexical analysis of a scoping review. Midwifery. 2021;98: 102986.
Xia H, Tan S, Huang S, Gan P, Zhong C, Lu M, Peng Y, Zhou X, Tang X. Scoping review and bibliometric analysis of the most influential publications in achalasia research from 1995 to 2020. Biomed Res Int. 2021;2021:8836395.
Vigliotti V, Taggart T, Walker M, Kusmastuti S, Ransome Y. Religion, faith, and spirituality influences on HIV prevention activities: a scoping review. PLoS ONE. 2020;15: e0234720.
van Heemskerken P, Broekhuizen H, Gajewski J, Brugha R, Bijlmakers L. Barriers to surgery performed by non-physician clinicians in sub-Saharan Africa-a scoping review. Hum Resour Health. 2020;18:51.
Pham MT, Rajić A, Greig JD, Sargeant JM, Papadopoulos A, McEwen SA. A scoping review of scoping reviews: advancing the approach and enhancing the consistency. Res Synth Methods. 2014;5:371–85.
Peters MDJ, Marnie C, Tricco AC, Pollock D, Munn Z, Alexander L, McInerney P, Godfrey CM, Khalil H. Updated methodological guidance for the conduct of scoping reviews. JBI Evid Synth. 2020;18:2119–26.
Tricco AC, Lillie E, Zarin W, O’Brien KK, Colquhoun H, Levac D, Moher D, Peters MDJ, Horsley T, Weeks L, et al. PRISMA Extension for Scoping Reviews (PRISMA-ScR): checklist and explanation. Ann Intern Med. 2018;169:467–73.
Nyanchoka L, Tudur-Smith C, Thu VN, Iversen V, Tricco AC, Porcher R. A scoping review describes methods used to identify, prioritize and display gaps in health research. J Clin Epidemiol. 2019;109:99–110.
Wolffe TAM, Whaley P, Halsall C, Rooney AA, Walker VR. Systematic evidence maps as a novel tool to support evidence-based decision-making in chemicals policy and risk management. Environ Int. 2019;130:104871.
Digital Solution Foundry and EPPI-Centre. EPPI-Mapper, Version 2.0.1. EPPI-Centre, UCL Social Research Institute, University College London. 2020. https://eppi.ioe.ac.uk/cms/Default.aspx?tabid=3790 .
South E, Rodgers M, Wright K, Whitehead M, Sowden A. Reducing lifestyle risk behaviours in disadvantaged groups in high-income countries: a scoping review of systematic reviews. Prev Med. 2022;154: 106916.
Download references
Acknowledgements
We would like to thank Melissa Harden, Senior Information Specialist, Centre for Reviews and Dissemination, for advice on developing the search strategy.
This work received no external funding.
Author information
Authors and affiliations.
Centre for Reviews and Dissemination, University of York, York, YO10 5DD, UK
Emily South & Mark Rodgers
You can also search for this author in PubMed Google Scholar
Contributions
Both authors conceptualised and designed the study and contributed to screening, data extraction and the interpretation of results. ES undertook the literature searches, analysed data, produced the data visualisations and drafted the manuscript. MR contributed to revising the manuscript, and both authors read and approved the final version.
Corresponding author
Correspondence to Emily South .
Ethics declarations
Ethics approval and consent to participate.
Not applicable.
Consent for publication
Competing interests.
The authors declare that they have no competing interests.
Additional information
Publisher’s note.
Springer Nature remains neutral with regard to jurisdictional claims in published maps and institutional affiliations.
Supplementary Information
Additional file 1..
Typology of data visualisation methods.
Additional file 2.
References of scoping reviews included in main dataset.
Rights and permissions
Open Access This article is licensed under a Creative Commons Attribution 4.0 International License, which permits use, sharing, adaptation, distribution and reproduction in any medium or format, as long as you give appropriate credit to the original author(s) and the source, provide a link to the Creative Commons licence, and indicate if changes were made. The images or other third party material in this article are included in the article's Creative Commons licence, unless indicated otherwise in a credit line to the material. If material is not included in the article's Creative Commons licence and your intended use is not permitted by statutory regulation or exceeds the permitted use, you will need to obtain permission directly from the copyright holder. To view a copy of this licence, visit http://creativecommons.org/licenses/by/4.0/ . The Creative Commons Public Domain Dedication waiver ( http://creativecommons.org/publicdomain/zero/1.0/ ) applies to the data made available in this article, unless otherwise stated in a credit line to the data.
Reprints and permissions
About this article
Cite this article.
South, E., Rodgers, M. Data visualisation in scoping reviews and evidence maps on health topics: a cross-sectional analysis. Syst Rev 12 , 142 (2023). https://doi.org/10.1186/s13643-023-02309-y
Download citation
Received : 21 February 2023
Accepted : 07 August 2023
Published : 17 August 2023
DOI : https://doi.org/10.1186/s13643-023-02309-y
Share this article
Anyone you share the following link with will be able to read this content:
Sorry, a shareable link is not currently available for this article.
Provided by the Springer Nature SharedIt content-sharing initiative
- Scoping review
- Evidence map
- Data visualisation
Systematic Reviews
ISSN: 2046-4053
- Submission enquiries: Access here and click Contact Us
- General enquiries: [email protected]

IMAGES
VIDEO
COMMENTS
Table of contents. Step 1: Write your hypotheses and plan your research design. Step 2: Collect data from a sample. Step 3: Summarize your data with descriptive statistics. Step 4: Test hypotheses or make estimates with inferential statistics.
For many researchers unfamiliar with qualitative research, determining how to conduct qualitative analyses is often quite challenging. Part of this challenge is due to the seemingly limitless approaches that a qualitative researcher might leverage, as well as simply learning to think like a qualitative researcher when analyzing data. From framework analysis (Ritchie & Spencer, 1994) to content ...
tative research is not in the mainstream of research and not at the core of methods training or teaching in general, ongoing research increasingly includes qualitative studies. These developments have led to an inter-esting gap, which forms a second level of proliferation: a variety of methods and approaches for data analysis have been
This article is a practical guide to conducting data analysis in general literature reviews. The general literature review is a synthesis and analysis of published research on a relevant clinical issue, and is a common format for academic theses at the bachelor's and master's levels in nursing, physiotherapy, occupational therapy, public health and other related fields.
INTRODUCTION. Scientific research is usually initiated by posing evidenced-based research questions which are then explicitly restated as hypotheses.1,2 The hypotheses provide directions to guide the study, solutions, explanations, and expected results.3,4 Both research questions and hypotheses are essentially formulated based on conventional theories and real-world processes, which allow the ...
Data Analysis and Presentation Techniques that Apply to both Survey and Interview Research. Create a documentation of the data and the process of data collection. Analyze the data rather than just describing it - use it to tell a story that focuses on answering the research question. Use charts or tables to help the reader understand the data ...
nominal. It is important to know w hat kind of data you are planning to collect or analyse as this w ill. affect your analysis method. A 12 step approach to quantitative data analysis. Step 1 ...
Definition of research in data analysis: According to LeCompte and Schensul, research data analysis is a process used by researchers to reduce data to a story and interpret it to derive insights. The data analysis process helps reduce a large chunk of data into smaller fragments, which makes sense. Three essential things occur during the data ...
How to conduct qualitative research? Given that qualitative research is characterised by flexibility, openness and responsivity to context, the steps of data collection and analysis are not as separate and consecutive as they tend to be in quantitative research [13, 14].As Fossey puts it: "sampling, data collection, analysis and interpretation are related to each other in a cyclical ...
quantitative (numbers) and qualitative (words or images) data. The combination of. quantitative and qualitative research methods is called mixed methods. For example, first, numerical data are ...
The Ultimate Guide to Qualitative Research - Part 1: The Basics. Learn the essential building blocks of qualitative research. This section covers everything from key definitions to research design, data collection methods, and important ethical considerations. Understand what qualitative research is and how it differs from quantitative research.
User Research Analysis Guide July 31, 2023. By Meg McMahon Analyzing the notes from a user research study is an essential part of answering the research questions that prompted the study. During the analysis, you or your team decide what insights are generated from the notes taken about the research studies. The insights should relate to the ...
Qualitative Research. Qualitative research is a type of research methodology that focuses on exploring and understanding people's beliefs, attitudes, behaviors, and experiences through the collection and analysis of non-numerical data. It seeks to answer research questions through the examination of subjective data, such as interviews, focus ...
Here are some tips for making user research analysis faster and easier on upcoming projects: Begin the user research by creating well defined questions and goals. Create tags based on each goal. Tag research notes and data as it's collected to speed up analysis later. Debrief after each research session.
This Guide is presented by the IPR Measurement Commission. The Communicator's Guide to Research, Analysis, and Evaluation was created to help public relations leaders understand how they can apply data, research, and analytics to uncover insights that inform strategic decision making, improve communication performance, and deliver meaningful ...
Step 1: Study for a degree. A bachelor's degree in a business-related subject, math, economics, or social science is typically the entry point to work as a research analyst, with some employers asking for a master's degree. According to Zippia, 70 percent of research analysts have a bachelor's degree, with a further 18 percent going on to ...
Research-based . evaluation to assess . the extent to which . the program met or exceeded the original research-based objectives. IV. TACTICAL CREATION. AND ACTIVATION: Research-based program execution to . pretest tactics and campaigns for their . ability to captivate stakeholders. III. DEVELOPING STRATEGY: Interpretation of research to ...
This will guide the entire process. 2. Collect and consolidate your data. Gather your data from all relevant sources using data analysis software. Ensure that the data is representative and actually covers the variables you want to analyze. 3. Select your analytical methods.
Scoping reviews and evidence maps are forms of evidence synthesis that aim to map the available literature on a topic and are well-suited to visual presentation of results. A range of data visualisation methods and interactive data visualisation tools exist that may make scoping reviews more useful to knowledge users. The aim of this study was to explore the use of data visualisation in a ...
GREET is the go-to tool to guide decision-making, research and development, and regulations related to transportation because it can assess a range of life-cycle energy, ... Regulated Emissions, and Energy use in Technologies) life cycle analysis (LCA) suite of models in 1994, with the first version released in 1995. Life cycle analysis is a ...
Reviewer: R. Sambasiva Rao. Fuzzy set theory, as propounded by Lotfi Zadeh in 1965, has grown by leaps and bounds, in applications as well as in its theoretical aspects. The major disciplines contributing to intelligent tools are neural nets, genetic algorithms, second-level expert systems, and fuzzy theory …. (More)
Step 4: Create a research design. The research design is a practical framework for answering your research questions. It involves making decisions about the type of data you need, the methods you'll use to collect and analyze it, and the location and timescale of your research. There are often many possible paths you can take to answering ...
There has also been a major change in where guns have been stolen from over the past decade. 10 Everytown Research analysis of FBI NIBRS data of 159 cities, 2013-2022, and ACS population data, 2013-2022, accessed January 2024. The rate of gun theft from cars was 39.9 in 2019, prior to the pandemic, and 60.2 in 2021, the last full year of ...
New research into B2B content marketing trends for 2024 reveals specifics of AI implementation, social media use, and budget forecasts, plus content success factors. Marketers talk AI, common challenges, best results, and more in the 14th annual B2B Content Marketing Benchmarks, Budgets, and Trends: Outlook for 2024.
1) First, tap "Futures" on the website homepage. 2) Select a product you would like to trade from the list here. BTCC currently offers daily and perpetual futures. 3) Check time to settlement. The settlement time of these futures type is different, you can check the time for settlement for each product here.