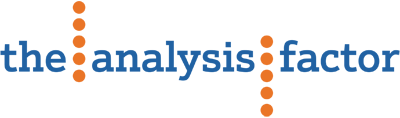

Cohort and Case-Control Studies: Pro’s and Con’s
by guest contributer 16 Comments
Two designs commonly used in epidemiology are the cohort and case-control studies. Both study causal relationships between a risk factor and a disease. What is the difference between these two designs? And when should you opt for the one or the other?
Cohort studies
Cohort studies begin with a group of people (a cohort) free of disease. The people in the cohort are grouped by whether or not they are exposed to a potential cause of disease. The whole cohort is followed over time to see if the development of new cases of the disease (or other outcome) differs between the groups with and without exposure.
For example, you could do a cohort study if you suspect there might be a causal relationship between the use of a certain water source and the incidence of diarrhea among children under five in a village with different water sources.
You select a group of children under five years, either all children of that age in the village, a random sample taken from the population register, or e.g. children living in the same area, or attending the same clinic. Then you classify them as either using the suspected water source or other water sources. You check e.g. after two weeks whether the children have had diarrhea.
You can then calculate how many diarrhea cases there were among those children using the suspected water source and those using other sources of water supply (cumulative incidence of diarrhea).
Case-control studies
The same problem could also be studied in a case-control study. A case-control study begins with the selection of cases (people with a disease) and controls (people without the disease). The controls should represent people who would have been study cases if they had developed the disease (population at risk).
The exposure status to a potential cause of disease is determined for both cases and controls. Then the occurrence of the possible cause of the disease could be calculated for both the cases and controls. To come back to the example, you may compare children who present themselves at a health center with diarrhea (cases) with children with other complaints, for example acute respiratory infections (controls). You determine which source of drinking water they had used. Then calculate the proportion of cases and controls that were exposed to the suspected water source.
Pro’s and con’s
On what basis do you decide to choose a cohort design or a case-control design ?
Cohort studies provide the best information about the causation of disease because you follow persons from exposure to the occurrence of the disease. With data from cohort studies you can calculate cumulative incidences. Cumulative incidences are the most direct measurement of the risk of developing disease.
An added advantage is that you can examine a range of outcomes/diseases caused by one exposure. For example, when heart disease, lung disease, renal disease are caused by smoking.
However, cohort studies are major undertakings. They may require long periods of follow-up since disease may occur a long time after exposure. Therefore, it is a very expensive study design.
Cohort studies work well for rare exposures –you can specifically select people exposed to a certain factor. But this design does not work for rare diseases –you would then need a large study group to find sufficient disease cases.
Case-control studies are relatively simple to conduct. They do not require a long follow-up period (as the disease has already developed), and are hence much cheaper. This design is especially useful for rare diseases (as you select the cases yourself), but not for rare causes (as you will probably not find these in sufficient number in your study). It is also very suitable for diseases with a long latent period, such as cancer.
However, case-control studies are less adept at showing a causal relationship than cohort studies. They are more prone to bias.
One example is recall bias: cases might recall certain exposures more clearly than controls, simply due to the fact that they have thought about what could have caused their disease.
by Annette Gerritsen, Ph.D.
About the Author: With expertise in epidemiology, biostatistics and quantitative research projects, Annette Gerritsen, Ph.D. provides s ervices to her clients focussing on the methodological soundness of each phase of an epidemiological study to ensure getting valid answers to the proposed research questions. She is the founder of Epi Result .

Reader Interactions
December 2, 2020 at 2:18 pm
Thank a a lot for simple explaining it clearly.
March 31, 2020 at 2:47 am
March 24, 2020 at 11:49 pm
what would be best study design to find out causal relationship between lung cancer and smoking?
February 4, 2019 at 8:32 pm
Super clear love it I’m only in eighth grade. I’m doing science Olympiad and this really helped me for disease detectives!!
June 5, 2018 at 1:17 am
The article is very easy to understand. Thank you very much!!!!
March 21, 2018 at 5:12 am
well understood thanks
November 14, 2017 at 8:44 pm
This was so helpful! Clear and concise.
May 10, 2017 at 10:45 am
the article is well simplified for easy understanding. Thanks.
July 14, 2014 at 1:49 pm
Well written, simple and clear explanation. I m no longer confused now! Thanks
November 4, 2013 at 1:53 am
Dear Annette Gerritsen, could you please explain the difference of interpretation between RR in cohort study and OR in case control study? I have read some books but it’s still not clear.
Best regards,
Sarumpaet Sori Muda
September 14, 2013 at 4:24 am
The article is clear,well written and easy to understand
April 18, 2013 at 11:22 pm
Wonderful work!!! Very clear than wat we were taught n class…….
April 16, 2013 at 2:50 pm
This article helped me much,it is self explanatory Thank you.
February 13, 2013 at 5:37 am
The article is clear. However, I would like you to help me on the methodology of a study that I would like to do.
I would like to do an intervention whereby I was thinking that data in the registers before the intervention would be used as a baseline and after the intervention would compare the treatment outcomes for baseline and end-line.
May you help me with how best can I can put this idea on paper?
December 21, 2012 at 8:09 pm
This article is really well written .It helped me alot to solve my confusion.thanks
October 14, 2012 at 7:14 pm
This article is well written out. It helped me answer questions on mid term take home exams. Thank you very much.
Leave a Reply Cancel reply
Your email address will not be published. Required fields are marked *
Privacy Overview
- En español – ExME
- Em português – EME
Case-control and Cohort studies: A brief overview
Posted on 6th December 2017 by Saul Crandon
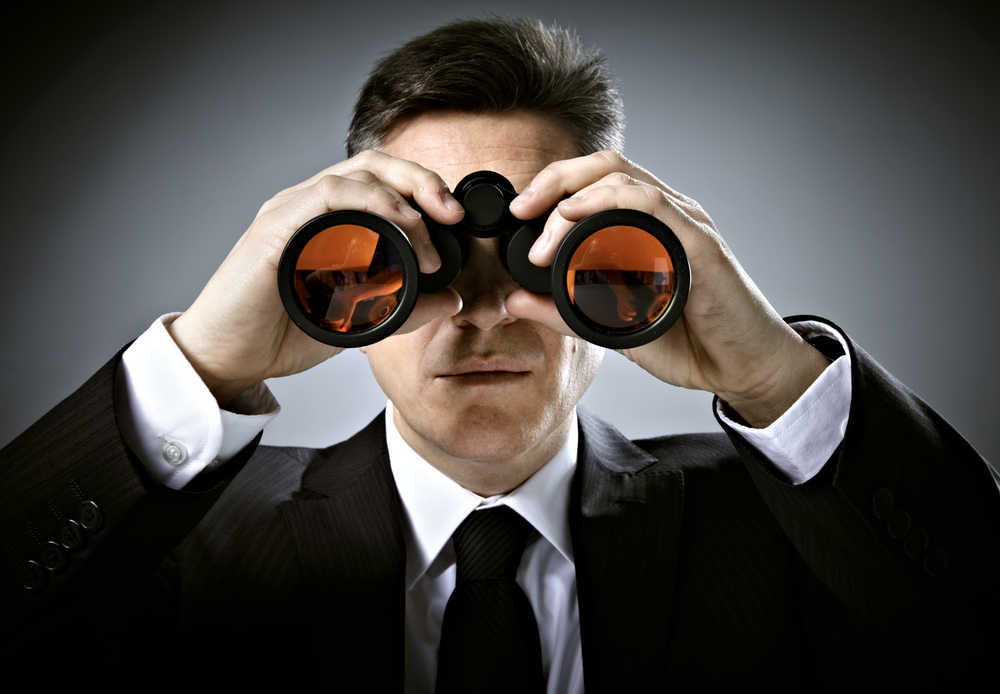
Introduction
Case-control and cohort studies are observational studies that lie near the middle of the hierarchy of evidence . These types of studies, along with randomised controlled trials, constitute analytical studies, whereas case reports and case series define descriptive studies (1). Although these studies are not ranked as highly as randomised controlled trials, they can provide strong evidence if designed appropriately.
Case-control studies
Case-control studies are retrospective. They clearly define two groups at the start: one with the outcome/disease and one without the outcome/disease. They look back to assess whether there is a statistically significant difference in the rates of exposure to a defined risk factor between the groups. See Figure 1 for a pictorial representation of a case-control study design. This can suggest associations between the risk factor and development of the disease in question, although no definitive causality can be drawn. The main outcome measure in case-control studies is odds ratio (OR) .
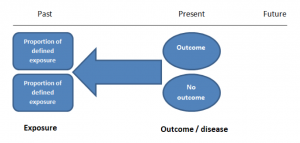
Figure 1. Case-control study design.
Cases should be selected based on objective inclusion and exclusion criteria from a reliable source such as a disease registry. An inherent issue with selecting cases is that a certain proportion of those with the disease would not have a formal diagnosis, may not present for medical care, may be misdiagnosed or may have died before getting a diagnosis. Regardless of how the cases are selected, they should be representative of the broader disease population that you are investigating to ensure generalisability.
Case-control studies should include two groups that are identical EXCEPT for their outcome / disease status.
As such, controls should also be selected carefully. It is possible to match controls to the cases selected on the basis of various factors (e.g. age, sex) to ensure these do not confound the study results. It may even increase statistical power and study precision by choosing up to three or four controls per case (2).
Case-controls can provide fast results and they are cheaper to perform than most other studies. The fact that the analysis is retrospective, allows rare diseases or diseases with long latency periods to be investigated. Furthermore, you can assess multiple exposures to get a better understanding of possible risk factors for the defined outcome / disease.
Nevertheless, as case-controls are retrospective, they are more prone to bias. One of the main examples is recall bias. Often case-control studies require the participants to self-report their exposure to a certain factor. Recall bias is the systematic difference in how the two groups may recall past events e.g. in a study investigating stillbirth, a mother who experienced this may recall the possible contributing factors a lot more vividly than a mother who had a healthy birth.
A summary of the pros and cons of case-control studies are provided in Table 1.
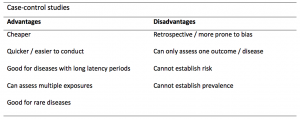
Table 1. Advantages and disadvantages of case-control studies.
Cohort studies
Cohort studies can be retrospective or prospective. Retrospective cohort studies are NOT the same as case-control studies.
In retrospective cohort studies, the exposure and outcomes have already happened. They are usually conducted on data that already exists (from prospective studies) and the exposures are defined before looking at the existing outcome data to see whether exposure to a risk factor is associated with a statistically significant difference in the outcome development rate.
Prospective cohort studies are more common. People are recruited into cohort studies regardless of their exposure or outcome status. This is one of their important strengths. People are often recruited because of their geographical area or occupation, for example, and researchers can then measure and analyse a range of exposures and outcomes.
The study then follows these participants for a defined period to assess the proportion that develop the outcome/disease of interest. See Figure 2 for a pictorial representation of a cohort study design. Therefore, cohort studies are good for assessing prognosis, risk factors and harm. The outcome measure in cohort studies is usually a risk ratio / relative risk (RR).
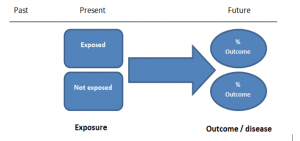
Figure 2. Cohort study design.
Cohort studies should include two groups that are identical EXCEPT for their exposure status.
As a result, both exposed and unexposed groups should be recruited from the same source population. Another important consideration is attrition. If a significant number of participants are not followed up (lost, death, dropped out) then this may impact the validity of the study. Not only does it decrease the study’s power, but there may be attrition bias – a significant difference between the groups of those that did not complete the study.
Cohort studies can assess a range of outcomes allowing an exposure to be rigorously assessed for its impact in developing disease. Additionally, they are good for rare exposures, e.g. contact with a chemical radiation blast.
Whilst cohort studies are useful, they can be expensive and time-consuming, especially if a long follow-up period is chosen or the disease itself is rare or has a long latency.
A summary of the pros and cons of cohort studies are provided in Table 2.
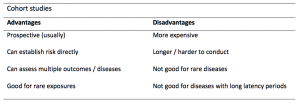
The Strengthening of Reporting of Observational Studies in Epidemiology Statement (STROBE)
STROBE provides a checklist of important steps for conducting these types of studies, as well as acting as best-practice reporting guidelines (3). Both case-control and cohort studies are observational, with varying advantages and disadvantages. However, the most important factor to the quality of evidence these studies provide, is their methodological quality.
- Song, J. and Chung, K. Observational Studies: Cohort and Case-Control Studies . Plastic and Reconstructive Surgery.  2010 Dec;126(6):2234-2242.
- Ury HK. Efficiency of case-control studies with multiple controls per case: Continuous or dichotomous data . Biometrics . 1975 Sep;31(3):643–649.
- von Elm E, Altman DG, Egger M, Pocock SJ, Gøtzsche PC, Vandenbroucke JP; STROBE Initiative. The Strengthening the Reporting of Observational Studies in Epidemiology (STROBE) statement: guidelines for reporting observational studies.  Lancet 2007 Oct;370(9596):1453-14577. PMID: 18064739.
Saul Crandon
Leave a reply cancel reply.
Your email address will not be published. Required fields are marked *
Save my name, email, and website in this browser for the next time I comment.
No Comments on Case-control and Cohort studies: A brief overview
Very well presented, excellent clarifications. Has put me right back into class, literally!
Very clear and informative! Thank you.
very informative article.
Thank you for the easy to understand blog in cohort studies. I want to follow a group of people with and without a disease to see what health outcomes occurs to them in future such as hospitalisations, diagnoses, procedures etc, as I have many health outcomes to consider, my questions is how to make sure these outcomes has not occurred before the “exposure disease”. As, in cohort studies we are looking at incidence (new) cases, so if an outcome have occurred before the exposure, I can leave them out of the analysis. But because I am not looking at a single outcome which can be checked easily and if happened before exposure can be left out. I have EHR data, so all the exposure and outcome have occurred. my aim is to check the rates of different health outcomes between the exposed)dementia) and unexposed(non-dementia) individuals.
Very helpful information
Thanks for making this subject student friendly and easier to understand. A great help.
Thanks a lot. It really helped me to understand the topic. I am taking epidemiology class this winter, and your paper really saved me.
Happy new year.
Wow its amazing n simple way of briefing ,which i was enjoyed to learn this.its very easy n quick to pick ideas .. Thanks n stay connected
Saul you absolute melt! Really good work man
am a student of public health. This information is simple and well presented to the point. Thank you so much.
very helpful information provided here
really thanks for wonderful information because i doing my bachelor degree research by survival model
Quite informative thank you so much for the info please continue posting. An mph student with Africa university Zimbabwe.
Thank you this was so helpful amazing
Apreciated the information provided above.
So clear and perfect. The language is simple and superb.I am recommending this to all budding epidemiology students. Thanks a lot.
Great to hear, thank you AJ!
I have recently completed an investigational study where evidence of phlebitis was determined in a control cohort by data mining from electronic medical records. We then introduced an intervention in an attempt to reduce incidence of phlebitis in a second cohort. Again, results were determined by data mining. This was an expedited study, so there subjects were enrolled in a specific cohort based on date(s) of the drug infused. How do I define this study? Thanks so much.
thanks for the information and knowledge about observational studies. am a masters student in public health/epidemilogy of the faculty of medicines and pharmaceutical sciences , University of Dschang. this information is very explicit and straight to the point
Very much helpful
Subscribe to our newsletter
You will receive our monthly newsletter and free access to Trip Premium.
Related Articles
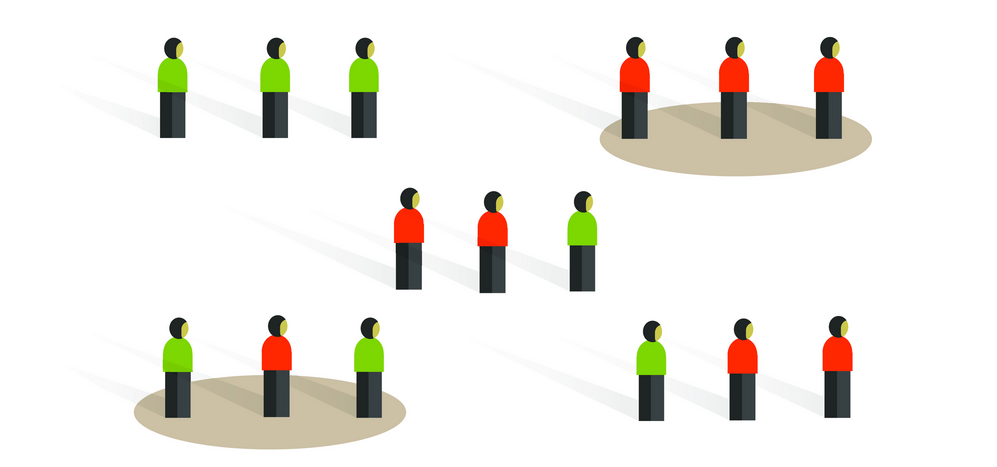
Cluster Randomized Trials: Concepts
This blog summarizes the concepts of cluster randomization, and the logistical and statistical considerations while designing a cluster randomized controlled trial.
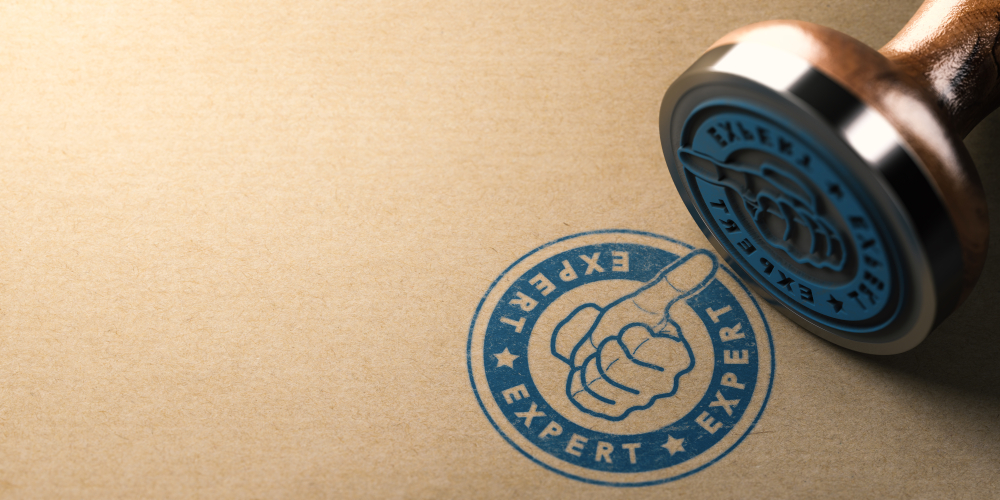
Expertise-based Randomized Controlled Trials
This blog summarizes the concepts of Expertise-based randomized controlled trials with a focus on the advantages and challenges associated with this type of study.
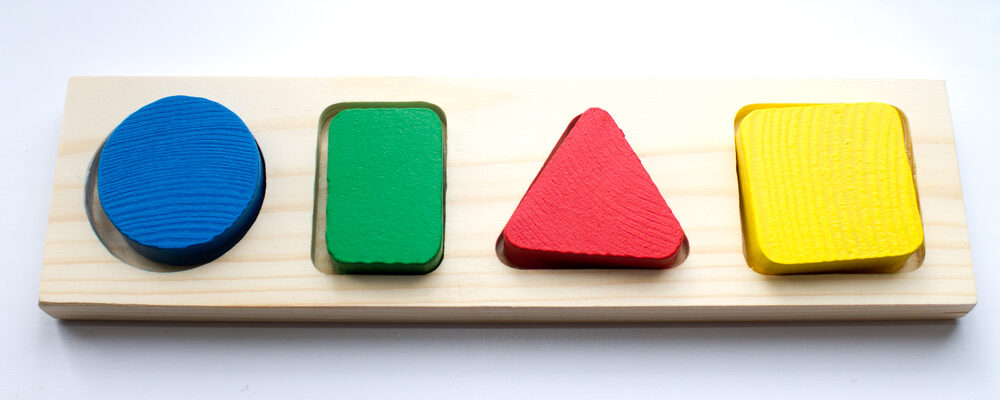
An introduction to different types of study design
Conducting successful research requires choosing the appropriate study design. This article describes the most common types of designs conducted by researchers.

Cohort Studies
- 1
- | 2
- | 3
- | 4
- | 5
- | 6

Advantages & Disadvantages of Cohort Studies
Disadvantages of prospective cohort studies, disadvantages of retrospective cohort studies.

Epi_Tools.XLSX
All Modules
Clarity of Temporal Sequence (Did the exposure precede the outcome?): Cohort studies more clearly indicate the temporal sequence between exposure and outcome, because in a cohort study, subjects are known to be disease-free at the beginning of the observation period when their exposure status is established. In case-control studies, one begins with diseased and non-diseased people and then acertains their prior exposures. This is a reasonable approach to establishing past exposures, but subjects may have difficulty remembering past exposures, and their recollection may be biased by having the outcome (recall bias).
Allow Calculation of Incidence: Cohort studies allow you to calculate the incidence of disease in exposure groups, so you can calculate:
- Absolute risk (incidence)
- Relative risk (risk ratio or rate ratio)
- Risk difference
- Attributable proportion (attributable risk %)
Facilitate Study of Rare Exposures: While a cohort design can be used to investigate common exposures (e.g., risk factors for cardiovascular disease and cancer in the Nurses' Health Study), they are particularly useful for evaluating the effects of rare or unusual exposures, because the investigators can make it a point to identify an adequate number of subjects who have an unusual exposure, e.g.,
- Exposure to toxic chemicals (Agent Orange)
- Adverse effects of drugs (e.g., thalidomide) or treatments (e.g., radiation treatments for ankylosing spondylitis)
- Unusual occupational exposures (e.g., asbestos, or solvents in tire manufacturing, )
Allow Examination of Multiple Effects of a Single Exposure

Avoid Selection Bias at Enrollment: Cohort studies, especially prospective cohort studies, reduce the possibility that the results will be biased by selecting subjects for the comparison group who may be more or less likely to have the outcome of interest, because in a cohort study the outcome is not known at baseline when exposure status is established. Nevertheless, selection bias can occur in retrospective cohort studies (since the outcomes have already occurred at the time of selection), and it can occur in prospective cohort studies as a result of differential loss to follow up.
The "Air Force Health Study" on agent orange illustrates these advantages.
- It was clear that the exposure preceded adverse outcomes among exposed subjects who developed problems.
- It was used to evaluate the effects of an unusual risk factors (agent orange).
- It allowed direct calculation of incidence rates.
- It enabled the investigators to study multiple outcomes of this single unusual exposure.
- The prospective component of the study was not biased by knowledge of outcome status, because the outcomes hadn't occurred at the time of enrollment.
Link to a v ideo on Agent Orange from the the New York Times

- You may have to follow large numbers of subjects for a long time.
- They can be very expensive and time consuming.
- They are not good for rare diseases.
- They are not good for diseases with a long latency.
- Differential loss to follow up can introduce bias.
- As with prospective cohort studies, they are not good for very rare diseases.
- If one uses records that were not designed for the study, the available data may be of poor quality.
- There is frequently an absence of data on potential confounding factors if the data was recorded in the past.
- It may be difficult to identify an appropriate exposed cohort and an appropriate comparison group.
- Differential losses to follow up can also bias retrospective cohort studies.
return to top | previous page | next page
Content ©2016. All Rights Reserved. Date last modified: September 21, 2016. Wayne W. LaMorte, MD, PhD, MPH
Case-control studies: advantages and disadvantages
Affiliation.
- 1 Centre for Medical and Healthcare Education, St George's, University of London, London, UK [email protected].
- PMID: 31419845
- DOI: 10.1136/bmj.f7707
Study Design 101
- Helpful formulas
- Finding specific study types
- Case Control Study
- Meta- Analysis
- Systematic Review
- Practice Guideline
- Randomized Controlled Trial
- Cohort Study
- Case Reports
A study that compares patients who have a disease or outcome of interest (cases) with patients who do not have the disease or outcome (controls), and looks back retrospectively to compare how frequently the exposure to a risk factor is present in each group to determine the relationship between the risk factor and the disease.
Case control studies are observational because no intervention is attempted and no attempt is made to alter the course of the disease. The goal is to retrospectively determine the exposure to the risk factor of interest from each of the two groups of individuals: cases and controls. These studies are designed to estimate odds.
Case control studies are also known as "retrospective studies" and "case-referent studies."
- Good for studying rare conditions or diseases
- Less time needed to conduct the study because the condition or disease has already occurred
- Lets you simultaneously look at multiple risk factors
- Useful as initial studies to establish an association
- Can answer questions that could not be answered through other study designs
Disadvantages
- Retrospective studies have more problems with data quality because they rely on memory and people with a condition will be more motivated to recall risk factors (also called recall bias).
- Not good for evaluating diagnostic tests because it’s already clear that the cases have the condition and the controls do not
- It can be difficult to find a suitable control group
Design pitfalls to look out for
Care should be taken to avoid confounding, which arises when an exposure and an outcome are both strongly associated with a third variable. Controls should be subjects who might have been cases in the study but are selected independent of the exposure. Cases and controls should also not be "over-matched."
Is the control group appropriate for the population? Does the study use matching or pairing appropriately to avoid the effects of a confounding variable? Does it use appropriate inclusion and exclusion criteria?
Fictitious Example
There is a suspicion that zinc oxide, the white non-absorbent sunscreen traditionally worn by lifeguards is more effective at preventing sunburns that lead to skin cancer than absorbent sunscreen lotions. A case-control study was conducted to investigate if exposure to zinc oxide is a more effective skin cancer prevention measure. The study involved comparing a group of former lifeguards that had developed cancer on their cheeks and noses (cases) to a group of lifeguards without this type of cancer (controls) and assess their prior exposure to zinc oxide or absorbent sunscreen lotions.
This study would be retrospective in that the former lifeguards would be asked to recall which type of sunscreen they used on their face and approximately how often. This could be either a matched or unmatched study, but efforts would need to be made to ensure that the former lifeguards are of the same average age, and lifeguarded for a similar number of seasons and amount of time per season.
Real-life Examples
Boubekri, M., Cheung, I., Reid, K., Wang, C., & Zee, P. (2014). Impact of windows and daylight exposure on overall health and sleep quality of office workers: a case-control pilot study . Journal of Clinical Sleep Medicine : JCSM : Official Publication of the American Academy of Sleep Medicine, 10 (6), 603-611. https://doi.org/10.5664/jcsm.3780
This pilot study explored the impact of exposure to daylight on the health of office workers (measuring well-being and sleep quality subjectively, and light exposure, activity level and sleep-wake patterns via actigraphy). Individuals with windows in their workplaces had more light exposure, longer sleep duration, and more physical activity. They also reported a better scores in the areas of vitality and role limitations due to physical problems, better sleep quality and less sleep disturbances.
Togha, M., Razeghi Jahromi, S., Ghorbani, Z., Martami, F., & Seifishahpar, M. (2018). Serum Vitamin D Status in a Group of Migraine Patients Compared With Healthy Controls: A Case-Control Study . Headache, 58 (10), 1530-1540. https://doi.org/10.1111/head.13423
This case-control study compared serum vitamin D levels in individuals who experience migraine headaches with their matched controls. Studied over a period of thirty days, individuals with higher levels of serum Vitamin D was associated with lower odds of migraine headache.
Related Formulas
- Odds ratio in an unmatched study
- Odds ratio in a matched study
Related Terms
A patient with the disease or outcome of interest.
Confounding
When an exposure and an outcome are both strongly associated with a third variable.
A patient who does not have the disease or outcome.
Matched Design
Each case is matched individually with a control according to certain characteristics such as age and gender. It is important to remember that the concordant pairs (pairs in which the case and control are either both exposed or both not exposed) tell us nothing about the risk of exposure separately for cases or controls.
Observed Assignment
The method of assignment of individuals to study and control groups in observational studies when the investigator does not intervene to perform the assignment.
Unmatched Design
The controls are a sample from a suitable non-affected population.
Now test yourself!
1. Case Control Studies are prospective in that they follow the cases and controls over time and observe what occurs.
a) True b) False
2. Which of the following is an advantage of Case Control Studies?
a) They can simultaneously look at multiple risk factors. b) They are useful to initially establish an association between a risk factor and a disease or outcome. c) They take less time to complete because the condition or disease has already occurred. d) b and c only e) a, b, and c
← Previous Next →
© 2011-2019, The Himmelfarb Health Sciences Library Questions? Ask us .

- Himmelfarb Intranet
- Privacy Notice
- Terms of Use
- GW is committed to digital accessibility. If you experience a barrier that affects your ability to access content on this page, let us know via the Accessibility Feedback Form .
Cookies on GOV.UK
We use some essential cookies to make this website work.
We’d like to set additional cookies to understand how you use GOV.UK, remember your settings and improve government services.
We also use cookies set by other sites to help us deliver content from their services.
You have accepted additional cookies. You can change your cookie settings at any time.
You have rejected additional cookies. You can change your cookie settings at any time.

Register to vote Register by 18 June to vote in the General Election on 4 July.
- Health and social care
- Public health
- Health improvement
Case-control study: comparative studies
How to use a case-control study to evaluate your digital health product.
This page is part of a collection of guidance on evaluating digital health products .
A case-control study is a type of observational study. It looks at 2 sets of participants. One group has the condition you are interested in (the cases) and one group does not have it (the controls).
In other respects, the participants in both groups are similar. You can then look at a particular factor that might have caused the condition, such as your digital product, and compare participants from the 2 groups in relation to that.
A case-control study is an observational study because you observe the effects on existing groups rather than designing an experiment where participants are allocated into different groups.
What to use it for
A case-control study can help you to find out if your digital product or service achieves its aims, so it can be useful when you have developed your product (summative evaluation).
It can be a useful method when it would be difficult or impossible to randomise participants, for example, if your product aims to help people with rare health conditions.
Case-control studies have many benefits.
- help to estimate the effects of your digital product when randomisation is not possible
- use existing data, which could be cheaper and easier
- operate with fewer participants compared to other designs
There can also be drawbacks of a case-control study.
For example:
- you need to pay careful attention to factors that may influence your results, confounding factors and biases – see explanation in ‘How to carry out a case-control study’ below
- there may be challenges when accessing pre-existing data
- you cannot draw definitive answers about the effects of your product as you haven’t randomly selected participants for your evaluation
How to carry out a case-control study
In a traditional case-control design, cases and controls are looked at retrospectively – that is, the health condition and the factor that might have caused it have already occurred when you start the study.
Sources of cases and controls typically include:
- routinely collected data at medical facilities
- disease registries
- cross-sectional surveys
Some researchers use the term prospective case-control study when, for example, a prospective group exposed to an intervention is compared to a retrospective control.
Choosing your control
Selecting an appropriate control is an important part of a case-control study. The comparison group should be as similar as possible to the source population that produced the cases. This means the participants will be similar to each other in terms of factors that may influence the outcomes you’re looking at. Ideally, they will only differ in whether they received your digital product (cases) or not (controls).
There are 2 main types of case-control design: matched and unmatched.
Essentially, in an unmatched case-control design, a shared control group is selected for all cases at random given certain attributes. In a matched case-control design, controls are selected case-by-case based on specified characteristics. You should pick characteristics that have an effect on the usage of digital devices and services.
Commonly used matching factors include:
- socio-economic status
However, think about other characteristics and attributes that might influence the use of your product, and the subsequent outcomes.
Confounding variables and biases
Confounding variables (variables other than the one you are interested in that may influence the results) and biases (errors that influence the sample selected and results observed) are important to consider when conducting any research. This is especially important in designs that are non-randomised.
- selection bias can happen when participants are assigned without randomisation
- attribution bias may occur when patients with unfavourable outcomes are less likely to attend follow-ups
Analysing your data
The analysis most commonly used in case-control studies is an odds ratio, which is the chance (odds) of the outcomes occurring in the case group versus the control group.
Example: Can telemedicine help with post-bariatric surgery care? A case-control design
In 2019, Wang and colleagues published a paper entitled Exploring the Effects of Telemedicine on Bariatric Surgery Follow-up: a Matched Case Control Study .
The study showed that people who go through bariatric surgery have better outcomes if they attend their follow-up appointments after surgery in comparison to those who do not. However, attending appointments can be challenging for people who live in remote areas. In Ontario, Canada, telemedicine suites were set up to enable healthcare provider-patient videoconferencing.
The researchers used a matched case-control study to investigate if telemedicine videoconferencing can support post-surgery appointment attendance rates in people who live further away from the hospital sites. They used the existing data from the bariatric surgery hospital programme to identify eligible patients.
All patients attending the bariatric surgery were offered telemedicine services. The cases were the participants who used telemedicine services; they were compared to those who did not (the controls).
Cases and controls were matched on various characteristics, specifically:
- time since bariatric surgery
- body mass index ( BMI )
- travel distance from the hospital site
Researchers measured:
- the percentage of appointments attended
- rates of dropout
- pre-and post-surgery weight and BMI
- various physical and psychological outcomes
They also calculated rurality index to classify patients into urban, non-urban and rural areas. These variables were used to compare cases (those who used telemedicine) and controls (those who did not).
During the study period, they identified that 487 patients of 1,262 who received bariatric surgery used telemedicine services. Of those, 192 agreed to participate in the study.
They found that patients who used telemedicine did as well as patients who attended in person, both in terms of appointment attendance rates and in terms of physical and psychological outcomes.
Moreover, the researchers found that the cases (telemedicine users) came from more rural areas than the controls. The authors argued that this demonstrated that telemedicine can help overcome the known challenges for patients in more rural areas to attend appointments.
Randomising patients to telemedicine or withdrawing the telemedicine would be difficult, undesirable and possibly unethical. Case-control was a good alternative to assess the potential impact on patient outcomes in a service that is already up and running.
More information and resources
A 2003 study by Mann provides an accessible overview of observational research methods, including an explanation of biases and confounding variables.
On the website for Strengthening the Reporting of Observational Studies in Epidemiology ( STROBE ), there is a checklist of items that should be included in reports of case-control studies .
A 2016 study by Pearce offers considerations for the analysis of a matched case-control study.
Examples of case-control studies in digital health
In a 2020 study by Heuvel and others , researchers assessed a new digital health tool to monitor women at increased risk of preeclampsia at home. They investigated if the digital tool allows for fewer antenatal visits without compromising women’s safety, and whether it positively affects pregnancy outcomes. This study used a prospective case group compared to a retrospective control group.
In a 2019 study by Depp and others , the research team examined whether schizophrenia symptoms were associated with mobility (measured using GPS sensors). They compared participants with schizophrenia to healthy controls and they found that less mobility was associated with greater symptoms of schizophrenia.
Related content
Is this page useful.
- Yes this page is useful
- No this page is not useful
Help us improve GOV.UK
Don’t include personal or financial information like your National Insurance number or credit card details.
To help us improve GOV.UK, we’d like to know more about your visit today. Please fill in this survey (opens in a new tab) .
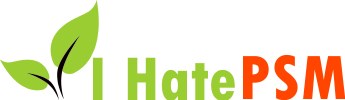
You are here
- [email protected]'s blog
Epidemiology: Advantages and Disadvantages of Case Control Studies
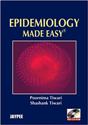
- Easy to conduct as no follow up is required
- No attrition, as no follow up is needed
- Gives faster results
- Inexpensive
- Suitable for rare and newly identified diseases
- More than one risk factors can be studied simultaneously
- Ethical problem lesser as disease has already occurred
Disadvantages
- The study depends upon the history given by the subject. Hence recall bias can occur
- Sometimes finding and appropriate control is difficult
- We can only establish an association between the disease and the risk factor as we don’t know what occurred first
- We can’t know the incidence hence can’t calculate the relative risk (RR) directly from a case-control study
Examples of case-control studies:
- Study of Reye’s syndrome
- Study of adenocarcinoma of vagina
- Oral contraceptives and thrombo-embolic phenomenon
- Thalidomide tragedy
References: Tiwari P. Epidemiology Made Easy. New Delhi: Jaypee Brothers; 2003 Gordis, L. (2014). Epidemiology (Fifth edition.). Philadelphia, PA: Elsevier Saunders. Bonita, R., Beaglehole, R., Kjellström, T., & World Health Organization. (2006). Basic epidemiology. Geneva: World Health Organization. Schneider, Dona, Lilienfeld, David E (Eds.), 4th ed. Lilienfeld’s Foundations of Epidemiology. New York: Oxford University Press; 2015 K. Park. Principles of Epidemiology and Epidemiologic Methods. In Park’s Textbook of Preventive and Social Medicine. 24th Ed. Jabalpur: Banarasidas Bhanot, 2017: pg 58 – 145
(adsbygoogle = window.adsbygoogle || []).push({ google_ad_client: "ca-pub-2296304216845141", enable_page_level_ads: true }); Steps in Investigation of an Epidemic: http://www.ihatepsm.com/blog/epidemiology-steps-investigation-epidemic Tests of Significance : http://www.ihatepsm.com/blog/epidemiology-tests-significance Monitoring and Evaluation : http://www.ihatepsm.com/blog/epidemiology-monitoring-and-evaluation Advantages and Disadvantages of Case Control Studies : http://www.ihatepsm.com/blog/epidemiology-advantages-and-disadvantages-c... Advantages and disadvantages of cohort study : http://www.ihatepsm.com/blog/epidemiology-advantages-and-disadvantages-c... Basic Concepts in Epidemiology: http://www.ihatepsm.com/blog/epidemiology-basic-concepts Types of Epidemiological Studies: http://www.ihatepsm.com/blog/epidemiology-types-epidemiological-studies Differences between Case – control and cohort study : http://www.ihatepsm.com/blog/epidemiology-differences-between-case-%E2%8... Uses of epidemiology: http://www.ihatepsm.com/blog/epidemiology-uses-epidemiology Blinding in Experimental Studies: http://www.ihatepsm.com/blog/blinding-experimental-studies Evaluation of a Screening Test : http://www.ihatepsm.com/blog/epidemiology-evaluation-screening-test

- Free Case Studies
- Business Essays
Write My Case Study
Buy Case Study
Case Study Help
- Case Study For Sale
- Case Study Services
- Hire Writer
Cohort Study vs Case-Control: Pros, Cons, and Differences
Case-control studies.
A case-control study is a kind of research design in which two subsisting groups varying in outcome are differentiated and classified on the basis of some conjectured casual characteristic.
Case-control research subjects chosen based on disease status and assessed for previous illness to a risk factor of interest. “Cases” are those determined to have the disease or outcome of interest. “Controls” are free from the disease or outcome of interest. The illness data can come from a variety of sources, like subsisting data in a medical record or by surveying the participants.You can think of this as a “flashback” study.
It accounts for the passage of time using a flashback technique to assess past characteristics or exposures and to groups of people, cases and controls.
Case-Control Study Design
At the present time, cases and controls are identified and past exposures are measured. The study determines the odds of having the exposure among cases and controls, and then compares these groups to determine the association between the exposure and the outcome. Unbiased selection of cases and controls is very crucial to this research design. Selection biases pose a substantial threat to the validity of study findings for this design.Case-control studies measure the probabilities of having an exposure or characteristics in the case and control populations.
These probabilities are then compared using the odds ratio – a measure of association.
Cohort Study
A cohort study, in other words a prospective study, is a research design which study subjects – disease-free at enrollment and chosen based exposure rank. Unexposed and exposed groups are followed for the same amount of time to determine who develops the disease of interest. This design meets the need to confirm a casual relation between exposure and outcome. Since the exposure is established first and the potential effect is captured prospectively, the temporal association is actually witnessed during the course of the study.You can think of it as a “motion picture” study.
This research design follows groups of individuals free from disease through a period of time in motion picture fashion to determine whether the disease develops.
Cohort Study Design
A cohort time at present time exposed and unexposed are recruited to be in the study, then they are followed prospectively to see if they amplify the outcome or disease of interest. The exposed and unexposed groups are then compared to determine the association with the outcome. Data from cohort studies are used to calculate the risk or rate of the health characteristics or disease.
Pros and Cons
Strengths of Case-Control Studies:
- Can be relatively quickly done;
- Can be relatively inexpensive;
- Good for diseases with short or long latency/duration;
- Good for rare diseases.
Weaknesses of Case-Control Studies:
- Inefficient for rare exposures;
- Usually unable to determine prevalence or the incidence in the population;
- More predisposed to bias, especially selection, and recall biases.
Strengths of Cohort Studies:
- Good for rare exposures;
- Exposure clearly precedes disease;
- Can examine multiple effects of an exposure;
- Able to determine incidence of disease in population.
Weaknesses of Cohort Studies:
- Can be expansive;
- Inefficient for rare diseases;
- Once begun, difficult to examine other study factors (exposures);
- The prospective study may take a long time if the disease has a long latency.
Related posts:
- Pros and Cons of a Matrix Organization
- Living Away from Home Country; pros and Cons
- Pros and Cons of Social Studies Teaching
- Pros and Cons if the Japanese MITI Model Issue
- Case Control vs Cohort Study
- Case Study: Fundamental Differences of Infrastructure
- What Is Cohort Study: Types, Study Design and Examples
Quick Links
Privacy Policy
Terms and Conditions
Testimonials
Our Services
Case Study Writing Services
Case Studies For Sale
Our Company
Welcome to the world of case studies that can bring you high grades! Here, at ACaseStudy.com, we deliver professionally written papers, and the best grades for you from your professors are guaranteed!
[email protected] 804-506-0782 350 5th Ave, New York, NY 10118, USA
Acasestudy.com © 2007-2019 All rights reserved.

Hi! I'm Anna
Would you like to get a custom case study? How about receiving a customized one?
Haven't Found The Case Study You Want?
For Only $13.90/page

An official website of the United States government
The .gov means it’s official. Federal government websites often end in .gov or .mil. Before sharing sensitive information, make sure you’re on a federal government site.
The site is secure. The https:// ensures that you are connecting to the official website and that any information you provide is encrypted and transmitted securely.
- Publications
- Account settings
Preview improvements coming to the PMC website in October 2024. Learn More or Try it out now .
- Advanced Search
- Journal List
- HHS Author Manuscripts

Observational Research Opportunities and Limitations
Edward j. boyko.
Epidemiologic Research and Information Center, VA Puget Sound Health Care System, Seattle, WA USA. University of Washington School of Medicine, Seattle, WA
Medical research continues to progress in its ability to identify treatments and characteristics associated with benefits and adverse outcomes. The principle engine for the evaluation of treatment efficacy is the randomized controlled trial (RCT). Due to the cost and other considerations, RCTs cannot address all clinically important decisions. Observational research often is used to address issues not addressed or not addressable by RCTs. This article provides an overview of the benefits and limitations of observational research to serve as a guide to the interpretation of this category of research designs in diabetes investigations. The potential for bias is higher in observational research but there are design and analysis features that can address these concerns although not completely eliminate them. Pharmacoepidemiologic research may provide important information regarding relative safety and effectiveness of diabetes pharmaceuticals. Such research must effectively address the important issue of confounding by indication in order to produce clinically meaningful results. Other methods such as instrumental variable analysis are being employed to enable stronger causal inference but these methods also require fulfillment of several key assumptions that may or may not be realistic. Nearly all clinical decisions involve probabilistic reasoning and confronting uncertainly, so a realistic goal for observational research may not be the high standard set by RCTs but instead the level of certainty needed to influence a diagnostic or treatment decision.
A major focus of medical research is the identification of causes of health outcomes, good and bad. The current gold standard method to accomplish this aim is the randomized controlled trial (RCT) ( Meldrum, 2000 ). The performance of a RCT requires strict specification of study conditions related to all aspects of its conduct, such as participant selection, treatment and control assignment arms, inclusion/exclusion criteria, randomization method, outcome measurement, and many other considerations. Such trials are difficult to mount due to the expense in terms of both time and money, and often lead to results that may be difficult to apply to a real-world setting due to either the rigor or complexity of the intervention or the selection process for participants that yields a population dissimilar from that seen in general clinical practice. A randomized controlled trial focuses on an assessment of the validity of its results at the expense of generalizability. For example, the Diabetes Prevention Program screened 158,177 subjects to yield 3,819 subjects who were eventually randomized to one of the four original arms ( Rubin et al., 2002 ). Other limitations of RCTs include a focus on treatment effects and not the ability to detect rarer adverse reactions; restrictions on diabetes duration at the time of trial entry, thereby yielding results that may not apply to persons with a different diabetes duration at the initiation of treatment; and high costs that limits the number of therapeutic comparisons. Regarding this last point, assessment of a new treatment for hyperglycemia requires comparison to existing accepted treatments, but the control population usually is restricted to fewer treatments than in current use, thereby limiting the ability to compare the new treatment to all existing treatments.
Given these considerations, observational research is often used to address important clinical questions in the absence of randomized clinical trial data, but may also make important potential contributions even when RCTs have been conducted. Examples include monitoring for long-term adverse events that did not appear during the time interval over which the RCT was conducted, or to assess whether the trial findings apply to a different population excluded from the trial due to younger or older age, gender, presence of comorbid conditions, or other factors. Observational research often also addresses other questions not suitable for randomized clinical trials, such as an exposure known to be harmful or in other ways unacceptable to participants or whose administration is inconsistent with ethical principles. Also, observational research can address other exposures that are not potentially under the control of the investigator, such as, for example, eye color, blood type, presence of a specific genetic marker, or elevations of blood pressure or plasma glucose concentration. Observational research may also provide preliminary data to justify the performance of a clinical trial, which might not have received sufficient funding support without the existence of such results.
This paper will review observational research methods applied to addressing questions of causation in diabetes research, with a particular focus on pharmacoepidemiology as an area of research where many important questions may be addressed regarding the relative merits of multiple pharmaceuticals for a given condition. There have been an increasing number of observational studies of the association between diabetes treatments and hard outcomes, such as death or CVD events. The increase in such studies likely has been facilitated by the availability of big data in general and specifically large pharmaceutical databases created by national health plans, large health care systems, or mail-order pharmacy providers ( Sobek et al., 2011 ). In addition, the ongoing development of diabetes pharmacotherapies approved based on ability to achieve an improvement in glycemic control but without data on hard outcomes may also provide the impetus to use such large databases for research on comparative safety and efficacy.
Observational Research Study Designs
Cohort and case-control studies.
The two most popular designs for investigating causal hypotheses are the cohort and case-control studies. Features are shown in Table 1 . The major difference between the two is that the cohort study begins with identification of the exposure status, whereas the case-control study begins with the identification of the outcome. A cohort study can be prospective, where exposed and non-exposed subjects are followed for the development of the outcome, or retrospective, where collected data can be used to identify both the exposure status at some past time point and the subsequent development of the outcome. A case-control study, on the other hand, can only look back in time for occurrence of the exposure. There are of course exceptions to these general statements. It is possible in some case-control studies to measure the exposure after the outcome in time if the exposure is invariant and if it is not related to a greater loss to follow-up among persons with the outcome due to mortality or other reasons. Examples of such exposures include genetic markers or an unchanging characteristic of adults such as femur length, eye color, or red blood cell type. Variations in these study designs include the case-cohort and case-only studies, which are described in detail elsewhere, and which a description of which will not be provided here ( DiPietro, 2010 ). Also, the relative merits of these study designs will not be discussed here but are covered in standard epidemiology texts.
Observational Study Designs for Assessment of Causal Relationships

Weaker Observational Research Designs
Other research designs are often used in studies reported in the medical literature. These include cross-sectional, case-series, and case-reports. The cross-sectional study has limited value in assessing a potential causal relationship since it may not be possible to determine whether the potential exposure preceded the outcome, except when the exposure does not vary over one’s life history, such as in the case of a genotype, ABO blood group, or eye color. Case-series and case reports are even more limited since it is not possible to assess if the outcome occurred more frequently among the persons included compared to a control population. Case reports do though have potential value in pharmaceutical safety research by generating potential signals that signify unexpected adverse events. Such monitoring is employed in the Food and Drug Administration’s Adverse Event Reporting System, and has led to changes in product labeling as well as restriction or outright removal of pharmaceuticals from the market due to safety concerns ( Wysowski & Swartz, 2005 ). Over 2 million case reports of adverse reactions were submitted between 1969–2002, resulting in only about 1% of marketed drugs being withdrawn or restricted. Therefore the noise-to-signal ratio for this method of surveillance is exceedingly high and presents an opportunity for other observational methods to better address this issue.
Observation Research for Causal Inference
Causal associations will always involve correlation, but the presence of a correlation does not imply causation. The challenge of observational research is to assess whether a correlation is present and then determine whether it may be due to a causal association. A list of criteria was developed by Dr. Austin Bradford Hill decades ago that is still referred to frequently today ( Hill, 1965 ), although reexamination of these criteria more recently has led to the conclusion that only one of the nine original features is really necessary for a causal relationship in a observational study ( Phillips & Goodman, 2004 ; Rothman & Greenland, 2005 ). The magnitude of the observed association, another Hill criterion, often figures into determinations about the presence of bias, with those of greater magnitude considered less likely to be due to bias and more likely due to a causal process ( Grimes & Schulz, 2002 ).
Examination of the features of an RCT provide some insight into the limitations of observational research in assessing causal associations. The randomization process provides the opportunity for equal distribution of risk factors for the outcome among persons assigned to the treatment and control. Thus any difference in the outcome between these two groups will not likely be due to unequal distribution of risk factors by treatment assignment. The use of randomization provides a way to approach the problem of not having complete knowledge about predictors of all clinically important outcomes. If we did have such knowledge then groups with exactly equal risks of the outcome could be assembled by the investigator. As we do not have such knowledge, the process of randomization utilizes chance to distribute both known and more importantly unknown risk factors for the outcome, and is most likely to achieve this aim with larger sample size ( Efird, 2011 ). Randomization, though, does not guarantee that the treatment and control group will have the same risk of the outcome. Accidents of randomization have occurred for known risk factors for outcomes as in the UGDP, where older subjects with a higher prevalence of cardiovascular disease risk factors were disproportionately assigned to the tolbutamide treatment arm ( Leibel, 1971 ). Such accidents also must occur for the unknown risk factors, although these would not be apparent to the investigator.
Bias in Observational Research
Confounding bias.
Observational research does not have the benefit of randomization to allocate by chance risk factors for an outcome of interest. Exposures to risk factors occur due to self-selection, medical provider prescription, in association with occupation, and for other reasons. When an exposure of interest is strongly associated with another exposure that is also related to the outcome, confounding bias is present, but methods exist to obtain an unbiased estimated of the exposure-disease association as long as the confounding factor is identified and measured accurately.
A cross-sectional study of a genetic marker (Gm haplotype Gm 3;5,13,14 ) and diabetes prevalence provides an example of confounding bias. Subjects included members of the Pima and Papago tribes of the Gila River Indian Community in Southern Arizona who underwent a medical history and examination every two years including assessment of diabetes status through oral glucose tolerance testing ( Knowler, Williams, Pettitt & Steinberg, 1988 ). Subjects were further characterized by degree of Indian heritage measured in eighths and referred to as “quantum.” A total of 4,640 subjects of either 0/8, 4/8, and 8/8 quantum were included in this analysis. There were 1,336 persons with and 3,304 persons without diabetes available for analysis, yielding a crude (unadjusted) overall odds ratio of 0.24 for the association between haplotype Gm 3;5,13,14 and diabetes prevalence ( Figure 1 , Panel A). This result supports a lower prevalence of diabetes in association with haplotype Gm 3;5,13,14 , but the unadjusted result represents a substantial overestimate due to confounding by Quantum. In Figure 1 panel B, subjects were divided by the three Quantum categories found in the sample, and within each of these the odds ratio is closer to 1.0 and therefore of smaller magnitude than the crude result. Note that collapsing the three tables in Panel B by summing the cells yields the single overall table shown in Panel A. Adjustment for these Quantum categories yields an odds ratio of 0.59, which is of smaller magnitude than the result seen in the unadjusted analysis ( Figure 1 , Panel B). Although the odds ratios vary across Quantum categories, a test for heterogeneity across these strata was non-significant (p=0.295). Therefore the null hypothesis that the odds ratios differed across Quantum strata could not be rejected.

Cross-sectional study of Native Americans of the Pima and Papago Indian tribes in Southern Arizona on the associations between the GM haplotype Gm 3;5,13,14 , native quantum, and diabetes mellitus prevalence. Panel A displays all participants combined with Native quantum of either 0/8, 4/8 or 8/8 by presence of diabetes mellitus in relation to Gm 3;5,13,14 presence or absence. The overall (crude) odds ratio for the association is shown. Panel B displays all participants from Panel A stratified by Native quantum, demonstrating confounding by Native quantum as judged by the discordance between the crude and stratified or Quantum-adjusted results. Panel C demonstrates that Quantum meets the criterion as a confounding variable due to its negative association with Gm 3;5,13,14 and positive association with diabetes prevalence.
Examination of the frequency of haplotype Gm 3;5,13,14 and diabetes prevalence across Indian heritage Quantum reveals the reason for the overestimation of the association in the unadjusted analysis. Diabetes occurred more frequently while the haplotype Gm 3;5,13,14 occurred less frequently among subjects with greater Indian heritage ( Figure 1 , Panel C). Adjustment for the imbalance in Quantum by haplotype Gm 3;5,13,14 in this specific example and in general any accurately measured confounding factor yields a less biased odds ratio that is closer to the true magnitude of the association between this haplotype and diabetes prevalence.
Another more recent example of confounding can be seen in a case-cohort European study of the association between artificially sweetened soft drinks and the risk of developing type 2 diabetes ( 2013 ). The unadjusted hazard ratio for the daily consumption of ≥ 250 g of this beverage type was 1.84 (95% CI 1.52 to 2.23) representing a statistically significant elevation in risk. After adjustment for daily energy intake and BMI, the hazard ratio diminished to 1.13 (95% CI 0.85 to 1.52) and was no longer statistically significant (p=0.24). The investigators concluded that consumption of artificially sweetened soft drinks was not associated with type 2 diabetes risk in their population.
Multiple methods exist to remove the bias from recognized, accurately measured confounding factors, but unfortunately there is no widely accepted option for handling unmeasured confounding factors and adjusting for this bias. In this regard observational research is unable to match the ability of a RCT to account for this potential bias. Methods have been developed to better assess whether associations represent causal pathways that will be described later in this paper.
Information Bias
Observational research can be susceptible to other types of bias. Information bias refers to inaccurate assessment of the outcome, the exposure, or potential confounding variables. An example includes measurement of nutritional intake, which is often assessed by research subjects completing a food frequency survey or 24-hour dietary recall. Even if subjects report these intakes correctly, the likelihood is low that this will reflect long-term dietary intake exactly. Attempts have been made to reduce the error of these measurements through biomarker calibration that in one study was based on a urinary nitrogen protocol to estimate daily protein consumption over a 24-hour period ( Tinker et al., 2011 ). This analysis revealed a slight increase in risk of incident diabetes in association with a 20% higher protein intake in grams (Hazard Ratio 1.05, 95% CI 1.03–1.07). Recalibrated results based on the results of the urinary nitrogen protocol yielded a substantially higher diabetes hazard ratio of 1.82 (95% CI 1.56–2.12) that after adjustment for BMI was reduced to 1.16 (95% CI 1.05–2.28). In this example, reduction of measurement error yielded a difference of greater magnitude than see in the analysis based on dietary self-reports only without objective validation, although theoretically more accurate measurements may yield smaller differences, depending on the type and magnitude of measurement error.
Selection Bias
Selection bias may produce factitious exposure-disease associations if the study population fails to mirror the target population of interest. For example, selection of control subjects from among hospitalized patients as might be the case in a study based on administrative data may not accurately depict smoking prevalence among controls, given that smoking is related to multiple diseases that would increase the risk for hospitalization. Effective observational research must recognize the potential for bias and attempt to minimize it both in the design and analysis, as well as accurately describing limitations of these data and the implications for study validity in reports of results.
Agreement and Discrepancies between Observational and Clinical Trial Research
One way to assess whether the potential biases of observational studies result in failure to detect true associations is by comparison of observational versus RCT results on the same questions. Since observational studies of treatments often precede definitive clinical trials, several authors have assessed agreement between similar hypotheses tested using the gold standard compared to observational designs, concluding that agreement between the two is high. A comparison of 136 reports published between 1985 to 1998 on 19 different treatments found excellent agreement, with the combined magnitude of the effect in observational studies lying within the 95% confidence intervals of the combined magnitude of the effect in RCTs for 17 of the 19 hypotheses tested ( Benson & Hartz, 2000 ). Another comparison focused on comparing the results of meta-analyses of observation and clinical trial research on five clinical questions that were identified through a search of five major medical journals from 1991 to 1995 ( Concato, Shah & Horwitz, 2000 ). These investigators concluded that average results of these studies were “remarkably similar.”
In contrast, other research has demonstrated discrepancies between RCT and observational designs. The Women’s Health Initiative (WHI) was a RCT of dietary and menopausal hormone interventions to assess these effects on mortality, cardiovascular disease, and cancer risk ( Prentice et al., 2005 ). Perhaps unique to this study was the establishment of a concurrent observational study accompanying the randomized clinical trial, thereby permitting direct comparison of reported associations by type of research design within the same study framework. In the trial/observational study of estrogen plus progestin for menopausal hormone replacement, marked differences were seen between the treatment and control groups by participation in the RCT or observational study ( Table 2 ). In the RCT, no important differences were seen by treatment assignment for race, educational level, BMI, or current smoking status. This was not true by estrogen-progestin exposure in the observational study, where exposed women were more likely to be White, having completed a college degree or higher, and less likely to be current smokers or obese. Outcomes occurred more frequently in the estrogen-progestin arm of the RCT, but less frequently in the corresponding arm of the observational study, except for venous thromboembolism ( Table 2 ). Hazard ratios for these comparisons adjusted for imbalances in baseline potential confounding factors show a harmful effect of estrogen-progestin use that is statistically significantly elevated in 2 of 3 outcomes and a discordance with the observational results due to null, somewhat protective hazard ratios or in the case of venous thromboembolism, an elevated hazard ratio of considerably smaller magnitude than in the clinical trial. Although good agreement between clinical trials and observational research occurs often, the example of the WHI prevents having complete confidence in the results of observational studies.
Comparison of baseline characteristics and outcomes in the randomized controlled trial and observational study of estrogen-progestin treatment in the Women’s Health Initiative (1994–2002).
Achievements of Observational Research
Despite the limitations of observational research design, many well-accepted causal associations in medicine are supported entirely or in part due to this type of investigation. Several examples include the association between hyperglycemia and diabetes complications including retinopathy, nephropathy, peripheral neuropathy, and ischemic heart disease ( 2013 ). Other well known examples include hypertension and stroke, smoking and lung cancer, asbestosis and mesothelioma, and LDL and HDL cholesterol concentrations and risk of ischemic heart disease ( Churg, 1988 ; Gordon, Kannel, Castelli & Dawber, 1981 ; Kannel, Wolf, Verter & McNamara, 1970 ; Pirie, Peto, Reeves, Green & Beral, 2013 ). In the case of complications due to hyperglycemia, high LDL-cholesterol concentration, and hypertension, clinical trials to reduce these levels have resulted in reductions in the rate of these outcomes, further supporting a causal association ( 1991 ; 1994 ; 1998 ; 1998 ). For many associations that involve an exposure that cannot be controlled by the investigator or should not be modified for ethical reasons, observational research may be the only avenue for direct testing of these associations in humans.
Causal Inference from Observational Research
The results of an observational research study are never interpreted in an information vacuum. Given the potential for bias with this study design, a number of other factors should be considered when weighing the strength of this evidence. First and foremost would be the replication of the finding in other observational research studies. Additional evidence to bolster the potential causal association would be support from the biological understanding of underlying mechanisms, animal experiments confirming that the exposure results in a similar outcome, and trend data in disease incidence following changes in exposure prevalence. For example, in the UK Million Women Study where median age was reported at 55 years, women who quit smoking completely at ages 25–34 or 35–44 years had only 3% and 10% of the excess mortality, respectively, seen among women who were continuing smokers ( Pirie, Peto, Reeves, Green & Beral, 2013 ). Coronary heart disease deaths in the U.S. declined by approximately 50% between 1980 to 2000. One analysis that addressed the reasons for this decline concluded that change in risk factors (reductions in total cholesterol concentration, systolic blood pressure, smoking, and physical inactivity) accounted for approximately 47% of this decrease ( Ford et al., 2007 ). These trends provide support for a causal association between smoking and lung cancer, and multiple cardiovascular disease risk factors and coronary death risk.
Pharmacoepidemiology
Many questions regarding the use of pharmaceuticals may never be answered through use of RCTs, thereby creating a need to address knowledge gaps using observational research. The specialized field of pharmacoepidemiology directly addresses these needs. The earliest appearance of the term “pharmacoepidemiology” on PubMed.com is in an article written in 1984 ( Lawson, 1984 ). The field of pharmacoepidemiology encompasses the use of observational research to assess pharmaceutical safety and effectiveness. For example, diabetes pharmaceuticals have received FDA approval based on efficacy at lowering glucose and safety, without the need to prove efficacy at preventing long-term complications. The sulfonylurea hypoglycemic agents glyburide and glipizide are in widespread use to manage the hyperglycemia of diabetes, but it is not clear whether one is associated with a greater reduction in hard outcomes such as mortality or diabetes complications, as this has not been tested in a clinical trial. Use of such surrogate endpoints as opposed to the hard outcomes one wishes to prevent has been criticized as an ineffective and potentially harmful approach to medication approval ( Fleming & DeMets, 1996 ; Psaty et al., 1999 ). Design of clinical trials to address hard as opposed to surrogate endpoints typically requires larger sample size, longer follow-up, and greater costs.
Observational research may also identify adverse effects associated with the use of pharmaceuticals that were not anticipated based on research conducted in support of the drug approval process. The withdrawal of the thiazolidinedione agent troglitazone from the U.S. market in 2000 followed reports on cases of severe liver toxicity during post-marketing surveillance. Similar data on a high number of reported cases of severe myopathies in cerivastatin users led to its withdrawal from the worldwide market in 2001 ( Furberg & Pitt, 2001 ). An observational study using administrative claims databases to assess the relative safety of lipid lowering medications in the U.S. between 2000–2004 reported a much higher risk for hospitalization for treatment of myopathy among cerivastatin users compared to users of other statin and non-stain lipid lowering agents ( Cziraky et al., 2006 ).
Confounding by Indication
As with other observational research designs, there are limitations to pharmacoepidemiology due to biases previous described, but in addition to these is the vexing phenomenon of confounding by indication, also referred to as channeling bias ( McMahon & MacDonald, 2000 ; Petri & Urquhart, 1991 ). This refers to an observed benefit (or harm) associated with a pharmaceutical due to the indications for treatment with it and not a medication effect. A hypothetical example of how confounding by indication results in outcome differences not due to medication effect is shown in Figure 2 , which provides an example of how the choice of a diabetes pharmaceutical may depend on the existence of a condition (higher serum creatinine reflecting lower GFR) associated with higher mortality risk ( Fox et al., 2012 ).

A hypothetical population of 2000 identical persons with type 2 diabetes differing only by renal function as measured by serum creatinine and assigned to either metformin or glipizide based on the serum creatinine level. The active treatment, though, is never dispensed, and instead substituted with a identical placebo. An expected difference in mortality is seen between the two groups given the association between poorer renal function and mortality in the glipizide group. This difference cannot be explained by the effect of the active pharmaceutical (since there was none) and therefore represents an example of confounding by indication.
Several approaches exist to the problem of confounding by indication. If there is no association between the indication for the pharmaceutical and the outcome of interest, then no bias will occur, since an association must also be present between both the indication and the outcome to yield a biased result. This same principle applies to all confounding factors ( van Stralen, Dekker, Zoccali & Jager, 2010 ). If the conditions for confounding are fulfilled, then statistical adjustment techniques are available to produce unbiased estimates of effect. Commonly used methods in biomedical research include linear regression analysis for continuous outcomes, logistic regression for categorical outcomes, and the Cox proportional hazards model for time-to-event outcomes. In addition, propensity scores have risen in popularity over the past decade. An “all fields” search of Pubmed conducted January 15, 2012 using the search term “propensity score” yielded 2,895 hits for the immediate past 5 years, and only 715 hits for the previous 5 years. The propensity score method models the probability of exposure in relation to predictor variables, and therefore estimates the likelihood, in the case of a pharmacoepidemiology study, of a subject receiving a particular pharmaceutical based on his or her characteristics ( Rubin, 2010 ). An additional step is required which uses standard previously mentioned adjustment methods to remove the bias associated with varying likelihood of receiving the pharmaceutical. Despite the rising popularity of this method, it has been demonstrated to be merely equivalent and sometimes inferior to standard multivariate adjustment methods ( Shah, Laupacis, Hux & Austin, 2005 ; Sturmer et al., 2006 ). Furthermore, propensity scores cannot address the issue of unmeasured confounding ( Cummings, 2008 ). So if the indications for the pharmaceutical cannot be determined from the other measured factors, neither multivariate adjustment or propensity scores will allow for adjustment and removal of bias.
Several design features of observational studies may increase the likelihood of confounding by indication but if recognized may be amenable to correction in the design or analysis phases of a study. Assessing outcomes for pharmaceuticals prescribed for different indications or by a comparison of populations who differ with regard to the presence of medication contraindications may introduce bias into comparisons. An assessment of the mortality risk associated with beta-blocker use compared to other antihypertensive medications should exclude participants in whom beta blockers but not other antihypertensive medications are prescribed for other indications, such as migraine headache or stage fright prophylaxis, as these conditions may be associated with better outcomes and lead to over-optimistic survival benefit. Also, failure to consider medication contraindications may lead to risk of the outcome differing by medication used, as seen in the example in Figure 2 which would lead to a higher frequency of subjects with renal insufficiency in the glipizide treatment group for hyperglycemia. To account for this potential bias, subjects with contraindications for use of any of the pharmaceuticals of interest in the comparison should be eliminated from the study. For example, recent studies of mortality and cardiovascular events among users of sulfonylurea or metformin monotherapy for treatment of diabetes in the Veterans Health Administration system excluded patients with serious medical conditions at baseline that might influence the prescription of diabetes medication ( Roumie et al., 2012 ; Wheeler et al., 2013 ). For example, some items on the list of exclusions were congestive heart failure, serum creatinine concentration of 1.5 mg/dl or greater, HIV, and other conditions described in this publication. Despite these design features and adjustment methods to correct for factors associated with a particular prescription that may also be associated with a different outcome risk, there will always be some uncertainty about the presence of bias due to residual confounding by indication.
Methods to Improve Causal Inference from Observational Research
Instrumental variables analysis has been promoted as a method to overcome the inability to exclude undetected confounding in observational research. This method involves identification of a factor that strongly predicts treatment (or exposure in an epidemiologic study not involving a pharmaceutical). This factor is referred to as an “instrument,” and it is used in a manner analogous to the intention to treat analysis employed in RCTs ( Thomas & Conti, 2004 ). A Mendelian Randomization study is a type of instrumental variable analysis that uses a genetic marker as the instrument ( Thomas & Conti, 2004 ). Although intriguing in concept, the difficulty is in the application, as this relies on finding an “instrument” that is (1) causally related to treatment but not unobserved risk factors for the outcome, and (2) influences the outcome only through its effect on treatment ( Hernan & Robins, 2006 ). This method is being explored in pharmacoepidemiologic investigations, with one example being use of physician prescribing preference for types of NSAIDS in the evaluation of the gastrointestinal toxicity of COX-2 inhibitors versus non-COX-2 inhibitor NSAIDS ( Brookhart, Wang, Solomon & Schneeweiss, 2006 ). This analysis reported a protective association with COX-2 inhibitors only in the instrumental variable analysis, leading the authors to conclude that this analysis resulted in a reduction in unmeasured confounding. Examples can also be found in the diabetes epidemiology literature, such as the lack of association between serum uric acid level and type 2 diabetes risk ( Pfister et al., 2011 ), and higher risk associated with lower sex hormone-binding globulin concentration ( Ding et al., 2009 ).
Conclusions
As it will not be possible to assess efficacy of all possible treatment comparisons in all possible groups of interest, or identify adverse (or unexpected beneficial) outcomes requiring longer follow-up or greater sample size using RCTs, observational research stands prepared to step forward to address these knowledge gaps. Much medical knowledge and practice currently rests on a foundation of observational research. Perhaps this is not noticed due to the gloss and novelty of recently completed RCTs. Little research has been conducted comparing results from observational and clinical trial designs, but that which has been completed finds generally good agreement in these findings. With any observational research finding, though, comes less certainly due to the inability to completely exclude the possibility of residual confounding, or in the case of a pharmaceutical, confounding by indication. However, the expectation of absolute certainty is unrealistic and inconsistent with the current practice of medicine, where decisions are made probabilistically, with the threshold for actions such as further testing or treatment varying widely depending on the comparative costs and benefits of true and false positive and negative decisions ( Boland & Lehmann, 2010 ; Pauker & Kassirer, 1980 ; Plasencia, Alderman, Baron, Rolfs & Boyko, 1992 ). Observational research definitely has had and will continue to have an important role in providing the information needed to improve medical decision-making. There is always room for improvement and the hope that the future will bring better methods to further reduce the uncertainty surrounding the validity of its results.
Acknowledgments
Grant Support: VA Epidemiologic Research and Information Center; the Diabetes Research Center at the University of Washington (DK-017047)
Thanks for James S. Floyd MD for his careful review of this manuscript. The work was supported by the VA Epidemiologic Research and Information Center; the Diabetes Research Center at the University of Washington (DK-017047); and VA Puget Sound Health Care System.
Publisher's Disclaimer: This is a PDF file of an unedited manuscript that has been accepted for publication. As a service to our customers we are providing this early version of the manuscript. The manuscript will undergo copyediting, typesetting, and review of the resulting proof before it is published in its final citable form. Please note that during the production process errors may be discovered which could affect the content, and all legal disclaimers that apply to the journal pertain.
- Prevention of stroke by antihypertensive drug treatment in older persons with isolated systolic hypertension. Final results of the Systolic Hypertension in the Elderly Program (SHEP). SHEP Cooperative Research Group. JAMA. 1991; 265 :3255–3264. [ PubMed ] [ Google Scholar ]
- 1994 Randomised trial of cholesterol lowering in 4444 patients with coronary heart disease: the Scandinavian Simvastatin Survival Study (4S) Lancet. 344 :1383–1389. [ PubMed ] [ Google Scholar ]
- Effect of intensive blood-glucose control with metformin on complications in overweight patients with type 2 diabetes (UKPDS 34). UK Prospective Diabetes Study (UKPDS) Group. Lancet. 1998; 352 :854–865. [ PubMed ] [ Google Scholar ]
- Intensive blood-glucose control with sulphonylureas or insulin compared with conventional treatment and risk of complications in patients with type 2 diabetes (UKPDS 33). UK Prospective Diabetes Study (UKPDS) Group. Lancet. 1998; 352 :837–853. [ PubMed ] [ Google Scholar ]
- Consumption of sweet beverages and type 2 diabetes incidence in European adults: results from EPIC-InterAct. Diabetologia. 2013; 56 :1520–1530. [ PubMed ] [ Google Scholar ]
- Diagnosis and classification of diabetes mellitus. Diabetes Care. 2013; 36 (Suppl 1):S67–74. [ PMC free article ] [ PubMed ] [ Google Scholar ]
- Benson K, Hartz AJ. A comparison of observational studies and randomized, controlled trials. N Engl J Med. 2000; 342 :1878–1886. [ PubMed ] [ Google Scholar ]
- Boland MV, Lehmann HP. A new method for determining physician decision thresholds using empiric, uncertain recommendations. BMC Med Inform Decis Mak. 2010; 10 :20. [ PMC free article ] [ PubMed ] [ Google Scholar ]
- Brookhart MA, Wang PS, Solomon DH, Schneeweiss S. Evaluating short-term drug effects using a physician-specific prescribing preference as an instrumental variable. Epidemiology. 2006; 17 :268–275. [ PMC free article ] [ PubMed ] [ Google Scholar ]
- Churg A. Chrysotile, tremolite, and malignant mesothelioma in man. Chest. 1988; 93 :621–628. [ PubMed ] [ Google Scholar ]
- Concato J, Shah N, Horwitz RI. Randomized, controlled trials, observational studies, and the hierarchy of research designs. N Engl J Med. 2000; 342 :1887–1892. [ PMC free article ] [ PubMed ] [ Google Scholar ]
- Cummings P. Propensity scores. Arch Pediatr Adolesc Med. 2008; 162 :734–737. [ PubMed ] [ Google Scholar ]
- Cziraky MJ, Willey VJ, McKenney JM, Kamat SA, Fisher MD, Guyton JR, et al. Statin safety: an assessment using an administrative claims database. Am J Cardiol. 2006; 97 :61C–68C. [ PubMed ] [ Google Scholar ]
- Ding EL, Song Y, Manson JE, Hunter DJ, Lee CC, Rifai N, et al. Sex hormone-binding globulin and risk of type 2 diabetes in women and men. N Engl J Med. 2009; 361 :1152–1163. [ PMC free article ] [ PubMed ] [ Google Scholar ]
- DiPietro NA. Methods in epidemiology: observational study designs. Pharmacotherapy. 2010; 30 :973–984. [ PubMed ] [ Google Scholar ]
- Efird J. Blocked randomization with randomly selected block sizes. Int J Environ Res Public Health. 2011; 8 :15–20. [ PMC free article ] [ PubMed ] [ Google Scholar ]
- Fleming TR, DeMets DL. Surrogate end points in clinical trials: are we being misled? Ann Intern Med. 1996; 125 :605–613. [ PubMed ] [ Google Scholar ]
- Ford ES, Ajani UA, Croft JB, Critchley JA, Labarthe DR, Kottke TE, et al. Explaining the decrease in U.S. deaths from coronary disease, 1980–2000. N Engl J Med. 2007; 356 :2388–2398. [ PubMed ] [ Google Scholar ]
- Fox CS, Matsushita K, Woodward M, Bilo HJ, Chalmers J, Heerspink HJ, et al. Associations of kidney disease measures with mortality and end-stage renal disease in individuals with and without diabetes: a meta-analysis. Lancet. 2012; 380 :1662–1673. [ PMC free article ] [ PubMed ] [ Google Scholar ]
- Furberg CD, Pitt B. Withdrawal of cerivastatin from the world market. Curr Control Trials Cardiovasc Med. 2001; 2 :205–207. [ PMC free article ] [ PubMed ] [ Google Scholar ]
- Gordon T, Kannel WB, Castelli WP, Dawber TR. Lipoproteins, cardiovascular disease, and death. The Framingham study. Arch Intern Med. 1981; 141 :1128–1131. [ PubMed ] [ Google Scholar ]
- Grimes DA, Schulz KF. Bias and causal associations in observational research. Lancet. 2002; 359 :248–252. [ PubMed ] [ Google Scholar ]
- Hernan MA, Robins JM. Instruments for causal inference: an epidemiologist’s dream? Epidemiology. 2006; 17 :360–372. [ PubMed ] [ Google Scholar ]
- Hill AB. The Environment and Disease: Association or Causation? Proceedings of the Royal Society of Medicine. 1965; 58 :295–300. [ PMC free article ] [ PubMed ] [ Google Scholar ]
- Kannel WB, Wolf PA, Verter J, McNamara PM. Epidemiologic assessment of the role of blood pressure in stroke. The Framingham study. JAMA. 1970; 214 :301–310. [ PubMed ] [ Google Scholar ]
- Knowler WC, Williams RC, Pettitt DJ, Steinberg AG. Gm3;5,13,14 and type 2 diabetes mellitus: an association in American Indians with genetic admixture. American journal of human genetics. 1988; 43 :520–526. [ PMC free article ] [ PubMed ] [ Google Scholar ]
- Lawson DH. Pharmacoepidemiology: a new discipline. Br Med J (Clin Res Ed) 1984; 289 :940–941. [ PMC free article ] [ PubMed ] [ Google Scholar ]
- Leibel B. An analysis of the University Group Diabetes Study Program: data results and conslusions. Can Med Assoc J. 1971; 105 :292–294. [ PMC free article ] [ PubMed ] [ Google Scholar ]
- McMahon AD, MacDonald TM. Design issues for drug epidemiology. Br J Clin Pharmacol. 2000; 50 :419–425. [ PMC free article ] [ PubMed ] [ Google Scholar ]
- Meldrum ML. A brief history of the randomized controlled trial. From oranges and lemons to the gold standard. Hematol Oncol Clin North Am. 2000; 14 :745–760. vii. [ PubMed ] [ Google Scholar ]
- Pauker SG, Kassirer JP. The threshold approach to clinical decision making. N Engl J Med. 1980; 302 :1109–1117. [ PubMed ] [ Google Scholar ]
- Petri H, Urquhart J. Channeling bias in the interpretation of drug effects. Stat Med. 1991; 10 :577–581. [ PubMed ] [ Google Scholar ]
- Pfister R, Barnes D, Luben R, Forouhi NG, Bochud M, Khaw KT, et al. No evidence for a causal link between uric acid and type 2 diabetes: a Mendelian randomisation approach. Diabetologia. 2011; 54 :2561–2569. [ PubMed ] [ Google Scholar ]
- Phillips CV, Goodman KJ. The missed lessons of Sir Austin Bradford Hill. Epidemiol Perspect Innov. 2004; 1 :3. [ PMC free article ] [ PubMed ] [ Google Scholar ]
- Pirie K, Peto R, Reeves GK, Green J, Beral V. The 21st century hazards of smoking and benefits of stopping: a prospective study of one million women in the UK. Lancet. 2013; 381 :133–141. [ PMC free article ] [ PubMed ] [ Google Scholar ]
- Plasencia CM, Alderman BW, Baron AE, Rolfs RT, Boyko EJ. A method to describe physician decision thresholds and its application in examining the diagnosis of coronary artery disease based on exercise treadmill testing. Med Decis Making. 1992; 12 :204–212. [ PubMed ] [ Google Scholar ]
- Prentice RL, Langer R, Stefanick ML, Howard BV, Pettinger M, Anderson G, et al. Combined postmenopausal hormone therapy and cardiovascular disease: toward resolving the discrepancy between observational studies and the Women’s Health Initiative clinical trial. Am J Epidemiol. 2005; 162 :404–414. [ PubMed ] [ Google Scholar ]
- Psaty BM, Weiss NS, Furberg CD, Koepsell TD, Siscovick DS, Rosendaal FR, et al. Surrogate end points, health outcomes, and the drug-approval process for the treatment of risk factors for cardiovascular disease. JAMA. 1999; 282 :786–790. [ PubMed ] [ Google Scholar ]
- Rothman KJ, Greenland S. Causation and causal inference in epidemiology. Am J Public Health. 2005; 95 (Suppl 1):S144–150. [ PubMed ] [ Google Scholar ]
- Roumie CL, Hung AM, Greevy RA, Grijalva CG, Liu X, Murff HJ, et al. Comparative effectiveness of sulfonylurea and metformin monotherapy on cardiovascular events in type 2 diabetes mellitus: a cohort study. Ann Intern Med. 2012; 157 :601–610. [ PMC free article ] [ PubMed ] [ Google Scholar ]
- Rubin DB. Propensity score methods. Am J Ophthalmol. 2010; 149 :7–9. [ PubMed ] [ Google Scholar ]
- Rubin RR, Fujimoto WY, Marrero DG, Brenneman T, Charleston JB, Edelstein SL, et al. The Diabetes Prevention Program: recruitment methods and results. Control Clin Trials. 2002; 23 :157–171. [ PubMed ] [ Google Scholar ]
- Shah BR, Laupacis A, Hux JE, Austin PC. Propensity score methods gave similar results to traditional regression modeling in observational studies: a systematic review. J Clin Epidemiol. 2005; 58 :550–559. [ PubMed ] [ Google Scholar ]
- Sobek M, Cleveland L, Flood S, Hall PK, King ML, Ruggles S, et al. Big Data: Large-Scale Historical Infrastructure from the Minnesota Population Center. Hist Methods. 2011; 44 :61–68. [ PMC free article ] [ PubMed ] [ Google Scholar ]
- Sturmer T, Joshi M, Glynn RJ, Avorn J, Rothman KJ, Schneeweiss S. A review of the application of propensity score methods yielded increasing use, advantages in specific settings, but not substantially different estimates compared with conventional multivariable methods. J Clin Epidemiol. 2006; 59 :437–447. [ PMC free article ] [ PubMed ] [ Google Scholar ]
- Thomas DC, Conti DV. Commentary: the concept of ‘Mendelian Randomization’ Int J Epidemiol. 2004; 33 :21–25. [ PubMed ] [ Google Scholar ]
- Tinker LF, Sarto GE, Howard BV, Huang Y, Neuhouser ML, Mossavar-Rahmani Y, et al. Biomarker-calibrated dietary energy and protein intake associations with diabetes risk among postmenopausal women from the Women’s Health Initiative. Am J Clin Nutr. 2011; 94 :1600–1606. [ PMC free article ] [ PubMed ] [ Google Scholar ]
- van Stralen KJ, Dekker FW, Zoccali C, Jager KJ. Confounding. Nephron Clin Pract. 2010; 116 :c143–147. [ PubMed ] [ Google Scholar ]
- Wheeler S, Moore K, Forsberg CW, Riley K, Floyd JS, Smith NL, et al. Mortality among veterans with type 2 diabetes initiating metformin, sulfonylurea or rosiglitazone monotherapy. Diabetologia 2013 [ PubMed ] [ Google Scholar ]
- Wysowski DK, Swartz L. Adverse drug event surveillance and drug withdrawals in the United States, 1969–2002: the importance of reporting suspected reactions. Arch Intern Med. 2005; 165 :1363–1369. [ PubMed ] [ Google Scholar ]
- - Google Chrome
Intended for healthcare professionals
- Access provided by Google Indexer
- My email alerts
- BMA member login
- Username * Password * Forgot your log in details? Need to activate BMA Member Log In Log in via OpenAthens Log in via your institution

Search form
- Advanced search
- Search responses
- Search blogs
- Nested case-control...
Nested case-control studies: advantages and disadvantages
- Related content
- Peer review
- Philip Sedgwick , reader in medical statistics and medical education 1
- 1 Centre for Medical and Healthcare Education, St George’s, University of London, London, UK
- p.sedgwick{at}sgul.ac.uk
Researchers investigated whether antipsychotic drugs were associated with venous thromboembolism. A population based nested case-control study design was used. Data were taken from the UK QResearch primary care database consisting of 7 267 673 patients. Cases were adult patients with a first ever record of venous thromboembolism between 1 January 1996 and 1 July 2007. For each case, up to four controls were identified, matched by age, calendar time, sex, and practice. Exposure to antipsychotic drugs was assessed on the basis of prescriptions on, or during the 24 months before, the index date. 1
There were 25 532 eligible cases (15 975 with deep vein thrombosis and 9557 with pulmonary embolism) and 89 491 matched controls. The primary outcome was the odds ratios for venous thromboembolism associated with antipsychotic drugs adjusted for comorbidity and concomitant drug exposure. When adjusted using logistic regression to control for potential confounding, prescription of antipsychotic drugs in the previous 24 months was significantly associated with an increased occurrence of venous thromboembolism compared with non-use (odds ratio 1.32, 95% confidence interval 1.23 to 1.42). The researchers concluded that prescription of antipsychotic drugs was associated with venous thromboembolism in a large primary care population.
Which of the following statements, if any, are true?
a) The nested case-control study is a retrospective design
b) The study design minimised selection bias compared with a case-control study
c) Recall bias was minimised compared with a case-control study
d) Causality could be inferred from the association between prescription of antipsychotic drugs and venous thromboembolism
Statements a , b , and c are true, whereas d is false.
The aim of the study was to investigate whether prescription of antipsychotic drugs was associated with venous thromboembolism. A nested case-control study design was used. The study design was an observational one that incorporated the concept of the traditional case-control study within an established cohort. This design overcomes some of the disadvantages associated with case-control studies, 2 while incorporating some of the advantages of cohort studies. 3 4
Data for the study above were extracted from the UK QResearch primary care database, a computerised register of anonymised longitudinal medical records for patients registered at more than 500 UK general practices. Patient data were recorded prospectively, the database having been updated regularly as patients visited their GP. Cases were all adult patients in the register with a first ever record of venous thromboembolism between 1 January 1996 and 1 July 2007. There were 25 532 cases in total. For each case, up to four controls were identified from the register, matched by age, calendar time, sex, and practice. In total, 89 491 matched controls were obtained. Data relating to prescriptions for antipsychotic drugs on, or during the 24 months before, the index date were extracted for the cases and controls. The index date was the date in the register when venous thromboembolism was recorded for the case. The cases and controls were compared to ascertain whether exposure to prescription of antipsychotic drugs was more common in one group than in the other. Despite the data for the cases and controls being collected prospectively, the nested case-control study is described as retrospective ( a is true) because it involved looking back at events that had already taken place and been recorded in the register.
Selection bias is of particular concern in the traditional case-control study. Described in a previous question, 5 selection bias is the systematic difference between the study participants and the population they are meant to represent with respect to their characteristics, including demographics and morbidity. Cases and controls are often selected through convenience sampling. Cases are typically recruited from hospitals or general practices because they are convenient and easily accessible to researchers. Controls are often recruited from the same hospital clinics or general practices as the cases. Therefore, the selected cases may not be representative of the population of all cases. Equally, the controls might not be representative of otherwise healthy members of the population. The above nested case-control study was population based, with the QResearch primary care database incorporating a large proportion of the UK population. The cases and controls were selected from the database and therefore should be more representative of the population than those in a traditional case-control study. Hence, selection bias was minimised by using the nested case-control study design ( b is true).
The traditional case-control study involves participants recalling information about past exposure to risk factors after identification as a case or control. The study design is prone to recall bias, as described in a previous question. 6 Recall bias is the systematic difference between cases and controls in the accuracy of information recalled. Recall bias will exist if participants have selective preconceptions about the association between the disease and past exposure to the risk factor(s). Cases may, for example, recall information more accurately than controls, possibly because of an association with the disease or outcome. Although in the study above the cases and controls were identified retrospectively, the data for the QResearch primary care database were collected prospectively. Therefore, there was no reason for any systematic differences between groups of study participants in the accuracy of the information collected. Therefore, recall bias was minimised compared with a traditional case-control study ( c is true).
Not all of the patient records in the UK QResearch primary care database were used to explore the association between prescription of antipsychotic drugs and development of venous thromboembolism. A nested case-control study was used instead, with cases and controls matched on age, calendar time, sex, and practice. This was because it was statistically more efficient to control for the effects of age, calendar time, sex, and practice by matching cases and controls on these variables at the design stage, rather than controlling for their potential confounding effects when the data were analysed. The matching variables were considered to be important factors that could potentially confound the association between prescription of antipsychotic drugs and venous thromboembolism, but they were not of interest as potential risk factors in themselves. Matching in case-control studies has been described in a previous question. 7
Unlike a traditional case-control study, the data in the example above were recorded prospectively. Therefore, it was possible to determine whether prescription of antipsychotic drugs preceded the occurrence of venous thromboembolism. Nonetheless, only association, and not causation, can be inferred from the results of the above nested case-control study ( d is false)—that is, those people who were exposed to prescribed antipsychotic drugs were more likely to have developed venous thromboembolism. This is because the observed association between prescribed antipsychotic drugs and occurrence of venous thromboembolism may have been due to confounding. In particular, it was not possible to measure and then control for, through statistical analysis, all factors that may have affected the occurrence of venous thromboembolism.
The example above is typical of a nested case-control study; the health records for a group of patients that have already been collected and stored in an electronic database are used to explore the association between one or more risk factors and a disease or condition. The management of such databases means it is possible for a variety of studies to be undertaken, each investigating the risk factors associated with different diseases or outcomes. Nested case-control studies are therefore relatively inexpensive to perform. However, the major disadvantage of nested case-control studies is that not all pertinent risk factors are likely to have been recorded. Furthermore, because many different healthcare professionals will be involved in patient care, risk factors and outcome(s) will probably not have been measured with the same accuracy and consistency throughout. It may also be problematic if the diagnosis of the disease or outcome changes with time.
Cite this as: BMJ 2014;348:g1532
Competing interests: None declared.
- ↵ Parker C, Coupland C, Hippisley-Cox J. Antipsychotic drugs and risk of venous thromboembolism: nested case-control study. BMJ 2010 ; 341 : c4245 . OpenUrl Abstract / FREE Full Text
- ↵ Sedgwick P. Case-control studies: advantages and disadvantages. BMJ 2014 ; 348 : f7707 . OpenUrl CrossRef
- ↵ Sedgwick P. Prospective cohort studies: advantages and disadvantages. BMJ 2013 ; 347 : f6726 . OpenUrl FREE Full Text
- ↵ Sedgwick P. Retrospective cohort studies: advantages and disadvantages. BMJ 2014 ; 348 : g1072 . OpenUrl FREE Full Text
- ↵ Sedgwick P. Selection bias versus allocation bias. BMJ 2013 ; 346 : f3345 . OpenUrl FREE Full Text
- ↵ Sedgwick P. What is recall bias? BMJ 2012 ; 344 : e3519 . OpenUrl FREE Full Text
- ↵ Sedgwick P. Why match in case-control studies? BMJ 2012 ; 344 : e691 . OpenUrl FREE Full Text
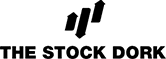
- Can I Buy Lottery...
Can I Buy Lottery Tickets with a Debit Card? An Authentic Guide
- Jun 05, 2024
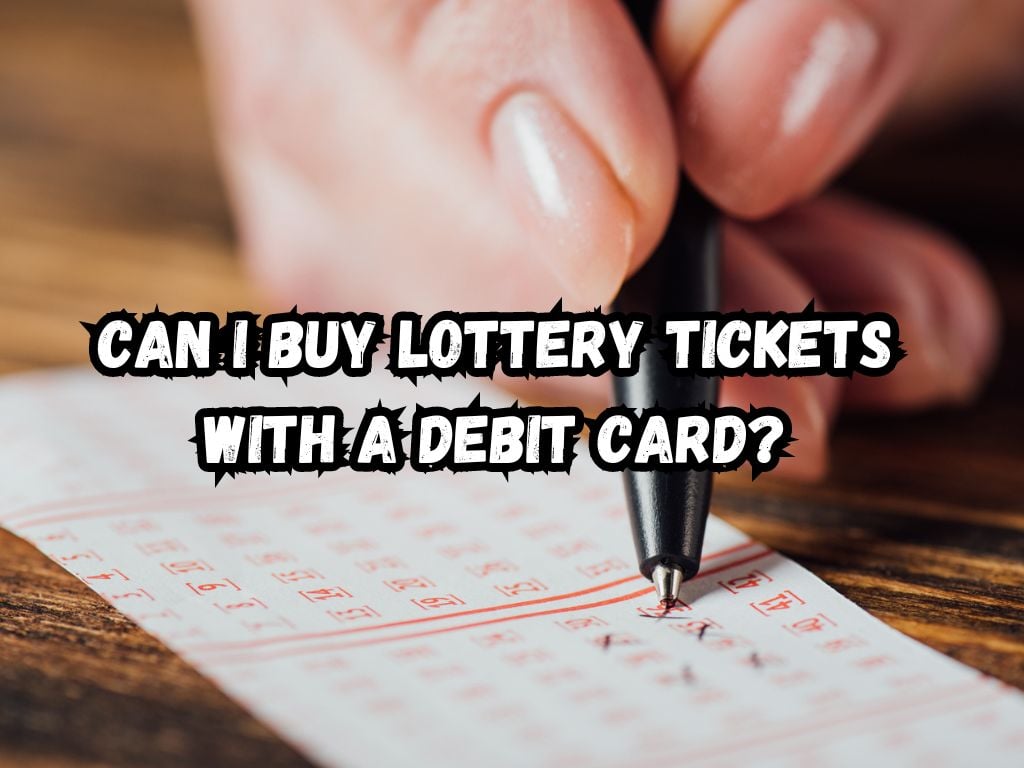
The lottery, a game of chance and dreams, captures the imagination of millions. With the advent of modern banking, many people are asking whether they can buy lottery tickets with a debit card.
This article explores the intricate details of purchasing lottery tickets with a debit card, state positions, and retailer policies that govern these transactions.
Can I Buy Lottery Tickets with a Debit Card?
The simple answer is, it depends. Various factors come into play when determining if you can use a debit card to procure lottery tickets.
It’s not a uniform yes or no across the board because state regulations and retailer policies in the US widely differ.
State Regulations and Variations
In the US, lottery regulations are governed at the state level, creating a patchwork of rules. Some states permit the use of debit cards for lottery ticket purchases, while others strictly prohibit it or leave it to the discretion of the retailers.
As of now, no federal law explicitly forbids the purchase of lottery tickets with debit cards.
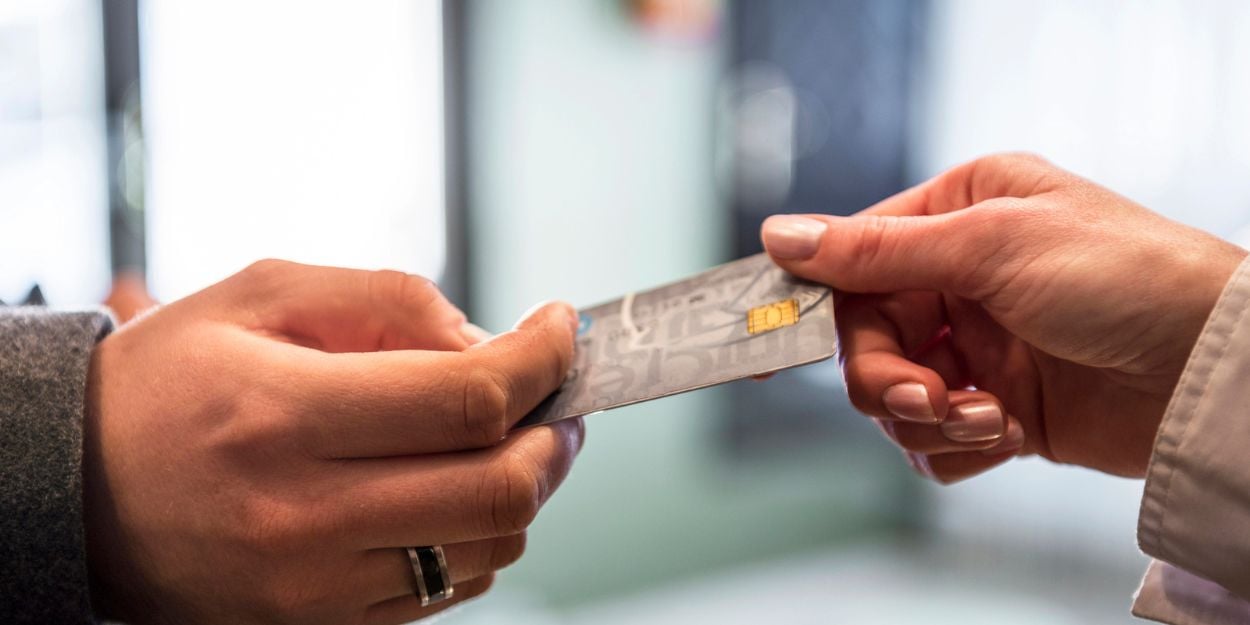
Retailers and Debit Card Acceptance
Lottery retailers may choose to accept or decline debit card payments for lottery tickets based on their own policies. Reasons for not accepting payment cards include avoiding transaction fees and reducing administrative burdens.
Large chains may have specific rules in place, so it’s prudent to inquire before attempting to make a purchase.
Pros and Cons of Using a Debit Card
Using a debit card to buy lottery tickets has its advantages. It’s a fast and convenient way to pay, directly pulling funds from your bank account.
However, one must also consider the drawbacks like added fees, possible spending limits, and it may not always be an option depending on your location.
How to Buy Lottery Tickets With a Debit Card
If you’re in a state and store that allows this type of purchase, buying lottery tickets with a debit card is straightforward.
You’ll simply swipe your card as you would for any other purchase and follow the prompts on the card reader or merchant system. Confirm any fees or unusual practices with the retailer first to avoid surprises.
Financial Tips and Responsible Gambling
When engaging in games of chance like the lottery, it’s vital to approach it with budgetary caution. Using a debit card linked to your bank can be a sound way to manage spending since it limits you to available funds.
Always factor lottery ticket purchases into your entertainment budget and avoid overspending. Gambling responsibly protects not only your finances but also your well-being.
Consumer Experiences and Case Studies
Consumer experiences with purchasing lottery tickets using debit cards vary widely, providing a wealth of insights. Take Jane from California, who appreciates the convenience and speed of using her debit card at her local convenience store .
Conversely, Tom in Texas encountered a hiccup when his bank flagged the transaction as suspicious, causing a temporary freeze on his account.
These case studies reflect the scope of outcomes—positive and negative—highlighting the importance of understanding one’s bank policies and the potential for unexpected issues when using debit cards for lottery ticket purchases.
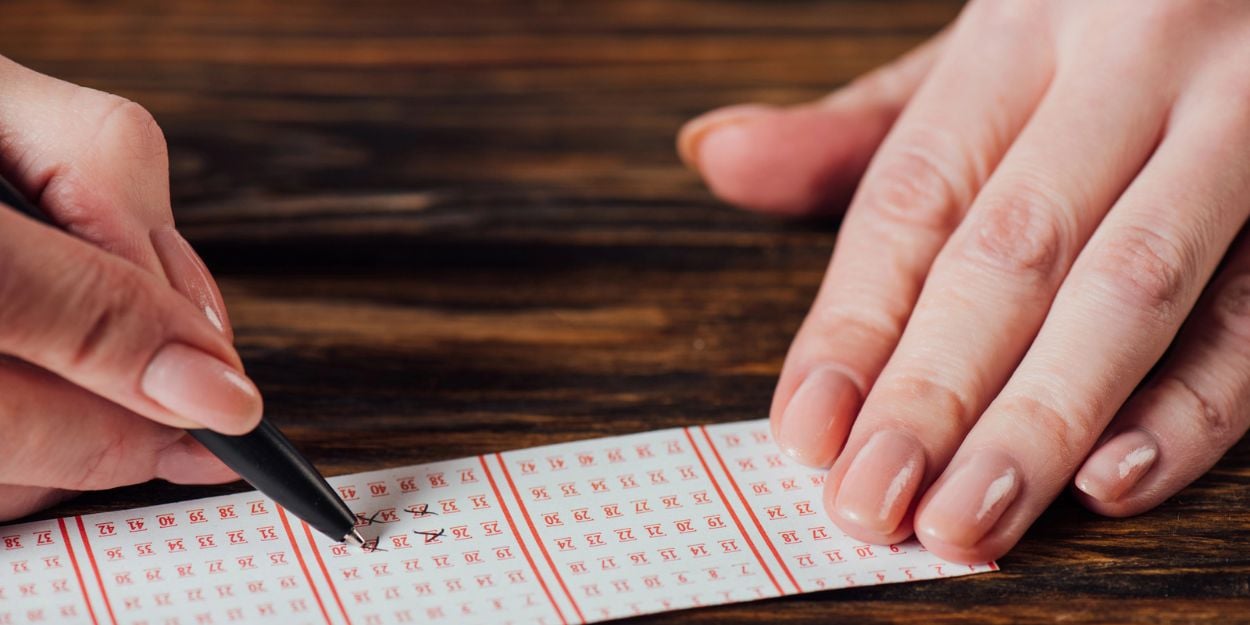
Frequently Asked Questions
Can i purchase lottery tickets with a debit card in every state.
No, you cannot purchase lottery tickets with a debit card in every state. Laws and regulations vary, with some states allowing it and others prohibiting or leaving it to retailer discretion.
Will using a debit card for lottery tickets incur additional charges?
Yes, using a debit card for lottery tickets may incur additional charges. Retailers or banks might impose transaction fees. It’s essential to check with your bank and the retailer beforehand.
What are the risks of buying lottery tickets with a debit card?
The risks of buying lottery tickets with a debit card include potential overspending and facing hidden fees. Also, transactions might be flagged by banks as suspicious, affecting your account.
Are there any benefits to buying lottery tickets with cash instead of a debit card?
Yes, buying lottery tickets with cash avoids potential transaction fees and overspending. It also ensures privacy and avoids electronic tracking of your lottery purchase habits.
Can you buy lottery tickets with a debit card at self-service kiosks?
Yes, you can buy lottery tickets with a debit card at self-service kiosks in locations where debit transactions for lottery purchases are permitted. However, availability may vary by state and retailer.
Debit card transactions for lottery tickets are a nuanced affair, with state laws and retailer policies creating a varied landscape across the country.
By staying informed and playing responsibly, you can enjoy the thrill of the lottery within your financial comfort zone.
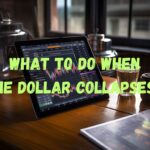
Copyright © 2024 THE STOCK DORK
- Affiliate Disclosure
- Cookie Policy
TechRepublic
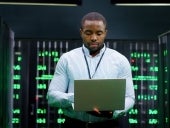
8 Best Data Science Tools and Software
Apache Spark and Hadoop, Microsoft Power BI, Jupyter Notebook and Alteryx are among the top data science tools for finding business insights. Compare their features, pros and cons.
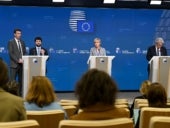
EU’s AI Act: Europe’s New Rules for Artificial Intelligence
Europe's AI legislation, adopted March 13, attempts to strike a tricky balance between promoting innovation and protecting citizens' rights.
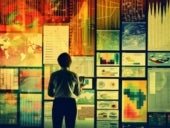
10 Best Predictive Analytics Tools and Software for 2024
Tableau, TIBCO Data Science, IBM and Sisense are among the best software for predictive analytics. Explore their features, pricing, pros and cons to find the best option for your organization.
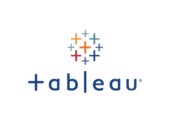
Tableau Review: Features, Pricing, Pros and Cons
Tableau has three pricing tiers that cater to all kinds of data teams, with capabilities like accelerators and real-time analytics. And if Tableau doesn’t meet your needs, it has a few alternatives worth noting.
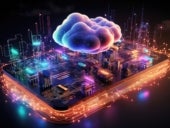
Top 6 Enterprise Data Storage Solutions for 2024
Amazon, IDrive, IBM, Google, NetApp and Wasabi offer some of the top enterprise data storage solutions. Explore their features and benefits, and find the right solution for your organization's needs.
Latest Articles
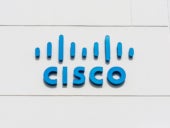
Cisco Live 2024: New Unified Observability Experience Packages Cisco & Splunk Insight Tools
The observability suite is the first major overhaul for Splunk products since the Cisco acquisition. Plus, Mistral AI makes a deal with Cisco’s incubator.
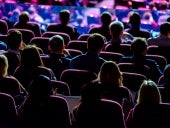
Top Tech Conferences & Events to Add to Your Calendar in 2024
A great way to stay current with the latest technology trends and innovations is by attending conferences. Read and bookmark our 2024 tech events guide.
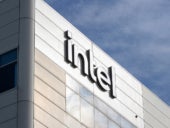
Intel Lunar Lake NPU Brings 48 TOPS of AI Acceleration
Competition for AI speed heats up. Plus, the first of the two new Xeon 6 processors is now available, and Gaudi 3 deals have been cinched with manufacturers.
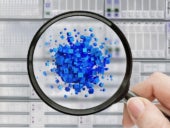
Cisco Live 2024: Cisco Unveils AI Deployment Solution With NVIDIA
A $1 billion commitment will send Cisco money to Cohere, Mistral AI and Scale AI.
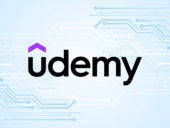
The 5 Best Udemy Courses That Are Worth Taking in 2024
Udemy is an online platform for learning at your own pace. Boost your career with our picks for the best Udemy courses for learning tech skills online in 2024.
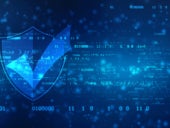
What Is Data Quality? Definition and Best Practices
Data quality refers to the degree to which data is accurate, complete, reliable and relevant for its intended use.
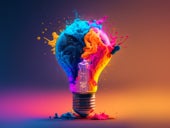
TechRepublic Premium Editorial Calendar: Policies, Checklists, Hiring Kits and Glossaries for Download
TechRepublic Premium content helps you solve your toughest IT issues and jump-start your career or next project.
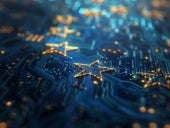
What is the EU’s AI Office? New Body Formed to Oversee the Rollout of General Purpose Models and AI Act
The AI Office will be responsible for enforcing the rules of the AI Act, ensuring its implementation across Member States, funding AI and robotics innovation and more.
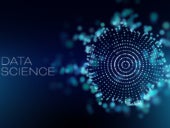
What is Data Science? Benefits, Techniques and Use Cases
Data science involves extracting valuable insights from complex datasets. While this process can be technically challenging and time-consuming, it can lead to better business decision-making.
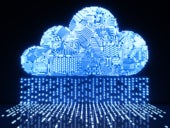
Gartner’s 7 Predictions for the Future of Australian & Global Cloud Computing
An explosion in AI computing, a big shift in workloads to the cloud, and difficulties in gaining value from hybrid cloud strategies are among the trends Australian cloud professionals will see to 2028.
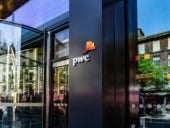
OpenAI Adds PwC as Its First Resale Partner for the ChatGPT Enterprise Tier
PwC employees have 100,000 ChatGPT Enterprise seats. Plus, OpenAI forms a new safety and security committee in their quest for more powerful AI, and seals media deals.
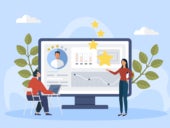
What Is Contact Management? Importance, Benefits and Tools
Contact management ensures accurate, organized and accessible information for effective communication and relationship building.
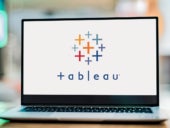
How to Use Tableau: A Step-by-Step Tutorial for Beginners
Learn how to use Tableau with this guide. From creating visualizations to analyzing data, this guide will help you master the essentials of Tableau.
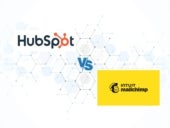
HubSpot CRM vs. Mailchimp (2024): Which Tool Is Right for You?
HubSpot and Mailchimp can do a lot of the same things. In most cases, though, one will likely be a better choice than the other for a given use case.
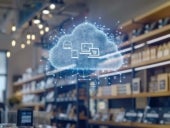
Top 5 Cloud Trends U.K. Businesses Should Watch in 2024
TechRepublic identified the top five emerging cloud technology trends that businesses in the U.K. should be aware of this year.
Create a TechRepublic Account
Get the web's best business technology news, tutorials, reviews, trends, and analysis—in your inbox. Let's start with the basics.
* - indicates required fields
Sign in to TechRepublic
Lost your password? Request a new password
Reset Password
Please enter your email adress. You will receive an email message with instructions on how to reset your password.
Check your email for a password reset link. If you didn't receive an email don't forgot to check your spam folder, otherwise contact support .
Welcome. Tell us a little bit about you.
This will help us provide you with customized content.
Want to receive more TechRepublic news?
You're all set.
Thanks for signing up! Keep an eye out for a confirmation email from our team. To ensure any newsletters you subscribed to hit your inbox, make sure to add [email protected] to your contacts list.

IMAGES
VIDEO
COMMENTS
Advantages and Disadvantages of Case-Control Studies. They are efficient for rare diseases or diseases with a long latency period between exposure and disease manifestation. They are less costly and less time-consuming; they are advantageous when exposure data is expensive or hard to obtain. They are advantageous when studying dynamic ...
A case-control study begins with the selection of cases (people with a disease) and controls (people without the disease). The controls should represent people who would have been study cases if they had developed the disease (population at risk). The exposure status to a potential cause of disease is determined for both cases and controls.
Introduction. Case-control and cohort studies are observational studies that lie near the middle of the hierarchy of evidence. These types of studies, along with randomised controlled trials, constitute analytical studies, whereas case reports and case series define descriptive studies (1). Although these studies are not ranked as highly as ...
A case-control study is a type of observational study commonly used to look at factors associated with diseases or outcomes. The case-control study starts with a group of cases, which are the individuals who have the outcome of interest. The researcher then tries to construct a second group of individuals called the controls, who are similar to ...
A case-control study is a type of observational study commonly used to look at factors associated with diseases or outcomes.[1] The case-control study starts with a group of cases, which are the individuals who have the outcome of interest. The researcher then tries to construct a second group of individuals called the controls, who are similar to the case individuals but do not have the ...
Revised on June 22, 2023. A case-control study is an experimental design that compares a group of participants possessing a condition of interest to a very similar group lacking that condition. Here, the participants possessing the attribute of study, such as a disease, are called the "case," and those without it are the "control.".
Case-control studies are one of the major observational study designs for performing clinical research. The advantages of these study designs over other study designs are that they are relatively quick to perform, economical, and easy to design and implement. Case-control studies are particularly appropriate for studying disease outbreaks, rare diseases, or outcomes of interest.
A case-control study can help provide extra insight on data that has already been collected. A case-control study is a way of carrying out a medical investigation to confirm or indicate what is ...
Case-control studies are one of the major observational study designs for performing clinical research. The advantages of these study designs over other study designs are that they are relatively quick to perform, economical, and easy to design and implement. Case-control studies are particularly appropriate for studying disease outbreaks, rare ...
Characteristics of Case-Control Studies. How do case-control studies fit into classifications of research design described in an earlier article? 1 Case-control studies are empirical studies that are based on samples, not individual cases or case series. They are cross-sectional because cases and controls are identified and evaluated for caseness, historical exposures, and confounding ...
General Overview of Case-Control Studies. In observational studies, also called epidemiologic studies, the primary objective is to discover and quantify an association between exposures and the outcome of interest, in hopes of drawing causal inference. Observational studies can have a retrospective study design, a prospective design, a cross ...
Cohort studies and case-control studies are two primary types of observational studies that aid in evaluating associations between diseases and exposures. In this review article, we describe these study designs, methodological issues, and provide examples from the plastic surgery literature. Keywords: observational studies, case-control study ...
Disadvantages of Retrospective Cohort Studies. As with prospective cohort studies, they are not good for very rare diseases. If one uses records that were not designed for the study, the available data may be of poor quality. There is frequently an absence of data on potential confounding factors if the data was recorded in the past.
Case-control studies: advantages and disadvantages BMJ. 2014 Jan 3:348:f7707. doi: 10.1136/bmj.f7707. Author Philip Sedgwick 1 Affiliation 1 Centre for Medical and Healthcare Education, St George's, University of London, London, UK [email protected]. PMID: 31419845 DOI: 10.1136 ...
A case-control study was conducted to investigate if exposure to zinc oxide is a more effective skin cancer prevention measure. The study involved comparing a group of former lifeguards that had developed cancer on their cheeks and noses (cases) to a group of lifeguards without this type of cancer (controls) and assess their prior exposure to ...
A case-control study was performed in the city of Samara, 700 miles south east of Moscow. Cases were 334 consecutive adults diagnosed as having culture confirmed pulmonary tuberculosis at any of the city's specialist tuberculosis clinics between 1 January 2003 and 31 December 2003. For each case, a control matched for year of birth and sex ...
A case-control study is a type of observational study. It looks at 2 sets of participants. One group has the condition you are interested in (the cases) and one group does not have it (the ...
The main advantages of a nested case-control study are as follows: (1) cost reduction and effort minimization, as only a fraction of the parent cohort requires the necessary outcome assessment; (2) reduced selection bias, as both case and control subjects are sampled from the same population; and (3) flexibility in analysis by allowing testing of a hypotheses in the future that is not ...
Case-control studies: Part 2. Arbitration agreement. September 2014:145 (3) In the previous article, I defined the case-control study and discussed issues regarding the selection of controls. In this second article devoted to case-control studies, I will discuss bias and the advantages and disadvantage of this clinical study design.
[email protected]'s blog. Epidemiology: Advantages and Disadvantages of Case Control Studies. Advantages. Easy to conduct as no follow up is required. No attrition, as no follow up is needed. Gives faster results. Inexpensive. Suitable for rare and newly identified diseases. More than one risk factors can be studied simultaneously.
Case-control study and cohort study both are types of observational study. Let's find out, in what cases, the case-control or cohort study should be implemented. ... Pros and Cons. Strengths of Case-Control Studies: Can be relatively quickly done; Can be relatively inexpensive; Good for diseases with short or long latency/duration;
Cohort and Case-Control Studies. The two most popular designs for investigating causal hypotheses are the cohort and case-control studies. Features are shown in Table 1.The major difference between the two is that the cohort study begins with identification of the exposure status, whereas the case-control study begins with the identification of the outcome.
Researchers investigated whether antipsychotic drugs were associated with venous thromboembolism. A population based nested case-control study design was used. Data were taken from the UK QResearch primary care database consisting of 7 267 673 patients. Cases were adult patients with a first ever record of venous thromboembolism between 1 January 1996 and 1 July 2007. For each case, up to four ...
HRM 572 Module 5-Case Study: Training Approaches-Pros & Cons The first training apparatus that will be examined is using technology to train employees, and this case study will start with the cons of using electronic components to improve staff. The first con to using technology to teach in today's era begins with the distracting demeanor that ...
Pros and Cons of Using a Debit Card. Using a debit card to buy lottery tickets has its advantages. It's a fast and convenient way to pay, directly pulling funds from your bank account. ... These case studies reflect the scope of outcomes—positive and negative—highlighting the importance of understanding one's bank policies and the ...
Big Data Big Data Tableau Review: Features, Pricing, Pros and Cons . Tableau has three pricing tiers that cater to all kinds of data teams, with capabilities like accelerators and real-time analytics.