

Understanding Nursing Research
- Primary Research
- Qualitative vs. Quantitative Research
- Experimental Design
- Is it a Nursing journal?
- Is it Written by a Nurse?
Secondary Research and Systematic Reviews
Comparative table.
- Quality Improvement Plans
Secondary Research is when researchers collect lots of research that has already been published on a certain subject. They conduct searches in databases, go through lots of primary research articles, and analyze the findings in those pieces of primary research. The goal of secondary research is to pull together lots of diverse primary research (like studies and trials), with the end goal of making a generalized statement. Primary research can only make statements about the specific context in which their research was conducted (for example, this specific intervention worked in this hospital with these participants), but secondary research can make broader statements because it compiled lots of primary research together. So rather than saying, "this specific intervention worked at this specific hospital with these specific participants, a piece of secondary research can say, "This intervention works at hospitals that serve this population."
Systematic Reviews are a kind of secondary research. The creators of systematic reviews are very intentional about their inclusion/exclusion criteria, or which articles they'll include in their review and the goal is to make a generalized statement so other researchers can build upon the practices or interventions they recommend. Use the chart below to understand the differences between a systematic review and a literature review.
Check out the video below to watch the Nursing and Health Sciences librarian describe the differences between primary and secondary research.
Literature Review | Systematic Review | Meta-Analysis |
---|---|---|
|
- "Literature Reviews and Systematic Reviews: What Is the Difference?" This article explains in depth the differences between Literature Reviews and Systematic Reviews. It is from the journal RADIOLOGIC TECHNOLOGY, Nov/Dec 2013, v. 85, #2. It is one to which Bell Library subscribes and meets copyright clearance requirements through our subscription to CCC.
- << Previous: Is it Written by a Nurse?
- Next: Permalinks >>
- Last Updated: May 23, 2024 4:16 PM
- URL: https://guides.library.tamucc.edu/nursingresearch
Log in using your username and password
- Search More Search for this keyword Advanced search
- Latest content
- Current issue
- Write for Us
- BMJ Journals
You are here
- Volume 14, Issue 3
- What is a systematic review?
- Article Text
- Article info
- Citation Tools
- Rapid Responses
- Article metrics

- Jane Clarke
- Correspondence to Jane Clarke 4 Prime Road, Grey Lynn, Auckland, New Zealand; janeclarkehome{at}gmail.com
https://doi.org/10.1136/ebn.2011.0049
Statistics from Altmetric.com
Request permissions.
If you wish to reuse any or all of this article please use the link below which will take you to the Copyright Clearance Center’s RightsLink service. You will be able to get a quick price and instant permission to reuse the content in many different ways.
A high-quality systematic review is described as the most reliable source of evidence to guide clinical practice. The purpose of a systematic review is to deliver a meticulous summary of all the available primary research in response to a research question. A systematic review uses all the existing research and is sometime called ‘secondary research’ (research on research). They are often required by research funders to establish the state of existing knowledge and are frequently used in guideline development. Systematic review findings are often used within the …
Competing interests None.
Read the full text or download the PDF:

An official website of the United States government
The .gov means it’s official. Federal government websites often end in .gov or .mil. Before sharing sensitive information, make sure you’re on a federal government site.
The site is secure. The https:// ensures that you are connecting to the official website and that any information you provide is encrypted and transmitted securely.
- Publications
- Account settings
- My Bibliography
- Collections
- Citation manager
Save citation to file
Email citation, add to collections.
- Create a new collection
- Add to an existing collection
Add to My Bibliography
Your saved search, create a file for external citation management software, your rss feed.
- Search in PubMed
- Search in NLM Catalog
- Add to Search
How to Do a Systematic Review: A Best Practice Guide for Conducting and Reporting Narrative Reviews, Meta-Analyses, and Meta-Syntheses
Affiliations.
- 1 Behavioural Science Centre, Stirling Management School, University of Stirling, Stirling FK9 4LA, United Kingdom; email: [email protected].
- 2 Department of Psychological and Behavioural Science, London School of Economics and Political Science, London WC2A 2AE, United Kingdom.
- 3 Department of Statistics, Northwestern University, Evanston, Illinois 60208, USA; email: [email protected].
- PMID: 30089228
- DOI: 10.1146/annurev-psych-010418-102803
Systematic reviews are characterized by a methodical and replicable methodology and presentation. They involve a comprehensive search to locate all relevant published and unpublished work on a subject; a systematic integration of search results; and a critique of the extent, nature, and quality of evidence in relation to a particular research question. The best reviews synthesize studies to draw broad theoretical conclusions about what a literature means, linking theory to evidence and evidence to theory. This guide describes how to plan, conduct, organize, and present a systematic review of quantitative (meta-analysis) or qualitative (narrative review, meta-synthesis) information. We outline core standards and principles and describe commonly encountered problems. Although this guide targets psychological scientists, its high level of abstraction makes it potentially relevant to any subject area or discipline. We argue that systematic reviews are a key methodology for clarifying whether and how research findings replicate and for explaining possible inconsistencies, and we call for researchers to conduct systematic reviews to help elucidate whether there is a replication crisis.
Keywords: evidence; guide; meta-analysis; meta-synthesis; narrative; systematic review; theory.
PubMed Disclaimer
Similar articles
- The future of Cochrane Neonatal. Soll RF, Ovelman C, McGuire W. Soll RF, et al. Early Hum Dev. 2020 Nov;150:105191. doi: 10.1016/j.earlhumdev.2020.105191. Epub 2020 Sep 12. Early Hum Dev. 2020. PMID: 33036834
- A Primer on Systematic Reviews and Meta-Analyses. Nguyen NH, Singh S. Nguyen NH, et al. Semin Liver Dis. 2018 May;38(2):103-111. doi: 10.1055/s-0038-1655776. Epub 2018 Jun 5. Semin Liver Dis. 2018. PMID: 29871017 Review.
- Publication Bias and Nonreporting Found in Majority of Systematic Reviews and Meta-analyses in Anesthesiology Journals. Hedin RJ, Umberham BA, Detweiler BN, Kollmorgen L, Vassar M. Hedin RJ, et al. Anesth Analg. 2016 Oct;123(4):1018-25. doi: 10.1213/ANE.0000000000001452. Anesth Analg. 2016. PMID: 27537925 Review.
- Summarizing systematic reviews: methodological development, conduct and reporting of an umbrella review approach. Aromataris E, Fernandez R, Godfrey CM, Holly C, Khalil H, Tungpunkom P. Aromataris E, et al. Int J Evid Based Healthc. 2015 Sep;13(3):132-40. doi: 10.1097/XEB.0000000000000055. Int J Evid Based Healthc. 2015. PMID: 26360830
- RAMESES publication standards: meta-narrative reviews. Wong G, Greenhalgh T, Westhorp G, Buckingham J, Pawson R. Wong G, et al. BMC Med. 2013 Jan 29;11:20. doi: 10.1186/1741-7015-11-20. BMC Med. 2013. PMID: 23360661 Free PMC article.
- Surveillance of Occupational Exposure to Volatile Organic Compounds at Gas Stations: A Scoping Review Protocol. Mendes TMC, Soares JP, Salvador PTCO, Castro JL. Mendes TMC, et al. Int J Environ Res Public Health. 2024 Apr 23;21(5):518. doi: 10.3390/ijerph21050518. Int J Environ Res Public Health. 2024. PMID: 38791733 Free PMC article. Review.
- Association between poor sleep and mental health issues in Indigenous communities across the globe: a systematic review. Fernandez DR, Lee R, Tran N, Jabran DS, King S, McDaid L. Fernandez DR, et al. Sleep Adv. 2024 May 2;5(1):zpae028. doi: 10.1093/sleepadvances/zpae028. eCollection 2024. Sleep Adv. 2024. PMID: 38721053 Free PMC article.
- Barriers to ethical treatment of patients in clinical environments: A systematic narrative review. Dehkordi FG, Torabizadeh C, Rakhshan M, Vizeshfar F. Dehkordi FG, et al. Health Sci Rep. 2024 May 1;7(5):e2008. doi: 10.1002/hsr2.2008. eCollection 2024 May. Health Sci Rep. 2024. PMID: 38698790 Free PMC article.
- Studying Adherence to Reporting Standards in Kinesiology: A Post-publication Peer Review Brief Report. Watson NM, Thomas JD. Watson NM, et al. Int J Exerc Sci. 2024 Jan 1;17(7):25-37. eCollection 2024. Int J Exerc Sci. 2024. PMID: 38666001 Free PMC article.
- Evidence for Infant-directed Speech Preference Is Consistent Across Large-scale, Multi-site Replication and Meta-analysis. Zettersten M, Cox C, Bergmann C, Tsui ASM, Soderstrom M, Mayor J, Lundwall RA, Lewis M, Kosie JE, Kartushina N, Fusaroli R, Frank MC, Byers-Heinlein K, Black AK, Mathur MB. Zettersten M, et al. Open Mind (Camb). 2024 Apr 3;8:439-461. doi: 10.1162/opmi_a_00134. eCollection 2024. Open Mind (Camb). 2024. PMID: 38665547 Free PMC article.
- Search in MeSH
LinkOut - more resources
Full text sources.
- Ingenta plc
- Ovid Technologies, Inc.
Other Literature Sources
- scite Smart Citations
Miscellaneous
- NCI CPTAC Assay Portal
- Citation Manager
NCBI Literature Resources
MeSH PMC Bookshelf Disclaimer
The PubMed wordmark and PubMed logo are registered trademarks of the U.S. Department of Health and Human Services (HHS). Unauthorized use of these marks is strictly prohibited.
- Locations and Hours
- UCLA Library
- Research Guides
- Biomedical Library Guides
Systematic Reviews
- Types of Literature Reviews
What Makes a Systematic Review Different from Other Types of Reviews?
- Planning Your Systematic Review
- Database Searching
- Creating the Search
- Search Filters and Hedges
- Grey Literature
- Managing and Appraising Results
- Further Resources
Reproduced from Grant, M. J. and Booth, A. (2009), A typology of reviews: an analysis of 14 review types and associated methodologies. Health Information & Libraries Journal, 26: 91–108. doi:10.1111/j.1471-1842.2009.00848.x
Aims to demonstrate writer has extensively researched literature and critically evaluated its quality. Goes beyond mere description to include degree of analysis and conceptual innovation. Typically results in hypothesis or mode | Seeks to identify most significant items in the field | No formal quality assessment. Attempts to evaluate according to contribution | Typically narrative, perhaps conceptual or chronological | Significant component: seeks to identify conceptual contribution to embody existing or derive new theory | |
Generic term: published materials that provide examination of recent or current literature. Can cover wide range of subjects at various levels of completeness and comprehensiveness. May include research findings | May or may not include comprehensive searching | May or may not include quality assessment | Typically narrative | Analysis may be chronological, conceptual, thematic, etc. | |
Mapping review/ systematic map | Map out and categorize existing literature from which to commission further reviews and/or primary research by identifying gaps in research literature | Completeness of searching determined by time/scope constraints | No formal quality assessment | May be graphical and tabular | Characterizes quantity and quality of literature, perhaps by study design and other key features. May identify need for primary or secondary research |
Technique that statistically combines the results of quantitative studies to provide a more precise effect of the results | Aims for exhaustive, comprehensive searching. May use funnel plot to assess completeness | Quality assessment may determine inclusion/ exclusion and/or sensitivity analyses | Graphical and tabular with narrative commentary | Numerical analysis of measures of effect assuming absence of heterogeneity | |
Refers to any combination of methods where one significant component is a literature review (usually systematic). Within a review context it refers to a combination of review approaches for example combining quantitative with qualitative research or outcome with process studies | Requires either very sensitive search to retrieve all studies or separately conceived quantitative and qualitative strategies | Requires either a generic appraisal instrument or separate appraisal processes with corresponding checklists | Typically both components will be presented as narrative and in tables. May also employ graphical means of integrating quantitative and qualitative studies | Analysis may characterise both literatures and look for correlations between characteristics or use gap analysis to identify aspects absent in one literature but missing in the other | |
Generic term: summary of the [medical] literature that attempts to survey the literature and describe its characteristics | May or may not include comprehensive searching (depends whether systematic overview or not) | May or may not include quality assessment (depends whether systematic overview or not) | Synthesis depends on whether systematic or not. Typically narrative but may include tabular features | Analysis may be chronological, conceptual, thematic, etc. | |
Method for integrating or comparing the findings from qualitative studies. It looks for ‘themes’ or ‘constructs’ that lie in or across individual qualitative studies | May employ selective or purposive sampling | Quality assessment typically used to mediate messages not for inclusion/exclusion | Qualitative, narrative synthesis | Thematic analysis, may include conceptual models | |
Assessment of what is already known about a policy or practice issue, by using systematic review methods to search and critically appraise existing research | Completeness of searching determined by time constraints | Time-limited formal quality assessment | Typically narrative and tabular | Quantities of literature and overall quality/direction of effect of literature | |
Preliminary assessment of potential size and scope of available research literature. Aims to identify nature and extent of research evidence (usually including ongoing research) | Completeness of searching determined by time/scope constraints. May include research in progress | No formal quality assessment | Typically tabular with some narrative commentary | Characterizes quantity and quality of literature, perhaps by study design and other key features. Attempts to specify a viable review | |
Tend to address more current matters in contrast to other combined retrospective and current approaches. May offer new perspectives | Aims for comprehensive searching of current literature | No formal quality assessment | Typically narrative, may have tabular accompaniment | Current state of knowledge and priorities for future investigation and research | |
Seeks to systematically search for, appraise and synthesis research evidence, often adhering to guidelines on the conduct of a review | Aims for exhaustive, comprehensive searching | Quality assessment may determine inclusion/exclusion | Typically narrative with tabular accompaniment | What is known; recommendations for practice. What remains unknown; uncertainty around findings, recommendations for future research | |
Combines strengths of critical review with a comprehensive search process. Typically addresses broad questions to produce ‘best evidence synthesis’ | Aims for exhaustive, comprehensive searching | May or may not include quality assessment | Minimal narrative, tabular summary of studies | What is known; recommendations for practice. Limitations | |
Attempt to include elements of systematic review process while stopping short of systematic review. Typically conducted as postgraduate student assignment | May or may not include comprehensive searching | May or may not include quality assessment | Typically narrative with tabular accompaniment | What is known; uncertainty around findings; limitations of methodology | |
Specifically refers to review compiling evidence from multiple reviews into one accessible and usable document. Focuses on broad condition or problem for which there are competing interventions and highlights reviews that address these interventions and their results | Identification of component reviews, but no search for primary studies | Quality assessment of studies within component reviews and/or of reviews themselves | Graphical and tabular with narrative commentary | What is known; recommendations for practice. What remains unknown; recommendations for future research |
- << Previous: Home
- Next: Planning Your Systematic Review >>
- Last Updated: Apr 17, 2024 2:02 PM
- URL: https://guides.library.ucla.edu/systematicreviews
Have a language expert improve your writing
Run a free plagiarism check in 10 minutes, automatically generate references for free.
- Knowledge Base
- Methodology
- Systematic Review | Definition, Examples & Guide
Systematic Review | Definition, Examples & Guide
Published on 15 June 2022 by Shaun Turney . Revised on 17 October 2022.
A systematic review is a type of review that uses repeatable methods to find, select, and synthesise all available evidence. It answers a clearly formulated research question and explicitly states the methods used to arrive at the answer.
They answered the question ‘What is the effectiveness of probiotics in reducing eczema symptoms and improving quality of life in patients with eczema?’
In this context, a probiotic is a health product that contains live microorganisms and is taken by mouth. Eczema is a common skin condition that causes red, itchy skin.
Table of contents
What is a systematic review, systematic review vs meta-analysis, systematic review vs literature review, systematic review vs scoping review, when to conduct a systematic review, pros and cons of systematic reviews, step-by-step example of a systematic review, frequently asked questions about systematic reviews.
A review is an overview of the research that’s already been completed on a topic.
What makes a systematic review different from other types of reviews is that the research methods are designed to reduce research bias . The methods are repeatable , and the approach is formal and systematic:
- Formulate a research question
- Develop a protocol
- Search for all relevant studies
- Apply the selection criteria
- Extract the data
- Synthesise the data
- Write and publish a report
Although multiple sets of guidelines exist, the Cochrane Handbook for Systematic Reviews is among the most widely used. It provides detailed guidelines on how to complete each step of the systematic review process.
Systematic reviews are most commonly used in medical and public health research, but they can also be found in other disciplines.
Systematic reviews typically answer their research question by synthesising all available evidence and evaluating the quality of the evidence. Synthesising means bringing together different information to tell a single, cohesive story. The synthesis can be narrative ( qualitative ), quantitative , or both.
Prevent plagiarism, run a free check.
Systematic reviews often quantitatively synthesise the evidence using a meta-analysis . A meta-analysis is a statistical analysis, not a type of review.
A meta-analysis is a technique to synthesise results from multiple studies. It’s a statistical analysis that combines the results of two or more studies, usually to estimate an effect size .
A literature review is a type of review that uses a less systematic and formal approach than a systematic review. Typically, an expert in a topic will qualitatively summarise and evaluate previous work, without using a formal, explicit method.
Although literature reviews are often less time-consuming and can be insightful or helpful, they have a higher risk of bias and are less transparent than systematic reviews.
Similar to a systematic review, a scoping review is a type of review that tries to minimise bias by using transparent and repeatable methods.
However, a scoping review isn’t a type of systematic review. The most important difference is the goal: rather than answering a specific question, a scoping review explores a topic. The researcher tries to identify the main concepts, theories, and evidence, as well as gaps in the current research.
Sometimes scoping reviews are an exploratory preparation step for a systematic review, and sometimes they are a standalone project.
A systematic review is a good choice of review if you want to answer a question about the effectiveness of an intervention , such as a medical treatment.
To conduct a systematic review, you’ll need the following:
- A precise question , usually about the effectiveness of an intervention. The question needs to be about a topic that’s previously been studied by multiple researchers. If there’s no previous research, there’s nothing to review.
- If you’re doing a systematic review on your own (e.g., for a research paper or thesis), you should take appropriate measures to ensure the validity and reliability of your research.
- Access to databases and journal archives. Often, your educational institution provides you with access.
- Time. A professional systematic review is a time-consuming process: it will take the lead author about six months of full-time work. If you’re a student, you should narrow the scope of your systematic review and stick to a tight schedule.
- Bibliographic, word-processing, spreadsheet, and statistical software . For example, you could use EndNote, Microsoft Word, Excel, and SPSS.
A systematic review has many pros .
- They minimise research b ias by considering all available evidence and evaluating each study for bias.
- Their methods are transparent , so they can be scrutinised by others.
- They’re thorough : they summarise all available evidence.
- They can be replicated and updated by others.
Systematic reviews also have a few cons .
- They’re time-consuming .
- They’re narrow in scope : they only answer the precise research question.
The 7 steps for conducting a systematic review are explained with an example.
Step 1: Formulate a research question
Formulating the research question is probably the most important step of a systematic review. A clear research question will:
- Allow you to more effectively communicate your research to other researchers and practitioners
- Guide your decisions as you plan and conduct your systematic review
A good research question for a systematic review has four components, which you can remember with the acronym PICO :
- Population(s) or problem(s)
- Intervention(s)
- Comparison(s)
You can rearrange these four components to write your research question:
- What is the effectiveness of I versus C for O in P ?
Sometimes, you may want to include a fourth component, the type of study design . In this case, the acronym is PICOT .
- Type of study design(s)
- The population of patients with eczema
- The intervention of probiotics
- In comparison to no treatment, placebo , or non-probiotic treatment
- The outcome of changes in participant-, parent-, and doctor-rated symptoms of eczema and quality of life
- Randomised control trials, a type of study design
Their research question was:
- What is the effectiveness of probiotics versus no treatment, a placebo, or a non-probiotic treatment for reducing eczema symptoms and improving quality of life in patients with eczema?
Step 2: Develop a protocol
A protocol is a document that contains your research plan for the systematic review. This is an important step because having a plan allows you to work more efficiently and reduces bias.
Your protocol should include the following components:
- Background information : Provide the context of the research question, including why it’s important.
- Research objective(s) : Rephrase your research question as an objective.
- Selection criteria: State how you’ll decide which studies to include or exclude from your review.
- Search strategy: Discuss your plan for finding studies.
- Analysis: Explain what information you’ll collect from the studies and how you’ll synthesise the data.
If you’re a professional seeking to publish your review, it’s a good idea to bring together an advisory committee . This is a group of about six people who have experience in the topic you’re researching. They can help you make decisions about your protocol.
It’s highly recommended to register your protocol. Registering your protocol means submitting it to a database such as PROSPERO or ClinicalTrials.gov .
Step 3: Search for all relevant studies
Searching for relevant studies is the most time-consuming step of a systematic review.
To reduce bias, it’s important to search for relevant studies very thoroughly. Your strategy will depend on your field and your research question, but sources generally fall into these four categories:
- Databases: Search multiple databases of peer-reviewed literature, such as PubMed or Scopus . Think carefully about how to phrase your search terms and include multiple synonyms of each word. Use Boolean operators if relevant.
- Handsearching: In addition to searching the primary sources using databases, you’ll also need to search manually. One strategy is to scan relevant journals or conference proceedings. Another strategy is to scan the reference lists of relevant studies.
- Grey literature: Grey literature includes documents produced by governments, universities, and other institutions that aren’t published by traditional publishers. Graduate student theses are an important type of grey literature, which you can search using the Networked Digital Library of Theses and Dissertations (NDLTD) . In medicine, clinical trial registries are another important type of grey literature.
- Experts: Contact experts in the field to ask if they have unpublished studies that should be included in your review.
At this stage of your review, you won’t read the articles yet. Simply save any potentially relevant citations using bibliographic software, such as Scribbr’s APA or MLA Generator .
- Databases: EMBASE, PsycINFO, AMED, LILACS, and ISI Web of Science
- Handsearch: Conference proceedings and reference lists of articles
- Grey literature: The Cochrane Library, the metaRegister of Controlled Trials, and the Ongoing Skin Trials Register
- Experts: Authors of unpublished registered trials, pharmaceutical companies, and manufacturers of probiotics
Step 4: Apply the selection criteria
Applying the selection criteria is a three-person job. Two of you will independently read the studies and decide which to include in your review based on the selection criteria you established in your protocol . The third person’s job is to break any ties.
To increase inter-rater reliability , ensure that everyone thoroughly understands the selection criteria before you begin.
If you’re writing a systematic review as a student for an assignment, you might not have a team. In this case, you’ll have to apply the selection criteria on your own; you can mention this as a limitation in your paper’s discussion.
You should apply the selection criteria in two phases:
- Based on the titles and abstracts : Decide whether each article potentially meets the selection criteria based on the information provided in the abstracts.
- Based on the full texts: Download the articles that weren’t excluded during the first phase. If an article isn’t available online or through your library, you may need to contact the authors to ask for a copy. Read the articles and decide which articles meet the selection criteria.
It’s very important to keep a meticulous record of why you included or excluded each article. When the selection process is complete, you can summarise what you did using a PRISMA flow diagram .
Next, Boyle and colleagues found the full texts for each of the remaining studies. Boyle and Tang read through the articles to decide if any more studies needed to be excluded based on the selection criteria.
When Boyle and Tang disagreed about whether a study should be excluded, they discussed it with Varigos until the three researchers came to an agreement.
Step 5: Extract the data
Extracting the data means collecting information from the selected studies in a systematic way. There are two types of information you need to collect from each study:
- Information about the study’s methods and results . The exact information will depend on your research question, but it might include the year, study design , sample size, context, research findings , and conclusions. If any data are missing, you’ll need to contact the study’s authors.
- Your judgement of the quality of the evidence, including risk of bias .
You should collect this information using forms. You can find sample forms in The Registry of Methods and Tools for Evidence-Informed Decision Making and the Grading of Recommendations, Assessment, Development and Evaluations Working Group .
Extracting the data is also a three-person job. Two people should do this step independently, and the third person will resolve any disagreements.
They also collected data about possible sources of bias, such as how the study participants were randomised into the control and treatment groups.
Step 6: Synthesise the data
Synthesising the data means bringing together the information you collected into a single, cohesive story. There are two main approaches to synthesising the data:
- Narrative ( qualitative ): Summarise the information in words. You’ll need to discuss the studies and assess their overall quality.
- Quantitative : Use statistical methods to summarise and compare data from different studies. The most common quantitative approach is a meta-analysis , which allows you to combine results from multiple studies into a summary result.
Generally, you should use both approaches together whenever possible. If you don’t have enough data, or the data from different studies aren’t comparable, then you can take just a narrative approach. However, you should justify why a quantitative approach wasn’t possible.
Boyle and colleagues also divided the studies into subgroups, such as studies about babies, children, and adults, and analysed the effect sizes within each group.
Step 7: Write and publish a report
The purpose of writing a systematic review article is to share the answer to your research question and explain how you arrived at this answer.
Your article should include the following sections:
- Abstract : A summary of the review
- Introduction : Including the rationale and objectives
- Methods : Including the selection criteria, search method, data extraction method, and synthesis method
- Results : Including results of the search and selection process, study characteristics, risk of bias in the studies, and synthesis results
- Discussion : Including interpretation of the results and limitations of the review
- Conclusion : The answer to your research question and implications for practice, policy, or research
To verify that your report includes everything it needs, you can use the PRISMA checklist .
Once your report is written, you can publish it in a systematic review database, such as the Cochrane Database of Systematic Reviews , and/or in a peer-reviewed journal.
A systematic review is secondary research because it uses existing research. You don’t collect new data yourself.
A literature review is a survey of scholarly sources (such as books, journal articles, and theses) related to a specific topic or research question .
It is often written as part of a dissertation , thesis, research paper , or proposal .
There are several reasons to conduct a literature review at the beginning of a research project:
- To familiarise yourself with the current state of knowledge on your topic
- To ensure that you’re not just repeating what others have already done
- To identify gaps in knowledge and unresolved problems that your research can address
- To develop your theoretical framework and methodology
- To provide an overview of the key findings and debates on the topic
Writing the literature review shows your reader how your work relates to existing research and what new insights it will contribute.
Cite this Scribbr article
If you want to cite this source, you can copy and paste the citation or click the ‘Cite this Scribbr article’ button to automatically add the citation to our free Reference Generator.
Turney, S. (2022, October 17). Systematic Review | Definition, Examples & Guide. Scribbr. Retrieved 2 July 2024, from https://www.scribbr.co.uk/research-methods/systematic-reviews/
Is this article helpful?
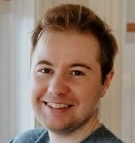
Shaun Turney
Other students also liked, what is a literature review | guide, template, & examples, exploratory research | definition, guide, & examples, what is peer review | types & examples.

Understanding research and critical appraisal
- Introduction
What is secondary research?
Secondary research study designs.
- Primary research
- Critical appraisal of research papers
- Useful terminology
- Further reading and helpful resources
The aim of secondary research is to produce a more or less systematic appraisal and/or synthesis of the existing primary research on a topic. There are numerous types of reviews which aim to summarise or synthesise the evidence on a topic, but here we will focus on two: meta-analyses and systematic reviews.
For a fuller discussion of the range of review types, their features and uses, see: Sutton, A. et al . (2019) 'Meeting the review family: exploring review types and associated information retrieval requirements', Health Information and Libraries Journal , 36 (3), pp. 202-222. doi:10.1111/hir.12276
Meta-analysis
A meta-analysis is a statistical synthesis of the results from multiple individual studies, usually randomised controlled trials (RCTs),
Carrying out a meta-analysis of studies allows results from multiple studies looking at the effect of an intervention to be combined, allowing for greater precision in the estimation of effects, and clarity over the direction and size of an effect. A meta-analysis can provide more conclusive evidence for or against the effectiveness of an intervention than individual studies alone.
A good meta-analysis should always be based on a systematic review of studies, and requires some homogeneity of participants, settings, interventions and outcome measures in the studies included.
Systematic review
A systematic review is not simply a literature review. A systematic review is a study which aims to synthesise all of the available primary research on a specific topic. The first step in a systematic review is a thorough search of all appropriate sources, including subject related databases, clinical trial registers and grey literature, in order to identify all of the relevant evidence. These searches should ideally be carried out by a librarian or information specialist in the field, or by others with a similar level of expertise. The systematic review itself should be carried out by two or more researchers, as a means of reducing possible bias.
All identified studies are screened for inclusion or exclusion according to strict criteria set out at the start of the study, and the data from those studies selected for inclusion is analysed and synthesised. Part of this process is an attempt to identify any potential source of bias in existing findings. A systematic review will offer a summary of the available research findings, and offer conclusions on the basis of these, taking into account any flaws or limitations in the original studies.
A systematic review can offer more generalisability and consistency of research findings than the individual studies on which it is based.
Systematic reviews may employ quantitative, qualitative (experiential), or mixed-methods approaches.
- << Previous: Introduction
- Next: Primary research >>
- Last Updated: Mar 26, 2024 4:38 PM
- URL: https://libguides.sgul.ac.uk/researchdesign
Have a language expert improve your writing
Run a free plagiarism check in 10 minutes, generate accurate citations for free.
- Knowledge Base
Methodology
- What is Secondary Research? | Definition, Types, & Examples
What is Secondary Research? | Definition, Types, & Examples
Published on January 20, 2023 by Tegan George . Revised on January 12, 2024.
Secondary research is a research method that uses data that was collected by someone else. In other words, whenever you conduct research using data that already exists, you are conducting secondary research. On the other hand, any type of research that you undertake yourself is called primary research .
Secondary research can be qualitative or quantitative in nature. It often uses data gathered from published peer-reviewed papers, meta-analyses, or government or private sector databases and datasets.
Table of contents
When to use secondary research, types of secondary research, examples of secondary research, advantages and disadvantages of secondary research, other interesting articles, frequently asked questions.
Secondary research is a very common research method, used in lieu of collecting your own primary data. It is often used in research designs or as a way to start your research process if you plan to conduct primary research later on.
Since it is often inexpensive or free to access, secondary research is a low-stakes way to determine if further primary research is needed, as gaps in secondary research are a strong indication that primary research is necessary. For this reason, while secondary research can theoretically be exploratory or explanatory in nature, it is usually explanatory: aiming to explain the causes and consequences of a well-defined problem.
Here's why students love Scribbr's proofreading services
Discover proofreading & editing
Secondary research can take many forms, but the most common types are:
Statistical analysis
Literature reviews, case studies, content analysis.
There is ample data available online from a variety of sources, often in the form of datasets. These datasets are often open-source or downloadable at a low cost, and are ideal for conducting statistical analyses such as hypothesis testing or regression analysis .
Credible sources for existing data include:
- The government
- Government agencies
- Non-governmental organizations
- Educational institutions
- Businesses or consultancies
- Libraries or archives
- Newspapers, academic journals, or magazines
A literature review is a survey of preexisting scholarly sources on your topic. It provides an overview of current knowledge, allowing you to identify relevant themes, debates, and gaps in the research you analyze. You can later apply these to your own work, or use them as a jumping-off point to conduct primary research of your own.
Structured much like a regular academic paper (with a clear introduction, body, and conclusion), a literature review is a great way to evaluate the current state of research and demonstrate your knowledge of the scholarly debates around your topic.
A case study is a detailed study of a specific subject. It is usually qualitative in nature and can focus on a person, group, place, event, organization, or phenomenon. A case study is a great way to utilize existing research to gain concrete, contextual, and in-depth knowledge about your real-world subject.
You can choose to focus on just one complex case, exploring a single subject in great detail, or examine multiple cases if you’d prefer to compare different aspects of your topic. Preexisting interviews , observational studies , or other sources of primary data make for great case studies.
Content analysis is a research method that studies patterns in recorded communication by utilizing existing texts. It can be either quantitative or qualitative in nature, depending on whether you choose to analyze countable or measurable patterns, or more interpretive ones. Content analysis is popular in communication studies, but it is also widely used in historical analysis, anthropology, and psychology to make more semantic qualitative inferences.
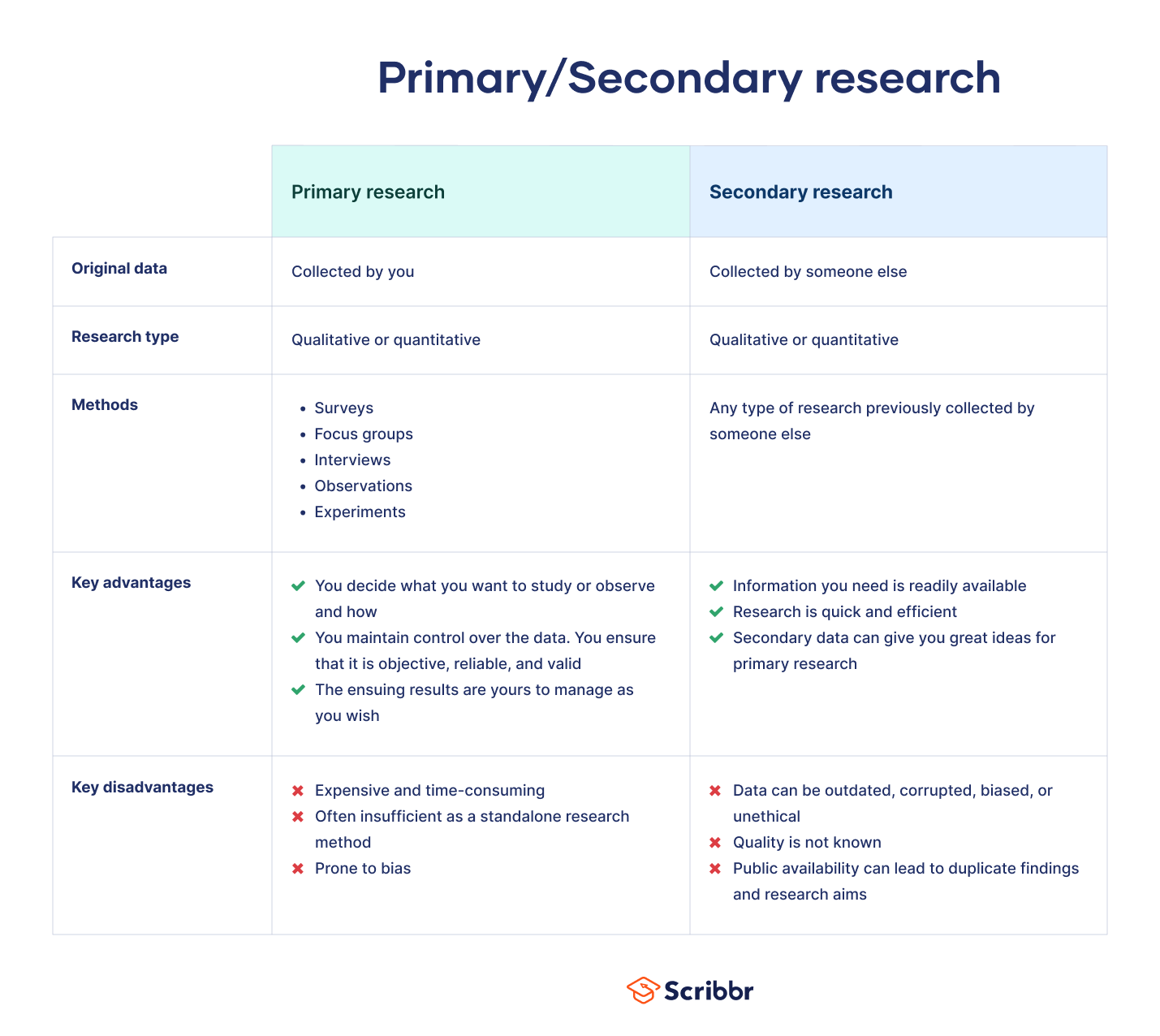
Secondary research is a broad research approach that can be pursued any way you’d like. Here are a few examples of different ways you can use secondary research to explore your research topic .
Secondary research is a very common research approach, but has distinct advantages and disadvantages.
Advantages of secondary research
Advantages include:
- Secondary data is very easy to source and readily available .
- It is also often free or accessible through your educational institution’s library or network, making it much cheaper to conduct than primary research .
- As you are relying on research that already exists, conducting secondary research is much less time consuming than primary research. Since your timeline is so much shorter, your research can be ready to publish sooner.
- Using data from others allows you to show reproducibility and replicability , bolstering prior research and situating your own work within your field.
Disadvantages of secondary research
Disadvantages include:
- Ease of access does not signify credibility . It’s important to be aware that secondary research is not always reliable , and can often be out of date. It’s critical to analyze any data you’re thinking of using prior to getting started, using a method like the CRAAP test .
- Secondary research often relies on primary research already conducted. If this original research is biased in any way, those research biases could creep into the secondary results.
Many researchers using the same secondary research to form similar conclusions can also take away from the uniqueness and reliability of your research. Many datasets become “kitchen-sink” models, where too many variables are added in an attempt to draw increasingly niche conclusions from overused data . Data cleansing may be necessary to test the quality of the research.
Receive feedback on language, structure, and formatting
Professional editors proofread and edit your paper by focusing on:
- Academic style
- Vague sentences
- Style consistency
See an example
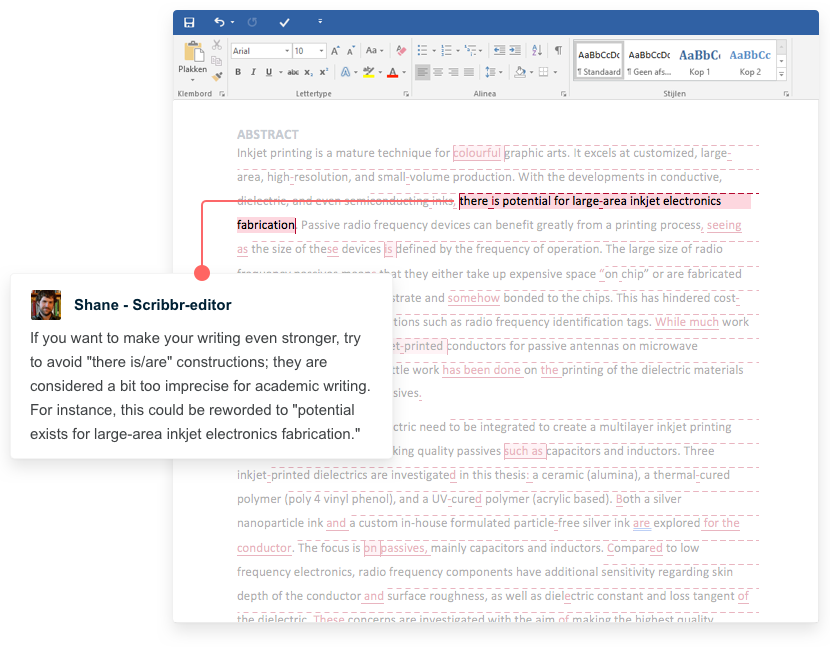
If you want to know more about statistics , methodology , or research bias , make sure to check out some of our other articles with explanations and examples.
- Normal distribution
- Degrees of freedom
- Null hypothesis
- Discourse analysis
- Control groups
- Mixed methods research
- Non-probability sampling
- Quantitative research
- Inclusion and exclusion criteria
Research bias
- Rosenthal effect
- Implicit bias
- Cognitive bias
- Selection bias
- Negativity bias
- Status quo bias
A systematic review is secondary research because it uses existing research. You don’t collect new data yourself.
The research methods you use depend on the type of data you need to answer your research question .
- If you want to measure something or test a hypothesis , use quantitative methods . If you want to explore ideas, thoughts and meanings, use qualitative methods .
- If you want to analyze a large amount of readily-available data, use secondary data. If you want data specific to your purposes with control over how it is generated, collect primary data.
- If you want to establish cause-and-effect relationships between variables , use experimental methods. If you want to understand the characteristics of a research subject, use descriptive methods.
Quantitative research deals with numbers and statistics, while qualitative research deals with words and meanings.
Quantitative methods allow you to systematically measure variables and test hypotheses . Qualitative methods allow you to explore concepts and experiences in more detail.
Sources in this article
We strongly encourage students to use sources in their work. You can cite our article (APA Style) or take a deep dive into the articles below.
George, T. (2024, January 12). What is Secondary Research? | Definition, Types, & Examples. Scribbr. Retrieved July 2, 2024, from https://www.scribbr.com/methodology/secondary-research/
Largan, C., & Morris, T. M. (2019). Qualitative Secondary Research: A Step-By-Step Guide (1st ed.). SAGE Publications Ltd.
Peloquin, D., DiMaio, M., Bierer, B., & Barnes, M. (2020). Disruptive and avoidable: GDPR challenges to secondary research uses of data. European Journal of Human Genetics , 28 (6), 697–705. https://doi.org/10.1038/s41431-020-0596-x
Is this article helpful?
Tegan George
Other students also liked, primary research | definition, types, & examples, how to write a literature review | guide, examples, & templates, what is a case study | definition, examples & methods, get unlimited documents corrected.
✔ Free APA citation check included ✔ Unlimited document corrections ✔ Specialized in correcting academic texts

How To Do Secondary Research or a Literature Review
- Secondary Research
- Literature Review
- Step 1: Develop topic
- Step 2: Develop your search strategy
- Step 3. Document search strategy and organize results
Before You Begin: Develop Search Terms
Where do i search, pubmed tips, psycinfo tips, using search history to formulate advanced searches, what about google scholar, use a citation manager, other places to search.
- Guide License
- Ethics & Integrity
- More Information
Paul V. Galvin Library

email: [email protected]
Chat with us:
Make a research appointment:, search our faq:.
Developing search terms starts with developing a research question. There are many ways to develop a research question, and your assignment may dictate which format to use, but the PICO framework is a very common format used in the health field.
- About PICO Format Information about forming a health-based medical question in the PICO format ( P opulation, I ntervention, C omparison/Control, O utcome)
An example research question using the PICO criteria:
Does group therapy [ I ntervention] lower the number of panic attacks per year [ O utcome] when compared to drug therapy [ C omparison/ C ontrol] for teenagers diagnosed with panic disorders [ P opulation]?
After you establish a question, you can begin developing keywords for the four PICO criteria (or if you're not using PICO, the main themes of the question), including synonyms you can think of. It can help to break this up into a chart, like the example below. In this case, it's best to break up any compound criteria such as "teenagers diagnosed with panic disorders," into distinct concepts like simply "teenagers" and "panic disorder."
(Concept 1A) | teenagers children youth |
(Concept 1B) (two Population concepts for this example question, because it was a compound population) | panic disorder generalized anxiety disorder
|
(Concept 2) | group therapy group counseling |
(Concept 3) | drug therapy pharmaceuticals anxiolytic |
(Concept 4) | panic attack panic |
For a psychology literature review, searching both PsycINFO and PubMed are your best bets. Both of these databases are very comprehensive. There will be some overlap between the two databases and some articles will appear during both searches, but you can filter for duplicates if you use a citation management program like Zotero (see later box about citation management). Searching tips for both databases follow.

How to search PubMed in a systematic way
1. create a saved search for all of the terms that represent a concept.
The most comprehensive way to search PubMed is to create a separate but comprehensive search for each of the terms related to the concepts (step 1), then combining all of those searches in a logical way (step 2). To do this, I would want to make one search string for all the potential terms used for each concept. I'll use the Intervention concept as an example. First, I will search for the first keyword I thought of to describe the concept, "group therapy."
Even though I searched just for "group therapy," the database interpreted my search in a different way. This is due to PubMed's algorithms. You can find the search details on the right side of the page:
Because this is how the database interpreted my search, I'll want to copy and paste that into the upper search box. We'll look at why in a bit.
From here, I would continue like this, searching for each phrase of word that describes my concept separately. When done, go to the Advanced Search to see your history:
As you can see, the simple searches I entered have the same number of results as the more complicated, database code that I copied and pasted. The reason I did that is to preserve the actual details as run by the database. From here, I can combine the detailed searches by applying Boolean logic. Because I want all possible terms that cover the same concept, the searches will be combined with OR. You can do this by clicking on the Add link next to searches 11 and 10, and separating by OR. Click search again and return to the search menu. There, you will have and you have one comprehensive list of all the terms related for one of your concepts (as number 12 below).
Make sure to sign in and save this history to preserve it in case you need to make changes in the future. Click on the number of the search and choose Save to NCBI (if you don't have a free NCBI account, you will need to create one first):
Repeat this step for all of your concepts, combining each separate search into one comprehensive search for that concept and saving it.
2. Combine the concept searches logically
After you have created a comprehensive search for each concept, think about how the searches should be combined. Often, this will be simply combining all the concept searches with AND (so you get the overlap between all the terms), but not always.
For example, some researchers don't include textual searches for an age group as key terms; instead, they rely on the database filters for that. This is up to personal preference but you will likely have slightly different results depending on what you choose.
For my sample question, I'm really researching two separate issues because I want to see both how group therapy works for panic attacks and also how drugs work, so that I can compare them. The reason I wouldn't want to search for ALL the concepts together in my example is that it's unlikely there are many articles explaining my exact issue (in other words, articles that compare the two therapies).
To search for relevant articles about the intervention of group therapy question, I'd want to combine my comprehensive searches made for the Population, Intervention, and the Outcome concepts with AND by using the advanced search:
I click Search and end up with 184 relevant results. You should of course save this final search as well!
3. Modify as needed
If you think of other terms to include in your searches, you should modify each concept search individually and then re-combine them.
Other tips:
- Sign up for a free NCBI account. This will allow you to customize your search criteria, save searches and search history, and organize articles into folders.
- PubMed's search algorithm uses something called Automatic Term Mapping (ATM), which automatically groups phrases and searches different fields for a thorough search. This is useful for simpler searches or when you don't need to document search strategy, but for literature search documentation, it's best to look at the Search Details box, modify the search as needed, and record that as your actual search method.
- use Medical Subject Headings (MeSH) to help find targeted results. You can use MeSH terms and the corresponding subheadings to find targeted results.
- PubMed Search Strategies Blog A good place to start to find starting point strategies on a topic similar to yours.

- In addition to using keywords, take advantage of the Thesaurus, which uses indexed terms. See more info about the index terms on the PsycINFO guide .
- Create an EBSCO account. This is separate from your MyIIT login and allows you to save search history, set up search alerts, and organize research.
- Avoid using quotation marks when searching. By leaving them off, the database will automatically search for slight variations of your keywords, such as plural versions or alternate spellings.
- Using the "Peer Reviewed" filter will limit results to only peer-reviewed journal articles. This will make up the majority of your literature, but if you'd like to also find items like books or conference proceedings, consider leaving that filter off.
- Note that your search results will vary by using Limiters, such as Age or Population Group, will provide you with different results than by using keywords for the same concept.
How to search PsycINFO in a systematic way
Similar to the PubMed strategy above, create a separate but comprehensive search for each of the terms related to the concepts (step 1), then combining all of those searches in a logical way (step 2). To do this, I would want to make one search string for all the potential terms used for each concept. I'll use the Intervention concept as an example. First, I will search for the first keyword I thought of to describe the concept, "group therapy." PsycINFO differs from PubMed because the search you enter is the search you get (except the database will apply slight variants such as British spelling, plurals, etc.). You can choose whether to search all fields or a specific field such as the Title (See the More Tips section if you'd like to use the database subject terms).

Repeat for all of your terms:

When you are done searching for each individual term separately, click on the Search History link underneath the search boxes. From there, first clear any terms left in the boxes above and then combine your searches using the checkboxes and the "Search with OR" link.

This will create a combined search, but unlike PubMed PsycINFO does not list the details of the words used, so it can help to immediately save and rename the search to something more descriptive. To save your searches, click on the search(es) you'd like to save and click on Save Searches/Alerts. You will need to make a separate EBSCO account to do this.

Once you have created separate, comprehensive searches for each concept, then combine them following the same strategy listed above in the PubMed box but using the "Search with AND" box on the search history page.
As explained above, when conducting a comprehensive literature review, it's very important to use a systematic approach. This is especially important when submitting an article for publication, because you're often required to submit the search strategy you used. Instead of combining words/phrases into one search on the home page of the database, it's a good idea to use your Search History instead. This will help you be sure that your search terms are being combined properly and in the right order. See the links below for more background and alternative explanations:
- PubMed Search Strategy For another explanation of searching PubMed, see this tutorial. The tutorial references "systematic reviews," but the search concept is similar to literature reviews.
- Example Search Methodology in an APA Paper Scroll to see a sample search method described in the Methodology section.
- Conducting a winning literature search Helpful article for finding health-based literature

In general, PsycINFO and PubMed are better bets to use than Google Scholar when conducting literature searches, because the search fields and algorithms are much more advancec. PsycINFO and PubMed also employ actual human indexers that review and categorize articles, whereas Google Scholar relies on keyword searching alone, so it's easier to get more complete and relevant results in PsycINFO or PubMed. It's also easier to keep track of your search strategy when using PubMed or PsycINFO.
- Zotero by IIT Galvin Library Last Updated Jul 1, 2024 2504 views this year
Using a citation manager requires a bit of a learning and adjustment period, but has a great payoff. Invest a bit of time to learn how to use one and you will benefit for the rest of your educational/professional career! Citation managers help you capture and organize references that you've found online, including the full text if available, and then help you to draft in-text citations and bibliographies. There are several available, but the library recommends Zotero if you aren't yet using a citation manager, because it is free, open-source, and very easy to use.
If you have a novel topic or one that has not yet been empirically studied extensively via research articles, you may need to supplement with dissertations, theses, or books.

- I-Share Catalog Find books from IIT and other universities here.

- << Previous: Step 3. Document search strategy and organize results
- Next: Ethics & Integrity >>
- Last Updated: Jul 2, 2024 11:55 AM
- URL: https://guides.library.iit.edu/litreview

Peer-Reviewed Literature: Peer-Reviewed Research: Primary vs. Secondary
- Peer-Reviewed Research: Primary vs. Secondary
- Types of Peer Review
- Identifying Peer-Reviewed Research
Peer Reviewed Research
Published literature can be either peer-reviewed or non-peer-reviewed. Official research reports are almost always peer reviewed while a journal's other content is usually not. In the health sciences, official research can be primary, secondary, or even tertiary. It can be an original experiment or investigation (primary), an analysis or evaluation of primary research (secondary), or findings that compile secondary research (tertiary). If you are doing research yourself, then primary or secondary sources can reveal more in-depth information.
Primary Research
Primary research is information presented in its original form without interpretation by other researchers. While it may acknowledge previous studies or sources, it always presents original thinking, reports on discoveries, or new information about a topic.
Health sciences research that is primary includes both experimental trials and observational studies where subjects may be tested for outcomes or investigated to gain relevant insight. Randomized Controlled Trials are the most prominent experimental design because randomized subjects offer the most compelling evidence for the effectiveness of an intervention. See the below graphic and below powerpoint for further information on primary research studies.
- Research Design
Secondary Research
Secondary research is an account of original events or facts. It is secondary to and retrospective of the actual findings from an experiment or trial. These studies may be appraised summaries, reviews, or interpretations of primary sources and often exclude the original researcher(s). In the health sciences, meta-analysis and systematic reviews are the most frequent types of secondary research.
- A meta-analysis is a quantitative method of combining the results of primary research. In analyzing the relevant data and statistical findings from experimental trials or observational studies, it can more accurately calculate effective resolutions regarding certain health topics.
- A systematic review is a summary of research that addresses a focused clinical question in a systematic, reproducible manner. In order to provide the single best estimate of effect in clinical decision making, primary research studies are pooled together and then filtered through an inclusion/exclusion process. The relevant data and findings are then compiled and synthesized to arrive at a more accurate conclusion about a specific health topic. Only peer-reviewed publications are used and analyzed in a methodology which may or may not include a meta-analysis.

- << Previous: Home
- Next: Types of Peer Review >>
- Last Updated: Sep 29, 2023 10:05 AM
- URL: https://ttuhsc.libguides.com/PeerReview

Exploring the Impact of Artificial Intelligence in Teaching and Learning of Science: A Systematic Review of Empirical Research
- Open access
- Published: 27 June 2024
Cite this article
You have full access to this open access article
- Firas Almasri 1 , 2 , 3 , 4
519 Accesses
Explore all metrics
The use of Artificial Intelligence (AI) in education is transforming various dimensions of the education system, such as instructional practices, assessment strategies, and administrative processes. It also plays an active role in the progression of science education. This systematic review attempts to render an inherent understanding of the evidence-based interaction between AI and science education. Specifically, this study offers a consolidated analysis of AI’s impact on students’ learning outcomes, contexts of its adoption, students’ and teachers’ perceptions about its use, and the challenges of its use within science education. The present study followed the PRISMA guidelines to review empirical papers published from 2014 to 2023. In total, 74 records met the eligibility for this systematic study. Previous research provides evidence of AI integration into a variety of fields in physical and natural sciences in many countries across the globe. The results revealed that AI-powered tools are integrated into science education to achieve various pedagogical benefits, including enhancing the learning environment, creating quizzes, assessing students’ work, and predicting their academic performance. The findings from this paper have implications for teachers, educational administrators, and policymakers.
Similar content being viewed by others
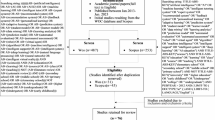
Artificial Intelligence in Science Education (2013–2023): Research Trends in Ten Years
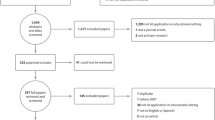
Systematic review of research on artificial intelligence applications in higher education – where are the educators?
Towards a Tripartite Research Agenda: A Scoping Review of Artificial Intelligence in Education Research
Avoid common mistakes on your manuscript.
Introduction
Artificial Intelligence (AI) is a broad field encompassing various technologies that have been developed over the past 50 years to enable machines to perform tasks traditionally requiring human intelligence, such as perceiving, reasoning, learning, and interacting (Ergen, 2019). However, recent advancements in generative AI (GenAI), particularly models like ChatGPT, have brought unprecedented attention to AI’s transformative potential across multiple industries (Hong et al., 2022 ; Lucci et al., 2022 ). Unlike predictive (pre-generative) AI which focuses predictions and decision making through a variety of machine learning and modelling techniques, Generative AI specializes in creating new content, such as text, images, and codes by using models of deep learning (Dai, 2023 ; Tang & Nichols, 2024 ). This distinction is essential to understand the breadth of AI applications in education.
Artificial intelligence in education (AIEd) is an evolving interdisciplinary arena incorporating AI technologies to renovate and enhance teaching and learning environments. Particularly, the application of AI in science teaching and learning is becoming more popular, even as interest in AI’s effects on general education is growing (Chiu et al., 2023 ; Gonzalez et al., 2017 ). More specifically, machine learning, a specific artificial intelligence technology, has been applied to automatically evaluate scientific models used in the education sector. Zhai et al. ( 2022 ) employed machine learning techniques to assess the quality of these models after gathering student responses to activities. Their research demonstrates how artificial intelligence can be used to automate assessment procedures and provide students with timely and detailed feedback on their work in the area of science education (Zhai, C Haudek, Zhai et al., 2020a , b , 2022 ). Similarly, Popenici and Kerr ( 2017 ) conducted a study to investigate the impact of AI on the teaching-learning process in higher education settings. Their study focused on how intelligent technologies are affecting student learning and traditional teaching approaches in education. Their research presents valuable insights into the incorporation of AI within science education contexts.
Zawacki-Richter et al. ( 2019 ), in their systematic assessment of AI applications in higher education, focused on the vital role that teachers can play in this domain. Their results suggest how important it is to explore and understand the needs and perceptions of teachers when integrating these technologies into teaching-learning settings. Likewise, Xu and Ouyang (2022) employed a systematic literature review method to identify and summarize research studies and classify the roles of AI in the educational system. Their findings advocate the use of AI is within the education environment to support its role in three ways: (1) AI as a new subject, (2) AI as an immediate mediator, and AI as a complementary aid to impact the teacher-learner, learner-self, and learner-learner relationships.
Though artificial intelligence has flourished in numerous domains within the education system, a comprehensive analysis of its role, advantages, and challenges in science education must be further explored through empirical investigations. This knowledge gap might prompt teachers, policymakers, and educational administrators to base decisions on patchy as well as limited information, lacking potential opportunities to enhance science teaching and learning with the help of AI. To fill this gap, the present paper provides a systematic review that comprehensively examines and consolidates AI’s impact on science education, evidenced by the empirical publications published from 2014 to 2023. While GenAI represents a significant leap in AI capabilities, this review considers the full spectrum of AI technologies, including both pre-GenAI and GenAI developments. In this way, we attempt to provide a holistic perspective on the current landscape, aiding stakeholders in leveraging AI’s potential while also considering its challenges and ethical implications for educational domains. The overarching objective of this review is to provide insights that could guide future research endeavors and advocate for evidence-based practices to enrich science education through the effective utilization of artificial intelligence.
Research Background
Overview of science education.
The goal of science education is not only to teach scientific knowledge but also to develop a scientifically literate populace capable of engaging in scientific reasoning and decision-making (Almasri, 2021 ; Grinnell, 2021 ). This aligns with the “Science for All” movement, emphasizing the importance of science education for all students, not just those pursuing careers in science (Almasri et al., 2022 ; Mansour, 2009 ). Students’ scientific literacy and critical thinking abilities are developed through the teaching and learning of scientific theories, procedures, and experiments in science education (Alharbi et al., 2022 ; Liu & Pásztor, 2022 ; Mogea, 2022 ; Zulyusri et al., 2023 ).
The nature of science education extends beyond content-based instruction to include student-centered activities and the development of scientific literacy for citizenship (Almasri et al., 2021 ; Irez, 2006a , b ; Kolstø, 2001 ). National development hinges significantly on robust contributions from the scientific community, driving economic growth and propelling the overall advancement of a nation (Hewapathirana & Almasri, 2022 ; Kola, 2013 ). The “Call to Action” for science education highlights an compelling necessity to improve educational approaches and make them consistent with the demands of the 21st century (Holme, 2021 ; Ibáñez & Delgado-Kloos, 2018 ). It is essential for developing students’ foundational knowledge, intriguing their curiosity, and getting them ready for STEM careers as per the contemporary world’s needs. Through AI incorporation, science education can be made more interesting, approachable, and pertinent for students of all ages and backgrounds by emphasizing experiential learning.
Prospects of Incorporating AI in Science Learning
With AI technology’s continuous evolution and popularity, the possibilities for its application in science education are promising but not without challenges. AI has the capability to transform the way science is taught and learned. One of the most compelling applications of AI in science education is its ability to simulate scientific experiments and provide virtual laboratory experiences to science learners. This ensures that students can practice and develop their scientific skills in a safe and controlled environment, potentially saving expenses and offering new opportunities for exploring scientific concepts that may not be feasible in traditional laboratory settings (Wahyono et al., 2019 ). However, these virtual experiences may lack the tactile and hands-on aspects of interaction with the physical world (Tang & Cooper, 2024 ), which are crucial for certain types of learning.
By leveraging AI, educators can also move away from traditional, one-size-fits-all approaches to education and instead provide personalized and interactive learning experiences for students. AI-powered algorithms can go beyond simply providing recommendations and assessments to conducting deep analyses of students’ learning patterns, allowing for highly personalized learning experiences (Zhai et al., 2021 ; Zhai et al., 2020a , b ). However, the effectiveness of these personalized learning systems depends heavily on the quality and representativeness of the data they are trained on, which can sometimes introduce biases and perpetuate existing inequities.
In addition, students can also benefit from immediate feedback and adaptive learning pathways, ensuring that they are able to address any misconceptions or gaps in their understanding of scientific phenomena (Mavroudi et al., 2018 ). AI can also help science educators track and monitor students’ progress more effectively, allowing for targeted interventions and support where necessary. Moreover, the use of AI can enable the development of interactive and immersive learning environments, making science education more engaging and accessible to students with diverse learning styles and needs. As AI continues to advance, the potential for its integration into science learning is likely to grow, presenting exciting opportunities to transform and elevate the science education experiences for students at all levels.
Anticipated Benefits of AI Implementation in Science Teaching
Artificial intelligence (AI) has numerous benefits in science education, profoundly affecting teaching and learning for science subjects. AI programs can study how students learn and change the material to fit each student’s needs, skills, and the way they learn. This way of creating educational material helps students learn better and faster. It lets them go at their own speed and in a way that matches how they like to learn (Zawacki-Richter et al., 2019 ). Also, AI-powered data analysis can help science teachers understand how well their students are doing in specific scientific subjects and where they might need extra help.
Another significant advantage is the improvement of exploratory learning through virtual labs and reenactments. AI-powered instruments have the potential to recreate complex logical tests, which may be illogical or hazardous to conduct in a conventional classroom setting. These virtual situations offer hands-on learning encounters and permit understudies to try distinctive scenarios, improving their understanding of scientific concepts (Ibáñez et al., 2018). This approach was not as supportive of extending understudy engagement but too valuable to democratize access to high-quality science instruction. AI devices can interface understudies and teachers over diverse geographies, empowering the trade of logical thoughts and cultivating a worldwide point of view on logical issues. This interconnecting also permits integrating differing datasets into the educational modules, uncovering understudies to real-world logical challenges and datasets (Holmes et al., 2023 ).
Ethical Considerations of AI Integration in Education
Even though artificial intelligence (AI) in education has bright futures, important ethical issues need to be resolved when integrating AI in the classroom. Many researchers have stressed considering the ethical implications and the need for character education in the era of AI (Burton et al., 2017 ; Cathrin & Wikandaru, 2023 ). The lack of critical reflection on the pedagogical and ethical implications and the risks of implementing AI applications in higher education underscores the need for a comprehensive ethical framework (Bozkurt et al., 2021 ).
Additionally, the integration of AI into educational settings presents new ethical obligations for teachers, necessitating a revaluation of ethical frameworks and responsibilities (Adams et al., 2022 ). Moreover, the introduction of ethics courses in academic training and capacity building of AI development actors can facilitate the integration of ethical values and the development of responsible AI (Kiemde & Kora, 2022 ). The ethical implications of AI in education extend beyond technical considerations to encompass broader societal impacts, such as privacy protection and social justice (Hermansyah et al., 2023 ).
Educators and students must understand, evaluate, and familiarize themselves with the uses of generative AI tools and consider their potential impacts on academic integrity. This involves recognizing when and how AI is used, assessing the reliability and validity of AI-generated outputs, and understanding the ethical and social implications of AI applications (Akgun & Greenhow, 2021 ). Moreover, the application of AI in education brings questions about educational equity and access to the fore. Systemic biases in AI algorithms and data can perpetuate inequities, making it crucial to address these biases effectively (Adams et al., 2022 ).
Purpose of the Study
The primary goal of the present study is to highlight the potential benefits as well as any disparities that might result from the widespread use of AI in science subjects. These discrepancies could be related to gaps in infrastructure, preparedness in the region, or accessibility. Eventually, the present investigation aims to furnish all relevant stakeholders, i.e., educators, learners, policymakers, and curriculum designers—with a deeper understanding of the current interaction between AI and science education.
The following research questions are addressed in this systematic review:
Impact on Learning Outcomes: How do AI tools impact student learning outcomes and engagement in science education?
Contexts of AI Adoption: What are the potential disparities in the uptake of AI tools within science education, considering differences among countries, educational levels, and subject areas?
Student and Teacher Perceptions: What are the perceptions and attitudes of students and educators towards the use of AI tools in science education?
Pedagogical Challenges: What are the identified challenges associated with using AI in science education?
Methodology
The authors worked diligently to explore how artificial intelligence contributes to science education thoroughly. We followed a structured process suggested by the widely used review methodology called Preferred Reporting Items for Systematic Reviews and Meta-Analyses (Page et al., 2021 ). The review approach of the current study comprised various stages – defining the study’s purpose along with specific research questions, formulating a protocol, an extensive literature search, a systematic screening process, extracting pertinent data, and synthesizing the findings. The sections below specifically mention how each of these steps was carried out for this study.
Search Strategy
We accessed a range of prominent digital repositories and databases to search the relevant literature. Particularly, IEEE Xplore, Springer, Tylor and Francis, ERIC (U.S. Dept. of Education), Science Direct, and Wiley were targeted to search the relevant literature. We also used Google Scholar and Google to make sure that we didn’t miss any important information. We used advanced search features to limit our search results to papers published between 2014 and 2023, ensuring that our search was focused and up to date (Piasecki et al., 2018 ).
We utilized a smart search strategy along with a range of search terms and operators to accomplish this. Our search strategy used a combination of key terms such as “artificial intelligence”, “AI”, “generative AI”, “ChatGPT,” “machine learning”, “robotics”, “intelligent system,” and “expert system” paired with descriptors like “science education,” “science learning,” or simply “science”. These combinations, along with their possible variations, were systematically applied to search within the papers’ titles, keywords, and abstracts. This search strategy was created with the aim to identify and consider a broad range of empirical work relating to the use of artificial intelligence in the teaching and learning of science.
Eligibility Criteria
Describing clear eligibility (inclusion and exclusion) criteria allows for setting boundaries for a systematic literature review. These criteria were aimed at creating a structured framework that facilitates the inclusion of studies meeting essential prerequisites while excluding those that don’t align with our research objectives. The inclusion criteria are as follows:
The paper must have employed empirical methods, such as quantitative, qualitative, or/and mixed methods, warranting a rigorous data collection and analysis approach.
The paper should have conducted research in an educational setting, encircling primary, middle, secondary, or higher education, emphasizing the applicability of the findings in educational environments.
A pivotal criterion necessitates the use of artificial intelligence in the study. This AI practice should have been applied to the teaching-learning process, and empirical data collected and integrated into the study.
Studies should be related to a science-related content area, spanning courses like chemistry, physics, biology, engineering, health sciences, or other related disciplines, ensuring applicability to the research topic.
The timeframe specified for publication years, from 2014 to 2023, targets to capture relevant studies within the past decade, ensuring the examination of recent developments in AI-based learning.
Our exclusion criteria were as follows:
Excluding studies that are not empirical in nature, such as theoretical papers, reviews, editorials, or opinion pieces, to maintain the focus on empirical research.
Studies written in languages other than English.
Studies that did not explicitly mention the AI use within a learning context.
Excluding studies that are solely available in abstract form and lack full-length publications.
The Screening Process
In the months of November and December 2023, we went on a thorough hunt for the required information. We started by searching through loads of databases and found 5,121 articles. After getting rid of duplicates, checking publication dates and titles, and looking at abstracts to see if they met the eligibility criteria for the present study, we ended up with 128 articles. From there, we excluded 41 studies because they didn’t really dive into science education. That left us with 87 articles that we pored over super carefully. We made sure they fit our criteria and answered our research questions before diving into them. From the pool of these 61 articles, ten (13) studies were identified as lacking clear empirical evidence regarding the use of artificial intelligence and were subsequently excluded. This process resulted in a final dataset of 74 articles that were included in the systematic review. See Table 1 for the list of studies included in our review. Figure 1 demonstrates a quick preview of the search strategy and the screening process.
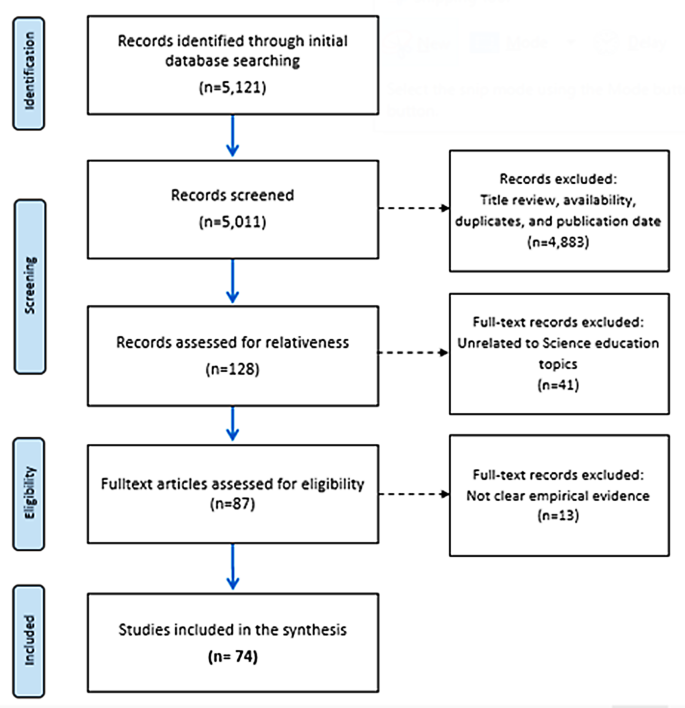
PRISMA review process
Coding and Analysis
We used a mix of qualitative and quantitative content analysis techniques to synthesize the findings of the empirical papers. To ensure inter-rater reliability in relation to the quality of article coding procedures, a small random sample consisting of 20 selected articles was independently coded by multiple raters. The calculated reliability level exceeded 92%, signifying a high degree of agreement across coding categories. We conducted a comprehensive examination of the studies from various perspectives. Firstly, we analyzed the characteristics of the data set, including the country where the studies were conducted, the journal name, the content area, and the educational level.
In this comprehensive review of the literature, we carefully evaluated seventy-four (74) empirical studies that deal with the incorporation of AI into science education. Numerous research approaches, such as mixed, qualitative, and quantitative approaches, were used in these studies. Examining the publication dates of the included papers revealed that they were dispersed over the review study’s 10-year focal period (2014 to 2023). The year 2023, with twenty-seven (27) papers, led the way, demonstrating researchers’ strong interest in the most recent research on the application of artificial intelligence in science education. This was followed by ten (10) studies in 2022, eight (08) studies in 2021, and nine (09) studies in year 2020. For more information on the year-wise publication, see Fig. 2 .
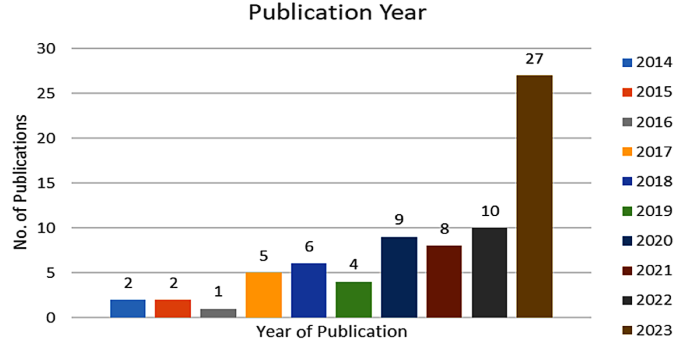
Year-wise publications
The review process of the present study involved the consolidation of findings pertaining to four distinct research questions, each of which is presented separately in the following sections.
RQ1: Impact on Outcomes Comparison
The first research question of the current study specifically addressed the primary intention of this systematic research i.e., analyzing the reported impact of AI-enhanced learning on students’ learning outcomes in science education. The empirical papers reviewed showed that artificial intelligence has been used within science education for a variety of purposes, such as engaging students in the learning process with a strong sense of motivation and interest (Balakrishnan, 2018 ), generating tests of science subjects (Aldabe & Maritxalar, 2014 ; Nasution & Education, 2023 ), scoring and providing personalized feedback on students’ assignments (Azcona et al., 2019 ; Maestrales et al., 2021 ; Mirchi et al., 2020 ), and predicting student performance (Blikstein et al., 2014 ; Buenaño-Fernández et al., 2019 ; Jiao et al., 2022a , b ).
AI-based tools were found to have a positive influence on student’ learning outcomes in science-related courses. The experimental group that was exposed to AI integration in their learning environments exhibited significantly higher scores in their academic tests compared to the control group who experienced traditional learning environments (Alneyadi & Wardat, 2023 ; Koć-Januchta et al., 2020 ). Ledesma and García ( 2017 ) and Lamb et al. ( 2021 ) highlighted AI’s capacity to identify complex concepts and enhance problem-solving skills significantly in subjects (Lamb et al., 2021 ; Ledesma & García, 2017 ). Ferrarelli and Iocchi ( 2021 ), Cochran et al. ( 2023 ), and Figueiredo and Paixão ( 2015 ) showcased how AI is helpful in fostering improved subject understanding and heightened motivation among students, particularly in physics and chemistry (Ferrarelli & Iocchi, 2021 ; Figueiredo et al., 2016 ).
Lee et al. ( 2022 ) argue that AI-based tools such as chatbots can help students become cognitively more active in the learning process(Lee et al., 2022 ). Likewise, Azcona ( 2019 ) suggests that personalized learning facilitated by AI can help reduce the gap between lower- and higher-performing students. Moreover, AI-powered education can empower students to predict their learning outcomes and strategically regulate their learning behavior (Buenaño-Fernández et al., 2019 ).
The effectiveness of different AI models varied across studies. Nguyen et al. ( 2023 ) highlighted the performance disparities among AI models like Google Bard, ChatGPT, and Bing Chat in addressing biology problems for Vietnamese students (Nguyen et al., 2023 ). While chatbots positively influenced online learning experiences, their impact on academic achievement remained variable (Almasri, 2022a ; Deveci Topal et al., 2021 ). In essence, these findings underscore the potential of AI to augment science education by enhancing student understanding, motivation, and engagement. However, they also underscore the importance of addressing challenges related to AI’s adaptability to subject matter and context and the need for continued exploration into AI’s comparative impact on academic achievement vis-à-vis traditional teaching methods in science education. Daher et al. ( 2023 ) pointed to AI’s limitations in comprehending specific subject matter, which could impact its effectiveness in aiding student learning. Cooper ( 2023 ) emphasized the need for educators to critically evaluate and adapt AI-generated resources to suit diverse teaching contexts.

RQ2: Contexts of AI Adoption
In our second research question, we aimed to explore the potential disparities in the uptake of AI tools within science education, considering differences among countries, educational levels, and subject areas. The results disclosed that artificial intelligence has been incorporated in a variety of subject areas within science education, including physical and natural sciences. The studies reviewed were highly dominated by investigations that did not specify any particular domain of science ( n = 15, 20.30%), but they preferred to use “Science” as the subject area in their papers. Next in line, was the subject of Physics with the second-highest number of papers ( n = 10, 13.50%). The list was continued by Biology and Programming with nine ( n = 9, 12.16%) and eight ( n = 8, 10.81%) papers, respectively. The subjects of Mathematics and Engineering occupied about 16% (with 06 papers each) of the total papers. Out of 74 studies, only five (05) studies were conducted to investigate the use of AI for AI education. The subjects of Computers/technology were focused on in four papers. Lastly, only one paper was centered around the use of artificial intelligence in Statistics and Earth Science. Figure 3 provides a summary of the content areas that were the focus of the papers included in our review.
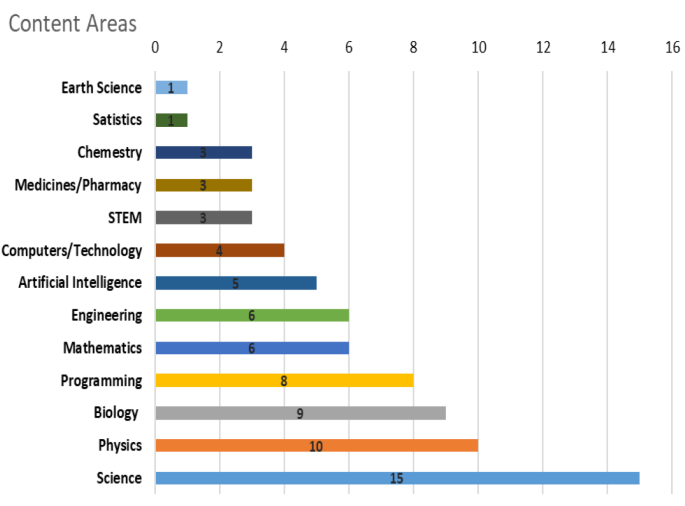
Studies distributed around subject areas within science education
While examining the various educational levels that benefited from the integration of artificial intelligence in some manner, we found that nearly half of the studies ( n = 35, 47%) belonged to undergraduate level, followed by high schools ( n = 15, 20%) and middle schools ( n = 7, 10%) respectively. Out of the total 74 papers, about 8% of the studies ( n = 6) were conducted in secondary school contexts. Likewise, 8% of the studies involved multiple levels of educational settings. In contrast, three of the studies (about 4%) were conducted in elementary school. Only 2% of the papers belonged to the college level, and only one study was conducted at the postgraduate and college levels. Figure 4 provides a quick distribution of the students in various educational contexts.
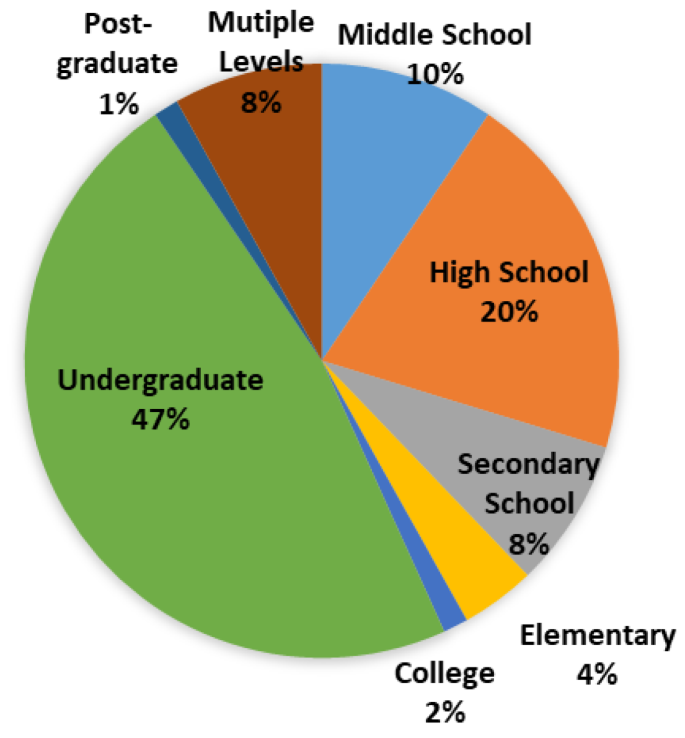
Studies distributed across various educational levels
Similarly, country-wise categorization of the papers exposed that about 38% of the studies ( n = 25) were conducted in the context of the United States. Germany ranked second in the list with six studies (8%). This was followed by four studies (5.4%) carried out in Turkey and Australia. UAE and Malaysia followed in the race, each with three papers. Eight countries, including Sweden, China, Mexico, Saudi Arabia, Spain, the Netherlands, Israel, and Taiwan, contributed about 21.6% of the total papers, each with two studies. The rest of the papers ( n = 10, 13.51%) were written in the context of 10 different countries across the globe (see Fig. 5 for details).
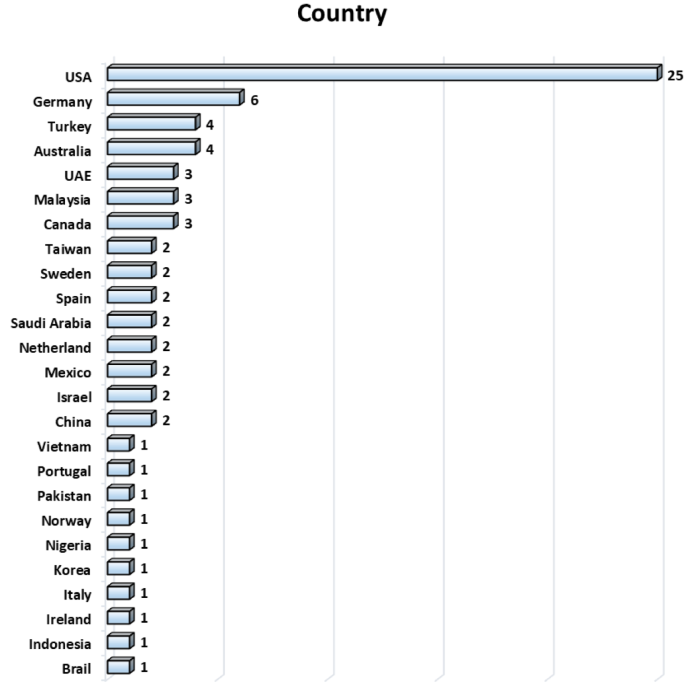
Country of research context
RQ3: Student and Teacher Perceptions
With our third research question, we attempted to explore science teachers’ and students’ perceptions regarding the integration of AI. The studies revealed multifaceted perspectives on the integration of AI in science education among both students and teachers. The effectiveness of AI tools in augmenting learning experiences garnered students’ attention. Students showcased increased engagement and improved subject understanding through AI-based interventions, indicating positive perceptions of AI’s efficacy in enhancing learning outcomes (Ferrarelli & Iocchi, 2021 ; Ledesma & García, 2017 ). For example, Bitzenbauer (2023) found that ChatGPT’s use in Physics classrooms favorably influenced students’ perceptions in Germany. Avelino et al. ( 2017 ) echoed this sentiment for undergraduate students in the United States.
Students reported their increased interest in science courses when AI was integrated into the learning environments. Students particularly admired the AI’s power to provide prediction and personalized feedback (Azcona et al., 2019 ). According to Elkhodr et al. ( 2023 ), science students perceive AI-based tools as useful and enjoyable learning resources, while most students showed a willingness to use them in the future.
Our analysis suggests that science teachers hold a high level of acceptance and positive attitudes toward AI’s utilization in the classroom. Teachers welcome its use with positive correlations to self-efficacy, ease of use, and behavioral intentions (Al Darayseh, 2023 ). They perceive this technology as the need of the hour to boost student engagement (Almasri, 2022b ; Nersa, 2020 ). Empirical papers included in the current study exposed fluctuating degrees of comfort and adaptability among educators and students in incorporating AI into their teaching and learning processes. Al Darayseh ( 2023 ) noted that science teachers exhibited favorable attitudes toward AI’s integration, possibly due to the perceived reduced effort in its utilization and their confidence in their essential skills to incorporate AI effectively.
There are several factors that influence teachers’ intentions and behavior regarding the use of AI, including self-esteem, expected benefits, ease of utilization, and their overall attitude toward AI applications. Teachers’ favorable disposition towards AI use is also due to their perception of reduced effort in its utilization.(Nja et al., 2023 ). Overall, teachers consider AI tools like ChatGPT to be helpful in designing science units, rubrics, and quizzes (Cooper, 2023 ). Yet, challenges associated with AI integration could influence students’ and teachers’ perceptions of AI’s reliability and accuracy in supporting educational goals, posing potential barriers to widespread acceptance and utilization.
RQ4: Pedagogical Challenges
Our analysis uncovered several challenges associated with the integration of AI in terms of complexities and limitations of its use within this particular domain of the education system. One prevalent challenge revolved around AI’s capability to comprehend and effectively address specific subject matter. Daher et al. ( 2023 ) highlighted instances where AI, like ChatGPT, encountered difficulties in understanding complex concepts in chemistry. They argue that the information provided by AI tools such as ChatGPT is limited because it depends on the data it was taught with. It might not have access to the latest or most complete knowledge in a particular domain.
Adaptability and contextual relevance emerged as significant concerns regarding the use of AI within science teaching. Cooper ( 2023 ) stressed that teachers critically evaluate AI-based resources and adapt them to their teaching contexts. He suggested that a one-size-fits-all approach might not suffice in accommodating the intricacies of varied educational environments. Another challenge pertained to the effectiveness and performance variability of different AI models. Nguyen et al. ( 2023 ) showcased the varying performance levels of different AI models, indicating disparities in their ability to address specific subject-related challenges. This variability in performance, as seen in different studies, implies the need for thorough evaluation and selection of appropriate AI tools tailored to the needs of specific subject areas. Furthermore, ethical considerations and limitations in AI’s current capabilities were notable concerns. Kieser et al. ( 2023 ) raised ethical issues regarding students using AI to fabricate data for class assignments. Addressing these challenges requires a nuanced approach that acknowledges the potential and constraints of AI while striving to optimize its role in enhancing science education effectively.
Discussions
The primary objective of this review was to investigate the interaction between artificial intelligence and science education. Our study uncovered a diverse landscape of AI usage within science education. Our results suggested that integrating AI tools in science education consistently improves students’ academic performance. This was evident in higher test scores and a better understanding of complex concepts compared to those in traditional learning environments (Alneyadi & Wardat, 2023 ; Koć-Januchta et al., 2020 ; Siddaway et al., 2019 ).
Literature suggests that integrating artificial intelligence into the teaching-learning process facilitates understanding complex scientific topics (Lamb et al., 2021 ; Ledesma & García, 2017 ). It also helps develop problem-solving skills considerably, leading to a better understanding of subjects, particularly in fields like physics and chemistry. Furthermore, it was revealed that science teachers use AI-driven tools to engage students effectively and foster their motivation and interest in science-related subjects (Balakrishnan, 2018 ). Personalized learning through AI tools helps bridge performance gaps between lower and higher-performing students (Azcona et al., 2019 ), contributing to a more equitable learning environment. AI-generated personalized feedback also contributed to students’ increased engagement in the learning process (Azcona et al., 2019 ; Maestrales et al., 2021 ; Mirchi et al., 2020 ).
The current systematic review suggests that the distribution of studies within various subject areas in science education showcases a dominant focus on science in general, followed by physics, biology, programming, and other specific science subjects. Some specific domains, like earth science and statistics, received comparatively the least attention in the reviewed literature.
The distribution of research papers across countries demonstrates certain disparities. The United States had a significantly higher number of studies compared to other nations. Germany ranked second on the list. Turkey and Australia followed, while UAE, Malaysia, and Canada contributed with a moderate number of studies. Several countries had minimal representation, with a diverse spread across multiple nations. Concentration of studies in certain countries like the United States and Germany might suggest varying levels of research infrastructure or prioritization of AI in education compared to other nations with fewer studies. This could potentially lead to disparities in the implementation and impact of AI tools in science education among different regions globally.
Our analysis found that students exhibit increased engagement and interest in science courses when AI tools are integrated into learning environments. This heightened interest is attributed to AI’s ability to provide predictions and personalized feedback (Jiao et al., 2022b ), making learning more engaging and enjoyable (Hewapathirana & Almasri, 2022 ). Students perceive AI-based tools as useful and beneficial for their learning experiences. They acknowledge AI’s effectiveness in improving subject understanding and express a willingness to continue using such tools in the future (Elkhodr et al., 2023 ).
Similar to students, science teachers also demonstrate positive attitudes and acceptance of AI tools in the classroom, correlating with perceived benefits in student engagement and their own teaching efficacy. Teachers view AI integration as a means to enhance student engagement, with some perceiving it as a way to reduce effort while teaching, leading to increased confidence in utilizing AI effectively (Al Darayseh, 2023 ). Specifically, teachers perceive ChatGPT a valuable resource for designing science units, rubrics, quizzes, and teaching aids, offering convenience and potential enhancement to their teaching methodologies.
While AI showed promise in improving learning outcomes, there are challenges related to its adaptability to subject matter and context. Some studies pointed out limitations in comprehending specific subjects, potentially impacting the effectiveness of AI in aiding student learning. Previous research suggests that AI tools like ChatGPT face difficulties in comprehending and addressing complex concepts in specific subject areas, as seen in instances within chemistry (Daher et al., 2023 ). The dependency on the data it was trained with limits its access to the latest or most comprehensive knowledge in particular domains. A uniform approach might not adequately cater to the complexities and nuances of varied educational environments, emphasizing the need for adaptable solutions (Cooper, 2023 ). Addressing these challenges requires a balanced approach that acknowledges AI’s potential and constraints in science education. Thus, teachers are advised to critically evaluate AI-generated resources and tailor them to diverse teaching contexts.
Our research provides important implications for teacher preparation and in-service professional development regarding AI in our society and implementing AI tools and processes in K-12 education (Antonenko & Abramowitz, 2023 ). As a whole, integrating artificial intelligence positively enhances the process and outcome of science education. However, there are certain limitations and challenges associated with its use. Providing training and support to educators to effectively utilize AI tools can enhance their confidence and capabilities in integrating these technologies into teaching practices. Moreover, establishing clear ethical guidelines and frameworks for the responsible use of AI in education can mitigate the risk of misuse and ensure ethical practices among students and educators.
Limitations
Some of the inherent limitations of this research review are discussed in this section. First, just like with other reviews, the search terms and strategies determine which research papers are included. Although a thorough and methodologically rigorous search was the goal, using different search terms might have turned up more articles that could have been included in the review. Furthermore, a few particular research databases were searched in order to find pertinent empirical literature for inclusion in this research review. An alternative methodological strategy would have involved restricting the search for research to a predetermined list of scholarly, peer-reviewed journals. A smaller sample of literature for inclusion may have occurred due to this strategy. However, greater control over validity, reliability, and credibility during the search and inclusion processes was sought to the best level. Lastly, we may have missed some grey literature, such as dissertations and conference proceedings, that was not indexed in the databases/repositories that we used.
This systematic review examined the impact, perceptions, and challenges associated with the integration of Artificial Intelligence (AI) in the teaching and learning of science. Our analysis uncovered a landscape rich in prospective benefits and challenges. The usage of AI in science education steadily established positive impacts on student learning outcomes. It encourages participation in the educational process, enhances comprehension of the subject, and boosts motivation in the students. Both students and teachers showed positive views of AI’s effectiveness and ease of use. Both acknowledged its potential to boost learning experiences. Nevertheless, issues arose from AI’s limited ability to understand particular subject matter, its inability to adjust to various educational contexts, and the variation in performance between various AI models. Ethical considerations regarding responsible use also appeared to be an important concern. Addressing these challenges demands a careful approach that considers thorough evaluation and adaptation to diverse contexts. Educators and policymakers should navigate these complexities to join the potential of AI in science education while ensuring ethical practices and maximizing its impact on students’ learning journey worldwide.
Adams, C., Pente, P., Lemermeyer, G., Turville, J., & Rockwell, G. (2022). Artificial intelligence and teachers’ new ethical obligations. The International Review of Information Ethics , 31 (1). https://doi.org/10.29173/irie483 .
Akgun, S., & Greenhow, C. (2021). Artificial intelligence in education: Addressing ethical challenges in K-12 settings. AI and Ethics , 2 , 431–440. https://doi.org/10.1007/s43681-021-00096-7 .
Article Google Scholar
Aldabe, I., & Maritxalar, M. (2014). Semantic similarity measures for the generation of science tests in basque. IEEE Transactions on Learning Technologies , 7 (4), 375–387. https://doi.org/10.1109/TLT.2014.2355831 .
Al Darayseh, A. (2023). Acceptance of artificial intelligence in teaching science: Science teachers’ perspective. Artificial Intelligence , 4 , 100132. https://doi.org/10.1016/j.caeai.2023.100132 .
Alharbi, S. M., Elfeky, A. I., & Ahmed, E. S. (2022). The effect of e-collaborative learning environment on development of critical thinking and higher order thinking skills. Journal of Positive School Psychology , 6 (6), 6848–6854.
Google Scholar
Almasri, F. (2021). Collaborative learning in science education: effects of student’s gender attitudes and achievement in science education University of Warwick, Retrieved from http://webcat.warwick.ac.uk/record=b3853208 .
Almasri, F. (2022a). The impact of e-learning, gender-groupings and learning pedagogies in biology undergraduate female and male students’ attitudes and achievement. Education and Information Technologies , 27 , 8329–8380. https://doi.org/10.1007/s10639-022-10967-z .
Almasri, F. (2022b). Simulations to teach science subjects: Connections among students’ engagement, self-confidence, satisfaction, and learning styles. Education and Information Technologies , 27 (5), 7161–7181. https://doi.org/10.1007/s10639-022-10940-w .
Almasri, F., Hewapathirana, G. I., Alhashem, F., Daniel, C. E., & Lee, N. (2022). The effect of gender composition and pedagogical approach on major and non-major undergraduates biology students’ achievement. Interactive Learning Environments , 1–33. https://doi.org/10.1080/10494820.2022.2066138 .
Almasri, F., Hewapathirana, G. I., Ghaddar, F., Lee, N., & Ibrahim, B. (2021). Measuring attitudes towards biology major and non-major: Effect of students’ gender, group composition, and learning environment. Plos One , 16 (5), e0251453. https://doi.org/10.1371/journal.pone.0251453 .
Alneyadi, S., & Wardat, Y. J. C. E. T. (2023). ChatGPT: Revolutionizing student achievement in the electronic magnetism unit for eleventh-grade students in Emirates schools. Contemporary Educational Technology , 15 (4), ep448. https://doi.org/10.30935/cedtech/13417 .
Antonenko, P., & Abramowitz, B. (2023). In-service teachers’(mis) conceptions of artificial intelligence in K-12 science education. Journal of Research on Technology in Education , 55 (1), 64–78. https://doi.org/10.1080/15391523.2022.2119450 .
Avelino, F., Wittmayer, J. M., Kemp, R., & Haxeltine, A. (2017). Game-changers and transformative social innovation. Ecology and Society, 22 (4). https://www.jstor.org/stable/26798984
Azcona, D., Hsiao, I. H., & Smeaton, A. F. (2019). Detecting students-at-risk in computer programming classes with learning analytics from students’ digital footprints. User Modeling and User-Adapted Interaction , 29 , 759–788. https://doi.org/10.1007/s11257-019-09234-7 .
Balakrishnan, B. (2018). Motivating engineering students learning via monitoring in personalized learning environment with tagging system. Computer Applications in Engineering Education , 26 (3), 700–710. https://doi.org/10.1002/cae.21924 .
Blikstein, P., Worsley, M., Piech, C., Sahami, M., Cooper, S., & Koller, D. (2014). Programming pluralism: Using learning analytics to detect patterns in the learning of computer programming. Journal of the Learning Sciences , 23 (4), 561–599. https://doi.org/10.1080/10508406.2014.954750 .
Bozkurt, A., Karadeniz, A., Baneres, D., Rodríguez, M. E., & Rodríguez, M. E. (2021). Artificial intelligence and reflections from educational landscape: A review of AI studies in half a century. Sustainability , 13 (2), 800. https://doi.org/10.3390/su13020800 .
Buenaño-Fernández, D., Gil, D., & Luján-Mora, S. (2019). Application of machine learning in predicting performance for computer engineering students: A case study. Sustainability , 11 (10), 2833. https://doi.org/10.3390/su11102833 .
Burton, E., Goldsmith, J., Koenig, S., Kuipers, B., Mattei, N., & Walsh, T. (2017). Ethical considerations in artificial intelligence courses. AI Magazine , 38 (2), 22–34. https://doi.org/10.1609/aimag.v38i2.2731 .
Cathrin, S., & Wikandaru, R. (2023). The future of character education in the era of artificial intelligence. Humanika Kajian Ilmiah Mata Kuliah Umum , 23 (1). https://doi.org/10.21831/hum.v23i1.59741 .
Chiu, T. K., Xia, Q., Zhou, X., Chai, C. S., & Cheng, M. (2023). Systematic literature review on opportunities, challenges, and future research recommendations of artificial intelligence in education. Computers and Education: Artificial Intelligence , 4 , 100118. https://doi.org/10.1016/j.caeai.2022.100118 .
Cochran, K., Cohn, C., Rouet, J. F., & Hastings, P. (2023, June). Improving automated evaluation of student text responses using gpt-3.5 for text data augmentation. In International conference on artificial intelligence in education (pp. 217–228). Springer Nature Switzerland. https://doi.org/10.1007/978-3-031-36272-9_18
Cooper, G. (2023). Examining science education in chatgpt: An exploratory study of generative artificial intelligence. Journal of Science Education and Technology , 32 (3), 444–452. https://doi.org/10.1007/s10956-023-10039-y .
Daher, W., Diab, H., & Rayan, A. (2023). Artificial intelligence generative tools and conceptual knowledge in problem solving in Chemistry. Information , 14 (7), 409. https://doi.org/10.3390/info14070409 .
Dai, Y. (2023). Negotiation of epistemological understandings and teaching practices between primary teachers and scientists about artificial intelligence in professional development. Research in Science Education , 53 (3), 577–591. https://doi.org/10.1007/s11165-022-10072-8 .
Deveci Topal, A., Eren, D., C., & Kolburan Geçer, A. (2021). Chatbot application in a 5th grade science course. Education and Information Technologies , 26 (5), 6241–6265. https://doi.org/10.1007/s10639-021-10627-8 .
Elkhodr, M., Gide, E., Wu, R., & Darwish, O. (2023). ICT students’ perceptions towards ChatGPT: An experimental reflective lab analysis. STEM Education , 3 (2), 70–88. https://doi.org/10.3934/steme.2023006 .
Ferrarelli, P., & Iocchi, L. (2021). Learning newtonian physics through programming robot experiments. Technology Knowledge and Learning , 26 (4), 789–824. https://doi.org/10.1007/s10758-021-09508-3
Figueiredo, M., Esteves, L., Neves, J., & Vicente, H. (2016). A data mining approach to study the impact of the methodology followed in chemistry lab classes on the weight attributed by the students to the lab work on learning and motivation. Chemistry Education Research and Practice , 17 (1), 156–171. https://doi.org/10.1039/C5RP00144G .
Figueiredo, M., & Paixão, F. (2015). O Papel da Observação na Compreensão da Natureza do Conhecimento Científico: Uma Proposta Didática Baseada nas Reações de Combustão. In Proceedings do Encontro Nacional de Educação em Ciências – ENEC 2015 - Ciência com Cultura (pp. 205–209). Lisboa.
Gonzalez, A. J., Hollister, J. R., DeMara, R. F., Leigh, J., Lanman, B., Lee, S. Y., & Wilder, B. (2017). AI in informal science education: Bringing turing back to life to perform the turing test. International Journal of Artificial Intelligence in Education , 27 , 353–384. https://doi.org/10.1007/s40593-017-0144-1 .
Grinnell, F. (2021). Scientific Inquiry, pluralism and complementarity. Scientific Inquiry Pluralism and Complementarity . https://doi.org/10.31235/osf.io/gejwv .
Hermansyah, M., Najib, A., Farida, A., Sacipto, R., & Rintyarna, B. S. (2023). Artificial intelligence and ethics: Building an artificial intelligence system that ensures privacy and social justice. International Journal of Science and Society , 5 (1), 154–168. https://doi.org/10.54783/ijsoc.v5i1.644 .
Hewapathirana, G., & Almasri, F. (2022). Active learning compared with lecture-based pedagogies in gender and socio-cultural context-specific major and non-major biology classes. Handbook of research on active Learning and Student Engagement in Higher Education (pp. 293–319). IGI Global.
Holmes, W., Bialik, M., & Fadel, C. (2023). Artificial intelligence in education . Globethics.
Holme, T. A. (2021). Considering the call to action for science education (Vol. 98, pp. 2739–2740). ACS.
Hong, F., Dou, W., & Chen, S. (2022). Research on the impact of artificial intelligence on government public service quality . Paper presented at the 2022 2nd International Conference on Public Management and Intelligent Society (PMIS 2022).
Ibáñez, M. B., & Delgado-Kloos, C. (2018). Augmented reality for STEM learning: A systematic review. Computers & Education , 123 , 109–123. https://doi.org/10.1016/j.compedu.2018.05.002 .
Irez, S. (2006a). Are we prepared? An assessment of preservice science teacher educators’ beliefs about nature of science. Science Education , 90 (6), 1113–1143. https://doi.org/10.1002/sce.20156 .
Irez, S. J. S. E. (2006b). Are we prepared? An assessment of preservice science teacher educators’ beliefs about nature of science. 90 (6), 1113–1143.
Jiao, P., Ouyang, F., Zhang, Q., & Alavi, A. H. (2022a). Artificial intelligence-enabled prediction model of student academic performance in online engineering education. Artificial Intelligence Review , 55 (8), 6321–6344. https://doi.org/10.1007/s10462-022-10155-y .
Jiao, P., Ouyang, F., Zhang, Q., & Alavi, A. H. (2022b). Artificial intelligence-enabled prediction model of student academic performance in online engineering education. Artificial Intelligence Review , 55 (8), 6321–6344.
Kiemde, S. M. A., & Kora, A. D. (2022). Towards an ethics of AI in Africa: Rule of education. AI and Ethics , 2 (1), 35–40. https://doi.org/10.1007/s43681-021-00106-8 .
Kieser, F., Wulff, P., Kuhn, J., & Küchemann, S. (2023). Educational data augmentation in physics education research using ChatGPT. Physical Review Physics Education Research, 19 (2), 020150.
Koć-Januchta, M. M., Schönborn, K. J., Tibell, L. A., Chaudhri, V. K., & Heller, H. C. (2020). Engaging with biology by asking questions: Investigating students’ interaction and learning with an artificial intelligence-enriched textbook. Journal of Educational Computing Research , 58 (6), 1190–1224.
Kok-Sing, T., & Nichols, K. (Eds.). (2024). Collection: Artificial intelligence in science education. Research in Science Education . https://link.springer.com/collections/dbihehajcd .
Kola, A. J. (2013). Importance of science education to national development and problems militating against its development. American Journal of Educational Research , 1 (7), 225–229. https://doi.org/10.12691/education-1-7-2 .
Kolstø, S. D. (2001). Scientific literacy for citizenship: Tools for dealing with the science dimension of controversial socioscientific issues. Science Education , 85 (3), 291–310. https://doi.org/10.1002/sce.1011 .
Lamb, R., Hand, B., & Kavner, A. (2021). Computational modeling of the effects of the science writing heuristic on student critical thinking in science using machine learning. Journal of Science Education and Technology , 30 , 283–297. https://doi.org/10.1007/s10956-020-09871-3 .
Ledesma, E. F. R., & García, J. J. G. (2017). Selection of mathematical problems in accordance with student’s learning style. International Journal of Advanced Computer Science Applications , 8 (3). https://doi.org/10.14569/IJACSA.2017.080316 .
Lee, H. S., Pallant, A., Pryputniewicz, S., Lord, T., Mulholland, M., & Liu, O. L. (2019). Automated text scoring and real-time adjustable feedback: Supporting revision of scientific arguments involving uncertainty. Science Education , 103 (3), 590–622.
Lee, Y. F., Hwang, G. J., & Chen, P. Y. (2022). Impacts of an AI-based cha bot on college students’ after-class review, academic performance, self-efficacy, learning attitude, and motivation. Educational Technology Research and Development , 70 (5), 1843–1865. https://doi.org/10.1007/s11423-022-10142-8 .
Liu, Y., & Pásztor, A. (2022). Effects of problem-based learning instructional intervention on critical thinking in higher education: A meta-analysis. Thinking Skills and Creativity , 45 , 101069. https://doi.org/10.1016/j.tsc.2022.101069 .
Lucci, S., Kopec, D., & Musa, S. M. (2022). Artificial intelligence in the 21st century: Mercury learning and information .
Maestrales, S., Zhai, X., Touitou, I., Baker, Q., Schneider, B., & Krajcik, J. (2021). Using machine learning to score multi-dimensional assessments of chemistry and physics. Journal of Science Education and Technology , 30 , 239–254. https://doi.org/10.1007/s10956-020-09895-9 .
Mansour, N. (2009). Science-technology-society (STS) a new paradigm in science education. Bulletin of Science Technology & Society , 29 (4), 287–297. https://doi.org/10.1177/0270467609336307 .
Mavroudi, A., Giannakos, M., & Krogstie, J. (2018). Supporting adaptive learning pathways through the use of learning analytics: Developments, challenges and future opportunities. Interactive Learning Environments, 26 (2), 206–220. https://doi.org/10.1080/10494820.2017.1292531
Mirchi, N., Bissonnette, V., Yilmaz, R., Ledwos, N., Winkler-Schwartz, A., & Del Maestro, R. F. J. P. (2020). The virtual operative assistant: An explainable artificial intelligence tool for simulation-based training in surgery and medicine. Plos One , 15 (2), e0229596. https://doi.org/10.1371/journal.pone.0229596 .
Mogea, T. (2022). Students’ critical thinking ability in English teaching and learning. Jurnal Pendidikan Dan Sastra Inggris , 2 (3), 157–171.
Nasution & Education. (2023). Using artificial intelligence to create biology multiple choice questions for higher education. Agricultural and Environmental Education , 2 (1).
Nersa, A. (2020). The coverage of children Rights in Amhara television children’s program
Nguyen, P., Trương, H., Nguyen, P., Bruneau, P., Cao, L., & Wang, J. (2023). Evaluation of Google Bard on Vietnamese High School Biology Examination.
Nja, C. O., Idiege, K. J., Uwe, U. E., Meremikwu, A. N., Ekon, E. E., Erim, C. M., & Umalili, B. (2023). Adoption of artificial intelligence in science teaching: From the vantage point of the African science teachers. Smart Learning Environments , 10 (1), 42. https://doi.org/10.1186/s40561-023-00261-x .
Page, M. J., McKenzie, J. E., Bossuyt, P. M., Boutron, I., Hoffmann, T. C., Mulrow, C. D., & Brennan, S. E. (2021). The PRISMA 2020 statement: An updated guideline for reporting systematic reviews. International Journal of Surgery , 88 , 105906. https://doi.org/10.1136/bmj.n71 .
Piasecki, J., Waligora, M., & Dranseika, V. (2018). Google search as an additional source in systematic reviews. Science and Engineering Ethics , 24 , 809–810.
Popenici, S. A., & Kerr, S. (2017). Exploring the impact of artificial intelligence on teaching and learning in higher education. Research and Practice in Technology Enhanced Learning , 12 (1), 1–13. https://doi.org/10.1186/s41039-017-0062-8 .
Siddaway, A. P., Wood, A. M., & Hedges, L. V. (2019). How to do a systematic review: A best practice guide for conducting and reporting narrative reviews, meta-analyses, and meta-syntheses. Annual Review of Psychology , 70 , 747–770. https://doi.org/10.1146/annurev-psych-010418-102803 .
Tang, K. S., & Cooper, G. (2024). The role of materiality in an era of generative artificial intelligence. Science & Education , 1–16. https://doi.org/10.1007/s11191-024-00508-0 .
Wahyono, I. D., Fadlika, I., Asfani, K., Putranto, H., Hammad, J., & Sunarti. (2019). New adaptive intelligence method for personalized adaptive laboratories. In 2019 International conference on electrical, electronics and information engineering (ICEEIE) (pp. 196–200). https://doi.org/10.1109/ICEEIE47180.2019.8981477
Zawacki-Richter, O., Marín, V. I., Bond, M., & Gouverneur, F. (2019). Systematic review of research on artificial intelligence applications in higher education–where are the educators? Journal of Educational Technology in Higher Education , 16 (1), 1–27. https://doi.org/10.1186/s41239-019-0171-0 .
Zhai, X., Haudek, C., Shi, K., Nehm, L. H., R., & Urban-Lurain, M. (2020a). From substitution to redefinition: A framework of machine learning‐based science assessment. Journal of Research in Science Teaching , 57 (9), 1430–1459. https://doi.org/10.1002/tea.21658 .
Zhai, X., He, P., & Krajcik, J. (2022). Applying machine learning to automatically assess scientific models. Journal of Research in Science Teaching , 59 (10), 1765–1794. https://doi.org/10.1002/tea.21773 .
Zhai, X., Shi, L., & Nehm, R. H. (2021). A Meta-analysis of machine learning-based Science assessments: Factors impacting machine-human score agreements. Journal of Science Education and Technology , 30 (3), 361–379. https://doi.org/10.1007/s10956-020-09875-z .
Zhai, X., Yin, Y., Pellegrino, J. W., Haudek, K. C., & Shi, L. (2020b). Applying machine learning in science assessment: A systematic review. Studies in Science Education , 56 (1), 111–151. https://doi.org/10.1080/03057267.2020.1735757 .
Zulyusri, Z., Elfira, I., Lufri, L., & Santosa, T. A. (2023). Literature study: Utilization of the PjBL model in science education to improve creativity and critical thinking skills. Jurnal Penelitian Pendidikan IPA , 9 (1), 133–143. https://doi.org/10.29303/jppipa.v9i1.2555 .
Download references
Acknowledgements
Author information, authors and affiliations.
College of Engineering and Sciences, International University of Science and Technology in Kuwait, Ardiya, Kuwait
Firas Almasri
Department of Physics, Toronto Metropolitan University, Toronto, ON, M5B 2K3, Canada
Centre for Education Studies, University of Warwick, Coventry, United Kingdom
Institute for Biomedical Engineering, Science and Technology (iBEST), A Partnership Between Toronto Metropolitan University and St. Michael’s Hospital, Toronto, ON, M5B 1T8, Canada
You can also search for this author in PubMed Google Scholar
Corresponding author
Correspondence to Firas Almasri .
Ethics declarations
Consent to publish.
I consent to publish identifiable details within the text in the Research in Science Education Journal.
Conflict of Interest
The authors reported no potential conflict of interest.
Additional information
Publisher’s note.
Springer Nature remains neutral with regard to jurisdictional claims in published maps and institutional affiliations.
Rights and permissions
Open Access This article is licensed under a Creative Commons Attribution 4.0 International License, which permits use, sharing, adaptation, distribution and reproduction in any medium or format, as long as you give appropriate credit to the original author(s) and the source, provide a link to the Creative Commons licence, and indicate if changes were made. The images or other third party material in this article are included in the article’s Creative Commons licence, unless indicated otherwise in a credit line to the material. If material is not included in the article’s Creative Commons licence and your intended use is not permitted by statutory regulation or exceeds the permitted use, you will need to obtain permission directly from the copyright holder. To view a copy of this licence, visit http://creativecommons.org/licenses/by/4.0/ .
Reprints and permissions
About this article
Almasri, F. Exploring the Impact of Artificial Intelligence in Teaching and Learning of Science: A Systematic Review of Empirical Research. Res Sci Educ (2024). https://doi.org/10.1007/s11165-024-10176-3
Download citation
Accepted : 07 June 2024
Published : 27 June 2024
DOI : https://doi.org/10.1007/s11165-024-10176-3
Share this article
Anyone you share the following link with will be able to read this content:
Sorry, a shareable link is not currently available for this article.
Provided by the Springer Nature SharedIt content-sharing initiative
- Artificial intelligence
- Science education
- Engineering students
- STEM learning
- Science learning
- Find a journal
- Publish with us
- Track your research

- Duke NetID Login
- 919.660.1100
- Duke Health Badge: 24-hour access
- Accounts & Access
- Databases, Journals & Books
- Request & Reserve
- Training & Consulting
- Request Articles & Books
- Renew Online
- Reserve Spaces
- Reserve a Locker
- Study & Meeting Rooms
- Course Reserves
- Pay Fines/Fees
- Recommend a Purchase
- Access From Off Campus
- Building Access
- Computers & Equipment
- Wifi Access
- My Accounts
- Mobile Apps
- Known Access Issues
- Report an Access Issue
- All Databases
- Article Databases
- Basic Sciences
- Clinical Sciences
- Dissertations & Theses
- Drugs, Chemicals & Toxicology
- Grants & Funding
- Interprofessional Education
- Non-Medical Databases
- Search for E-Journals
- Search for Print & E-Journals
- Search for E-Books
- Search for Print & E-Books
- E-Book Collections
- Biostatistics
- Global Health
- MBS Program
- Medical Students
- MMCi Program
- Occupational Therapy
- Path Asst Program
- Physical Therapy
- Researchers
- Community Partners
Conducting Research
- Archival & Historical Research
- Black History at Duke Health
- Data Analytics & Viz Software
- Data: Find and Share
- Evidence-Based Practice
- NIH Public Access Policy Compliance
- Publication Metrics
- Qualitative Research
- Searching Animal Alternatives
Systematic Reviews
- Test Instruments
Using Databases
- JCR Impact Factors
- Web of Science
Finding & Accessing
- COVID-19: Core Clinical Resources
- Health Literacy
- Health Statistics & Data
- Library Orientation
Writing & Citing
- Creating Links
- Getting Published
- Reference Mgmt
- Scientific Writing
Meet a Librarian
- Request a Consultation
- Find Your Liaisons
- Register for a Class
- Request a Class
- Self-Paced Learning
Search Services
- Literature Search
- Systematic Review
- Animal Alternatives (IACUC)
- Research Impact
Citation Mgmt
- Other Software
Scholarly Communications
- About Scholarly Communications
- Publish Your Work
- Measure Your Research Impact
- Engage in Open Science
- Libraries and Publishers
- Directions & Maps
- Floor Plans
Library Updates
- Annual Snapshot
- Conference Presentations
- Contact Information
- Gifts & Donations
- What is a Systematic Review?
Types of Reviews
- Manuals and Reporting Guidelines
- Our Service
- 1. Assemble Your Team
- 2. Develop a Research Question
- 3. Write and Register a Protocol
- 4. Search the Evidence
- 5. Screen Results
- 6. Assess for Quality and Bias
- 7. Extract the Data
- 8. Write the Review
- Additional Resources
- Finding Full-Text Articles
Review Typologies
There are many types of evidence synthesis projects, including systematic reviews as well as others. The selection of review type is wholly dependent on the research question. Not all research questions are well-suited for systematic reviews.
- Review Typologies (from LITR-EX) This site explores different review methodologies such as, systematic, scoping, realist, narrative, state of the art, meta-ethnography, critical, and integrative reviews. The LITR-EX site has a health professions education focus, but the advice and information is widely applicable.
Review the table to peruse review types and associated methodologies. Librarians can also help your team determine which review type might be appropriate for your project.
Reproduced from Grant, M. J. and Booth, A. (2009), A typology of reviews: an analysis of 14 review types and associated methodologies. Health Information & Libraries Journal, 26: 91-108. doi:10.1111/j.1471-1842.2009.00848.x
|
|
|
|
|
|
| Aims to demonstrate writer has extensively researched literature and critically evaluated its quality. Goes beyond mere description to include degree of analysis and conceptual innovation. Typically results in hypothesis or mode | Seeks to identify most significant items in the field | No formal quality assessment. Attempts to evaluate according to contribution | Typically narrative, perhaps conceptual or chronological | Significant component: seeks to identify conceptual contribution to embody existing or derive new theory |
| Generic term: published materials that provide examination of recent or current literature. Can cover wide range of subjects at various levels of completeness and comprehensiveness. May include research findings | May or may not include comprehensive searching | May or may not include quality assessment | Typically narrative | Analysis may be chronological, conceptual, thematic, etc. |
| Map out and categorize existing literature from which to commission further reviews and/or primary research by identifying gaps in research literature | Completeness of searching determined by time/scope constraints | No formal quality assessment | May be graphical and tabular | Characterizes quantity and quality of literature, perhaps by study design and other key features. May identify need for primary or secondary research |
| Technique that statistically combines the results of quantitative studies to provide a more precise effect of the results | Aims for exhaustive, comprehensive searching. May use funnel plot to assess completeness | Quality assessment may determine inclusion/ exclusion and/or sensitivity analyses | Graphical and tabular with narrative commentary | Numerical analysis of measures of effect assuming absence of heterogeneity |
| Refers to any combination of methods where one significant component is a literature review (usually systematic). Within a review context it refers to a combination of review approaches for example combining quantitative with qualitative research or outcome with process studies | Requires either very sensitive search to retrieve all studies or separately conceived quantitative and qualitative strategies | Requires either a generic appraisal instrument or separate appraisal processes with corresponding checklists | Typically both components will be presented as narrative and in tables. May also employ graphical means of integrating quantitative and qualitative studies | Analysis may characterise both literatures and look for correlations between characteristics or use gap analysis to identify aspects absent in one literature but missing in the other |
| Generic term: summary of the [medical] literature that attempts to survey the literature and describe its characteristics | May or may not include comprehensive searching (depends whether systematic overview or not) | May or may not include quality assessment (depends whether systematic overview or not) | Synthesis depends on whether systematic or not. Typically narrative but may include tabular features | Analysis may be chronological, conceptual, thematic, etc. |
| Method for integrating or comparing the findings from qualitative studies. It looks for ‘themes’ or ‘constructs’ that lie in or across individual qualitative studies | May employ selective or purposive sampling | Quality assessment typically used to mediate messages not for inclusion/exclusion | Qualitative, narrative synthesis | Thematic analysis, may include conceptual models |
| Assessment of what is already known about a policy or practice issue, by using systematic review methods to search and critically appraise existing research | Completeness of searching determined by time constraints | Time-limited formal quality assessment | Typically narrative and tabular | Quantities of literature and overall quality/direction of effect of literature |
| Preliminary assessment of potential size and scope of available research literature. Aims to identify nature and extent of research evidence (usually including ongoing research) | Completeness of searching determined by time/scope constraints. May include research in progress | No formal quality assessment | Typically tabular with some narrative commentary | Characterizes quantity and quality of literature, perhaps by study design and other key features. Attempts to specify a viable review |
| Tend to address more current matters in contrast to other combined retrospective and current approaches. May offer new perspectives | Aims for comprehensive searching of current literature | No formal quality assessment | Typically narrative, may have tabular accompaniment | Current state of knowledge and priorities for future investigation and research |
| Seeks to systematically search for, appraise and synthesis research evidence, often adhering to guidelines on the conduct of a review | Aims for exhaustive, comprehensive searching | Quality assessment may determine inclusion/exclusion | Typically narrative with tabular accompaniment | What is known; recommendations for practice. What remains unknown; uncertainty around findings, recommendations for future research |
| Combines strengths of critical review with a comprehensive search process. Typically addresses broad questions to produce ‘best evidence synthesis’ | Aims for exhaustive, comprehensive searching | May or may not include quality assessment | Minimal narrative, tabular summary of studies | What is known; recommendations for practice. Limitations |
| Attempt to include elements of systematic review process while stopping short of systematic review. Typically conducted as postgraduate student assignment | May or may not include comprehensive searching | May or may not include quality assessment | Typically narrative with tabular accompaniment | What is known; uncertainty around findings; limitations of methodology |
| Specifically refers to review compiling evidence from multiple reviews into one accessible and usable document. Focuses on broad condition or problem for which there are competing interventions and highlights reviews that address these interventions and their results | Identification of component reviews, but no search for primary studies | Quality assessment of studies within component reviews and/or of reviews themselves | Graphical and tabular with narrative commentary | What is known; recommendations for practice. What remains unknown; recommendations for future research |
- << Previous: What is a Systematic Review?
- Next: Manuals and Reporting Guidelines >>
- Last Updated: Jun 18, 2024 9:41 AM
- URL: https://guides.mclibrary.duke.edu/sysreview
- Duke Health
- Duke University
- Duke Libraries
- Medical Center Archives
- Duke Directory
- Seeley G. Mudd Building
- 10 Searle Drive
- [email protected]
Log in using your username and password
- Search More Search for this keyword Advanced search
- Latest content
- Current issue
- Browse by collection
- BMJ Journals
You are here
- Online First
- Intracoronary thrombolysis in ST-elevation myocardial infarction: a systematic review and meta-analysis
- Article Text
- Article info
- Citation Tools
- Rapid Responses
- Article metrics

- http://orcid.org/0000-0002-3678-2855 Rajan Rehan 1 , 2 ,
- http://orcid.org/0000-0002-7235-3919 Sohaib Virk 3 ,
- http://orcid.org/0000-0002-0180-0072 Christopher C Y Wong 4 , 5 ,
- Freda Passam 6 ,
- Jamie Layland 7 ,
- Anthony Keech 8 ,
- Andy Yong 4 ,
- http://orcid.org/0000-0001-7712-6750 Harvey D White 9 ,
- William Fearon 10 ,
- Martin Ng 1 , 11
- 1 Royal Prince Alfred Hospital , Camperdown , New South Wales , Australia
- 2 Concord Hospital , Concord , New South Wales , Australia
- 3 Systematic Reviews , CORE Group , Sydney , New South Wales , Australia
- 4 Cardiology , Concord Repatriation General Hospital , Concord , New South Wales , Australia
- 5 Stanford Hospital , Stanford , California , USA
- 6 Department of Hematology , University of Sydney , Sydney , New South Wales , Australia
- 7 Monash University , Melbourne , Victoria , Australia
- 8 NHMRC Clinical Trials Centre , The University of Sydney , Sydney , New South Wales , Australia
- 9 Cardiology Department , Green Lane Cardiovascular Service and Green Lane Cardiovascular Research Unit, Auckland City Hospital , Auckland , New Zealand
- 10 Stanford University , Stanford , California , USA
- 11 Department of Cardiology , The University of Sydney , Sydney , New South Wales , Australia
- Correspondence to Professor Martin Ng, Department of Cardiology, The University of Sydney, Sydney, New South Wales, Australia; Martin.ng{at}sydney.edu.au
Background Despite restoration of epicardial blood flow in acute ST-elevation myocardial infarction (STEMI), inadequate microcirculatory perfusion is common and portends a poor prognosis. Intracoronary (IC) thrombolytic therapy can reduce microvascular thrombotic burden; however, contemporary studies have produced conflicting outcomes.
Objectives This meta-analysis aims to evaluate the efficacy and safety of adjunctive IC thrombolytic therapy at the time of primary percutaneous coronary intervention (PCI) among patients with STEMI.
Methods Comprehensive literature search of six electronic databases identified relevant randomised controlled trials. The primary outcome was major adverse cardiac events (MACE). The pooled risk ratio (RR) and weighted mean difference (WMD) with a 95% CI were calculated.
Results 12 studies with 1915 patients were included. IC thrombolysis was associated with a significantly lower incidence of MACE (RR=0.65, 95% CI 0.51 to 0.82, I 2 =0%, p<0.0004) and improved left ventricular ejection fraction (WMD=1.87; 95% CI 1.07 to 2.67; I 2 =25%; p<0.0001). Subgroup analysis demonstrated a significant reduction in MACE for trials using non-fibrin (RR=0.39, 95% CI 0.20 to 0.78, I 2 =0%, p=0.007) and moderately fibrin-specific thrombolytic agents (RR=0.62, 95% CI 0.47 to 0.83, I 2 =0%, p=0.001). No significant reduction was observed in studies using highly fibrin-specific thrombolytic agents (RR=1.10, 95% CI 0.62 to 1.96, I 2 =0%, p=0.75). Furthermore, there were no significant differences in mortality (RR=0.91; 95% CI 0.48 to 1.71; I 2 =0%; p=0.77) or bleeding events (major bleeding, RR=1.24; 95% CI 0.47 to 3.28; I 2 =0%; p=0.67; minor bleeding, RR=1.47; 95% CI 0.90 to 2.40; I 2 =0%; p=0.12).
Conclusion Adjunctive IC thrombolysis at the time of primary PCI in patients with STEMI improves clinical and myocardial perfusion parameters without an increased rate of bleeding. Further research is needed to optimise the selection of thrombolytic agents and treatment protocols.
- Acute Coronary Syndrome
- Myocardial Infarction
- Meta-Analysis
- Atherosclerosis
Data availability statement
All data relevant to the study are included in the article or uploaded as supplemental information.
This is an open access article distributed in accordance with the Creative Commons Attribution Non Commercial (CC BY-NC 4.0) license, which permits others to distribute, remix, adapt, build upon this work non-commercially, and license their derivative works on different terms, provided the original work is properly cited, appropriate credit is given, any changes made indicated, and the use is non-commercial. See: http://creativecommons.org/licenses/by-nc/4.0/ .
https://doi.org/10.1136/heartjnl-2024-324078
Statistics from Altmetric.com
Request permissions.
If you wish to reuse any or all of this article please use the link below which will take you to the Copyright Clearance Center’s RightsLink service. You will be able to get a quick price and instant permission to reuse the content in many different ways.
WHAT IS ALREADY KNOWN ON THIS TOPIC
ST-elevation myocardial infarction (STEMI) is a significant cause of morbidity and mortality worldwide. Microvascular obstruction affects about half of patients with STEMI, leading to adverse outcomes. Previous studies on adjunctive intracoronary thrombolysis have shown inconsistent results.
WHAT THIS STUDY ADDS
This meta-analysis demonstrates that adjunctive intracoronary thrombolysis during primary percutaneous coronary intervention (PCI) significantly reduces major adverse cardiac events and improves left ventricular ejection fraction. Furthermore, it significantly improves myocardial perfusion parameters without increasing bleeding risk.
HOW THIS STUDY MIGHT AFFECT RESEARCH, PRACTICE OR POLICY
Adjunctive intracoronary thrombolysis in patients with STEMI undergoing primary PCI shows promise for clinical benefit. Future studies should identify high-risk patients for microcirculatory dysfunction to optimise treatment strategies and clinical outcomes.
Introduction
Ischaemic heart disease remains a leading cause of morbidity and mortality worldwide. 1 2 ST-elevation myocardial infarction (STEMI) occurs due to coronary vessel occlusion causing transmural myocardial ischaemia and subsequent necrosis. 3 The cornerstone of contemporary management involves prompt reopening of the occluded coronary artery with percutaneous coronary intervention (PCI). 4 5 Despite restoring epicardial blood flow, roughly 50% of patients fail to achieve adequate microvascular perfusion. 6 This phenomenon, known as microvascular obstruction (MVO), is predictive of a poor cardiac prognosis driven by left ventricular remodelling and larger infarct size. 7–9
In patients with STEMI, MVO is characterised by distal embolisation of atherothrombotic debris and fibrin-rich microvascular thrombi. 10 A growing body of evidence supports the efficacy of adjunctive low-dose intracoronary (IC) thrombolysis in this population. Sezer et al performed the first randomised controlled trial (RCT), demonstrating an improvement in myocardial perfusion with low-dose IC streptokinase post-PCI. 11 Subsequent studies focused on newer fibrin-specific agents with a lower propensity for systemic bleeding. 12 Despite encouraging results, many studies were inadequately powered and yielded conflicting outcomes. This meta-analysis aims to evaluate the efficacy and safety of adjunctive IC thrombolytic therapy at the time of primary PCI in patients with STEMI.
The present study was conducted in accordance with the Preferred Reporting Items for Systematic Reviews and Meta-Analyses (PRISMA) statement. 13
Search strategy and study selection
Electronic searches were performed using PubMed, Ovid Medline, Cochrane Library, ProQuest, ACP Journal Club and Google Scholar from their dates of inception to January 2022. The search terms “STEMI” AND “intracoronary” AND (“thrombolysis” OR “tenecteplase” OR “alteplase” OR “prourokinase” OR “urokinase” OR “streptokinase”) were combined as both keywords and Medical Subject Headings terms, with filters for RCTs. This was supplemented by hand searching the bibliographies of review articles and all potentially relevant studies.
Two reviewers (RR and SV) independently screened the title and abstracts of articles identified in the search. Full-text publications were subsequently reviewed separately if either reviewer considered the manuscript as being potentially eligible. Any disagreements regarding final study inclusion were resolved by discussion and consensus with a third reviewer (CCYW).
Eligibility criteria
Studies were included if they met following inclusion criteria: (1) RCT, (2) STEMI population, (3) IC thrombolysis given to treatment group with comparison with a control group (CG) receiving no thrombolytic therapy, (4) major adverse cardiovascular event (MACE) was an outcome reported.
All publications were limited to those involving human subjects and no restrictions were based on language. Reviews, meta-analyses, abstracts, case reports, conference presentations, editorials and expert opinions were excluded. When institutions published duplicate studies with accumulating numbers of patients or increased lengths of follow-up, only the most complete reports were included for assessment.
Data extraction and quality assessment
Two investigators (RR and SV) independently extracted data from text, tables and figures. Any discrepancies were resolved by discussion and consensus with a third reviewer (CCYW). For each of the included trials, the following data were extracted: publication year, number of patients, baseline characteristics of participants, treatment details (including specific agents administered), follow-up duration and endpoints.
Study quality and risk of bias were critically appraised using the updated Cochrane Collaboration Risk-of-Bias Tool V.2. 14 Five domains of bias were evaluated: (1) randomisation process, (2) deviations from study protocol, (3) missing outcome data, (4) outcome measurement and (5) selective reporting of results.
The predetermined primary endpoint was MACE, which represented a composite outcome as defined by each individual study. While the individual components of MACE were generally consistent across studies, minor discrepancies existed ( online supplemental table 1 ). Secondary outcomes included clinical endpoints (mortality, heart failure (HF), major and minor bleeding), myocardial perfusion endpoints (thrombolysis in myocardial infarction (TIMI) flow grade 3, TIMI myocardial perfusion grade (TMPG), corrected TIMI frame count (CTFC), ST-resolution (STR)) and echocardiographic parameters (left ventricular ejection fraction (LVEF)). Subgroup analysis for MACE was conducted based on fibrin specificity of the thrombolytic agent. This classification comprised non-fibrin-specific agents (streptokinase and urokinase), moderately fibrin-specific agents (prourokinase) and highly fibrin-specific agents (alteplase and tenectaplase). Clinical outcomes were assessed at the end of the follow-up period, which ranged from 1 to 12 months, while echocardiographic parameters were evaluated within a time frame of 1–6 months.
Supplemental material
Statistical analysis.
The mean difference (MD) or relative risk (RR) was used as summary statistics and reported with 95% CIs. Meta-analyses were performed using random-effects models to take into account the anticipated clinical and methodological diversity between studies. The I 2 statistic was used to estimate the percentage of total variation across studies due to heterogeneity rather than chance, with values exceeding 50% indicative of considerable heterogeneity. For meta-analysis of continuous data, values presented as median and IQR were converted to mean and SD using the quantile method previously described by Wan et al . 15 For subgroup analyses, a standard test of heterogeneity was used to assess for significant difference between subgroups with p<0.05 considered statistically significant.
Meta-regression analyses were performed to explore potential heterogeneity with the following moderator variables individually assessed for significance: publication year, mean age, proportion of male participants, percentage of left anterior descending artery infarcts, proportion of smokers, as well as baseline prevalence of diabetes, hypertension and dyslipidaemia.
Publication bias was assessed for the primary endpoint of MACE using funnel plots comparing log of point estimates with their SE. Egger’s linear regression method and Begg’s rank correlation test were used to detect funnel plot asymmetry. 16 17 Statistical analysis was conducted with Review Manager V.5.3.5 (Cochrane Collaboration, Oxford, UK) and Comprehensive Meta-Analysis V.3.0 (Biostat, Englewood, New Jersey, USA). All p values were two sided, and values <0.05 were considered statistically significant.
A total of 245 unique records were identified through electronic searches using six online databases, from which 85 duplicates were removed. Of these, 120 were excluded based on title and abstract alone. After screening the full text of the remaining 40 articles, 12 studies 18–29 were found to meet the inclusion criteria, as summarised on the PRISMA flow chart in figure 1 .
- Download figure
- Open in new tab
- Download powerpoint
Preferred Reporting Items for Systematic Reviews and Meta-Analyses flow chart of literature search and study selection.
IC thrombolysis was examined in 12 studies (n=1030 received IC thrombolysis and 885 no IC thrombolysis). Included studies used non-fibrin-specific (streptokinase, urokinase), moderately fibrin-specific (prourokinase) and highly fibrin-specific thrombolytic (alteplase, tenecteplase) agents. The timing and delivery of IC thrombolytic therapy varied between studies. A complete summary of study characteristics and baseline participant characteristics is presented in tables 1 and 2 , respectively. Primary and secondary outcomes are summarised in online supplemental table 2 . According to the revised Cochrane tool, the overall risk of bias assessment for procedural measures was judged to be ‘low risk’ in two studies, ‘some concerns’ in eight studies and ‘high risk’ in two studies ( online supplemental figure 1 ).
- View inline
Summary of studies investigating intracoronary thrombolysis for patients with STEMI
Summary of baseline patient characteristics in studies investigating intracoronary thrombolysis for patients with STEMI
Clinical outcomes
All 12 RCTs reported the incidence of MACE. Compared with the CG, IC thrombolysis treatment significantly improved the occurrence of MACE at the end of follow-up (RR=0.65, 95% CI 0.51 to 0.82, I 2 =0%, p<0.0004; figure 2 ). Subgroup analysis demonstrated a significant reduction in MACE for trials using non-fibrin (RR=0.39, 95% CI 0.20 to 0.78, I 2 =0%, p=0.007) and moderately fibrin-specific thrombolysis (RR=0.62, 95% CI 0.47 to 0.83, I 2 =0%, p=0.001). MACE was observed at a similar rate in studies using highly fibrin-specific thrombolysis (RR=1.10, 95% CI 0.62 to 1.96, I 2 =0%, p=0.75). Test for subgroup difference was not significant (p=0.07). Furthermore, IC thrombolysis was associated with an improvement of LVEF (weighted MD (WMD)=1.87; 95% CI, 1.07 to 2.67; I 2 =25%; p<0.0001; online supplemental figure 2 ). There was a trend towards lower incidence of HF hospitalisation (RR=0.66; 95% CI 0.42 to 1.05; I 2 =0%; p=0.08; online supplemental figure 3 ), though not statistically significant. No significant differences were observed in mortality (RR=0.95; 95% CI 0.50 to 1.81; I 2 =0%; p=0.88; online supplemental figure 4 ), major bleeding (RR=1.24; 95% CI 0.47 to 3.28; I 2 =0%; p=0.67; online supplemental figure 5 ) and minor bleeding events (RR=1.47; 95% CI 0.90 to 2.40; I 2 =0%; p=0.12; online supplemental figure 6 ) between the two groups.
Forest plot displaying relative risk for major adverse cardiovascular events with intracoronary (IC) thrombolysis (stratified by fibrin-specific and non-fibrin-specific agents) or placebo in ST-elevation myocardial infarction. Squares and diamonds=risk ratios. Lines=95% CIs.
Myocardial perfusion outcomes
In patients with STEMI, IC thrombolysis significantly improved TIMI flow grade 3 (RR=1.09; 95% CI 1.02 to 1.15; I 2 =63%; p=0.006), TMPG (RR=1.38; 95% CI 1.13 to 1.68; I 2 =54%; p=0.001), complete STR (RR=1.20; 95% CI 1.10 to 1.31; I 2 =51%; p<0.0001) and CTFC (WMD=−4.58; 95% CI −6.23 to –2.72; I 2 =41%; p<0.0001) when compared with the CG ( figure 3 ).
Forest plots of myocardial perfusion outcomes with intracoronary (IC) thrombolysis or placebo in ST-elevation myocardial infarction. (A) Thrombolysis in myocardial infarction (TIMI) flow grade 3. (B) TIMI myocardial perfusion grade 3. (C) ST-segment resolution. (D) Corrected TIMI frame count. Squares and diamonds=risk ratios/weighted mean difference. Lines=95% CIs.
Meta-regression results
For primary endpoint of MACE, meta-regression analyses did not identify the following moderator variables as significant effect modifiers: publication year (p=0.97), proportion of male (p=0.23), prevalence of diabetes (p=0.44), proportion of smokers (p=0.68), prevalence of dyslipidaemia (p=0.44) and prevalence of hypertension (p=0.21).
Publication bias
Both Egger’s linear regression method (p=0.73) and Begg’s rank correlation test (p=0.63) suggested that publication bias was not an influencing factor when MACE was selected as the primary endpoint.
The present meta-analysis examined 12 RCTs that included 1915 patients with STEMI undergoing primary PCI. All trials evaluated the efficacy and safety of IC thrombolytic agents compared with a CG. The main findings were that patients administered IC thrombolysis had: (1) significantly lower incidence of MACE, (2) improvement in LVEF and (3) superior myocardial perfusion parameters (TIMI flow grade 3, TMPG, CTFC and complete STR). Notably, there were no significant differences observed in mortality and bleeding events in both groups.
Mortality rates following STEMI remain high, with 30-day mortality rates ranging from 5.4% to 14% and 1-year mortality rates ranging from 6.6% to 17.5%. 30 Despite the increased availability of primary PCI facilities and advancements in reperfusion strategies, there has been limited improvement in STEMI mortality rates. 31 Moreover, complications such as HF, arrhythmia, repeat revascularisation and reinfarction continue to be prevalent. 32–34 Despite restoring epicardial blood flow through PCI, MVO is evident in almost half of patients with STEMI. 6 It is characterised by distal embolisation of atherothrombotic debris, de novo microvascular thrombosis formation and plugging of circulating blood cells. 35 Furthermore, the upregulation of inflammatory mediators leads to intramyocardial haemorrhage and further microvascular necrosis. 36 37 These mechanistic pathways contribute to a larger infarct size, adverse myocardial remodelling and worse prognosis. 7 8 38
Thrombolytic therapy is an effective treatment for acute coronary thrombosis. 39 It inhibits red blood cell aggregation and dissolves thrombi to facilitate adequate microvascular perfusion. 40 41 Thrombolytic agents are commonly classified based on their affinity for fibrin. Streptokinase and urokinase lack fibrin specificity, indiscriminately activating both circulating and clot-bound plasminogen. Prourokinase has moderate fibrin specificity with a propensity for activation on fibrin surfaces, although systemic fibrinogen degradation has been observed. Alteplase and tenectaplase are highly fibrin specific, activating fibrin-bound plasminogen with minimal impact on circulating free plasminogen.
Utilisation of a facilitated PCI strategy with adjunctive intravenous thrombolysis improves coronary flow acutely, 42 however, causes paradoxical activation of thrombin, leading to increased bleeding. 43 44 As a result, clinicians considered the administration of IC thrombolytic therapy. Encouraging results from an open-chest animal model 45 led to the first randomised trial using adjunctive IC streptokinase in 41 patients with STEMI undergoing primary PCI. 11 In the IC streptokinase group, patients demonstrated improved coronary flow reserve, index of microcirculatory resistance (IMR) and CTFC 2 days after primary PCI. 11 Further RCTs with moderately fibrin-specific thrombolytic agents (prourokinase) demonstrated similar results with improved myocardial perfusion parameters. 19 20 22 23 26–28 Notably, the T-TIME Study, a large RCT of 440 patients comparing a highly fibrin-specific thrombolytic agent (alteplase) against placebo, reported different outcomes. At 3-month follow-up, there were no significant differences in rates of death or HF hospitalisation between groups. In addition, microvascular obstruction (% left ventricular mass) on cardiac magnetic resonance (CMR) between groups at 2–7 days did not differ. The ICE T-TIMI trial, which also used a highly fibrin-specific thrombolytic agent (tenecteplase), investigated its efficacy in 40 patients. This small study administered two fixed doses of 4 mg of IC tenecteplase and evaluated the primary endpoint of culprit lesion per cent diameter stenosis after the first bolus of tenecteplase or placebo. The results indicated no significant difference in the primary endpoint between the two groups.
In an initial meta-analysis of six RCTs investigating the use of IC thrombolysis in patients with STEMI compared with placebo, findings revealed a reduction in MVO but no impact on MACE. 46 Subsequent analyses, including studies with larger sample sizes or focusing on specific thrombolytic agents, have since been conducted with varied results. 47 48 Our meta-analysis, which is the largest to date, demonstrates that adjunctive IC thrombolysis in patients with STEMI improves both clinical and microcirculation outcomes. Although bleeding events did not significantly increase, it is plausible that a tradeoff may exist for reducing MACE. Notably, subgroup analysis for MACE demonstrated no significant benefit for highly fibrin-specific agents ( figure 2 ).
Intuitively, fibrin-specific thrombolytics are presumed to offer inherent advantages over their less fibrin-specific counterparts. In vivo studies have revealed that administration of alteplase in patients with STEMI induced shorter periods of thrombin and kallikrein activation, less reduction in fibrinogen, and a decrease in D-dimer and plasmin–antiplasmin complexes compared with streptokinase. 49 In this regard, tenecteplase demonstrates superior performance relative to alteplase with almost no paradoxical procoagulant effect due to reduced activation of thrombin and the kallikrein–factor XII system. 50
Nonetheless, other variables may diminish the significance of fibrin specificity. It has been argued that administration of IC alteplase, a short-acting thrombolytic with a half-life of 4–6 min, before flow optimisation with stenting may have contributed to the negative results seen in T-TIME. Although prourokinase has a similarly short half-life and was also given before stenting in multiple studies, it was associated with better results. 19 20 22 23 26–28 The therapeutic efficacy of prourokinase predominantly relies on its conversion to urokinase, a non-fibrin-specific direct plasminogen activator, potentially resulting in a prolonged duration of action. Furthermore, inducing a systemic fibrinolytic state with a non-selective agent may be paradoxically desirable in patients receiving adjunctive IC thrombolytics during primary PCI. This approach can potentially prevent further thrombus reaccumulation and embolisation to the microcirculation, especially in a highly thrombogenic environment. In contrast, fibrin-specific agents may heighten the risk of rethrombosis and reocclusion due to their limited impact on systemic fibrinogen depletion. Nevertheless, such varied outcomes across these studies could be attributed to the heterogeneous methodologies used.
Despite encouraging results, future studies targeting patients at the highest risk of MVO with appropriately powered sample sizes are required. The ongoing RESTORE-MI (Restoring Microcirculatory Perfusion in STEMI) trial ( NCT03998319 ) has a unique approach in which all study participants will undergo assessment of microvascular integrity after primary PCI prior to inclusion. Only patients with objective evidence of microvascular dysfunction (IMR value >32) following reperfusion will be randomised to treatment with IC tenecteplase or placebo. The primary endpoint measured will be cardiovascular mortality and rehospitalisation for HF at 24 months, in addition to infarct size on CMR at 6 months post-PCI. This study may potentially support a novel therapeutic approach towards treating MVO in patients with STEMI in the future.
Limitations
Several key limitations should be considered when interpreting the findings of the present meta-analysis. First, several studies were subject to bias due to issues in randomisation and blinding, leading to an increased chance of type 1 (false-positive) error. In addition, the sample size of individual studies, except for the T-TIME trial, was relatively small. Second, inconsistencies in the duration of follow-up and the definition of clinical outcomes, such as MACE, were observed among the studies. Third, interventional protocols varied between RCTs. For example, IC thrombolytic therapy differed in agent, dosage, timing and route of administration. Initial studies used non-fibrin-specific agents, while contemporary studies moved towards newer fibrin-specific therapy. Besides Sezer et al , 25 all other studies administered IC thrombolysis therapy prior to stent implantation. 18–24 26–29 Within the latter group, some delivered before flow restoration, 19 21 29 though most did so after balloon dilation or thrombus aspiration. 18 20 22–24 26–28 Similarly, the methods of IC administration of the agents varied between non-selective delivery through guiding catheters 24 25 to selective delivery via IC catheters. 18–24 26–29 Furthermore, antiplatelet, anticoagulant and glycoprotein IIb/IIIa inhibitors (GPI) regimens also differed ( table 1 ). Finally, patient selection was diverse between studies. Though regression analysis did not detect any significant effect modifiers, total ischaemic time was omitted due to significant heterogeneity in reporting.
Impaired myocardial perfusion remains a clinical challenge in patients with STEMI. Despite its limitations, this meta-analysis favours the use of IC thrombolytic therapy during primary PCI. Overall, IC thrombolysis reduced the incidence of MACE and improved myocardial perfusion markers without increasing the risk of bleeding. Future clinical trials should be appropriately powered for clinical outcomes and focus on patients at high risk of microcirculatory dysfunction.
Ethics statements
Patient consent for publication.
Not applicable.
Ethics approval
- Chierchia S ,
- Lawton JS ,
- Tamis-Holland JE ,
- Bangalore S , et al
- Agewall S , et al
- Sorajja P ,
- Costantini C , et al
- van de Hoef TP ,
- Meuwissen M , et al
- Regenfus M ,
- Schlundt C ,
- Krähner R , et al
- Fearon WF ,
- Ng M , et al
- Holmes DR ,
- Herrmann J , et al
- Gören T , et al
- Liberati A ,
- Tetzlaff J , et al
- Sterne JAC ,
- Savović J ,
- Page MJ , et al
- Liu J , et al
- Davey Smith G ,
- Schneider M , et al
- Hao GZ , et al
- Gibson CM ,
- Gopalakrishnan L , et al
- Liu Z , et al
- Du X , et al
- McCartney PJ ,
- Maznyczka AM , et al
- Aslanger E , et al
- Wu H , et al
- Feng Q , et al
- Wang Y , et al
- Pelliccia F ,
- Tanzilli G , et al
- Mozaffarian D ,
- Benjamin EJ ,
- Go AS , et al
- Chandra M , et al
- Melenovský V ,
- Stehlik J , et al
- Windecker S ,
- Myat A , et al
- Rathor P , et al
- Konijnenberg LSF ,
- Duncker DJ , et al
- Betgem RP ,
- de Waard GA ,
- Nijveldt R , et al
- Yellon DM ,
- Hausenloy DJ
- Messalli G ,
- Dymarkowski S , et al
- ↵ Randomised trial of intravenous streptokinase, oral aspirin, both, or neither among 17,187 cases of suspected acute myocardial infarction: ISIS-2. ISIS-2 (Second international study of infarct survival) collaborative group . Lancet 1988 ; 2 : 349 – 60 . doi:10.1016/S0140-6736(88)92833-4 OpenUrl PubMed Web of Science
- Schwartz RS ,
- Farb A , et al
- Zalewski J ,
- Godlewski J , et al
- Hillis WS ,
- Been M , et al
- ↵ Primary versus Tenecteplase-facilitated percutaneous coronary intervention in patients with ST-segment elevation acute myocardial infarction (ASSENT-4 PCI): randomised trial . The Lancet 2006 ; 367 : 569 – 78 . doi:10.1016/S0140-6736(06)68147-6 OpenUrl
- Tendera M ,
- de Belder MA , et al
- Armiger LC ,
- White HD , et al
- Alyamani M ,
- Campbell S ,
- Navarese E , et al
- Tian W , et al
- Li ZP , et al
- Hoffmeister HM ,
- Kastner C , et al
- Ehlers R , et al
Supplementary materials
Supplementary data.
This web only file has been produced by the BMJ Publishing Group from an electronic file supplied by the author(s) and has not been edited for content.
- Data supplement 1
X @RajanRehan23
Contributors RR—conceptualisation, methodology, data analysis, writing (original draft preparation), reviewing and editing the final manuscript. SV—methodology, data analysis. CCYW—conceptualisation, methodology, data analysis. FP—supervision, writing (reviewing and editing). JL—supervision, writing (reviewing and editing). AK—supervision, writing (reviewing and editing). AY—conceptualisation, methodology, writing (reviewing and editing). HDW—conceptualisation, methodology, writing (reviewing and editing). WF—conceptualisation, methodology, writing (reviewing and editing). MN—conceptualisation, methodology, supervision, writing (reviewing and editing), guarantor.
Funding This study is funded by the National Health and Medical Research Council (2022150).
Competing interests JL has received minor honoraria from Abbott Vascular, Boehringer Ingelheim and Bayer. AY has received minor honoraria and research support from Abbot Vascular and Philips Healthcare. WF has received research support from Abbott Vascular and Medtronic; and has minor stock options with HeartFlow. MN has received research support from Abbot Vascular. HDW has received grant support paid to the institution and fees for serving on Steering Committees of the ODYSSEY trial from Sanofi and Regeneron Pharmaceuticals, the ISCHEMIA and MINT Study from the National Institutes of Health, the STRENGTH trial from Omthera Pharmaceuticals, the HEART-FID Study from American Regent, the DAL-GENE Study from DalCor Pharma UK, the AEGIS-II Study from CSL Behring, the CLEAR OUTCOMES Study from Esperion Therapeutics, and the SOLIST-WHF and SCOREDS trials from Sanofi Aventis Australia. The remaining authors have nothing to disclose.
Patient and public involvement Patients and/or the public were not involved in the design, or conduct, or reporting, or dissemination plans of this research.
Provenance and peer review Not commissioned; externally peer reviewed.
Supplemental material This content has been supplied by the author(s). It has not been vetted by BMJ Publishing Group Limited (BMJ) and may not have been peer-reviewed. Any opinions or recommendations discussed are solely those of the author(s) and are not endorsed by BMJ. BMJ disclaims all liability and responsibility arising from any reliance placed on the content. Where the content includes any translated material, BMJ does not warrant the accuracy and reliability of the translations (including but not limited to local regulations, clinical guidelines, terminology, drug names and drug dosages), and is not responsible for any error and/or omissions arising from translation and adaptation or otherwise.
Read the full text or download the PDF:
- Skip to primary navigation
- Skip to main content
- Skip to primary sidebar
information for practice
news, new scholarship & more from around the world
- gary.holden@nyu.edu
- @ Info4Practice
Evidence Synthesis Ireland: ‘Studies Within A Review’ (SWAR) programme of research is an exciting way to evaluate alternative options when conducting a review process (e.g. study selection, data extraction, reporting the findings) to provide much-needed evidence about how these steps in the review processes can be improved (Call closes: Sept 3)

An official website of the United States government
The .gov means it’s official. Federal government websites often end in .gov or .mil. Before sharing sensitive information, make sure you’re on a federal government site.
The site is secure. The https:// ensures that you are connecting to the official website and that any information you provide is encrypted and transmitted securely.
- Publications
- Account settings
Preview improvements coming to the PMC website in October 2024. Learn More or Try it out now .
- Advanced Search
- Journal List
- J Otolaryngol Head Neck Surg

Systematic and other reviews: criteria and complexities
Robert t. sataloff.
1 Editor-in-Chief, Journal of Voice, Philadephia, USA
2 Editor Emeritus, Ear, Nose and Throat Journal, Philadephia, USA
Matthew L. Bush
3 Assistant Editor, Otology & Neurotology, Lexington, USA
Rakesh Chandra
4 Editor-in-Chief, Ear, Ear, Nose and Throat Journal, Nashville, USA
Douglas Chepeha
5 Editor-in-Chief, Journal of Otolaryngology – Head & Neck Surgery, Toronto, Canada
Brian Rotenberg
6 Editor-in-Chief, Journal of Otolaryngology – Head & Neck Surgery, London, Canada
Edward W. Fisher
7 Senior Editor, Journal of Laryngology and Otology, Birmingham, UK
David Goldenberg
8 Editor-in-Chief, Operative Techniques in Otolaryngology – Head and Neck Surgery, Hershey, USA
Ehab Y. Hanna
9 Editor-in-Chief, Head & Neck, Houston, USA
Joseph E. Kerschner
10 Editor-in-Chief, International Journal of Pediatric Otorhinolaryngology, Milwaukee, USA
Dennis H. Kraus
11 Co-Editor-in-Chief, Journal of Neurological Surgery Part B: Skull Base, New York, USA
John H. Krouse
12 Editor-in-Chief, Otolaryngology – Head and Neck Surgery, Philadelphia, USA
13 Editor-in-Chief, OTO-Open, Philadelphia, USA
14 Editor-in-Chief, Journal for Oto-Rhino-Laryngology, Head and Neck Surgery, Philadelphia, USA
15 Editor-in-Chief, World Journal of Otorhinolaryngology – Head and Neck Surgery, Philadelphia, USA
Michael Link
16 Co-Editor-in-Chief, Journal of Neurological Surgery Part B: Skull Base, Rochester, USA
Lawrence R. Lustig
17 Editor-in-Chief, Otology & Neurotology, New York, USA
Samuel H. Selesnick
18 Editor-in-Chief, The Laryngoscope, New York, USA
Raj Sindwani
19 Editor-in-Chief, American Journal of Rhinology & Allergy, Cleveland, USA
Richard J. Smith
20 Editor-in-Chief, Annals of Otology, Rhinology & Laryngology, Iowa City, USA
James Tysome
21 Editor-in-Chief, Clinical Otolaryngology, Cambridge, UK
Peter C. Weber
22 Editor-in-Chief, American Journal of Otolaryngology, Boston, USA
D. Bradley Welling
23 Editor-in-Chief, Laryngoscope Investigative Otolaryngology, Boston, USA
Review articles can be extremely valuable. They synthesize information for readers, often provide clarity and valuable insights into a topic; and good review articles tend to be cited frequently. Review articles do not require Institutional Review Board (IRB) approval if the data reviewed are public (including private and government databases) and if the articles reviewed have received IRB approval previously. However, some institutions require IRB review and exemption for review articles. So, authors should be familiar with their institution’s policy. In assessing and interpreting review articles, it is important to understand the article’s methodology, scholarly purpose and credibility. Many readers, and some journal reviewers, are not aware that there are different kinds of review articles with different definitions, criteria and academic impact [ 1 ]. In order to understand the importance and potential application of a review article, it is valuable for readers and reviewers to be able to classify review articles correctly.
Systematic reviews
Authors often submit articles that include the term “systematic” in the title without realizing that that term requires strict adherence to specific criteria. A systematic review follows explicit methodology to answer a well-defined research question by searching the literature comprehensively, evaluating the quantity and quality of research evidence rigorously, and analyzing the evidence to synthesize an answer to the research question. The evidence gathered in systematic reviews can be qualitative or quantitative. However, if adequate and comparable quantitative data are available then a meta-analysis can be performed to assess the weighted and summarized effect size of the studies included. Depending on the research question and the data collected, systematic reviews may or may not include quantitative meta-analyses; however, meta-analyses should be performed in the setting of a systematic review to ensure that all of the appropriate data were accessed. The components of a systematic review can be found in an important article by Moher et al. published in 2009 that defined requirements for systematic reviews and meta-analyses [ 2 ].
In order to optimize reporting of meta-analyses, an international group developed the Quality of Reporting of Meta-Analyses (QUOROM) statement at a meeting in 1996 that led to publication of the QUOROM statement in 1999 [ 3 ]. Moher et al. revised that document and re-named the guidelines the Preferred Reporting Items for Systematic Reviews and Meta-Analyses (PRISMA). The PRISMA statement included both meta-analyses and systematic reviews, and the authors incorporated definitions established by the Cochrane Collaboration [ 4 ]. The PRISMA statement established the current standard for systematic reviews. To qualify as a systematic review, the methods section should acknowledge use of the PRISMA guidelines, and all PRISMA components should be incorporated strictly in all facets of the paper from the research question to the discussion. The PRISMA statement includes a checklist of 27 items that must be included when reporting a systematic review or meta-analysis [ 2 ]. A downloadable version of this checklist can be used by authors, reviewers, and journal editorial staff to ensure compliance with recommended components [ 5 ]. All 27 will not be listed in this brief editorial (although authors and reviewers are encouraged to consult the article by Moher et al. and familiarize themselves with all items), but a few will be highlighted.
The research question, as reflected in the title, should be a hypothesis-based specific research inquiry. The introduction must describe the rationale for the review and provide a specific goal or set of goals to be addressed. The type of systematic review, according to the Cochrane Collaboration, is based on the research question being asked and may assess diagnostic test accuracy, review prognostic studies evidence, evaluate intervention effect, scrutinize research methodology, or summarize qualitative evidence [ 6 ].
In the methods section, the participants, interventions, comparisons, outcomes and study design (PICOS) must be put forward. In addition to mentioning compliance with PRISMA, the methods section should state whether a review protocol exists and, if so, where it can be accessed (including a registration number). Systematic reviews are eligible for registration in the International Prospective Register of Systematic Reviews (PROSPERO) as established at the University of York (York, UK). When PROSPERO is used (it is available but not required for systematic reviews), registration should occur at the initial protocol stage of the review, and the final paper should direct to the information in the register. The methods section also must include specific study characteristics including databases used, years considered, languages of articles included, specific inclusion and exclusion criteria for studies; and rationale for each criterion must be included. Which individuals specifically performed searches should be noted. Electronic search strategy (with a full description of at least one electronic search strategy sufficient to allow replication of the search), process for article selection, data variables sought, assumptions and simplifications, methods for assessing bias risk of each individual study (such as selective reporting in individual studies) and utilization of this information in data synthesis, principal summary measures (risk ratio, hazard ratio, difference in means, etc.), methods of data management and combining study results, outcome level assessment, and other information should be reported.
The results section should include the number of studies identified, screened, evaluated for eligibility (including rationale for exclusion), and those included in the final synthesis. A PRISMA flow diagram should be included to provide this information succinctly [ 7 ]. The results also should include the study characteristics, study results, risk of bias within and across studies, and a qualitative or quantitative synthesis of the results of the included studies. This level of rigor in acquiring and evaluating the evidence of each individual study is one of the criteria that distinguishes systematic reviews from other categories. If the systematic review involves studies with paired samples and quantitative data, a summary of data should be provided for each intervention group along with effect estimates and confidence intervals for all outcomes of each study. If a meta-analysis is performed, then synthesized effect size should be reported with confidence intervals and measures of consistency (i.e. – data heterogeneity such as I 2 ) for each meta-analysis, and assessment of bias risk across studies. A forest plot, which provides a graphical presentation of the meta-analysis results, should be included.
The discussion section should summarize the main findings commenting on the strength of evidence for each outcome, as well as relevance to healthcare providers, policymakers and other key stake-holders; limitations of the study and outcomes; and conclusions highlighting the interpretation of results in the context of other research, and implications for future research.
Without adhering to of all of these criteria and the others listed in the PRISMA statement and checklist, the review does not qualify to be classified as “systematic”.
Meta-analyses
Meta-analyses, when feasible based on available and comparable quantitative data, supplement a systematic review evaluation, by adding a secondary statistical analysis of the pooled weighted outcomes of similar studies. This adds a level of objectivity in the synthesis of the review’s findings. Meta-analyses are appropriate when at least 2 individual studies contain paired samples (experimental group and control group) and provide quantitative outcome data and sample size. Studies that lack a control group may over-estimate the effect size of the experimental intervention or condition being studied and are not ideal for meta-analyses [ 8 ]. It also should be remembered that the conclusions of a meta-analysis are only as valid as the data on which the analysis is based. If the articles included are flawed, then the conclusions of the meta-analysis also may be flawed. Systematic reviews and meta-analyses are the most rigorous categories of review.
Other types of reviews
Mixed methods reviews.
Systematic reviews typically contain a single type of data, either qualitative or quantitative; however, mixed methods reviews bring together a combination of data types or study types. This approach may be utilized when quantitative data, in the setting of an intervention study, only provide a narrow perspective of the efficacy or effectiveness of the intervention. The addition of qualitative data or qualitative studies may provide a more complete picture of the knowledge, attitudes, and behaviors of clinicians, patients or researchers regarding that intervention. This type of review could involve collecting either the quantitative or the qualitative data using systematic review methodology, but often the qualitative data are gathered using a convenience sampling. Many qualitative studies provide useful insights into clinical management and/or implementation of research interventions; and incorporating them into a mixed methods review may provide valuable perspective on a wide range of literature. Mixed methods reviews are not necessarily systematic in nature; however, authors conducting mixed methods reviews should follow systematic review methodology, when possible.
Literature and narrative reviews
Literature reviews include peer-reviewed original research, systematic reviews, and meta-analyses, but also may include conference abstracts, books, graduate degree theses, and other non-peer reviewed publications. The methods used to identify and evaluate studies should be specified, but they are less rigorous and comprehensive than those required for systematic reviews. Literature reviews can evaluate a broad topic but do not specifically articulate a specific question, nor do they synthesize the results of included studies rigorously. Like mixed method reviews, they provide an overview of published information on the topic, although they may be less comprehensive than integrative reviews; and, unlike systematic reviews, they do not need to support evidence-based clinical or research practices, or highlight high-quality evidence for the reader. Narrative reviews are similar to literature reviews and evaluate the same scope of literature. The terms sometimes are used interchangeably, and author bias in article selection and data interpretation is a potential concern in literature and narrative reviews.
Umbrella reviews
An umbrella review integrates previously published, high-quality reviews such as systematic reviews and meta-analyses. Its purpose is to synthesize information in previously published systematic reviews and meta-analyses into one convenient paper.
Rapid review
A rapid review uses systematic review methodology to evaluate existing research. It provides a quick synthesis of evidence and is used most commonly to assist in emergent decision-making such as that required to determine whether COVID-19 vaccines should receive emergent approval.
Scoping, mapping, and systematized reviews
If literature has not been reviewed comprehensively in a specific subject that is varied and complex, a mapping review (also called scoping review) may be useful to organize initial understanding of the topic and its available literature. While mapping reviews may be helpful in crystallizing research findings and may be published, they are particularly useful in helping to determine whether a topic is amenable to systematic review, and to help organize and direct the approach of the systematic review or other reviews of the subject. Systematized reviews are used most commonly by students. The systematized review provides initial assessment of a topic that is potentially appropriate for a systematic review, but a systematized review does not meet the rigorous criteria of a systematic review and has substantially more limited value. Additional types of reviews exist including critical review, state-of-the-art review, and others.
Reviews can be invaluable; but they also can be misleading. Systematic reviews and meta-analyses provide readers with the greatest confidence that rigorous efforts have attempted to eliminate bias and ensure validity, but even they have limitations based upon the strengths and weaknesses of the literature that they have assessed (and the skill and objectivity with which the authors have executed the review). Risks of bias, incomplete information and misinformation increase as the rigor of review methodology decreases. While review articles may summarize research related to a topic for readers, non-systematic reviews lack the rigor to answer adequately hypothesis-driven research questions that can influence evidence-based practice. Journal authors, reviewers, editorial staff, and should be cognizant of the strengths and weaknesses of review methodology and should consider them carefully as they assess the value of published review articles, particularly as they determine whether the information presented should alter their patient care.
Authors’ contributions
The author(s) read and approved the final manuscript.
Declarations
The authors declare no competing interests.
This article is co-published in the following journals: Journal of Voice, Otology & Neurotology, Ear, Nose and Throat Journal, Journal of Laryngology and Otology, Operative Techniques in Otolaryngology – Head and Neck Surgery, Head & Neck, International Journal of Pediatric Otorhinolaryngology, Journal of Neurological Surgery Part B: Skull Base, Otolaryngology – Head and Neck Surgery, World Journal of Otorhinolaryngology – Head and Neck Surgery, The Laryngoscope, American Journal of Rhinology & Allergy, Annals of Otology, Rhinology & Laryngology, Clinical Otolaryngology, American Journal of Otolaryngology, Laryngoscope Investigative Otolaryngology.
Publisher’s Note
Springer Nature remains neutral with regard to jurisdictional claims in published maps and institutional affiliations.

IMAGES
VIDEO
COMMENTS
A systematic review collects secondary data, and is a synthesis of all available, ... 2015) - although a systematic review may be an inappropriate or unnecessary research methodology for answering many research questions. Systematic reviews can be inadvisable for a variety of reasons. It may be that the topic is too new and there are not ...
A systematic review collects secondary data, and is a synthesis of all available, ... 2015) - although a systematic review may be an inappropriate or unnecessary research methodology for answering many research questions. Systematic reviews can be inadvisable for a variety of reasons. It may be that the topic is too new and there are not ...
Systematic Reviews are a kind of secondary research. The creators of systematic reviews are very intentional about their inclusion/exclusion criteria, or which articles they'll include in their review and the goal is to make a generalized statement so other researchers can build upon the practices or interventions they recommend.
It is easy to confuse systematic reviews and meta-analyses. A systematic review is an objective, reproducible method to find answers to a certain research question, by collecting all available studies related to that question and reviewing and analyzing their results. A meta-analysis differs from a systematic review in that it uses statistical ...
Less familiar and more challenging meta-analytical approaches used in secondary research include individual participant data ... Systematic review: ... Sampson M, Tricco AC, et al. Epidemiology and reporting characteristics of systematic reviews of biomedical research: a cross-sectional study. PLoS Med. 2016; 13 (5):1-31. doi: 10.1371/journal ...
A high-quality systematic review is described as the most reliable source of evidence to guide clinical practice. The purpose of a systematic review is to deliver a meticulous summary of all the available primary research in response to a research question. A systematic review uses all the existing research and is sometime called 'secondary research' (research on research). They are often ...
Systematic reviews are characterized by a methodical and replicable methodology and presentation. They involve a comprehensive search to locate all relevant published and unpublished work on a subject; a systematic integration of search results; and a critique of the extent, nature, and quality of evidence in relation to a particular research question.
A systematic review is a type of review that uses repeatable methods to find, select, and synthesize all available evidence. It answers a clearly formulated research question and explicitly states the methods used to arrive at the answer. Example: Systematic review. In 2008, Dr. Robert Boyle and his colleagues published a systematic review in ...
Rapid review. Assessment of what is already known about a policy or practice issue, by using systematic review methods to search and critically appraise existing research. Completeness of searching determined by time constraints. Time-limited formal quality assessment. Typically narrative and tabular.
A systematic review is a type of review that uses repeatable methods to find, select, and synthesise all available evidence. It answers a clearly formulated research question and explicitly states the methods used to arrive at the answer. Example: Systematic review. In 2008, Dr Robert Boyle and his colleagues published a systematic review in ...
A systematic review is a scholarly synthesis of the evidence on a clearly presented topic using critical methods to identify, define and assess research on the topic. A systematic review extracts and interprets data from published studies on the topic (in the scientific literature), then analyzes, describes, critically appraises and summarizes interpretations into a refined evidence-based ...
Literature reviews establish the foundation of academic inquires. However, in the planning field, we lack rigorous systematic reviews. In this article, through a systematic search on the methodology of literature review, we categorize a typology of literature reviews, discuss steps in conducting a systematic literature review, and provide suggestions on how to enhance rigor in literature ...
Primary research is collecting data directly from patients or population, while secondary research is the analysis of data already collected through primary research. A review is an article that summarizes a number of primary studies and may draw conclusions on the topic of interest which can be traditional (unsystematic) or systematic.
The aim of secondary research is to produce a more or less systematic appraisal and/or synthesis of the existing primary research on a topic. There are numerous types of reviews which aim to summarise or synthesise the evidence on a topic, but here we will focus on two: meta-analyses and systematic reviews.
The research that falls into a somewhat less common category is secondary research. This article covers all the basics of secondary research. ... Like any primary research, systematic reviews and meta-analysis also need a protocol for the methodology. Such protocols can be published in a similar manner as done in case of primary research articles.
Secondary research is a research method that uses data that was collected by someone else. In other words, whenever you conduct research using data that already exists, you are conducting secondary research. On the other hand, any type of research that you undertake yourself is called primary research. Example: Secondary research.
How To Do Secondary Research or a Literature Review. Step-by-step guide to forming keywords and searching for articles for a literature review. Secondary Research; Literature Review; ... The tutorial references "systematic reviews," but the search concept is similar to literature reviews. Example Search Methodology in an APA Paper.
The term systematic review has two meanings, as follows: (1) a literature review that employs a systematic (hence the name), replicable process of selecting primary studies for. Table 1: The Seven Types of Literature Review. Type Systematic Purpose Primary Studies Analysis Approach.
1. INTRODUCTION. Evidence synthesis is a prerequisite for knowledge translation. 1 A well conducted systematic review (SR), often in conjunction with meta‐analyses (MA) when appropriate, is considered the "gold standard" of methods for synthesizing evidence related to a topic of interest. 2 The central strength of an SR is the transparency of the methods used to systematically search ...
It is secondary to and retrospective of the actual findings from an experiment or trial. These studies may be appraised summaries, reviews, or interpretations of primary sources and often exclude the original researcher(s). In the health sciences, meta-analysis and systematic reviews are the most frequent types of secondary research.
The use of Artificial Intelligence (AI) in education is transforming various dimensions of the education system, such as instructional practices, assessment strategies, and administrative processes. It also plays an active role in the progression of science education. This systematic review attempts to render an inherent understanding of the evidence-based interaction between AI and science ...
Not all research questions are well-suited for systematic reviews. Review Typologies (from LITR-EX) This site explores different review methodologies such as, systematic, scoping, realist, narrative, state of the art, meta-ethnography, critical, and integrative reviews. The LITR-EX site has a health professions education focus, but the advice ...
Background Despite restoration of epicardial blood flow in acute ST-elevation myocardial infarction (STEMI), inadequate microcirculatory perfusion is common and portends a poor prognosis. Intracoronary (IC) thrombolytic therapy can reduce microvascular thrombotic burden; however, contemporary studies have produced conflicting outcomes. Objectives This meta-analysis aims to evaluate the ...
In the previous six articles in this series on study designs, we have looked at different types of primary research study designs which are used to answer research questions. In this article, we describe the systematic review, a type of secondary research design that is used to summarize the results of prior primary research studies. Systematic reviews are considered the highest level of ...
Evidence Synthesis Ireland: 'Studies Within A Review' (SWAR) programme of research is an exciting way to evaluate alternative options when conducting a review process (e.g. study selection, data extraction, reporting the findings) to provide much-needed evidence about how these steps in the review processes can be improved (Call closes: Sept 3)
Within this context, this review aimed to synthesise existing research focused on provide improvements on primary/secondary student assessment using some AI tool. Thus, nine original research studies (641 participants), published between 2010 and 2023, met the inclusion criteria defined in this systematic literature review.
A systematic review follows explicit methodology to answer a well-defined research question by searching the literature comprehensively, evaluating the quantity and quality of research evidence rigorously, and analyzing the evidence to synthesize an answer to the research question. The evidence gathered in systematic reviews can be qualitative ...