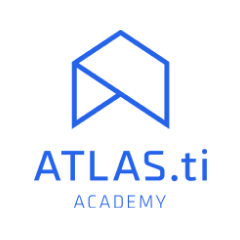

The Ultimate Guide to Qualitative Research - Part 1: The Basics
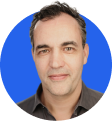
- Introduction and overview
- What is qualitative research?
- What is qualitative data?
- Examples of qualitative data
- Qualitative vs. quantitative research
- Mixed methods
- Qualitative research preparation
- Theoretical perspective
- Theoretical framework
- Literature reviews
Research question
- Conceptual framework
- Conceptual vs. theoretical framework
Data collection
- Qualitative research methods
- Focus groups
- Observational research
What is a case study?
Applications for case study research, what is a good case study, process of case study design, benefits and limitations of case studies.
- Ethnographical research
- Ethical considerations
- Confidentiality and privacy
- Power dynamics
- Reflexivity
Case studies
Case studies are essential to qualitative research , offering a lens through which researchers can investigate complex phenomena within their real-life contexts. This chapter explores the concept, purpose, applications, examples, and types of case studies and provides guidance on how to conduct case study research effectively.
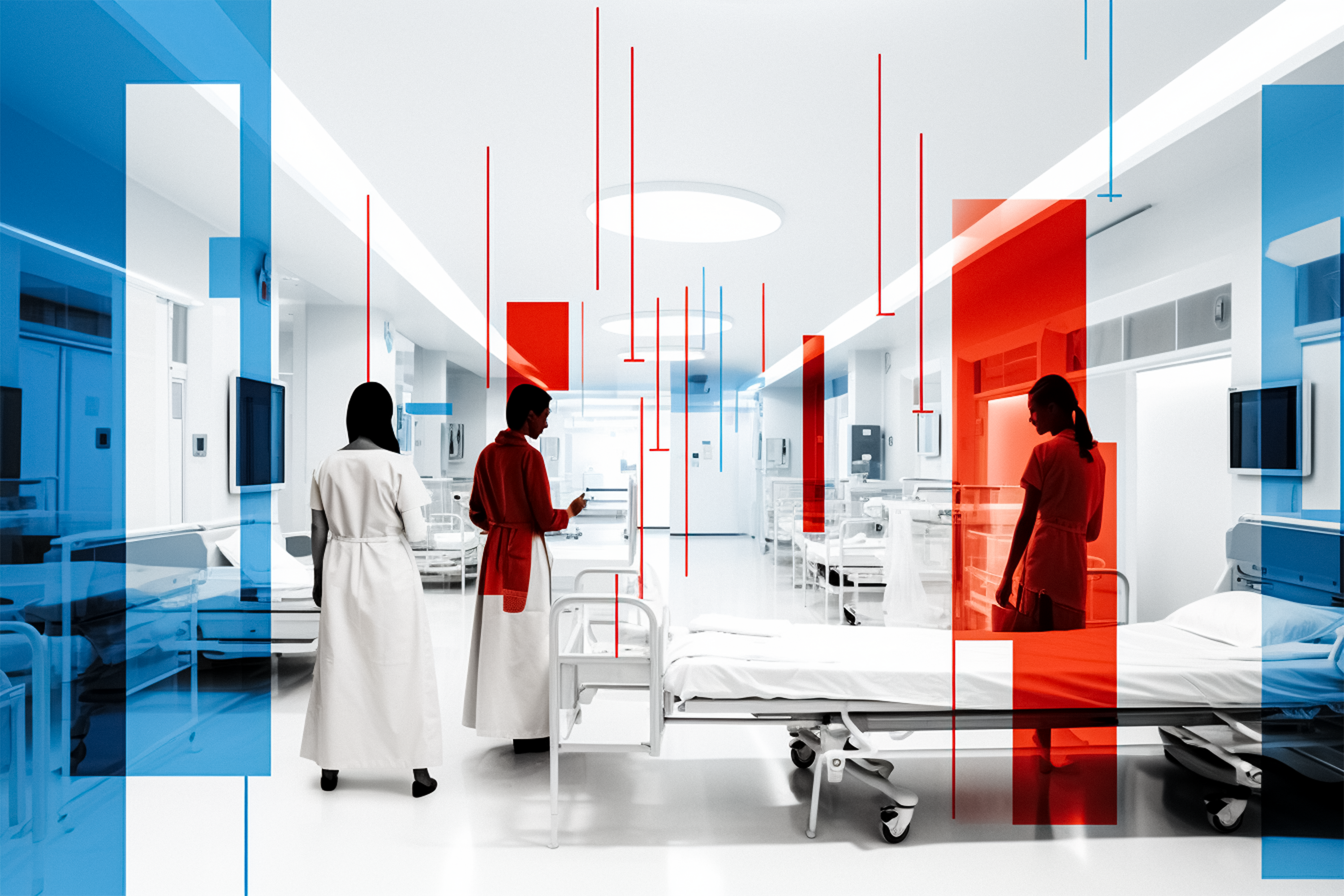
Whereas quantitative methods look at phenomena at scale, case study research looks at a concept or phenomenon in considerable detail. While analyzing a single case can help understand one perspective regarding the object of research inquiry, analyzing multiple cases can help obtain a more holistic sense of the topic or issue. Let's provide a basic definition of a case study, then explore its characteristics and role in the qualitative research process.
Definition of a case study
A case study in qualitative research is a strategy of inquiry that involves an in-depth investigation of a phenomenon within its real-world context. It provides researchers with the opportunity to acquire an in-depth understanding of intricate details that might not be as apparent or accessible through other methods of research. The specific case or cases being studied can be a single person, group, or organization – demarcating what constitutes a relevant case worth studying depends on the researcher and their research question .
Among qualitative research methods , a case study relies on multiple sources of evidence, such as documents, artifacts, interviews , or observations , to present a complete and nuanced understanding of the phenomenon under investigation. The objective is to illuminate the readers' understanding of the phenomenon beyond its abstract statistical or theoretical explanations.
Characteristics of case studies
Case studies typically possess a number of distinct characteristics that set them apart from other research methods. These characteristics include a focus on holistic description and explanation, flexibility in the design and data collection methods, reliance on multiple sources of evidence, and emphasis on the context in which the phenomenon occurs.
Furthermore, case studies can often involve a longitudinal examination of the case, meaning they study the case over a period of time. These characteristics allow case studies to yield comprehensive, in-depth, and richly contextualized insights about the phenomenon of interest.
The role of case studies in research
Case studies hold a unique position in the broader landscape of research methods aimed at theory development. They are instrumental when the primary research interest is to gain an intensive, detailed understanding of a phenomenon in its real-life context.
In addition, case studies can serve different purposes within research - they can be used for exploratory, descriptive, or explanatory purposes, depending on the research question and objectives. This flexibility and depth make case studies a valuable tool in the toolkit of qualitative researchers.
Remember, a well-conducted case study can offer a rich, insightful contribution to both academic and practical knowledge through theory development or theory verification, thus enhancing our understanding of complex phenomena in their real-world contexts.
What is the purpose of a case study?
Case study research aims for a more comprehensive understanding of phenomena, requiring various research methods to gather information for qualitative analysis . Ultimately, a case study can allow the researcher to gain insight into a particular object of inquiry and develop a theoretical framework relevant to the research inquiry.
Why use case studies in qualitative research?
Using case studies as a research strategy depends mainly on the nature of the research question and the researcher's access to the data.
Conducting case study research provides a level of detail and contextual richness that other research methods might not offer. They are beneficial when there's a need to understand complex social phenomena within their natural contexts.
The explanatory, exploratory, and descriptive roles of case studies
Case studies can take on various roles depending on the research objectives. They can be exploratory when the research aims to discover new phenomena or define new research questions; they are descriptive when the objective is to depict a phenomenon within its context in a detailed manner; and they can be explanatory if the goal is to understand specific relationships within the studied context. Thus, the versatility of case studies allows researchers to approach their topic from different angles, offering multiple ways to uncover and interpret the data .
The impact of case studies on knowledge development
Case studies play a significant role in knowledge development across various disciplines. Analysis of cases provides an avenue for researchers to explore phenomena within their context based on the collected data.
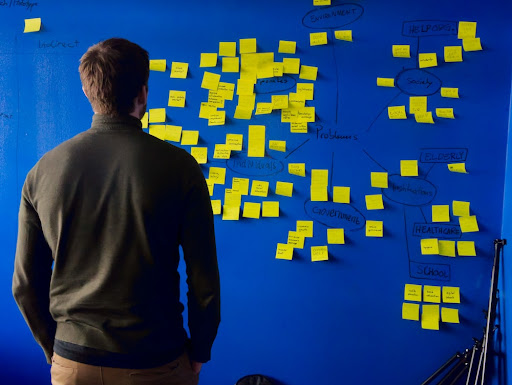
This can result in the production of rich, practical insights that can be instrumental in both theory-building and practice. Case studies allow researchers to delve into the intricacies and complexities of real-life situations, uncovering insights that might otherwise remain hidden.
Types of case studies
In qualitative research , a case study is not a one-size-fits-all approach. Depending on the nature of the research question and the specific objectives of the study, researchers might choose to use different types of case studies. These types differ in their focus, methodology, and the level of detail they provide about the phenomenon under investigation.
Understanding these types is crucial for selecting the most appropriate approach for your research project and effectively achieving your research goals. Let's briefly look at the main types of case studies.
Exploratory case studies
Exploratory case studies are typically conducted to develop a theory or framework around an understudied phenomenon. They can also serve as a precursor to a larger-scale research project. Exploratory case studies are useful when a researcher wants to identify the key issues or questions which can spur more extensive study or be used to develop propositions for further research. These case studies are characterized by flexibility, allowing researchers to explore various aspects of a phenomenon as they emerge, which can also form the foundation for subsequent studies.
Descriptive case studies
Descriptive case studies aim to provide a complete and accurate representation of a phenomenon or event within its context. These case studies are often based on an established theoretical framework, which guides how data is collected and analyzed. The researcher is concerned with describing the phenomenon in detail, as it occurs naturally, without trying to influence or manipulate it.
Explanatory case studies
Explanatory case studies are focused on explanation - they seek to clarify how or why certain phenomena occur. Often used in complex, real-life situations, they can be particularly valuable in clarifying causal relationships among concepts and understanding the interplay between different factors within a specific context.
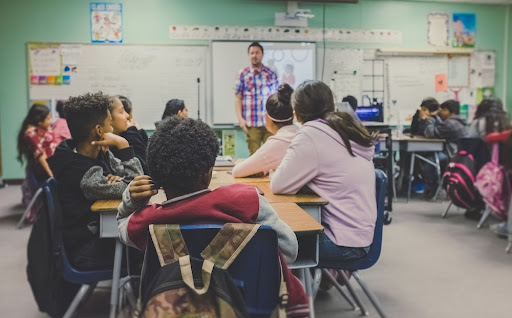
Intrinsic, instrumental, and collective case studies
These three categories of case studies focus on the nature and purpose of the study. An intrinsic case study is conducted when a researcher has an inherent interest in the case itself. Instrumental case studies are employed when the case is used to provide insight into a particular issue or phenomenon. A collective case study, on the other hand, involves studying multiple cases simultaneously to investigate some general phenomena.
Each type of case study serves a different purpose and has its own strengths and challenges. The selection of the type should be guided by the research question and objectives, as well as the context and constraints of the research.
The flexibility, depth, and contextual richness offered by case studies make this approach an excellent research method for various fields of study. They enable researchers to investigate real-world phenomena within their specific contexts, capturing nuances that other research methods might miss. Across numerous fields, case studies provide valuable insights into complex issues.
Critical information systems research
Case studies provide a detailed understanding of the role and impact of information systems in different contexts. They offer a platform to explore how information systems are designed, implemented, and used and how they interact with various social, economic, and political factors. Case studies in this field often focus on examining the intricate relationship between technology, organizational processes, and user behavior, helping to uncover insights that can inform better system design and implementation.
Health research
Health research is another field where case studies are highly valuable. They offer a way to explore patient experiences, healthcare delivery processes, and the impact of various interventions in a real-world context.
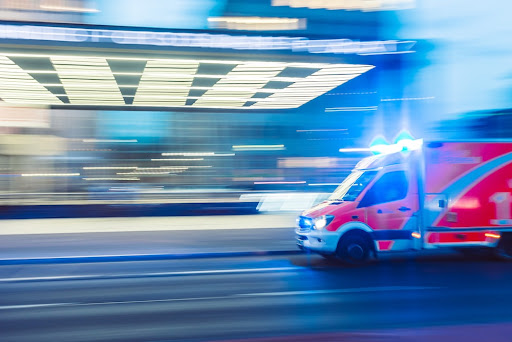
Case studies can provide a deep understanding of a patient's journey, giving insights into the intricacies of disease progression, treatment effects, and the psychosocial aspects of health and illness.
Asthma research studies
Specifically within medical research, studies on asthma often employ case studies to explore the individual and environmental factors that influence asthma development, management, and outcomes. A case study can provide rich, detailed data about individual patients' experiences, from the triggers and symptoms they experience to the effectiveness of various management strategies. This can be crucial for developing patient-centered asthma care approaches.
Other fields
Apart from the fields mentioned, case studies are also extensively used in business and management research, education research, and political sciences, among many others. They provide an opportunity to delve into the intricacies of real-world situations, allowing for a comprehensive understanding of various phenomena.
Case studies, with their depth and contextual focus, offer unique insights across these varied fields. They allow researchers to illuminate the complexities of real-life situations, contributing to both theory and practice.
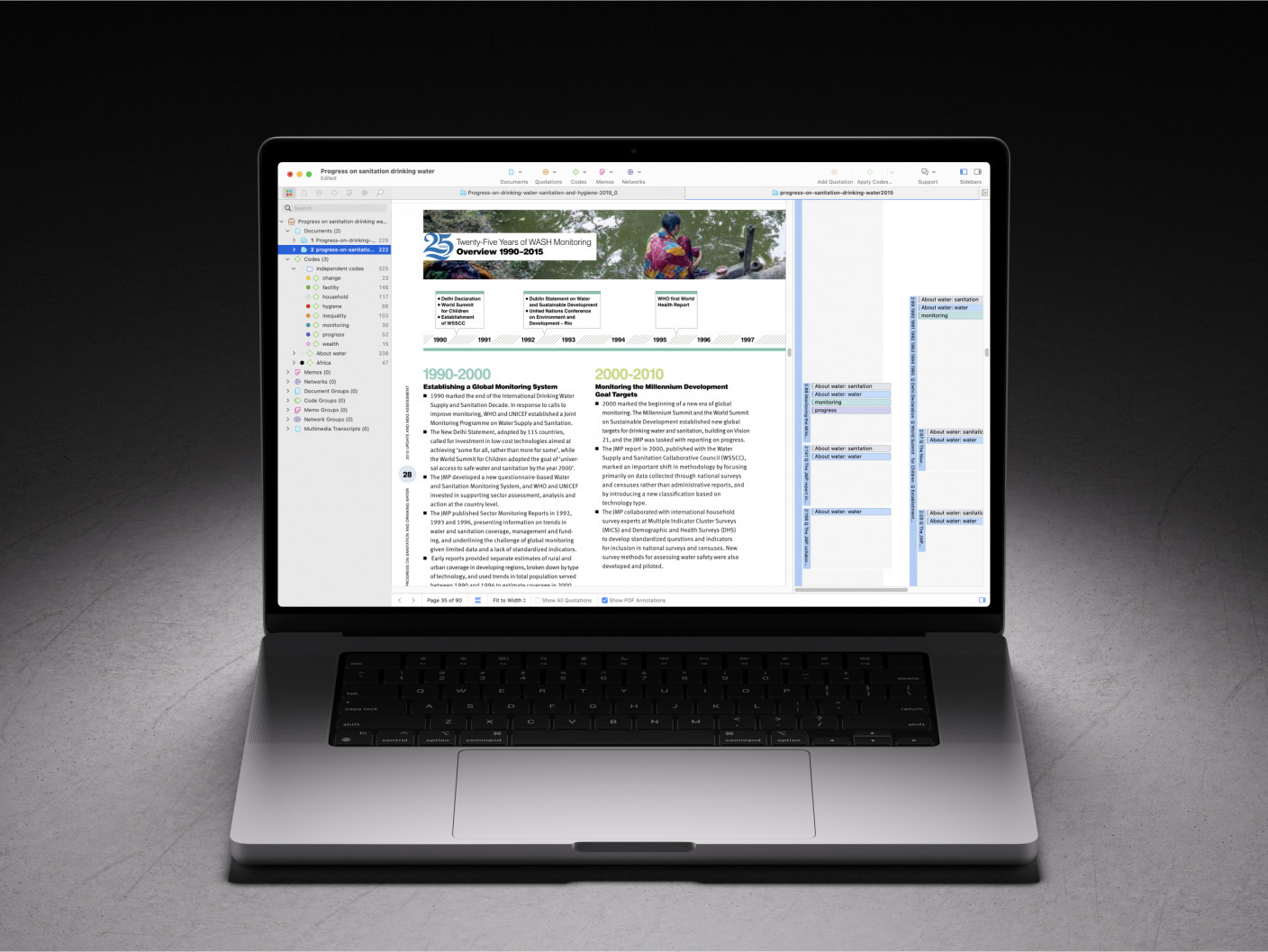
Whatever field you're in, ATLAS.ti puts your data to work for you
Download a free trial of ATLAS.ti to turn your data into insights.
Understanding the key elements of case study design is crucial for conducting rigorous and impactful case study research. A well-structured design guides the researcher through the process, ensuring that the study is methodologically sound and its findings are reliable and valid. The main elements of case study design include the research question , propositions, units of analysis, and the logic linking the data to the propositions.
The research question is the foundation of any research study. A good research question guides the direction of the study and informs the selection of the case, the methods of collecting data, and the analysis techniques. A well-formulated research question in case study research is typically clear, focused, and complex enough to merit further detailed examination of the relevant case(s).
Propositions
Propositions, though not necessary in every case study, provide a direction by stating what we might expect to find in the data collected. They guide how data is collected and analyzed by helping researchers focus on specific aspects of the case. They are particularly important in explanatory case studies, which seek to understand the relationships among concepts within the studied phenomenon.
Units of analysis
The unit of analysis refers to the case, or the main entity or entities that are being analyzed in the study. In case study research, the unit of analysis can be an individual, a group, an organization, a decision, an event, or even a time period. It's crucial to clearly define the unit of analysis, as it shapes the qualitative data analysis process by allowing the researcher to analyze a particular case and synthesize analysis across multiple case studies to draw conclusions.
Argumentation
This refers to the inferential model that allows researchers to draw conclusions from the data. The researcher needs to ensure that there is a clear link between the data, the propositions (if any), and the conclusions drawn. This argumentation is what enables the researcher to make valid and credible inferences about the phenomenon under study.
Understanding and carefully considering these elements in the design phase of a case study can significantly enhance the quality of the research. It can help ensure that the study is methodologically sound and its findings contribute meaningful insights about the case.
Ready to jumpstart your research with ATLAS.ti?
Conceptualize your research project with our intuitive data analysis interface. Download a free trial today.
Conducting a case study involves several steps, from defining the research question and selecting the case to collecting and analyzing data . This section outlines these key stages, providing a practical guide on how to conduct case study research.
Defining the research question
The first step in case study research is defining a clear, focused research question. This question should guide the entire research process, from case selection to analysis. It's crucial to ensure that the research question is suitable for a case study approach. Typically, such questions are exploratory or descriptive in nature and focus on understanding a phenomenon within its real-life context.
Selecting and defining the case
The selection of the case should be based on the research question and the objectives of the study. It involves choosing a unique example or a set of examples that provide rich, in-depth data about the phenomenon under investigation. After selecting the case, it's crucial to define it clearly, setting the boundaries of the case, including the time period and the specific context.
Previous research can help guide the case study design. When considering a case study, an example of a case could be taken from previous case study research and used to define cases in a new research inquiry. Considering recently published examples can help understand how to select and define cases effectively.
Developing a detailed case study protocol
A case study protocol outlines the procedures and general rules to be followed during the case study. This includes the data collection methods to be used, the sources of data, and the procedures for analysis. Having a detailed case study protocol ensures consistency and reliability in the study.
The protocol should also consider how to work with the people involved in the research context to grant the research team access to collecting data. As mentioned in previous sections of this guide, establishing rapport is an essential component of qualitative research as it shapes the overall potential for collecting and analyzing data.
Collecting data
Gathering data in case study research often involves multiple sources of evidence, including documents, archival records, interviews, observations, and physical artifacts. This allows for a comprehensive understanding of the case. The process for gathering data should be systematic and carefully documented to ensure the reliability and validity of the study.
Analyzing and interpreting data
The next step is analyzing the data. This involves organizing the data , categorizing it into themes or patterns , and interpreting these patterns to answer the research question. The analysis might also involve comparing the findings with prior research or theoretical propositions.
Writing the case study report
The final step is writing the case study report . This should provide a detailed description of the case, the data, the analysis process, and the findings. The report should be clear, organized, and carefully written to ensure that the reader can understand the case and the conclusions drawn from it.
Each of these steps is crucial in ensuring that the case study research is rigorous, reliable, and provides valuable insights about the case.
The type, depth, and quality of data in your study can significantly influence the validity and utility of the study. In case study research, data is usually collected from multiple sources to provide a comprehensive and nuanced understanding of the case. This section will outline the various methods of collecting data used in case study research and discuss considerations for ensuring the quality of the data.
Interviews are a common method of gathering data in case study research. They can provide rich, in-depth data about the perspectives, experiences, and interpretations of the individuals involved in the case. Interviews can be structured , semi-structured , or unstructured , depending on the research question and the degree of flexibility needed.
Observations
Observations involve the researcher observing the case in its natural setting, providing first-hand information about the case and its context. Observations can provide data that might not be revealed in interviews or documents, such as non-verbal cues or contextual information.
Documents and artifacts
Documents and archival records provide a valuable source of data in case study research. They can include reports, letters, memos, meeting minutes, email correspondence, and various public and private documents related to the case.
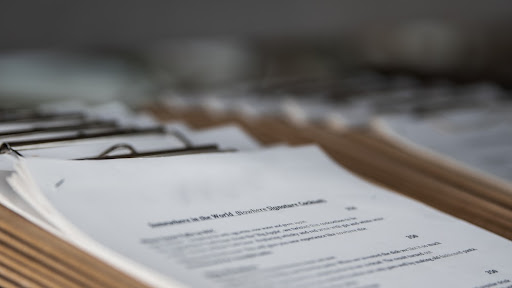
These records can provide historical context, corroborate evidence from other sources, and offer insights into the case that might not be apparent from interviews or observations.
Physical artifacts refer to any physical evidence related to the case, such as tools, products, or physical environments. These artifacts can provide tangible insights into the case, complementing the data gathered from other sources.
Ensuring the quality of data collection
Determining the quality of data in case study research requires careful planning and execution. It's crucial to ensure that the data is reliable, accurate, and relevant to the research question. This involves selecting appropriate methods of collecting data, properly training interviewers or observers, and systematically recording and storing the data. It also includes considering ethical issues related to collecting and handling data, such as obtaining informed consent and ensuring the privacy and confidentiality of the participants.
Data analysis
Analyzing case study research involves making sense of the rich, detailed data to answer the research question. This process can be challenging due to the volume and complexity of case study data. However, a systematic and rigorous approach to analysis can ensure that the findings are credible and meaningful. This section outlines the main steps and considerations in analyzing data in case study research.
Organizing the data
The first step in the analysis is organizing the data. This involves sorting the data into manageable sections, often according to the data source or the theme. This step can also involve transcribing interviews, digitizing physical artifacts, or organizing observational data.
Categorizing and coding the data
Once the data is organized, the next step is to categorize or code the data. This involves identifying common themes, patterns, or concepts in the data and assigning codes to relevant data segments. Coding can be done manually or with the help of software tools, and in either case, qualitative analysis software can greatly facilitate the entire coding process. Coding helps to reduce the data to a set of themes or categories that can be more easily analyzed.
Identifying patterns and themes
After coding the data, the researcher looks for patterns or themes in the coded data. This involves comparing and contrasting the codes and looking for relationships or patterns among them. The identified patterns and themes should help answer the research question.
Interpreting the data
Once patterns and themes have been identified, the next step is to interpret these findings. This involves explaining what the patterns or themes mean in the context of the research question and the case. This interpretation should be grounded in the data, but it can also involve drawing on theoretical concepts or prior research.
Verification of the data
The last step in the analysis is verification. This involves checking the accuracy and consistency of the analysis process and confirming that the findings are supported by the data. This can involve re-checking the original data, checking the consistency of codes, or seeking feedback from research participants or peers.
Like any research method , case study research has its strengths and limitations. Researchers must be aware of these, as they can influence the design, conduct, and interpretation of the study.
Understanding the strengths and limitations of case study research can also guide researchers in deciding whether this approach is suitable for their research question . This section outlines some of the key strengths and limitations of case study research.
Benefits include the following:
- Rich, detailed data: One of the main strengths of case study research is that it can generate rich, detailed data about the case. This can provide a deep understanding of the case and its context, which can be valuable in exploring complex phenomena.
- Flexibility: Case study research is flexible in terms of design , data collection , and analysis . A sufficient degree of flexibility allows the researcher to adapt the study according to the case and the emerging findings.
- Real-world context: Case study research involves studying the case in its real-world context, which can provide valuable insights into the interplay between the case and its context.
- Multiple sources of evidence: Case study research often involves collecting data from multiple sources , which can enhance the robustness and validity of the findings.
On the other hand, researchers should consider the following limitations:
- Generalizability: A common criticism of case study research is that its findings might not be generalizable to other cases due to the specificity and uniqueness of each case.
- Time and resource intensive: Case study research can be time and resource intensive due to the depth of the investigation and the amount of collected data.
- Complexity of analysis: The rich, detailed data generated in case study research can make analyzing the data challenging.
- Subjectivity: Given the nature of case study research, there may be a higher degree of subjectivity in interpreting the data , so researchers need to reflect on this and transparently convey to audiences how the research was conducted.
Being aware of these strengths and limitations can help researchers design and conduct case study research effectively and interpret and report the findings appropriately.
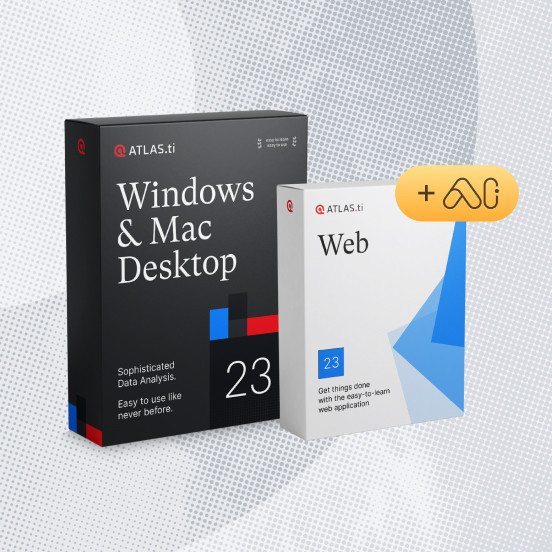
Ready to analyze your data with ATLAS.ti?
See how our intuitive software can draw key insights from your data with a free trial today.
- Search Menu
Sign in through your institution
- Browse content in Arts and Humanities
- Browse content in Archaeology
- Anglo-Saxon and Medieval Archaeology
- Archaeological Methodology and Techniques
- Archaeology by Region
- Archaeology of Religion
- Archaeology of Trade and Exchange
- Biblical Archaeology
- Contemporary and Public Archaeology
- Environmental Archaeology
- Historical Archaeology
- History and Theory of Archaeology
- Industrial Archaeology
- Landscape Archaeology
- Mortuary Archaeology
- Prehistoric Archaeology
- Underwater Archaeology
- Urban Archaeology
- Zooarchaeology
- Browse content in Architecture
- Architectural Structure and Design
- History of Architecture
- Residential and Domestic Buildings
- Theory of Architecture
- Browse content in Art
- Art Subjects and Themes
- History of Art
- Industrial and Commercial Art
- Theory of Art
- Biographical Studies
- Byzantine Studies
- Browse content in Classical Studies
- Classical Literature
- Classical Reception
- Classical History
- Classical Philosophy
- Classical Mythology
- Classical Art and Architecture
- Classical Oratory and Rhetoric
- Greek and Roman Papyrology
- Greek and Roman Archaeology
- Greek and Roman Epigraphy
- Greek and Roman Law
- Late Antiquity
- Religion in the Ancient World
- Digital Humanities
- Browse content in History
- Colonialism and Imperialism
- Diplomatic History
- Environmental History
- Genealogy, Heraldry, Names, and Honours
- Genocide and Ethnic Cleansing
- Historical Geography
- History by Period
- History of Emotions
- History of Agriculture
- History of Education
- History of Gender and Sexuality
- Industrial History
- Intellectual History
- International History
- Labour History
- Legal and Constitutional History
- Local and Family History
- Maritime History
- Military History
- National Liberation and Post-Colonialism
- Oral History
- Political History
- Public History
- Regional and National History
- Revolutions and Rebellions
- Slavery and Abolition of Slavery
- Social and Cultural History
- Theory, Methods, and Historiography
- Urban History
- World History
- Browse content in Language Teaching and Learning
- Language Learning (Specific Skills)
- Language Teaching Theory and Methods
- Browse content in Linguistics
- Applied Linguistics
- Cognitive Linguistics
- Computational Linguistics
- Forensic Linguistics
- Grammar, Syntax and Morphology
- Historical and Diachronic Linguistics
- History of English
- Language Evolution
- Language Reference
- Language Variation
- Language Families
- Language Acquisition
- Lexicography
- Linguistic Anthropology
- Linguistic Theories
- Linguistic Typology
- Phonetics and Phonology
- Psycholinguistics
- Sociolinguistics
- Translation and Interpretation
- Writing Systems
- Browse content in Literature
- Bibliography
- Children's Literature Studies
- Literary Studies (Romanticism)
- Literary Studies (American)
- Literary Studies (Modernism)
- Literary Studies (Asian)
- Literary Studies (European)
- Literary Studies (Eco-criticism)
- Literary Studies - World
- Literary Studies (1500 to 1800)
- Literary Studies (19th Century)
- Literary Studies (20th Century onwards)
- Literary Studies (African American Literature)
- Literary Studies (British and Irish)
- Literary Studies (Early and Medieval)
- Literary Studies (Fiction, Novelists, and Prose Writers)
- Literary Studies (Gender Studies)
- Literary Studies (Graphic Novels)
- Literary Studies (History of the Book)
- Literary Studies (Plays and Playwrights)
- Literary Studies (Poetry and Poets)
- Literary Studies (Postcolonial Literature)
- Literary Studies (Queer Studies)
- Literary Studies (Science Fiction)
- Literary Studies (Travel Literature)
- Literary Studies (War Literature)
- Literary Studies (Women's Writing)
- Literary Theory and Cultural Studies
- Mythology and Folklore
- Shakespeare Studies and Criticism
- Browse content in Media Studies
- Browse content in Music
- Applied Music
- Dance and Music
- Ethics in Music
- Ethnomusicology
- Gender and Sexuality in Music
- Medicine and Music
- Music Cultures
- Music and Media
- Music and Culture
- Music and Religion
- Music Education and Pedagogy
- Music Theory and Analysis
- Musical Scores, Lyrics, and Libretti
- Musical Structures, Styles, and Techniques
- Musicology and Music History
- Performance Practice and Studies
- Race and Ethnicity in Music
- Sound Studies
- Browse content in Performing Arts
- Browse content in Philosophy
- Aesthetics and Philosophy of Art
- Epistemology
- Feminist Philosophy
- History of Western Philosophy
- Metaphysics
- Moral Philosophy
- Non-Western Philosophy
- Philosophy of Language
- Philosophy of Mind
- Philosophy of Perception
- Philosophy of Action
- Philosophy of Law
- Philosophy of Religion
- Philosophy of Science
- Philosophy of Mathematics and Logic
- Practical Ethics
- Social and Political Philosophy
- Browse content in Religion
- Biblical Studies
- Christianity
- East Asian Religions
- History of Religion
- Judaism and Jewish Studies
- Qumran Studies
- Religion and Education
- Religion and Health
- Religion and Politics
- Religion and Science
- Religion and Law
- Religion and Art, Literature, and Music
- Religious Studies
- Browse content in Society and Culture
- Cookery, Food, and Drink
- Cultural Studies
- Customs and Traditions
- Ethical Issues and Debates
- Hobbies, Games, Arts and Crafts
- Lifestyle, Home, and Garden
- Natural world, Country Life, and Pets
- Popular Beliefs and Controversial Knowledge
- Sports and Outdoor Recreation
- Technology and Society
- Travel and Holiday
- Visual Culture
- Browse content in Law
- Arbitration
- Browse content in Company and Commercial Law
- Commercial Law
- Company Law
- Browse content in Comparative Law
- Systems of Law
- Competition Law
- Browse content in Constitutional and Administrative Law
- Government Powers
- Judicial Review
- Local Government Law
- Military and Defence Law
- Parliamentary and Legislative Practice
- Construction Law
- Contract Law
- Browse content in Criminal Law
- Criminal Procedure
- Criminal Evidence Law
- Sentencing and Punishment
- Employment and Labour Law
- Environment and Energy Law
- Browse content in Financial Law
- Banking Law
- Insolvency Law
- History of Law
- Human Rights and Immigration
- Intellectual Property Law
- Browse content in International Law
- Private International Law and Conflict of Laws
- Public International Law
- IT and Communications Law
- Jurisprudence and Philosophy of Law
- Law and Society
- Law and Politics
- Browse content in Legal System and Practice
- Courts and Procedure
- Legal Skills and Practice
- Primary Sources of Law
- Regulation of Legal Profession
- Medical and Healthcare Law
- Browse content in Policing
- Criminal Investigation and Detection
- Police and Security Services
- Police Procedure and Law
- Police Regional Planning
- Browse content in Property Law
- Personal Property Law
- Study and Revision
- Terrorism and National Security Law
- Browse content in Trusts Law
- Wills and Probate or Succession
- Browse content in Medicine and Health
- Browse content in Allied Health Professions
- Arts Therapies
- Clinical Science
- Dietetics and Nutrition
- Occupational Therapy
- Operating Department Practice
- Physiotherapy
- Radiography
- Speech and Language Therapy
- Browse content in Anaesthetics
- General Anaesthesia
- Neuroanaesthesia
- Clinical Neuroscience
- Browse content in Clinical Medicine
- Acute Medicine
- Cardiovascular Medicine
- Clinical Genetics
- Clinical Pharmacology and Therapeutics
- Dermatology
- Endocrinology and Diabetes
- Gastroenterology
- Genito-urinary Medicine
- Geriatric Medicine
- Infectious Diseases
- Medical Toxicology
- Medical Oncology
- Pain Medicine
- Palliative Medicine
- Rehabilitation Medicine
- Respiratory Medicine and Pulmonology
- Rheumatology
- Sleep Medicine
- Sports and Exercise Medicine
- Community Medical Services
- Critical Care
- Emergency Medicine
- Forensic Medicine
- Haematology
- History of Medicine
- Browse content in Medical Skills
- Clinical Skills
- Communication Skills
- Nursing Skills
- Surgical Skills
- Medical Ethics
- Browse content in Medical Dentistry
- Oral and Maxillofacial Surgery
- Paediatric Dentistry
- Restorative Dentistry and Orthodontics
- Surgical Dentistry
- Medical Statistics and Methodology
- Browse content in Neurology
- Clinical Neurophysiology
- Neuropathology
- Nursing Studies
- Browse content in Obstetrics and Gynaecology
- Gynaecology
- Occupational Medicine
- Ophthalmology
- Otolaryngology (ENT)
- Browse content in Paediatrics
- Neonatology
- Browse content in Pathology
- Chemical Pathology
- Clinical Cytogenetics and Molecular Genetics
- Histopathology
- Medical Microbiology and Virology
- Patient Education and Information
- Browse content in Pharmacology
- Psychopharmacology
- Browse content in Popular Health
- Caring for Others
- Complementary and Alternative Medicine
- Self-help and Personal Development
- Browse content in Preclinical Medicine
- Cell Biology
- Molecular Biology and Genetics
- Reproduction, Growth and Development
- Primary Care
- Professional Development in Medicine
- Browse content in Psychiatry
- Addiction Medicine
- Child and Adolescent Psychiatry
- Forensic Psychiatry
- Learning Disabilities
- Old Age Psychiatry
- Psychotherapy
- Browse content in Public Health and Epidemiology
- Epidemiology
- Public Health
- Browse content in Radiology
- Clinical Radiology
- Interventional Radiology
- Nuclear Medicine
- Radiation Oncology
- Reproductive Medicine
- Browse content in Surgery
- Cardiothoracic Surgery
- Gastro-intestinal and Colorectal Surgery
- General Surgery
- Neurosurgery
- Paediatric Surgery
- Peri-operative Care
- Plastic and Reconstructive Surgery
- Surgical Oncology
- Transplant Surgery
- Trauma and Orthopaedic Surgery
- Vascular Surgery
- Browse content in Science and Mathematics
- Browse content in Biological Sciences
- Aquatic Biology
- Biochemistry
- Bioinformatics and Computational Biology
- Developmental Biology
- Ecology and Conservation
- Evolutionary Biology
- Genetics and Genomics
- Microbiology
- Molecular and Cell Biology
- Natural History
- Plant Sciences and Forestry
- Research Methods in Life Sciences
- Structural Biology
- Systems Biology
- Zoology and Animal Sciences
- Browse content in Chemistry
- Analytical Chemistry
- Computational Chemistry
- Crystallography
- Environmental Chemistry
- Industrial Chemistry
- Inorganic Chemistry
- Materials Chemistry
- Medicinal Chemistry
- Mineralogy and Gems
- Organic Chemistry
- Physical Chemistry
- Polymer Chemistry
- Study and Communication Skills in Chemistry
- Theoretical Chemistry
- Browse content in Computer Science
- Artificial Intelligence
- Computer Architecture and Logic Design
- Game Studies
- Human-Computer Interaction
- Mathematical Theory of Computation
- Programming Languages
- Software Engineering
- Systems Analysis and Design
- Virtual Reality
- Browse content in Computing
- Business Applications
- Computer Games
- Computer Security
- Computer Networking and Communications
- Digital Lifestyle
- Graphical and Digital Media Applications
- Operating Systems
- Browse content in Earth Sciences and Geography
- Atmospheric Sciences
- Environmental Geography
- Geology and the Lithosphere
- Maps and Map-making
- Meteorology and Climatology
- Oceanography and Hydrology
- Palaeontology
- Physical Geography and Topography
- Regional Geography
- Soil Science
- Urban Geography
- Browse content in Engineering and Technology
- Agriculture and Farming
- Biological Engineering
- Civil Engineering, Surveying, and Building
- Electronics and Communications Engineering
- Energy Technology
- Engineering (General)
- Environmental Science, Engineering, and Technology
- History of Engineering and Technology
- Mechanical Engineering and Materials
- Technology of Industrial Chemistry
- Transport Technology and Trades
- Browse content in Environmental Science
- Applied Ecology (Environmental Science)
- Conservation of the Environment (Environmental Science)
- Environmental Sustainability
- Environmentalist Thought and Ideology (Environmental Science)
- Management of Land and Natural Resources (Environmental Science)
- Natural Disasters (Environmental Science)
- Nuclear Issues (Environmental Science)
- Pollution and Threats to the Environment (Environmental Science)
- Social Impact of Environmental Issues (Environmental Science)
- History of Science and Technology
- Browse content in Materials Science
- Ceramics and Glasses
- Composite Materials
- Metals, Alloying, and Corrosion
- Nanotechnology
- Browse content in Mathematics
- Applied Mathematics
- Biomathematics and Statistics
- History of Mathematics
- Mathematical Education
- Mathematical Finance
- Mathematical Analysis
- Numerical and Computational Mathematics
- Probability and Statistics
- Pure Mathematics
- Browse content in Neuroscience
- Cognition and Behavioural Neuroscience
- Development of the Nervous System
- Disorders of the Nervous System
- History of Neuroscience
- Invertebrate Neurobiology
- Molecular and Cellular Systems
- Neuroendocrinology and Autonomic Nervous System
- Neuroscientific Techniques
- Sensory and Motor Systems
- Browse content in Physics
- Astronomy and Astrophysics
- Atomic, Molecular, and Optical Physics
- Biological and Medical Physics
- Classical Mechanics
- Computational Physics
- Condensed Matter Physics
- Electromagnetism, Optics, and Acoustics
- History of Physics
- Mathematical and Statistical Physics
- Measurement Science
- Nuclear Physics
- Particles and Fields
- Plasma Physics
- Quantum Physics
- Relativity and Gravitation
- Semiconductor and Mesoscopic Physics
- Browse content in Psychology
- Affective Sciences
- Clinical Psychology
- Cognitive Psychology
- Cognitive Neuroscience
- Criminal and Forensic Psychology
- Developmental Psychology
- Educational Psychology
- Evolutionary Psychology
- Health Psychology
- History and Systems in Psychology
- Music Psychology
- Neuropsychology
- Organizational Psychology
- Psychological Assessment and Testing
- Psychology of Human-Technology Interaction
- Psychology Professional Development and Training
- Research Methods in Psychology
- Social Psychology
- Browse content in Social Sciences
- Browse content in Anthropology
- Anthropology of Religion
- Human Evolution
- Medical Anthropology
- Physical Anthropology
- Regional Anthropology
- Social and Cultural Anthropology
- Theory and Practice of Anthropology
- Browse content in Business and Management
- Business Ethics
- Business History
- Business Strategy
- Business and Technology
- Business and Government
- Business and the Environment
- Comparative Management
- Corporate Governance
- Corporate Social Responsibility
- Entrepreneurship
- Health Management
- Human Resource Management
- Industrial and Employment Relations
- Industry Studies
- Information and Communication Technologies
- International Business
- Knowledge Management
- Management and Management Techniques
- Operations Management
- Organizational Theory and Behaviour
- Pensions and Pension Management
- Public and Nonprofit Management
- Strategic Management
- Supply Chain Management
- Browse content in Criminology and Criminal Justice
- Criminal Justice
- Criminology
- Forms of Crime
- International and Comparative Criminology
- Youth Violence and Juvenile Justice
- Development Studies
- Browse content in Economics
- Agricultural, Environmental, and Natural Resource Economics
- Asian Economics
- Behavioural Finance
- Behavioural Economics and Neuroeconomics
- Econometrics and Mathematical Economics
- Economic History
- Economic Methodology
- Economic Systems
- Economic Development and Growth
- Financial Markets
- Financial Institutions and Services
- General Economics and Teaching
- Health, Education, and Welfare
- History of Economic Thought
- International Economics
- Labour and Demographic Economics
- Law and Economics
- Macroeconomics and Monetary Economics
- Microeconomics
- Public Economics
- Urban, Rural, and Regional Economics
- Welfare Economics
- Browse content in Education
- Adult Education and Continuous Learning
- Care and Counselling of Students
- Early Childhood and Elementary Education
- Educational Equipment and Technology
- Educational Strategies and Policy
- Higher and Further Education
- Organization and Management of Education
- Philosophy and Theory of Education
- Schools Studies
- Secondary Education
- Teaching of a Specific Subject
- Teaching of Specific Groups and Special Educational Needs
- Teaching Skills and Techniques
- Browse content in Environment
- Applied Ecology (Social Science)
- Climate Change
- Conservation of the Environment (Social Science)
- Environmentalist Thought and Ideology (Social Science)
- Natural Disasters (Environment)
- Social Impact of Environmental Issues (Social Science)
- Browse content in Human Geography
- Cultural Geography
- Economic Geography
- Political Geography
- Browse content in Interdisciplinary Studies
- Communication Studies
- Museums, Libraries, and Information Sciences
- Browse content in Politics
- African Politics
- Asian Politics
- Chinese Politics
- Comparative Politics
- Conflict Politics
- Elections and Electoral Studies
- Environmental Politics
- European Union
- Foreign Policy
- Gender and Politics
- Human Rights and Politics
- Indian Politics
- International Relations
- International Organization (Politics)
- International Political Economy
- Irish Politics
- Latin American Politics
- Middle Eastern Politics
- Political Behaviour
- Political Economy
- Political Institutions
- Political Theory
- Political Methodology
- Political Communication
- Political Philosophy
- Political Sociology
- Politics and Law
- Politics of Development
- Public Policy
- Public Administration
- Quantitative Political Methodology
- Regional Political Studies
- Russian Politics
- Security Studies
- State and Local Government
- UK Politics
- US Politics
- Browse content in Regional and Area Studies
- African Studies
- Asian Studies
- East Asian Studies
- Japanese Studies
- Latin American Studies
- Middle Eastern Studies
- Native American Studies
- Scottish Studies
- Browse content in Research and Information
- Research Methods
- Browse content in Social Work
- Addictions and Substance Misuse
- Adoption and Fostering
- Care of the Elderly
- Child and Adolescent Social Work
- Couple and Family Social Work
- Developmental and Physical Disabilities Social Work
- Direct Practice and Clinical Social Work
- Emergency Services
- Human Behaviour and the Social Environment
- International and Global Issues in Social Work
- Mental and Behavioural Health
- Social Justice and Human Rights
- Social Policy and Advocacy
- Social Work and Crime and Justice
- Social Work Macro Practice
- Social Work Practice Settings
- Social Work Research and Evidence-based Practice
- Welfare and Benefit Systems
- Browse content in Sociology
- Childhood Studies
- Community Development
- Comparative and Historical Sociology
- Economic Sociology
- Gender and Sexuality
- Gerontology and Ageing
- Health, Illness, and Medicine
- Marriage and the Family
- Migration Studies
- Occupations, Professions, and Work
- Organizations
- Population and Demography
- Race and Ethnicity
- Social Theory
- Social Movements and Social Change
- Social Research and Statistics
- Social Stratification, Inequality, and Mobility
- Sociology of Religion
- Sociology of Education
- Sport and Leisure
- Urban and Rural Studies
- Browse content in Warfare and Defence
- Defence Strategy, Planning, and Research
- Land Forces and Warfare
- Military Administration
- Military Life and Institutions
- Naval Forces and Warfare
- Other Warfare and Defence Issues
- Peace Studies and Conflict Resolution
- Weapons and Equipment

- < Previous chapter
- Next chapter >
6 Case Study and Selection
- Published: March 2024
- Cite Icon Cite
- Permissions Icon Permissions
This chapter presents and critically examines best practices for case study research and case selection. In reviewing prominent case study criteria that prioritize objective, analytical needs of research design (“appropriateness”), the chapter argues that overlooked considerations of feasibility (including positionality, resources, and skills) and interest play equally important roles when selecting and studying cases. The chapter concludes by arguing for an iterative process of research design, one that equally weighs pragmatic considerations and academic concerns of best fit. This strategy hopes to make qualitative research more accessible and transparent and to diversify the types of cases we study and questions we ask.
Signed in as
Institutional accounts.
- Google Scholar Indexing
- GoogleCrawler [DO NOT DELETE]
Personal account
- Sign in with email/username & password
- Get email alerts
- Save searches
- Purchase content
- Activate your purchase/trial code
- Add your ORCID iD
Institutional access
Sign in with a library card.
- Sign in with username/password
- Recommend to your librarian
- Institutional account management
- Get help with access
Access to content on Oxford Academic is often provided through institutional subscriptions and purchases. If you are a member of an institution with an active account, you may be able to access content in one of the following ways:
IP based access
Typically, access is provided across an institutional network to a range of IP addresses. This authentication occurs automatically, and it is not possible to sign out of an IP authenticated account.
Choose this option to get remote access when outside your institution. Shibboleth/Open Athens technology is used to provide single sign-on between your institution’s website and Oxford Academic.
- Click Sign in through your institution.
- Select your institution from the list provided, which will take you to your institution's website to sign in.
- When on the institution site, please use the credentials provided by your institution. Do not use an Oxford Academic personal account.
- Following successful sign in, you will be returned to Oxford Academic.
If your institution is not listed or you cannot sign in to your institution’s website, please contact your librarian or administrator.
Enter your library card number to sign in. If you cannot sign in, please contact your librarian.
Society Members
Society member access to a journal is achieved in one of the following ways:
Sign in through society site
Many societies offer single sign-on between the society website and Oxford Academic. If you see ‘Sign in through society site’ in the sign in pane within a journal:
- Click Sign in through society site.
- When on the society site, please use the credentials provided by that society. Do not use an Oxford Academic personal account.
If you do not have a society account or have forgotten your username or password, please contact your society.
Sign in using a personal account
Some societies use Oxford Academic personal accounts to provide access to their members. See below.
A personal account can be used to get email alerts, save searches, purchase content, and activate subscriptions.
Some societies use Oxford Academic personal accounts to provide access to their members.
Viewing your signed in accounts
Click the account icon in the top right to:
- View your signed in personal account and access account management features.
- View the institutional accounts that are providing access.
Signed in but can't access content
Oxford Academic is home to a wide variety of products. The institutional subscription may not cover the content that you are trying to access. If you believe you should have access to that content, please contact your librarian.
For librarians and administrators, your personal account also provides access to institutional account management. Here you will find options to view and activate subscriptions, manage institutional settings and access options, access usage statistics, and more.
Our books are available by subscription or purchase to libraries and institutions.
- About Oxford Academic
- Publish journals with us
- University press partners
- What we publish
- New features
- Open access
- Rights and permissions
- Accessibility
- Advertising
- Media enquiries
- Oxford University Press
- Oxford Languages
- University of Oxford
Oxford University Press is a department of the University of Oxford. It furthers the University's objective of excellence in research, scholarship, and education by publishing worldwide
- Copyright © 2024 Oxford University Press
- Cookie settings
- Cookie policy
- Privacy policy
- Legal notice
This Feature Is Available To Subscribers Only
Sign In or Create an Account
This PDF is available to Subscribers Only
For full access to this pdf, sign in to an existing account, or purchase an annual subscription.
Case Selection Techniques in Case Study Research: A Menu of Qualitative and Quantitative Options
- Related Documents
Case Selection for Case‐Study Analysis: Qualitative and Quantitative Techniques
This article presents some guidance by cataloging nine different techniques for case selection: typical, diverse, extreme, deviant, influential, crucial, pathway, most similar, and most different. It also indicates that if the researcher is starting from a quantitative database, then methods for finding influential outliers can be used. In particular, the article clarifies the general principles that might guide the process of case selection in case-study research. Cases are more or less representative of some broader phenomenon and, on that score, may be considered better or worse subjects for intensive analysis. The article then draws attention to two ambiguities in case-selection strategies in case-study research. The first concerns the admixture of several case-selection strategies. The second concerns the changing status of a case as a study proceeds. Some case studies follow only one strategy of case selection.
Case Selection and Hypothesis Testing
This chapter discusses quantitative and qualitative practices of case-study selection when the goal of the analysis is to evaluate causal hypotheses. More specifically, it considers how the different causal models used in the qualitative and quantitative research cultures shape the kind of cases that provide the most leverage for hypothesis testing. The chapter examines whether one should select cases based on their value on the dependent variable. It also evaluates the kinds of cases that provide the most leverage for causal inference when conducting case-study research. It shows that differences in research goals between quantitative and qualitative scholars yield distinct ideas about best strategies of case selection. Qualitative research places emphasis on explaining particular cases; quantitative research does not.
Paying Attention to the Trees in the Forest, or a Call to Examine Agency-Specific Stories
Public administration scholarship needs to strike a better balance between large sample studies and in-depth case studies. The availability of large data sets has led us to engage in empirical research that is broad in scope but is frequently devoid of rich context. In-depth case studies can help to explain why we observe particular relationships and can help us to clarify gaps and inconsistencies in theory. Our argument for more case studies aims to encourage researchers to bridge insights from qualitative and quantitative research through triangulation. We describe the value of case study research, and qualitative and quantitative design options. We then propose opportunities for case study research in public personnel scholarship on patronage pressures, performance management, and diversity management.
Case Selection Techniques in Case Study Research
Blending the focused ethnographic method and case study research: implications regarding case selection and generalization of results.
In this article, we present the benefits of blending the methodological characteristics of the focused ethnographic method (FEM) and case study research (CSR) for a study on auxiliary work processes in a hospital in Barcelona, Spain. We argue that incorporating CSR logic and principles in the FEM produces a better form of inquiry, as this combination improves the quality of data and the ability to make comparisons in addition to enhancing the transferability of findings. These better outcomes are achieved because the characteristics of the case study methodological design reinforce many of the strengths of the FEM, such as its thematic concreteness and its targeted data collection. The role played by the theoretical framework of the study thus makes it easier to focus on the key variables of the initial theoretical model and to introduce a logic of multiple case comparison.
Qualitative Case Study Research Design
Qualitative case study research can be a valuable tool for answering complex, real-world questions. This method is often misunderstood or neglected due to a lack of understanding by researchers and reviewers. This tutorial defines the characteristics of qualitative case study research and its application to a broader understanding of stuttering that cannot be defined through other methodologies. This article will describe ways that data can be collected and analyzed.
Intersecting Mixed Methods and Case Study Research: Design Possibilities and Challenges
Robert k. yin. (2014). case study research design and methods (5th ed.). thousand oaks, ca: sage. 282 pages., cooperative banks & local government units - areas of collaboration (poland case study - research results), from the ‘frying-pan’ into the ‘fire’ antidotes to confirmatory bias in case-study research, export citation format, share document.
Organizing Your Social Sciences Research Assignments
- Annotated Bibliography
- Analyzing a Scholarly Journal Article
- Group Presentations
- Dealing with Nervousness
- Using Visual Aids
- Grading Someone Else's Paper
- Types of Structured Group Activities
- Group Project Survival Skills
- Leading a Class Discussion
- Multiple Book Review Essay
- Reviewing Collected Works
- Writing a Case Analysis Paper
- Writing a Case Study
- About Informed Consent
- Writing Field Notes
- Writing a Policy Memo
- Writing a Reflective Paper
- Writing a Research Proposal
- Generative AI and Writing
- Acknowledgments
A case study research paper examines a person, place, event, condition, phenomenon, or other type of subject of analysis in order to extrapolate key themes and results that help predict future trends, illuminate previously hidden issues that can be applied to practice, and/or provide a means for understanding an important research problem with greater clarity. A case study research paper usually examines a single subject of analysis, but case study papers can also be designed as a comparative investigation that shows relationships between two or more subjects. The methods used to study a case can rest within a quantitative, qualitative, or mixed-method investigative paradigm.
Case Studies. Writing@CSU. Colorado State University; Mills, Albert J. , Gabrielle Durepos, and Eiden Wiebe, editors. Encyclopedia of Case Study Research . Thousand Oaks, CA: SAGE Publications, 2010 ; “What is a Case Study?” In Swanborn, Peter G. Case Study Research: What, Why and How? London: SAGE, 2010.
How to Approach Writing a Case Study Research Paper
General information about how to choose a topic to investigate can be found under the " Choosing a Research Problem " tab in the Organizing Your Social Sciences Research Paper writing guide. Review this page because it may help you identify a subject of analysis that can be investigated using a case study design.
However, identifying a case to investigate involves more than choosing the research problem . A case study encompasses a problem contextualized around the application of in-depth analysis, interpretation, and discussion, often resulting in specific recommendations for action or for improving existing conditions. As Seawright and Gerring note, practical considerations such as time and access to information can influence case selection, but these issues should not be the sole factors used in describing the methodological justification for identifying a particular case to study. Given this, selecting a case includes considering the following:
- The case represents an unusual or atypical example of a research problem that requires more in-depth analysis? Cases often represent a topic that rests on the fringes of prior investigations because the case may provide new ways of understanding the research problem. For example, if the research problem is to identify strategies to improve policies that support girl's access to secondary education in predominantly Muslim nations, you could consider using Azerbaijan as a case study rather than selecting a more obvious nation in the Middle East. Doing so may reveal important new insights into recommending how governments in other predominantly Muslim nations can formulate policies that support improved access to education for girls.
- The case provides important insight or illuminate a previously hidden problem? In-depth analysis of a case can be based on the hypothesis that the case study will reveal trends or issues that have not been exposed in prior research or will reveal new and important implications for practice. For example, anecdotal evidence may suggest drug use among homeless veterans is related to their patterns of travel throughout the day. Assuming prior studies have not looked at individual travel choices as a way to study access to illicit drug use, a case study that observes a homeless veteran could reveal how issues of personal mobility choices facilitate regular access to illicit drugs. Note that it is important to conduct a thorough literature review to ensure that your assumption about the need to reveal new insights or previously hidden problems is valid and evidence-based.
- The case challenges and offers a counter-point to prevailing assumptions? Over time, research on any given topic can fall into a trap of developing assumptions based on outdated studies that are still applied to new or changing conditions or the idea that something should simply be accepted as "common sense," even though the issue has not been thoroughly tested in current practice. A case study analysis may offer an opportunity to gather evidence that challenges prevailing assumptions about a research problem and provide a new set of recommendations applied to practice that have not been tested previously. For example, perhaps there has been a long practice among scholars to apply a particular theory in explaining the relationship between two subjects of analysis. Your case could challenge this assumption by applying an innovative theoretical framework [perhaps borrowed from another discipline] to explore whether this approach offers new ways of understanding the research problem. Taking a contrarian stance is one of the most important ways that new knowledge and understanding develops from existing literature.
- The case provides an opportunity to pursue action leading to the resolution of a problem? Another way to think about choosing a case to study is to consider how the results from investigating a particular case may result in findings that reveal ways in which to resolve an existing or emerging problem. For example, studying the case of an unforeseen incident, such as a fatal accident at a railroad crossing, can reveal hidden issues that could be applied to preventative measures that contribute to reducing the chance of accidents in the future. In this example, a case study investigating the accident could lead to a better understanding of where to strategically locate additional signals at other railroad crossings so as to better warn drivers of an approaching train, particularly when visibility is hindered by heavy rain, fog, or at night.
- The case offers a new direction in future research? A case study can be used as a tool for an exploratory investigation that highlights the need for further research about the problem. A case can be used when there are few studies that help predict an outcome or that establish a clear understanding about how best to proceed in addressing a problem. For example, after conducting a thorough literature review [very important!], you discover that little research exists showing the ways in which women contribute to promoting water conservation in rural communities of east central Africa. A case study of how women contribute to saving water in a rural village of Uganda can lay the foundation for understanding the need for more thorough research that documents how women in their roles as cooks and family caregivers think about water as a valuable resource within their community. This example of a case study could also point to the need for scholars to build new theoretical frameworks around the topic [e.g., applying feminist theories of work and family to the issue of water conservation].
Eisenhardt, Kathleen M. “Building Theories from Case Study Research.” Academy of Management Review 14 (October 1989): 532-550; Emmel, Nick. Sampling and Choosing Cases in Qualitative Research: A Realist Approach . Thousand Oaks, CA: SAGE Publications, 2013; Gerring, John. “What Is a Case Study and What Is It Good for?” American Political Science Review 98 (May 2004): 341-354; Mills, Albert J. , Gabrielle Durepos, and Eiden Wiebe, editors. Encyclopedia of Case Study Research . Thousand Oaks, CA: SAGE Publications, 2010; Seawright, Jason and John Gerring. "Case Selection Techniques in Case Study Research." Political Research Quarterly 61 (June 2008): 294-308.
Structure and Writing Style
The purpose of a paper in the social sciences designed around a case study is to thoroughly investigate a subject of analysis in order to reveal a new understanding about the research problem and, in so doing, contributing new knowledge to what is already known from previous studies. In applied social sciences disciplines [e.g., education, social work, public administration, etc.], case studies may also be used to reveal best practices, highlight key programs, or investigate interesting aspects of professional work.
In general, the structure of a case study research paper is not all that different from a standard college-level research paper. However, there are subtle differences you should be aware of. Here are the key elements to organizing and writing a case study research paper.
I. Introduction
As with any research paper, your introduction should serve as a roadmap for your readers to ascertain the scope and purpose of your study . The introduction to a case study research paper, however, should not only describe the research problem and its significance, but you should also succinctly describe why the case is being used and how it relates to addressing the problem. The two elements should be linked. With this in mind, a good introduction answers these four questions:
- What is being studied? Describe the research problem and describe the subject of analysis [the case] you have chosen to address the problem. Explain how they are linked and what elements of the case will help to expand knowledge and understanding about the problem.
- Why is this topic important to investigate? Describe the significance of the research problem and state why a case study design and the subject of analysis that the paper is designed around is appropriate in addressing the problem.
- What did we know about this topic before I did this study? Provide background that helps lead the reader into the more in-depth literature review to follow. If applicable, summarize prior case study research applied to the research problem and why it fails to adequately address the problem. Describe why your case will be useful. If no prior case studies have been used to address the research problem, explain why you have selected this subject of analysis.
- How will this study advance new knowledge or new ways of understanding? Explain why your case study will be suitable in helping to expand knowledge and understanding about the research problem.
Each of these questions should be addressed in no more than a few paragraphs. Exceptions to this can be when you are addressing a complex research problem or subject of analysis that requires more in-depth background information.
II. Literature Review
The literature review for a case study research paper is generally structured the same as it is for any college-level research paper. The difference, however, is that the literature review is focused on providing background information and enabling historical interpretation of the subject of analysis in relation to the research problem the case is intended to address . This includes synthesizing studies that help to:
- Place relevant works in the context of their contribution to understanding the case study being investigated . This would involve summarizing studies that have used a similar subject of analysis to investigate the research problem. If there is literature using the same or a very similar case to study, you need to explain why duplicating past research is important [e.g., conditions have changed; prior studies were conducted long ago, etc.].
- Describe the relationship each work has to the others under consideration that informs the reader why this case is applicable . Your literature review should include a description of any works that support using the case to investigate the research problem and the underlying research questions.
- Identify new ways to interpret prior research using the case study . If applicable, review any research that has examined the research problem using a different research design. Explain how your use of a case study design may reveal new knowledge or a new perspective or that can redirect research in an important new direction.
- Resolve conflicts amongst seemingly contradictory previous studies . This refers to synthesizing any literature that points to unresolved issues of concern about the research problem and describing how the subject of analysis that forms the case study can help resolve these existing contradictions.
- Point the way in fulfilling a need for additional research . Your review should examine any literature that lays a foundation for understanding why your case study design and the subject of analysis around which you have designed your study may reveal a new way of approaching the research problem or offer a perspective that points to the need for additional research.
- Expose any gaps that exist in the literature that the case study could help to fill . Summarize any literature that not only shows how your subject of analysis contributes to understanding the research problem, but how your case contributes to a new way of understanding the problem that prior research has failed to do.
- Locate your own research within the context of existing literature [very important!] . Collectively, your literature review should always place your case study within the larger domain of prior research about the problem. The overarching purpose of reviewing pertinent literature in a case study paper is to demonstrate that you have thoroughly identified and synthesized prior studies in relation to explaining the relevance of the case in addressing the research problem.
III. Method
In this section, you explain why you selected a particular case [i.e., subject of analysis] and the strategy you used to identify and ultimately decide that your case was appropriate in addressing the research problem. The way you describe the methods used varies depending on the type of subject of analysis that constitutes your case study.
If your subject of analysis is an incident or event . In the social and behavioral sciences, the event or incident that represents the case to be studied is usually bounded by time and place, with a clear beginning and end and with an identifiable location or position relative to its surroundings. The subject of analysis can be a rare or critical event or it can focus on a typical or regular event. The purpose of studying a rare event is to illuminate new ways of thinking about the broader research problem or to test a hypothesis. Critical incident case studies must describe the method by which you identified the event and explain the process by which you determined the validity of this case to inform broader perspectives about the research problem or to reveal new findings. However, the event does not have to be a rare or uniquely significant to support new thinking about the research problem or to challenge an existing hypothesis. For example, Walo, Bull, and Breen conducted a case study to identify and evaluate the direct and indirect economic benefits and costs of a local sports event in the City of Lismore, New South Wales, Australia. The purpose of their study was to provide new insights from measuring the impact of a typical local sports event that prior studies could not measure well because they focused on large "mega-events." Whether the event is rare or not, the methods section should include an explanation of the following characteristics of the event: a) when did it take place; b) what were the underlying circumstances leading to the event; and, c) what were the consequences of the event in relation to the research problem.
If your subject of analysis is a person. Explain why you selected this particular individual to be studied and describe what experiences they have had that provide an opportunity to advance new understandings about the research problem. Mention any background about this person which might help the reader understand the significance of their experiences that make them worthy of study. This includes describing the relationships this person has had with other people, institutions, and/or events that support using them as the subject for a case study research paper. It is particularly important to differentiate the person as the subject of analysis from others and to succinctly explain how the person relates to examining the research problem [e.g., why is one politician in a particular local election used to show an increase in voter turnout from any other candidate running in the election]. Note that these issues apply to a specific group of people used as a case study unit of analysis [e.g., a classroom of students].
If your subject of analysis is a place. In general, a case study that investigates a place suggests a subject of analysis that is unique or special in some way and that this uniqueness can be used to build new understanding or knowledge about the research problem. A case study of a place must not only describe its various attributes relevant to the research problem [e.g., physical, social, historical, cultural, economic, political], but you must state the method by which you determined that this place will illuminate new understandings about the research problem. It is also important to articulate why a particular place as the case for study is being used if similar places also exist [i.e., if you are studying patterns of homeless encampments of veterans in open spaces, explain why you are studying Echo Park in Los Angeles rather than Griffith Park?]. If applicable, describe what type of human activity involving this place makes it a good choice to study [e.g., prior research suggests Echo Park has more homeless veterans].
If your subject of analysis is a phenomenon. A phenomenon refers to a fact, occurrence, or circumstance that can be studied or observed but with the cause or explanation to be in question. In this sense, a phenomenon that forms your subject of analysis can encompass anything that can be observed or presumed to exist but is not fully understood. In the social and behavioral sciences, the case usually focuses on human interaction within a complex physical, social, economic, cultural, or political system. For example, the phenomenon could be the observation that many vehicles used by ISIS fighters are small trucks with English language advertisements on them. The research problem could be that ISIS fighters are difficult to combat because they are highly mobile. The research questions could be how and by what means are these vehicles used by ISIS being supplied to the militants and how might supply lines to these vehicles be cut off? How might knowing the suppliers of these trucks reveal larger networks of collaborators and financial support? A case study of a phenomenon most often encompasses an in-depth analysis of a cause and effect that is grounded in an interactive relationship between people and their environment in some way.
NOTE: The choice of the case or set of cases to study cannot appear random. Evidence that supports the method by which you identified and chose your subject of analysis should clearly support investigation of the research problem and linked to key findings from your literature review. Be sure to cite any studies that helped you determine that the case you chose was appropriate for examining the problem.
IV. Discussion
The main elements of your discussion section are generally the same as any research paper, but centered around interpreting and drawing conclusions about the key findings from your analysis of the case study. Note that a general social sciences research paper may contain a separate section to report findings. However, in a paper designed around a case study, it is common to combine a description of the results with the discussion about their implications. The objectives of your discussion section should include the following:
Reiterate the Research Problem/State the Major Findings Briefly reiterate the research problem you are investigating and explain why the subject of analysis around which you designed the case study were used. You should then describe the findings revealed from your study of the case using direct, declarative, and succinct proclamation of the study results. Highlight any findings that were unexpected or especially profound.
Explain the Meaning of the Findings and Why They are Important Systematically explain the meaning of your case study findings and why you believe they are important. Begin this part of the section by repeating what you consider to be your most important or surprising finding first, then systematically review each finding. Be sure to thoroughly extrapolate what your analysis of the case can tell the reader about situations or conditions beyond the actual case that was studied while, at the same time, being careful not to misconstrue or conflate a finding that undermines the external validity of your conclusions.
Relate the Findings to Similar Studies No study in the social sciences is so novel or possesses such a restricted focus that it has absolutely no relation to previously published research. The discussion section should relate your case study results to those found in other studies, particularly if questions raised from prior studies served as the motivation for choosing your subject of analysis. This is important because comparing and contrasting the findings of other studies helps support the overall importance of your results and it highlights how and in what ways your case study design and the subject of analysis differs from prior research about the topic.
Consider Alternative Explanations of the Findings Remember that the purpose of social science research is to discover and not to prove. When writing the discussion section, you should carefully consider all possible explanations revealed by the case study results, rather than just those that fit your hypothesis or prior assumptions and biases. Be alert to what the in-depth analysis of the case may reveal about the research problem, including offering a contrarian perspective to what scholars have stated in prior research if that is how the findings can be interpreted from your case.
Acknowledge the Study's Limitations You can state the study's limitations in the conclusion section of your paper but describing the limitations of your subject of analysis in the discussion section provides an opportunity to identify the limitations and explain why they are not significant. This part of the discussion section should also note any unanswered questions or issues your case study could not address. More detailed information about how to document any limitations to your research can be found here .
Suggest Areas for Further Research Although your case study may offer important insights about the research problem, there are likely additional questions related to the problem that remain unanswered or findings that unexpectedly revealed themselves as a result of your in-depth analysis of the case. Be sure that the recommendations for further research are linked to the research problem and that you explain why your recommendations are valid in other contexts and based on the original assumptions of your study.
V. Conclusion
As with any research paper, you should summarize your conclusion in clear, simple language; emphasize how the findings from your case study differs from or supports prior research and why. Do not simply reiterate the discussion section. Provide a synthesis of key findings presented in the paper to show how these converge to address the research problem. If you haven't already done so in the discussion section, be sure to document the limitations of your case study and any need for further research.
The function of your paper's conclusion is to: 1) reiterate the main argument supported by the findings from your case study; 2) state clearly the context, background, and necessity of pursuing the research problem using a case study design in relation to an issue, controversy, or a gap found from reviewing the literature; and, 3) provide a place to persuasively and succinctly restate the significance of your research problem, given that the reader has now been presented with in-depth information about the topic.
Consider the following points to help ensure your conclusion is appropriate:
- If the argument or purpose of your paper is complex, you may need to summarize these points for your reader.
- If prior to your conclusion, you have not yet explained the significance of your findings or if you are proceeding inductively, use the conclusion of your paper to describe your main points and explain their significance.
- Move from a detailed to a general level of consideration of the case study's findings that returns the topic to the context provided by the introduction or within a new context that emerges from your case study findings.
Note that, depending on the discipline you are writing in or the preferences of your professor, the concluding paragraph may contain your final reflections on the evidence presented as it applies to practice or on the essay's central research problem. However, the nature of being introspective about the subject of analysis you have investigated will depend on whether you are explicitly asked to express your observations in this way.
Problems to Avoid
Overgeneralization One of the goals of a case study is to lay a foundation for understanding broader trends and issues applied to similar circumstances. However, be careful when drawing conclusions from your case study. They must be evidence-based and grounded in the results of the study; otherwise, it is merely speculation. Looking at a prior example, it would be incorrect to state that a factor in improving girls access to education in Azerbaijan and the policy implications this may have for improving access in other Muslim nations is due to girls access to social media if there is no documentary evidence from your case study to indicate this. There may be anecdotal evidence that retention rates were better for girls who were engaged with social media, but this observation would only point to the need for further research and would not be a definitive finding if this was not a part of your original research agenda.
Failure to Document Limitations No case is going to reveal all that needs to be understood about a research problem. Therefore, just as you have to clearly state the limitations of a general research study , you must describe the specific limitations inherent in the subject of analysis. For example, the case of studying how women conceptualize the need for water conservation in a village in Uganda could have limited application in other cultural contexts or in areas where fresh water from rivers or lakes is plentiful and, therefore, conservation is understood more in terms of managing access rather than preserving access to a scarce resource.
Failure to Extrapolate All Possible Implications Just as you don't want to over-generalize from your case study findings, you also have to be thorough in the consideration of all possible outcomes or recommendations derived from your findings. If you do not, your reader may question the validity of your analysis, particularly if you failed to document an obvious outcome from your case study research. For example, in the case of studying the accident at the railroad crossing to evaluate where and what types of warning signals should be located, you failed to take into consideration speed limit signage as well as warning signals. When designing your case study, be sure you have thoroughly addressed all aspects of the problem and do not leave gaps in your analysis that leave the reader questioning the results.
Case Studies. Writing@CSU. Colorado State University; Gerring, John. Case Study Research: Principles and Practices . New York: Cambridge University Press, 2007; Merriam, Sharan B. Qualitative Research and Case Study Applications in Education . Rev. ed. San Francisco, CA: Jossey-Bass, 1998; Miller, Lisa L. “The Use of Case Studies in Law and Social Science Research.” Annual Review of Law and Social Science 14 (2018): TBD; Mills, Albert J., Gabrielle Durepos, and Eiden Wiebe, editors. Encyclopedia of Case Study Research . Thousand Oaks, CA: SAGE Publications, 2010; Putney, LeAnn Grogan. "Case Study." In Encyclopedia of Research Design , Neil J. Salkind, editor. (Thousand Oaks, CA: SAGE Publications, 2010), pp. 116-120; Simons, Helen. Case Study Research in Practice . London: SAGE Publications, 2009; Kratochwill, Thomas R. and Joel R. Levin, editors. Single-Case Research Design and Analysis: New Development for Psychology and Education . Hilldsale, NJ: Lawrence Erlbaum Associates, 1992; Swanborn, Peter G. Case Study Research: What, Why and How? London : SAGE, 2010; Yin, Robert K. Case Study Research: Design and Methods . 6th edition. Los Angeles, CA, SAGE Publications, 2014; Walo, Maree, Adrian Bull, and Helen Breen. “Achieving Economic Benefits at Local Events: A Case Study of a Local Sports Event.” Festival Management and Event Tourism 4 (1996): 95-106.
Writing Tip
At Least Five Misconceptions about Case Study Research
Social science case studies are often perceived as limited in their ability to create new knowledge because they are not randomly selected and findings cannot be generalized to larger populations. Flyvbjerg examines five misunderstandings about case study research and systematically "corrects" each one. To quote, these are:
Misunderstanding 1 : General, theoretical [context-independent] knowledge is more valuable than concrete, practical [context-dependent] knowledge. Misunderstanding 2 : One cannot generalize on the basis of an individual case; therefore, the case study cannot contribute to scientific development. Misunderstanding 3 : The case study is most useful for generating hypotheses; that is, in the first stage of a total research process, whereas other methods are more suitable for hypotheses testing and theory building. Misunderstanding 4 : The case study contains a bias toward verification, that is, a tendency to confirm the researcher’s preconceived notions. Misunderstanding 5 : It is often difficult to summarize and develop general propositions and theories on the basis of specific case studies [p. 221].
While writing your paper, think introspectively about how you addressed these misconceptions because to do so can help you strengthen the validity and reliability of your research by clarifying issues of case selection, the testing and challenging of existing assumptions, the interpretation of key findings, and the summation of case outcomes. Think of a case study research paper as a complete, in-depth narrative about the specific properties and key characteristics of your subject of analysis applied to the research problem.
Flyvbjerg, Bent. “Five Misunderstandings About Case-Study Research.” Qualitative Inquiry 12 (April 2006): 219-245.
- << Previous: Writing a Case Analysis Paper
- Next: Writing a Field Report >>
- Last Updated: May 7, 2024 9:45 AM
- URL: https://libguides.usc.edu/writingguide/assignments

An official website of the United States government
The .gov means it’s official. Federal government websites often end in .gov or .mil. Before sharing sensitive information, make sure you’re on a federal government site.
The site is secure. The https:// ensures that you are connecting to the official website and that any information you provide is encrypted and transmitted securely.
- Publications
- Account settings
Preview improvements coming to the PMC website in October 2024. Learn More or Try it out now .
- Advanced Search
- Journal List

Case selection and causal inferences in qualitative comparative research
Thomas plümper.
1 Department of Socioeconomics, Vienna University of Economics and Business, Vienna, Austria
Vera E. Troeger
2 Department of Economics, University of Warwick, Coventry, United Kingdom
Eric Neumayer
3 Department of Geography and Environment, London School of Economics and Political Science, London, United Kingdom
Associated Data
Replication files for the Monte Carlo simulations can be accessed here: Troeger, Vera Eva; Plümper, Thomas; Neumayer, Eric, 2017, "Replication Data for: Case selection and causal inferences in qualitative comparative research", doi: 10.7910/DVN/3H5EDP , Harvard Dataverse, V1.
Traditionally, social scientists perceived causality as regularity. As a consequence, qualitative comparative case study research was regarded as unsuitable for drawing causal inferences since a few cases cannot establish regularity. The dominant perception of causality has changed, however. Nowadays, social scientists define and identify causality through the counterfactual effect of a treatment. This brings causal inference in qualitative comparative research back on the agenda since comparative case studies can identify counterfactual treatment effects. We argue that the validity of causal inferences from the comparative study of cases depends on the employed case-selection algorithm. We employ Monte Carlo techniques to demonstrate that different case-selection rules strongly differ in their ex ante reliability for making valid causal inferences and identify the most and the least reliable case selection rules.
Introduction
We demonstrate that the validity of causal inferences based on the qualitative comparison of cases depends on the data-generating process and on the choice of case-selection algorithm. While the first factor is beyond the influence of scientists, researchers can freely choose the algorithm that determines the selection of cases. Of course, methodologists have long since been aware of the importance of case-selection for qualitative comparative research [ 1 , 2 , 3 ]. One can trace back systematic theoretical and methodological reasoning on case-selection to at least John Stuart Mill [ 4 ]. After all this time, one might expect that the optimal case-selection algorithms are known. Yet, this is only partially the case. We offer one of the first rigorous analyses of the relative performance of both simple and more complex case-selection rules under conditions of relevance to real world comparative research [ 5 ].
Specifically, we vary the size of the total set of cases from which specific cases are selected, we vary the degrees to which the causal factor of interest is correlated with confounding factors, and we vary the “signal-to-noise ratio”, that is, the (relative) strength of the effect of the causal factor of interest. Using a Monte Carlo design we compare the relative performance of 11 case-selection algorithms, partly following suggestions of qualitative methodologists and partly derived from common practice in comparative case analyses. The very best case-selection algorithm results in an estimated average effect that is almost a hundred times closer to the true effect than the worst algorithm. We also evaluate the conditions conducive to higher validity of causal inferences from qualitative comparative research. We find that the best selection algorithms exhibit relatively high ex ante reliability for making valid inferences if: a) the explanatory variable of interest exerts a strong influence on the dependent variable relative to random noise and confounding factors, b) the variable of interest is not too strongly correlated with confounding variables, and c) the dependent variable is not dichotomous. More importantly, while the best algorithms are still fairly reliable even in the presence of strong stochastic influences on the dependent variable and other complications, the worst algorithms are highly unreliable even if the conditions are met under which qualitative comparative research works best.
Our research contributes to both qualitative and quantitative methodological debates. Quantitative researchers assume that it is impossible to derive valid causal inferences from qualitative comparative research methods. However, we argue that this assumption is outdated because the concept of causality as regularity [ 6 , 4 , 7 ] has been superseded by the concept of causality as counterfactual effect [ 8 , 9 , 10 ]. In fact, the counterfactual concept of causation requires only a single case for causal inference if only it were possible to observe the counterfactual [ 11 , 12 , 13 ]. In the absence of directly observable counterfactual outcomes, the closest methodological equivalents according to the ‘identification school’ are randomization of treatment [ 14 ] and stratification of treatment and control group [ 15 ] through case-selection. It is this latter research strategy of rule- or model-based case-selection that demands a re-evaluation of qualitative comparative designs.
The logic of causal inference typically invoked by quantitative methodologists therefore also applies to qualitative comparative methods: if two or more cases are identical in all relevant dimensions but vary in the treatment, causal inference is internally valid. In addition, our research demonstrates that if these two cases are sampled so that the difference in the treatment is maximized the precision of the computed causal effect is large. We understand of course that these optimal conditions often do not exist and that selected cases vary in more dimensions than the treatment effect. Analyzing how different case-selection rules perform as a function of different conditions in which they must operate is exactly the purpose of our contribution.
As for the debate amongst qualitative methodologists, our results first and foremost speak to qualitative comparative researchers who, in the tradition of John Stuart Mill, draw inferences from the comparison of two sufficiently similar cases that vary in respect to the variable of interest (the ‘treatment’). Yet, the research design logic supported by our results also applies to scholars who compare a single case at two or more different points in time with a ‘treatment’ occurring in between the first and the last observation of the selected single case. These research designs are comparative in nature, and thus our findings that inferences are most likely to be valid if researchers maximize the variance of the variable of interest and minimize the variance of the confounding factors for selecting the case or cases they analyze over time also holds for a comparison of two different observations in time of a single case.
Yet, our research also contrasts with some of the acquired wisdom of qualitative methodologists. We agree that qualitative research, including the in-depth study of one or more cases and the comparative study of cases, can serve many other purposes and are, arguably, better suited for inductive purposes such as theory and concept development [ 16 , 17 ]. Qualitative research often seeks to generate ideas about the data-generating process so that little knowledge of the data-generating process can be assumed to exist prior to the case selection. Clearly, the logic of case selection for deductive causal inference research differs from the logic of case selection for inductive research. We therefore do not believe that our results can or indeed should be extended to inductive research. Importantly, however, many empirical qualitative researchers do make causal inferences and generalize their findings from the analyzed cases to a broader population. Our analysis enables those qualitative researchers who do wish to make causal inferences based on the comparative analysis of cases to understand how case-selection rules differ with respect to their ex ante reliability for detecting the direction and strength of a causal effect. Crucially, given limited knowledge about the data-generating process, we show that the relatively best-performing algorithms remain best-performing no matter what the underlying data-generating process (of those we have analyzed).
Qualitative researchers might struggle with a second aspect of our research design. Qualitative comparative researchers hardly ever estimate the strength of an effect and thus an analysis of effect strengths must seem irrelevant for them (but see [ 18 ]). Yet, we do not compute the effect strength from a comparison of two cases to tempt qualitative researchers to quantify effect strengths. We merely compute the effect strength and compare it to the assumed true effect size to have an indicator against which we can judge the ex ante reliability of selection algorithms. Computing the effect size is a tool, not the goal. Even if qualitative comparative researchers only intend to make inferences on the direction of a causal effect, they should agree that the expected deviation of an implied effect strength estimate from the truth–called root mean squared error by the quantitative tribe–is a good indicator for the relative ex ante reliability of case-selection algorithms: The larger this deviation, the more likely that even the inferred direction of an effect is wrong.
The paper is organized as follows: the next section shows that the now dominant modern concept of causality as counterfactual analysis implies that one can make causal inferences based on qualitative comparative analysis. One cannot make such inferences with certainty, however, and the validity of inferences will crucially depend on how cases are selected. We review what methodologists have advised on the selection of cases in qualitative comparative research in section 3. This informs our choice of selection algorithms that we subject to Monte Carlo analysis, though we also add some original algorithms to test whether and, if so, how much better they can perform. Section 4 describes these algorithms, the Monte Carlo design and how we evaluate the relative performance of the case-selection algorithms. Section 5 presents results from the Monte Carlo simulations.
Causal inference and qualitative comparative research
Causality as regularity dominated the philosophy of science at least from Hume to Popper. Hume [ 5 ] argued that scientists cannot have knowledge of causality beyond observed regularities in associations of events. He therefore suggests inferring causality through a systematic comparison of situations in which the presumed causal factor is present or absent, or varies in strength. The concept of causality as regularity became the central element of Hempel and Oppenheim’s [ 19 ] deductive-nomological model of scientific explanation. Hempel was also the first to develop the concept further to include statistical inference [ 20 ]. In Popper’s conception of a non-degenerative research program [ 7 ], a single falsification effectively leads to the rejection of the tested hypothesis or, worse, the theory from which the hypothesis derives. The “regularity” perspective culminates in the definition of science as “unbroken, natural regularity” [ 21 ].
This “strict regularity” concept of causality had ambiguous implications for comparative social science qualitative researchers’ ability to make causal inferences. On the one hand, the analysis of a small number of cases cannot establish regularity. On the other hand, if, conversely, a single deviant case suffices to refute a causal claim or even a theory, as Popper believes, then strength in numbers does not exist [ 22 , 23 , 17 ]. The “strict regularity” perspective is dead, however, because a) not all regularities are causal (“correlation is not causation”) and b) causality can be probabilistic rather than deterministic and can thus exist without strict regularity.
Probabilistic causal mechanisms paved the way for an interpretation of regularity as statistical regularity. Yet, not even the brilliant idea of statistical inference saved the regularity concept of causality. If correlation is not causality, then high correlation does not imply causality either and low correlation and statistical insignificance may indicate low-probability causality and a lack of sufficient variation rather than the absence of causality. Eventually, this insight eliminated the support for the causality as regularity view.
Over the last three decades, the concept of causality as regularity was replaced by the counterfactual concept of causality, also called the potential outcomes framework. Its understanding of causality is tautological: causality exists if a cause exerts a causal effect on the outcome, and a cause exerts a causal effect on the outcome when the relation is causal. This tautology seems to be the main reason why scholars advancing the counterfactual perspective [ 9 , 10 , 24 , 25 ] focus on causal inference and the identification of causal effects rather than on causality itself [ 24 ].
According to the counterfactual concept of causality, causality is perfectly identified if one observes the outcome given treatment and the outcome given no treatment at the same time for the same person(s). Naturally, this is impossible. Hence, a counterfactual analysis starts with a ‘missing data’ problem and then immediately turns to ‘second-best’ options for inferring causality. If one cannot observe the potential or counterfactual outcome for any one single case, then one needs to resort to comparing the outcomes of different cases. This raises the challenge that either one must make sure that the cases compared are equal or sufficiently similar in all dimensions that matter or that one can render the influence of all potential confounders irrelevant. Otherwise, no causal effect has been ‘identified’.
The approach generally preferred by identification scholars–what they call the “gold standard”–aspires to render potential confounders irrelevant by randomizing treatment across a large number of cases in a controlled experiment (but see [ 25 , 26 ]). Though practically all actual experiments fall way short of the ideal of experimental designs, the randomization of treatments in a sample where N approaches infinity guarantees that the treatment will be uncorrelated with both observable and, crucially, unobservable confounders. Because of this lack of correlation with any potential confounder, any observable difference in outcomes between the two groups must be due to the treatment. If one assumes causal homogeneity among cases and assumes away that potential confounders might condition the effect of treatment, then ideal experiments will not only have identified a cause-effect relationship but will also allow the calculation of the unbiased effect size.
Clearly, from the experimentalist viewpoint, qualitative small-N comparative research is useless for causal inferences. In fact, so is everything else. Its diehard proponents explicitly argue that experiments are a necessary condition for causal inference. For example, Light, Singer, and Willett [ 27 ] claim that “to establish a causal link, you must conduct an experiment (…). Only experimental inquiries allow you to determine whether a treatment causes an outcome to change.” This claim wrongly assumes that identification is a necessary condition for causal inference, whereas in fact perfect identification is only a necessary condition for making causal inferences that are valid with certainty. The idea that one can only make causal inferences if scientists are certain about having identified a cause-effect relationship via experiments is absurd, however. If the claim was correct, scientists would not be able to infer that more education causes higher lifetime income, or that smoking causes lung cancer. For that matter, social scientists would not be able to explore much of interest. The quest for causal inference in the social sciences is not about certainty; it is about how to deal with uncertainty and how much uncertainty about the validity of inferences can be tolerated.
More importantly, making certainty a prerequisite for causal inference runs into a logical problem for the social sciences because experiments that social scientists are able to conduct do not generate inferences that are valid with certainty. Even ignoring causal heterogeneity and potential conditionalities [ 28 ], the confounding-factors problem can only be solved asymptotically, that is, by increasing the sample size to infinity. With a finite number of participants, randomization of treatment does not suffice to render treatment uncorrelated to unobserved confounders like mood, experience, knowledge, or intelligence, and often to even observed confounders like age, sex, income, or education. As a remedy, many experimenters control for observable differences in addition to randomizing treatment. Since it is impossible to control for all factors that influence human behavior, not least because some of them may be unobserved, the problem of confounders can be reduced but not eliminated by experiments. Yet, if experiments only increase the probability that causal inferences are correct, then the strict dichotomy between experiments and all other research methods that Light, Singer, and Willett make is unjustified.
The second approach to solving the “missing data” problem in the counterfactual concept of causality argues that causal effects are identified if cases can be selected so as to guarantee that all the relevant properties of the treatment group exactly match the properties of the control group [ 29 , 30 , 31 ]. Identification via selection or matching on the properties of the treatment and control groups requires perfect knowledge of all the factors that influence outcomes and also that one can match cases on these properties. As with experiments, falling short of this ideal will mean that a causal effect has not been identified with certainty, but does not render causal inference impossible. For experimentalists, matching is far inferior to experiments because they doubt one can know all the relevant properties (one can know the so-called data-generating process) and even if one could know these properties, one cannot measure all of these properties, some of which are unobservable, and thus one cannot match on them.
This second approach substitutes impossible counterfactual analyses with a possible analysis of cases that have been carefully selected to be homogeneous with respect to confounding variables. This strategy is obviously encouraging for causal inference based on case comparison. Nothing in this matching approach suggests that the validity of causal inferences depends on the number of cases. If cases are homogeneous, causal inferences based on small-N qualitative comparative methods become possible, and the validity of these causal inferences depends on the employed selection rule.
Qualitative comparative researchers have always made arguments that closely resemble matching [ 5 ]: if two cases are identical in all relevant dimensions but vary in the dimension of interest (the treatment), then it is possible to directly infer causality and to compute a causal effect size. This possibility does not imply that causal inference from qualitative comparative research is optimal or easy, however. Of course, there is the issue of knowing all relevant dimensions and finding at least two cases which are identical in all these dimensions. There are other difficulties, too: First, if causal processes are stochastic, as they are bound to be, then a single small-N comparative analysis, which cannot control for noise and random errors, will not reveal the truth but some random deviation from the truth. Matching cases in a quantitative analysis with large N therefore can be superior—though the greater difficulty of adequately matching a larger number of cases means that any positive effect on the validity of causal inferences from efficiency gains may be defeated by the negative effect due to problems in matching. Second, perfect homogeneity among cases on all confounding factors can only be achieved if researchers know the true data-generating process, which is unlikely to be the case even if qualitative researchers argue that their in-depth study of cases allow them to know much more about this process than quantitative researchers do [ 32 , 33 ]. In the absence of knowledge of the true data-generating process, qualitative comparative researchers should make sure that selected cases do not differ in respect to known strong confounding factors. The potential for bias grows with the strength of the potentially confounding factor (for which no controls have been included), and the size of the correlation between the variable of interest and the confounder.
Case-selection and qualitative comparisons
Methodological advice on the selection of cases in qualitative research stands in a long tradition. John Stuart Mill in his A System of Logic , first published in 1843, proposed five methods meant to enable researchers to make causal inferences: the method of agreement, the method of difference, the double method of agreement and difference, the method of residues, and the method of concomitant variation [ 4 ]. Methodologists have questioned and criticized the usefulness and general applicability of Mill’s methods [ 34 , 35 ]. However, without doubt Mill’s proposals had a major and lasting impact on the development of the two most prominent modern methods, namely the “most similar” and “most different” comparative case-study designs [ 1 , 36 , 37 ].
Yet, as Seawright and Gerring [ 3 ] point out, these and other methods of case-selection are “poorly understood and often misapplied”. Qualitative researchers mean very different things when they invoke the same terms “most similar” or “most different” and usually the description of their research design is not precise enough to allow readers to assess exactly how cases have been chosen. Seawright and Gerring have therefore provided a formal definition and classification of these and other techniques of case-selection. They [ 3 ] suggest that “in its purest form” the “most similar” design chooses cases which appear to be identical on all controls ( z ) but different in the variable of interest ( x ). Lijphart [ 1 ] suggested what might be regarded a variant of this method that asks researchers to maximize “the ratio between the variance of the operative variables and the variance of the control variables”.
Naturally, the “most similar” technique is not easily applied because researchers find it difficult to match cases such that they are identical on all control variables. As Seawright and Gerring [ 3 ] concede: “Unfortunately, in most observational studies, the matching procedure described previously–known as exact matching–is impossible.” This impossibility has three sources: first, researchers usually do not know the true model and thus cannot match on all control variables. Second, even if known to affect the dependent variable, many variables remain unobserved. And third, even if all necessary pieces of information are available, two cases that are identical in all excluded variables may not exist.
Qualitative comparative researchers prefer the “most similar” technique, despite ambiguity in its definition and practical operationalization, to its main rival, the “most different” design. Seawright and Gerring [ 3 ] believe that this dominance of “most similar” over “most different” design is well justified. Defining the “most different” technique as choosing two cases that are identical in the outcome y and in the main variable of interest x but different in all control variables z , they argue that this technique does not generate much leverage. They criticize three points: first, the chosen cases never represent the entire population (if x can in fact vary in the population). Second, the lack of variation in x renders it impossible to identify causal effects. And third, elimination of rival hypotheses is impossible. As Gerring [ 38 ] formulates poignantly: “There is little point in pursuing cross-unit analysis if the units in question do not exhibit variation on the dimensions of theoretical interest and/or the researcher cannot manage to hold other, potentially confounding, factors constant.”
For comparative case studies, Seawright and Gerring also identify a third selection technique, which they label the “diverse” technique. It selects cases so as to “represent the full range of values characterizing X, Y, or some particular X/Y relationship” [ 3 ]. This definition is somewhat ambiguous and vague (“some particular relationship”), but one of the selection algorithms used below in our MC simulations captures the essence of this technique by simultaneously maximizing variation in y and x .
Perhaps surprisingly, King, Keohane and Verba’s [ 39 ] seminal contribution to qualitative research methodology discusses case-selection only from the perspective of unit homogeneity–broadly understood as constant effect assumption–and selection bias–defined as non-random selection of cases that are not statistically representative of the population. Selecting cases in a way that does not avoid selection bias negatively affects the generalizability of inferences. Random sampling from the population of cases would clearly avoid selection bias. Thus, given the prominence of selection bias in King et al.’s discussion of case-selection, the absence of random sampling in comparative research may appear surprising. But it is not. Random selection of cases leads to inferences which are correct on average when the number of conducted case studies approaches infinity, but the sampling deviation is extremely large. As a consequence, the reliability of single studies of randomly sampled cases remains low. The advice King and his co-authors give on case-selection, then, lends additional credibility to commonly chosen practices by qualitative comparative researchers, namely to avoid truncation of the dependent variable, to avoid selection on the dependent variable, while at the same time selecting according to the categories of the “key causal explanatory variable”. King et al. [ 39 ] also repeatedly claim that increasing the number of observations makes causal inferences more reliable. Qualitative methodologists have argued that this view, while correct in principle, does not do justice to qualitative research [ 40 , 41 , 42 ]. More importantly, they also suggest that the extent to which the logic of quantitative research can be superimposed on qualitative research designs has limits.
While there is a growing consensus on the importance of case-selection for comparative research, as yet very little overall agreement has emerged concerning the use of central terminology and the relative advantages of different case-selection rules. Scholars largely agree that random sampling is unsuitable for qualitative comparative research (but see [ 5 ]), but disagreement on sampling on the dependent variable, and the appropriate use of information from observable confounding factors persists. Our Monte Carlo analysis will shed light on this issue by exploring which selection algorithms are best suited under a variety of assumptions about the data-generating process.
A Monte Carlo analysis of case-selection algorithms
In statistics, Monte Carlo experiments are employed to compare the performance of estimators. The term Monte Carlo experiments describes a broad set of techniques that randomly draw values from a probability distribution to add error to a predefined equation that serves as data-generating process. Since the truth is known, it is straightforward to compare the estimated or computed effects to the true effects. An estimator performs the better the smaller the average distance between the estimated effect and the truth. This average distance is usually called the root mean squared error.
Our Monte Carlo experiments follow this common practice in statistics and merely replace the estimators by a case-selection rule or algorithm. We compare selection rules commonly used in applied qualitative comparative research, as well as various simple permutations and extensions. Without loss of generality, we assume a data-generating process in which the dependent variable y is a linear function of a variable of interest x , a control variable z and an error term ε . Since we can interpret z as a vector of k control variables, we can generalize findings to analyses with multiple controls.
Case-selection algorithms
Ignoring for the time being standard advice against sampling on the dependent variable, researchers might wish to maximize variation of y , maximize variation of x , minimize variation of z or some combination thereof. Employing addition and subtraction, the two most basic functions to aggregate information on more than one variable, leads to seven permutations of information from which to choose; together with random sampling this results in eight simple case-selection algorithms–see Table 1 . The mathematical description of the selection algorithms, as shown in the last column of the table, relies on the set-up of the Monte Carlo analyses (described in the next section). In general, for each variable we generate Euclidean distance matrices, which are N×N matrices representing the difference or distance in a set of cases i and j forming the case-dyad ij . Starting from these distance matrices, we select two cases that follow a specific selection rule. For example, max(x) only considers the explanatory variable of interest, thereby ignoring the distance matrices for the dependent variable y and the control variable z . With max(x) , we select the two cases that represent the cell of the distance matrix with the largest distance value. We refrain from analyzing case-selection algorithms for qualitative research with more than two cases. Note, however, that all major results we show here carry over to selecting more than two cases based on a single algorithm. However, we do not yet know whether all our results carry over to analyses of more than two cases when researchers select cases based on different algorithms–a topic we will revisit in future research.
Algorithm 1 does not use information (other than that a case belongs to the population), and samples cases randomly. We include this algorithm for completeness and because qualitative methodologists argue that random sampling–the gold standard for sampling in quantitative research–does not work well in small-N comparative research.
We incorporate the second algorithm–pure sampling on the dependent variable without regard to variation of either x or z –for the same completeness reason. Echoing Geddes [ 43 ], many scholars have argued that sampling on the dependent variable biases the results [ 39 , 44 , 45 ]. Geddes demonstrates that “selecting on the dependent variable” lies at the core of invalid results generated from qualitative comparative research in fields as diverse as economic development, social revolution, and inflation.
But does Geddes’s compelling critique of sampling on the dependent variable imply that applied researchers should entirely ignore information on the dependent variable when they also use information on the variable of interest or the confounding factors? Algorithms 5, 6, and 8 help us to explore this question. These rules include selection on the dependent variable in addition to selection on x and/or z . Theoretically, these algorithms should perform better than the algorithm 2, but we are more interested in analyzing how these biased algorithms perform in comparison to their counterparts, namely algorithms 3, 4, and 7, which, respectively, maximize variation of x , minimize variation of z , and simultaneously maximize variation of x and minimize variation of z , just as algorithms 5, 6 and 8 do, but this time without regard to variation of y .
Theoretically, one would expect algorithm 7 to outperform algorithms 3 and 4. Qualitative methodlogists such as Gerring and Seawright and Gerring [ 17 , 3 ] expect this outcome and we concur. Using more information must be preferable to using less information when it comes to sampling. This does not imply, however, that algorithm 7 necessarily offers the optimal selection rule for qualitative comparative research. Since information from at least two different variables has to be aggregated, researchers have at their disposal multiple possible algorithms that all aggregate information in different ways. For example, in addition to the simple unweighted sum (or difference) that we assume in Table 1 , one can aggregate by multiplying or dividing the distances, and one can also weight the individual components.
Lijphart [ 1 ] has suggested an alternative function for aggregation, namely maximizing the ratio of the variance in x and z : max[dist(x)/dist(z)] . We include Lijphart’s suggestion as our algorithm 9 even though it suffers from a simple problem which reduces its usefulness: when the variance of the control variable z is smaller than 1.0, the variance of what Lijphart calls the operative variable x becomes increasingly unimportant for case-selection (unless of course the variation of the control variables is very similar across different pairs of cases). We solve this problem by also including in the competition an augmented version of Lijphart’s suggestion. This algorithm 10 adds one to the denominator of the algorithm proposed by Lijphart: max[dist(x)/(1+dist(z))] . Observe that adding one to the denominator prevents the algorithm from converging to min[dist(z)] when dist(z) becomes small. Finally, we add a variance-weighted version of algorithm 7 as our final algorithm 11 to check whether weighting improves on the simple algorithms. Table 2 summarizes the additional analyzed algorithms that aggregate information using more complicated functions.
Note that thus far we have given the selection algorithms formal and technical labels, avoiding terminology of case-selection rules commonly used in the literature. Nevertheless, there are connections between some of the above algorithms and the terminology commonly used in the literature. For example, algorithms 2, 3 and 5 are variants of selection rules described by Seawright and Gerring [ 3 ] as “diverse” case-selection rules. Algorithms 2, 5, 6, and 8 all use information on variation of the dependent variable and are thus variants of selection on the dependent variable. More importantly, algorithms 4 and 7 as well as algorithms 9 to 11 seem to be variants of the most similar design. However, we do not call any of these algorithms “selection on the dependent variable” or “most similar”. The reason is that, as discussed above, there is a lack of consensus on terminology and different scholars prefer different labels and often mean different things when they invoke rules such as “sampling on the dependent variable” or “most similar”.
The Monte Carlo design
The use of Monte Carlo techniques may appear to be strange to qualitative researchers. However, Monte Carlo simulations are perfectly suited for the purpose of exploring the ex ante reliability of case-selection algorithms. As we have explained above, Monte Carlo simulations provide insights into the expected accuracy of inferences given certain pre-defined properties of the data-generating process. While they are commonly used to compare estimators, one can equally use them to compare the performance of different sampling rules.
Monte Carlo simulations allow us to systematically change the data-generating process, and to explore the comparative advantages of different selection algorithms depending on the assumptions we make about the data-generating process. Possible systematic changes include variation in the assumed level of correlation between explanatory variables, the relative importance of uncertainty, the level of measurement error, and so on. Unsystematic changes are modelled by repeated random draws of the error term.
Specifically, we define various data-generating processes from which we draw a number of random samples, and then select two cases from each sample according to a specific algorithm, as defined above. As a consequence of the unaccounted error process, the computed effects from the various Monte Carlo simulations will deviate somewhat from the truth. Yet, since we confront all selection algorithms to the same set of data-generating processes, including the same error processes, performance differences must result from the algorithms themselves. These differences occur because different algorithms will select different pairs of cases i and j , and, as a consequence, the computed effect and the distance of this effect from the true effect differ. Our analysis explores to what extent a comparison of two cases allows researchers to estimate the effect that one explanatory variable, called x , exerts on a dependent variable, called y . We assume that this dependent variable y is a function of x , a single control variable z , which is observed, and some error term ε : y i = βx i + γz i + ε i , where β, γ represent coefficients and ε is an iid error process. Obviously, as var( ε ) approaches zero, the data-generating process becomes increasingly deterministic. We follow the convention of quantitative methodology and assume that the error term is randomly drawn from a standard normal distribution. Note, however, that since we are not interested in asymptotic properties of case-selection algorithms, we could as well draw the error term from different distributions. This would have no consequence other than adding systematic bias to all algorithms alike. The process resembles what Gerring and McDermott [ 46 ] call a “spatial comparison” (a comparison across n observations), but our conclusions equally apply to “longitudinal” (a comparison across t periods) and “dynamic comparisons” (a comparison across n · t observations). We conducted simulations with both a continuous and a binary dependent variable. We report results for the continuous variable in detail in the next section and briefly summarize the results for the binary dependent variable with full results reported in the appendices.
There are different ways to think about the error term. First, usually scientists implicitly assume that the world is not perfectly determined and they allow for multiple equilibria which depend on random constellations or the free will of actors. In this respect, the error term accounts for the existence of behavioral randomness. Second, virtually all social scientists acknowledge the existence of systematic and unsystematic measurement error. The error term can be perceived as accounting for information that is partly uncertain. And third, the error term can be interpreted as model uncertainty–that is, as unobserved omitted variables also exerting an influence on the dependent variable. Only if randomness and free will, measurement error, and model uncertainty did not exist, would the inclusion of an error term make no sense.
We always draw x and z from a normal distribution, but, of course, alternative assumptions are possible. Given the low number of observations, it comes without loss in generality that we draw ε from a normal distribution with mean zero and standard deviation of 1.5; and, unless otherwise stated, all true coefficients take the value of 1.0; the standard deviation of variables is 1.0; correlations are 0.0; and the number of observations N, representing the size of the sample from which researchers can select cases, equals 100.

Evaluating the results from the Monte Carlo simulations
We compare the reliability of inference on effect strength. Specifically, the effect size of x on y from a comparative case study with two cases equals
where subscripts [ i , j ] represent the two selected cases. We take the root mean squared error (RMSE) as our measure for the reliability of causal inference as it reacts to both bias and inefficiency. The RMSE is defined as
This criterion not only incorporates bias (the average deviation of the computed effect from the true effect), but also accounts for inefficiency, which is a measure of the sampling variation of the computed effect that reflects the influence of random noise on the computed effect. Qualitative researchers cannot appropriately control for the influence of noise on estimates. The best they can do to account for randomness is to choose a case-selection algorithm that responds less than others to noise. Naturally, these are case-selection algorithms that make best use of information. In quantitative research, the property characterizing the best use of information is called efficiency , and we see no reason to deviate from this terminology.
Results from the Monte Carlo analysis of case-selection algorithms
We conduct three sets of MC simulations, in which we vary the parameters of the data-generating process, and evaluate the effect of this variation on the precision with which the algorithms approach the true coefficients together with the efficiency of the estimation. In each type of analysis we draw 1,000 samples from the underlying data-generating process. In the first set of simulations, we change the number of observations from which the two cases are chosen ( i = 1,…N), thereby varying the size of the sample, i.e., the total number of cases from which researchers can select two cases. In the second set of simulations, we vary the correlation between x and z –that is, the correlation between the variable of interest and the confounding factor. In the final set of simulations, we vary the variance of x and thus the effect size or explanatory power of x relative to the effect size of the confounding factor z .
Analyzing the impact of varying the number of analyzed cases on the validity of inferences in qualitative comparative research may seem strange at first glance. After all, qualitative researchers usually study a fairly limited number of cases. In fact, in our Monte Carlo analyses we generate effects by looking at a single pair of cases selected by each of the case-selection algorithms. So why should the number of cases from which we select the two cases matter? The reason is that if qualitative researchers can choose from a larger number of cases about which they have theoretically relevant information, they will be able to select a better pair of cases given the chosen algorithm. The more information researchers have before they select cases, the more reliable their inferences should thus become. In other words, N does not represent the number of cases analyzed, but the number of the total set of cases from which the analyzed cases are chosen.
By varying the correlation between x and the control variable z we can analyze the impact of confounding factors on the performance of the case-selection algorithms. With increasing correlation, inferences should become less reliable. Thereby, we look at the effect of potential model misspecification on the validity of inference in qualitative comparative research. While quantitative researchers can eliminate the potential for bias from correlated control variables by including these on the right-hand-side of the regression model, qualitative researchers have to use appropriate case-selection rules to reduce the potential for bias.
Finally, in varying the standard deviation of x we analyze the impact of varying the strength of the effect of the variable of interest on the dependent variable. The larger this relative effect size of the variable of interest, the more reliable causal inferences should become. The smaller the effect of the variable of interest x on y in comparison to the effect on y of the control or confounding variables z , the harder it is to identify the effect correctly, and the less valid the inferences become–especially when the researcher does not know the true specification of the model.
Table 3 reports the Monte Carlo results obtained when we only vary the size of the sample from which we draw the two cases we compare. In this set of simulations, we do not allow for systematic correlation between the variable of interest x and the confounding factor z . The deviations of computed effects from the true effect occur because of “normal” sampling error, and how efficiently the algorithm deals with the available information.
Note: corr(x,z) = 0, SD(x) = 1
The table displays the root mean squared error. Smaller numbers indicate higher reliability.
Observe, first, that of the basic case-selection algorithms, max(x)min(z) performs up to 100 times better with respect to the average deviation from the true effect (the root mean squared error) than the poorest-performing competitors, namely random , which draws two cases randomly from the sample, and max(y) , which purely selects on the dependent variable. The drawback from selecting on the dependent variable declines if researchers additionally take into account variation of x and/or variation of z , but these algorithms 5, 6, and 8 are typically inferior to their counterparts 3, 4, and 7, which ignore variation of the dependent variable. Accordingly, selection on the dependent variable not only leads to unreliable inferences that are likely to be wrong, it also makes other selection algorithms less reliable. Hence, researchers should not pay attention to variation in the dependent variable y when they select cases. By selecting cases on the variable of interest x while at the same time controlling for the influence of confounding factors, researchers are likely to choose cases which vary in their outcome if x indeed exerts an effect on y .
Maximizing variation of x while at the same time minimizing variation of z appears optimal. Algorithm 7 uses subtraction as a basic function for aggregating information from more than one variable. Would using a more complicated function dramatically improve the performance of case-selection? The results reported in Table 3 show that, at least for this set of simulations, this is not the case. Algorithm 7 performs roughly 10 percent better than the augmented version of Lijphart’s proposal ( augmented lijphart ), and while algorithm 11, the variance-weighted version of algorithm 7, is very slightly superior, not much separates the performance of the two.
Another interesting finding from Table 3 is that only four algorithms become systematically more reliable when the population size from which we draw two cases increases. These four algorithms are: max(x) , max(x)min(z) and its weighted variant, weighted max(x)min(z) , as well as augmented lijphart . Algorithms need to have a certain quality to generate, in expectation, improvements in the validity of causal inferences when the population size becomes larger. Random selection, for example, only improves on average if the increase in population size leads to relatively more “onliers” than “outliers”. This may be the case, but there is no guarantee. When researchers use relatively reliable case-selection algorithms, however, an increase in the size of the sample, on which information is available, improves causal inferences unless one adds extreme outliers to the sample. Inferences become more reliable if cases are selected from a larger sample of cases for which researchers have sufficient information. We are not making any normative claim about enlarging the population size, because the improvements of enlarging the population from which cases are selected has to be discounted by the deteriorations caused by an increase in case heterogeneity caused by an enlarged sample.
The results from Table 3 support King, Keohane and Verba’s [ 39 ] arguments against both random selection and sampling on the dependent variable. At first sight, our results seem to differ from Herron and Quinn’s [ 5 ] finding that “simple random sampling outperforms most methods of case selection” even when the number of analyzed cases “is as small as 5 or 7”. However, our results are consistent with Herron and Quinn’s finding that random sampling is not reliable when the number of cases is two. In fact, the number of cases required to make random sampling a viable strategy depends on the heterogeneity of cases and the signal-to-noise ratio of the causal effect of interest: the more homogeneous and stronger the effect researchers are interested in, the better the performance of random selection of cases and the lower the number of cases for sufficiently reliable inferences.
In Table 4 , we report the results of Monte Carlo simulations from varying the correlation between the variable of interest x and the confounding factor z .
Note: SD(x) = 1.0, N = 100, SD(z) = 1.0, Varying Correlation (x,z). The table displays the root mean squared error. Smaller numbers indicate higher reliability.
Note that all substantive results from Table 3 remain valid if we allow for correlation between the variable of interest and the confounding factor. In particular, algorithm 11, which weights the individual components of the best-performing simple case-selection algorithm 7, performs only very slightly better; while the performance gap between simple algorithm max(x)min(z) , based on subtraction, and the augmented Lijphart algorithm ( augmented lijphart ), which uses the ratio as aggregation function, increases only marginally. Table 4 also demonstrates that correlation between the variable of interest and confounding factors renders causal inferences from qualitative comparative research less reliable. Over all simulations and algorithms, the RMSE increases by at least 100 percent when the correlation between x and z increases from 0.0 to either -0.9 or +0.9.
Finally, we examine how algorithms respond to variation in the strength of the effect of the variable of interest. In this final set of simulations for which results are reported in Table 5 we vary the standard deviation of the explanatory factor x ; a small standard deviation indicates a small effect of x on y relative to the effect exerted from z on y . The results show that the performance of all case-selection algorithms suffers from a low “signal-to-noise” ratio. As one would expect, the smaller the effect of the variable of interest x on y relative to the effect of z on y , the less reliable the causal inferences from comparative case study research becomes. Yet, we find that the algorithms which performed best in the previous two sets of simulations also turn out to be least vulnerable to a small effect of the variable of interest. Accordingly, while inferences do become more unreliable when the effect of the variable of interest becomes small relative to the total variation of the dependent variable, comparative case studies are not simply confined to analyzing the main determinant of the phenomenon of interest if one of the top performing case-selection algorithms are used. As in the previous sets of simulations, we find that little is gained by employing more complicated functions for aggregating information from more than one variable as, for example, the ratio ( augmented lijphart ) or weighting by the variance of x and z ( weighted max(x)min(z) ). Sticking to the most basic aggregation function has little cost, if any.
Note: corr(x,z) = 0.0, N = 100, SD(z) = 1.0, Varying SD(x)
We now briefly report results from additional Monte Carlo simulations which we show in full in the appendix to the paper ( S1 File ). First, weighting x and z by their respective sample range becomes more important when the data-generating process includes correlation between x and z and the effect of x on y is relatively small (see Table A in S1 File ). In this case, weighting both the variation of x and z before using the max(x)min(z) selection rule for identifying two cases slightly increases the reliability of causal inferences.
Second, we also conducted the full range of Monte Carlo simulations with a dichotomous dependent variable (see Tables B- E in S1 File ). We find that the algorithms that perform best with a continuous dependent variable also dominate with respect to reliability when we analyze dichotomous dependent variables. Yet, causal inferences from qualitative comparative case study research become far less reliable when the dependent variable is dichotomous for all selection algorithms compared to the case of a continuous dependent variable. The root mean squared error roughly doubles for the better-performing algorithms. As a consequence, causal inferences with a binary dependent variable and an additional complication (either a non-trivial correlation between x and z or a relatively small effect of x on y ) are not reliable. Accordingly, qualitative researchers should not throw away variation by dichotomizing their dependent variable. Where the dependent variable is dichotomous, qualitative comparative research is confined to what most qualitative researchers actually do in these situations: trying to identify strong and deterministic relationships or necessary conditions [ 47 , 48 ]. In both cases, the strong deterministic effect of x on y compensates for the low level of information in the data.
Case-selection rules employed in qualitative research resemble ‘matching’ algorithms developed by identification scholars in quantitative research and thus can be employed to derive causal inferences. They also share their most important shortcoming: the extent to which causal inferences from selected samples are valid is partly determined by the extent of knowledge of the data-generating process. The more is known about the “true model”, the better researchers can select cases to maximize the ex ante reliability of their causal inferences.
Our major contribution has been to guide qualitative comparative researchers on what are the selection rules with the highest ex ante reliability for the purpose of making causal inferences under a range of conditions regarding the underlying data-generating process. The validity of causal inferences from qualitative comparative research will necessarily always be uncertain but following our guidance will allow qualitative comparative researchers to maximize the imperfect validity of their inferences.
Qualitative comparative researchers can take away six important concrete lessons from our Monte Carlo simulations: First, ceteris paribus, selecting cases from a larger set of potential cases gives more reliable results. Qualitative researchers often deal with extremely small samples. Sometimes nothing can be done to increase sample size, but where there are no binding constraints it can well be worth the effort expanding the sample from which cases can be selected. Second, for all the better-performing selection algorithms, it holds that ignoring information on the dependent variable for the purpose of selecting cases makes inferences much more reliable. Tempting though it may seem, qualitative comparative researchers should not select on the dependent variable at all. Third, selecting cases based on both the variable of interest and confounding factors improves the ex ante reliability of causal inferences in comparison to selection algorithms that consider just the variable of interest or just confounding factors–even if this means that one no longer chooses the cases that match most closely on confounding factors. These algorithms are relatively best-performing, no matter what the underlying data-generating process (of those we have analyzed). This is a crucial lesson because qualitative comparative researchers might not have much knowledge about the kind of data-generating process they are dealing with. Fourth, correlation between the variable of interest and confounding factors renders the selection algorithms less reliable. The same holds if the analyzed effect is weak. This reinforces existing views that qualitative case comparison is most suitable for studying strong and deterministic causal relationships [ 47 , 48 ]. Fifth, the reliability of case-selection rules depends on the variation in the dependent variable scholars can analyze. Accordingly, unless there are very strong over-riding theoretical or conceptual reasons, throwing away information by dichotomizing the dependent variable is a bad idea. A continuous dependent variable allows for more valid inferences; a dichotomous dependent variable should only be used if there is no alternative. Sixth, employing basic functions for aggregating information from more than one variable (such as maximizing the difference between variation of x and variation of z ) does not reduce by much the ex ante reliability of case-selection compared to more complicated aggregation functions (such as maximizing the ratio or the variance-weighted difference). The only exceptions occur if x and z are highly correlated and the effect of x on y is relatively small compared to the effect of z on y . As a general rule, one does not lose much by opting for the most basic aggregation function.
In conclusion, our Monte Carlo study is broadly consistent with the views of qualitative methodologists. After all, the best- or nearly best-performing algorithms in our analysis of alternative selection algorithms appear to be variants of the most similar design, which in turn draws on Przeworski and Teune’s [ 35 ] and Lijphart’s [ 49 ] suggestions for case-selection. However, we are the first to provide systematic evidence that upholds existing recommendations in the presence of stochastic error processes. In addition, we demonstrated that simple functions for linking variation of the explanatory variable with variation of the confounding variables perform relatively well in general. There is little reason to resort to more advanced functions unless the explanatory variable has a weak effect and is strongly correlated with the confounding variables. One important area for further analysis comes from settings in which comparative qualitative researchers assess claims about two or more causal factors interacting with each other.
Supporting information
Funding statement.
The authors received no funding for this work.
Data Availability
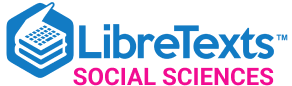
- school Campus Bookshelves
- menu_book Bookshelves
- perm_media Learning Objects
- login Login
- how_to_reg Request Instructor Account
- hub Instructor Commons
Margin Size
- Download Page (PDF)
- Download Full Book (PDF)
- Periodic Table
- Physics Constants
- Scientific Calculator
- Reference & Cite
- Tools expand_more
- Readability
selected template will load here
This action is not available.
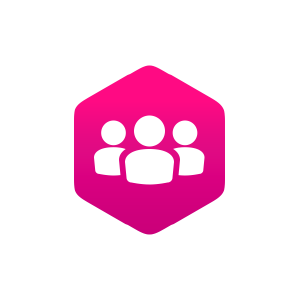
2.3: Case Selection (Or, How to Use Cases in Your Comparative Analysis)
- Last updated
- Save as PDF
- Page ID 135832
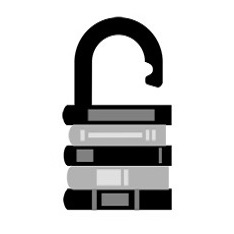
- Dino Bozonelos, Julia Wendt, Charlotte Lee, Jessica Scarffe, Masahiro Omae, Josh Franco, Byran Martin, & Stefan Veldhuis
- Victor Valley College, Berkeley City College, Allan Hancock College, San Diego City College, Cuyamaca College, Houston Community College, and Long Beach City College via ASCCC Open Educational Resources Initiative (OERI)
\( \newcommand{\vecs}[1]{\overset { \scriptstyle \rightharpoonup} {\mathbf{#1}} } \)
\( \newcommand{\vecd}[1]{\overset{-\!-\!\rightharpoonup}{\vphantom{a}\smash {#1}}} \)
\( \newcommand{\id}{\mathrm{id}}\) \( \newcommand{\Span}{\mathrm{span}}\)
( \newcommand{\kernel}{\mathrm{null}\,}\) \( \newcommand{\range}{\mathrm{range}\,}\)
\( \newcommand{\RealPart}{\mathrm{Re}}\) \( \newcommand{\ImaginaryPart}{\mathrm{Im}}\)
\( \newcommand{\Argument}{\mathrm{Arg}}\) \( \newcommand{\norm}[1]{\| #1 \|}\)
\( \newcommand{\inner}[2]{\langle #1, #2 \rangle}\)
\( \newcommand{\Span}{\mathrm{span}}\)
\( \newcommand{\id}{\mathrm{id}}\)
\( \newcommand{\kernel}{\mathrm{null}\,}\)
\( \newcommand{\range}{\mathrm{range}\,}\)
\( \newcommand{\RealPart}{\mathrm{Re}}\)
\( \newcommand{\ImaginaryPart}{\mathrm{Im}}\)
\( \newcommand{\Argument}{\mathrm{Arg}}\)
\( \newcommand{\norm}[1]{\| #1 \|}\)
\( \newcommand{\Span}{\mathrm{span}}\) \( \newcommand{\AA}{\unicode[.8,0]{x212B}}\)
\( \newcommand{\vectorA}[1]{\vec{#1}} % arrow\)
\( \newcommand{\vectorAt}[1]{\vec{\text{#1}}} % arrow\)
\( \newcommand{\vectorB}[1]{\overset { \scriptstyle \rightharpoonup} {\mathbf{#1}} } \)
\( \newcommand{\vectorC}[1]{\textbf{#1}} \)
\( \newcommand{\vectorD}[1]{\overrightarrow{#1}} \)
\( \newcommand{\vectorDt}[1]{\overrightarrow{\text{#1}}} \)
\( \newcommand{\vectE}[1]{\overset{-\!-\!\rightharpoonup}{\vphantom{a}\smash{\mathbf {#1}}}} \)
Learning Objectives
By the end of this section, you will be able to:
- Discuss the importance of case selection in case studies.
- Consider the implications of poor case selection.
Introduction
Case selection is an important part of any research design. Deciding how many cases, and which cases, to include, will clearly help determine the outcome of our results. If we decide to select a high number of cases, we often say that we are conducting large-N research. Large-N research is when the number of observations or cases is large enough where we would need mathematical, usually statistical, techniques to discover and interpret any correlations or causations. In order for a large-N analysis to yield any relevant findings, a number of conventions need to be observed. First, the sample needs to be representative of the studied population. Thus, if we wanted to understand the long-term effects of COVID, we would need to know the approximate details of those who contracted the virus. Once we know the parameters of the population, we can then determine a sample that represents the larger population. For example, women make up 55% of all long-term COVID survivors. Thus, any sample we generate needs to be at least 55% women.
Second, some kind of randomization technique needs to be involved in large-N research. So not only must your sample be representative, it must also randomly select people within that sample. In other words, we must have a large selection of people that fit within the population criteria, and then randomly select from those pools. Randomization would help to reduce bias in the study. Also, when cases (people with long-term COVID) are randomly chosen they tend to ensure a fairer representation of the studied population. Third, your sample needs to be large enough, hence the large-N designation for any conclusions to have any external validity. Generally speaking, the larger the number of observations/cases in the sample, the more validity we can have in the study. There is no magic number, but if using the above example, our sample of long-term COVID patients should be at least over 750 people, with an aim of around 1,200 to 1,500 people.
When it comes to comparative politics, we rarely ever reach the numbers typically used in large-N research. There are about 200 fully recognized countries, with about a dozen partially recognized countries, and even fewer areas or regions of study, such as Europe or Latin America. Given this, what is the strategy when one case, or a few cases, are being studied? What happens if we are only wanting to know the COVID-19 response in the United States, and not the rest of the world? How do we randomize this to ensure our results are not biased or are representative? These and other questions are legitimate issues that many comparativist scholars face when completing research. Does randomization work with case studies? Gerring suggests that it does not, as “any given sample may be widely representative” (pg. 87). Thus, random sampling is not a reliable approach when it comes to case studies. And even if the randomized sample is representative, there is no guarantee that the gathered evidence would be reliable.
One can make the argument that case selection may not be as important in large-N studies as they are in small-N studies. In large-N research, potential errors and/or biases may be ameliorated, especially if the sample is large enough. This is not always what happens, errors and biases most certainly can exist in large-N research. However, incorrect or biased inferences are less of a worry when we have 1,500 cases versus 15 cases. In small-N research, case selection simply matters much more.
This is why Blatter and Haverland (2012) write that, “case studies are ‘case-centered’, whereas large-N studies are ‘variable-centered’". In large-N studies we are more concerned with the conceptualization and operationalization of variables. Thus, we want to focus on which data to include in the analysis of long-term COVID patients. If we wanted to survey them, we would want to make sure we construct questions in appropriate ways. For almost all survey-based large-N research, the question responses themselves become the coded variables used in the statistical analysis.
Case selection can be driven by a number of factors in comparative politics, with the first two approaches being the more traditional. First, it can derive from the interests of the researcher(s). For example, if the researcher lives in Germany, they may want to research the spread of COVID-19 within the country, possibly using a subnational approach where the researcher may compare infection rates among German states. Second, case selection may be driven by area studies. This is still based on the interests of the researcher as generally speaking scholars pick areas of studies due to their personal interests. For example, the same researcher may research COVID-19 infection rates among European Union member-states. Finally, the selection of cases selected may be driven by the type of case study that is utilized. In this approach, cases are selected as they allow researchers to compare their similarities or their differences. Or, a case might be selected that is typical of most cases, or in contrast, a case or cases that deviate from the norm. We discuss types of case studies and their impact on case selection below.
Types of Case Studies: Descriptive vs. Causal
There are a number of different ways to categorize case studies. One of the most recent ways is through John Gerring. He wrote two editions on case study research (2017) where he posits that the central question posed by the researcher will dictate the aim of the case study. Is the study meant to be descriptive? If so, what is the researcher looking to describe? How many cases (countries, incidents, events) are there? Or is the study meant to be causal, where the researcher is looking for a cause and effect? Given this, Gerring categorizes case studies into two types: descriptive and causal.
Descriptive case studies are “not organized around a central, overarching causal hypothesis or theory” (pg. 56). Most case studies are descriptive in nature, where the researchers simply seek to describe what they observe. They are useful for transmitting information regarding the studied political phenomenon. For a descriptive case study, a scholar might choose a case that is considered typical of the population. An example could involve researching the effects of the pandemic on medium-sized cities in the US. This city would have to exhibit the tendencies of medium-sized cities throughout the entire country. First, we would have to conceptualize what we mean by ‘a medium-size city’. Second, we would then have to establish the characteristics of medium-sized US cities, so that our case selection is appropriate. Alternatively, cases could be chosen for their diversity . In keeping with our example, maybe we want to look at the effects of the pandemic on a range of US cities, from small, rural towns, to medium-sized suburban cities to large-sized urban areas.
Causal case studies are “organized around a central hypothesis about how X affects Y” (pg. 63). In causal case studies, the context around a specific political phenomenon or phenomena is important as it allows for researchers to identify the aspects that set up the conditions, the mechanisms, for that outcome to occur. Scholars refer to this as the causal mechanism , which is defined by Falleti & Lynch (2009) as “portable concepts that explain how and why a hypothesized cause, in a given context, contributes to a particular outcome”. Remember, causality is when a change in one variable verifiably causes an effect or change in another variable. For causal case studies that employ causal mechanisms, Gerring divides them into exploratory case-selection, estimating case-selection, and diagnostic case-selection. The differences revolve around how the central hypothesis is utilized in the study.
Exploratory case studies are used to identify a potential causal hypothesis. Researchers will single out the independent variables that seem to affect the outcome, or dependent variable, the most. The goal is to build up to what the causal mechanism might be by providing the context. This is also referred to as hypothesis generating as opposed to hypothesis testing. Case selection can vary widely depending on the goal of the researcher. For example, if the scholar is looking to develop an ‘ideal-type’, they might seek out an extreme case. An ideal-type is defined as a “conception or a standard of something in its highest perfection” (New Webster Dictionary). Thus, if we want to understand the ideal-type capitalist system, we want to investigate a country that practices a pure or ‘extreme’ form of the economic system.
Estimating case studies start with a hypothesis already in place. The goal is to test the hypothesis through collected data/evidence. Researchers seek to estimate the ‘causal effect’. This involves determining if the relationship between the independent and dependent variables is positive, negative, or ultimately if no relationship exists at all. Finally, diagnostic case studies are important as they help to “confirm, disconfirm, or refine a hypothesis” (Gerring 2017). Case selection can also vary in diagnostic case studies. For example, scholars can choose an least-likely case, or a case where the hypothesis is confirmed even though the context would suggest otherwise. A good example would be looking at Indian democracy, which has existed for over 70 years. India has a high level of ethnolinguistic diversity, is relatively underdeveloped economically, and a low level of modernization through large swaths of the country. All of these factors strongly suggest that India should not have democratized, or should have failed to stay a democracy in the long-term, or have disintegrated as a country.
Most Similar/Most Different Systems Approach
The discussion in the previous subsection tends to focus on case selection when it comes to a single case. Single case studies are valuable as they provide an opportunity for in-depth research on a topic that requires it. However, in comparative politics, our approach is to compare. Given this, we are required to select more than one case. This presents a different set of challenges. First, how many cases do we pick? This is a tricky question we addressed earlier. Second, how do we apply the previously mentioned case selection techniques, descriptive vs. causal? Do we pick two extreme cases if we used an exploratory approach, or two least-likely cases if choosing a diagnostic case approach?
Thankfully, an English scholar by the name of John Stuart Mill provided some insight on how we should proceed. He developed several approaches to comparison with the explicit goal of isolating a cause within a complex environment. Two of these methods, the 'method of agreement' and the 'method of difference' have influenced comparative politics. In the 'method of agreement' two or more cases are compared for their commonalities. The scholar looks to isolate the characteristic, or variable, they have in common, which is then established as the cause for their similarities. In the 'method of difference' two or more cases are compared for their differences. The scholar looks to isolate the characteristic, or variable, they do not have in common, which is then identified as the cause for their differences. From these two methods, comparativists have developed two approaches.
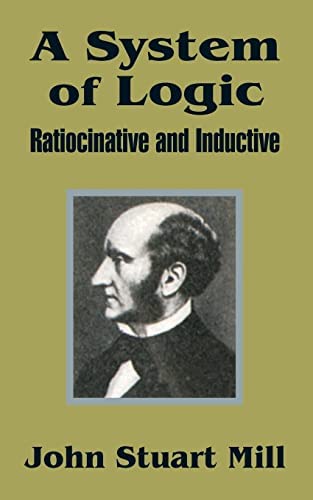
What Is the Most Similar Systems Design (MSSD)?
This approach is derived from Mill’s ‘method of difference’. In a Most Similar Systems Design Design, the cases selected for comparison are similar to each other, but the outcomes differ in result. In this approach we are interested in keeping as many of the variables the same across the elected cases, which for comparative politics often involves countries. Remember, the independent variable is the factor that doesn’t depend on changes in other variables. It is potentially the ‘cause’ in the cause and effect model. The dependent variable is the variable that is affected by, or dependent on, the presence of the independent variable. It is the ‘effect’. In a most similar systems approach the variables of interest should remain the same.
A good example involves the lack of a national healthcare system in the US. Other countries, such as New Zealand, Australia, Ireland, UK and Canada, all have robust, publicly accessible national health systems. However, the US does not. These countries all have similar systems: English heritage and language use, liberal market economies, strong democratic institutions, and high levels of wealth and education. Yet, despite these similarities, the end results vary. The US does not look like its peer countries. In other words, why do we have similar systems producing different outcomes?
What Is the Most Different Systems Design (MDSD)?
This approach is derived from Mill’s ‘method of agreement’. In a Most Different System Design, the cases selected are different from each other, but result in the same outcome. In this approach, we are interested in selecting cases that are quite different from one another, yet arrive at the same outcome. Thus, the dependent variable is the same. Different independent variables exist between the cases, such as democratic v. authoritarian regime, liberal market economy v. non-liberal market economy. Or it could include other variables such as societal homogeneity (uniformity) vs. societal heterogeneity (diversity), where a country may find itself unified ethnically/religiously/racially, or fragmented along those same lines.
A good example involves the countries that are classified as economically liberal. The Heritage Foundation lists countries such as Singapore, Taiwan, Estonia, Australia, New Zealand, as well as Switzerland, Chile and Malaysia as either free or mostly free. These countries differ greatly from one another. Singapore and Malaysia are considered flawed or illiberal democracies (see chapter 5 for more discussion), whereas Estonia is still classified as a developing country. Australia and New Zealand are wealthy, Malaysia is not. Chile and Taiwan became economically free countries under the authoritarian military regimes, which is not the case for Switzerland. In other words, why do we have different systems producing the same outcome?
- Help & FAQ
Case-Selection Techniques in Case Study Research: A Menu of Qualitative and Quantitative Options
- Political Science
Research output : Contribution to journal › Article › peer-review
Fingerprint
- Quantitative Social Sciences 100%
- Selection Procedure Social Sciences 100%
- Approach Social Sciences 100%
- Case Study Research Social Sciences 100%
- Case Study Psychology 100%
- Research Psychology 100%
- sampling INIS 100%
- Analysis Social Sciences 66%
T1 - Case-Selection Techniques in Case Study Research: A Menu of Qualitative and Quantitative Options
AU - Seawright, Jason
N2 - How can scholars select cases from a large universe for in-depth case study analysis? Random sampling is not typically a viable approach when the total number of cases to be selected is small. Hence attention to purposive modes of sampling is needed. Yet, while the existing qualitative literature on case selection offers a wide range of suggestions for case selection, most techniques discussed require in-depth familiarity of each case. Seven case selection procedures are considered, each of which facilitates a different strategy for within-case analysis. The case selection procedures considered focus on typical, diverse, extreme, deviant, influential, most similar, and most different cases. For each case selection procedure, quantitative approaches are discussed that meet the goals of the approach, while still requiring information that can reasonably be gathered for a large number of cases.
AB - How can scholars select cases from a large universe for in-depth case study analysis? Random sampling is not typically a viable approach when the total number of cases to be selected is small. Hence attention to purposive modes of sampling is needed. Yet, while the existing qualitative literature on case selection offers a wide range of suggestions for case selection, most techniques discussed require in-depth familiarity of each case. Seven case selection procedures are considered, each of which facilitates a different strategy for within-case analysis. The case selection procedures considered focus on typical, diverse, extreme, deviant, influential, most similar, and most different cases. For each case selection procedure, quantitative approaches are discussed that meet the goals of the approach, while still requiring information that can reasonably be gathered for a large number of cases.
M3 - Article
JO - Political Research Quarterly
JF - Political Research Quarterly
- Open access
- Published: 11 May 2024
The reliability of the College of Intensive Care Medicine of Australia and New Zealand “Hot Case” examination
- Kenneth R. Hoffman 1 , 2 ,
- David Swanson 3 ,
- Stuart Lane 4 ,
- Chris Nickson 1 , 2 ,
- Paul Brand 5 &
- Anna T. Ryan 3
BMC Medical Education volume 24 , Article number: 527 ( 2024 ) Cite this article
1246 Accesses
5 Altmetric
Metrics details
High stakes examinations used to credential trainees for independent specialist practice should be evaluated periodically to ensure defensible decisions are made. This study aims to quantify the College of Intensive Care Medicine of Australia and New Zealand (CICM) Hot Case reliability coefficient and evaluate contributions to variance from candidates, cases and examiners.
This retrospective, de-identified analysis of CICM examination data used descriptive statistics and generalisability theory to evaluate the reliability of the Hot Case examination component. Decision studies were used to project generalisability coefficients for alternate examination designs.
Examination results from 2019 to 2022 included 592 Hot Cases, totalling 1184 individual examiner scores. The mean examiner Hot Case score was 5.17 (standard deviation 1.65). The correlation between candidates’ two Hot Case scores was low (0.30). The overall reliability coefficient for the Hot Case component consisting of two cases observed by two separate pairs of examiners was 0.42. Sources of variance included candidate proficiency (25%), case difficulty and case specificity (63.4%), examiner stringency (3.5%) and other error (8.2%). To achieve a reliability coefficient of > 0.8 a candidate would need to perform 11 Hot Cases observed by two examiners.
The reliability coefficient for the Hot Case component of the CICM second part examination is below the generally accepted value for a high stakes examination. Modifications to case selection and introduction of a clear scoring rubric to mitigate the effects of variation in case difficulty may be helpful. Increasing the number of cases and overall assessment time appears to be the best way to increase the overall reliability. Further research is required to assess the combined reliability of the Hot Case and viva components.
Peer Review reports
Credentialling medical specialists requires defined performance standards [ 1 , 2 ] and traditionally relies upon high stakes examinations to assess trainees against those standards [ 3 , 4 , 5 ]. These examinations substitute for controlling quality of care by attempting to control progression through training programs for the safety of both patients and society. Specialist colleges are also expected to provide transparent and fair assessment processes, to ensure defensible decisions are made regarding trainee progression and specialist credentialling [ 6 ].
The College of Intensive Care Medicine of Australia and New Zealand (CICM) second part examination was introduced in 1979 and has undergone many revisions [ 3 ]. It has two components: a written examination and, if completed successfully, an oral examination. The oral examination includes an eight-station viva assessment and two clinical “Hot Case” assessments. This Hot Case component targets the highest level of assessment on Miller’s Pyramid [ 7 ], ‘Does’, requiring candidates to be assessed in the workplace performing real-world tasks. Of the candidates who have passed the written examination successfully, only 35% pass both Hot Cases [ 8 ]. It is therefore important to evaluate both the validity of inferences from this examination component and the reliability or reproducibility of the results [ 9 ].
Reliability describes the degree to which variation in scores reflects true variability in candidates’ proficiency, rather than measurement error. This is dependent on the task, examiner stringency and assessment context [ 10 ]. Reliability can be quantified using the reliability coefficient, with 0 representing a completely unreliable assessment and 1 representing a completely reliable assessment. The minimum standard generally considered acceptable for high stakes medical examinations is a reliability coefficient greater than 0.8 [ 11 , 12 , 13 , 14 ].
Generalisability theory (G-theory) provides the statistical basis for combining multiple sources of variance into a single analysis [ 15 ]. This enables the calculation of an overall reliability coefficient and calculation of the contribution from candidates, cases and examiners to examination reliability. G-theory also provides the basis for conducting decision studies (D-studies) that statistically project reliability based on alternate assessment designs.
To date, no information on the reliability of the CICM second part examination has been published. Given the implications of incorrect credentialling decisions for trainees, patients and society, the Hot Case reliability coefficient should be quantified.
Examination format
The second part examination prior to COVID-19 was held twice yearly with candidates invited to the oral component in a single Australian city. Trainees complete two Hot Cases within metropolitan intensive care units (ICU) with 20 min allocated for each: 10 min to examine an ICU patient, followed by 10 min with paired examiners to present their findings and answer questions regarding investigations and clinical management.
Format changes occurred during the COVID-19 pandemic. The first oral examination was cancelled in 2020, with trainees deferring to the second sitting. Additionally, travel restrictions meant candidates sat the Hot Case component in their home city with local examiners from the second sitting in 2020 to the second sitting in 2021. From 2022 onwards, the oral examination has been held in Sydney, Melbourne, or both.
Hot Cases are marked out of 10 by two CICM examiners using a rating scale that scores candidates based on how comfortable examiners would be supervising them. An acceptable pass standard (5/10) indicates an examiner is comfortable to leave the candidate in charge of the ICU with minimal supervision. There is no specific scoring rubric, although examiner pairs cooperatively determine clinical signs that should be identified, nominate investigations and imaging to show a candidate, and specify discussion questions. Expected levels of knowledge, interpretation and clinical management are defined prospectively. An automatic fail for the entire oral examination is triggered if candidates fail both Hot Cases and obtain a Hot Case component mark < 40% of the possible marks.
Examiner calibration
Examiners undergo calibration training prior to the examination. They independently score the candidate, then discuss their individual scores and rationale. Examiners can then amend their score before recording final scores in the examination database. Each Hot Case is marked by separate pairs of examiners, to prevent bias from a candidates first case performance influencing their second case score. Following the examination, results are presented to the whole examiner cohort for further discussion and explanation.
Data collection
The CICM provided access to their examination database from the second sitting of 2012 (2012-2) through to the first sitting of 2022 (2022-1). For each de-identified candidate, the written mark, overall Hot Case mark, viva mark, and overall examination mark were obtained. The Hot Case specific data included the cases used, examiners present and individual examiner marks, with a total of four scores per candidate (two examiner scores for each Hot Case).
Analysis was restricted to 2019-1 to 2022-1 due to data recording inconsistency providing insufficient data for G-theory analysis. Additionally, changes occurred from 2019-1 with the introduction of the Angoff standard setting method [ 16 , 17 ] for the written examination. This altered final score calculation with the written examination functioning as a barrier examination, although the written score no longer contributes to the final examination score. Candidates were included if they sat the oral examination for the first time in 2019 or later and, if they failed, subsequent attempts were recorded.
Statistical analysis
Statistical analysis used Microsoft Excel and SPSS. Continuous examination scores were summarised using mean and standard deviation. Categorical variables were reported as counts and percentages. Frequency distributions (histograms) were used to graph overall examination component results. A p-value of < 0.05 indicated statistical significance. Comparisons of examiner marks and relationships between examination components were analysed with Pearson’s correlation coefficient and visually represented with scatterplots.
G-theory analysis was used to calculate an overall reliability coefficient for the Hot Case examination, and the factors contributing to variance. As examiners observed multiple candidates and candidates performed multiple Hot Cases, the design was partially crossed. However, as the case identification numbers used in the examination were recorded variably, the initial design was modified to treat cases as nested within candidates for the analysis. The variance factors being analysed included candidate proficiency, examiner stringency, case to case performance variability (case specificity) and other unspecified measurement error. These were reported with variance components, square roots of variance components and percentage of total variance. G-theory was used to conduct D-studies exploring the impact of alternate assessment designs on overall generalisability coefficients and associated standard errors of measurement. The D-study calculated the generalisability coefficient based on the equation listed in Fig. 1 .

Generalisability coefficient equation
Overall, there were 889 candidate oral examination attempts from 2012-2 to 2022-1. After exclusion of candidate oral examination attempts prior to the 2019-1 sitting, exclusion of candidates with first attempts prior to 2019-1 and exclusion of one candidate with missing Hot Case scores, there were 296 candidate oral examination attempts analysed. This included 166 first attempts, 100 s and 30 third attempts. This resulted in 592 Hot Case results and 1184 individual examiner Hot Case scores. The recruitment, exclusion and analysis of the sample are presented in Fig. 2 .
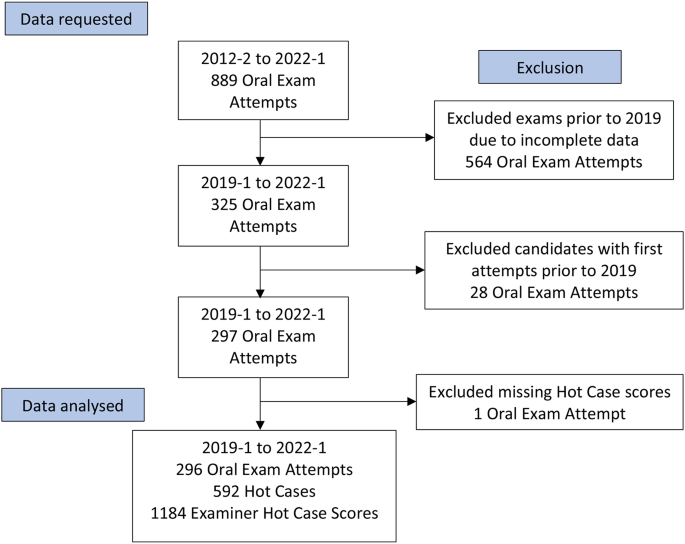
CONSORT style diagram demonstrating the sample size from data request through to the sample available for analysis
The mean and standard deviation of individual examiner Hot Case scores from all examiners was 5.17 and 1.65 respectively. Of the 1184 Hot Case individual examiner scores, 645 (54.5%) achieved a score 5 or greater, and 539 (45.5%) scored less than 5. The distribution of individual examiner Hot Case scores is presented in Fig. 3 . First attempt candidates scored higher than those repeating (5.25 (SD1.63) vs. 4.89 (SD1.66) p = < 0.01).
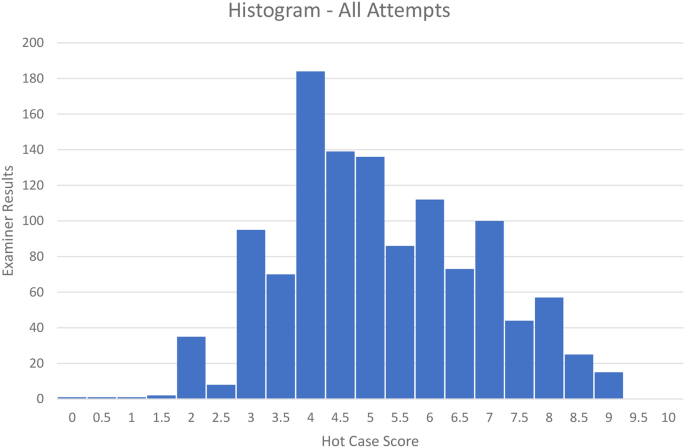
Histogram showing individual examiner Hot Case scores for all attempts
Scores on each Hot Case are calculated as the mean of the two individual examiner Hot Case scores. Overall, 312 of 592 Hot Cases were passed (52.7%). The correlation coefficient between candidates first and second Hot Cases was low at 0.30 (Fig. 4 ).
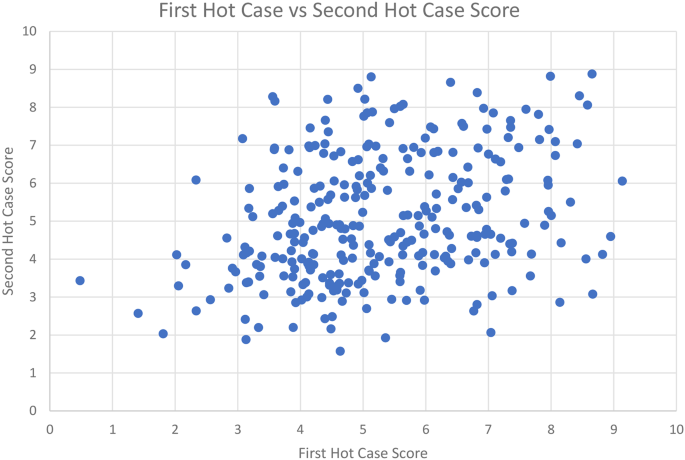
The correlation between each candidate’s first and second Hot Case scores. A jitter function was applied to spread overlying points
The correlation coefficient between examiners observing the same case (inter-rater agreement) was high at 0.91 (Fig. 5 ).
The summary of sources of variance for individual examiner Hot Case scores is presented in Table 1 .
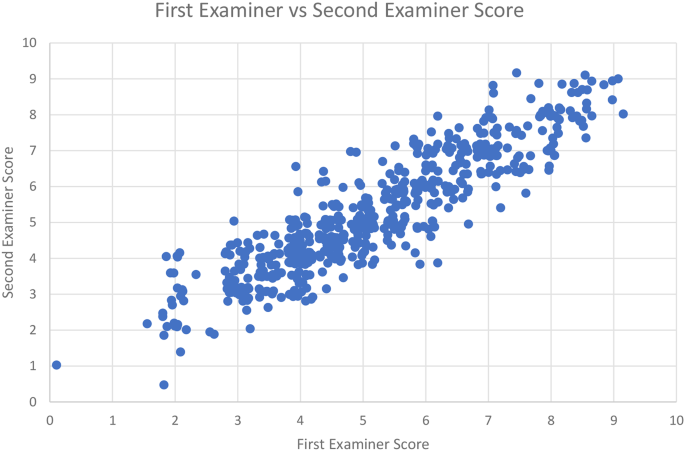
Comparison between Hot Case scores from the first and second examiners. A jitter function was applied to spread overlying points
The overall generalisability coefficient of the Hot Case component including two separate cases observed by two examiners each was 0.42.
The results for the D-studies are presented in Table 2 . To achieve a generalisability coefficient of 0.8 or greater, 11 Hot Cases with two examiners would be needed. A graph comparing the generalisability coefficients for one and two examiners is presented in Fig. 6 .
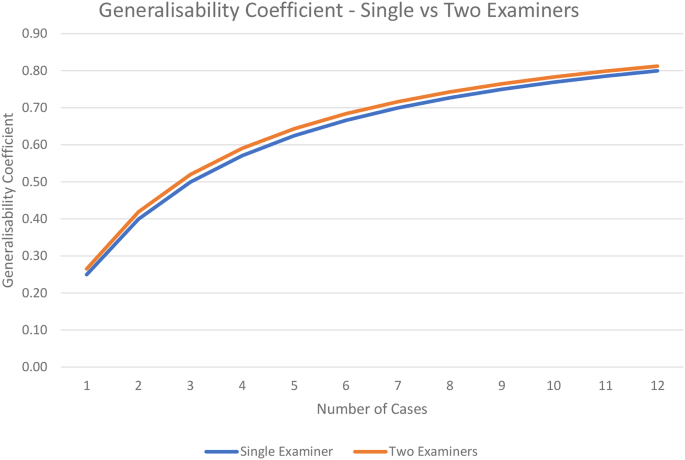
Generalisability coefficients with a variable number of cases comparing examination designs with one and two examiners observing each case
The current examination format with two Hot Cases observed by two examiners has a reliability coefficient of 0.42. To achieve the widely accepted standard for high stakes examinations of a reliability coefficient of > 0.8 requires each candidate to sit 11 Hot Cases with two examiners.
These results are similar to The Royal Australasian College of Physicians (RACP) 60-minute long case examination observed by two examiners which has a reliability coefficient of 0.38 [ 18 ]. When the assessment time is lengthened with two long cases and four short cases, the RACP achieved a reliability coefficient of 0.71. The RACP continues to use long case examinations, as they are valued by examiners and trainees as an authentic measure of competence with an educational impact from examination preparation [ 18 ]. Educational impact is commonly cited as a reason to retain clinical examinations [ 4 , 19 , 20 ].
G-theory analysis demonstrates that examiners appear well calibrated, as examiner variance was responsible for only 3.5% of overall variance in Hot Case scores. Therefore, adding additional examiners would not substantially improve reliability. However, this conclusion may be affected by the extent of discussion between the examiners prior to recording their amended final scores. If discussion influences the opinions of an examiner strongly, it is likely there will be higher correlation between examiner scores. To evaluate this effect, independent examiner scores would need to be recorded prior to discussion, with clear guidelines around acceptable amendments to scores.
The finding that the majority of Hot Case variance (63.4%) arises from case variation is consistent with anecdotal reports from examination candidates who describe case difficulty as a “lucky dip”. This finding is consistent with the poor correlation (0.30) between candidates’ first and second Hot Cases. Whilst examiners preview the Hot Case patient, there is no formal method of quantifying and adjusting for the difficulty of each case. According to Kane’s Validity Framework [ 21 ], it is difficult to argue that the assessment is valid if the initial scoring and subsequent generalisation of those scores are based more on case specificity than candidate proficiency, particularly when the implications of the results are significant for candidates and patient safety. The CICM has introduced the Angoff method [ 16 ] for the written examination to account for variation in question difficulty and an appropriate standard setting method for the Hot Case component may mitigate this degree of case variability to some extent. The CICM has avoided the use of norm referenced assessments where candidates are compared with their peers so that all candidates deemed competent are eligible to pass. This is appropriate given the low number of candidates in each sitting, the low number of candidates taken to each case and high variability in case difficulty.
Case specificity is the concept that candidate performance is dependent on the case used and is a major issue in specialist credentialling examinations [ 4 ]. Problem solving ability and clinical reasoning are based on prior clinical experience, areas of particular interest and background knowledge. Candidate performance may be highly case specific, meaning limited numbers of examination cases have detrimental effects on reliability [ 4 , 5 , 22 ]. In the literature, increasing case numbers or overall assessment time is commonly proposed as a method of obtaining more generalisable results [ 6 , 18 , 23 , 24 ]. However, having a candidate pass overall, but clearly fail a component of a credentialling examination may be difficult to justify as defensible from the perspective of patient safety and societal obligations.
The individual examiner Hot Case scores (5.17, SD 1.65) are close to the 50% pass fail boundary. This makes examiners’ decision making difficult, with potentially small differences in performance determining a pass or fail. This is demonstrated in the histogram in Fig. 3 , with a large proportion of trainees scoring a 4.5 or 5, the junction between a pass and a fail. This dichotomisation should be supported by a clear rubric defining what constitutes a minimally competent performance. This will also give candidates clearer performance expectations and may mitigate variability due to case difficulty and specificity by defining expected competencies which are independent of the case difficulty.
Assessing the quality of future care using examination performance as a substitute marker of competence has limitations [ 11 ]. There are concerns from a validity point of view regarding decision making based on short periods of assessment [ 6 , 9 , 10 , 18 , 25 ]. As such, credentialling examinations should focus on identifying low end outliers, a possible true risk to patients and society without further training. Rather than failing candidates with a borderline performance, the focus should be on increasing the sample size to guide decision making. Additional Hot Cases for those with a borderline performance on the oral examination is a possible solution, to increase the reliability for defensible decision making. Summative Hot Cases performed during the training program, but not at the time of the final examination, is another option to increase available data through a transition to a programmatic style of longitudinal assessment.
Restricting the analysis for candidates who sat the written from the 2019-1 sitting onwards was necessitated by the quality of the available dataset. This aided analysis as the Angoff method was introduced for the written paper in 2019 [ 17 ] with the written score no longer counting toward the overall examination score. Candidates are now considered to have passed or failed the written, and then to pass the oral examination they require > 50% from the Hot Case component (worth 30 marks) and viva component (worth 40 marks) combined. This results in a higher benchmark to pass the examination overall, as previously a strong written mark could contribute to an overall pass despite a weaker oral performance.
This research fills a gap in the current understanding of credentialling intensive care physicians. However, it should be taken in context of the overall assessment process. If high stakes assessment requires a reliability coefficient of > 0.8, this value should be the benchmark for the combined oral examination including the Hot Cases and viva component. Further research is required to assess how the Hot Case component and the viva component interact to form the overall reliability of the oral examination.
The strengths of this study include the originality, the predefined statistical plan, the large cohort and the collaboration with the CICM to provide previously unexamined data for an independent analysis. Additionally, the use of descriptive statistics, G-theory analysis and D-studies provides a comprehensive picture of the Hot Case examination reliability in its current format.
Study limitations include dataset consistency issues that restricted the study period, the focus specifically on the Hot Case component without an in-depth analysis of the other components of the examination, the focus on traditional psychometric evaluation and the potential overestimation of examiner calibration due to revision of examiner scores after discussion. Evaluating examination performance without external measures of candidate ability is a research design that focuses on the examination itself. Assessment research is often not truly focussed on candidate competence as this is very difficult to study, so it inevitably evaluates the process rather than the product. As such, identifying poor reliability as a weakness of the Hot Case examination does not detract from potential validity in the overall examination process.
Several implications and unanswered questions remain. Firstly, examiners appear well calibrated, but discussion and score amendment may be significant. Secondly, with additional examiner time, reliability could be increased by challenging candidates with borderline results with additional cases upon which decisions are made. Thirdly, this research highlights the importance of a scoring rubric and robust processes for data capture. Finally, further research is required to assess how the Hot Case and viva examination interact to test the overall reliability of the oral examination. This should be supported by research aiming to assess the validity of the Hot Case as a method of evaluating clinical competence by comparing it with other forms of assessment and workplace competency.
Hot Cases have long been a method of assessment in ICU training in Australia and New Zealand, with perceived benefits from the perspective of stakeholder acceptance and educational impact. Changes to the current examination format to increase reliability would solidify its role in the credentialling process by addressing concerns within the ICU community.
The reliability of the CICM Hot Case examination is less than the generally accepted standard for a high stakes credentialling examination. Further examiner training is unlikely to improve the reliability as the examiners appear to be well calibrated. Modifications to case selection and the introduction of a clear scoring rubric to mitigate the effects of variation in case difficulty may be helpful, but are unlikely to improve reliability substantially due to case specificity. Increasing the number of cases and overall assessment time appears to be the best way to increase the overall reliability. Further research is required to assess how the Hot Case and viva results interact to quantify the reliability of the oral examination in its entirety, and to evaluate the validity of the examination format in making credentialling decisions.
Data availability
The datasets analysed during the current study are not publicly available, but are available from the corresponding author on reasonable request.
Burch VC, Norman GR, Schmidt HG, van der Vleuten CP. Are specialist certification examinations a reliable measure of physician competence? Adv Health Sci Educ Theory Pract. 2008;13(4):521–33. https://doi.org/10.1007/s10459-007-9063-5 .
Article Google Scholar
Norcini J, Anderson MB, Bollela V, Burch V, Costa MJ, Duvivier R, Hays R, Palacios Mackay MF, Roberts T, Swanson D. Consensus framework for good assessment. Med Teach. 2018;40(11):1102–9. https://doi.org/10.1080/0142159X.2018.1500016 .
Lee RP, Venkatesh B, Morley P. Evidence-based evolution of the high stakes postgraduate intensive care examination in Australia and New Zealand. Anaesth Intensive Care. 2009;37(4):525–31. https://doi.org/10.1177/0310057X0903700422 .
Turnbull J, Turnbull J, Jacob P, Brown J, Duplessis M, Rivest J. Contextual considerations in summative competency examinations: relevance to the long case. Acad Med. 2005;80(12):1133–7. https://doi.org/10.1097/00001888-200512000-00014 .
Memon MA, Joughin GR, Memon B. Oral assessment and postgraduate medical examinations: establishing conditions for validity, reliability and fairness. Adv Health Sci Educ Theory Pract. 2010;15(2):277–89. https://doi.org/10.1007/s10459-008-9111-9 .
Lane AS, Roberts C, Khanna P. Do we know who the Person with the Borderline score is, in standard-setting and decision-making. Health Prof Edu. 2020;6(4):617–25. https://doi.org/10.1016/j.hpe.2020.07.001 .
Miller GE. The assessment of clinical skills/competence/performance. Acad Med. 1990;65(9 Suppl):S63–7. https://doi.org/10.1097/00001888-199009000-00045 . PMID: 2400509.
College of Intensive Care Medicine of Australia and New Zealand. Second part examination: previous exam reports [Internet]. CICM. 2022 [updated 2023; cited 2023 Oct 30]. https://www.cicm.org.au/CICM_Media/CICMSite/Files/Exams/2022-1-SP-Exam-Report.pdf .
Hoffman K, Nickson CP, Ryan AT, Lane S. Too hot to handle? The validity and reliability of the college of intensive Care Medicine Hot Case examination. Crit Care Resusc. 2022;24(1):87–92. https://doi.org/10.51893/2022.1.L .
Van Der Vleuten C. The assessment of professional competence: developments, research and practical implications. Adv Health Sci Educ. 1996;1:41–67. https://doi.org/10.1007/BF00596229 .
Bloch R, Norman G. Generalizability theory for the perplexed: a practical introduction and guide: AMEE Guide 68. Med Teach. 2012;34(11):960–92. https://doi.org/10.3109/0142159X.2012.703791 .
Moonen-van Loon JM, Overeem K, Donkers HH, van der Vleuten CP, Driessen EW. Composite reliability of a workplace-based assessment toolbox for postgraduate medical education. Adv Health Sci Educ Theory Pract. 2013;18(5):1087–102. https://doi.org/10.1007/s10459-013-9450-z .
Crossley J, Davies H, Humphris G, Jolly B. Generalisability: a key to unlock professional assessment. Med Educ. 2002;36(10):972–8. https://doi.org/10.1046/j.1365-2923.2002.01320.x .
Weller JM, Castanelli DJ, Chen Y, Jolly B. Making robust assessments of specialist trainees’ workplace performance. Br J Anaesth. 2017;118(2):207–14. https://doi.org/10.1093/bja/aew412 .
Cronbach LJ, Gleser GC, Nanda H, Rajaratnam N. The dependability of behavioral measurements: theory of generalizability for scores and profiles. New York: Wiley; 1972.
Google Scholar
Angoff WH. Scales, norms, and equivalent scores. Educational Measurement. Second Edition. Washington: American Coucil on Education; 1971.
Karcher C. The Angoff method in the written exam of the College of Intensive Care Medicine of Australia and New Zealand: setting a new standard. Crit Care Resusc. 2019;21(1):6–8.
Wilkinson TJ, Campbell PJ, Judd SJ. Reliability of the long case. Med Educ. 2008;42(9):887–93. https://doi.org/10.1111/j.1365-2923.2008.03129.x .
Tey C, Chiavaroli N, Ryan A. Perceived educational impact of the medical student long case: a qualitative study. BMC Med Educ. 2020;20:257. https://doi.org/10.1186/s12909-020-02182-6 .
Sim J, Daniel E. The long case as an assessment tool of clinical skills in summative assessment: a necessary evil. Int Med J. 2015;22:537–40.
Kane MT. Validating the interpretations and uses of test scores. J Educ Meas. 2013;50:1–73.
Swanson DB, Norman GR, Linn RL. Performance-based assessment: lessons from the health professions. Educ Res. 1995;24:5e11.
Wass V, Jolly B. Does observation add to the validity of the long case? Med Educ. 2001;35(8):729–34. https://doi.org/10.1046/j.1365-2923.2001.01012.x .
Van Der Vleuten C, Schuwirth L. Assessing professional competence: from methods to programmes. Med Educ. 2005;39(3):309–17. https://doi.org/10.1111/j.1365-2929.2005.02094.x .
Dijkstra J, Galbraith R, Hodges B, et al. Expert validation of fit-for-purpose guidelines for designing programmes of assessment. BMC Med Educ. 2012;1712:20. https://doi.org/10.1186/1472-6920-12-20 .
Download references
The CICM provided staff time for data extraction and de-identification prior to release. There was no additional funding provided for the research.
Author information
Authors and affiliations.
Intensive Care Unit, The Alfred Hospital, Melbourne, Australia
Kenneth R. Hoffman & Chris Nickson
Department of Epidemiology and Preventative Medicine, School of Public Health, Monash University, Melbourne, Australia
Department of Medical Education, Melbourne Medical School, University of Melbourne, Melbourne, Australia
David Swanson & Anna T. Ryan
Sydney Medical School, The University of Sydney, Sydney, Australia
Stuart Lane
College of Intensive Care Medicine of Australia and New Zealand, Melbourne, Australia
You can also search for this author in PubMed Google Scholar
Contributions
KH conceived and designed the study, analysed and interpreted the data and drafted and revised the manuscript. DS designed the study, performed the analysis, interpreted the data and revised the manuscript. SL contributed to the conception, design and interpretation of the study and revised the manuscript. CN contributed to the conception, design and interpretation of the study and revised the manuscript. PB contributed to data acquisition and analysis and revised the manuscript. AR contributed to the conception, design and interpretation of the study and revised the manuscript.
Corresponding author
Correspondence to Kenneth R. Hoffman .
Ethics declarations
Ethical approval and consent to participate.
Ethics approval was provided by the University of Melbourne Low Risk Human Ethics Committee (Project number 2022-23964-28268-3). The consent requirement was waived by the University of Melbourne Low Risk Human Ethics Committee as analysis was retrospective using de-identified data with no foreseeable risk to participants in accordance with the Australian National Statement on Ethical Conduct in Human Research 2023.
Consent for publication
Not applicable.
Competing interests
KH (Fellow of the CICM), AR (No conflicts of interest), SL (Fellow of the CICM, CICM Second Part examiner 2011-2023, CICM Second Part examination committee 2019-2023, Chair of the CICM Second Part examination panel 2020-2023, CICM First Part examiner 2012-2019), CN (Fellow of the CICM, CICM First Part examiner 2017-2023, CICM First Part examination committee 2019-2023, CICM Supervisor of Training 2018-2023), DS (No conflicts of interest), PB (Employed by the CICM in the position of Information, Communication and Technology Manager).
Additional information
Publisher’s note.
Springer Nature remains neutral with regard to jurisdictional claims in published maps and institutional affiliations.
Rights and permissions
Open Access This article is licensed under a Creative Commons Attribution 4.0 International License, which permits use, sharing, adaptation, distribution and reproduction in any medium or format, as long as you give appropriate credit to the original author(s) and the source, provide a link to the Creative Commons licence, and indicate if changes were made. The images or other third party material in this article are included in the article’s Creative Commons licence, unless indicated otherwise in a credit line to the material. If material is not included in the article’s Creative Commons licence and your intended use is not permitted by statutory regulation or exceeds the permitted use, you will need to obtain permission directly from the copyright holder. To view a copy of this licence, visit http://creativecommons.org/licenses/by/4.0/ . The Creative Commons Public Domain Dedication waiver ( http://creativecommons.org/publicdomain/zero/1.0/ ) applies to the data made available in this article, unless otherwise stated in a credit line to the data.
Reprints and permissions
About this article
Cite this article.
Hoffman, K.R., Swanson, D., Lane, S. et al. The reliability of the College of Intensive Care Medicine of Australia and New Zealand “Hot Case” examination. BMC Med Educ 24 , 527 (2024). https://doi.org/10.1186/s12909-024-05516-w
Download citation
Received : 21 February 2024
Accepted : 03 May 2024
Published : 11 May 2024
DOI : https://doi.org/10.1186/s12909-024-05516-w
Share this article
Anyone you share the following link with will be able to read this content:
Sorry, a shareable link is not currently available for this article.
Provided by the Springer Nature SharedIt content-sharing initiative
- Intensive care
- Examination
- Credentialling
- Reliability
- Generalisability theory
BMC Medical Education
ISSN: 1472-6920
- Submission enquiries: [email protected]
- General enquiries: [email protected]

Case CCC Group Studies the Impact of Clinical Trial Design on Recruitment of Diverse Populations
An inter-organizational group of researchers and administrators from Case CCC recently published their work, "Impact of clinical trial design on recruitment of racial and ethnic minorities," in the Journal of Cancer Education. Case CCC affiliates include Population and Cancer Prevention Program members Debora Bruno, MD, University Hospitals (UH); Yilun Sun, PhD, Case Western Reserve University (CWRU) School of Medicine (SOM); Shearwood McClelland, MD, UH; Cancer Imaging Program member Jennifer Dorth, MD, UH; Developmental Therapeutics member Smitha Krishnamurthi, MD, Cleveland Clinic; Case CCC Clinical Research Office Director Kevin Hoy, PhD; and staff member Bob Lanese, MS.
To develop effective and safe cancer therapies for a diverse population, it is essential for treatment trials to accrue robust differences in age, gender, race, and ethnicity among and between participants. However, studies show that racial and ethnic minorities, women, and older adult patients are least likely to enroll in oncology treatment trials compared to White patients, men, and younger populations.
Thus, in this study, the group questioned how oncology treatment trial design influences enrollment of racial and ethnic minorities. While extant literature on this topic is limited, the researchers suggest that rigorous identification of clinical trial design parameters associated favorably with minority accrual provides educational opportunities for individuals interested in designing more representative treatment trials. Read their method and what they observed by clicking the link below.
Read the Article
Thank you for visiting nature.com. You are using a browser version with limited support for CSS. To obtain the best experience, we recommend you use a more up to date browser (or turn off compatibility mode in Internet Explorer). In the meantime, to ensure continued support, we are displaying the site without styles and JavaScript.
- View all journals
- My Account Login
- Explore content
- About the journal
- Publish with us
- Sign up for alerts
- Open access
- Published: 21 May 2024
Optimizing multi-spectral ore sorting incorporating wavelength selection utilizing neighborhood component analysis for effective arsenic mineral detection
- Natsuo Okada 1 ,
- Hiromasa Nozaki 1 ,
- Shinichiro Nakamura 1 ,
- Elsa Pansilvania Andre Manjate 2 ,
- Angesom Gebretsadik 1 , 4 ,
- Yoko Ohtomo 3 ,
- Takahiko Arima 3 &
- Youhei Kawamura 3
Scientific Reports volume 14 , Article number: 11544 ( 2024 ) Cite this article
Metrics details
- Environmental sciences
- Imaging and sensing
- Scientific data
- Optical spectroscopy
Arsenic contamination not only complicates mineral processing but also poses environmental and health risks. To address these challenges, this research investigates the feasibility of utilizing Hyperspectral imaging combined with machine learning techniques for the identification of arsenic-containing minerals in copper ore samples, with a focus on practical application in sorting and processing operations. Through experimentation with various copper sulfide ores, Neighborhood Component Analysis (NCA) was employed to select essential wavelength bands from Hyperspectral data, subsequently used as inputs for machine learning algorithms to identify arsenic concentrations. Results demonstrate that by selecting a subset of informative bands using NCA, accurate mineral identification can be achieved with a significantly reduced the size of dataset, enabling efficient processing and analysis. Comparison with other wavelength selection methods highlights the superiority of NCA in optimizing classification accuracy. Specifically, the identification accuracy showed 91.9% or more when utilizing 8 or more bands selected by NCA and was comparable to hyperspectral data analysis with 204 bands. The findings suggest potential for cost-effective implementation of multispectral cameras in mineral processing operations. Future research directions include refining machine learning algorithms, exploring broader applications across diverse ore types, and integrating hyperspectral imaging with emerging sensor technologies for enhanced mineral processing capabilities.
Similar content being viewed by others
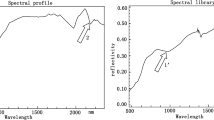
Application of hyperspectral remote sensing for supplementary investigation of polymetallic deposits in Huaniushan ore region, northwestern China
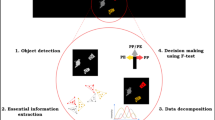
Systematic reduction of hyperspectral images for high-throughput plastic characterization
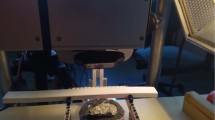
A 2D hyperspectral library of mineral reflectance, from 900 to 2500 nm
Introduction.
Mineral-intensive technologies such as renewable energy and electric vehicles will be in high demand as climate change is addressed and a sustainable energy future is transitioned. Despite this, the mining sector, especially in copper, is facing considerable difficulties due to the growing demand 1 , 2 . The depletion of high-grade ore and the rise of high-arsenic copper resources are more prominent issues in this field. Arsenic in low-grade copper ores not only makes mineral processing more difficult, but also causes environmental and health concerns due to the presence of arsenic in wastewater and exhaust gas 3 , 4 . The correlation between arsenic exposure and a range of health problems highlights the pressing requirement for ecologically viable methods in the mining sector. The incorporation of modern technologies such as hyperspectral imaging into sensor-based ore sorting systems has significant potential in this situation 5 . Ore sorting systems can successfully separate high-arsenic ores from valuable material by utilizing the precise and accurate analysis of mineral composition provided by hyperspectral imaging. This not only mitigates environmental dangers but also reduces processing costs. This strategy not only tackles the difficulties presented by ores containing high levels of arsenic, but also aids in the advancement of a mining industry that is environmentally friendly, in line with the objectives of the Paris Agreement.
Sensor-based ore sorting has become a crucial technology in mineral processing, providing numerous advantages that transform conventional mining methods. With sensor-based ore sorting systems, valuable minerals can be selectively extracted from ore streams according to their unique physical and chemical properties based on advanced sensor technologies. This process of selective extraction maximizes the efficient use of resources by effectively separating valuable ore from nonvaluable materials (or gangue minerals). In the field of mineral processing, Sensor-based ore sorting is a vital component as it enhances ore grades and minimizes the amount of waste material that is processed 6 . Evidence demonstrates that it effectively decreases the usage of energy, water, and reagents, while also minimizing the formation of fine waste, by disposing of trash before undergoing additional processing 7 , 8 . To successfully apply sensor-based sorting, it is crucial to select a sensing approach that can efficiently distinguish between ore and waste 9 . Sensor fusion, the integration of data from several sensing systems, has the potential to enhance the characterization of the detected material and improve sorting capability 4 . Microwave imaging (MWI) is a promising technique that can penetrate deeply into rock particles and serve as an additional approach for analyzing ores with significant differences in electromagnetic characteristics 10 . The efficacy of MWI in ore sorting has been validated by simulations and tests, affirming its capability to segregate valuable minerals or metals from unproductive particles. The utilization of sensor-based ore sorting presents substantial advantages in terms of reducing costs and enhancing efficiency in mineral processing.
Hyperspectral imaging
Ore sorting techniques can be significantly enhanced by leveraging hyperspectral imaging technology, which offers unparalleled capabilities for mineral characterization and classification. Hyperspectral imaging allows ore sorting systems to analyze the distinct spectral fingerprints of minerals over a wide range of wavelengths, unlike traditional sorting methods that only consider physical attributes like size, shape, and density. This enables the identification and differentiation of minerals by analyzing their unique chemical compositions and optical features. Hyperspectral imaging is used in sensor-based ore sorting to analyze ore streams in real-time without causing damage 5 . This technique offers important details on the mineralogy and quality of the material being processed. By using hyperspectral imaging technology into sorting systems, mining companies can enhance their efficiency, precision, and selectivity in segregating valuable minerals from waste material. As a result, mineral processing enterprises have higher rates of recovery, lower costs of processing, and increased profitability.
Curse of dimensionality and feature reduction
The processing of hyperspectral data is more challenging than that of other types of data due to the sheer volume of information collected, which may be affected by issues with its dimensions. High-dimensional spectral bands in hyperspectral images are often highly similar, which makes them susceptible to the "curse of dimensionality," a phenomenon that affects many traditional algorithms 11 . Within the domain of hyperspectral ore sorting systems, the notion of wavelength selection arises as a crucial strategy for enhancing sorting efficiency and precision. Wavelength selection is the process of strategically identifying and using wavelengths of electromagnetic radiation (light) that provide the most useful information for differentiating between various minerals or compounds in an ore stream. Through the analysis of distinct spectral patterns displayed by minerals at different wavelengths, the process of wavelength selection allows ore sorting systems to concentrate on the specific spectral bands that are most effective in distinguishing the desired minerals. By employing this focused method, the precision, effectiveness, and dependability of mineral identification and separation procedures are enhanced, resulting in better utilization of resources and increased operational performance in mineral processing. The process of choosing the right wavelength is also extremely important to reduce the likelihood of incorrect positive and negative results, maximize the rate at which valuable minerals are recovered, and to reduce the waste stream losses of potentially valuable materials. The significance of ore sorting lies in its ability to facilitate efficient and precise separation of valuable ore from waste or gangue materials. Based on their unique reflectance or absorption properties, sensors can effectively distinguish ore from gangue by using specific wavelengths, such as visible or mid-infrared ones. This enhances the system's ability to choose and efficiently sort materials, especially when working with intricate ores or comparable substances. Utilizing wavelength selection can improve the ability of photometric sensors to distinguish between different substances and simplify the creation of new sensors for the purpose of sorting ores and characterizing minerals 12 . A variety of advanced techniques are used to analyze multidimensional spectrum data and extract relevant features from hyperspectral data, such as spectral features extraction and machine learning algorithms i.e. linear regression, K-means clustering, neural network 13 , 14 , 15 .
The intricate nature and extensive dimensions of multi-spectral data require the application of sophisticated data classification techniques such as Neighborhood Component Analysis (NCA). Advanced data classification techniques like NCA are needed to handle multi-spectral data due to several reasons. To begin with, hyperspectral data typically includes a substantial number of spectral bands, which might provide computing difficulties during processing and analysis 16 . The issue can be addressed by using NCA, which involves lowering the dimensionality of the data. This would lead to improved processing and classification efficiency 17 . Additionally, it is essential to note that conventional classification methods designed for multispectral data may not be appropriate for hyperspectral data, as the latter offers more intricate and comprehensive spectral information 18 . The NCA method can effectively handle hyperspectral data with a high number of dimensions. It achieves improved classification accuracies by taking into account both spectral and spatial information 19 . Additionally, NCA offers advantages such as low computational requirements and shorter classification times 20 . Therefore, advanced techniques like NCA are essential for accurately classifying hyperspectral data while overcoming the challenges associated with high dimensionality and detailed spectral information.
In this study, Neighborhood Component Analysis (NCA) was applied as a preprocessing step to reduce the dimension of Hyperspectral (HS) data of arsenic-bearing minerals by identifying several wavelength bands important for mineral identification. Then the identified wavelength bands were used as inputs to train machine learning algorithms for identifying Arsenic (As) minerals concentration in simulated ore materials. Multispectral (MS) cameras are more cost-effective and provide faster data collecting and processing compared to HS cameras; hence, they are projected to enable mineral identification utilizing data from a few wavelength bands. The HS data of arsenic-bearing minerals (enargite) were used NCA a machine learning method, as a band selector, and identified several wavelength bands important for mineral identification. Then, the data containing only the minimum number of wavelengths were analyzed for identification of mineral contents/ratios using machine learning algorithms. These will improve the selectivity of wavelengths, considering the ore characteristics produced by each mine. In addition, the application of the herein proposed machine learning algorithm for HS images analysis is expected to improve the efficiency of ore selectivity, i.e. improve the speed of the ore sorting process.
Literature review
To develop environmentally sustainable resources, it’s essential to develop advanced metal recovery technology for these high-grade arsenic ores, and Sensor-Based Ore Sorting (SBOS) can achieve this. SBOS, when implemented as a presorting process before the normal beneficiation process, can reduce the amount of ore that must be processed to produce a certain amount of value-added metal, which has a significant impact on the economics of the mine and the plant as a whole 21 , 22 , 23 . It can also reduce the environmental impact by reducing the tailings produced in the subsequent beneficiation process. Non-destructively classified tailings are geotechnically stable and can be easily stored due to their low moisture content 24 . Robben and Wotruba highlighted that the introduction of SBOS would have an impact on both the environmental and economic aspects of the mineral processing process 25 . However, the authors pointed out that SBOS is still in the market entry stage of the mineral industry and further technological development is required.
Mineral analysis requires knowledge of crystallography as well as chemical analysis 26 . However, the methods commonly used for mineral analysis, such as Electron Probe Micro Analyzer (EPMA), X-ray diffraction (XRD) and Scanning Electron Microscope (SEM), are relatively time-consuming and depend on experience 27 , they are not realistic in terms of identification speed, convenience, and economy when used in actual mineral processing operations.
Therefore, SBOS has been developed as a form of mineral identification suitable for beneficiation. In recent years, more and more equipment has been installed that can withstand larger production scales 25 . SBOS methods have utilized a range of sensing technologies, including X-ray transmission, X-ray fluorescence, optical sensing, and inductive sensing 9 , 10 , 28 . Furthermore, the utilization of area-scan cameras and predictive tracking systems that rely on machine learning approaches have demonstrated potential in minimizing characterization and separation errors 29 . Researchers have also studied the combination of data from several sensing approaches to improve the sorting ability of these systems 6 . While different SBOS methods have been developed and introduced particularly focusing on SWIR data, there are few studies or methods on mineral identification/sorting using VNIR short-wavelength HS data. However, there is growing interest in visible to near-infrared (VNIR) spectroscopy for mineral identification, and in some recent studies VNIR wavelengths have been used to classify rocks and minerals 30 , 31 .
Sensor-based ore sorting methods and technologies have the potential to significantly improve ore grades and reduce waste in mineral processing 6 . These methods, which rely on the electromagnetic spectrum, can be classified based on their characteristics and limitations 28 . An example of a successful method for sorting complicated ores is the utilization of hyperspectral short-wave infrared sensors in conjunction with machine learning, as demonstrated by Tusa 32 . Sensor-based ore sorting can be applied at various stages in the process flow diagram, making it a versatile and valuable tool in the mining industry 33 .
In the field of remote sensing, mineral identification in the near-infrared region has been widely used 34 , 35 and they have shown excellent performance in ore classification. On the other hand, the high cost of HS cameras and the time required for data acquisition have been barriers to their application in actual operations, where immediate classification is required. In a previous study 24 , 36 , HS data of minerals were analyzed by deep learning to identify minerals. The use of deep learning allows the creation of more versatile and simplified learning models compared to conventional machine learning or identification methods that combine multiple machine learning models. However, since HS images consist of several hundred spectral bands, there is a high correlation between proximity spectra, and data analysis without preprocessing is highly redundant and computationally intensive. Therefore, dimensionality reduction is necessary as a preprocessing step for a large amount of data to be generated.
Dimensionality reduction methods for HS commonly fall into two categories: band extraction and wavelength selection. The band extraction methods map a high-dimensional feature space to a low-dimensional space; therefore, cannot preserve the original physical interpretation of the image and is not applicable as a dimensionality reduction method 37 . While the wavelength selection method can maintain the original physical interpretation of the images. According to a review by Sun and Du 38 , wavelength selection methods can be categorized into six groups: ranking-based methods, searching-based methods, clustering-based methods, sparsity-based methods, embedding learning-based methods, and hybrid scheme-based methods.
A variety of studies have explored different techniques for wavelength selection and spectral data classification in mineral processing. Ghosh 39 introduced an infrared thermography-based method for sorting alumina-rich iron ores, while Kern 40 suggested utilizing short-wavelength infrared and dual-energy X-ray transmission sensors for the Hammerlein Sn–In–Zn deposit. Phiri 41 investigated the potential of near-infrared sensors for separating carbonate-rich gangue from copper-bearing particles in a copper–gold ore sample. Tusa 32 advanced the field by evaluating hyperspectral short-wave infrared sensors, combined with machine learning methods, for pre-sorting complex ores. These studies collectively illustrate the potential of various wavelength selection techniques for enhancing the efficiency and effectiveness of ore sorting systems.
Furthermore, numerous research endeavors have investigated the implementation of machine learning algorithms to automate the task of wavelength selection and spectral data classification. Passos 42 introduced an automated deep learning pipeline to optimize neural architecture and hyperparameters for spectral classification. Duan 43 proposed a template matching approach achieving high accuracy without training, while Wang 44 developed a multifunctional optical spectrum analysis technique utilizing support vector machines for optimal accuracy and speed. Baskir 45 presented a MATLAB toolbox for user-friendly wavelength selection and automated spectral region selection. These investigations collectively underscore the potential of machine learning in automating and enhancing the process of wavelength selection and spectral data classification.
In addition to this, Advancements in hyperspectral imaging technology have significantly expanded the potential applications of this technology 46 . However, the complexity of hyperspectral data, including its high dimensionality and size, requires innovative methodologies for effective processing and analysis 47 . These challenges have led to the development of a range of image processing and machine learning analysis pipelines 46 . Notably, hyperspectral imaging finds application in microscopy, enabling the capture and identification of different spectral signatures in a single-pass evaluation 48 .
The effectiveness of machine learning algorithms, particularly Neighborhood Component Analysis (NCA), for multi-spectral data classification in mineral processing has been highlighted in recent research. Jahoda 49 and Sinaice 50 both emphasize the advantages of combining spectroscopic methods with machine learning for mineral identification. Jahoda 49 specifically highlights the superiority of machine learning methods in this context, while Sinaice 50 proposes a system integrating hyperspectral imaging, NCA, and machine learning for rock and mineral classification. These findings are further supported by Carey 51 , who stresses the importance of spectrum preprocessing and a weighted-neighbors classifier for optimal mineral spectrum matching performance.
In their previous study Okada et al. 24 , developed a basic technology of SBOS, using hyperspectral (HS) imaging and deep learning as an expert system for mineral identification. HS is promising as SBOS to avoid As-containing copper minerals technic instead. In that study, HS imaging was used as a sensor to collect the intensity of wavelength data, which was then used to train deep learning algorithms for mineral identification. The HS image is cube-shaped data with dimensions in the wavelength and spatial directions, with wavelength data from the visible to near-infrared regions (400 ~ 1000 nm, 204 bands). Minerals (hematite, chalcopyrite, galena) identification was performed by analyzing detailed wavelength data of 204 bands in the shorter wavelength range of 400–1000 nm (from the visible light region to a part of the near-infrared region) using deep learning. However, the HS data used in that study consisted of 204 high-dimensional data, which required heavy computational resources. In addition, the HS camera itself is expensive, which was a barrier to its introduction/implementation in the operating site (mineral processing plant).
Yokoya and Iwasaki 52 reported that, since each pixel provides continuous electromagnetic spectral characteristics, it’s possible to obtain detailed information about the target object. Owing to the high spectral resolution HS imaging is applied in fields such as remote sensing and quality control of food deep and pharmaceuticals. Robben et al. 53 pointed out that, minerals show specific absorption characteristics in the near-infrared region from 1300 to 2550 nm due to vibrations of the bonding molecules contained in each mineral. A skilled expert can identify some minerals visually (Color), and the continuous electromagnetic spectrum in the short wavelength region is considered to contain optical data with mineral-specific physical properties.
Sorting machines that use MS images with a reduced number of dimensions are now technically feasible. They sort by acquiring specific wavelength information predetermined for each ore type. However, even for the same type of mineral, there are subtle differences in the formation and shape of each mine that affect the spectra. Additionally, the light environment inside each plant varies, which also affects the spectrum. Based on these factors, it is suggested that ore selectivity could be improved by defining the wavelength to be acquired for each ore type. To achieve this, we propose a framework that allows for the selection of spectral bands based on the characteristics of the ore produced. This framework will greatly support the tuning of the sorting process. As a case study, we will use a mineral sample containing arsenic.
The literature review highlights various gaps in current mineral processing practices, emphasizing the need for innovative approaches to improve efficiency and sustainability. While Sensor-Based Ore Sorting (SBOS) offers promise for environmentally friendly metal recovery, further technological development is required to enhance its effectiveness and practicality in operational settings. Traditional mineral analysis methods are time-consuming and impractical for real-time processing, prompting the exploration of faster and more economical techniques. Additionally, the application of machine learning algorithms and hyperspectral imaging for mineral identification presents computational challenges and limitations in the practical implementation due to the high dimensionality of data.
In response to these challenges, the proposed framework integrates Neighborhood Component Analysis (NCA) and machine learning algorithms to address the complexities of mineral identification and sorting using multi-spectral data. By reducing data dimensionality and identifying crucial wavelength bands, the framework enables efficient mineral identification while considering the unique characteristics of each ore type. Furthermore, by utilizing multi-spectral cameras with reduced dimensions, the framework enhances sorting efficiency and selectivity, paving the way for more sustainable mining practices and improved operational outcomes in mineral processing plants. In this study, a clustering-based method, Neighborhood Components Analysis (NCA), was used to perform dimensionality reduction and wavelength selection on HS data. After the selection, the selected bands were learned by machine learning algorithms to experiment with mineral identification. It is expected that mineral identification using fewer wavelengths than HS data will enable data acquisition with less expensive MS cameras and increase the efficiency of mineral identification.
Methodology
This study was conducted according to the flowchart in Fig. 1 . After acquiring hyperspectral data, dimensionality reduction is performed using NCA to enable mineral identification using a multispectral camera.
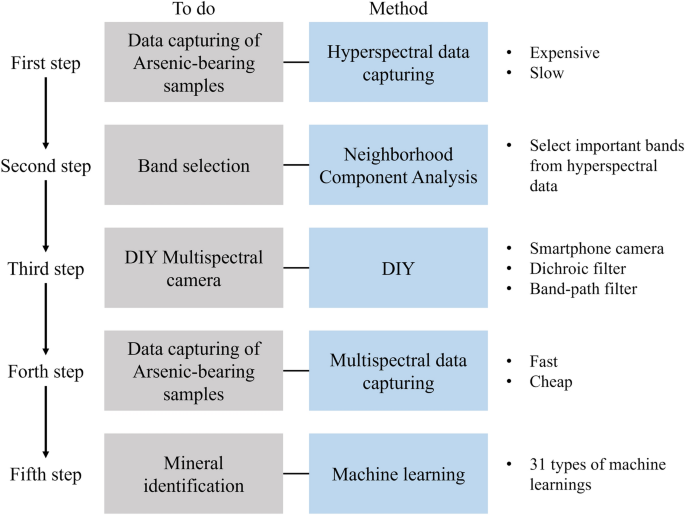
A summary of a flowchart of the research.
Methodology for hyperspectral (HS) imaging
A HS camera is a camera that can acquire intensity of wavelength data with more than 100 spectrally oriented wavelength bands in addition to two-dimensional planar data. Spectral Imaging's Specim IQ 54 , used in this study, can capture the intensity of wavelengths from 400 to 1000 nm. It is capable of spectroscopy in 204 wavelength bands and can capture continuous electromagnetic spectra for each wavelength of approximately 3 nm. Minerals are thought to have a unique intensity of wavelength patterns, and these characteristic intensities of wavelength patterns were learned using machine learning.
Bands selection using neighborhood component analysis (NCA)
The high redundancy of HS data and the resulting high dimensional data are fatal flaws in SBOS, which require high-speed ore sorting. Therefore, by using NCA, only the most important wavelength bands of HS data are selected, and the MS sensor installed in SBOS acquires only those bands for faster analysis. Figure 2 shows the flow of wavelength selection by NCA.
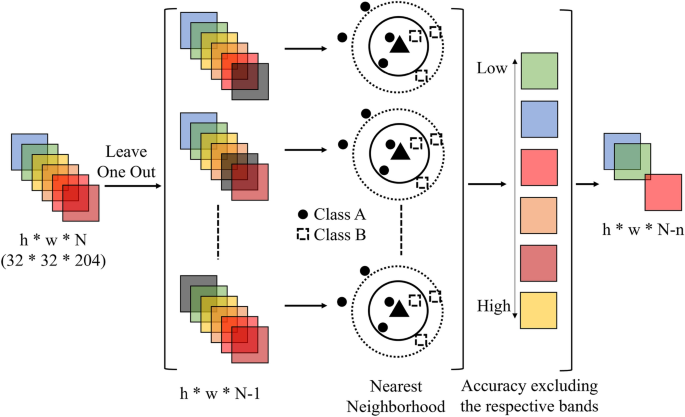
An overview of the NCA algorithm, which combines the Leave-One-Out and neighborhood methods to indicate the importance of the input bands.
NCA is a type of machine learning algorithm/technique used for wavelength selection as well as clustering. It is a nonparametric process for a dataset and does not require parametric assumptions such as normal distribution. The data learning method uses a data classification method called Leave-One-Out (LOO), in which only one example is extracted from a set of n data as test data and the rest as training data, and the validation is repeated so that all the data become test data one at a time. Index number is represented as i . In NCA, the LOO probability was calculated to optimize the distance function with the weight as a function of probability p , as shown in Eq. ( 1 ) 55 , 56 .
Here, transforming the right-hand side of F(w) which is the function of weight w , is shown as in Eqs. ( 2 )–( 4 ).
To obtain the weight vector w , f(w) = -F(w) and f i (w) = -F i (w) , as in Eq. ( 5 ) below. The weight vector w was obtained by analyzing HS data with NCA to identify the wavelength bands that are important for mineral identification.
In this study, the formulas shown in Eqs. ( 1 ) through (3) were run using MATLAB. NCA was performed on hyperspectral data. The weights were calculated by Eq. ( 3 ).
- Machine learning
Edgar and Manz 57 defined machine learning as a field of study that looks at using computational algorithms to turn empirical data into usable models. In this study, the wavelength bands that are important for mineral identification among the mineral wavelength data were selected using NCA. These bands were then used as input to train a total of 33 different types of machine learning algorithms, as shown in Table 1 . The use of a variety of machine learning algorithms allows for a comprehensive evaluation of overall performance. However, it is important to address the concerns raised regarding the number of classifiers and the parameter configurations.
A diverse set of 33 machine learning algorithms was employed to comprehensively evaluate their performance. Although the use of numerous classifiers might seem excessive, it was intended to ensure a thorough exploration of different methodologies. However, it is acknowledged that optimizing parameters for each classifier is necessary to achieve maximum accuracy. Instead of providing detailed parameter configurations, the number of wavelengths (from 1 to 100 bands) was varied to determine the optimal number of bands for each individual method. Furthermore, rigorous methods, including cross-validation, were employed to fine-tune the parameters of each classifier, thereby ensuring the robustness of the results.
Case study and experimental procedures
Figure 3 shows the experimental situation and conceptual diagram of the imaging experiment. The experiments were conducted in a dark room to avoid light source interference. In addition, to avoid shadows on the sample, the target ore was illuminated from two directions by halogen lights from the left, and right of the camera. Halogen lights can uniformly illuminate the wavelength range from 500 to 3000 nm, which is the wavelength range used in this study. The filament of the halogen light heats up to a very high temperature as time passes, and the wavelength peak of the halogen light shifts to a higher wavelength. However, prior experiments confirmed that the effect was negligible.
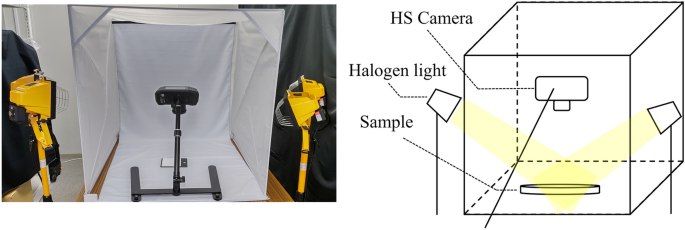
The sample is illuminated from two directions by a halogen light source, and the wavelength of the reflected light from the sample is acquired with a HS camera. The left figure shows the actual experimental scene, and the right figure shows the schematic diagram of the experiment.
The surface of the powdered mineral was smoothed and photographed from 15 cm away. Although a single exposure takes about one minute, depending on the exposure time, the camera is heated strongly by the illumination;therefore, the camera was used for only about one hour. The illuminance of the light source was measured at each shooting with an illuminance meter. However, since the camera automatically adjusts the exposure time and corrects the illumination using a white plate, the effects of these factors were disregarded from the analysis test conducted beforehand. Before photographing the sample, it’s necessary to photograph a White Reference as a way of calibrating the camera. Thus, the intensity of wavelength data acquired in subsequent photography is obtained as the reflection intensity relative to the reflection intensity of the White Reference. The HS data taken by the camera can be shown as the intensity of wavelength data of wavelengths on the computer. The data acquired by a single HS camera is represented as a three-dimensional data cube with depth in the wavelength direction in addition to the vertical and horizontal quadratic planes. There are 512 pixels in the vertical plane, 512 pixels in the horizontal plane, and 204 pixels in the wavelength band that corresponds to the depth part. In this study, the vertical and horizontal pixels were divided into 16 equal parts to create 256 data cubes of 32 pixels each in the vertical and horizontal directions and 204 pixels in the depth direction. Each of these divided data cubes was then averaged to be 1 pixel in height and width, and the data set shown in Fig. 4 was prepared with 1 pixel in height and width and 204 pixels in depth.
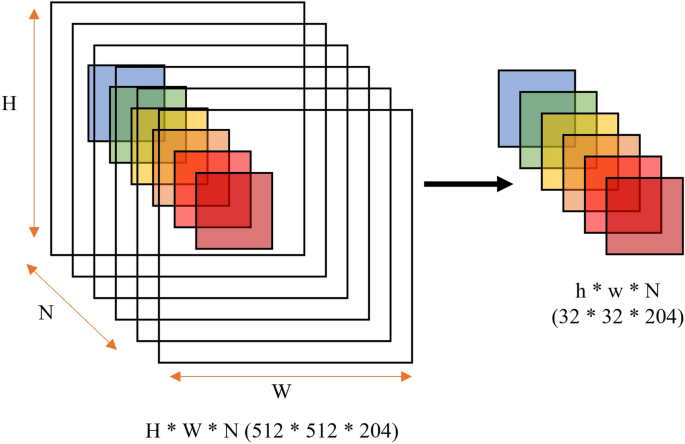
Pre-processing method for HS data, where H is height, W is width, and N is the number of bands. The data were cut out along the band direction to have height h and width w.
Target minerals for the experiment
Fourteen samples with different concentrations of arsenic, copper, and iron as shown in Table 2 were prepared by mixing powdered copper ore deposits collected from the Chuquicamata Mine 58 and powdered quartz as shown in Fig. 5 . The copper ore deposits comprise 76.98% of quartz and 8.74% of sulfide minerals (enargite, chalcopyrite, pyrite) with other minor components. The main host mineral for As is enargite Cu3AsS4. Ore samples with various grades were simulated by dilution of powdered quartz. By making the samples in powder form, it was possible to distribute the components evenly, and the acquired HS data were thought to contain the intensity of wavelengths of each mineral component evenly. Each sample was diluted with powdered quartz to achieve the same concentration (in weight ratio units) within the group. In smelters, a penalty occurs at arsenic concentrations of 0.2–0.5% or higher in the copper ore to be smelted/processed; therefore, it’s necessary to determine the arsenic concentration. The arsenic grades used as samples in this study were of the order of 0.2% to 0.5%, or arsenic concentration were aligned to investigate whether they are detectable for even lower As concentration. Group A was prepared for those with relatively high arsenic concentrations (avg. 0.23 wt%), Group B for those with moderate concentrations (avg. 0.10 wt%), and Group C for those with low arsenic concentrations (0.06 wt%). The chemical composition of each sample was determined using an Inductively Coupled Plasma (ICP) optical emission spectrometer (SPS-5510, Hitachi High-tech, Tokyo, Japan. SII became a 100% subsidiary of Hitachi High-tech in 2013). As, Cu, Fe and En (enargite) concentrations in each sample are shown in Table 2 . After capturing HS data using the HS camera, the elements were used in machine learning.
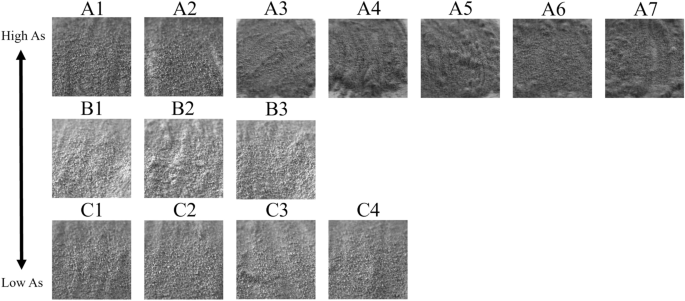
Samples images of 14 arsenic samples diluted by quartz.
Acquired hyperspectral (HS) data
The averaged HS data of the test samples A, B, and C obtained by the HS camera (14 types in total) are shown in Fig. 6 . The horizontal axis represents the wavelength (nm), the vertical axis represents the relative intensity normalized by White Reference, and each line represents the HS data obtained from the captured sample. Each HS line corresponds to the average of the data contained in 16 × 16 pixels, from A1 to C4, with different colors for each type. The overall trend is that the data is almost horizontal in the visible light region (400–800 nm), but characteristic protrusions are seen in some parts of the data. In the near-infrared region (800–1000 nm), the reflection intensity tends to increase. The reflection intensity at both ends of the spectrum is also higher. In other wavelength bands as well, the reflectance intensity shows many peaks of up and down fluctuations that cannot be observed by the human naked eye. In addition, the overall reflection intensity tends to increase as the quartz concentration increases, because the dark, low reflection intensity enargite was diluted with white quartz, which has a high reflection intensity. However, in the preliminary research 36 using color images conducted beforehand, it was difficult to determine the color of the sample surface, i.e., only by the size of the reflectance intensity. In practical/field application of the SBOS, the light source environment varies depending on the location where the SBOS is installed, and the reflection intensity is thought to vary depending on the light source environment.
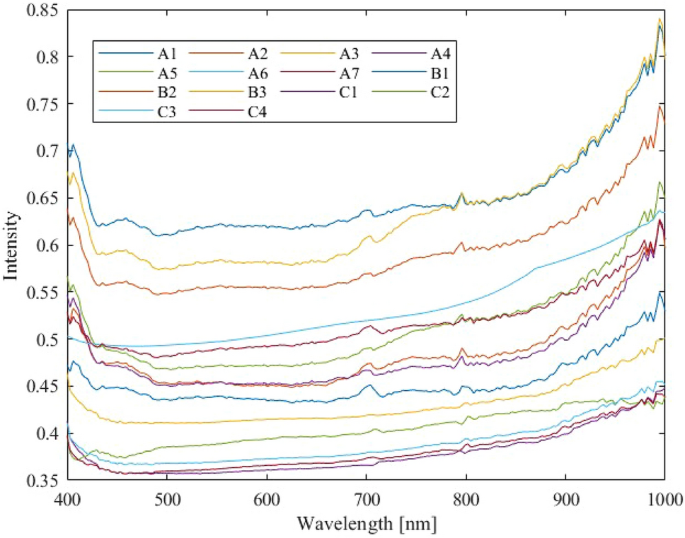
Distinctive protrusions appear at 700 nm and 800 nm; the spectral intensity tends to increase in the near-infrared region after 800 nm.
Although there are differences in the degree of peaking and the shape of the intensity of wavelengths depending on the spectral intensity of each sample, the basic shape of the wavelengths remains unchanged. These acquired data are now input to the machine learning algorithms which are trained to learn features of the data to identify As concentration.
Result and discussion
Analysis of machine learning using selected bands by neighborhood component analysis (nca), bands selection using nca.
NCA was performed on the input HS data, and the weights, which indicate the importance of each wavelength band in mineral identification, were calculated as shown in Fig. 7 . The vertical axis shows the feature weights, and the horizontal axis shows the wavelength band classifications from 1 to 204. A larger weight indicates that the data in that wavelength band is important, while a smaller weight indicates that the data is unimportant. In the figure, the feature weight is almost zero in the band bands 30 ~ 50 (488 ~ 547 nm), 120 ~ 130 (752 ~ 782 nm) 180 ~ 190 (929 ~ 958 nm). In other bands, the feature weight is more than 1, but it is not concentrated in some wavelength bands, but widely distributed in important wavelength bands. Considering the large amount of information and redundancy of HS data, the feature weight is low. Avoiding the analysis of wavelength data can save computational resources. Therefore, in this study, we analyze the wavelength data set with high feature weight (MS data) selected by NCA to perform data processing faster than analyzing HS data.
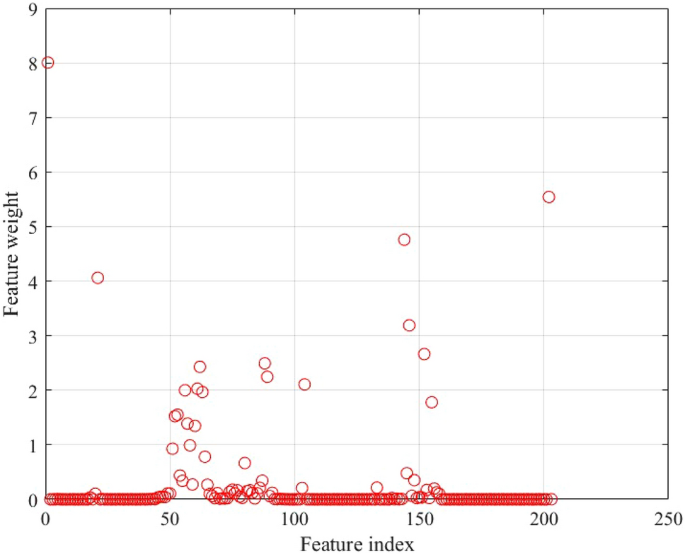
Shows each band weighted by NCA. The vertical axis shows feature weight and the horizontal axis shows the number of bands.
Multispectral (MS) data analysis
Using the selected intensity of wavelength data, mineral identification was performed with various machine learning algorithms as shown in Table 1 . Since different combinations of machine learning algorithms and data sets used have different compatibility, in this study multiple machine learning algorithms were trained on the same data set to find the best performing combination or machine learning algorithm. From the wavelength selection by NCA, we created datasets with the top 25, 20, 15, 10, 9, 8, 7, 6, 5, 4, 3, 2, and 1 band each with high feature weight values. Then, each dataset was trained once with 33 different machine learning algorithms using the machine learning application MATLAB.
In addition, RGB images (3 bands) and HS data (204 bands) were analyzed in the same manner and compared to the identification accuracy of the MS data. The result is shown in Figs. 8 and 9 . The plots include the change in the number of bands selected by the NCA and the corresponding change in the accuracy of mineral determination by machine learning. It is noteworthy that the machine learning models are sequentially selected and presented for the best-performing models as outlined in Table 1 . Figures 8 and 9 also compares the discrimination accuracy of the RGB images, HS data, and MS data.
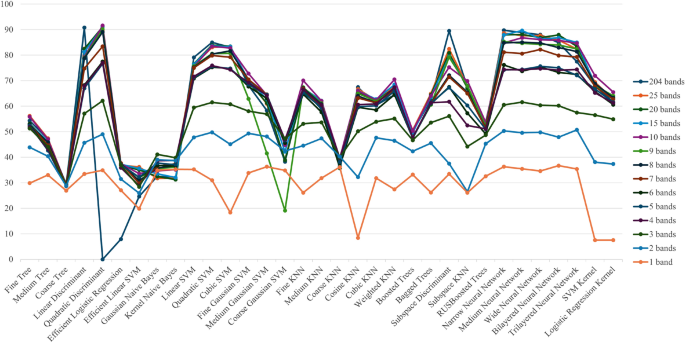
Machine learning results (Validation accuracy).
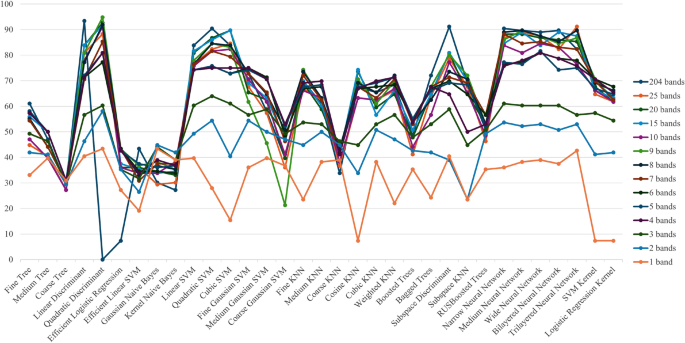
Machine learning results (Test accuracy).
Figure 9 shows that the machine learning identification results for the 15–25 bands of data selected by NCA are high, exceeding 90%. It also shows that the identification accuracy in those bands is almost the same as that of the HS data, indicating that mineral assemblages can be identified with high accuracy with approximately one-tenth or less of the data volume. This suggests the potential to reduce both computational and economic costs. The data analysis of 6 to 10 bands showed a high identification result of more than 80%, while the identification accuracy tended to decrease as the number of selected bands decreased. The analysis of 3-wavelength selection in particular shows lower identification accuracy than that for RGB images that also have 3 bands.
These results indicate that the arsenic minerals used in this study can be identified with more than 80% accuracy by selecting 6 or more bands in the NCA, and, by using 15 or more bands, the identification accuracy is almost equivalent to the analysis of HS data. On the other hand, when the number of bands selected by NCA is less than 5, the accuracy of arsenic concentration identification is significantly reduced, and perhaps a different algorithm (other than the machine learning algorithm employed in this study) may improve the accuracy of identification when using less than 5 bands.
While the identification of specific wavelengths for new ores necessitates the use of a hyperspectral camera, it is important to note that this camera is primarily employed for the training of AI models, rather than for ore sorting. This implies that with the use of a single hyperspectral camera, AI models can be trained for multiple ores, thereby offering a cost-effective solution in the long term.
Comparison of wavelength selection methods
In this study, NCA was employed as the wavelength selection method. However, as mentioned above, there are a wide variety of wavelength selection methods for HS data. Therefore, a performance comparison of representative wavelength selection methods is conducted here for simplicity. In this article, the following five-wavelength selection methods (MRMR(Minimum Redundancy Maximum Relevance) 59 , Chi-square distribution test 60 , ANOVA 61 , Kruskal–Wallis (KW) test 62 , and ReliefF 63 ) were used for comparison, and the accuracy was compared by using a machine learning to identify arsenic concentration only in the selected bands.
To give an overview of each wavelength selection method, MRMR minimizes redundancy by selecting data sets that are not similar for paired data. The Chi-square variance test compares the difference between the variance values of the data and those of the assumed data. ANOVA also analyzes variance but compares significant differences between groups; the KW test is a nonparametric method of ANOVA. RelieF then matches and ranks features between pairs of data.
Each is a different wavelength selection algorithm, and their effectiveness for the data set used in this study compared to NCA is compared. The following Table 3 shows the selected bands ranking by the aforementioned five methods and the NCA.
Table 3 shows that among the wavelength bands at 400 ~ 1000 nm, the bands at the ends near 400 nm and 1000 nm tend to be selected mainly by Chi2, ReliefF, ANOVA, and KW methods. While the wavelength bands at the edges of those bands are selected for NCA and MRMR, the bands selected are widely scattered, and this is especially true for NCA. While the Chi2, ReliefF, ANOVA, and KW methods are algorithms that mainly analyze the dispersion values of the data, NCA/MRMR is an algorithm that uses the nearest neighbor method and redundancy, which may be the result of the differences caused by these algorithms.
Since the bands selected by the band selectors were different, they performed machine learning classification using only the wavelength data selected by them and compared which band selector was the best. The number of bands to be trained varied from a ranking of 10 to 1. The learning results are shown in Fig. 10 below.
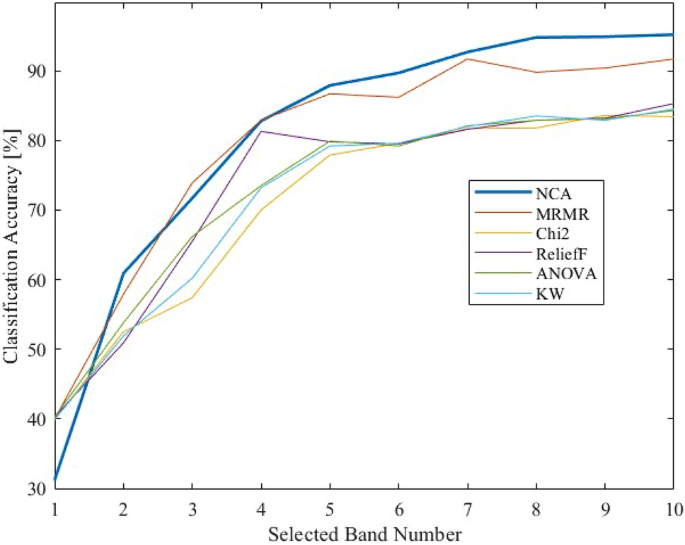
Wavelength selection method comparison of classification accuracy (NCA, MRMR, Chi2, ReliefF, ANOVA, and Kruskal–Wallis).
Figure 10 shows that the NCA method outperformed other wavelength selection methods for the present sample in the band number range of 4–10. Table 3 confirms that this is because other methods react strongly to components at the edge of the acquired wavelengths, such as 400 nm and 1000 nm, which are outside the 400 to 1000 nm region. It is important to note that hyperspectral cameras are less accurate for edge wavelength data due to their functioning. The accuracy of the selection method, other than NCA, may have been affected. This is due to the selection of wavelength bands with large errors and data variations, resulting in lower accuracy. In contrast, NCA selects bands in the wavelength range from visible light to near-infrared light, resulting in higher classification accuracy.
One possible reason is the influence of the absorption spectra in the chosen bands. The absorption spectrum of a mineral can be attributed to two processes, electronic and vibrational 64 , but especially in the short wavelength band, it is caused by the energy order of electron transitions. In the near-infrared wavelength band, absorption spectra are caused by molecular contraction and other processes. In the ore type used in this study, these absorption spectra are thought to be the cause of the characteristic spectral shape.
In this study, more than 30 machine learning models were created and tested 15 times each using these models. Despite the limitations of the paper preventing the presentation of the mixing matrices for all of them, Figures (Figs. 11 , 12 , 13 , 14 , 15 , 16 , 17 , 18 , 19 and 20 ) show the mixing matrices for the training models trained using only the data reduced in dimensionality using NCA. As illustrated in, the positive predictive values (PPV) values displayed in blue exhibit a concurrent decline with each reduction in the number of bands, whereas the false discovery rates (FDR) values displayed in red demonstrate an upward trend. Additionally, it can be observed that the false discovery rates within the same group tend to increase as the number of bands decreases, whereas the false discovery rates across groups exhibit a comparatively lower increase.
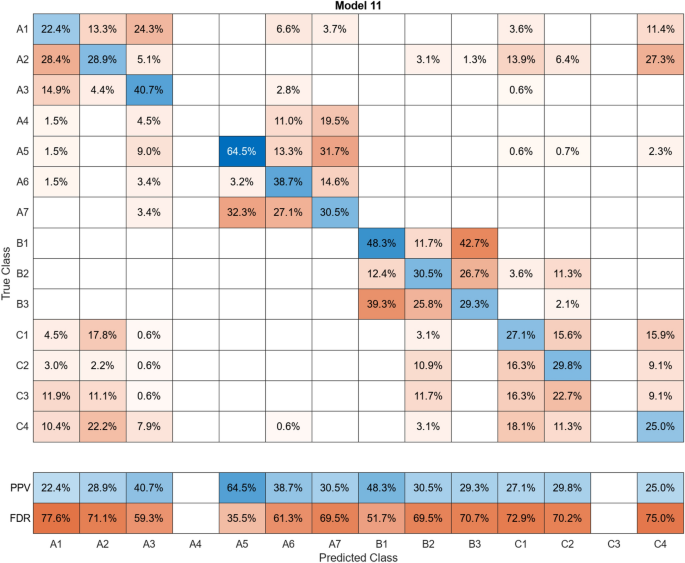
Confusion Matrix of NCA results using 1 band.
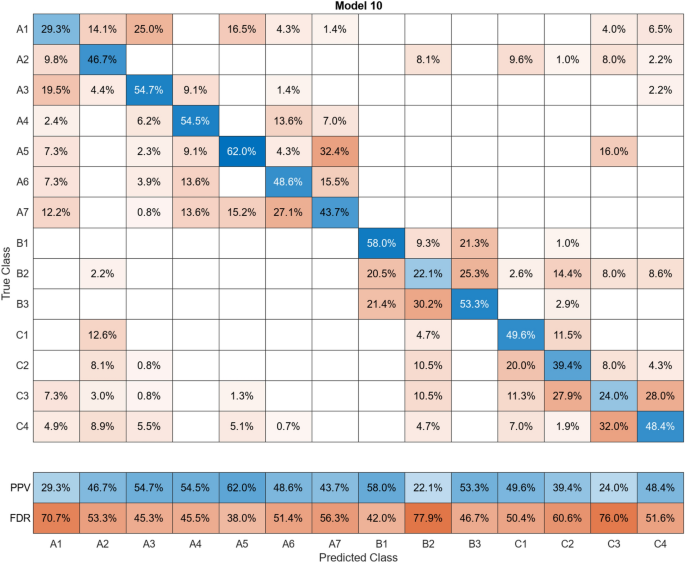
Confusion Matrix of NCA results using 2 bands.
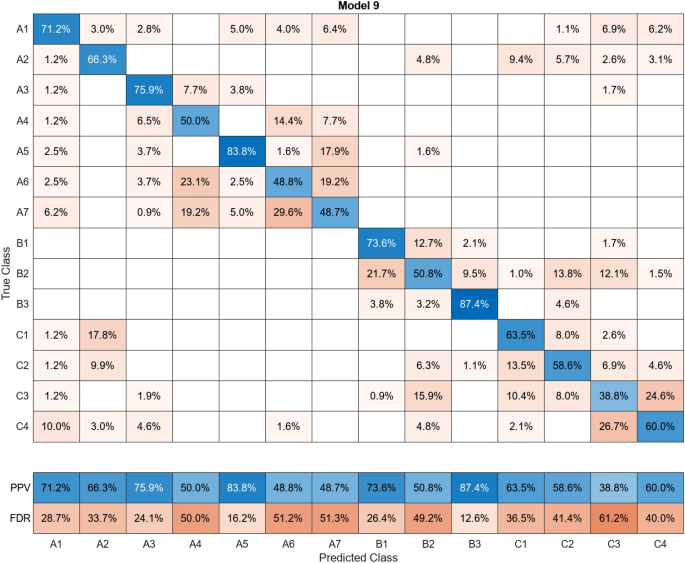
Confusion Matrix of NCA results using 3 bands.
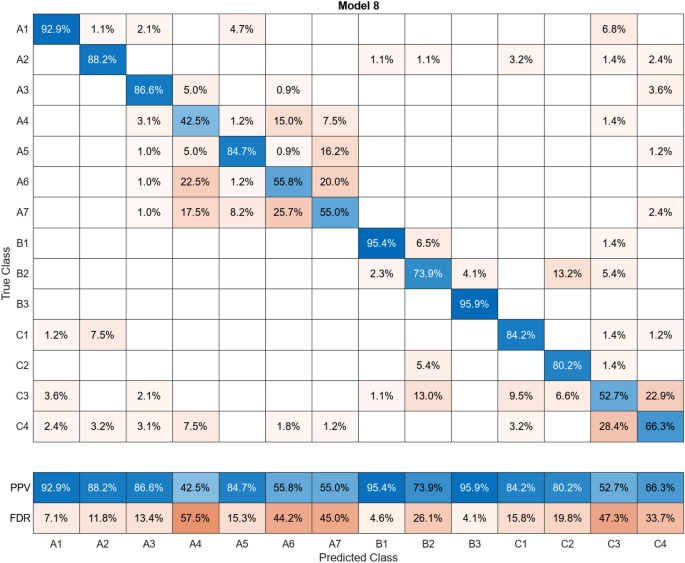
Confusion Matrix of NCA results using 4 bands.
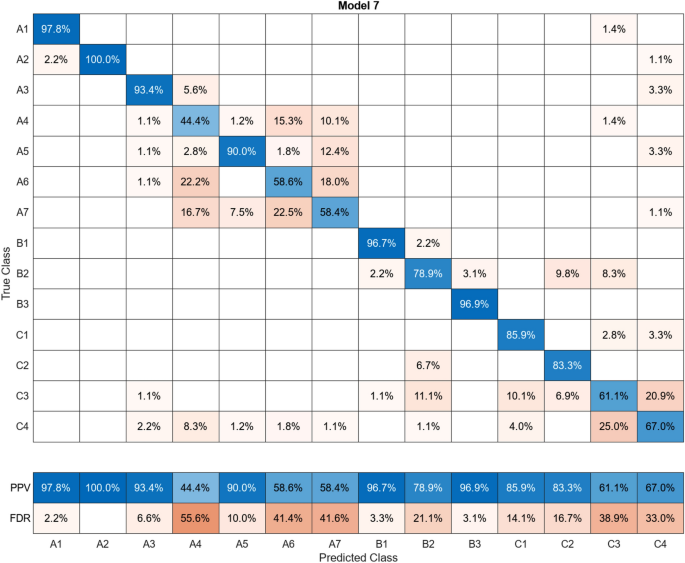
Confusion Matrix of NCA results using 5 bands.
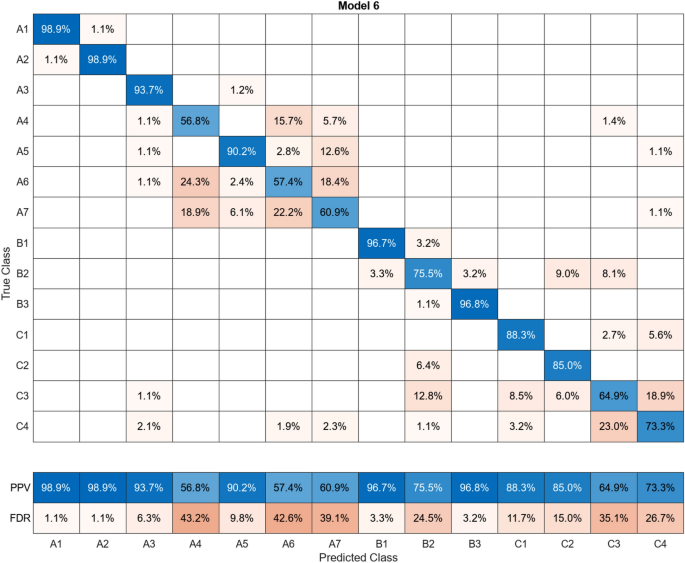
Confusion Matrix of NCA results using 6 bands.
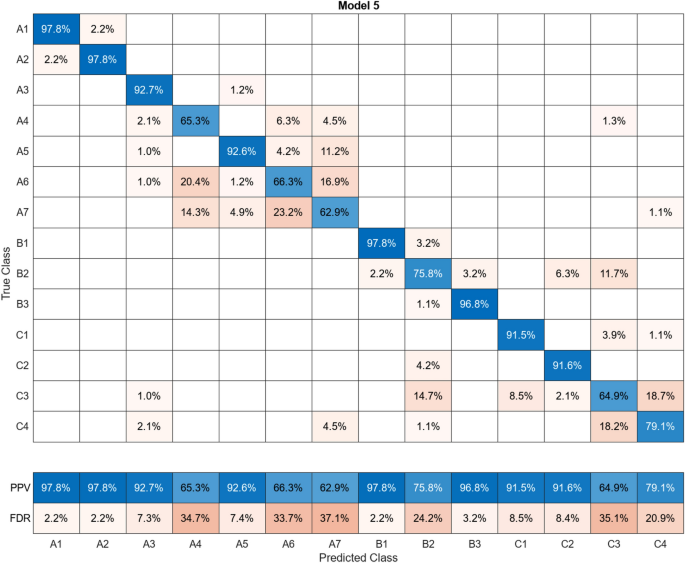
Confusion Matrix of NCA results using 7 bands.
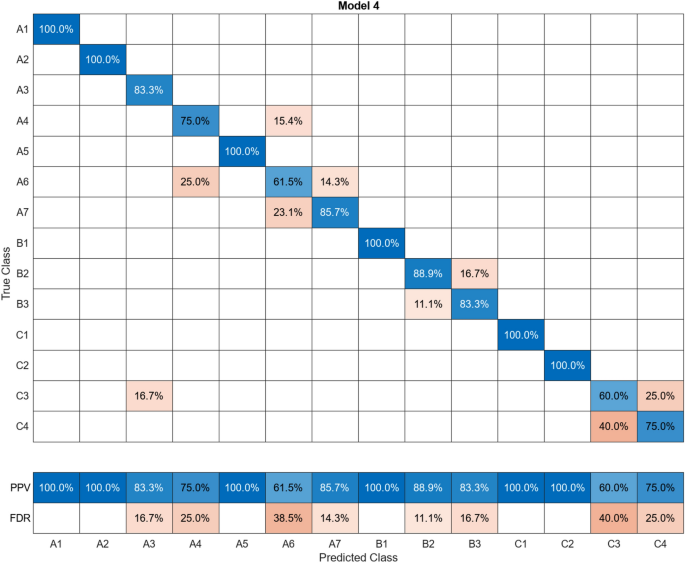
Confusion Matrix of NCA results using 8 bands.
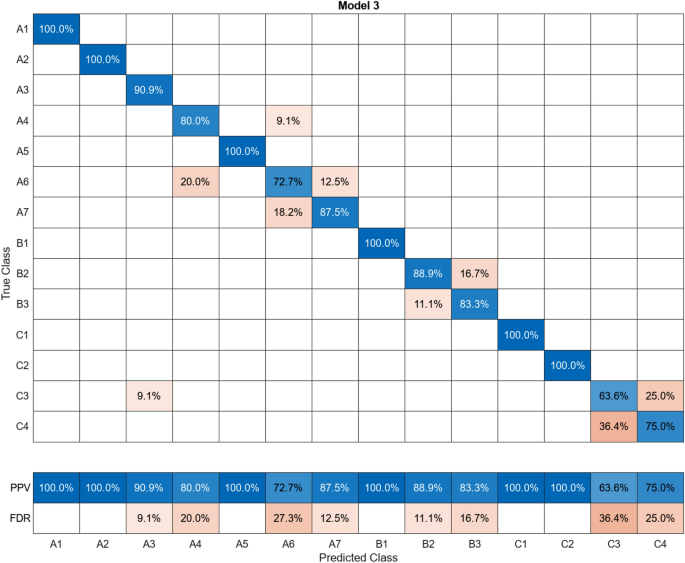
Confusion Matrix of NCA results using 9 bands.
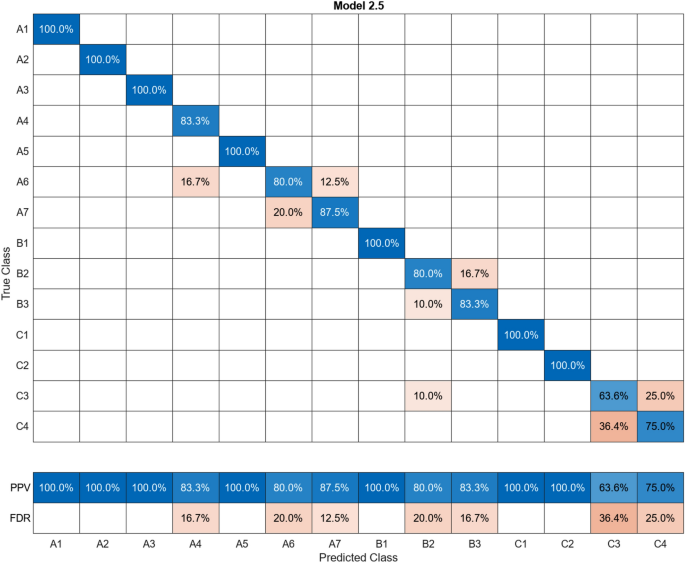
Confusion Matrix of NCA results using 10 bands.
However, the band selector that selected those edge bands cannot be regarded as an excellent band selector because the identification accuracy itself is low (Supplemental materials). Therefore, the wavelength selection method using NCA, which showed an identification accuracy of more than 90% with less than one-tenth of the HS data, was found to be superior by comparison. Furthermore, given that the bands selected by NCA were widely distributed, it was found that there existed not only absorption spectra resulting from vibrational processes of molecules in the far-infrared region in conventional mineral identification, but also characteristic optical property values of the minerals themselves in the visible and near-infrared regions.
Regarding the calculation and processing of HS data, a large amount of calculation resources was required because the data consisted of 204 dimensional wavelengths. In addition, the HS camera itself is expensive, which has been a barrier to its introduction into actual operation. This study shows that identification can be performed by using MS data, which greatly reduces the dimension of data. The Specim IQ HS camera used in this study acquires approximately 300 MB of data in a single shot, whereas MS data acquires only about 3 MB of data. Also, the practical scanning time for Specim IQ is approximately 60 s (depending on the exposure time), while MS cameras can capture images in a few seconds, depending on the type of equipment used. In terms of price, HS cameras are generally expensive, costing tens of thousands of dollars or more, whereas MS cameras are expected to significantly reduce costs. As described above, the dimensionality reduction of HS data using NCA is expected to reduce the amount of data (reduction of computation cost), scan time, and price.
Limitations and future works
This experiment was conducted in an idealized environment with homogenized and perfect lighting; to improve the classification accuracy by AI and use it in actual operations, the training data must be tuned to improve the robustness of the data. The research may encounter difficulties in extrapolating its findings due to its focus on arsenic-containing minerals found in samples of copper ore. To improve the usefulness of the methodology in various situations, it would be advantageous to include a wider variety of mineral compositions and types of ore in future research. The practicality of incorporating hyperspectral imaging and machine learning algorithms may also be constrained by issues such as equipment availability and cost. Taking steps to address these limitations will lead to a greater acceptance of the methodology within the mining industry. Moreover, discrepancies in the composition of the ore and the prevailing climatic circumstances may influence the efficacy of the suggested approach. Examining the resilience of the technique under various settings would yield vital insights into its practical usefulness.
In order to ascertain the efficacy and feasibility of the suggested approach in real-life scenarios, further research endeavors could encompass the implementation of field trials or pilot studies inside active mining settings. Additional refinement of machine learning algorithms and approaches has the potential to improve the precision and effectiveness of mineral identification, especially in situations that involve intricate ore compositions. Investigating the potential for combining hyperspectral imaging with new sensor technologies may result in more efficient and economical methods for mineral processing and sorting. The environmental implications of the proposed approach, including energy consumption and waste production, must also be evaluated in order to guarantee long-term viability. Creating intuitive interfaces and software tools for analyzing and visualizing data could help mining sector personnel embrace hyperspectral imaging and machine learning approaches.
The combination of hyperspectral imaging (HS) and sensor-based ore sorting (SBOS) systems, particularly in addressing challenges within the copper extraction industry, presents a promising solution amidst increasing demand for minerals in renewable energy and electric vehicles. The depletion of high-grade ore and the emergence of high-arsenic copper resources pose significant obstacles to sustainable mining practices. Arsenic contamination complicates mineral processing and raises environmental and health concerns. Nevertheless, by incorporating contemporary technologies like hyperspectral imaging into SBOS systems, it becomes feasible to address these difficulties with efficiency. Sensor-based ore sorting systems offer advantages in selectively extracting valuable minerals based on their unique properties, optimizing resource utilization, and reducing processing costs. Hyperspectral imaging enhances these systems by providing detailed mineral characterization, enabling precise mineral identification and classification based on spectral fingerprints. By leveraging techniques like Neighborhood Component Analysis (NCA) for wavelength selection, ore sorting systems can focus on specific spectral bands that are most effective in distinguishing desired minerals, thereby improving efficiency and selectivity.
Machine learning was performed on each of the top 25, 20, 15, and 10 ~ 1 band data sets selected as a result of the NCA analysis to show the change in identification accuracy with the number of bands. By selecting the most accurate data from the multiple machine learning results, statistical errors caused by differences in compatibility between the data and machine learning were eliminated. As a result, the machine learning results for the 6–25 bands selected by NCA were almost the same as the identification accuracy of the HS data with 204 bands. On the other hand, the identification accuracy decreased significantly for bands 1–5. This indicates that arsenic-containing minerals can be identified with a high accuracy of more than 90% by selecting 6 or more bands in the NCA and performing machine learning classification. At the same time, similar tests were conducted using major band selectors other than NCA (MRMR, Chi2, ANOVA, KW, and ReliefF) as verification experiments, and NCA showed the highest performance.
This research has shown that with the integration of the NCA, identification accuracy of arsenic-containing minerals can exceed 90% with just a fraction of the original HS data volume. This approach not only rivals the performance of full HS data but also offers greater efficiency and cost-effectiveness. Comparison experiments with other wavelength selection methods reaffirm the superiority of NCA in terms of classification accuracy and efficiency. Moreover, the ability to customize wavelength selection according to ore characteristics further enhances the adaptability and effectiveness of ore sorting systems. This research underscores the transformative potential of hyperspectral imaging and machine learning in revolutionizing mineral processing and sorting technologies. This strategy addresses the difficulties related to high-dimensional data and enhances the accuracy of categorization. Further research endeavors could explore field trials, refine machine learning algorithms, and investigate new sensor technologies to advance the efficacy and applicability of these methodologies in real-world mining operations.
Data availability
The data used in the study are available from the corresponding author upon reasonable request.
Abbreviations
Hyperspectral
Inductively coupled plasma
Leave-one-out
Multispectral
- Neighborhood component analysis
Number of bands
- Sensor-based ore sorting
Elshkaki, A., Graedel, T., Ciacci, L. & Change, B. R. Copper demand, supply, and associated energy use to 2050. Glob. Environ. Chang. 39 , 305–315 (2016).
Article Google Scholar
Pell, R. et al. Towards sustainable extraction of technology materials through integrated approaches. Nat. Rev. Earth Environ. 2 (10), 665–679. https://doi.org/10.1038/s43017-021-00211-6 (2021).
Article ADS Google Scholar
Ferreccio, C. & Sancha, A. M. Arsenic exposure and its impact on health in Chile. J. Health Popul. Nutr. 24 , 164–175 (2006).
PubMed Google Scholar
Mohammed Abdul, K. S., Jayasinghe, S. S., Chandana, E. P. S., Jayasumana, C. & De Silva, P. M. C. S. Arsenic and human health effects: A review. Environ. Toxicol. Pharmacol. 40 (3), 828–846. https://doi.org/10.1016/J.ETAP.2015.09.016 (2015).
Article CAS Google Scholar
Okada, N. et al. Automated identification of mineral types and grain size using hyperspectral imaging and deep learning for mineral processing. Minerals https://doi.org/10.3390/min10090809 (2020).
Peukert, D., Xu, C. & Dowd, P. A review of sensor-based sorting in mineral processing: The potential benefits of sensor fusion. Minerals 12 (11), 1364–1364. https://doi.org/10.3390/MIN12111364 (2022).
Article ADS CAS Google Scholar
Zhang, Z., Liu, Y., Hu, Q., Zhang, Z., & Liu, Y. Competitive voting-based multi-class prediction for ore selection. In IEEE International Conference on Automation Science and Engineering , vol. 2020-Augus, pp. 514–519. doi: https://doi.org/10.1109/CASE48305.2020.9217017 (2020)
Mahlangu, T., Moemise, N., Ramakokovhu, M. M., Olubambi, P. A. & Shongwe, M. B. Separation of kimberlite from waste rocks using sensor-based sorting at Cullinan diamond mine. J. S. Afr. Inst. Min. Metall. 116 (4), 343–347. https://doi.org/10.17159/2411-9717/2016/V116N4A7 (2016).
Normanyo, E., Ofosu, R. A. & Acquah, D. S. On sensor-based ore sorting. Ghana Min. J. 22 (1), 56–61. https://doi.org/10.4314/GM.V22I1.7 (2022).
Duan, B., Bobicki, E. R. & Hum, S. V. Identification of valuable minerals or metals in ores using microwave imaging. IEEE Trans. Antennas Propag. 70 (12), 12189–12198. https://doi.org/10.1109/TAP.2022.3209711 (2022).
Rashedul Islam, M. D., & Ahmed, B. Spectral–spatial dimensionality reduction for hyperspectral image classification. in 2022 25th International Conference on Computer and Information Technology (ICCIT) , pp. 282–287. doi: https://doi.org/10.1109/ICCIT57492.2022.10054891 (2022)
Jang, H., Shin, S., Jeong, S., & Hwang, E. Likelihood based wavelength selection for sorting metals by laser-induced breakdown spectroscopy, in Optics InfoBase Conference Papers . doi: https://doi.org/10.1364/EE.2016.ETU3A.2 (2016).
Hariharan, J., Ampatzidis, Y., Abdulridha, J. & Batuman, O. Useful feature extraction and machine learning techniques for identifying unique pattern signatures present in hyperspectral image data. Hyperspectr. Imaging Perspect. Recent Adv. Appl. https://doi.org/10.5772/INTECHOPEN.107436 (2023).
Paikaray, B. K., Pramanik, J., & Samal, A. K. An introductory approach to spectral image analysis using machine learning classifiers, in Proceedings of 2022 1st IEEE International Conference on Industrial Electronics: Developments and Applications, ICIDeA 2022 , pp. 198–201. doi: https://doi.org/10.1109/ICIDEA53933.2022.9970023 (2022)
Yousuff, M. & Babu, R. Enhancing the classification metrics of spectroscopy spectrums using neural network based low dimensional space. Earth Sci. Inf. 16 (1), 825–844. https://doi.org/10.1007/S12145-022-00917-1 (2022).
Sinaice, B. B., Owada, N., Utsuki, S., Bagai, Z. B., Shemang, E., & Kawamura, Y. Employing NCA as a band reduction tool in rock identification from hyperspectral processing, in Rock mechanics and engineering geology in volcanic fields , pp. 427–434. doi: https://doi.org/10.1201/9781003293590-53 (2022)
Hiremath, S. K. Advances in principal component analysis in multispectral satellite imagery. Pune Institute of Computer Technology. [Online]. Available: https://typeset.io/papers/advances-in-principal-component-analysis-in-multispectral-1ethjso5i1
Fujisawa, T. & Unno, M. Advanced spectral analysis of complex molecular system. Mol. Laser Spectrosc. Adv. Appl. 3 , 25–54. https://doi.org/10.1016/B978-0-323-91249-5.00002-8 (2022).
Miller, C. I., Thomas, J. J., Kim, A. M., Metcalf, J. P. & Olsen, R. C. Application of image classification techniques to multispectral lidar point cloud data. Proc. SPIE 9832 , 286–297. https://doi.org/10.1117/12.2223257 (2016).
Ghamisi, P. Spectral and spatial classification of hyperspectral data. [Online]. Available: https://typeset.io/papers/spectral-and-spatial-classification-of-hyperspectral-data-3h0c216vh7
Buxton, M., & Benndorf, J. The use of sensor derived data in optimization along the mine-value-chain an overview and assessment of techno-economic significance. in Proceedings of the 15th international ISM congress, Aachen, Germany , pp. 324–336 (2013).
Young, A., Veras, M., Neto, D., Otávio, P., & Sampaio, C. Applications of automatic sensor based sorting to mining ores (2017).
Wotruba, H. (2016) Sensor sorting technology-is the minerals industry missing a chance?.
Okada, N. et al. Automated identification of mineral types and grain size using hyperspectral imaging and deep learning for mineral processing. Minerals 10 (9), 809 (2020).
Robben, C. & Wotruba, H. Sensor-based ore sorting technology in mining—past, present and future. Minerals 9 (9), 523 (2019).
Ware, N. G. Analytical methods: Mineral analysis, in Encyclopedia of geology , pp. 107–118 (2004). doi: https://doi.org/10.1016/B0-12-369396-9/00098-8 .
Pirrie, D. & Rollinson, G. K. Unlocking the applications of automated mineral analysis. Geol. Today 27 (6), 226–235. https://doi.org/10.1111/J.1365-2451.2011.00818.X (2011).
Modise, E. G., Zungeru, A. M., Mtengi, B. & Ude, A. U. Sensor-based ore sorting—A review of current use of electromagnetic spectrum in sorting. IEEE Access 10 , 112307–112326. https://doi.org/10.1109/ACCESS.2022.3216296 (2022).
Maier, G. et al. Simulation study and experimental validation of a neural network-based predictive tracking system for sensor-based sorting. Technisches Messen 90 (7–8), 489–499. https://doi.org/10.1515/TEME-2023-0033/PDF (2023).
Sinaice, B. B., Kawamura, Y., Kim, J., Okada, N., Kitahara, I., & Jang, H. Application of deep learning approaches in igneous rock hyperspectral imaging . 2020. doi: https://doi.org/10.1007/978-3-030-33954-8_29 (2019)
Sinaice, B. B. et al. Spectral angle mapping and AI methods applied in automatic identification of placer deposit magnetite using multispectral camera mounted on UAV. Minerals 12 (2), 268. https://doi.org/10.3390/min12020268 (2022).
Tuşa, L. et al. Evaluating the performance of hyperspectral short-wave infrared sensors for the pre-sorting of complex ores using machine learning methods. Miner. Eng. 146 , 106150. https://doi.org/10.1016/J.MINENG.2019.106150 (2020).
Robben, C. & Wotruba, H. Sensor-based ore sorting technology in mining—Past, present and future. Minerals 9 (9), 523. https://doi.org/10.3390/MIN9090523 (2019).
Sabins, F. F. Remote sensing for mineral exploration. Ore Geol. Rev. 14 (3–4), 157–183. https://doi.org/10.1016/S0169-1368(99)00007-4 (1999).
van der Meer, F. D. et al. Multi- and hyperspectral geologic remote sensing: A review. Int. J. Appl. Earth Obs. Geoinf. 14 (1), 112–128. https://doi.org/10.1016/J.JAG.2011.08.002 (2012).
Okada, N. et al. Classification of arsenic bearing minerals using hyperspectral imaging and deep learning for mineral processing. J. MMIJ https://doi.org/10.2473/journalofmmij.137.1 (2021).
Li, S., Wu, H., Wan, D. & Zhu, J. An effective feature selection method for hyperspectral image classification based on genetic algorithm and support vector machine. Knowl. Based Syst. https://doi.org/10.1016/j.knosys.2010.07.003 (2011).
Sun, W. & Du, Q. Hyperspectral band selection: a review. IEEE Geosci. Remote Sens. Mag. 7 (2), 118–139 (2019).
Ghosh, A., Nayak, B., Das, T. K. & Palit Sagar, S. A non-invasive technique for sorting of alumina-rich iron ores. Miner. Eng. 45 , 55–58. https://doi.org/10.1016/J.MINENG.2012.12.004 (2013).
Kern, M., Tusa, L., Leißner, T., van den Boogaart, K. G. & Gutzmer, J. Optimal sensor selection for sensor-based sorting based on automated mineralogy data. J. Clean. Prod. 234 , 1144–1152. https://doi.org/10.1016/J.JCLEPRO.2019.06.259 (2019).
Phiri, T., Glass, H. J. & Mwamba, P. Development of a strategy and interpretation of the NIR spectra for application in automated sorting. Miner. Eng. 127 , 224–231. https://doi.org/10.1016/J.MINENG.2018.08.011 (2018).
Passos, D. & Mishra, P. An automated deep learning pipeline based on advanced optimisations for leveraging spectral classification modelling. Chemom. Intell. Lab. Syst. https://doi.org/10.1016/J.CHEMOLAB.2021.104354 (2021).
Duan, F. Q., Liu, R., Guo, P., Zhou, M. Q. & Wu, F. C. Automated spectral classification using template matching. Res. Astron. Astrophys. 9 (3), 341–348. https://doi.org/10.1088/1674-4527/9/3/009 (2009).
Wang, D. et al. Machine learning-based multifunctional optical spectrum analysis technique. IEEE Access 7 , 19726–19737. https://doi.org/10.1109/ACCESS.2019.2895409 (2019).
Baskir, I. M. & Drozd, A. V. New Matlab software for wavelength selection. Chemom. Intell. Lab. Syst. 66 (1), 89–91. https://doi.org/10.1016/S0169-7439(03)00002-9 (2003).
Nalepa, J. Recent advances in multi-and hyperspectral image analysis. Sensors https://doi.org/10.3390/S21186002 (2021).
Article PubMed PubMed Central Google Scholar
Ghamisi, P., Yokoya, N., Li, J., Liao, W., Liu, S., Plaza, J., Rasti, B. and Plaza, A. Advances in hyperspectral image and signal processing: A comprehensive overview of the state of the art. ieeexplore.ieee.org IEEE Geoscience and Remote Sensing Magazine, 2017•ieeexplore.ieee.org . (2017)
Schultz, R. A., Nielsen, T., Zavaleta, J. R., Ruch, R., Wyatt, R., Garner, H. R. Hyperspectral imaging: A novel approach for microscopic analysis. Wiley Online Library Cytometry, 2001•Wiley Online Library , doi: https://doi.org/10.1002/1097-0320(20010401)43:4<239::AID-CYTO1056>3.0.CO;2-Z (2001)
Jahoda, P. et al. Machine learning for recognizing minerals from multispectral data. Analyst 146 (1), 184–195. https://doi.org/10.1039/D0AN01483D (2021).
Article ADS CAS PubMed Google Scholar
Sinaice, B. B. et al. Coupling nca dimensionality reduction with machine learning in multispectral rock classification problems. Minerals https://doi.org/10.3390/MIN11080846 (2021).
Carey, C., Boucher, T., Mahadevan, S., Bartholomew, P. & Dyar, M. D. Machine learning tools formineral recognition and classification from Raman spectroscopy. J. Raman Spectrosc. 46 (10), 894–903. https://doi.org/10.1002/JRS.4757 (2015).
Yokoya, N. & Iwasaki, A. Hyperspectral image processing for advanced earth observation. J. Jpn. Soc. Artif. Intell. 29 (4), 357–365 (2014).
Google Scholar
Robben, M., Wotruba, H., Balthasar, D., & Rehrmann, V. NIR spectral imaging in the minerals industry. Universitätsbibliothek der RWTH Aachen (2009).
Specim IQ - Specim. Accessed 17 February 2024. [Online]. Available: https://www.specim.com/iq/
Yang, W., Wang, K. & Zuo, W. Neighborhood component feature selection for high-dimensional data. J. Comput. 7 (1), 161–168. https://doi.org/10.4304/jcp.7.1.161-168 (2012).
Neighborhood Component Analysis (NCA) Feature Selection - MATLAB & Simulink. Accessed 17 March 2024. [Online]. Available: https://www.mathworks.com/help/stats/neighborhood-component-analysis.html
Edgar, T., & Manz, D. Research methods for cyber security (2017)
Ossandón, G., Fréraut, R., Gustafson, L. B., Lindsay, D. D., & Zentilli, M. Geology of the Chuquicamata mine: A progress report. pubs.geoscienceworld.org Economic Geology, 2001•pubs.geoscienceworld.org , vol. 96, pp. 249–270 (2001).
Che, J. et al. Maximum Relevance Minimum Common Redundancy Feature Selection for Nonlinear Data (Elsevier, 2017).
Book Google Scholar
Lancaster, H. O. & Seneta, E. Chi-square distribution. Encycl. Biostat. https://doi.org/10.1002/0470011815.B2A15018 (2005).
Ding, H., Feng, P. M., Chen, W. & Lin, H. Identification of bacteriophage virion proteins by the ANOVA feature selection and analysis. Mol. BioSyst. https://doi.org/10.1039/C4MB00316K (2014).
Article PubMed Google Scholar
Vargha, A. & Delaney, H. D. The Kruskal-Wallis test and stochastic homogeneity. J. Educ. Behav. Stat. 23 (2), 170–192. https://doi.org/10.3102/10769986023002170 (1998).
Robnik-Šikonja, M. & Kononenko, I. Theoretical and empirical analysis of ReliefF and RReliefF. Mach. Learn. 53 (1–2), 23–69. https://doi.org/10.1023/A:1025667309714 (2003).
Clark, R. N. Spectroscopy of rocks and minerals, and principles of spectroscopy (2020).
Download references
Acknowledgements
The researchers would like to acknowledge the JSPS KAKENHI for funding this work.
This work was supported by JSPS KAKENHI Grant Number JP23KJ0029.
Author information
Authors and affiliations.
Division of Sustainable Resources Engineering, Graduate School of Engineering, Hokkaido University, Kita-13, Nishi-8, Sapporo, 060-8628, Japan
Natsuo Okada, Hiromasa Nozaki, Shinichiro Nakamura & Angesom Gebretsadik
Division of Engineering, Instituto Superior Politécnico de Tete, Tete, Mozambique
Elsa Pansilvania Andre Manjate
Division of Sustainable Resources Engineering, Faculty of Engineering, Hokkaido University, Kita-13, Nishi-8, Sapporo, 060-8628, Japan
Yoko Ohtomo, Takahiko Arima & Youhei Kawamura
Department of Mining Engineering, Aksum University, 7080, Aksum, Tigray, Ethiopia
Angesom Gebretsadik
You can also search for this author in PubMed Google Scholar
Contributions
Conceptualization, N.O., Y.O., T.A., and Y.K.; Methodology, N.O.; Software, N.O., H.N., and S.N.; Validation, N.O.; Formal analysis, N.O. and A.G.; Investigation, N.O.,T.A., and E.P.A.M; Resources, Y.K. and Y.O.; Data curation, N.O., H.N., S.N.; Writing—original draft, N.O.; Writing—review & editing, E.P.A.M., A.G., Y.O., and Y.K.; Visualization, N.O., S.N., and H.N.; Supervision, Y.O.,T.A., and Y.K.; Project administration, Y.K.; Funding acquisition, Y.K. All authors have read and agreed to the published version of the manuscript.
Corresponding author
Correspondence to Natsuo Okada .
Ethics declarations
Competing interests.
The authors declare no competing interests.
Additional information
Publisher's note.
Springer Nature remains neutral with regard to jurisdictional claims in published maps and institutional affiliations.
Supplementary Information
Supplementary information., rights and permissions.
Open Access This article is licensed under a Creative Commons Attribution 4.0 International License, which permits use, sharing, adaptation, distribution and reproduction in any medium or format, as long as you give appropriate credit to the original author(s) and the source, provide a link to the Creative Commons licence, and indicate if changes were made. The images or other third party material in this article are included in the article's Creative Commons licence, unless indicated otherwise in a credit line to the material. If material is not included in the article's Creative Commons licence and your intended use is not permitted by statutory regulation or exceeds the permitted use, you will need to obtain permission directly from the copyright holder. To view a copy of this licence, visit http://creativecommons.org/licenses/by/4.0/ .
Reprints and permissions
About this article
Cite this article.
Okada, N., Nozaki, H., Nakamura, S. et al. Optimizing multi-spectral ore sorting incorporating wavelength selection utilizing neighborhood component analysis for effective arsenic mineral detection. Sci Rep 14 , 11544 (2024). https://doi.org/10.1038/s41598-024-62166-0
Download citation
Received : 26 March 2024
Accepted : 14 May 2024
Published : 21 May 2024
DOI : https://doi.org/10.1038/s41598-024-62166-0
Share this article
Anyone you share the following link with will be able to read this content:
Sorry, a shareable link is not currently available for this article.
Provided by the Springer Nature SharedIt content-sharing initiative
- Mineral processing
- Wavelength selection
By submitting a comment you agree to abide by our Terms and Community Guidelines . If you find something abusive or that does not comply with our terms or guidelines please flag it as inappropriate.
Quick links
- Explore articles by subject
- Guide to authors
- Editorial policies
Sign up for the Nature Briefing: AI and Robotics newsletter — what matters in AI and robotics research, free to your inbox weekly.

- ISU Navigate
- Faculty & Staff
- Virtual Tour
Common Searches
- Academic Calendar
- Transcripts
- Scholarships
- Event Tickets
- Health Center
- APA Style Guide
- Financial Aid
New technique for case study development published
May 9, 2024
Kevin Parker, ISU professor emeritus, recently published two papers in Communications of the Association for Information Systems (CAIS). Each paper was published by CAIS in their IS Education section, which has a 7% acceptance rate.
Modular Design of Teaching Cases: Reducing Workload While Maximizing Reusability presents a modular case study development concept for better managing the development of case studies. The approach achieves project extensibility through reusable case study modules, while at the same time helping to reduce instructor workload and solution reuse by students. The approach is based on the concept of creating different variations of a case study each semester by adding or replacing existing descriptive modules with new modules.
Wind Riders of the Lost River Range: A Modular Project-Based Case for Software Development focuses on the information technology needs of a simulated specialty sports shop in central Idaho that concentrates on wind sports equipment, like hang gliders, paragliders, and snowkites. The case study consists of a core case that describes both the IT system currently in use and the new system that provides updated business support. Students are tasked with analyzing the system and designing a new system that delivers enhanced functionality. This evolutionary case study is based on the Modular Design of Teaching Cases and consists of the core case and 17 modules that can be swapped in or out of both the current or future system to produce a wide variety of combinations and variations of the case study.
Categories:
Research University News
case selection and the comparative method: introducing the case selector
- Published: 14 August 2017
- Volume 17 , pages 422–436, ( 2018 )
Cite this article
- timothy prescott 1 &
- brian r. urlacher 1
670 Accesses
2 Citations
5 Altmetric
Explore all metrics
We introduce a web application, the Case Selector ( http://und.edu/faculty/brian.urlacher ), that facilitates comparative case study research designs by creating an exhaustive comparison of cases from a dataset on the dependent, independent, and control variables specified by the user. This application was created to aid in systematic and transparent case selection so that researchers can better address the charge that cases are ‘cherry picked.’ An examination of case selection in a prominent study of rebel behaviour in civil war is then used to illustrate different applications of the Case Selector.
This is a preview of subscription content, log in via an institution to check access.
Access this article
Price includes VAT (Russian Federation)
Instant access to the full article PDF.
Rent this article via DeepDyve
Institutional subscriptions
Similar content being viewed by others
Case Study Research
Qualitative Comparative Analysis
Ahmed, A. and Sil, R. (2012) ‘When Multi-method Research Subverts Methodological Pluralism—or, Why We Still Need Single-Method Research’, Perspectives on Politics 10(4): 935–953.
Article Google Scholar
Asher, H. (2016) Polling and the Public: What every citizen should know , Washington, DC: CQ Press.
Google Scholar
Balcells, L. (2010) ‘Rivalry and Revenge: Violence Against Civilians in Conventional Civil Wars’, International Studies Quarterly 54(2): 291–313.
Brady, H. E. and Collier, D. (eds) (2004) Rethinking Social Inquiry: Diverse Tools, Shared Standards , Lanhan, MD: Rowman and Littlefield.
Dafoe, A. and Kelsey, N. (2014) ‘Observing the capitalist peace: Examining market-mediated signaling and other mechanisms’, Journal of Peace Research 51(5): 619–633.
DeRouen Jr, K., Ferguson, M. J., Norton, S., Park, Y. H., Lea, J., and Streat-Bartlett, A. (2010) ‘Civil War Peace Agreement Implementation and State Capacity’, Journal of Peace Research 47(3): 333–346.
Dogan, M. (2009) ‘Strategies in Comparative Sociology’, New Frontiers in Comparative Sociology , Leiden, Netherlands: Brill, pp. 13–44.
Durkheim, E. (1982) [1895] The Rules of Sociological Method , New York, NY: Free Press.
Book Google Scholar
Eck, K and Hultman, L. (2007) ‘One-Sided Violence Against Civilians in War: Insights from New Fatality Data’, Journal of Peace Research 44(2): 233–246.
Fearon, J. D. and Laitin, D. D. (2008) ‘Integrating Qualitative and Quantitative Methods’, The Oxford Handbook of Political Methodology , New York, NY: Oxford University Press. pp. 756–776.
Freedman, D. A. (2008) ‘Do the Ns Justify the Means?’, Qualitative & Multi - Method Research 6(2): 4–6.
George, A. L. and Bennett, A. (2004) Case Studies and Theory Development in the Social Sciences , Cambridge, MA: The MIT Press.
Gerring, J. and Cojocaru, L. (2016) ‘Selecting Cases for Intensive Analysis: A Diversity of Goals and Methods’, Sociological Methods & Research 45(3): 392–423.
Gerring, J. (2001) Social Science Methodology: A Criterial Framework, Cambridge, UK: University of Cambridge Press.
Gerring, J. (2004) ‘What Is a Case Study and What Is It Good for?’, American Political Science Review 98(2): 341–354.
Gerring, J. (2007) Case Study Research: Principles and Practices , Cambridge, UK: Cambridge University Press.
Glynn, A. N., and Ichino, N. (2016) ‘Increasing Inferential Leverage in the Comparative Method Placebo Tests in Small-n Research’, Sociological Methods & Research 45(3): 598–629.
Iacus, S. M., King, G., Porro, G., and Katz, J. N. (2012) ‘Causal Inference Without Balance Checking: Coarsened Exact Matching’, Political Analysis 20(1): 1–24.
Kohli, A., Evans, P., Katzenstein, P. J., Przeworski, A., Rudolph, S. H., Scott, J. C., and Skocpol, T. (1995) ‘The Role of Theory in Comparative Politics: A Symposium’, World Politics 48(1), 1–49.
Kalyvas, S. N. 2006. The Logic of Violence in Civil War , Cambridge, UK: Cambridge University Press.
King, G., Keohane, R. O., and Verba, S. (1994) Designing Social Inquiry: Scientific Inference in Qualitative Research , Princeton, NJ: Princeton University Press.
Kuperman, A. J. (2004) ‘Is Partition Really the Only Hope? Reconciling Contradictory Findings About Ethnic Civil Wars’, Security Studies 13(4): 314–349.
Lijphart, A. (1971) ‘Comparative Politics and Comparative Method, American Political Science Review 65(3): 682–698.
Maoz, Z. (2002) ‘Case Study Methodology in International Studies: From Storytelling to Hypothesis Testing’, Evaluating Methodology in International Studies , Ann Arbor, MI: University of Michigan Press, pp. 161–186.
Mahoney, J. (2010) ‘After KKV: The New Methodology of Qualitative Research’, World Politics , 62(1): 120–147.
Mill, J. S. (1872) A System of Logic . London, England: Longmans, Green, Reader, and Dyer.
Nielsen, Richard A. 2016. Case Selection via Matching. Sociological Methods & Research 45(3): 569 - 597.
Peters, B. G. (1998) Comparative politics: Theory and methods , Washington Square, NY: New York University Press.
Przeworski, A. and Teune, H. (1970) The Logic of Comparative Social Inquiry , New York, NY: Wiley-Interscience.
Ragin, C. C. (2014) The Comparative Method: Moving Beyond Qualitative and Quantitative Strategies . Berkley, CA: University of California Press.
Ragin, C. C., Berg-Schlosser, D., and de Meur, G. (1996) ‘Political Methodology: Qualitative Methods’, A New Handbook of Political Science , New York, NY: Oxford University Press, pp. 749–769.
Sambanis, N. (2004a) ‘What is Civil War? Conceptual and Empirical Complexities of an Operational Definition’, Journal of Conflict Resolution 48(6): 814–858.
Sambanis, N. (2004b) ‘Using Case Studies to Expand Economic Models of Civil War’, Perspectives on Politics 2(2): 259–279.
Seawright, J. and Gerring, J. (2008) ‘Case Selection Techniques in Case Study Research: A Menu of Qualitative and Quantitative Options’, Political Research Quarterly 61(2): 294–308.
Schrodt, P. A. (2014) ‘Seven Deadly Sins of Contemporary Quantitative Political Analysis’, Journal of Peace Research 51(2): 287–300.
Slater, D. and Ziblatt, D. (2013) ‘The Enduring Indispensability of the Controlled Comparison’, Comparative Political Studies 46(10): 1301–1327.
Snyder, R. (2001) ‘Scaling Down: The Subnational Comparative Method’, Studies in Comparative International Development 36(1): 93–110.
Tarrow, S. (2010) ‘The Strategy of Paired Comparison: Toward a Theory of Practice’, Comparative Political Studies 43(2): 230–259.
Weinstein, J. M. (2007) Inside Rebellion: The Politics of Insurgent Violence , Cambridge, UK: Cambridge University Press.
Wood, R. M. (2010) ‘Rebel Capability and Strategic Violence Against Civilians’, Journal of Peace Research 47(5): 601–614.
Yang, Z., Matsumura, Y. Kuwata, S., Kusuoka, H., and Takeda, H. (2003) ‘Similar Case Retrieval from the Database of Laboratory Test Results’, Journal of Medical Systems 27(3): 271–281.
Yin, R. K. (2003) Case Study Research: Design and Method , Thousand Oaks, CA: Sage Publications, Inc.
Download references
Acknowledgements
The authors would like to thank the anonymous reviewers for their insightful comments and feedback over the course of the review processes. This project has been significantly improved by their suggestions. The authors have also agreed to provide access to the Case Selector through their faculty webpages at their affiliated institutions.
Author information
Authors and affiliations.
Political Science Department, University of North Dakota, 293 Centennial Drive, Grand Forks, ND, USA
timothy prescott & brian r. urlacher
You can also search for this author in PubMed Google Scholar
Corresponding author
Correspondence to brian r. urlacher .
Rights and permissions
Reprints and permissions
About this article
prescott, t., urlacher, b.r. case selection and the comparative method: introducing the case selector. Eur Polit Sci 17 , 422–436 (2018). https://doi.org/10.1057/s41304-017-0128-5
Download citation
Published : 14 August 2017
Issue Date : September 2018
DOI : https://doi.org/10.1057/s41304-017-0128-5
Share this article
Anyone you share the following link with will be able to read this content:
Sorry, a shareable link is not currently available for this article.
Provided by the Springer Nature SharedIt content-sharing initiative
- comparative method
- case selection
- qualitative methods
- Find a journal
- Publish with us
- Track your research

IMAGES
VIDEO
COMMENTS
Hence attention to purposive modes of sampling is needed. Yet, while the existing qualitative literature on case selection offers a wide range of suggestions for case selection, most techniques discussed require in-depth familiarity of each case. Seven case selection procedures are considered, each of which facilitates a different strategy for ...
A good research question guides the direction of the study and informs the selection of the case, the methods of collecting data, and the analysis techniques. A well-formulated research question in case study research is typically clear, focused, and complex enough to merit further detailed examination of the relevant case(s). Propositions
It follows that case‐selection procedures in case‐study research may build upon prior cross‐case analysis and that they depend, at the very least, upon certain assumptions about the broader population. In certain circumstances, the case‐selection procedure may be structured by a quantitative analysis of the larger population. Here ...
Abstract. This chapter presents and critically examines best practices for case study research and case selection. In reviewing prominent case study criteria that prioritize objective, analytical needs of research design ("appropriateness"), the chapter argues that overlooked considerations of feasibility (including positionality, resources, and skills) and interest play equally important ...
Case selection is the primordial task of the case study researcher, for in choosing cases, one also sets out an agenda for studying those cases. This means that case selection and case analysis are inter twined to a much greater extent in case study research than in large-Af cross-case analysis. Indeed, the method of choosing cases and ...
Revised on November 20, 2023. A case study is a detailed study of a specific subject, such as a person, group, place, event, organization, or phenomenon. Case studies are commonly used in social, educational, clinical, and business research. A case study research design usually involves qualitative methods, but quantitative methods are ...
Figure 3.1 gives a snapshot of the types of case studies and basic selection strategies and additionally demonstrates that distinguishing between the three dimensions introduced in Chapter 1 - research goals, levels of analysis, and variants of causal effects - is central for case studies and case selection. 2.
This chapter discusses quantitative and qualitative practices of case-study selection when the goal of the analysis is to evaluate causal hypotheses. More specifically, it considers how the different causal models used in the qualitative and quantitative research cultures shape the kind of cases that provide the most leverage for hypothesis ...
Abstract. For most researchers, case selection defines method: a few cases of a particular phenomenon make a study 'qualitative' but a lot of cases turns it into a 'quantitative' analysis. Usually a case is equated with a country, and there is often an implicit presumption that some sort of history will be traced.
"Case Selection Techniques in Case Study Research." Political Research Quarterly 61 (June 2008): 294-308. Structure and Writing Style. The purpose of a paper in the social sciences designed around a case study is to thoroughly investigate a subject of analysis in order to reveal a new understanding about the research problem and, in so doing ...
Case-selection and qualitative comparisons. Methodological advice on the selection of cases in qualitative research stands in a long tradition. John Stuart Mill in his A System of Logic, first published in 1843, proposed five methods meant to enable researchers to make causal inferences: the method of agreement, the method of difference, the double method of agreement and difference, the ...
and Case Selection Each empirical field of study can be described by the cases ("units") analysed, the characteristics of cases ("variables") being considered and the number of times each unit is observed ("observations"). In macro-qualitative small-N situations, which is the domain we are concerned
7.2.1 Case Selection Before the Processual Turn . Before "process tracing" entered the lexicon of social scientists, the dominant case selection strategy in case study research sought to maximize causal leverage via comparison, particularly via the "methods of agreement and difference" of John Stuart Reference Mill Mill (1843 [1974]: 388-391).
Case-selection is the primordial task of the case study researcher, for in choosing cases one also sets out an agenda for studying those cases. This means that case selection and case analysis are intertwined to a much greater extent in case study research than in large-N cross-case analysis.
Second, case selection may be driven by area studies. This is still based on the interests of the researcher as generally speaking scholars pick areas of studies due to their personal interests. For example, the same researcher may research COVID-19 infection rates among European Union member-states. Finally, the selection of cases selected may ...
Case selection via matching can also be criticized from the opposite direc-tion: When enoughdata are available toselectcases from a relativelylarge pool, perhaps regression analysis should be preferred over qualitative case studies. This critique ignores the goals of case study researchers, most of whom are try-ingtotracecausalmechanisms ...
T1 - Case-Selection Techniques in Case Study Research: A Menu of Qualitative and Quantitative Options. AU - Seawright, Jason. PY - 2008. Y1 - 2008. N2 - How can scholars select cases from a large universe for in-depth case study analysis? Random sampling is not typically a viable approach when the total number of cases to be selected is small.
The vendor provided the use of the AI workstation, but did not have any input on study design, case selection, data analysis and interpretation, or manuscript preparation. The algorithm provided case-level cancer detection and risk scores for screening DBT examinations. The case score ranges from 0 to 100, and per the vendor ...
In addition, case selection and access in case study research also pose practical challenges to qualitative researchers. Case selection and access is a context-situated process, which involves the dynamic interactions between researchers and the various parties in the process. Simply following the existing literature or listing a number of ...
Background High stakes examinations used to credential trainees for independent specialist practice should be evaluated periodically to ensure defensible decisions are made. This study aims to quantify the College of Intensive Care Medicine of Australia and New Zealand (CICM) Hot Case reliability coefficient and evaluate contributions to variance from candidates, cases and examiners. Methods ...
For instance, Li et al. (Citation 2020) argued that continuous investment in research and development is among the key determinants of eco-innovation in renewable energy.Additionally, recent studies by Achmad et al. (Citation 2023) underscore the pivotal role of government support, policies and regulatory frameworks, while Chen and Liu (Citation 2020) emphasize the significance of customer ...
With the advancement of information technology, the Internet is pivotal in today's society, serving as a global connectivity platform. Leveraging Internet technology within an enterprise can improve operational efficiency and curtail costs. However, traditional Internet platform selection methods cannot simultaneously handle quantitative and qualitative information, fuzzy semantics, and ...
However, studies show that racial and ethnic minorities, women, and older adult patients are least likely to enroll in oncology treatment trials compared to White patients, men, and younger populations. Thus, in this study, the group questioned how oncology treatment trial design influences enrollment of racial and ethnic minorities.
Abstract. This chapter examines the criteria for selecting the cases analysed in this study. It explains the application of critical discourse analysis (CDA) as its principal empirical method. It discusses CDA methods and data treatment as well as the empirical analysis of CDA results. Finally, it compares and summarises the case selection ...
In this study, a clustering-based method, Neighborhood Components Analysis (NCA), was used to perform dimensionality reduction and wavelength selection on HS data.
The case study consists of a core case that describes both the IT system currently in use and the new system that provides updated business support. Students are tasked with analyzing the system and designing a new system that delivers enhanced functionality. This evolutionary case study is based on the Modular Design of Teaching Cases and ...
Thus, case selection for both case study designs and public opinion polling is best understood as a mix of systematic method and art. 7. A similar strategy is proposed by Sambanis , who calls for case study research designs that focus on cases predicted well and predicted poorly by regression models. 8.