
- University of Texas Libraries

Literature Reviews
Steps in the literature review process.
- What is a literature review?
- Define your research question
- Determine inclusion and exclusion criteria
- Choose databases and search
- Review Results
- Synthesize Results
- Analyze Results
- Librarian Support
- You may need to some exploratory searching of the literature to get a sense of scope, to determine whether you need to narrow or broaden your focus
- Identify databases that provide the most relevant sources, and identify relevant terms (controlled vocabularies) to add to your search strategy
- Finalize your research question
- Think about relevant dates, geographies (and languages), methods, and conflicting points of view
- Conduct searches in the published literature via the identified databases
- Check to see if this topic has been covered in other discipline's databases
- Examine the citations of on-point articles for keywords, authors, and previous research (via references) and cited reference searching.
- Save your search results in a citation management tool (such as Zotero, Mendeley or EndNote)
- De-duplicate your search results
- Make sure that you've found the seminal pieces -- they have been cited many times, and their work is considered foundational
- Check with your professor or a librarian to make sure your search has been comprehensive
- Evaluate the strengths and weaknesses of individual sources and evaluate for bias, methodologies, and thoroughness
- Group your results in to an organizational structure that will support why your research needs to be done, or that provides the answer to your research question
- Develop your conclusions
- Are there gaps in the literature?
- Where has significant research taken place, and who has done it?
- Is there consensus or debate on this topic?
- Which methodological approaches work best?
- For example: Background, Current Practices, Critics and Proponents, Where/How this study will fit in
- Organize your citations and focus on your research question and pertinent studies
- Compile your bibliography
Note: The first four steps are the best points at which to contact a librarian. Your librarian can help you determine the best databases to use for your topic, assess scope, and formulate a search strategy.
Videos Tutorials about Literature Reviews
This 4.5 minute video from Academic Education Materials has a Creative Commons License and a British narrator.
Recommended Reading
- Last Updated: Oct 26, 2022 2:49 PM
- URL: https://guides.lib.utexas.edu/literaturereviews
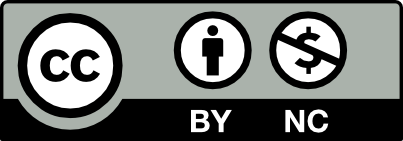

Research Process :: Step by Step
- Introduction
- Select Topic
- Identify Keywords
- Background Information
- Develop Research Questions
- Refine Topic
- Search Strategy
- Popular Databases
- Evaluate Sources
- Types of Periodicals
- Reading Scholarly Articles
- Primary & Secondary Sources
- Organize / Take Notes
- Writing & Grammar Resources
- Annotated Bibliography
- Literature Review
- Citation Styles
- Paraphrasing
- Privacy / Confidentiality
- Research Process
- Selecting Your Topic
- Identifying Keywords
- Gathering Background Info
- Evaluating Sources

Organize the literature review into sections that present themes or identify trends, including relevant theory. You are not trying to list all the material published, but to synthesize and evaluate it according to the guiding concept of your thesis or research question.
What is a literature review?
A literature review is an account of what has been published on a topic by accredited scholars and researchers. Occasionally you will be asked to write one as a separate assignment, but more often it is part of the introduction to an essay, research report, or thesis. In writing the literature review, your purpose is to convey to your reader what knowledge and ideas have been established on a topic, and what their strengths and weaknesses are. As a piece of writing, the literature review must be defined by a guiding concept (e.g., your research objective, the problem or issue you are discussing, or your argumentative thesis). It is not just a descriptive list of the material available, or a set of summaries
A literature review must do these things:
- be organized around and related directly to the thesis or research question you are developing
- synthesize results into a summary of what is and is not known
- identify areas of controversy in the literature
- formulate questions that need further research
Ask yourself questions like these:
- What is the specific thesis, problem, or research question that my literature review helps to define?
- What type of literature review am I conducting? Am I looking at issues of theory? methodology? policy? quantitative research (e.g. on the effectiveness of a new procedure)? qualitative research (e.g., studies of loneliness among migrant workers)?
- What is the scope of my literature review? What types of publications am I using (e.g., journals, books, government documents, popular media)? What discipline am I working in (e.g., nursing psychology, sociology, medicine)?
- How good was my information seeking? Has my search been wide enough to ensure I've found all the relevant material? Has it been narrow enough to exclude irrelevant material? Is the number of sources I've used appropriate for the length of my paper?
- Have I critically analyzed the literature I use? Do I follow through a set of concepts and questions, comparing items to each other in the ways they deal with them? Instead of just listing and summarizing items, do I assess them, discussing strengths and weaknesses?
- Have I cited and discussed studies contrary to my perspective?
- Will the reader find my literature review relevant, appropriate, and useful?
Ask yourself questions like these about each book or article you include:
- Has the author formulated a problem/issue?
- Is it clearly defined? Is its significance (scope, severity, relevance) clearly established?
- Could the problem have been approached more effectively from another perspective?
- What is the author's research orientation (e.g., interpretive, critical science, combination)?
- What is the author's theoretical framework (e.g., psychological, developmental, feminist)?
- What is the relationship between the theoretical and research perspectives?
- Has the author evaluated the literature relevant to the problem/issue? Does the author include literature taking positions she or he does not agree with?
- In a research study, how good are the basic components of the study design (e.g., population, intervention, outcome)? How accurate and valid are the measurements? Is the analysis of the data accurate and relevant to the research question? Are the conclusions validly based upon the data and analysis?
- In material written for a popular readership, does the author use appeals to emotion, one-sided examples, or rhetorically-charged language and tone? Is there an objective basis to the reasoning, or is the author merely "proving" what he or she already believes?
- How does the author structure the argument? Can you "deconstruct" the flow of the argument to see whether or where it breaks down logically (e.g., in establishing cause-effect relationships)?
- In what ways does this book or article contribute to our understanding of the problem under study, and in what ways is it useful for practice? What are the strengths and limitations?
- How does this book or article relate to the specific thesis or question I am developing?
Text written by Dena Taylor, Health Sciences Writing Centre, University of Toronto
http://www.writing.utoronto.ca/advice/specific-types-of-writing/literature-review
- << Previous: Annotated Bibliography
- Next: Step 5: Cite Sources >>
- Last Updated: May 13, 2024 11:24 AM
- URL: https://libguides.uta.edu/researchprocess
University of Texas Arlington Libraries 702 Planetarium Place · Arlington, TX 76019 · 817-272-3000
- Internet Privacy
- Accessibility
- Problems with a guide? Contact Us.
Purdue Online Writing Lab Purdue OWL® College of Liberal Arts
Writing a Literature Review
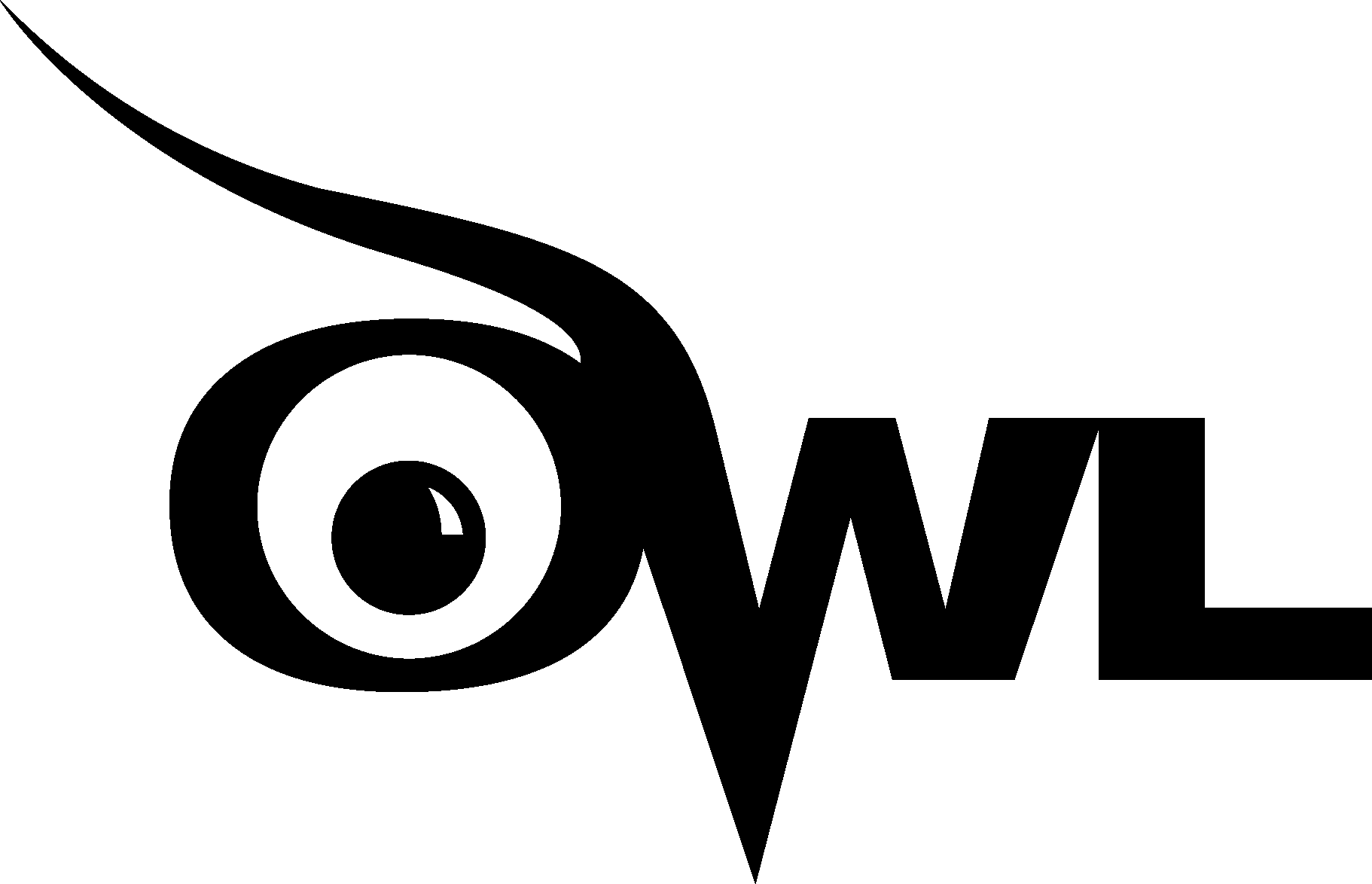
Welcome to the Purdue OWL
This page is brought to you by the OWL at Purdue University. When printing this page, you must include the entire legal notice.
Copyright ©1995-2018 by The Writing Lab & The OWL at Purdue and Purdue University. All rights reserved. This material may not be published, reproduced, broadcast, rewritten, or redistributed without permission. Use of this site constitutes acceptance of our terms and conditions of fair use.
A literature review is a document or section of a document that collects key sources on a topic and discusses those sources in conversation with each other (also called synthesis ). The lit review is an important genre in many disciplines, not just literature (i.e., the study of works of literature such as novels and plays). When we say “literature review” or refer to “the literature,” we are talking about the research ( scholarship ) in a given field. You will often see the terms “the research,” “the scholarship,” and “the literature” used mostly interchangeably.
Where, when, and why would I write a lit review?
There are a number of different situations where you might write a literature review, each with slightly different expectations; different disciplines, too, have field-specific expectations for what a literature review is and does. For instance, in the humanities, authors might include more overt argumentation and interpretation of source material in their literature reviews, whereas in the sciences, authors are more likely to report study designs and results in their literature reviews; these differences reflect these disciplines’ purposes and conventions in scholarship. You should always look at examples from your own discipline and talk to professors or mentors in your field to be sure you understand your discipline’s conventions, for literature reviews as well as for any other genre.
A literature review can be a part of a research paper or scholarly article, usually falling after the introduction and before the research methods sections. In these cases, the lit review just needs to cover scholarship that is important to the issue you are writing about; sometimes it will also cover key sources that informed your research methodology.
Lit reviews can also be standalone pieces, either as assignments in a class or as publications. In a class, a lit review may be assigned to help students familiarize themselves with a topic and with scholarship in their field, get an idea of the other researchers working on the topic they’re interested in, find gaps in existing research in order to propose new projects, and/or develop a theoretical framework and methodology for later research. As a publication, a lit review usually is meant to help make other scholars’ lives easier by collecting and summarizing, synthesizing, and analyzing existing research on a topic. This can be especially helpful for students or scholars getting into a new research area, or for directing an entire community of scholars toward questions that have not yet been answered.
What are the parts of a lit review?
Most lit reviews use a basic introduction-body-conclusion structure; if your lit review is part of a larger paper, the introduction and conclusion pieces may be just a few sentences while you focus most of your attention on the body. If your lit review is a standalone piece, the introduction and conclusion take up more space and give you a place to discuss your goals, research methods, and conclusions separately from where you discuss the literature itself.
Introduction:
- An introductory paragraph that explains what your working topic and thesis is
- A forecast of key topics or texts that will appear in the review
- Potentially, a description of how you found sources and how you analyzed them for inclusion and discussion in the review (more often found in published, standalone literature reviews than in lit review sections in an article or research paper)
- Summarize and synthesize: Give an overview of the main points of each source and combine them into a coherent whole
- Analyze and interpret: Don’t just paraphrase other researchers – add your own interpretations where possible, discussing the significance of findings in relation to the literature as a whole
- Critically Evaluate: Mention the strengths and weaknesses of your sources
- Write in well-structured paragraphs: Use transition words and topic sentence to draw connections, comparisons, and contrasts.
Conclusion:
- Summarize the key findings you have taken from the literature and emphasize their significance
- Connect it back to your primary research question
How should I organize my lit review?
Lit reviews can take many different organizational patterns depending on what you are trying to accomplish with the review. Here are some examples:
- Chronological : The simplest approach is to trace the development of the topic over time, which helps familiarize the audience with the topic (for instance if you are introducing something that is not commonly known in your field). If you choose this strategy, be careful to avoid simply listing and summarizing sources in order. Try to analyze the patterns, turning points, and key debates that have shaped the direction of the field. Give your interpretation of how and why certain developments occurred (as mentioned previously, this may not be appropriate in your discipline — check with a teacher or mentor if you’re unsure).
- Thematic : If you have found some recurring central themes that you will continue working with throughout your piece, you can organize your literature review into subsections that address different aspects of the topic. For example, if you are reviewing literature about women and religion, key themes can include the role of women in churches and the religious attitude towards women.
- Qualitative versus quantitative research
- Empirical versus theoretical scholarship
- Divide the research by sociological, historical, or cultural sources
- Theoretical : In many humanities articles, the literature review is the foundation for the theoretical framework. You can use it to discuss various theories, models, and definitions of key concepts. You can argue for the relevance of a specific theoretical approach or combine various theorical concepts to create a framework for your research.
What are some strategies or tips I can use while writing my lit review?
Any lit review is only as good as the research it discusses; make sure your sources are well-chosen and your research is thorough. Don’t be afraid to do more research if you discover a new thread as you’re writing. More info on the research process is available in our "Conducting Research" resources .
As you’re doing your research, create an annotated bibliography ( see our page on the this type of document ). Much of the information used in an annotated bibliography can be used also in a literature review, so you’ll be not only partially drafting your lit review as you research, but also developing your sense of the larger conversation going on among scholars, professionals, and any other stakeholders in your topic.
Usually you will need to synthesize research rather than just summarizing it. This means drawing connections between sources to create a picture of the scholarly conversation on a topic over time. Many student writers struggle to synthesize because they feel they don’t have anything to add to the scholars they are citing; here are some strategies to help you:
- It often helps to remember that the point of these kinds of syntheses is to show your readers how you understand your research, to help them read the rest of your paper.
- Writing teachers often say synthesis is like hosting a dinner party: imagine all your sources are together in a room, discussing your topic. What are they saying to each other?
- Look at the in-text citations in each paragraph. Are you citing just one source for each paragraph? This usually indicates summary only. When you have multiple sources cited in a paragraph, you are more likely to be synthesizing them (not always, but often
- Read more about synthesis here.
The most interesting literature reviews are often written as arguments (again, as mentioned at the beginning of the page, this is discipline-specific and doesn’t work for all situations). Often, the literature review is where you can establish your research as filling a particular gap or as relevant in a particular way. You have some chance to do this in your introduction in an article, but the literature review section gives a more extended opportunity to establish the conversation in the way you would like your readers to see it. You can choose the intellectual lineage you would like to be part of and whose definitions matter most to your thinking (mostly humanities-specific, but this goes for sciences as well). In addressing these points, you argue for your place in the conversation, which tends to make the lit review more compelling than a simple reporting of other sources.
Have a language expert improve your writing
Run a free plagiarism check in 10 minutes, automatically generate references for free.
- Knowledge Base
- Dissertation
- What is a Literature Review? | Guide, Template, & Examples
What is a Literature Review? | Guide, Template, & Examples
Published on 22 February 2022 by Shona McCombes . Revised on 7 June 2022.
What is a literature review? A literature review is a survey of scholarly sources on a specific topic. It provides an overview of current knowledge, allowing you to identify relevant theories, methods, and gaps in the existing research.
There are five key steps to writing a literature review:
- Search for relevant literature
- Evaluate sources
- Identify themes, debates and gaps
- Outline the structure
- Write your literature review
A good literature review doesn’t just summarise sources – it analyses, synthesises, and critically evaluates to give a clear picture of the state of knowledge on the subject.
Instantly correct all language mistakes in your text
Be assured that you'll submit flawless writing. Upload your document to correct all your mistakes.
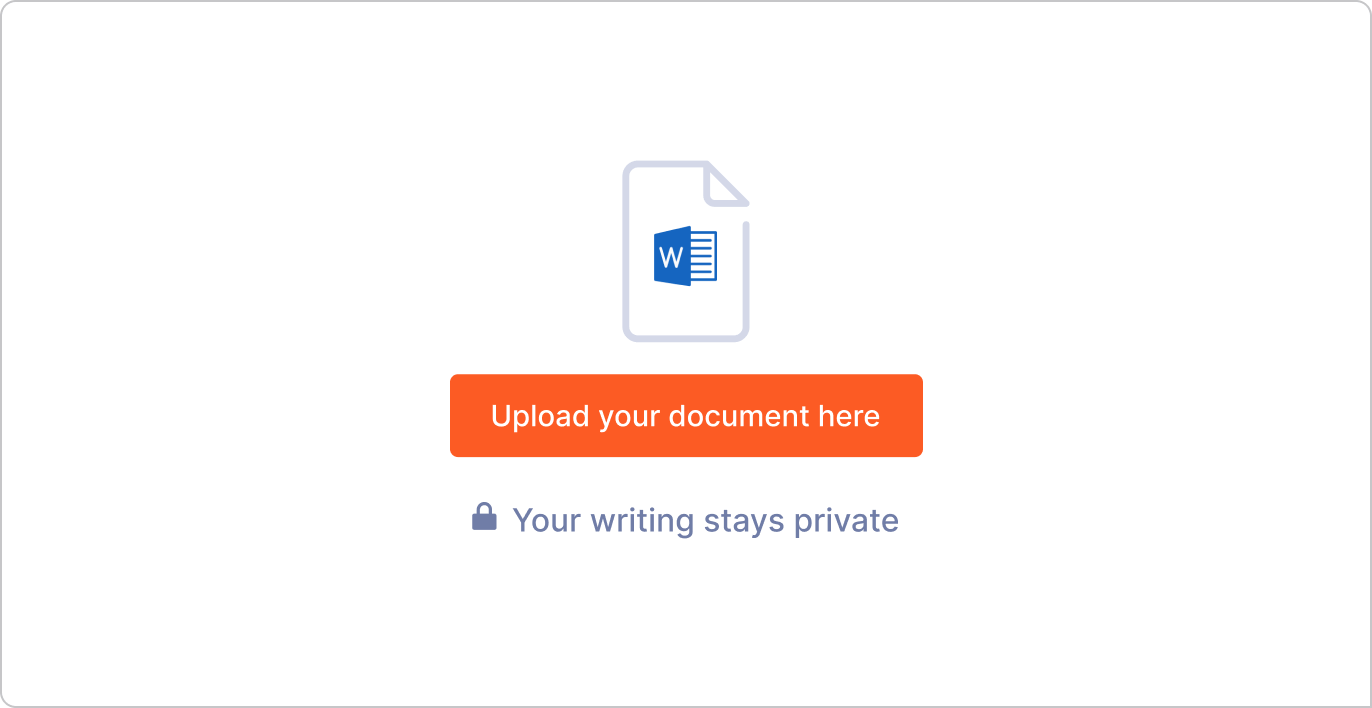
Table of contents
Why write a literature review, examples of literature reviews, step 1: search for relevant literature, step 2: evaluate and select sources, step 3: identify themes, debates and gaps, step 4: outline your literature review’s structure, step 5: write your literature review, frequently asked questions about literature reviews, introduction.
- Quick Run-through
- Step 1 & 2
When you write a dissertation or thesis, you will have to conduct a literature review to situate your research within existing knowledge. The literature review gives you a chance to:
- Demonstrate your familiarity with the topic and scholarly context
- Develop a theoretical framework and methodology for your research
- Position yourself in relation to other researchers and theorists
- Show how your dissertation addresses a gap or contributes to a debate
You might also have to write a literature review as a stand-alone assignment. In this case, the purpose is to evaluate the current state of research and demonstrate your knowledge of scholarly debates around a topic.
The content will look slightly different in each case, but the process of conducting a literature review follows the same steps. We’ve written a step-by-step guide that you can follow below.
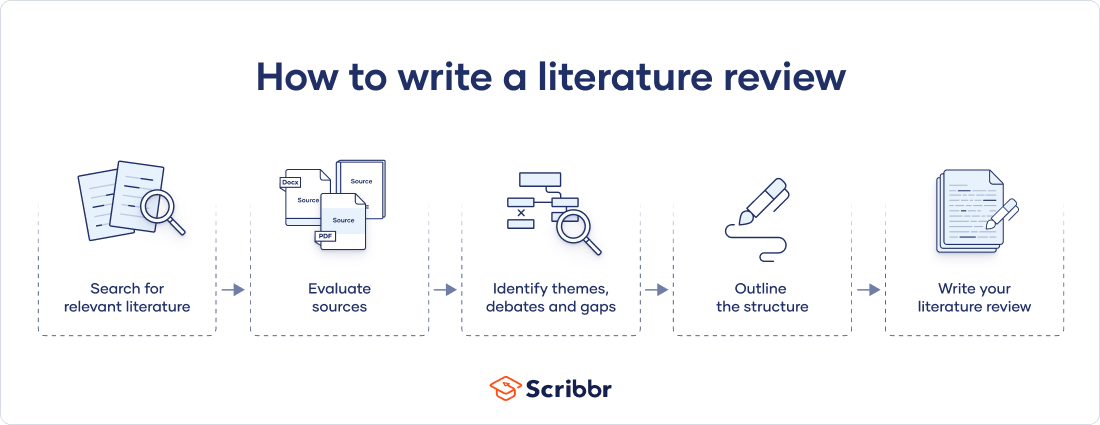
The only proofreading tool specialized in correcting academic writing
The academic proofreading tool has been trained on 1000s of academic texts and by native English editors. Making it the most accurate and reliable proofreading tool for students.
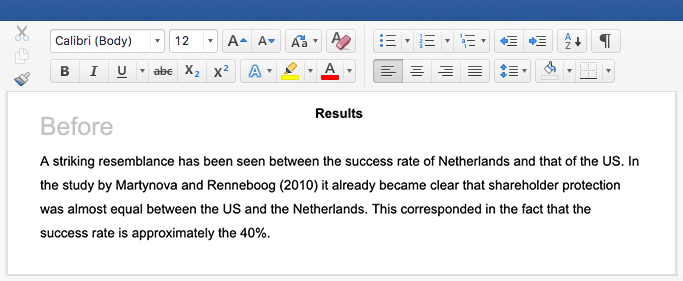
Correct my document today
Writing literature reviews can be quite challenging! A good starting point could be to look at some examples, depending on what kind of literature review you’d like to write.
- Example literature review #1: “Why Do People Migrate? A Review of the Theoretical Literature” ( Theoretical literature review about the development of economic migration theory from the 1950s to today.)
- Example literature review #2: “Literature review as a research methodology: An overview and guidelines” ( Methodological literature review about interdisciplinary knowledge acquisition and production.)
- Example literature review #3: “The Use of Technology in English Language Learning: A Literature Review” ( Thematic literature review about the effects of technology on language acquisition.)
- Example literature review #4: “Learners’ Listening Comprehension Difficulties in English Language Learning: A Literature Review” ( Chronological literature review about how the concept of listening skills has changed over time.)
You can also check out our templates with literature review examples and sample outlines at the links below.
Download Word doc Download Google doc
Before you begin searching for literature, you need a clearly defined topic .
If you are writing the literature review section of a dissertation or research paper, you will search for literature related to your research objectives and questions .
If you are writing a literature review as a stand-alone assignment, you will have to choose a focus and develop a central question to direct your search. Unlike a dissertation research question, this question has to be answerable without collecting original data. You should be able to answer it based only on a review of existing publications.
Make a list of keywords
Start by creating a list of keywords related to your research topic. Include each of the key concepts or variables you’re interested in, and list any synonyms and related terms. You can add to this list if you discover new keywords in the process of your literature search.
- Social media, Facebook, Instagram, Twitter, Snapchat, TikTok
- Body image, self-perception, self-esteem, mental health
- Generation Z, teenagers, adolescents, youth
Search for relevant sources
Use your keywords to begin searching for sources. Some databases to search for journals and articles include:
- Your university’s library catalogue
- Google Scholar
- Project Muse (humanities and social sciences)
- Medline (life sciences and biomedicine)
- EconLit (economics)
- Inspec (physics, engineering and computer science)
You can use boolean operators to help narrow down your search:
Read the abstract to find out whether an article is relevant to your question. When you find a useful book or article, you can check the bibliography to find other relevant sources.
To identify the most important publications on your topic, take note of recurring citations. If the same authors, books or articles keep appearing in your reading, make sure to seek them out.
You probably won’t be able to read absolutely everything that has been written on the topic – you’ll have to evaluate which sources are most relevant to your questions.
For each publication, ask yourself:
- What question or problem is the author addressing?
- What are the key concepts and how are they defined?
- What are the key theories, models and methods? Does the research use established frameworks or take an innovative approach?
- What are the results and conclusions of the study?
- How does the publication relate to other literature in the field? Does it confirm, add to, or challenge established knowledge?
- How does the publication contribute to your understanding of the topic? What are its key insights and arguments?
- What are the strengths and weaknesses of the research?
Make sure the sources you use are credible, and make sure you read any landmark studies and major theories in your field of research.
You can find out how many times an article has been cited on Google Scholar – a high citation count means the article has been influential in the field, and should certainly be included in your literature review.
The scope of your review will depend on your topic and discipline: in the sciences you usually only review recent literature, but in the humanities you might take a long historical perspective (for example, to trace how a concept has changed in meaning over time).
Remember that you can use our template to summarise and evaluate sources you’re thinking about using!
Take notes and cite your sources
As you read, you should also begin the writing process. Take notes that you can later incorporate into the text of your literature review.
It’s important to keep track of your sources with references to avoid plagiarism . It can be helpful to make an annotated bibliography, where you compile full reference information and write a paragraph of summary and analysis for each source. This helps you remember what you read and saves time later in the process.
You can use our free APA Reference Generator for quick, correct, consistent citations.
Prevent plagiarism, run a free check.
To begin organising your literature review’s argument and structure, you need to understand the connections and relationships between the sources you’ve read. Based on your reading and notes, you can look for:
- Trends and patterns (in theory, method or results): do certain approaches become more or less popular over time?
- Themes: what questions or concepts recur across the literature?
- Debates, conflicts and contradictions: where do sources disagree?
- Pivotal publications: are there any influential theories or studies that changed the direction of the field?
- Gaps: what is missing from the literature? Are there weaknesses that need to be addressed?
This step will help you work out the structure of your literature review and (if applicable) show how your own research will contribute to existing knowledge.
- Most research has focused on young women.
- There is an increasing interest in the visual aspects of social media.
- But there is still a lack of robust research on highly-visual platforms like Instagram and Snapchat – this is a gap that you could address in your own research.
There are various approaches to organising the body of a literature review. You should have a rough idea of your strategy before you start writing.
Depending on the length of your literature review, you can combine several of these strategies (for example, your overall structure might be thematic, but each theme is discussed chronologically).
Chronological
The simplest approach is to trace the development of the topic over time. However, if you choose this strategy, be careful to avoid simply listing and summarising sources in order.
Try to analyse patterns, turning points and key debates that have shaped the direction of the field. Give your interpretation of how and why certain developments occurred.
If you have found some recurring central themes, you can organise your literature review into subsections that address different aspects of the topic.
For example, if you are reviewing literature about inequalities in migrant health outcomes, key themes might include healthcare policy, language barriers, cultural attitudes, legal status, and economic access.
Methodological
If you draw your sources from different disciplines or fields that use a variety of research methods , you might want to compare the results and conclusions that emerge from different approaches. For example:
- Look at what results have emerged in qualitative versus quantitative research
- Discuss how the topic has been approached by empirical versus theoretical scholarship
- Divide the literature into sociological, historical, and cultural sources
Theoretical
A literature review is often the foundation for a theoretical framework . You can use it to discuss various theories, models, and definitions of key concepts.
You might argue for the relevance of a specific theoretical approach, or combine various theoretical concepts to create a framework for your research.
Like any other academic text, your literature review should have an introduction , a main body, and a conclusion . What you include in each depends on the objective of your literature review.
The introduction should clearly establish the focus and purpose of the literature review.
If you are writing the literature review as part of your dissertation or thesis, reiterate your central problem or research question and give a brief summary of the scholarly context. You can emphasise the timeliness of the topic (“many recent studies have focused on the problem of x”) or highlight a gap in the literature (“while there has been much research on x, few researchers have taken y into consideration”).
Depending on the length of your literature review, you might want to divide the body into subsections. You can use a subheading for each theme, time period, or methodological approach.
As you write, make sure to follow these tips:
- Summarise and synthesise: give an overview of the main points of each source and combine them into a coherent whole.
- Analyse and interpret: don’t just paraphrase other researchers – add your own interpretations, discussing the significance of findings in relation to the literature as a whole.
- Critically evaluate: mention the strengths and weaknesses of your sources.
- Write in well-structured paragraphs: use transitions and topic sentences to draw connections, comparisons and contrasts.
In the conclusion, you should summarise the key findings you have taken from the literature and emphasise their significance.
If the literature review is part of your dissertation or thesis, reiterate how your research addresses gaps and contributes new knowledge, or discuss how you have drawn on existing theories and methods to build a framework for your research. This can lead directly into your methodology section.
A literature review is a survey of scholarly sources (such as books, journal articles, and theses) related to a specific topic or research question .
It is often written as part of a dissertation , thesis, research paper , or proposal .
There are several reasons to conduct a literature review at the beginning of a research project:
- To familiarise yourself with the current state of knowledge on your topic
- To ensure that you’re not just repeating what others have already done
- To identify gaps in knowledge and unresolved problems that your research can address
- To develop your theoretical framework and methodology
- To provide an overview of the key findings and debates on the topic
Writing the literature review shows your reader how your work relates to existing research and what new insights it will contribute.
The literature review usually comes near the beginning of your dissertation . After the introduction , it grounds your research in a scholarly field and leads directly to your theoretical framework or methodology .
Cite this Scribbr article
If you want to cite this source, you can copy and paste the citation or click the ‘Cite this Scribbr article’ button to automatically add the citation to our free Reference Generator.
McCombes, S. (2022, June 07). What is a Literature Review? | Guide, Template, & Examples. Scribbr. Retrieved 13 May 2024, from https://www.scribbr.co.uk/thesis-dissertation/literature-review/
Is this article helpful?
Shona McCombes
Other students also liked, how to write a dissertation proposal | a step-by-step guide, what is a theoretical framework | a step-by-step guide, what is a research methodology | steps & tips.
Harvey Cushing/John Hay Whitney Medical Library
- Collections
- Research Help
YSN Doctoral Programs: Steps in Conducting a Literature Review
- Biomedical Databases
- Global (Public Health) Databases
- Soc. Sci., History, and Law Databases
- Grey Literature
- Trials Registers
- Data and Statistics
- Public Policy
- Google Tips
- Recommended Books
- Steps in Conducting a Literature Review
What is a literature review?
A literature review is an integrated analysis -- not just a summary-- of scholarly writings and other relevant evidence related directly to your research question. That is, it represents a synthesis of the evidence that provides background information on your topic and shows a association between the evidence and your research question.
A literature review may be a stand alone work or the introduction to a larger research paper, depending on the assignment. Rely heavily on the guidelines your instructor has given you.
Why is it important?
A literature review is important because it:
- Explains the background of research on a topic.
- Demonstrates why a topic is significant to a subject area.
- Discovers relationships between research studies/ideas.
- Identifies major themes, concepts, and researchers on a topic.
- Identifies critical gaps and points of disagreement.
- Discusses further research questions that logically come out of the previous studies.
APA7 Style resources
APA Style Blog - for those harder to find answers
1. Choose a topic. Define your research question.
Your literature review should be guided by your central research question. The literature represents background and research developments related to a specific research question, interpreted and analyzed by you in a synthesized way.
- Make sure your research question is not too broad or too narrow. Is it manageable?
- Begin writing down terms that are related to your question. These will be useful for searches later.
- If you have the opportunity, discuss your topic with your professor and your class mates.
2. Decide on the scope of your review
How many studies do you need to look at? How comprehensive should it be? How many years should it cover?
- This may depend on your assignment. How many sources does the assignment require?
3. Select the databases you will use to conduct your searches.
Make a list of the databases you will search.
Where to find databases:
- use the tabs on this guide
- Find other databases in the Nursing Information Resources web page
- More on the Medical Library web page
- ... and more on the Yale University Library web page
4. Conduct your searches to find the evidence. Keep track of your searches.
- Use the key words in your question, as well as synonyms for those words, as terms in your search. Use the database tutorials for help.
- Save the searches in the databases. This saves time when you want to redo, or modify, the searches. It is also helpful to use as a guide is the searches are not finding any useful results.
- Review the abstracts of research studies carefully. This will save you time.
- Use the bibliographies and references of research studies you find to locate others.
- Check with your professor, or a subject expert in the field, if you are missing any key works in the field.
- Ask your librarian for help at any time.
- Use a citation manager, such as EndNote as the repository for your citations. See the EndNote tutorials for help.
Review the literature
Some questions to help you analyze the research:
- What was the research question of the study you are reviewing? What were the authors trying to discover?
- Was the research funded by a source that could influence the findings?
- What were the research methodologies? Analyze its literature review, the samples and variables used, the results, and the conclusions.
- Does the research seem to be complete? Could it have been conducted more soundly? What further questions does it raise?
- If there are conflicting studies, why do you think that is?
- How are the authors viewed in the field? Has this study been cited? If so, how has it been analyzed?
Tips:
- Review the abstracts carefully.
- Keep careful notes so that you may track your thought processes during the research process.
- Create a matrix of the studies for easy analysis, and synthesis, across all of the studies.
- << Previous: Recommended Books
- Last Updated: Jan 4, 2024 10:52 AM
- URL: https://guides.library.yale.edu/YSNDoctoral
Libraries | Research Guides
Literature reviews, what is a literature review, learning more about how to do a literature review.
- Planning the Review
- The Research Question
- Choosing Where to Search
- Organizing the Review
- Writing the Review
A literature review is a review and synthesis of existing research on a topic or research question. A literature review is meant to analyze the scholarly literature, make connections across writings and identify strengths, weaknesses, trends, and missing conversations. A literature review should address different aspects of a topic as it relates to your research question. A literature review goes beyond a description or summary of the literature you have read.
- Sage Research Methods Core Collection This link opens in a new window SAGE Research Methods supports research at all levels by providing material to guide users through every step of the research process. SAGE Research Methods is the ultimate methods library with more than 1000 books, reference works, journal articles, and instructional videos by world-leading academics from across the social sciences, including the largest collection of qualitative methods books available online from any scholarly publisher. – Publisher
- Next: Planning the Review >>
- Last Updated: May 2, 2024 10:39 AM
- URL: https://libguides.northwestern.edu/literaturereviews
- UConn Library
- Literature Review: The What, Why and How-to Guide
- Introduction
Literature Review: The What, Why and How-to Guide — Introduction
- Getting Started
- How to Pick a Topic
- Strategies to Find Sources
- Evaluating Sources & Lit. Reviews
- Tips for Writing Literature Reviews
- Writing Literature Review: Useful Sites
- Citation Resources
- Other Academic Writings
What are Literature Reviews?
So, what is a literature review? "A literature review is an account of what has been published on a topic by accredited scholars and researchers. In writing the literature review, your purpose is to convey to your reader what knowledge and ideas have been established on a topic, and what their strengths and weaknesses are. As a piece of writing, the literature review must be defined by a guiding concept (e.g., your research objective, the problem or issue you are discussing, or your argumentative thesis). It is not just a descriptive list of the material available, or a set of summaries." Taylor, D. The literature review: A few tips on conducting it . University of Toronto Health Sciences Writing Centre.
Goals of Literature Reviews
What are the goals of creating a Literature Review? A literature could be written to accomplish different aims:
- To develop a theory or evaluate an existing theory
- To summarize the historical or existing state of a research topic
- Identify a problem in a field of research
Baumeister, R. F., & Leary, M. R. (1997). Writing narrative literature reviews . Review of General Psychology , 1 (3), 311-320.
What kinds of sources require a Literature Review?
- A research paper assigned in a course
- A thesis or dissertation
- A grant proposal
- An article intended for publication in a journal
All these instances require you to collect what has been written about your research topic so that you can demonstrate how your own research sheds new light on the topic.
Types of Literature Reviews
What kinds of literature reviews are written?
Narrative review: The purpose of this type of review is to describe the current state of the research on a specific topic/research and to offer a critical analysis of the literature reviewed. Studies are grouped by research/theoretical categories, and themes and trends, strengths and weakness, and gaps are identified. The review ends with a conclusion section which summarizes the findings regarding the state of the research of the specific study, the gaps identify and if applicable, explains how the author's research will address gaps identify in the review and expand the knowledge on the topic reviewed.
- Example : Predictors and Outcomes of U.S. Quality Maternity Leave: A Review and Conceptual Framework: 10.1177/08948453211037398
Systematic review : "The authors of a systematic review use a specific procedure to search the research literature, select the studies to include in their review, and critically evaluate the studies they find." (p. 139). Nelson, L. K. (2013). Research in Communication Sciences and Disorders . Plural Publishing.
- Example : The effect of leave policies on increasing fertility: a systematic review: 10.1057/s41599-022-01270-w
Meta-analysis : "Meta-analysis is a method of reviewing research findings in a quantitative fashion by transforming the data from individual studies into what is called an effect size and then pooling and analyzing this information. The basic goal in meta-analysis is to explain why different outcomes have occurred in different studies." (p. 197). Roberts, M. C., & Ilardi, S. S. (2003). Handbook of Research Methods in Clinical Psychology . Blackwell Publishing.
- Example : Employment Instability and Fertility in Europe: A Meta-Analysis: 10.1215/00703370-9164737
Meta-synthesis : "Qualitative meta-synthesis is a type of qualitative study that uses as data the findings from other qualitative studies linked by the same or related topic." (p.312). Zimmer, L. (2006). Qualitative meta-synthesis: A question of dialoguing with texts . Journal of Advanced Nursing , 53 (3), 311-318.
- Example : Women’s perspectives on career successes and barriers: A qualitative meta-synthesis: 10.1177/05390184221113735
Literature Reviews in the Health Sciences
- UConn Health subject guide on systematic reviews Explanation of the different review types used in health sciences literature as well as tools to help you find the right review type
- << Previous: Getting Started
- Next: How to Pick a Topic >>
- Last Updated: Sep 21, 2022 2:16 PM
- URL: https://guides.lib.uconn.edu/literaturereview

Literature Reviews
What is a literature review.
- Literature Review Process
Purpose of a Literature Review
- Choosing a Type of Review
- Developing a Research Question
- Searching the Literature
- Searching Tips
- ChatGPT [beta]
- Documenting your Search
- Using Citation Managers
- Concept Mapping
- Writing the Review
- Further Resources
The Library's Subject Specialists are happy to help with your literature reviews! Find your Subject Specialist here .

If you have questions about this guide, contact Librarian Jamie Niehof ([email protected]).
A literature review is an overview of the available research for a specific scientific topic. Literature reviews summarize existing research to answer a review question, provide context for new research, or identify important gaps in the existing body of literature.
An incredible amount of academic literature is published each year, by estimates over two million articles .
Sorting through and reviewing that literature can be complicated, so this Research Guide provides a structured approach to make the process more manageable.
THIS GUIDE IS AN OVERVIEW OF THE LITERATURE REVIEW PROCESS:
- Getting Started (asking a research question | defining scope)
- Organizing the Literature
- Writing the Literature Review (analyzing | synthesizing)
A literature search is a systematic search of the scholarly sources in a particular discipline. A literature review is the analysis, critical evaluation and synthesis of the results of that search. During this process you will move from a review of the literature to a review for your research. Your synthesis of the literature is your unique contribution to research.
WHO IS THIS RESEARCH GUIDE FOR?
— those new to reviewing the literature
— those that need a refresher or a deeper understanding of writing literature reviews
You may need to do a literature review as a part of a course assignment, a capstone project, a master's thesis, a dissertation, or as part of a journal article. No matter the context, a literature review is an essential part of the research process.

WHAT IS THE PURPOSE OF A LITERATURE REVIEW?
A literature review is typically performed for a specific reason. Even when assigned as an assignment, the goal of the literature review will be one or more of the following:
- To communicate a project's novelty by identifying a research gap

- An overview of research issues , methodologies or results relevant to field
- To explore the volume and types of available studies
- To establish familiarity with current research before carrying out a new project
- To resolve conflicts amongst contradictory previous studies
Reviewing the literature helps you understand a research topic and develop your own perspective.
A LITERATURE REVIEW IS NOT :
- An annotated bibliography – which is a list of annotated citations to books, articles and documents that includes a brief description and evaluation for each entry
- A literary review – which is a critical discussion of the merits and weaknesses of a literary work
- A book review – which is a critical discussion of the merits and weaknesses of a particular book
- Next: Choosing a Type of Review >>
- Last Updated: May 9, 2024 11:44 AM
- URL: https://guides.lib.umich.edu/litreview
News alert: UC Berkeley has announced its next university librarian
Secondary menu
- Log in to your Library account
- Hours and Maps
- Connect from Off Campus
- UC Berkeley Home
Search form
Conducting a literature review: why do a literature review, why do a literature review.
- How To Find "The Literature"
- Found it -- Now What?
Besides the obvious reason for students -- because it is assigned! -- a literature review helps you explore the research that has come before you, to see how your research question has (or has not) already been addressed.
You identify:
- core research in the field
- experts in the subject area
- methodology you may want to use (or avoid)
- gaps in knowledge -- or where your research would fit in
It Also Helps You:
- Publish and share your findings
- Justify requests for grants and other funding
- Identify best practices to inform practice
- Set wider context for a program evaluation
- Compile information to support community organizing
Great brief overview, from NCSU
Want To Know More?
- Next: How To Find "The Literature" >>
- Last Updated: Apr 25, 2024 1:10 PM
- URL: https://guides.lib.berkeley.edu/litreview
Get science-backed answers as you write with Paperpal's Research feature
What is a Literature Review? How to Write It (with Examples)
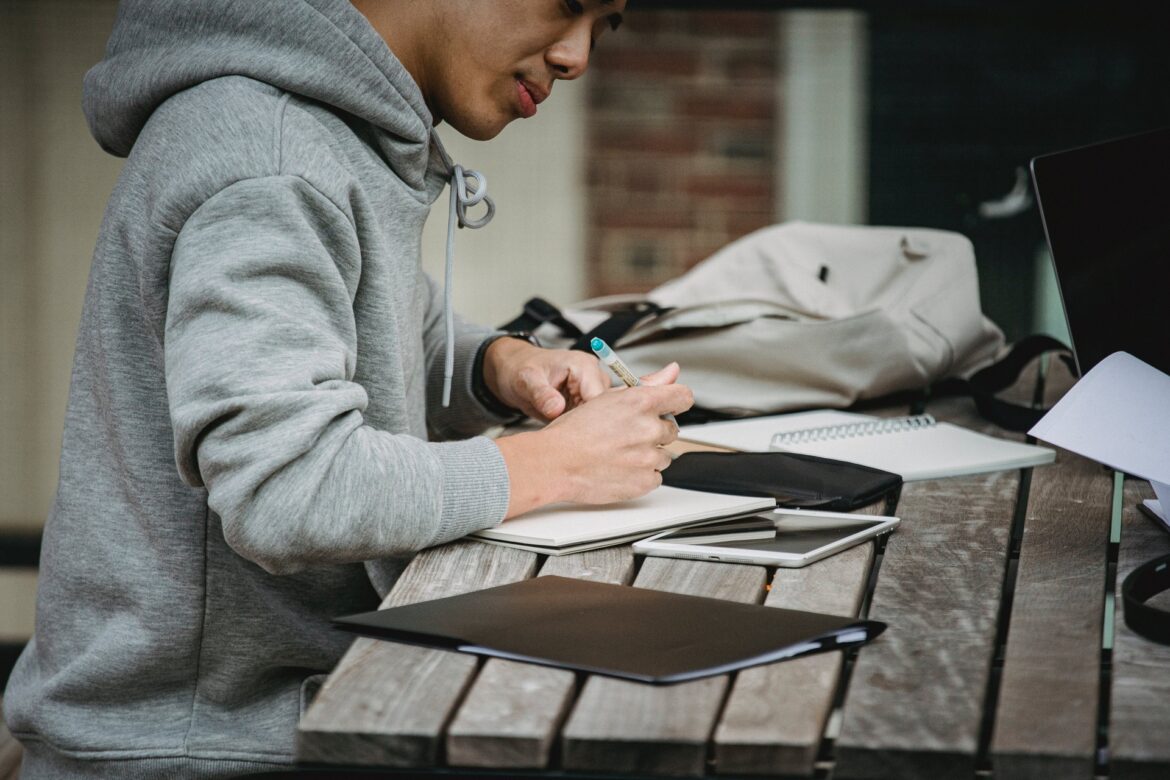
A literature review is a critical analysis and synthesis of existing research on a particular topic. It provides an overview of the current state of knowledge, identifies gaps, and highlights key findings in the literature. 1 The purpose of a literature review is to situate your own research within the context of existing scholarship, demonstrating your understanding of the topic and showing how your work contributes to the ongoing conversation in the field. Learning how to write a literature review is a critical tool for successful research. Your ability to summarize and synthesize prior research pertaining to a certain topic demonstrates your grasp on the topic of study, and assists in the learning process.
Table of Contents
- What is the purpose of literature review?
- a. Habitat Loss and Species Extinction:
- b. Range Shifts and Phenological Changes:
- c. Ocean Acidification and Coral Reefs:
- d. Adaptive Strategies and Conservation Efforts:
- How to write a good literature review
- Choose a Topic and Define the Research Question:
- Decide on the Scope of Your Review:
- Select Databases for Searches:
- Conduct Searches and Keep Track:
- Review the Literature:
- Organize and Write Your Literature Review:
- Frequently asked questions
What is a literature review?
A well-conducted literature review demonstrates the researcher’s familiarity with the existing literature, establishes the context for their own research, and contributes to scholarly conversations on the topic. One of the purposes of a literature review is also to help researchers avoid duplicating previous work and ensure that their research is informed by and builds upon the existing body of knowledge.
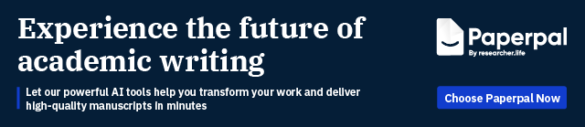
What is the purpose of literature review?
A literature review serves several important purposes within academic and research contexts. Here are some key objectives and functions of a literature review: 2
- Contextualizing the Research Problem: The literature review provides a background and context for the research problem under investigation. It helps to situate the study within the existing body of knowledge.
- Identifying Gaps in Knowledge: By identifying gaps, contradictions, or areas requiring further research, the researcher can shape the research question and justify the significance of the study. This is crucial for ensuring that the new research contributes something novel to the field.
- Understanding Theoretical and Conceptual Frameworks: Literature reviews help researchers gain an understanding of the theoretical and conceptual frameworks used in previous studies. This aids in the development of a theoretical framework for the current research.
- Providing Methodological Insights: Another purpose of literature reviews is that it allows researchers to learn about the methodologies employed in previous studies. This can help in choosing appropriate research methods for the current study and avoiding pitfalls that others may have encountered.
- Establishing Credibility: A well-conducted literature review demonstrates the researcher’s familiarity with existing scholarship, establishing their credibility and expertise in the field. It also helps in building a solid foundation for the new research.
- Informing Hypotheses or Research Questions: The literature review guides the formulation of hypotheses or research questions by highlighting relevant findings and areas of uncertainty in existing literature.
Literature review example
Let’s delve deeper with a literature review example: Let’s say your literature review is about the impact of climate change on biodiversity. You might format your literature review into sections such as the effects of climate change on habitat loss and species extinction, phenological changes, and marine biodiversity. Each section would then summarize and analyze relevant studies in those areas, highlighting key findings and identifying gaps in the research. The review would conclude by emphasizing the need for further research on specific aspects of the relationship between climate change and biodiversity. The following literature review template provides a glimpse into the recommended literature review structure and content, demonstrating how research findings are organized around specific themes within a broader topic.
Literature Review on Climate Change Impacts on Biodiversity:
Climate change is a global phenomenon with far-reaching consequences, including significant impacts on biodiversity. This literature review synthesizes key findings from various studies:
a. Habitat Loss and Species Extinction:
Climate change-induced alterations in temperature and precipitation patterns contribute to habitat loss, affecting numerous species (Thomas et al., 2004). The review discusses how these changes increase the risk of extinction, particularly for species with specific habitat requirements.
b. Range Shifts and Phenological Changes:
Observations of range shifts and changes in the timing of biological events (phenology) are documented in response to changing climatic conditions (Parmesan & Yohe, 2003). These shifts affect ecosystems and may lead to mismatches between species and their resources.
c. Ocean Acidification and Coral Reefs:
The review explores the impact of climate change on marine biodiversity, emphasizing ocean acidification’s threat to coral reefs (Hoegh-Guldberg et al., 2007). Changes in pH levels negatively affect coral calcification, disrupting the delicate balance of marine ecosystems.
d. Adaptive Strategies and Conservation Efforts:
Recognizing the urgency of the situation, the literature review discusses various adaptive strategies adopted by species and conservation efforts aimed at mitigating the impacts of climate change on biodiversity (Hannah et al., 2007). It emphasizes the importance of interdisciplinary approaches for effective conservation planning.
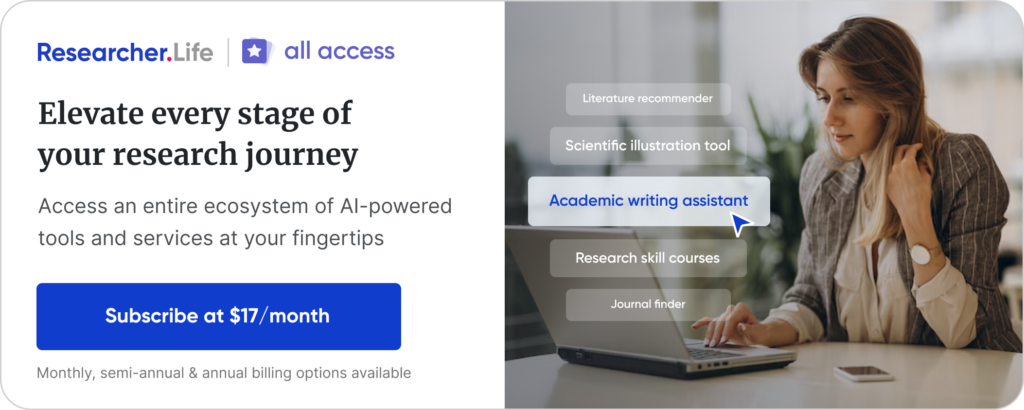
How to write a good literature review
Writing a literature review involves summarizing and synthesizing existing research on a particular topic. A good literature review format should include the following elements.
Introduction: The introduction sets the stage for your literature review, providing context and introducing the main focus of your review.
- Opening Statement: Begin with a general statement about the broader topic and its significance in the field.
- Scope and Purpose: Clearly define the scope of your literature review. Explain the specific research question or objective you aim to address.
- Organizational Framework: Briefly outline the structure of your literature review, indicating how you will categorize and discuss the existing research.
- Significance of the Study: Highlight why your literature review is important and how it contributes to the understanding of the chosen topic.
- Thesis Statement: Conclude the introduction with a concise thesis statement that outlines the main argument or perspective you will develop in the body of the literature review.
Body: The body of the literature review is where you provide a comprehensive analysis of existing literature, grouping studies based on themes, methodologies, or other relevant criteria.
- Organize by Theme or Concept: Group studies that share common themes, concepts, or methodologies. Discuss each theme or concept in detail, summarizing key findings and identifying gaps or areas of disagreement.
- Critical Analysis: Evaluate the strengths and weaknesses of each study. Discuss the methodologies used, the quality of evidence, and the overall contribution of each work to the understanding of the topic.
- Synthesis of Findings: Synthesize the information from different studies to highlight trends, patterns, or areas of consensus in the literature.
- Identification of Gaps: Discuss any gaps or limitations in the existing research and explain how your review contributes to filling these gaps.
- Transition between Sections: Provide smooth transitions between different themes or concepts to maintain the flow of your literature review.
Conclusion: The conclusion of your literature review should summarize the main findings, highlight the contributions of the review, and suggest avenues for future research.
- Summary of Key Findings: Recap the main findings from the literature and restate how they contribute to your research question or objective.
- Contributions to the Field: Discuss the overall contribution of your literature review to the existing knowledge in the field.
- Implications and Applications: Explore the practical implications of the findings and suggest how they might impact future research or practice.
- Recommendations for Future Research: Identify areas that require further investigation and propose potential directions for future research in the field.
- Final Thoughts: Conclude with a final reflection on the importance of your literature review and its relevance to the broader academic community.
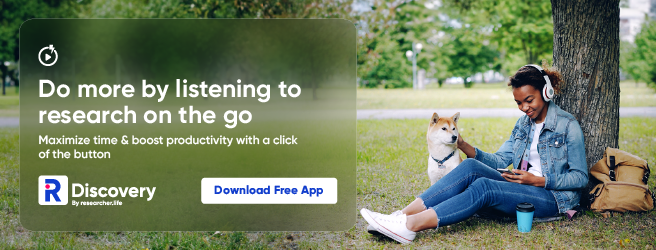
Conducting a literature review
Conducting a literature review is an essential step in research that involves reviewing and analyzing existing literature on a specific topic. It’s important to know how to do a literature review effectively, so here are the steps to follow: 1
Choose a Topic and Define the Research Question:
- Select a topic that is relevant to your field of study.
- Clearly define your research question or objective. Determine what specific aspect of the topic do you want to explore?
Decide on the Scope of Your Review:
- Determine the timeframe for your literature review. Are you focusing on recent developments, or do you want a historical overview?
- Consider the geographical scope. Is your review global, or are you focusing on a specific region?
- Define the inclusion and exclusion criteria. What types of sources will you include? Are there specific types of studies or publications you will exclude?
Select Databases for Searches:
- Identify relevant databases for your field. Examples include PubMed, IEEE Xplore, Scopus, Web of Science, and Google Scholar.
- Consider searching in library catalogs, institutional repositories, and specialized databases related to your topic.
Conduct Searches and Keep Track:
- Develop a systematic search strategy using keywords, Boolean operators (AND, OR, NOT), and other search techniques.
- Record and document your search strategy for transparency and replicability.
- Keep track of the articles, including publication details, abstracts, and links. Use citation management tools like EndNote, Zotero, or Mendeley to organize your references.
Review the Literature:
- Evaluate the relevance and quality of each source. Consider the methodology, sample size, and results of studies.
- Organize the literature by themes or key concepts. Identify patterns, trends, and gaps in the existing research.
- Summarize key findings and arguments from each source. Compare and contrast different perspectives.
- Identify areas where there is a consensus in the literature and where there are conflicting opinions.
- Provide critical analysis and synthesis of the literature. What are the strengths and weaknesses of existing research?
Organize and Write Your Literature Review:
- Literature review outline should be based on themes, chronological order, or methodological approaches.
- Write a clear and coherent narrative that synthesizes the information gathered.
- Use proper citations for each source and ensure consistency in your citation style (APA, MLA, Chicago, etc.).
- Conclude your literature review by summarizing key findings, identifying gaps, and suggesting areas for future research.
The literature review sample and detailed advice on writing and conducting a review will help you produce a well-structured report. But remember that a literature review is an ongoing process, and it may be necessary to revisit and update it as your research progresses.
Frequently asked questions
A literature review is a critical and comprehensive analysis of existing literature (published and unpublished works) on a specific topic or research question and provides a synthesis of the current state of knowledge in a particular field. A well-conducted literature review is crucial for researchers to build upon existing knowledge, avoid duplication of efforts, and contribute to the advancement of their field. It also helps researchers situate their work within a broader context and facilitates the development of a sound theoretical and conceptual framework for their studies.
Literature review is a crucial component of research writing, providing a solid background for a research paper’s investigation. The aim is to keep professionals up to date by providing an understanding of ongoing developments within a specific field, including research methods, and experimental techniques used in that field, and present that knowledge in the form of a written report. Also, the depth and breadth of the literature review emphasizes the credibility of the scholar in his or her field.
Before writing a literature review, it’s essential to undertake several preparatory steps to ensure that your review is well-researched, organized, and focused. This includes choosing a topic of general interest to you and doing exploratory research on that topic, writing an annotated bibliography, and noting major points, especially those that relate to the position you have taken on the topic.
Literature reviews and academic research papers are essential components of scholarly work but serve different purposes within the academic realm. 3 A literature review aims to provide a foundation for understanding the current state of research on a particular topic, identify gaps or controversies, and lay the groundwork for future research. Therefore, it draws heavily from existing academic sources, including books, journal articles, and other scholarly publications. In contrast, an academic research paper aims to present new knowledge, contribute to the academic discourse, and advance the understanding of a specific research question. Therefore, it involves a mix of existing literature (in the introduction and literature review sections) and original data or findings obtained through research methods.
Literature reviews are essential components of academic and research papers, and various strategies can be employed to conduct them effectively. If you want to know how to write a literature review for a research paper, here are four common approaches that are often used by researchers. Chronological Review: This strategy involves organizing the literature based on the chronological order of publication. It helps to trace the development of a topic over time, showing how ideas, theories, and research have evolved. Thematic Review: Thematic reviews focus on identifying and analyzing themes or topics that cut across different studies. Instead of organizing the literature chronologically, it is grouped by key themes or concepts, allowing for a comprehensive exploration of various aspects of the topic. Methodological Review: This strategy involves organizing the literature based on the research methods employed in different studies. It helps to highlight the strengths and weaknesses of various methodologies and allows the reader to evaluate the reliability and validity of the research findings. Theoretical Review: A theoretical review examines the literature based on the theoretical frameworks used in different studies. This approach helps to identify the key theories that have been applied to the topic and assess their contributions to the understanding of the subject. It’s important to note that these strategies are not mutually exclusive, and a literature review may combine elements of more than one approach. The choice of strategy depends on the research question, the nature of the literature available, and the goals of the review. Additionally, other strategies, such as integrative reviews or systematic reviews, may be employed depending on the specific requirements of the research.
The literature review format can vary depending on the specific publication guidelines. However, there are some common elements and structures that are often followed. Here is a general guideline for the format of a literature review: Introduction: Provide an overview of the topic. Define the scope and purpose of the literature review. State the research question or objective. Body: Organize the literature by themes, concepts, or chronology. Critically analyze and evaluate each source. Discuss the strengths and weaknesses of the studies. Highlight any methodological limitations or biases. Identify patterns, connections, or contradictions in the existing research. Conclusion: Summarize the key points discussed in the literature review. Highlight the research gap. Address the research question or objective stated in the introduction. Highlight the contributions of the review and suggest directions for future research.
Both annotated bibliographies and literature reviews involve the examination of scholarly sources. While annotated bibliographies focus on individual sources with brief annotations, literature reviews provide a more in-depth, integrated, and comprehensive analysis of existing literature on a specific topic. The key differences are as follows:
References
- Denney, A. S., & Tewksbury, R. (2013). How to write a literature review. Journal of criminal justice education , 24 (2), 218-234.
- Pan, M. L. (2016). Preparing literature reviews: Qualitative and quantitative approaches . Taylor & Francis.
- Cantero, C. (2019). How to write a literature review. San José State University Writing Center .
Paperpal is an AI writing assistant that help academics write better, faster with real-time suggestions for in-depth language and grammar correction. Trained on millions of research manuscripts enhanced by professional academic editors, Paperpal delivers human precision at machine speed.
Try it for free or upgrade to Paperpal Prime , which unlocks unlimited access to premium features like academic translation, paraphrasing, contextual synonyms, consistency checks and more. It’s like always having a professional academic editor by your side! Go beyond limitations and experience the future of academic writing. Get Paperpal Prime now at just US$19 a month!
Related Reads:
- Empirical Research: A Comprehensive Guide for Academics
- How to Write a Scientific Paper in 10 Steps
- Life Sciences Papers: 9 Tips for Authors Writing in Biological Sciences
- What is an Argumentative Essay? How to Write It (With Examples)
6 Tips for Post-Doc Researchers to Take Their Career to the Next Level
Self-plagiarism in research: what it is and how to avoid it, you may also like, how paperpal’s research feature helps you develop and..., how paperpal is enhancing academic productivity and accelerating..., how to write a successful book chapter for..., academic editing: how to self-edit academic text with..., 4 ways paperpal encourages responsible writing with ai, what are scholarly sources and where can you..., how to write a hypothesis types and examples , measuring academic success: definition & strategies for excellence, what is academic writing: tips for students, why traditional editorial process needs an upgrade.


How to write a Literature Review: Literature review process
- Literature review process
- Purpose of a literature review
- Evaluating sources
- Managing sources
- Request a literature search
- Selecting the approach to use
- Quantitative vs qualitative method
- Summary of different research methodologies
- Research design vs research methodology
- Diagram: importance of research
- Attributes of a good research scholar
Step 1: Select a topic
- Select a topic you can manage in the time frame you have to complete your project.
- Establish your research questions and organize your literature into logical categories around the subject/ topic areas of your questions. Your research questions must be specific enou gh to guide you to the relevant literature.
- Make sure you understand the concept of ‘broader’ and ‘narrower’ terms. The narrower your topic, the easier it will be to limit the number of sources you need to read in order to get a good survey of the literature.
Step 2: Identify the most relevant sources on your topic
Use a variety of resources - locate books , journals , and documents that contain useful information and ideas on your topic. Internet sites , theses & dissertations , conference papers , ePrints and government or industry reports can also be included. Do not rely solely on electronic full-text material which is more easily available. Reference sources such as dictionaries can assist in defining terminology, and encyclopaedias may provide useful introductions to your topic by experts in the field and will list key references.
Step 3 : Search and refine
- Unisa has a number of databases that provide full text access to articles, that allow you to refine your search to ‘peer reviewed’ journals. These are scholarly journals which go through a rigorous process of quality assessment by several researchers or subject specialists in the academic community before they are accepted for publication.
- Use the And, Or, Not operators, Wildcards and Logical Brackets when searching in the databases. For instance, you can use And to narrow your search while the operator OR expands your search. Not, on the other hand, helps to exclude irrelevant information from your search results. Please click here for more information on searching.
Literature review process - an overview
Step 3: search and refine.
- Unisa has a number of databases that provide full text access to articles, that allow you to refine your search to ‘peer reviewed’ journals. These are scholarly journals which go through a rigorous process of quality assessment by several researchers or subject specialists in the academic community before they are accepted for publication.
- Use the And, Or, Not operators, Wildcards and Logical Brackets when searching in the databases. For instance, you can use And to narrow your search while the operator OR expands your search. Not, on the other hand, helps to exclude irrelevant information from your search results. Please click here for more information on searching.
How do I write a literature review
See the chapter below for a helpful overview of the literature review process, especially the sections on how to analyse the literature you have gathered and how to write up your literature review:
Literature Reviews and Bibliographic Searches. 2006. In V. Desai, & R. Potter (Eds.), Doing Development Research. (pp. 209-222). London, England: SAGE Publications, Ltd. Available at: http://0-dx.doi.org.oasis.unisa.ac.za/10.4135/9781849208925.n22 (A student will be prompted at some stage for his/ her student number and myUnisa password. A staff member will be prompted at some stage for his/ her Unisa Network username and login password).
This book is available in the Sage Research Methods Online database.
Step 4: Read and analyse
Group the sources into the themes and sub-themes of your topic. As you read widely but selectively in your topic area, consider what themes or issues connect your sources together.
- Do they present one or different solutions?
- Is there an aspect of the field that is missing?
- How well do they present the material and do they portray it according to an appropriate theory?
- Do they reveal a trend in the field?
- A raging debate?
- Pick one of these themes to focus the organization of your review.
Step 5: Write the literature review
You can organize the review in many ways; for example, you can center the review historically (how the topic has been dealt with over time); or center it on the theoretical positions surrounding your topic (those for a position vs. those against, for example); or you can focus on how each of your sources contributes to your understanding of your project.
Your literature review should include:
- an introduction which explains how your review is organized.
- a body which contains the headings and subheadings that provide a map to show the various perspectives of your argument. In other words the body contains the evaluation of the materials you want to include on your topic.
- a summary .
Some of the information on this page is indebted to the sources below:
Caldwell College Library
Monmouth University Library
University of Cape Town Libraries
- << Previous: Home
- Next: Purpose of a literature review >>
- Last Updated: Apr 30, 2024 1:19 PM
- URL: https://libguides.unisa.ac.za/literature_review
- Library databases
- Library website
Library Guide to Capstone Literature Reviews: Role of the Literature Review
The role of the literature review.
Your literature review gives readers an understanding of the scholarly research on your topic.
In your literature review you will:
- demonstrate that you are a well-informed scholar with expertise and knowledge in the field by giving an overview of the current state of the literature
- find a gap in the literature, or address a business or professional issue, depending on your doctoral study program; the literature review will illustrate how your research contributes to the scholarly conversation
- provide a synthesis of the issues, trends, and concepts surrounding your research

Be aware that the literature review is an iterative process. As you read and write initial drafts, you will find new threads and complementary themes, at which point you will return to search, find out about these new themes, and incorporate them into your review.
The purpose of this guide is to help you through the literature review process. Take some time to look over the resources in order to become familiar with them. The tabs on the left side of this page have additional information.
Short video: Research for the Literature Review
Short Video: Research for the Literature Review
(4 min 10 sec) Recorded August 2019 Transcript
Literature review as a dinner party
To think about the role of the literature review, consider this analogy: pretend that you throw a dinner party for the other researchers working in your topic area. First, you’d need to develop a guest list.
- The guests of honor would be early researchers or theorists; their work likely inspired subsequent studies, ideas, or controversies that the current researchers pursue.
- Then, think about the important current researchers to invite. Which guests might agree with each other? Which others might provide useful counterpoints?
- You likely won’t be able to include everyone on the guest list, so you may need to choose carefully so that you don’t leave important figures out.
- Alternatively, if there aren’t many researchers working in your topic area, then your guest list will need to include people working in other, related areas, who can still contribute to the conversation.
After the party, you describe the evening to a friend. You’ll summarize the evening’s conversation. Perhaps one guest made a comment that sparked a conversation, and then you describe who responded and how the topic evolved. There are other conversations to share, too. This is how you synthesize the themes and developments that you find in your research. Thinking about your literature research this way will help you to present your dinner party (and your literature review) in a lively and engaging way.
Short video: Empirical research
Video: How to locate and identify empirical research for your literature review
(6 min 16 sec) Recorded May 2020 Transcript
Here are some useful resources from the Writing Center, the Office of Research and Doctoral Services, and other departments within the Office of Academic Support. Take some time to look at what is available to help you with your capstone/dissertation.
- Familiarize yourself with Walden support
- Doctoral Capstone Resources website
- Capstone writing resources
- Office of Student Research Administration
- Office of Research and Doctoral Services
- Visit the Writing Center
You can watch recorded webinars on the literature review in our Library Webinar Archives .
- Next Page: Scope
- Office of Student Disability Services
Walden Resources
Departments.
- Academic Residencies
- Academic Skills
- Career Planning and Development
- Customer Care Team
- Field Experience
- Military Services
- Student Success Advising
- Writing Skills
Centers and Offices
- Center for Social Change
- Office of Academic Support and Instructional Services
- Office of Degree Acceleration
- Office of Student Affairs
Student Resources
- Doctoral Writing Assessment
- Form & Style Review
- Quick Answers
- ScholarWorks
- SKIL Courses and Workshops
- Walden Bookstore
- Walden Catalog & Student Handbook
- Student Safety/Title IX
- Legal & Consumer Information
- Website Terms and Conditions
- Cookie Policy
- Accessibility
- Accreditation
- State Authorization
- Net Price Calculator
- Contact Walden
Walden University is a member of Adtalem Global Education, Inc. www.adtalem.com Walden University is certified to operate by SCHEV © 2024 Walden University LLC. All rights reserved.
This paper is in the following e-collection/theme issue:
Published on 13.5.2024 in Vol 26 (2024)
Suitability of the Current Health Technology Assessment of Innovative Artificial Intelligence-Based Medical Devices: Scoping Literature Review
Authors of this article:

- Line Farah 1, 2 * , PharmD ;
- Isabelle Borget 2, 3, 4 , PharmB, PhD ;
- Nicolas Martelli 2, 5 , PharmD, PhD ;
- Alexandre Vallee 6 * , MD, PhD
1 Innovation Center for Medical Devices Department, Foch Hospital, Suresnes, France
2 Groupe de Recherche et d'accueil en Droit et Economie de la Santé Department, University Paris-Saclay, Orsay, France
3 Department of Biostatistics and Epidemiology, Gustave Roussy, University Paris-Saclay, Villejuif, France
4 Oncostat U1018, Inserm, Équipe Labellisée Ligue Contre le Cancer, University Paris-Saclay, Villejuif, France
5 Pharmacy Department, Georges Pompidou European Hospital, Paris, France
6 Department of Epidemiology and Public Health, Foch Hospital, Suresnes, France
*these authors contributed equally
Corresponding Author:
Line Farah, PharmD
Innovation Center for Medical Devices Department
Foch Hospital
40 rue Worth
Suresnes, 92150
Phone: 33 952329655
Email: [email protected]
Background: Artificial intelligence (AI)–based medical devices have garnered attention due to their ability to revolutionize medicine. Their health technology assessment framework is lacking.
Objective: This study aims to analyze the suitability of each health technology assessment (HTA) domain for the assessment of AI-based medical devices.
Methods: We conducted a scoping literature review following the PRISMA (Preferred Reporting Items for Systematic Reviews and Meta-Analyses) methodology. We searched databases (PubMed, Embase, and Cochrane Library), gray literature, and HTA agency websites.
Results: A total of 10.1% (78/775) of the references were included. Data quality and integration are vital aspects to consider when describing and assessing the technical characteristics of AI-based medical devices during an HTA process. When it comes to implementing specialized HTA for AI-based medical devices, several practical challenges and potential barriers could be highlighted and should be taken into account (AI technological evolution timeline, data requirements, complexity and transparency, clinical validation and safety requirements, regulatory and ethical considerations, and economic evaluation).
Conclusions: The adaptation of the HTA process through a methodological framework for AI-based medical devices enhances the comparability of results across different evaluations and jurisdictions. By defining the necessary expertise, the framework supports the development of a skilled workforce capable of conducting robust and reliable HTAs of AI-based medical devices. A comprehensive adapted HTA framework for AI-based medical devices can provide valuable insights into the effectiveness, cost-effectiveness, and societal impact of AI-based medical devices, guiding their responsible implementation and maximizing their benefits for patients and health care systems.
Introduction
Artificial intelligence (AI) has emerged as a transformative technology with vast potential across various sectors, including health care [ 1 , 2 ]. In this field, AI-based medical devices have garnered significant attention due to their ability to revolutionize diagnosis, treatment, and patient monitoring [ 3 ]. These devices use advanced algorithms and machine learning techniques to analyze complex medical data sets, thereby providing valuable insights and support to health care professionals in their decision-making. To meet the ever-increasing demand for integration of AI-based medical devices into clinical practice, their efficient evaluation through adapted health technology assessment (HTA) is now crucial [ 4 ]. Several frameworks have been published showing an adaptation of items for reporting clinical trials related to AI-based medical devices ( Table 1 ). However, there is still a lack of a much needed adaptation of the standard HTA framework to better suit the assessment of AI-based health care technologies.
a HTA: health technology assessment.
b CONSORT-AI: Consolidated Standards of Reporting Trials–Artificial Intelligence.
c CONSORT: Consolidated Standards of Reporting Trials.
d SPIRIT-AI: Standard Protocol Items: Recommendations for Interventional Trials–Artificial Intelligence.
e SPIRIT: Standard Protocol Items: Recommendations for Interventional Trials.
f TRIPOD-AI: Transparent Reporting of a Multivariable Prediction Model for Individual Prognosis or Diagnosis-Artificial Intelligence.
g TRIPOD: Transparent Reporting of a Multivariable Prediction Model for Individual Prognosis or Diagnosis.
An HTA involves a systematic evaluation of a medical technology’s clinical, economic, ethical, and social implications to determine its overall value and impact on health care delivery [ 9 ]. The European Network for Health Technology Assessment has designed an HTA Core Model that provides a methodological framework for production and sharing of HTA information [ 5 ]. It evaluates the following nine domains: (1) health problem and current use of technology, (2) description and technical characteristics of the technology, (3) safety, (4) clinical effectiveness, (5) costs and economic evaluation, (6) ethical analysis, (7) organizational aspects, (8) patients and social aspects, and (9) legal aspects ( Multimedia Appendix 1 ).
A full understanding of the capabilities, limitations, and specificities of AI-based medical devices is paramount to complete these HTA domain assessments and, thereby, inform evidence-based decision-making and allow for policy development and the responsible integration of these technologies into health care systems [ 10 , 11 ]. Much uncertainty remains with regard to the reliability of AI-based medical devices, data issues, and regulatory processes, resulting in multiple challenges faced by HTA agencies assessing new technologies and delivering their approval [ 4 ]. Nevertheless, AI-based medical devices require strict regulations and specific legislations [ 12 - 14 ]. Over the last few decades, the evaluation of AI-based medical devices through an HTA process has received growing interest, as shown by the increase from 1 published article in 1990 to 94 in December 2023, with 484 articles in total during the period 1990-2023 ( Table 2 ).
The objective of this review was to critically assess the comprehensive suitability of the current HTA process for AI-based medical devices. By evaluating the performance and capabilities of AI-based medical devices across multiple dimensions, this review aimed to inform health care professionals, policy makers, and researchers about the challenges and opportunities associated with these technologies. Ultimately, this review sought to facilitate evidence-based decision-making; promote responsible implementation; and maximize the potential benefits of AI-based medical devices in improving health care quality, accessibility, and outcomes.
To this end, we analyzed the suitability of each HTA domain for the assessment of AI-based medical devices and proposed an adapted HTA framework.
Search Strategies
A scoping literature review was conducted following the PRISMA (Preferred Reporting Items for Systematic Reviews and Meta-Analyses) methodology [ 15 , 16 ]. All articles related to HTA methods for AI-based medical devices were selected. Data extraction focused on assessment criteria, methodological evaluations, and results.
The search terms and strategy outlined in Textbox 1 were used for the scoping review.
Database and search strategy
- “technology assessment, biomedical” [MeSH term] OR (“technology” [all fields] AND “assessment” [all fields] AND “biomedical” [all fields]) OR “biomedical technology assessment” [all fields] OR (“health” [all fields] AND “technology” [all fields] AND “assessment” [all fields]) OR “health technology assessment” [all fields]
- “equipment and supplies” [MeSH term] OR (“equipment” [all fields] AND “supplies” [all fields]) OR “equipment and supplies” [all fields] OR (“medical” [all fields] AND “device” [all fields]) OR “medical device” [all fields]
- “artificial intelligence” [MeSH term] OR (“artificial” [all fields] AND “intelligence” [all fields]) OR “artificial intelligence” [all fields]
- (“artificial intelligence” OR “artificial intelligence-based medical device”) AND “technology assessment”
- (technology assessment) and (artificial intelligence)
We searched multiple databases: PubMed, Embase, and the Cochrane Library. Additional articles were retrieved manually from the gray literature and from HTA agency websites (Bundesinstitut für Arzneimittel und Medizinprodukte [Federal Institute for Drugs and Medical Devices in Germany], Haute Autorité de Santé [French Health Authority], National Health Service, National Institute for Health and Care Excellence, National Institute of Public Health, International Network of Agencies for Health Technology Assessment, and the European Network for Health Technology Assessment).
Study Selection Process
The studies were selected by 2 reviewers (LF and AV). After removing duplicates, both reviewers independently screened the abstracts to select eligible articles and then analyzed full-text reports for eligibility. A third party (NM) resolved the possible discrepancies highlighted during the selection process should a consensus not be reached. An extraction database was used to list the selected articles meeting the inclusion criteria to ensure that all eligible articles were included.
The inclusion and exclusion criteria were related to (1) language (this review was limited to the English and French languages), (2) article type (reviews and primary research were included, whereas other article types were excluded, such as abstracts, commentaries, editorials, letters to the editor, case reports, case series, animal studies, phase-1 and phase-2 studies, pilot studies, duplicate studies, irrelevant studies, studies with the wrong aim, and studies available in abstract form only), (3) type of technology (only AI-based medical devices were eligible), and (4) type of evaluation (HTA articles were included).
Data Extraction
In total, 2 analysts (LF and AV) independently extracted data items from the selected articles. A third party was involved to resolve any discrepancies highlighted during the selection process. The following data were extracted from each article: (1) general characteristics of the studies (authors, journal, and publication date), (2) study objective, and (3) HTA assessment domain for AI-based medical devices related to the article.
Methodological Quality Appraisal
Neither the methodological quality nor the risk of bias of the included articles were assessed, consistent with scoping review guidance [ 15 ].
Application Example of the HTA of an AI-Based Medical Device
Concerning each HTA domain, we added a case study of the HTA of several AI-based medical devices in diabetes to illustrate our recommendations.
Scoping Review Results
The literature search resulted in 775 citations summarized in the PRISMA flowchart ( Figure 1 ). After removal of 32.6% (253/775) of duplicates, we carried out an initial screening of the remaining 522 publications, resulting in 129 (24.7%) potentially relevant full-text papers. After further screening, we assessed 77 reports for eligibility, based on which we excluded 11 (14%) for not referring to AI-based medical devices and 2 (3%) for not being HTAs. We then included a further 14 records from 59 potential citations available from HTA agency websites and gray literature.

This gave a total of 78 included articles covering one or multiple HTA domains, the distribution of which is provided at the bottom of Figure 1 .
We then summarized the data collection for each selected article in Multimedia Appendix 2 [ 1 , 2 , 4 , 9 , 10 , 17 - 89 ].
Suitability of Each HTA Domain for the Assessment of AI-Based Medical Devices
As domain 1, health problem and current use of technology, is suitable for any type of medical technology and systematically addressed these technologies, we focused on the suitability of the HTA domains (from 1 to 9).
Domains 1 and 2 (Health Problem, Current Use, Description, and Technical Characteristics of the Technology)
Data quality and integration are vital aspects to consider when describing and assessing the technical characteristics of AI-based medical devices during an HTA process [ 17 ]. These devices often rely on accessing and analyzing diverse health care data sources, including electronic health records, medical images, genetic data, and wearable device data. Therefore, it is essential to evaluate their ability to seamlessly integrate and exchange data with existing health care systems [ 18 ].
First, interoperability refers to the ability of AI-based medical devices to interact and communicate with other health care technologies and systems [ 10 ]. This includes the ability to access and use data from different sources, such as laboratory systems, imaging archives, and patient health records. The assessment should consider whether the devices adhere to relevant data standards and protocols, ensuring efficient data exchange and compatibility with existing health care infrastructures [ 19 ].
Second, data integration involves the ability of AI-based medical devices to aggregate and analyze data from multiple sources to provide comprehensive and accurate insights [ 20 ]. The HTA should assess whether the devices can handle different data types, formats, and resolutions and whether they can effectively integrate and harmonize data from disparate sources.
In addition, data privacy and security considerations are crucial when evaluating AI-based medical devices [ 21 ]. The assessment should examine whether the devices comply with relevant data protection regulations, use appropriate data anonymization and encryption techniques, and have robust security measures in place to protect patient information [ 22 ].
Therefore, a comprehensive HTA should address both the interoperability and data integration capabilities of AI-based medical devices, ensuring that they can seamlessly interact with existing health care systems and integrate data from multiple sources and that they adhere to data privacy and security standards. By evaluating all these aspects, the HTA could determine the devices’ feasibility, scalability, and potential impact on health care delivery.
Domain 3 (Safety) and Domain 4 (Clinical Effectiveness)
The assessment of clinical effectiveness and the impact on patient outcomes is a crucial aspect when evaluating AI-based medical devices through a comprehensive HTA [ 4 ]. While accuracy and performance metrics are important, it is essential to determine how these devices translate into tangible benefits for patients and health care delivery [ 23 ].
Clinical utility refers to the extent to which the AI-based medical device improves clinical decision-making, patient outcomes, and health care processes [ 24 ]. The HTA should examine whether the device provides actionable and reliable information that potentially helps health care professionals make more accurate diagnoses or improve treatment plans or monitoring strategies [ 25 , 26 ]. It should also evaluate the potential impact of the device on patient outcomes by assessing, for example, improved survival rates, reduced complications, or enhanced quality of life [ 27 ].
To assess clinical utility, the HTA should consider the device’s performance in relevant clinical scenarios and its ability to address specific clinical questions or challenges. This may involve evaluating the device’s performance against established clinical guidelines or expert opinions as well as considering the device’s potential to fill gaps in clinical practice or enhance existing diagnostic or treatment methods.
Furthermore, the HTA should examine the broader impact of AI-based medical devices on health care systems and resource allocation [ 21 ]. This includes evaluating the devices’ potential to optimize resource use, reduce health care costs, or improve workflow efficiency. Economic evaluations such as cost-effectiveness analyses can provide insights into the value for money and long-term cost savings associated with the adoption of these devices [ 28 , 29 ].
A comprehensive HTA should thoroughly assess the clinical effectiveness and impact on patient outcomes of AI-based medical devices, examining their performance in relevant clinical scenarios, their alignment with clinical guidelines, and their potential to improve health care processes and resource allocation. By evaluating these aspects, the HTA can provide a holistic understanding of the AI devices’ effectiveness and their potential to positively transform health care delivery [ 21 ].
AI-based medical devices must undergo rigorous clinical validation to assess their performance and reliability in real-world health care settings [ 30 , 31 ]. Clinical validation involves evaluating the device’s accuracy, sensitivity, specificity, and overall diagnostic or prognostic performance. In addition, the devices should be tested across diverse patient populations and compared against gold-standard reference methods or expert opinions [ 32 ].
Furthermore, the use of real-world evidence (RWE) is crucial for a comprehensive HTA [ 33 ]. RWE involves gathering data from routine clinical practice, electronic health records, and other sources to evaluate the device’s effectiveness and safety in real-world settings [ 34 ]. These data can help assess the device’s performance in a broader patient population and identify any potential limitations or biases that may arise in specific clinical scenarios.
Robust clinical validation studies and the integration of RWE provide critical evidence for the evaluation of AI-based medical devices in an HTA process [ 4 ]. These studies should include a sufficient sample size, appropriate study design, and statistical analysis to ensure the validity and generalizability of the results [ 35 - 38 ]. By considering clinical validation and RWE adapted to AI-based medical devices, HTA can provide valuable insights into their clinical utility and impact in real-world health care settings, facilitating evidence-based decision-making for their adoption and use.
A lack of safety evaluation was highlighted in a systematic review, showing that only 9% of AI-based medical device studies evaluated safety criteria [ 4 ]. However, safety is crucial for the confidence in and adoption of AI-based technologies for both patients and health care professionals [ 39 ]. While these devices have the potential to improve diagnosis and treatment outcomes, their integration into clinical practice must be accompanied by robust safety evaluations [ 2 , 40 ]. Identifying and mitigating potential risks, such as algorithmic bias, data privacy breaches, and algorithm failures, is essential to protect patient well-being and maintain trust in these innovative technologies [ 41 ].
To ensure the safety of AI-based medical devices, it is crucial to establish standardized evaluation frameworks and guidelines [ 39 ]. These frameworks should encompass rigorous testing methodologies, validation procedures, and continuous monitoring of AI performance. Collaboration among stakeholders, including manufacturers, regulatory agencies, health care providers, and researchers, is essential to develop and implement comprehensive safety evaluation protocols. Addressing the safety gap in AI-based medical devices not only ensures patient welfare but also instills confidence in health care professionals to embrace and use these technologies effectively [ 40 ]. By prioritizing safety evaluation, we can unleash the full potential of AI-based medical devices, leading to transformative advancements in health care delivery [ 1 ].
Domain 5 (Costs and Economic Evaluation)
Costs and economic evaluation play a crucial role in the comprehensive assessment of health technology adoption, particularly in the context of the HTA of AI-based medical devices [ 4 , 9 , 42 ]. While AI technologies show huge potential to improve health care delivery, reduce time to diagnosis, and save money, their economic impact requires careful consideration to ensure their successful integration and sustainability [ 23 ].
When assessing AI-based medical devices through the HTA process, economic evaluations involve analyzing the costs and benefits associated with the adoption and use of the technology [ 4 ]. These evaluations go beyond the upfront costs of acquiring the AI-based medical device; they encompass various factors, such as training, infrastructure modifications, maintenance, and ongoing operational costs [ 43 ]. Cost analysis also includes improved patient outcomes, reduced hospital readmissions, and shortened hospital stays. By conducting economic evaluations, decision makers can gauge the cost-effectiveness and cost utility to weigh the affordability of AI-based medical devices for their particular use against the potential mid- and long-term cost savings considered [ 44 ].
HTA evaluates the suitability of AI-based medical devices by considering their potential economic benefits, risks, and ethical implications [ 45 , 46 ]. By incorporating economic evaluation into the HTA process, decision makers can make evidence-based choices about the allocation of limited health care resources and prioritize interventions that provide the greatest value for money [ 4 , 47 ].
Finally, considering cost savings and conducting economic evaluations as part of the HTA process helps facilitate the adoption of AI-based medical devices [ 37 ]. It provides decision makers with the necessary information to determine the financial feasibility and potential return on investment associated with implementing these technologies. HTA ensures that AI-based medical devices are suitable for integration into the health care system, fostering confidence in their effectiveness, efficiency, and cost utility [ 4 , 43 , 48 ].
Domain 6 (Ethical Analysis) and Domain 8 (Patients and Social Aspects)
Ethical and societal implications are critical aspects that must be considered when evaluating the suitability of AI-based medical devices for a comprehensive HTA [ 45 ]. The integration of AI technologies into health care raises important ethical concerns that need to be addressed to ensure responsible and equitable use [ 49 ].
Data privacy and patient consent are primary ethical considerations [ 50 ]. The HTA should evaluate whether AI-based medical devices adhere to strict data protection regulations, maintain patient confidentiality, and obtain appropriate informed consent for data use. It is essential to assess whether the devices have mechanisms in place to handle sensitive patient information securely and protect it against unauthorized access or data breaches.
Algorithmic fairness and bias are additional ethical concerns [ 51 ]. AI algorithms can inadvertently perpetuate biases present in the data used for training, resulting in unequal treatment or access to health care resources [ 52 ]. The HTA should assess whether the devices have been evaluated for fairness and bias and consider the steps taken to mitigate any identified biases [ 53 ].
Moreover, the HTA should examine the impact of AI-based medical devices on health care disparities and access to care [ 54 ]. It is crucial to assess whether the devices have the potential to exacerbate existing inequalities or whether they can contribute to reducing disparities by improving health care access, particularly for underserved populations.
Accountability and transparency in AI decision-making processes are also important ethical considerations [ 55 ]. The HTA should evaluate whether the AI devices provide clear explanations for their outputs and ensure that health care professionals and patients can understand and challenge the device’s recommendations [ 21 ].
A comprehensive HTA should thoroughly examine the ethical and societal implications of AI-based medical devices, ensuring that they prioritize patient privacy, fairness, and equitable access to care; fostering trust; and ensuring that these devices align with societal values and goals.
To ensure the adoption of AI-based medical devices through a truthfully AI concept, explainability, interpretability, and transparency are important considerations for their comprehensive HTA [ 56 ]. Indeed, these devices often use complex algorithms and machine and deep learning techniques, resulting in their operating as “black boxes” that reach decisions and recommendations that are challenging to understand [ 55 ]. However, explainability and transparency are essential to ensure trust, accountability, and acceptance of AI-based technologies in health care. First, Explainability refers to the ability to understand and interpret the reasoning behind the device’s outputs [ 56 ]. It involves providing clinicians and users with transparent explanations of how the AI algorithm processes input data and generates results. This enables health care professionals to trust the device’s recommendations and make informed decisions based on the provided information. The HTA should evaluate the extent to which AI-based medical devices can provide interpretable and understandable explanations of their decision-making processes [ 17 , 46 ]. Second, Transparency involves disclosing important information about the AI-based medical devices, including the data used for training, algorithmic methodologies used, and potential limitations or biases [ 57 ]. Transparency promotes trust and allows stakeholders to assess the device’s reliability and potential risks. The HTA should assess whether the device manufacturers provide clear documentation and information to health care professionals and patients about the device’s capabilities, limitations, and potential errors [ 21 , 58 , 59 ]. Transparency is closely linked to regulatory considerations [ 60 , 61 ]. The HTA should consider whether the device complies with relevant regulatory standards and whether the manufacturers have provided the necessary documentation and evidence to support their ethical claims [ 21 , 62 , 63 ].
A comprehensive HTA process should address concerns regarding the explainability and transparency of AI-based medical devices, trust, accountability, and ethical implications related to the use of AI in health care [ 45 , 46 ].
Domain 7 (Organizational Aspects)
Organizational aspects play a crucial role in the effective integration of AI-based medical devices into health care systems [ 64 ]. To ensure consistency, transparency, and comparability in the evaluation process, there is a growing need for a robust methodological framework that provides standardized guidelines for assessing the organizational impact of the implementation of AI-based medical devices [ 65 ]. According to a descriptive analysis led in German hospitals, the main barriers to AI-based medical device adoption were lack of resources (staff, knowledge, and financial) [ 66 ].
Clear indicators are needed to measure the organizational readiness for and impact of AI-based medical devices [ 4 , 10 , 67 ]. Several criteria have been highlighted, such as (1) health care workplace readiness and stakeholder acceptance, (2) AI-based medical device organization alignment assessment, and (3) business plan (financing and investments) [ 64 ].
Domain 9 (Legal Aspects)
The roles and responsibilities of health care professionals are also impacted by these AI solutions [ 68 ]. It is necessary to evaluate whether they complement or replace health care professionals’ expertise and whether additional training, supervision, or support is required for their optimal use [ 69 , 70 ]. As AI technologies could evolve and become more prevalent in health care, it is crucial to ensure that these devices comply with existing legal frameworks and regulations [ 49 , 71 ]. HTA plays a pivotal role in evaluating the legal implications of AI-based medical devices by assessing factors such as data privacy, security, liability, and regulatory compliance [ 72 ].
One key legal aspect to consider is data privacy and protection [ 73 , 74 ]. AI-based medical devices often rely on vast amounts of patient data for training and decision-making. Therefore, it is essential to evaluate whether these devices adhere to relevant data protection laws, such as the General Data Protection Regulation in the European Union [ 75 , 76 ]. HTA examines the measures taken by AI-based medical device manufacturers to safeguard patient privacy, including data anonymization, encryption, and secure data storage practices [ 37 ]. These issues are highlighted in the Artificial Intelligence Act published in June 2023 by the European Commission [ 77 , 78 ].
Cybersecurity is another critical consideration [ 21 , 79 ]. As AI-based medical devices handle sensitive patient data and make critical health care decisions, it is crucial to assess the security measures implemented to prevent unauthorized access, data breaches, or tampering [ 80 ]. HTA evaluates the robustness of the security protocols implemented by device manufacturers and their compliance with industry standards and regulations [ 21 , 81 ].
Liability is also a significant legal aspect to be addressed in the context of AI-based medical devices [ 82 , 83 ]. When errors or adverse events occur due to the use of these devices, determining liability can be complex [ 84 ]. HTA examines the legal frameworks and liability guidelines pertaining to AI technologies, including whether clear guidelines exist regarding the responsibility of manufacturers, health care providers, and users in case of device malfunctions or errors [ 85 ]. Assessing liability aspects within the HTA process helps establish accountability and ensures that legal frameworks adequately address potential risks [ 86 ].
Regulatory compliance is a crucial consideration when assessing the suitability of AI-based medical devices for HTA [ 87 ]. Depending on the jurisdiction, AI devices need to undergo regulatory approval processes before being introduced into the market [ 88 ]. HTA examines whether AI-based medical device manufacturers have obtained the necessary regulatory approvals, such as clearance from the relevant health authorities or certification from regulatory bodies such as the Food and Drug Administration (FDA) in the United States or notified bodies in Europe [ 89 ]. This evaluation ensures that AI-based medical devices comply with existing regulations and are fit for clinical use [ 61 ].
Recommendations to Adapt the 9 HTA Domains for AI-Based Medical Devices
Taking into account the previous considerations, some recommendations could be proposed to adapt and personalize these standard HTA domains to AI-based medical devices’ specificities. Therefore, we suggest 4 main recommendations by domain in Table 3 .
a ML: machine learning.
b DL: deep learning.
c DMS: diabetes management system.
d FDA: Food and Drug Administration.
The adaptation of the HTA process through a methodological framework for AI-based medical devices enhances the comparability of results across different evaluations and jurisdictions [ 90 - 92 ]. It promotes consistency in the assessment methodologies, reporting formats, and presentation of findings, enabling decision makers to make informed choices based on reliable and comparable evidence. This standardization contributes to the overall credibility and acceptance of HTA outcomes related to AI-based medical devices [ 93 ]. In addition, a methodological framework would address the need for appropriate expertise and skills to conduct the HTA of AI-based medical devices [ 94 - 99 ]. It would outline the qualifications and competencies required for the individuals involved in the assessment, including knowledge of AI technologies [ 46 ]. By defining the necessary expertise, the framework supports the development of a skilled workforce capable of conducting robust and reliable HTAs of AI-based medical devices ( Figure 2 ).

Evaluating the trade-offs and weighing different features of AI-based medical devices is indeed a complex and important task, especially in domains such as health care where the impact on human lives is significant. The acceptability of AI-based medical devices should be assessed on a case-by-case basis considering various factors, including, for instance, performance, accuracy, cost, explainability, and the specific context in which they are being used.
Trade-offs between accuracy and cost are common in AI. It may be acceptable for AI to increase follow-up care costs if it significantly improves accuracy and patient outcomes. For example, if an AI system can detect diseases at an earlier stage, it might lead to more effective treatment and, ultimately, lower overall health care costs in the long run.
The balance between explainability and performance is a critical consideration. While explainable algorithms are preferred for safety and transparency reasons, there may be cases in which a highly complex, unexplainable algorithm outperforms explainable ones. In such cases, the trade-off between transparency and performance should be carefully evaluated based on the specific use case and potential risks.
The minimum performance for adding this technology to the tools available to human clinicians depends on the specific task and the level of trust that patients, health care providers, and regulators have in the technology. Some key factors to consider include the following: (1) The complexity of the task—AI-based medical devices should excel in tasks that are well defined and data driven, but they may not replace human clinicians in tasks requiring complex decision-making, empathy, or ethical considerations. (2) Safety and reliability—AI-based medical devices should demonstrate a high level of safety and reliability, ideally surpassing the performance of human clinicians in terms of avoiding errors. (3) Ethical considerations—AI-based medical devices should adhere to ethical standards, including patient privacy, informed consent, and unbiased decision-making, which are often considered even more important than performance metrics. (4) Regulatory approval—regulatory bodies often establish performance thresholds for AI-based medical devices. Compliance with these thresholds is essential for market acceptance.
In general, AI should aim to complement and enhance the capabilities of human clinicians rather than completely replacing them. The specific threshold for acceptable performance will vary across applications and contexts, and it should be determined through a combination of rigorous testing, peer-reviewed studies, and input from health care professionals and patients.
It is important to note that ethical considerations, patient safety, and the potential for bias should always be at the forefront of these discussions, and AI-based medical devices should not be adopted solely for the sake of automation or cost reduction if they compromise these critical aspects of health care.
Use Case of the Application of the Aforementioned HTA Recommendations for AI: AI-Based Medical Devices in Pathways for Patients With Diabetes
Concerning the HTA domain 3 on the safety of an AI-based medical device, the potential risk of patient injury of an insulin delivery AI-based medical device system should be taken into account in the risk management process of algorithm development [ 96 ]. In the case of an evolutive deep learning–based medical device without continuous ongoing safety assessment, a wrong dosage administration due to an AI error, for instance, could provoke a serious adverse event such as an acid ketosis coma for a patient with diabetes.
A risk management plan should be available for users and regularly updated with the impact of the AI changes on the safety plan.
In relation to the HTA domain 4, which focuses on the effectiveness of an AI-based medical device, the FDA in the United States has proposed a regulatory framework for modifications to evolutive AI- and machine learning–based software as a medical device with modification guidance focused on the risk to users or patients resulting from the AI changes [ 100 ]. They have asked for an Algorithm Change Protocol with specific methods in place to achieve and control the risks of the anticipated types of modifications related to performance, use, or inputs. A continuous clinical effectiveness assessment could be an interesting approach for an AI-based medical device detection system for diabetes foot ulcer for patients needing adaptative treatment modifications to prevent ulcer development [ 101 ].
Concerning the HTA domain 5, to evaluate a diabetic retinopathy screening AI-based medical device, clinical effectiveness is not sufficient. The cost-effectiveness of different types of AI diabetic retinopathy screening should be compared with no screening and ophthalmologist screening. A recent study demonstrated that AI-based screening was the most cost-effective, not only saving costs but also improving the quality of life of patients with diabetes [ 44 ]. In this case, the long-term assessment of the economic impact of AI introduction in diabetic retinopathy screening highlighted the added value of this technology.
The HTA domain 7 on organizational impact has to assess how the AI-based medical device can be effectively integrated into the health care pathway and prevent wasteful spending. More thorough attention must be paid to the following aspects: (1) evaluating needs and determining the added value of the implementation of the AI-based medical device; (2) assessing workplace preparedness, including stakeholder acceptance of the introduction of the AI-based medical device and involvement; and (3) analyzing the alignment between AI technology and organizational structure [ 64 ]. Decision makers and technology advocates need to address the complexities of AI more comprehensively and understand the systemic challenges that its adoption poses to health care organizations and systems. As an example, consider an AI tool used for diagnosing diabetic retinopathy in a primary care setting, such as by a family physician or nurse [ 102 ]. In theory, this could lead to shorter waiting times for patients. However, if the health care organization faces challenges such as a shortage of specialized staff (eg, ophthalmologists), insufficient organization of care pathways, and lack of specialized facilities for proper management and follow-up after diagnosis, the introduction of AI might adversely affect the quality of care and patient experience. In such a scenario, the AI application might merely transfer the delay from primary to secondary care, failing to address the fundamental issue.
Finally, concerning the last domains (6, 8, and 9) about ethical, patient, social, and legal aspects, AI-based continuous glucose monitoring and insulin pumps should also be assessed on ethical and legal aspects [ 103 ]. While citizen juries have generally shown support for AI in research and treatment, concerns remain. The risks of data theft and privacy breaches necessitate careful consideration of ethical and legal issues for patients. Although AI can aid in decision-making, it cannot wholly substitute a physician’s expertise. Effective regulations and systems designed to ensure safety, reduce bias, and enhance transparency are essential.
By implementing these recommendations, stakeholders can foster the responsible integration, regulation, and evaluation of AI-based medical devices. These measures can enhance the evidence base, address ethical concerns, and maximize the potential benefits of these AI technologies in improving health care outcomes while protecting patient safety, privacy, and equity.
Practical Challenges and Potential Barriers to Implementing HTAs Specific for AI-Based Medical Devices
When it comes to implementing specialized HTAs for AI-based medical devices, several practical challenges and potential barriers could be encountered and should be taken into account: First, AI technological evolution timeline—AI technologies evolve at a much faster pace compared to traditional medical devices, making it challenging for HTA frameworks to keep up with the latest developments and assess their long-term impact effectively. AI-based medical devices have a short product lifetime, between 12 and 18 months, in contrast to drug products. This shorter life cycle highlights the need for evolutive and fast-track HTA processes for AI-based medical devices. Second, data requirements and quality—AI systems rely heavily on large data sets for training and validation. Ensuring the availability of high-quality, representative data is a significant challenge. There is also the issue of data privacy and security, which must be addressed. The availability and quality of data and evidence required for conducting HTAs on AI-based medical devices present a complex and evolving landscape. Assessing the feasibility and challenges associated with gathering such data is crucial for robust evaluations. First, the availability of data can vary significantly depending on the AI-based medical device. While some devices may have access to vast amounts of high-quality real-world patient data, others might face limitations due to the novelty of the technology or issues related to data privacy. Second, the quality of data is paramount as inaccurate or biased data can lead to flawed assessments. Ensuring data accuracy, representativeness, and relevance is a constant challenge in AI-based medical device evaluations [ 104 ]. The rapid pace of AI development can result in limited long-term data, making it difficult to assess the device’s sustained performance and safety [ 105 ]. Balancing the need for robust evidence with the dynamic nature of AI technologies is a significant challenge that HTA organizations must address to provide valuable insights for informed decision-making in health care. Third, complexity and transparency—the complex algorithms used in AI-based medical devices can be difficult to understand and assess, leading to issues with transparency and explainability [ 17 ]. This complexity can pose a challenge for regulators and assessors in HTA processes. Fourth, clinical validation and safety requirements—generating robust clinical evidence to demonstrate the safety, efficacy, and effectiveness of AI-based medical devices can be challenging. This includes proving that these devices perform consistently across diverse patient populations. Moreover, to account for a life cycle that would include updates that may improve performance, one solution to investigate could be the proposition of the FDA in the United States in 2019 to experiment with a “dynamic certification” [ 100 ]. It allows for the re-evaluation of the AI-based medical device in case of a substantial modification of the indication of the medical device or the way to deliver the diagnosis, for instance. Fifth, regulatory and ethical considerations—adapting existing regulatory frameworks to accommodate AI-based medical devices, addressing ethical concerns such as bias, and ensuring equitable access are critical challenges [ 106 ]. Sixth, economic evaluation—determining the cost-effectiveness of AI-based medical devices, especially when benefits might be indirect or long term, poses a unique challenge for HTA [ 93 ]. Seventh, stakeholder engagement and trust—building trust among health care providers, patients, and policy makers regarding the reliability, trustworthiness, and usefulness of AI-based medical devices is crucial but challenging [ 107 ]. Eighth, integration into health care systems and interoperability—the integration of AI-based medical devices into existing health care workflows and systems can be complex and resource intensive [ 18 ]. Ninth, global and local applicability—ensuring that AI-based medical devices are effective and appropriate for use in different global and local contexts, considering varying health care systems and population needs, is another significant barrier.
Practically, one suggestion to implement such frameworks could be to implement these recommendations in the future European clinical joint assessment guidelines. As they are being currently discussed in a European project on a common HTA process for connected medical devices that includes AI-based medical devices, it could be the opportunity to tackle these challenges at the European level [ 108 ].
Addressing these challenges requires a concerted effort from regulators, health care providers, technology developers, and other stakeholders in the health care ecosystem.
Conclusions
AI-based medical devices have the potential to transform health care delivery, but the suitability of the current comprehensive HTA requires careful adaptation of the evaluation across the 9 dimensions. While these AI devices show promise in improving accuracy, safety, and efficiency, there is a need for robust clinical validation, integration into workflows, economic evaluation, and addressing of ethical and legal implications. A comprehensive adapted HTA framework for AI-based medical devices can provide valuable insights into their effectiveness, cost-effectiveness, and societal impact, guiding their responsible implementation and maximizing their benefits for patients and health care systems.
Data Availability
The data sets generated during and analyzed during this study are available from the corresponding author on reasonable request.
Authors' Contributions
LF and AV contributed to the conceptualization. LF and AV contributed to the methodology. LF, AV, NM, and IB contributed to the article selection. LF and AV contributed to the writing and original draft preparation. NM and IB contributed to the writing and review and editing. LF and AV contributed to the validation. The authors have read and agreed to the published version of the manuscript.
Conflicts of Interest
None declared.
The 9 health technology assessment (HTA) domains for the assessment of artificial intelligence–based medical devices adapted from the HTA Core Model proposed by the European Network for Health Technology Assessment.
Summary results of the scoping review and general characteristics of the studies included in the scoping review.
- Bajwa J, Munir U, Nori A, Williams B. Artificial intelligence in healthcare: transforming the practice of medicine. Future Healthc J. Jul 2021;8(2):e188-e194. [ CrossRef ] [ Medline ]
- Bohr A, Memarzadeh K. The rise of artificial intelligence in healthcare applications. In: Artificial Intelligence in Healthcare. Cambridge, MA. Academic Press; 2020.
- Park CW, Seo SW, Kang N, Ko B, Choi BW, Park CM, et al. Artificial intelligence in health care: current applications and issues. J Korean Med Sci. Nov 02, 2020;35(42):e379. [ CrossRef ] [ Medline ]
- Farah L, Davaze-Schneider J, Martin T, Nguyen P, Borget I, Martelli N. Are current clinical studies on artificial intelligence-based medical devices comprehensive enough to support a full health technology assessment? A systematic review. Artif Intell Med. Jun 2023;140:102547. [ CrossRef ] [ Medline ]
- HTA core model. European Network for Health Technology Assessment. URL: https://www.eunethta.eu/hta-core-model/ [accessed 2021-10-20]
- Liu X, Cruz Rivera S, Moher D, Calvert MJ, Denniston AK, SPIRIT-AI and CONSORT-AI Working Group. Reporting guidelines for clinical trial reports for interventions involving artificial intelligence: the CONSORT-AI extension. Nat Med. Sep 2020;26(9):1364-1374. [ CrossRef ] [ Medline ]
- Rivera SC, Liu X, Chan AW, Denniston AK, Calvert MJ, SPIRIT-AICONSORT-AI Working Group. Guidelines for clinical trial protocols for interventions involving artificial intelligence: the SPIRIT-AI Extension. BMJ. Sep 09, 2020;370:m3210. [ CrossRef ] [ Medline ]
- Collins GS, Dhiman P, Andaur Navarro CL, Ma J, Hooft L, Reitsma JB, et al. Protocol for development of a reporting guideline (TRIPOD-AI) and risk of bias tool (PROBAST-AI) for diagnostic and prognostic prediction model studies based on artificial intelligence. BMJ Open. Jul 09, 2021;11(7):e048008. [ CrossRef ] [ Medline ]
- Chen Y. Health technology assessment and economic evaluation: is it applicable for the traditional medicine? Integr Med Res. Mar 2022;11(1):100756. [ CrossRef ] [ Medline ]
- He J, Baxter SL, Xu J, Xu J, Zhou X, Zhang K. The practical implementation of artificial intelligence technologies in medicine. Nat Med. Jan 2019;25(1):30-36. [ CrossRef ] [ Medline ]
- Giordano C, Brennan M, Mohamed B, Rashidi P, Modave F, Tighe P. Accessing artificial intelligence for clinical decision-making. Front Digit Health. Jun 25, 2021;3:645232. [ CrossRef ] [ Medline ]
- Vayena E, Blasimme A, Cohen IG. Machine learning in medicine: addressing ethical challenges. PLoS Med. Nov 6, 2018;15(11):e1002689. [ CrossRef ] [ Medline ]
- Dzobo K, Adotey S, Thomford NE, Dzobo W. Integrating artificial and human intelligence: a partnership for responsible innovation in biomedical engineering and medicine. OMICS. May 2020;24(5):247-263. [ CrossRef ] [ Medline ]
- Zawati M, Lang M. What's in the box?: uncertain accountability of machine learning applications in healthcare. Am J Bioeth. Nov 2020;20(11):37-40. [ CrossRef ] [ Medline ]
- Peters MD, Godfrey C, McInerney P, Khalil H, Larsen P, Marnie C, et al. Best practice guidance and reporting items for the development of scoping review protocols. JBI Evid Synth. Apr 01, 2022;20(4):953-968. [ CrossRef ] [ Medline ]
- Pollock D, Peters MD, Khalil H, McInerney P, Alexander L, Tricco AC, et al. Recommendations for the extraction, analysis, and presentation of results in scoping reviews. JBI Evid Synth. Mar 01, 2023;21(3):520-532. [ CrossRef ] [ Medline ]
- Farah L, Murris JM, Borget I, Guilloux A, Martelli NM, Katsahian SI. Assessment of performance, interpretability, and explainability in artificial intelligence–based health technologies: what healthcare stakeholders need to know. Mayo Clin Proc Digit Health. Jun 2023;1(2):120-138. [ CrossRef ]
- Lehne M, Sass J, Essenwanger A, Schepers J, Thun S. Why digital medicine depends on interoperability. NPJ Digit Med. Aug 20, 2019;2:79. [ CrossRef ] [ Medline ]
- Esmaeilzadeh P. Use of AI-based tools for healthcare purposes: a survey study from consumers' perspectives. BMC Med Inform Decis Mak. Jul 22, 2020;20(1):170. [ CrossRef ] [ Medline ]
- Zia A, Aziz M, Popa I, Khan SA, Hamedani AF, Asif AR. Artificial intelligence-based medical data mining. J Pers Med. Aug 24, 2022;12(9):1359. [ CrossRef ] [ Medline ]
- Ming J, He Y, Yang Y, Hu M, Zhao X, Liu J, et al. Health technology assessment of medical devices: current landscape, challenges, and a way forward. Cost Eff Resour Alloc. Oct 05, 2022;20(1):54. [ CrossRef ] [ Medline ]
- Filkins BL, Kim JY, Roberts B, Armstrong W, Miller MA, Hultner ML, et al. Privacy and security in the era of digital health: what should translational researchers know and do about it? Am J Transl Res. Mar 15, 2016;8(3):1560-1580. [ Medline ]
- Johnson KB, Wei WQ, Weeraratne D, Frisse ME, Misulis K, Rhee K, et al. Precision medicine, AI, and the future of personalized health care. Clin Transl Sci. Jan 2021;14(1):86-93. [ CrossRef ] [ Medline ]
- Sloane EJ, Silva RJ. Artificial intelligence in medical devices and clinical decision support systems. In: Clinical Engineering Handbook (Second Edition). Cambridge, MA. Academic Press; 2020.
- Steuten LM. Early stage health technology assessment for precision biomarkers in oral health and systems medicine. OMICS. Jan 2016;20(1):30-35. [ CrossRef ] [ Medline ]
- Javaid M, Haleem A, Pratap Singh R, Suman R, Rab S. Significance of machine learning in healthcare: features, pillars and applications. Int J Intell Networks. 2022;3:58-73. [ CrossRef ]
- Rowland SP, Fitzgerald JE, Holme T, Powell J, McGregor A. What is the clinical value of mHealth for patients? NPJ Digit Med. Jan 13, 2020;3:4. [ CrossRef ] [ Medline ]
- Voets MM, Veltman J, Slump CH, Siesling S, Koffijberg H. Systematic review of health economic evaluations focused on artificial intelligence in healthcare: the tortoise and the cheetah. Value Health. Mar 2022;25(3):340-349. [ CrossRef ] [ Medline ]
- Kirisits A, Redekop WK. The economic evaluation of medical devices: challenges ahead. Appl Health Econ Health Policy. Feb 2013;11(1):15-26. [ CrossRef ] [ Medline ]
- Park SH, Choi J, Byeon JS. Key principles of clinical validation, device approval, and insurance coverage decisions of artificial intelligence. Korean J Radiol. Mar 2021;22(3):442-453. [ CrossRef ] [ Medline ]
- Tsopra R, Fernandez X, Luchinat C, Alberghina L, Lehrach H, Vanoni M, et al. A framework for validating AI in precision medicine: considerations from the European ITFoC consortium. BMC Med Inform Decis Mak. Oct 02, 2021;21(1):274. [ CrossRef ] [ Medline ]
- Bolboacă SD. Medical diagnostic tests: a review of test anatomy, phases, and statistical treatment of data. Comput Math Methods Med. May 28, 2019;2019:1891569. [ CrossRef ] [ Medline ]
- Hogervorst MA, Pontén J, Vreman RA, Mantel-Teeuwisse AK, Goettsch WG. Real world data in health technology assessment of complex health technologies. Front Pharmacol. Feb 10, 2022;13:837302. [ CrossRef ] [ Medline ]
- Simon GE, Bindman AB, Dreyer NA, Platt R, Watanabe JH, Horberg M, et al. When can we trust real-world data to evaluate new medical treatments? Clin Pharmacol Ther. Jan 2022;111(1):24-29. [ CrossRef ] [ Medline ]
- Pongiglione B, Torbica A, Blommestein H, de Groot S, Ciani O, Walker S, et al. Do existing real-world data sources generate suitable evidence for the HTA of medical devices in Europe? Mapping and critical appraisal. Int J Technol Assess Health Care. Apr 26, 2021;37(1):e62. [ CrossRef ] [ Medline ]
- Pongiglione B, Torbica A. How real can we get in generating real world evidence? Exploring the opportunities of routinely collected administrative data for evaluation of medical devices. Health Econ. Sep 2022;31 Suppl 1(Suppl 1):25-43. [ CrossRef ] [ Medline ]
- Zemplényi A, Tachkov K, Balkanyi L, Németh B, Petykó ZI, Petrova G, et al. Recommendations to overcome barriers to the use of artificial intelligence-driven evidence in health technology assessment. Front Public Health. Apr 26, 2023;11:1088121. [ CrossRef ] [ Medline ]
- Daubner-Bendes R, Kovács S, Niewada M, Huic M, Drummond M, Ciani O, et al. Quo Vadis HTA for medical devices in central and eastern Europe? Recommendations to address methodological challenges. Front Public Health. Jan 08, 2021;8:612410. [ CrossRef ] [ Medline ]
- Larson DB, Harvey H, Rubin DL, Irani N, Tse JR, Langlotz CP. Regulatory frameworks for development and evaluation of artificial intelligence-based diagnostic imaging algorithms: summary and recommendations. J Am Coll Radiol. Mar 2021;18(3 Pt A):413-424. [ CrossRef ] [ Medline ]
- Choudhury A, Asan O. Role of artificial intelligence in patient safety outcomes: systematic literature review. JMIR Med Inform. Jul 24, 2020;8(7):e18599. [ CrossRef ] [ Medline ]
- Belenguer L. AI bias: exploring discriminatory algorithmic decision-making models and the application of possible machine-centric solutions adapted from the pharmaceutical industry. AI Ethics. 2022;2(4):771-787. [ CrossRef ] [ Medline ]
- Binder L, Ghadban M, Sit C, Barnard K. Health technology assessment process for oncology drugs: impact of CADTH changes on public payer reimbursement recommendations. Curr Oncol. Mar 01, 2022;29(3):1514-1526. [ CrossRef ] [ Medline ]
- Wolff J, Pauling J, Keck A, Baumbach J. The economic impact of artificial intelligence in health care: systematic review. J Med Internet Res. Feb 20, 2020;22(2):e16866. [ CrossRef ] [ Medline ]
- Abràmoff MD, Roehrenbeck C, Trujillo S, Goldstein J, Graves AS, Repka MX, et al. A reimbursement framework for artificial intelligence in healthcare. NPJ Digit Med. Jun 09, 2022;5(1):72. [ CrossRef ] [ Medline ]
- Bélisle-Pipon JC, Couture V, Roy MC, Ganache I, Goetghebeur M, Cohen IG. What makes artificial intelligence exceptional in health technology assessment? Front Artif Intell. Nov 02, 2021;4:736697. [ CrossRef ] [ Medline ]
- Alami H, Lehoux P, Auclair Y, de Guise M, Gagnon MP, Shaw J, et al. Artificial intelligence and health technology assessment: anticipating a new level of complexity. J Med Internet Res. Jul 07, 2020;22(7):e17707. [ CrossRef ] [ Medline ]
- Love-Koh J, Peel A, Rejon-Parrilla JC, Ennis K, Lovett R, Manca A, et al. The future of precision medicine: potential impacts for health technology assessment. Pharmacoeconomics. Dec 2018;36(12):1439-1451. [ CrossRef ] [ Medline ]
- Gomez Rossi J, Rojas-Perilla N, Krois J, Schwendicke F. Cost-effectiveness of artificial intelligence as a decision-support system applied to the detection and grading of melanoma, dental caries, and diabetic retinopathy. JAMA Netw Open. Mar 01, 2022;5(3):e220269. [ CrossRef ] [ Medline ]
- Naik N, Hameed BM, Shetty DK, Swain D, Shah M, Paul R, et al. Legal and ethical consideration in artificial intelligence in healthcare: who takes responsibility? Front Surg. Mar 14, 2022;9:862322. [ CrossRef ] [ Medline ]
- Haynes CL, Cook GA, Jones MA. Legal and ethical considerations in processing patient-identifiable data without patient consent: lessons learnt from developing a disease register. J Med Ethics. May 2007;33(5):302-307. [ CrossRef ] [ Medline ]
- McCradden MD, Joshi S, Mazwi M, Anderson JA. Ethical limitations of algorithmic fairness solutions in health care machine learning. Lancet Digit Health. May 2020;2(5):e221-e223. [ CrossRef ] [ Medline ]
- Gianfrancesco MA, Tamang S, Yazdany J, Schmajuk G. Potential biases in machine learning algorithms using electronic health record data. JAMA Intern Med. Nov 01, 2018;178(11):1544-1547. [ CrossRef ] [ Medline ]
- Fletcher RR, Nakeshimana A, Olubeko O. Addressing fairness, bias, and appropriate use of artificial intelligence and machine learning in global health. Front Artif Intell. Apr 15, 2021;3:561802. [ CrossRef ] [ Medline ]
- Tachkov K, Zemplenyi A, Kamusheva M, Dimitrova M, Siirtola P, Pontén J, et al. Barriers to use artificial intelligence methodologies in health technology assessment in central and east European countries. Front Public Health. Jul 14, 2022;10:921226. [ CrossRef ] [ Medline ]
- Durán JM, Jongsma KR. Who is afraid of black box algorithms? On the epistemological and ethical basis of trust in medical AI. J Med Ethics. Mar 18, 2021. (forthcoming). [ CrossRef ] [ Medline ]
- Amann J, Blasimme A, Vayena E, Frey D, Madai VI, Precise4Q consortium. Explainability for artificial intelligence in healthcare: a multidisciplinary perspective. BMC Med Inform Decis Mak. Nov 30, 2020;20(1):310. [ CrossRef ] [ Medline ]
- Kiseleva A, Kotzinos D, De Hert P. Transparency of AI in healthcare as a multilayered system of accountabilities: between legal requirements and technical limitations. Front Artif Intell. May 30, 2022;5:879603. [ CrossRef ] [ Medline ]
- Baltaxe E, Hsieh HW, Roca J, Cano I. The assessment of medical device software supporting health care services for chronic patients in a tertiary hospital: overarching study. J Med Internet Res. Jan 04, 2023;25:e40976. [ CrossRef ] [ Medline ]
- Garfield S, Polisena J, S Spinner D, Postulka A, Y Lu C, Tiwana SK, et al. Health technology assessment for molecular diagnostics: practices, challenges, and recommendations from the medical devices and diagnostics special interest group. Value Health. 2016;19(5):577-587. [ CrossRef ] [ Medline ]
- Fraser AG, Biasin E, Bijnens B, Bruining N, Caiani EG, Cobbaert K, et al. Artificial intelligence in medical device software and high-risk medical devices - a review of definitions, expert recommendations and regulatory initiatives. Expert Rev Med Devices. Jun 2023;20(6):467-491. [ CrossRef ] [ Medline ]
- Beckers R, Kwade Z, Zanca F. The EU medical device regulation: implications for artificial intelligence-based medical device software in medical physics. Phys Med. Mar 2021;83:1-8. [ CrossRef ] [ Medline ]
- Melvin T, Torre M. New medical device regulations: the regulator's view. EFORT Open Rev. Jun 03, 2019;4(6):351-356. [ CrossRef ] [ Medline ]
- Fleetcroft C, McCulloch P, Campbell B. IDEAL as a guide to designing clinical device studies consistent with the new European Medical Device Regulation. BMJ Surg Interv Health Technol. Mar 04, 2021;3(1):e000066. [ CrossRef ] [ Medline ]
- Alami H, Lehoux P, Denis JL, Motulsky A, Petitgand C, Savoldelli M, et al. Organizational readiness for artificial intelligence in health care: insights for decision-making and practice. J Health Organ Manag. Dec 03, 2020. (forthcoming). [ CrossRef ] [ Medline ]
- Segur-Ferrer J, Moltó-Puigmartí C, Pastells-Peiró R, Vivanco-Hidalgo RM. Methodological frameworks and dimensions to be taken into consideration in digital health technology assessment: protocol for a scoping review. JMIR Res Protoc. Oct 11, 2022;11(10):e39905. [ CrossRef ] [ Medline ]
- Weinert L, Müller J, Svensson L, Heinze O. Perspective of information technology decision makers on factors influencing adoption and implementation of artificial intelligence technologies in 40 German hospitals: descriptive analysis. JMIR Med Inform. Jun 15, 2022;10(6):e34678. [ CrossRef ] [ Medline ]
- de Hond AA, Leeuwenberg AM, Hooft L, Kant IM, Nijman SW, van Os HJ, et al. Guidelines and quality criteria for artificial intelligence-based prediction models in healthcare: a scoping review. NPJ Digit Med. Jan 10, 2022;5(1):2. [ CrossRef ] [ Medline ]
- Ahuja AS. The impact of artificial intelligence in medicine on the future role of the physician. PeerJ. 2019;7:e7702. [ CrossRef ] [ Medline ]
- Widrig D, Tag B. HTA and its legal issues: a framework for identifying legal issues in health technology assessment. Int J Technol Assess Health Care. Dec 2014;30(6):587-594. [ CrossRef ] [ Medline ]
- Vella Bonanno P, Bucsics A, Simoens S, Martin AP, Oortwijn W, Gulbinovič J, et al. Proposal for a regulation on health technology assessment in Europe - opinions of policy makers, payers and academics from the field of HTA. Expert Rev Pharmacoecon Outcomes Res. Jun 2019;19(3):251-261. [ CrossRef ] [ Medline ]
- McKee M, Wouters OJ. The challenges of regulating artificial intelligence in healthcare comment on "clinical decision support and new regulatory frameworks for medical devices: are we ready for it? - a viewpoint paper". Int J Health Policy Manag. 2023;12:7261. [ CrossRef ] [ Medline ]
- Hordern V. Data protection compliance in the age of digital health. Eur J Health Law. Jun 2016;23(3):248-264. [ CrossRef ] [ Medline ]
- Stanberry B. The legal and ethical aspects of telemedicine. 2: data protection, security and European law. J Telemed Telecare. 1998;4(1):18-24. [ CrossRef ] [ Medline ]
- Poullet Y. Legal aspects of data protection in medical informatics. Stud Health Technol Inform. 1991;1:138-160. [ Medline ]
- Dove ES, Chen J. To what extent does the EU general data protection regulation (GDPR) apply to citizen scientist-led health research with mobile devices? J Law Med Ethics. Mar 2020;48(1_suppl):187-195. [ CrossRef ] [ Medline ]
- Marovic B, Curcin V. Impact of the European general data protection regulation (GDPR) on health data management in a European Union candidate country: a case study of Serbia. JMIR Med Inform. Apr 17, 2020;8(4):e14604. [ CrossRef ] [ Medline ]
- EU Artificial Intelligence Act. URL: https://artificialintelligenceact.eu/ [accessed 2023-07-24]
- Proposal for a regulation of the European parliament and of the council laying down harmonised rules on artificial intelligence (Artificial Intelligence Act) and amending certain union legislative acts. European Union. 2021. URL: https://eur-lex.europa.eu/legal-content/EN/TXT/?uri=CELEX:52021PC0206 [accessed 2023-07-24]
- Camara C, Peris-Lopez P, Tapiador JE. Security and privacy issues in implantable medical devices: a comprehensive survey. J Biomed Inform. Jun 2015;55:272-289. [ CrossRef ] [ Medline ]
- Migliore A, Ratti M, Cerbo M, Jefferson T. Health Technology Assessment: managing the introduction and use of medical devices in clinical practice in Italy. Expert Rev Med Devices. May 2009;6(3):251-257. [ CrossRef ] [ Medline ]
- Pisapia A, Banfi G, Tomaiuolo R. The novelties of the regulation on health technology assessment, a key achievement for the European Union health policies. Clin Chem Lab Med. Jul 26, 2022;60(8):1160-1163. [ CrossRef ] [ Medline ]
- Maliha G, Gerke S, Cohen IG, Parikh RB. Artificial intelligence and liability in medicine: balancing safety and innovation. Milbank Q. Sep 2021;99(3):629-647. [ CrossRef ] [ Medline ]
- Jassar S, Adams SJ, Zarzeczny A, Burbridge BE. The future of artificial intelligence in medicine: medical-legal considerations for health leaders. Healthc Manage Forum. May 2022;35(3):185-189. [ CrossRef ] [ Medline ]
- Samore MH, Evans RS, Lassen A, Gould P, Lloyd J, Gardner RM, et al. Surveillance of medical device-related hazards and adverse events in hospitalized patients. JAMA. Jan 21, 2004;291(3):325-334. [ CrossRef ] [ Medline ]
- Bleher H, Braun M. Diffused responsibility: attributions of responsibility in the use of AI-driven clinical decision support systems. AI Ethics. 2022;2(4):747-761. [ CrossRef ] [ Medline ]
- Street J, Stafinski T, Lopes E, Menon D. Defining the role of the public in Health Technology Assessment (HTA) and HTA-informed decision-making processes. Int J Technol Assess Health Care. Apr 2020;36(2):87-95. [ CrossRef ] [ Medline ]
- Massella M, Dri DA, Gramaglia D. Regulatory considerations on the use of machine learning based tools in clinical trials. Health Technol (Berl). 2022;12(6):1085-1096. [ CrossRef ] [ Medline ]
- Marcus HJ, Payne CJ, Hughes-Hallett A, Marcus AP, Yang GZ, Darzi A, et al. Regulatory approval of new medical devices: cross sectional study. BMJ. May 20, 2016;353:i2587. [ CrossRef ] [ Medline ]
- Milam ME, Koo CW. The current status and future of FDA-approved artificial intelligence tools in chest radiology in the United States. Clin Radiol. Feb 2023;78(2):115-122. [ CrossRef ] [ Medline ]
- Vreman RA, Mantel-Teeuwisse AK, Hövels AM, Leufkens HG, Goettsch WG. Differences in health technology assessment recommendations among European jurisdictions: the role of practice variations. Value Health. Jan 2020;23(1):10-16. [ CrossRef ] [ Medline ]
- Allen N, Walker SR, Liberti L, Salek S. Health technology assessment (HTA) case studies: factors influencing divergent HTA reimbursement recommendations in Australia, Canada, England, and Scotland. Value Health. Mar 2017;20(3):320-328. [ CrossRef ] [ Medline ]
- Agboola F, Wright AC, Herron-Smith S, Mathur D, Rind D. Evaluation of diversity of clinical trials informing health technology assessments in the United States: a 5-year analysis of institute for clinical and economic review assessments. Value Health. Sep 2023;26(9):1345-1352. [ CrossRef ] [ Medline ]
- Hendrix N, Veenstra DL, Cheng M, Anderson NC, Verguet S. Assessing the economic value of clinical artificial intelligence: challenges and opportunities. Value Health. Mar 2022;25(3):331-339. [ CrossRef ] [ Medline ]
- Nielsen CP, Lauritsen SW, Kristensen FB, Bistrup ML, Cecchetti A, Turk E. Involving stakeholders and developing a policy for stakeholder involvement in the European network for health technology assessment, EUnetHTA. Int J Technol Assess Health Care. Dec 2009;25 Suppl 2:84-91. [ CrossRef ] [ Medline ]
- Scott AM, Wale JL, HTAi PatientCitizen Involvement in HTA Interest Group‚ Patient InvolvementEducation Working Group. Patient advocate perspectives on involvement in HTA: an international snapshot. Res Involv Engagem. Jan 10, 2017;3:2. [ CrossRef ] [ Medline ]
- Gagnon MP, Desmartis M, Gagnon J, St-Pierre M, Gauvin FP, Rhainds M, et al. Introducing the patient's perspective in hospital health technology assessment (HTA): the views of HTA producers, hospital managers and patients. Health Expect. Dec 2014;17(6):888-900. [ CrossRef ] [ Medline ]
- Gagnon M, Desmartis M, Gagnon J, St-Pierre M, Rhainds M, Coulombe M, et al. Framework for user involvement in health technology assessment at the local level: views of health managers, user representatives, and clinicians. Int J Technol Assess Health Care. Jan 2015;31(1-2):68-77. [ CrossRef ] [ Medline ]
- Angelis A, Kanavos P. Value-based assessment of new medical technologies: towards a robust methodological framework for the application of multiple criteria decision analysis in the context of health technology assessment. Pharmacoeconomics. May 2016;34(5):435-446. [ CrossRef ] [ Medline ]
- Wale JL, Thomas S, Hamerlijnck D, Hollander R. Patients and public are important stakeholders in health technology assessment but the level of involvement is low - a call to action. Res Involv Engagem. Jan 05, 2021;7(1):1. [ CrossRef ] [ Medline ]
- Artificial intelligence and machine learning (AI/ML)-enabled medical devices. U.S. Food & Drug Administration. Oct 19, 2023. URL: https://tinyurl.com/m8ba5aw4 [accessed 2023-11-25]
- Cassidy B, Hoon Yap M, Pappachan JM, Ahmad N, Haycocks S, O'Shea C, et al. Artificial intelligence for automated detection of diabetic foot ulcers: a real-world proof-of-concept clinical evaluation. Diabetes Res Clin Pract. Nov 2023;205:110951. [ CrossRef ] [ Medline ]
- Mackenzie SC, Sainsbury CA, Wake DJ. Diabetes and artificial intelligence beyond the closed loop: a review of the landscape, promise and challenges. Diabetologia. Feb 2024;67(2):223-235. [ CrossRef ] [ Medline ]
- Sridhar GR, Lakshmi G. Ethical issues of artificial intelligence in diabetes mellitus. Med Res Arch. Aug 2023;11(8):1-8. [ CrossRef ]
- Zhang L, Zhang L. Artificial intelligence for remote sensing data analysis: a review of challenges and opportunities. IEEE Geosci Remote Sens Mag. Apr 13, 2022;10(2):270-294. [ FREE Full text ] [ CrossRef ]
- Challen R, Denny J, Pitt M, Gompels L, Edwards T, Tsaneva-Atanasova K. Artificial intelligence, bias and clinical safety. BMJ Qual Saf. Mar 2019;28(3):231-237. [ CrossRef ] [ Medline ]
- Beil M, Proft I, van Heerden D, Sviri S, van Heerden PV. Ethical considerations about artificial intelligence for prognostication in intensive care. Intensive Care Med Exp. Dec 10, 2019;7(1):70. [ CrossRef ] [ Medline ]
- Bærøe K, Miyata-Sturm A, Henden E. How to achieve trustworthy artificial intelligence for health. Bull World Health Organ. Apr 01, 2020;98(4):257-262. [ CrossRef ] [ Medline ]
- Julian E, Gianfrate F, Sola-Morales O, Mol P, Bergmann JF, Salmonson T, et al. How can a joint European health technology assessment provide an 'additional benefit' over the current standard of national assessments? : insights generated from a multi-stakeholder survey in hematology/oncology. Health Econ Rev. Jun 02, 2022;12(1):30. [ CrossRef ] [ Medline ]
Abbreviations
Edited by Y Zhuang; submitted 02.08.23; peer-reviewed by A de Hond, E Vashishtha, I Cano, N Hendrix; comments to author 28.10.23; revised version received 17.12.23; accepted 28.12.23; published 13.05.24.
©Line Farah, Isabelle Borget, Nicolas Martelli, Alexandre Vallee. Originally published in the Journal of Medical Internet Research (https://www.jmir.org), 13.05.2024.
This is an open-access article distributed under the terms of the Creative Commons Attribution License (https://creativecommons.org/licenses/by/4.0/), which permits unrestricted use, distribution, and reproduction in any medium, provided the original work, first published in the Journal of Medical Internet Research, is properly cited. The complete bibliographic information, a link to the original publication on https://www.jmir.org/, as well as this copyright and license information must be included.
- Open access
- Published: 09 May 2024
Machine learning models for abstract screening task - A systematic literature review application for health economics and outcome research
- Jingcheng Du 1 ,
- Ekin Soysal 1 , 3 ,
- Dong Wang 2 ,
- Long He 1 ,
- Bin Lin 1 ,
- Jingqi Wang 1 ,
- Frank J. Manion 1 ,
- Yeran Li 2 ,
- Elise Wu 2 &
- Lixia Yao 2
BMC Medical Research Methodology volume 24 , Article number: 108 ( 2024 ) Cite this article
143 Accesses
3 Altmetric
Metrics details
Systematic literature reviews (SLRs) are critical for life-science research. However, the manual selection and retrieval of relevant publications can be a time-consuming process. This study aims to (1) develop two disease-specific annotated corpora, one for human papillomavirus (HPV) associated diseases and the other for pneumococcal-associated pediatric diseases (PAPD), and (2) optimize machine- and deep-learning models to facilitate automation of the SLR abstract screening.
This study constructed two disease-specific SLR screening corpora for HPV and PAPD, which contained citation metadata and corresponding abstracts. Performance was evaluated using precision, recall, accuracy, and F1-score of multiple combinations of machine- and deep-learning algorithms and features such as keywords and MeSH terms.
Results and conclusions
The HPV corpus contained 1697 entries, with 538 relevant and 1159 irrelevant articles. The PAPD corpus included 2865 entries, with 711 relevant and 2154 irrelevant articles. Adding additional features beyond title and abstract improved the performance (measured in Accuracy) of machine learning models by 3% for HPV corpus and 2% for PAPD corpus. Transformer-based deep learning models that consistently outperformed conventional machine learning algorithms, highlighting the strength of domain-specific pre-trained language models for SLR abstract screening. This study provides a foundation for the development of more intelligent SLR systems.
Peer Review reports
Introduction
Systematic literature reviews (SLRs) are an essential tool in many areas of health sciences, enabling researchers to understand the current knowledge around a topic and identify future research and development directions. In the field of health economics and outcomes research (HEOR), SLRs play a crucial role in synthesizing evidence around unmet medical needs, comparing treatment options, and preparing the design and execution of future real-world evidence studies. SLRs provide a comprehensive and transparent analysis of available evidence, allowing researchers to make informed decisions and improve patient outcomes.
Conducting a SLR involves synthesizing high-quality evidence from biomedical literature in a transparent and reproducible manner, and seeks to include all available evidence on a given research question, and provides some assessment regarding quality of the evidence [ 1 , 2 ]. To conduct an SLR one or more bibliographic databases are queried based on a given research question and a corresponding set of inclusion and exclusion criteria, resulting in the selection of a relevant set of abstracts. The abstracts are reviewed, further refining the set of articles that are used to address the research question. Finally, appropriate data is systematically extracted from the articles and summarized [ 1 , 3 ].
The current approach to conducting a SLR is through manual review, with data collection, and summary done by domain experts against pre-specified eligibility criteria. This is time-consuming, labor-intensive, expensive, and non-scalable given the current more-than linear growth of the biomedical literature [ 4 ]. Michelson and Reuter estimate that each SLR costs approximately $141,194.80 and that on average major pharmaceutical companies conduct 23.36 SLRs, and major academic centers 177.32 SLRs per year, though the cost may vary based on the scope of different reviews [ 4 ]. Clearly automated methods are needed, both from a cost/time savings perspective, and for the ability to effectively scan and identify increasing amounts of literature, thereby allowing the domain experts to spend more time analyzing the data and gleaning the insights.
One major task of SLR project that involves large amounts of manual effort, is the abstract screening task. For this task, selection criteria are developed and the citation metadata and abstract for articles tentatively meeting these criteria are retrieved from one or more bibliographic databases (e.g., PubMed). The abstracts are then examined in more detail to determine if they are relevant to the research question(s) and should be included or excluded from further consideration. Consequently, the task of determining whether articles are relevant or not based on their titles, abstracts and metadata can be treated as a binary classification task, which can be addressed by natural language processing (NLP). NLP involves recognizing entities and relationships expressed in text and leverages machine-learning (ML) and deep-learning (DL) algorithms together with computational semantics to extract information. The past decade has witnessed significant advances in these areas for biomedical literature mining. A comprehensive review on how NLP techniques in particular are being applied for automatic mining and knowledge extraction from biomedical literature can be found in Zhao et al. [ 5 ].
Materials and methods
The aims of this study were to: (1) identify and develop two disease-specific corpora, one for human papillomavirus (HPV) associated diseases and the other for pneumococcal-associated pediatric diseases suitable for training the ML and DL models underlying the necessary NLP functions; (2) investigate and optimize the performance of the ML and DL models using different sets of features (e.g., keywords, Medical Subject Heading (MeSH) terms [ 6 ]) to facilitate automation of the abstract screening tasks necessary to construct a SLR. Note that these screening corpora can be used as training data to build different NLP models. We intend to freely share these two corpora with the entire scientific community so they can serve as benchmark corpora for future NLP model development in this area.
SLR corpora preparation
Two completed disease-specific SLR studies by Merck & Co., Inc., Rahway, NJ, USA were used as the basis to construct corpora for abstract-level screening. The two SLR studies were both relevant to health economics and outcome research, including one for human papillomavirus (HPV) associated diseases (referred to as the HPV corpus), and one for pneumococcal-associated pediatric diseases (which we refer to as the PAPD corpus). Both of the original SLR studies contained literature from PubMed/MEDLINE and EMBASE. Since we intended for the screening corpora to be released to the community, we only kept citations found from PubMed/MEDLINE in the finalized corpora. Because the original SLR studies did not contain the PubMed ID (PMID) for each article, we matched each article’s citation information (if available) against PubMed and then collected meta-data such as authors, journals, keywords, MeSH terms, publication types, etc., using PubMed Entrez Programming Utilities (E-utilities) Application Programming Interface (API). The detailed description of the two corpora can be seen in Table 1 . Both of the resulting corpora are publicly available at [ https://github.com/Merck/NLP-SLR-corpora ].
Machine learning algorithms
Although deep learning algorithms have demonstrated superior performance on many NLP tasks, conventional machine learning algorithms have certain advantages, such as low computation costs and faster training and prediction speed.
We evaluated four traditional ML-based document classification algorithms, XGBoost [ 7 ], Support Vector Machines (SVM) [ 8 ], Logistic regression (LR) [ 9 ], and Random Forest [ 10 ] on the binary inclusion/exclusion classification task for abstract screening. Salient characteristics of these models are as follows:
XGBoost: Short for “eXtreme Gradient Boosting”, XGBoost is a boosting-based ensemble of algorithms that turn weak learners into strong learners by focusing on where the individual models went wrong. In Gradient Boosting, individual weak models train upon the difference between the prediction and the actual results [ 7 ]. We set max_depth at 3, n_estimators at 150 and learning rate at 0.7.
Support vector machine (SVM): SVM is one of the most robust prediction methods based on statistical learning frameworks. It aims to find a hyperplane in an N-dimensional space (where N = the number of features) that distinctly classifies the data points [ 8 ]. We set C at 100, gamma at 0.005 and kernel as radial basis function.
Logistic regression (LR): LR is a classic statistical model that in its basic form uses a logistic function to model a binary dependent variable [ 9 ]. We set C at 5 and penalty as l2.
Random forest (RF): RF is a machine learning technique that utilizes ensemble learning to combine many decision trees classifiers through bagging or bootstrap aggregating [ 10 ]. We set n_estimators at 100 and max_depth at 14.
These four algorithms were trained for both the HPV screening task and the PAPD screening task using the corresponding training corpus.
For each of the four algorithms, we examined performance using (1) only the baseline feature criteria (title and abstract of each article), and (2) with five additional meta-data features (MeSH, Authors, Keywords, Journal, Publication types.) retrieved from each article using the PubMed E-utilities API. Conventionally, title and abstract are the first information a human reviewer would depend on when making a judgment for inclusion or exclusion of an article. Consequently, we used title and abstract as the baseline features to classify whether an abstract should be included at the abstract screening stage. We further evaluated the performance with additional features that can be retrieved by PubMed E-utilities API, including MeSH terms, authors, journal, keywords and publication type. For baseline evaluation, we concatenated the titles and abstracts and extracted the TF-IDF (term frequency-inverse document frequency) vector for the corpus. TF-IDF evaluates how relevant a word is to a document in a collection of documents. For additional features, we extracted TF-IDF vector using each feature respectively and then concatenated the extracted vectors with title and abstract vector. XGBoost was selected for the feature evaluation process, due to its relatively quick computational running time and robust performance.
Deep learning algorithms
Conventional ML methods rely heavily on manually designed features and suffer from the challenges of data sparsity and poor transportability when applied to new use cases. Deep learning (DL) is a set of machine learning algorithms based on deep neural networks that has advanced performance of text classification along with many other NLP tasks. Transformer-based deep learning models, such as BERT (Bidirectional encoder representations from transformers), have achieved state-of-the-art performance in many NLP tasks [ 11 ]. A Transformer is an emerging architecture of deep learning models designed to handle sequential input data such as natural language by adopting the mechanisms of attention to differentially weigh the significance of each part of the input data [ 12 ]. The BERT model and its variants (which use Transformer as a basic unit) leverage the power of transfer learning by first pre-training the models over 100’s of millions of parameters using large volumes of unlabeled textual data. The resulting model is then fine-tuned for a particular downstream NLP application, such as text classification, named entity recognition, relation extraction, etc. The following three BERT models were evaluated against both the HPV and Pediatric pneumococcal corpus using two sets of features (title and abstract versus adding all additional features into the text). For all BERT models, we used Adam optimizer with weight decay. We set learning rate at 1e-5, batch size at 8 and number of epochs at 20.
BERT base: this is the original BERT model released by Google. The BERT base model was pre-trained on textual data in the general domain, i.e., BooksCorpus (800 M words) and English Wikipedia (2500 M words) [ 11 ].
BioBERT base: as the biomedical language is different from general language, the BERT models trained on general textual data may not work well on biomedical NLP tasks. BioBERT was further pre-trained (based on original BERT models) in the large-scale biomedical corpora, including PubMed abstracts (4.5B words) and PubMed Central Full-text articles (13.5B words) [ 13 ].
PubMedBERT: PubMedBERT was pre-trained from scratch using abstracts from PubMed. This model has achieved state-of-the-art performance on several biomedical NLP tasks on Biomedical Language Understanding and Reasoning Benchmark [ 14 ].
Text pre-processing and libraries that were used
We have removed special characters and common English words as a part of text pre-processing. Default tokenizer from scikit-learn was adopted for tokenization. Scikit-learn was also used for TF-IDF feature extraction and machine learning algorithms implementation. Transformers libraries from Hugging Face were used for deep learning algorithms implementation.
Evaluation datasets were constructed from the HPV and Pediatric pneumococcal corpora and were split into training, validation and testing sets with a ratio of 8:1:1 for the two evaluation tasks: (1) ML algorithms performance assessment; and (2) DL algorithms performance assessment. Models were fitted on the training sets, and model hyperparameters were optimized on the validation sets and the performance were evaluated on the testing sets. The following major metrics are expressed by the noted calculations:
Where True positive is an outcome where the model correctly predicts the positive (e.g., “included” in our tasks) class. Similarly, a True negative is an outcome where the model correctly predicts the negative class (e.g., “excluded” in our tasks). False positive is an outcome where the model incorrectly predicts the positive class, and a False negative is an outcome where the model incorrectly predicts the negative class. We have repeated all experiments five times and reported the mean scores with standard deviation.
Table 2 shows the baseline comparison using different feature combinations for the SLR text classification tasks using XGBoost. As noted, adding additional features in addition to title and abstract was effective in further improving the classification accuracy. Specifically, using all available features for the HPV classification increased accuracy by ? ∼ 3% and F1 score by ? ∼ 3%; using all available features for Pediatric pneumococcal classification increased accuracy by ? ∼ 2% and F1 score by ? ∼ 4%. As observed, adding additional features provided a stronger boost in precision, which contributed to the overall performance improvement.
The comparison of the article inclusion/exclusion classification task for four machine learning algorithms with all features is shown in Table 3 . XGBoost achieved the highest accuracy and F-1 scores in both tasks. Table 4 shows the comparison between XGBoost and deep learning algorithms on the classification tasks for each disease. Both XGBoost and deep learning models consistently have achieved higher accuracy scores when using all features as input. Among all models, BioBERT has achieved the highest accuracy at 0.88, compared with XGBoost at 0.86. XGBoost has the highest F1 score at 0.8 and the highest recall score at 0.9 for inclusion prediction.
Discussions and conclusions
Abstract screening is a crucial step in conducting a systematic literature review (SLR), as it helps to identify relevant citations and reduces the effort required for full-text screening and data element extraction. However, screening thousands of abstracts can be a time-consuming and burdensome task for scientific reviewers. In this study, we systematically investigated the use of various machine learning and deep learning algorithms, using different sets of features, to automate abstract screening tasks. We evaluated these algorithms using disease-focused SLR corpora, including one for human papillomavirus (HPV) associated diseases and another for pneumococcal-associated pediatric diseases (PADA). The publicly available corpora used in this study can be used by the scientific community for advanced algorithm development and evaluation. Our findings suggest that machine learning and deep learning algorithms can effectively automate abstract screening tasks, saving valuable time and effort in the SLR process.
Although machine learning and deep learning algorithms trained on the two SLR corpora showed some variations in performance, there were also some consistencies. Firstly, adding additional citation features significantly improved the performance of conventional machine learning algorithms, although the improvement was not as strong in transformer-based deep learning models. This may be because transformer models were mostly pre-trained on abstracts, which do not include additional citation information like MeSH terms, keywords, and journal names. Secondly, when using only title and abstract as input, transformer models consistently outperformed conventional machine learning algorithms, highlighting the strength of subject domain-specific pre-trained language models. When all citation features were combined as input, conventional machine learning algorithms showed comparable performance to deep learning models. Given the much lower computation costs and faster training and prediction time, XGBoost or support vector machines with all citation features could be an excellent choice for developing an abstract screening system.
Some limitations remain for this study. Although we’ve evaluated cutting-edge machine learning and deep learning algorithms on two SLR corpora, we did not conduct much task-specific customization to the learning algorithms, including task-specific feature engineering and rule-based post-processing, which could offer additional benefits to the performance. As the focus of this study is to provide generalizable strategies for employing machine learning to abstract screening tasks, we leave the task-specific customization to future improvement. The corpora we evaluated in this study mainly focus on health economics and outcome research, the generalizability of learning algorithms to another domain will benefit from formal examination.
Extensive studies have shown the superiority of transformer-based deep learning models for many NLP tasks [ 11 , 13 , 14 , 15 , 16 ]. Based on our experiments, however, adding features to the pre-trained language models that have not seen these features before may not significantly boost their performance. It would be interesting to find a better way of encoding additional features to these pre-trained language models to maximize their performance. In addition, transfer learning has proven to be an effective technique to improve the performance on a target task by leveraging annotation data from a source task [ 17 , 18 , 19 ]. Thus, for a new SLR abstract screening task, it would be worthwhile to investigate the use of transfer learning by adapting our (publicly available) corpora to the new target task.
When labeled data is available, supervised machine learning algorithms can be very effective and efficient for article screening. However, as there is increasing need for explainability and transparency in NLP-assisted SLR workflow, supervised machine learning algorithms are facing challenges in explaining why certain papers fail to fulfill the criteria. The recent advances in large language models (LLMs), such as ChatGPT [ 20 ] and Gemini [ 21 ], show remarkable performance on NLP tasks and good potentials in explainablity. Although there are some concerns on the bias and hallucinations that LLMs could bring, it would be worthwhile to evaluate further how LLMs could be applied to SLR tasks and understand the performance of using LLMs to take free-text article screening criteria as the input and provide explainanation for article screening decisions.
Data availability
The annotated corpora underlying this article are available at https://github.com/Merck/NLP-SLR-corpora .
Bullers K, Howard AM, Hanson A, et al. It takes longer than you think: librarian time spent on systematic review tasks. J Med Libr Assoc. 2018;106:198–207. https://doi.org/10.5195/jmla.2018.323 .
Article PubMed PubMed Central Google Scholar
Carver JC, Hassler E, Hernandes E et al. Identifying Barriers to the Systematic Literature Review Process. In: 2013 ACM / IEEE International Symposium on Empirical Software Engineering and Measurement . 2013. 203–12. https://doi.org/10.1109/ESEM.2013.28 .
Lame G. Systematic literature reviews: an introduction. Proc Des Society: Int Conf Eng Des. 2019;1:1633–42. https://doi.org/10.1017/dsi.2019.169 .
Article Google Scholar
Michelson M, Reuter K. The significant cost of systematic reviews and meta-analyses: a call for greater involvement of machine learning to assess the promise of clinical trials. Contemp Clin Trials Commun. 2019;16:100443. https://doi.org/10.1016/j.conctc.2019.100443 .
Recent advances in. biomedical literature mining | Briefings in Bioinformatics | Oxford Academic. https://academic.oup.com/bib/article/22/3/bbaa057/5838460?login=true (accessed 30 May 2022).
Medical Subject Headings - Home Page. https://www.nlm.nih.gov/mesh/meshhome.html (accessed 30 May 2022).
Chen T, Guestrin C, XGBoost:. A Scalable Tree Boosting System. In: Proceedings of the 22nd ACM SIGKDD International Conference on Knowledge Discovery and Data Mining . New York, NY, USA: Association for Computing Machinery 2016. 785–94. https://doi.org/10.1145/2939672.2939785 .
Noble WS. What is a support vector machine? Nat Biotechnol. 2006;24:1565–7. https://doi.org/10.1038/nbt1206-1565 .
Article CAS PubMed Google Scholar
Logistic Regression . https://doi.org/10.1007/978-1-4419-1742-3 (accessed 30 May 2022).
Random forest classifier for remote sensing classification. International Journal of Remote Sensing: Vol 26, No 1. https://www.tandfonline.com/doi/abs/10.1080/01431160412331269698 (accessed 30 May 2022).
Devlin J, Chang M-W, Lee K, et al. BERT: pre-training of Deep Bidirectional transformers for Language understanding. arXiv. 2019. https://doi.org/10.48550/arXiv.1810.04805 .
Vaswani A, Shazeer N, Parmar N et al. Attention is All you Need. In: Advances in Neural Information Processing Systems . Curran Associates, Inc. 2017. https://proceedings.neurips.cc/paper/2017/hash/3f5ee243547dee91fbd053c1c4a845aa-Abstract.html (accessed 30 May 2022).
BioBERT. a pre-trained biomedical language representation model for biomedical text mining | Bioinformatics | Oxford Academic. https://academic.oup.com/bioinformatics/article/36/4/1234/5566506 (accessed 3 Jun 2020).
Gu Y, Tinn R, Cheng H, et al. Domain-specific Language Model Pretraining for Biomedical Natural Language Processing. ACM Trans Comput Healthc. 2021;3(2):1–2. https://doi.org/10.1145/3458754 .
Article CAS Google Scholar
Chen Q, Du J, Allot A, et al. LitMC-BERT: transformer-based multi-label classification of biomedical literature with an application on COVID-19 literature curation. arXiv. 2022. https://doi.org/10.48550/arXiv.2204.08649 .
Chen Q, Allot A, Leaman R, et al. Multi-label classification for biomedical literature: an overview of the BioCreative VII LitCovid Track for COVID-19 literature topic annotations. arXiv. 2022. https://doi.org/10.48550/arXiv.2204.09781 .
Kermany DS, Goldbaum M, Cai W, et al. Identifying Medical diagnoses and Treatable diseases by Image-based deep learning. Cell. 2018;172:1122–e11319. https://doi.org/10.1016/j.cell.2018.02.010 .
Howard J, Ruder S. Universal Language Model fine-tuning for text classification. arXiv. 2018. https://doi.org/10.48550/arXiv.1801.06146 .
Do CB, Ng AY. Transfer learning for text classification. In: Advances in Neural Information Processing Systems . MIT Press. 2005. https://proceedings.neurips.cc/paper/2005/hash/bf2fb7d1825a1df3ca308ad0bf48591e-Abstract.html (accessed 30 May 2022).
Achiam J et al. Gpt-4 technical report. arXiv preprint arXiv:2303.08774 (2023).
https:// gemini.google.com/app/a4dcd2e2d7672354 . (accessed 01 Feb 2024).
Download references
Acknowledgements
We thank Dr. Majid Rastegar-Mojarad for conducting some additional experiments during revision.
This research was supported by Merck Sharp & Dohme LLC, a subsidiary of Merck & Co., Inc., Rahway, NJ, USA.
Author information
Authors and affiliations.
Intelligent Medical Objects, Houston, TX, USA
Jingcheng Du, Ekin Soysal, Long He, Bin Lin, Jingqi Wang & Frank J. Manion
Merck & Co., Inc, Rahway, NJ, USA
Dong Wang, Yeran Li, Elise Wu & Lixia Yao
McWilliams School of Biomedical Informatics, University of Texas Health Science Center at Houston, Houston, TX, USA
Ekin Soysal
You can also search for this author in PubMed Google Scholar
Contributions
Study concept and design: JD and LY Corpus preparation: DW, YL and LY Experiments: JD and ES Draft of the manuscript: JD, DW, FJM and LY Acquisition, analysis, or interpretation of data: JD, ES, DW and LY Critical revision of the manuscript for important intellectual content: JD, ES, DW, LH, BL, JW, FJM, YL, EW, LY Study supervision: LY.
Corresponding author
Correspondence to Lixia Yao .
Ethics declarations
Disclaimers.
The content is the sole responsibility of the authors and does not necessarily represent the official views of Merck & Co., Inc., Rahway, NJ, USA or Intelligent Medical Objects.
Ethics approval and consent to participate
Not applicable.
Consent for publication
Competing interests.
DW is an employee of Merck Sharp & Dohme LLC, a subsidiary of Merck & Co., Inc., Rahway, NJ, USA. EW, YL, and LY were employees of Merck Sharp & Dohme LLC, a subsidiary of Merck & Co., Inc., Rahway, NJ, USA for this work. JD, LH, JW, and FJM are employees of Intelligent Medical Objects. ES was an employee of Intelligent Medical Objects during his contributions, and is currently an employee of EBSCO Information Services. All the other authors declare no competing interest.
Additional information
Publisher’s note.
Springer Nature remains neutral with regard to jurisdictional claims in published maps and institutional affiliations.
Rights and permissions
Open Access This article is licensed under a Creative Commons Attribution 4.0 International License, which permits use, sharing, adaptation, distribution and reproduction in any medium or format, as long as you give appropriate credit to the original author(s) and the source, provide a link to the Creative Commons licence, and indicate if changes were made. The images or other third party material in this article are included in the article’s Creative Commons licence, unless indicated otherwise in a credit line to the material. If material is not included in the article’s Creative Commons licence and your intended use is not permitted by statutory regulation or exceeds the permitted use, you will need to obtain permission directly from the copyright holder. To view a copy of this licence, visit http://creativecommons.org/licenses/by/4.0/ . The Creative Commons Public Domain Dedication waiver ( http://creativecommons.org/publicdomain/zero/1.0/ ) applies to the data made available in this article, unless otherwise stated in a credit line to the data.
Reprints and permissions
About this article
Cite this article.
Du, J., Soysal, E., Wang, D. et al. Machine learning models for abstract screening task - A systematic literature review application for health economics and outcome research. BMC Med Res Methodol 24 , 108 (2024). https://doi.org/10.1186/s12874-024-02224-3
Download citation
Received : 19 May 2023
Accepted : 18 April 2024
Published : 09 May 2024
DOI : https://doi.org/10.1186/s12874-024-02224-3
Share this article
Anyone you share the following link with will be able to read this content:
Sorry, a shareable link is not currently available for this article.
Provided by the Springer Nature SharedIt content-sharing initiative
- Machine learning
- Deep learning
- Text classification
- Article screening
- Systematic literature review
BMC Medical Research Methodology
ISSN: 1471-2288
- General enquiries: [email protected]
Advertisement
A detailed review on word embedding techniques with emphasis on word2vec
- Published: 03 October 2023
- Volume 83 , pages 37979–38007, ( 2024 )
Cite this article
- S. Joshua Johnson 1 ,
- M. Ramakrishna Murty 1 &
- I. Navakanth 2
807 Accesses
3 Citations
Explore all metrics
Text data has been growing drastically in the present day because of digitalization. The Internet, being flooded with millions of documents every day, makes the task of text processing by human beings relatively complex, which is neither adaptable nor successful. Many machine learning algorithms cannot interpret the raw text in its original format, as these algorithms purely need numbers as inputs to accomplish any task (say, classification, regression). A better way to represent text for computers, to understand and process text efficiently and effectively is needed. Word embedding is one such technique. Word embedding, or the encoding of words as vectors, has received much interest as a feature learning technique for natural language processing in recent times. This review presents a better way of understanding and working with word embeddings. Many researchers, who are non-experts in using different text processing techniques, would not know where to start their exploration due to a lack of comprehensive material. This review provides an overview of several word embedding strategies and the entire working procedure of word2vec,both in theory and mathematical perspectives which provides researchers with detailed information so that they may rapidly get to work on their research. Research results of standard word embedding techniques have also been included to better understand how word embedding have been improved from the past years to most recent findings.
This is a preview of subscription content, log in via an institution to check access.
Access this article
Price includes VAT (Russian Federation)
Instant access to the full article PDF.
Rent this article via DeepDyve
Institutional subscriptions
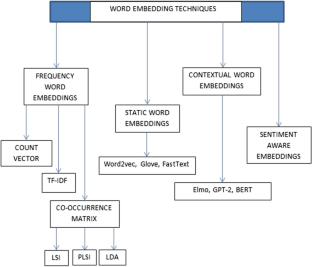
Similar content being viewed by others
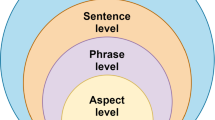
A survey on sentiment analysis methods, applications, and challenges
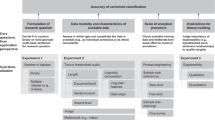
Sentiment Analysis in the Age of Generative AI
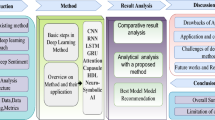
"Challenges and future in deep learning for sentiment analysis: a comprehensive review and a proposed novel hybrid approach"
https://www.analyticsvidhya.com/blog/2017/06/word-embeddings-count-word2veec/
Mcdonald, Scott. (2008). Testing the distributional hypothesis: The influence of context on judgements of semantic similarity.
Hillebrand L, Biesner D, Bauckhage C, Sifa R (2021) Interpretable Topic Extraction and Word Embedding Learning Using Non-Negative Tensor DEDICOM. Mach Learn Knowl Extraction 3(1):123–167. https://doi.org/10.3390/make3010007
Article Google Scholar
Levy O, Goldberg Y (2014) Neural word embedding as implicit matrix factorization. In: Proceedings of the 27th international conference on neural information processing systems, vol 2, no. December, pp 2177–2185. https://doi.org/10.5555/2969033.2969070
Pennington, J.; Socher, R.; Manning, C. Glove: Global Vectors for Word Representation. In Proceedings of the 2014 Conference on Empirical Methods in Natural Language Processing (EMNLP), Association for Computational Linguistics, Doha, Qatar, 25–29 October 2014. 1532–1543.
Deerwester S, Dumias ST, Furmas GW, Lander TK, Harshman R (1990) Indexing by latent semantic analysis. J Am Soc Inf Sci 41(6):391–407 (https://asistdl.onlinelibrary.wiley.com/doi/abs/10.1002/(SICI)1097-4571(199009)41:63.0.CO;2-9)
Hofmann T (1999) Probabilistic latent semantic analysis. In:Thomas H (ed) Probabilistic latent semantic analysis. Proceedings of the Fifteenth conference on Uncertainty in artificial intelligence. Morgan Kaufmann Publisher Inc. 289–296
https://medium.com/nanonets/topic-modeling-with-lsa-psla-lda-and-lda2vec-555ff65b0b05
Blei DM, Ng AY, Jordan MI (2003) Latent dirichlet allocation. J Mach Learn Res 3:993–1022
Google Scholar
Lebret R, Collobert R (2014) Word embeddings through Hellinger PCA. In: 14th Conference of the european chapter of the association for computational linguistics 2014, EACL 2014, 482–490
Pennington J, Socher R, Manning C (2014) Glove: global vectors for word representation. In: Proceedings of the 2014 conference on empirical methods in natural language processing (EMNLP), pp 1532–1543
Efficient Estimation of Word Representations in Vector Space Tomas Mikolov Google Inc., Mountain View, CA [email protected] Kai Chen Google Inc., Mountain View, CA [email protected] Greg Corrado Google Inc., Mountain View, CA [email protected] Jeffrey Dean Google Inc., Mountain View, CA [email protected] arXiv:1301.3781v3 [cs.CL] 7 Sep 2013
arXiv:1411.2738v4 [cs.CL] 5 Jun 2016word2vec Parameter Learning Explained Xin Rong [email protected]
Huang EH, Socher R, Manning CD, Ng AY (2012) Improve Word Representation via Global Context and Multiple Word prototypes. In: Proceedings of the 50th annual meeting of the association for computational linguistics no. July.JejuIslan: Korea: Association for Computational Linguistics, 873–882
Sen P, Ganguly D, Jones G (2019) Word-Node2Vec: improving word embedding with document-level non-local word cooccurrences. In: Proceedings of the 2019 conference of the north american chapter of the association for computational linguistics human language technologies, Volume 1 (Long and Short Papers), pp 1041–1051. [Online]. Available: https://www.aclweb.org/anthology/N19–1109
Reisinger J, Mooney RJ (2010) Multi-prototype vector-space models of word meaning. In: NAACL HLT 2010 - human language technologies: the 2010 annual conference of the north american chapter of the association for computational linguistics, proceedings of the main conference, no June, 109–117
Wu Z, Giles CL (2015) Sense-aware semantic analysis: A multi-prototype word representation model using wikipedia. Proc Nat Conf Artif Intell 3:2188–2194
Neelakantan A, Shankar J, Passos A, McCallum A (2014) Efficient non-parametric estimation of multiple embeddings perword in vector space. In: EMNLP 2014 - 2014 Conference on empirical methods in natural language processing, proceedings of the conference, 1059–1069
Li J, Jurafsky D (2015) Do multi-sense embeddings improve natural language understanding? In: Conference proceedings - EMNLP 2015: conference on empirical methods in natural language processing no. September 1722–1732
Chen X, Liu Z, Sun M (2014) A unified model for word sense representation and disambiguation. In: EMNLP 2014 - 2014 Conference on empirical methods in natural language processing, proceedings of the conference, 1025–1035
Tian F, Dai H, Bian J, Gao B, Zhang R, Chen E, Liu TY (2014) A probabilistic model for learning multi-prototypeword embeddings. In: COLING 2014 - 25th International conference on computational linguistics, proceedings of COLING 2014: technical papers, 151–160
Peters M, Neumann M, Iyyer M, Gardner M, Clark C, Lee K,Zettlemoyer L (2018) Deep contextualized word representations.In: Proceedings of the 2018 conference of the north American chapter of the association for computational linguistics: humanlanguage, Vol. 1 (Long Papers) Technologies. New Orleans,Louisiana: Association for Computational Linguistics, pp 2227–2237. [Online]. Available: https://www.aclweb.org/anthology/N18-1202
Devlin J, Chang M-W, Lee K, Toutanova K (2019) BERT: pretraining of deep bidirectional transformers for language understanding. In: Proceedings of the 2019 conference of the north american chapter of the association for computational linguistics: human language technologies. Minneapolis, Minnesota: Association for Computational Linguistics, pp 4171–4186. [Online]. Available: https://www.aclweb.org/anthology/N19-1423
Yang Z, Dai Z, Yang Y, Carbonell J, Salakhutdinov R, Le QV (2019) XLNet: generalized autoregressive pretraining for language understanding. In: Advances in neural information processing systems (NIPS 2019), pre-proceedings. Curran Associates, pp 5754–5764.[Online]. Available: http://papers.nips.cc/paper/8812-xlnet-generalized-autoregressive -pretr aining-for-language-understanding.pdf
Maas AL, Daly RE, Pham PT, Huang D, Ng AY, Potts C (2011) Learning word vectors for sentiment analysis. In: Proceedings of the 49th annual meeting of the association for computational linguistics (ACL-2011), no. February, pp 142–150. [Online]. Available: https://www.aclweb.org/anthology/P11-1015
Mikolov T, Chen K, Corrado G, Dean J (2013) Distributed representations of words and phrases and their compositionality. In: In Advances in neural information processing systems, 3111–3119
Zhang Z, Lan M (2016) Learning sentiment-inherent word embedding for word-level and sentence-level sentiment analysis. In: Proceedings of 2015 international conference on asian language processing, IALP 2015, 1: 94–97
Yu L-C, Wang J, Lai KR, Zhang X (2017) Refining word embeddings for sentiment analysis. In: Proceedings of the 2017 conference on empirical methods in natural language processing Copenhagen, pp 534–539. [Online]. Available: https://www.aclweb.org/anthology/D17–1056
Rezaeinia SM, Rahmani R, Ghodsi A, Veisi H (2019) Sentiment analysis based on improved pre-trained word embeddings. Expert SystAppl 117:139–147. https://doi.org/10.1016/j.eswa.2018.08.044
Rahimi Z, Homayounpour MM (2021) TensSent: a tensor based sentimental word embedding method. ApplIntell. https://doi.org/10.1007/s10489-020-02163-8
Using Latent Semantic Indexing to Filter Spam Kevin R. Gee Dept. of Computer Science and Engineering The University of Texas at Arlington Arlington, TX 76019 [email protected]
K. Weinberger, A. Dasgupta, J. Langford, A. Smola, and J. Attenberg, “Feature hashing for large scale multitask learning,” in Proceedings of the 26th Annual International Conference on Machine Learning. ACM, 2009, 1113–1120.
G. Salton and M. McGill, editors.Introduction to Modern Information Retrieval.McGraw-Hill, 1983.
https://www.analyticsvidhya.com/blog/2020/02/quick-introduction-bag-of-words-bow-tf-idf/
Golub G, Reinsch C (1970) Singular value decomposition and least squares solutions. NumerischeMathematik 14(5):403–420
Comparative analysis of word embeddings for capturing word similarities , Martina Toshevska, Frosina Stojanovska and Jovan Kalajdjieski Faculty of Computer Science and Engineering, Ss. Cy.
Liu Z, Li M, Liu Y, Ponraj M (2011) Performance evaluation of Latent Dirichlet Allocation in text mining. Eighth International Conference on Fuzzy Systems and Knowledge Discovery (FSKD) 2011:2695–2698. https://doi.org/10.1109/FSKD.2011.6020066
T. Mikolov, W.T. Yih, G. Zweig. Linguistic Regularities in Continuous Space Word Representations. NAACL HLT 2013.
Tshitoyan V, Dagdelen J, Weston L et al (2019) Unsupervised word embeddings capture latent knowledge from materials science literature. Nature 571:95–98. https://doi.org/10.1038/s41586-019-1335-8
Download references
Author information
Authors and affiliations.
CSE, Anil Neerukonda Institute of Technology and Sciences(Autonomous), Visakhapatnam, India
S. Joshua Johnson & M. Ramakrishna Murty
CSE, Maturi Venkata Subba Rao (MVSR) Engineering College(Autonomous), Hyderabad, India
I. Navakanth
You can also search for this author in PubMed Google Scholar
Ethics declarations
Conflict of interest.
The authors certify that there is no conflict of interest in the subject matter discussed in this manuscript.
Additional information
Publisher's note.
Springer Nature remains neutral with regard to jurisdictional claims in published maps and institutional affiliations.
Rights and permissions
Springer Nature or its licensor (e.g. a society or other partner) holds exclusive rights to this article under a publishing agreement with the author(s) or other rightsholder(s); author self-archiving of the accepted manuscript version of this article is solely governed by the terms of such publishing agreement and applicable law.
Reprints and permissions
About this article
Johnson, S.J., Murty, M.R. & Navakanth, I. A detailed review on word embedding techniques with emphasis on word2vec. Multimed Tools Appl 83 , 37979–38007 (2024). https://doi.org/10.1007/s11042-023-17007-z
Download citation
Received : 29 March 2022
Revised : 19 September 2022
Accepted : 11 September 2023
Published : 03 October 2023
Issue Date : April 2024
DOI : https://doi.org/10.1007/s11042-023-17007-z
Share this article
Anyone you share the following link with will be able to read this content:
Sorry, a shareable link is not currently available for this article.
Provided by the Springer Nature SharedIt content-sharing initiative
- Word Embeddings
- Neural Networks
- Find a journal
- Publish with us
- Track your research

IMAGES
VIDEO
COMMENTS
Literature Review and Research Design by Dave Harris This book looks at literature review in the process of research design, and how to develop a research practice that will build skills in reading and writing about research literature--skills that remain valuable in both academic and professional careers. Literature review is approached as a process of engaging with the discourse of scholarly ...
Examples of literature reviews. Step 1 - Search for relevant literature. Step 2 - Evaluate and select sources. Step 3 - Identify themes, debates, and gaps. Step 4 - Outline your literature review's structure. Step 5 - Write your literature review.
In writing the literature review, your purpose is to convey to your reader what knowledge and ideas have been established on a topic, and what their strengths and weaknesses are. As a piece of writing, the literature review must be defined by a guiding concept (e.g., your research objective, the problem or issue you are discussing, or your ...
A literature review is a document or section of a document that collects key sources on a topic and discusses those sources in conversation with ... A literature review can be a part of a research paper or scholarly article, usually falling after the introduction and before the research methods sections. ... More info on the research process is ...
A literature review is a survey of scholarly sources on a specific topic. It provides an overview of current knowledge, allowing you to identify relevant theories, methods, and gaps in the existing research. There are five key steps to writing a literature review: Search for relevant literature. Evaluate sources. Identify themes, debates and gaps.
A systematic review can be explained as a research method and process for identifying and critically appraising relevant research, as well as for collecting and analyzing data from said research (Liberati et al., 2009). The aim of a systematic review is to identify all empirical evidence that fits the pre-specified inclusion criteria to answer ...
A literature review is important because it: Explains the background of research on a topic. Demonstrates why a topic is significant to a subject area. Discovers relationships between research studies/ideas. Identifies major themes, concepts, and researchers on a topic. Identifies critical gaps and points of disagreement.
A literature review is a review and synthesis of existing research on a topic or research question. A literature review is meant to analyze the scholarly literature, make connections across writings and identify strengths, weaknesses, trends, and missing conversations. A literature review should address different aspects of a topic as it ...
Example: Predictors and Outcomes of U.S. Quality Maternity Leave: A Review and Conceptual Framework: 10.1177/08948453211037398 ; Systematic review: "The authors of a systematic review use a specific procedure to search the research literature, select the studies to include in their review, and critically evaluate the studies they find." (p. 139).
A literature search is a systematic search of the scholarly sources in a particular discipline. A literature review is the analysis, critical evaluation and synthesis of the results of that search. During this process you will move from a review of the literature to a review for your research. Your synthesis of the literature is your unique ...
From each study, we extracted information on the following two subtopics: (1) the definition, typology, and purpose of literature review and (2) the literature review process. The literature review process is further broken down into subtopics on formulating the research problem, developing and validating the review protocol, searching the ...
This book looks at literature review in the process of research design, and how to develop a research practice that will build skills in reading and writing about research literature--skills that remain valuable in both academic and professional careers. Literature review is approached as a process of engaging with the discourse of scholarly ...
A formal literature review is an evidence-based, in-depth analysis of a subject. There are many reasons for writing one and these will influence the length and style of your review, but in essence a literature review is a critical appraisal of the current collective knowledge on a subject. Rather than just being an exhaustive list of all that ...
Literature search. Fink has defined research literature review as a "systematic, explicit and reproducible method for identifying, evaluating, and synthesizing the existing body of completed and recorded work produced by researchers, scholars and practitioners."[]Review of research literature can be summarized into a seven step process: (i) Selecting research questions/purpose of the ...
A literature review is a critical analysis and synthesis of existing research on a particular topic. It provides an overview of the current state of knowledge, identifies gaps, and highlights key findings in the literature. 1 The purpose of a literature review is to situate your own research within the context of existing scholarship ...
Systematic vs. Literature Review Systematic Review Literature Review Definition High-level overview of primary research on a focused question that identifies, selects, synthesizes, and appraises all high quality research evidence relevant to that question Qualitatively summarizes evidence on a topic using informal or
Establish your research questions and organize your literature into logical categories around the subject/ topic areas of your questions. Your research questions must be specific enough to guide you to the relevant literature. Make sure you understand the concept of 'broader' and 'narrower' terms. The narrower your topic, the easier it ...
The literature review is not a comprehensive history of your topic, but a way to provide context to your reader about research that has preceded your study. Be aware that the literature review is an iterative process.
The literature review is a vital part of medical education research and should occur throughout the research process to help researchers design a strong study and effectively communicate study results and importance. To achieve these goals, researchers are advised to plan and execute the literature review carefully.
This article is a practical guide to conducting data analysis in general literature reviews. The general literature review is a synthesis and analysis of published research on a relevant clinical issue, and is a common format for academic theses at the bachelor's and master's levels in nursing, physiotherapy, occupational therapy, public health and other related fields.
9.3. Types of Review Articles and Brief Illustrations. EHealth researchers have at their disposal a number of approaches and methods for making sense out of existing literature, all with the purpose of casting current research findings into historical contexts or explaining contradictions that might exist among a set of primary research studies conducted on a particular topic.
APA's clinical practice guidelines (CPGs) and professional practice guidelines (PPGs) are the result of a complex and lively process of decision-making, interdisciplinary discussion, literature review, public comment, APA approval, and more. Here's a quick rundown of how they come together: Clinical practice guidelines
Methods: We conducted a scoping literature review following the PRISMA (Preferred Reporting Items for Systematic Reviews and Meta-Analyses) methodology. We searched databases (PubMed, Embase, and Cochrane Library), gray literature, and HTA agency websites. Results: A total of 10.1% (78/775) of the references were included.
Background research on process intensification in the food industry for sustainable food process operations offers insightful and useful methodologies to maximise resource utilisation, minimise waste, improve product quality, and guarantee the long-term sustainability of food processing operations (Sharma et al., 2020). This narrative review ...
Systematic literature reviews (SLRs) are critical for life-science research. However, the manual selection and retrieval of relevant publications can be a time-consuming process. This study aims to (1) develop two disease-specific annotated corpora, one for human papillomavirus (HPV) associated diseases and the other for pneumococcal-associated pediatric diseases (PAPD), and (2) optimize ...
Background. Systematic literature searching is recognised as a critical component of the systematic review process. It involves a systematic search for studies and aims for a transparent report of study identification, leaving readers clear about what was done to identify studies, and how the findings of the review are situated in the relevant evidence.
We performed a systematic literature review following the methodology described in the PRISMA framework, to provide a novel comprehensive and systematic overview of what is present in the literature regarding the obstacles stakeholders perceive with regards to agroforestry adoption, gathered through participatory research methods, which are ...
Research on interactive alignment encompasses various perspectives, including the recognition of linguistic coordination patterns, mental representation processes between interlocutors, and linguistic and behavioral convergence. In the absence of a literature review that presents the advances in the study of interactive alignment, this study identified 64 theoretical and empirical articles ...
Aim of this research is to review the advanced techniques made available to generate high-quality word vectors for efficient word embedding generation. Previously, feature engineering in Natural Language Processing (NLP) required the creation of appropriate numerical functions to capture significant textual properties like the noun-to-pronoun ...