Functional annotation of protein sequences
Overview Questions: How to perform functional annotation on protein sequences? Objectives: Perform functional annotation using EggNOG-mapper and InterProScan Requirements: Introduction to Galaxy Analyses Time estimation: 1 hour Level: Introductory Introductory Supporting Materials: Datasets Workflows FAQs instances Available on these Galaxies Known Working UseGalaxy.eu ✅ ⭐️ UseGalaxy.org (Main) ✅ ⭐️ UseGalaxy.org.au ✅ ⭐️ UseGalaxy.cz ✅ UseGalaxy.fr ✅ Possibly Working UseGalaxy.no Containers docker_image Docker image Published: Jul 20, 2022 Last modification: Jan 8, 2024 License: Tutorial Content is licensed under Creative Commons Attribution 4.0 International License . The GTN Framework is licensed under MIT purl PURL : https://gxy.io/GTN:T00173 version Revision: 17
When performing the structural annotation of a genome sequence, you get the position of each gene, but you don’t have information about their name of their function. That’s the goal of functional annotation .
In this short tutorial, we will run the most commonly used tools to perform functional annotation, starting from the predicted protein sequences of a few example genes.
For a more complete view of how this step integrates into a whole genome sequencing and annotation process, you can have a look at the Funannotate tutorial .
Agenda In this tutorial, we will cover: Data upload Functional annotation EggNOG Mapper InterProScan Conclusion

Data upload
We will annotate a small set of protein sequences . These sequences were predicted from the gene structures obtained in the Funannotate tutorial ? Though these sequences from from a fungal species, you can run the same tools on proteins from any organisms, including prokaryotes.
Hands-on: Data upload Create a new history for this tutorial Tip: Creating a new history Click the new-history icon at the top of the history panel:
Import the files from Zenodo or from the shared data library ( GTN - Material -> genome-annotation -> Functional annotation of protein sequences ):
Tip: Importing via links Copy the link location Click galaxy-upload Upload Data at the top of the tool panel Select galaxy-wf-edit Paste/Fetch Data Paste the link(s) into the text field Press Start Close the window
Tip: Importing data from a data library As an alternative to uploading the data from a URL or your computer, the files may also have been made available from a shared data library : Go into Shared data (top panel) then Data libraries Navigate to the correct folder as indicated by your instructor. On most Galaxies tutorial data will be provided in a folder named GTN - Material –> Topic Name -> Tutorial Name . Select the desired files Click on Add to History galaxy-dropdown near the top and select as Datasets from the dropdown menu In the pop-up window, choose “Select history” : the history you want to import the data to (or create a new one) Click on Import
Functional annotation
Eggnog mapper.
EggNOG Mapper compares each protein sequence of the annotation to a huge set of ortholog groups from the EggNOG database . In this database, each ortholog group is associated with functional annotation like Gene Ontology (GO) terms or KEGG pathways . When the protein sequence of a new gene is found to be very similar to one of these ortholog groups, the corresponding functional annotation is transfered to this new gene.
Hands-on eggNOG Mapper ( Galaxy version 2.1.8+galaxy3) with the following parameters: param-file “Fasta sequences to annotate” : proteins.fasta (Input dataset) “Version of eggNOG Database” : select the latest version available In “Output Options” : “Exclude header lines and stats from output files” : No
The output of this tool is a tabular file, where each line represents a gene from our annotation, with the functional annotation that was found by EggNOG-mapper. It includes a predicted protein name, GO terms, EC numbers, KEGG identifiers, …
Display the file and explore which kind of identifiers were found by EggNOG Mapper.
InterProScan
InterPro is a huge integrated database of protein families. Each family is characterized by one or muliple signatures (i.e. sequence motifs) that are specific to the protein family, and corresponding functional annotation like protein names or Gene Ontology (GO) . A good proportion of the signatures are manually curated, which means they are of very good quality.
InterProScan is a tool that analyses each protein sequence from our annotation to determine if they contain one or several of the signatures from InterPro. When a protein contains a known signature, the corresponding functional annotation will be assigned to it by InterProScan .
InterProScan itself runs multiple applications to search for the signatures in the protein sequences. It is possible to select exactly which ones we want to use when launching the analysis (by default all will be run).
Hands-on InterProScan ( Galaxy version 5.59-91.0+galaxy3) with the following parameters: param-file “Protein FASTA File” : proteins.fasta (Input dataset) “InterProScan database” : select the latest version available “Use applications with restricted license, only for non-commercial use?” : Yes (set it to No if you run InterProScan for commercial use) “Output format” : Tab-separated values format (TSV) and XML
Comment To speed up the processing by InterProScan during this tutorial, you can disable Pfam and PANTHER applications. When analysing real data, it is adviced to keep them enabled. When some applications are disabled, you will of course miss the corresponding results in the output of InterProScan .
The output of this tool is both a tabular file and an XML file. Both contain the same information, but the tabular one is more readable for a Human: each line represents a gene from our annotation, with the different domains and motifs that were found by InterProScan.
If you display the TSV file you should see something like this:

Each line correspond to a motif found in one of the annotated proteins. The most interesting columns are:
- Column 1: the protein identifier
- Column 5: the identifier of the signature that was found in the protein sequence
- Column 4: the databank where this signature comes from (InterProScan regroups several motifs databanks)
- Column 6: the human readable description of the motif
- Columns 7 and 8: the position where the motif was found
- Column 9: a score for the match (if available)
- Column 12 and 13: identifier of the signature integrated in InterPro (if available). Have a look an example webpage for IPR036859 on InterPro.
- The following columns contains various identifiers that were assigned to the protein based on the match with the signature (Gene ontology term, Reactome, …)
The XML output file contains the same information in a computer-friendly format, we will use it in the next step.
Congratulations for reaching the end of this tutorial! Now you know how to perform the functional annotation of a set of protein sequences, using EggNOG mapper and InterProScan.
If you want to collect more functional annotation, you can try to run the NCBI BLAST+ blastp ( Galaxy version 2.10.1+galaxy2) or Diamond ( Galaxy version 2.0.15+galaxy0) tools against the UniProt or NR databases (Diamond runs much faster on big datasets). These tools will search for similarities between your protein sequences and the ones already described in big international databases.
Also note that many other more specialised tools exist to collect even more functional annotation, in particular for certain species (prokaryotes forexample), or enzyme/protein families.
You've Finished the Tutorial
Please also consider filling out the Feedback Form as well!
Key points EggNOG Mapper compares sequences to a database of annotated orthologous sequences InterProScan detects known motifs in protein sequences
Frequently Asked Questions
Did you use this material as an instructor? Feel free to give us feedback on how it went . Did you use this material as a learner or student? Click the form below to leave feedback.
Citing this Tutorial
- Anthony Bretaudeau, Functional annotation of protein sequences (Galaxy Training Materials) . https://training.galaxyproject.org/training-material/topics/genome-annotation/tutorials/functional/tutorial.html Online; accessed TODAY
- Hiltemann, Saskia, Rasche, Helena et al., 2023 Galaxy Training: A Powerful Framework for Teaching! PLOS Computational Biology 10.1371/journal.pcbi.1010752
- Batut et al., 2018 Community-Driven Data Analysis Training for Biology Cell Systems 10.1016/j.cels.2018.05.012
BibTeX @misc{genome-annotation-functional, author = "Anthony Bretaudeau", title = "Functional annotation of protein sequences (Galaxy Training Materials)", year = "", month = "", day = "" url = "\url{https://training.galaxyproject.org/training-material/topics/genome-annotation/tutorials/functional/tutorial.html}", note = "[Online; accessed TODAY]" } @article{Hiltemann_2023, doi = {10.1371/journal.pcbi.1010752}, url = {https://doi.org/10.1371%2Fjournal.pcbi.1010752}, year = 2023, month = {jan}, publisher = {Public Library of Science ({PLoS})}, volume = {19}, number = {1}, pages = {e1010752}, author = {Saskia Hiltemann and Helena Rasche and Simon Gladman and Hans-Rudolf Hotz and Delphine Larivi{\`{e}}re and Daniel Blankenberg and Pratik D. Jagtap and Thomas Wollmann and Anthony Bretaudeau and Nadia Gou{\'{e}} and Timothy J. Griffin and Coline Royaux and Yvan Le Bras and Subina Mehta and Anna Syme and Frederik Coppens and Bert Droesbeke and Nicola Soranzo and Wendi Bacon and Fotis Psomopoulos and Crist{\'{o}}bal Gallardo-Alba and John Davis and Melanie Christine Föll and Matthias Fahrner and Maria A. Doyle and Beatriz Serrano-Solano and Anne Claire Fouilloux and Peter van Heusden and Wolfgang Maier and Dave Clements and Florian Heyl and Björn Grüning and B{\'{e}}r{\'{e}}nice Batut and}, editor = {Francis Ouellette}, title = {Galaxy Training: A powerful framework for teaching!}, journal = {PLoS Comput Biol} Computational Biology} }
These individuals or organisations provided funding support for the development of this resource
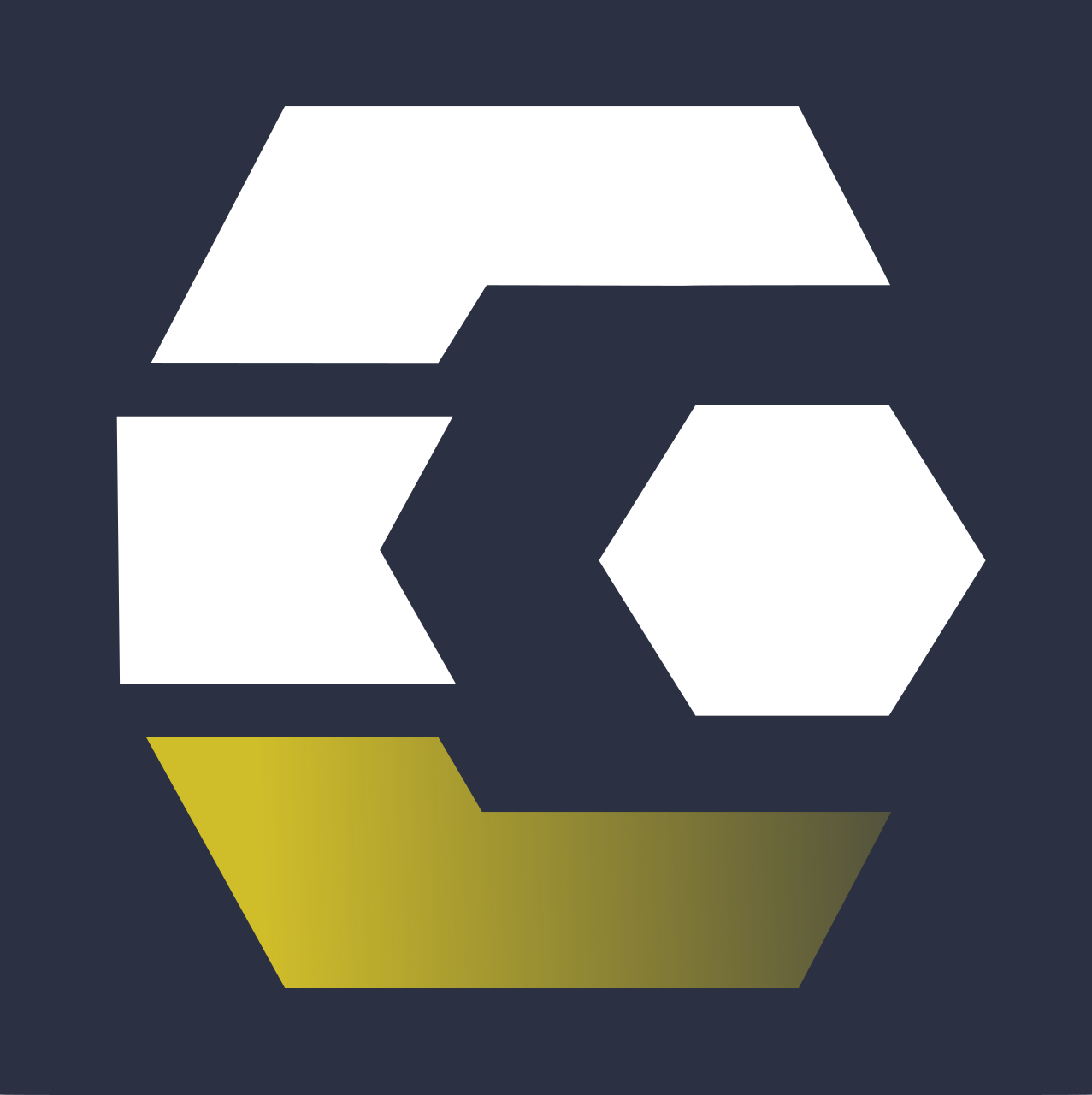
Galaxy Administrators: Install the missing tools You can use Ephemeris's shed-tools install command to install the tools used in this tutorial. shed-tools install [-g GALAXY] [-a API_KEY] -t <(curl https://training.galaxyproject.org/training-material/api/topics/genome-annotation/tutorials/functional/tutorial.json | jq .admin_install_yaml -r) Alternatively you can copy and paste the following YAML --- install_tool_dependencies: true install_repository_dependencies: true install_resolver_dependencies: true tools: - name: diamond owner: bgruening revisions: e8ac2b53f262 tool_panel_section_label: NCBI Blast tool_shed_url: https://toolshed.g2.bx.psu.edu/ - name: interproscan owner: bgruening revisions: 74810db257cc tool_panel_section_label: Annotation tool_shed_url: https://toolshed.g2.bx.psu.edu/ - name: ncbi_blast_plus owner: devteam revisions: 0e3cf9594bb7 tool_panel_section_label: NCBI Blast tool_shed_url: https://toolshed.g2.bx.psu.edu/ - name: eggnog_mapper owner: galaxyp revisions: 844fa988236b tool_panel_section_label: Proteomics tool_shed_url: https://toolshed.g2.bx.psu.edu/
Functional annotation and pathway analysis
Articles (1 in this collection), survconvmixer: robust and interpretable cancer survival prediction based on convmixer using pathway-level gene expression images, authors (first, second and last of 4).
- Yuanning Liu
- Content type: Research
- Open Access
- Published: 27 March 2024
- Article: 133
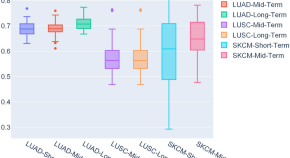
Participating journals
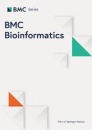
BMC Bioinformatics
- Find a journal
- Publish with us
- Track your research

An official website of the United States government
The .gov means it’s official. Federal government websites often end in .gov or .mil. Before sharing sensitive information, make sure you’re on a federal government site.
The site is secure. The https:// ensures that you are connecting to the official website and that any information you provide is encrypted and transmitted securely.
- Publications
- Account settings
Preview improvements coming to the PMC website in October 2024. Learn More or Try it out now .
- Advanced Search
- Journal List
- Plant Physiol
- v.135(2); 2004 Jun

Functional Annotation of the Arabidopsis Genome Using Controlled Vocabularies 1
Controlled vocabularies are increasingly used by databases to describe genes and gene products because they facilitate identification of similar genes within an organism or among different organisms. One of The Arabidopsis Information Resource's goals is to associate all Arabidopsis genes with terms developed by the Gene Ontology Consortium that describe the molecular function, biological process, and subcellular location of a gene product. We have also developed terms describing Arabidopsis anatomy and developmental stages and use these to annotate published gene expression data. As of March 2004, we used computational and manual annotation methods to make 85,666 annotations representing 26,624 unique loci. We focus on associating genes to controlled vocabulary terms based on experimental data from the literature and use The Arabidopsis Information Resource-developed PubSearch software to facilitate this process. Each annotation is tagged with a combination of evidence codes, evidence descriptions, and references that provide a robust means to assess data quality. Annotation of all Arabidopsis genes will allow quantitative comparisons between sets of genes derived from sources such as microarray experiments. The Arabidopsis annotation data will also facilitate annotation of newly sequenced plant genomes by using sequence similarity to transfer annotations to homologous genes. In addition, complete and up-to-date annotations will make unknown genes easy to identify and target for experimentation. Here, we describe the process of Arabidopsis functional annotation using a variety of data sources and illustrate several ways in which this information can be accessed and used to infer knowledge about Arabidopsis and other plant species.
Arabidopsis is an annual plant of the Brassicaceae family and is commonly found in temperate regions of the world. Its suitability for molecular and genetic experiments has made it one of the most widely studied plants today. It was the first plant genome to be completely sequenced and remains the most completely sequenced eukaryotic genome to date ( Arabidopsis Genome Initiative, 2000 ). Approximately 13,000 researchers around the world are currently engaged in unraveling the functions of this genome and applying the knowledge gained to other plants. When the sequence of the Arabidopsis genome was first reported ( Arabidopsis Genome Initiative, 2000 ), the annotation included a total of 25,498 predicted protein-coding genes. Of these, 69% were classified into nine functional categories using the PEDANT analysis system ( Frishman et al., 2001 ): cellular metabolism, transcription, plant defense, signaling, growth, protein fate, intracellular transport, transport, and protein synthesis. The remaining 30% of gene products could not be assigned to any of these categories. The most recent version of the Arabidopsis genome annotation (The Institute for Genome Research [TIGR] release 5.0) includes 26,207 protein-coding genes and 3,786 pseudogenes ( ftp://ftp.tigr.org/pub/data/a_thaliana/ath1/ ). While computational methods can shed some light on the general categories to which many of these genes belong, experimental approaches are essential to confirm computational predictions and supply the function of genes in cases where no computational prediction is currently possible. Given the large number of uncharacterized genes, experimental characterization of groups of genes, rather than single genes, is essential if significant progress is to be made in the near future. To meet this challenge, the projects initiated under the National Science Foundation 2010 initiative, as well as those supported by other funding agencies such as Deutsche Forschungsgemeinschaft (German Research Foundation), aim to decipher the function of every Arabidopsis gene by the year 2010 ( MASC Committee, 2003 ) by combining high-throughput approaches with domain expertise. About 20,500 unique genes are currently being studied by various functional genomics project investigators ( http://www.arabidopsis.org/info/2010_projects/index.jsp ). The results of this massive experimental effort need to be summarized, stored in an easily accessible manner, and combined with information available from studies of individual genes.
A central goal of The Arabidopsis Information Resource (TAIR) project is to integrate information from various data sources and present the research community with a comprehensive view of each Arabidopsis gene. Functional annotation is defined as the process of collecting information about and describing a gene's biological identity—its various aliases, molecular function, biological role(s), subcellular location, and its expression domains within the plant. At TAIR, we obtain this information from reading the published literature and by soliciting contributions from the research community as well as from computational analyses of the genome sequence. We present the collated information in two ways: (1) in a short summary for each gene that contains its essential attributes and (2) as multiple gene-term associations (or annotations) between a controlled vocabulary term and the gene product. Each annotation is associated with an evidence code, an evidence description, and a reference on which the association is based. We use the Gene Ontology (GO) vocabularies ( www.geneontology.org ; GO Consortium, 2001 ) as well as TAIR's Arabidopsis anatomy and developmental stage ontologies as the sources for the controlled vocabulary terms.
Controlled Vocabularies
A controlled vocabulary is a standardized, restricted set of defined terms designed to reduce ambiguity in describing a concept. For example, one publication might refer to enzyme A as having phytochromobilin synthase activity, while another says that enzyme B has phytochromobilin:ferredoxin oxidoreductase activity. Both enzyme A and enzyme B perform identical functions; the terms describing them are synonymous. Without an explicitly defined standard term, searching for all gene products with this function is difficult and requires knowledge of all possible synonyms.
The GO vocabularies are gaining widespread acceptance within the scientific community as the standard set of terms to use for functional annotation ( Dwight et al., 2002 ; Camon et al., 2003 ; Hazbun et al., 2003 ; Hennig et al., 2003 ; Kanapin et al., 2003 ; King et al., 2003 ; Sprague et al., 2003 ). The terms are organized into three categories that represent molecular functions, biological processes, and subcellular compartments ( GO Consortium, 2001 ). Molecular function terms describe the biochemical activity performed by a gene product (e.g. kinase activity). Biological process terms describe the ordered assembly of more than one molecular function (e.g. flower development). Cellular component terms describe the subcellular compartments of a cell (e.g. nucleus). The terms are used to describe these separate aspects of a gene product's biological identity. The vocabularies are developed and maintained by a consortium of model organism databases (MODs). Curators from the MODs work together to ensure that the terms are uniformly agreed upon, clearly defined, and broadly applicable to a wide taxonomic range of species. As a GO consortium member since 2000, TAIR has been instrumental in modifying and expanding the vocabularies so that they can be used to accurately describe plant genes. The consortium maintains a central database ( http://www.godatabase.org/cgi-bin/go.cgi ) that stores the gene-term associations contributed by its member MODs. Having a central repository for all annotation information allows one to retrieve groups of genes from multiple species that are associated with a single term. There are currently 16,808 terms: 8,181 for biological processes, 7,278 for molecular functions, and 1,379 for cellular components ( http://www.geneontology.org/index.shtml#downloads ).
Most of the terms have explicit definitions, and all of them are arranged in an ontology, a structured hierarchy with defined relationships between terms ( GO Consortium, 2001 ). The term definitions and relationships between terms are intended to reflect the current state of knowledge about a particular term. The terms are organized such that the broader concepts, or parent terms, appear on the top level on the tree structure and are composed of more specific concepts, or child terms. Broader concepts, for example, the term plastid, are used to group more specific concepts, such as amyloplast, chloroplast, chromoplast, and etioplast together. Parent-child relationships are structured such that a child term can be either an instance of or part of a parent term. Thus, a chloroplast is an instance of a plastid, while a plastid is a part of the cytoplasm. Additionally, a child term may have more than one parent term and inherits the characteristics of each parent term. To accommodate instances of multiple parentages, parent-child relationships between terms are represented using a directed acyclic graph (DAG) rather than a simple hierarchy ( Fig. 1 ). In such cases, each parent-child relationship reflects a different aspect of this term's definition. Terms and their relationships with one another are added to, evaluated, and updated on a regular basis to keep pace with the knowledge in that field.

Visualizing controlled vocabularies and DAGs. TAIR's Keyword Browser ( http://www.arabidopsis.org/servlets/Search?action=new_search&type=keyword ) allows users to navigate through the parent-child relationships of the ontologies, look up definitions, and view associated data. Hyperlinks are underlined, and clicking on them will open data pages that list the associated information in greater detail. Section A offers an option to view various data type associated with the term. Section B provides the term name, its identification, and an explicit definition of the term. Section C is a legend for interpreting the icons within the tree structure. Section D allows one to browse any listed ontology other than the one being viewed. Section E illustrates the multiple parentage concept in a DAG using the biological process term germination. In this example, germination is an instance of three different parent terms: cell differentiation, post-embryonic development, and physiological process.
Since the scope of GO does not extend to terms describing supracellular structures and developmental stages, we used the principles underlying the GO ontologies to develop two additional sets of controlled vocabulary terms describing Arabidopsis anatomy and developmental stages that can be used to describe gene expression patterns and mutant phenotypes. Under the auspices of the Plant Ontology Consortium ( www.plantontology.org ), we are collaborating with Gramene, Maize Genetics and Genomics Database (MaizeGDB), the Missouri Botanical Garden, and the University of Missouri (St. Louis) to merge these terms into a common vocabulary that will be used to annotate gene expression and phenotypes of major groups of agriculturally and economically important plants.
Current State of Functional Annotation of the Arabidopsis Genome
Annotation of all Arabidopsis genes to controlled vocabulary terms that describe their biological identity is an ongoing process begun by TAIR in 2002. As of March 2004, we associated a total of 26,624 loci to 1,095 biological process terms, 1,146 molecular function terms, 260 cellular component terms, 120 anatomy terms, and 33 developmental stage terms for a total of 85,666 annotations. Of these, 33,733 annotations to 20,260 loci were manual annotations done by a curator. One gene may have multiple process, function, and/or component annotations, depending on the amount of information available in its associated literature. We have identified approximately 3,600 Arabidopsis genes that have been described in about 6,500 publications obtained from PubMed, Agricola, BIOSIS, and the meeting abstracts of the International Conference on Arabidopsis Research and have assigned at least one GO term to nearly all of these genes. Annotations are made not only to sequenced protein-coding genes and pseudogenes but also to approximately 570 mapped genetic loci where the molecular sequence has not been identified and the only information available pertains to their mutant phenotypes.
We have also used computational methods to generate annotations to a large number of genes, many of which have not been described in the literature. There are currently about 42,500 annotations from INTERPRO2GO mapping, about 11,500 annotations based on TargetP predictions, about 600 from Metacyc2GO mapping, and about 350 from a string matching algorithm. Taking these annotations into account, 20,818 genes (69% of the genome) have at least one GO annotation from TAIR. Upon integration of TIGR's GO annotations, the total number of Arabidopsis genes with at least one GO assignment to a known term increases to 22,570 genes, including protein coding genes, pseudogenes, and genetic loci, covering approximately 75% of the genome ( Table I ). This results in a 6% increase in functional classification since the initial Arabidopsis Genome Initiative genome analysis in 2000, which covered 69% of the genome.
Arabidopsis genome functional annotation statistics as of March 4, 2004
Number of Annotations | Number of Genes Annotated | |
---|---|---|
Functional annotations made by TAIR and TIGR | 121,933 | 28,331 |
Biological process annotations: | ||
Known | 25,955 | 14,621 |
Unknown | 13,241 | 12,853 |
Total annotated | 39,196 | 27,469 |
Unannotated | n/a | 3,713 |
Molecular function annotations: | ||
Known | 36,686 | 16,432 |
Unknown | 11,657 | 11,588 |
Total | 48,343 | 27,959 |
Unannotated | n/a | 3,223 |
Cellular component annotations: | ||
Known | 22,115 | 15,752 |
Unknown | 11,323 | 10,951 |
Total annotated | 33,438 | 26,703 |
Unannotated | n/a | 4,479 |
Functional annotations made by TAIR | 85,666 | 26,624 |
TAIR GO annotations | 84,708 | 30,063 |
TAIR computational annotations to GO | 50,975 | 19,218 |
TAIR manual annotations to GO | 33,733 | 20,260 |
TAIR annotations to anatomy and temporal ontology | 958 | 443 |
TAIR annotations to anatomy ontology | 867 | 423 |
TAIR annotations to temporal ontology | 91 | 76 |
n/a, Not applicable.
Since not all genes, even those that have been described in the literature, have been characterized in detail, curators assign the terms molecular function unknown, cellular component unknown, or biological process unknown to any gene that has been manually inspected and does not have any evidence (in the literature or by computational prediction) to support a known process, function, or subcellular component annotation. For example, a gene that has been shown in expression studies to be involved in the biological process response to pathogen may have an undetermined molecular function or subcellular localization. Annotations to the unknown GO terms are useful for delineating what is unknown about a gene and informs the user that the literature for these genes has been inspected and no information on a known function, process, or location was available at the time of annotation. Including associations to unknown terms, 28,331 genes (94% of the genome) have at least one GO annotation. Unannotated genes, which have not yet been assigned a term by computational methods or by a curator, reflect the ongoing nature of this annotation project.
To get an overview of the distribution of the annotations within each ontology, we have chosen some of the high-level terms from each GO hierarchy that are useful for grouping genes into broad categories. Taking the earlier example of plastids, the more specific terms chromoplast, etioplast, chloroplast, and amyloplast can be represented by the single parent term plastid. These high-level terms, called GOslims, are a simplified version of the full ontologies composed of about 40, as opposed to several thousand, terms per ontology. There are several GOslims in use by the GO Consortium; TAIR uses one developed with plant annotations in mind ( ftp://ftp.geneontology.org/go/GO_slims/ ). Using the plant GOslim terms, we have classified the genome into an array of broad functional categories that aid in assessing the distribution of genes among different functions, processes, and subcellular locations ( Fig. 2 ). The resulting distribution shows that most cellular component annotations are to unknown (35%), membrane (24%), and plastid (13%). Molecular function annotations are largely to unknown (26%), followed by transferase activity and catalytic activity (both 10%). The most common biological processes are unknown (37%), transport (8%), and metabolism (7%). The current distribution of genes in known GOslim categories may not accurately reflect biological reality because of the large proportion of computationally derived annotations. As the number of unknown genes is decreased by further experimentation and refinement of computational methods, the number of genes within each category will more accurately reflect the actual distributions of functions, processes, and subcellular locations.

Functional classification of the whole Arabidopsis genome representing the distribution of genes based on their annotations to terms in the GO cellular component (a), GO molecular function (b), and GO biological process vocabularies (c).
Annotation of Temporal and Spatial Gene Expression Data
In addition to making GO annotations, we have also been using controlled vocabularies to describe the anatomical parts and developmental stages in which a gene is expressed. As part of this effort, we have annotated the protein and/or mRNA expression patterns of more than 400 genes (about 900 annotations; Table I ). Combining the GO annotations with the anatomy and temporal annotations for a given gene provides a comprehensive view of the role of a gene in the cell.
Accessing Arabidopsis Controlled Vocabulary Annotations
To enable the research community to effectively use these controlled vocabulary annotations, we have developed several tools to search, browse, and download them from TAIR's Web site. Table II provides a complete set of URLs where tools to access the vocabularies and annotations at TAIR and related Web sites can be found. The main search tools for finding genes and associated terms include TAIR's Gene Search and Keyword Browser. The Gene Search allows users to specify the vocabulary type, term name, and many gene-related attributes. Search results are displayed on the Gene detail page ( Fig. 3a ), which links to the Term Annotation detail ( Fig. 3b ) and Gene Annotation detail pages ( Fig. 3c ). Browsing of all the controlled vocabularies and their associated genes can be done using the TAIR Keyword Browser ( Fig. 1 ). One can retrieve GO annotations and plant GOslim mappings for a list of Arabidopsis Genome Initiative locus codes (i.e. AT1G01010) by entering or uploading a locus list into the TAIR GO annotation search, functional categorization, and download tool ( http://www.arabidopsis.org/tools/bulk/go/index.jsp ). The complete annotation set can be downloaded by ftp (file transfer protocol).

Display of controlled vocabulary association on the TAIR Gene detail page (a), which summarizes information relevant to gene, the Term Annotation detail page (b), which displays all annotations made to the term in question, and the Gene Annotation detail page (c), which displays all controlled vocabulary annotations made to that gene. These pages are interlinked so that one can get from one page to the next by clicking on the appropriate hyperlink.
Useful Web site links to aid the searching with controlled vocabularies
Page Names | URL | Usage |
---|---|---|
TAIR Gene search | Search for genes using controlled vocabularies | |
TAIR Keyword Browser | Search for or browse controlled vocabulary terms; view term details and term relationships | |
TAIR GO bulk download | Download GO annotations and functionally categorize a set of genes | |
TAIR and TIGR GO annotations | Download GO annotations for the whole Arabidopsis genome | |
TAIR anatomy and temporal ontologies | Download Arabidopsis anatomy and temporal ontologies | |
TAIR anatomy annotations | Download anatomy annotations for the whole genome | |
TAIR temporal annotations | Download temporal annotations for the whole genome | |
GO consortium | Gene Ontology Web site | |
GO database browser | Search for terms and annotations in the GO database | |
OBO | Open Biological Ontologies Web site, which hosts most of the controlled vocabularies | |
Plant Ontology consortium | Plant Ontology Web site |
Components of Controlled Vocabulary Annotations
A controlled vocabulary association has several parts as defined by the GO Consortium: gene name, associated term and ID, evidence code, reference, annotation date, and annotating database/person ( GO Consortium, 2001 ). To these standard GO annotation components, we have added two fields to present a complete picture of the annotation to the users: evidence description and relationship type. These fields are not submitted to the GO database and are displayed only on TAIR Web site pages.
The combination of evidence code, evidence description, and reference defines the basis for annotation and provides the information necessary for a user to interpret an annotation correctly. The evidence code indicates how the association between the gene and the term is supported. There are 11 evidence codes in use by TAIR and TIGR (see Table III ). Annotations derived from computational predictions that have not been reviewed by a curator are given the evidence code IEA (inferred from electronic annotation). Annotations that have been reviewed by a curator are given one of the other evidence codes depending on the type of experimental evidence that was used to make the association. The evidence description provides additional information on the evidence used to support the annotation. In the example shown in Figure 3c , the association between the gene PDF2 and the term epidermal cell differentiation is supported by an IMP (inferred from mutant phenotype) evidence code with an evidence description of analysis of visible trait. Here, the phrase analysis of visible trait provides information about the type of method used to support the association between the gene and the GO term. Evidence descriptions used by TAIR are also a controlled vocabulary currently composed of 107 descriptions. Table IV shows an example of the evidence descriptions used in conjunction with the IPI (inferred from physical interaction) evidence code. Finally, the reference linked to each association gives users a concrete source where the experimental evidence can be found and read about in greater depth. We strive to capture all relevant data, including conflicting views, permitting users to evaluate the supporting evidence themselves.
Evidence codes used in functional annotations
Evidence Code Abbreviation | Evidence Code Definition |
---|---|
Computational: | |
IEA | Inferred from electronic annotation |
Manual: | |
IDA | Inferred from direct assay |
IMP | Inferred from mutant phenotype |
IEP | Inferred from expression pattern |
ISS | Inferred from sequence similarity |
IGI | Inferred from genetic interaction |
IPI | Inferred from physical interaction |
TAS | Traceable author statement |
NAS | Nontraceable author statement |
ND | No biological data available |
IC | Inferred by curator |
Unique fields used in TAIR functional annotations
Unique Field Name | Description | Number of Annotations |
---|---|---|
Relationship type | Has | 23,400 |
Located in | 29,701 | |
Involved in | 29,087 | |
Functions as | 760 | |
Expressed in | 857 | |
Related to | 639 | |
Functions in | 194 | |
Is subunit of | 111 | |
Constituent of | 75 | |
Expressed during | 60 | |
Required for | 31 | |
Not involved in | 31 | |
Regulates | 23 | |
Not expressed in | 24 | |
Is down-regulated by | 20 | |
Expressed only in | 20 | |
Not functions as | 6 | |
Not located in | 6 | |
Represses | 3 | |
Expressed only during | 5 | |
Not required for | 2 | |
None | 38,067 | |
Evidence Description | Yeast two-hybrid assay | 56 |
(For IPI evidence code) | Coimmunoprecipitation | 28 |
Copurification | 4 | |
Yeast one-hybrid | 5 | |
Cosedimentation | 3 | |
Sos-recruitment assay | 2 | |
Far-western analysis | 2 | |
Split-ubiquitin assay | 1 | |
None | 36,729 |
Relationship type refers to terms that define the association between the gene and the controlled vocabulary term. For example, Figure 3a displays several annotations, one of which states that PDF2 is involved in epidermal cell differentiation. Here, involved in is the relationship type that links the gene PDF2 with the controlled vocabulary term epidermal cell differentiation. The relationship type provides a specific context for the association between the term and the gene that can be used for searching and data mining purposes. It also allows the annotation to be read in a more logical, sentence-like format, helping users understand the functional annotation more intuitively. There are 21 relationship types currently in use by TAIR ( Table IV ). The relationship types that include the word not allow curators to capture specific negative results that have been described in the literature, which may be contrary to previously known data. The GO consortium has recognized the utility of TAIR's relationship types and may move toward adding them to the current standard for consortium-wide GO annotations.
DISCUSSION AND CONCLUSION
Advantages to using controlled vocabularies.
There are several advantages to using controlled vocabularies for functional annotation of a genome. First, it allows one to perform powerful intraspecies and cross-species genome queries. For example, one can identify all of the genes in Arabidopsis that are associated to the term NADH dehydrogenase activity using the TAIR gene search ( Fig. 4a ), or one can identify all of the genes in the central GO database that are associated to the same term using the AmiGO browser ( Fig. 4b ).

Searching with controlled vocabulary terms within one species and across multiple species. a, Screenshot from a TAIR Web page showing a partial list of all Arabidopsis genes associated to the GO term NADH dehydrogenase activity. This page can be retrieved by entering the GO term on the TAIR gene search page ( http://www.arabidopsis.org/servlets/Search?action=new_search&type=gene ). b, Screenshot from a GO Web page showing a partial list of genes from multiple organisms associated to the term NADH dehydrogenase activity. This page can be reached by entering the GO term on the GO database/ontology browser ( http://www.godatabase.org/cgi-bin/go.cgi ) or by clicking on the GO database hyperlink from the TAIR keyword detail page.
Second, one can quantitatively assess the similarity/dissimilarity of any two sets of genes or genomes by comparing the distribution of their annotations among GOslim categories. Functional categorization of the whole genome using GOslim terms provides researchers the ability to view the distribution of the entire genome into categories describing cellular location, molecular function, and biological process. This large-scale view may assist in directing future research to areas that are in need of more attention. Exploring these areas of biology may reduce the number of unknown genes and lead to better understanding of the overall nature of the genome. The plant GOslim terms are also useful in classifying and comparing smaller sets of genes, such as those identified by common expression patterns in a microarray experiment. In a previous section, we described the retrieval of annotations for lists of genes. In addition to getting the association counts in a tabular format, users can also draw pie charts (such as those in Fig. 2 ) based on the GOslim mapping for analysis and presentation purposes. A researcher can group the genes in one data set and compare their distribution among GOslim categories to a second set of genes or the genome as a whole to determine which categories are overrepresented or underrepresented.
Third, one can use the annotated genome of any one species to transfer knowledge to another genome. Since Arabidopsis has the most comprehensive functional annotation of any plant genome, its annotation can serve as a foundation upon which the functional annotation of other plant genomes such as rice ( Oryza sativa ), tomato ( Lycopersicon esculentum ), cotton ( Gossypium hirsutum ), maize ( Zea mays ), and related Brassica species can be built. For example, there are approximately 22,000 tentative tomato consensus sequences (TIGR Tomato Gene Index version 9.0, April 2003) that have been generated from approximately 182,000 tomato expressed sequence tags in several sequencing projects. Many of these tentative consensus sequences have >50% amino acid sequence similarity to an Arabidopsis protein over the entire sequence length ( http://aztec.stanford.edu/cold/cgi-bin/analysis.cgi ). Transferring at least the molecular function annotations of the Arabidopsis genes to the homologous tomato sequences with an IEA evidence code would be a reasonable first step in annotating the tomato genome. Expanding this example to a large-scale transfer of annotations makes the construction of a scaffold functional annotation of a new plant genome possible. This approach is also valid for smaller sets of genes. Researchers focusing on other plant species can find Arabidopsis genes similar to their genes of interest using sequence similarity methods. This gene list can be used to obtain functional annotation from the Arabidopsis genome (see above), which can be used to infer information and suggest experiments for these other systems.
Finally, complete functional annotation of a genome allows detailed evaluation of known versus unknown genes in that genome. For example, one can easily assess the number of genes with unknown molecular function, biological process, or cellular component. The lack of information in the literature, which is reflected by the unknown annotation, could guide researchers to a set of genes in need of further research. In addition, evidence codes can be used to determine to what extent a gene has been characterized. For example, a gene whose sequence is similar to known glycosyl transferases but has no experimental evidence for the activity may be annotated to glycosyl transferase activity with an ISS (inferred from sequence similarity) evidence code indicating that no experimental evidence supporting this prediction exists. By using a combination of GO terms and evidence codes, a researcher looking for a new project can get an up-to-date view of genes still requiring experimental characterization.
TAIR's annotations using controlled vocabularies are based on clearly defined sources of evidence, either experimental or computational. Both methods have their advantages—computational data can supply hypotheses that suggest experimental approaches and supply a basic level of annotation for genes not yet characterized experimentally. Experimental data, on the other hand, provides confirmation of a gene's biological role and also provides the basis for future computational analysis. When it is available, experimental data must take precedence over computational data, but both kinds of information are useful in combination to examine relationships between structure and function and answer evolutionary questions.
Continuing and Expanding Functional Annotation of the Arabidopsis Genome
Once we have captured the basic information for each published gene, we will be faced with the task of keeping the functional annotations up to date, including adding new genes as they are described and capturing new information about existing genes. Keeping the annotations current is essential to reflecting the most recent state of knowledge about the genome. The most efficient way to accomplish both of these tasks will be to switch from our current gene-based curation approach to a paper-based approach in which we will extract all relevant information from new papers (approximately 100 per month) as they are incorporated into TAIR's PubSearch database. New genes will be annotated with GO terms describing their identity or with unknown terms to indicate missing information. For existing genes, we will use new information to replace existing unknown annotations with the appropriate GO terms, add GO and TAIR terms for newly described phenomena, and update existing known annotations based upon the latest experimental data. We also regularly update annotations based on comments from the research community. Since our user community is ultimately the best judge of the annotation quality, we strongly encourage them to contact us if we have made erroneous annotations or incorrectly captured data from the literature. Researchers can give their feedback by (1) adding comments to genes by clicking on the Add My Comments button on each gene detail page, (2) e-mailing us directly at gro.sispodibara@rotaruc , or (3) giving us comments in person when at scientific meetings such as the International Conference on Arabidopsis Research or the Annual Meeting of the American Society of Plant Biologists.
Building on our experience in extracting gene-related information from the literature, we are in the early stages of the next large task of annotation of mutant and natural variant alleles and their associated germ plasms and phenotypes. Incorporation of data into TAIR will capture what processes and/or expression patterns are disrupted or modified as a result of allelic variance. From a survey of almost 8,400 full-text Arabidopsis articles held in-house at TAIR, there are about 5,000 unique alleles described to varying degrees in the literature. Allele-related data is extremely complex and challenging to curate, and we anticipate that this project will last several years. Initially, we will describe the phenotypes using text summaries similar to gene descriptions. We will then move to using controlled vocabularies for describing basic phenotypes as well. Along with many other model organism databases, we have participated in a series of Phenotype Ontology meetings that discussed the need for a controlled vocabulary to describe phenotypes ( http://obo.sourceforge.net/pheno/ ). Such a vocabulary would facilitate querying and comparison of phenotypes between different species. The common desire for a phenotype annotation standard has led to the development of a prototype controlled vocabulary (available from http://obo.sourceforge.net/ ) that will be modified and updated by TAIR and the other databases in much the same way as the GO vocabularies.
We have begun capturing information in the literature pertaining to genetic interactions and will expand this effort to cover signal transduction and transcriptional regulation pathways. Finally, we will begin making more complex associations by including environmental condition or genotype information in our annotations as well as by tying annotations to two separate controlled vocabularies to each other. Examples of this kind of information include: gene X is expressed in the radicle during germination or gene Y is expressed in the nucleus in the ecotype Columbia-0 but in the cytoplasm in the ecotype Landsberg erecta . Other types of composite annotations could capture conditional subcellular localization depending on phosphorylation status of the protein or association of a signal molecule. A combination of these types of annotations with the existing controlled vocabulary annotations will provide the researcher with a more complete summary of a gene's identity in a computationally accessible format.
MATERIALS AND METHODS
Computational annotation methods.
The following methods were used to computationally generate GO assignments: (1) INTERPRO2GO transfer, a mapping between all Arabidopsis proteins containing INTERPRO domains ( Mulder et al., 2003 ) and the corresponding GO identification assigned to the individual INTERPRO domain using the INTERPRO2GO mapping file ( http://www.geneontology.org/external2go/interpro2go ). (2) TargetP analysis ( Emanuelsson et al., 2000 ), which uses a pattern recognition program that detects consensus targeting sequences within the entire predicted Arabidopsis proteome. The subcellular locations determined by this analysis were mapped to the corresponding GO term. (3) Metacyc2go transfer. The metacyc2go mapping file ( http://www.geneontology.org/external2go/metacyc2go ) is used to generate GO annotations in a manner similar to the INTERPRO2GO mapping, in which GO identifications for particular metabolic processes and functions were assigned to genes that had been annotated to Metacyc biochemical pathways and reactions ( Krieger et al., 2004 ). (4) String matching, an algorithm in which gene descriptions obtained from TIGR were matched to a corresponding GO term. All annotations derived using these methods are given the IEA evidence code and associated to a reference describing the analysis in detail. Our computational analyses are repeated on each successive genome release to ensure that they remain up to date.
Manually Reviewed Annotation Methods
We also associate genes with controlled vocabulary terms based on evidence found in the published literature. This entails obtaining appropriate papers that describe Arabidopsis genes, reading the papers, and associating the controlled vocabulary terms to the genes along with the evidence supporting the association. To facilitate literature-based annotation, we developed PubSearch, a literature curation software package that stores gene, paper, and controlled vocabulary data, automatically indexes the literature against genes and controlled vocabulary terms, and provides a user-friendly Web interface for manual verification of matches and curation ( http://pubsearch.org/ ). PubSearch is maintained by TAIR and is one of the literature curation tools for the Generic Model Organism Database project. Its source code is available under the General Public License from Sourceforge ( http://www.gmod.org ). PubSearch is both extensible, allowing new types of biological objects to be added, and flexible, allowing programmatic implementation of different curation strategies. The software automatically assigns new genes each day to individual curators and displays the number of genes completed and in progress. The criteria used by PubSearch for selecting genes to be curated are modified according to the priorities of the curation team. All curation at TAIR is stored in the PubSearch database, and updates are sent to the production database on a weekly basis. Table V gives an overview of the data types that are stored in the TAIR installation of the PubSearch database.
PubSearch data types and statistics
Data Types | Numbers | |
---|---|---|
All literature records | 21,532 | |
Research papers | 16,427 | |
Research papers with abstracts | 11,888 | |
Articles with full text | 8,633 | |
Gene names (including aliases) | 118,484 | |
Controlled vocabulary terms | 17,178 | |
Anatomy terms | 268 | |
Developmental stage terms | 102 | |
GO molecular function terms | 7,278 | |
GO biological process terms | 8,181 | |
GO cellular component terms | 1,379 | |
Hits between terms and articles | 177,210 | |
Curator-reviewed hits between genes and articles | 19,974 | |
Valid hits | 15,604 | |
Invalid hits | 4,301 | |
Maybe hits | 69 |
The stored titles and abstracts of publications are first indexed against the gene names and aliases to generate hits, or associations, between papers and genes. For example, a paper that mentions the gene HST in its abstract will be associated with the gene HST. Because gene symbols are often not unique (for example, there are two GPX genes, two PUP1 genes, etc.), each match of a gene to an abstract is verified by a curator if the association is correct. Thus, several gene entries may exist with the same gene symbol but with different associated publications. After verification, the set of articles associated to a gene serves as the reading material for the curator who is updating a specific gene's annotations. The automated association of genes to papers frees curators from the need to search the literature for gene-related articles each time a gene record is updated or revisited.
We use the following procedure in extracting information from each gene's associated body of literature. First, the most recent paper or review about the gene is read to determine whether the process, function, and/or cellular location are known. If some or all of these aspects are known, the original paper describing the details of the experiments leading to that conclusion is located and the relevant information (i.e. subcellular localization method) is translated into a GO term, evidence code, and description. Each gene and annotation is stamped with the date it was last modified and the name of the annotating curator.
We select the most specific GO term that is appropriate for describing that aspect of the gene's identity. For example, we would select Ser/Thr kinase activity rather than enzyme activity to describe a Ser/Thr kinase. If the appropriate term is not present in the ontologies, curators propose a new term together with a definition and parentage and enter it as a temporary term through the PubSearch user interface. Annotations made to the new terms are not released to the public until the term has been accepted and added to the GO/TAIR vocabularies. Two members of our curation team periodically go through the list of proposed terms and, after review and consultation with the GO consortium and/or the rest of the TAIR curation team, add it to the appropriate vocabulary, at which point the term becomes available for the entire community to use.
Finally, we incorporate annotations made by external groups such as individual researchers sending corrections by mail, gene family experts sending annotations in spreadsheet files, and major database groups such as TIGR. TIGR has been annotating genes based on their membership in paralogous gene families. This has resulted in annotation of 21,893 genes. TIGR's paralogous family groupings are based on sequence similarity, identification of Pfam and TIGRFAM domain signatures, and potential novel domains in the Arabidopsis proteome ( Wortman et al., 2003 ). GO terms that are associated with certain protein domains are then ascribed to all gene products that are members of paralogous families, if they are deemed appropriate. In cases where some members of the paralogous family had been described in the literature, annotations for biological process and/or cellular component were added as well.
Quality Control Methods
We employ several methods to assure a consistent and accurate standard of annotation. First, to minimize variability in annotation between curators, individual annotations are randomly selected and checked by verifying the association between the gene and the controlled vocabulary term. Rules for making associations are clarified when necessary. Second, at the level of data input, the curation software checks ensure that all the necessary fields are filled in to complete an annotation. User interfaces for editing information are designed to minimize human error. Third, at the level of data exchange between the PubSearch database and the TAIR production and GO databases, a number of software checks ensure data integrity (e.g. that annotations made to temporary terms are not sent out and that all references used in the annotation are present in the TAIR database). Fourth, we have implemented a method of computationally updating annotations based on a combination of evidence code and whether the association is made to an unknown term or not. Annotations of a gene to unknown terms are updated when an annotation of the same gene to a known term in the same ontology is made. Annotations with an IEA evidence code are replaced when a curator adds a non-IEA based annotation to the gene using a term in the same ontology. Finally, we incorporate feedback from the scientific community who provide corrections to the annotations or point out papers that were missing from our database.
1 This work was supported by the National Science Foundation (grant no. DBI–9978564) and the National Institutes of Health (grant no. HG02273–03).
www.plantphysiol.org/cgi/doi/10.1104/pp.104.040071 .
- Arabidopsis Genome Initiative (2000) Analysis of the genome sequence of the flowering plant Arabidopsis thaliana. Nature 408 : 796–815 [ PubMed ] [ Google Scholar ]
- Camon E, Magrane M, Barrell D, Binns D, Fleischmann W, Kersey P, Mulder N, Oinn T, Maslen J, Cox A, Apweiler R (2003) The Gene Ontology Annotation (GOA) project: implementation of GO in SWISS-PROT, TrEMBL, and InterPro. Genome Res 13 : 662–672 [ PMC free article ] [ PubMed ] [ Google Scholar ]
- Consortium GO (2001) Creating the gene ontology resource: design and implementation. Genome Res 11 : 1425–1433 [ PMC free article ] [ PubMed ] [ Google Scholar ]
- Dwight SS, Harris MA, Dolinski K, Ball CA, Binkley G, Christie KR, Fisk DG, Issel-Tarver L, Schroeder M, Sherlock G, et al (2002) Saccharomyces Genome Database (SGD) provides secondary gene annotation using the Gene Ontology (GO). Nucleic Acids Res 30 : 69–72 [ PMC free article ] [ PubMed ] [ Google Scholar ]
- Emanuelsson O, Nielsen H, Brunak S, Svon Heijne G (2000) Predicting subcellular localization of proteins based on their N-terminal amino acid sequence. J Mol Biol 300 : 1005–1016 [ PubMed ] [ Google Scholar ]
- Frishman D, Albermann K, Hani J, Heumann K, Metanomski A, Zollner A, Mewes HW (2001) Functional and structural genomics using PEDANT. Bioinformatics 17 : 44–57 [ PubMed ] [ Google Scholar ]
- Hazbun TR, Malmstrom L, Anderson S, Graczyk BJ, Fox B, Riffle M, Sundin BA, Aranda JD, McDonald WH, Chiu CH, et al (2003) Assigning function to yeast proteins by integration of technologies. Mol Cell 12 : 1353–1365 [ PubMed ] [ Google Scholar ]
- Hennig S, Groth D, Lehrach H (2003) Automated Gene Ontology annotation for anonymous sequence data. Nucleic Acids Res 31 : 3712–3715 [ PMC free article ] [ PubMed ] [ Google Scholar ]
- Kanapin A, Batalov S, Davis MJ, Gough J, Grimmond S, Kawaji H, Magrane M, Matsuda H, Schonbach C, Teasdale RD, Yuan Z (2003) Mouse proteome analysis. Genome Res 13 : 1335–1344 [ PMC free article ] [ PubMed ] [ Google Scholar ]
- King OD, Lee JC, Dudley AM, Janse DM, Church GM, Roth FP (2003) Predicting phenotype from patterns of annotation. Bioinformatics 19 (suppl. 1) : I183–I189 [ PubMed ] [ Google Scholar ]
- Krieger CJ, Zhang P, Mueller LA, Wang A, Paley S, Arnaud M, Pick J, Rhee SY, Karp PD (2004) MetaCyc: a multiorganism database of metabolic pathways and enzymes. Nucleic Acids Res 32 : D438–D442 [ PMC free article ] [ PubMed ] [ Google Scholar ]
- MASC Committee (2003) The Multinational Coordinated Arabidopsis thaliana Functional Genomics Project: Annual Report 2003. MASC Committee, Madison, WI
- Mulder NJ, Apweiler R, Attwood TK, Bairoch A, Barrell D, Bateman A, Binns D, Biswas M, Bradley P, Bork P, et al (2003) The InterPro Database, 2003 brings increased coverage and new features. Nucleic Acids Res 31 : 315–318 [ PMC free article ] [ PubMed ] [ Google Scholar ]
- Sprague J, Clements D, Conlin T, Edwards P, Frazer K, Schaper K, Segerdell E, Song P, Sprunger B, Westerfield M (2003) The Zebrafish Information Network (ZFIN): the zebrafish model organism database. Nucleic Acids Res 31 : 241–243 [ PMC free article ] [ PubMed ] [ Google Scholar ]
- Wortman JR, Haas BJ, Hannick LI, Smith RK Jr, Maiti R, Ronning CM, Chan AP, Yu C, Ayele M, Whitelaw CA, et al (2003) Annotation of the Arabidopsis genome. Plant Physiol 132 : 461–468 [ PMC free article ] [ PubMed ] [ Google Scholar ]
- A-Z Publications
Annual Review of Animal Biosciences
Volume 7, 2019, review article, functional annotation of animal genomes (faang): current achievements and roadmap.
- Elisabetta Giuffra 1 , Christopher K. Tuggle 2 , and FAANG Consortium 1,2
- View Affiliations Hide Affiliations Affiliations: 1 Génétique Animale et Biologie Intégrative (GABI), Institut National de la Recherche Agronomique (INRA), AgroParisTech, Université Paris Saclay, 78350 Jouy-en-Josas, France; email: [email protected] 2 Department of Animal Science, Iowa State University, Ames, Iowa 50011, USA; email: [email protected]
- Vol. 7:65-88 (Volume publication date February 2019) https://doi.org/10.1146/annurev-animal-020518-114913
- First published as a Review in Advance on November 14, 2018
- Copyright © 2019 by Annual Reviews. All rights reserved
Functional annotation of genomes is a prerequisite for contemporary basic and applied genomic research, yet farmed animal genomics is deficient in such annotation. To address this, the FAANG (Functional Annotation of Animal Genomes) Consortium is producing genome-wide data sets on RNA expression, DNA methylation, and chromatin modification, as well as chromatin accessibility and interactions. In addition to informing our understanding of genome function, including comparative approaches to elucidate constrained sequence or epigenetic elements, these annotation maps will improve the precision and sensitivity of genomic selection for animal improvement. A scientific community–driven effort has already created a coordinated data collection and analysis enterprise crucial for the success of this global effort. Although it is early in this continuing process, functional data have already been produced and application to genetic improvement reported. The functional annotation delivered by the FAANG initiative will add value and utility to the greatly improved genome sequences being established for domesticated animal species.
Article metrics loading...
Full text loading...
Literature Cited
- 1. Tuggle CK , Towfic F , Honavar V 2011 . Introduction to systems biology for animal scientists. Systems Biology and Livestock Science MFW te Pas, H Woelders, A Bannick 1– 30 Malden, MA: John Wiley & Sons [Google Scholar]
- 2. Suravajhala P , Kogelman LJ , Kadarmideen HN 2016 . Multi-omic data integration and analysis using systems genomics approaches: methods and applications in animal production, health and welfare. Genet. Sel. Evol. 48 : 38 [Google Scholar]
- 3. Loor JJ , Vailati-Riboni M , McCann JC , Zhou Z , Bionaz M 2015 . Triennial Lactation Symposium: nutrigenomics in livestock: systems biology meets nutrition. J. Anim. Sci. 93 : 5554– 74 [Google Scholar]
- 4. Kell DB , Oliver SG 2004 . Here is the evidence, now what is the hypothesis? The complementary roles of inductive and hypothesis-driven science in the post-genomic era. Bioessays 26 : 99– 105 [Google Scholar]
- 5. Meuwissen TH , Hayes BJ , Goddard ME 2001 . Prediction of total genetic value using genome-wide dense marker maps. Genetics 157 : 1819– 29 [Google Scholar]
- 6. Goddard ME , Kemper KE , MacLeod IM , Chamberlain AJ , Hayes BJ 2016 . Genetics of complex traits: prediction of phenotype, identification of causal polymorphisms and genetic architecture. Proc. R. Soc. B Biol. Sci. 283 : 20160569 [Google Scholar]
- 7. Hayes BJ , Lewin HA , Goddard ME 2013 . The future of livestock breeding: genomic selection for efficiency, reduced emissions intensity, and adaptation. Trends Genet 29 : 206– 14 [Google Scholar]
- 8. Hayes BJ , Bowman PJ , Chamberlain AJ , Goddard ME 2009 . Invited review: genomic selection in dairy cattle: progress and challenges. J. Dairy Sci. 92 : 433– 43 [Google Scholar]
- 9. Lund MS , Su G , Janss L , Guldbrandtsen B , Brøndum RF 2014 . Genomic evaluation of cattle in a multi-breed context. Livest. Sci. 166 : 101– 10 [Google Scholar]
- 10. Wang M , Hancock TP , Chamberlain JA , Vander Jagt CJ , Pryce JE et al. 2018 . Putative bovine topological association domains and CTCF binding motifs can reduce the search space for causative regulatory variants of complex traits. BMC Genom 19 : 395 [Google Scholar]
- 11. Kellis M , Wold B , Snyder MP , Bernstein BE , Kundaje A et al. 2014 . Defining functional DNA elements in the human genome. PNAS 111 : 6131– 38 [Google Scholar]
- 12. Ritchie MD , Holzinger ER , Li R , Pendergrass SA , Kim D 2015 . Methods of integrating data to uncover genotype-phenotype interactions. Nat. Rev. Genet. 16 : 85– 97 [Google Scholar]
- 13. Brookes AJ , Robinson PN 2015 . Human genotype-phenotype databases: aims, challenges and opportunities. Nat. Rev. Genet. 16 : 702– 15 [Google Scholar]
- 14. Chakravorty S , Hegde M 2017 . Gene and variant annotation for Mendelian disorders in the era of advanced sequencing technologies. Annu. Rev. Genom. Hum. Genet. 18 : 229– 56 [Google Scholar]
- 15. Meadows JRS , Lindblad-Toh K 2017 . Dissecting evolution and disease using comparative vertebrate genomics. Nat. Rev. Genet. 18 : 624– 36 [Google Scholar]
- 16. Schmidt D , Wilson MD , Ballester B , Schwalie PC , Brown GD et al. 2010 . Five-vertebrate ChIP-seq reveals the evolutionary dynamics of transcription factor binding. Science 328 : 1036– 40 [Google Scholar]
- 17. Yue F , Cheng Y , Breschi A , Vierstra J , Wu W et al. 2014 . A comparative encyclopedia of DNA elements in the mouse genome. Nature 515 : 355– 64 [Google Scholar]
- 18. Villar D , Berthelot C , Aldridge S , Rayner TF , Lukk M et al. 2015 . Enhancer evolution across 20 mammalian species. Cell 160 : 554– 66 [Google Scholar]
- 19. Elsik CG , Tellam RL , Worley KC , Gibbs RA , Muzny DM et al. 2009 . The genome sequence of taurine cattle: a window to ruminant biology and evolution. Science 324 : 522– 28 [Google Scholar]
- 20. Groenen MA , Archibald AL , Uenishi H , Tuggle CK , Takeuchi Y et al. 2012 . Analyses of pig genomes provide insight into porcine demography and evolution. Nature 491 : 393– 98 [Google Scholar]
- 21. Archibald AL , Flicek P , Birney E 2012 . Enabling the reading of genome sequences for farmed and companion animals—a proposal for ENCODE consortia Presented at the 33rd Conference of the International Society for Animal Genetics, Cairns, Aust., July 15– 20 https://www.isag.us/2012/docs/ISAG_2012_Abstracts.pdf [Google Scholar]
- 22. Andersson L , Archibald AL , Bottema CD , Brauning R , Burgess SC et al. 2015 . Coordinated international action to accelerate genome-to-phenome with FAANG, the Functional Annotation of Animal Genomes project. Genome Biol 16 : 57 [Google Scholar]
- 23. Tuggle CK , Giuffra E , White SN , Clarke L , Zhou H et al. 2016 . GO-FAANG meeting: a Gathering On Functional Annotation of Animal Genomes. Anim. Genet. 47 : 528– 33 [Google Scholar]
- 24. Int. Chick. Genome Seq. Consort. 2004 . Sequence and comparative analysis of the chicken genome provide unique perspectives on vertebrate evolution. Nature 432 : 695– 716 [Google Scholar]
- 25. Lindblad-Toh K , Wade CM , Mikkelsen TS , Karlsson EK , Jaffe DB et al. 2005 . Genome sequence, comparative analysis and haplotype structure of the domestic dog. Nature 438 : 803– 19 [Google Scholar]
- 26. Wade CM , Giulotto E , Sigurdsson S , Zoli M , Gnerre S et al. 2009 . Genome sequence, comparative analysis, and population genetics of the domestic horse. Science 326 : 865– 67 [Google Scholar]
- 27. Jiang Y , Xie M , Chen W , Talbot R , Maddox JF et al. 2014 . The sheep genome illuminates biology of the rumen and lipid metabolism. Science 344 : 1168– 73 [Google Scholar]
- 28. Dong Y , Xie M , Jiang Y , Xiao N , Du X et al. 2013 . Sequencing and automated whole-genome optical mapping of the genome of a domestic goat ( Capra hircus ). Nat. Biotechnol. 31 : 135– 41 [Google Scholar]
- 29. Berthelot C , Brunet F , Chalopin D , Juanchich A , Bernard M et al. 2014 . The rainbow trout genome provides novel insights into evolution after whole-genome duplication in vertebrates. Nat. Commun. 5 : 3657 [Google Scholar]
- 30. Warr A , Robert C , Hume D , Archibald AL , Deeb N , Watson M 2015 . Identification of low-confidence regions in the pig reference genome (Sscrofa10.2). Front. Genet. 6 : 338 [Google Scholar]
- 31. Bickhart DM , Rosen BD , Koren S , Sayre BL , Hastie AR et al. 2017 . Single-molecule sequencing and chromatin conformation capture enable de novo reference assembly of the domestic goat genome. Nat. Genet. 49 : 643– 50 [Google Scholar]
- 32. Birney E , Hudson TJ , Green ED , Gunter C , Eddy S et al. 2009 . Prepublication data sharing. Nature 461 : 168– 70 [Google Scholar]
- 33. Macqueen DJ , Primmer CR , Houston RD , Nowak BF , Bernatchez L et al. 2017 . Functional Annotation of All Salmonid Genomes (FAASG): an international initiative supporting future salmonid research, conservation and aquaculture. BMC Genom 18 : 484 [Google Scholar]
- 34. Soneson C , Love MI , Robinson MD 2015 . Differential analyses for RNA-seq: Transcript-level estimates improve gene-level inferences. F1000Research 4 : 1521 [Google Scholar]
- 35. Mortazavi A , Williams BA , McCue K , Schaeffer L , Wold B 2008 . Mapping and quantifying mammalian transcriptomes by RNA-Seq. Nat. Methods 5 : 621– 28 [Google Scholar]
- 36. Wang Z , Gerstein M , Snyder M 2009 . RNA-Seq: a revolutionary tool for transcriptomics. Nat. Rev. Genet. 10 : 57– 63 [Google Scholar]
- 37. Robert C , Watson M 2015 . Errors in RNA-Seq quantification affect genes of relevance to human disease. Genome Biol 16 : 177 [Google Scholar]
- 38. Clark EL , Bush SJ , McCulloch MEB , Farquhar IL , Young R et al. 2017 . A high resolution atlas of gene expression in the domestic sheep ( Ovis aries ). PLOS Genet 13 : e1006997 [Google Scholar]
- 39. Kuo RI , Tseng E , Eory L , Paton IR , Archibald AL , Burt DW 2017 . Normalized long read RNA sequencing in chicken reveals transcriptome complexity similar to human. BMC Genom 18 : 323 [Google Scholar]
- 40. Imanishi T , Itoh T , Suzuki Y , O'Donovan C , Fukuchi S et al. 2004 . Integrative annotation of 21,037 human genes validated by full-length cDNA clones. PLOS Biol 2 : e162 [Google Scholar]
- 41. Chamberlain AJ , Vander Jagt CJ , Hayes BJ , Khansefid M , Marett LC et al. 2015 . Extensive variation between tissues in allele specific expression in an outbred mammal. BMC Genom 16 : 993 [Google Scholar]
- 42. Engreitz JM , Haines JE , Perez EM , Munson G , Chen J et al. 2016 . Local regulation of gene expression by lncRNA promoters, transcription and splicing. Nature 539 : 452– 55 [Google Scholar]
- 43. Hezroni H , Koppstein D , Schwartz MG , Avrutin A , Bartel DP , Ulitsky I 2015 . Principles of long noncoding RNA evolution derived from direct comparison of transcriptomes in 17 species. Cell Rep 11 : 1110– 22 [Google Scholar]
- 44. Djebali S , Davis CA , Merkel A , Dobin A , Lassmann T et al. 2012 . Landscape of transcription in human cells. Nature 489 : 101– 8 [Google Scholar]
- 45. Anthon C , Tafer H , Havgaard JH , Thomsen B , Hedegaard J et al. 2014 . Structured RNAs and synteny regions in the pig genome. BMC Genom 15 : 459 [Google Scholar]
- 46. Bush SJ , Muriuki C , McCulloch MEB , Farquhar IL , Clark EL , Hume DA 2018 . Cross-species inference of long non-coding RNAs greatly expands the ruminant transcriptome. Genet. Sel. Evol. 50 : 20 [Google Scholar]
- 47. Bush SJ , Muriuki C , McCulloch MEB , Farquhar IL , Clark EL , Hume DA 2018 . Cross-species inference of long non-coding RNAs greatly expands the ruminant transcriptome. Genet. Sel. Evol. 50 : 20 [Google Scholar]
- 48. Foissac S , Djebali S , Munyard K , Villa-Vialaneix N , Rau A et al. 2018 . Livestock genome annotation: transcriptome and chromatin structure profiling in cattle, goat, chicken and pig. bioRxiv https://doi.org/10.1101/316091 [Crossref] [Google Scholar]
- 49. Muret K , Klopp C , Wucher V , Esquerré D , Legeai F et al. 2017 . Long noncoding RNA repertoire in chicken liver and adipose tissue. Genet. Sel. Evol. 49 : 6 [Google Scholar]
- 50. Weikard R , Hadlich F , Hammon HM , Frieten D , Gerbert C et al. 2018 . Long noncoding RNAs are associated with metabolic and cellular processes in the jejunum mucosa of pre-weaning calves in response to different diets. Oncotarget 9 : 21052– 69 [Google Scholar]
- 51. Koufariotis LT , Chen YP , Chamberlain A , Vander Jagt C , Hayes BJ 2015 . A catalogue of novel bovine long noncoding RNA across 18 tissues. PLOS ONE 10 : e0141225 [Google Scholar]
- 52. Scott EY , Mansour T , Bellone RR , Brown CT , Mienaltowski MJ et al. 2017 . Identification of long non-coding RNA in the horse transcriptome. BMC Genom 18 : 511 [Google Scholar]
- 53. Wang X , Zhang FX , Wang ZM , Wang Q , Wang HF et al. 2016 . Histone H3K9 acetylation influences growth characteristics of goat adipose-derived stem cells in vitro . . Genet. Mol. Res 15 : gmr15048954 [Google Scholar]
- 54. Kociucka B , Stachecka J , Szydlowski M , Szczerbal I 2017 . Rapid communication: the correlation between histone modifications and expression of key genes involved in accumulation of adipose tissue in the pig. J. Anim. Sci. 95 : 4514– 19 [Google Scholar]
- 55. Byrne K , McWilliam S , Vuocolo T , Gondro C , Cockett NE , Tellam RL 2014 . Genomic architecture of histone 3 lysine 27 trimethylation during late ovine skeletal muscle development. Anim. Genet. 45 : 427– 38 [Google Scholar]
- 56. Li C , Guo S , Zhang M , Gao J , Guo Y 2015 . DNA methylation and histone modification patterns during the late embryonic and early postnatal development of chickens. Poult. Sci. 94 : 706– 21 [Google Scholar]
- 57. He Y , Yu Y , Zhang Y , Song J , Mitra A et al. 2012 . Genome-wide bovine H3K27me3 modifications and the regulatory effects on genes expressions in peripheral blood lymphocytes. PLOS ONE 7 : e39094 [Google Scholar]
- 58. Xiao S , Xie D , Cao X , Yu P , Xing X et al. 2012 . Comparative epigenomic annotation of regulatory DNA. Cell 149 : 1381– 92 [Google Scholar]
- 59. Jahan S , Xu W , He S , Gonzalez C , Delcuve GP , Davie JR 2016 . The chicken erythrocyte epigenome. Epigenet. Chromatin 9 : 19 [Google Scholar]
- 60. Mitra A , Luo J , He Y , Gu Y , Zhang H et al. 2015 . Histone modifications induced by MDV infection at early cytolytic and latency phases. BMC Genom 16 : 311 [Google Scholar]
- 61. Messerschmidt DM , Knowles BB , Solter D 2014 . DNA methylation dynamics during epigenetic reprogramming in the germline and preimplantation embryos. Genes Dev 28 : 812– 28 [Google Scholar]
- 62. Jones PA 2012 . Functions of DNA methylation: islands, start sites, gene bodies and beyond. Nat. Rev. Genet. 13 : 484– 92 [Google Scholar]
- 63. Schübeler D 2015 . Function and information content of DNA methylation. Nature 517 : 321– 26 [Google Scholar]
- 64. Coleman-Derr D , Zilberman D 2012 . DNA methylation, H2A.Z, and the regulation of constitutive expression. Cold Spring Harb. Symp. Quant. Biol. 77 : 147– 54 [Google Scholar]
- 65. Suzuki MM , Bird A 2008 . DNA methylation landscapes: provocative insights from epigenomics. Nat. Rev. Genet. 9 : 465– 76 [Google Scholar]
- 66. Li M , Wu H , Luo Z , Xia Y , Guan J et al. 2012 . An atlas of DNA methylomes in porcine adipose and muscle tissues. Nat. Commun. 3 : 850 [Google Scholar]
- 67. Bang WY , Kim SW , Kwon SG , Hwang JH , Kim TW et al. 2013 . Swine liver methylomes of Berkshire, Duroc and Landrace breeds by MeDIPS. Anim. Genet. 44 : 463– 66 [Google Scholar]
- 68. Ibeagha-Awemu EM , Zhao X 2015 . Epigenetic marks: regulators of livestock phenotypes and conceivable sources of missing variation in livestock improvement programs. Front. Genet. 6 : 302 [Google Scholar]
- 69. Lan X , Cretney EC , Kropp J , Khateeb K , Berg MA et al. 2013 . Maternal diet during pregnancy induces gene expression and DNA methylation changes in fetal tissues in sheep. Front. Genet. 4 : 49 [Google Scholar]
- 70. Namous H , Peñagaricano F , Del Corvo M , Capra E , Thomas DL et al. 2018 . Integrative analysis of methylomic and transcriptomic data in fetal sheep muscle tissues in response to maternal diet during pregnancy. BMC Genom 19 : 123 [Google Scholar]
- 71. Jenkins TG , Carrell DT 2012 . The sperm epigenome and potential implications for the developing embryo. Reproduction 143 : 727– 34 [Google Scholar]
- 72. Kropp J , Carrillo JA , Namous H , Daniels A , Salih SM et al. 2017 . Male fertility status is associated with DNA methylation signatures in sperm and transcriptomic profiles of bovine preimplantation embryos. BMC Genom 18 : 280 [Google Scholar]
- 73. Verma A , Rajput S , De S , Kumar R , Chakravarty AK , Datta TK 2014 . Genome-wide profiling of sperm DNA methylation in relation to buffalo ( Bubalus bubalis ) bull fertility. Theriogenology 82 : 750– 59.e1 [Google Scholar]
- 74. Lee JR , Hong CP , Moon JW , Jung YD , Kim DS et al. 2014 . Genome-wide analysis of DNA methylation patterns in horse. BMC Genom 15 : 598 [Google Scholar]
- 75. Schachtschneider KM , Madsen O , Park C , Rund LA , Groenen MA , Schook LB 2015 . Adult porcine genome-wide DNA methylation patterns support pigs as a biomedical model. BMC Genom 16 : 743 [Google Scholar]
- 76. Choi M , Lee J , Le MT , Nguyen DT , Park S et al. 2015 . Genome-wide analysis of DNA methylation in pigs using reduced representation bisulfite sequencing. DNA Res 22 : 343– 55 [Google Scholar]
- 77. Schachtschneider KM , Liu Y , Rund LA , Madsen O , Johnson RW et al. 2016 . Impact of neonatal iron deficiency on hippocampal DNA methylation and gene transcription in a porcine biomedical model of cognitive development. BMC Genom 17 : 856 [Google Scholar]
- 78. Song L , Crawford GE 2010 . DNase-seq: a high-resolution technique for mapping active gene regulatory elements across the genome from mammalian cells. Cold Spring Harb. Protoc. 2010 : pdb.prot5384 [Google Scholar]
- 79. Buenrostro JD , Giresi PG , Zaba LC , Chang HY , Greenleaf WJ 2013 . Transposition of native chromatin for fast and sensitive epigenomic profiling of open chromatin, DNA-binding proteins and nucleosome position. Nat. Methods 10 : 1213– 18 [Google Scholar]
- 80. Buenrostro JD , Wu B , Chang HY , Greenleaf WJ 2015 . ATAC-seq: a method for assaying chromatin accessibility genome-wide. Curr. Protoc. Mol. Biol. 109 : 21.9.1– 9 [Google Scholar]
- 81. Corces MR , Trevino AE , Hamilton EG , Greenside PG , Sinnott-Armstrong NA et al. 2017 . An improved ATAC-seq protocol reduces background and enables interrogation of frozen tissues. Nat. Methods 14 : 959– 62 [Google Scholar]
- 82. Lanctôt C , Cheutin T , Cremer M , Cavalli G , Cremer T 2007 . Dynamic genome architecture in the nuclear space: regulation of gene expression in three dimensions. Nat. Rev. Genet. 8 : 104– 15 [Google Scholar]
- 83. Dekker J , Rippe K , Dekker M , Kleckner N 2002 . Capturing chromosome conformation. Science 295 : 1306– 11 [Google Scholar]
- 84. Fanucchi S , Shibayama Y , Burd S , Weinberg MS , Mhlanga MM 2013 . Chromosomal contact permits transcription between coregulated genes. Cell 155 : 606– 20 [Google Scholar]
- 85. Pombo A , Dillon N 2015 . Three-dimensional genome architecture: players and mechanisms. Nat. Rev. Mol. Cell Biol. 16 : 245– 57 [Google Scholar]
- 86. Lieberman-Aiden E , van Berkum NL , Williams L , Imakaev M , Ragoczy T et al. 2009 . Comprehensive mapping of long-range interactions reveals folding principles of the human genome. Science 326 : 289– 93 [Google Scholar]
- 87. Nora EP , Lajoie BR , Schulz EG , Giorgetti L , Okamoto I et al. 2012 . Spatial partitioning of the regulatory landscape of the X-inactivation centre. Nature 485 : 381– 85 [Google Scholar]
- 88. Noordermeer D , Leleu M , Schorderet P , Joye E , Chabaud F , Duboule D 2014 . Temporal dynamics and developmental memory of 3D chromatin architecture at Hox gene loci. eLife 3 : e02557 [Google Scholar]
- 89. Rao SS , Huntley MH , Durand NC , Stamenova EK , Bochkov ID et al. 2014 . A 3D map of the human genome at kilobase resolution reveals principles of chromatin looping. Cell 159 : 1665– 80 [Google Scholar]
- 90. Dixon JR , Selvaraj S , Yue F , Kim A , Li Y et al. 2012 . Topological domains in mammalian genomes identified by analysis of chromatin interactions. Nature 485 : 376– 80 [Google Scholar]
- 91. Pope BD , Ryba T , Dileep V , Yue F , Wu W et al. 2014 . Topologically associating domains are stable units of replication-timing regulation. Nature 515 : 402– 5 [Google Scholar]
- 92. Javierre BM , Burren OS , Wilder SP , Kreuzhuber R , Hill SM et al. 2016 . Lineage-specific genome architecture links enhancers and non-coding disease variants to target gene promoters. Cell 167 : 1369– 84.e19 [Google Scholar]
- 93. Conesa A , Madrigal P , Tarazona S , Gomez-Cabrero D , Cervera A et al. 2016 . A survey of best practices for RNA-seq data analysis. Genome Biol 17 : 13 [Google Scholar]
- 94. Derrien T , Estellé J , Marco Sola S , Knowles DG , Raineri E et al. 2012 . Fast computation and applications of genome mappability. PLOS ONE 7 : e30377 [Google Scholar]
- 95. Johnson DS , Mortazavi A , Myers RM , Wold B 2007 . Genome-wide mapping of in vivo protein-DNA interactions. Science 316 : 1497– 502 [Google Scholar]
- 96. Krueger F , Kreck B , Franke A , Andrews SR 2012 . DNA methylome analysis using short bisulfite sequencing data. Nat. Methods 9 : 145– 51 [Google Scholar]
- 97. Zerbino DR , Wilder SP , Johnson N , Juettemann T , Flicek PR 2015 . The Ensembl regulatory build. Genome Biol 16 : 56 [Google Scholar]
- 98. Harrison PW , Fan J , Richardson D , Clarke L , Zerbino D et al. 2018 . FAANG, establishing metadata standards, validation and best practice for the farmed and companion animal community. Anim. Genet. 49 : 520– 26 [Google Scholar]
- 99. Wilkinson MD , Dumontier M , Aalbersberg IJ , Appleton G , Axton M et al. 2016 . The FAIR Guiding Principles for scientific data management and stewardship. Sci. Data 3 : 160018 [Google Scholar]
- 100. Raney BJ , Dreszer TR , Barber GP , Clawson H , Fujita PA et al. 2014 . Track data hubs enable visualization of user-defined genome-wide annotations on the UCSC Genome Browser. Bioinformatics 30 : 1003– 5 [Google Scholar]
- 101. Casper J , Zweig AS , Villarreal C , Tyner C , Speir ML et al. 2018 . The UCSC Genome Browser database: 2018 update. Nucleic Acids Res 46 : D762– D69 [Google Scholar]
- 102. Zerbino DR , Achuthan P , Akanni W , Amode MR , Barrell D et al. 2018 . Ensembl 2018. Nucleic Acids Res 46 : D754– D61 [Google Scholar]
- 103. Sloan CA , Chan ET , Davidson JM , Malladi VS , Strattan JS et al. 2016 . ENCODE data at the ENCODE portal. Nucleic Acids Res 44 : D726– 32 [Google Scholar]
- 104. Bujold D , Morais DAL , Gauthier C , Côté C , Caron M et al. 2016 . The International Human Epigenome Consortium Data Portal. Cell Syst 3 : 496– 9.e2 [Google Scholar]
- 105. Misztal I , Legarra A 2017 . Invited review: efficient computation strategies in genomic selection. Animal 11 : 731– 36 [Google Scholar]
- 106. Veerkamp RF , Bouwman AC , Schrooten C , Calus MP 2016 . Genomic prediction using preselected DNA variants from a GWAS with whole-genome sequence data in Holstein-Friesian cattle. Genet. Sel. Evol. 48 : 95 [Google Scholar]
- 107. Brøndum RF , Su G , Janss L , Sahana G , Guldbrandtsen B et al. 2015 . Quantitative trait loci markers derived from whole genome sequence data increases the reliability of genomic prediction. J. Dairy Sci. 98 : 4107– 16 [Google Scholar]
- 108. MacLeod IM , Bowman PJ , Vander Jagt CJ , Haile-Mariam M , Kemper KE et al. 2016 . Exploiting biological priors and sequence variants enhances QTL discovery and genomic prediction of complex traits. BMC Genom 17 : 144 [Google Scholar]
- 109. Pérez-Enciso M , Rincón JC , Legarra A 2015 . Sequence- vs. chip-assisted genomic selection: Accurate biological information is advised. Genet. Sel. Evol. 47 : 43 [Google Scholar]
- 110. Schaub MA , Boyle AP , Kundaje A , Batzoglou S , Snyder M 2012 . Linking disease associations with regulatory information in the human genome. Genome Res 22 : 1748– 59 [Google Scholar]
- 111. Kircher M , Witten DM , Jain P , O'Roak BJ , Cooper GM , Shendure J 2014 . A general framework for estimating the relative pathogenicity of human genetic variants. Nat. Genet. 46 : 310– 15 [Google Scholar]
- 112. Gulko B , Hubisz MJ , Gronau I , Siepel A 2015 . A method for calculating probabilities of fitness consequences for point mutations across the human genome. Nat. Genet. 47 : 276– 83 [Google Scholar]
- 113. Nguyen QH , Tellam RL , Naval-Sanchez M , Porto-Neto LR , Barendse W et al. 2018 . Mammalian genomic regulatory regions predicted by utilizing human genomics, transcriptomics, and epigenetics data. Gigascience 7 : 1– 17 [Google Scholar]
- 114. Wang M , Hancock TP , MacLeod IM , Pryce JE , Cocks BG , Hayes BJ 2017 . Putative enhancer sites in the bovine genome are enriched with variants affecting complex traits. Genet. Sel. Evol. 49 : 56 [Google Scholar]
- 115. Bouwman AC , Daetwyler HD , Chamberlain AJ , Ponce CH , Sargolzaei M et al. 2018 . Meta-analysis of genome-wide association studies for cattle stature identifies common genes that regulate body size in mammals. Nat. Genet. 50 : 362– 67 [Google Scholar]
- 116. Koufariotis LT , Chen YP , Stothard P , Hayes BJ 2018 . Variance explained by whole genome sequence variants in coding and regulatory genome annotations for six dairy traits. BMC Genom 19 : 237 [Google Scholar]
- 117. Klann TS , Black JB , Gersbach CA 2018 . CRISPR-based methods for high-throughput annotation of regulatory DNA. Curr. Opin. Biotechnol. 52 : 32– 41 [Google Scholar]
- 118. Lau CH , Suh Y 2018 . CRISPR-based strategies for studying regulatory elements and chromatin structure in mammalian gene control. Mamm. Genome 29 : 205– 28 [Google Scholar]
- 119. Claussnitzer M , Dankel SN , Kim KH , Quon G , Meuleman W et al. 2015 . FTO obesity variant circuitry and adipocyte browning in humans. N. Engl. J. Med. 373 : 895– 907 [Google Scholar]
- 120. Hanssen LLP , Kassouf MT , Oudelaar AM , Biggs D , Preece C et al. 2017 . Tissue-specific CTCF-cohesin-mediated chromatin architecture delimits enhancer interactions and function in vivo. Nat . . Cell Biol 19 : 952– 61 [Google Scholar]
- 121. Wu Y , Zeng J , Zhang F , Zhu Z , Qi T et al. 2018 . Integrative analysis of omics summary data reveals putative mechanisms underlying complex traits. Nat. Commun. 9 : 918 [Google Scholar]
- 122. Kungulovski G , Jeltsch A 2016 . Epigenome editing: state of the art, concepts, and perspectives. Trends Genet 32 : 101– 13 [Google Scholar]
- 123. Hilton IB , D'Ippolito AM , Vockley CM , Thakore PI , Crawford GE et al. 2015 . Epigenome editing by a CRISPR-Cas9-based acetyltransferase activates genes from promoters and enhancers. Nat. Biotechnol. 33 : 510– 17 [Google Scholar]
- 124. Morgan SL , Mariano NC , Bermudez A , Arruda NL , Wu F et al. 2017 . Manipulation of nuclear architecture through CRISPR-mediated chromosomal looping. Nat. Commun. 8 : 15993 [Google Scholar]
- 125. Powell RH , Behnke MS 2017 . WRN conditioned media is sufficient for in vitro propagation of intestinal organoids from large farm and small companion animals. Biol. Open 6 : 698– 705 [Google Scholar]
- 126. Khalil HA , Lei NY , Brinkley G , Scott A , Wang J et al. 2016 . A novel culture system for adult porcine intestinal crypts. Cell Tissue Res 365 : 123– 34 [Google Scholar]
- 127. van der Hee B , Loonen LMP , Taverne N , Taverne-Thiele JJ , Smidt H , Wells JM 2018 . Optimized procedures for generating an enhanced, near physiological 2D culture system from porcine intestinal organoids. Stem Cell Res 28 : 165– 71 [Google Scholar]
- 128. Meijerink E , Neuenschwander S , Fries R , Dinter A , Bertschinger HU et al. 2000 . A DNA polymorphism influencing α(1,2)fucosyltransferase activity of the pig FUT1 enzyme determines susceptibility of small intestinal epithelium to Escherichia coli F18 adhesion. Immunogenetics 52 : 129– 36 [Google Scholar]
- 129. Driehuis E , Clevers H 2017 . CRISPR/Cas 9 genome editing and its applications in organoids. Am. J. Physiol. Gastrointest. Liver Physiol. 312 : G257– G65 [Google Scholar]
- 130. MacHugh DE , Larson G , Orlando L 2017 . Taming the past: ancient DNA and the study of animal domestication. Annu. Rev. Anim. Biosci. 5 : 329– 51 [Google Scholar]
- 131. Schubert M , Jónsson H , Chang D , Der Sarkissian C , Ermini L et al. 2014 . Prehistoric genomes reveal the genetic foundation and cost of horse domestication. PNAS 111 : E5661– 69 [Google Scholar]
- 132. Gaunitz C , Fages A , Hanghøj K , Albrechtsen A , Khan N et al. 2018 . Ancient genomes revisit the ancestry of domestic and Przewalski's horses. Science 360 : 111– 14 [Google Scholar]
- 133. Librado P , Gamba C , Gaunitz C , Der Sarkissian C , Pruvost M et al. 2017 . Ancient genomic changes associated with domestication of the horse. Science 356 : 442– 45 [Google Scholar]
- 134. Librado P , Der Sarkissian C , Ermini L , Schubert M , Jónsson H et al. 2015 . Tracking the origins of Yakutian horses and the genetic basis for their fast adaptation to subarctic environments. PNAS 112 : E6889– 97 [Google Scholar]
- 135. Tilgner H , Jahanbani F , Blauwkamp T , Moshrefi A , Jaeger E et al. 2015 . Comprehensive transcriptome analysis using synthetic long-read sequencing reveals molecular co-association of distant splicing events. Nat. Biotechnol. 33 : 736– 42 [Google Scholar]
- 136. Mercer TR , Gerhardt DJ , Dinger ME , Crawford J , Trapnell C et al. 2011 . Targeted RNA sequencing reveals the deep complexity of the human transcriptome. Nat. Biotechnol. 30 : 99– 104 [Google Scholar]
- 137. Lagarde J , Uszczynska-Ratajczak B , Carbonell S , Pérez-Lluch S , Abad A et al. 2017 . High-throughput annotation of full-length long noncoding RNAs with capture long-read sequencing. Nat. Genet. 49 : 1731– 40 [Google Scholar]
- 138. Jain M , Fiddes IT , Miga KH , Olsen HE , Paten B , Akeson M 2015 . Improved data analysis for the MinION nanopore sequencer. Nat. Methods 12 : 351– 56 [Google Scholar]
- 139. Takahashi H , Lassmann T , Murata M , Carninci P 2012 . 5′ End-centered expression profiling using cap-analysis gene expression and next-generation sequencing. Nat. Protoc. 7 : 542– 61 [Google Scholar]
- 140. Batut P , Gingeras TR 2013 . RAMPAGE: promoter activity profiling by paired-end sequencing of 5′-complete cDNAs. Curr. Protoc. Mol. Biol. 104 : 25B.11.1– 16 [Google Scholar]
- 141. Chang H , Lim J , Ha M , Kim VN 2014 . TAIL-seq: genome-wide determination of poly(A) tail length and 3′ end modifications. Mol. Cell 53 : 1044– 52 [Google Scholar]
- 142. Mifsud B , Tavares-Cadete F , Young AN , Sugar R , Schoenfelder S et al. 2015 . Mapping long-range promoter contacts in human cells with high-resolution capture Hi-C. Nat. Genet. 47 : 598– 606 [Google Scholar]
- 143. Ramani V , Cusanovich DA , Hause RJ , Ma W , Qiu R et al. 2016 . Mapping 3D genome architecture through in situ DNase Hi-C. Nat. Protoc. 11 : 2104– 21 [Google Scholar]
- 144. Mumbach MR , Rubin AJ , Flynn RA , Dai C , Khavari PA et al. 2016 . HiChIP: efficient and sensitive analysis of protein-directed genome architecture. Nat. Methods 13 : 919– 22 [Google Scholar]
- 145. Fang R , Yu M , Li G , Chee S , Liu T et al. 2016 . Mapping of long-range chromatin interactions by proximity ligation-assisted ChIP-seq. Cell Res 26 : 1345– 48 [Google Scholar]
Data & Media loading...
Supplementary Data
Download Supplemental Figure 1 (PDF).
Download Supplemental Table 1 (PDF).
- Article Type: Review Article
Most Read This Month
Most cited most cited rss feed, effects of heat stress on postabsorptive metabolism and energetics, porcine reproductive and respiratory syndrome virus (prrsv): pathogenesis and interaction with the immune system, amino acid nutrition in animals: protein synthesis and beyond, african swine fever epidemiology and control, genomic selection in dairy cattle: the usda experience *, the genome 10k project: a way forward, animal models of aging research: implications for human aging and age-related diseases *, accelerating improvement of livestock with genomic selection, 1000 bull genomes project to map simple and complex genetic traits in cattle: applications and outcomes, porcine circovirus type 2 (pcv2): pathogenesis and interaction with the immune system.
previous episode
Binning and functional annotation, lesson home, functional annotation.
Overview Teaching: 40 min Exercises: 10 min Questions How can we add functional annotation to our bins? Objectives Define what funtional annotation is Know how to use prokka for functional annotation
What is functional annotation?
Now we have our binned MAGs, we can start to think about what functions genes contained within their genomes do. We can do this via functional annotation - a way to collect information about and describe a DNA sequence.
Next lesson we will talk about taxonomic annotation , which tells us which organisms are present in the metagenome assembly. This lesson, however, we will do some brief functional annotation to get more information about the potential metabolic capacity of the organism we are annotating. This is possible because there is software available which uses features in DNA sequences to predict where genes start and end, allowing us to predict which genes are in our MAGs.
A high quality functional annotation is important because it is very useful for lots of downstream analyses. For instance, if we were looking for genes that have a particular function, we would only be able to do that if we were able to predict the location of the genes in these assemblies.
For example, the paper this data is pulled from uses functional annotation of MAGs to look for genes associated with denitrification pathways. The abundance of these genes is then linked to N 2 O flux rates at different sites.
In this lesson we will only be doing a very small amount of functional annotation using the tool Prokka for rapid prokaryotic genome annotation. This is intended as a taster to give you an idea what you can use your MAGs for. There are many other routes to be taken regarding functional annotation, some of which will be discussed briefly at the end of this episode.
As with taxonomic annotation, effectiveness is determined by the database that the MAG sequence is being compared to. If you do not use the appropriate database you may not end up with many annotated sequences. In particular, Prokka (the tool we will use in this episode) annotates archaea and bacterial genomes. If you are trying to annotate a fungal genome or a eukaryote, you will need to use something different.
How do we perform functional annotation?
Software choices We are using Prokka here as it is still the software most commonly used. However, the program is no longer being updated. One recent alternative that is being actively developed is Bakta .
Prokka identifies candidate genes in a iterative process. First it uses Prodigal (another command line tool) to find candidate genes.These are then compared against databases of known protein sequences in order to determine their function. If you like, you can read more about Prokka in this 2014 paper .
Prokka has been pre-installed on our instance. First, let’s create a directory inside analysis where we can store our outputs from Prokka.
For now we will annotate just one MAG at a time with Prokka. In the previous episode we produced 90 MAGs of varying quality. In this example, we will start with the MAG bin.45.fa , as this MAG had the fairly high completeness (57.76%) and only 1.72% contamination.
Before we start we’ll need to activate a conda environment to run the software.
Activating an environment
Environments are a way of installing a piece of software so that it is isolated, so that things installed within an environment, do not affect other software installed at system wide level. For some pieces of software, the requirements for different dependency versions, such different versions of python mean this is an easy way to have multiple pieces of software installed without conflicts. One popular way to manage environments is to use conda which is a popular environment manager. We will not discuss using conda in detail, so for further information of how to use it, here is a Carpentries course that covers how to use conda in more detail.
For this course we have created a conda environment containing prokka. In order to use this we will need to use the conda activate command:
You will be able to tell you have activated your environment because your prompt should go from looking like this, with (base) at the beginning…
…to having (prokka) at the beginning. If you forget whether you are in an the prokka environment, look back to see what the prompt looks like.
Now let’s take a look at the help page for Prokka using the -h flag.
Prokka Help documentation Name: Prokka 1.12 by Torsten Seemann <[email protected]> Synopsis: rapid bacterial genome annotation Usage: prokka [options] <contigs.fasta>General: --help This help --version Print version and exit --docs Show full manual/documentation --citation Print citation for referencing Prokka --quiet No screen output (default OFF) --debug Debug mode: keep all temporary files (default OFF) Setup: --listdb List all configured databases --setupdb Index all installed databases --cleandb Remove all database indices --depends List all software dependencies Outputs: --outdir [X] Output folder [auto] (default '') --force Force overwriting existing output folder (default OFF) --prefix [X] Filename output prefix [auto] (default '') --addgenes Add 'gene' features for each 'CDS' feature (default OFF) --addmrna Add 'mRNA' features for each 'CDS' feature (default OFF) --locustag [X] Locus tag prefix [auto] (default '') --increment [N] Locus tag counter increment (default '1') --gffver [N] GFF version (default '3') --compliant Force Genbank/ENA/DDJB compliance: --addgenes --mincontiglen 200 --centre XXX (default OFF) --centre [X] Sequencing centre ID. (default '') --accver [N] Version to put in Genbank file (default '1') Organism details: --genus [X] Genus name (default 'Genus') --species [X] Species name (default 'species') --strain [X] Strain name (default 'strain') --plasmid [X] Plasmid name or identifier (default '') Annotations: --kingdom [X] Annotation mode: Archaea|Bacteria|Mitochondria|Viruses (default 'Bacteria') --gcode [N] Genetic code / Translation table (set if --kingdom is set) (default '0') --gram [X] Gram: -/neg +/pos (default '') --usegenus Use genus-specific BLAST databases (needs --genus) (default OFF) --proteins [X] FASTA or GBK file to use as 1st priority (default '') --hmms [X] Trusted HMM to first annotate from (default '') --metagenome Improve gene predictions for highly fragmented genomes (default OFF) --rawproduct Do not clean up /product annotation (default OFF) --cdsrnaolap Allow [tr]RNA to overlap CDS (default OFF) Computation: --cpus [N] Number of CPUs to use [0=all] (default '8') --fast Fast mode - only use basic BLASTP databases (default OFF) --noanno For CDS just set /product="unannotated protein" (default OFF) --mincontiglen [N] Minimum contig size [NCBI needs 200] (default '1') --evalue [n.n] Similarity e-value cut-off (default '1e-06') --rfam Enable searching for ncRNAs with Infernal+Rfam (SLOW!) (default '0') --norrna Don't run rRNA search (default OFF) --notrna Don't run tRNA search (default OFF) --rnammer Prefer RNAmmer over Barrnap for rRNA prediction (default OFF)
Looking at the help page tells us how to construct our basic command, which looks like this:
- --outdir mydir tells Prokka that the ‘output directory’ is mydir
- --prefix mygenome tells Prokka that the output files should all be labelled mygenome
- contigs.fa is the file we want Prokka to annotate
Prokka produces multiple different file types, which you can see in the table below. We are mainly interested in .faa and .tsv but many of the other files are useful for submission to different databases.
Suffix | Description of file contents |
---|---|
.fna | FASTA file of original input contigs (nucleotide) |
.faa | FASTA file of translated coding genes (protein) |
.ffn | FASTA file of all genomic features (nucleotide) |
.fsa | Contig sequences for submission (nucleotide) |
.tbl | Feature table for submission |
.sqn | Sequin editable file for submission |
.gbk | Genbank file containing sequences and annotations |
.gff | GFF v3 file containing sequences and annotations |
.log | Log file of Prokka processing output |
.txt | Annotation summary statistics |
.tsv | Tab-separated file of all features: locus_tag,ftype,len_bp,gene,EC_number,COG,product |
This should take around 1-2 minutes on the instance so we will not be running the command in the background.
Exercise 1: Recap of Prokka command Test yourself! What do each of these parts of the command signal? --outdir bin.45 --prefix bin.45 ../binning/assembly_ERR5000342.fasta.metabat-bins1500-YYYMMDD_HHMMSS/bin.45.fa Solution bin.45 is the name of the directory where Prokka will place its output files bin.45 will be the name of each output file e.g. bin.45.tsv or bin.45.faa This is the file path for the file we want Prokka to annotate
When you initially run the command you should see similar to the following.
And you should see the following when the command has finished:
Now prokka has finished running, we can exit the conda environment and our prompt should return to base . In order to do this we need to use the conda deactivate command, which is as follows:
Your prompt should return from something like this:
If we navigate into the bin.45 output file we can use ls to see that Prokka has generated many files.
As mentioned previously, the two files we are most interested in are those with the extension .tsv and .faa :
- the .tsv file contains information about every gene identified by Prokka
- the .faa file is a FASTA file containing the amino acid sequence of every gene that has been identified.
We can take a look at the .tsv file using head .
This file gives us a list of all the sequences that Prokka has identified as being protein-coding, along with the gene name (if there is one) and the protein product (again, if there is one).
You will notice that some of the output are labelled simply “hypothetical protein”. This means the locus in questions looks like a protein-coding gene, but there isn’t a match for it in any of the databases used by Prokka to label genes.
Others have a gene and product name, meaning Prokka was able to successfully identify them as a specific gene. The product column tells you the name of the protein this gene codes for.
We can then look at the .faa file to see the sequences of these proteins.
Now we have information about the various genes (and the proteins they code for) present in one of our bins. What can we do with this information?
Relating genes to an online database
There are tools available which allow you to visualise the proteins in your bin and how they fit into different metabolic pathways. Some of these are available through your browser.
One such tool is BlastKOALA , where you can upload the .faa file we just looked at and get back a breakdown of the proteins mapped to the KEGG database (a database of molecular interaction maps). The output looks like this:
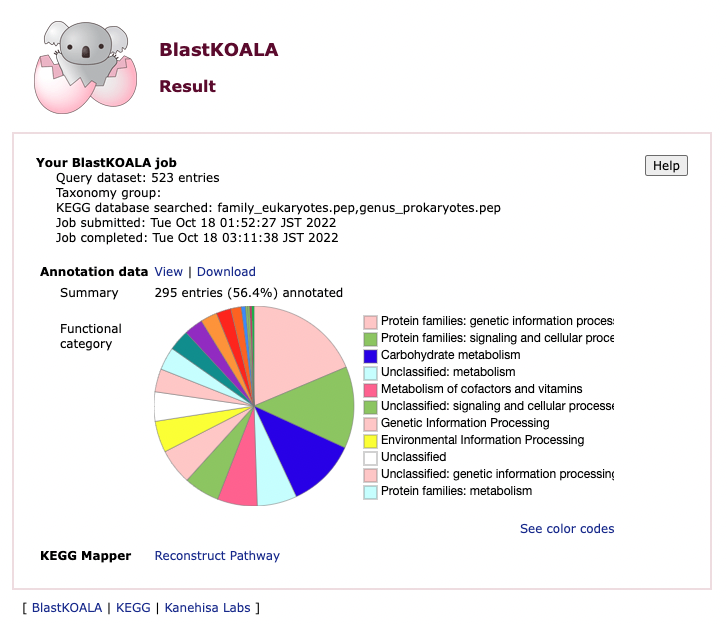
Using an annotation tool like this can help you understand more about the genes and pathways present in your sample(s). For example, as previously described, the paper this data is pulled from uses functional annotation of MAGs to look for genes associated with denitrification pathways.
Building a tree from the 16S sequence
Another option is to build a taxonomic tree to see what organisms your MAG is related to. This is possible using 16S rRNA sequences, which Prokka identifies automatically during its analysis.
Once you have an rRNA sequence you can run it through a search tool such as BLAST to find sequences which match it and what species they belong to.
We won’t be covering how to do this in detail as part of this course but you can read some instructions on how to use both BlastKOALA and BLAST towards the end of this episode of our previous Metagenomics course.
Key Points Functional annotation allows us to look at the metabolic capacity of a metagenome Prokka can be used to predict genes in our assembly
Thank you for visiting nature.com. You are using a browser version with limited support for CSS. To obtain the best experience, we recommend you use a more up to date browser (or turn off compatibility mode in Internet Explorer). In the meantime, to ensure continued support, we are displaying the site without styles and JavaScript.
- View all journals
- Explore content
- About the journal
- Publish with us
- Sign up for alerts
- Open access
- Published: 29 January 2018
GO FEAT: a rapid web-based functional annotation tool for genomic and transcriptomic data
- Fabricio Almeida Araujo 1 ,
- Debmalya Barh 2 ,
- Artur Silva ORCID: orcid.org/0000-0002-4082-1132 1 ,
- Luis Guimarães 1 &
- Rommel Thiago Juca Ramos 1
Scientific Reports volume 8 , Article number: 1794 ( 2018 ) Cite this article
31k Accesses
73 Citations
Metrics details
- Computational platforms and environments
- Data integration
Downstream analysis of genomic and transcriptomic sequence data is often executed by functional annotation that can be performed by various bioinformatics tools and biological databases. However, a full fast integrated tool is not available for such analysis. Besides, the current available software is not able to produce analytic lists of annotations and graphs to help users in evaluating the output results. Therefore, we present the Gene Ontology Functional Enrichment Annotation Tool (GO FEAT), a free web platform for functional annotation and enrichment of genomic and transcriptomic data based on sequence homology search. The analysis can be customized and visualized as per users’ needs and specifications. GO FEAT is freely available at http://computationalbiology.ufpa.br/gofeat/ and its source code is hosted at https://github.com/fabriciopa/gofeat .
Similar content being viewed by others
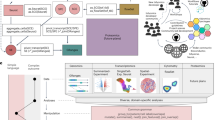
The tidyomics ecosystem: enhancing omic data analyses
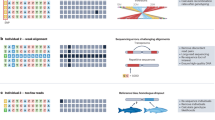
Next-generation data filtering in the genomics era
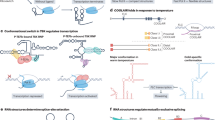
Identification of RNA structures and their roles in RNA functions
Introduction.
Giving biological meaning to genomic and transcriptomic data is laborious and time consuming, especially considering the large amount of data generated by high-throughput technologies 1 and the number of tools, web-servers and databases developed for this purpose 2 . The biological analysis is often given by functional annotation through Gene Ontology (GO) database 3 which is widely used as the gene functions dictionary. Besides, it’s very usual to perform data functional enrichment by the integration of several databases such as: UniProt 4 , InterPro 5 , KEGG 6 , Pfam 7 , NCBI 8 and SEED 9 .
Many tools are available for the annotation process: Blast2GO 10 , AmiGO 11 , GOrilla 12 , REVIGO 13 , QuickGO 14 , NaviGO 15 . However, these tools have limitations: a) not all are completely and freely available; b) installation, configuration and command line are complex; c) lack of visual interface; d) limited capacity or sequence number limitation for analysis e) difficulty to share and export results. To address these issues, we developed GO FEAT, a free, on-line, user friendly platform for functional annotation and enrichment of genomic and transcriptomic data based on sequence homology search, allowing users to export the results to different output formats, to generate reports, tables, GO charts and graphs that help them with downstream analysis.
GO FEAT is developed in PHP as back-end programming language. HTML5, CSS3 and JavaScript are used as front-end programming language, and PERL is adapted for remote connection scripts. To store the records from the tool we used MySQL RDBMS. All remote calling is made by public REST API (EMBL-EBI’s public API for Blast, UniProt for database integration, QuickGO for ontologies and SEED’s public API for SEED). The user can share their data to other users, export data to several formats, and generate Gene Ontology charts (general and by type of ontology).
GO FEAT receives a multi-fasta file (nucleotide or protein) as an input, once a project is registered or assigned. The pipeline (Fig. 1 ) proceed to search for homology with e-value defined by the user and then annotate the homologs using public databases. After the submission, each sequence is queued to the processing line. The processing starts with the remote BLAST 16 using the EMBL-EBI public API 17 or local DIAMOND 18 aligner. GO FEAT automatically identifies the type of sequence to be searched (nucleotide or protein) and runs the specific program: BLASTx for nucleotide sequences or BLASTp for protein sequences. The next step is to integrate the result from the alignment to UniProt, NCBI Protein, KEGG, InterPro, Pfam and Gene Ontology databases via UniProt public API and SEED database via SEED public API. After the integration, the results are processed and displayed in graphs, charts, and tables to simplify the analysis.
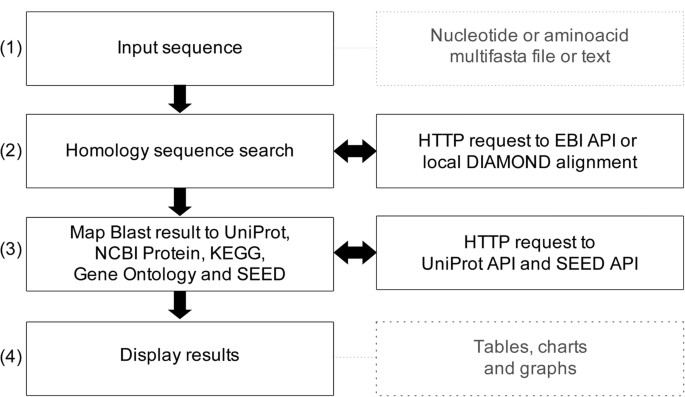
GO FEAT pipeline steps. (1) A multi-fasta file containing any number of sequences (nucleotide or protein) is used as input. (2) Each sequence is used as query against EBI database through EBI public API or local DIAMOND. (3) The alignment results are mapped to UniProt, NCBI Protein, KEGG, GO databases by UniProt public API and SEED database by SEED public API. Finally, (4) the results are displayed in tables, charts and graphs.
Since the EBI servers restrict the number of request to 30 at time, a queue control parameter was developed to optimize the server’s resources. For projects with 100 or less sequences, resources are allocated dynamically for maximum of 10 users simultaneously (3 requests for each project). If resources are available, the projects can receive more than 3 requests. Projects with more than 100 sequences are put in a queue for local alignment using DIAMOND that process batches of 500 sequences at a time. This allows the server’s resources usage to be optimized and more sequences can be processed at the same time.
To compare the results from GO FEAT with other tools, we performed the functional annotation in six different scenarios: a random sequence with 500 bp from Escherichia coli ; the full genome of Escherichia coli K-12 MG1655 (4140 CDS and average size of 321 bp) [RefSeq NC_000913.3]; the full genome of Drosophila melanogaster BDGP6 (30482 CDS and CDS average size of 668 bp) [Assembly GCA_000001215.4]; the full genome of Nostoc sp. PCC 7107 (5237 CDS and CDS average size of 330 bp) [RefSeq NC_019676.1]; the transcriptomic data from E. coli response to five different perturbations (4092 CDS and CDS average size of 326 bp); 19 and the transcriptomic data from M. tuberculosis response to macrophages (4076 CDS and CDS average size of 332 bp) 20 . The results of this comparison are shown in the next section.
GO FEAT was developed to be executed in any modern internet browser. Also, it has a clean and easy-to-use graphic interface. It’s not required any kind of installation of any tool or software and users can execute projects without previous registration.
Project manager
GO FEAT provides a project manager to facilitate the categorization of each analysis performed by registered users. In the project manager, it’s possible to check the project’s progress, export data to several formats and share projects to other people users to avoid running the same project multiple times.
Reports, charts and graphs
GO FEAT allows different ways for result visualization: spreadsheet reports present sequences in tables corresponding to its Blast result, which are integrated to several databases, to perform searches and export results; it’s also possible to view the results in graphs and charts, which are divided by molecular function, cellular component and biological process. On each one, it is possible to view all GO terms of each category together with the sequences identification. Finally, the user can view the GO terms with its acyclic graph, downloaded through the Quick GO API.
Benchmarking
For a 500 bp random sequence chosen from Escherichia coli ’s genome, GO FEAT takes around 4 minutes for full functional annotation and enrichment while Blast2GO takes around 14 minutes for the same sequence. Direct Blast to NCBI website takes around 2 minutes, however, the mapping between the blast result and other databases are not automatically made. At UniProt, the function annotation and enrichment takes around 2 minutes. Since NCBI’s Blast does not perform a full functional annotation and UniProt website has limitations regarding the number of sequences, they will not be included in further analysis. For complete genomes of model organisms, GO FEAT needs around 5 hours for Escherichia coli and 30 hours for Drosophila melanogaster . For transcriptomic data, 5 hours were required to peform the functional annotation described in the Jozefczuk’s paper and 5 hours to perform the functional annotation described in Rohde’s paper. Blast2GO was unable to perform the full annotation and enrichment of any complete genome or transcriptomic data analysis in less than 10 days. For non-model organism such as Nostoc sp. PCC 7107, around 4 hours is required to finish the processing in GO FEAT. The time varies depending on server loads of the remote APIs. At full load, around 11 hours was necessary to process 10 projects, each one with 1000 different sequences from Drosophila melanogaster and CDS average size of 603 bp. Regarding functionalities, GO FEAT presents useful features in comparison to other functional annotation tools (Table 1 ) and rapidly process the input sequence and generates the results.
Limitations
GO FEAT was developed to perform functional annotations on previously predicted genes, coding DNA sequences (CDS), open reading frames (ORF) or transcripts. Thus, large sequences, such as full genomes or contigs, are not suitable to be used as inputs in GO FEAT due to size limitation of alignment softwares.
Conclusions
Functional characterization of biological sequences is a required step in the analysis of biological data. GO FEAT is an annotation platform integrated with several databases which can be used for different datasets, such as: coding sequences identified after gene prediction and sequences produced after new sequence assembly of next-generation sequencing data. The user can share results with collaborators through graphic interface and can export the results to many formats. Since the tool uses API to access various databases, the annotations are based on most recent and updated data from those databases.
We are committed to maintain GO FEAT for at least 2 years and expect to improve its performance as our computational infrastructure grows. For future works, we plan on adding a prediction step before the functional annotation so users can input large sequences, exporting the predicted sequences.
Goodwin, S., McPherson, J. D. & McCombie, W. R. Coming of age: ten years of next-generation sequencing technologies. Nature Reviews Genetics 17 , 333–351 (2016).
Article CAS PubMed Google Scholar
Kumar, S. & Dudley, J. Bioinformatics software for biologists in the genomics era. Bioinformatics 23 , 1713–1717 (2007).
Ashburner, M. et al . Gene ontology: tool for the unification of biology. Nature Genetics 25 , 25–29 (2009).
Article Google Scholar
Uniprot: the universal protein knowledgebase. Nucleic Acids Research 45 , D158–D169 (2017).
Finn, R., Attwood, T. & Babbitt, P. et al . Interpro in 2017—beyond protein family and domain annotations. Nucleic Acids Research 45 (Database issue), D190–D199 (2017).
Article PubMed Google Scholar
Kanehisa, M., Furumichi, M., Tanabe, M., Sato, Y. & Morishima, K. Kegg: new perspectives on genomes, pathways, diseases and drugs. Nucleic Acids Research 45 , D353–D361 (2017).
Finn, R. D. et al . Pfam: clans, web tools and services. Nucleic Acids Research 34 , D247–D251 (2006).
Database resources of the national center for biotechnology information. Nucleic Acids Research 44 , D7–D19 (2016).
Overbeek, R. et al . The seed and the rapid annotation of microbial genomes using subsystems technology (rast). Nucleic Acids Research 42 , D206–D214 (2014).
Conesa, A. et al . Blast2go: a universal tool for annotation, visualization and analysis in functional genomics research. Bioinformatics 21 , 3674–3676 (2005).
Carbon, S. et al . Amigo: online access to ontology and annotation data. Bioinformatics 25 , 288–289 (2009).
Eden, E., Navon, R., Steinfeld, I., Lipson, D. &Yakhini, Z. Gorilla: a tool for discovery and visualization of enriched go terms in ranked gene lists. BMC Bioinformatics 10 (2009).
Supek, F., Bošnjak, M., Škunca, N. & Šmuc, T. Revigo summarizes and visualizes long lists of gene ontology terms. PLOS ONE 6 , 1–9 (2011).
Binns, D. et al . Quickgo: a web-based tool for gene ontology searching. Bioinformatics 25 , 3045–3046 (2009).
Article CAS PubMed PubMed Central Google Scholar
Wei, Q., Khan, I., Ding, Z., Yerneni, S. & Kihara, D. Navigo: interactive tool for visualization and functional similarity and coherence analysis with gene ontology. BMC Bioinformatics 18 (2017).
Altschul, S. F., Gish, W., Miller, W., Myers, E. W. & Lipman, D. J. Basic local alignment search tool. Journal of Molecular Biology 215 , 403–410 (1990).
CAS PubMed Google Scholar
Lopez, R., Cowley, A., Li, W. & McWilliam, H. Using embl-ebi services via web interface and programmatically via web services. Current Protocols in Bioinformatics 48 (2014).
Buchfink, B., Xie, C. & Huson, D. H. Fast and sensitive protein alignment using diamond. Nature Methods 12 , 59–60 (2015).
Jozefczuk, S. et al . Metabolomic and transcriptomic stress response of escherichia coli. Molecular Systems Biology 6 (2010).
Rohde, K. H., Abramovitch, R. B. &Russell, D. G. Mycobacterium tuberculosis invasion of macrophages: Linking bacterial gene expression to environmental cues. Cell Host Microbe 2 (2007).
Download references
Acknowledgements
This work has been supported by the CNPq (Conselho Nacional de Pesquisa Científica) grant #421528/2016-8, CAPES (Coordenação de Aperfeiçoamento de Pessoal de Nível Superior) and PROPESP/UFPA (Pró-Reitoria de Pesquisa e Pós-Graduação/Universidade Federal do Pará).
Author information
Authors and affiliations.
Universidade Federal do Pará, Instituto de Ciências Biológicas, Rua Augusto Corrêa, 01 - Guamá, Belém, PA, Brazil
Fabricio Almeida Araujo, Artur Silva, Luis Guimarães & Rommel Thiago Juca Ramos
Centre for Genomics and Applied Gene Technology, Institute of Integrative Omics and Applied Biotechnology (IIOAB), Nonakuri, Purba Medinipur, WB-721172, India
Debmalya Barh
You can also search for this author in PubMed Google Scholar
Contributions
Rommel Thiago Juca Ramos and Fabricio Almeida Araujo conceived the program’s idea and developed it. Debmalya Barh, Artur Silva and Luis Guimarães evaluated the biological informations and defined the databases to be integrated. All authors reviewed the manuscript.
Corresponding author
Correspondence to Rommel Thiago Juca Ramos .
Ethics declarations
Competing interests.
The authors declare that they have no competing interests.
Additional information
Publisher's note: Springer Nature remains neutral with regard to jurisdictional claims in published maps and institutional affiliations.
Rights and permissions
Open Access This article is licensed under a Creative Commons Attribution 4.0 International License, which permits use, sharing, adaptation, distribution and reproduction in any medium or format, as long as you give appropriate credit to the original author(s) and the source, provide a link to the Creative Commons license, and indicate if changes were made. The images or other third party material in this article are included in the article’s Creative Commons license, unless indicated otherwise in a credit line to the material. If material is not included in the article’s Creative Commons license and your intended use is not permitted by statutory regulation or exceeds the permitted use, you will need to obtain permission directly from the copyright holder. To view a copy of this license, visit http://creativecommons.org/licenses/by/4.0/ .
Reprints and permissions
About this article
Cite this article.
Araujo, F., Barh, D., Silva, A. et al. GO FEAT: a rapid web-based functional annotation tool for genomic and transcriptomic data. Sci Rep 8 , 1794 (2018). https://doi.org/10.1038/s41598-018-20211-9
Download citation
Received : 04 October 2017
Accepted : 15 January 2018
Published : 29 January 2018
DOI : https://doi.org/10.1038/s41598-018-20211-9
Share this article
Anyone you share the following link with will be able to read this content:
Sorry, a shareable link is not currently available for this article.
Provided by the Springer Nature SharedIt content-sharing initiative
This article is cited by
Microrna cross-talk between monilinia fungal pathogens and peach host.
- Kubra Arslan
- Hilal Ozkilinc
Phytoparasitica (2024)
Bioinformatics insight in shallow genome sequence: a case study of Corymbia hybrid (C. citriodora × C. torelliana)
- Arzoo Shamoon
- Maneesh S. Bhandari
- Shailesh Pandey
Proceedings of the National Academy of Sciences, India Section B: Biological Sciences (2024)
Genomic and metabolomic insights into the antimicrobial compounds and plant growth-promoting potential of Bacillus velezensis Q-426
- Ruochen Fan
- Chunshan Quan
BMC Genomics (2023)
Chromosome-length genome assembly of Teladorsagia circumcincta – a globally important helminth parasite in livestock
- Shamshad Ul Hassan
- Eng Guan Chua
- Parwinder Kaur
Recent advances in genome annotation and synthetic biology for the development of microbial chassis
- Saltiel Hamese
- Kanganwiro Mugwanda
- Deepak B. Thimiri Govinda Raj
Journal of Genetic Engineering and Biotechnology (2023)
By submitting a comment you agree to abide by our Terms and Community Guidelines . If you find something abusive or that does not comply with our terms or guidelines please flag it as inappropriate.

Quick links
- Explore articles by subject
- Guide to authors
- Editorial policies
Sign up for the Nature Briefing newsletter — what matters in science, free to your inbox daily.

- Open access
- Published: 15 February 2019
FunMappOne: a tool to hierarchically organize and visually navigate functional gene annotations in multiple experiments
- Giovanni Scala 1 , 2 , 3 ,
- Angela Serra 1 , 2 ,
- Veer Singh Marwah 1 , 2 ,
- Laura Aliisa Saarimäki 1 , 2 &
- Dario Greco 1 , 2 , 3
BMC Bioinformatics volume 20 , Article number: 79 ( 2019 ) Cite this article
9291 Accesses
32 Citations
9 Altmetric
Metrics details
Functional annotation of genes is an essential step in omics data analysis. Multiple databases and methods are currently available to summarize the functions of sets of genes into higher level representations, such as ontologies and molecular pathways. Annotating results from omics experiments into functional categories is essential not only to understand the underlying regulatory dynamics but also to compare multiple experimental conditions at a higher level of abstraction. Several tools are already available to the community to represent and compare functional profiles of omics experiments. However, when the number of experiments and/or enriched functional terms is high, it becomes difficult to interpret the results even when graphically represented. Therefore, there is currently a need for interactive and user-friendly tools to graphically navigate and further summarize annotations in order to facilitate results interpretation also when the dimensionality is high.
We developed an approach that exploits the intrinsic hierarchical structure of several functional annotations to summarize the results obtained through enrichment analyses to higher levels of interpretation and to map gene related information at each summarized level. We built a user-friendly graphical interface that allows to visualize the functional annotations of one or multiple experiments at once. The tool is implemented as a R-Shiny application called FunMappOne and is available at https://github.com/grecolab/FunMappOne .
FunMappOne is a R-shiny graphical tool that takes in input multiple lists of human or mouse genes, optionally along with their related modification magnitudes, computes the enriched annotations from Gene Ontology, Kyoto Encyclopedia of Genes and Genomes, or Reactome databases, and reports interactive maps of functional terms and pathways organized in rational groups. FunMappOne allows a fast and convenient comparison of multiple experiments and an easy way to interpret results.
Functional annotation of large sets of significant genes is often the final step of omics data analysis. However, when multiple genes are selected during differential analysis, it becomes almost impossible to understand the altered biological processes by manually inspecting the individual genes. This task is even more difficult when comparing functional profiles derived from two or more related experiments at the gene level, for different sets of functionally related genes may be specifically affected in different experimental conditions.
A multitude of tools are already available to the community to graphically represent enriched functional annotations from single pair-wise comparisons [ 1 – 4 ]. When considering multiple experiments, these methods require to run separate analyses for each experiment and subsequently collate the results for comparison. The complexity of this task increases with the number of considered experiments, especially for users who are not familiar with advanced techniques of data manipulation. Some tools allow the visualization of the enriched Gene Ontology terms from multiple experiments [ 5 – 7 ]. However, as they are typically implemented in R, they require a certain degree of programming expertise in order to produce the desired visualizations. Moreover, since these methods usually offer a static graphical output, the produced plots become difficult to read and interpret when large number of functional terms need to be displayed.
An important aspect of some functional annotations is the possibility to derive a hierarchical structure for their base terms, such as for Kyoto Encyclopedia of Genes and Genomes (KEGG) pathways [ 8 ], Reactome pathways [ 9 ] and Gene Ontology terms [ 10 ]. This structure can be used to organize the functional terms and summarize sets of related functions in super classes. This feature can be further exploited to reduce the dimensionality of sets of enriched terms and to abstract the underlying biological functions to higher levels of interpretation.
Here we present FunMappOne, an R-shiny user-friendly software with a simple graphical interface that takes in input lists of human or mouse genes from multiple experiments, optionally with their gene-associated metrics, such as fold change and p -value. It provides functionalities to statistically evaluate over-represented biological terms from Gene Ontology, KEGG, or Reactome databases, graphically summarize, and navigate them.
The three-level hierarchy
In order to reduce the dimensionality of the sets of enriched terms, we introduced the concept of hierarchical summarization, that is the possibility to explore enriched terms at higher functional levels. To do this, a hierarchy is needed to group terms in super-classes. By definition, this structure needs to be represented as a direct acyclic graph, with a root category (representing the functional annotation) and a series of meta-terms (real terms or functional groups), defining progressively specialized group of terms. This structure is naturally found in the intrinsic organization of KEGG and Reactome pathways while it can be easily derived for Gene Ontology terms, as described in the next section. An important factor for the hierarchy definition and construction is the number of levels of the hierarchy, namely the depth of the corresponding graph structure: KEGG has an intrinsic structure based on three levels, while Reactome pathways and gene ontology can have more than three levels that are not uniformly distributed (the hierarchical chain of meta-terms can have different length for different terms). Having many summarization levels has the advantage of making more specialized grouping of terms but would also complicate the task for the user to reduce the set dimensionality and obtain easier views of the enrichment data. For this reason, we chose to follow the KEGG philosophy and homogenize the three hierarchies (KEGG, Reactome and Gene Ontology) in order to have three levels of summarization from the terms to the root. The detailed implementation of the hierarchies is described in the following section.
Hierarchy definition
Figure 1 shows the implemented procedure to define hierarchical structures for KEGG pathways (panel A), Gene Ontology terms (panel B) and Reactome pathways (panel C), respectively. For each annotation type, a three-level hierarchy was defined.
For KEGG pathways (Fig. 1 a), the three levels of BRITE functional hierarchy was used [ 8 ].
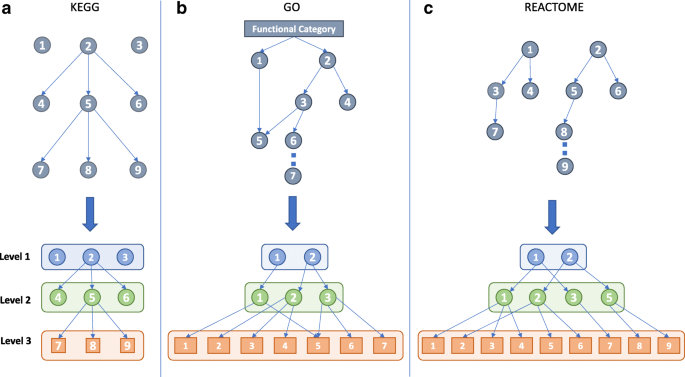
Definition of the hierarchies. For each functional annotation type, a model reflecting the relationship between functional terms and levels in their original structure is shown above the corresponding generated hierarchy. Panel a , b and c report hierarchy generation models for KEGG, Gene Ontology and Reactome, respectively. In the second and third panel, different numbers indicate different functional terms. In panel b , “CAT” can be one of the Gene Ontology categories BP, CC or MF
For each Gene Ontology category CAT (Biological Processes - BP, Cellular Components - CC, and Molecular Functions - MF), a three-level hierarchy was extracted by first considering the graph GO_CAT rooted in CAT (Fig. 1 b). Then, the acyclic directed subgraph GO_CAT_ac was computed by considering only the edges representing the relationship “is_a” or “part_of” in GO_CAT. Finally, a new graph GO_CAT_hier was built by considering all the nodes in GO_CAT_ac, and adding, for each node t i , all the edges in the path [ t i ,…, t r −1 ] if the path [ t i ,…, t r −1 , C A T ] of length at most 3 already existed in GO_CAT_ac. For the paths [ t i ,…, t r −2 , t r −1 , C A T ] in GO_CAT_ac of length greater than 3, only the arcs forming the sequence [ t i , t r −2 , t r −1 ] were added to GO_CAT_hier.
For the Reactome pathways (Fig. 1 C), the set of root nodes Rs were considered and a three-level hierarchy was explicated. First, the associated graph R E A C T _ R S i rooted in CAT was selected. Next, for each node t i the edges [ t i , t r −1 , R S i ] were added if the path [ t i , t r −1 , R S i ] belonged to R E A C T _ R S i . If the path [ t i ,…, t r −2 , t r −1 , R S i ] existed in R E A C T _ R S i , only the edges forming the sequence [ t i , t r −2 , R S i ] were added to the new graph representing the hierarchy.
FunMappOne algorithm workflow
Figure 2 shows the FunMappOne algorithm workflow. The input is provided as N lists of genes, one for each experimental condition to compare and, optionally, N lists of modifications (e.g. the fold-change or the p -value) associated with each gene. For each experiment analyzed, the enriched terms in the chosen functional annotation are computed by using the gProfiler R package [ 4 ], and a matrix Ter[NxM] is created, where M is the total number of enriched terms. Each element Ter[i,j] is associated with the hypergeometric test p -value of term j for the genes in the i-th list. Optionally, Ter[i,j] can also be associated with a value that summarizes the modification values (e.g. the median fold change) of the genes from the i-th list intersecting the gene set of the term j.
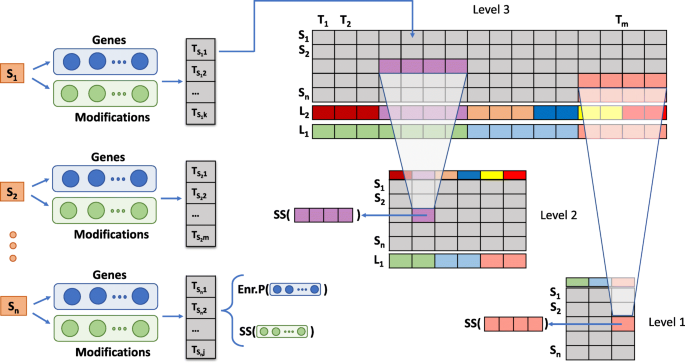
FunMappOne workflow. The tool accepts as input gene lists and modification values for every experimental condition S 1 ,…, S n for which the enrichment will be carried out. The analysis performed on the j-th sample will results in a set of enriched terms T s j 1 ,…, T sjk with an associated p -value (Enr.P) from the enrichment function applied on the gene list, or a value coming from the application of a summary statistic (SS) on the associated modification values. A matrix with n rows associated to samples and m columns associated with the enriched terms is then specified to represent the data structure beneath Level 3 representation of the data. Matrices associated to higher hierarchical levels are composed by n rows and as many columns as the categories of the level. Each cell of a higher level matrix contains a value obtained by applying SS to the terms belonging to the associated category from the Level 3 matrix
To summarize the information at a higher level of interpretation, a new matrix T e r i [NxK] is created, where i =1,2 is the desired height of the chosen annotation hierarchy and K is the number of different terms at level i. Each element T e r i [i,j] is then associated with a summary statics (e.g. the median p -value) of the elements Ter[i,k] for all k such that the term k is a descendant j in the reference hierarchy.
Finally, given a matrix T e r i [NxK] representing the enrichment at level i as defined above, the possibility to reorder and cluster experiments, based on a given distance function D k , l , is implemented. This is computed between the vectors T e r i [k,] and T e r i [l,] using, alternatively, a distance based on the Jaccard index on the number of common enriched terms, the Euclidean distance on the values associated with terms, or a combination of these two.
In the first case, the Jaccard index J k , l is computed as \(\frac {|Terms(k) \cap Terms(l)|}{|Terms(k) \cup Terms(l)|}\) , where T e r m s ( x ) is the set of enriched terms for the experiment x and D k , l is set as 1− J k , l .
In the second case, the set c o m m ( k , l )= T e r m s ( k )∩ T e r m s ( l )| is first considered, where T e r m s ( x ) is the set of enriched terms for the experimental condition x , then if | c o m m ( k , l )|≥0 the Euclidean distance D E k , l on the sub-vectors T e r i [ k , c o m m ] and T e r i [ l , c o m m ] is computed. A combination of the two methods is implemented by creating the mean distance matrix M k =( D + D E 01 )/2, where D is the matrix of the Jaccard index and D E 01 is the Euclidean distance matrix scaled in the range [0,1]. In this way, the experimental conditions are clustered together not only when they share the same enriched terms, but also considering how similar are the enriched terms with respect to their enrichment p -value or summary statistic. A hierarchical clustering function is then applied to the matrix using a linkage method between complete, single and ward.
Results and discussion
The analytical approach presented above was implemented using R-shiny. The typical analysis is performed by three interaction steps: i) input of gene lists and modifications, ii) graphical visualization of enriched terms and iii) interactive navigation of the results. A step-by-step user manual is available in Additional file 1 .
In the first step, the application provides a simple graphical interface, where the user can submit a spreadsheet file with the lists of genes associated to each experimental condition of interest and (optionally) their modification information (e.g. the associated fold change from a differential expression analysis). The input spreadsheet contains a sheet for every experimental condition, named with a condition id. In every sheet, two columns are provided, containing the gene identifiers (Entrez Gene, Gene Symbol, or Ensembl gene ids) and, optionally, their modifications, respectively. Furthermore, an additional sheet is required, containing two columns with the condition id and the condition grouping information, respectively.
The user is then asked to choose the species (human or mouse), a functional annotation (Gene Ontology - BP, Gene Ontology - CC, Gene Ontology - MF, KEGG, Reactome), a summarization function (min, median, mean, max) to annotate and summarize the enriched terms with provided modifications, a p -value correction method (gSCS [ 4 ], bonferroni, fdr), and a statistical significance threshold for the enriched functional terms. If the amplitude of gene modification (e.g. fold change, p -value) is provided, the user selects whether the summarized value of the enriched terms is plotted in a color-scale associated to its value, or with three colors only (negative, zero, positive); this latter feature is useful when emphasis is given to the dominant sign of the modification in the term. Moreover, if gene modification values are provided in the input, the user can choose the type of information that will be associated to the enriched terms: the term enrichment p -value, the provided modification value, or a combination of term enrichment p -values (Enr.P) and modification values (MVs), specified as M V ×− l o g ( E n r . P ). Alternatively, if only gene lists without providing modification values are uploaded, the enrichment p -value for each enriched term will be displayed.
After loading the needed files, a dedicated panel in the software graphical environment shows the content of the provided tables, along with a summary of each column.
After clicking the “Generate Map” button, the tool computes the enrichment and shows the “Plot Maps” panel. After selecting the desired visualization options and clicking the “Plot Map” button, the tool shows the map of enriched terms as a grid (Fig. 3 ), where columns represent experimental conditions, eventually grouped based on the provided information, and rows represent the enriched terms grouped and colored based on the corresponding hierarchy class.
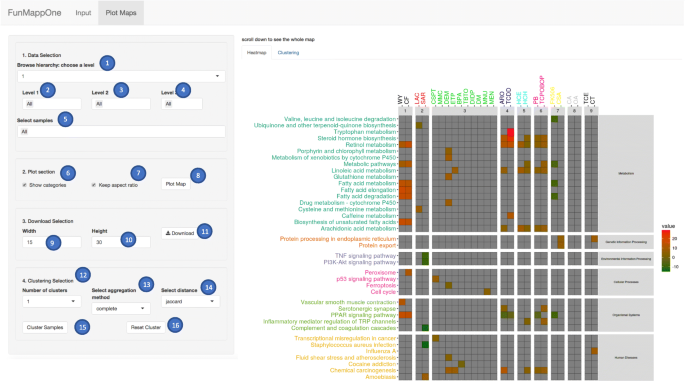
Interactive Map Visualization. The user can select the level of hierarchy to visualize (1) as well as a subset of elements to be plotted at each level of hierarchy (2-4). Furthermore, the user can select a subset of the conditions (5). In the “Plot section” the user can select to show the categories (6) and to keep the aspect ratio (7) for the plot. By clicking the button “Plot Map” (8) the updated map is visualized. After specifying the desired height (9) and width (10) for the pdf that will be downloaded, the user can save the image by clicking the “Download” button (11). Experiments can be clustered by selecting the number of clusters (12), the desired clustering function (13), the distance function (14), and then clicking the “Cluster samples” button (15). The map can be reset to the initial visualization with the predefined grouping by clicking the “Reset cluster” button (16)
The user can interact with the generated enrichment map in three different ways: i) by selecting the level at which the map is displayed, ii) by specifying one or more categories of terms to be displayed from a desired level of hierarchy, iii) by choosing a subset of experimental conditions to be plotted. The selection of the summarization level is performed via a drop-down menu. Once the desired level is selected and the “Plot Map” button is clicked, the panel with the results is automatically updated, providing a new map where the rows correspond to the categories of the chosen level, grouped by their super classes in the hierarchy. The color of the cells in the new map is associated with the summarized value of all the enriched terms in the experimental condition column belonging to the category row.
The concept of level categories can be used to select subsets of rows of interest. This is done by selecting, for each represented level, the categories/terms of interest. The tool subsequently updates the map reporting only category/terms from the selected set, thus allowing a more compact view of the portion of interest of the map. Similarly, the user can specify a subset of experiments to be plotted.
Finally, the columns of the map can be reordered by grouping experimental conditions having similar enrichment profiles. This is accomplished by selecting a desired number of groups, a distance function among Jaccard, Euclidean and “Jaccard+Euclidean”, and a clustering linkage method between complete, single, and ward. In the “Clustering” sub-tab of “Plot Maps”, FunMappOne provides a visualization of the cluster dendrogram as well as the partitioning based on the number of desired clusters. This functionality can help in selecting the most appropriate number of clusters to be displayed. Finally, the current view of the map can be exported in various graphical formats.
We finally provide a comparison among FunMappOne features and those offered by a selection of currently available tools for functional annotation having close scope to FunMappOne. Table 1 shows the comparison of FunMappOne with the following gene functional analysis tools: DAVID [ 1 ], Enrichr [ 2 ], ToppGene [ 3 ], g:profiler [ 4 ], clusterProfiler [ 5 ], Goplot [ 6 ] and BACA [ 7 ]. As shown in Table 1 , most of the other tools offer the possibility to analyze KEGG pathways, Reactome pathways and Gene Ontology, also with a graphic representation of the enrichment results. Only Goplot offers the possibility to map gene associated values to terms, while Enrichr and g:profiler are the only tools offering a web based graphical user interface. None of the other tools offer the possibility to summarize results and to cluster functional profiles from multiple experiments. To our knowledge, FunMappOne is the only tool providing all of these functionalities in a user friendly graphical interface.
We showcase the functionalities of FunMappOne on a transcriptome dataset of mouse hepatocytes exposed to 26 chemical compounds with different carcinogenic potential [ 11 ]. While Schaap et al. defined the similarity between the mechanism of action of a pair of chemicals at the level of individual genes, we tested the hypothesis that significant similarity patterns can be observed also at the functional annotation level. An excel file (Additional file 2 ) containing the originally described lists of the 30 most up-regulated and 30 most down-regulated genes in each compound-to-control comparison, along with the corresponding t-statistics, was uploaded to FunMappOne.
The annotation was performed by selecting the “KEGG” option and “gSCS” as multiple testing correction method with “0.05” as significance threshold (Additional file 3 ). For the plotting, the “median” function was chosen as summary statistics and colors were associated to the summarized modification direction of enriched terms by selecting the “sign” option (Additional file 3 ). Chemical exposures were finally ordered based on the “Jaccard” distance on the number of shared terms, and further clustered into 11 groups using hierarchical clustering and “complete” aggregation method.
Additional file 3 shows the KEGG enrichment map at the level 1 (Additional file 3 A), level 2 (Additional file 3 B), and at the individual pathway level 3 (Additional file 3 C).
Our analysis confirmed many similarities originally described by Schaap and collaborators, such as the one between Wyeth-14643 (WY) and Clofibrate (CF), which in our analysis were grouped together with Tacrolimus (FK506) in cluster 11 (Additional file 3 C). These chemicals modulate PPAR signalling pathway and fatty acid metabolism related genes, which we observed to be significantly enriched. Moreover, we identified a large cluster of compounds (cluster 6) characterized by no significantly enriched pathway, whose pairwise similarity of their mechanism of action were also described in the original report, but with a low significance [ 11 ].
Interestingly, enriched alteration of pathways related to steroid hormone biosynthesis and chemical carcinogenesis was observed in a group of known carcinogenic compounds clustered together (cluster 5). The visualizations produced at higher levels of the pathway hierarchy help the user to immediately observe that the chemicals in cluster 5 alter the genes in metabolic pathways and human diseases (Additional file 3 A). When the visualization at level 2 is inspected, the notion that lipid metabolism and cancer pathways are enriched also easily emerges. This functionality of FunMappOne becomes very effective when analyzing richer functional annotations, such as gene ontology, where the number of enriched terms can be significantly higher (as shown in Additional file 4 ).
We present FunMappOne, a web based standalone application that enables users to graphically inspect, navigate, and compare functional annotations in multiple experiments at different levels of abstraction. This tool facilitates the analyses of multiple experimental conditions through a simple user interface and dynamic graphical representations of the relevant functional categories. The FunMappOne software is open-source and distributed under the AGPL-3 license.
Availability and requirements
Project name: FunMappOne
Project home page: https://github.com/Greco-Lab/FunMappOne
Operating system(s): Cross-platform
Programming language: R
Other requirements: Shiny
License: AGPL-3
Any restrictions to use by non-academics: For commercial use and modifications please contact the corresponding author.
Abbreviations
Aroclor 1254
Biological processes
Bisphenol A
Calyculin A
Cellular components
Cyclosporin A
Carbon tetrachloride
Diethyl maleate
Diisodecyl phthalate
Term enrichment p -value
Heptachlor epoxide
β-Hexachlorocyclohexane
Kyoto Encyclopedia of Genes and Genomes
Lead acetate
Molecular functions
Mitomycin C
N-Methyl-N-nitrosourea
Gene modification value
Okadaic acid
Phenobarbital
Sodium arsenite
Summary statistics
Tributyltin oxide
1,1,1,-Trichloroethane
2,3,7,8-Tetrachlorodibenzo-p-dioxin
1,4-Bis[2-(3,5-dichloropyridyloxy)]benzene
Wyeth-14643
Huang DW, Sherman BT, Lempicki RA. Systematic and integrative analysis of large gene lists using DAVID bioinformatics resources. Nat Protoc. 2009; 4(1):44–57. https://doi.org/10.1038/nprot.2008.211. 9411012 .
Kuleshov MV, Jones MR, Rouillard AD, Fernandez NF, Duan Q, Wang Z, Koplev S, Jenkins SL, Jagodnik KM, Lachmann A, McDermott MG, Monteiro CD, Gundersen GW, Ma’ayan A. Enrichr: a comprehensive gene set enrichment analysis web server 2016 update. Nucleic Acids Res. 2016; 44(Web Server issue):90–7. https://doi.org/10.1093/nar/gkw377 .
Chen J, Bardes EE, Aronow BJ, Jegga AG. {ToppGene} {Suite} for gene list enrichment analysis and candidate gene prioritization. Nucleic Acids Res. 2009; 37(Web Server issue):305–11. https://doi.org/10.1093/nar/gkp427 .
Reimand J, Kull M, Peterson H, Hansen J, Vilo J. g:{Profiler}—a web-based toolset for functional profiling of gene lists from large-scale experiments. Nucleic Acids Res. 2007; 35(Web Server issue):193–200. https://doi.org/10.1093/nar/gkm226 .
Yu G, Wang L-G, Han Y, He Q-Y. {clusterProfiler}: an {R} {Package} for {Comparing} {Biological} {Themes} {Among} {Gene} {Clusters}. OMICS : J Integr Biol. 2012; 16(5):284–7. https://doi.org/10.1089/omi.2011.0118 .
Walter W, Sánchez-Cabo F, Ricote M. {GOplot}: an {R} package for visually combining expression data with functional analysis. Bioinforma (Oxford, England). 2015; 31(17):2912–4. https://doi.org/10.1093/bioinformatics/btv300 .
Fortino V, Alenius H, Greco D. {BACA}: bubble {chArt} to compare annotations. BMC Bioinformatics. 2015;16(1). https://doi.org/10.1186/s12859-015-0477-4 .
Kanehisa M, Sato Y, Kawashima M, Furumichi M, Tanabe M. KEGG as a reference resource for gene and protein annotation. Nucleic Acids Res. 2016; 44(D1):457–62. https://doi.org/10.1093/nar/gkv1070 .
Fabregat A, Jupe S, Matthews L, Sidiropoulos K, Gillespie M, Garapati P, Haw R, Jassal B, Korninger F, May B, Milacic M, Roca CD, Rothfels K, Sevilla C, Shamovsky V, Shorser S, Varusai T, Viteri G, Weiser J, Wu G, Stein L, Hermjakob H, D’Eustachio P. The Reactome Pathway Knowledgebase. Nucleic Acids Res. 2018; 46(D1):649–55. http://dx.doi.org/10.1093/nar/gkx1132 . NIHMS150003 .
Ashburner M, Ball CA, Blake JA, Botstein D, Butler H, Cherry JM, Davis AP, Dolinski K, Dwight SS, Eppig JT, Harris MA, Hill DP, Issel-Tarver L, Kasarskis A, Lewis S, Matese JC, Richardson JE, Ringwald M, Rubin GM, Sherlock G. Gene ontology: Tool for the unification of biology. 2000. https://doi.org/10.1038/75556 . http://www.ncbi.nlm.nih.gov/pubmed/10802651 . http://www.pubmedcentral.nih.gov/articlerender.fcgi?artid=PMC3037419 . http://www.nature.com/doifinder/10.1038/75556 .
Schaap MM, Wackers PFK, Zwart EP, Huijskens I, Jonker MJ, Hendriks G, Breit TM, van Steeg H, van de Water B, Luijten M. A novel toxicogenomics-based approach to categorize (non-)genotoxic carcinogens. Arch Toxicol. 2015; 89(12):2413–27. https://doi.org/10.1007/s00204-014-1368-6 .
Download references
Acknowledgements
Not applicable.
This study was supported by the Academy of Finland (grant agreements 275151 and 292307).
Availability of data and materials
The FunMappOne tool, its source code and the example test data used in this manuscript are available at https://github.com/Greco-Lab/FunMappOne .
Author information
Authors and affiliations.
Faculty of Medicine and Life Sciences, University of Tampere, Arvo Ylpön katu 34 - Arvo building, Tampere, FI-33014, Finland
Giovanni Scala, Angela Serra, Veer Singh Marwah, Laura Aliisa Saarimäki & Dario Greco
BioMediTech Institute, University of Tampere, Arvo Ylpön katu 34 - Arvo building, Tampere, FI-33014, Finland
Institute of Biotechnology, University of Helsinki, Viikinkaari 5d, Helsinki, FI-00014, Finland
Giovanni Scala & Dario Greco
You can also search for this author in PubMed Google Scholar
Contributions
GS and DG conceived the application and coordinated the project. GS, AS and VSM developed the FunMappOne tool. GS, LAS, and DG analyzed and interpreted the results of the case study. GS, AS, VSM, DG and LAS drafted the manuscript. All authors read and approved the final manuscript.
Ethics declarations
Ethics approval and consent to participate, consent for publication, competing interests.
The authors declare that they have no competing interests.
Publisher’s Note
Springer Nature remains neutral with regard to jurisdictional claims in published maps and institutional affiliations.
Additional files
Additional file 1.
FunMappOne user manual. User manual for the FunMappOne tool. (DOCX 1940 kb)
Additional file 2
Excel file containing input data for the case study. The excel file is composed of one sheet for each exposure and a last sheet containing grouping information. Each exposure sheet is named with the exposure ID and contains two columns containing the list of selected genes and the associated t-statistics, respectively. The last sheet contains two columns: one reporting the list of exposure IDs and another the corresponding group. (XLSX 63 kb)
Additional file 3
Case study KEGG enrichment maps. KEGG enrichment maps showing modification direction after clustering analysis with 11 clusters. Panel A (top) shows enrichment results summarized at KEGG Level 1, panel B (middle) shows enrichment results summarized at KEGG Level 2, panel C (bottom) shows enrichment results summarized at KEGG Level 3 (pathways level). (PPTX 6869 kb)
Additional file 4
Level 1,2,3 Reactome and Gene Ontology (BP, CC, MF) maps for the proposed case study. Reactome maps have been produced by providing “Additional file 1” as input and choosing “Reactome” enrichment, annotation was performed using “Bonferroni” as multiple testing correction method with “0.001” as significance threshold. Three classes of Gene Ontology maps have been produced by providing “Additional file 1” as input and choosing “GO” and alternatively “BP”, “CC” or “MF” enrichment, annotation was performed using “Bonferroni” as multiple testing correction method with “0.001” as significance threshold. In both cases, for the plotting “median” was chosen as summary statistics and map colors were associated to the summarized each term modification direction by choosing the sign option. (PDF 3044 kb)
Rights and permissions
Open Access This article is distributed under the terms of the Creative Commons Attribution 4.0 International License ( http://creativecommons.org/licenses/by/4.0/ ), which permits unrestricted use, distribution, and reproduction in any medium, provided you give appropriate credit to the original author(s) and the source, provide a link to the Creative Commons license, and indicate if changes were made. The Creative Commons Public Domain Dedication waiver( http://creativecommons.org/publicdomain/zero/1.0/ ) applies to the data made available in this article, unless otherwise stated.
Reprints and permissions
About this article
Cite this article.
Scala, G., Serra, A., Marwah, V.S. et al. FunMappOne: a tool to hierarchically organize and visually navigate functional gene annotations in multiple experiments. BMC Bioinformatics 20 , 79 (2019). https://doi.org/10.1186/s12859-019-2639-2
Download citation
Received : 16 July 2018
Accepted : 18 January 2019
Published : 15 February 2019
DOI : https://doi.org/10.1186/s12859-019-2639-2
Share this article
Anyone you share the following link with will be able to read this content:
Sorry, a shareable link is not currently available for this article.
Provided by the Springer Nature SharedIt content-sharing initiative
- Functional annotation
- Pathway visualization
- Ontology visualization
- Gene Ontology
BMC Bioinformatics
ISSN: 1471-2105
- General enquiries: [email protected]
Frequently Asked Questions
1. What is DAVID?
DAVID was originally designed as a web-based functional annotation tool, particularly for gene-enrichment analysis on the DAVID knowledgebase which contained annotations and gene accessions linked by LocusLink IDs in the 2003 version (v1.x). As the result of continuous improvement, DAVID provides a large integrated annotation knowledgebase based on the "DAVID Gene Concept" since v2.x, a method to agglomerate heterogeneous and widely distributed public databases. Besides functional annotation, it also provides an enhanced set of bioinformatics tools to summarize the relevant biological patterns for users to quickly understand the biological themes under study. To address the challenges of systems biology, DAVID will keep being upgraded and more tools will be developed.
2. What tools does DAVID provide to analyze my gene list?
DAVID provides an integrated knowledgebase collected from the most common bioinformatic resources ( see update of knowledgebase for details ). To leverage the knowledgebase, four sets of comprehensive tools have been developed including: Functional Annotation Tools ; Gene Functional Classification Tool ; Gene ID Conversion Tool ; Gene Name Batch Viewer . With the Functional Annotation Tools, users can perform functional annotation mapping (Functional Annotation Table), functional annotation enrichment analysis (Functional Annotation Chart), and functional annotation clustering based on the relationship of genes to annotation within the user's list (Functional Annotation Clustering). The Gene Functional Classification Tool generates a gene-to-gene similarity matrix of the genes in the user's list based on shared functional annotation from multiple functional annotation categories. This novel clustering algorithm classifies highly related genes into functionally related groups. With the Gene ID Conversion Tool, users can convert a list of gene IDs/accessions to other identifier types with the comprehensive gene ID mapping repository from the DAVID Knowledgebase. The ambiguous or contaminating accessions in a user's list can also be detected and determined by users with this tool. The Gene Name Batch viewer is able to quickly list all gene names for a given gene list to quickly view the genes which are present in a user's experiment.
3. What accession numbers and gene identifiers does DAVID accept?
DAVID accepts a wide range of gene/protein identifiers. Users can view all the identifier options from the drop-down selection menu in Upload tab of the List Manager on the left side of the user interface .
4. What file formats can be uploaded/downloaded by DAVID?
Plain text (*.txt), tab-delimited files can be uploaded by DAVID. For the single gene list, the first column of your file must contain the gene identifier and the second column may contain an optional value (e.g., fold change, p-value, correlation, cluster number, experimental group, etc.). Remove column headings and save the file as a Tab delimited text file . To convert an excel file to this format choose File>Save As> then under save as type choose Text (Tab delimited) (*.txt) . To save your annotated gene list from your browser to your hard drive as an excel file simply choose File>Save As> then type yourfilename.xlsx and save to your hard drive. You can then open this file in Microsoft excel and perform typical excel-type analysis. User can also upload multiple gene lists at once from one file. The file format is tab-delimited text file, with each column representing one list. The first row (header) should contain the names of the individual lists and all lists should be of the same id type (i.e. ENTREZ_GENE_ID).
5. Who can use DAVID?
DAVID is free to use for all users. Please see the license section for more details.
6. Where does DAVID's knowledgebase come from and how current is it?
The DAVID knowledgebase agglomerates species-specific gene/protein identifiers and their annotations from a variety of public genomic resources (e.g. NCBI, Uniprot, Ensembl, Gene Ontology, KEGG, Reactome, etc.). The DAVID Knowledgebase contains tens of millions of identifiers from tens of thousands of species allowing agglomeration of a diverse array of functional and sequence annotation, greatly enriching the level of biological information available for a given gene (e.g. gene/protein ids, protein functional domains, gene ontology, pathways, disease associations, general descriptions, protein-protein interactions, literature, small molecule interactions, etc.). However, DAVID does not check the quality or accuracy of all original annotation data. If you happen to find annotation errors, please give us your feedback in the DAVID forum or contact us . For more details on content coverage and collection dates, including the last update, please refer to the update section .
7. Who do I contact if I find an annotation error?
DAVID tries to aggregate biological knowledge into an organized structure that allows the efficient dissemination of functional annotations across genome-scale datasets. DAVID does not guarantee the quality or accuracy of annotation data. If you happen to find annotation errors, please give us your feedback in the DAVID forum or contact us .
8. How are genes counted in DAVID Chart Report?
DAVID counts the number of unique DAVID gene Ids corresponding to the input gene list. If two or more identifiers represent alternatively spliced forms of the same gene they will be counted as one and reflected as such in the histograms.
9. Why are there different levels for GO Annotation?
The structured vocabulary created by the Gene Ontology Consortium is a pseudo-hierarchy or directed acyclic graph (DAG). The different levels provided by DAVID allows users to annotate lists of genes at different levels within the DAG. Level 1 represents the most general categories and provides the most coverage, whereas Level 5 provides more specific information and less coverage. Users may also annotate their gene lists with all annotations available at all levels and for some genes there will be more than 5 levels. Additionally, users can choose to use only a subset of the more specific terms represented by the GO_Fat categories. The GO level specific, All and Fat categories provide GO annotations based on the data provided by the original source (NCBI, Uniprot and Ensembl) and DAVID additionally maps the gene to the lineage of the directly annotated term (i.e. parents, grandparents, etc.). GO terms annotated directly by the annotation source are represented by the GO_Direct categories which are the GO categories selected by default in DAVID. Of note, the fact that proteins are frequently involved in numerous biological processes is reflected in the Gene Ontology structure. Thus, genes may be annotated with several categories and be counted in each annotation category by the charting tools.
10. What does it mean to have empty chart report?
An empty chart report means that there are no annotations passing the specified thresholds. It does not mean that annotation does not exist for any of the genes in the list. Options can be adjusted at the top of the Chart report result page. By dropping the Count and EASE thresholds to 1, any annotation in the selected categories associated with any gene in the list and in the background will be in the results.
11. How do I cite DAVID?
DAVID users may publish or otherwise publicly disclose the results obtained from DAVID. Please acknowledge DAVID in your publications by citing the following two references:
- Huang DW, Sherman BT, Lempicki RA. Systematic and integrative analysis of large gene lists using DAVID Bioinformatics Resources. Nature Protoc. 2009;4(1):44-57. [ PubMed ]
- Huang DW, Sherman BT, Lempicki RA. Bioinformatics enrichment tools: paths toward the comprehensive functional analysis of large gene lists. Nucleic Acids Res. 2009;37(1):1-13. [ PubMed
12. What is the purpose of the minimum number of hits and maximum p-value thresholds?
One way of looking at it is that the thresholds allow you to filter the result, i.e. Show me annotations with 3 or more genes that are enriched in my list (significant p-value). If you show all categories including those with only one hit, a lot of non-specific results will be displayed. The current defaults for minimum number of hits (Count) is 2 and for p-value (EASE) is 0.1 which may be adjusted in the options found at the top of the Chart report result page.
13. What journal articles have cited DAVID or EASE?
Please refer to the DAVID Google Scholar page .
14. Not all of my genes are annotated! Why?
The reason for this is that the functional annotation of genomes is incomplete and the types of annotation that any given gene may have can differ. For example, when using DAVID, you may find a gene that has GO classifications and no functional summary text, while another gene has functional summary text and no GO classifications, and still others will have no annotation whatsoever. This is why the database behind DAVID is continually updated, giving researchers access to the current state of functional annotation, which indeed is always changing. Another reason is that some user input identifiers cannot be mapped to any known genes in the DAVID Knowledgebase. One other consideration is to make sure that the genes in your list are found in the background set that you have selected in DAVID otherwise, DAVID will ignore them.
15. How can I use DAVID functional analysis modules programmatically?
DAVID provides a set of APIs and web services for outside applications to directly interact with DAVID.
16. What are the choices of population background in DAVID enrichment analysis?
The enrichment analysis compares the annotation composition based on your gene list to that of a background population of genes. In this sense, the selection of a background population will affect the results significantly. The DAVID default background is the set of corresponding genome-wide genes for the species with the highest representation in the user's list. The default background is a good choice for studies in a genome-wide scope or close to genome-wide scope. More background choices are available in DAVID, including Affymetrix and Illumina array backgrounds. The pre-built Affymetrix and Illumina backgrounds can be selected through the "Background" tab of the Gene List Manager on the left side of the interface. Affymetrix and Illumina backgrounds will be a better choice for a gene list derived from Affymetrix microarray or Illumina studies, respectively. Users may also input a customized background by uploading in the List Manager on the left side of the interface, similar to submitting a gene list. Customized backgrounds will be a better choice for studies far below a genome-wide scope, such as targeted gene experiments.
17. Does DAVID limit the maximum number of genes in a list?
The goal of DAVID's design is to be able to efficiently upload and analyze a list consisting of <=3000 genes. All DAVID tools have been tested with lists in this range and should return results in a few seconds to no more than a few minutes. If running time is longer than a few minutes, please contact the DAVID Bioinformatic Team for help. Please note that Functional Annotation Clustering and Gene Functional Classification have a 3000 gene limit.
18. What is the format requirement for my input gene list?
You can either load a gene list from a file or paste a gene list to the text box. DAVID was designed to accept the data starting from the first row without header (i.e. accession). The gene list must be in a format of one gene in one row and only the first column is considered in the analysis. DAVID is case insensitive for the accessions/IDs. Since the DAVID list manager is centralized, the format requirement to submit a gene list are the same for ALL DAVID tools. In addition, the submitted gene lists could be used as customized background genes in the enrichment analysis based on your choice at step 3. The indication of a successful submission is that you should see the corresponding gene lists listed by list tab or background tab. Moreover, an expected gene # should also be associated with the gene lists. Example: 1000_at 1001_at 1002_at
19. Which DAVID tools to choose?
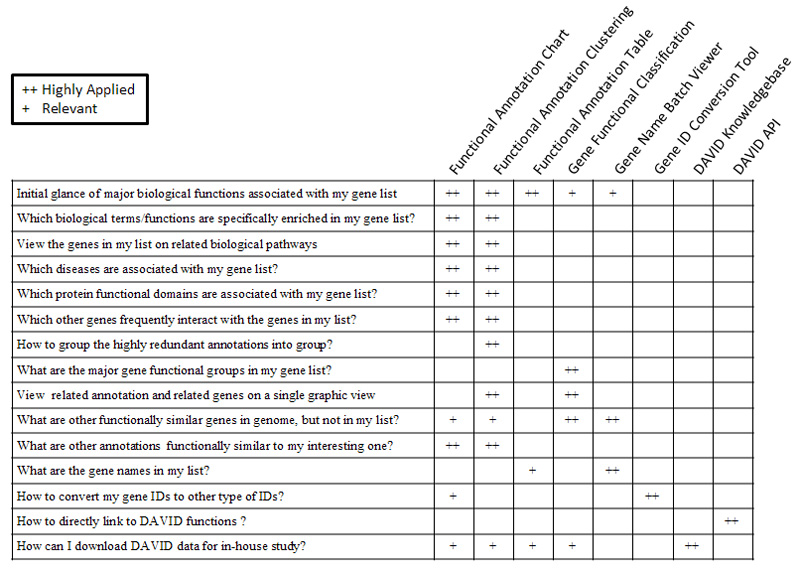
20. Why does DAVID give empty results after I walk away for awhile?
The session timeout of DAVID is set to 30 minutes. In other words, if your web browser has no activity with DAVID for 30 minutes, your session will end, and you will need to re-submit your gene list to DAVID and restart your analysis.
Last edited December 10, 2020
- Uppmax login
- Cheat sheets
Functional annotation
- What is needed for functional annotation?
- How to do functional annotation?
- understand the different tools/processes to do a functional annotation
- be able to run a functional annotation
Prerequisites
For this exercise you need to be logged in to Uppmax.
Setup the folder structure:
Introduction
Functional annotation is the process during which we try to put names to faces - what do genes that we have annotated and curated? Basically all existing approaches accomplish this by means of similarity. If a translation product has strong similarity to a protein that has previously been assigned a function, the function in this newly annotated transcript is probably the same. Of course, this thinking is a bit problematic (where do other functional annotations come from…?) and the method will break down the more distant a newly annotated genome is to existing reference data. A complementary strategy is to scan for more limited similarity - specifically to look for the motifs of functionally characterized protein domains. It doesn’t directly tell you what the protein is doing exactly, but it can provide some first indication.
In this exercise we will use an approach that combines the search for full-sequence similarity by means of ‘Blast’ against large public databases with more targeted characterization of functional elements through the InterproScan pipeline. Interproscan is a meta-search engine that can compare protein queries against numerous databases. The output from Blast and Interproscan can then be used to add some information to our annotation.
Prepare the input data
Since we do not wish to spend too much time on this, we will again limit our analysis to chromosome 4. It is also probably best to choose the analysis with ab-initio predictions enabled (unless you found the other build to be more convincing). Maker produces a protein fasta file (called “annotations.proteins.fa”) together with the annotation and this file should be located in your maker directory.
Move in the proper folder:
Now link the annotation you choose to work with. The command will looks like:
Interproscan approach
Interproscan combines a number of searches for conserved motifs and curated data sets of protein clusters etc. This step may take fairly long time. It is recommended to paralellize it for huge amount of data by doing analysis of chunks of tens or hundreds proteins.
Perform InterproScan analysis
InterproScan can be run through a website or from the command line on a linux server. Here we are interested in the command line approach. Interproscan allows to look up pathways, families, domains, sites, repeats, structural domains and other sequence features.
Launch Interproscan with the option -h if you want have a look about all the parameters.
- The ‘-app’ option allows defining the database used. Here we will use the PfamA,ProDom and SuperFamily databases. - Interproscan uses an internal database that related entries in public databases to established GO terms. By running the ‘-goterms’ option, we can add this information to our data set. - If you enable the InterPro lookup (‘-iprlookup’), you can also get the InterPro identifier corresponding to each motif retrieved: for example, the same motif is known as PF01623 in Pfam and as IPR002568 in InterPro. - The option ‘-pa’ provides mappings from matches to pathway information (MetaCyc,UniPathway,KEGG,Reactome).
This analyse will fail.

If you did not have a look at the maker_final.faa, please have look and find a solution to make interproscan run.

Rerun the previous interproscan command.
The analysis should take 2-3 secs per protein request - depending on how many sequences you have submitted, you can make a fairly deducted guess regarding the running time. You will obtain 3 result files with the following extension ‘.gff3’, ‘.tsv’ and ‘.xml’. Explanation of these output are available »here« .
load the retrieved functional information in your annotation file:
Next, you could write scripts of your own to merge interproscan output into your annotation. Incidentally, Maker comes with utility scripts that can take InterProscan output and add it to a Maker annotation file (you need to load maker).
- ipr_update_gff: adds searchable tags to the gene and mRNA features in the GFF3 files. - iprscan2gff3: adds physical viewable features for domains that can be displayed in JBrowse, Gbrowse, and Web Apollo.
We also created a script that can do the merging between the structural annotation and the interpro results :
Where a match is found, the new file will now include features called Dbxref and/or Ontology_term in the gene and transcript feature field (9th column). The improved annotation is the gff file inside the maker_final.interpro folder.
BLAST approach
Blast searches provide an indication about potential homology to known proteins. A ‘full’ Blast analysis can run for several days and consume several GB of Ram. Consequently, for a huge amount of data it is recommended to parallelize this step doing analysis of chunks of tens or hundreds proteins. This approach can be used to give a name to the genes and a function to the transcripts.
Perform Blast searches from the command line on Uppmax:
To run Blast on your data, use the Ncbi Blast+ package against a Drosophila-specific database (included in the folder we have provided for you, under $data/blastdb/uniprot_dmel/uniprot_dmel.fa ) - of course, any other NCBI database would also work:
Against the Drosophila-specific database, the blast search takes about 2 secs per protein request - depending on how many sequences you have submitted, you can make a fairly deducted guess regarding the running time.
load the retrieved information in your annotation file:
Now you should be able to use the following script:
That will add the name attribute to the “gene” feature and the description attribute (corresponding to the product information) to the “mRNA” feature into you annotation file. The improved annotation is the gff file inside the maker_final.interpro.blast folder.

Set nice IDs
The purpose is to modify the ID value by something more convenient (i.e FLYG00000001 instead of maker-4-exonerate_protein2genome-gene-8.41).
The improved annotation is the gff file inside the maker_final.interpro.blast.ID folder.
Polish your file for a nice display within Webapollo
For a nice display of a gff file within Webapollo some modification might be needed. As example the attribute product is not displayed in Webapollo, whereas renaming it description will work out.
Visualise the final annotation
Transfer the final_annotation.gff file to your computer using scp in a new terminal:
Load the file in into the genome portal called drosophila_melanogaster_chr4 in the Webapollo genome browser available at the address http://annotation-prod.scilifelab.se:8080/NBIS_course/ . Here find the WebApollo instruction
Wonderfull ! isn’t it ?
What’s next?
Because of Makers’ compatibility with GMOD standards, a functional annotation created in one or both of this way can be loaded into e.g. WebApollo and will save annotators a lot of work when e.g. adding meta data to transcript models.
- Disulfide Bond
- Glycosylation Analysis of Protein
- Phosphoproteomics Service
- Ubiquitinated-proteomics
- S-Nitrosylation
- Methyl-proteomics
- Acetyl-proteomics
- Characterization of Protein SUMOylation
- Co-immunoprecipitation/mass spectrometry (co-IP/MS)
- Crosslinking Protein Interaction Analysis
- Far-Western Blot Analysis Service
- Pull-Down Assay
- Label Transfer Protein Interaction Analysis
- BioID-MS Service
- Chemical Cross-linking Mass Spectrometry (CX-MS) Service
- Tandem Affinity Purification (TAP)-MS Service
- TurboID Service
- IP-MS Protein Interactomics Solution
- Isoelectric Point Analysis Service
- Protein Sample Preparation
- Digestion (in-gel or in-solution)
- Molecular Mass Determination Service
- Protein Purity and Homogeneity Characterization
- Sequence Analysis of Peptides or Proteins
- Membrane Protein Identification
- Accurate Mass Determination
- Shotgun Protein Identification
- iTRAQ-based Proteomics Aanalysis
- TMT based proteomics service
- SILAC-based Proteomics Analysis
- Absolute Quantification (AQUA)
- Label-free Quantification
- Semi-quantitative Proteomics Analysis
- Parallel Reaction Monitoring (PRM)
- SRM & MRM
- DIA Quantitative Proteomics Service
- Top Down-based Sequencing
- Top Down-based Characterization of PTMs
- Characterization of Protein Structure
- Characterization of Peptide Biomarkers
- Comprehensive Peptidomics Service
- Subcellular Proteomics
- Exosome Proteomics
- Cell Surface Proteomics
- Plasma/Serum Proteomics Service
- Metaproteomics Service
- Customized Experiments
- GC-MS/MS Un-targeted Metabolomics
- LC-MS/MS Untargeted Metabolomics
- Metabolomics Research Solution of Gut Microbiota
- Serum Untargeted Metabolomics
- Urine Untargeted Metabolomics
- Cerebrospinal Fluid Untargeted Metabolomics
- Exosome Untargeted Metabolomics
- Targeted Metabolomics
- Metabolic Flux Analysis (MFA)
- Unknown Metabolites Identification
- Xenobiotic Metabolites Analysis
- Plant Metabolomics Service
- Plant Untargeted Lipidomics
- Yeast Untargeted Lipidomics
- Mammals Untargeted Lipidomics
- Short Chain Fatty Acids
- Total Fatty Acids
- Eicosanoids
- Fatty Acid Metabolism
- Sphingolipid Metabolism
- Sterol Lipids Analysis Service
- Glycerolipids
- Glycerophospholipids
- Phospholipids
- Fatty Acids Metabolomics Service
- Phytoceramides Quantitative Analysis
- Targeted Phosphoinositides Analysis Service
- Cholestero LC-MS Analysis Service
- MALDI-Imaging Lipidomics
- Exosome Lipidomics
- N-Glycan Profiling
- O-Glycan Profiling
- N-Glycosylation Site Occupation
- O-Glycosylation Site Occupation
- N-Glycan Linkage Analysis
- O-Glycan Linkage Analysis
- Lignin Content Analysis
- Cellulose Content Analysis
- Glycan Quantification
- Glycan Sequencing
- Structural Characterization of Glycans
- Glycopeptides Analysis
- Glyco-gene Microarray Assay
- Lectin Microarray Assay
- Glycan Microarray Assay
- Microbial Glycan Microarray Assay
- Glycopeptide Microarray Assay
- Polysaccharide Isolation and Purification Service
- Determination of the Absolute Configuration
- Identification of the Anomeric Configuration
- Molecular Weight Determination of Polysaccharide
- Starch / Amylose Content Analysis
- Amylopectin Chain Length Distribution Profiling
- Granule Size Distribution Analysis
- Gelatinization Temperature Determination
- Thermal Stability Analysis
- Peptidoglycan Structure Analysis
- Integrated Transcriptomics and Metabolomics Analysis
- Integrated Transcriptomic and Proteomic Analysis
- Integrated Transcriptomic and Lipidomics Analysis
- Integrated Proteomics and Metabolomics Analysis
- Integrated Proteomics and Lipidomics Analysis
- Integrated Transcriptomic, Proteomic, and Metabolomic Analysis
- Integrated Metagenomic and Metabolomic Analysis
- Integrated Metabolomics and Microbiomics Analysis
- Integrated Genomics and Transcriptomics Analysis
- Integrated Analysis of DNA Methylation and Transcriptome
- Metabolite Genome-Wide Association Study (mGWAS) Service
- Peptide Purity Analysis
- Structure Activity Relationship (SAR) Analysis
Functional Annotation and Enrichment Analysis Service
- Clustering Analysis Service
- Statistical Analysis Service
- Network Analysis Service
- Proteomic Analysis of Post-translational Modifications Service
- Bioinformatic Univariate Analysis Service
- Multivariate Analysis Service
- Clustering Analysis Service for Metabolomics
- Bioinformatic Data Preprocess and Normalization Service
- Protein Sequence Analysis Service
- Protein Structure Analysis Service
- Protein Evolution Analysis Service
- Flow Cytometry Service
- De Novo Peptides/Proteins Sequencing
- Peptide Mapping
- De Novo Antibody Sequencing
- Amino Acid Analysis (AAA)
- Host Cell Protein Analysis
- Bioanalysis of DNA Methylations
- Residual DNA Testing
- Customized Synthesized Peptide/Proteins
- Biacore Service
- Environment
- Chromatography Technology
- Spectroscopy Technology
- Mass Spectrometry Platform
- Knowledge Bases
- Support Documents
- Distributor
- Order Online
- Bioinformatics Service
- Bioinformatics for Proteomics
- Service Details
Functional annotation and enrichment analysis has been widely used in bioinformatics of omics research. Creative Proteomics can provide our customers multiple functional annotation and enrichment analysis services, such as GO annotation analysis and GO enrichment analysis , KEGG annotation and KEGG enrichment analysis , COG / KOG annotation , domain annotation and enrichment analysis, and subcellular localization . As one of the leading omics industry company in the world, we are open to help you with Functional Annotation and Enrichment Analysis Service.
What Is Functional Analysis
Common methods for gene (protein) functional analysis include metabolic signaling pathway analysis and Gene Ontology (GO) analysis. Additionally, there are other analyses such as Clusters of Orthologous Groups of proteins (COG) and protein domain analysis.
GO and pathway analyses both study gene function, but they have differences. GO primarily focuses on studying gene function, while pathway analysis involves the study of gene and protein functions. GO categorizes gene functions into three major classes: Biological Process (BP), Molecular Function (MF), and Cellular Component (CC). Among these, GO BP analysis is commonly used. Common pathway data sources include KEGG, Reactome, Biocarta, etc.
Functional analysis can be divided into two categories: functional annotation analysis and functional enrichment analysis.
What Is Functional Annotation
Functional annotation is the process of attaching biological information to sequences of genes or proteins. The basic level of annotation is using sequence alignment tool BLAST for finding similarities, and then annotating genes or proteins based on that. Nevertheless, nowadays more and more additional information of biological functions is added to the annotation system. The additional information allows hand-operated annotation to distinguish genes or proteins that have the same annotation. With many genomes sequenced, computational annotation approaches to characterize genes and proteins from their sequence are increasingly important.
Functional annotation consists of three main steps:
Identifying portions of the genome that do not code for proteins
Identifying elements on the genome, a process called gene prediction
Attaching biological information to these elements.
Functional annotation analysis involves annotating genes with GO terms and pathway information. For example, the DDR1 gene may be associated with 20 biological processes (GO BP), such as GO:0001558 regulation of cell growth, GO:0007155 cell adhesion, and GO:0031100 organ regeneration.
What Is Functional Enrichment Analysis
Functional enrichment analysis is a method to determine classes of genes or proteins that are over-represented in a large group of genes or proteins, and may have relations with disease phenotypes. This approach uses statistical methods to determine significantly enriched groups of genes. In GSEA, DNA microarrays, or RNA-Seq, are still carried out and compared between two distinct categories, but focusing on a gene set instead of a single gene in a long list. Researchers analyze whether the most of genes in the set is located in the extremes of the list: The top and bottom of the list represent the largest differences in expression between the two types. If the gene set falls at either the top (over-expressed) or bottom (under-expressed), it is considered to be related to the phenotypic differences.
The general steps of enrichment analysis provided by Creative Proteomics are summarized below:
Calculate a p-value that represents the amount to which the proteins in the set are over-represented at either the top or bottom of the list.
Evaluate the statistical significance of a node or pathway based on the p-value.
P-value for each set is normalized and a false discovery rate is calculated for multiple hypothesis testing.
Functional enrichment analysis refers to analyzing a gene set to identify significantly enriched functions using the hypergeometric distribution algorithm. By using enrichment analysis, we can summarize a comprehensive overview of events based on many seemingly scattered differentially expressed genes. For example, we can conclude that the TP53 signaling pathway is related to the occurrence of gastric cancer, rather than stating that the occurrence of gastric cancer is associated with the seven genes BAX, BID, ABL1, ATM, BCL2, BOK, and CDKN1A.
Application
Up to date, functional annotation and enrichment analysis has obtained Important achievements in variety of scientific research fields, such as:
Cancer cell profiling
Complex disorders (such as schizophrenia)
Spontaneous preterm birth
Genome-wide association studies
Creative Proteomics can provide the following services
GO annotation analysis
GO enrichment analysis
Directed acyclic graph (DAG)
KEGG pathway annotation
KEGG pathway enrichment
COG annotation
KOG annotation
Domain annotation
Domain enrichment
Subcellular localization
How to place an order:

*If your organization requires signing of a confidentiality agreement, please contact us by email
Now, bioinformaticians at Creative Proteomics is opening to provide our customers functional annotation and enrichment analysis service. With years of experience in the computational sciences and knowledge of these powerful technologies, you will find what you need from the best. Contact Us for all the detailed information!
Liver Extrahepatic Bile Duct and Gallbladder Genes in a Biliary Atresia Mouse Model
Research Objective
Biliary atresia (BA) is a condition where there is blockage in the intrahepatic and extrahepatic bile ducts, leading to obstructive cholangiopathy and ultimately resulting in liver failure. Rotavirus infection can induce BA-like disease in mice. Therefore, we constructed a time expression profile of biliary atresia in neonatal mice infected with rotavirus. We analyzed the differentially expressed genes and their functional roles in the disease samples to elucidate the molecular mechanisms of the biliary atresia model.
Methods and Results
I Experimental Design:
Control samples treated with normal saline, with time points at 3 days (Day3_NS), 7 days (Day7_NS), and ** days (Day**NS), with 3 samples at each time point.
Experimental samples treated with rotavirus, with time points at 3 days (Day3_RRV), 7 days (Day7_RRV), and ** days (Day**_RRV), with 3 samples at each time point.
Microarray Preparation: Microarrays were created for gene expression analysis.
II Expression Data Preprocessing and Differential Expression Analysis of Microarray Data:
Gene expression values were used for Venn diagram analysis across multiple datasets to rapidly identify important genes and observe similarities and differences among differentially expressed genes at the three time points.
The results of the Venn diagram analysis are shown in Figure 1. There were 115 upregulated genes simultaneously appearing at all three time points, while there was only one downregulated gene. Subsequent analysis will focus on these 116 genes.
III Functional Enrichment Analysis of Differentially Expressed Genes:
Enrichment analysis tools were used to separately analyze the upregulated and downregulated genes at each time point for their involvement in GO functions and KEGG pathways. The VennPlex Venn diagram provided the expression direction consistency of the 116 differentially expressed genes across the three time points.
IV Intersection Gene Analysis:
Combining the results from the VennPlex Venn diagram analysis, we identified genes that showed consistent expression direction across the three time points. Transcription factors regulating these genes were predicted. We selected transcription factor-target gene relationships from the transfac and jaspar databases for this predictive analysis.
Online Inquiry
Please submit a detailed description of your project. We will provide you with a customized project plan to meet your research requests. You can also send emails directly to for inquiries.
- Search Menu
- Sign in through your institution
- Advance articles
- Collections
- Author Guidelines
- Submission Site
- Open Access Options
- About ISME Communications
- About the International Society for Microbial Ecology
- Editorial Board
- Self-Archiving Policy
- Journals on Oxford Academic
- Books on Oxford Academic

Article Contents
Conflicts of interest, data availability.
- < Previous
Driving through stop signs: predicting stop codon reassignment improves functional annotation of bacteriophages
- Article contents
- Figures & tables
- Supplementary Data
Ryan Cook, Andrea Telatin, George Bouras, Antonio Pedro Camargo, Martin Larralde, Robert A Edwards, Evelien M Adriaenssens, Driving through stop signs: predicting stop codon reassignment improves functional annotation of bacteriophages, ISME Communications , Volume 4, Issue 1, January 2024, ycae079, https://doi.org/10.1093/ismeco/ycae079
- Permissions Icon Permissions
The majority of bacteriophage diversity remains uncharacterized, and new intriguing mechanisms of their biology are being continually described. Members of some phage lineages, such as the Crassvirales , repurpose stop codons to encode an amino acid by using alternate genetic codes. Here, we investigated the prevalence of stop codon reassignment in phage genomes and its subsequent impacts on functional annotation. We predicted 76 genomes within INPHARED and 712 vOTUs from the Unified Human Gut Virome Catalogue (UHGV) that repurpose a stop codon to encode an amino acid. We re-annotated these sequences with modified versions of Pharokka and Prokka, called Pharokka-gv and Prokka-gv, to automatically predict stop codon reassignment prior to annotation. Both tools significantly improved the quality of annotations, with Pharokka-gv performing best. For sequences predicted to repurpose TAG to glutamine (translation table 15), Pharokka-gv increased the median gene length (median of per genome median) from 287 to 481 bp for UHGV sequences (67.8% increase) and from 318 to 550 bp for INPHARED sequences (72.9% increase). The re-annotation increased median coding capacity from 66.8% to 90.0% and from 69.0% to 89.8% for UHGV and INPHARED sequences predicted to use translation table 15. Furthermore, the proportion of genes that could be assigned functional annotation increased, including an increase in the number of major capsid proteins that could be identified. We propose that automatic prediction of stop codon reassignment before annotation is beneficial to downstream viral genomic and metagenomic analyses.
Bacteriophages, hereafter phages, are increasingly recognized as a vital component of microbial communities in all environments where they have been studied in detail [ 1–3 ]. Phages are known to drive bacterial evolution and community composition through predator–prey dynamics and their potential as agents of horizontal gene transfer [ 4 , 5 ]. The use of viral metagenomics, or viromics, has massively expanded our understanding of global viral diversity and shed light on the ecological roles that phages play [ 1–3 ].
Much of the study into viral communities has been conducted on the human gut. Here, viromics has uncovered ecologically important viruses that are difficult to bring into culture using standard laboratory techniques [ 6 ], shown the potential roles of viruses in disease states [ 3 ], and allowed for the recovery of enormous phage genomes larger than any brought into culture [ 7 ]. As the majority of phage diversity remains uncharacterized, new and enigmatic diversification mechanisms are being described continually, including the potential use of alternative translation tables.
Lineage-specific stop codon reassignment has been described previously in bacteriophages [ 8 , 9 ], whereby a stop codon is repurposed to encode an amino acid. Notably, annotations of Lak “megaphages” assembled from metagenomes were observed to exhibit unusually low coding density (~70%) when genes are predicted using the standard bacterial, archaeal, and plant plastid genetic code (translation table 11) [ 7 ], much lower than the value observed for most cultured phages of ~90% [ 10 ]. The Lak megaphages were predicted to repurpose the TAG stop codon into an as-of-yet unknown amino acid [ 7 ]. More recently, uncultured members of Crassvirales have been predicted to repurpose TAG to glutamine (translation table 15) and TGA to tryptophan (translation table 4) [ 9 ], and since then, the use of translation table 15 has been experimentally validated in two phages belonging to Crassvirales [ 11 ]. Although the reasons for stop codon reassignment in viruses are not yet understood, it has been suggested that stop codon reassignment is involved in the regulation of lytic genes that are involved in late-stage infection [ 12 ].
As stop codon reassignment may be widespread in human gut viruses, we trained a fork of Prodigal [ 13 ], named prodigal-gv, to predict stop codon reassignment in phages [ 14 ] and implemented it in the pyrodigal-gv library to provide efficient Cython bindings to Prodigal-gv with pyrodigal [ 15 ]. Additionally, the virus discovery tool geNomad incorporates pyrodigal-gv to predict stop codon reassignment for viral sequences identified in metagenomes and viromes [ 14 ]. Similarly, others have developed a tool for the detection of stop codon reassignment named MgCod [ 16 ]. However, the detection of translation table 15 still has limited support in many tools, and the impacts of stop codon reassignment on functional annotation are rarely considered in viral genomics and metagenomics.
To assess the extent of stop codon reassignment in studied phage genomes and the impacts on functional annotation, we extracted phage genomes from INPHARED [ 10 ] and predicted those using alternative stop codons. We also added high-quality and complete vOTUs from the Unified Human Gut Virome Catalogue (UHGV; https://github.com/snayfach/UHGV ) predicted to use alternative codons. The viral genomes were re-annotated using modified versions of the commonly used annotation pipelines Prokka [ 17 ] and Pharokka [ 18 ], implementing prodigal-gv and pyrodigal-gv for gene prediction (see Supplementary Methods). Hereafter, the modified versions are referred to as Prokka-gv and Pharokka-gv.
From INPHARED, 49 genomes (0.24%) were predicted to use translation table 15, and 27 (0.13%) were predicted to use translation table 4. From the UHGV, 666 vOTUs (1.2%) were predicted to use translation table 15, and 46 (0.08%) were predicted to use translation table 4. These genomes and vOTUs were not constrained to one particular clade of viruses, being predicted to occur on both dsDNA viruses of the realm Duplodnaviria and ssDNA viruses of the realm Monodnaviria . At the family level, we see clear lineages of viruses that conserve this feature, such as the Suoliviridae of Crassvirales ; however, it also appears sporadically in other families that are not widely known to re-purpose stop codons, such as the Demerecviridae ( Supplementary Table S1 ). The appearance of stop codon repurposing on distant lineages of viruses suggests this is a phenomenon that has arisen on multiple occasions. The lower frequency of these genomes in cultured isolates (INPHARED) versus human viromes (UHGV) may be due to culturing and sequencing biases, perhaps including modifications to DNA that are known to be recalcitrant to sequencing.
Although the mechanism for stop codon reassignment in phages is not fully understood, suppressor tRNAs are suggested to play a role [ 8 , 19 ]. Consistent with previous findings, we found 375/715 (52.4%) phages predicted to use translation table 15 encoded at least one suppressor tRNA corresponding to the amber stop codon (Sup-CTA tRNA), and 11/73 (15.1%) of those predicted to use translation table 4 encoded at least one suppressor tRNA corresponding to the opal stop codon (Sup-TCA tRNA) [ 8 , 19 , 20 ]. Although fewer of those predicted to use translation table 4 encoded the relevant suppressor tRNA, 22/27 (81%) of the INPHARED phages predicted to use translation table 4 were viruses of Mycoplasma or Spiroplasma . As Mycoplasma and Sprioplasma are known to use translation table 4, many of the viruses predicted to use translation table 4 may be simply using the same translation table as their host.
Prediction of stop codon reassignment led to improved annotations for both Prokka and Pharokka, although the extent of this varied with the two datasets, translation tables, and annotation pipelines tested ( Fig. 1 ; Supplementary Table S2 ; Supplementary Results). As Pharokka-gv outperformed Prokka-gv on all metrics tested, only Pharokka-gv is discussed further, and the equivalent results for Prokka-gv can be found in Supplementary Results. Despite using the same method for initially predicting ORFs, Prokka-gv filters more predicted ORFs than Pharokka-gv, which likely caused the difference in results.

Re-annotating with predicted stop codon reassignment increases the quality of annotations. Comparison of ( A ) median predicted gene length (bp), ( B ) coding capacity (%), and ( C ) proportion of unannotated “hypothetical” proteins for INPHARED genomes and UHGV vOTUs annotated with Pharokka (translation table 11 only) and Pharokka-gv (prediction of stop codon reassignment), grouped by dataset and predicted stop codon reassignment. Grey lines indicate pairing of the genomes across the two annotation strategies tested. Asterisk indicates significance at P ≤ 10e-10 with P determined by a paired sample T test and adjusted with the Benjamini–Hochberg procedure.
The largest improvements to annotations were observed for sequences predicted to use translation table 15, for which Pharokka-gv increased the median gene length (median of per genome medians) from 287 to 481 bp for UHGV sequences (67.8% increase) and from 318 to 550 bp for INPHARED sequences (72.9% increase; Fig. 1A ). This was also reflected in an increase of median coding capacity from 66.8% to 90.0% for UHGV and 69.0% to 89.8% for INPHARED ( Fig. 1B ). Overall, these improved gene calls led to an increased gene length and a reduction in the number of predicted genes per kb ( Supplementary Table S2 ). This was mirrored by an increase in the proportion of predicted proteins that could be assigned functions, with the median proportion of unannotated “hypothetical proteins” decreasing from 83.1% to 76.4% for UHGV and from 84% to 76.4% for INPHARED ( Fig. 1C ). As it is commonly used as a phylogenetic marker for bacteriophages, we investigated how commonly the major capsid protein (MCP) could be identified with and without predicted stop codon reassignment [ 21 ]. For those viruses we predicted to use translation table 15, annotation using the default translation table 11 only resulted in the MCP being identified in 407/715 (56.9%) of the genomes. In contrast, using translation table 15 with Pharokka-gv, we could identify the MCP in 475/715 (66.4%).
When investigating the sequences for which translation table 4 was predicted to be optimal, a substantial increase was also observed for UHGV sequences, with Pharokka-gv increasing median gene length (median of per genome medians) from 350 to 518 bp (a 48.0% increase in length; Fig. 1A ), resulting in an increase of median coding capacity from 78.0% to 90.4% ( Fig. 1B ), and a decrease in the median proportion of unannotated hypothetical proteins from 79.3% to 73.2% ( Fig. 1C ). However, the same was not observed for the 27 INPHARED genomes predicted to use translation table 4. Reannotation resulted in a modest increase in median gene length (median of per genome medians) from 573 to 588 bp (a 2.6% increase in length; Fig. 1A ). Median coding capacity was not increased, with both Pharokka and Pharokka-gv obtaining 89.1% ( Fig. 1B ). As the median gene length and coding capacity for INPHARED sequences predicted to use translation table 4 are in line with expected values, their prediction to use an alternate translation table may not be true. Similarly, many of these sequences belong to the viruses Mycoplasma and Sprioplasma , bacteria that are known to use translation table 4. Perhaps similarities of these viruses and their hosts have led to the prediction of translation table 4. Reassuringly, the prediction of translation table 4 has not hindered the quality of annotations for those genomes that have not observed a clear improvement in functional annotation.
The analysis of viral (meta)genomes relies on accurate protein predictions, with predicted ORFs being used in common analyses, including (pro)phage prediction, functional annotation, and phylogenetic analyses. The clear differences in protein predictions with/without predicted stop codon reassignment will likely have downstream impacts upon these analyses. However, this phenomenon is not yet widely considered in viral (meta)genomics. We have demonstrated the impacts of stop codon reassignment in the functional annotation of phages and provided tools for the automatic prediction and annotation of viral genomes that repurpose stop codons. Our analysis highlights the need for accurate viral ORF prediction and further experimental validation to elucidate the mechanisms of stop codon reassignment.
The authors declare no conflicts of interest.
This research was supported by the BBSRC Institute Strategic Programme Food Microbiome and Health BB/X011054/1 and its constituent projects BBS/E/F/000PR13631 and BBS/E/F/000PR13633; and by the BBSRC Institute Strategic Programme Microbes and Food Safety BB/X011011/1 and its constituent projects BBS/E/F/000PR13634, BBS/E/F/000PR13635, and BBS/E/F/000PR13636. R.C. and E.M.A. were supported by the BBSRC grant Bacteriophages in Gut Health BB/W015706/1. This research was supported in part by the NBI Research Computing through the High-Performance Computing cluster. We gratefully acknowledge CLIMB-BIG-DATA infrastructure (MR/T030062/1) support for the provision of cloud resources. R.A.E. was supported by an award from the NIH NIDDK RC2DK116713 and an award from the Australian Research Council DP220102915. The work conducted by the US Department of Energy Joint Genome Institute ( https://ror.org/04xm1d337 ) and the National Energy Research Scientific Computing Center ( https://ror.org/05v3mvq14 ) is supported by the US Department of Energy Office of Science user facilities, operated under contract no. DE-AC02-05CH11231.
The genomes used in this analysis are from two publicly available datasets; INPHARED ( https://github.com/RyanCook94/inphared ) and the Unified Human Gut Virome (UHGV; https://github.com/snayfach/UHGV ). The details of included sequences are shown in Supplementary Table S1 . The code for Prokka-gv is available on GitHub ( https://github.com/telatin/metaprokka ). The code for Pharokka is available on GitHub ( https://github.com/gbouras13/pharokka ). The code for Prodigal-gv is available on GitHub ( https://github.com/apcamargo/prodigal-gv ). The code for Pyrodigal-gv is available on GitHub ( https://github.com/althonos/pyrodigal-gv ).
Gregory AC , Zayed AA , Conceição-Neto N et al. Marine DNA viral macro- and microdiversity from pole to pole . Cell 2019 ; 177 : 1109 – 1123.e14 . https://doi.org/10.1016/j.cell.2019.03.040
- Google Scholar
Roux S , Emerson JB . Diversity in the soil virosphere: to infinity and beyond? Trends Microbiol 2022 ; 30 : 1025 – 35 . https://doi.org/10.1016/j.tim.2022.05.003
Clooney AG , Sutton TDS , Shkoporov AN et al. Whole-Virome analysis sheds light on viral dark matter in inflammatory bowel disease . Cell Host Microbe 2019 ; 26 : 764 – 778.e5 . https://doi.org/10.1016/j.chom.2019.10.009
Borodovich T , Shkoporov AN , Ross RP et al. Phage-mediated horizontal gene transfer and its implications for the human gut microbiome . Gastroenterol Rep (Oxf) 2022 ; 10 : goac012 . https://doi.org/10.1093/gastro/goac012
Brown TL , Charity OJ , Adriaenssens EM . Ecological and functional roles of bacteriophages in contrasting environments: marine, terrestrial and human gut . Curr Opin Microbiol 2022 ; 70 : 102229 . https://doi.org/10.1016/j.mib.2022.102229
Dutilh BE , Cassman N , McNair K et al. A highly abundant bacteriophage discovered in the unknown sequences of human faecal metagenomes . Nat Commun 2014 ; 5 : 4498 . https://doi.org/10.1038/ncomms5498
Devoto AE , Santini JM , Olm MR et al. Megaphages infect Prevotella and variants are widespread in gut microbiomes . Nat Microbiol 2019 ; 4 :693–700.
Ivanova NN , Schwientek P , Tripp HJ et al. Stop codon reassignments in the wild . Science 2014 ; 344 : 909 – 13 . https://doi.org/10.1126/science.1250691
Yutin N , Benler S , Shmakov SA et al. Analysis of metagenome-assembled viral genomes from the human gut reveals diverse putative CrAss-like phages with unique genomic features . Nat Commun 2021 ; 12 : 1044 . https://doi.org/10.1038/s41467-021-21350-w
Cook R , Brown N , Redgwell T et al. INfrastructure for a PHAge REference database: identification of large-scale biases in the current collection of cultured phage genomes . Phage 2021 ; 2 : 214 – 23 Cold Spring Harbor Laboratory .
Peters SL , Borges AL , Giannone RJ et al. Experimental validation that human microbiome phages use alternative genetic coding . Nat Commun 2022 ; 13 : 5710 . https://doi.org/10.1038/s41467-022-32979-6
Borges AL , Lou YC , Sachdeva R et al. Widespread stop-codon recoding in bacteriophages may regulate translation of lytic genes . Nat Microbiol 2022 ; 7 : 918 – 27 . https://doi.org/10.1038/s41564-022-01128-6
Hyatt D , Chen GL , LoCascio PF et al. Prodigal: prokaryotic gene recognition and translation initiation site identification . BMC Bioinformatics 2010 ; 11 :119. BioMed Central . https://doi.org/10.1186/1471-2105-11-119
Camargo AP , Roux S , Schulz F et al. Identification of mobile genetic elements with geNomad . Nat Biotechnol 2023 . https://doi.org/10.1038/s41587-023-01953-y
Larralde MP . Python bindings and interface to prodigal, an efficient method for gene prediction in prokaryotes . J Open Source Softw 2022 ; 7 :4296. https://doi.org/10.21105/joss.04296
Pfennig A , Lomsadze A , Borodovsky M . MgCod: gene prediction in phage genomes with multiple genetic codes . J Mol Biol 2023 ; 435 : 168159 . https://doi.org/10.1016/j.jmb.2023.168159
Seemann T . Prokka: rapid prokaryotic genome annotation . Bioinformatics 2014 ; 30 : 2068 – 9 . https://doi.org/10.1093/bioinformatics/btu153
Bouras G , Nepal R , Houtak G et al. Pharokka: a fast scalable bacteriophage annotation tool . Bioinformatics 2023 ; 39 :btac776. https://doi.org/10.1093/bioinformatics/btac776
Pfennig A , Lomsadze A , Borodovsky M . Annotation of phage genomes with multiple genetic codes . bioRxiv . 2022.2006.2029.495998 2022 . https://doi.org/10.1101/2022.06.29.495998
Chan PP , Lowe TM . tRNAscan-SE: searching for tRNA genes in genomic sequences . Methods Mol Biol 2019 ; 1962 : 1 – 14 . https://doi.org/10.1007/978-1-4939-9173-0_1
Simmonds P , Adriaenssens EM , Zerbini FM et al. Four principles to establish a universal virus taxonomy . PLoS Biol 2023 ; 21 : e3001922 . https://doi.org/10.1371/journal.pbio.3001922
Telatin A , Fariselli P , Birolo G . SeqFu: a suite of Utilities for the Robust and Reproducible Manipulation of sequence files . Bioengineering 2021 ; 8 : 59 . https://doi.org/10.3390/bioengineering8050059
Terzian P , Olo Ndela E , Galiez C et al. PHROG: families of prokaryotic virus proteins clustered using remote homology . NAR Genomics and Bioinformatics . Oxford Academic , 2021 ; 3 :lqab067.
Team, R. C . R: A Language and Environment for Statistical Computing . R Foundation for Statistical Computing , Vienna, 2018 .
Google Preview
Benjamini Y , Hochberg Y . Journal of the Royal Statistical Society: Series B (Methodological) , Vol. 57 . John Wiley & Sons, Ltd , Hoboken, 1995 , 289 – 300 . https://doi.org/10.1111/j.2517-6161.1995.tb02031.x
Wickham H . Ggplot2: Elegant Graphics for Data Analysis , 2nd edn. Springer International Publishing , New York, 2016 .
Supplementary data
Month: | Total Views: |
---|---|
June 2024 | 268 |
Email alerts
Related articles in, citing articles via.
- Advertising & Corporate Services
- Journals Career Network
Affiliations
- Online ISSN 2730-6151
- Copyright © 2024 International Society for Microbial Ecology
- About Oxford Academic
- Publish journals with us
- University press partners
- What we publish
- New features
- Open access
- Institutional account management
- Rights and permissions
- Get help with access
- Accessibility
- Advertising
- Media enquiries
- Oxford University Press
- Oxford Languages
- University of Oxford
Oxford University Press is a department of the University of Oxford. It furthers the University's objective of excellence in research, scholarship, and education by publishing worldwide
- Copyright © 2024 Oxford University Press
- Cookie settings
- Cookie policy
- Privacy policy
- Legal notice
This Feature Is Available To Subscribers Only
Sign In or Create an Account
This PDF is available to Subscribers Only
For full access to this pdf, sign in to an existing account, or purchase an annual subscription.

PlantRNA-FM: An Interpretable RNA Foundation Model for Exploration Functional RNA Motifs in Plants
- Find this author on Google Scholar
- Find this author on PubMed
- Search for this author on this site
- ORCID record for Haopeng Yu
- For correspondence: [email protected]
- Info/History
- Supplementary material
- Preview PDF
The complex 'language' of plant RNA encodes a vast array of biological regulatory elements that orchestrate crucial aspects of plant growth, development, and adaptation to environmental stresses. Recent advancements in foundation models (FMs) have demonstrated their unprecedented potential to decipher complex 'language' in biology. In this study, we introduced PlantRNA-FM, a novel high-performance and interpretable RNA FM specifically designed based on RNA features including both sequence and structure. PlantRNA-FM was pre-trained on an extensive dataset, integrating RNA sequences and RNA structure information from 1,124 distinct plant species. PlantRNA-FM exhibits superior performance in plant-specific downstream tasks, such as plant RNA annotation prediction and RNA translation efficiency (TE) prediction. Compared to the second-best FMs, PlantRNA-FM achieved an F1 score improvement of up to 52.45% in RNA genic region annotation prediction and up to 15.30% in translation efficiency prediction, respectively. Our PlantRNA-FM is empowered by our interpretable framework that facilitates the identification of biologically functional RNA sequence and structure motifs, including both RNA secondary and tertiary structure motifs across transcriptomes. Through experimental validations, we revealed novel translation-associated RNA motifs in plants. Our PlantRNA-FM also highlighted the importance of the position information of these functional RNA motifs in genic regions. Taken together, our PlantRNA-FM facilitates the exploration of functional RNA motifs across the complexity of transcriptomes, empowering plant scientists with novel capabilities for programming RNA codes in plants.
Competing Interest Statement
The authors have declared no competing interest.
The title mistakenly included a number, which has now been removed.
https://huggingface.co/yangheng/PlantRNA-FM
View the discussion thread.
Supplementary Material
Thank you for your interest in spreading the word about bioRxiv.
NOTE: Your email address is requested solely to identify you as the sender of this article.

Citation Manager Formats
- EndNote (tagged)
- EndNote 8 (xml)
- RefWorks Tagged
- Ref Manager
- Tweet Widget
- Facebook Like
- Google Plus One
Subject Area
- Bioinformatics
- Animal Behavior and Cognition (5415)
- Biochemistry (12219)
- Bioengineering (9147)
- Bioinformatics (30177)
- Biophysics (15490)
- Cancer Biology (12596)
- Cell Biology (18082)
- Clinical Trials (138)
- Developmental Biology (9757)
- Ecology (14629)
- Epidemiology (2067)
- Evolutionary Biology (18786)
- Genetics (12557)
- Genomics (17234)
- Immunology (12324)
- Microbiology (29059)
- Molecular Biology (12064)
- Neuroscience (63273)
- Paleontology (464)
- Pathology (1939)
- Pharmacology and Toxicology (3373)
- Physiology (5193)
- Plant Biology (10815)
- Scientific Communication and Education (1710)
- Synthetic Biology (3007)
- Systems Biology (7547)
- Zoology (1692)

IMAGES
VIDEO
COMMENTS
What is functional annotation Functional annotation is defined as the process of collecting information about and describing a [genome feature's]biological identity—its various aliases, molecular function, biological role(s), subcellular location, and its expression domains within the [organism] February 20, 2020 Functional Annotation 14
Genome annotation is a multi-level process that includes prediction of protein-coding genes, as well as other functional genome units such as structural RNAs, tRNAs, small RNAs, pseudogenes, control regions, direct and inverted repeats, insertion sequences, transposons and other mobile elements.
By definition, functional annotation (more precisely, functional prediction) deals with proteins whose functions are unknown, and the rate of experimental testing of predictions is extremely slow. We believe that it is possible to design an objective test of the accuracy of genome annotation in the following manner. The protein set encoded in a ...
One measure of the extent of functional annotation is the number of Gene Ontology (GO) annotations that have been curated from experimental results reported in publications. Eighty-five percent of experimental GO annotations are for genes in 10 well-studied organisms, only one of which is a prokaryote ( Table 1 ).
The Database for Annotation, Visualization and Integrated Discovery () provides a comprehensive set of functional annotation tools for investigators to understand the biological meaning behind large lists of genes.These tools are powered by the comprehensive DAVID Knowledgebase built upon the DAVID Gene concept which pulls together multiple sources of functional annotations.
Functional annotation is a fundamental step in omics data analysis. The annotation of genes and proteins describes their complete biological identities, including biological function, pathways they are involved in and localization. Pathway analysis, also called functional enrichment analysis, is used to identify particularly abundant pathways ...
Functional annotation is defined as the process of collecting information about and describing a gene's biological identity—its various aliases, molecular function, biological role(s), subcellular location, and its expression domains within the plant. At TAIR, we obtain this information from reading the published literature and by soliciting ...
Genome annotation is the process of deriving the structural and functional information of a protein or gene from a raw data set using different analysis, comparison, estimation, precision, and other mining techniques. From: Bioinformatics, 2022. Add to Mendeley.
Functional Annotation is a way of organising and understanding the vast amount of information contained in a genome, and it's an essential tool for scientist...
Functional annotation of genomes is a prerequisite for contemporary basic and applied genomic research, yet farmed animal genomics is deficient in such annotation. To address this, the FAANG (Functional Annotation of Animal Genomes) Consortium is producing genome-wide data sets on RNA expression, DNA methylation, and chromatin modification, as well as chromatin accessibility and interactions.
The Functional Annotation of Animal Genomes consortium was formed to collaboratively annotate the functional elements in animal genomes, starting with domesticated animals.
Functional genomics is a field of molecular biology that attempts to describe gene (and protein) functions and interactions. Functional genomics make use of the vast data generated by genomic and transcriptomic projects (such as genome sequencing projects and RNA sequencing ). Functional genomics focuses on the dynamic aspects such as gene ...
Functional annotation consists of attaching biological information to genomic elements: biochemical function, biological function, involved regulation and interactions, and expression. These steps may involve both biological experiments and in silico analysis. Proteogenomics based approaches utilize information from expressed proteins, often ...
• The best functional annotation systems use human beings who read the literature before assigning a function to a gene. Functional Annotation Some difficulties • Different people use different words for the same function • They mean different things by the same word. • The context in which a gene was found may not be associated with its
Using an annotation tool like this can help you understand more about the genes and pathways present in your sample(s). For example, as previously described, the paper this data is pulled from uses functional annotation of MAGs to look for genes associated with denitrification pathways. Building a tree from the 16S sequence
Background High-throughput sequencing has increased the number of available microbial genomes recovered from isolates, single cells, and metagenomes. Accordingly, fast and comprehensive functional gene annotation pipelines are needed to analyze and compare these genomes. Although several approaches exist for genome annotation, these are typically not designed for easy incorporation into ...
Downstream analysis of genomic and transcriptomic sequence data is often executed by functional annotation that can be performed by various bioinformatics tools and biological databases. However ...
Functional annotation can be performed through probabilistic methods. The distribution of hydrophilic and hydrophobic amino acids indicates whether a protein is located in a solution or membrane. Specific sequence motifs provide information on posttranslational modifications and final location of any given protein.
Most interpretations of genomic analyses rely on accurate functional annotations of the proteins encoded by more than 500 000 genomes sequenced to date. By different estimates, only half the predicted sequenced proteins carry an accurate functional annotation, and this percentage varies drastically between different organismal lineages.
Functional annotation of genes is an essential step in omics data analysis. Multiple databases and methods are currently available to summarize the functions of sets of genes into higher level representations, such as ontologies and molecular pathways. Annotating results from omics experiments into functional categories is essential not only to understand the underlying regulatory dynamics but ...
Please note that Functional Annotation Clustering and Gene Functional Classification have a 3000 gene limit. 18. What is the format requirement for my input gene list? You can either load a gene list from a file or paste a gene list to the text box. DAVID was designed to accept the data starting from the first row without header (i.e. accession).
Functional annotation is the process during which we try to put names to faces - what do genes that we have annotated and curated? Basically all existing approaches accomplish this by means of similarity. If a translation product has strong similarity to a protein that has previously been assigned a function, the function in this newly ...
Functional annotation is the process of attaching biological information to sequences of genes or proteins. The basic level of annotation is using sequence alignment tool BLAST for finding similarities, and then annotating genes or proteins based on that. Nevertheless, nowadays more and more additional information of biological functions is ...
Furthermore, the proportion of genes that could be assigned functional annotation increased, including an increase in the number of major capsid proteins that could be identified. We propose that automatic prediction of stop codon reassignment before annotation is beneficial to downstream viral genomic and metagenomic analyses.
The complex 'language' of plant RNA encodes a vast array of biological regulatory elements that orchestrate crucial aspects of plant growth, development, and adaptation to environmental stresses. Recent advancements in foundation models (FMs) have demonstrated their unprecedented potential to decipher complex 'language' in biology. In this study, we introduced PlantRNA-FM, a novel high ...