Qualitative case study data analysis: an example from practice
Affiliation.
- 1 School of Nursing and Midwifery, National University of Ireland, Galway, Republic of Ireland.
- PMID: 25976531
- DOI: 10.7748/nr.22.5.8.e1307
Aim: To illustrate an approach to data analysis in qualitative case study methodology.
Background: There is often little detail in case study research about how data were analysed. However, it is important that comprehensive analysis procedures are used because there are often large sets of data from multiple sources of evidence. Furthermore, the ability to describe in detail how the analysis was conducted ensures rigour in reporting qualitative research.
Data sources: The research example used is a multiple case study that explored the role of the clinical skills laboratory in preparing students for the real world of practice. Data analysis was conducted using a framework guided by the four stages of analysis outlined by Morse ( 1994 ): comprehending, synthesising, theorising and recontextualising. The specific strategies for analysis in these stages centred on the work of Miles and Huberman ( 1994 ), which has been successfully used in case study research. The data were managed using NVivo software.
Review methods: Literature examining qualitative data analysis was reviewed and strategies illustrated by the case study example provided. Discussion Each stage of the analysis framework is described with illustration from the research example for the purpose of highlighting the benefits of a systematic approach to handling large data sets from multiple sources.
Conclusion: By providing an example of how each stage of the analysis was conducted, it is hoped that researchers will be able to consider the benefits of such an approach to their own case study analysis.
Implications for research/practice: This paper illustrates specific strategies that can be employed when conducting data analysis in case study research and other qualitative research designs.
Keywords: Case study data analysis; case study research methodology; clinical skills research; qualitative case study methodology; qualitative data analysis; qualitative research.
- Case-Control Studies*
- Data Interpretation, Statistical*
- Nursing Research / methods*
- Qualitative Research*
- Research Design
- AI & NLP
- Churn & Loyalty
- Customer Experience
- Customer Journeys
- Customer Metrics
- Feedback Analysis
- Product Experience
- Product Updates
- Sentiment Analysis
- Surveys & Feedback Collection
- Try Thematic
Welcome to the community
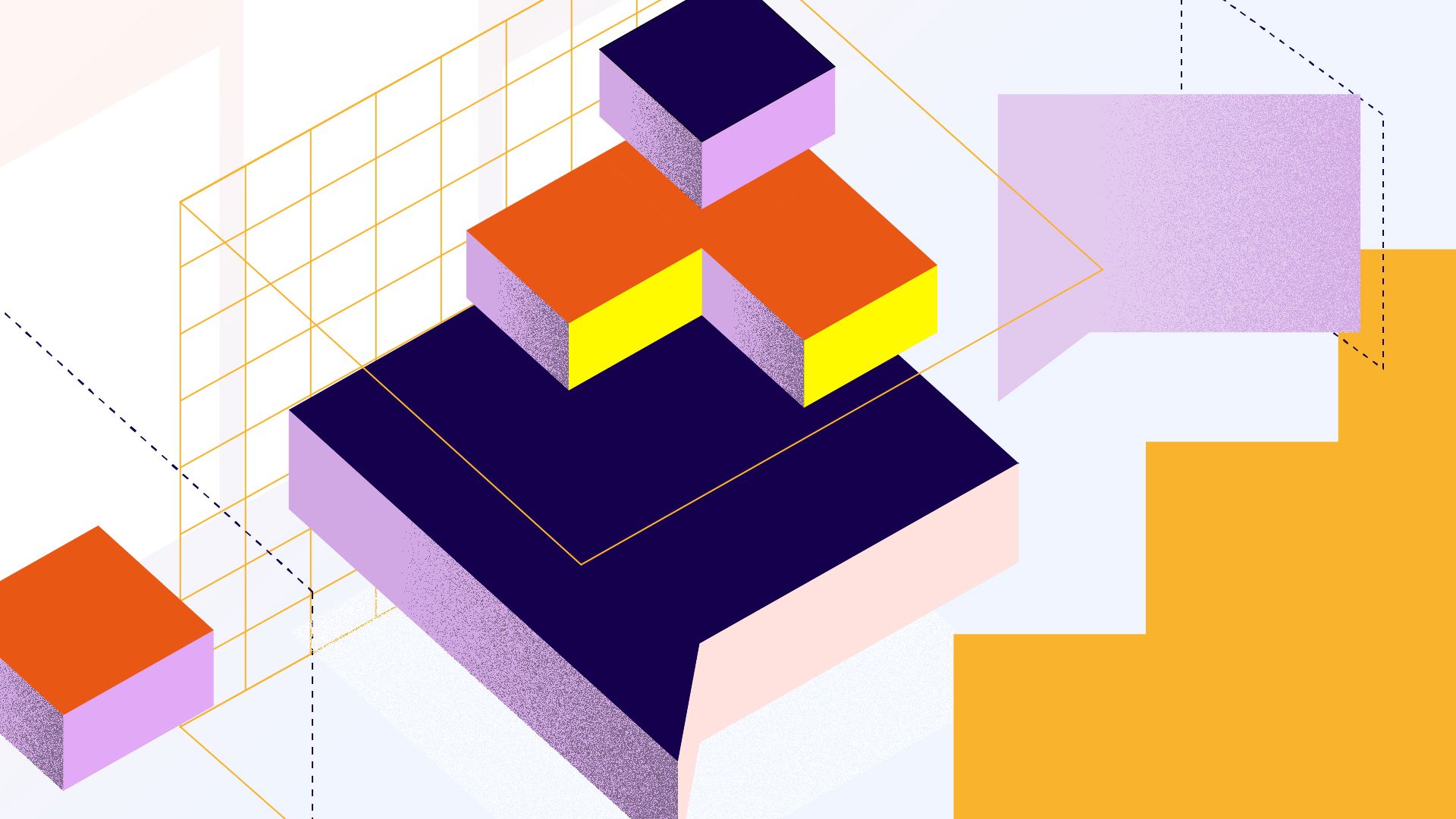

Qualitative Data Analysis: Step-by-Step Guide (Manual vs. Automatic)
When we conduct qualitative methods of research, need to explain changes in metrics or understand people's opinions, we always turn to qualitative data. Qualitative data is typically generated through:
- Interview transcripts
- Surveys with open-ended questions
- Contact center transcripts
- Texts and documents
- Audio and video recordings
- Observational notes
Compared to quantitative data, which captures structured information, qualitative data is unstructured and has more depth. It can answer our questions, can help formulate hypotheses and build understanding.
It's important to understand the differences between quantitative data & qualitative data . But unfortunately, analyzing qualitative data is difficult. While tools like Excel, Tableau and PowerBI crunch and visualize quantitative data with ease, there are a limited number of mainstream tools for analyzing qualitative data . The majority of qualitative data analysis still happens manually.
That said, there are two new trends that are changing this. First, there are advances in natural language processing (NLP) which is focused on understanding human language. Second, there is an explosion of user-friendly software designed for both researchers and businesses. Both help automate the qualitative data analysis process.
In this post we want to teach you how to conduct a successful qualitative data analysis. There are two primary qualitative data analysis methods; manual & automatic. We will teach you how to conduct the analysis manually, and also, automatically using software solutions powered by NLP. We’ll guide you through the steps to conduct a manual analysis, and look at what is involved and the role technology can play in automating this process.
More businesses are switching to fully-automated analysis of qualitative customer data because it is cheaper, faster, and just as accurate. Primarily, businesses purchase subscriptions to feedback analytics platforms so that they can understand customer pain points and sentiment.
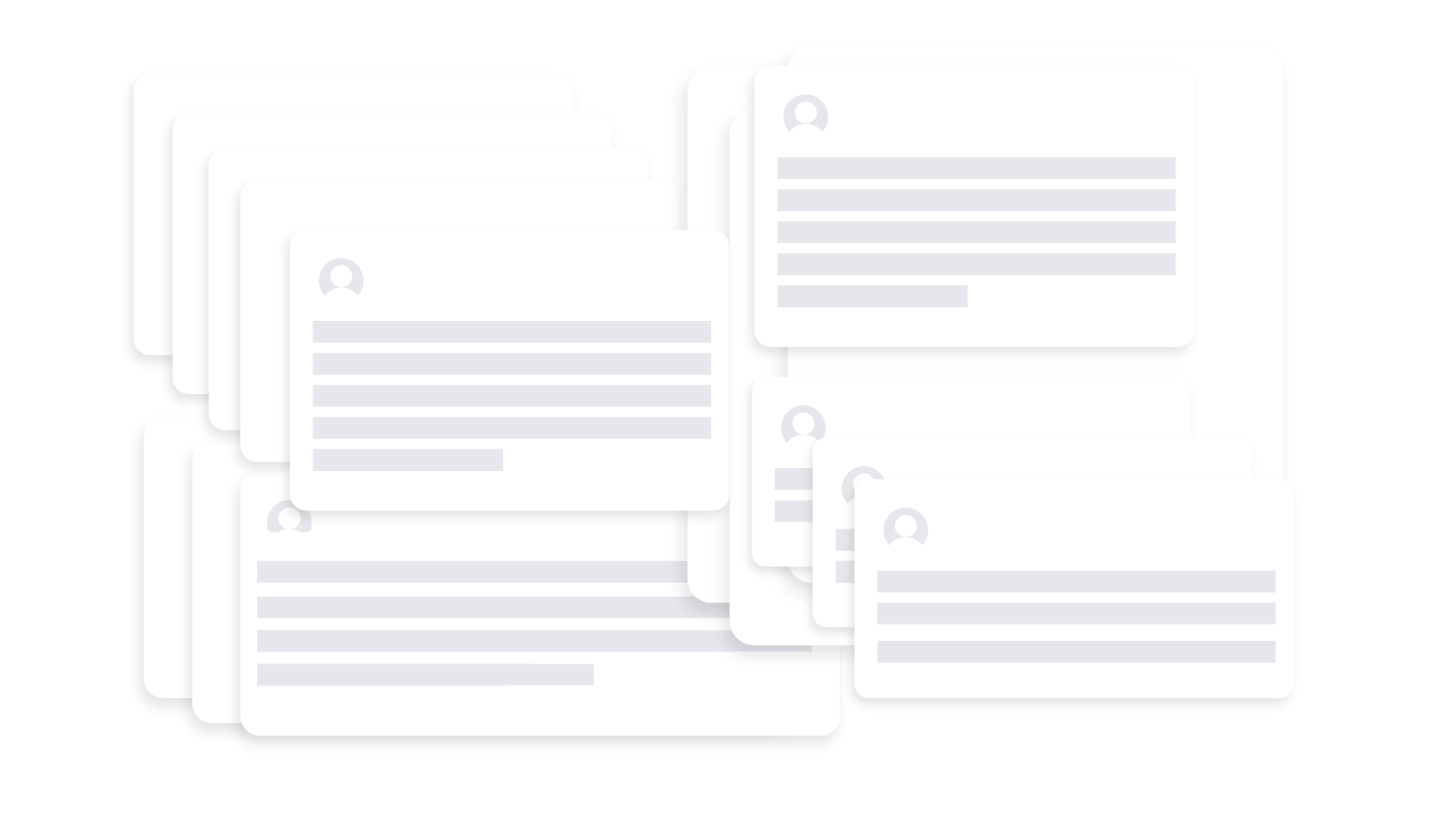
We’ll take you through 5 steps to conduct a successful qualitative data analysis. Within each step we will highlight the key difference between the manual, and automated approach of qualitative researchers. Here's an overview of the steps:
The 5 steps to doing qualitative data analysis
- Gathering and collecting your qualitative data
- Organizing and connecting into your qualitative data
- Coding your qualitative data
- Analyzing the qualitative data for insights
- Reporting on the insights derived from your analysis
What is Qualitative Data Analysis?
Qualitative data analysis is a process of gathering, structuring and interpreting qualitative data to understand what it represents.
Qualitative data is non-numerical and unstructured. Qualitative data generally refers to text, such as open-ended responses to survey questions or user interviews, but also includes audio, photos and video.
Businesses often perform qualitative data analysis on customer feedback. And within this context, qualitative data generally refers to verbatim text data collected from sources such as reviews, complaints, chat messages, support centre interactions, customer interviews, case notes or social media comments.
How is qualitative data analysis different from quantitative data analysis?
Understanding the differences between quantitative & qualitative data is important. When it comes to analyzing data, Qualitative Data Analysis serves a very different role to Quantitative Data Analysis. But what sets them apart?
Qualitative Data Analysis dives into the stories hidden in non-numerical data such as interviews, open-ended survey answers, or notes from observations. It uncovers the ‘whys’ and ‘hows’ giving a deep understanding of people’s experiences and emotions.
Quantitative Data Analysis on the other hand deals with numerical data, using statistics to measure differences, identify preferred options, and pinpoint root causes of issues. It steps back to address questions like "how many" or "what percentage" to offer broad insights we can apply to larger groups.
In short, Qualitative Data Analysis is like a microscope, helping us understand specific detail. Quantitative Data Analysis is like the telescope, giving us a broader perspective. Both are important, working together to decode data for different objectives.
Qualitative Data Analysis methods
Once all the data has been captured, there are a variety of analysis techniques available and the choice is determined by your specific research objectives and the kind of data you’ve gathered. Common qualitative data analysis methods include:
Content Analysis
This is a popular approach to qualitative data analysis. Other qualitative analysis techniques may fit within the broad scope of content analysis. Thematic analysis is a part of the content analysis. Content analysis is used to identify the patterns that emerge from text, by grouping content into words, concepts, and themes. Content analysis is useful to quantify the relationship between all of the grouped content. The Columbia School of Public Health has a detailed breakdown of content analysis .
Narrative Analysis
Narrative analysis focuses on the stories people tell and the language they use to make sense of them. It is particularly useful in qualitative research methods where customer stories are used to get a deep understanding of customers’ perspectives on a specific issue. A narrative analysis might enable us to summarize the outcomes of a focused case study.
Discourse Analysis
Discourse analysis is used to get a thorough understanding of the political, cultural and power dynamics that exist in specific situations. The focus of discourse analysis here is on the way people express themselves in different social contexts. Discourse analysis is commonly used by brand strategists who hope to understand why a group of people feel the way they do about a brand or product.
Thematic Analysis
Thematic analysis is used to deduce the meaning behind the words people use. This is accomplished by discovering repeating themes in text. These meaningful themes reveal key insights into data and can be quantified, particularly when paired with sentiment analysis . Often, the outcome of thematic analysis is a code frame that captures themes in terms of codes, also called categories. So the process of thematic analysis is also referred to as “coding”. A common use-case for thematic analysis in companies is analysis of customer feedback.
Grounded Theory
Grounded theory is a useful approach when little is known about a subject. Grounded theory starts by formulating a theory around a single data case. This means that the theory is “grounded”. Grounded theory analysis is based on actual data, and not entirely speculative. Then additional cases can be examined to see if they are relevant and can add to the original grounded theory.
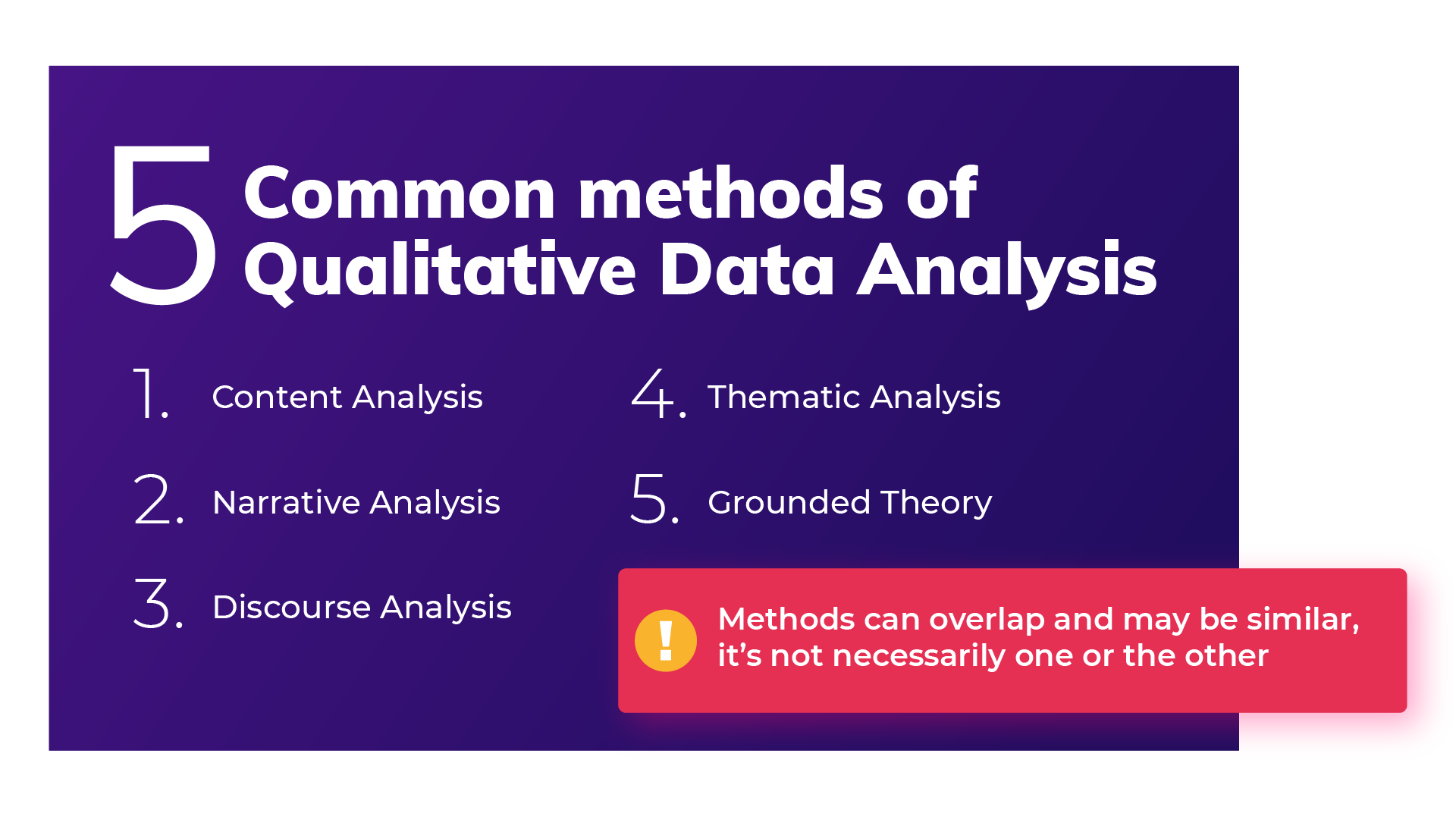
Challenges of Qualitative Data Analysis
While Qualitative Data Analysis offers rich insights, it comes with its challenges. Each unique QDA method has its unique hurdles. Let’s take a look at the challenges researchers and analysts might face, depending on the chosen method.
- Time and Effort (Narrative Analysis): Narrative analysis, which focuses on personal stories, demands patience. Sifting through lengthy narratives to find meaningful insights can be time-consuming, requires dedicated effort.
- Being Objective (Grounded Theory): Grounded theory, building theories from data, faces the challenges of personal biases. Staying objective while interpreting data is crucial, ensuring conclusions are rooted in the data itself.
- Complexity (Thematic Analysis): Thematic analysis involves identifying themes within data, a process that can be intricate. Categorizing and understanding themes can be complex, especially when each piece of data varies in context and structure. Thematic Analysis software can simplify this process.
- Generalizing Findings (Narrative Analysis): Narrative analysis, dealing with individual stories, makes drawing broad challenging. Extending findings from a single narrative to a broader context requires careful consideration.
- Managing Data (Thematic Analysis): Thematic analysis involves organizing and managing vast amounts of unstructured data, like interview transcripts. Managing this can be a hefty task, requiring effective data management strategies.
- Skill Level (Grounded Theory): Grounded theory demands specific skills to build theories from the ground up. Finding or training analysts with these skills poses a challenge, requiring investment in building expertise.
Benefits of qualitative data analysis
Qualitative Data Analysis (QDA) is like a versatile toolkit, offering a tailored approach to understanding your data. The benefits it offers are as diverse as the methods. Let’s explore why choosing the right method matters.
- Tailored Methods for Specific Needs: QDA isn't one-size-fits-all. Depending on your research objectives and the type of data at hand, different methods offer unique benefits. If you want emotive customer stories, narrative analysis paints a strong picture. When you want to explain a score, thematic analysis reveals insightful patterns
- Flexibility with Thematic Analysis: thematic analysis is like a chameleon in the toolkit of QDA. It adapts well to different types of data and research objectives, making it a top choice for any qualitative analysis.
- Deeper Understanding, Better Products: QDA helps you dive into people's thoughts and feelings. This deep understanding helps you build products and services that truly matches what people want, ensuring satisfied customers
- Finding the Unexpected: Qualitative data often reveals surprises that we miss in quantitative data. QDA offers us new ideas and perspectives, for insights we might otherwise miss.
- Building Effective Strategies: Insights from QDA are like strategic guides. They help businesses in crafting plans that match people’s desires.
- Creating Genuine Connections: Understanding people’s experiences lets businesses connect on a real level. This genuine connection helps build trust and loyalty, priceless for any business.
How to do Qualitative Data Analysis: 5 steps
Now we are going to show how you can do your own qualitative data analysis. We will guide you through this process step by step. As mentioned earlier, you will learn how to do qualitative data analysis manually , and also automatically using modern qualitative data and thematic analysis software.
To get best value from the analysis process and research process, it’s important to be super clear about the nature and scope of the question that’s being researched. This will help you select the research collection channels that are most likely to help you answer your question.
Depending on if you are a business looking to understand customer sentiment, or an academic surveying a school, your approach to qualitative data analysis will be unique.
Once you’re clear, there’s a sequence to follow. And, though there are differences in the manual and automatic approaches, the process steps are mostly the same.
The use case for our step-by-step guide is a company looking to collect data (customer feedback data), and analyze the customer feedback - in order to improve customer experience. By analyzing the customer feedback the company derives insights about their business and their customers. You can follow these same steps regardless of the nature of your research. Let’s get started.
Step 1: Gather your qualitative data and conduct research (Conduct qualitative research)
The first step of qualitative research is to do data collection. Put simply, data collection is gathering all of your data for analysis. A common situation is when qualitative data is spread across various sources.
Classic methods of gathering qualitative data
Most companies use traditional methods for gathering qualitative data: conducting interviews with research participants, running surveys, and running focus groups. This data is typically stored in documents, CRMs, databases and knowledge bases. It’s important to examine which data is available and needs to be included in your research project, based on its scope.
Using your existing qualitative feedback
As it becomes easier for customers to engage across a range of different channels, companies are gathering increasingly large amounts of both solicited and unsolicited qualitative feedback.
Most organizations have now invested in Voice of Customer programs , support ticketing systems, chatbot and support conversations, emails and even customer Slack chats.
These new channels provide companies with new ways of getting feedback, and also allow the collection of unstructured feedback data at scale.
The great thing about this data is that it contains a wealth of valubale insights and that it’s already there! When you have a new question about user behavior or your customers, you don’t need to create a new research study or set up a focus group. You can find most answers in the data you already have.
Typically, this data is stored in third-party solutions or a central database, but there are ways to export it or connect to a feedback analysis solution through integrations or an API.
Utilize untapped qualitative data channels
There are many online qualitative data sources you may not have considered. For example, you can find useful qualitative data in social media channels like Twitter or Facebook. Online forums, review sites, and online communities such as Discourse or Reddit also contain valuable data about your customers, or research questions.
If you are considering performing a qualitative benchmark analysis against competitors - the internet is your best friend, and review analysis is a great place to start. Gathering feedback in competitor reviews on sites like Trustpilot, G2, Capterra, Better Business Bureau or on app stores is a great way to perform a competitor benchmark analysis.
Customer feedback analysis software often has integrations into social media and review sites, or you could use a solution like DataMiner to scrape the reviews.
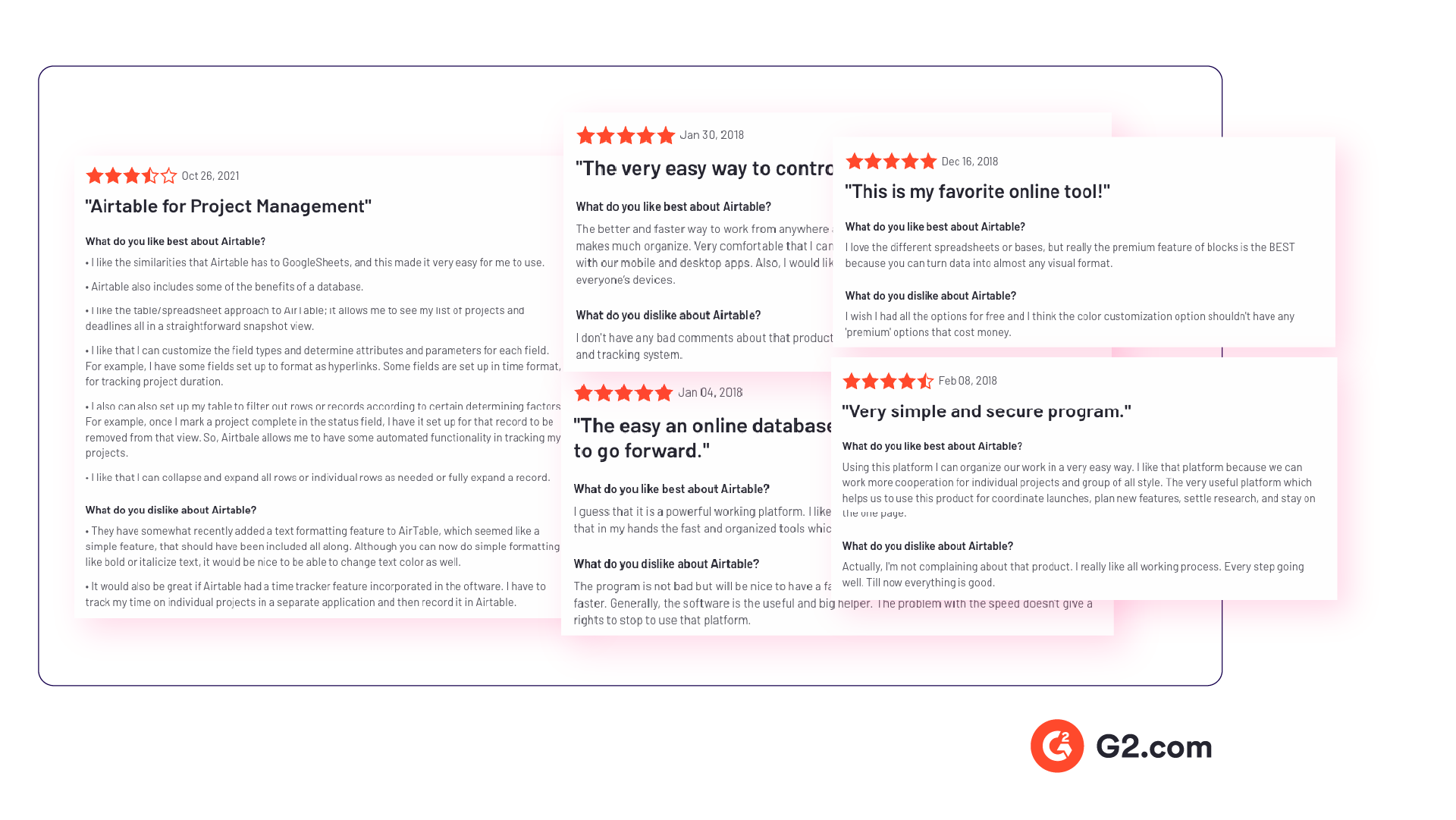
Step 2: Connect & organize all your qualitative data
Now you all have this qualitative data but there’s a problem, the data is unstructured. Before feedback can be analyzed and assigned any value, it needs to be organized in a single place. Why is this important? Consistency!
If all data is easily accessible in one place and analyzed in a consistent manner, you will have an easier time summarizing and making decisions based on this data.
The manual approach to organizing your data
The classic method of structuring qualitative data is to plot all the raw data you’ve gathered into a spreadsheet.
Typically, research and support teams would share large Excel sheets and different business units would make sense of the qualitative feedback data on their own. Each team collects and organizes the data in a way that best suits them, which means the feedback tends to be kept in separate silos.
An alternative and a more robust solution is to store feedback in a central database, like Snowflake or Amazon Redshift .
Keep in mind that when you organize your data in this way, you are often preparing it to be imported into another software. If you go the route of a database, you would need to use an API to push the feedback into a third-party software.
Computer-assisted qualitative data analysis software (CAQDAS)
Traditionally within the manual analysis approach (but not always), qualitative data is imported into CAQDAS software for coding.
In the early 2000s, CAQDAS software was popularised by developers such as ATLAS.ti, NVivo and MAXQDA and eagerly adopted by researchers to assist with the organizing and coding of data.
The benefits of using computer-assisted qualitative data analysis software:
- Assists in the organizing of your data
- Opens you up to exploring different interpretations of your data analysis
- Allows you to share your dataset easier and allows group collaboration (allows for secondary analysis)
However you still need to code the data, uncover the themes and do the analysis yourself. Therefore it is still a manual approach.
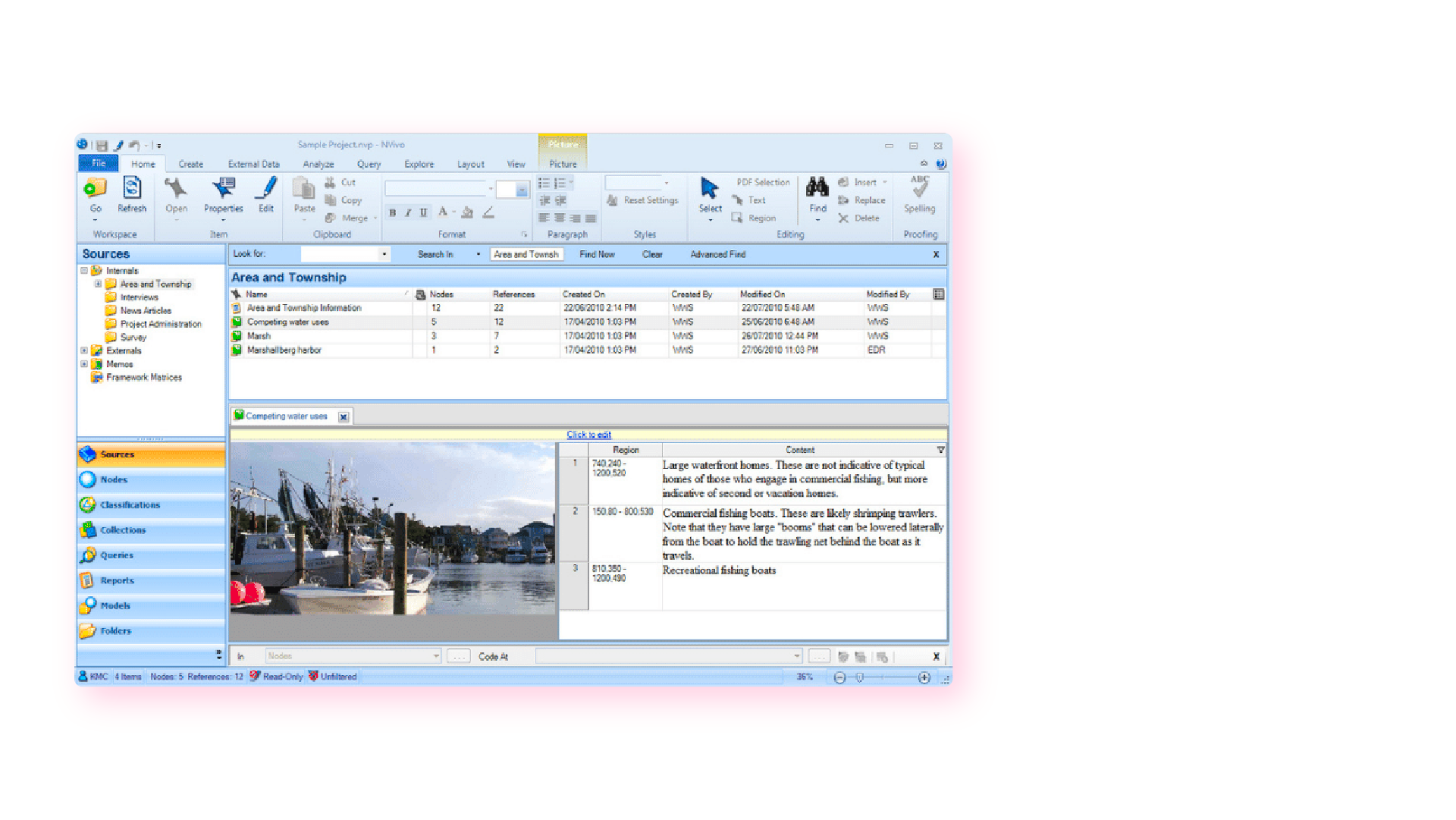
Organizing your qualitative data in a feedback repository
Another solution to organizing your qualitative data is to upload it into a feedback repository where it can be unified with your other data , and easily searchable and taggable. There are a number of software solutions that act as a central repository for your qualitative research data. Here are a couple solutions that you could investigate:
- Dovetail: Dovetail is a research repository with a focus on video and audio transcriptions. You can tag your transcriptions within the platform for theme analysis. You can also upload your other qualitative data such as research reports, survey responses, support conversations, and customer interviews. Dovetail acts as a single, searchable repository. And makes it easier to collaborate with other people around your qualitative research.
- EnjoyHQ: EnjoyHQ is another research repository with similar functionality to Dovetail. It boasts a more sophisticated search engine, but it has a higher starting subscription cost.
Organizing your qualitative data in a feedback analytics platform
If you have a lot of qualitative customer or employee feedback, from the likes of customer surveys or employee surveys, you will benefit from a feedback analytics platform. A feedback analytics platform is a software that automates the process of both sentiment analysis and thematic analysis . Companies use the integrations offered by these platforms to directly tap into their qualitative data sources (review sites, social media, survey responses, etc.). The data collected is then organized and analyzed consistently within the platform.
If you have data prepared in a spreadsheet, it can also be imported into feedback analytics platforms.
Once all this rich data has been organized within the feedback analytics platform, it is ready to be coded and themed, within the same platform. Thematic is a feedback analytics platform that offers one of the largest libraries of integrations with qualitative data sources.
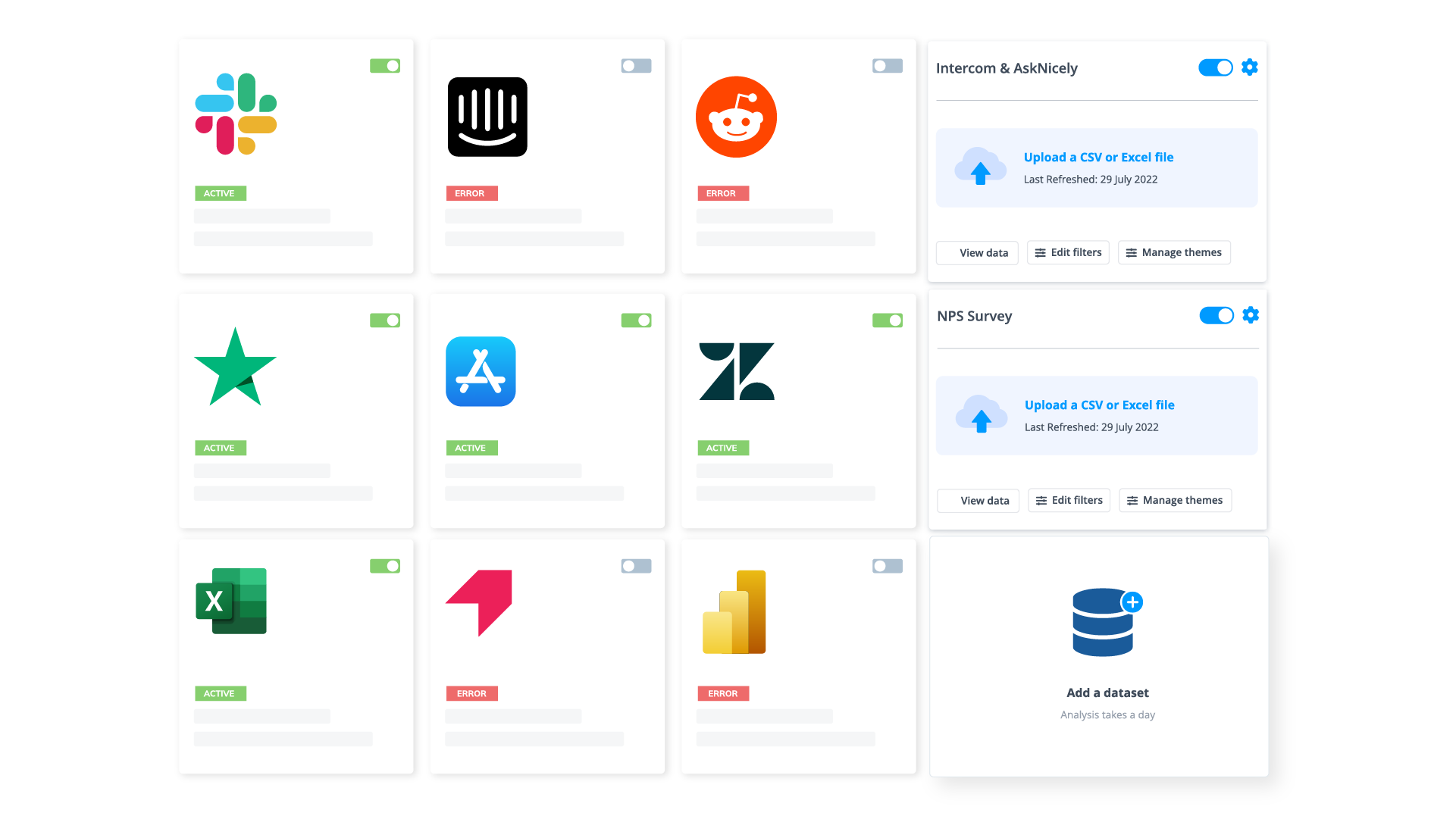
Step 3: Coding your qualitative data
Your feedback data is now organized in one place. Either within your spreadsheet, CAQDAS, feedback repository or within your feedback analytics platform. The next step is to code your feedback data so we can extract meaningful insights in the next step.
Coding is the process of labelling and organizing your data in such a way that you can then identify themes in the data, and the relationships between these themes.
To simplify the coding process, you will take small samples of your customer feedback data, come up with a set of codes, or categories capturing themes, and label each piece of feedback, systematically, for patterns and meaning. Then you will take a larger sample of data, revising and refining the codes for greater accuracy and consistency as you go.
If you choose to use a feedback analytics platform, much of this process will be automated and accomplished for you.
The terms to describe different categories of meaning (‘theme’, ‘code’, ‘tag’, ‘category’ etc) can be confusing as they are often used interchangeably. For clarity, this article will use the term ‘code’.
To code means to identify key words or phrases and assign them to a category of meaning. “I really hate the customer service of this computer software company” would be coded as “poor customer service”.
How to manually code your qualitative data
- Decide whether you will use deductive or inductive coding. Deductive coding is when you create a list of predefined codes, and then assign them to the qualitative data. Inductive coding is the opposite of this, you create codes based on the data itself. Codes arise directly from the data and you label them as you go. You need to weigh up the pros and cons of each coding method and select the most appropriate.
- Read through the feedback data to get a broad sense of what it reveals. Now it’s time to start assigning your first set of codes to statements and sections of text.
- Keep repeating step 2, adding new codes and revising the code description as often as necessary. Once it has all been coded, go through everything again, to be sure there are no inconsistencies and that nothing has been overlooked.
- Create a code frame to group your codes. The coding frame is the organizational structure of all your codes. And there are two commonly used types of coding frames, flat, or hierarchical. A hierarchical code frame will make it easier for you to derive insights from your analysis.
- Based on the number of times a particular code occurs, you can now see the common themes in your feedback data. This is insightful! If ‘bad customer service’ is a common code, it’s time to take action.
We have a detailed guide dedicated to manually coding your qualitative data .
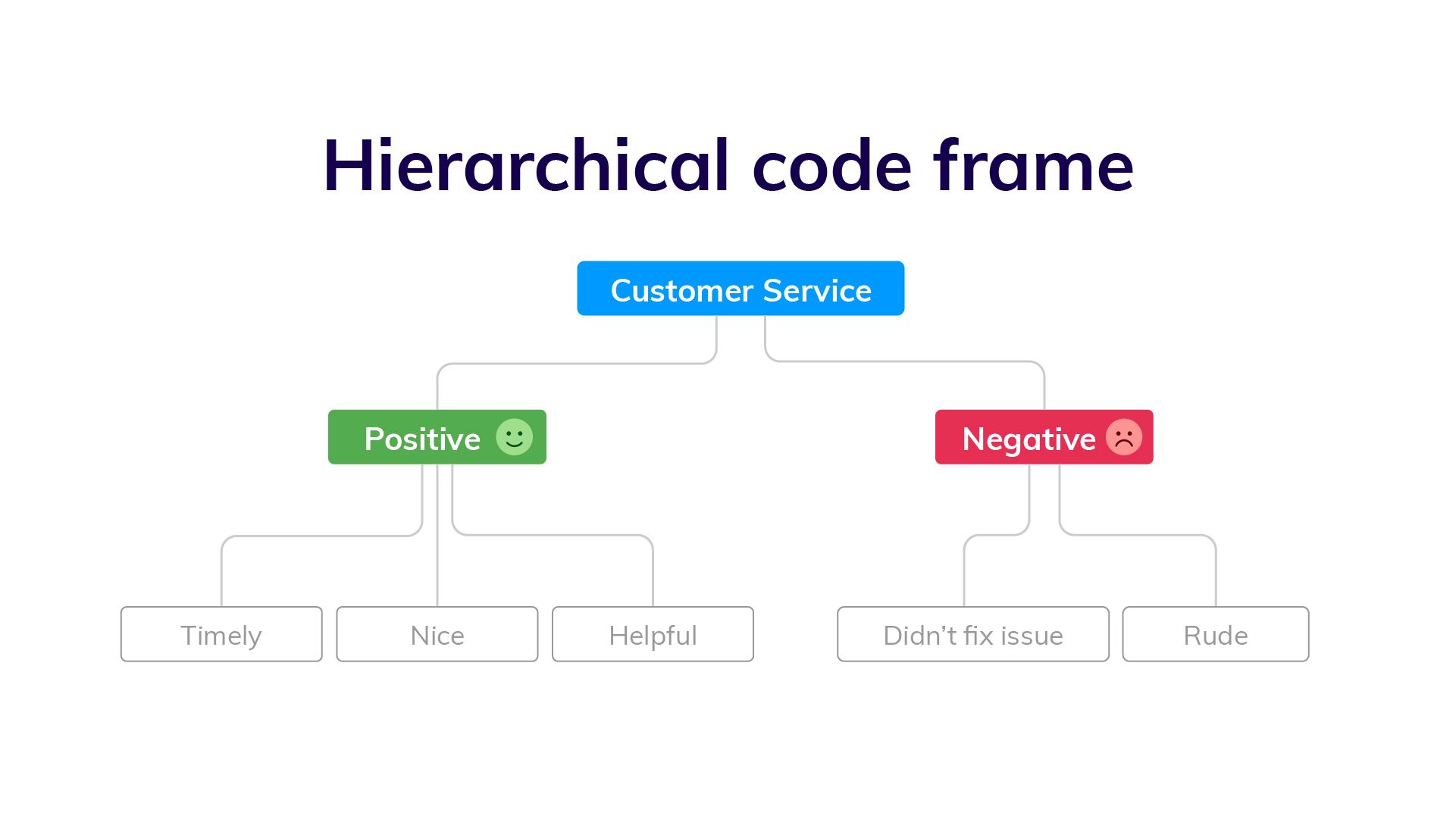
Using software to speed up manual coding of qualitative data
An Excel spreadsheet is still a popular method for coding. But various software solutions can help speed up this process. Here are some examples.
- CAQDAS / NVivo - CAQDAS software has built-in functionality that allows you to code text within their software. You may find the interface the software offers easier for managing codes than a spreadsheet.
- Dovetail/EnjoyHQ - You can tag transcripts and other textual data within these solutions. As they are also repositories you may find it simpler to keep the coding in one platform.
- IBM SPSS - SPSS is a statistical analysis software that may make coding easier than in a spreadsheet.
- Ascribe - Ascribe’s ‘Coder’ is a coding management system. Its user interface will make it easier for you to manage your codes.
Automating the qualitative coding process using thematic analysis software
In solutions which speed up the manual coding process, you still have to come up with valid codes and often apply codes manually to pieces of feedback. But there are also solutions that automate both the discovery and the application of codes.
Advances in machine learning have now made it possible to read, code and structure qualitative data automatically. This type of automated coding is offered by thematic analysis software .
Automation makes it far simpler and faster to code the feedback and group it into themes. By incorporating natural language processing (NLP) into the software, the AI looks across sentences and phrases to identify common themes meaningful statements. Some automated solutions detect repeating patterns and assign codes to them, others make you train the AI by providing examples. You could say that the AI learns the meaning of the feedback on its own.
Thematic automates the coding of qualitative feedback regardless of source. There’s no need to set up themes or categories in advance. Simply upload your data and wait a few minutes. You can also manually edit the codes to further refine their accuracy. Experiments conducted indicate that Thematic’s automated coding is just as accurate as manual coding .
Paired with sentiment analysis and advanced text analytics - these automated solutions become powerful for deriving quality business or research insights.
You could also build your own , if you have the resources!
The key benefits of using an automated coding solution
Automated analysis can often be set up fast and there’s the potential to uncover things that would never have been revealed if you had given the software a prescribed list of themes to look for.
Because the model applies a consistent rule to the data, it captures phrases or statements that a human eye might have missed.
Complete and consistent analysis of customer feedback enables more meaningful findings. Leading us into step 4.
Step 4: Analyze your data: Find meaningful insights
Now we are going to analyze our data to find insights. This is where we start to answer our research questions. Keep in mind that step 4 and step 5 (tell the story) have some overlap . This is because creating visualizations is both part of analysis process and reporting.
The task of uncovering insights is to scour through the codes that emerge from the data and draw meaningful correlations from them. It is also about making sure each insight is distinct and has enough data to support it.
Part of the analysis is to establish how much each code relates to different demographics and customer profiles, and identify whether there’s any relationship between these data points.
Manually create sub-codes to improve the quality of insights
If your code frame only has one level, you may find that your codes are too broad to be able to extract meaningful insights. This is where it is valuable to create sub-codes to your primary codes. This process is sometimes referred to as meta coding.
Note: If you take an inductive coding approach, you can create sub-codes as you are reading through your feedback data and coding it.
While time-consuming, this exercise will improve the quality of your analysis. Here is an example of what sub-codes could look like.
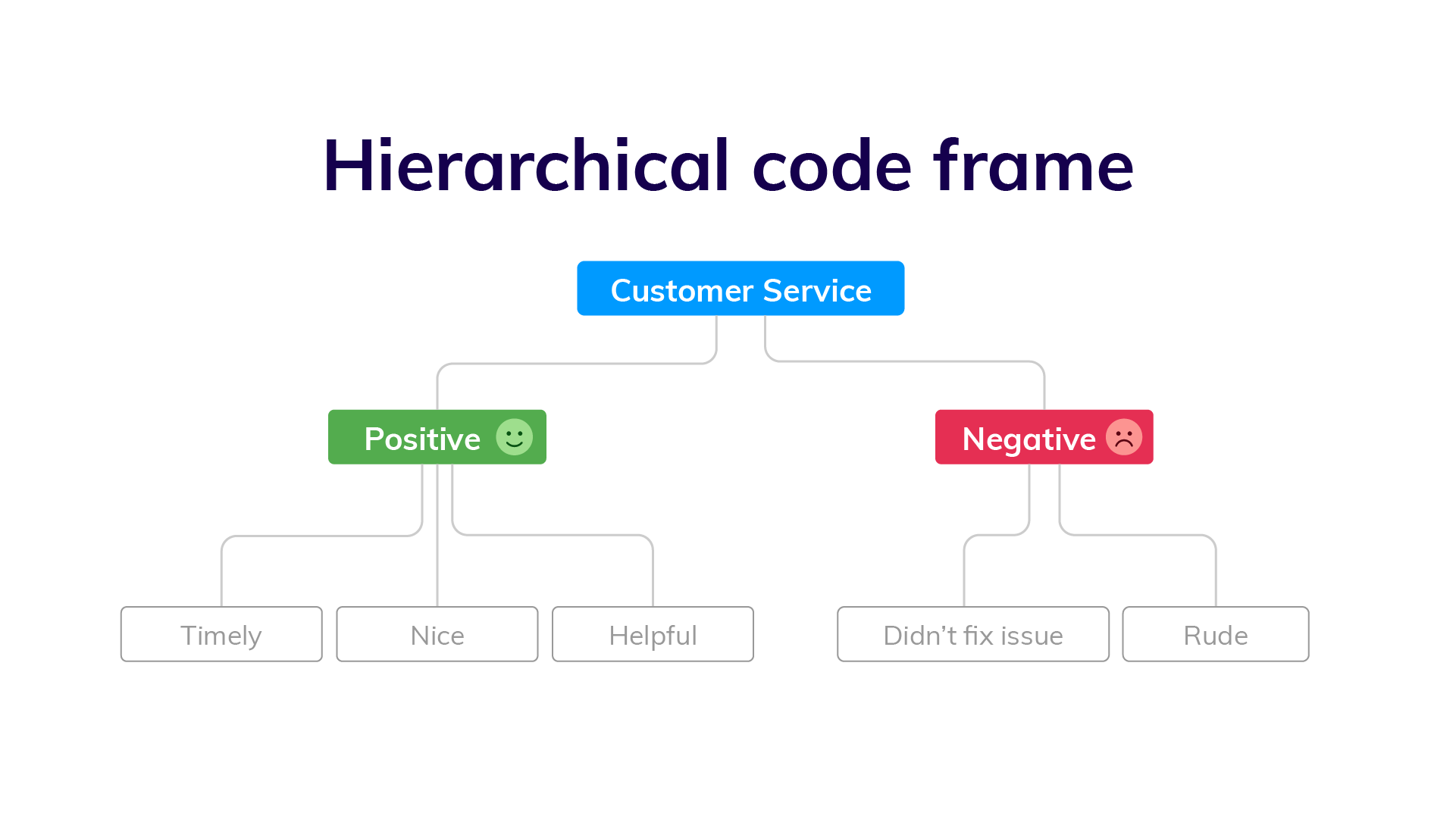
You need to carefully read your qualitative data to create quality sub-codes. But as you can see, the depth of analysis is greatly improved. By calculating the frequency of these sub-codes you can get insight into which customer service problems you can immediately address.
Correlate the frequency of codes to customer segments
Many businesses use customer segmentation . And you may have your own respondent segments that you can apply to your qualitative analysis. Segmentation is the practise of dividing customers or research respondents into subgroups.
Segments can be based on:
- Demographic
- And any other data type that you care to segment by
It is particularly useful to see the occurrence of codes within your segments. If one of your customer segments is considered unimportant to your business, but they are the cause of nearly all customer service complaints, it may be in your best interest to focus attention elsewhere. This is a useful insight!
Manually visualizing coded qualitative data
There are formulas you can use to visualize key insights in your data. The formulas we will suggest are imperative if you are measuring a score alongside your feedback.
If you are collecting a metric alongside your qualitative data this is a key visualization. Impact answers the question: “What’s the impact of a code on my overall score?”. Using Net Promoter Score (NPS) as an example, first you need to:
- Calculate overall NPS
- Calculate NPS in the subset of responses that do not contain that theme
- Subtract B from A
Then you can use this simple formula to calculate code impact on NPS .
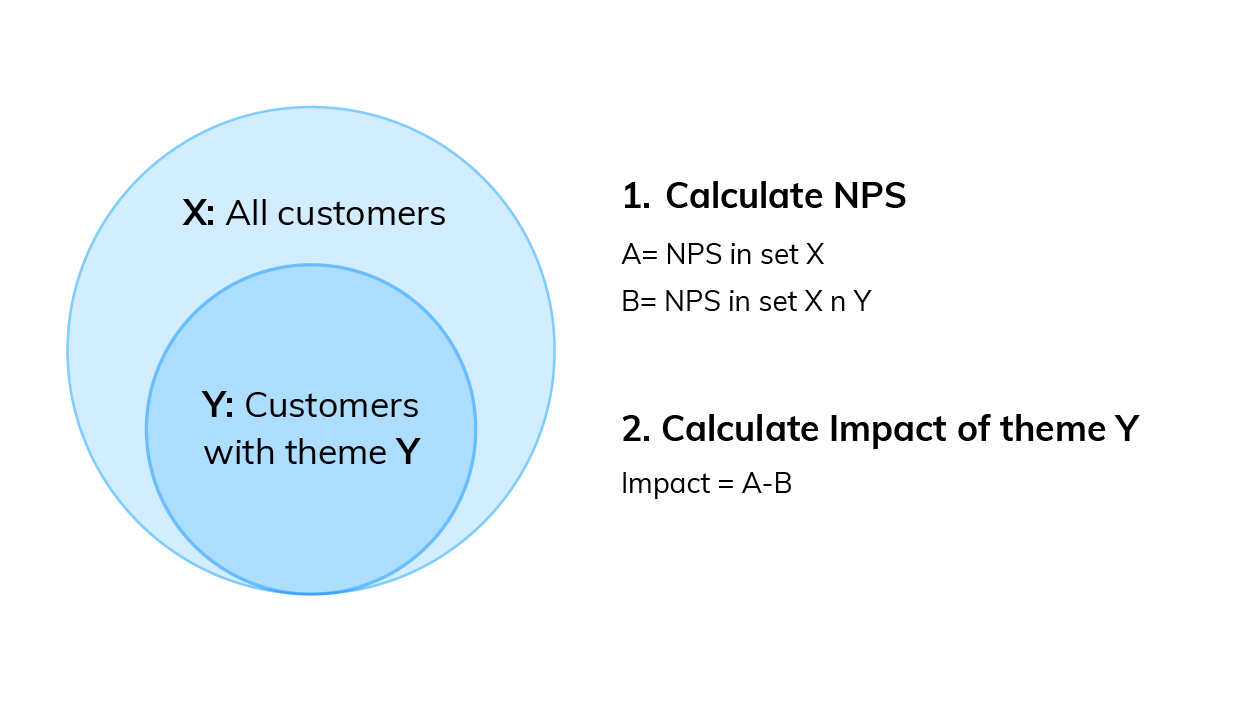
You can then visualize this data using a bar chart.
You can download our CX toolkit - it includes a template to recreate this.
Trends over time
This analysis can help you answer questions like: “Which codes are linked to decreases or increases in my score over time?”
We need to compare two sequences of numbers: NPS over time and code frequency over time . Using Excel, calculate the correlation between the two sequences, which can be either positive (the more codes the higher the NPS, see picture below), or negative (the more codes the lower the NPS).
Now you need to plot code frequency against the absolute value of code correlation with NPS. Here is the formula:
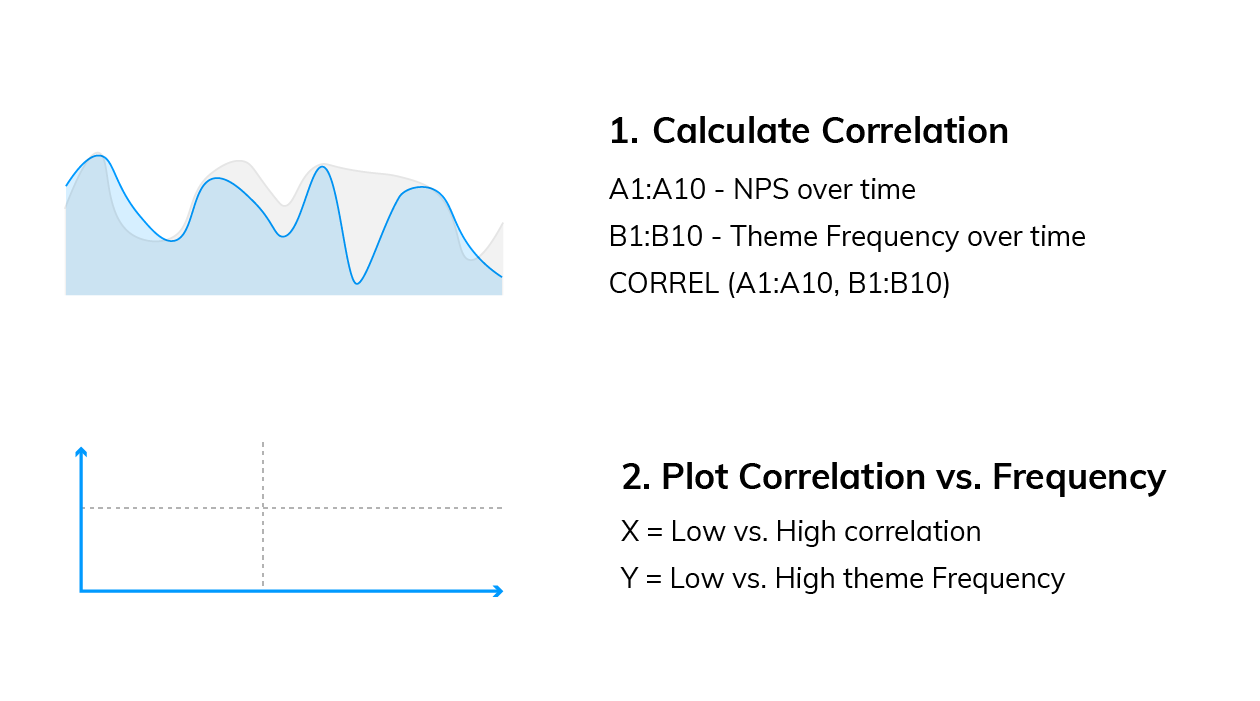
The visualization could look like this:
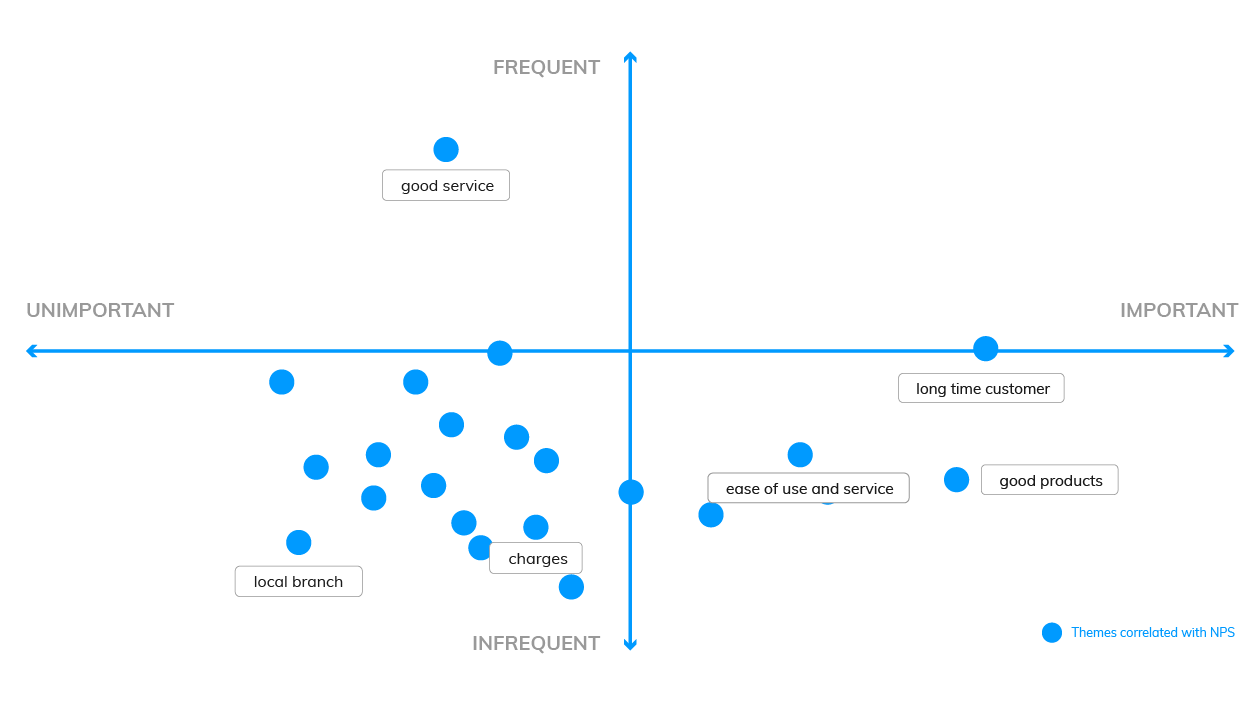
These are two examples, but there are more. For a third manual formula, and to learn why word clouds are not an insightful form of analysis, read our visualizations article .
Using a text analytics solution to automate analysis
Automated text analytics solutions enable codes and sub-codes to be pulled out of the data automatically. This makes it far faster and easier to identify what’s driving negative or positive results. And to pick up emerging trends and find all manner of rich insights in the data.
Another benefit of AI-driven text analytics software is its built-in capability for sentiment analysis, which provides the emotive context behind your feedback and other qualitative textual data therein.
Thematic provides text analytics that goes further by allowing users to apply their expertise on business context to edit or augment the AI-generated outputs.
Since the move away from manual research is generally about reducing the human element, adding human input to the technology might sound counter-intuitive. However, this is mostly to make sure important business nuances in the feedback aren’t missed during coding. The result is a higher accuracy of analysis. This is sometimes referred to as augmented intelligence .
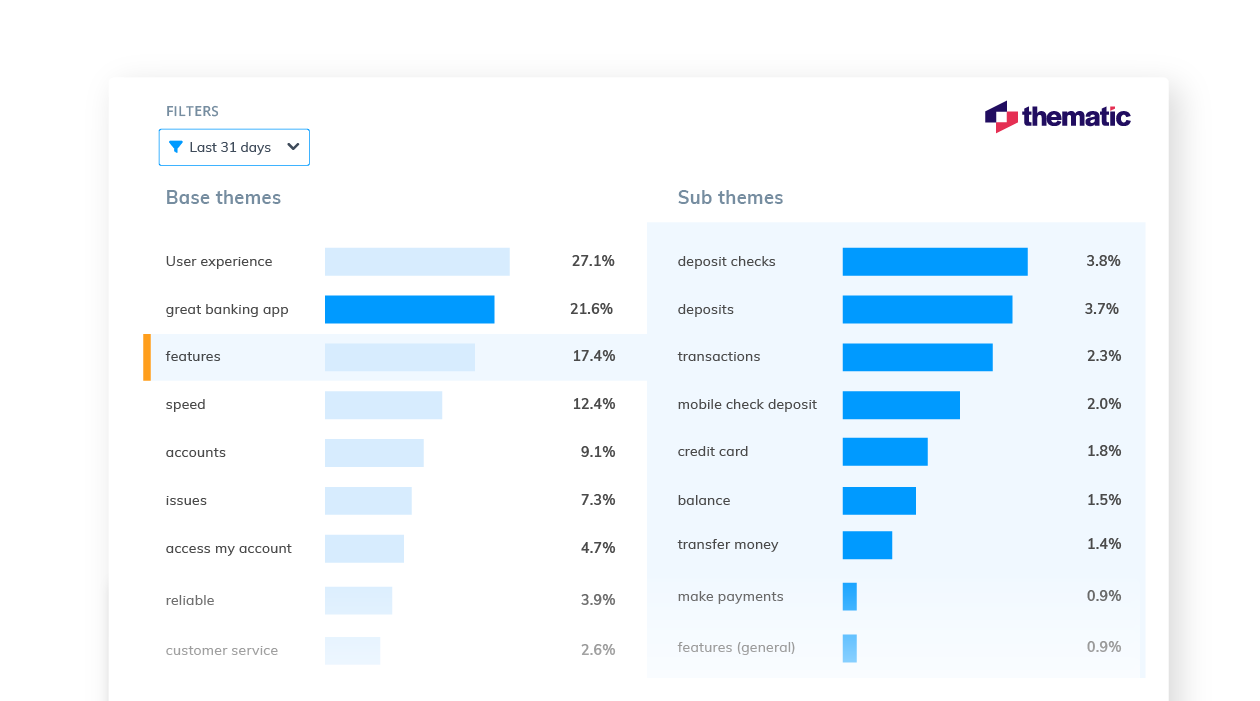
Step 5: Report on your data: Tell the story
The last step of analyzing your qualitative data is to report on it, to tell the story. At this point, the codes are fully developed and the focus is on communicating the narrative to the audience.
A coherent outline of the qualitative research, the findings and the insights is vital for stakeholders to discuss and debate before they can devise a meaningful course of action.
Creating graphs and reporting in Powerpoint
Typically, qualitative researchers take the tried and tested approach of distilling their report into a series of charts, tables and other visuals which are woven into a narrative for presentation in Powerpoint.
Using visualization software for reporting
With data transformation and APIs, the analyzed data can be shared with data visualisation software, such as Power BI or Tableau , Google Studio or Looker. Power BI and Tableau are among the most preferred options.
Visualizing your insights inside a feedback analytics platform
Feedback analytics platforms, like Thematic, incorporate visualisation tools that intuitively turn key data and insights into graphs. This removes the time consuming work of constructing charts to visually identify patterns and creates more time to focus on building a compelling narrative that highlights the insights, in bite-size chunks, for executive teams to review.
Using a feedback analytics platform with visualization tools means you don’t have to use a separate product for visualizations. You can export graphs into Powerpoints straight from the platforms.
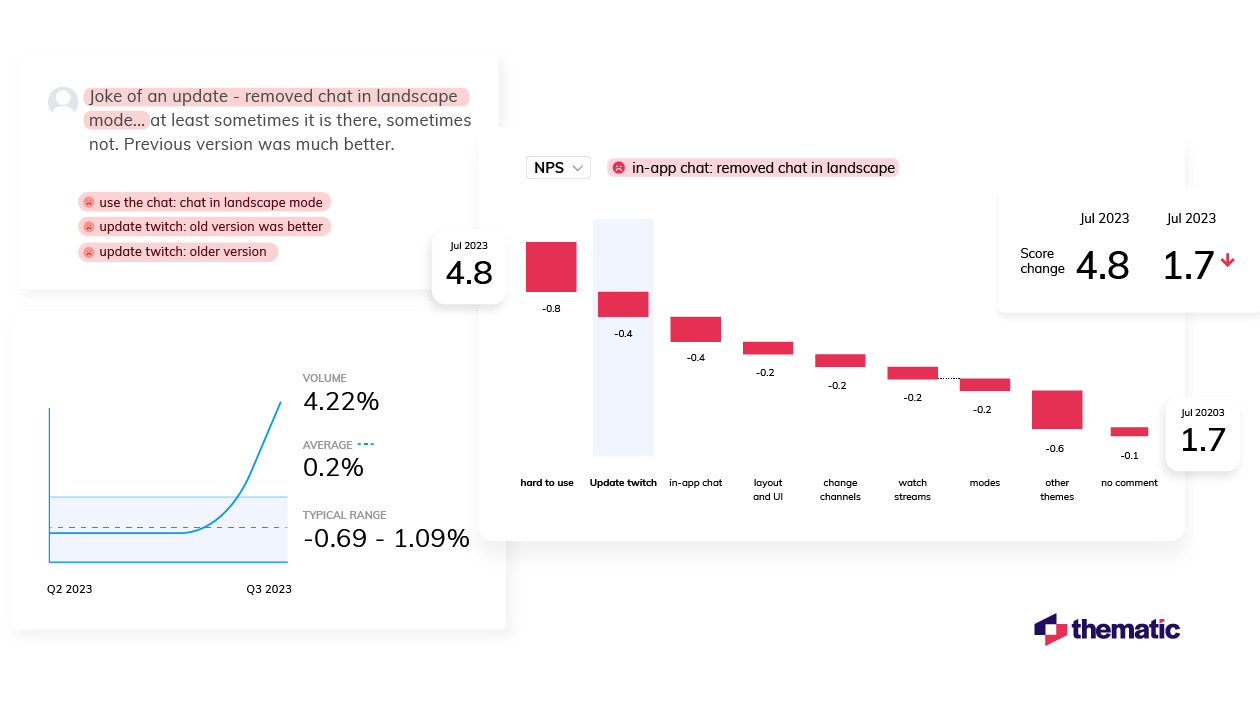
Conclusion - Manual or Automated?
There are those who remain deeply invested in the manual approach - because it’s familiar, because they’re reluctant to spend money and time learning new software, or because they’ve been burned by the overpromises of AI.
For projects that involve small datasets, manual analysis makes sense. For example, if the objective is simply to quantify a simple question like “Do customers prefer X concepts to Y?”. If the findings are being extracted from a small set of focus groups and interviews, sometimes it’s easier to just read them
However, as new generations come into the workplace, it’s technology-driven solutions that feel more comfortable and practical. And the merits are undeniable. Especially if the objective is to go deeper and understand the ‘why’ behind customers’ preference for X or Y. And even more especially if time and money are considerations.
The ability to collect a free flow of qualitative feedback data at the same time as the metric means AI can cost-effectively scan, crunch, score and analyze a ton of feedback from one system in one go. And time-intensive processes like focus groups, or coding, that used to take weeks, can now be completed in a matter of hours or days.
But aside from the ever-present business case to speed things up and keep costs down, there are also powerful research imperatives for automated analysis of qualitative data: namely, accuracy and consistency.
Finding insights hidden in feedback requires consistency, especially in coding. Not to mention catching all the ‘unknown unknowns’ that can skew research findings and steering clear of cognitive bias.
Some say without manual data analysis researchers won’t get an accurate “feel” for the insights. However, the larger data sets are, the harder it is to sort through the feedback and organize feedback that has been pulled from different places. And, the more difficult it is to stay on course, the greater the risk of drawing incorrect, or incomplete, conclusions grows.
Though the process steps for qualitative data analysis have remained pretty much unchanged since psychologist Paul Felix Lazarsfeld paved the path a hundred years ago, the impact digital technology has had on types of qualitative feedback data and the approach to the analysis are profound.
If you want to try an automated feedback analysis solution on your own qualitative data, you can get started with Thematic .
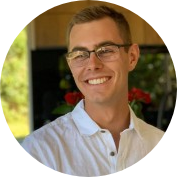
Community & Marketing
Tyler manages our community of CX, insights & analytics professionals. Tyler's goal is to help unite insights professionals around common challenges.
We make it easy to discover the customer and product issues that matter.
Unlock the value of feedback at scale, in one platform. Try it for free now!
- Questions to ask your Feedback Analytics vendor
- How to end customer churn for good
- Scalable analysis of NPS verbatims
- 5 Text analytics approaches
- How to calculate the ROI of CX
Our experts will show you how Thematic works, how to discover pain points and track the ROI of decisions. To access your free trial, book a personal demo today.
Recent posts
When two major storms wreaked havoc on Auckland and Watercare’s infrastructurem the utility went through a CX crisis. With a massive influx of calls to their support center, Thematic helped them get inisghts from this data to forge a new approach to restore services and satisfaction levels.
Become a qualitative theming pro! Creating a perfect code frame is hard, but thematic analysis software makes the process much easier.
Qualtrics is one of the most well-known and powerful Customer Feedback Management platforms. But even so, it has limitations. We recently hosted a live panel where data analysts from two well-known brands shared their experiences with Qualtrics, and how they extended this platform’s capabilities. Below, we’ll share the

Research Writing and Analysis
- NVivo Group and Study Sessions
- SPSS This link opens in a new window
- Statistical Analysis Group sessions
- Using Qualtrics
- Dissertation and Data Analysis Group Sessions
- Defense Schedule - Commons Calendar This link opens in a new window
- Research Process Flow Chart
- Research Alignment Chapter 1 This link opens in a new window
- Step 1: Seek Out Evidence
- Step 2: Explain
- Step 3: The Big Picture
- Step 4: Own It
- Step 5: Illustrate
- Annotated Bibliography
- Literature Review This link opens in a new window
- Systematic Reviews & Meta-Analyses
- How to Synthesize and Analyze
- Synthesis and Analysis Practice
- Synthesis and Analysis Group Sessions
- Problem Statement
- Purpose Statement
- Conceptual Framework
- Theoretical Framework
- Locating Theoretical and Conceptual Frameworks This link opens in a new window
- Quantitative Research Questions
- Qualitative Research Questions
- Trustworthiness of Qualitative Data
- Analysis and Coding Example- Qualitative Data
- Thematic Data Analysis in Qualitative Design
- Dissertation to Journal Article This link opens in a new window
- International Journal of Online Graduate Education (IJOGE) This link opens in a new window
- Journal of Research in Innovative Teaching & Learning (JRIT&L) This link opens in a new window
Writing a Case Study

What is a case study?

A Case study is:
- An in-depth research design that primarily uses a qualitative methodology but sometimes includes quantitative methodology.
- Used to examine an identifiable problem confirmed through research.
- Used to investigate an individual, group of people, organization, or event.
- Used to mostly answer "how" and "why" questions.
What are the different types of case studies?

Note: These are the primary case studies. As you continue to research and learn
about case studies you will begin to find a robust list of different types.
Who are your case study participants?

What is triangulation ?
Validity and credibility are an essential part of the case study. Therefore, the researcher should include triangulation to ensure trustworthiness while accurately reflecting what the researcher seeks to investigate.

How to write a Case Study?
When developing a case study, there are different ways you could present the information, but remember to include the five parts for your case study.

Was this resource helpful?
- << Previous: Thematic Data Analysis in Qualitative Design
- Next: Journal Article Reporting Standards (JARS) >>
- Last Updated: May 29, 2024 8:05 AM
- URL: https://resources.nu.edu/researchtools


An official website of the United States government
The .gov means it’s official. Federal government websites often end in .gov or .mil. Before sharing sensitive information, make sure you’re on a federal government site.
The site is secure. The https:// ensures that you are connecting to the official website and that any information you provide is encrypted and transmitted securely.
- Publications
- Account settings
Preview improvements coming to the PMC website in October 2024. Learn More or Try it out now .
- Advanced Search
- Journal List
- HHS Author Manuscripts

Qualitative Secondary Analysis: A Case Exemplar
Judith ann tate.
The Ohio State University, College of Nursing
Mary Beth Happ
Qualitative secondary analysis (QSA) is the use of qualitative data collected by someone else or to answer a different research question. Secondary analysis of qualitative data provides an opportunity to maximize data utility particularly with difficult to reach patient populations. However, QSA methods require careful consideration and explicit description to best understand, contextualize, and evaluate the research results. In this paper, we describe methodologic considerations using a case exemplar to illustrate challenges specific to QSA and strategies to overcome them.
Health care research requires significant time and resources. Secondary analysis of existing data provides an efficient alternative to collecting data from new groups or the same subjects. Secondary analysis, defined as the reuse of existing data to investigate a different research question ( Heaton, 2004 ), has a similar purpose whether the data are quantitative or qualitative. Common goals include to (1) perform additional analyses on the original dataset, (2) analyze a subset of the original data, (3) apply a new perspective or focus to the original data, or (4) validate or expand findings from the original analysis ( Hinds, Vogel, & Clarke-Steffen, 1997 ). Synthesis of knowledge from meta-analysis or aggregation may be viewed as an additional purpose of secondary analysis ( Heaton, 2004 ).
Qualitative studies utilize several different data sources, such as interviews, observations, field notes, archival meeting minutes or clinical record notes, to produce rich descriptions of human experiences within a social context. The work typically requires significant resources (e.g., personnel effort/time) for data collection and analysis. When feasible, qualitative secondary analysis (QSA) can be a useful and cost-effective alternative to designing and conducting redundant primary studies. With advances in computerized data storage and analysis programs, sharing qualitative datasets has become easier. However, little guidance is available for conducting, structuring procedures, or evaluating QSA ( Szabo & Strang, 1997 ).
QSA has been described as “an almost invisible enterprise in social research” ( Fielding, 2004 ). Primary data is often re-used; however, descriptions of this practice are embedded within the methods section of qualitative research reports rather than explicitly identified as QSA. Moreover, searching or classifying reports as QSA is difficult because many researchers refrain from identifying their work as secondary analyses ( Hinds et al., 1997 ; Thorne, 1998a ). In this paper, we provide an overview of QSA, the purposes, and modes of data sharing and approaches. A unique, expanded QSA approach is presented as a methodological exemplar to illustrate considerations.
QSA Typology
Heaton (2004) classified QSA studies based on the relationship between the secondary and primary questions and the scope of data analyzed. Types of QSA included studies that (1) investigated questions different from the primary study, (2) applied a unique theoretical perspective, or (3) extended the primary work. Heaton’s literature review (2004) showed that studies varied in the choice of data used, from selected portions to entire or combined datasets.
Modes of Data Sharing
Heaton (2004) identified three modes of data sharing: formal, informal and auto-data. Formal data sharing involves accessing and analyzing deposited or archived qualitative data by an independent group of researchers. Historical research often uses formal data sharing. Informal data sharing refers to requests for direct access to an investigator’s data for use alone or to pool with other data, usually as a result of informal networking. In some instances, the primary researchers may be invited to collaborate. The most common mode of data sharing is auto-data, defined as further exploration of a qualitative data set by the primary research team. Due to the iterative nature of qualitative research, when using auto-data, it may be difficult to determine where the original study questions end and discrete, distinct analysis begins ( Heaton, 1998 ).
An Exemplar QSA
Below we describe a QSA exemplar conducted by the primary author of this paper (JT), a member of the original research team, who used a supplementary approach to examine concepts revealed but not fully investigated in the primary study. First, we describe an overview of the original study on which the QSA was based. Then, the exemplar QSA is presented to illustrate: (1) the use of auto-data when the new research questions are closely related to or extend the original study aims ( Table 1 ), (2) the collection of additional clinical record data to supplement the original dataset and (3) the performance of separate member checking in the form of expert review and opinion. Considerations and recommendations for use of QSA are reviewed with illustrations taken from the exemplar study ( Table 2 ). Finally, discussion of conclusions and implications is included to assist with planning and implementation of QSA studies.
Research question comparison
Application of the Exemplar Qualitative Secondary Analysis (QSA)
Aitken, L. M., Marshall, A. P., Elliott, R., & McKinley, S. (2009). Critical care nurses' decision making: sedation assessment and management in intensive care. Journal of Clinical Nursing, 18 (1), 36–45.
Morse, J., & Field, P. (1995). Qualitative research methods for health professionals. (2nd ed.). Thousand Oaks, CA: Sage Publishing.
Patel, R. P., Gambrell, M., Speroff, T.,…Strength, C. (2009). Delirium and sedation in the intensive care unit: Survey of behaviors and attitudes of 1384 healthcare professionals. Critical Care Medicine, 37 (3), 825–832.
Shehabi, Y., Botha, J. A., Boyle, M. S., Ernest, D., Freebairn, R. C., Jenkins, I. R., … Seppelt, I. M. (2008). Sedation and delirium in the intensive care unit: an Australian and New Zealand perspective. Anaesthesia & Intensive Care, 36 (4), 570–578.
Tanios, M. A., de Wit, M., Epstein, S. K., & Devlin, J. W. (2009). Perceived barriers to the use of sedation protocols and daily sedation interruption: a multidisciplinary survey. Journal of Critical Care, 24 (1), 66–73.
Weinert, C. R., & Calvin, A. D. (2007). Epidemiology of sedation and sedation adequacy for mechanically ventilated patients in a medical and surgical intensive care unit. Critical Care Medicine , 35(2), 393–401.
The Primary Study
Briefly, the original study was a micro-level ethnography designed to describe the processes of care and communication with patients weaning from prolonged mechanical ventilation (PMV) in a 28-bed Medical Intensive Care Unit ( Broyles, Colbert, Tate, & Happ, 2008 ; Happ, Swigart, Tate, Arnold, Sereika, & Hoffman, 2007 ; Happ et al, 2007 , 2010 ). Both the primary study and the QSA were approved by the Institutional Review Board at the University of Pittsburgh. Data were collected by two experienced investigators and a PhD student-research project coordinator. Data sources consisted of sustained field observations, interviews with patients, family members and clinicians, and clinical record review, including all narrative clinical documentation recorded by direct caregivers.
During iterative data collection and analysis in the original study, it became apparent that anxiety and agitation had an effect on the duration of ventilator weaning episodes, an observation that helped to formulate the questions for the QSA ( Tate, Dabbs, Hoffman, Milbrandt & Happ, 2012 ). Thus, the secondary topic was closely aligned as an important facet of the primary phenomenon. The close, natural relationship between the primary and QSA research questions is demonstrated in the side-by-side comparison in Table 1 . This QSA focused on new questions which extended the original study to recognition and management of anxiety or agitation, behaviors that often accompany mechanical ventilation and weaning but occur throughout the trajectory of critical illness and recovery.
Considerations when Undertaking QSA ( Table 2 )
Practical advantages.
A key practical advantage of QSA is maximizing use of existing data. Data collection efforts represent a significant percentage of the research budget in terms of cost and labor ( Coyer & Gallo, 2005 ). This is particularly important in view of the competition for research funding. Planning and implementing a qualitative study involves considerable time and expertise not only for data collecting (e.g., interviews, participant observation or focus group), but in establishing access, credibility and relationships ( Thorne, 1994 ) and in conducting the analysis. The cost of QSA is often seen as negligible since the outlay of resources for data collection is assumed by the original study. However, QSA incurs costs related to storage, researcher’s effort for review of existing data, analysis, and any further data collection that may be necessary.
Another advantage of QSA is access to data from an assembled cohort. In conducting original primary research, practical concerns arise when participants are difficult to locate or reluctant to divulge sensitive details to a researcher. In the case of vulnerable critically ill patients, participation in research may seem an unnecessary burden to family members who may be unwilling to provide proxy consent ( Fielding, 2004 ). QSA permits new questions to be asked of data collected previously from these vulnerable groups ( Rew, Koniak-Griffin, Lewis, Miles, & O'Sullivan, 2000 ), or from groups or events that occur with scarcity ( Thorne, 1994 ). Participants’ time and effort in the primary study therefore becomes more worthwhile. In fact, it is recommended that data already collected from existing studies of vulnerable populations or about sensitive topics be analyzed prior to engaging new participants. In this way, QSA becomes a cumulative rather than a repetitive process ( Fielding, 2004 ).
Data Adequacy and Congruency
Secondary researchers must determine that the primary data set meets the needs of the QSA. Data may be insufficient to answer a new question or the focus of the QSA may be so different as to render the pursuit of a QSA impossible ( Heaton, 1998 ). The underlying assumptions, sampling plan, research questions, and conceptual framework selected to answer the original study question may not fit the question posed during QSA ( Coyer & Gallo, 2005 ). The researchers of the primary study may have selectively sampled participants and analyzed the resulting data in a manner that produced a narrow or uneven scope of data ( Hinds et al., 1997 ). Thus, the data needed to fully answer questions posed by the QSA may be inadequately addressed in the primary study. A critical review of the existing dataset is an important first step in determining whether the primary data fits the secondary questions ( Hinds et al., 1997 ).
Passage of Time
The timing of the QSA is another important consideration. If the primary study and secondary study are performed sequentially, findings of the original study may influence the secondary study. On the other hand, studies performed concurrently offer the benefit of access to both the primary research team and participants member checking ( Hinds et al., 1997 ).
The passage of time since the primary study was conducted can also have a distinct effect on the usefulness of the primary dataset. Data may be outdated or contain a historical bias ( Coyer & Gallo, 2005 ). Since context changes over time, characteristics of the phenomena of interest may have changed. Analysis of older datasets may not illuminate the phenomena as they exist today.( Hinds et al., 1997 ) Even if participants could be re-contacted, their perspectives, memories and experiences change. The passage of time also has an affect on the relationship of the primary researchers to the data – so auto-data may be interpreted differently by the same researcher with the passage of time. Data are bound by time and history, therefore, may be a threat to internal validity unless a new investigator is able to account for these effects when interpreting data ( Rew et al., 2000 ).
Researcher stance/Context involvement
Issues related to context are a major source of criticism of QSA ( Gladstone, Volpe, & Boydell, 2007 ). One of the hallmarks of qualitative research is the relationship of the researcher to the participants. It can be argued that removing active contact with participants violates this premise. Tacit understandings developed in the field may be difficult or impossible to reconstruct ( Thorne, 1994 ). Qualitative fieldworkers often react and redirect the data collection based on a growing knowledge of the setting. The setting may change as a result of external or internal factors. Interpretation of researchers as participants in a unique time and social context may be impossible to re-construct even if the secondary researchers were members of the primary team ( Mauthner, Parry, & Milburn, 1998 ). Because the context in which the data were originally produced cannot be recovered, the ability of the researcher to react to the lived experience may be curtailed in QSA ( Gladstone et al., 2007 ). Researchers utilize a number of tactics to filter and prioritize what to include as data that may not be apparent in either the written or spoken records of those events ( Thorne, 1994 ). Reflexivity between the researcher, participants and setting is impossible to recreate when examining pre-existing data.
Relationship of QSA Researcher to Primary Study
The relationship of the QSA researcher to the primary study is an important consideration. When the QSA researcher is not part of the original study team, contractual arrangements detailing access to data, its format, access to the original team, and authorship are required ( Hinds et al., 1997 ). The QSA researcher should assess the condition of the data, documents including transcripts, memos and notes, and clarity and flow of interactions ( Hinds et al., 1997 ). An outline of the original study and data collection procedures should be critically reviewed ( Heaton, 1998 ). If the secondary researcher was not a member of the original study team, access to the original investigative team for the purpose of ongoing clarification is essential ( Hinds et al., 1997 ).
Membership on the original study team may, however, offer the secondary researcher little advantage depending on their role in the primary study. Some research team members may have had responsibility for only one type of data collection or data source. There may be differences in involvement with analysis of the primary data.
Informed Consent of Participants
Thorne (1998) questioned whether data collected for one study purpose can ethically be re-examined to answer another question without participants’ consent. Many institutional review boards permit consent forms to include language about the possibility of future use of existing data. While this mechanism is becoming routine and welcomed by researchers, concerns have been raised that a generic consent cannot possibly address all future secondary questions and may violate the principle of full informed consent ( Gladstone et al., 2007 ). Local variations in study approval practices by institutional review boards may influence the ability of researchers to conduct a QSA.
Rigor of QSA
The primary standards for evaluating rigor of qualitative studies are trustworthiness (logical relationship between the data and the analytic claims), fit (the context within which the findings are applicable), transferability (the overall generalizability of the claims) and auditabilty (the transparency of the procedural steps and the analytic moves processes) ( Lincoln & Guba, 1991 ). Thorne suggests that standard procedures for assuring rigor can be modified for QSA ( Thorne, 1994 ). For instance, the original researchers may be viewed as sources of confirmation while new informants, other related datasets and validation by clinical experts are sources of triangulation that may overcome the lack of access to primary subjects ( Heaton, 2004 ; Thorne, 1994 ).
Our observations, derived from the experience of posing a new question of existing qualitative data serves as a template for researchers considering QSA. Considerations regarding quality, availability and appropriateness of existing data are of primary importance. A realistic plan for collecting additional data to answer questions posed in QSA should consider burden and resources for data collection, analysis, storage and maintenance. Researchers should consider context as a potential limitation to new analyses. Finally, the cost of QSA should be fully evaluated prior to making a decision to pursue QSA.
Acknowledgments
This work was funded by the National Institute of Nursing Research (RO1-NR07973, M Happ PI) and a Clinical Practice Grant from the American Association of Critical Care Nurses (JA Tate, PI).
Publisher's Disclaimer: This is a PDF file of an unedited manuscript that has been accepted for publication. As a service to our customers we are providing this early version of the manuscript. The manuscript will undergo copyediting, typesetting, and review of the resulting proof before it is published in its final citable form. Please note that during the production process errors may be discovered which could affect the content, and all legal disclaimers that apply to the journal pertain.
Disclosure statement: Drs. Tate and Happ have no potential conflicts of interest to disclose that relate to the content of this manuscript and do not anticipate conflicts in the foreseeable future.
Contributor Information
Judith Ann Tate, The Ohio State University, College of Nursing.
Mary Beth Happ, The Ohio State University, College of Nursing.
- Broyles L, Colbert A, Tate J, Happ MB. Clinicians’ evaluation and management of mental health, substance abuse, and chronic pain conditions in the intensive care unit. Critical Care Medicine. 2008; 36 (1):87–93. [ PubMed ] [ Google Scholar ]
- Coyer SM, Gallo AM. Secondary analysis of data. Journal of Pediatric Health Care. 2005; 19 (1):60–63. [ PubMed ] [ Google Scholar ]
- Fielding N. Getting the most from archived qualitative data: Epistemological, practical and professional obstacles. International Journal of Social Research Methodology. 2004; 7 (1):97–104. [ Google Scholar ]
- Gladstone BM, Volpe T, Boydell KM. Issues encountered in a qualitative secondary analysis of help-seeking in the prodrome to psychosis. Journal of Behavioral Health Services & Research. 2007; 34 (4):431–442. [ PubMed ] [ Google Scholar ]
- Happ MB, Swigart VA, Tate JA, Arnold RM, Sereika SM, Hoffman LA. Family presence and surveillance during weaning from prolonged mechanical ventilation. Heart & Lung: The Journal of Acute and Critical Care. 2007; 36 (1):47–57. [ PMC free article ] [ PubMed ] [ Google Scholar ]
- Happ MB, Swigart VA, Tate JA, Hoffman LA, Arnold RM. Patient involvement in health-related decisions during prolonged critical illness. Research in Nursing & Health. 2007; 30 (4):361–72. [ PubMed ] [ Google Scholar ]
- Happ MB, Tate JA, Swigart V, DiVirgilio-Thomas D, Hoffman LA. Wash and wean: Bathing patients undergoing weaning trials during prolonged mechanical ventilation. Heart & Lung: The Journal of Acute and Critical Care. 2010; 39 (6 Suppl):S47–56. [ PMC free article ] [ PubMed ] [ Google Scholar ]
- Heaton J. Secondary analysis of qualitative data. Social Research Update. 1998;(22) [ Google Scholar ]
- Heaton J. Reworking Qualitative Data. London: SAGE Publications; 2004. [ Google Scholar ]
- Hinds PS, Vogel RJ, Clarke-Steffen L. The possibilities and pitfalls of doing a secondary analysis of a qualitative data set. Qualitative Health Research. 1997; 7 (3):408–424. [ Google Scholar ]
- Lincoln YS, Guba EG. Naturalistic inquiry. Beverly Hills, CA: Sage Publishing; 1991. [ Google Scholar ]
- Mauthner N, Parry O, Milburn K. The data are out there, or are they? Implications for archiving and revisiting qualitative data. Sociology. 1998; 32 :733–745. [ Google Scholar ]
- Rew L, Koniak-Griffin D, Lewis MA, Miles M, O'Sullivan A. Secondary data analysis: new perspective for adolescent research. Nursing Outlook. 2000; 48 (5):223–229. [ PubMed ] [ Google Scholar ]
- Szabo V, Strang VR. Secondary analysis of qualitative data. Advances in Nursing Science. 1997; 20 (2):66–74. [ PubMed ] [ Google Scholar ]
- Tate JA, Dabbs AD, Hoffman LA, Milbrandt E, Happ MB. Anxiety and agitation in mechanically ventilated patients. Qualitative health research. 2012; 22 (2):157–173. [ PMC free article ] [ PubMed ] [ Google Scholar ]
- Thorne S. Secondary analysis in qualitative research: Issues and implications. In: Morse JM, editor. Critical Issues in Qualitative Research. Second. Thousand Oaks, CA: SAGE; 1994. [ Google Scholar ]
- Thorne S. Ethical and representational issues in qualitative secondary analysis. Qualitative Health Research. 1998; 8 (4):547–555. [ PubMed ] [ Google Scholar ]
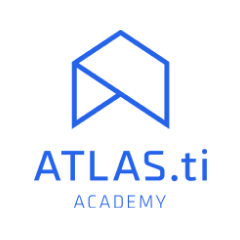
What Is a Research Design? | Definition, Types & Guide
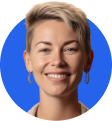
Introduction
Parts of a research design, types of research methodology in qualitative research, narrative research designs, phenomenological research designs, grounded theory research designs.
- Ethnographic research designs
Case study research design
Important reminders when designing a research study.
A research design in qualitative research is a critical framework that guides the methodological approach to studying complex social phenomena. Qualitative research designs determine how data is collected, analyzed, and interpreted, ensuring that the research captures participants' nuanced and subjective perspectives. Research designs also recognize ethical considerations and involve informed consent, ensuring confidentiality, and handling sensitive topics with the utmost respect and care. These considerations are crucial in qualitative research and other contexts where participants may share personal or sensitive information. A research design should convey coherence as it is essential for producing high-quality qualitative research, often following a recursive and evolving process.
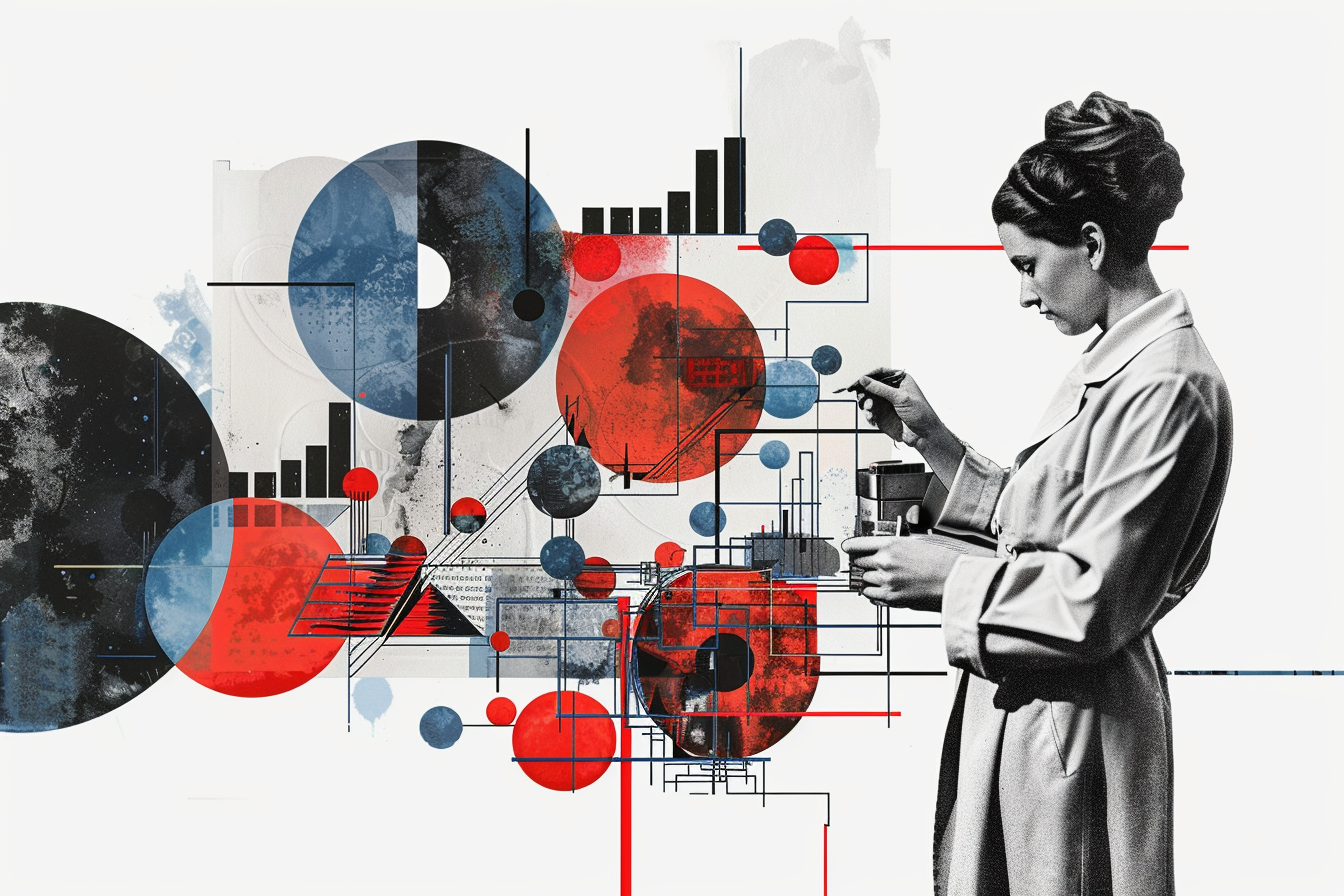
Theoretical concepts and research question
The first step in creating a research design is identifying the main theoretical concepts. To identify these concepts, a researcher should ask which theoretical keywords are implicit in the investigation. The next step is to develop a research question using these theoretical concepts. This can be done by identifying the relationship of interest among the concepts that catch the focus of the investigation. The question should address aspects of the topic that need more knowledge, shed light on new information, and specify which aspects should be prioritized before others. This step is essential in identifying which participants to include or which data collection methods to use. Research questions also put into practice the conceptual framework and make the initial theoretical concepts more explicit. Once the research question has been established, the main objectives of the research can be specified. For example, these objectives may involve identifying shared experiences around a phenomenon or evaluating perceptions of a new treatment.
Methodology
After identifying the theoretical concepts, research question, and objectives, the next step is to determine the methodology that will be implemented. This is the lifeline of a research design and should be coherent with the objectives and questions of the study. The methodology will determine how data is collected, analyzed, and presented. Popular qualitative research methodologies include case studies, ethnography , grounded theory , phenomenology, and narrative research . Each methodology is tailored to specific research questions and facilitates the collection of rich, detailed data. For example, a narrative approach may focus on only one individual and their story, while phenomenology seeks to understand participants' lived common experiences. Qualitative research designs differ significantly from quantitative research, which often involves experimental research, correlational designs, or variance analysis to test hypotheses about relationships between two variables, a dependent variable and an independent variable while controlling for confounding variables.
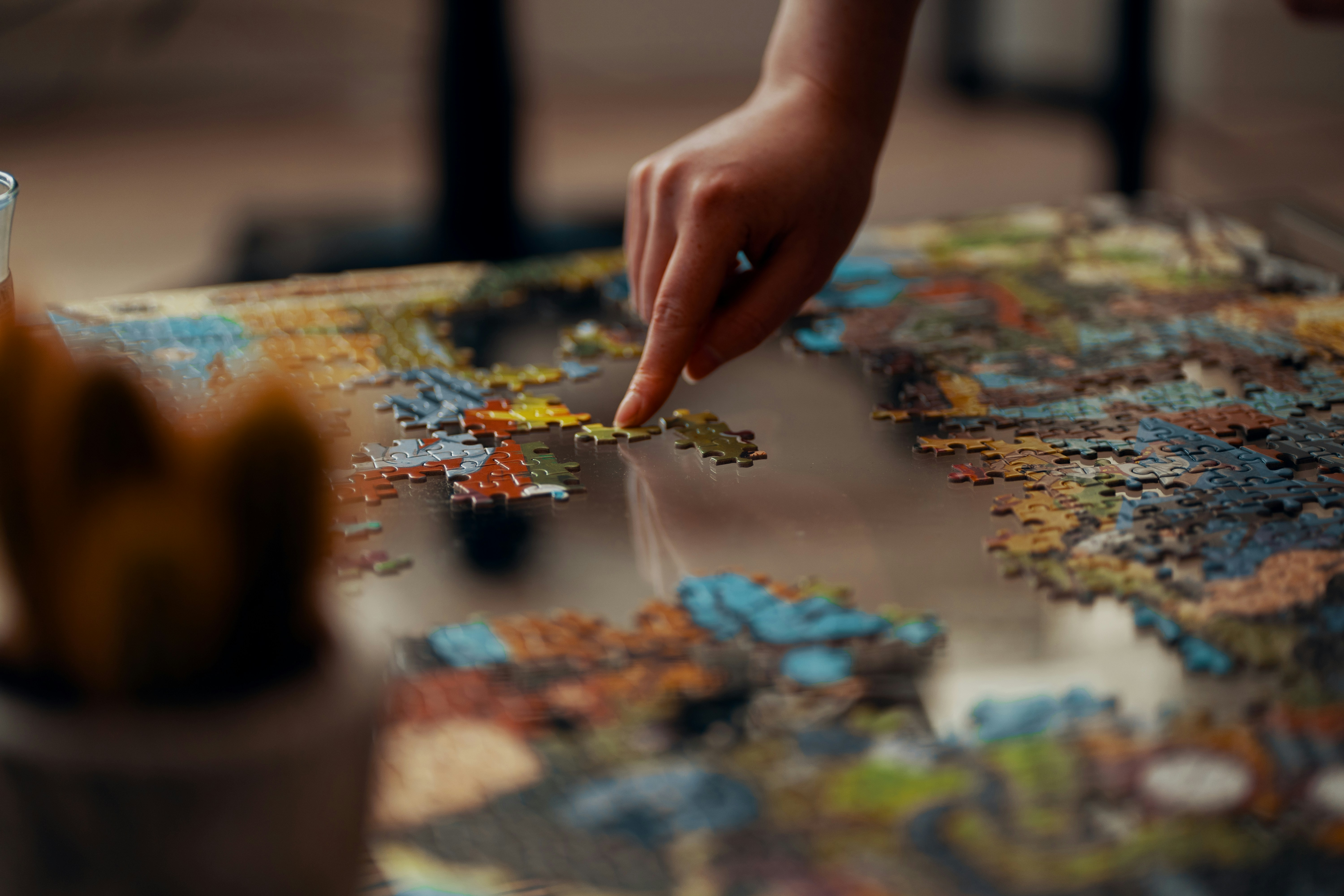
Literature review
After the methodology is identified, conducting a thorough literature review is integral to the research design. This review identifies gaps in knowledge, positioning the new study within the larger academic dialogue and underlining its contribution and relevance. Meta-analysis, a form of secondary research, can be particularly useful in synthesizing findings from multiple studies to provide a clear picture of the research landscape.
Data collection
The sampling method in qualitative research is designed to delve deeply into specific phenomena rather than to generalize findings across a broader population. The data collection methods—whether interviews, focus groups, observations, or document analysis—should align with the chosen methodology, ethical considerations, and other factors such as sample size. In some cases, repeated measures may be collected to observe changes over time.
Data analysis
Analysis in qualitative research typically involves methods such as coding and thematic analysis to distill patterns from the collected data. This process delineates how the research results will be systematically derived from the data. It is recommended that the researcher ensures that the final interpretations are coherent with the observations and analyses, making clear connections between the data and the conclusions drawn. Reporting should be narrative-rich, offering a comprehensive view of the context and findings.
Overall, a coherent qualitative research design that incorporates these elements facilitates a study that not only adds theoretical and practical value to the field but also adheres to high quality. This methodological thoroughness is essential for achieving significant, insightful findings. Examples of well-executed research designs can be valuable references for other researchers conducting qualitative or quantitative investigations. An effective research design is critical for producing robust and impactful research outcomes.
Each qualitative research design is unique, diverse, and meticulously tailored to answer specific research questions, meet distinct objectives, and explore the unique nature of the phenomenon under investigation. The methodology is the wider framework that a research design follows. Each methodology in a research design consists of methods, tools, or techniques that compile data and analyze it following a specific approach.
The methods enable researchers to collect data effectively across individuals, different groups, or observations, ensuring they are aligned with the research design. The following list includes the most commonly used methodologies employed in qualitative research designs, highlighting how they serve different purposes and utilize distinct methods to gather and analyze data.
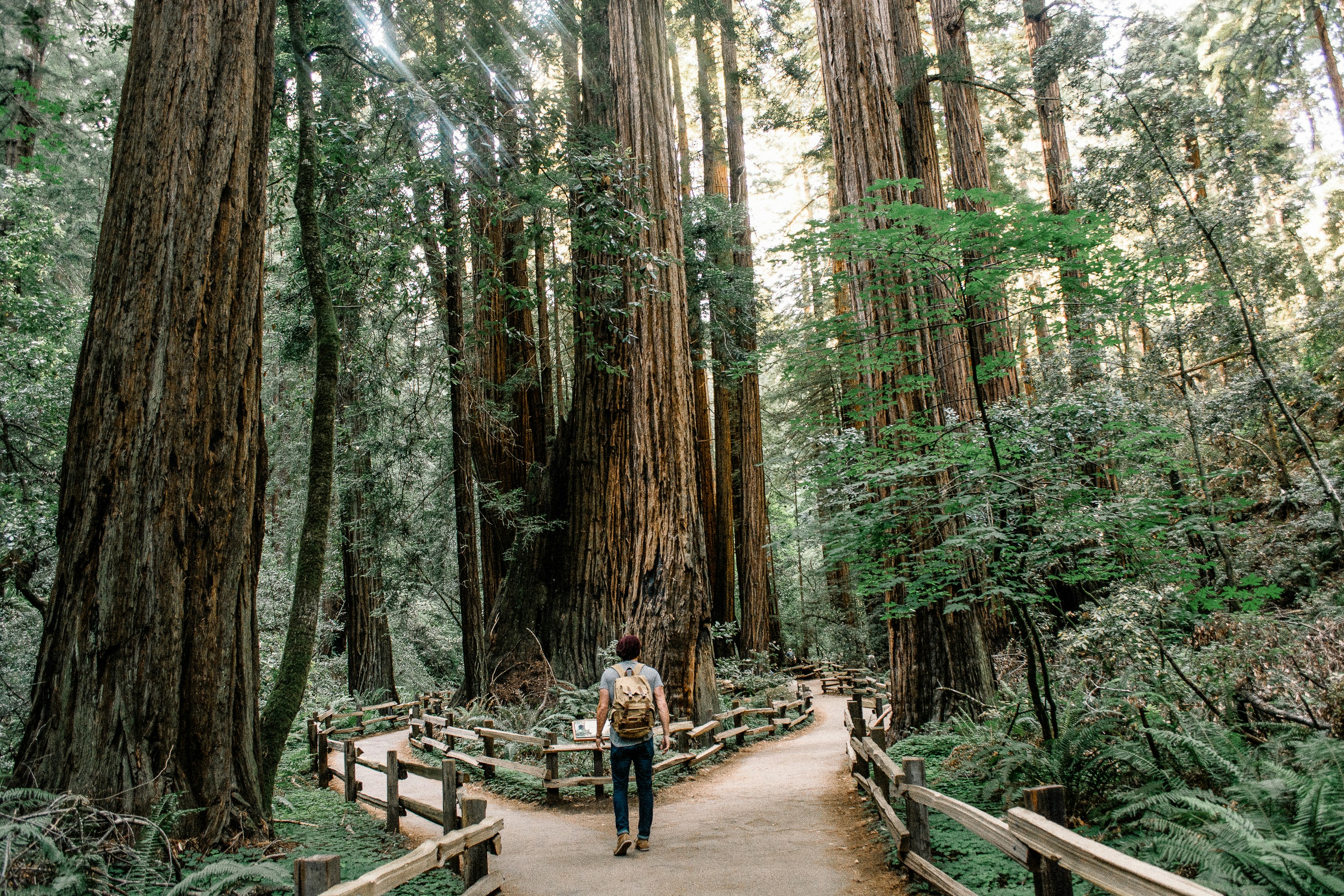
The narrative approach in research focuses on the collection and detailed examination of life stories, personal experiences, or narratives to gain insights into individuals' lives as told from their perspectives. It involves constructing a cohesive story out of the diverse experiences shared by participants, often using chronological accounts. It seeks to understand human experience and social phenomena through the form and content of the stories. These can include spontaneous narrations such as memoirs or diaries from participants or diaries solicited by the researcher. Narration helps construct the identity of an individual or a group and can rationalize, persuade, argue, entertain, confront, or make sense of an event or tragedy. To conduct a narrative investigation, it is recommended that researchers follow these steps:
Identify if the research question fits the narrative approach. Its methods are best employed when a researcher wants to learn about the lifestyle and life experience of a single participant or a small number of individuals.
Select the best-suited participants for the research design and spend time compiling their stories using different methods such as observations, diaries, interviewing their family members, or compiling related secondary sources.
Compile the information related to the stories. Narrative researchers collect data based on participants' stories concerning their personal experiences, for example about their workplace or homes, their racial or ethnic culture, and the historical context in which the stories occur.
Analyze the participant stories and "restore" them within a coherent framework. This involves collecting the stories, analyzing them based on key elements such as time, place, plot, and scene, and then rewriting them in a chronological sequence (Ollerenshaw & Creswell, 2000). The framework may also include elements such as a predicament, conflict, or struggle; a protagonist; and a sequence with implicit causality, where the predicament is somehow resolved (Carter, 1993).
Collaborate with participants by actively involving them in the research. Both the researcher and the participant negotiate the meaning of their stories, adding a credibility check to the analysis (Creswell & Miller, 2000).
A narrative investigation includes collecting a large amount of data from the participants and the researcher needs to understand the context of the individual's life. A keen eye is needed to collect particular stories that capture the individual experiences. Active collaboration with the participant is necessary, and researchers need to discuss and reflect on their own beliefs and backgrounds. Multiple questions could arise in the collection, analysis, and storytelling of individual stories that need to be addressed, such as: Whose story is it? Who can tell it? Who can change it? Which version is compelling? What happens when narratives compete? In a community, what do the stories do among them? (Pinnegar & Daynes, 2006).
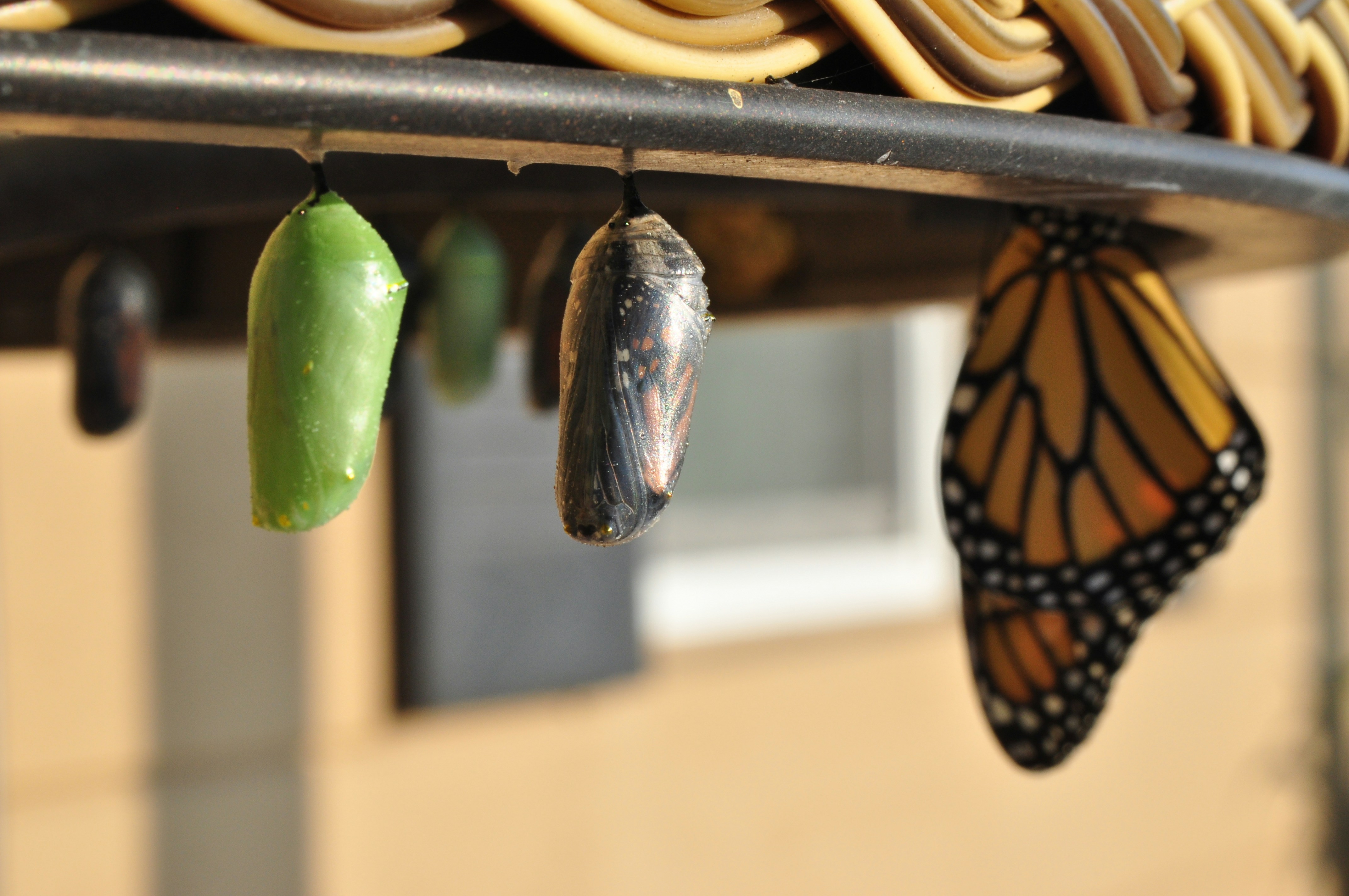
Make the most of your data with ATLAS.ti
Powerful tools in an intuitive interface, ready for you with a free trial today.
A research design based on phenomenology aims to understand the essence of the lived experiences of a group of people regarding a particular concept or phenomenon. Researchers gather deep insights from individuals who have experienced the phenomenon, striving to describe "what" they experienced and "how" they experienced it. This approach to a research design typically involves detailed interviews and aims to reach a deep existential understanding. The purpose is to reduce individual experiences to a description of the universal essence or understanding the phenomenon's nature (van Manen, 1990). In phenomenology, the following steps are usually followed:
Identify a phenomenon of interest . For example, the phenomenon might be anger, professionalism in the workplace, or what it means to be a fighter.
Recognize and specify the philosophical assumptions of phenomenology , for example, one could reflect on the nature of objective reality and individual experiences.
Collect data from individuals who have experienced the phenomenon . This typically involves conducting in-depth interviews, including multiple sessions with each participant. Additionally, other forms of data may be collected using several methods, such as observations, diaries, art, poetry, music, recorded conversations, written responses, or other secondary sources.
Ask participants two general questions that encompass the phenomenon and how the participant experienced it (Moustakas, 1994). For example, what have you experienced in this phenomenon? And what contexts or situations have typically influenced your experiences within the phenomenon? Other open-ended questions may also be asked, but these two questions particularly focus on collecting research data that will lead to a textural description and a structural description of the experiences, and ultimately provide an understanding of the common experiences of the participants.
Review data from the questions posed to participants . It is recommended that researchers review the answers and highlight "significant statements," phrases, or quotes that explain how participants experienced the phenomenon. The researcher can then develop meaningful clusters from these significant statements into patterns or key elements shared across participants.
Write a textual description of what the participants experienced based on the answers and themes of the two main questions. The answers are also used to write about the characteristics and describe the context that influenced the way the participants experienced the phenomenon, called imaginative variation or structural description. Researchers should also write about their own experiences and context or situations that influenced them.
Write a composite description from the structural and textural description that presents the "essence" of the phenomenon, called the essential and invariant structure.
A phenomenological approach to a research design includes the strict and careful selection of participants in the study where bracketing personal experiences can be difficult to implement. The researcher decides how and in which way their knowledge will be introduced. It also involves some understanding and identification of the broader philosophical assumptions.
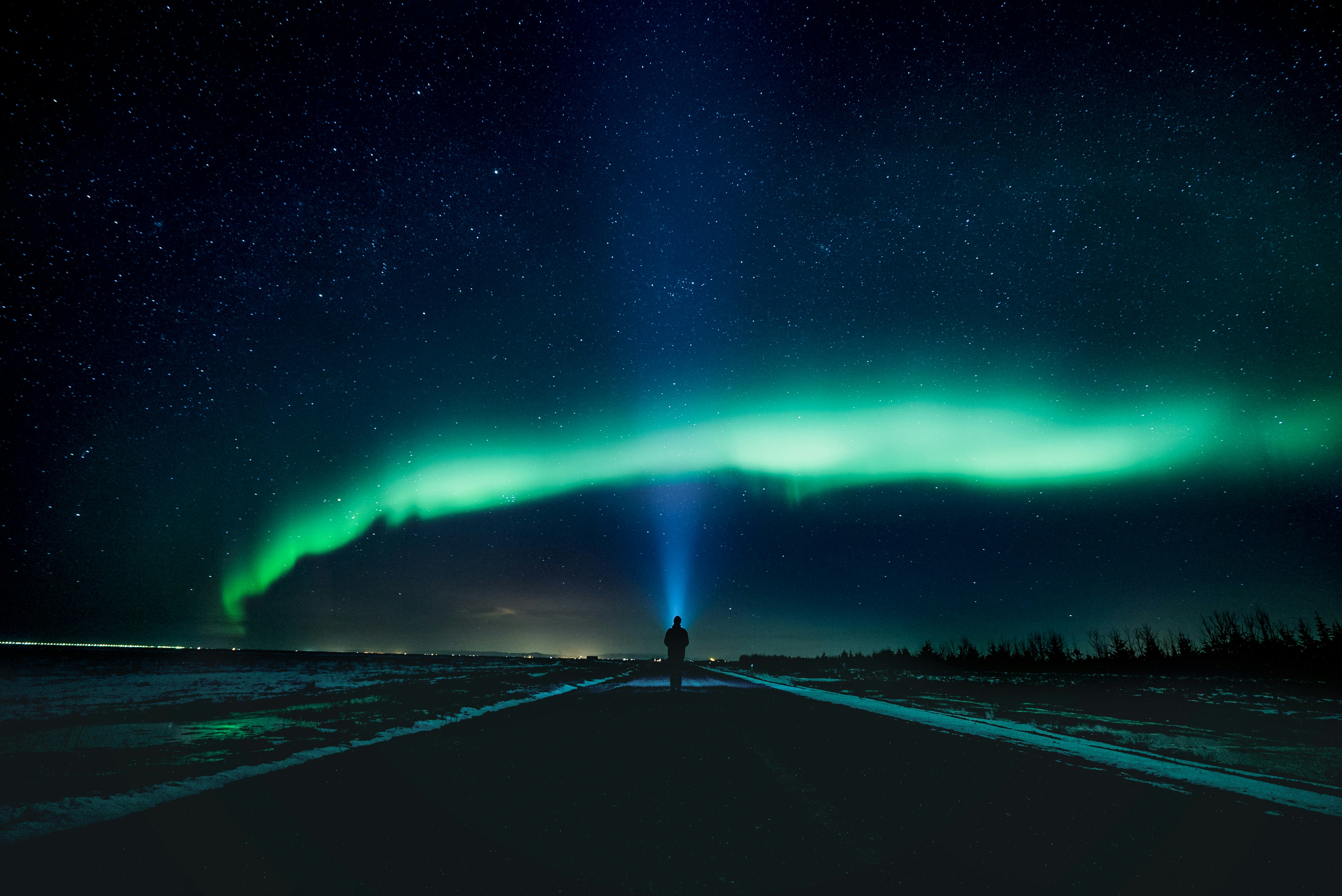
Grounded theory is used in a research design when the goal is to inductively develop a theory "grounded" in data that has been systematically gathered and analyzed. Starting from the data collection, researchers identify characteristics, patterns, themes, and relationships, gradually forming a theoretical framework that explains relevant processes, actions, or interactions grounded in the observed reality. A grounded theory study goes beyond descriptions and its objective is to generate a theory, an abstract analytical scheme of a process. Developing a theory doesn't come "out of nothing" but it is constructed and based on clear data collection. We suggest the following steps to follow a grounded theory approach in a research design:
Determine if grounded theory is the best for your research problem . Grounded theory is a good design when a theory is not already available to explain a process.
Develop questions that aim to understand how individuals experienced or enacted the process (e.g., What was the process? How did it unfold?). Data collection and analysis occur in tandem, so that researchers can ask more detailed questions that shape further analysis, such as: What was the focal point of the process (central phenomenon)? What influenced or caused this phenomenon to occur (causal conditions)? What strategies were employed during the process? What effect did it have (consequences)?
Gather relevant data about the topic in question . Data gathering involves questions that are usually asked in interviews, although other forms of data can also be collected, such as observations, documents, and audio-visual materials from different groups.
Carry out the analysis in stages . Grounded theory analysis begins with open coding, where the researcher forms codes that inductively emerge from the data (rather than preconceived categories). Researchers can thus identify specific properties and dimensions relevant to their research question.
Assemble the data in new ways and proceed to axial coding . Axial coding involves using a coding paradigm or logic diagram, such as a visual model, to systematically analyze the data. Begin by identifying a central phenomenon, which is the main category or focus of the research problem. Next, explore the causal conditions, which are the categories of factors that influence the phenomenon. Specify the strategies, which are the actions or interactions associated with the phenomenon. Then, identify the context and intervening conditions—both narrow and broad factors that affect the strategies. Finally, delineate the consequences, which are the outcomes or results of employing the strategies.
Use selective coding to construct a "storyline" that links the categories together. Alternatively, the researcher may formulate propositions or theory-driven questions that specify predicted relationships among these categories.
Develop and visually present a matrix that clarifies the social, historical, and economic conditions influencing the central phenomenon. This optional step encourages viewing the model from the narrowest to the broadest perspective.
Write a substantive-level theory that is closely related to a specific problem or population. This step is optional but provides a focused theoretical framework that can later be tested with quantitative data to explore its generalizability to a broader sample.
Allow theory to emerge through the memo-writing process, where ideas about the theory evolve continuously throughout the stages of open, axial, and selective coding.
The researcher should initially set aside any preconceived theoretical ideas to allow for the emergence of analytical and substantive theories. This is a systematic research approach, particularly when following the methodological steps outlined by Strauss and Corbin (1990). For those seeking more flexibility in their research process, the approach suggested by Charmaz (2006) might be preferable.
One of the challenges when using this method in a research design is determining when categories are sufficiently saturated and when the theory is detailed enough. To achieve saturation, discriminant sampling may be employed, where additional information is gathered from individuals similar to those initially interviewed to verify the applicability of the theory to these new participants. Ultimately, its goal is to develop a theory that comprehensively describes the central phenomenon, causal conditions, strategies, context, and consequences.
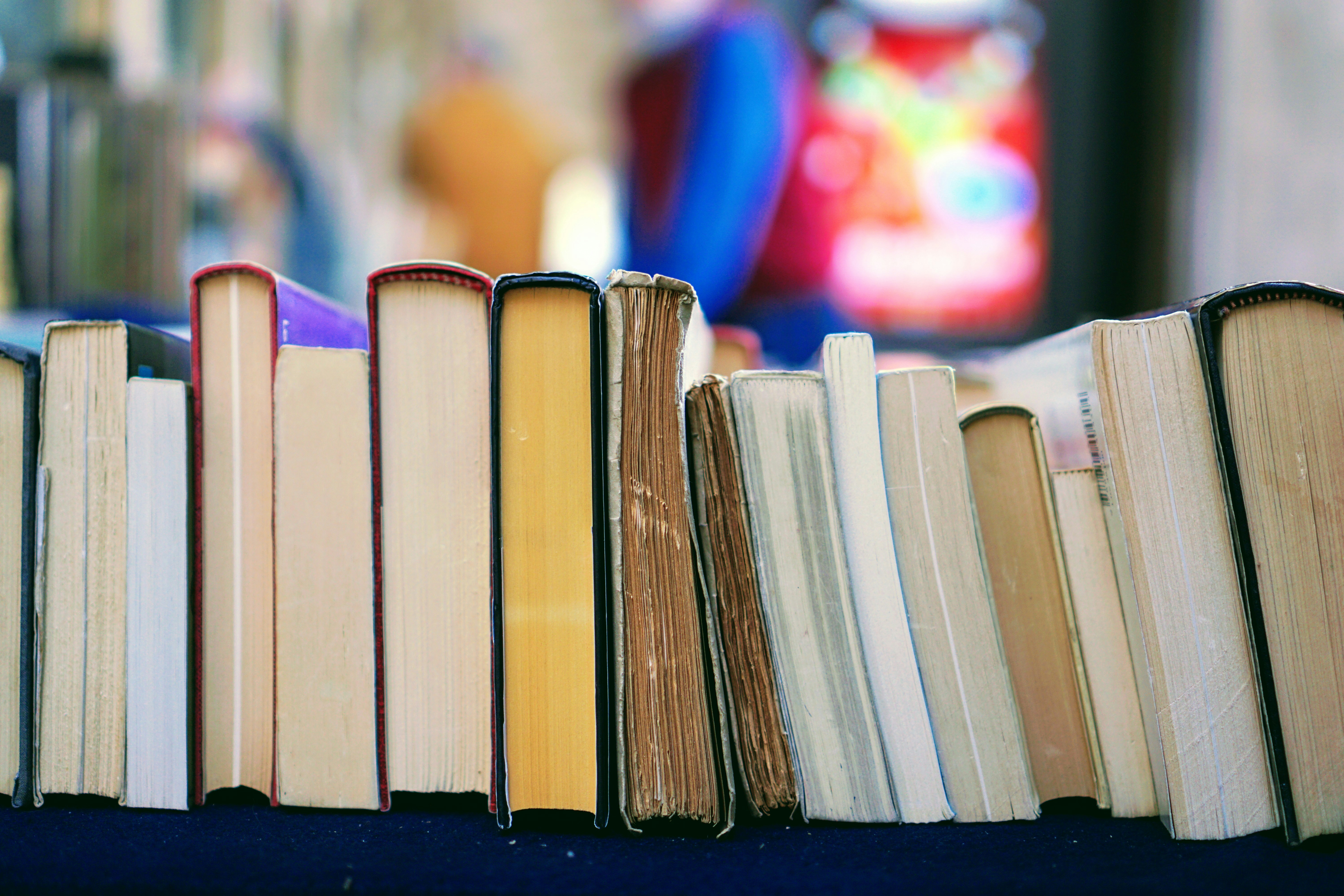
Ethnographic research design
An ethnographic approach in research design involves the extended observation and data collection of a group or community. The researcher immerses themselves in the setting, often living within the community for long periods. During this time, they collect data by observing and recording behaviours, conversations, and rituals to understand the group's social dynamics and cultural norms. We suggest following these steps for ethnographic methods in a research design:
Assess whether ethnography is the best approach for the research design and questions. It's suitable if the goal is to describe how a cultural group functions and to delve into their beliefs, language, behaviours, and issues like power, resistance, and domination, particularly if there is limited literature due to the group’s marginal status or unfamiliarity to mainstream society.
Identify and select a cultural group for your research design. Choose one that has a long history together, forming distinct languages, behaviours, and attitudes. This group often might be marginalized within society.
Choose cultural themes or issues to examine within the group. Analyze interactions in everyday settings to identify pervasive patterns such as life cycles, events, and overarching cultural themes. Culture is inferred from the group members' words, actions, and the tension between their actual and expected behaviours, as well as the artifacts they use.
Conduct fieldwork to gather detailed information about the group’s living and working environments. Visit the site, respect the daily lives of the members, and collect a diverse range of materials, considering ethical aspects such as respect and reciprocity.
Compile and analyze cultural data to develop a set of descriptive and thematic insights. Begin with a detailed description of the group based on observations of specific events or activities over time. Then, conduct a thematic analysis to identify patterns or themes that illustrate how the group functions and lives. The final output should be a comprehensive cultural portrait that integrates both the participants (emic) and the researcher’s (etic) perspectives, potentially advocating for the group’s needs or suggesting societal changes to better accommodate them.
Researchers engaging in ethnography need a solid understanding of cultural anthropology and the dynamics of sociocultural systems, which are commonly explored in ethnographic research. The data collection phase is notably extensive, requiring prolonged periods in the field. Ethnographers often employ a literary, quasi-narrative style in their narratives, which can pose challenges for those accustomed to more conventional social science writing methods.
Another potential issue is the risk of researchers "going native," where they become overly assimilated into the community under study, potentially jeopardizing the objectivity and completion of their research. It's crucial for researchers to be aware of their impact on the communities and environments they are studying.
The case study approach in a research design focuses on a detailed examination of a single case or a small number of cases. Cases can be individuals, groups, organizations, or events. Case studies are particularly useful for research designs that aim to understand complex issues in real-life contexts. The aim is to provide a thorough description and contextual analysis of the cases under investigation. We suggest following these steps in a case study design:
Assess if a case study approach suits your research questions . This approach works well when you have distinct cases with defined boundaries and aim to deeply understand these cases or compare multiple cases.
Choose your case or cases. These could involve individuals, groups, programs, events, or activities. Decide whether an individual or collective, multi-site or single-site case study is most appropriate, focusing on specific cases or themes (Stake, 1995; Yin, 2003).
Gather data extensively from diverse sources . Collect information through archival records, interviews, direct and participant observations, and physical artifacts (Yin, 2003).
Analyze the data holistically or in focused segments . Provide a comprehensive overview of the entire case or concentrate on specific aspects. Start with a detailed description including the history of the case and its chronological events then narrow down to key themes. The aim is to delve into the case's complexity rather than generalize findings.
Interpret and report the significance of the case in the final phase . Explain what insights were gained, whether about the subject of the case in an instrumental study or an unusual situation in an intrinsic study (Lincoln & Guba, 1985).
The investigator must carefully select the case or cases to study, recognizing that multiple potential cases could illustrate a chosen topic or issue. This selection process involves deciding whether to focus on a single case for deeper analysis or multiple cases, which may provide broader insights but less depth per case. Each choice requires a well-justified rationale for the selected cases. Researchers face the challenge of defining the boundaries of a case, such as its temporal scope and the events and processes involved. This decision in a research design is crucial as it affects the depth and value of the information presented in the study, and therefore should be planned to ensure a comprehensive portrayal of the case.
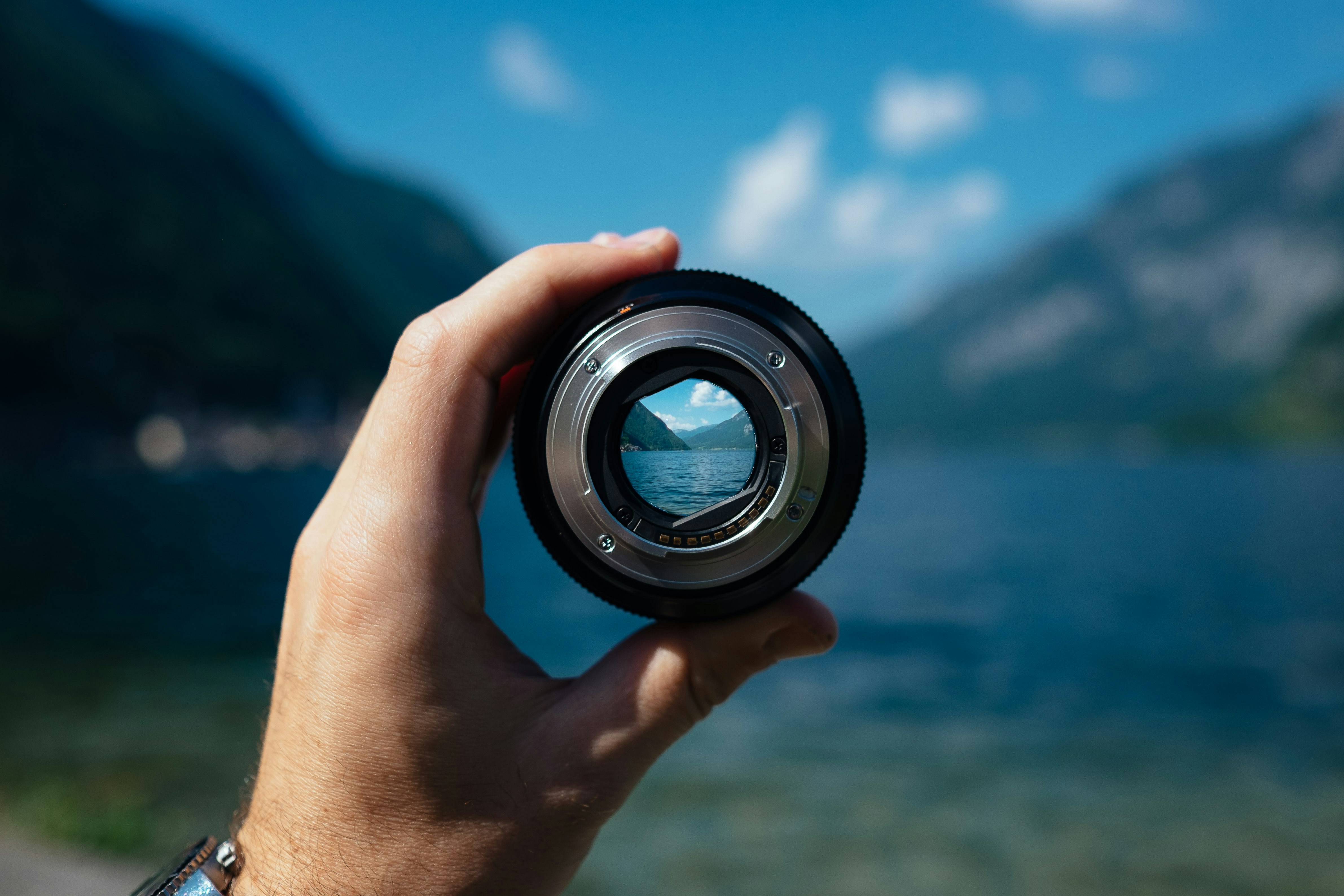
Qualitative and quantitative research designs are distinct in their approach to data collection and data analysis. Unlike quantitative research, which focuses on numerical data and statistical analysis, qualitative research prioritizes understanding the depth and richness of human experiences, behaviours, and interactions.
Qualitative methods in a research design have to have internal coherence, meaning that all elements of the research project—research question, data collection, data analysis, findings, and theory—are well-aligned and consistent with each other. This coherence in the research study is especially crucial in inductive qualitative research, where the research process often follows a recursive and evolving path. Ensuring that each component of the research design fits seamlessly with the others enhances the clarity and impact of the study, making the research findings more robust and compelling. Whether it is a descriptive research design, explanatory research design, diagnostic research design, or correlational research design coherence is an important element in both qualitative and quantitative research.
Finally, a good research design ensures that the research is conducted ethically and considers the well-being and rights of participants when managing collected data. The research design guides researchers in providing a clear rationale for their methodologies, which is crucial for justifying the research objectives to the scientific community. A thorough research design also contributes to the body of knowledge, enabling researchers to build upon past research studies and explore new dimensions within their fields. At the core of the design, there is a clear articulation of the research objectives. These objectives should be aligned with the underlying concepts being investigated, offering a concise method to answer the research questions and guiding the direction of the study with proper qualitative methods.
Carter, K. (1993). The place of a story in the study of teaching and teacher education. Educational Researcher, 22(1), 5-12, 18.
Charmaz, K. (2006). Constructing grounded theory. London: Sage.
Creswell, J. W., & Miller, D. L. (2000). Determining validity in qualitative inquiry. Theory Into Practice, 39(3), 124-130.
Lincoln, Y. S., & Guba, E. G. (1985). Naturalistic inquiry. Newbury Park, CA: Sage.
Moustakas, C. (1994). Phenomenological research methods. Thousand Oaks, CA: Sage.
Ollerenshaw, J. A., & Creswell, J. W. (2000, April). Data analysis in narrative research: A comparison of two “restoring” approaches. Paper presented at the annual meeting of the American Educational Research Association, New Orleans, LA.
Stake, R. E. (1995). The art of case study research. Thousand Oaks, CA: Sage.
Strauss, A., & Corbin, J. (1990). Basics of qualitative research: Grounded theory procedures and techniques. Newbury Park, CA: Sage.
van Manen, M. (1990). Researching lived experience: Human science for an action sensitive pedagogy. Ontario, Canada: University of Western Ontario.
Yin, R. K. (2003). Case study research: Design and methods (3rd ed.). Thousand Oaks, CA: Sage
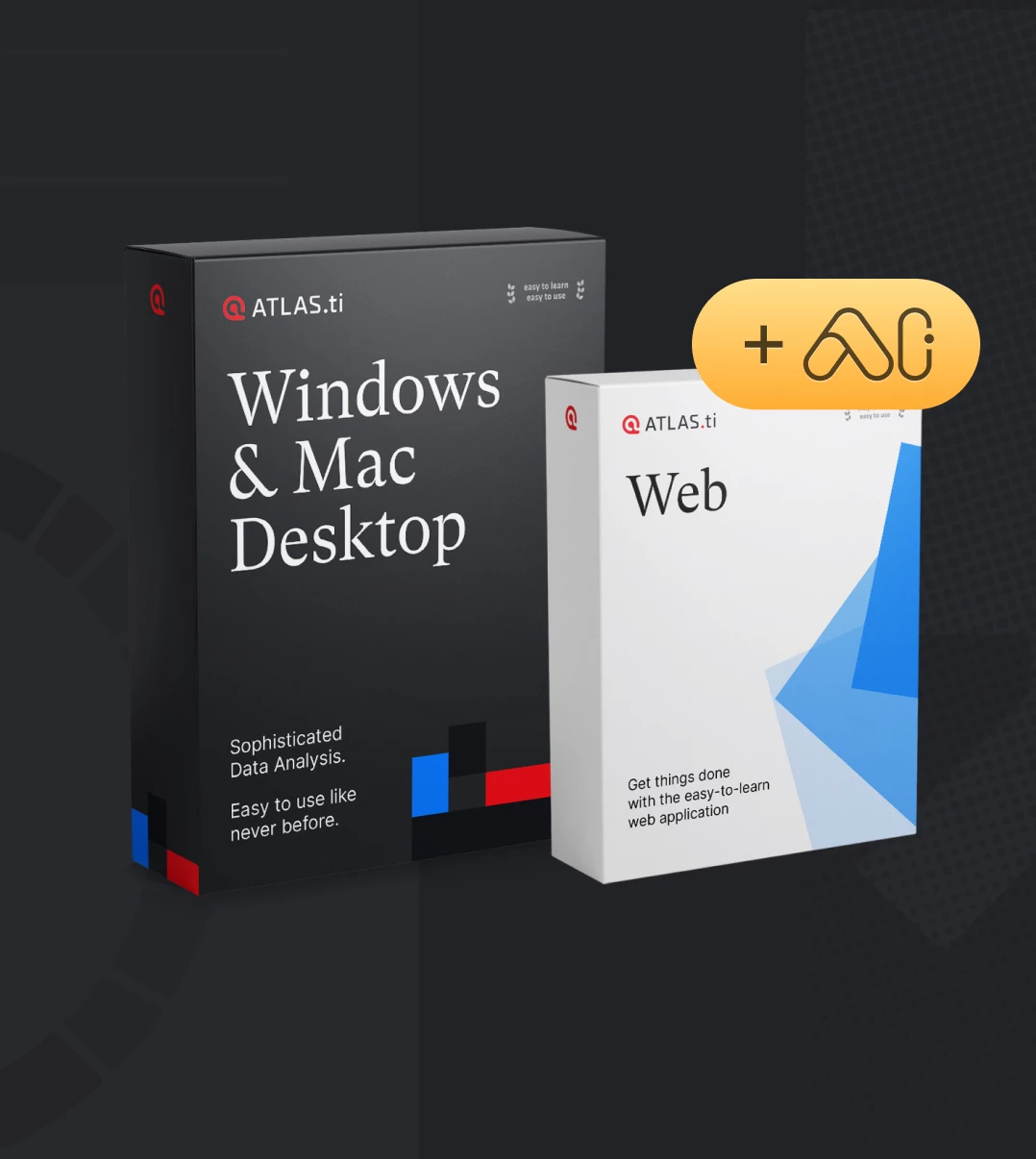
Whatever your research objectives, make it happen with ATLAS.ti!
Download a free trial today.
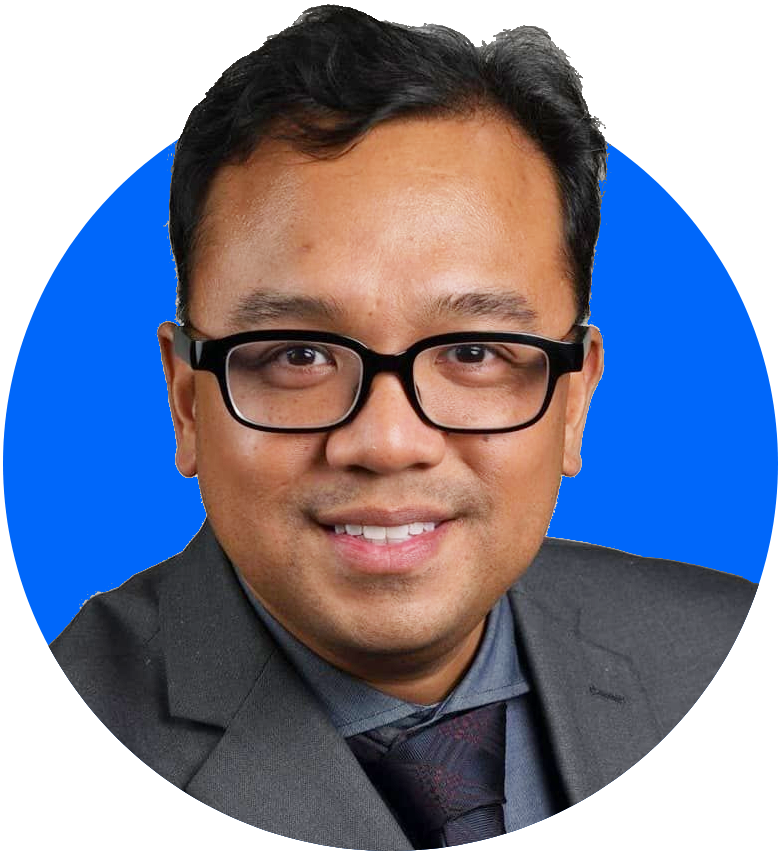
- Cancer Nursing Practice
- Emergency Nurse
- Evidence-Based Nursing
- Learning Disability Practice
- Mental Health Practice
- Nurse Researcher
- Nursing Children and Young People
- Nursing Management
- Nursing Older People
- Nursing Standard
- Primary Health Care
- RCN Nursing Awards
- Nursing Live
- Nursing Careers and Job Fairs
- CPD webinars on-demand
- --> Advanced -->

- Clinical articles
- Expert advice
- Career advice
- Revalidation
Data analysis Previous Next
Qualitative case study data analysis: an example from practice, catherine houghton lecturer, school of nursing and midwifery, national university of ireland, galway, republic of ireland, kathy murphy professor of nursing, national university of ireland, galway, ireland, david shaw lecturer, open university, milton keynes, uk, dympna casey senior lecturer, national university of ireland, galway, ireland.
Aim To illustrate an approach to data analysis in qualitative case study methodology.
Background There is often little detail in case study research about how data were analysed. However, it is important that comprehensive analysis procedures are used because there are often large sets of data from multiple sources of evidence. Furthermore, the ability to describe in detail how the analysis was conducted ensures rigour in reporting qualitative research.
Data sources The research example used is a multiple case study that explored the role of the clinical skills laboratory in preparing students for the real world of practice. Data analysis was conducted using a framework guided by the four stages of analysis outlined by Morse ( 1994 ): comprehending, synthesising, theorising and recontextualising. The specific strategies for analysis in these stages centred on the work of Miles and Huberman ( 1994 ), which has been successfully used in case study research. The data were managed using NVivo software.
Review methods Literature examining qualitative data analysis was reviewed and strategies illustrated by the case study example provided.
Discussion Each stage of the analysis framework is described with illustration from the research example for the purpose of highlighting the benefits of a systematic approach to handling large data sets from multiple sources.
Conclusion By providing an example of how each stage of the analysis was conducted, it is hoped that researchers will be able to consider the benefits of such an approach to their own case study analysis.
Implications for research/practice This paper illustrates specific strategies that can be employed when conducting data analysis in case study research and other qualitative research designs.
Nurse Researcher . 22, 5, 8-12. doi: 10.7748/nr.22.5.8.e1307
This article has been subject to double blind peer review
None declared
Received: 02 February 2014
Accepted: 16 April 2014
Case study data analysis - case study research methodology - clinical skills research - qualitative case study methodology - qualitative data analysis - qualitative research
User not found
Want to read more?
Already have access log in, 3-month trial offer for £5.25/month.
- Unlimited access to all 10 RCNi Journals
- RCNi Learning featuring over 175 modules to easily earn CPD time
- NMC-compliant RCNi Revalidation Portfolio to stay on track with your progress
- Personalised newsletters tailored to your interests
- A customisable dashboard with over 200 topics
Alternatively, you can purchase access to this article for the next seven days. Buy now
Are you a student? Our student subscription has content especially for you. Find out more
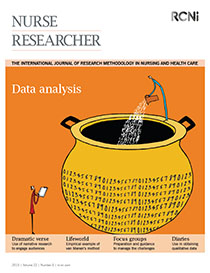
15 May 2015 / Vol 22 issue 5
TABLE OF CONTENTS
DIGITAL EDITION
- LATEST ISSUE
- SIGN UP FOR E-ALERT
- WRITE FOR US
- PERMISSIONS
Share article: Qualitative case study data analysis: an example from practice
We use cookies on this site to enhance your user experience.
By clicking any link on this page you are giving your consent for us to set cookies.
- Article Writing Affordable Article Writing Services
- Blog Writing Blogs that optimise your visibility
- Product Description Website that optimise your visibility
- Website Writing Website that optimise your visibility
- Proofreading Website that optimise your visibility
- Translation Website that optimise your visibility
- Agriculture Affordable Article Writing Services
- Health & Beauty Blogs that optimise your visibility
- Automotive Website that optimise your visibility
- Sports & fitness Website that optimise your visibility
- Real Estate Website that optimise your visibility
- Entertainment Website that optimise your visibility
- Blogs Affordable Article Writing Services
- Samples Blogs that optimise your visibility
- Case Study Website that optimise your visibility
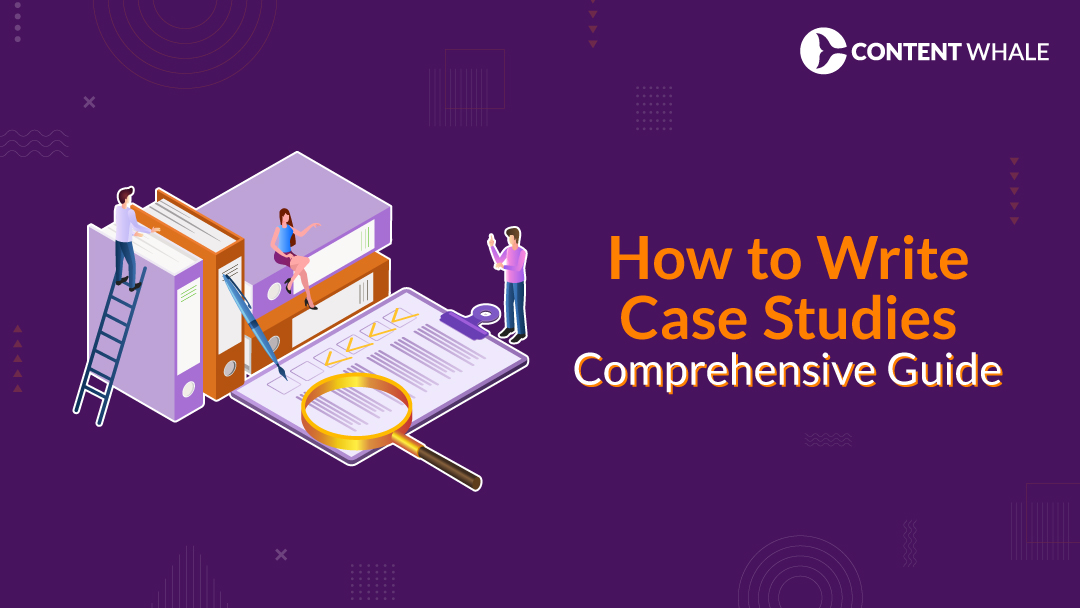
“How to Write Case Studies: A Comprehensive Guide”
Case studies are essential for marketing and research, offering in-depth insights into successes and problem-solving methods. This blog explains how to write case studies, including steps for creating them, tips for analysis, and case study examples. You'll also find case study templates to simplify the process. Effective case studies establish credibility, enhance marketing efforts, and provide valuable insights for future projects.
Case studies are detailed examinations of subjects like businesses, organizations, or individuals. They are used to highlight successes and problem-solving methods. They are crucial in marketing, education, and research to provide concrete examples and insights.
This blog will explain how to write case studies and their importance. We will cover different applications of case studies and a step-by-step process to create them. You’ll find tips for conducting case study analysis, along with case study examples and case study templates.
Effective case studies are vital. They showcase success stories and problem-solving skills, establishing credibility. This guide will teach you how to create a case study that engages your audience and enhances your marketing and research efforts.
What are Case Studies?
1. Definition and Purpose of a Case Study
Case studies are in-depth explorations of specific subjects to understand dynamics and outcomes. They provide detailed insights that can be generalized to broader contexts.
2. Different Types of Case Studies
- Exploratory: Investigates an area with limited information.
- Explanatory: Explains reasons behind a phenomenon.
- Descriptive: Provides a detailed account of the subject.
- Intrinsic : Focuses on a unique subject.
- Instrumental: Uses the case to understand a broader issue.
3. Benefits of Using Case Studies
Case studies offer many benefits. They provide real-world examples to illustrate theories or concepts. Businesses can demonstrate the effectiveness of their products or services. Researchers gain detailed insights into specific phenomena. Educators use them to teach through practical examples. Learning how to write case studies can enhance your marketing and research efforts.
Understanding how to create a case study involves recognizing these benefits. Case study examples show practical applications. Using case study templates can simplify the process.
5 Steps to Write a Case Study
1. Identifying the Subject or Case
Choose a subject that aligns with your objectives and offers valuable insights. Ensure the subject has a clear narrative and relevance to your audience. The subject should illustrate key points and provide substantial learning opportunities. Common subjects include successful projects, client stories, or significant business challenges.
2. Conducting Thorough Research and Data Collection
Gather comprehensive data from multiple sources. Conduct interviews with key stakeholders, such as clients, team members, or industry experts. Use surveys to collect quantitative data. Review documents, reports, and any relevant records. Ensure the information is accurate, relevant, and up-to-date. This thorough research forms the foundation for how to write case studies that are credible and informative.
3. Structuring the Case Study
Organize your case study into these sections:
- Introduction: Introduce the subject and its significance. Provide an overview of what will be covered.
- Background: Provide context and background information. Describe the subject’s history, environment, and any relevant details.
- Case Presentation: Detail the case, including the problem or challenge faced. Discuss the actions taken to address the issue.
- Analysis: Analyze the data and discuss the findings. Highlight key insights, patterns, and outcomes.
- Conclusion: Summarize the outcomes and key takeaways. Reflect on the broader implications and lessons learned.
4. Writing a Compelling Introduction
The introduction should grab the reader’s attention. Start with a hook, such as an interesting fact, quote, or question. Provide a brief overview of the subject and its importance. Explain why this case is relevant and worth studying. An engaging introduction sets the stage for how to create a case study that keeps readers interested.
5. Providing Background Information and Context
Give readers the necessary background to understand the case. Include details about the subject’s history, environment, and any relevant circumstances. Explain the context in which the case exists, such as the industry, market conditions, or organizational culture. Providing a solid foundation helps readers grasp the significance of the case and enhances the credibility of your study.
Understanding how to write a case study involves meticulous research and a clear structure. Utilizing case study examples and templates can guide you through the process, ensuring you present your findings effectively. These steps are essential for writing informative, engaging, and impactful case studies.
How to Write Case Study Analysis
1. Analyzing the Data Collected
Examine the data to identify patterns, trends, and key findings. Use qualitative and quantitative methods to ensure a comprehensive analysis. Validate the data’s accuracy and relevance to the subject. Look for correlations and causations that can provide deeper insights.
2. Identifying Key Issues and Problems
Pinpoint the main issues or challenges faced by the subject. Determine the root causes of these problems. Use tools like SWOT analysis (Strengths, Weaknesses, Opportunities, Threats) to get a clear picture. Prioritize the issues based on their impact and urgency.
3. Discussing Possible Solutions and Their Implementation
Explore various solutions that address the identified issues. Compare the potential effectiveness of each solution. Discuss the steps taken to implement the chosen solutions. Highlight the decision-making process and the rationale behind it. Include any obstacles faced during implementation and how they were overcome.
4. Evaluating the Results and Outcomes
Assess the outcomes of the implemented solutions. Use metrics and KPIs (Key Performance Indicators) to measure success. Compare the results with the initial objectives and expectations. Discuss any deviations and their reasons. Provide evidence to support your evaluation, such as before-and-after data or testimonials.
5. Providing Insights and Lessons Learned
Reflect on the insights gained from the case study. Discuss what worked well and what didn’t. Highlight lessons that can be applied to similar situations. Provide actionable recommendations for future projects. This section should offer valuable takeaways for the readers, helping them understand how to create a case study that is insightful and practical.
Mastering how to write case studies involves understanding each part of the analysis. Use case study examples to see how these elements are applied. Case study templates can help you structure your work. Knowing how to make a case study analysis will make your findings clear and actionable.
Case Study Examples and Templates
1. Showcasing Successful Case Studies
Georgia tech athletics increase season ticket sales by 80%.
Georgia Tech Athletics aimed to enhance their season ticket sales and engagement with fans. Their initial strategy involved multiple outbound phone calls without targeting. They partnered with Salesloft to improve their sales process with a more structured inbound approach. This allowed sales reps to target communications effectively. As a result, Georgia Tech saw an 80% increase in season ticket sales, with improved employee engagement and fan relationships.
WeightWatchers Revamps Enterprise Sales Process with HubSpot
WeightWatchers sought to improve their sales efficiency. Their previous system lacked automation, requiring extensive manual effort. By adopting HubSpot’s CRM, WeightWatchers streamlined their sales process. The automation capabilities of HubSpot allowed them to manage customer interactions more effectively. This transition significantly enhanced their operational efficiency and sales performance.
2. Breakdown of What Makes These Examples Effective
These case study examples are effective due to their clear structure and compelling storytelling. They:
- Identify the problem: Each case study begins by outlining the challenges faced by the client.
- Detail the solution: They explain the specific solutions implemented to address these challenges.
- Showcase the results: Quantifiable results and improvements are highlighted, demonstrating the effectiveness of the solutions.
- Use visuals and quotes: Incorporating images, charts, and client testimonials enhances engagement and credibility.
3. Providing Case Study Templates
To assist in creating your own case studies, here are some recommended case study templates:
1. General Case Study Template
- Suitable for various industries and applications.
- Includes sections for background, problem, solution, and results.
- Helps provide a structured narrative for any case study.
2. Data-Driven Case Study Template
- Focuses on presenting metrics and data.
- Ideal for showcasing quantitative achievements.
- Structured to highlight significant performance improvements and achievements.
3. Product-Specific Case Study Template
- Emphasizes customer experiences and satisfaction with a specific product.
- Highlights benefits and features of the product rather than the process.
4. Tips for Customizing Templates to Fit Your Needs
When using case study templates, tailor them to match the specific context of your study. Consider the following tips:
- Adapt the language and tone: Ensure it aligns with your brand voice and audience.
- Include relevant visuals: Add charts, graphs, and images to support your narrative.
- Personalize the content: Use specific details about the subject to make the case study unique and relatable.
Utilizing these examples and templates will guide you in how to write case studies effectively. They provide a clear framework for how to create a case study that is engaging and informative. Learning how to make a case study becomes more manageable with these resources and examples.
Tips for Creating Compelling Case Studies
1. Using Storytelling Techniques to Engage Readers
Incorporate storytelling techniques to make your case study engaging. A compelling narrative holds the reader’s attention.
2. Including Quotes and Testimonials from Participants
Add quotes and testimonials to add credibility. Participant feedback enhances the authenticity of your study.
3. Visual Aids: Charts, Graphs, and Images to Support Your Case
Use charts, graphs, and images to illustrate key points. Visual aids help in better understanding and retention.
4. Ensuring Clarity and Conciseness in Writing
Write clearly and concisely to maintain reader interest. Avoid jargon and ensure your writing is easy to follow.
5. Highlighting the Impact and Benefits
Emphasize the positive outcomes and benefits. Show how the subject has improved or achieved success.
Understanding how to write case studies involves using effective storytelling and visuals. Case study examples show how to engage readers, and case study templates help organize your content. Learning how to make a case study ensures that it is clear and impactful.

Benefits of Using Case Studies
1. Establishing Authority and Credibility
How to write case studies can effectively establish your authority. Showcasing success stories builds credibility in your field.
2. Demonstrating Practical Applications of Your Product or Service
Case study examples demonstrate how your product or service solves real-world problems. This practical evidence is convincing for potential clients.
3. Enhancing Marketing and Sales Efforts
Use case studies to support your marketing and sales strategies. They highlight your successes and attract new customers.
4. Providing Valuable Insights for Future Projects
Case studies offer insights that can guide future projects. Learning how to create a case study helps in applying these lessons effectively.
5. Engaging and Educating Your Audience
Case studies are engaging and educational. They provide detailed examples and valuable lessons. Using case study templates can make this process easier and more effective. Understanding how to make a case study ensures you can communicate these benefits clearly.
Writing effective case studies involves thorough research, clear structure, and engaging content. By following these steps, you’ll learn how to write case studies that showcase your success stories and problem-solving skills. Use the case study examples and case study templates provided to get started. Well-crafted case studies are valuable tools for marketing, research, and education. Start learning how to make a case study today and share your success stories with the world.
What is the purpose of a case study?
A case study provides detailed insights into a subject, illustrating successes and solutions. It helps in understanding complex issues.
How do I choose a subject for my case study?
Select a subject that aligns with your objectives and offers valuable insights. Ensure it has a clear narrative.
What are the key components of a case study analysis?
A case study analysis includes data collection, identifying key issues, discussing solutions, evaluating outcomes, and providing insights.
Where can I find case study templates?
You can find downloadable case study templates online. They simplify the process of creating a case study.
How can case studies benefit my business?
Case studies establish credibility, demonstrate practical applications, enhance marketing efforts, and provide insights for future projects. Learning how to create a case study can significantly benefit your business.
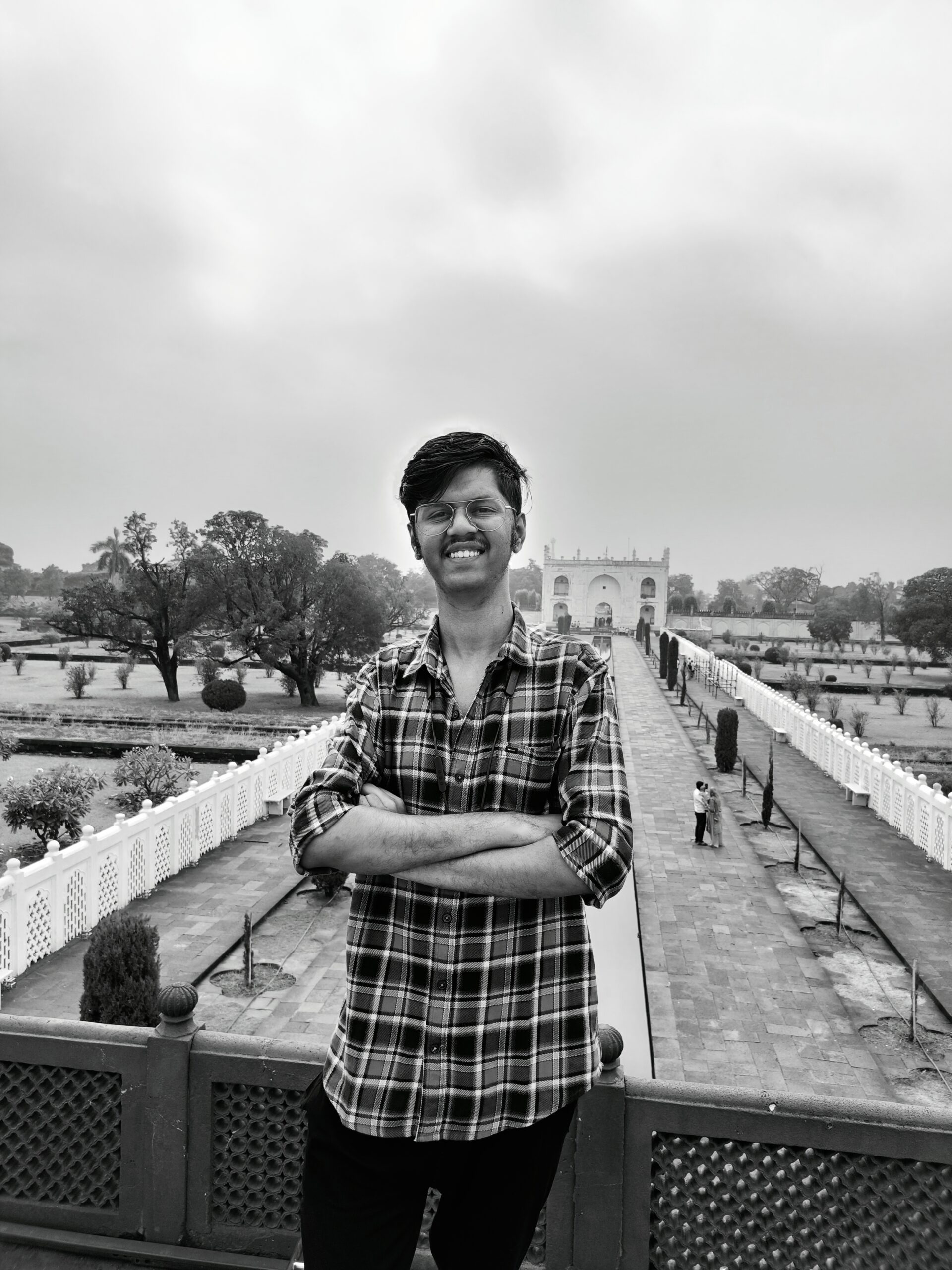
I am currently pursuing my Masters in Communication and Journalism from University of Mumbai. I am the author of four self published books. I am interested inv writing for films and TV. I run a blog where I write about film reviews.
More details for blogs
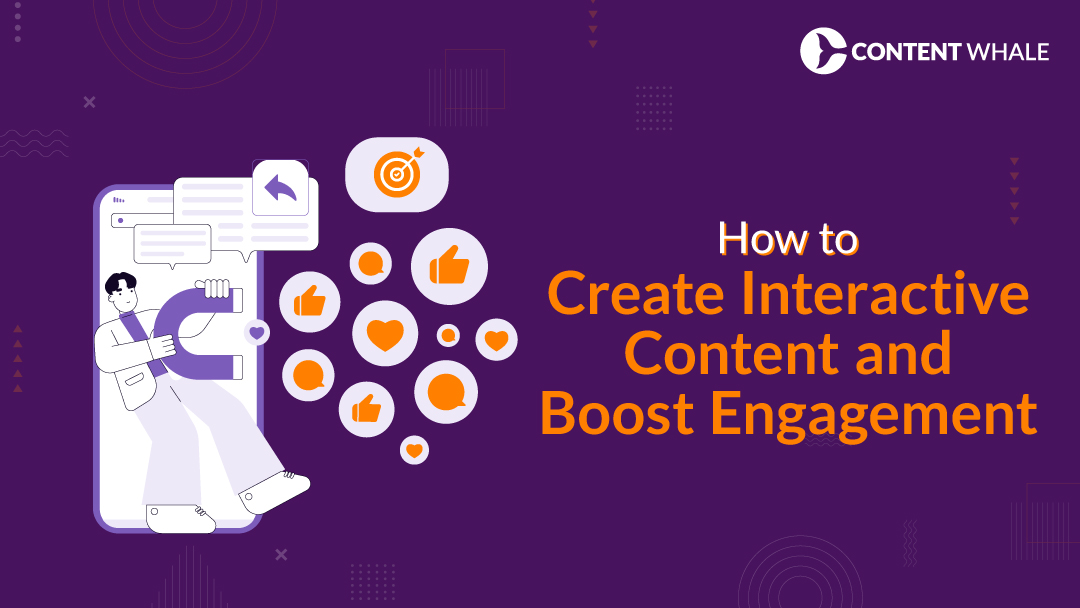
How to Create Interactive Content and Boost Engagement
Learn how to create interactive content to engage your audience. Discover tools, strategies, and benefits of using interactive elements.
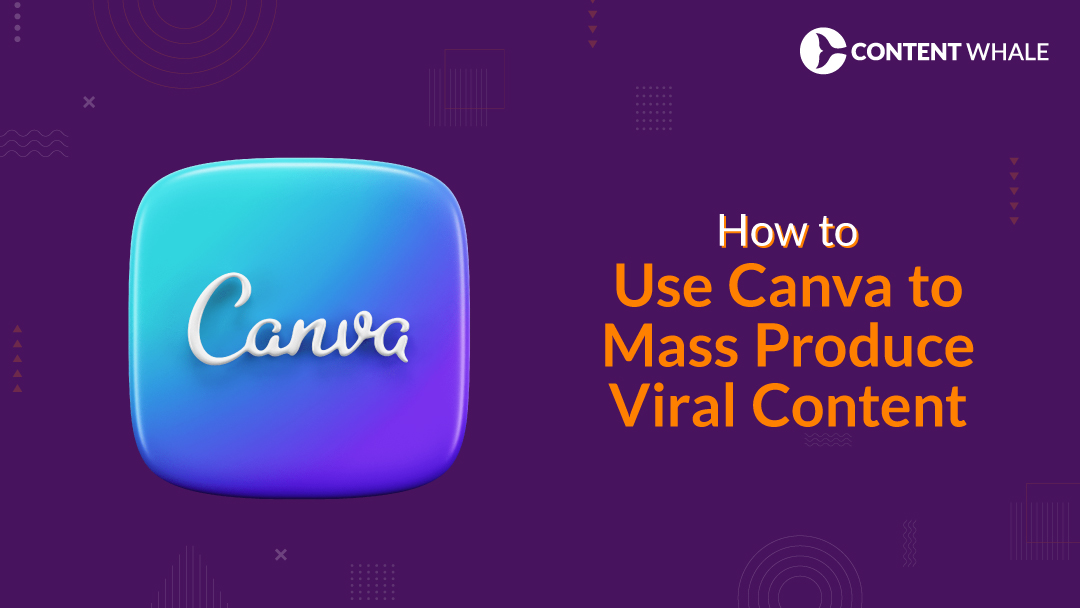
How to Use Canva to Mass Produce Viral Content
Discover how to use Canva to mass produce viral content with these expert tips and strategies. Boost your content creation game today!
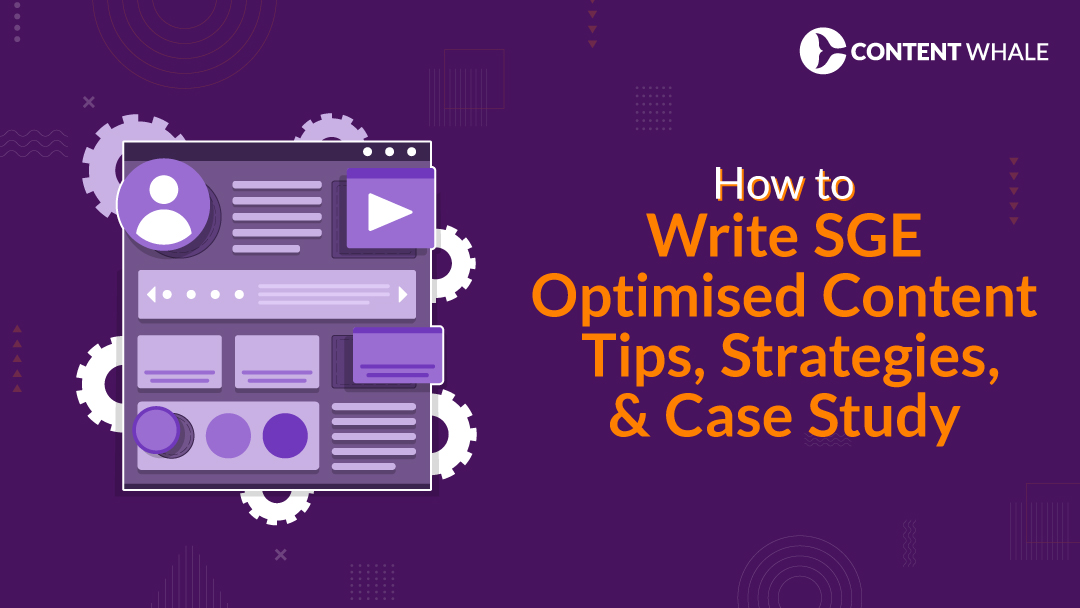
SGE Ranking Strategies: How to Get Rankings on SGEs
Learn how to create answers that rank for SGE with our comprehensive guide on SGE ranking strategies. Powerful tips and examples!
Need assistance with something
Speak with our expert right away to receive free service-related advice.
- Open access
- Published: 03 June 2024
The use of evidence to guide decision-making during the COVID-19 pandemic: divergent perspectives from a qualitative case study in British Columbia, Canada
- Laura Jane Brubacher ORCID: orcid.org/0000-0003-2806-9539 1 , 2 ,
- Chris Y. Lovato 1 ,
- Veena Sriram 1 , 3 ,
- Michael Cheng 1 &
- Peter Berman 1
Health Research Policy and Systems volume 22 , Article number: 66 ( 2024 ) Cite this article
34 Accesses
1 Altmetric
Metrics details
The challenges of evidence-informed decision-making in a public health emergency have never been so notable as during the COVID-19 pandemic. Questions about the decision-making process, including what forms of evidence were used, and how evidence informed—or did not inform—policy have been debated.
We examined decision-makers' observations on evidence-use in early COVID-19 policy-making in British Columbia (BC), Canada through a qualitative case study. From July 2021- January 2022, we conducted 18 semi-structured key informant interviews with BC elected officials, provincial and regional-level health officials, and civil society actors involved in the public health response. The questions focused on: (1) the use of evidence in policy-making; (2) the interface between researchers and policy-makers; and (3) key challenges perceived by respondents as barriers to applying evidence to COVID-19 policy decisions. Data were analyzed thematically, using a constant comparative method. Framework analysis was also employed to generate analytic insights across stakeholder perspectives.
Overall, while many actors’ impressions were that BC's early COVID-19 policy response was evidence-informed, an overarching theme was a lack of clarity and uncertainty as to what evidence was used and how it flowed into decision-making processes. Perspectives diverged on the relationship between 'government' and public health expertise, and whether or not public health actors had an independent voice in articulating evidence to inform pandemic governance. Respondents perceived a lack of coordination and continuity across data sources, and a lack of explicit guidelines on evidence-use in the decision-making process, which resulted in a sense of fragmentation. The tension between the processes involved in research and the need for rapid decision-making was perceived as a barrier to using evidence to inform policy.
Conclusions
Areas to be considered in planning for future emergencies include: information flow between policy-makers and researchers, coordination of data collection and use, and transparency as to how decisions are made—all of which reflect a need to improve communication. Based on our findings, clear mechanisms and processes for channeling varied forms of evidence into decision-making need to be identified, and doing so will strengthen preparedness for future public health crises.
Peer Review reports
The challenges of evidence-informed decision-making Footnote 1 in a public health emergency have never been so salient as during the COVID-19 pandemic, given its unprecedented scale, rapidly evolving virology, and multitude of global information systems to gather, synthesize, and disseminate evidence on the SARS-CoV-2 virus and associated public health and social measures [ 1 , 2 , 3 ]. Early in the COVID-19 pandemic, rapid decision-making became central for governments globally as they grappled with crucial decisions for which there was limited evidence. Critical questions exist, in looking retrospectively at these decision-making processes and with an eye to strengthening future preparedness: Were decisions informed by 'evidence'? What forms of evidence were used, and how, by decision-makers? [ 4 , 5 , 6 ].
Scientific evidence, including primary research, epidemiologic research, and knowledge synthesis, is one among multiple competing influences that inform decision-making processes in an outbreak such as COVID-19 [ 7 ]. Indeed, the use of multiple forms of evidence has been particularly notable as it applies to COVID-19 policy-making. Emerging research has also documented the important influence of ‘non-scientific’ evidence such as specialized expertise and experience, contextual information, and level of available resources [ 8 , 9 , 10 ]. The COVID-19 pandemic has underscored the politics of evidence-use in policy-making [ 11 ]; what evidence is used and how can be unclear, and shaped by political bias [ 4 , 5 ]. Moreover, while many governments have established scientific advisory boards, the perspectives of these advisors were reportedly largely absent from COVID-19 policy processes [ 6 ]. How evidence and public health policy interface—and intersect—is a complex question, particularly in the dynamic context of a public health emergency.
Within Canada, a hallmark of the public health system and endorsed by government is evidence-informed decision-making [ 12 ]. In British Columbia (BC), Canada, during the early phases of COVID-19 (March—June 2020), provincial public health communication focused primarily on voluntary compliance with recommended public health and social measures, and on supporting those most affected by the pandemic. Later, the response shifted from voluntary compliance to mandatory enforceable government orders [ 13 ]. Like many other jurisdictions, the government’s public messaging in BC asserted that the province took an approach to managing the COVID-19 pandemic and developing related policy that was based on scientific evidence, specifically. For example, in March 2021, in announcing changes to vaccination plans, Dr. Bonnie Henry, the Provincial Health Officer, stated, " This is science in action " [ 14 ]. As a public health expert with scientific voice, the Provincial Health Officer has been empowered to speak on behalf of the BC government across the COVID-19 pandemic progression. While this suggests BC is a jurisdiction which has institutionalized scientifically-informed decision-making as a core tenet of effective public health crisis response, it remains unclear as to whether BC’s COVID-19 response could, in fact, be considered evidence-informed—particularly from the perspectives of those involved in pandemic decision-making and action. Moreover, if evidence-informed, what types of evidence were utilized and through what mechanisms, how did this evidence shape decision-making, and what challenges existed in moving evidence to policy and praxis in BC’s COVID-19 response?
The objectives of this study were: (1) to explore and characterize the perspectives of BC actors involved in the COVID-19 response with respect to evidence-use in COVID-19 decision-making; and (2) to identify opportunities for and barriers to evidence-informed decision-making in BC’s COVID-19 response, and more broadly. This inquiry may contribute to identifying opportunities for further strengthening the synthesis and application of evidence (considered broadly) to public health policy and decision-making, particularly in the context of future public health emergencies, both in British Columbia and other jurisdictions.
Study context
This qualitative study was conducted in the province of British Columbia (BC), Canada, a jurisdiction with a population of approximately five million people [ 15 ]. Within BC’s health sector, key actors involved in the policy response to COVID-19 included: elected officials, the BC Government’s Ministry of Health (MOH), the Provincial Health Services Authority (PHSA), Footnote 2 the Office of the Provincial Health Officer (PHO), Footnote 3 the BC Centre for Disease Control (BCCDC), Footnote 4 and Medical Health Officers (MHOs) and Chief MHOs at regional and local levels.
Health research infrastructure within the province includes Michael Smith Health Research BC [ 16 ] and multiple post-secondary research and education institutions (e.g., The University of British Columbia). Unlike other provincial (e.g., Ontario) and international (e.g., UK) jurisdictions, BC did not establish an independent, formal scientific advisory panel or separate organizational structure for public health intelligence in COVID-19. That said, a Strategic Research Advisory Council was established, reporting to the MOH and PHO, to identify COVID-19 research gaps and commission needed research for use within the COVID-19 response [ 17 ].
This research was part of a multidisciplinary UBC case study investigating the upstream determinants of the COVID-19 response in British Columbia, particularly related to institutions, politics, and organizations and how these interfaced with, and affected, pandemic governance [ 18 ]. Ethics approval for this study was provided by the University of British Columbia (UBC)’s Institutional Research Ethics Board (Certificate #: H20-02136).
Data collection
From July 2021 to January 2022, 18 semi-structured key informant interviews were conducted with BC elected officials, provincial and regional-level health officials, and civil society actors (e.g., within non-profit research organizations, unions) (Table 1 ). Initially, respondents were purposively sampled, based on their involvement in the COVID-19 response and their positioning within the health system organizational structure. Snowball sampling was used to identify additional respondents, with the intent of representing a range of organizational roles and actor perspectives. Participants were recruited via email invitation and provided written informed consent to participate.
Interviews were conducted virtually using Zoom® videoconferencing, with the exception of one hybrid in-person/Zoom® interview. Each interview was approximately one hour in duration. One to two research team members led each interview. The full interview protocol focused on actors’ descriptions of decision-making processes across the COVID-19 pandemic progression, from January 2020 to the date of the interviews, and they were asked to identify key decision points (e.g., emergency declaration, business closures) [see Additional File 1 for the full semi-structured interview guide]. For this study, we used a subset of interview questions focused on evidence-use in the decision-making process, and the organizational structures or actors involved, in BC's early COVID-19 pandemic response (March–August 2020). Questions were adapted to be relevant to a respondent’s expertise and particular involvement in the response. ‘Evidence’ was left undefined and considered broadly by the research team (i.e., both ‘scientific’/research-based and ‘non-scientific’ inputs) within interview questions, and therefore at the discretion of the participant as to what inputs they perceived and described as ‘evidence’ that informed or did not inform pandemic decision-making. Interviews were audio-recorded over Zoom® with permission and transcribed using NVivo Release 1.5© software. Each transcript was then manually verified for accuracy by 1–2 members of the research team.
Data analysis
An inductive thematic analysis was conducted, using a constant comparative method, to explore points of divergence and convergence across interviews and stakeholder perspectives [ 19 ]. Transcripts were inductively coded in NVivo Release 1.5© software, which was used to further organize and consolidate codes, generate a parsimonious codebook to fit the data, and retrieve interview excerpts [ 20 ]. Framework analysis was also employed as an additional method for generating analytic insights across stakeholder perspectives and contributed to refining the overall coding [ 21 ]. Triangulation across respondents and analytic methods, as well as team collaboration in reviewing and refining the codebook, contributed to validity of the analysis [ 22 ].
How did evidence inform early COVID-19 policy-making in BC?
Decision-makers described their perceptions on the use of evidence in policy-making; the interface between researchers and policy-makers; and specific barriers to evidence-use in policy-making within BC’s COVID-19 response. In discussing the use of evidence, respondents focused on ‘scientific’ evidence; however, they noted a lack of clarity as to how and what evidence flowed into decision-making. They also acknowledged that ‘scientific’ evidence was one of multiple factors influencing decisions. The themes described below reflect the narrative underlying their perspectives.
Perceptions of evidence-use
Multiple provincial actors generally expressed confidence or had an overall impression that decisions were evidence-based (IDI5,9), stating definitively that, "I don’t think there was a decision we made that wasn’t evidence-informed" (IDI9) and that "the science became a driver of decisions that were made" (IDI5). However, at the regional health authority level, one actor voiced skepticism that policy decisions were consistently informed by scientific evidence specifically, stating, "a lot of decisions [the PHO] made were in contrast to science and then shifted to be by the science" ( IDI6). The evolving nature of the available evidence and scientific understanding of the virus throughout the pandemic was acknowledged. For instance, one actor stated that, "I’ll say the response has been driven by the science; the science has been changing…from what I’ve seen, [it] has been a very science-based response" (IDI3).
Some actors narrowed in on certain policy decisions they believed were or were not evidence-informed. Policy decisions in 2020 that actors believed were directly informed by scientific data included the early decision to restrict informal, household gatherings; to keep schools open for in-person learning; to implement a business safety plan requirement across the province; and to delay the second vaccine dose for maximum efficacy. One provincial public health actor noted that an early 2020 decision made, within local jurisdictions, to close playgrounds was not based on scientific evidence. Further, the decision prompted public health decision-makers to centralize some decision-making to the provincial level, to address decisions being made 'on the ground' that were not based on scientific evidence (IDI16). Similarly, they added that the policy decision to require masking in schools was not based on scientific evidence; rather, "it's policy informed by the noise of your community." As parents and other groups within the community pushed for masking, this was "a policy decision to help schools stay open."
Early in the pandemic response, case data in local jurisdictions were reportedly used for monitoring and planning. These "numerator data" (IDI1), for instance case or hospitalization counts, were identified as being the primary mode of evidence used to inform decisions related to the implementation or easing of public health and social measures. The ability to generate epidemiological count data early in the pandemic due to efficient scaling up of PCR testing for COVID-19 was noted as a key advantage (IDI16). As the pandemic evolved in 2020, however, perspectives diverged in relation to the type of data that decision-makers relied on. For example, it was noted that BCCDC administered an online, voluntary survey to monitor unintended consequences of public health and social measures and inform targeted interventions. Opinions varied on whether this evidence was successfully applied in decision-making. One respondent emphasized this lack of application of evidence and perceived that public health orders were not informed by the level and type of evidence available, beyond case counts: "[In] a communicable disease crisis like a pandemic, the collateral impact slash damage is important and if you're going to be a public health institute, you actually have to bring those to the front, not just count cases" (IDI1).
There also existed some uncertainty and a perceived lack of transparency or clarity as to how or whether data analytic ‘entities’, such as BCCDC or research institutions, fed directly into decision-making. As a research actor shared, "I’m not sure that I know quite what all those channels really look like…I’m sure that there’s a lot of improvement that could be driven in terms of how we bring strong evidence to actual policy and practice" (IDI14). Another actor explicitly named the way information flowed into decision-making in the province as "organic" (IDI7). They also noted the lack of a formal, independent science advisory panel for BC’s COVID-19 response, which existed in other provincial and international jurisdictions. Relatedly, one regional health authority actor perceived that the committee that was convened to advise the province on research, and established for the purpose of applying research to the COVID-19 response, "should have focused more on knowledge translation, but too much time was spent commissioning research and asking what kinds of questions we needed to ask rather than looking at what was happening in other jurisdictions" (IDI6). Overall, multiple actors noted a lack of clarity around application of evidence and who is responsible for ensuring evidence is applied. As a BCCDC actor expressed, in relation to how to prevent transmission of COVID-19:
We probably knew most of the things that we needed to know about May of last year [2020]. So, to me, it’s not even what evidence you need to know about, but who’s responsible for making sure that you actually apply the evidence to the intervention? Because so many of our interventions have been driven by peer pressure and public expectation rather than what we know to be the case [scientifically] (IDI1).
Some described the significance of predictive disease modelling to understand the COVID-19 trajectory and inform decisions, as well as to demonstrate to the public the effectiveness of particular measures, which "help[ed] sustain our response" (IDI2). Others, however, perceived that "mathematical models were vastly overused [and] overvalued in decision-making around this pandemic" (IDI1) and that modellers stepped outside their realm of expertise in providing models and policy recommendations through the public media.
Overall, while many actors’ impressions were that the response was evidence-informed, an overarching theme was a lack of clarity and uncertainty with respect to how evidence actually flowed into decision-making processes, as well as what specific evidence was used and how. Participants noted various mechanisms created or already in place prior to COVID-19 that fed data into, and facilitated, decision-making. There was an acknowledgement that multiple forms of evidence—including scientific data, data on public perceptions, as well as public pressure—appeared to have influenced decision-making.
Interface between researchers and policy-makers
There was a general sense that the Ministry supported the use of scientific and research-based evidence specifically. Some actors identified particular Ministry personnel as being especially amenable to research and focused on data to inform decisions and implementation. More broadly, the government-research interface was characterized by one actor as an amicable one, a "research-friendly government", and that the Ministry of Health (MOH), specifically, has a research strategy whereby, "it’s literally within their bureaucracy to become a more evidence-informed organization" (IDI11). The MOH was noted to have funded a research network intended to channel evidence into health policy and practice, and which reported to the research side of the MOH.
Other actors perceived relatively limited engagement with the broader scientific community. Some perceived an overreliance on 'in-house expertise' or a "we can do that [ourselves] mentality" within government that precluded academic researchers’ involvement, as well as a sense of "not really always wanting to engage with academics to answer policy questions because they don’t necessarily see the value that comes" (IDI14). With respect to the role of research, an actor stated:
There needs to be a provincial dialogue around what evidence is and how it gets situated, because there’s been some tension around evidence being produced and not used or at least not used in the way that researchers think that it should be (IDI11).
Those involved in data analytics within the MOH acknowledged a challenge in making epidemiological data available to academic researchers, because "at the time, you’re just trying to get decisions made" (IDI7). Relatedly, a research actor described the rapid instigation of COVID-19 research and pivoting of academic research programs to respond to the pandemic, but perceived a slow uptake of these research efforts from the MOH and PHSA for decision-making and action. Nevertheless, they too acknowledged the challenge of using research evidence, specifically, in an evolving and dynamic pandemic:
I think we’ve got to be realistic about what research in a pandemic situation can realistically contribute within very short timelines. I mean, some of these decisions have to be made very quickly...they were intuitive decisions, I think some of them, rather than necessarily evidence-based decisions (IDI14).
Relatedly, perspectives diverged on the relationship between 'government' and public health expertise, and whether or not public health actors had an independent voice in articulating evidence to inform governance during the pandemic. Largely from Ministry stakeholders, and those within the PHSA, the impressions were that Ministry actors were relying on public health advice and scientific expertise. As one actor articulated, "[the] government actually respected and acknowledged and supported public health expertise" (IDI9). Others emphasized a "trust of the people who understood the problem" (IDI3)—namely, those within public health—and perceived that public health experts were enabled "to take a lead role in the health system, over politics" (IDI12). This perspective was not as widely held by those in the public health sector, as one public health actor expressed, "politicians and bureaucrats waded into public health practice in a way that I don't think was appropriate" and that, "in the context of a pandemic, it’s actually relatively challenging to bring true expert advice because there’s too many right now. Suddenly, everybody’s a public health expert, but especially bureaucrats and politicians." They went on to share that the independence of public health to speak and act—and for politicians to accept independent public health advice—needs to be protected and institutionalized as "core to good governance" (IDI1). Relatedly, an elected official linked this to the absence of a formal, independent science table to advise government and stated that, "I think we should have one established permanently. I think we need to recognize that politicians aren't always the best at discerning scientific evidence and how that should play into decision-making" (IDI15).
These results highlight the divergent perspectives participants had as to the interface between research and policy-making and a lack of understanding regarding process and roles.
Challenges in applying evidence to policy decisions
Perspectives converged with respect to the existence of numerous challenges with and barriers to applying evidence to health policy and decision-making. These related to the quality and breadth of available data, both in terms of absence and abundance. For instance, as one public health actor noted in relation to health policy-making, "you never have enough information. You always have an information shortage, so you're trying to make the best decisions you can in the absence of usually really clear information" (IDI8). On the other hand, as evidence emerged en masse across jurisdictions in the pandemic, there were challenges with synthesizing evidence in a timely fashion for 'real-time' decision-making. A regional health authority actor highlighted this challenge early in the COVID-19 pandemic and perceived that there was not a provincial group bringing new synthesized information to decision-makers on a daily basis (IDI6). Other challenges related to the complexity of the political-public health interface with respect to data and scientific expertise, which "gets debated and needs to be digested by the political process. And then decisions are made" (IDI5). This actor further expressed that debate among experts needs to be balanced with efficient crisis response, that one has to "cut the debate short. For the sake of expediency, you need to react."
It was observed that, in BC’s COVID-19 response, data was gathered from multiple sources with differing data collection procedures, and sometimes with conflicting results—for instance, 'health system data' analyzed by the PHSA and 'public health data' analyzed by the BCCDC. This was observed to present challenges from a political perspective in discerning "who’s actually getting the 'right' answers" (IDI7). An added layer of complexity was reportedly rooted in how to communicate such evidence to the public and "public trust in the numbers" (IDI7), particularly as public understanding of what evidence is, how it is developed, and why it changes, can influence public perceptions of governance.
Finally, as one actor from within the research sector noted, organizationally and governance-wise, the system was "not very well set up to actually use research evidence…if we need to do better at using evidence in practice, we need to fix some of those things. And we actually know what a lot of those things are." For example , "there’s no science framework for how organizations work within that" and " governments shy away from setting science policy " (IDI11). This challenge was framed as having a macro-level dimension, as higher-level leadership structures were observed to not incentivize the development and effective use of research among constituent organizations, and also micro-level implications. From their perspective, researchers will struggle without such policy frameworks to obtain necessary data-sharing agreements with health authorities, nor will they be able to successfully navigate other barriers to conducting action-oriented research that informs policy and practice.
Similarly, a research actor perceived that the COVID-19 pandemic highlighted pre-existing fragmentation, "a pretty disjointed sort of enterprise" in how research is organized in the province:
I think pandemics need strong leadership and I think pandemic research response needed probably stronger leadership than it had. And I think that’s to do with [how] no one really knew who was in charge because no one really was given the role of being truly in charge of the research response (IDI14).
This individual underscored that, at the time of the interview, there were nearly 600 separate research projects being conducted in BC that focused on COVID-19. From their perspective, this reflected the need for more centralized direction to provide leadership, coordinate research efforts, and catalyze collaborations.
Overall, respondents perceived a lack of coordination and continuity across data sources, and a lack of explicit guidelines on evidence-use in the decision-making process, which resulted in a sense of fragmentation. The tension between the processes involved in research and the need for rapid decision-making was perceived as a barrier to using evidence to inform policy.
This study explored the use of evidence to inform early COVID-19 decision-making within British Columbia, Canada, from the perspectives of decision-makers themselves. Findings underscore the complexity of synthesizing and applying evidence (i.e., ‘scientific’ or research-based evidence most commonly discussed) to support public health policy in 'real-time', particularly in the context of public health crisis response. Despite a substantial and long-established literature on evidence-based clinical decision-making [ 23 , 24 ], understanding is more limited as to how public health crisis decision-making can be evidence-informed or evidence-based. By contributing to a growing global scholarship of retrospective examinations of COVID-19 decision-making processes [ 25 , 26 , 27 , 28 ], our study aimed to broaden this understanding and, thus, support the strengthening of public health emergency preparedness in Canada, and globally.
Specifically, based on our findings on evidence-based public health practice, we found that decision-makers clearly emphasized ‘evidence-based’ or ‘evidence-informed’ as meaning ‘scientific’ evidence. They acknowledged other forms of evidence such as professional expertise and contextual information as influencing factors. We identified four key points related to the process of evidence-use in BC's COVID-19 decision-making, with broader implications as well:
Role Differences: The tensions we observed primarily related to a lack of clarity among the various agencies involved as to their respective roles and responsibilities in a public health emergency, a finding that aligns with research on evidence-use in prior pandemics in Canada [ 29 ]. Relatedly, scientists and policy-makers experienced challenges with communication and information-flow between one another and the public, which may reflect their different values and standards, framing of issues and goals, and language [ 30 ].
Barriers to Evidence-Use: Coordination and consistency in how data are collected across jurisdictions reportedly impeded efficiency and timeliness of decision-making. Lancaster and Rhodes (2020) suggest that evidence itself should be treated as a process, rather than a commodity, in evidence-based practice [ 31 ]. Thus, shifting the dialogue from 'barriers to evidence use' to an approach that fosters dialogue across different forms of evidence and different actors in the process may be beneficial.
Use of Evidence in Public Health versus Medicine: Evidence-based public health can be conflated with the concept of evidence-based medicine, though these are distinct in the type of information that needs to be considered. While ‘research evidence’ was the primary type of evidence used, other important types of evidence informed policy decisions in the COVID-19 public health emergency—for example, previous experience, public values, and preferences. This concurs with Brownson’s (2009) framework of factors driving decision-making in evidence-based public health [ 32 ]. Namely, that a balance between multiple factors, situated in particular environmental and organizational context, shapes decision-making: 1) best available research evidence; 2) clients'/population characteristics, state, needs, values, and preferences; and 3) resources, including a practitioner’s expertise. Thus, any evaluation of evidence-use in public health policy must take into consideration this multiplicity of factors at play, and draw on frameworks specific to public health [ 33 ]. Moreover, public health decision-making requires much more attention to behavioural factors and non-clinical impacts, which is distinct from the largely biology-focused lens of evidence-based medicine.
Transparency: Many participants emphasized a lack of explanation about why certain decisions were made and a lack of understanding about who was involved in decisions and how those decisions were made. This point was confirmed by a recent report on lessons learned in BC during the COVID-19 pandemic in which the authors describe " the desire to know more about the reasons why decisions were taken " as a " recurring theme " (13:66). These findings point to a need for clear and transparent mechanisms for channeling evidence, irrespective of the form used, into public health crisis decision-making.
Our findings also pointed to challenges associated with the infrastructure for utilizing research evidence in BC policy-making, specifically a need for more centralized authority on the research side of the public health emergency response to avoid duplication of efforts and more effectively synthesize findings for efficient use. Yet, as a participant questioned, what is the realistic role of research in a public health crisis response? Generally, most evidence used to inform crisis response measures is local epidemiological data or modelling data [ 7 ]. As corroborated by our findings, challenges exist in coordinating data collection and synthesis of these local data across jurisdictions to inform 'real-time' decision-making, let alone to feed into primary research studies [ 34 ].
On the other hand, as was the case in the COVID-19 pandemic, a 'high noise' research environment soon became another challenge as data became available to researchers. Various mechanisms have been established to try and address these challenges amid the COVID-19 pandemic, both to synthesize scientific evidence globally and to create channels for research evidence to support timely decision-making. For instance: 1) research networks and collaborations are working to coordinate research efforts (e.g., COVID-END network [ 35 ]); 2) independent research panels or committees within jurisdictions provide scientific advice to inform decision-making; and 3) research foundations, funding agencies, and platforms for knowledge mobilization (e.g., academic journals) continue to streamline funding through targeted calls for COVID-19 research grant proposals, or for publication of COVID-19 research articles. While our findings describe the varied forms of evidence used in COVID-19 policy-making—beyond scientific evidence—they also point to the opportunity for further investments in infrastructure that coordinates, streamlines, and strengthens collaborations between health researchers and decision-makers that results in timely uptake of results into policy decisions.
Finally, in considering these findings, it is important to note the study's scope and limitations: We focused on evidence use in a single public health emergency, in a single province. Future research could expand this inquiry to a multi-site analysis of evidence-use in pandemic policy-making, with an eye to synthesizing lessons learned and best practices. Additionally, our sample of participants included only one elected official, so perspectives were limited from this type of role. The majority of participants were health officials who primarily referred to and discussed evidence as ‘scientific’ or research-based evidence. Further work could explore the facilitators and barriers to evidence-use from the perspectives of elected officials and Ministry personnel, particularly with respect to the forms of evidence—considered broadly—and other varied inputs, that shape decision-making in the public sphere. This could include a more in-depth examination of policy implementation and how the potential societal consequences of implementation factor into public health decision-making.
We found that the policy decisions made during the initial stages of the COVID-19 pandemic were perceived by actors in BC's response as informed by—not always based on—scientific evidence, specifically; however, decision-makers also considered other contextual factors and drew on prior pandemic-related experience to inform decision-making, as is common in evidence-based public health practice [ 32 ]. The respondents' experiences point to specific areas that need to be considered in planning for future public health emergencies, including information flow between policy-makers and researchers, coordination in how data are collected, and transparency in how decisions are made—all of which reflect a need to improve communication. Furthermore, shifting the discourse from evidence as a commodity to evidence-use as a process will be helpful in addressing barriers to evidence-use, as well as increasing understanding about the public health decision-making process as distinct from clinical medicine. Finally, there is a critical need for clear mechanisms that channel evidence (whether ‘scientific’, research-based, or otherwise) into health crisis decision-making, including identifying and communicating the decision-making process to those producing and synthesizing evidence. The COVID-19 pandemic experience is an opportunity to reflect on what needs to be done to guild our public health systems for the future [ 36 , 37 ]. Understanding and responding to the complexities of decision-making as we move forward, particularly with respect to the synthesis and use of evidence, can contribute to strengthening preparedness for future public health emergencies.
Availability of data and materials
The data that support the findings of this study are not publicly available to maintain the confidentiality of research participants.
The terms 'evidence-informed' and 'evidence-based' decision-making are used throughout this paper, though are distinct. The term 'evidence-informed' suggests that evidence is used and considered, though not necessarily solely determinative in decision-making [ 38 ].
The Provincial Health Services Authority (PHSA) works with the Ministry of Health (MOH) and regional health authorities to oversee the coordination and delivery of programs.
The Office of the Provincial Health Officer (PHO) has binding legal authority in the case of an emergency, and responsibility to monitor the health of BC’s population and provide independent advice to Ministers and public offices on public health issues.
The British Columbia Centre for Disease Control (BCCDC) is a program of the PHSA and provides provincial and national disease surveillance, detection, treatment, prevention, and consultation.
Abbreviations
British Columbia
British Columbia Centre for Disease Control
Coronavirus Disease 2019
Medical Health Officer
Ministry of Health
Provincial Health Officer
Provincial Health Services Authority
Severe Acute Respiratory Syndrome Coronavirus—2
University of British Columbia
Rubin O, Errett NA, Upshur R, Baekkeskov E. The challenges facing evidence-based decision making in the initial response to COVID-19. Scand J Public Health. 2021;49(7):790–6.
Article PubMed Google Scholar
Williams GA, Ulla Díez SM, Figueras J, Lessof S, Ulla SM. Translating evidence into policy during the COVID-19 pandemic: bridging science and policy (and politics). Eurohealth (Lond). 2020;26(2):29–48.
Google Scholar
Vickery J, Atkinson P, Lin L, Rubin O, Upshur R, Yeoh EK, et al. Challenges to evidence-informed decision-making in the context of pandemics: qualitative study of COVID-19 policy advisor perspectives. BMJ Glob Heal. 2022;7(4):1–10.
Piper J, Gomis B, Lee K. “Guided by science and evidence”? The politics of border management in Canada’s response to the COVID-19 pandemic. Front Polit Sci. 2022;4
Cairney P. The UK government’s COVID-19 policy: what does “Guided by the science” mean in practice? Front Polit Sci. 2021;3(March):1–14.
Colman E, Wanat M, Goossens H, Tonkin-Crine S, Anthierens S. Following the science? Views from scientists on government advisory boards during the COVID-19 pandemic: a qualitative interview study in five European countries. BMJ Glob Heal. 2021;6(9):1–11.
Salajan A, Tsolova S, Ciotti M, Suk JE. To what extent does evidence support decision making during infectious disease outbreaks? A scoping literature review. Evid Policy. 2020;16(3):453–75.
Article Google Scholar
Cairney P. The UK government’s COVID-19 policy: assessing evidence-informed policy analysis in real time. Br Polit. 2021;16(1):90–116.
Lancaster K, Rhodes T, Rosengarten M. Making evidence and policy in public health emergencies: lessons from COVID-19 for adaptive evidence-making and intervention. Evid Policy. 2020;16(3):477–90.
Yang K. What can COVID-19 tell us about evidence-based management? Am Rev Public Adm. 2020;50(6–7):706–12.
Parkhurst J. The politics of evidence: from evidence-based policy to the good governance of evidence. Abingdon: Routledge; 2017.
Office of the Prime Minister. Minister of Health Mandate Letter [Internet]. 2021. https://pm.gc.ca/en/mandate-letters/2021/12/16/minister-health-mandate-letter
de Faye B, Perrin D, Trumpy C. COVID-19 lessons learned review: Final report. Victoria, BC; 2022.
First Nations Health Authority. Evolving vaccination plans is science in action: Dr. Bonnie Henry. First Nations Health Authority. 2021.
BC Stats. 2021 Sub-provincial population estimates highlights. Vol. 2021. Victoria, BC; 2022.
Michael Smith Health Research BC [Internet]. 2023. healthresearchbc.ca. Accessed 25 Jan 2023.
Michael Smith Health Research BC. SRAC [Internet]. 2023. https://healthresearchbc.ca/strategic-provincial-advisory-committee-srac/ . Accessed 25 Jan 2023.
Brubacher LJ, Hasan MZ, Sriram V, Keidar S, Wu A, Cheng M, et al. Investigating the influence of institutions, politics, organizations, and governance on the COVID-19 response in British Columbia, Canada: a jurisdictional case study protocol. Heal Res Policy Syst. 2022;20(1):1–10.
Braun V, Clarke V. Using thematic analysis in psychology. Qual Res Psychol. 2006;3:77–101.
DeCuir-Gunby JT, Marshall PL, McCulloch AW. Developing and using a codebook for the analysis of interview data: an example from a professional development research project. Field Methods. 2011;23(2):136–55.
Gale NK, Heath G, Cameron E, Rashid S, Redwood S. Using the framework method for the analysis of qualitative data in multi-disciplinary health research. BMC Med Res Methodol. 2013;13(117):1–8.
Creswell JW, Miller DL. Determining validity in qualitative inquiry. Theory Pract. 2000;39(3):124–30.
Sackett D. How to read clinical journals: I. Why to read them and how to start reading them critically. Can Med Assoc J. 1981;1245:555–8.
Evidence Based Medicine Working Group. Evidence-based medicine: a new approach to teaching the practice of medicine. JAMA Netw. 1992;268(17):2420–5.
Allin S, Fitzpatrick T, Marchildon GP, Quesnel-Vallée A. The federal government and Canada’s COVID-19 responses: from “we’re ready, we’re prepared” to “fires are burning.” Heal Econ Policy Law. 2022;17(1):76–94.
Bollyky TJ, Hulland EN, Barber RM, Collins JK, Kiernan S, Moses M, et al. Pandemic preparedness and COVID-19: an exploratory analysis of infection and fatality rates, and contextual factors associated with preparedness in 177 countries, from Jan 1, 2020, to Sept 30, 2021. Lancet. 2022;6736(22):1–24.
Kuhlmann S, Hellström M, Ramberg U, Reiter R. Tracing divergence in crisis governance: responses to the COVID-19 pandemic in France, Germany and Sweden compared. Int Rev Adm Sci. 2021;87(3):556–75.
Haldane V, De Foo C, Abdalla SM, Jung AS, Tan M, Wu S, et al. Health systems resilience in managing the COVID-19 pandemic: lessons from 28 countries. Nat Med. 2021;27(6):964–80.
Article CAS PubMed Google Scholar
Rosella LC, Wilson K, Crowcroft NS, Chu A, Upshur R, Willison D, et al. Pandemic H1N1 in Canada and the use of evidence in developing public health policies—a policy analysis. Soc Sci Med. 2013;83:1–9.
Article PubMed PubMed Central Google Scholar
Saner M. A map of the interface between science & policy. Ottawa, Ontario; 2007. Report No.: January 1.
Lancaster K, Rhodes T. What prevents health policy being “evidence-based”? New ways to think about evidence, policy and interventions in health. Br Med Bull. 2020;135(1):38–49.
Brownson RC, Fielding JE, Maylahn CM. Evidence-based public health: a fundamental concept for public health practice. Annu Rev Public Health. 2009;30:175–201.
Rychetnik L, Frommer M, Hawe P, Shiell A. Criteria for evaluating evidence on public health interventions. J Epidemiol Community Health. 2002;56:119–27.
Article CAS PubMed PubMed Central Google Scholar
Khan Y, Brown A, Shannon T, Gibson J, Généreux M, Henry B, et al. Public health emergency preparedness: a framework to promote resilience. BMC Public Health. 2018;18(1):1–16.
COVID-19 Evidence Network to Support Decision-Making. COVID-END [Internet]. 2023. https://www.mcmasterforum.org/networks/covid-end . Accessed 25 Jan 2023.
Canadian Institutes of Health Research. Moving forward from the COVID-19 pandemic: 10 opportunities for strengthening Canada’s public health systems. 2022.
Di Ruggiero E, Bhatia D, Umar I, Arpin E, Champagne C, Clavier C, et al. Governing for the public’s health: Governance options for a strengthened and renewed public health system in Canada. 2022.
Adjoa Kumah E, McSherry R, Bettany-Saltikov J, Hamilton S, Hogg J, Whittaker V, et al. Evidence-informed practice versus evidence-based practice educational interventions for improving knowledge, attitudes, understanding, and behavior toward the application of evidence into practice: a comprehensive systematic review of undergraduate studen. Campbell Syst Rev. 2019;15(e1015):1–19.
Download references
Acknowledgements
We would like to extend our gratitude to current and former members of the University of British Columbia Working Group on Health Systems Response to COVID-19 who contributed to various aspects of this study, including Shelly Keidar, Kristina Jenei, Sydney Whiteford, Dr. Md Zabir Hasan, Dr. David M. Patrick, Dr. Maxwell Cameron, Mahrukh Zahid, Dr. Yoel Kornreich, Dr. Tammi Whelan, Austin Wu, Shivangi Khanna, and Candice Ruck.
Financial support for this work was generously provided by the University of British Columbia's Faculty of Medicine (Grant No. GR004683) and Peter Wall Institute for Advanced Studies (Grant No. GR016648), as well as a Canadian Institutes of Health Research Operating Grant (Grant No. GR019157). These funding bodies were not involved in the design of the study, the collection, analysis or interpretation of data, or in the writing of this manuscript.
Author information
Authors and affiliations.
School of Population and Public Health, University of British Columbia, Vancouver, Canada
Laura Jane Brubacher, Chris Y. Lovato, Veena Sriram, Michael Cheng & Peter Berman
School of Public Health Sciences, University of Waterloo, Waterloo, Canada
Laura Jane Brubacher
School of Public Policy and Global Affairs, University of British Columbia, Vancouver, Canada
Veena Sriram
You can also search for this author in PubMed Google Scholar
Contributions
CYL, PB, and VS obtained funding for and designed the study. LJB, MC, and PB conducted data collection. LJB and VS analyzed the qualitative data. CYL and LJB collaboratively wrote the manuscript. All authors read and approved the final manuscript.
Corresponding author
Correspondence to Laura Jane Brubacher .
Ethics declarations
Ethics approval and consent to participate.
This case study received the approval of the UBC Behavioural Research Ethics Board (Certificate # H20-02136). Participants provided written informed consent.
Consent for publication
Not applicable.
Competing interests
The authors declare that they have no competing interests.
Additional information
Publisher's note.
Springer Nature remains neutral with regard to jurisdictional claims in published maps and institutional affiliations.
Supplementary Information
Additional file 1..
Semi-structured interview guide [* = questions used for this specific study]
Rights and permissions
Open Access This article is licensed under a Creative Commons Attribution 4.0 International License, which permits use, sharing, adaptation, distribution and reproduction in any medium or format, as long as you give appropriate credit to the original author(s) and the source, provide a link to the Creative Commons licence, and indicate if changes were made. The images or other third party material in this article are included in the article's Creative Commons licence, unless indicated otherwise in a credit line to the material. If material is not included in the article's Creative Commons licence and your intended use is not permitted by statutory regulation or exceeds the permitted use, you will need to obtain permission directly from the copyright holder. To view a copy of this licence, visit http://creativecommons.org/licenses/by/4.0/ . The Creative Commons Public Domain Dedication waiver ( http://creativecommons.org/publicdomain/zero/1.0/ ) applies to the data made available in this article, unless otherwise stated in a credit line to the data.
Reprints and permissions
About this article
Cite this article.
Brubacher, L.J., Lovato, C.Y., Sriram, V. et al. The use of evidence to guide decision-making during the COVID-19 pandemic: divergent perspectives from a qualitative case study in British Columbia, Canada. Health Res Policy Sys 22 , 66 (2024). https://doi.org/10.1186/s12961-024-01146-2
Download citation
Received : 08 February 2023
Accepted : 29 April 2024
Published : 03 June 2024
DOI : https://doi.org/10.1186/s12961-024-01146-2
Share this article
Anyone you share the following link with will be able to read this content:
Sorry, a shareable link is not currently available for this article.
Provided by the Springer Nature SharedIt content-sharing initiative
- Decision-making
- Public health
- Policy-making
- Qualitative
Health Research Policy and Systems
ISSN: 1478-4505
- Submission enquiries: Access here and click Contact Us
- General enquiries: [email protected]
- Open access
- Published: 05 June 2024
Experiences of medical students and faculty regarding the use of long case as a formative assessment method at a tertiary care teaching hospital in a low resource setting: a qualitative study
- Jacob Kumakech 1 ,
- Ian Guyton Munabi 2 ,
- Aloysius Gonzaga Mubuuke 3 &
- Sarah Kiguli 4
BMC Medical Education volume 24 , Article number: 621 ( 2024 ) Cite this article
Metrics details
Introduction
The long case is used to assess medical students’ proficiency in performing clinical tasks. As a formative assessment, the purpose is to offer feedback on performance, aiming to enhance and expedite clinical learning. The long case stands out as one of the primary formative assessment methods for clinical clerkship in low-resource settings but has received little attention in the literature.
To explore the experiences of medical students and faculty regarding the use of the Long Case Study as a formative assessment method at a tertiary care teaching hospital in a low-resource setting.
Methodology
A qualitative study design was used. The study was conducted at Makerere University, a low-resource setting. The study participants were third- and fifth-year medical students as well as lecturers. Purposive sampling was utilized to recruit participants. Data collection comprised six Focus Group Discussions with students and five Key Informant Interviews with lecturers. The qualitative data were analyzed by inductive thematic analysis.
Three themes emerged from the study: ward placement, case presentation, and case assessment and feedback. The findings revealed that students conduct their long cases at patients’ bedside within specific wards/units assigned for the entire clerkship. Effective supervision, feedback, and marks were highlighted as crucial practices that positively impact the learning process. However, challenges such as insufficient orientation to the long case, the super-specialization of the hospital wards, pressure to hunt for marks, and inadequate feedback practices were identified.
The long case offers students exposure to real patients in a clinical setting. However, in tertiary care teaching hospitals, it’s crucial to ensure proper design and implementation of this practice to enable students’ exposure to a variety of cases. Adequate and effective supervision and feedback create valuable opportunities for each learner to present cases and receive corrections.
Peer Review reports
The long case serves as an authentic assessment method for evaluating medical students’ competence in clinical tasks [ 1 ]. This form of assessment requires students to independently spend time with patients taking their medical history, conducting physical examinations, and formulating diagnosis and management plans. Subsequently, students present their findings to senior clinicians for discussion and questioning [ 2 , 3 ]. While developed countries increasingly adopt simulation-based assessments for formative evaluation, logistical challenges hinder the widespread use of such methods in developing countries [ 4 ]. Consequently, the low-resource countries heavily rely on real patient encounters for formative assessment. The long case is one such method predominantly used as a primary formative assessment method during clinical clerkship and offers a great opportunity for feedback [ 5 ]. The assessment grounds students’ learning into practice by providing them with rich opportunities to interact with patients and have the feel of medical practice. The long case thus bridges the gap between theory and practice, immersing students in the real tasks of a physician [ 1 ]. The complexity of clinical scenarios and the anxiety associated with patient encounters may not be well replicated in simulation-based assessments because diseases often have atypical presentations not found in textbooks. Assessment methods should thus utilize authentic learning experiences to provide learners with applications of learning that they would expect to encounter in real life [ 6 ]. This requires medical education and the curriculum to focus attention on assessment because it plays a significant role in driving learning [ 7 ]. The long case thus remains crucial in medical education as one of the best ways of preparing for practice. It exposes the student repeatedly to taking medical history, examining patients, making clinical judgments, deciding treatment plans, and collaborating with senior clinicians.
The long case, however, has faced significant criticism in the medical education literature due to perceived psychometric deficiencies [ 8 , 9 , 10 ]. Consequently, many universities have begun to adopt assessment methods that yield more reliable and easily defensible results [ 2 ] due to concerns over the low reliability, generalizability, and validity of the long case, coupled with rising litigations and student appeals [ 11 , 12 ]. Despite these shortcomings, the long case remains an educationally valuable assessment tool that provides diagnostic feedback essential for the learning process during clinical clerkship [ 13 ]. Teachers can utilize long-case results to pinpoint neglected areas or teaching deficiencies and align with course outcomes.
However, there is a paucity of research into the long case as a formative assessment tool. A few studies conducted in developed countries highlighted its role in promoting a holistic approach to patient care, fostering students’ clinical skills, and a driving force for students to spend time with patients [ 2 , 13 ], . There is a notable absence of literature on the use of long case as a formative assessment method in low-resource countries, and no published work is available at Makerere University where it has been used for decades. This underscores the importance of conducting research in this area to provide insight into the effectiveness, challenges, and potentials for improvement. Therefore, this study aimed to investigate the experiences of medical students and faculty regarding the utilization of the long case as a formative assessment method within the context of a tertiary care teaching hospital in a low-resource setting.
Study design
This was an exploratory qualitative study.
Study setting
The research was conducted at Makerere University within the Department of Internal Medicine. The Bachelor of Medicine and Bachelor of Surgery (MBChB) degree at Makerere University is a five-year program with the first two years for pre-clinical (biomedical Sciences) course and the last three years dedicated to clinical clerkship. Medical students do Internal Medicine clerkships in third- and fifth-year at the two tertiary teaching hospitals namely; Mulago and Kiruddu National Referral Hospitals. The students are introduced to the long case in third-year as Junior Clerks and later in the fifth-year as Senior Clerks. During clerkship, students are assigned to various medical wards, where they interact with patients, take medical history from them, perform physical examinations, and develop diagnosis and management plans. Subsequently, students present their long cases to lecturers or postgraduate students, often in the presence of their peers, followed by feedback and comprehensive case discussions. Students are afforded ample time to prepare and present their cases during ward rounds, at their discretion. The students are formatively assessed and a mark is awarded on a scale of one to ten in the student’s logbook. Each student is required to make a minimum of ten long cases over the seven weeks of clerkship.
Study participants
The study participants were third- and fifth-year medical students who had completed junior and senior clerkship respectively, as well as lecturers who possessed at least five years of experience with the long case. The participants were selected through purposive sampling. The sample size for the study was determined by data saturation.
Data collection
Data were collected through Focus Group Discussions (FGDs) and Key Informant Interviews (KIIs). A total of 36 medical students participated in FGDs, reflecting on their experiences with the long case. Five faculty members participated in individual KIIs. The students were mobilized by their class representative and a brief recruitment presentation was made at the study site while the lecturers were approached via email and telephone invitation.
Six FGDs were conducted, three for junior clerks and three for senior clerks. Each FGD comprised of 5–7 participants with balanced male and female gender representation. Data saturation was achieved by the fifth FGD, at which point no additional new information emerged. A research assistant proficient in qualitative research methods moderated the FGDs. The discussions lasted between 55 min and 1 h 10 min and were audio recorded. The Principal Investigator attended all the FGDs to document interactions and record his perspectives and non-verbal cues of participants.
Semi-structured KIIs were used to collect data from Internal Medicine faculty. Five KIIs were conducted, and data saturation was achieved by the fourth interview, at which point no new theme emerged. The Principal Investigator conducted the KIIs via Zoom. Each interview lasted between 25 and 50 min and all were audio recorded. A research assistant proficient in qualitative methods attended all the Zoom meetings. The data collected were securely stored on a hard drive and Google Drive with password protection to prevent unauthorized access.
Data analysis
Data analysis was done through inductive thematic analysis method. Following each FGD or KII session, the data collection team listened to the recordings to familiarize themselves with the data and develop general ideas regarding the participants’ perspectives. The data were transcribed verbatim by the researchers to generate text data. Two separate transcripts were generated by the Principal Investigator and a research assistant. The transcripts were then compared and manually reviewed by the research team to compare the accuracy with the audio recordings. After transcript harmonization, data cleaning was done for both FGDs and KIIs transcripts.
The transcribed data from both FGDs and KIIs underwent inductive thematic analysis as aggregated data. This involved initial line-by-line coding, followed by focused coding where the relationships between initial codes were explored and similar codes were grouped. Throughout the analysis, the principle of constant comparison was applied, where emerging codes were compared for similarities and differences.
Study results
Socio-demographics.
A total of 36 medical students participated in the FGDs, comprising 18 junior clerks and 19 senior clerks. The participants were aged between 21 and 25 years except two participants who were aged above 25 (30 and 36 years old). Among the third-year students, there were 10 male and 9 female participants while the fifth-year student comprised of 8 male and 10 female participants.
Five lecturers participated in the Key Informant Interviews, three of whom were females and two male participants. They were aged between 40 and 50 years, and all had over 10 years of experience with the long case. The faculty members included one consultant physician, one associate professor, two senior lecturers, and one lecturer.
Themes that emerged
Three themes emerged from the study: ward placement, case presentations, and case assessment and feedback.
Theme 1: Ward placement
The study findings disclosed that medical students are assigned to specific wards for the duration of their clerkship. The specialization of medical wards was found to significantly restrict students’ exposure to limited disease conditions found only in their allocated ward.
With the super-specialization of the units, there is some bias on what they do learn; if a particular group is rotating on the cardiology unit, they will obviously have a bias to learn the history and physical exam related to cardiovascular disease (KII 1).
The students, particularly junior clerks, expressed dissatisfaction with the lack of proper and standardized orientation to the long case on the wards. This deficiency led to wastage of time and a feeling of being unwelcome in the clerkship.
Some orient you when you reach the ward but others you reach and you are supposed to pick up on your own. I expect orientation, then taking data from us, what they expect us to do, and what we expect from them, taking us through the clerkship sessions (FGD 4 Participant 1).
Students’ exposure to cases in other wards poses significant challenges; the study found that as some lecturers facilitate visits to different wards for scheduled teaching sessions, others don’t, resulting in missed learning opportunities. Additionally, some lecturers leave the burden on students’ personal initiative to explore cases in other wards.
We actually encourage them to go through the different specialties because when you are faced with a patient, you will not have to choose which one to see and not to see (KII 4).
Imagine landing on a stroke patient when you have been in the infectious disease ward or getting a patient with renal condition when you have been in the endocrinology ward can create problems (FGD 6 Participant 3).
Theme 2 Case presentation
Medical students present their long case to lecturers and postgraduate students. However, participants revealed variations among lecturers regarding their preferences on how they want students to present their cases. While some prefer to listen to the entire history and examination, others prefer only a summary, and some prefer starting from the diagnosis.
The practice varies depending on the lecturer, as everyone does it their own way. There are some, who listen to your history, examination, and diagnosis, and then they go into basic discussion of the case; others want only a summary. Some lecturers come and tell you to start straight away from your diagnosis, and then they start treating you backward (FGD 6 Participant 3).
The students reported limited observation of their skills due a little emphasis placed by examiners on physical examination techniques, as well as not providing the students with the opportunity to propose treatment plans.
When we are doing these physical examinations on the ward no one is seeing you. You present your physical examination findings, but no one saw how you did it. You may think you are doing the right thing during the ward rotations, but actually your skills are bad (FGD 4 Participant 6).
They don’t give us time to propose management plans. The only time they ask for how you manage a patient is during the summative long case, yet during the ward rotation, they were not giving us the freedom to give our opinion on how we would manage the patient.(FGD 2Participant 6).
Supervision was reportedly dependent on the ward to which the student was allocated. Additionally, the participants believe that the large student-to-lecturer ratio negatively affects the opportunity to present.
My experience was different in years three and five. In year three, we had a specialist every day on the ward, but in year five, we would have a specialist every other day, sometimes even once a week. When I compare year five with year three, I think I was even a better doctor in year three than right now (FGD 1 Participant 1).
Clinical training is like nurturing somebody to behave or conduct themselves in a certain way. Therefore, if the numbers are large, the impacts per person decrease, and the quality decreases (KII 5).
Theme C: Case assessment and feedback
The study found that a student’s long case is assessed both during the case presentation on the ward and through the case write-up, with marks awarded accordingly.
They present to the supervisor and then also write it up, so at a later time you also mark the sheet where they have written up the cases; so they are assessed at presentation and write up (KII 2).
The mark awarded was reportedly a significant motivator for students to visit wards and clerk patients, but students also believe that the pressure to hunt for marks tends to override the goal of the formative assessment.
Your goal there is to learn, but most of us go with the goal of getting signatures; signature-based learning. The learning, you realize probably comes on later if you have the individual morale to go and learn (FGD 1 participant 1).
Feedback is an integral part of any formative assessment. While students receive feedback from lecturers, the participants were concerned about the absence of a formal channel for soliciting feedback from students.
Of course, teachers provide feedback to students because it is a normal part of teaching. However, it is not a common routine to solicit feedback about how teaching has gone. So maybe that is something that needs to be improved so that we know if we have been effective teachers (KII 3).
Whereas the feedback intrigues students to read more to compensate for their knowledge gap, they decried several encounters with demeaning, intimidating, insulting, demotivating, and embarrassing feedback from assessors.
Since we are given a specific target of case presentation we are supposed to make in my training , if I make the ten, I wouldn’t want to present again. Why would I receive other negative comments for nothing? They truly have a personality effect on the student, and students feel low self-esteem (FGD 1, Participant 4).
This study aimed to investigate the experiences of medical students and faculty regarding the use of the long case as a formative assessment method at a tertiary care teaching hospital in a low-resource setting. This qualitative research provides valuable insights into the current practices surrounding the long case as a formative assessment method in such a setting.
The study highlighted the patient bedside as the primary learning environment for medical students. Bedside teaching plays a crucial role in fostering the development of skills such as history-taking and physical examination, as well as modeling professional behaviors and directly observing learners [ 14 , 15 ]. However, the specialization of wards in tertiary hospitals means that students may not be exposed to certain conditions found in other wards. This lack of exposure can lead to issues of case specificity, which has been reported in various literature as a cause of low reliability and generalizability of the long case [ 16 , 17 ]. Participants in the study expressed feeling like pseudo-specialists based on their ward allocations. This is partly attributed to missing scheduled teachings and poor management of opportunities to clerk and present patients on other wards. Addressing these challenges is essential for enhancing the effectiveness of the long case as a formative assessment method in medical education.
Proper orientation at the beginning of a clerkship is crucial for clarifying the structure and organization, defining students’ roles, and providing insights into clinical supervisors’ perspectives [ 18 ]. However, the study revealed that orientation into the long case was unsatisfactory, resulting in time wastage and potentially hindering learning. Effective orientation requires dedicated time and should involve defining expectations and goals, as well as guiding students through the steps of history-taking and physical examination during the initial weeks of the rotation. Contrary to this ideal approach, the medical students reported being taken through systemic examinations when the clerkship was nearing its end, highlighting a significant gap in the orientation process. Proper orientation is very important since previous studies have also documented the positive impact of orientation on student performance [ 19 ]. Therefore, addressing the shortcomings in orientation practices identified in this study is essential for optimizing learning outcomes and ensuring that students are adequately prepared to engage in the long case.
There was reportedly a significant variation in the way students present their long cases, with some lecturers preferring only a case summary, while others expect a complete presentation or begin with a diagnosis. While this diversity in learning styles may expose students to both familiar and unfamiliar approaches, providing a balance of comfort and tension [ 20 ], it’s essential for students to first be exposed to familiar methods before transitioning to less familiar ones to expand their ability to use diverse learning styles. The variation observed in this context may be attributed to time constraints, as lecturers may aim to accommodate the large number of students within the available time. Additionally, a lack of standardized practices could also contribute to this variation. Therefore, there is a pressing need for standardized long-case practices to ensure a consistent experience for students and to meet the desired goals of the assessment. Standardizing the long case practice would not only provide a uniform experience for students but also enhance the reliability, validity, and perception of fairness of the assessment [ 9 , 21 ]. It would ensure that all students are evaluated using the same criteria, reducing potential biases and disparities in grading. Additionally, standardized practices facilitate better alignment with learning objectives and promote more effective feedback mechanisms [ 22 ].
Related to the above, students reported limited observation of skills and little emphasis placed on them to learn physical examination techniques. This finding resonates with the research conducted by Abdalla and Shorbagi in 2018, where many students reported a lack of observation during history-taking and physical examination [ 23 ]. The importance of observation is underscored by the fact that students often avoid conducting physical examinations, as highlighted in Pavlakis & Laurent’s study among postgraduate trainees in 2001 [ 24 ]. This study sheds more light on the critical role of observation in forcing medical students to master clinical assessment and practical skills. The study also uncovered that students are rarely given the opportunity to propose management plans during case presentations, which hampers their confidence and learning of clinical decision-making. These findings likely stem from the large student-to-lecturer ratio and little attention given to these aspects of the long case during the planning of the assessment method. The result is students not receiving the necessary guidance and support to develop their clinical and decision-making skills. Therefore, addressing these issues by putting more emphasis on observation of student-patient interaction, management plan, and having a smaller student group is vital to ensure that medical students receive comprehensive training and are adequately prepared for their future roles as physicians.
The study found that the marks awarded for the long case serve as the primary motivator for students. This finding aligns with previous research indicating that the knowledge that each long case is part of assessment drives students to perform their duties diligently [ 2 , 25 ]. It underscores the crucial role that assessment plays in driving learning processes. However, the pressures to obtain marks and signatures reportedly hinder students’ engagement in learning. This could be attributed to instances where some lecturers relax on supervision or are absent, leaving students to struggle to find someone to assess them. Inadequate supervision by attending physicians has been identified in prior studies as one of the causes of insufficient clinical experience [ 26 ], something that need to be dealt with diligently. While the marks awarded are a motivating factor, it is essential to understand other underlying motivations of medical students to engage in the long case and their impact on the learning process.
Feedback is crucial for the long case to fulfill its role as an assessment for learning. The study participants reported that feedback is provided promptly as students present their cases. This immediate feedback is essential for identifying errors and learning appropriate skills to enhance subsequent performance. However, the feedback process appears to be unilateral, with students receiving feedback from lecturers but lacking a structured mechanism for providing feedback themselves. One reason for the lack of student feedback may be a perceived intimidating approach from lecturers which discourages students from offering their input. It is thus important to establish a conducive environment where students feel comfortable providing feedback without fear of negative repercussions. The study underscores the significance of feedback from students in improving the learning process. This aligns with the findings of Hattie and Timperley (2007), who emphasized that feedback received from learners contributes significantly to improvements in student learning [ 27 ]. Therefore, it is essential to implement strategies to encourage and facilitate bidirectional feedback between students and lecturers in the context of the long case assessment. This could involve creating formal channels for students to provide feedback anonymously or in a structured format, fostering open communication, and addressing any perceived barriers to feedback exchange [ 28 ]. By promoting a culture of feedback reciprocity, educators can enhance the effectiveness of the long case as an assessment tool.
Conclusions
In conclusion, the long case remains a cornerstone of formative assessment during clerkship in many medical schools, particularly in low-resource countries. However, its effectiveness is challenged by limitations such as case specificity in tertiary care hospitals, which can affect the assessment’s reliability and generalizability. The practice of awarding marks in formative assessment serves as a strong motivator for students but also creates tension, especially when there is inadequate contact with lecturers. This can lead to a focus on hunting for marks at the expense of genuine learning. Thus adequate supervision and feedback practices are vital for ensuring the success of the long case as an assessment for learning.
Furthermore, there is a need to foster standardized long case practice to ensure that scheduled learning activities are completed and that all students clerk and present patients with different conditions from various wards. This will promote accountability among both lecturers and students and ensure a consistent and uniform experience with the long case as an assessment for learning, regardless of the ward a student is assigned.
Data availability
The data supporting the study results of this article can be accessed from the Makerere University repository, titled “Perceptions of Medical Students and Lecturers of the Long Case Practices as Formative Assessment in Internal Medicine Clerkship at Makerere University,” available on DSpace. The identifier is http://hdl.handle.net/10570/13032 . Additionally, the raw data are securely stored with the researchers in Google Drive.
Dare AJ, Cardinal A, Kolbe J, Bagg W. What can the history tell us? An argument for observed history-taking in the trainee intern long case assessment. N Z Med J. 2008;121 1282:51–7.
Google Scholar
Tey C, Chiavaroli N, Ryan A. Perceived educational impact of the medical student long case: a qualitative study. BMC Med Educ. 2020;20(1):1–9.
Article Google Scholar
Jayasinghe R. Mastering the Medical Long Case. Elsevier Health Sciences; 2009.
Martinerie L, Rasoaherinomenjanahary F, Ronot M, Fournier P, Dousset B, Tesnière A, Mariette C, Gaujoux S, Gronnier C. Health care simulation in developing countries and low-resource situations. J Continuing Educ Health Professions. 2018;38(3):205–12.
van der Vleuten C. Making the best of the long case. Lancet (London England). 1996;347(9003):704–5.
Reeves TC, Okey JR. Alternative assessment for constructivist learning environments. Constructivist Learn Environments: Case Stud Instructional Des. 1996;191:202.
Biggs J. What the student does: teaching for enhanced learning. High Educ Res Dev. 1999;18(1):141.
Michael A, Rao R, Goel V. The long case: a case for revival? Psychiatrist. 2013;37(12):377–81.
Benning T, Broadhurst M. The long case is dead–long live the long case: loss of the MRCPsych long case and holism in psychiatry. Psychiatr Bull. 2007;31(12):441–2.
Burn W, Brittlebank A. The long case: the case against its revival: Commentary on… the long case. Psychiatrist. 2013;37(12):382–3.
Norcini JJ. The death of the long case? Bmj 2002;324(7334):408–9.
Pell G, Roberts T. Setting standards for student assessment. Int J Res Method Educ. 2006;29(1):91–103.
Masih CS, Benson C. The long case as a formative Assessment Tool–views of medical students. Ulster Med J. 2019;88(2):124.
Peters M, Ten Cate O. Bedside teaching in medical education: a literature review. Perspect Med Educ. 2014;3(2):76–88.
Wölfel T, Beltermann E, Lottspeich C, Vietz E, Fischer MR, Schmidmaier R. Medical ward round competence in internal medicine–an interview study towards an interprofessional development of an Entrustable Professional Activity (EPA). BMC Med Educ. 2016;16(1):1–10.
Wilkinson TJ, Campbell PJ, Judd SJ. Reliability of the long case. Med Educ. 2008;42(9):887–93.
Sood R. Long case examination-can it be improved. J Indian Acad Clin Med. 2001;2(4):252–5.
Atherley AE, Hambleton IR, Unwin N, George C, Lashley PM, Taylor CG. Exploring the transition of undergraduate medical students into a clinical clerkship using organizational socialization theory. Perspect Med Educ. 2016;5:78–87.
Owusu GA, Tawiah MA, Sena-Kpeglo C, Onyame JT. Orientation impact on performance of undergraduate students in University of Cape Coast (Ghana). Int J Educational Adm Policy Stud. 2014;6(7):131–40.
Vaughn L, Baker R. Teaching in the medical setting: balancing teaching styles, learning styles and teaching methods. Med Teach. 2001;23(6):610–2.
Olson CJ, Rolfe I, Hensley. The effect of a structured question grid on the validity and perceived fairness of a medical long case assessment. Med Educ. 2000;34(1):46–52.
Jensen-Doss A, Hawley KM. Understanding barriers to evidence-based assessment: clinician attitudes toward standardized assessment tools. J Clin Child Adolesc Psychol. 2010;39(6):885–96.
Abdalla ME, Shorbagi S. Challenges faced by medical students during their first clerkship training: a cross-sectional study from a medical school in the Middle East. J Taibah Univ Med Sci. 2018;13(4):390–4.
Pavlakis N, Laurent R. Role of the observed long case in postgraduate medical training. Intern Med J. 2001;31(9):523–8.
Teoh NC, Bowden FJ. The case for resurrecting the long case. BMJ. 2008;336(7655):1250–1250.
Mulindwa F, Andia I, McLaughlin K, Kabata P, Baluku J, Kalyesubula R, Kagimu M, Ocama P. A quality improvement project assessing a new mode of lecture delivery to improve postgraduate clinical exposure time in the Department of Internal Medicine, Makerere University, Uganda. BMJ Open Qual. 2022;11(2):e001101.
Hattie J, Timperley H. The power of feedback. Rev Educ Res. 2007;77(1):81–112.
Weallans J, Roberts C, Hamilton S, Parker S. Guidance for providing effective feedback in clinical supervision in postgraduate medical education: a systematic review. Postgrad Med J. 2022;98(1156):138–49.
Download references
Acknowledgements
Not applicable.
This research was supported by the Fogarty International Centre of the National Institute of Health under award number 1R25TW011213. The content is solely the responsibility of the author and does not necessarily represent the official views of the National Institute of Health.
Author information
Authors and affiliations.
School of Medicine, Department of Paediatrics & Child Health, Makerere University, Kampala, Uganda
Jacob Kumakech
School of Biomedical Sciences, Department of Anatomy, Makerere University, Kampala, Uganda
Ian Guyton Munabi
School of Medicine, Department of Radiology, Makerere University, Kampala, Uganda
Aloysius Gonzaga Mubuuke
School of Medicine, Department of Pediatrics & Child Health, Makerere University, Kampala, Uganda
Sarah Kiguli
You can also search for this author in PubMed Google Scholar
Contributions
JK contributed to the conception and design of the study, as well as the acquisition, analysis, and interpretation of the data. He also drafted the initial version of the work and approved the submitted version. He agrees to be personally accountable for his contribution and to ensure that any questions related to the accuracy or integrity of any part of the work, even those in which he was not personally involved, are appropriately investigated and resolved, with the resolution documented in the literature.IMG contributed to the analysis and interpretation of the data. He also made major corrections to the first draft of the manuscript and approved the submitted version. He agrees to be personally accountable for his contribution and to ensure that any questions related to the accuracy or integrity of any part of the work, even those in which he was not personally involved, are appropriately investigated and resolved, with the resolution documented in the literature.MA contributed to the analysis and interpretation of the data. He made major corrections to the first draft of the manuscript and approved the submitted version. He agrees to be personally accountable for his contribution and to ensure that any questions related to the accuracy or integrity of any part of the work, even those in which he was not personally involved, are appropriately investigated and resolved, with the resolution documented in the literature.SK made major corrections to the first draft and the final corrections for the submitted version of the work. She agrees to be personally accountable for her contribution and to ensure that any questions related to the accuracy or integrity of any part of the work, even those in which she was not personally involved, are appropriately investigated and resolved, with the resolution documented in the literature.
Corresponding author
Correspondence to Jacob Kumakech .
Ethics declarations
Ethical approval.
Ethical approval to conduct the study was obtained from the Makerere University School of Medicine Research and Ethics Committee, with ethics ID Mak-SOMREC-2022-524. Informed consent was obtained from all participants using the Mak-SOMREC informed consent form.
Consent for publication
Competing interests.
The authors declare no competing interests.
Additional information
Publisher’s note.
Springer Nature remains neutral with regard to jurisdictional claims in published maps and institutional affiliations.
Rights and permissions
Open Access This article is licensed under a Creative Commons Attribution 4.0 International License, which permits use, sharing, adaptation, distribution and reproduction in any medium or format, as long as you give appropriate credit to the original author(s) and the source, provide a link to the Creative Commons licence, and indicate if changes were made. The images or other third party material in this article are included in the article’s Creative Commons licence, unless indicated otherwise in a credit line to the material. If material is not included in the article’s Creative Commons licence and your intended use is not permitted by statutory regulation or exceeds the permitted use, you will need to obtain permission directly from the copyright holder. To view a copy of this licence, visit http://creativecommons.org/licenses/by/4.0/ . The Creative Commons Public Domain Dedication waiver ( http://creativecommons.org/publicdomain/zero/1.0/ ) applies to the data made available in this article, unless otherwise stated in a credit line to the data.
Reprints and permissions
About this article
Cite this article.
Kumakech, J., Munabi, I.G., Mubuuke, A.G. et al. Experiences of medical students and faculty regarding the use of long case as a formative assessment method at a tertiary care teaching hospital in a low resource setting: a qualitative study. BMC Med Educ 24 , 621 (2024). https://doi.org/10.1186/s12909-024-05589-7
Download citation
Received : 04 April 2024
Accepted : 22 May 2024
Published : 05 June 2024
DOI : https://doi.org/10.1186/s12909-024-05589-7
Share this article
Anyone you share the following link with will be able to read this content:
Sorry, a shareable link is not currently available for this article.
Provided by the Springer Nature SharedIt content-sharing initiative
- Formative assessment
- Medical education
- Low-resource setting
BMC Medical Education
ISSN: 1472-6920
- Submission enquiries: [email protected]
- General enquiries: [email protected]
- Study protocol
- Open access
- Published: 04 June 2024
Understanding the processes underpinning IMPlementing IMProved Asthma self-management as RouTine (IMP 2 ART) in primary care: study protocol for a process evaluation within a cluster randomised controlled implementation trial
- J. Sheringham ORCID: orcid.org/0000-0003-3468-129X 1 ,
- L. Steed 2 ,
- K. McClatchey 3 ,
- B. Delaney 4 ,
- A. Barat 2 ,
- V. Hammersley 5 ,
- V. Marsh 5 ,
- N. J. Fulop 1 ,
- S. J. C. Taylor 2 &
- H. Pinnock 5
Trials volume 25 , Article number: 359 ( 2024 ) Cite this article
Metrics details
Providing supported self-management for people with asthma can reduce the burden on patients, health services and wider society. Implementation, however, remains poor in routine clinical practice. IMPlementing IMProved Asthma self-management as RouTine (IMP 2 ART) is a UK-wide cluster randomised implementation trial that aims to test the impact of a whole-systems implementation strategy, embedding supported asthma self-management in primary care compared with usual care. To maximise opportunities for sustainable implementation beyond the trial, it is necessary to understand how and why the IMP 2 ART trial achieved its clinical and implementation outcomes.
A mixed-methods process evaluation nested within the IMP 2 ART trial will be undertaken to understand how supported self-management was implemented (or not) by primary care practices, to aid interpretation of trial findings and to inform scaling up and sustainability. Data and analysis strategies have been informed by mid-range and programme-level theory. Quantitative data will be collected across all practices to describe practice context, IMP 2 ART delivery (including fidelity and adaption) and practice response. Case studies undertaken in three to six sites, supplemented by additional interviews with practice staff and stakeholders, will be undertaken to gain an in-depth understanding of the interaction of practice context, delivery, and response. Synthesis, informed by theory, will combine analyses of both qualitative and quantitative data. Finally, implications for the scale up of asthma self-management implementation strategies to other practices in the UK will be explored through workshops with stakeholders.
This mixed-methods, theoretically informed, process evaluation seeks to provide insights into the delivery and response to a whole-systems approach to the implementation of supported self-management in asthma care in primary care. It is underway at a time of significant change in primary care in the UK. The methods have, therefore, been developed to be adaptable to this changing context and to capture the impact of these changes on the delivery and response to research and implementation processes.
Peer Review reports
Asthma places a major burden on patients, health services and wider society. Providing self-management education to people with asthma, supported by a personalised action plan and regular review, can reduce this burden, by preventing unscheduled healthcare use and improving asthma control [ 1 ]. Several studies have demonstrated that the implementation of supported self-management in primary care, however, remains low [ 2 ]. Enhancing the implementation of supported self-management in primary care requires a whole-systems approach—i.e. a combination of patient education, professional training and organisational support [ 3 ].
IMP 2 ART is a whole-systems, evidence-based implementation strategy developed to help primary care practices to implement supported self-management for asthma patients [ 4 , 5 , 6 , 7 , 8 ]. Evaluations of such implementation strategies are complex and require consideration of the clinical effectiveness, the implementation success and the process by which such outcomes are achieved. Process evaluations play a particularly crucial role in this understanding. They unpick the ‘black box’ of interventions by understanding who received what, how and the process through which it impacted (or not) outcomes and inform potential mechanisms for sustainability. There is plentiful guidance that process evaluations should use mid-range theory in their design and delivery [ 9 ]. There is increasing recognition that process evaluations could and should seek to develop these mid-range theories to improve the design and evaluation of future implementation studies [ 10 ].
This paper describes the protocol for the process evaluation taking place alongside a cluster randomised trial of the IMPlementing IMProved Asthma self-management as RouTine (IMP 2 ART) strategy in UK primary care practices [ref: ISRCTN15448074]. It sets out how we seek to measure the delivery and response to IMP 2 ART, how we seek to understand the trial’s effectiveness findings and how it may contribute to the development of theory.
IMP 2 ART cluster randomised trial
IMP 2 ART is a UK-wide cluster randomised implementation trial that aims to test the impact of a whole-systems implementation strategy embedding supported asthma self-management in primary care compared with usual care on both clinical and implementation outcomes. The main trial protocol and the IMP 2 ART strategy are described in McClatchey et al. [ 5 ].
Programme theory
IMP 2 ART combined the mid-range implementation and behaviour change theories of iPARIHS [ 9 ] and capability, opportunity, and motivation required for behaviour change (COM-B) [ 11 ] to develop a programme-level theory of how IMP 2 ART can support practices to implement supported self-management in asthma [ 12 ]. The programme theory states the central hypothesis of IMP 2 ART, i.e. that facilitation plays a critical role in implementation success. Facilitation encompasses both the inputs of a trained facilitator and the delivery of IMP 2 ART implementation strategies. Facilitation is expected to achieve its impacts through increasing staff capability, motivation and opportunity towards supported self-management. It is also expected that tailoring by facilitators to take account of practice context, particularly capacity, culture and leadership will be an important aspect of their interactions with practices. Moreover, we expect that the relationship between the facilitation and practice response to IMP 2 ART will be influenced by practice context.
IMP 2 ART strategies
The IMP 2 ART strategies comprise multiple components directed at patients, professionals and the organisation, supported by expert nurse facilitation for 12 months, summarised in a logic model (Fig. 1 ) and described in greater detail in McClatchey et al. (2023) [ 5 ].
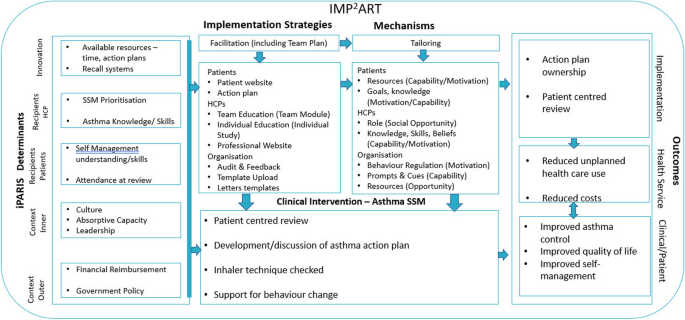
IMP2ART’s logic mode [ 12 ]. Facilitation through trained specialist asthma nurses acts as a catalyst for the use of MP2ART strategies in practices, which in turn aims to optimise professionals’ capacity, motivation and opportunity to deliver supported self-management to patients with asthma. The nature of the delivery of IMP2ART and the response to facilitation and the IMP2ART strategies will vary between practices and will be strongly influenced through practice context
While all strategies will be made available to all implementation practices, it is expected that practices will choose which IMP 2 ART strategies they use and how they adapt them to their own context. In all implementation practices, the following ‘core’ strategies will be delivered: facilitation and ongoing support from a facilitator (12 months), monthly audit and feedback reports highlighting practice-supported self-management performance (e.g. number of action plans provided) [ 4 ], and access to education modules (a team education module to highlight the whole team role in supported self-management; an individual module for clinicians delivering asthma reviews) [ 5 ] and access to a ‘Living with Asthma’ website with practice and patient resources [ 8 ].
The cluster randomised controlled trial takes place in England and Scotland with a target recruitment of 144 practices.
An internal pilot of IMP 2 ART was conducted in 2021 with the first 12 practices recruited to the trial, primarily to optimise trial design as the feasibility of the components of the implementation strategy had already been tested and refined [ 4 , 5 , 6 ]. The pilot also provided an opportunity to test and refine some of the bespoke data collection and analysis approaches for the process evaluation [ 13 ].
Aims and objectives
The IMP 2 ART process evaluation has two primary aims:
To explain the IMP 2 ART trial’s clinical and implementation outcome findings
To identify learning, in relation to IMP 2 ART outcomes, to inform the design, scaling up and sustainability of implementation strategies to improve supported self-management of asthma in primary care
The evaluation is structured to achieve five interrelated objectives, with associated research questions, see Table 1 .
The process evaluation is based on the Medical Research Council guidelines for the process evaluation of complex interventions [ 14 ], also drawing on Grant et al.’s process evaluation framework for cluster randomised trials [ 15 ]. Aligned with the Medical Research Council recommendations for the evaluation at different stages of development, an internal pilot process evaluation has already been conducted and will be reported elsewhere [ 13 ]. The process evaluation incorporates learning from the pilot and focuses on three key dimensions: implementation strategy delivery (including fidelity and adaptation), practice response and practice context.
As shown in Fig. 2 , we will primarily use quantitative analysis to achieve objectives 1 and 2 and an in-depth qualitative approach to achieve objective 3, culminating in a mixed-methods synthesis supported by additional interviews to achieve objectives 4 and 5. In line with Fetters et al.’s description of an interactive approach, we will iterate data collection and analysis through the use of interim (formative) analyses and discussion of emerging findings during the process evaluation [ 16 ]. We will adopt a critical realist perspective, which is in keeping with our aim to derive causative explanations for IMP 2 ART’s findings, whilst acknowledging that we can only capture aspects of reality [ 17 ].
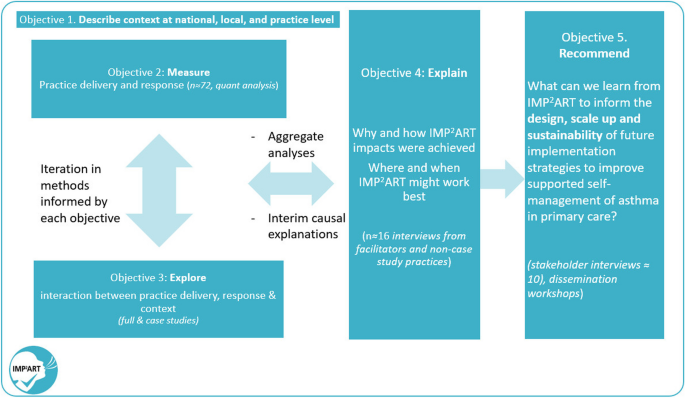
Relationship between objectives in IMP2ART’s process evaluation
There are no specific reporting guidelines for process evaluations, so we have drawn on suggestions from Moore et al. [ 14 ] StaRI guidelines for reporting implementation studies [ 18 ] and used the TRIPLE C reporting principles for case study evaluations as a guide to the case study element [ 19 ].
Data collection and collation
The process evaluation will use quantitative data from all 144 recruited practices (control and implementation), with a focus on the practices allocated to the implementation arm. A subset of practices will be invited to take part in further qualitative data collection, as case studies or as one-off interviews. Additional qualitative interview data will be collected from IMP 2 ART’s facilitators and national stakeholders, described in more detail below.
Data for the process evaluation comes from a range of sources, which are summarised in Fig. 3 , and described in more detail below. Table 2 summarises their links to our research questions.
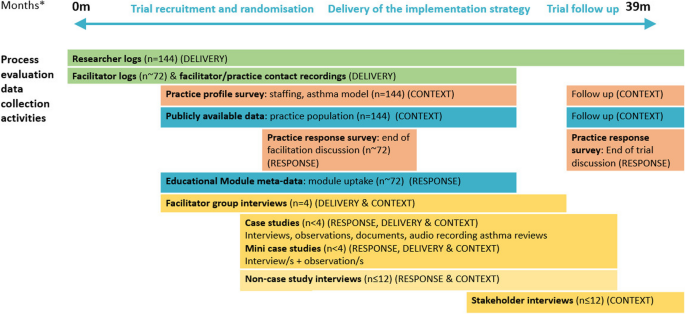
IMP2ART process evaluation data collection timeline. *Months refer to IMP2ART trial duration. All practices will participate in the trial for 24 months. Practices assigned to the implementation arm will receive 12 months of active facilitation and 12 months of follow-up
Researcher logs
Researchers will keep detailed notes about all practices approached, practices showing interest and the proportion who agree to participate. Where available, reasons for participation and non-participation will be noted to inform the potential for scaling up ( aim 2 ). Reasons for withdrawal (if available) will be noted. For those practices randomised to the trial, researchers will continue to log contacts and delivery of IMP2ART tools such as audit and feedback reports sent and templates uploaded.
Facilitator logs and facilitator/practice contact recordings
Facilitators will keep logs of all practice contacts, including duration and nature of contact (e.g. email, phone or video call). The practice introductory workshop and the end of the facilitation meeting, both conducted via video calls, will be video recorded, and data from the call (duration, attendees and comments) will be downloaded. In addition, the facilitator will complete an observation form shortly after to structure impressions of the practice.
Publicly available data and practice profile survey (n = 144)
Details on all trial practices (control and implementation) will be obtained where possible from publicly available sources at the start of the trial on practice characteristics (e.g. population size, ethnicity, deprivation), supplemented with a survey of all practices to obtain information on their model of asthma management. In line with StaRI, we will examine both initial and changes in context [ 18 ]. To obtain changes in the practice context, key details of this profile will be reviewed in discussion with practices at the end of the trial meeting.
Educational online module usage data (n ~ 72)
Practice completion of the online educational modules will be logged automatically. We will store data on logins and completion of both modules at the practice level. The use of the team module will be recorded for each practice as it is designed to be used and discussed in groups.
Practice response survey (n ~ 72)
We will collect self-reported implementation of IMP 2 ART strategies at 12 months (end of facilitation) and 24 months (end of trial). We have drawn on Proctor et al.’s taxonomy of implementation outcomes to identify different aspects of implementation relevant to IMP 2 ART and will focus on different outcomes at different points during the trial, e.g. acceptability and adoption in initial measures, adaptation at the mid-point and sustainability towards the end of the trial [ 21 ].
Facilitator group interviews (n < 8)
IMP 2 ART’s four trained asthma nurse facilitators will be interviewed as a group at four points in the trial. Interviews will focus on their evolving experiences and learning as they progress from delivering their initial IMP 2 ART workshops and becoming experienced facilitators to their experiences of ending the facilitation process with practices. These interviews will serve a formative purpose, to provide an alert of any problems in the delivery of IMP 2 ART and to inform data collection strategies (e.g. to highlight practices that have engaged in IMP 2 ART in specific ways for non-case study interviews). They will also serve a summative purpose in providing insights into the IMP 2 ART delivery, particularly on the evolving interaction between delivery and practice context.
Full case studies (n ≤ 4) and mini-case studies (n ≤ 4)
We will seek to explore from multiple perspectives how IMP 2 ART fits with a practice’s culture and routines, absorptive capacity and leadership over the 2 years in which practices participate in the trial.
A case study methodology, as described by Yin, is applicable where an in-depth investigation of a contemporary phenomenon is needed within a real-life context and where the boundaries between context and the phenomenon are not clear [ 22 ]. This fits with the fact that context and intervention are intentionally very closely interlinked in IMP 2 ART because the strategies have been designed to be adapted in response to practice context.
We have designed flexibility into our case study methodology, recognising that general practices are under significant pressure and may not be able to commit to 2 years of data collection. We have therefore developed a mini-case study adaptation of our full method, as a bridge between one-off interviews and full case studies.
Case study selection: From the implementation group practices, we will approach case studies to try and reflect diversity in baseline asthma management and population characteristics.
Data collection: For full case studies, we will seek to collect several sources of bespoke data at key intervals in the practice’s participation in the trial (Table 2 ).
Interviews ( n ≤ 12/case study) with key actors will be conducted at early, mid- and later stages of the trial to track how implementation changes and changes in practice context and to explore the potential for sustainability. Key actors may be individuals who deliver supported self-management in the practice (usually nurses, but may also be healthcare assistants, pharmacists, GPs); people in an administrative role who contribute to, or might be affected by, the implementation of supported self-management (e.g. practice managers, prescribing clerks, receptionists); people making decisions about self-management (e.g. GP partners, practice managers); and lay members of a practice user group if they are involved in the IMP 2 ART initiative (see example topic guide, Supplementary data file 1).
These will often be repeat interviews with the same individuals (or sometimes new individuals who have taken over a role of a previous participant). Researchers will tailor topic guides to the stage of the practice’s participation in IMP 2 ART, i.e. focusing on context and expectations in early interviews and asking for reflections on IMP 2 ART and sustainability at later interviews.
Observation of activities ( n ≤ 20 h/case), e.g. training sessions, practice meetings, facilitator visits, shadowing practice staff and routine review consultations. Observation field notes will focus on the practice context, the processes by which practices implement self-management and evidence of adopting/adapting the IMP. 2 ART implementation strategy (see example observation guide, Supplementary data file 1)
Documentary analysis ( n ≤ 40/case), e.g. anonymised personalised asthma action plans, meeting minutes, asthma review procedures and policies
Audio-recording of asthma clinics n > 3 asthma clinics/case study practice
For mini-case studies, we will seek at least one face-to-face interview and an observation and draw on data already collected at other trial time points (e.g. end-of-facilitation meeting). Data collection will be influenced by the stage at which the practice has reached by the time of recruitment (Table 3 ).
Data collection tools are based on the IMP 2 ART programme theory, reflecting particularly the mid-range i-PARIHS and COM-B frameworks. Each tool is designed to be tailored to ensure data gathering is aligned with participants’ roles and the stage of trial participation (see Supplementary data file 1 for examples of topic guides and observation tools).
Non-case study interviews (n ≤ 12)
Up to 12 non-case study interviews will be undertaken informed by preliminary findings from other data sources (e.g. facilitator feedback) and learning from prior IMP 2 ART research [ 23 ], so that we can explore the applicability of our emerging themes in a range of contexts. From the pool of non-case study practices, we will recruit a key informant (GP, nurse or practice manager) able to discuss the implementation of supported self-management in their practice in a semi-structured interview. Practices will be selected because they offer a contrasting context to our case study practices, use novel approaches to implementation, or are outliers in terms of outcomes/processes (e.g. where facilitator notes suggest very low or very high engagement with IMP 2 ART or exhibit innovative adaption). Interviews will be informed by the ongoing process analysis and will seek views on emerging themes.
Stakeholder interviews (n ≤ 10)
We will arrange focussed interviews with stakeholders to explore the generalisability of emerging themes and/or policy perspectives. These may represent national or regional opinion leaders in asthma care or healthcare management with whom we can test out emerging themes. They may also include IMP 2 ART collaborators who can give a view on the feasibility and value of embedding IMP 2 ART approaches beyond the trial if they are found to be effective.
Data management and analysis
Many of the sources will include data that could be analysed qualitatively and quantitatively. For example, the researcher logs will include dates of key activities for all practices, but for some practices, there will also be researcher field notes (e.g. practice feedback). Key variables will be extracted from the data sources and analysed quantitatively for all practices. We will also select data to be analysed qualitatively alongside case study and additional interview data to supplement the case studies or where data provide evidence that contributes to the programme theory.
Quantitative
Data management.
The main quantitative analysis of IMP 2 ART will be conducted at the practice level so a core dataset will be formed at the practice level from all the sources and imported into Excel.
Quantitative descriptive analysis will be conducted on data from all implementation practices to answer our objective 1 research questions related to IMP 2 ART delivery, practice response and summary practice characteristics.
Fidelity and adaptation
The concept of fidelity has been variably defined and interpreted [ 24 ]. In some conceptualisations, fidelity is synonymous with adherence, with maximising adherence being a goal of intervention delivery [ 25 ]. In implementation research, however, adaptation of a strategy—changes in its content, format or delivery—to align the innovation with important characteristics of local context is often critical [ 26 ]. This is reflected in the StaRI guidelines which recommend reporting of both fidelity (in terms of core strategies to be delivered) and also adaptations made [ 18 ]. In IMP 2 ART, we therefore measure both fidelity of delivery for core strategies, whilst also seeking to capture adaptations and the rationale for these.
The dimensions of fidelity measured relate to the five recommended key domains of the NIH Behaviour Change Consortium (BCC) Treatment Fidelity Framework—treatment design, training, delivery, receipt and enactment [ 27 ] (with a focus on the delivery of IMP 2 ART), receipt (practice response to IMP 2 ART) and enactment (delivery of supported self-management with patients).
To allow for a more in-depth understanding of the delivery of facilitation and its potential mechanisms of action, a sub-sample of video-recorded introductory facilitation and end-of-facilitation workshops will be coded to understand the activities and processes of facilitation used. A study-specific tool has been developed for this purpose. A sample of at least 10% ( n = 7) of practices will be selected at random stratified to ensure at least one workshop per facilitator. In addition, all workshops from case studies will be coded [ 28 ]. Each workshop will be independently coded by two individuals following training to ensure consistency in the application of the tool.
Qualitative
All interviews will be audio-recorded, transcribed verbatim and anonymised. Researchers will take structured field notes for all case study observations which will be stored in a central repository. The research team will work together to build consistency in the process of both data collection and analysis. Activities to enhance consistency include all researchers watching and writing field notes on an initial IMP 2 ART workshop recording from a case study site and then discussing the similarities and differences in their observations.
As advised by Yin for explanatory case studies [ 22 ], our analytic strategy for both case study data and the additional interviews will be in part guided by the theoretical propositions we have already set out, and in part inductive, guided by the data [ 12 ]. These propositions have informed the data analysis tools we have developed to organise and redescribe the data for each case. In line with critical realist theory, we also recognise the fallibility of theory and remain open to drawing on other theories or frameworks to support data interpretation [ 17 ].
Mid-range and programme theories have guided an initial coding framework, which we will iterate following researcher discussions in light of the data (see Fig. 4 , stage 1). We will use the coded data to identify themes, and with reference to evidence from quantitative data sources (see mixed methods synthesis), we will produce both standardised descriptions of each case and a timeline. These documents will be used for cross-case comparisons to identify similarities and differences between cases (see Fig. 4 , stages 2–3). As shown in step 4, wider interviews will be coded using the initial coding framework, with refinements carried out as described in step 1. The coding of these interviews will be used to identify further themes.
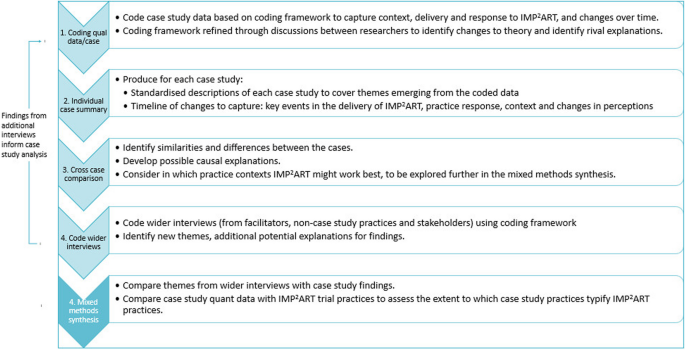
Analytical stages for qualitative and mixed methods analysis
Mixed methods synthesis and interpretation
In the mixed methods synthesis, we will seek to address objectives 4 and 5 providing explanation for results and making recommendations for future practice.
We will integrate quantitative data analysis (objectives 1 and 2), case study findings (objective 3) and the themes from additional interview data from group interviews with IMP 2 ART’s four facilitators; non-case study practice staff ( n ≤ 12); and wider stakeholders ( n ≤ 10). We will use these data to interrogate and refine the explanations derived from the case studies about which practice contexts and how IMP 2 ART might work best (objective 4).
As shown in Figs. 1 and 4 , we will conduct the analysis iteratively through frequent sharing of interim analyses, to enable us to draw on learning from each data source to refine the analysis and interpretation of the data as a whole. For example, the qualitative analysis may help us select variables to examine quantitative associations between contextual characteristics and delivery/response to IMP 2 ART.
We will use integrated analysis to produce a narrative synthesis across all the data sources that critically assess the evidence for the initial programme theory [ 12 ]. If possible, we will look to understand the mechanisms and moderators that enable the use of the IMP 2 ART implementation strategies to increase supported self-management of asthma in general practice.
Preliminary findings will be shared at three end-of-programme workshops in each of the main research sites (London, Sheffield and Lothian) to share preliminary findings from the IMP 2 ART programme, gauge the application of our findings to a broader range of contexts and explore the potential for scale-up and transferability of the findings to other practices in England and Scotland.
This protocol describes a nested process evaluation to aid the interpretation of the IMP 2 ART trial results and—in its own right—seeks to provide insights into the delivery and response to a whole-systems approach to the implementation of supported self-management in asthma care in general practice.
Methodological considerations
This process evaluation has been informed by a comprehensive pilot evaluation across the first twelve practices to participate in the trial and substantial research on strategies to implement supported self-management in primary care [ 7 , 23 , 29 ]. The pilot experience in IMP 2 ART indicated that the implementation strategy was feasible and acceptable and enabled testing and refinement of core elements of the process evaluation methods [ 13 ]. It is important to note that conditions in pilots may be different from those in the main trial [ 20 ], which necessitates exploration of some of the pilot themes with a wider sample. By conducting the process evaluation in parallel with the trial, there is the potential for interim process evaluation findings to influence peripheral content and delivery to maximise the chance of a successful outcome [ 28 ].
The inclusion of a multisite case study within the process evaluation is also now more widely recognised as a significant opportunity to provide evidence about context and transferability, and also to support elucidating causal inferences, particularly in trials like IMP 2 ART where the pathway from intervention delivery to impact is likely to be non-linear [ 28 ].
Limitations and challenges
IMP 2 ART is taking place at a time of considerable change and uncertainty in general practice. This uncertainty may affect both practice engagement with the implementation strategy and their participation in the process evaluation data collection. We have sought to minimise the burden for practices through using publicly available data where possible to describe characteristics and routine data for many of the measurements. Whilst maintaining consistency, we have also sought to make the process evaluation methods flexible so we can adapt to changing circumstances. For example, we have introduced an adapted case study design in recognition that many IMP 2 ART practices are not able to commit to longitudinal case studies over the entire 2 years of their trial participation. Whilst this may reduce the depth of our case studies, it will enable us to explore a wider range of contexts. We have also conducted an interim analysis of process evaluation data to identify where our methods need additional iteration.
The process evaluation is highly complex, both in terms of its combinations of theory and in its involvement of a large multidisciplinary research team split across multiple sites. Additional complexity can arise because of the differences in philosophical perspectives across the different disciplines contributing to IMP 2 ART. In order to build consistency across a large team working with these multiple perspectives, we have worked to create common understandings of all the theoretical perspectives on which IMP 2 ART is drawn [ 12 ]. We have also worked to maximise alignments between philosophical and methodological perspectives. For example, we recognise the possible philosophical misalignment between a trial, which implicitly takes a positivist perspective, and the process evaluation’s critical realist perspective, which considers that research can, at best, only capture a small part of reality [ 17 ]. Our choice of a critical realist approach, however, allows us to recognise the relevance of the trial’s findings to the reality of primary care practice and recommends the use of theory as a way of getting closer to identifying causal explanations for the trial’s findings.
It is not feasible to examine every process or outcome to the same depth. Our focus in the process evaluation is on IMP 2 ART’s main focus, helping general practices to implement supported self-management. It means, however, that while trial outcomes will be at the patient level, the process evaluation has limited opportunities to understand the impacts of IMP 2 ART on patients. We will seek to address this gap through some future additional linked projects, for example, to interview patients in some of our sites (Supplementary data file 2).
Study status
This protocol of the process evaluation is version 2.0. Version 1 was approved by ethics in 2019. Significant changes to the process evaluation since version 1 include the addition of adapted—mini-case studies—alongside full case studies.
Recruitment for the process evaluation began in July 2022. At the time of protocol submission (January 2024), the process evaluation was part way through the recruitment of case study sites (4/6 recruited) and had not started recruitment of non-case study interviews. Data collection is anticipated to be complete by 31 December 2024.
Availability of data and materials
Availability of data will depend on the data source. Due to the confidentiality of NHS routine data, trial and other data extracted from practices will not be made available. Other quantitative data may be made available via the University of Edinburgh DataShare if the practices are non-identifiable. By its nature, it will not be possible to anonymise the qualitative interview data for public availability. Requests for secondary use should be directed to the trial manager.
Abbreviations
Capability, Opportunity, Motivation – as determinants of Behaviour
General practitioner
Health Service and Delivery Research
IMPlementing IMProved Asthma self-management as RouTine
Integrated-Promoting Action on Research Implementation in Health Services
National Institute for Health Research
National Health Service
Randomised controlled trial
Research Ethics Committee
United Kingdom
Pinnock H, Parke HL, Panagioti M, Daines L, Pearce G, Epiphaniou E, Bower P, Sheikh A, Griffiths CJ, Taylor SJC, et al. Systematic meta-review of supported self-management for asthma: a healthcare perspective. BMC Med. 2017;15(1):64. https://doi.org/10.1186/s12916-017-0823-7 .
Article PubMed PubMed Central Google Scholar
Wiener-Ogilvie S, Pinnock H, Huby G, Sheikh A, Partridge MR, Gillies J. Do practices comply with key recommendations of the British Asthma Guideline? If not, why not? Prim Care Respir J. 2007;16(6):369–77. https://doi.org/10.3132/pcrj.2007.00074 .
Taylor SJC, Pinnock H, Epiphaniou E, Pearce G, Parke H. A rapid synthesis of the evidence on interventions supporting self-management for people with long-term conditions. (PRISMS Practical Systematic Review of Self-Management Support for long-term conditions) Health Serv Deliv Res. 2014;2:54.
McClatchey K, Sheldon A, Steed L, Sheringham J, Holmes S, Preston M, Appiagyei F, Price D, Taylor SJC, Pinnock H, et al. Development of theoretically informed audit and feedback: an exemplar from a complex implementation strategy to improve asthma self-management in UK primary care. J Eval Clin Pract. 2023. https://doi.org/10.1111/jep.13895 .
Article PubMed Google Scholar
McClatchey K, Marsh V, Steed L, Holmes S, Taylor SJC, Wiener-Ogilvie S, Neal J, Last R, Saxon A, Pinnock H, et al. Developing a theoretically informed education programme within the context of a complex implementation strategy in UK primary care: an exemplar from the IMP2ART trial. Trials. 2022;23(1):350. https://doi.org/10.1186/s13063-022-06147-6 .
McClatchey K, Sheldon A, Steed L, Sheringham J, Appiagyei F, Price D, Hammersley V, Taylor S, Pinnock H. Development of a patient-centred electronic review template to support self-management in primary care: a mixed-methods study. BJGP Open 2023, 7 (2), BJGPO.2022.0165. https://doi.org/10.3399/bjgpo.2022.0165 .
Morrissey M, Shepherd E, Kinley E, McClatchey K, Pinnock H. Effectiveness and perceptions of using templates in long-term condition reviews: a systematic synthesis of quantitative and qualitative studies. Br J Gen Pract. 2021;71(710):e652–9. https://doi.org/10.3399/bjgp.2020.0963 .
Harvey G, Kitson A. PARIHS revisited: from heuristic to integrated framework for the successful implementation of knowledge into practice. Implement Sci. 2016;11:33. https://doi.org/10.1186/s13012-016-0398-2 .
Kislov R, Pope C, Martin GP, Wilson PM. Harnessing the power of theorising in implementation science. Implement Sci. 2019;14(1):103. https://doi.org/10.1186/s13012-019-0957-4 .
Michie S, van Stralen MM, West R. The behaviour change wheel: a new method for characterising and designing behaviour change interventions. Implement Sci. 2011;6:42. https://doi.org/10.1186/1748-5908-6-42 .
Steed L, Sheringham J, McClatchey K, Hammersley V, Marsh V, Morgan N, Jackson T, Holmes S, Taylor S, Pinnock H. IMP(2)ART: development of a multi-level programme theory integrating the COM-B model and the iPARIHS framework, to enhance implementation of supported self-management of asthma in primary care. Implement Sci Commun. 2023;4(1):136. https://doi.org/10.1186/s43058-023-00515-2 .
McClatchey K, Sheringham J, Barat A, Delaney B, Searle B, Marsh V, Hammersley V, Taylor S, Pinnock H. IMPlementing IMProved Asthma self-management as RouTine (IMP2ART) in primary care: internal pilot for a cluster randomised controlled trial. Eur Respir J. 2022;60(suppl 66):394. https://doi.org/10.1183/13993003.congress-2022.394 .
Article Google Scholar
Moore GF, Audrey S, Barker M, Bond L, Bonell C, Hardeman W, Moore L, O’Cathain A, Tinati T, Wight D, et al. Process evaluation of complex interventions: Medical Research Council guidance. BMJ 2015, 350. https://doi.org/10.1136/bmj.h1258 .
Grant A, Treweek S, Dreischulte T, Foy R, Guthrie B. Process evaluations for cluster-randomised trials of complex interventions: a proposed framework for design and reporting. Trials. 2013;14:15. https://doi.org/10.1186/1745-6215-14-15 .
Fetters MD, Curry LA, Creswell JW. Achieving integration in mixed methods designs-principles and practices. Health Serv Res. 2013;48(6 Pt 2):2134–56. https://doi.org/10.1111/1475-6773.12117 .
Pinnock H, Barwick M, Carpenter CR, Eldridge S, Grandes G, Griffiths CJ, Rycroft-Malone J, Meissner P, Murray E, Patel A, et al. Standards for Reporting Implementation Studies (StaRI) Statement. BMJ 2017, 356. https://doi.org/10.1136/bmj.i6795 .
Shaw SE, Paparini S, Murdoch J, Green J, Greenhalgh T, Hanckel B, James HM, Petticrew M, Wood GW, Papoutsi C. TRIPLE C reporting principles for case study evaluations of the role of context in complex interventions. BMC Med Res Methodol. 2023;23(1):115. https://doi.org/10.1186/s12874-023-01888-7 .
Proctor E, Silmere H, Raghavan R, Hovmand P, Aarons G, Bunger A, Griffey R, Hensley M. Outcomes for implementation research: conceptual distinctions, measurement challenges, and research agenda. Adm Policy Ment Health. 2011;38(2):65–76. https://doi.org/10.1007/s10488-010-0319-7 .
Yin RK. Case study research and applications; Thousand Oaks. California: SAGE Publications Inc; 2018.
Google Scholar
Fingertips: Public Health Data - National General Practice Profiles. Office for Health Improvement and Disparities. https://fingertips.phe.org.uk/profile/general-practice . Accessed 4 Jan 2024.
Morrow S, Daines L, Wiener-Ogilvie S, Steed L, McKee L, Caress A-L, Taylor SJC, Pinnock H. Exploring the perspectives of clinical professionals and support staff on implementing supported self-management for asthma in UK general practice: an IMP2ART qualitative study. Primary Care Respiratory Medicine. 2017;27(1):45. https://doi.org/10.1038/s41533-017-0041-y .
Carroll C, Patterson M, Wood S, Booth A, Rick J, Balain S. A conceptual framework for implementation fidelity. Implement Sci. 2007;2(1):40. https://doi.org/10.1186/1748-5908-2-40 .
Borrelli B. The assessment, monitoring, and enhancement of treatment fidelity in public health clinical trials. J Public Health Dent. 2011;71(s1):S52-s63. https://doi.org/10.1111/j.1752-7325.2011.00233.x .
McCleary N, Andrews A, Buelo A, Captieux M, Morrow S, Wiener-Ogilvie S, Fletcher M, Steed L, Taylor SJC, Pinnock H. IMP2ART systematic review of education for healthcare professionals implementing supported self-management for asthma. npj Primary Care Respiratory Medicine 2018, 28 (1), 42. https://doi.org/10.1038/s41533-018-0108-4 .
Moore GF, Evans RE, Hawkins J, Littlecott H, Melendez-Torres GJ, Bonell C, Murphy S. From complex social interventions to interventions in complex social systems: future directions and unresolved questions for intervention development and evaluation. Evaluation. 2019;25(1):23–45. https://doi.org/10.1177/1356389018803219 .
Grant A, Bugge C, Wells M. Designing process evaluations using case study to explore the context of complex interventions evaluated in trials. Trials. 2020;21(1):982. https://doi.org/10.1186/s13063-020-04880-4 .
McIntyre SA, Francis JJ, Gould NJ, Lorencatto F. The use of theory in process evaluations conducted alongside randomized trials of implementation interventions: a systematic review. Translational Behavioral Medicine. 2018;10(1):168–78. https://doi.org/10.1093/tbm/iby110(acccessed6/28/2022) .
Paparini S, Papoutsi C, Murdoch J, Green J, Petticrew M, Greenhalgh T, Shaw SE. Evaluating complex interventions in context: systematic, meta-narrative review of case study approaches. BMC Med Res Methodol. 2021;21(1):225. https://doi.org/10.1186/s12874-021-01418-3 .
Fletcher AJ. Applying critical realism in qualitative research: methodology meets method. Int J Soc Res Methodol. 2017;20(2):181–94. https://doi.org/10.1080/13645579.2016.1144401 .
Download references
Acknowledgements
We are grateful for the contributions of the Asthma UK Centre for Applied Research patient panel and IMP 2 ART Programme Group for their input into the design of the process evaluation by providing feedback on plans for the process evaluation as it developed.
The National Institute for Health and Care Research (NIHR) Programme Grants for Applied Research (reference number RP-PG-1016–20008). JS and ST are supported by NIHR ARC North Thames. The views expressed are those of the authors and not necessarily those of the NIHR or the Department of Health and Social Care. The funder had no role in the design of this study and will not have any role during its execution, analyses, interpretation of the data or decision to publish. The Asthma UK Centre for Applied Research (reference Asthma UK: AC-2012–01) funded some pre-grant work on the theoretical development of the implementation strategy. Education for Health developed the education modules, and Optimum Patient Care developed the templates and audit and feedback components of the implementation strategy.
Author information
Authors and affiliations.
Institute of Epidemiology and Health Care, UCL, London, WC1E 6BT, UK
J. Sheringham & N. J. Fulop
Wolfson Institute of Population Health, Queen Mary University of London, London, UK
L. Steed, A. Barat & S. J. C. Taylor
University of Dundee, Dundee, UK
K. McClatchey
School of Health and Related Research, The University of Sheffield, Sheffield, UK
Asthma UK Centre for Applied Research, Usher Institute, University of Edinburgh, Edinburgh, UK
V. Hammersley, V. Marsh & H. Pinnock
You can also search for this author in PubMed Google Scholar
Contributions
HP, SJCT, KM, VM, VH, NJF, JS and LS contributed to the design of the study. JS drafted the manuscript. LS, HP, SJCT, KM, VH, VM, NJF, AB and BD contributed to the drafts of the manuscript. All authors read, commented on and approved the final manuscript.
Corresponding author
Correspondence to J. Sheringham .
Ethics declarations
Consent for publication.
Not applicable. This protocol contains no baseline or pilot data.
Competing interests
The authors declare that they have no competing interests.
Additional information
Publisher’s note.
Springer Nature remains neutral with regard to jurisdictional claims in published maps and institutional affiliations.
Supplementary Information
13063_2024_8179_moesm1_esm.docx.
Additional file 1. Data collection tools. a. Practice staff interview schedule (case study – early). b. General observation form (case study). c. Post-workshop facilitator observation form.
13063_2024_8179_MOESM2_ESM.docx
Additional file 2. Examples of potential allied process evaluation projects to enable additional exploration of IMP2ART’s delivery, response and context.
Rights and permissions
Open Access This article is licensed under a Creative Commons Attribution 4.0 International License, which permits use, sharing, adaptation, distribution and reproduction in any medium or format, as long as you give appropriate credit to the original author(s) and the source, provide a link to the Creative Commons licence, and indicate if changes were made. The images or other third party material in this article are included in the article's Creative Commons licence, unless indicated otherwise in a credit line to the material. If material is not included in the article's Creative Commons licence and your intended use is not permitted by statutory regulation or exceeds the permitted use, you will need to obtain permission directly from the copyright holder. To view a copy of this licence, visit http://creativecommons.org/licenses/by/4.0/ . The Creative Commons Public Domain Dedication waiver ( http://creativecommons.org/publicdomain/zero/1.0/ ) applies to the data made available in this article, unless otherwise stated in a credit line to the data.
Reprints and permissions
About this article
Cite this article.
Sheringham, J., Steed, L., McClatchey, K. et al. Understanding the processes underpinning IMPlementing IMProved Asthma self-management as RouTine (IMP 2 ART) in primary care: study protocol for a process evaluation within a cluster randomised controlled implementation trial. Trials 25 , 359 (2024). https://doi.org/10.1186/s13063-024-08179-6
Download citation
Received : 11 February 2024
Accepted : 16 May 2024
Published : 04 June 2024
DOI : https://doi.org/10.1186/s13063-024-08179-6
Share this article
Anyone you share the following link with will be able to read this content:
Sorry, a shareable link is not currently available for this article.
Provided by the Springer Nature SharedIt content-sharing initiative
- Process evaluation
- Implementation
- Primary care
- Self-management
ISSN: 1745-6215
- Submission enquiries: Access here and click Contact Us
- General enquiries: [email protected]

IMAGES
VIDEO
COMMENTS
A case study is one of the most commonly used methodologies of social research. This article attempts to look into the various dimensions of a case study research strategy, the different epistemological strands which determine the particular case study type and approach adopted in the field, discusses the factors which can enhance the effectiveness of a case study research, and the debate ...
Themes generation and coding is the most recognized data analysis method in qualitative empirical material. The authors interpreted the raw data for case studies with the help of a four-step interpretation process (PESI).
Furthermore, the ability to describe in detail how the analysis was conducted ensures rigour in reporting qualitative research. Data sources: The research example used is a multiple case study that explored the role of the clinical skills laboratory in preparing students for the real world of practice. Data analysis was conducted using a ...
Case studies play a significant role in knowledge development across various disciplines. Analysis of cases provides an avenue for researchers to explore phenomena within their context based on the collected data. Analysis of qualitative data from case study research can contribute to knowledge development.
The types of qualitative research included: 24 case studies, 19 generic qualitative studies, and eight phenomenological studies. Notably, about half of the articles reported analyzing their qualitative data via content analysis and a constant comparative method, which was also commonly referred to as a grounded theory approach and/or inductive ...
Step 1: Gather your qualitative data and conduct research (Conduct qualitative research) The first step of qualitative research is to do data collection. Put simply, data collection is gathering all of your data for analysis. A common situation is when qualitative data is spread across various sources.
Revised on November 20, 2023. A case study is a detailed study of a specific subject, such as a person, group, place, event, organization, or phenomenon. Case studies are commonly used in social, educational, clinical, and business research. A case study research design usually involves qualitative methods, but quantitative methods are ...
McMaster University, West Hamilton, Ontario, Canada. Qualitative case study methodology prov ides tools for researchers to study. complex phenomena within their contexts. When the approach is ...
The purpose of case study research is twofold: (1) to provide descriptive information and (2) to suggest theoretical relevance. Rich description enables an in-depth or sharpened understanding of the case. It is unique given one characteristic: case studies draw from more than one data source. Case studies are inherently multimodal or mixed ...
Qualitative case study methodology is an appropriate strategy for exploring phenomena such as lived experiences, events, and the contexts in which they occur (Houghton et al. 2014;Miles and ...
The specific strategies for analysis in these stages centred on the work of Miles and Huberman ( 1994 ), which has been successfully used in case study research. The data were managed using NVivo software. Review methods Literature examining qualitative data analysis was reviewed and strategies illustrated by the case study example provided.
As case study research is a flexible research method, qualitative data analysis methods are commonly used [176]. The basic objective of the analysis is, as in any other analysis, to derive conclusions from the data, keeping a clear chain of evidence.
Aims of Qualitative Data Analysis The analysis of qualitative data can have several aims. The first aim may be to describe a phenomenon in some or greater detail. The phenomenon can be the subjective experi-ences of a specific individual or group (e.g. the way people continue to live after a fatal diagnosis). This can focus on the case (indi-
According to the book Understanding Case Study Research, case studies are "small scale research with meaning" that generally involve the following: The study of a particular case, or a number of cases. That the case will be complex and bounded. That it will be studied in its context. That the analysis undertaken will seek to be holistic.
After describing qualitative data and strategies for analysis, this chapter examines five broad classifications of designs: case study, phenomenological, ethnographic, narrative, and mixed methods. These designs require complex collection of data as sources of evidence for claims about the meaning of the data.
Definitions of qualitative case study research. Case study research is an investigation and analysis of a single or collective case, intended to capture the complexity of the object of study (Stake, 1995).Qualitative case study research, as described by Stake (), draws together "naturalistic, holistic, ethnographic, phenomenological, and biographic research methods" in a bricoleur design ...
Further, often the contribution of a qualitative case study research (QCSR) emerges from the 'extension of a theory' or 'developing deeper understanding—fresh meaning of a phenomenon'. ... Thematic analysis of qualitative data: AMEE Guide No. 131. Medical Teacher. Crossref. PubMed. Google Scholar. Ryan G. W., & Bernard H. R. (2003 ...
A Case study is: An in-depth research design that primarily uses a qualitative methodology but sometimes includes quantitative methodology. Used to examine an identifiable problem confirmed through research. Used to investigate an individual, group of people, organization, or event. Used to mostly answer "how" and "why" questions.
propose an approach to the analysis of case study data by logically linking the data to a series of propositions and then interpreting the subsequent information. Like the Yin (1994) strategy, the Miles and Huberman (1994) process of analysis of case study data, although quite detailed, may still be insufficient to guide the novice researcher.
Qualitative secondary analysis (QSA) is the use of qualitative data collected by someone else or to answer a different research question. Secondary analysis of qualitative data provides an opportunity to maximize data utility particularly with difficult to reach patient populations. However, QSA methods require careful consideration and ...
Assess if a case study approach suits your research questions. This approach works well when you have distinct cases with defined boundaries and aim to deeply understand these cases or compare multiple cases. ... which focuses on numerical data and statistical analysis, qualitative research prioritizes understanding the depth and richness of ...
Data sources The research example used is a multiple case study that explored the role of the clinical skills laboratory in preparing students for the real world of practice. Data analysis was conducted using a framework guided by the four stages of analysis outlined by Morse ( 1994 ): comprehending, synthesising, theorising and recontextualising.
How to Write Case Study Analysis 1. Analyzing the Data Collected. ... Use qualitative and quantitative methods to ensure a comprehensive analysis. Validate the data's accuracy and relevance to the subject. Look for correlations and causations that can provide deeper insights. 2. Identifying Key Issues and Problems
Therefore, the purpose of this paper is to provide a concise explanation of four common qualitative approaches, case study, ethnography, narrative, and phenomenology, demonstrating how each approach is linked to specific types of data collection and analysis. ... Qualitative data analysis: conceptual and practical considerations. Health ...
Study context. This qualitative study was conducted in the province of British Columbia (BC), Canada, a jurisdiction with a population of approximately five million people [].Within BC's health sector, key actors involved in the policy response to COVID-19 included: elected officials, the BC Government's Ministry of Health (MOH), the Provincial Health Services Authority (PHSA), Footnote 2 ...
The qualitative data were analyzed by inductive thematic analysis. Three themes emerged from the study: ward placement, case presentation, and case assessment and feedback. The findings revealed that students conduct their long cases at patients' bedside within specific wards/units assigned for the entire clerkship.
Case studies undertaken in three to six sites, supplemented by additional interviews with practice staff and stakeholders, will be undertaken to gain an in-depth understanding of the interaction of practice context, delivery, and response. Synthesis, informed by theory, will combine analyses of both qualitative and quantitative data.
The following key attributes of the case study methodology can be underlined. 1. Case study is a research strategy, and not just a method/technique/process of data collection. 2. A case study involves a detailed study of the concerned unit of analysis within its natural setting. A de-contextualised study has no relevance in a case study ...
We conducted a qualitative study as part of the mixed-methods implementation evaluation of the SC4C trial. We collected data through virtual and in-person focus groups at the general practices and phone, virtual and in-person interviews. Data was analysed using an iterative hybrid inductive-deductive thematic analysis.
A qualitative-descriptive lens, as outlined by Sandelowski 27,28 provided a useful approach for analysis of the in-depth interviews. 29 Using a text matrix (constructed simply with word-processing software) allowed analysis of each participant by data source (interview, case presentation, hub recommendations) and of participants from one to ...