
Dementia UK: Second Edition - Overview
- Health Service & Population Research
- NIHR Maudsley Biomedical Research Centre (BRC)
- LSE London School of Economics & Political Science
Research output : Book/Report › Commissioned report
Original language | English |
---|---|
Publisher | |
Number of pages | 61 |
Publication status | Published - 10 Sept 2014 |
Other files and links
- publisher's website link
Fingerprint
- Dementia Medicine and Dentistry 100%
- Total Number Mathematics 100%
- Total Cost Mathematics 100%
- Worst Case Mathematics 100%
- Standards Computer Science 100%
- Current Projection Computer Science 100%
- Prevalence Medicine and Dentistry 54%
- Health Care Cost Medicine and Dentistry 36%
T1 - Dementia UK: Second Edition - Overview
AU - Prince, Martin
AU - Knapp, Martin
AU - Guerchet, Maelenn
AU - McCrone, Paul
AU - Prina, Matthew
AU - Comas-Herrera, A
AU - Wittenberg, Raphael
AU - Adelaja, Bayo
AU - Hu, Bo
AU - King, Derek
AU - Rehill, Amritpal
AU - Salimkumar, Dhanya
PY - 2014/9/10
Y1 - 2014/9/10
N2 - This overview document provides an update on the figures presented in the first edition of Dementia UK (Alzheimer’s Society, 2007). It is an overview of the full report, Dementia UK: Second edition, which will be published by Alzheimer’s Society in autumn 2014. This document presents the best available evidence for the current cost and prevalence of dementia in the UK.The full report was commissioned by Alzheimer’s Society. It was researched and written by King’s College London and London School of Economics in 2014.Some of the key findings from the report are:• The total age-standardised 65+ population prevalence of dementia is 7.1% (based on 2013 data).• This equals one in every 79 (1.3%) of the entire UK population, and 1 in every 14 of the population aged 65 years and over.• At the current estimated rate of prevalence, there will be 850,000 people with dementia in the UK in 2015.• Compared to the 2007 estimates, the current prevalence consensus estimates are slightly higher for the youngest (65 to 69) and oldest (90+) age bands and slightly lower for the intermediate age groups (80–89).• The total number of people with dementia in the UK would increase to over 1 million by 2025 and over 2 million by 2051 if age-specific prevalence remains stable, and increases are only driven by demographic ageing.• This is a worst-case scenario. Improvements to education standards, cardiovascular health, activity levels and other known risk factors may all help reduce dementia incidence and prevalence in the future. However, available research in this area is not sufficient to allow us to forecast this in our current projections.• There are over 40,000 people with early-onset dementia (under the age of 65 years) in the UK.• The total cost of dementia to society in the UK is £26.3 billion, with an average cost of£32, 250 per person.• £4.3 billion is spent on healthcare costs.• £10.3 billion is spent on social care (publicly and privately funded).• £11.6 billion is contributed by the work of unpaid carers of people with dementia.
AB - This overview document provides an update on the figures presented in the first edition of Dementia UK (Alzheimer’s Society, 2007). It is an overview of the full report, Dementia UK: Second edition, which will be published by Alzheimer’s Society in autumn 2014. This document presents the best available evidence for the current cost and prevalence of dementia in the UK.The full report was commissioned by Alzheimer’s Society. It was researched and written by King’s College London and London School of Economics in 2014.Some of the key findings from the report are:• The total age-standardised 65+ population prevalence of dementia is 7.1% (based on 2013 data).• This equals one in every 79 (1.3%) of the entire UK population, and 1 in every 14 of the population aged 65 years and over.• At the current estimated rate of prevalence, there will be 850,000 people with dementia in the UK in 2015.• Compared to the 2007 estimates, the current prevalence consensus estimates are slightly higher for the youngest (65 to 69) and oldest (90+) age bands and slightly lower for the intermediate age groups (80–89).• The total number of people with dementia in the UK would increase to over 1 million by 2025 and over 2 million by 2051 if age-specific prevalence remains stable, and increases are only driven by demographic ageing.• This is a worst-case scenario. Improvements to education standards, cardiovascular health, activity levels and other known risk factors may all help reduce dementia incidence and prevalence in the future. However, available research in this area is not sufficient to allow us to forecast this in our current projections.• There are over 40,000 people with early-onset dementia (under the age of 65 years) in the UK.• The total cost of dementia to society in the UK is £26.3 billion, with an average cost of£32, 250 per person.• £4.3 billion is spent on healthcare costs.• £10.3 billion is spent on social care (publicly and privately funded).• £11.6 billion is contributed by the work of unpaid carers of people with dementia.
UR - http://www.alzheimers.org.uk/dementiauk
M3 - Commissioned report
BT - Dementia UK: Second Edition - Overview
PB - Alzheimer's Society
Dementia: policy, services and statistics overview
- Research Briefing
- Community care
- Health services
- Elizabeth Parkin
- Tim Jarrett
This briefing examines policies in England to improve dementia diagnosis, care, support and research. Health is a devolved matter, and the note also briefly outlines dementia strategies in Scotland, Wales and Northern Ireland. It also provides statistics on rates of dementia.
Documents to download
Dementia: policy, services and statistics overview (344 KB , PDF)
There are an estimated 850,000 people with dementia in the UK. By 2040, the number of people with the condition is expected to double.
The UK Government has set an objective for England to be a world leader in fighting dementia and has committed to improving diagnosis, care and support, and research.
The Challenge on Dementia 2020 , published in February 2015, set out what the UK Government wanted to achieve by 2020 in order for England to be:
- the best country in the world for dementia care and support and for people with dementia, their carers and families to live
- the best place in the world to undertake research into dementia and other neurodegenerative diseases
Some of the key objectives of the Challenge were:
- Equal access to diagnosis for everyone
- GPs playing a lead role in ensuring coordination and continuity of care for people with dementia
- Every person diagnosed with dementia having meaningful care following their diagnosis
- All NHS staff having received training on dementia appropriate to their role.
The Challenge on Dementia 2020 also contained the commitment to spend £300 million on dementia research over the five years to March 2020. This commitment was delivered a year early with £344 million spent on dementia research over the four years to 31 March 2019. [1]
The NHS Long Term Plan , published on 7 January 2019, also commits the NHS in England to continuing to improve the care provided to people with dementia and their carers.
The Government has said that it aims to bring forward proposals for a new strategy later in 2021, which will set out plans for dementia care, support, awareness, and research in England. The Government is also currently working on ways to significantly boost further research on dementia including medical and care interventions. [2]
A separate Library briefing is available on The effect of the covid-19 outbreak on people affected by dementia (November 2020).
[1] PQ 144985 [on Dementia: Research], 3 February 2021
[2] PQ 171711 [on Dementia: Research], 25 March 2021
Share this with
- Facebook Share this with Facebook
- Twitter Share this with Twitter
- LinkedIn Share this with LinkedIn
- Email Share this with Email
Related posts
Nhs dentistry in england.
An overview of NHS dentistry in England, including a discussion of current challenges facing providers and the Government response.
NHS strike action in England
Strikes have been taking place across the NHS in England. This briefing looks at when and why some NHS workers are striking and explains the latest pay deals.
Key Employment Rights
This briefing provides an overview of a range of key statutory employment rights in Great Britain, including unfair dismissal, deduction from wages and working time.
- Work and incomes
Europe PMC requires Javascript to function effectively.
Either your web browser doesn't support Javascript or it is currently turned off. In the latter case, please turn on Javascript support in your web browser and reload this page.
![ARUK-FOR-A-CURE logo Core-colours-RGB[1] alzheimer's research uk for a cure logo](https://www.alzheimersresearchuk.org/wp-content/uploads/2023/05/ARUK-FOR-A-CURE-logo-Core-colours-RGB1-1024x192.png)
Policy reports

Tackling Inequalities in Dementia Risk
October 2023 Health inequalities in the UK are damaging brain health, reducing life expectancy, and incurring significant costs – to individuals and society. These disparities are avoidable, unjust, and on the rise.

Tipping Point: The Future of Dementia
September 2023
Our new report sets out a series of calls for party leaders ahead of the next general election. We believe these recommendations are vital to tackle the increasing pressure that dementia places on society, the NHS, and the economy.

Investing in dementia research boosts UK economy
If nothing changes, one in two of us will be directly affected by dementia, either by developing the condition ourselves, caring for someone, or both.

Addressing value and affordability
Following a workshop with stakeholders from the NHS, the National Institute for Health and Care Excellence (NICE), industry and academia, we examine the challenges and opportunities in assessing the value and affordability of new Alzheimer’s medicines.

Establishing a Clinical Trials Network
The creation of a ‘clinical trials network’ has huge potential to dramatically change the landscape for dementia research in the UK. Here, we set out our recommendations for how such a network could operate and deliver successful outcomes.

A joint statement on the voluntary scheme for branded medicines pricing and access (VPAS)
Patient charities set out a shared vision for the next VPAS scheme.

Improving dementia diagnosis: what could change in primary care?
November 2022
The search for life-changing dementia treatments is beginning to yield tangible results. Now is the time for primary care to adapt to diagnosing people at the earliest stages of dementia, when treatments are most likely to be effective.

Five steps to delivering life-changing treatments for dementia
August 2022
Alzheimer’s Research UK is calling on the new Prime Minister to follow five key steps to ensure the UK can be a world leader in dementia clinical research and help deliver life-changing treatments.

The Impact of Dementia on Women
Dementia devastates the lives of everyone it touches, but our analysis shows that women are bearing a disproportionate weight of the impact.

Dementia Attitudes Monitor: Wave 2 (2021)
September 2021 Wave 2 builds on findings from Wave 1 (2018), shining a light on the UK’s attitudes towards dementia.

Are we ready to deliver disease modifying treatments?
Old Age Psychiatrists’ views on diagnosing and treating Alzheimer’s disease before dementia

The Right to Know: Accurate and Earlier Diagnosis of Dementia
Our vision of how to progressively reform and build dementia diagnostics capability in the UK.

Translating Science into breakthroughs: the future of late-stage dementia clinical trials in the UK
People with dementia are at risk of missing out on new treatments if the UK does not improve how it hosts clinical trials, according to a new report from Alzheimer’s Research UK.

Brain Health: A new way to think about dementia risk reduction
January 2021
How we can use ‘brain health’ to transform the public’s attitude towards dementia risk reduction.

Delivering Dementia – Plan to find life-changing treatments
January 2020
Alzheimer’s Research UK has called on government to invest in six priority research areas, which will help to deliver a life-changing treatment for dementia.

Detecting and diagnosing Alzheimer’s disease
December 2019
Our report reveals public attitudes to early diagnosis of Alzheimer’s.

Dementia Attitudes Monitor: Wave 1 (2018)
January 2019
A comprehensive review of public attitudes towards dementia and research, identifying key misperceptions and opportunities for shaping public attitudes towards the condition.

No time to lose: An action plan for dementia
September 2018
The five actions government can take to improve the lives of people with dementia.

Brain Health consensus statement
Driving progress in dementia risk reduction by rallying health stakeholders around ‘brain health’.

Thinking Differently: preparing today to implement future dementia treatments
Analysis of how future dementia treatments will impact our health system and the taskforce we’re launching to overcome the unique challenges these treatments present.
- Next »
Privacy overview
Cookie | Duration | Description |
---|---|---|
__adal_ca | 5 months 27 days | Adalyser sets this cookie to stores which advertising campaign drove a user to visit, stores traffic source and campaign data. |
__adal_cw | 7 days | Adalyser sets this cookie to tie back conversion events to earlier visits and stores a visit timestamp. |
__adal_id | 1 year 1 month 4 days | Adalyser stores the Device ID generated by this cookie as a unique identifier for the device. |
__adal_ses | 30 minutes | Adalyser sets this cookie to determine whether there is an active session and which conversions have taken place in this session to prevent duplicates, stores a list of events in this session. |
_fbp | 3 months | This cookie is set by Facebook to deliver advertisement when they are on Facebook or a digital platform powered by Facebook advertising after visiting this website. |
_opt_expid | less than a minute | Set by Google Analytics, this cookie is created when running a redirect experiment. It stores the experiment ID, the variant ID and the referrer to the page that is being redirected. |
_pin_unauth | 1 year | Pinterest set this cookie to group actions for users who cannot be identified. |
ai_user | 1 year | Microsoft Azure sets this cookie as a unique user identifier cookie, enabling counting of the number of users accessing the application over time. |
B | 1 year | This Cookie is used by Yahoo to provide ads, contents or analytics. |
bscookie | 2 years | This cookie is a browser ID cookie set by Linked share Buttons and ad tags. |
fr | 3 months | The cookie is set by Facebook to show relevant advertisments to the users and measure and improve the advertisements. The cookie also tracks the behavior of the user across the web on sites that have Facebook pixel or Facebook social plugin. |
guest_id | 1 year 1 month 4 days | Twitter sets this cookie to identify and track the website visitor. It registers if a user is signed in to the Twitter platform and collects information about ad preferences. |
guest_id_ads | 1 year 1 month 4 days | Twitter sets this cookie to identify and track the website visitor. |
guest_id_marketing | 1 year 1 month 4 days | Twitter sets this cookie to identify and track the website visitor. |
id | 1 month | The main purpose of this cookie is targeting and advertising. It is used to create a profile of the user''s interest and to show relevant ads on their site. This Cookie is set by DoubleClick which is owned by Google. |
IDE | 1 year 24 days | Used by Google DoubleClick and stores information about how the user uses the website and any other advertisement before visiting the website. This is used to present users with ads that are relevant to them according to the user profile. |
li_sugr | 3 months | LinkedIn sets this cookie to collect user behaviour data to optimise the website and make advertisements on the website more relevant. |
muc_ads | 1 year 1 month 4 days | Twitter sets this cookie to collect user behaviour and interaction data to optimize the website. |
MUID | 1 year 24 days | Bing sets this cookie to recognise unique web browsers visiting Microsoft sites. This cookie is used for advertising, site analytics, and other operations. |
NID | 6 months | Google sets the cookie for advertising purposes; to limit the number of times the user sees an ad, to unwanted mute ads, and to measure the effectiveness of ads. |
personalization_id | 1 year 1 month 4 days | Twitter sets this cookie to integrate and share features for social media and also store information about how the user uses the website, for tracking and targeting. |
rl_anonymous_id | never | RudderStack set this cookie to store statistical data of users' behaviour on the website, which can be used for internal analytics by the website operator. |
rl_group_id | never | RudderStack sets this cookie to collect user activity on the web. |
rl_group_trait | never | Rudderstack sets this cookie, which is used to store performed actions on the website. |
rl_page_init_referrer | never | Rudderstack sets this cookie, which is used to store performed actions on the website. |
rl_page_init_referring_domain | never | Rudderstack sets this cookie, which is used to store performed actions on the website. |
rl_trait | never | Rudderstack sets this cookie, which is used to store performed actions on the website. |
rl_user_id | never | RudderStack set this cookie to store a unique user ID for the Marketing/Tracking purpose. |
SUID | 12 hours | Google Analytics sets this cookie to collect data on user preferences and/or interaction with web campaign content (Microsoft). |
test_cookie | 15 minutes | This cookie is set by doubleclick.net. The purpose of the cookie is to determine if the users'' browser supports cookies. |
VISITOR_INFO1_LIVE | 5 months 27 days | This cookie is set by Youtube. Used to track the information of the embedded YouTube videos on a website. |
yt-remote-connected-devices | never | YouTube sets this cookie to store the user's video preferences using embedded YouTube videos. |
yt-remote-device-id | never | YouTube sets this cookie to store the user's video preferences using embedded YouTube videos. |
yt.innertube::nextId | never | YouTube sets this cookie to register a unique ID to store data on what videos from YouTube the user has seen. |
yt.innertube::requests | never | YouTube sets this cookie to register a unique ID to store data on what videos from YouTube the user has seen. |
Cookie | Duration | Description |
---|---|---|
_ga | 2 years | This cookie is installed by Google Analytics. The cookie is used to calculate visitor, session, campaign data and keep track of site usage for the site''s analytics report. The cookies store information anonymously and assign a randomly generated number to identify unique visitors. |
_ga_* | 1 year 1 month 4 days | Google Analytics sets this cookie to store and count page views. |
_ga_TR76MGPH49 | 2 years | The _ga cookie, installed by Google Analytics, calculates visitor, session and campaign data and also keeps track of site usage for the site's analytics report. The cookie stores information anonymously and assigns a randomly generated number to recognize unique visitors. |
_gaexp_rc | less than a minute | Google Analytics sets this cookie to determine a user's inclusion in an experiment and the expiry of experiments that a user has been included in. |
_gat_UA-* | 1 minute | Google Analytics sets this cookie for user behaviour tracking. |
_gcl_au | 3 months | This cookie is used by Google Analytics to understand user interaction with the website. |
_gid | 1 day | This cookie is installed by Google Analytics. The cookie is used to store information of how visitors use a website and helps in creating an analytics report of how the wbsite is doing. The data collected including the number visitors, the source where they have come from, and the pages viisted in an anonymous form. |
_hjRecordingEnabled | never | Hotjar sets this cookie when a Recording starts and is read when the recording module is initialized, to see if the user is already in a recording in a particular session. |
_hjRecordingLastActivity | never | Hotjar sets this cookie when a user recording starts and when data is sent through the WebSocket. |
_hjSession_* | 30 minutes | Hotjar sets this cookie to ensure data from subsequent visits to the same site is attributed to the same user ID, which persists in the Hotjar User ID, which is unique to that site. |
_hjSessionUser_* | 1 year | Hotjar sets this cookie to ensure data from subsequent visits to the same site is attributed to the same user ID, which persists in the Hotjar User ID, which is unique to that site. |
ai_session | 30 minutes | This is a unique anonymous session identifier cookie set by Microsoft Application Insights software to gather statistical usage and telemetry data for apps built on the Azure cloud platform. |
attribution_user_id | 1 year | This cookie is set by Typeform for usage statistics and is used in context with the website's pop-up questionnaires and messengering. |
FPID | session | Google Tag Manager sets this cookie for server-side tagging. |
FPLC | session | Google Tag Manager sets this cookie for tracking between domains. |
ln_or | 1 day | Linkedin sets this cookie to registers statistical data on users' behaviour on the website for internal analytics. |
MUIDB | 1 year 24 days | Bing sets this cookie to determine how the user uses the website and any advertising that the end user may have seen before visiting the said website. |
vuid | 2 years | This domain of this cookie is owned by Vimeo. This cookie is used by vimeo to collect tracking information. It sets a unique ID to embed videos to the website. |
Cookie | Duration | Description |
---|---|---|
__cf_bm | 30 minutes | This cookie is set by CloudFare. The cookie is used to support Cloudfare Bot Management. |
_EDGE_S | session | Bing sets this cookie to display map content using Bing Maps. |
_EDGE_V | 1 year 24 days | Bing sets this cookie to display map content using Bing Maps. |
_gaexp | 23 days 11 hours 9 minutes | Google Optimize sets this cookie to determine a user's inclusion in an experiment and the expiration of experiments a user has been included in. |
aka_debug | No description | |
bcookie | 2 years | This cookie is set by linkedIn. The purpose of the cookie is to enable LinkedIn functionalities on the page. |
cfid | 20 days 2 hours | Used in conjunction with cftoken this cookie helps to uniquely identify a client device (browser) to enable the site to maintain user session variables. |
lang | session | This cookie is used to store the language preferences of a user to serve up content in that stored language the next time user visit the website. |
li_gc | 5 months 27 days | Linkedin set this cookie for storing visitor's consent regarding using cookies for non-essential purposes. |
lidc | 1 day | This cookie is set by LinkedIn and used for routing. |
Cookie | Duration | Description |
---|---|---|
ADRUM_BTa | This cookie is used to optimize the visitor experience on the website by detecting errors on the website and share the information to support staff. | |
sync_active | never | This cookie is set by Vimeo and contains data on the visitor's video-content preferences, so that the website remembers parameters such as preferred volume or video quality. |
YSC | session | This cookies is set by Youtube and is used to track the views of embedded videos. |
Cookie | Duration | Description |
---|---|---|
_gat_UA-21273952-1 | 1 minute | No description |
_hjAbsoluteSessionInProgress | 30 minutes | No description |
_hjFirstSeen | 30 minutes | No description |
_hjid | 1 year | This cookie is set by Hotjar. This cookie is set when the customer first lands on a page with the Hotjar script. It is used to persist the random user ID, unique to that site on the browser. This ensures that behavior in subsequent visits to the same site will be attributed to the same user ID. |
_hjIncludedInPageviewSample | 2 minutes | No description |
_hjIncludedInSessionSample | 2 minutes | No description |
_hjTLDTest | session | No description |
A3 | 1 year | No description |
aff | 1 month | No description |
AnalyticsSyncHistory | 1 month | No description |
asst | 30 minutes | No description |
AWSALB | 7 days | AWSALB is a cookie generated by the Application load balancer in the Amazon Web Services. It works slightly different from AWSELB. |
cass | 2 hours | No description |
cftoken | 20 days 2 hours | Used in conjunction with cfid this cookie helps to uniquely identify a client device (browser) to enable the site to maintain user session variables. |
CONSENT | 16 years 10 months 12 days 14 hours 5 minutes | No description |
gdId | 10 years | No description |
gdsid | 6 hours | No description |
GSESSIONID | 2 hours | No description |
MXP_TRACKINGID | 30 years | No description |
player | 1 year | No description |
RUL | 1 year | RUL writes these cookies: GDPR - your consent that is collected on this page (valid for one year). PHPSESSID - session identifier for repository part of RUL, valid as long as the browser window is open (valid for session duration). random number - session identifier for content part of RUL, valid as long as the browser window is open. startSz - font size. This cookie is written only if you change the font size using ""Bigger font"" or ""Smaller font"" link. dkUser - written only when you log-in, which changes the display of certain page elements (valid for session duration). Above mentioned cookies are mandatory for our website to function properly. |
SameSite | No description | |
site | No description | |
trs | 1 year | No description |
UserMatchHistory | 1 month | Linkedin - Used to track visitors on multiple websites, in order to present relevant advertisement based on the visitor''s preferences. |
Cookie | Duration | Description |
---|---|---|
__adal | session | Description is currently not available. |
_hjIncludedInSessionSample_2773626 | 2 minutes | Description is currently not available. |
_hjIncludedInSessionSample_66910 | 2 minutes | Description is currently not available. |
AWSALBTG | 7 days | No description available. |
AWSALBTGCORS | 7 days | No description available. |
ccc | 1 month | Description is currently not available. |
is_eu | never | No description available. |
m | 1 year 1 month 4 days | No description available. |
Path | session | Description is currently not available. |
SRCHHPGUSR | 1 year 24 days | No description available. |
tf_respondent_cc | 6 months | Description is currently not available. |
WFESessionId | session | No description available. |
- Research article
- Open access
- Published: 29 May 2024
Specification curve analysis to identify heterogeneity in risk factors for dementia: findings from the UK Biobank
- Renhao Luo 1 ,
- Dena Zeraatkar 2 ,
- Maria Glymour 3 ,
- Randall J. Ellis 1 ,
- Hossein Estiri 4 &
- Chirag J. Patel 1
BMC Medicine volume 22 , Article number: 216 ( 2024 ) Cite this article
1380 Accesses
17 Altmetric
Metrics details
In 2020, the Lancet Commission identified 12 risk factors as priorities for prevention of dementia, and other studies identified APOE e4/e4 genotype and family history of Alzheimer’s disease strongly associated with dementia outcomes; however, it is unclear how robust these relationships are across dementia subtypes and analytic scenarios. Specification curve analysis (SCA) is a new tool to probe how plausible analytical scenarios influence outcomes.
We evaluated the heterogeneity of odds ratios for 12 risk factors reported from the Lancet 2020 report and two additional strong associated non-modifiable factors ( APOE e4/e4 genotype and family history of Alzheimer’s disease) with dementia outcomes across 450,707 UK Biobank participants using SCA with 5357 specifications across dementia subtypes (outcomes) and analytic models (e.g., standard demographic covariates such as age or sex and/or 14 correlated risk factors).
SCA revealed variable dementia risks by subtype and age, with associations for TBI and APOE e4/e4 robust to model specification; in contrast, diabetes showed fluctuating links with dementia subtypes. We found that unattributed dementia participants had similar risk factor profiles to participants with defined subtypes.
Conclusions
We observed heterogeneity in the risk of dementia, and estimates of risk were influenced by the inclusion of a combination of other modifiable risk factors; non-modifiable demographic factors had a minimal role in analytic heterogeneity. Future studies should report multiple plausible analytic scenarios to test the robustness of their association. Considering these combinations of risk factors could be advantageous for the clinical development and evaluation of novel screening models for different types of dementia.
Peer Review reports
In 2020, the Lancet Commission Report highlighted 12 potentially correlated and modifiable risk factors as targets for potential dementia prevention, intervention, and care for different age groups: education, hearing loss, traumatic brain injury (TBI), hypertension, alcohol consumption, obesity, smoking, depression, social isolation, physical inactivity, diabetes, and air pollution [ 1 ]. Additionally, previous studies identified non-modifiable risk factors, APOE e4/e4 genotype [ 2 ], and family history of Alzheimer’s disease [ 3 , 4 ], which are also strongly associated with dementia outcomes. While these factors can be prioritized based on the size of the risk (e.g., the magnitude of the odds ratio), it is unclear whether risk estimates are heterogeneous across demographics (e.g., age, gender, and ethnicity), operationalizations of dementia in the health record (e.g., as coded or non-coded subtypes, such as Alzheimer’s disease, and/or age of onset), or the co-occurrence/correlation of the major risk factors (e.g., a participant having both hypertension and diabetes).
Sources of heterogeneity in estimated effects of risk factors may be biological differences or the study design itself, such as the covariates selected as adjustment factors. Furthermore, given that the diagnosis and treatment of dementia involve an evolving, complex, and interdisciplinary approach in the clinical world and nonuniform progress among various populations [ 5 ], understanding the heterogeneity of dementia can offer a more robust basis for the early diagnosis and care of patients. In fact, the Lancet 2020 report has reported differences in risk across studies and demographic stratum. For example, the report documents large differences between study heterogeneity for traumatic brain injury (TBI) ( I 2 of 99%) [ 1 ]. Digging in deeper, two studies on military veterans showed men have an increased dementia risk after TBI than women [ 6 , 7 ]. Moreover, a Swedish study on TBI, adjusted for age, civil status, education, and pension, showed a larger risk of dementia than a Danish study, which only adjusted for sex [ 8 , 9 ]. These results led to specific clinical care suggestions about TBI in the Lancet report, among other suggestions for other risk factors [ 1 ]. It is unclear what factors within studies contribute to the differences in risk estimates that emerge. Further still, the risk factors (e.g., obesity and diabetes) may be correlated with one another and provide “redundant” information. Modeling them together is required to attain an accurate risk estimate for one risk factor that is independent of the others.
Importantly, although there is unambiguous theoretical guidance about selecting covariates to estimate causal effects [ 10 ], applied researchers face tremendous ambiguity. Imperfect covariate measurement, unclear life course timing of occurrence of potential covariates compared to the exposure [ 11 ], and confusion of criteria for confounders versus mediators lead to inconsistent covariate sets across analyses. We claim that associations that are minimally influenced by the selection of alternative and plausible covariate sets are the most convincing. Moreover, the sensitivity and combinational effects of covariate sets are important to understand for future analyses [ 12 ]. Therefore, a specification curve analysis (SCA), an approach to analyze and visualize comprehensive sources of heterogeneity transparently, can enable us to identify specifications that are biological and clinically meaningful [ 13 ].
Here, we first apply SCA to systematically investigate the impact of 2 age groups, 5 different demographic variables, and 14 risk factors with a total of 1445 analytical specifications on the associations between risk factors and dementia-specific ICD 9/10 diagnosis codes [ 13 , 14 ]. We considered specifications such as age groups and different dementia disease coding “subtypes” (as characterized by administrative International Classification of Disease [ICD] codes, pre-defined ICD codes for hospital admission records and death certificate records, and self-reported information), including AD, dementia that is unattributable to AD, frontotemporal dementia, or vascular dementia. For each, we compare models controlling for different covariate sets, such as adjustment for gender and/or ethnicity. Second, we selected risk factors across the spectrum of analytic robustness to test their association in multivariate modeling scenarios or different combinations of the risk factors themselves with a total of 3912 analytical specifications. Lastly, we examined the relationship between unattributed dementia patients and known dementia-type patients based on their risk factor profiles.
Study population
UK Biobank (UKB) is a detailed prospective study of 502,505 participants. The participants’ phenotypic and genetic information was collected between 2006 and 2010 when they were aged between 40 and 69 years in one of 22 assessment centers across England, Scotland, and Wales. During the visit, physical measurements were taken, and phenotypic information was collected by answering many questions about their health status and lifestyles via touch-screen or nurse-led questionnaires [ 15 ]. In addition, the participants’ genetic information was obtained from their biosamples, and the samples underwent genome-wide genotyping using the UK Biobank Axiom Array. This array directly measures approximately 850,000 variants, and more than 90 million variants were imputed using the Haplotype Reference Consortium and UK10K + 1000 Genomes reference panels [ 16 ]. All participants consent to the study. The UKB study application of this study is 52887. The Harvard internal review board (IRB) deemed the research to be non-human subjects research (IRB: IRB16-2145).
In this study, we only included participants greater than 45 years old in the analyses, which left 450,707 participants. We divided the study population into two different groups: midlife (age at dementia diagnosis between 45 and 65) and late-life (age at dementia diagnosis greater than 65), consistent with the 2020 Lancet report [ 1 ]. Additional file 2: Table S1 shows the definition used for each risk factor, and Additional file 2: Table S2 shows the dataset’s prevalence of participants with different types of dementia. The rest of the participant’s ages were defined as when they attended the first visit when grouping.
Dementia case ascertainment
We focus on different dementia subtypes, including all-cause dementia, Alzheimer’s disease, vascular dementia, and frontotemporal dementia. The source of the report and the date of the report for all four types of dementia reports were obtained from the algorithmically defined dementia outcomes, which combine participants’ self-reported medical conditions, linked hospital diagnoses, and death registries provided by the UK Biobank group and validated by a different study [ 17 , 18 ]. The patient’s diagnosis age is determined by the date of the specific dementia report date. The distribution of age at dementia diagnosis is shown in Additional file 1: Figure S1. For each subtype of dementia, the binary variable was defined as one if a given patient has that specific dementia diagnosis and zero if the patient does not have particular dementia subtypes. Additionally, we extracted “unattributed dementia” participants as the dementia participants who were in the all-cause dementia group but did not receive any specific diagnosis (e.g., ICD codes, hospital admission records, self-reports) for frontotemporal dementia, AD, and/or vascular dementia. The number of unattributed dementia is 1189 participants.
Risk factors ascertainment
We identified the 31 Lancet Commission risk factor variables from 14 risk factors measured in the UK Biobank participants. We adopted the definitions of the modifiable variables from the 2020 Lancet report with some adjudication when extracting the data from the UK Biobank (see definitions in Additional file 2: Table S1). All risk factor variables were obtained from either the self-report or self-report combined with ICD 9/10 code diagnosis and other well-known clinical criteria when available (see prevalence of each risk factor in Additional file 2: Table S2). We used the risk factors with only self-reports available at the participants’ visits between 2006 and 2010, as shown in the questionnaires. For the risk factors with sources from self-reported and ICD code, we convert them into a binary table, and “Yes” annotates the participant as affirmative to the condition based on either source, whereas “No” annotates negative of the condition in the participant.
The Lancet Commission reported “social isolation” as one of the risk factors, and here, we use self-reported “loneliness” as a proxy of social isolation. We obtained the APOE e4/e4 genotype information from the imputed chromosome 19 file provided by the UK Biobank and extracted the genotype for the two APOE SNPs, rs429358 and rs7412, by using PLINK2 (v2.00a3.1LM) [ 19 ]. Based on the previous literature, the e4/e4 genotype corresponds to SNP alleles “CC” in rs429358 and “CC” in rs7412 [ 2 ]. After extracting the genotype information, we constructed a binary table for all participants, with “Yes” for carrying the allele and “No” for not carrying the allele.
Specification curve analysis (SCA)
In this study, we conducted a “specification curve analysis”, which is an approach that systematically considers all reasonable analytical choices to address a particular research question [ 13 ]. “Specifications” may include, but are not limited to, covariate choice or causal model, inclusion criteria, definitions of the outcome (here, dementia subtypes), and the ways the risk factors are processed and cleaned. Here, we produced 5357 unique specifications, and these include all combinations of dementia subtype outcomes with three categories of experimental variables, specifically, 5 dementia subtypes, 2 age groups, 5 covariate or model choices, and 14 risk factors (31 risk factor variables) with/without combinations (Fig. 1 ). The risk factors were selected based on modifiable and non-modifiable factors suggested by the 2020 Lancet report [ 1 ], and non-modifiable genetic factors, APOE e4/e4 [ 20 ], and family history of Alzheimer’s disease [ 3 ] that showed robust association with dementia. While adjusting for different demographics, we excluded 105 specifications, resulting in large confidence intervals, and have 1445 specifications for all risk factors. Additionally, we want to explore the combinatorial effects of different risk factors to mimic participants with multiple conditions. To do that, we selected risk factors with robust association (see the definition in the section below) and built models with multiple risk factors as the covariates and adjusted for gender, age, and ethnicity, resulting in an additional 3912 specifications. These specifications constituted our analysis set. We visualized the odds ratio (OR) results from each specification on a specification curve, providing a range of possible outcomes and allowing us to view the impact of our analytical choices on the results. The results of the specification curve analysis are shown in Fig. 2 for all risk factors (1445 specifications) and Fig. 3 with selected risk factors with combinational effects (3912 specifications). The odds ratios were the outcomes from different logistic regression models described in the section below.
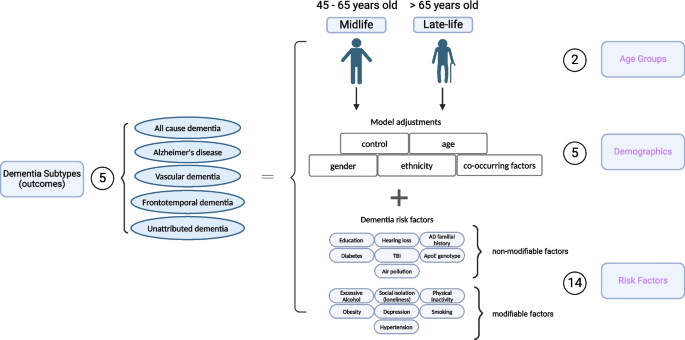
Specifications of risk in dementia subtypes. In our specification curve analysis (SCA), we modeled the dementia outcomes with three categories of experimental variables shown in purple, including “Age Groups,” “Demographics,” and “Risk Factors.” Specifically, we compared five different dementia subtypes, two age groups, five different model adjustments, and 14 different risk factors (31 different risk factor levels). Additionally, we selected robust risk factors and built models with different combinations of the risk factors for the subsequent SCA analysis. Figure created with Biorender
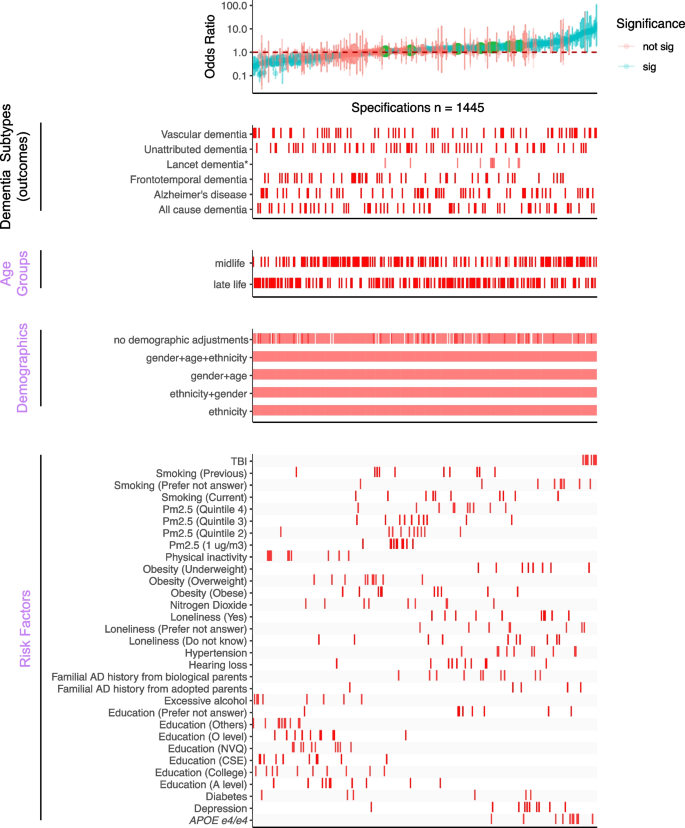
Overview of the SCA results for modeling dementia subtype outcomes with three categories of experimental variables, “Age Groups,” “Demographics,” and “Risk Factors.” All odds ratios are shown with 95% confidence intervals and colored by the significance of the model outputs in the top panel. The bottom four panels show the distribution of correspondents in each model. There are 1445 specifications from this SCA. “Sig” denotes nominal significance ( p < 0.05). * Lancet dementia refers to the odds ratios reported from the 2020 Lancet report [ 1 ] shown in green circles in the top panel and is not included in the total specification number
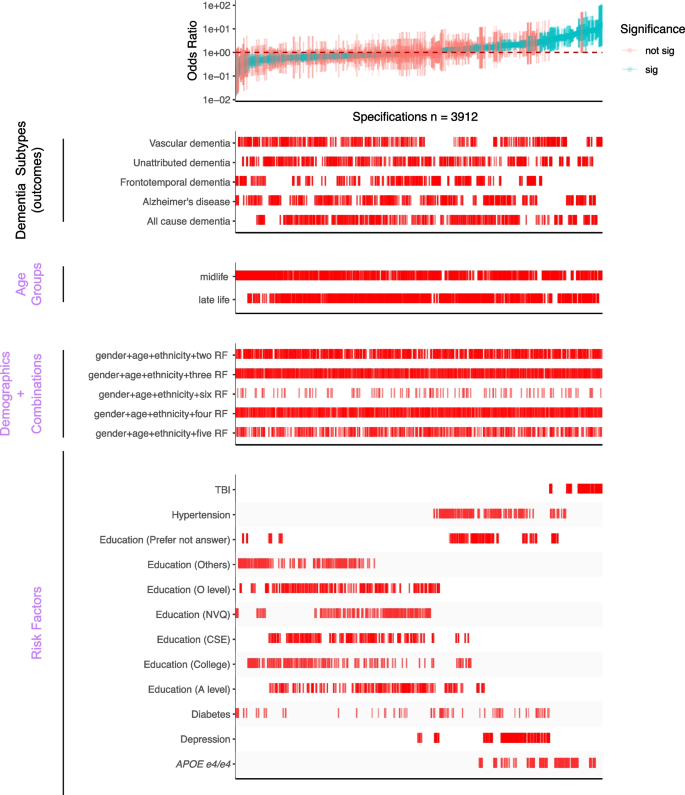
SCA results for modeling dementia subtype outcomes with three categories of experimental variables, including different combinations of selected risk factors (bottom panel). All odds ratios are shown with 95% confidence intervals and colored by the significance of the model outputs. There are a total of 3912 specifications from this SCA. “Sig” denotes nominal significance ( p < 0.05). “RF” in the “Demographic + Combination” panel refers to selected risk factors in the “Risk Factors” panel
Threshold for assessment of “robust” associations
We applied a set of rules to claim a risk factor as “robust”: simply, given an outcome (e.g., all-cause dementia or AD), we deemed a risk factor as robust if the IQR of the OR is all on one side of no association (OR = 1). We only considered the risk factors that resulted in significant ORs. The selected risk factors were included in the combinational effect analysis, shown in Fig. 3 .
Statistical analyses
The study overview is shown in Fig. 1 . All the analyses were performed using R v4.0.1 on the Harvard Medical School high-performance cluster. The prevalence of each risk factor was calculated by the number of positive cases of the risk factor, regardless of dementia status, divided by the total number of participants with the positive and negative of that risk factor. In this study, we built different logistic regression models corresponding to each dementia subtype in each age group, as shown in the results of SCA (Figs. 2 and 3 ). The gender and ethnicity information was extracted from the UKB data for all participants. We reported the odds ratio (OR), p -values, and 95% confidence interval based on the estimates from each model. We eliminated the risk factors for each age group with less than ten responses from the analyses. A risk factor is considered significant when the p -value is less than 0.05 (no adjustment for multiple hypotheses).
We excluded risk factors with less than 10 responses within the dementia category, and those are mainly “physical inactivity” and “excessive alcohol consumption” in midlife AD, vascular and frontotemporal dementia, and late-life in frontotemporal dementia. The summarized ORs for specifications are shown in Tables 1 and 2 . The summary statistics for selected robust risk factors are shown in Additional file 2: Table S3. The age- and gender-adjusted ORs for each dementia subtype are shown in Additional file 2: Table S4. To test the statistical significance between ORs from the UK Biobank cohort and the Lancet report, we used the following formula to calculate z and obtained the p -value, Z = (beta_1 − beta_2)/sqrt(se(beta_1) 2 + se(beta_2) 2 ). Lastly, to examine the similarity of the ORs between different outcomes, we estimated Pearson’s correlation on the ORs between the unattributed dementia participants and the subtype participants and reported the correlation coefficients with the p -values.
Through two specification curve analyses—one encompassing a comprehensive range of established dementia risk factors and the other focusing on a subset of particularly stable risk factors to study combinational effects—we identified heterogeneity in the risk for various dementia subtypes and across different age groups. Nonetheless, modifications in age categorization or demographic factors exerted negligible influence on the associations between risk determinants and dementia manifestations. Of particular significance were traumatic brain injury (TBI) and the APOE e4/e4 allele, which demonstrated consistent associations with all examined dementia subtypes across all analytical conditions. In contrast, risk factors such as diabetes exhibited variable correlations with diverse dementia outcomes. Furthermore, individuals with unattributed dementia participants displayed risk profiles that were analogous to those with definitive subtypes, transcending age delineations.
In this study, we included 450,707 participants from the UK Biobank with ages greater than 45 years old and divided them into two groups: midlife (45–65 years old) and late-life (greater than 65 years old). The midlife group consists of about 83% of all the participants. UK Biobank participants accrued 2710 all-cause dementia (44.5% female, about 0.6% of all UK Biobank participants), 1005 Alzheimer’s Disease (48.9% female), 539 vascular dementia (36.4% female), and 113 frontotemporal dementia (43.4% female) diagnoses during follow-up. There are 20%, 13%, 26.5%, and 12.4% of all-cause dementia, Alzheimer’s disease, frontotemporal dementia, and vascular dementia participants in the midlife group. The results from SCA analyses are summarized by the median ORs, their interquartile ranges (IQR), and the percentage of significant model outputs in Tables 1 and 2 . The definitions we used to query the risk factors are shown in Additional file 2: Table S1. Details about the number of participants included for each risk factor among different age groups and prevalence can be found in Additional file 1: Figure S1 and Additional file 2: Table S2.
Specification curve analysis to illustrate analytic heterogeneity in different models
Our comprehensive specification curve analysis revealed significant findings regarding dementia risk factors. The comprehensive specifications consist of three categories of experimental variables to model the dementia subtypes as the outcome, outlined in Fig. 1 . Out of 1445 models in Fig. 2 , 716 showed statistically significant results ( p < 0.05). Considering the directionality, the median OR of all the significant models greater than or equal to 1 is 2.09 [1.49–3.39], and less than 1 is 0.42 [0.33–0.58]. There are 917 models with ORs greater than or equal to 1.
We presented the details of the comprehensive specifications of our models in Table 1 . The age groups showed that more midlife participants contributed to large ORs than late-life participants. We did not observe distinguishable differences in estimates depending on covariate sets in any specifications. For the same risk factor (e.g., diabetes) under different model adjustments, the odds ratio varied. Diabetes had inconsistent associations in different age groups (midlife median OR 0.52 [0.37–0.96] and late-life median OR 1.86 [1.22–2.51]). On the other hand, most other risk factors had similar associations with dementia regardless of the age group evaluated. PM2.5 ORs differed based on analytic specification; for example, the top quintile has a midlife median OR 1.43 [1.15–1.45] and a late-life median OR 1.39 [1.29–1.49], which is higher compared to the second quintile (midlife median OR 1.10 [1.08–1.16] and late-life median OR 1.21 [1.19–1.23]).
The Lancet study’s risk factors had varying levels of robustness, with some showing smaller odds ratios (ORs) in the UK Biobank (Additional file 1: Figure S2). Traumatic brain injury (TBI) showed a median OR of 8.43 [7.19–10.58] for midlife and 8.29 [7.47–9.27] for the late-life group in both studies, with significant midlife OR difference. Depression ORs were higher in UK Biobank across all ages (midlife = 2.11 [2.04–2.32], late-life = 2.09 [1.89–2.25]), while hypertension ORs were higher in the Lancet but lower in UK Biobank (midlife = 1.69 [1.61–2.18], late-life: 2.37 [1.45–3.12]). No significant late-life risk factor differences were noted. Inconsistencies in excessive alcohol consumption and physical inactivity associations between studies may relate to UK Biobank’s smaller sample size, as shown in Additional file 2: Table S2. These disparities suggest population differences as a potential cause of heterogeneity in risk factor impacts. Furthermore, we are interested in studying the impacts of multiple risk factors in one model on the ORs.
Specification curve analyses highlight several robust risk factors in association with dementia outcomes
To test the robustness of six selected risk factors (TBI, APOE e4/e4 , hypertension, diabetes, depression, and education) from the previous specification curve analyses, we executed 3912 multivariate logistic regression models with different combinations of the selected risk factors (while adjusting for age, gender, and ethnicity) (Fig. 3 and Table 2 ). In these multivariate models, we assessed each risk factor by (a) the number of times the OR is greater than or less than 1 and (b) the interquartile range of the OR (Fig. 4 and Additional file 2: Table S3) to assess their heterogeneity.
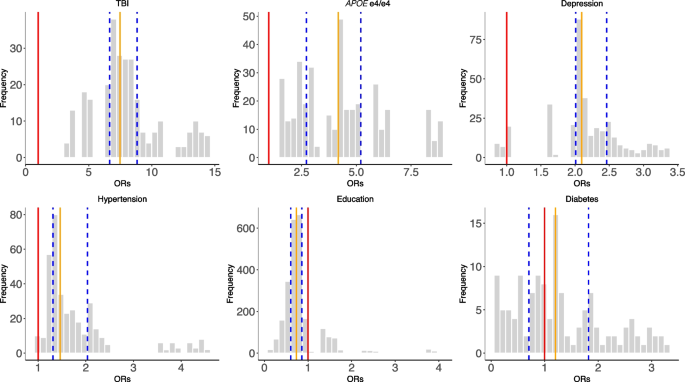
The distributions of odds ratios among the selected risk factors across all combinations of SCA analyses (Fig. 3 ). The red line represents OR = 1, the orange line represents the median ORs, and the blue lines define the IQRs
In Fig. 4 , we showed the distribution of the ORs among the selected risk factors. 100% of models that included APOE e4/e4 (ORs = 1.56–8.76), 100% of models that included TBI (ORs = 3.42–14.48), 97% of models with hypertension (ORs = 0.97–4.54), and 93% depression outputs (ORs = 0.87–3.36) are positively associated (OR > 1) with dementia outcomes. Further, higher than high school education categories were all negatively (OR < 1) associated with dementia outcomes (e.g., 84% in A level, 96% in O level, and 90% in college and CSEs). In contrast, diabetes has mixed and non-robust model outputs (ORs = 0.11–3.28; 55% of models have ORs less than 1) from the combinations of risk factors, suggesting inconsistent association trends for diabetes in disease outcomes in the presence of other correlated risk factors.
To assess the robustness of these risk factors (except diabetes) in different dementia subtypes, we compared the OR generated from the no-demographic-adjusted models with the odds ratios from the multivariate models with all six risk factors (Additional file 1: Figure S3). We observed positive correlations between the simple and multi-risk factor models in all dementia subtypes. The highest correlation was in unattributed dementia ( R 2 = 0.995, p -value = 2.425e − 10), and frontotemporal dementia showed the lowest correlation ( R 2 = 0.694, p -value = 0.002). These findings suggest the robustness of the five risk factors’ associations with dementia outcomes, regardless of model adjustments.
Heterogeneity of risk factors within and across different age groups and dementia subtypes
Next, we compared the ORs for the risk factors within each age group with different justifications (Fig. 5 , Additional file 1: Figure S4, and Additional file 2: Table S4). The range of ORs in the age- and gender-adjusted models for APOE e4/e4 in midlife is from 1.57 to 5.09, and in late-life is from 2.77 to 8.78. Similarly, the ranges of ORs for TBI in age- and gender-adjusted models are 6.81 to 13.97 for midlife and 6.92 to 10.15 for late life. In contrast, some risk factors present small differences between dementia subtypes, such as continuous PM2.5 (1 µg/m3). The OR range in PM2.5 (per 1 µg/m3) is in the age- and gender-adjusted model in midlife which is between 0.88 and 1.21, and the range in late life is between 1.13 and 1.17.
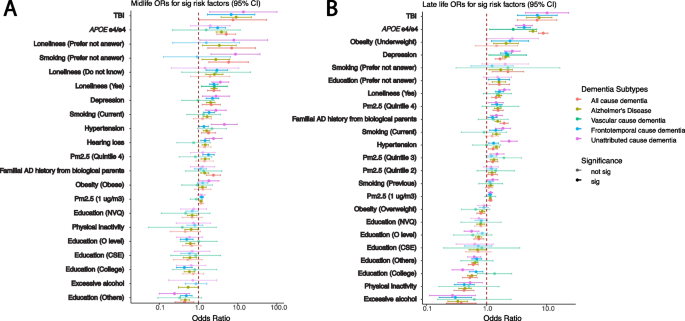
Heterogeneity between different dementia subtypes among significant risk factors identified in age- and gender-adjusted models from the all-cause dementia participants in respective age groups. The ORs and 95% confidence intervals from gender- and age-adjusted models from significant all-cause dementia outputs ranked by the ORs from high to low in midlife ( A ) and late-life ( B ) groups. The ORs in the plots are colored by the dementia subtypes, and transparency indicates the significance
There are risk factors that have a significant association ( p < 0.05) in one age group but not in the other one, and vice versa. Hearing loss is significant in all-cause dementia in the midlife group but becomes non-significant in late life. Overweight is significant in the late-life group for all-cause dementia participants but not in the midlife group. Additionally, within subtypes, some risk factors differ across age groups. For example, in the age- and gender-adjusted model (Fig. 5 ), APOE e4/e4 has significant ORs, from high to low, in AD (5.09 [2.92–8.87]), all-cause dementia (3.85 [2.82–5.24]), and unattributed cause dementia (3.08 [1.93–4.9]) among the midlife participants. Moreover, the APOE e4/e4 ORs are significant among the late-life participants, displaying a different OR ranking and overall larger ORs compared to the midlife group in the age- and gender-adjusted models: AD (8.78 [7.29–10.57]), all-cause dementia (5.90 [5.13–6.78]), unattributed cause dementia (4.23 [3.31–5.40]), vascular dementia (4.19 [3.03–5.79]), and frontotemporal dementia (2.77 [1.12–6.86]). Similarly, intra-group variation in OR rankings also exists in other risk factors, including depression, diabetes, hypertension, loneliness, and TBI.
Heterogeneity of risk factors for unattributed dementia
In the UKB, there are 1189 participants out of 2732 all-cause dementia participants who were not coded into the dementia subtypes (specify how you classified unattributed dementia). We refer to those participants as “unattributed” dementia participants. To understand the risk factors for those participants, we calculated the ORs for those people separately for each age group (Additional file 2: Table S4). Similar to other dementia subtypes, TBI (OR with 95% CI for midlife 6.82 [1.69–27.48] and for late-life OR 6.98 [3.25–14.97]) and APOE e4/e4 genotypes (OR with 95% CI for midlife 3.08 [1.93–4.90] and for late-life OR 4.23 [3.31–5.40]) were among the top risk factors with high odds ratios. Moreover, the rankings of the risk factors in both midlife and late-life groups are similar to the all-cause dementia participants. In midlife, familial AD history from adopted parents, loneliness, depression, smoking, hearing loss, hypertension, and quintile 4 of the PM2.5 are the significant risk factors with ORs greater than 1. In late life, underweight, depression, loneliness, smoking, quintile 4 of the PM2.5, and familial AD history from biological parents have significant odds ratios in all models with ORs greater than 1. Significant education levels have ORs less than 1 in both age groups.
To further investigate the concordance of risk factors between the unattributed dementia participants and known dementia participants, we correlated the ORs of the risk factors between unattributed dementia to each other specific subtype. In the midlife group, the unattributed dementia group had Pearson correlation coefficients of 0.439, 0.651, and 0.703 with vascular dementia, AD, and frontotemporal dementia, respectively (Fig. 6 A and Additional file 1: Figure S5A). While most risk factors have similar ORs in the comparisons, some risk factors, such as APOE e4/e4 , showed the highest ORs across specifications. In the vascular dementia comparison, the APOE e4/e4 has a higher OR in the unattributed dementia cause group than in vascular dementia. In contrast, it has lower ORs in unattributed dementia when compared to AD. The ORs from unattributed participants significantly correlated with those in AD and vascular dementia participants with Pearson’s correlation coefficients of 0.838 and 0.934, respectively (Fig. 6 B and Additional file 1: Figure S5B), whereas a low correlation with the frontotemporal dementia participants with a correlation coefficient of 0.491. In all the late-life comparisons, both TBI and APOE e4/e4 have high ORs. Based on the Pearson correlation results, the unattributed participants are more correlated with participants having frontotemporal dementia in the midlife group and vascular dementia and AD in the life group.
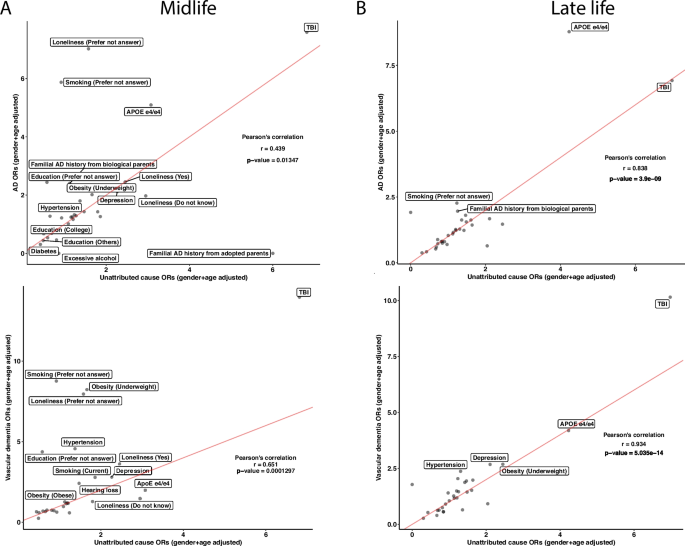
Unattributed cause dementia ORs in all risk factors and their correlations with known cause dementia. We subset the participants with unattributed causes of dementia and reported the ORs on the risk factors in both midlife and late life. We ran correlations on the ORs between the known subtypes of dementia with unattributed causes of dementia in midlife ( A ) and late-life ( B ) in AD and vascular dementia. Lastly, we calculated Pearson’s correlation for each comparison and reported the correlation coefficients and the p -values. The red lines in the correlation plots show when the slope is 1. There are N = 131, 67, and 296 participants in the midlife group for AD, vascular dementia, and unattributed cause dementia, respectively, and N = 874, 472, and 873 participants in the late-life group for AD, vascular dementia, and unattributed cause dementia, respectively
In this study, we attempt to disentangle the heterogeneity in dementia arising from variation in examining a number of phenotypes or subtypes and adjustment covariates using the tools of the “specification curve analysis” (SCA). Analytic decisions made during modeling risk factors associated with dementia are dependent on assumptions, and these assumptions may bias results and induce heterogeneity. We performed SCA to study how all articulated analytical specifications (e.g., classification of subtype, age of diagnosis, and risk factors in a multivariate model) may affect the OR estimates and their precision. The space of possible assumptions we explore includes both study designs, for example, (a) covariates and risk factors in the model; (b) outcomes, such as all-comers dementia and ICD-encoded Alzheimer’s disease; and (c) age at the outcome. In our study, we systematically examined 5357 specifications, including 1445 specifications for all risk factors and 3912 specifications for selected risk factors with combinations. These specifications and factors are reasonable assumptions to make when assessing evidence for dementia but are not made explicit when developing recommendations such as the Lancet 2020 report (we considered all specifications from the report in the present study). For instance, we included both known modifiable (such as smoking and physical inactivity) and non-modifiable risk factors (such as TBI and air pollution) for dementia in our specifications for modeling. The specification curves are important to document to understand sources of variation in OR estimates rather than one monolithic estimate. Specifically, our results highlighted risk factors that consistently have high odds ratios across dementia types but also showed the risk factors with highly varying ORs across covariates, age groups, and dementia outcomes. Several risk factors remained robust when modeled in complex multivariate scenarios (e.g., TBI and APOE e4/e4 ). This database of specifications may provide a map for future researchers to guide future study plans in their cohort studies to identify modifiable risk factors in dementia systematically.
Examples of heterogeneity and robust associations
We discuss several specific associations between risk factors and dementia. TBI, we found, had a high OR across all specifications (median OR across risk factor specifications of midlife 8.29 [7.47–9.27] and late-life 8.43 [7.19–10.58]) and a large range across different combinations of specifications in the late-life group with median OR ranged from 5 (modeling 6 concurrent risk factors) to 7.23 (modeling 2 concurrent risk factors). In other studies, we also observe large OR ranges [ 6 , 21 , 22 ]. For instance, the Lancet 2020 reported an OR of only 1.8 [1.5–2.2] [ 1 ]. Moreover, the comparison between our results and the Lancet report also showed heterogeneity among different populations. The MIRAGE study, on the other hand, reported that participants (average age 69.9 ± 9) with head injury have an OR of 4.0 [2.9–5.5] [ 23 ]. In addition, the APOE e4/e4 genotype is strongly associated with dementia risk in all specifications. The median ORs across all specifications are 3.05 [1.99–3.85] for the midlife group and 4.18 [3.84–5.83] for the late-life group. This strong association is in line with previous studies and well documented by those studies [ 24 ].
Previous studies on hypertension [ 25 ], diabetes [ 26 , 27 ], and depression [ 28 ] have claimed causal correlations with dementia. In our study, diabetes has a variety of associations, where the ORs were larger than 1 and less than 1 depending on the analytic scenario, whereas hypertension and depression are examples of more robust and consistent associations with respect to the direction of OR. The degree of heterogeneity implies that these risk factors will require larger sample sizes and more precise outcome measures, potentially beyond ICD codes, to estimate their causal association, if any. These results also suggested that the large heterogeneity in diabetes (and other non-robust risk factors) could be potentially due to the different specifications and their correlation with other risk factors. Another group of robust associations is education. In our analysis, having a higher education level, including college and other professional training (e.g., nursing), mostly resulted in a negative association with dementia outcomes in all specifications. However, this relationship is not linear (e.g., the risks are different between A-level and CSE), which aligns with the previous results [ 29 ].
The role of outcome coding on heterogeneity of odds ratios
The lack of robustness of risk estimates can be driven by clinical/biological differences, such as subtypes of dementia, including Alzheimer’s disease (AD), vascular dementia, and frontotemporal dementia. However, these differing outcomes are represented by a set of disease codes in a health registry setting despite their complex clinical manifestation. We emphasize it is impossible to know the true diagnosis or subtype of dementia in these cases.
To surmount the obstacles associated with dementia classification, one viable strategy may involve the refinement of diagnostic codes to encompass not only the clinical manifestations observable through current standards, neuroimaging, and biomarkers [ 5 ] but also data pertaining to risk factors. By adopting this approach, a more comprehensive profile of dementia could be documented, thereby enriching the foundation for future dementia research.
Relatedly, our method of incorporating various combinations of risk factors, such as TBI, APOE e4/e4 , hypertension, etc., indicates that these robust association risk factors should be evaluated collectively to assess dementia risk and diagnosis accurately. Evaluating these factors individually may result in biased assessments or assessments that underperform in accuracy. Considering these risk factor combinations could be clinically beneficial in developing and appraising new models for screening for dementia subtypes.
In the analyzed instance of the UK Biobank, 1189 participants (43.5% of all-cause dementia participants) were not categorized into one of the known dementia subtypes by ICD codes. These participants have specification curves that resemble vascular dementia and AD participants [ 15 ].
Implications
In this investigation, we have identified and quantified analytic sources of analytic heterogeneity in risk factors for AD. We hypothesize that the heterogeneity in ORs that arise from age and subtypes may be due to differing etiologies between subtypes or “errors” in subtyping, such as misclassification.
Secondly, risk estimates for individual factors often neglect adjustments that account for correlations with other risk factors. Given that modifiable risk factors considered in Lancet 2020 are interrelated [ 30 , 31 ], it is crucial to analyze them concurrently in a multivariate regression model to obtain independent estimates. While the role of age is known in APOE -derived risk for AD [ 20 ], the role of age in modifiable risk factors is elusive. Future investigations should estimate the degree to which subtype heterogeneity is driven by analytic specification, such as inclusion criteria, versus biological differences. Specifically, future studies could validate the combinational effects in a different dataset, such as the All of Us cohort [ 32 ], to test if the robustness of risk factors could be replicated and generalizable. Additionally, incorporating the current diagnosis methods, neuroimaging, and biomarker testing into the specifications would also help to improve the accuracy of diagnosing cognitive decline and dementia not captured via the disease codes utilized in this study.
Thirdly, while we identified how risk factors might differ across subtypes, what remains is how intervening on any one or multiple factors might induce change in risk [ 33 ]. For example, a few studies have tried to establish the causal relationship between risk factors and dementia outcomes, but they resulted in either positive or negative associations when different assumptions were considered [ 34 , 35 , 36 ].
Strength and limitations
Here, we compared the odds ratios of modifiable and non-modifiable risk factors directly in different age groups and studied the changes in different risk factors between age groups to highlight differences and similarities between the UK Biobank population and the Lancet report [ 1 ]. The SCA highlighted the different effects of each risk factor, age group, and subtype of dementia described by different models. Future surveillance programs should describe the odds ratios of these factors as the case mix changes. For example, in a similar study examining the Lancet reported dementia risk factors on the US population, different ethnicities yielded different population attributable fractions (PAF) [ 21 ]. As a result, comprehensive assessments of dementia patients using multiple techniques should be taken to classify and later validate subtypes of dementia.
In this study, the sample size for certain risk factors was small. Moreover, we used self-reported data to ascertain non-genomic risk factors (Additional file 2: Table S1), which may limit the replicability of our findings in other datasets due to variability in questionnaire design. Third, a previous study showed that the positive predicted values for all-cause dementia and dementia subtype cases vary [ 18 ], which may also impact the replicability of the results in different datasets and the general population.
In the current study, we observed heterogeneity in the risk of dementia, and estimates of risk factors were influenced by the inclusion of a combination of other risk factors but not demographic factors. It is important for future recommendations and reports of risk to include multiple plausible analytical scenarios that consider correlated risk factors to assess the strength and accuracy of risk estimates.
Availability of data and materials
All the results of this study are included in the figure or in the supplementary information. The UK Biobank dataset is not publicly available. Researchers interested in accessing the dataset must apply and be approved by the UK Biobank management team. See details: https://www.ukbiobank.ac.uk/enable-your-research/apply-for-access .
Abbreviations
- Alzheimer’s disease
Confidence interval
International Classification of Diseases
Population attributable fractions
- Specification curve analysis
Traumatic brain injury
Livingston G, Huntley J, Sommerlad A, Ames D, Ballard C, Banerjee S, et al. Dementia prevention, intervention, and care: 2020 report of the lancet commission. Lancet. 2020;396(10248):413–46.
Article PubMed PubMed Central Google Scholar
Lumsden AL, Mulugeta A, Zhou A, Hyppönen E. Apolipoprotein E (APOE) genotype-associated disease risks: a phenome-wide, registry-based, case-control study utilising the UK Biobank. eBioMedicine. 2020;59:102954.
Article CAS PubMed PubMed Central Google Scholar
Liu JZ, Erlich Y, Pickrell JK. Case–control association mapping by proxy using family history of disease. Nat Genet. 2017;49(3):325–31.
Article CAS PubMed Google Scholar
Marioni RE, Harris SE, Zhang Q, McRae AF, Hagenaars SP, Hill WD, et al. GWAS on family history of Alzheimer’s disease. Transl Psychiatry. 2018;8(1):99.
Atri A. The Alzheimer’s disease clinical spectrum: diagnosis and management. Med Clin North Am. 2019;103(2):263–93.
Article PubMed Google Scholar
Barnes DE, Byers AL, Gardner RC, Seal KH, Boscardin WJ, Yaffe K. Association of mild traumatic brain injury with and without loss of consciousness with dementia in US military veterans. JAMA Neurol. 2018;75(9):1055.
Yaffe K, Lwi SJ, Hoang TD, Xia F, Barnes DE, Maguen S, et al. Military-related risk factors in female veterans and risk of dementia. Neurology. 2019;92(3):e205-11.
Nordström A, Nordström P. Traumatic brain injury and the risk of dementia diagnosis: a nationwide cohort study. Menon D, editor. PLoS Med. 2018;15(1):e1002496.
Fann JR, Ribe AR, Pedersen HS, Fenger-Grøn M, Christensen J, Benros ME, et al. Long-term risk of dementia among people with traumatic brain injury in Denmark: a population-based observational cohort study. Lancet Psychiatry. 2018;5(5):424–31.
Pearl J. Causality. Cambridge University Press; 2009. Available from: https://books.google.com/books?id=f4nuexsNVZIC .
Gilsanz P, Young JG, Glymour MM, Tchetgen Tchetgen EJ, Eng CW, Koenen KC, et al. Marginal structural models for life-course theories and social epidemiology: definitions, sources of bias, and simulated illustrations. Am J Epidemiol. 2022;191(2):349–59.
Patel CJ, Burford B, Ioannidis JP. Assessment of vibration of effects due to model specification can demonstrate the instability of observational associations. J Clin Epidemiol. 2015;68(9):1046–58. https://doi.org/10.1016/j.jclinepi.2015.05.029 .
Simonsohn U, Simmons JP, Nelson LD. Specification curve analysis. Nat Hum Behav. 2020;4(11):1208–14.
Ciria LF, Román-Caballero R, Vadillo MA, Holgado D, Luque-Casado A, Perakakis P, et al. An umbrella review of randomized control trials on the effects of physical exercise on cognition. Nat Hum Behav. 2023. Available from: https://www.nature.com/articles/s41562-023-01554-4 . Cited 2023 May 15.
Sudlow C, Gallacher J, Allen N, Beral V, Burton P, Danesh J, et al. UK Biobank: an open access resource for identifying the causes of a wide range of complex diseases of middle and old age. PLoS Med. 2015;12(3):e1001779.
Bycroft C, Freeman C, Petkova D, Band G, Elliott LT, Sharp K, et al. The UK Biobank resource with deep phenotyping and genomic data. Nature. 2018;562(7726):203–9.
Wilkinson T, Ly A, Schnier C, Rannikmäe K, Bush K, Brayne C, et al. Identifying dementia cases with routinely collected health data: a systematic review. Alzheimers Dement. 2018;14(8):1038–51.
Wilkinson T, Schnier C, Bush K, Rannikmäe K, Henshall DE, Lerpiniere C, et al. Identifying dementia outcomes in UK Biobank: a validation study of primary care, hospital admissions and mortality data. Eur J Epidemiol. 2019;34(6):557–65.
Chen ZL, Meng JM, Cao Y, Yin JL, Fang RQ, Fan SB, et al. A high-speed search engine pLink 2 with systematic evaluation for proteome-scale identification of cross-linked peptides. Nat Commun. 2019;10(1):3404.
Whitwell JL, Tosakulwong N, Weigand SD, Graff-Radford J, Ertekin-Taner N, Machulda MM, et al. Relationship of APOE, age at onset, amyloid and clinical phenotype in Alzheimer disease. Neurobiol Aging. 2021;108:90–8.
Lee M, Whitsel E, Avery C, Hughes TM, Griswold ME, Sedaghat S, et al. Variation in population attributable fraction of dementia associated with potentially modifiable risk factors by race and ethnicity in the US. JAMA Netw Open. 2022;5(7):e2219672.
Gardner RC, Burke JF, Nettiksimmons J, Kaup A, Barnes DE, Yaffe K. Dementia risk after traumatic brain injury vs nonbrain trauma: the role of age and severity. JAMA Neurol. 2014;71(12):1490.
Guo Z, Cupples LA, Kurz A, Auerbach SH, Volicer L, Chui H, et al. Head injury and the risk of AD in the MIRAGE study. Neurology. 2000;54(6):1316–23.
Farrer LA. Effects of age, sex, and ethnicity on the association between apolipoprotein E genotype and Alzheimer disease: a meta-analysis. JAMA. 1997;278(16):1349.
Hughes D, Judge C, Murphy R, Loughlin E, Costello M, Whiteley W, et al. Association of blood pressure lowering with incident dementia or cognitive impairment: a systematic review and meta-analysis. JAMA. 2020;323(19):1934.
Chatterjee S, Peters SAE, Woodward M, Mejia Arango S, Batty GD, Beckett N, et al. Type 2 diabetes as a risk factor for dementia in women compared with men: a pooled analysis of 2.3 million people comprising more than 100,000 cases of dementia. Diabetes Care. 2016;39(2):300–7.
Zheng B, Su B, Price G, Tzoulaki I, Ahmadi-Abhari S, Middleton L. Glycemic control, diabetic complications, and risk of dementia in patients with diabetes: results from a large U.K. cohort study. Diabetes Care. 2021;44:1556-63.
Singh-Manoux A, Dugravot A, Fournier A, Abell J, Ebmeier K, Kivimäki M, et al. Trajectories of depressive symptoms before diagnosis of dementia: a 28-year follow-up study. JAMA Psychiat. 2017;74(7):712.
Article Google Scholar
Sharp ES, Gatz M. Relationship between education and dementia: an updated systematic review. Alzheimer Dis Assoc Disord. 2011;25(4):289–304.
Patel CJ, Ioannidis JPA. Placing epidemiological results in the context of multiplicity and typical correlations of exposures. J Epidemiol Community Health. 2014;68(11):1096–100.
Patel CJ, Ioannidis JPA. Studying the elusive environment in large scale. JAMA. 2014;311(21):2173.
All of Us Research Program Investigators. The “All of Us” research program. N Engl J Med. 2019;381:668–76. https://doi.org/10.1056/NEJMsr1809937 .
Ngandu T, Lehtisalo J, Solomon A, Levälahti E, Ahtiluoto S, Antikainen R, et al. A 2 year multidomain intervention of diet, exercise, cognitive training, and vascular risk monitoring versus control to prevent cognitive decline in at-risk elderly people (FINGER): a randomised controlled trial. Lancet. 2015;385(9984):2255–63.
Desai R, John A, Saunders R, Marchant NL, Buckman JEJ, Charlesworth G, et al. Examining the lancet commission risk factors for dementia using Mendelian randomisation. BMJ Ment Health. 2023;26(1):e300555.
Serrano-Pozo A, Das S, Hyman BT. APOE and Alzheimer’s disease: advances in genetics, pathophysiology, and therapeutic approaches. Lancet Neurol. 2021;20(1):68–80.
Koutsodendris N, Blumenfeld J, Agrawal A, Traglia M, Grone B, Zilberter M, et al. Neuronal APOE4 removal protects against tau-mediated gliosis, neurodegeneration and myelin deficits. Nat Aging. 2023. Available from: https://www.nature.com/articles/s43587-023-00368-3 . Cited 2023 Mar 4.
Download references
Acknowledgements
Our study utilized the UK Biobank Resource under the approved project number 52887. We extend our thanks to both the participants and the data management team. We also would like to thank all the members of the Patel lab for providing constructive feedback on this project.
This research has been funded by the National Institutes of Health, National Institute on Aging (NIA), through grant number 1RF1AG074372 and NIEHS R01ES032470.
Author information
Authors and affiliations.
Department of Biomedical Informatics, Harvard Medical School, Boston, MA, USA
Renhao Luo, Randall J. Ellis & Chirag J. Patel
Department of Anesthesia and Department of Health Research Methods, Evidence and Impact, McMaster University, Hamilton, Canada
Dena Zeraatkar
Department of Epidemiology, School of Public Health, Boston University, Boston, MA, USA
Maria Glymour
Department of Medicine, Harvard Medical School, Boston, MA, USA
Hossein Estiri
You can also search for this author in PubMed Google Scholar
Contributions
RL, CJP: conceptualized the study, data curation, formal analysis, methodology, visualization, writing—original draft. RL, DZ, MG, RE, HE, CJP: provided input to modify the study design, validation, writing—review and editing. CJP: funding acquisition. All authors read and approved the final manuscript.
Authors’ Twitter handles
RL: @Renhao4.
DZ: @DenaZera.
MG: @MariaGlymour.
RJE: @randalljellis.
HE: @Hossein_Estiri.
CJP: @chiragjp.
Corresponding author
Correspondence to Chirag J. Patel .
Ethics declarations
Ethics approval and consent to participate.
UK Biobank has ethical approval from the NHS National Research Ethics Service, and all participants gave written permission for their anonymized data to be utilized in research and publications when they enrolled [ 11 ]. The UKB study application of this study is 52887. The Harvard internal review board (IRB) deemed the research to be non-human subjects research (IRB: IRB16-2145).

Consent for publication
Not applicable.
Competing interests
The authors declare that they have no competing interests.
Additional information
Publisher’s note.
Springer Nature remains neutral with regard to jurisdictional claims in published maps and institutional affiliations.
Supplementary Information
12916_2024_3424_moesm1_esm.docx.
Additional file 1: Figure S1. Distribution of participants’ age used in this study. Figure S2. Comparison of the risk factors’ odds ratios between UKB and the Lancet 2020 report. Figure S3. Correlation between the simple and complex models for dementia subtypes. Figure S4. Non-significant risk factors results across different dementia subtypes, in complementary to Fig. 5 . Figure S5. Correlation between frontotemporal dementia and the unattributed cause of dementia participants.
12916_2024_3424_MOESM2_ESM.docx
Additional file 2: Supplementary Table S1. Definitions of risk factors. Supplementary Table S2. Prevalence of different dementia risk factors in different age groups. Supplementary Table S3. Summary stats for odds ratios from selected risk factors (Fig. 4 ). Supplementary Table S4. Gender and age adjusted model outputs in midlife and late life group.
Rights and permissions
Open Access This article is licensed under a Creative Commons Attribution 4.0 International License, which permits use, sharing, adaptation, distribution and reproduction in any medium or format, as long as you give appropriate credit to the original author(s) and the source, provide a link to the Creative Commons licence, and indicate if changes were made. The images or other third party material in this article are included in the article's Creative Commons licence, unless indicated otherwise in a credit line to the material. If material is not included in the article's Creative Commons licence and your intended use is not permitted by statutory regulation or exceeds the permitted use, you will need to obtain permission directly from the copyright holder. To view a copy of this licence, visit http://creativecommons.org/licenses/by/4.0/ . The Creative Commons Public Domain Dedication waiver ( http://creativecommons.org/publicdomain/zero/1.0/ ) applies to the data made available in this article, unless otherwise stated in a credit line to the data.
Reprints and permissions
About this article
Cite this article.
Luo, R., Zeraatkar, D., Glymour, M. et al. Specification curve analysis to identify heterogeneity in risk factors for dementia: findings from the UK Biobank. BMC Med 22 , 216 (2024). https://doi.org/10.1186/s12916-024-03424-w
Download citation
Received : 05 January 2024
Accepted : 13 May 2024
Published : 29 May 2024
DOI : https://doi.org/10.1186/s12916-024-03424-w
Share this article
Anyone you share the following link with will be able to read this content:
Sorry, a shareable link is not currently available for this article.
Provided by the Springer Nature SharedIt content-sharing initiative
- All-cause dementia
- Vascular dementia
- Frontotemporal dementia
- Risk factors
BMC Medicine
ISSN: 1741-7015
- Submission enquiries: [email protected]
- General enquiries: [email protected]
Academia.edu no longer supports Internet Explorer.
To browse Academia.edu and the wider internet faster and more securely, please take a few seconds to upgrade your browser .
Enter the email address you signed up with and we'll email you a reset link.
- We're Hiring!
- Help Center
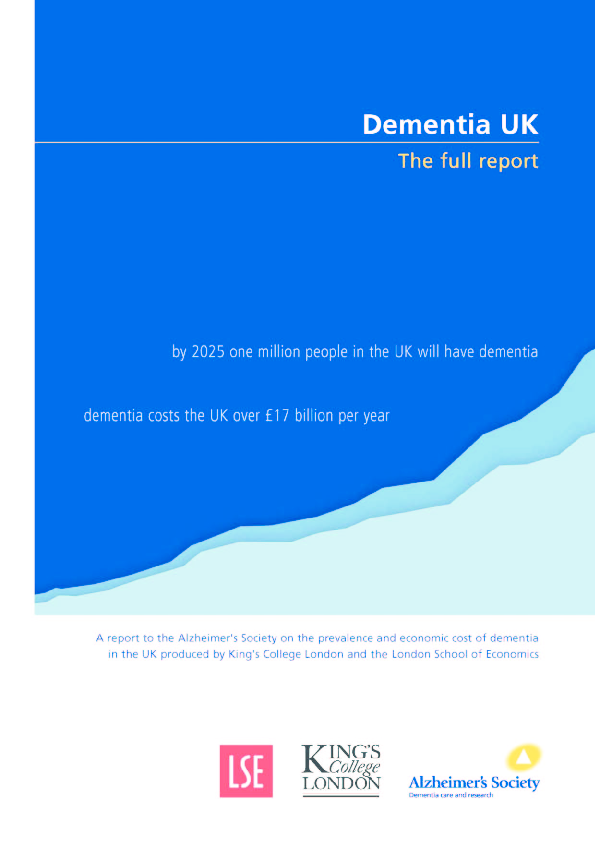
Dementia UK Full Report-

Related Papers
Australian and New Zealand Journal of Psychiatry
Perminder Sachdev
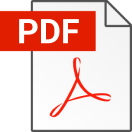
Journal of Public Mental Health
Lee Knifton
Dementia is "a global challenge and a public health priority" according to Dr Margaret Chan, director general of the World Health Organization in a speech she delivered to a recent G8 summit on the condition. Her sentiments are shared by many current political leaders including Barack Obama and David Cameron, the UK prime minister who hosted the summit in London last year. He described it as "the biggest challenge we face today" (UK Department of Health, 2012). In addition to organising international initiatives, many countries including Australia, Japan, Korea, Netherlands, Norway, France and the UK have also recently published national dementia plans setting out their own strategies to tackle this challenge (Alzheimer's Disease International). The plan outlined by the Obama administration is possibly the most ambitious and has called for an effective treatment by 2025 (US Department of Health and Human Services, 2011).
Research briefing paper
Catrin Jones
This paper is published as part of the Assembly’s pilot Academic Fellowship Scheme, which enables academics to work at the Assembly on a specific project, for the mutual benefit of the academic and the Assembly. As Wales enters a new phase in tackling dementia this paper outlines examples of innovative work in Wales, the UK and internationally set in the context of the WHO Global Plan of Action on the Public Health Response to Dementia 2017- 2025.
Disability & Society
George Rook
Adelina Comas-Herrera
The ageing of the population is focusing attention on the needs of older people, the services required to meet them, and how to finance those services in affordable, efficient, politically acceptable ways. These are enduring themes of PSSRU research, exemplified by the work of the Long-Term Care Finance programme at LSE (pp. 1 4–1 5) and the Unit's analyses for the 2006 Wanless report. Recent dementia studies at LSE have continued in this vein.
Dementia (London, England)
Hervé Platel
Given that there may well be no significant advances in drug development before 2025, prevention of dementia-Alzheimer's disease through the management of vascular and lifestyle-related risk factors may be a more realistic goal than treatment. Level of education and cognitive reserve assessment in neuropsychological testing deserve attention, as well as cultural, social, and economic aspects of caregiving. Assistive technologies for dementia care remain complex. Serious games are emerging as virtual educational and pleasurable tools, designed for individual and cooperative skill building. Public policies are likely to pursue improving awareness and understanding of dementia; providing good quality early diagnosis and intervention for all; improving quality of care from diagnosis to the end of life, using clinical and economic end points; delivering dementia strategies quicker, with an impact on more people. Dementia should remain presented as a stand-alone concept, distinct from...
Amanda Johnson
Karl Stroetmann
Egumgbe-Ezeh Chinonye C
Alternative therapies in health and medicine
Loading Preview
Sorry, preview is currently unavailable. You can download the paper by clicking the button above.
RELATED PAPERS
Aging Health
Oscar Blanco-Sanchez
- We're Hiring!
- Help Center
- Find new research papers in:
- Health Sciences
- Earth Sciences
- Cognitive Science
- Mathematics
- Computer Science
- Academia ©2024
- Open access
- Published: 09 February 2022
A systematic review and meta-analysis of studies on screening for mild cognitive impairment in primary healthcare
- Leila Karimi 1 ,
- Alireza Mahboub–Ahari 2 ,
- Leila Jahangiry 3 , 1 ,
- Homayoun Sadeghi-Bazargani 4 &
- Mostafa Farahbakhsh 5
BMC Psychiatry volume 22 , Article number: 97 ( 2022 ) Cite this article
4617 Accesses
10 Citations
Metrics details
Cognitive disorders and dementia have an important effect on individual independence and orientation. According to the Alzheimer's Disease International (ADI) 75% of people with dementia are not diagnosed; this may be as high as 90% in some low- and middle-income countries. This systematic review and meta-analysis aimed to identify the test performance of screening tools and compare them pairwise. The findings of our study can support countries in planning to establish and care for mild cognitive impairment in primary health centers.
Medline (PubMed), Scopus, Cochrane, Dare, All EBM Reviews, CRD (OVID), and Proquest were searched from 2012 to November 2021. The risk of bias was assessed through the QUADAS-2 instrument. Given the high heterogeneity between studies, a random-effects model was used to calculate the pooled effect sizes for diagnostic accuracy measures (sensitivity, specificity, and area under curve indices). I 2 test was used for assessing heterogeneity and predefined subgroup analyses were performed using participants’ age, country’s income, and sample size of studies.
A systematic search identified 18,132 records, of which, 20 studies were included in the quality assessment, and six were included in quantitative analysis. None of the studies had examined the feasibility or efficiency of mass screening. According to a pairwise comparison, IQCODE, AD8 and GPCOG showed equal or better diagnostic performance relative to the MMSE in terms of sensitivity and specificity. The random-effect model for the MMSE showed the pooled sensitivity equal to 0.73 (95% CI 0.57–0.90), the pooled specificity equal to 0.83 (95% CI 0.75—0.90), and the pooled AUC equal to 0.88 (95% CI 0.83–0.93).
Several benefits have been attached to short tests making them a suitable choice for use in primary healthcare settings. Considering factors such as accuracy, time of application, ease of scoring, and utilization charges, tests such as IQCODE, AD8, and GPCOG or appropriate combination with counterpart tools seem to be good alternatives to the use of the MMSE in primary care.
Peer Review reports
Cognitive disorders and dementia have an important effect on individual independence and orientation. Alzheimer’s is characterized by impaired memory and dysfunction; it is one of the areas of aphasia, apraxia, amnesia, and dysfunction, which has a significant impact on individual and social functioning [ 1 ]. According to the World Alzheimer Report, over 55 million people worldwide live with dementia, and this number is expected to increase to 78 million by 2030. According to the mentioned report, 75% of people with dementia are not diagnosed; this may be as high as 90% in some low- and middle-income countries [ 2 ].
Additional research by the National Institute on Aging (NIA) at the National Institutes of Health (NIH) and the Alzheimer's Association (NIA-AA) highlighted modernizing concept in Alzheimer’s disease diagnosis [ 3 ]. The research groups introduced Alzheimer’s disease in a continuum with three discrete phases including preclinical, Mild Cognitive Impairment (MCI), and dementia. They suggested that Alzheimer’s disease (AD) is a pathophysiological construct similar to other diseases such as diabetes and osteoporosis. By using biomarkers, a clinical specialist might detect the disease in a person based on symptoms [ 4 ]. However, physicians are less likely to be able to diagnose cognitive disorders by formal examining or performing daily visits [ 5 ], therefore, up to 76% of patients are diagnosed only in moderate or severe dementia [ 6 , 7 , 8 ]. Early diagnosis of cognitive impairment can give patients and their families the opportunity to receive care in the early stages of the disease; this will lead to a better prognosis and improve living standards. Although early detection of cognitive impairment cannot halt the onset of the disorder, and existing treatments cannot reverse the course of the disease, the health, psychological, and social benefits of early detection are important enough to make a screening program worthwhile [ 9 ]. Werner et al. [ 10 ] conducted a systematic review to investigate dementia diagnosis disclosure among the patients and their families. Based on their findings, most studies have been positive about the disclosure of the disease. The patients' families have acknowledged that they were initially skeptical about the disease disclosure, then they later adapted it. Awareness of the diagnosis has led to better planning and preparation for the future.
There has been a growing interest among researchers and health systems for the early identification of people at risk of developing dementia. In fact, early accurate diagnosis of AD is a major global health priority [ 11 ]. The global action plan of the World Health Organization (WHO) on the public health response to dementia targets at least 50% of countries to diagnose 50% of the estimated number of people with dementia by 2025 [ 2 ]. The US Preventive Services Task Force (USPSTF) in its last update, reported that there was insufficient published evidence of better clinical outcomes as a result of routine screening for cognitive impairment in older adults. However, the Task Force recognized that the use of cognitive assessment tools can increase the detection of cognitive impairment [ 12 ]. Subsequently, the Patient Protection and Affordable Care Act (PPACA) in the United States recommended early diagnosis of cognitive impairment during the annual wellness visit. The workgroup developed ten recommendations for improving the early detection and care for dementia, concerning the implementation of cognitive screening practice in personalized healthcare [ 13 ]. According to the principals of Annals Wellness Visits (AWV), the early detection process is likely to occur in a primary care setting by using brief screening tests (taking a minimum time to administer), used by non-physician practitioners. Therefore, it is necessary to have easy-to-score, quick, open access, and sensitive tests to identify people with dementia in primary healthcare [ 14 ]. In recent years, systematic reviews and meta-analyses have attempted to identify diagnostic accuracy of both comprehensive and brief instruments for cognitive impairment and Alzheimer’s [ 15 , 16 ]. Most of them have examined cognitive screening measures in secondary or tertiary care settings where the practice is run by physicians or neuropsychologist experts. The test performance of screening tools has not been widely assessed in the literature. In the study by Pelegrini et al. [ 15 ], diagnostic strategies in primary healthcare settings have been examined across low and middle-income countries. In spite of the short time interval of literature search (2013 to 2018), the study has only reported a sort of diagnostic criteria for screening tests’ performance and compared it among countries from different income streams. However, the gap of suitable instruments for use in primary healthcare settings has still been remained questionable. Lin et al., in an updated systematic review, attempted to address the benefits, harms, and diagnostic accuracy of brief screening instruments to detect cognitive impairment in community -dwelling older adults [ 16 ]. In spite of their conclusion in favor of the benefits of using brief instruments, they have not recognized empirical evidence on screening to improve decision-making. Considering the importance of early diagnosis for cognitive impairment as well as the consensus on primary care setting as the best start setting for assessment, our systematic review and meta-analysis aimed to identify test performance of screening tools and compare them pairwise. The findings of our study can support countries in planning to establish dementia care in primary health care centers.
The present systematic review was conducted in accordance with the preferred report items for systematic review and meta-analysis studies (PRISMA) [ 17 ]. The systematic review protocol was registered in the International Prospective Register of Systematic Reviews (PROSPERO) database with the code CRD42020156638.
Inclusion and exclusion criteria
All English original studies including a) screening early detection of cognitive disorders in a primary care setting, b) using short questionnaires (according to the Alzheimer Association, the questionnaires that take less than 5 min to administer), c) and reporting sensitivity, specificity, positive and negative predictive values, and AUC measures for diagnostic tests and d) screening mild dementia were searched. The exclusion criteria were: a) studies that only examined the characteristics of diagnostic methods, b) or evaluated patient or provider’s opinion about the instruments, c) studies applied laboratory markers or imaging techniques to diagnose a particular type of dementia or Alzheimer's disease.
Data sources and search strategy
Databases including Medline (PubMed), Scopus, Cochrane, Dare, All EBM Reviews, Center for Research and Dissemination (CRD) via OVID, and Proquest were searched from the beginning of 2012 to November 2021. A search strategy is presented below for PubMed. A supplementary search across the references list and citations of included studies were also performed in Google Scholar to find related articles.
(TITLE-ABS-KEY (dementia OR Alzheimer OR "Cognitive Disorders" OR "Cognitive impairment" OR "Cognition Disorders" OR "cognitive decline" OR "cognitive loss") AND TITLE-ABS-KEY (screening OR "Early detection" OR "early diagnosis")) AND PUBYEAR > 2012 AND PUBYEAR < 2021.
Selection of studies
The study selection was independently done by two authors (LK and LJ). Any disagreement was resolved by the systematic review consultant (HS) or the clinical consultant (MF). After eliminating duplicates in the reference management software (EndNote) and manually (sorting by the title and year of the study), the titles and abstracts of the studies were screened according to the inclusion criteria. At this stage, screening programs were identified and studies that met the exclusion criteria were excluded. For the studies without the original article, the authors contacted the corresponding author (send an email or message in www.researchgate.net ). If the reply message was not received after sending the message, the article was removed.
Data extraction
An Excel form was designed by the research team then administered to gather information about the author, year, country, population and place of the study, sample size, index, and reference test, reported outcome, and cut-off point. Data were independently extracted by (LK) and (AM) and sent to the (LJ) step by step for review and approval.
Risk of bias and quality assessment
In order to assess the risk of bias in the studies, The Quality Assessment of Diagnostic Accuracy Studies-2 (QUADAS-2) tools were used [ 17 ]. This tool has four domains of patient selection (three questions), index test (two questions), reference test (two questions), flow, and time (four questions). The probability of bias existence is reported in three levels of bias: low, uncertain, and high. Concerns about the usability of each domain are also reported in three forms: low, high, and unspecified. In fact, the purpose of this question is to evaluate the ability of the domain to answer the research question. In order to evaluate the quality of the studies, a software program designed by the QUADAS group was used. In this program, questions of each domain are listed, which by entering studies and evaluating them, the program allows the researcher to produce graphs and evaluation results in the form of excel tables. The risk of bias was assessed by LK and AM. In cases where clinical or epidemiological consultation was required, cases were raised and resolved with consulting professors (HS and MF). For minimizing biases and increasing reliability, selecting the studies for this systematic review was conducted through dual revision by two researchers. Cohen’s Kapa coefficient statistic was used for reporting the agreement.
Outcome measurement criteria
The outcome of interest consisted of the diagnostic accuracy indices of the screening tests, including sensitivity, specificity, or data that could be used to derive these values.
Summary of study findings and statistical analysis
In order to evaluate the accuracy of diagnostic screening tools, sensitivity and specificity of indices and reference tests were compared and reported in terms of study number and sample size. Given the high heterogeneity between studies, a random-effects model was used to calculate the pooled specificity, sensitivity, and AUC. I 2 test was used for assessing heterogeneity and predefined subgroup analyses were performed using participants’ age, country’s income, and sample size of studies. The data were analyzed using STATA version 14 (STATA Corp, College Station, TX, USA). P-values of less than 0.05 were considered statistically significant. Publication bias test was conducted by funnel plot analysis .
Ethical considerations
The present study has been approved in Tabriz University of Medical Sciences (NO. IR.TBZMED.VCR.REC.1398.139).
Studies characteristics
Systematic search identified 18,155 records, of which 9,858 articles were duplicates, and 8,245 records were not relevant which were excluded at initial screening of title and abstracts. After reviewing the title and abstract of the studies, 56 original articles were selected for the study. Of these, 35 studies were excluded because of not having eligible criteria. Finally twenty-one studies met the inclusion criteria for the systematic review and were included in the qualitative evaluation (Fig. 1 ). Characteristics of the studies were presented in Table 1 , share of countries from the 21 final studies including Australia ( n = 1) [ 18 ], China ( n = 2) [ 19 , 20 ], England ( n = 1) [ 21 ], Germany ( n = 3) [ 22 , 23 , 24 ], Greece ( n = 2) [ 25 , 26 ], Indonesia ( n = 1) [ 27 ], Italy ( n = 1) [ 28 ], Iran [ 29 ], Singapore ( n = 1) [ 30 ], Portugal ( n = 1) [ 31 ], Malaysia ( n = 3) [ 32 , 33 , 34 ], Turkey ( n = 1) [ 35 ], and USA ( n = 3) [ 36 , 37 , 38 ] were studied.
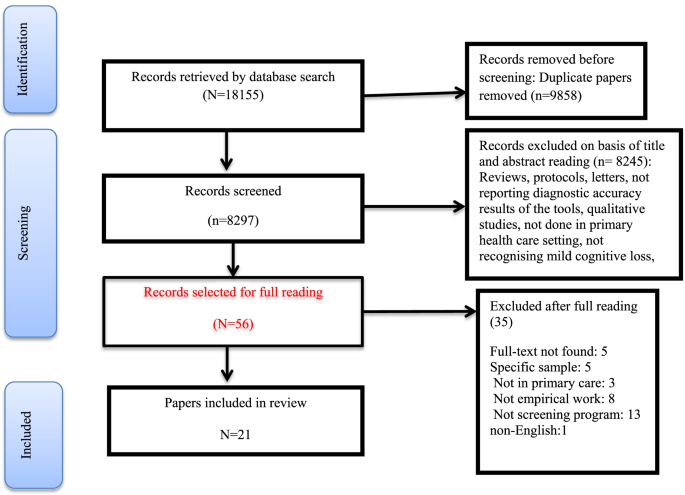
Study selection process
According to World Bank classification of countries by income [ 39 ], fourteen studies were conducted in high income countries (Australia, England, Germany, Greece, Italy, Singapore, Portugal, and USA) and seven studies were conducted in upper-middle and low income countries (China, Indonesia, Malaysia, Turkey and Iran).
The studies mainly examined the age groups of 60 years and older, but in one study, the age group of 45 to 90 years was recruited [ 28 ]. In total, the present studies had totally 21,196 sample sizes that were performed in the general population. Short screening tools were used in all of the studies. The most widely used tool was the Mini Mental Status Examination (MMSE). The possibility of cognitive impairment was examined, so that in 17 studies (85%) [ 18 , 19 , 20 , 21 , 22 , 23 , 24 , 26 , 28 , 29 , 30 , 31 , 32 , 33 , 34 , 35 , 36 ], MMSE was used as a reference or index test. Due to the fact that the purpose of this study was to evaluate screening programs in the primary care ward, all studies were performed in primary care centers or family physician office. Screening was performed by family physicians or nurses or health care workers, and those whose cognitive status was positive at the first level (cognitive impairment), were referred to the secondary level (specialist clinics or psychiatrists or hospitals).
Screening tests
As an index test, all studies used short tools to diagnose cognitive disorders. MMSE were used in 14 studies [ 18 , 19 , 20 , 21 , 22 , 23 , 25 , 26 , 27 , 28 , 29 , 30 , 32 , 33 , 34 , 38 ], General Practitioner Assessment of Cognition (GPCOG) in two studies [ 18 , 26 ], Test Your Memory (TYM) in two study [ 26 , 29 ], Early Dementia Questionnaire (EDQ) in two studies [ 32 , 33 ], Ascertain Dementia 8-item (AD8) in one study [ 30 ], the Informant Questionnaire On Cognitive Decline in the Elderly (IQCODE) in one study [ 37 ], the Picture version of the Free and Cued Selective Reminding Test with Immediate Recall (pFCSRT + IR) in two studies [ 36 , 38 ], Malay Version Rowland Universal Dementia Assessment Scale (M-RUDAS) in one study [ 24 ], a new screening method to support diagnosis of dementia (DemTect) in one study [ 34 ], and the Consortium to Establish a Registry for Alzheimer's Disease (CERAD) in one study [ 27 ]. Also, as a reference test, 10 studies have used the agreement of psychiatrists or geriatricians [ 20 , 21 , 23 , 25 , 27 , 28 , 31 , 35 , 36 , 37 ], one study [ 19 ] used CAMCOG, eight studies used MMSE [ 20 , 24 , 26 , 29 , 30 , 33 , 34 , 37 ] and two studies used MOCA [ 19 , 30 ] (Table 1 ).
EDQ and MMSE
The accuracy of EDQ diagnosis and its comparison with MMSE has been studied in two studies [ 32 , 33 ]. In these studies, the sensitivity for EDQ was (0.669, 0.799) and the specificity was (0.477, 0.651). Positive and negative predictive values for EDQ were 23.5% and 93.2%, respectively. In one study, EDQ was compared to MMSE [ 32 ]. The prevalence of dementia was estimated 52.3% by using EDQ and 15.2% by using MMSE. Based on the findings of these two studies, EDQ has been introduced as a suitable alternative tool for MMSE for screening in primary care settings. Since this tool is tailored with the patients’ symptoms in a specific condition, so it has a high accuracy of diagnosis. Given the high negative predictive value of this test, the researchers believed that fewer cases of patients would be concealed from screening. Also, as this tool is more powerful than MMSE in diagnosing patients in the early stages of the disease, it has high power for detecting patients in early stage of cognitive disorders.
GPCOG and MMSE
The comparison of these two tests has been done in only one study [ 18 ]. In this study, the mean area under the curve (AUC) for GPCOG and MMSE was estimated to be 0.92 and 0.91%, respectively. However, there were no statistically significant differences between the two parameters. The sensitivity of GPCOG at the cut-off point of 11/10 and the sensitivity of MMSE at the cut-off point of 24/23 were estimated to be 0.79 and 0.51, respectively, which was also statistically significant. Researchers have reported better performance for GPCOG than MMSE despite spending less time for interviewing.
AD8, MMSE, and MOCA
The diagnostic features of the AD8, MMSE, and MOCA tools have been compared in a study [ 30 ] by using ROC curve. In order to evaluate the accuracy of diagnosis of these tools, a panel of experts has been used as the reference standard. Based on the findings, among people over 60 years with a cut-off point of 3.4, the sub-curve area criterion (AUC) for AD8 is equal to 0.97 with a 95% confidence interval (0.95—0.99), with sensitivity of 0.91, positive predictive value of 0.63, and negative predictive value of 0.97. For MOCA with a cut-off point of 16.17 AUC, sensitivity, specificity, positive predictive value and negative predictive value were 0.94 (0.92- 0.97), 0.84, 0.89, 0.56 and 0.97, respectively. The AD8 is superior to the MMSE and has similar performance to the MOCA. The AD8 showed similar performance among people over 75 years of age. In the Yang study [ 19 ], MMSE and MOCA were used among elderly population. Although the purpose of this study was not to compare the two tools, both instruments performed well in terms of evaluator agreement. In the Larner’s study, AUC of 0.64, sensitivity and specificity were reported 0.80 and 0.86, respectively, for MMSE (index test) compared to MOCA (reference test). Due to the low sensitivity of MMSE, researchers have not considered this tool suitable for use in screening in low prevalence areas for cognitive impairment and have introduced alternative tools such as MOCA with more efficiency. The researchers believed that, regardless of the cost of using MMSE and copyright considerations, it is not suitable for use in primary care in low prevalence conditions.
SIS and MMSE
The Short Screening Tool (SIS) [ 20 ] was derived from the MMSE tool. The different cutting points for the sensitivity of SIS have been reported. The most suitable cutting point is three, which has the sensitivity equal to 0.86, the specificity of 0.87, and AUC 95% CI: 0.93 (0.89–0.97). Researchers have found good validity for the SIS and believed that the summary of the SIS reduces the interview time and it is suitable for use among illiterate elderly population.
pFCSRT + IR and MMSE
Grobber [ 38 ] compared the diagnostic characteristics of two combined tools picture version of the Free and Cued Selective Reminding Test with Immediate Recall (pFCSRT) plus IR and MMSE . The AUC for pFCSRT + IR was greater than the MMSE (86% vs. 72%, P < 0.026). For diagnosis of dementia with the same specificity (81%), the sensitivity of MMSE was 48% (cut-off point less than 24) and the sensitivity of pFCSRT + AR was 70% (cut-off point less than 27). The sensitivity was reported 74% for both tests (cut point less than 28 for pFCSRT) and (cut point more than > 26) for MMSE. The specificity of pFCSRT was 75% and MMSE was 62%. The accuracy of pFCSRT was superior to MMSE. These tools take 10 to 15 min to be completed.
Pooled estimation of diagnostic accuracy of MMSE test
Aggregation of the values reported in seven studies for the sensitivity, specificity, and AUC of the MMSE test were used for meta-analysis. The cumulative sensitivity, specificity, and AUC analysis was conducted only for MMSE instrument. Due to the high heterogeneity in the studies, it was not possible to perform pooled analysis for all instruments. The diagnostic performance of the instruments used in the studies was systematically reviewed comparatively, the findings of which are presented in the following section. The random effect model for the MMSE showed the pooled sensitivity equal to 0.73 (95% CI 0.57–0.90) (Fig. 2 ), the pooled specificity equal to 0.83 (95% CI 0.75—0.90) (Fig. 3 ), and the pooled AUC 0.88 (95% CI 0.83–0.93) (Fig. 4 ).
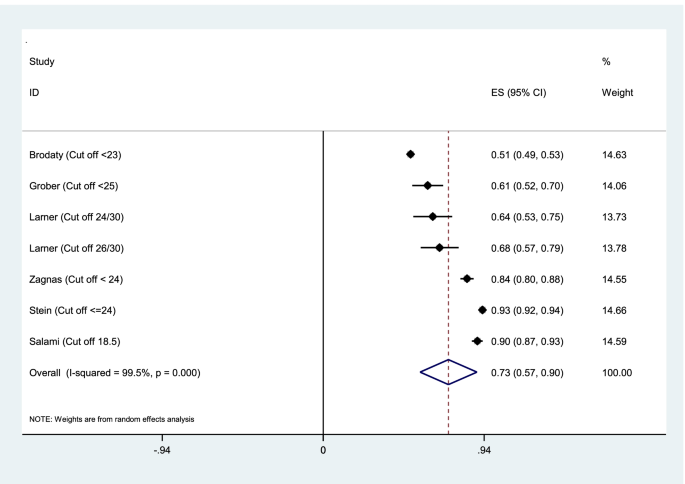
Results of aggregation of MMSE test sensitivity values in identifying cognitive disorders
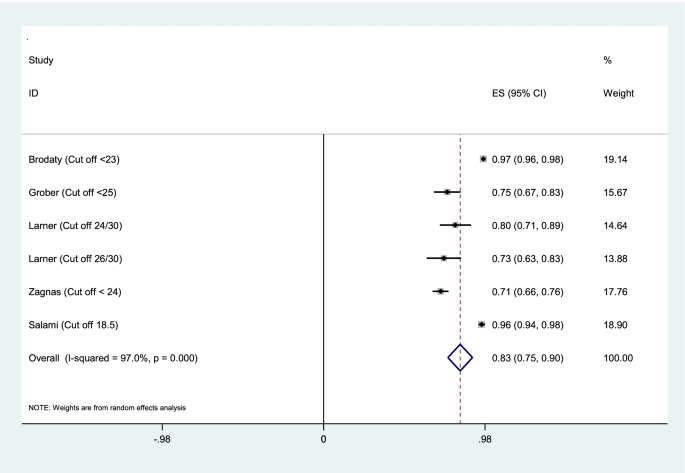
Results of aggregation of MMSE specificity feature values in identifying cognitive disorders
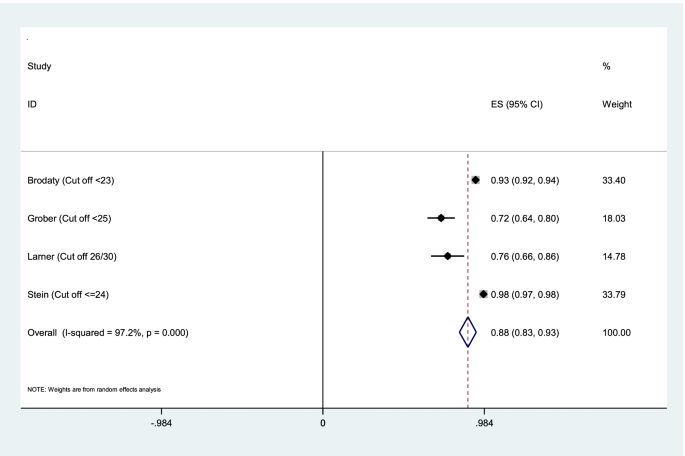
Results of aggregation of area under curve for MMSE test in identifying cognitive disorders
The risk of bias in the studies is shown in Table 2 . Also, the risk of bias and concern about the applicability of each domain of quality assessment studies based on QUADAS2 tool were shown in Fig. 5 . Kapa coefficient score was estimated 0.908 ( P < 0.0001) indicating strong agreement between two screening researchers.
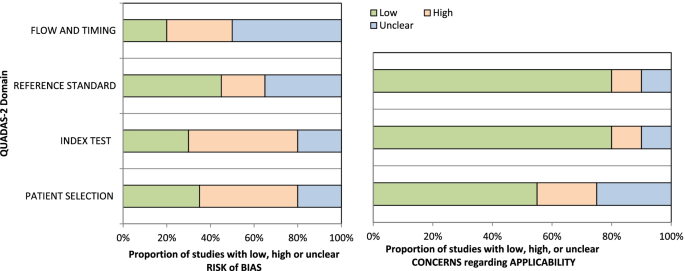
Risk of bias and concern about the applicability of each domain of quality assessment studies based on QUADAS2 tools
Subgroup analysis
Table 3 shows the results based on the sensitivity, specificity, and AUC of MMSE according to subgroup analyses to explore the origin of the heterogeneity between the studies. The random-effects pooled estimation for sensitivity was 0.71 (95% CI 0.53–0.88; p < 0.001), for specificity was 0.81 (95% CI 0.67–0.95; p < 0.001), and for AUC was 0.73 (95% CI 0.67–0.80; p < 0.001) for participants aged 75 years and older. The higher random effect pooled estimation for sensitivity for the groups with respect to country’s income was for 0.91 low income countries (95% CI 0.89–0.94). The higher random effect pooled estimation for specificity was 0.97 (95% CI 0.96–0.97; p < 0.001) and for AUC was 0.97 (0.64–0.94; p < 0.001), respectively, for the groups with respect to sample size > 1000.
Publication bias
Publication bias was highlighted and confirmed by funnel plots. The funnel plots in Fig. 6 testing publication within diagnostic accuracy of MMSE tool. The graphical results point to asymmetry with a majority of the studies clustering to the left of the mean. Large studies are shown at the top of the graph, and smaller studies are shown at the bottom.
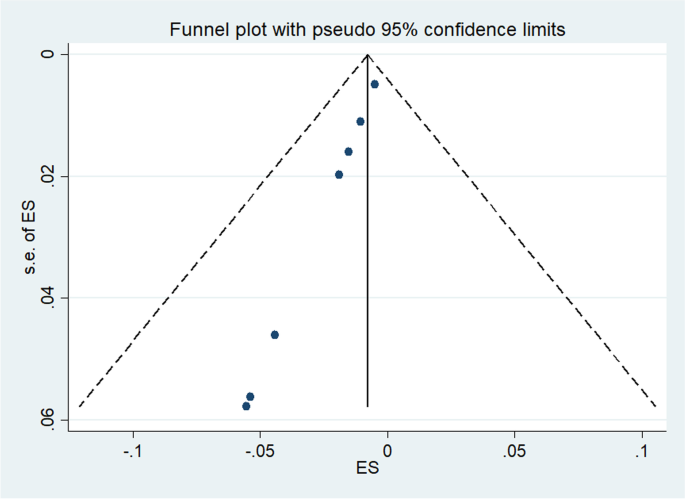
Funnel plot
The findings of the systematic review showed that the MMSE questionnaire is the most widely used tool and has been used as an indicator or reference test in most studies.
The findings of the present systematic review showed that there is insufficient evidence for community-based screening programs. The included final 21 studies in the systematic review also performed early detection of cognitive disorders on cross-sectional samples of the population and reported the accuracy of diagnosis of these tools. Of the 21 final studies, two studies [ 24 , 27 ] recommended routine screening for cognitive disorders and three studies recommended against screening [ 19 , 31 , 36 ] that have pointed to the inability to implement community based screening, especially in low-income countries. Some substantial barriers of screening for cognitive disorders in low-income countries were highlighted such as limited resources for serving large population, insufficient training, and shortage of general physicians [ 19 ]. Another issues like living of most of older adults in remote rural areas or urban areas without having access the centers where offer routine screening tests [ 40 ]. These factors, along with other epidemiological and social factors like low educational level, low socio-economic status of older population, time and financial constraints, diagnostic uncertainty, stigma [ 35 ], and access of such people to health care centers contribute to the pause and challenging of screening programs in low income countries [ 5 , 41 ] However, Koch et al. [ 35 ] in a rapid appraisal of barriers to the diagnosis of cognitive disorders and dementia stated that health care systems were accountable for the several mentioned barriers [ 42 ]. Eichler [ 24 ] and Pandahita [ 27 ] agreements for performing routine screening were the high percentage of undiagnosed patients in primary care settings and also the fact that the proposed screening test did not provide enough information about the feasibility of screening. Therefore, these two studies would not be recognized sufficient evidence for screening cognitive disorders. The findings are in line with the recommendations of the US Preventive Services Committee Task Force (USPSTF) in 2003, 2011, 2014 and most recently in 2020. The committee believes that there was no evidence to prove the screening program could improve the current care process [ 12 ]. The Alzheimer's Association of the United States cites this evidence and recommends the inclusion of an early detection program for cognitive disorders in the annual geriatric visits [ 5 , 43 ]. Iliffe et al. [ 43 ] stated that they were not able to identify an advantage for routine screening test, but they considered the possibility of early detection in primary care. Therefore, the program for diagnosing cognitive disorders is beyond the informal observation by a physician and is an ongoing process that is diagnosed during various stages of senile disorder. Counselling and interviewing before and after the diagnosis of the disorder is an important part of the diagnosis process and the use of caregivers and elderly people would be effective in diagnosing the disease [ 43 ]. The National Institute for Clinical Excellence (NICE) and the UK National Health System's advisory did not consider routine screening to be cost-effectiveness in their recommendations in 2006.
More than 12 different tools have been used in the final studies. MMSE tool is the most widely used and common tool in this field. Comparison of instruments showed that IQCODE, GPCOG, AD8, MOCA, PFCSRT + IR and EDQ instruments had detection power equal to or higher than MMSE. Even the MMSE short tool had good diagnostic performance. The present finding shows that the above tools can replace MMSE in the diagnosis of cognitive disorders and dementia. In addition, MMSE because of being long, not free and is biased towards the literacy level of the participants, the Alzheimer's Association has introduced six criteria for selecting the right tool, including evaluation time of less than 5 min, validation evidence in primary care, usability by non-medical staff, appropriate psychometric properties, insensitivity to literacy, language and culture bias, and it’s free availability. The Alzheimer's Association based on the findings of the previously published systematic review studies [ 44 , 45 , 46 , 47 ] showed appropriate tools for assessing patients' cognition, including GPCOG, Mini-Cog, and MIS, and interviews with IQCODE, AD8, and GPCOG caregivers. Our systematic review findings are also in line with the recommendations of the Alzheimer's Association. MOCA, IQCODE, GPCOG and MMSE instruments have also been validated in Iran [ 29 , 48 , 49 ], but participants were recruited from the general population setting rather than the primary care units. Consistent with our study, a review study on brief cognitive screening instruments found that MMSE is the most frequently used cognitive screening tool in the community and primary care. The study also highlighted that mini cognition (Mini-cog), memory impairment screen (MIS), and the general practitioner assessment of cognition (GPCOG) were beneficial in primary care setting and recommended for use [ 47 ]. Based on the findings, practicality, psychometric properties of instruments, validation in a community, general population, or referring people for primary care setting, as well as utility, efficacy, and administration time were major criteria for implementing the cognitive screening instruments in primary care and community programs especially in low income countries.
Limitations
The available studies were carried out in the variety of high and middle income countries. There was no study in low level country to clarify the advantages or disadvantages of screening programs in these countries. Overall, additional researches are needed to identify the best screening tool in low income countries.
There was insufficient evidence for routine and general screening to identify cognitive disorders. However, due to the high incidence of undiagnosed patients and the benefits of early diagnosis in caregiver management, the integration of early diagnosis into annual or periodic geriatric care programs has been used in most high-income countries. The use of non-medical staff in the initial assessment can be suggested as a suitable option, especially in countries that face a shortage of medical staff. Although MMSE is the most widely used diagnostic tool, according to the current systematic review, MOCA, GPCOG and MIS tools can be used to evaluate patients and IQCODE, AD8 and GPCOG tools can be used to evaluate their caregivers with equal or better performance than MMSE.
Availability of data and material
The data collection tools and datasets generated and/or analyzed during the current study are available from the corresponding author on reasonable request.
Abbreviations
A Quick Test of Cognitive Speed,
Rapid Recognition Screening
Six-Item Screener
Preferred report items for systematic review and meta-analysis
Quality Assessment of Diagnostic Accuracy Studies-2
Annual wellness visit
Mini–mental state examination
General practitioner assessment of cognition
Ascertain dementia 8-item
Test your memory
Early Dementia Questionnaire
The picture version of the Free and Cued Selective Reminding Test with Immediate
The Recall Malay Version Rowland Universal Dementia Assessment Scale
A new screening method to support diagnosis of dementia
Pink Jta, O'Brien Jpooap, Robinson Lpopc, ageing, Longson DclpcoGC, on behalf of the Guideline C: Guidelines: Dementia: assessment, management and support: summary of updated NICE guidance. BMJ. 2018;23(361):k2438.
Gauthier S R-NP, Morais JA, & Webster C. : World Alzheimer Report 2021: Journey through the diagnosis of dementia. In . London, England: Alzheimer’s Disease International.; 2021.
Jack CR Jr, Bennett DA, Blennow K, Carrillo MC, Dunn B, Haeberlein SB, Holtzman DM, Jagust W, Jessen F, Karlawish J. NIA-AA research framework: toward a biological definition of Alzheimer’s disease. Alzheimers Dement. 2018;14(4):535–62.
Article PubMed PubMed Central Google Scholar
Santos CY, Snyder PJ, Wu W-C, Zhang M, Echeverria A, Alber J. Pathophysiologic relationship between Alzheimer’s disease, cerebrovascular disease, and cardiovascular risk: a review and synthesis. Alzheimers Dement (Amst). 2017;7:69–87.
Article Google Scholar
Cordell CB, Borson S, Boustani M, Chodosh J, Reuben D, Verghese J, Thies W, Fried LB. Alzheimer’s Association recommendations for operationalizing the detection of cognitive impairment during the Medicare Annual Wellness Visit in a primary care setting. Alzheimers Dement. 2013;9(2):141–50.
Article PubMed Google Scholar
Bradford A, Kunik ME, Schulz P, Williams SP, Singh H. Missed and delayed diagnosis of dementia in primary care: prevalence and contributing factors. Alzheimer Dis Assoc Disord. 2009;23(4):306–14.
Bianchetti A, Ferrara N, Padovani A, Scarpini E, Trabucchi M, Maggi S. Timely detection of mild cognitive impairment in Italy: an expert opinion. Journal of Alzheimer’s disease : JAD. 2019;68(4):1401–14.
Woods B, Arosio F, Diaz A, Gove D, Holmerová I, Kinnaird L, Mátlová M, Okkonen E, Possenti M, Roberts J, et al. Timely diagnosis of dementia? family carers’ experiences in 5 European countries. Int J Geriatr Psychiatry. 2019;34(1):114–21.
Dhedhi SA, Swinglehurst D, Russell J. “Timely” diagnosis of dementia: what does it mean? A narrative analysis of GPs’ accounts. BMJ open. 2014;4(3):e004439.
Werner P, Goldstein D, Karpas DS, Chan L, Lai C. Help-seeking for dementia: a systematic review of the literature. Alzheimer Dis Assoc Disord. 2014;28(4):299–310.
Petersen RC, Roberts RO, Knopman DS, Boeve BF, Geda YE, Ivnik RJ, Smith GE, Jack CR Jr. Mild cognitive impairment: ten years later. Arch Neurol. 2009;66(12):1447–55.
Patnode CD, Perdue LA, Rossom RC, Rushkin MC, Redmond N, Thomas RG, Lin JS. Screening for cognitive impairment in older adults: updated evidence report and systematic review for the US Preventive Services Task Force. JAMA. 2020;323(8):764–85.
Rosenbaum S. The patient protection and affordable care act: implications for public health policy and practice. Public Health Rep. 2011;126(1):130–5.
National Collaborating Centre for Mental H: National Institute for Health and Clinical Excellence: Guidance. In: Dementia: A NICE-SCIE Guideline on Supporting People With Dementia and Their Carers in Health and Social Care. edn. Leicester (UK): British Psychological Society Copyright © 2007, The British Psychological Society & The Royal College of Psychiatrists.; 2007.
Pelegrini LNC, Mota GMP, Ramos CF, Jesus E, Vale FAC. Diagnosing dementia and cognitive dysfunction in the elderly in primary health care: a systematic review. Dement Neuropsychol. 2019;13(2):144–53.
Lin JS, O’Connor E, Rossom RC, Perdue LA, Eckstrom E. Screening for cognitive impairment in older adults: A systematic review for the U.S. Preventive Services Task Force. Ann Intern Med. 2013;159(9):601–12.
PubMed Google Scholar
Whiting PF, Rutjes AW, Westwood ME, Mallett S, Deeks JJ, Reitsma JB, Leeflang MM, Sterne JA, Bossuyt PM. QUADAS-2: a revised tool for the quality assessment of diagnostic accuracy studies. Ann Intern Med. 2011;155(8):529–36.
Brodaty H, Connors MH, Loy C, Teixeira-Pinto A, Stocks N, Gunn J, Mate KE, Pond CD. Screening for dementia in primary care: a comparison of the GPCOG and the MMSE. Dement Geriatr Cogn Disord. 2016;42(5–6):323–30.
Yang Y, Xiao LD, Deng L, Wang Y, Li M, Ullah S. Nurse-led cognitive screening model for older adults in primary care. Geriatr Gerontol Int. 2015;15(6):721–8.
Xue J, Chiu HFK, Liang J, Zhu T, Jiang Y, Chen S. Validation of the Six-Item Screener to screen for cognitive impairment in primary care settings in China. Aging Ment Health. 2018;22(4):453–7.
Larner AJ. Mini-Mental State Examination: diagnostic test accuracy study in primary care referrals. Neurodegenerative disease management. 2018;8(5):301–5.
Stein J, Luppa M, Kaduszkiewicz H, Eisele M, Weyerer S, Werle J, Bickel H, Mösch E, Wiese B, Prokein J, et al. Is the Short Form of the Mini-Mental State Examination (MMSE) a better screening instrument for dementia in older primary care patients than the original MMSE? Results of the German study on ageing, cognition, and dementia in primary care patients (AgeCoDe). Psychol Assess. 2015;27(3):895–904.
Thyrian JR, Eichler T, Michalowsky B, Wucherer D, Reimann M, Hertel J, Richter S, Dreier A, Hoffmann W. Community-dwelling people screened positive for dementia in primary care: a comprehensive, multivariate descriptive analysis using data from the DelpHi-Study. Journal of Alzheimer’s disease : JAD. 2016;52(2):609–17.
Eichler T, Thyrian JR, Hertel J, Michalowsky B, Wucherer D, Dreier A, Kilimann I, Teipel S, Hoffmann W. Rates of formal diagnosis of dementia in primary care: the effect of screening. Alzheimers Dement (Amst). 2015;1(1):87–93.
Zaganas IV, Simos P, Basta M, Kapetanaki S, Panagiotakis S, Koutentaki I, Fountoulakis N, Bertsias A, Duijker G, Tziraki C. The Cretan aging cohort: cohort description and burden of dementia and mild cognitive impairment. Am J Alzheimers Dis Other Demen. 2019;34(1):23–33.
Iatraki E, Simos PG, Bertsias A, Duijker G, Zaganas I, Tziraki C, Vgontzas AN, Lionis C. Cognitive screening tools for primary care settings: examining the “Test Your Memory” and “General Practitioner assessment of Cognition” tools in a rural aging population in Greece. Eur J Gen Pract. 2017;23(1):171–8.
Pandhita SG, Sutrisna B, Wibowo S, Adisasmita AC, Rahardjo TBW, Amir N, Rustika R, Kosen S, Syarif S, Wreksoatmodjo BR. Decision tree clinical algorithm for screening of mild cognitive impairment in the Elderly in Primary Health Care: Development, Test of Accuracy, and Time-Effectiveness Analysis. Neuroepidemiology. 2020;54(3):243–50.
Petrazzuoli F, Palmqvist S, Thulesius H, Buono N, Pirrotta E, Cuffari A, Cambielli M, D'Urso M, Farinaro C, Chiumeo F, Marsala V, Wiig EH. A Quick Test of Cognitive Speed: norm-referenced criteria for 121 Italian adults aged 45 to 90 years. Int Psychogeriatr. 2014:1–8. https://doi.org/10.1017/S1041610214000787 . Epub ahead of print.
Salami M, Alinaghipour A, Daneshvar R, Hamidi GA, Agahi A, Soheili M, Akbari H, Esmaeili Taba SM. Adapted MMSE and TYM cognitive tests: how much powerful in screening for Alzheimer’s disease in Iranian people. Aging Ment Health. 2020;24(6):1010–7.
Article CAS PubMed Google Scholar
Chan QL, Xu X, Shaik MA, Chong SST, Hui RJY, Chen CL-H, Dong Y. Clinical utility of the informant AD8 as a dementia case finding instrument in primary healthcare. J Alzheimers Dis. 2016;49(1):121–7.
Teixeira L, Dos Santos PM, Alves S, Azevedo MJ, Duarte MG, Leuschner A, Paúl C. Screening of dementia in Portuguese primary care: Methodology, assessment tools, and main results. Front Med. 2017;13(4):197.
Arabi Z, Aziz NA, Abdul Aziz AF, Razali R, Wan Puteh SE. Early Dementia Questionnaire (EDQ): a new screening instrument for early dementia in primary care practice. BMC Fam Pract. 2013;14:49.
Arabi Z, Syed Abdul Rahman SA, Hazmi H, Hamdin N. Reliability and construct validity of the Early Dementia Questionnaire (EDQ). BMC Geriatr. 2016;16(1):202.
Shaaban J, Aziz AA, Abdullah Z, Razak AA. Validation of the malay version of rowland universal dementia assessment scale (m-rudas) among elderly attending primary care clinic. International Medical Journal. 2013;20(5):555–8.
Google Scholar
KocOkudur S, Dokuzlar O, Kaya D, Soysal P, Isik AT. Triple test plus rapid cognitive screening test: a combination of clinical signs and a tool for cognitive assessment in older adults. Diagnostics. 2019;9(3):97.
Grober E, Ehrlich AR, Troche Y, Hahn S, Lipton RB. Screening older Latinos for dementia in the primary care setting. J Int Neuropsychol Soc. 2014;20(8):848–55.
Grober E, Mowrey WB, Ehrlich AR, Mabie P, Hahn S, Lipton RB. Two-stage screening for early dementia in primary care. J Clin Exp Neuropsychol. 2016;38(9):1038–49.
Grober E, Wakefield D, Ehrlich AR, Mabie P, Lipton RB. Identifying memory impairment and early dementia in primary care. Alzheimers Dement (Amst). 2017;6:188–95.
https://datatopics.worldbank.org/world-development-indicators/the-world-by-income-and-region.html Accessed 14 Jun
Cullen B, O’Neill B, Evans JJ, Coen RF, Lawlor BA. A review of screening tests for cognitive impairment. J Neurol Neurosurg Psychiatry. 2007;78(8):790–9.
Brucki SMD, Nitrini R. Subjective memory impairment in a rural population with low education in the Amazon rainforest: an exploratory study. Int Psychogeriatr. 2008;21(1):164–71.
Tabrizi JS, HaghGoshayie E, Doshmangir L, Yousefi M. New public management in Iran’s health complex: a management framework for primary health care system. Primary health care research & development. 2018;19(3):264–76.
Iliffe S, Robinson L, Brayne C, Goodman C, Rait G, Manthorpe J, Ashley P. Primary care and dementia: 1. diagnosis, screening and disclosure. Int J Geriatr Psychiatry. 2009;24(9):895–901.
Setter SM, Neumiller JJ, Weeks DL, Borson S, Scanlan JM, Sonnett TE. Screening for undiagnosed cognitive impairment in homebound older adults. The Consultant pharmacist : the journal of the American Society of Consultant Pharmacists. 2009;24(4):299–305.
Holsinger T, Boustani M, Abbot D, Williams JW. Acceptability of dementia screening in primary care patients. Int J Geriatr Psychiatry. 2011;26(4):373–9.
Milne A, Culverwell A, Guss R, Tuppen J, Whelton R. Screening for dementia in primary care: a review of the use, efficacy and quality of measures. Int Psychogeriatr. 2008;20(5):911–26.
Ismail Z, Rajji TK, Shulman KI. Brief cognitive screening instruments: an update. Int J Geriatr Psychiatry. 2010;25(2):111–20.
Badrkhahan SZ, Sikaroodi H, Sharifi F, Kouti L, Noroozian M. Validity and reliability of the Persian version of the Montreal Cognitive Assessment (MoCA-P) scale among subjects with Parkinson’s disease. Appl Neuropsychol Adult. 2020;27(5):431–9.
Foroughan M, Jafari Z, Farahani I, Rashedi V. Validity and Reliability of the Informant Questionnaire on Cognitive Decline in the Elderly (IQCODE): Preliminary Findings Among the Older Population of Iran. GeroPsych. 2019;32:145–51.
Download references
Acknowledgements
We acknowledge the contributions of Tabriz University of Medical Sciences, Tabriz, Iran for providing facilities to the study.
Tabriz University of Medical Sciences provided facilities.
Author information
Authors and affiliations.
Health Education and Health Promotion Department, School of Public Health, Tabriz University of Medical Sciences, Tabriz, Iran
Leila Karimi & Leila Jahangiry
School of Management and Medical Informatics, Tabriz Health Services Management Research Center, Tabriz University of Medical Sciences, Tabriz, Iran
Alireza Mahboub–Ahari
Research Center for Evidence Based Medicine, Tabriz University of Medical Sciences, Tabriz, Iran
Leila Jahangiry
Road Traffic Injury Research Center, Tabriz University of Medical Sciences, Tabriz, Iran
Homayoun Sadeghi-Bazargani
Psychiatrics Research Center, Tabriz University of Medical Sciences, Tabriz, Iran
Mostafa Farahbakhsh
You can also search for this author in PubMed Google Scholar
Contributions
LJ is responsible for the study design. LJ and LK did the analyses. LJ and AMA were responsible for data interpretation. LK, AMA, HSB, MF helped in the study design and data gathering, LK and AMA helped in the drafting of the manuscript. All authors have read and approved the final manuscript.
Corresponding author
Correspondence to Leila Jahangiry .
Ethics declarations
Ethics approval and consent to participate.
The study received ethical approval from the Ethics Committee of Tabriz University of Medical Sciences (NO: IR.TBZMED.REC. 1398. 139).
Consent for publication
Not applicable
Competing interests
The authors declare no conflicts of interest.
Additional information
Publisher’s note.
Springer Nature remains neutral with regard to jurisdictional claims in published maps and institutional affiliations.
Rights and permissions
Open Access This article is licensed under a Creative Commons Attribution 4.0 International License, which permits use, sharing, adaptation, distribution and reproduction in any medium or format, as long as you give appropriate credit to the original author(s) and the source, provide a link to the Creative Commons licence, and indicate if changes were made. The images or other third party material in this article are included in the article's Creative Commons licence, unless indicated otherwise in a credit line to the material. If material is not included in the article's Creative Commons licence and your intended use is not permitted by statutory regulation or exceeds the permitted use, you will need to obtain permission directly from the copyright holder. To view a copy of this licence, visit http://creativecommons.org/licenses/by/4.0/ . The Creative Commons Public Domain Dedication waiver ( http://creativecommons.org/publicdomain/zero/1.0/ ) applies to the data made available in this article, unless otherwise stated in a credit line to the data.
Reprints and permissions
About this article
Cite this article.
Karimi, L., Mahboub–Ahari, A., Jahangiry, L. et al. A systematic review and meta-analysis of studies on screening for mild cognitive impairment in primary healthcare. BMC Psychiatry 22 , 97 (2022). https://doi.org/10.1186/s12888-022-03730-8
Download citation
Received : 25 February 2021
Accepted : 25 January 2022
Published : 09 February 2022
DOI : https://doi.org/10.1186/s12888-022-03730-8
Share this article
Anyone you share the following link with will be able to read this content:
Sorry, a shareable link is not currently available for this article.
Provided by the Springer Nature SharedIt content-sharing initiative
- Early diagnosis
- Mild cognitive impairment
- Primary health care
BMC Psychiatry
ISSN: 1471-244X
- Submission enquiries: [email protected]
- General enquiries: [email protected]

Sildenafil (‘Viagra’) improves brain blood flow and could help to prevent dementia
A new trial conducted by the University of Oxford reveals that sildenafil, commonly known as Viagra, enhances blood flow to the brain and improves the function of brain blood vessels in patients at a heightened risk of vascular dementia.
This new study, published in Circulation Research , marks a potentially pivotal step in the fight against this debilitating condition.
Dr. Alastair Webb, as Associate Professor at the Wolfson Centre for Prevention of Stroke and Dementia at Oxford University said: 'This is the first trial to show that sildenafil gets into the blood vessels in the brain in people with this condition, improving blood flow and how responsive these blood vessels are. These two key factors are associated with chronic damage to the small blood vessels in the brain, which is the commonest cause of vascular dementia. This demonstrates the potential of this well-tolerated, widely-available drug to prevent dementia, which needs testing in larger trials'.
The significance of this research lies in its potential to transform the treatment and prevention of vascular dementia, which currently lacks specific therapies. Chronic damage to the small blood vessels in the brain is not only the leading cause of vascular dementia but also contributes to 30% of strokes and 80% of brain bleeds. High blood pressure, reduced blood flow to the brain, and impaired blood vessel function exacerbate these conditions, making the findings of this trial particularly crucial.
The OxHARP trial was a meticulously designed double-blind, placebo-controlled study involving 75 participants who had experienced a minor stroke and showed signs of mild to moderate small vessel disease. Each participant received sildenafil, a placebo, and cilostazol (a similar drug) over three-week periods in a randomised order. The study employed cardiovascular physiology tests, ultrasound, and functional MRI scans to evaluate the drugs' effects.
Key findings include:
- Sildenafil increased blood flow in both large and small brain vessels as measured by ultrasound and MRI scans.
- Sildenafil enhanced the blood flow response to carbon dioxide, indicating improved cerebrovascular function.
- Both sildenafil and cilostazol lowered blood vessel resistance in the brain.
- Sildenafil caused fewer side effects compared to cilostazol, particularly with less incidence of diarrhoea.
Looking ahead, the next steps involve larger-scale trials to confirm these findings and explore sildenafil's potential in preventing vascular dementia on a broader scale.
This research was generously funded by the Wellcome Trust and supported by the National Institute for Health and Care Research, with special thanks to all the partners and participants involved.
Professor Peter Rothwell, Founding Director of the Wolfson Centre for Prevention of Stroke and Dementia said: 'Professor Webb's findings are very encouraging and highlight the potential for preventing vascular dementia using existing drugs that target the underlying reduction in flow in the small blood vessels in the brain.'
Read the full research paper here - Cerebrovascular Effects of Sildenafil in Small Vessel Disease: The OxHARP Trial

DISCOVER MORE
- Support Oxford's research
- Partner with Oxford on research
- Study at Oxford
- Research jobs at Oxford
You can view all news or browse by category
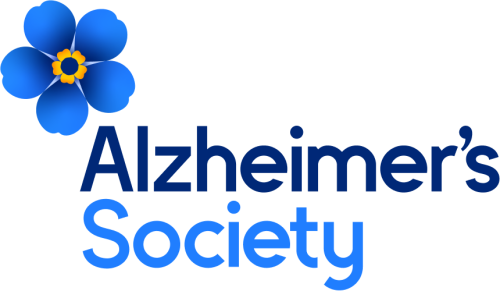
General Election 2024: Make Dementia a Priority
At this General Election candidates from all political parties must acknowledge that dementia is the UK’s biggest killer, and the greatest health and social care challenge we face.
Through eleven General Elections, Alzheimer’s Society has campaigned tirelessly for dementia to be a priority with our national decision makers. From Thatcher to Blair, Brown to Sunak, years have rolled by, but we’re still waiting.
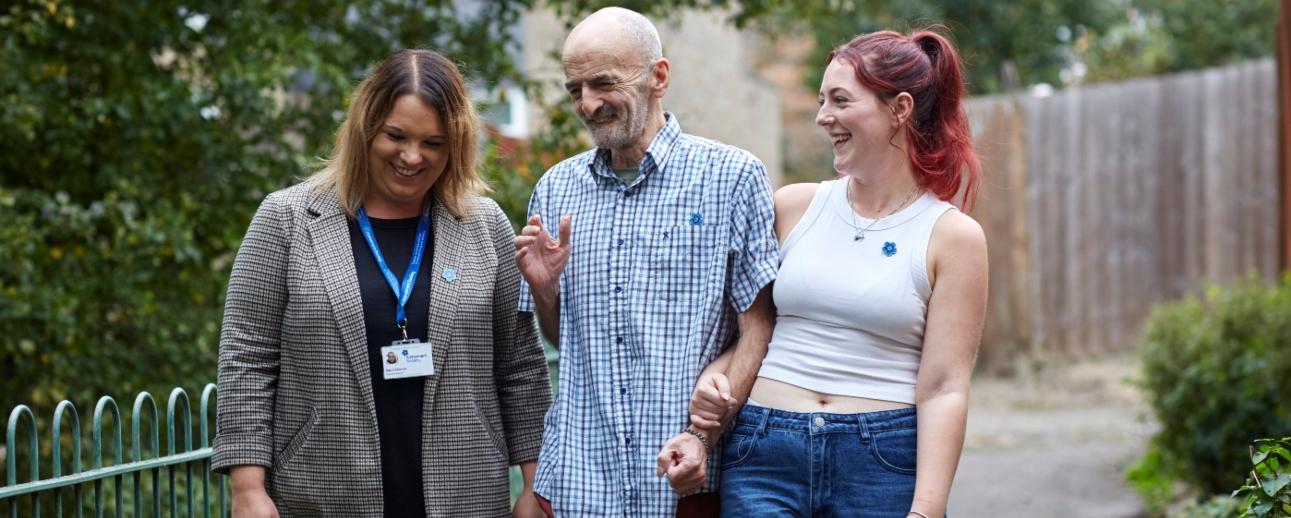
Why the next Government must Make Dementia a Priority
A third of people living with dementia in England and Northern Ireland and half of people living with dementia in Wales do not have a diagnosis. This means they can’t access the vital care and support they deserve and will not be able to receive new treatments for which they may be eligible, if approved.
The dementia diagnosis rate targets across the UK are not ambitious enough. For no other condition would it be acceptable for so many people to be in the dark about their diagnosis.
While the potential of new disease modifying treatments (DMTs) represent a defining moment for Alzheimer’s disease, the NHS is not ready to deliver breakthrough treatments if they become available.
Dementia diagnosis is not widely enough available, nor is it happening sufficiently early or accurately. Healthcare system preparedness is key, which requires investment in diagnostic equipment and specialised workforce.
Whilst there is hope for the future with treatments in the pipeline, we must also appreciate hundreds of thousands of people living with dementia are reliant on the social care system now and may never be eligible for these new drugs.
It is vital we have a social care system set up to provide quality, personalised care, delivered by a well-trained and supported workforce.
The next Government must:
Increase dementia diagnosis rates and accelerating system readiness for disease modifying treatments (dmts) .
- New, ambitious dementia diagnosis rate targets should be set for England, Wales and Northern Ireland accompanied by funded plans to deliver early, accurate diagnosis at scale. Plans must be published by healthcare systems across the UK on how disease-modifying treatments will be delivered, including through investment in the diagnostic workforce and infrastructure.
Deliver a social care workforce strategy
- We need a long-term social care workforce strategy so that we have enough well-trained and supported staff to provide high quality, personalised dementia care to everyone who needs it.
- The strategy must include a requirement for evidence-based dementia training for all staff supporting people living with dementia to Tier 2 of the Dementia Training Standards Framework or equivalent.
Decisions relating to health (including how to roll out any future treatments) and social care are devolved. If you would like to know more about what we are doing in Wales and Northern Ireland, please contact [email protected] .
Sign our pledge
A third of people living with dementia in England and Northern Ireland and half of those living with dementia in Wales do not have a diagnosis, meaning they can't access vital support or treatment. Sign our pledge calling for dementia to be made a priority by the next UK Government.
Think this page could be useful to someone? Share it:
- Email this page to a friend.
Sign up for dementia support by email
Our regular support email includes the latest dementia advice, resources, real stories and more.
You can change what you receive at any time and we will never sell your details to third parties. Here’s our Privacy Policy .

IMAGES
COMMENTS
THE FULL REPORT Project directors: Professor Martin Knapp and Professor Martin Prince Research team: Dr Emiliano Albanese, ... 3.1 Number of people in the UK with dementia (2005) 24 3.2 Projected increases in the number of people with dementia in the UK, by age group (2005 -2051) 24
It's really important to us to be open and transparent with everyone who comes into contact with us. Our annual reports will show you what we've been working on for the past year and the years before. Annual report 2023 /6.35 MB. Annual report 2022 /6.50 MB. Impact Report 2022-23 /1.44 MB.
The All-Party Parliamentary Group on Dementia reported, in July 2013, an estimate of nearly 25,000 people living with dementia from BAME communities in England and Wales. This number is expected to grow to nearly 50,000 by 2026 and over 172,000 by 2051. This is nearly a seven-fold increase in 40 years.
In the UK, currently around 700,000 people have dementia but this is estimated to rise to 1 million by 2020 and 1.7 million by 2050, an increase of over 150% [ 1 ]. The current costs of caring for ...
Dementia UK Annual Report 2022-23 5. Every three minutes, someone in the UK develops dementia: a progressive and complex condition that can be devastating for the whole family. And too ... Nurses in areas without full-time nurse services, to reach even more families In hospitals We will: • create new Admiral Nurse posts to support
It is an overview of the full report, Dementia UK: Second edition, which will be published by Alzheimer's Society in autumn 2014. This document presents the best available evidence for the current cost and prevalence of dementia in the UK.The full report was commissioned by Alzheimer's Society. It was researched and written by King's ...
The Dementia UK report was published in 2014. Read our latest report for up-to-date projections on the scale and cost of dementia until 2040. The 2014 Dementia UK report found that there will be 850,000 people living with dementia in the UK by 2015 and that dementia costs the UK £26 billion a year. The research, commissioned through King's ...
2 Dementia UK mpact report 2021-2022. Dementia UK Impact report 2021-2022 3. There are over 200 types of dementia - the most common are Alzheimer's disease, vascular dementia, Lewy body dementia, ... four new full-time Admiral Nurse posts to work in the clinics. We are currently working on plans to launch
economic benefits in the UK between 2020 and 2040. • Full-time salaries in dementia R&D are on average 41% higher than the average salary across all jobs in a region. Accordingly, full-time salaries in dementia administration staff are on average 24.5% higher than the average salary across all jobs in a region.
3. Worldwide, dementia is responsible for 11.2% of all years lived with disability among people aged over 60 years. In the UK alone, there are now almost 700,000 people living with dementia, equivalent to 1.1% of the UK population, at a cost of £17 billion per year, and prevalence is projected to rise by 38% over the next 15 years.
THE FULL REPORT Project directors: Professor Martin Knapp and Professor Martin Prince Research team: Dr Emiliano Albanese, ... 3.1 Number of people in the UK with dementia (2005) 23 3.2 Projected increases in the number of people with dementia in the UK, by age group (2005 -2051) 23
Download full report Download 'Dementia: policy, services and statistics overview' report (344 KB , PDF) There are an estimated 850,000 people with dementia in the UK. By 2040, the number of people with the condition is expected to double. The UK Government has set an objective for England to be a world leader in fighting dementia and has ...
Abstract. This report establishes an accurate estimate of the numbers of people in the UK who currently have dementia. It also provides authoritative estimates for the numbers of people who will have dementia in the years up to 2051. The services and treatments currently provided to support people with dementia are reviewed.
The prevalence rate of dementia among older people in the UK is estimated to be 7.1% in 2019 (Figure 1). Among the 885,000 people with dementia in the UK, 127,000 have mild dementia, 246,000 have moderate dementia and 511,000 have severe dementia (Table 1). Of the four countries, England has the highest overall prevalence rate of dementia
Translating Science into breakthroughs: the future of late-stage dementia clinical trials in the UK. May 2021. People with dementia are at risk of missing out on new treatments if the UK does not improve how it hosts clinical trials, according to a new report from Alzheimer's Research UK.
2 Dementia UK Impact Report 2022-23. Dementia UK Impact Report 2022-23 3. Dementia is an umbrella term for a range of progressive conditions that ... supporters - and we are committed to full transparency regarding how we spend this money. We encourage creativity and innovation
The number of older people, including those living with dementia, is rising, as younger age mortality declines. However, the age-specific incidence of dementia has fallen in many countries, probably because of improvements in education, nutrition, health care, and lifestyle changes. Overall, a growing body of evidence supports the nine potentially modifiable risk factors for dementia modelled ...
The source of the report and the date of the report for all four types of dementia reports were obtained from the algorithmically defined dementia outcomes, which combine participants' self-reported medical conditions, linked hospital diagnoses, and death registries provided by the UK Biobank group and validated by a different study [17, 18 ...
Dementia UK Full Report-Dementia UK Full Report-Flo Xanji. See Full PDF Download PDF. See Full PDF Download PDF. Related Papers. ... (pp. 1 4-1 5) and the Unit's analyses for the 2006 Wanless report. Recent dementia studies at LSE have continued in this vein. Download Free PDF View PDF. Dementia (London, England)
An international team has developed a method for predicting dementia with over 80 per cent accuracy and up to nine years pre-diagnosis. Home; ... on record with the UK Biobank. The findings showed that the model had accurately predicted onset of dementia up to nine years before an official diagnosis was made, and with greater than 80 per cent ...
8 Dementia UK Annual Report 2021 • Young onset dementia affects people aged 65 and under • Over 42,300 people, or 5.2% of the total number of people with dementia, are estimated to have a diagnosis of young onset dementia, although the actual number may be much higher
Cognitive disorders and dementia have an important effect on individual independence and orientation. Alzheimer's is characterized by impaired memory and dysfunction; it is one of the areas of aphasia, apraxia, amnesia, and dysfunction, which has a significant impact on individual and social functioning [].According to the World Alzheimer Report, over 55 million people worldwide live with ...
This new study, published in Circulation Research, marks a potentially pivotal step in the fight against this debilitating condition.. Dr. Alastair Webb, as Associate Professor at the Wolfson Centre for Prevention of Stroke and Dementia at Oxford University said: 'This is the first trial to show that sildenafil gets into the blood vessels in the brain in people with this condition, improving ...
In 2017 life expectancy at age 65 was 20.9 years for women and 18.6 years for men (ONS, 2018l). It is projected that 23.4% of male and 29.2% of female babies born in 2018 will survive to the age of 100 (ONS, 2018k). Increases in life expectancy in the UK have stalled since 2011.
together for a full-day workshop to agree through consensus the final priorities. The ... People with dementia who live alone report more loneliness and less satisfaction with life2. ... Dementia UK is a registered charity in England and Wales (1039404) and Scotland (SC 047429). ...
1 BACKGROUND. Hypertension is a highly prevalent condition affecting one-third of adults globally and is an established risk factor for cardiovascular (CV) and cerebrovascular disease. 1-3 Additionally, it is estimated that more than 65 million people live with dementia worldwide, with this number projected to exceed 175 million by 2050. 4 Individuals with hypertension are at an increased risk ...
General Election 2024: Make Dementia a Priority. At this General Election candidates from all political parties must acknowledge that dementia is the UK's biggest killer, and the greatest health and social care challenge we face. Through eleven General Elections, Alzheimer's Society has campaigned tirelessly for dementia to be a priority ...