An official website of the United States government
Here’s how you know
Official websites use .gov A .gov website belongs to an official government organization in the United States.
Secure .gov websites use HTTPS A lock ( Lock Locked padlock icon ) or https:// means you’ve safely connected to the .gov website. Share sensitive information only on official, secure websites.

- Health Topics
- Drugs & Supplements
- Medical Tests
- Medical Encyclopedia
- About MedlinePlus
- Customer Support
- How are genetic conditions treated or managed?
Many genetic disorders result from gene changes that are present in essentially every cell in the body. As a result, these disorders often affect many body systems, and most cannot be cured. However, approaches may be available to treat or manage some of the associated signs and symptoms.
For a group of genetic conditions called inborn errors of metabolism, which result from genetic changes that disrupt the production of specific enzymes, treatments sometimes include dietary changes or replacement of the particular enzyme that is missing. Limiting certain substances in the diet can help prevent the buildup of potentially toxic substances that are normally broken down by the enzyme. In some cases, enzyme replacement therapy can help make up for the enzyme shortage. These treatments are used to manage existing signs and symptoms and may help prevent future complications. An example of an inborn error of metabolism is phenylketonuria (PKU) .
For other genetic conditions, treatment and management strategies are designed to improve particular signs and symptoms associated with the disorder. These approaches vary by disorder and are specific to an individual's health needs. For example, a genetic disorder associated with a heart defect might be treated with surgery to repair the defect or with a heart transplant. Conditions that are characterized by defective blood cell formation, such as sickle cell disease , can sometimes be treated with a bone marrow transplant. Bone marrow transplantation can allow the formation of normal blood cells and, if done early in life, may help prevent episodes of pain and other future complications.
Some genetic changes are associated with an increased risk of future health problems, such as certain forms of cancer. One well-known example is familial breast cancer related to mutations in the BRCA1 and BRCA2 genes. Management may include more frequent cancer screening or preventive (prophylactic) surgery to remove the tissues at highest risk of becoming cancerous.
Genetic disorders may cause such severe health problems that they are incompatible with life. In the most severe cases, these conditions may cause a miscarriage of an affected embryo or fetus. In other cases, affected infants may be stillborn or die shortly after birth. Although few treatments are available for these severe genetic conditions, health professionals can often provide supportive care, such as pain relief or mechanical breathing assistance, to the affected individual.
Most treatment strategies for genetic disorders do not alter the underlying genetic mutation; however, a few disorders have been treated with gene therapy . This experimental technique involves changing a person's genes to prevent or treat a disease. Gene therapy, along with many other treatment and management approaches for genetic conditions, are under study in clinical trials.

Topics in the Genetic Consultation chapter
- What is a genetic consultation?
- Why might someone have a genetic consultation?
- What happens during a genetic consultation?
- How can I find a genetics professional in my area?
- What is the prognosis of a genetic condition?
- How are genetic conditions diagnosed?
Other chapters in Help Me Understand Genetics
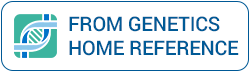
Genetics Home Reference has merged with MedlinePlus. Genetics Home Reference content now can be found in the "Genetics" section of MedlinePlus. Learn more
The information on this site should not be used as a substitute for professional medical care or advice. Contact a health care provider if you have questions about your health.
12.1 Mendel’s Experiments and the Laws of Probability
Learning objectives.
In this section, you will explore the following questions:
- Why was Mendel’s experimental work so successful?
- How do the sum and product rules of probability predict the outcomes of monohybrid crosses involving dominant and recessive alleles?
Connection for AP ® Courses
Genetics is the science of heredity. Austrian monk Gregor Mendel set the framework for genetics long before chromosomes or genes had been identified, at a time when meiosis was not well understood. Working with garden peas, Mendel found that crosses between true-breeding parents (P) that differed in one trait (e.g., color: green peas versus yellow peas) produced first generation (F1) offspring that all expressed the trait of one parent (e.g., all green or all yellow). Mendel used the term dominant to refer to the trait that was observed, and recessive to denote that non-expressed trait, or the trait that had “disappeared” in this first generation. When the F1 offspring were crossed with each other, the F2 offspring exhibited both traits in a 3:1 ratio. Other crosses (e.g., height: tall plants versus short plants) generated the same 3:1 ratio (in this example, tall to short) in the F2 offspring. By mathematically examining sample sizes, Mendel showed that genetic crosses behaved according to the laws of probability, and that the traits were inherited as independent events. In other words, Mendel used statistical methods to build his model of inheritance.
As you have likely noticed, the AP Biology course emphasizes the application of mathematics. Two rules of probability can be used to find the expected proportions of different traits in offspring from different crosses. To find the probability of two or more independent events (events where the outcome of one event has no influence on the outcome of the other event) occurring together, apply the product rule and multiply the probabilities of the individual events. To find the probability that one of two or more events occur, apply the sum rule and add their probabilities together.
The content presented in this section supports the learning objectives outlined in Big Idea 3 of the AP ® Biology Curriculum Framework. The AP ® learning objectives merge essential knowledge content with one or more of the seven science practices. These objectives provide a transparent foundation for the AP ® Biology course, along with inquiry-based laboratory experiences, instructional activities, and AP ® exam questions.
Teacher Support
Two rules of probability are used in solving genetics problems: the rule of multiplication and the rule of addition. The probability that independent events will occur simultaneously is the product of their individual probabilities. If two dices are tossed, what is the probability of landing two ones? A die has 6 faces, and assuming the die is not loaded, each face has the same probability of outcome. The probability of obtaining the number 1 is equal to the number on the die divided by the total number of sides: 1 6 1 6 . The probability of rolling two ones is equal to 1 6 × 1 6 = 1 36 1 6 × 1 6 = 1 36 .
The probability that any one of a set of mutually exclusive events will occur is the sum of their individual probabilities. The probability of rolling a 1 or a 2 is equal to 1 6 + 1 6 = 1 3 1 6 + 1 6 = 1 3 because the two outcomes are mutually exclusive. If we roll a 1, it cannot be a 2.
Tell students that Gregor Mendel was a monk who had received a solid scientific education and had excelled at mathematics. He brought this knowledge of science into his experiments with peas.
Engage students in describing what makes a good organism to study genetics. One approach is to ask the class if they would use elephants to study genetics. The disadvantages of using elephants actually highlight the advantages of using peas, corn, fruit flies, or mice for genetics studies: short life cycle, easy to maintain and handle, large number of offspring for statistical analysis, etc.
The concepts of statistics are not intuitive. Practice with dice and coins. Explain that the probability ratios are achieved with large numbers of trials.
Dominant traits are the ones expressed in a dominant/recessive situation. They do not usually repress the recessive trait. A dominant trait is not necessarily the most common trait in a population. For example, type O blood is a recessive trait, but it is the most frequent blood group in many ethnic groups. A dominant trait can be lethal. A dominant allele is not better than the recessive allele. Whether a trait is beneficial depends on the environment. Give the example of wing color in moths. Dark pigmentation is beneficial in a polluted environment where predators would not pick up the moths on dark tree barks. For example, the population peppered moths in 19th century London shifted so that their wing colors were darker to blend in with the soot of the Industrial Revolution. After pollution levels dropped, light pigmentation became more prevalent because it helped the moths to escape notice.
Johann Gregor Mendel (1822–1884) ( Figure 12.2 ) was a lifelong learner, teacher, scientist, and man of faith. As a young adult, he joined the Augustinian Abbey of St. Thomas in Brno in what is now the Czech Republic. Supported by the monastery, he taught physics, botany, and natural science courses at the secondary and university levels. In 1856, he began a decade-long research pursuit involving inheritance patterns in honeybees and plants, ultimately settling on pea plants as his primary model system (a system with convenient characteristics used to study a specific biological phenomenon to be applied to other systems). In 1865, Mendel presented the results of his experiments with nearly 30,000 pea plants to the local Natural History Society. He demonstrated that traits are transmitted faithfully from parents to offspring independently of other traits and in dominant and recessive patterns. In 1866, he published his work, Experiments in Plant Hybridization, 1 in the proceedings of the Natural History Society of Brünn.
Mendel’s work went virtually unnoticed by the scientific community that believed, incorrectly, that the process of inheritance involved a blending of parental traits that produced an intermediate physical appearance in offspring; this hypothetical process appeared to be correct because of what we know now as continuous variation. Continuous variation results from the action of many genes to determine a characteristic like human height. Offspring appear to be a “blend” of their parents’ traits when we look at characteristics that exhibit continuous variation. The blending theory of inheritance asserted that the original parental traits were lost or absorbed by the blending in the offspring, but we now know that this is not the case. Mendel was the first researcher to see it. Instead of continuous characteristics, Mendel worked with traits that were inherited in distinct classes (specifically, violet versus white flowers); this is referred to as discontinuous variation . Mendel’s choice of these kinds of traits allowed him to see experimentally that the traits were not blended in the offspring, nor were they absorbed, but rather that they kept their distinctness and could be passed on. In 1868, Mendel became abbot of the monastery and exchanged his scientific pursuits for his pastoral duties. He was not recognized for his extraordinary scientific contributions during his lifetime. In fact, it was not until 1900 that his work was rediscovered, reproduced, and revitalized by scientists on the brink of discovering the chromosomal basis of heredity.
Mendel’s Model System
Mendel’s seminal work was accomplished using the garden pea, Pisum sativum , to study inheritance. This species naturally self-fertilizes, such that pollen encounters ova within individual flowers. The flower petals remain sealed tightly until after pollination, preventing pollination from other plants. The result is highly inbred, or “true-breeding,” pea plants. These are plants that always produce offspring that look like the parent. By experimenting with true-breeding pea plants, Mendel avoided the appearance of unexpected traits in offspring that might occur if the plants were not true breeding. The garden pea also grows to maturity within one season, meaning that several generations could be evaluated over a relatively short time. Finally, large quantities of garden peas could be cultivated simultaneously, allowing Mendel to conclude that his results did not come about simply by chance.
Mendelian Crosses
Mendel performed hybridizations , which involve mating two true-breeding individuals that have different traits. In the pea, which is naturally self-pollinating, this is done by manually transferring pollen from the anther of a mature pea plant of one variety to the stigma of a separate mature pea plant of the second variety. In plants, pollen carries the male gametes (sperm) to the stigma, a sticky organ that traps pollen and allows the sperm to move down the pistil to the female gametes (ova) below. To prevent the pea plant that was receiving pollen from self-fertilizing and confounding his results, Mendel painstakingly removed all of the anthers from the plant’s flowers before they had a chance to mature.
Plants used in first-generation crosses were called P 0 , or parental generation one, plants ( Figure 12.3 ). Mendel collected the seeds belonging to the P 0 plants that resulted from each cross and grew them the following season. These offspring were called the F 1 , or the first filial ( filial = offspring, daughter or son), generation. Once Mendel examined the characteristics in the F 1 generation of plants, he allowed them to self-fertilize naturally. He then collected and grew the seeds from the F 1 plants to produce the F 2 , or second filial, generation. Mendel’s experiments extended beyond the F 2 generation to the F 3 and F 4 generations, and so on, but it was the ratio of characteristics in the P 0 −F 1 −F 2 generations that were the most intriguing and became the basis for Mendel’s postulates.
Garden Pea Characteristics Revealed the Basics of Heredity
In his 1865 publication, Mendel reported the results of his crosses involving seven different characteristics, each with two contrasting traits. A trait is defined as a variation in the physical appearance of a heritable characteristic. The characteristics included plant height, seed texture, seed color, flower color, pea pod size, pea pod color, and flower position. For the characteristic of flower color, for example, the two contrasting traits were white versus violet. To fully examine each characteristic, Mendel generated large numbers of F 1 and F 2 plants, reporting results from 19,959 F 2 plants alone. His findings were consistent.
What results did Mendel find in his crosses for flower color? First, Mendel confirmed that he had plants that bred true for white or violet flower color. Regardless of how many generations Mendel examined, all self-crossed offspring of parents with white flowers had white flowers, and all self-crossed offspring of parents with violet flowers had violet flowers. In addition, Mendel confirmed that, other than flower color, the pea plants were physically identical.
Once these validations were complete, Mendel applied the pollen from a plant with violet flowers to the stigma of a plant with white flowers. After gathering and sowing the seeds that resulted from this cross, Mendel found that 100 percent of the F 1 hybrid generation had violet flowers. Conventional wisdom at that time would have predicted the hybrid flowers to be pale violet or for hybrid plants to have equal numbers of white and violet flowers. In other words, the contrasting parental traits were expected to blend in the offspring. Instead, Mendel’s results demonstrated that the white flower trait in the F 1 generation had completely disappeared.
Importantly, Mendel did not stop his experimentation there. He allowed the F 1 plants to self-fertilize and found that, of F 2 -generation plants, 705 had violet flowers and 224 had white flowers. This was a ratio of 3.15 violet flowers per one white flower, or approximately 3:1. When Mendel transferred pollen from a plant with violet flowers to the stigma of a plant with white flowers and vice versa, he obtained about the same ratio regardless of which parent, male or female, contributed which trait. This is called a reciprocal cross —a paired cross in which the respective traits of the male and female in one cross become the respective traits of the female and male in the other cross. For the other six characteristics Mendel examined, the F 1 and F 2 generations behaved in the same way as they had for flower color. One of the two traits would disappear completely from the F 1 generation only to reappear in the F 2 generation at a ratio of approximately 3:1 ( Table 12.1 ).
Upon compiling his results for many thousands of plants, Mendel concluded that the characteristics could be divided into expressed and latent traits. He called these, respectively, dominant and recessive traits. Dominant traits are those that are inherited unchanged in a hybridization. Recessive traits become latent, or disappear, in the offspring of a hybridization. The recessive trait does, however, reappear in the progeny of the hybrid offspring. An example of a dominant trait is the violet-flower trait. For this same characteristic (flower color), white-colored flowers are a recessive trait. The fact that the recessive trait reappeared in the F 2 generation meant that the traits remained separate (not blended) in the plants of the F 1 generation. Mendel also proposed that plants possessed two copies of the trait for the flower-color characteristic, and that each parent transmitted one of its two copies to its offspring, where they came together. Moreover, the physical observation of a dominant trait could mean that the genetic composition of the organism included two dominant versions of the characteristic or that it included one dominant and one recessive version. Conversely, the observation of a recessive trait meant that the organism lacked any dominant versions of this characteristic.
So why did Mendel repeatedly obtain 3:1 ratios in his crosses? To understand how Mendel deduced the basic mechanisms of inheritance that lead to such ratios, we must first review the laws of probability.
Science Practice Connection for AP® Courses
Think about it.
Students are performing a cross involving seed color in garden pea plants. Yellow seed color is dominant to green seed color. What F1 offspring would be expected when cross true-breeding plants with green seeds with true-breading plants with yellow seeds? Express the answer(s) as percentage.
This question is an application of Learning Objectives 3.14 and Science Practice 2.2 because students are applying a mathematical routine (probability) to determine a Mendelian pattern of inheritance.
Possible answer:
Probability basics.
Probabilities are mathematical measures of likelihood. The empirical probability of an event is calculated by dividing the number of times the event occurs by the total number of opportunities for the event to occur. It is also possible to calculate theoretical probabilities by dividing the number of times that an event is expected to occur by the number of times that it could occur. Empirical probabilities come from observations, like those of Mendel. Theoretical probabilities come from knowing how the events are produced and assuming that the probabilities of individual outcomes are equal. A probability of one for some event indicates that it is guaranteed to occur, whereas a probability of zero indicates that it is guaranteed not to occur. An example of a genetic event is a round seed produced by a pea plant. In his experiment, Mendel demonstrated that the probability of the event “round seed” occurring was one in the F 1 offspring of true-breeding parents, one of which has round seeds and one of which has wrinkled seeds. When the F 1 plants were subsequently self-crossed, the probability of any given F 2 offspring having round seeds was now three out of four. In other words, in a large population of F 2 offspring chosen at random, 75 percent were expected to have round seeds, whereas 25 percent were expected to have wrinkled seeds. Using large numbers of crosses, Mendel was able to calculate probabilities and use these to predict the outcomes of other crosses.
The Product Rule and Sum Rule
Mendel demonstrated that the pea-plant characteristics he studied were transmitted as discrete units from parent to offspring. As will be discussed, Mendel also determined that different characteristics, like seed color and seed texture, were transmitted independently of one another and could be considered in separate probability analyses. For instance, performing a cross between a plant with green, wrinkled seeds and a plant with yellow, round seeds still produced offspring that had a 3:1 ratio of green:yellow seeds (ignoring seed texture) and a 3:1 ratio of round:wrinkled seeds (ignoring seed color). The characteristics of color and texture did not influence each other.
The product rule of probability can be applied to this phenomenon of the independent transmission of characteristics. The product rule states that the probability of two independent events occurring together can be calculated by multiplying the individual probabilities of each event occurring alone. To demonstrate the product rule, imagine that you are rolling a six-sided die (D) and flipping a penny (P) at the same time. The die may roll any number from 1–6 (D # ), whereas the penny may turn up heads (P H ) or tails (P T ). The outcome of rolling the die has no effect on the outcome of flipping the penny and vice versa. There are 12 possible outcomes of this action ( Table 12.2 ), and each event is expected to occur with equal probability.
Of the 12 possible outcomes, the die has a 2/12 (or 1/6) probability of rolling a two, and the penny has a 6/12 (or 1/2) probability of coming up heads. By the product rule, the probability that you will obtain the combined outcome 2 and heads is: (D 2 ) x (P H ) = (1/6) x (1/2) or 1/12 ( Table 12.3 ). Notice the word “and” in the description of the probability. The “and” is a signal to apply the product rule. For example, consider how the product rule is applied to the dihybrid cross: the probability of having both dominant traits (for example, yellow and round) in the F 2 progeny is the product of the probabilities of having the dominant trait for each characteristic, as shown here:
On the other hand, the sum rule of probability is applied when considering two mutually exclusive outcomes that can come about by more than one pathway. The sum rule states that the probability of the occurrence of one event or the other event, of two mutually exclusive events, is the sum of their individual probabilities. Notice the word “or” in the description of the probability. The “or” indicates that you should apply the sum rule. In this case, let’s imagine you are flipping a penny (P) and a quarter (Q). What is the probability of one coin coming up heads and one coin coming up tails? This outcome can be achieved by two cases: the penny may be heads (P H ) and the quarter may be tails (Q T ), or the quarter may be heads (Q H ) and the penny may be tails (P T ). Either case fulfills the outcome. By the sum rule, we calculate the probability of obtaining one head and one tail as [(P H ) × (Q T )] + [(Q H ) × (P T )] = [(1/2) × (1/2)] + [(1/2) × (1/2)] = 1/2 ( Table 12.3 ). You should also notice that we used the product rule to calculate the probability of P H and Q T , and also the probability of P T and Q H , before we summed them. Again, the sum rule can be applied to show the probability of having at least one dominant trait in the F 2 generation of a dihybrid cross:
To use probability laws in practice, it is necessary to work with large sample sizes because small sample sizes are prone to deviations caused by chance. The large quantities of pea plants that Mendel examined allowed him to calculate the probabilities of the traits appearing in his F 2 generation. As you will learn, this discovery meant that when parental traits were known, the offspring’s traits could be predicted accurately even before fertilization.
- 1 Johann Gregor Mendel, Versuche über Pflanzenhybriden Verhandlungen des naturforschenden Vereines in Brünn, Bd. IV für das Jahr , 1865 Abhandlungen, 3–47. [go here for the English translation here ]
As an Amazon Associate we earn from qualifying purchases.
This book may not be used in the training of large language models or otherwise be ingested into large language models or generative AI offerings without OpenStax's permission.
Want to cite, share, or modify this book? This book uses the Creative Commons Attribution License and you must attribute OpenStax.
Access for free at https://openstax.org/books/biology-ap-courses/pages/1-introduction
- Authors: Julianne Zedalis, John Eggebrecht
- Publisher/website: OpenStax
- Book title: Biology for AP® Courses
- Publication date: Mar 8, 2018
- Location: Houston, Texas
- Book URL: https://openstax.org/books/biology-ap-courses/pages/1-introduction
- Section URL: https://openstax.org/books/biology-ap-courses/pages/12-1-mendels-experiments-and-the-laws-of-probability
© Apr 26, 2024 OpenStax. Textbook content produced by OpenStax is licensed under a Creative Commons Attribution License . The OpenStax name, OpenStax logo, OpenStax book covers, OpenStax CNX name, and OpenStax CNX logo are not subject to the Creative Commons license and may not be reproduced without the prior and express written consent of Rice University.

An official website of the United States government
The .gov means it’s official. Federal government websites often end in .gov or .mil. Before sharing sensitive information, make sure you’re on a federal government site.
The site is secure. The https:// ensures that you are connecting to the official website and that any information you provide is encrypted and transmitted securely.
- Publications
- Account settings
Preview improvements coming to the PMC website in October 2024. Learn More or Try it out now .
- Advanced Search
- Journal List
- CBE Life Sci Educ
- v.18(2); Summer 2019
Problem Solving in Genetics: Content Hints Can Help
Jennifer s. avena.
a Department of Molecular, Cellular, and Developmental Biology, University of Colorado–Boulder, Boulder, CO 80309
Jennifer K. Knight
Associated data.
Problem solving is an integral part of doing science, yet it is challenging for students in many disciplines to learn. We explored student success in solving genetics problems in several genetics content areas using sets of three consecutive questions for each content area. To promote improvement, we provided students the choice to take a content-focused prompt, termed a “content hint,” during either the second or third question within each content area. Overall, for students who answered the first question in a content area incorrectly, the content hints helped them solve additional content-matched problems. We also examined students’ descriptions of their problem solving and found that students who improved following a hint typically used the hint content to accurately solve a problem. Students who did not improve upon receipt of the content hint demonstrated a variety of content-specific errors and omissions. Overall, ultimate success in the practice assignment (on the final question of each topic) predicted success on content-matched final exam questions, regardless of initial practice performance or initial genetics knowledge. Our findings suggest that some struggling students may have deficits in specific genetics content knowledge, which when addressed, allow the students to successfully solve challenging genetics problems.
INTRODUCTION
Problem solving has been defined in the literature as engaging in a decision-making process leading to a goal, in which the course of thought needed to solve the problem is not certain ( Novick and Bassok, 2005 ; Bassok and Novick, 2012 ; National Research Council, 2012 ; Prevost and Lemons, 2016 ). Ample research shows that students have difficulty learning how to solve complex problems in many disciplines. For example, in biology and chemistry, students often omit critical information or recall information incorrectly and/or apply information incorrectly to a problem ( Smith and Good, 1984 ; Smith, 1988 ; Prevost and Lemons, 2016 ). Furthermore, across many disciplines, researchers have found that experts use different procedural processes than nonexperts when solving problems ( Chi et al. , 1981 ; Smith and Good, 1984 ; Smith et al. , 2013 ). While students often identify problems based on superficial features, such as the type of organism discussed in a problem, experts identify primary concepts and then link the concept with strategies on how to solve such a problem ( Chi et al. , 1981 ; Smith and Good, 1984 ; Smith et al. , 2013 ). Experts also often check their work and problem solutions more frequently than nonexperts ( Smith and Good, 1984 ; Smith, 1988 ). Given the difficulties students have in problem solving and the value of such skills to their future careers, there is clearly a need for undergraduate educators to assist students in developing problem-solving skills ( American Association for the Advancement of Science, 2011 ; National Research Council, 2012 ).
Two kinds of knowledge have been described in the literature as important for solving problems: domain specific and domain general. Domain-specific knowledge is knowledge about a specific field, including the content (declarative knowledge), the procedural processes used to solve problems (procedural knowledge), and how to apply content and process when solving problems (conditional knowledge; Alexander and Judy, 1988 ). Domain-general knowledge is knowledge that can be used across many contexts ( Alexander and Judy, 1988 ; Prevost and Lemons, 2016 ). A third category, strategic knowledge, is defined as knowledge about problem-solving strategies that can be domain specific or domain general ( Chi, 1981 ; Alexander and Judy, 1988 ). Research suggests that domain-specific knowledge is needed, but may not be sufficient, for applying strategic knowledge to solve problems ( Alexander and Judy, 1988 ; Alexander et al. , 1989 ). Thus, helping students learn to solve problems likely requires teaching them how to activate their content knowledge, apply their knowledge to a problem, and logically think through the problem-solving procedure.
Previous research suggests that receiving help in a variety of forms, including procedure-based prompts ( Mevarech and Amrany, 2008 ), a combination of multiple content- and procedure-based prompts ( Pol et al. , 2008 ), and models ( Stull et al. , 2012 ), can be beneficial to learning. Not surprisingly, accessing relevant prior knowledge has been shown to positively influence performance ( Dooling and Lachman, 1971 ; Bransford and Johnson, 1972 ; Gick and Holyoak, 1980 ). For example, in genetics, successful problem solvers often identify similarities between problems, whereas unsuccessful problem solvers do not ( Smith, 1988 ). Previous research also suggests that receiving procedural guidance can be beneficial to learning. In a study that asked students to examine different problems with related solutions, prompting students to consider previously reviewed problems helped most students subsequently solve a challenging problem ( Gick and Holyoak, 1980 ). In another study, when students received guidance that included identifying similarities to other problems as well as other procedural skills, such as planning and checking their work, they were better able to solve subsequent problems than in the absence of such guidance ( Mevarech and Amrany, 2008 ). However, although accessing prior knowledge is important, it is also important that students understand how to apply their prior knowledge to a given problem ( Bransford and Johnson, 1972 ). Thus, while students may realize they need additional information to solve a problem, if they cannot make sense of this information in the context of a given problem, the information is unlikely to be useful.
In addition to knowledge, students need practice. Within the field of psychology, many studies have examined the association between practice and performance. Completing a practice test leads to better performance on a subsequent final test compared with other conditions in which students do not test themselves, such as studying or completing an unrelated or no activity (e.g., Roediger and Karpicke, 2006 ; Adesope et al. , 2017 ). In a meta-analysis, this effect, termed the “testing effect,” was found to occur regardless of whether feedback was given and regardless of the time between the practice test and the final test ( Adesope et al. , 2017 ). The benefits of practice testing on later performance can occur not only when using the same questions (retention) but also when students are asked to transfer information to nonidentical questions, including questions that require application of concepts. In one of the few studies on the testing effect using transfer questions, students who took practice tests performed better on transfer questions on a final test for both factual (i.e., a single fact in a sentence) and conceptual (i.e., a cohesive idea across multiple sentences) questions than those who studied but did not take practice tests ( Butler, 2010 ). This study also found that those who performed well on their practice tests were more likely to do well than those who performed poorly on their practice tests 1 week after practice on a subsequent final test, which included conceptual questions that required application ( Butler, 2010 ).
In the current study, we focused on whether students who are incorrectly solving a problem can apply content knowledge given to them as a prompt to correctly solve subsequent genetics problems. We address the following questions: 1) Does providing a single content-focused prompt help students answer similar questions during subsequent practice, and does this practice help on later exams? 2) When unable to apply content prompts, what content errors and omissions do students make that lead them to continue to answer incorrectly?
Participants
We invited students enrolled in an introductory-level undergraduate genetics course for biology majors (total of 416 students in the course) at a 4-year institution during Spring 2017 to complete each of two practice assignments containing content related to course exams. The first practice assignment was taken immediately before a unit exam, and the second assignment was taken either immediately before the next unit exam or after this exam in preparation for the cumulative final exam (see Supplemental Figure S1 for timeline). Each assignment was offered online (using the survey platform Qualtrics) for up to 6 points of extra credit (650 total course points). Students received 4 points for answering the question with an explanation of their problem-solving process and an additional 2 points if they answered correctly. The practice assignments were announced in class and by email, with encouragement to complete the assignment as preparation for an upcoming exam. Students had the option to consent to have their answers used for research purposes, and all students who completed the assignment received credit regardless of their consent.
Course Performance Metrics
Students in the course were given the option to complete the Genetics Concept Assessment (GCA; Smith et al. , 2008 ) online at the beginning of the semester (within the first week of classes) for participation extra credit. The 25 GCA questions address eight of the 11 learning objectives taught in this course. Initial performance on the GCA is reported as the pretest. Students answered the same GCA questions again on the cumulative final exam, for credit, along with instructor-generated questions that also addressed the content from practice assignments along with other course content. The instructor-generated questions on the final exam comprised 15% of the student’s final course grade, and the GCA questions comprised just under 8% of the student’s final course grade.
Practice Assignment Content
We selected content areas known to be challenging for genetics students ( Smith et al. , 2008 ; Smith and Knight, 2012 ) and developed sets of questions on the following five topics: calculation of the probability of inheritance across multiple generations (“probability”), prediction of the cause of an incorrect chromosome number after meiosis (“nondisjunction”), interpretation of a gel and pedigree to determine inheritance patterns (“gel/pedigree”), prediction of the probability of an offspring’s genotype using linked genes (“recombination”), and determination of the parental germ line from which a gene is imprinted (“imprinting”).
For each content area, we wrote three questions intended to be isomorphic that had the following characteristics: they addressed the same underlying concept but used different superficial characteristics, targeted higher-order cognitive processes as assessed by Bloom’s level ( Bloom et al. , 1956 ), contained the same amount of information, and required students to perform similar processes to solve the problem. The questions were in constructed-response format but had a single correct answer, and each question also had a coinciding visual aid (example in Figure 1 ; see all questions in the Supplemental Material). The questions were initially based on previously used exam questions in the course and were tested and modified through individual think-aloud interviews (16 students and seven genetics faculty) and/or a focus group (three students).

Example of a practice question used for problem solving on the content of nondisjunction. Each question in the study had a visual aid, was constructed response, and had a single correct answer.
The three questions within a given content area (referred to as a “trio”) were given sequentially in the practice assignments, with the first, second, and third questions referred to as “Q1,” “Q2,” and “Q3,” respectively. For each problem-solving assignment, we randomized for each student the order of the three questions within each content area and the order in which each content area was presented. In the first problem-solving assignment, to prevent fatigue, students answered two of three randomly assigned content areas (probability, nondisjunction, and gel/pedigree), and for the second assignment, students completed questions on both recombination and imprinting.
Experimental Conditions
We developed content-focused prompts (referred to hereafter as “content hints”) based on common student errors revealed during in-class questions and previous exams for this course and/or during individual student think-aloud interviews. Each hint addressed the most common student error and contained only a single content idea ( Table 1 ). In each online practice assignment, we randomly assigned students to one of two conditions: an optional content hint when taking the second question of a content trio (hint at Q2) or an optional content hint when taking the third question of a content trio (hint at Q3). The first question (Q1) served as a baseline measure of performance for all students. At Q2, we compared the performance of students in the two conditions to determine the effect of a hint versus practice only. At Q3, we compared the performance of students within each condition with their performance on Q2 to determine whether performance was maintained (for hint at Q2 condition) or the hint improved performance compared with Q2 (for hint at Q3 condition). Using this randomized design, we could examine the differential effect of practice versus a hint, while still giving all students a chance to receive hints.
Content hints
Either at Q2 or at Q3 (depending on the condition), students were asked to respond to the following question: “Do you want a hint to solve this problem (No penalty)? If so, click here.” If they clicked, the hint appeared immediately below the problem, so students could see the hint while solving the problem. By asking students to select the hint rather than just showing it to everyone, we could track who chose to take a hint and thus distinguish between hint takers and non–hint takers. We did not provide real-time feedback to students, because the provided hints were intended to serve as a scaffolding mechanism without individual feedback. In addition, it would have been challenging to provide feedback, because the online platform used did not allow for personalized feedback and because the student answers were constructed response and could not be automatically graded.
Problem-Solving Content and Errors
We instructed students to explain in writing their thinking and the steps they were taking to solve the problem before they provided the final answer to each question ( Prevost and Lemons, 2016 ). Students were not allowed to return to a question once answered. The instructions at the beginning of the assignment outlined an example of how to do this (see the Supplemental Material), and students were able to reread the instructions and an example, if desired, during the assignment. In this study, we only tracked student performance and their use of language regarding the content hint, not their thinking or problem-solving steps.
We categorized student content-specific errors and omissions and also the use of language related to the content hint. The two authors reviewed a selection of student answers to develop an initial set of codes. We then independently coded, over three iterations, the same 66 of 456 selected answers. After each iteration, we discussed our codes to come to a consensus and revised the coding scheme as needed to represent student answers. We coded an additional 19 answers to reach a final interrater agreement of 85% (Cohen’s kappa of 0.83). Because we had coded and agreed upon 19% of the student answers at this point and our agreement was above acceptable levels ( Landis and Koch, 1977 ), we then each coded half of the remaining 371 answers independently and discussed and resolved any concerns.
Statistical Analysis
We scored student answers on the practice assignments as incorrect (0) or correct (1) and used performance data only from students who provided a final answer to all possible questions in one or both assignments. We analyzed data from 233 students: 133 students completed both practice assignments, 54 students completed only the first assignment, and 46 students completed only the second assignment. Where content areas are not specified, we report results on all content areas together. We analyzed patterns at the level of the individual answer and used logistic regressions to compare answer performance between conditions, content areas, and progression groups, treating performance on one content area as independent from another content area. A student’s performance within a single content area for Q1, Q2, and Q3 was treated as dependent (i.e., a repeated measure), and we used McNemar’s test to analyze differences in percentage correct between questions. To examine trends at the student level, we used ordinary least-squares (OLS) regression analysis.
For the analysis of student content language use and content errors, we excluded any trios in which one answer could not be coded (i.e., no problem solving described: 36 answers) or for which there was not enough explanation to be interpretable (31 answers). A total of 342 answers are discussed in this study. We used logistic regression to compare the presence of content-specific language between differing groups within the same hint condition.
For the GCA and instructor-generated final exam questions, we report performance as percentage correct. We excluded GCA pretest scores for individuals who took less than 6 minutes to complete the online questionnaire with the GCA or did not finish at least 85% of the questions. For both the GCA and the instructor-generated final exam (a total of 150 points), a subset of questions addressed the same content areas as the practice assignment questions and are termed “practice-related” questions in this study. For the GCA, practice-related questions included one multiple-choice question per content area (questions 10, 20, 24, 25) for a total of 8 points. For the instructor-generated final exam, there were two short-answer questions on nondisjunction and recombination and one multiple-choice question on probability, worth a total of 21 points. We also calculated performance on the remaining questions from the GCA and from the instructor-generated final exam (“practice-unrelated” questions). We used OLS regression analysis to examine the association between a student’s practice assignment and exam performance, and we report unstandardized beta coefficients. We used average performance on practice Q3 questions (“practice Q3 correct”), a measure of practice success, as the predictor. We also included average performance on Q1 (“practice Q1 correct”) in the regression models. For assessment performance analyses, we examined only students who completed both practice assignments (three total content areas) to ensure that all practice predictor variables were calculated based on the same number of questions (three Q3s and Q1s). Out of 133 students who completed both practice assignments, 109 students completed the GCA pre- and posttest and instructor-generated final exam and thus were included in the OLS models. The OLS regression model was the following for the GCA and instructor-generated exam questions, both practice related and practice unrelated:

We also compared assessment outcomes for students who completed the GCA at both time points and the final exam but did not complete any practice assignments ( n = 35) with those who completed all assessments and practice assignments (via OLS or independent t tests, as indicated). For this analysis, the OLS regression model was the following for the GCA and instructor-generated exam questions, both practice-related and practice-unrelated:

We used Stata v. 15.0 and R v. 3.3.3 (dplyr, VennDiagram, statmod, VGAM, irr packages) for all statistical tests. The cutoff for statistical significance was defined as an alpha of 0.05.
Human Subjects Approval
This work was reviewed by the University of Colorado Institutional Review Board, and the use of human subjects was approved (protocols 16-0511 and 15-0380).
Practice Problem-Solving Performance: Question Difficulty
By randomizing the order in which students answered each question within a content area, we were able to use student performance on the first question to compare the difficulty of each of the three questions. For all content areas except imprinting, the questions were isomorphic (χ 2 , p > 0.05), and answering the imprinting question did not influence student performance on recombination questions (taking recombination question first vs. second in the practice assignment; logistic regression, p > 0.05). Therefore, from this point on, all data presented represent the four remaining content areas: probability, nondisjunction, gel/pedigree, and recombination.
Two hundred thirty-three students answered a total of 553 trios of questions (Q1, Q2, Q3). The number of trios answered varies for each content area, because not all students answered all questions or completed both assignments: In the first assignment, students answered trios in two out of three content areas (randomly assigned), and in the second assignment, all students answered the trio of questions on recombination. We first examined the performance of all students across all four content areas and then for each content area individually ( Table 2 ). For all content areas combined, student performance increased from question 1 (Q1) to questions 2 (Q2) and 3 (Q3). Upon examination of each content area individually, however, we found that the percentage of correct answers increased from Q1 to Q3 in recombination and gel/pedigree, but not for the content areas of nondisjunction and probability. In comparing Q1 performance between content areas, students had a higher percent correct for gel/pedigree and nondisjunction questions than for probability and recombination questions and a higher percent correct for probability than for recombination ( Table 2 ).
Performance on practice problem-solving questions a
Hint Choice
Although all students were given the option to receive a content hint for each content area during practice assignments, they only took this option in 68% of trios overall (Supplemental Table S1). Students who were offered the hint at Q2 were equally likely as those who were offered the hint at Q3 to take a hint for any given content area. For the most difficult content area (recombination), students chose to take a hint more often than for the easier content area of gel/pedigree. When looking at performance across all content areas combined, students who took a hint in a given trio scored significantly lower on all three questions than students who did not take a hint in a given trio (Supplemental Table S2). This pattern, while not always significant, was also seen in each individual content area (Supplemental Table S2). Additionally, across all content areas combined, answers did not show improvement, on average, from Q1 to Q3 in trios in which a hint was not taken, while they did in trios in which a hint was taken. This difference was also significant in the individual content area of recombination, but not the other content areas (Supplemental Table S2). To maintain reasonable sample sizes in our analyses, we combined all content areas together for the remainder of the data in this paper regarding practice performance.
We also characterized students’ initial Q1 performance based on frequency of taking a hint. To best represent whether a student had a consistent pattern in hint choice, we focused on only the students who completed questions in both practice assignments (the maximum of three content areas). Of the 133 students who completed both assignments, 14 students never chose to take a hint, 56 students sometimes chose to take a hint, and 63 students always chose to take a hint when offered. Students who never took a hint performed better on Q1 than students who always took a hint (Supplemental Table S3). We have not further analyzed answer trios in which a student chose not to take a hint for several reasons. We did not have a randomization process for hint presentation: all students were given the option, and those who did not take a hint chose not to do so for reasons that we could not directly examine. In addition, because so few of the students in the study chose to never take a hint, and because we were primarily interested in the effect of taking a content hint on student success, we focused on the students who did take a hint, randomized to either Q2 or Q3 within a trio.
Content Hints Help a Subset of Students
To examine the immediate effect of a content hint on student performance, we focused the remainder of our analyses on situations in which students took a hint. We used Q1 as a baseline measure of student performance in a given content area. Because students were offered a hint either at Q2 or at Q3, we compared student performance at Q2 in the presence or absence of a hint for this question. To examine whether performance was maintained (for hint at Q2 condition) or whether the hint improved performance compared with Q2 (for hint at Q3 condition), we examined performance at Q3. For the students who took a hint, we first looked at aggregate data at the level of individual answers, binning answers into Q1 correct versus incorrect and then looking at performance on the subsequent two questions ( Figure 2 ). As shown in Figure 2 A, if students answered Q1 correctly within a trio, 15% went on to answer Q2 incorrectly (without a hint), indicating that practice itself may not help these students who initially answer correctly. Students who did receive a hint at Q2 performed the same as those who did not, indicating the drop in performance from Q1 to Q2 was not due to the hint. In a given trio, Q3 performance also did not differ based on when a hint was received, and performance, on average, did not change from Q2 to Q3, indicating that a hint did not positively or negatively impact performance for these students who initially answer correctly.

The effect of a hint differs depending on Q1 correctness. (A) Q1 incorrect: the percent of correct answers for Q2 and Q3 is shown for trios in which a hint was taken at Q2 ( n = 84 trios) or at Q3 ( n = 110 trios). *, p < 0.05; all else NS, p > 0.05 (logistic regression between conditions; McNemar’s test between Q2 and Q3 for each condition). (B) Q1 correct: the percent of correct answers for Q2 and Q3 is shown for trios in which a hint was taken at Q2 ( n = 89 trios) or at Q3 ( n = 91 trios). There were no significant differences between conditions (logistic regression, p > 0.05) or between Q2 and Q3 (McNemar’s test, p > 0.05).
If students answered Q1 incorrectly within a trio, 21% went on to answer Q2 correctly without a hint, suggesting that practice alone can help these students who initially answer incorrectly ( Figure 2 B). However, a significantly higher percent of students answered correctly upon receiving a hint at Q3. Students who took the hint at Q2 were significantly more likely to get Q2 correct than students who had not yet taken a hint, indicating the hint provides an added benefit beyond practice itself. A similar percent of the students who took a hint at Q2 also answered Q3 correctly, indicating that, on average, they maintained performance on a subsequent question after the hint was taken. By the third question in a content area, all students had received a hint, some at Q2 and some at Q3. Those who took a hint at Q3 performed equivalently on Q3 to those who had taken a hint at Q2, indicating that students benefited similarly at the end of practicing a given content area, regardless of when the hint was received.
To examine how individual students performed sequentially on a trio of questions, we followed the progression of individual students from Q1 to Q3 ( Figures 3 and and4). 4 ). Students took a hint at Q2 in 173 trios of questions ( Figure 3 ). Of these, 49% of students in a given trio answered Q1 incorrectly. Thirty-seven percent of those moved on to get Q2 correct when they received a hint, and then 68% of those went on to get Q3 correct. Thus, the majority, but not all students, maintained this improvement from Q2 to Q3. Students took a hint at Q3 in 201 trios of questions ( Figure 4 ). Of these, 55% of students in a given trio answered Q1 incorrectly. Seventy-nine percent of those also got Q2 incorrect, and then 26% of those moved on to get Q3 correct when they received a hint. As seen in Figures 3 and and4, 4 , while a hint helped some students answer a subsequent question correctly, a hint did not help all students; some students answered Q1, Q2, and Q3 incorrectly despite taking a hint.

Student-level progression across answer trios in which a hint was taken at Q2. Percent of correct answers is shown with the number of answers in each category (e.g., Q1 incorrect) in parentheses. Arrows indicate the percent of answers that track to the next category. Bolded arrows signify categories of trios that were analyzed for content-specific language use and errors/omissions: trios with Q1 incorrect but Q2 and Q3 correct (011 group) and those with all three answers incorrect (000 group).

Student-level progression for answer trios in which a hint was taken at Q3. Percentage of correct answers is shown with the number of answers in each category (e.g., Q1 incorrect) in parentheses. Arrows indicate the percent of answers that track to the next category. Bolded arrows signify categories of trios that were analyzed for content-specific language use and errors/omissions: trios with Q1 and Q2 incorrect but Q3 correct (001 group) and those with all three answers incorrect (000 group).
Content-Specific Language Use and Errors or Omissions
To further explore why the hint did not help some students but did help others, we examined how students used the given content hint. We categorized within a student’s documented problem-solving answer 1) the presence of language that reflected the content described in the hint (coded as present or absent; Table 3 ), and 2) the types of content errors and omissions made in solving the problem, tracking both correctness and language use across the three questions (Q1, Q2, Q3) for each content area ( Table 4 ). Only the following selection of students who answered Q1 incorrectly and took a hint were considered for this analysis (see bolded arrows in Figures 3 and and4): 4 ): students in a given trio who answered Q2 and Q3 correctly after taking a hint at Q2 (defined as 011), those who answered correctly after taking a hint at Q3 (defined as 001), and those who answered incorrectly on all three questions (defined as 000). Students who shifted from incorrect at Q1 to correct at Q2 or Q3 (011 and 001 students, respectively) more often used language associated with the content of the hint than students who answered all three questions incorrectly. In cases in which students took a hint at Q2, 83% of answers in the 011 group contained language reflecting the hint content compared with 55% in the 000 group ( n = 40 and 74 Q2 and Q3 answers, respectively; logistic regression, odds ratio [OR] = 3.8, p < 0.01). Similarly, when students took a hint at Q3, 91% of answers in the 001 group contained language reflecting the hint content compared with 60% in the 000 group ( n = 23 and 60 Q3 answers, respectively; logistic regression, OR = 9.2, p < 0.01).
Presence of language reflecting content in hint criteria, coded only in answers during and after receipt of a hint
Content errors and omissions codes
Students who continued to answer incorrectly (000 group) displayed a wide variety of content-specific errors and omissions, including multiple errors or omissions within a single answer. Figure 5 shows these errors and omissions for Q1 through Q3 categorized by content area, with each error type or omission represented by different colored circles. For each content area, the orange shading represents an error or omission related to the hint content; the other colors represent different errors or omissions specific to each content area and not related to the content hint. Details for each content area for the 000 group are given in the following sections.

Presence of content errors and omissions in incorrect answers in four critical content areas in genetics. The number of answers in which each content error/omission code was observed is shown, with overlap in color indicating the presence of multiple errors/omissions within a single answer. Only 000 progression groups are shown for all questions Q1–Q3. In each case, orange shading indicates an error aligned with the hint content.
Recombination
In the recombination questions, the most common error in the 000 group was no use of map units to solve the problem (57% of 143 answers; Figure 5 A, orange oval). In addition, students made three other types of errors, sometimes in addition to the most common error. In some answers, while map units were used, they were used incorrectly (29%; Figure 5 A, blue oval). Students also made errors in gamete-type identification in which they incorrectly assigned the type of gamete (recombinant or parental) or assigned the probability of recombination to the nonrecombinant gamete (22%; Figure 5 A, green oval). Less often, students incorrectly identified the desired genotype to solve the problem (4%; Figure 5 A, magenta oval). Even after receiving the hint defining map distance, many students made the most common error of not using map units to solve the problem (“No use of map units”; 49% of 67 answers), even though some of these students ( n = 12) used the content language of the hint.
Probability
In the probability questions in this study, students needed to appropriately assign offspring having a probability of 2/3 for a certain genotype based on information about the parents and the mode of inheritance (due to one possible offspring genotype from a parental mating being eliminated). The two most common errors in the 000 group were incorrectly assigning at least one genotype or probability (which includes not using the 2/3 probability correctly; 81% of 67 answers; Figure 5 B, orange circle) and not using or improperly using the product rule for multiplying multiple independent probabilities (64%; Figure 5 B, green circle). These two errors were most commonly present in combination in the same answer (40%; Figure 5 B). While not as common, student answers sometimes contained the error of inaccurate use of modes of inheritance or calculations, either alone or in combination with other errors (21%; Figure 5 B, blue circle). Even after receiving the hint about the 2/3 probability, many students made incorrect genotype or probability assignments (“Genotype/probability misassignment”; 70% of 33 answers), even though some of these students ( n = 5) used the content language of the hint.
Gel/Pedigree
Gel/pedigree was one of the two higher-performing categories (the other being nondisjunction), so there are fewer answers in the 000 group. In these problems, students were asked to interpret both a gel and pedigree to determine inheritance patterns. To most accurately answer the gel/pedigree questions, examination of the molecular gel information to inform the number of chromosome copies present was needed. The omission of not discussing the number of alleles per gene in males and females was most common (91% of 23 answers; Figure 5 C, orange circle), and while only a few answers contained this single omission, many answers contained this omission in addition to other errors/omissions of not clearly using the provided gel (57% total; Figure 5 C, green circle) and incompletely defining a mode of inheritance (26% total; Figure 5 C, blue circle). Even after receiving the hint about X chromosome allele number, many students made the most common omission of not discussing the number of alleles per gene in males and females (“No discussion of copy number”; 88% of 8 answers), and none of these students used the content language of the hint.
Nondisjunction
In the nondisjunction problems, students were asked to identify the cause of an incorrect chromosome number after meiosis. Three errors in understanding of meiosis were present at similar levels in answers in the 000 group, including students not accurately describing homologues versus sister chromatids and/or in what phase they separated at the metaphase plate (30% of 33 answers; Figure 5 D, orange circle), students not sufficiently understanding that phases in meiosis (I or II) should be considered and differentiated (42%; Figure 5 D, green circle), and students not understanding the typical outcome of meiosis or how errors could occur (33%; Figure 5 D, blue circle). After receiving the hint describing chromosome alignment during meiosis, several students still made the error of not accurately describing homologues versus sister chromatids and/or in what phases they separated in meiosis (“Incorrect chromosome definition/separation rules”; 38% of 13 answers), even though some of these students ( n = 3) used the content language of the hint.
Practice Is Associated with Higher Longer-Term Assessment Performance
In addition to the immediate impact of a hint on student performance during a practice assignment, we also examined whether practice itself was associated with longer-term performance on a final exam. Of the 233 students who completed practice assignments, 133 completed both assignments, and 100 completed only one assignment. To ensure that all practice predictor variables were calculated based on the same number of questions (three Q1s and Q3s), we focused on only the students who completed both practice assignments. Of the 133 students who completed both assignments, 109 of these students completed the GCA pre- and posttest and instructor-generated final exam: These are the students included in the final analyses reported in Table 5 and Supplemental Tables S4 and S5. Using the mean performance on Q3 practice questions as a measure of “success” in the practice assignments (Supplemental Table S4), we found that, for students who completed both practice assignments, success in practice significantly predicted both GCA posttest and instructor-generated question performance for practice-related questions (controlling for mean Q1 performance and GCA pretest performance; Table 5 , models 1 and 2). These students also had significantly higher scores on practice-unrelated GCA posttest and instructor-generated questions ( Table 5 , models 3 and 4).
OLS regression estimates of the association between practice performance and final exam performance a
* p < 0.05.
** p < 0.01.
*** p < 0.001.
Finally, we examined whether there was a difference in final exam performance between students who did not complete any practice assignments and those who completed both assignments. There were 35 students who did not complete any practice assignments but did complete the GCA pre- and posttest and instructor-generated final exam. We used GCA pretest scores to control for potential differences in incoming genetics knowledge between the group of students who completed both practice assignments and those who completed none, although we could not control for other factors, such as motivation or interest. There was no significant difference in the GCA pretest scores between these two groups (Supplemental Table S4), but students who completed the practice questions had higher GCA posttest and instructor-generated final exam scores than students who did not practice (Supplemental Table S5).
Content Hints Help a Subset of Students during Problem-Solving Practice
We administered genetics practice problems to students on concepts that had already been presented and practiced in class. Overall, we found that some students benefit from this practice, in particular if they initially answer incorrectly. Owing to the design of our study, each student completed at least one question (Q1) within a content area without any assistance. Students then received a hint on one of the subsequent questions. This provided students with the opportunity to struggle through the first question for each concept on their own before receiving assistance. An initial struggle without assistance, followed by feedback, has been shown to help students’ future performance ( Kapur and Bielaczyc, 2012 ), and although we did not provide feedback to students about whether they were correct or incorrect in their initial answers, we gave all students a chance to receive scaffolding via a content hint. For students who had initially answered Q1 incorrectly, when they took a content hint while answering Q2, 37% answered correctly, while only 21% of students answered this question correctly if they did not take a hint at Q2. This difference of 16% indicates that, although practice alone can help, practice with content scaffolding helps more students. In addition, we have demonstrated that students benefit from a content hint regardless of whether they receive that hint at the second question or at the third question. This suggests that students who are learning from the hint at Q2 are able to apply this knowledge in answering the next question. Once they receive a key piece of content, the students who use the hint successfully continue to do so on future problems.
Not all students in this study chose to take an offered hint when solving practice problems. Students who did not take a hint for a particular trio had a higher Q1 score than students who did take a hint. Along with these baseline differences in performance, several possible factors could have influenced students’ choices. One component of student choice could relate to self-regulatory capacity in monitoring their understanding ( Aleven et al. , 2003 ). Students who did not take a hint may have felt confident in their problem-solving ability and thus chose not to view additional information they felt they already knew. In a study that examined students’ use of three-dimensional molecular models to assist in drawing molecular representations, some students did not use models even when the models were placed directly into their hands ( Stull et al. , 2012 ). Some of these students reported thinking they did not need the models to answer the given questions ( Stull et al. , 2012 ). This supports the idea that students who do not use provided hints may simply feel they do not need them. On the other hand, 29% of the students in our study who did not take a hint answered the first question incorrectly, indicating their confidence was misplaced. Similarly, in a study that offered computer-tailored hints for solving problems, even students predicted to benefit from hints did not always take them ( Aleven et al. , 2006 ). In the current study, due to the constructed-response nature of the questions, students could not receive immediate feedback on whether they correctly answered a question. Thus, there would be value in examining whether immediate feedback on performance would influence students’ future choices. Because we could not discover students’ rationales for not taking a hint in this study, we cannot make any further conclusions about their choices.
Utility of a Single Content Idea
We showed that the inclusion of just one content idea as a hint helped some initially struggling students understand a concept, potentially by activating their prior knowledge related to the hint content. In looking at these students’ problem solving, we found that students who improved in a given content trio (011 and 001 groups) more often used language similar to the content of the hint than students who consistently answered incorrectly in a given trio (000 group). Thus, for students helped by the hint, this particular piece of content likely was critical for correctly solving the problem. Adding to previous frameworks ( Alexander and Judy, 1988 ; Alexander et al. , 1989 ), we suggest that this declarative (content) knowledge is the component of domain-specific knowledge that is needed to effectively apply procedural (e.g., strategic) knowledge to accurately solve a problem. In future studies, we plan to further explore the details of students’ procedural processes during problem solving and to determine whether a student’s inability to recall a piece of information is the main reason for an incorrect answer or whether there are additional higher-order cognitive skills and processes required for correct problem solving.
Some students continued to answer all questions in a content trio incorrectly (000 group) despite a content hint. These students often had multiple gaps in content knowledge or made content errors or omissions not related to the content hint. In future studies, students could receive tailored content hint(s) to match all errors that are present; this could allow us to determine whether the lack of content is the reason for incorrect answers, rather than a lack of procedural process skills. In one previous study, a computer program for solving problems that provides tailored hints and feedback was used to specifically assist in genetics problem solving, providing up to four hints specific to each component of a given problem (the Genetics Cognitive Tutor; Corbett et al. , 2010 ). The authors found a significant improvement in learning from pre- to postcompletion of this program ( Corbett et al. , 2010 ).
In cases in which students consistently answered incorrectly (000 group), some used language related to the content hint but made errors when trying to apply the hint in their explanations. If students have inaccurate knowledge on how to apply content, even when correct content ideas are provided, a hint may be insufficient. Indeed, Smith (1988) found that unsuccessful problem solvers can often identify important pieces of information but do not know how to apply this information. In this case, providing more scaffolding to a student, such as by providing students with worked examples of similar problems (e.g., Sweller and Cooper, 1985 ; Renkl and Atkinson, 2010 ) or providing more guided hints and feedback via a cognitive tutor (e.g., Corbett et al. , 2010 ), may be needed.
These students who consistently answer incorrectly may also be lacking critical problem-solving skills. In this study, we focused on the use and application of content knowledge, but in future studies, we will examine the problem-solving processes taken by students who answer correctly and compare these with the processes used by students who answer incorrectly. Certain skills may be particularly critical, such as displaying metacognitive ability (the knowledge and regulation of one’s own cognition). Activating prior knowledge by identifying similarities between problems is an effective metacognitive skill to help orient oneself to a problem ( Gick and Holyoak, 1980 ; Smith, 1988 ; Meijer et al. , 2006 ), and using this behavior in combination with several other metacognitive skills, including planning and checking work, can improve problem-solving ability ( Mevarech and Amrany, 2008 ). Thus, a prompt that asks students to explain how the content in a hint is related to information the student has used previously to solve a problem may be helpful, as it may elicit their prior knowledge of solving similar problems.
Content-Specific Errors and Omissions
Recombination..
For the topic of recombination, students who answered consistently incorrectly (000 group) did not often use map units to determine the probability of offspring when considering two linked genes; instead, many students attempted to solve the problem using Punnett squares and/or the logic of solving a probability question for genes on different chromosomes. Even when students used map units, they often either performed incorrect calculations or assigned recombinant probabilities to the incorrect genotypes. This suggests that the conceptual idea behind calculating probability of inheritance using linked genes is challenging.
Probability.
Students struggled in calculating the probability that an unaffected child of two heterozygotes would be a heterozygote. Instead of considering information in the pedigree that would allow them to eliminate one of the genotype possibilities (homozygous recessive), students often assumed that the probability of a heterozygote offspring of carriers would be 1/2 rather than 2/3. For students who answered these questions consistently incorrectly (000 group), the most common error included the combination of not using the probability of 2/3 with failing to use the product rule appropriately to account for multiple generations. This suggests that struggling students do not understand the broader concept of how to consider multiple generations when determining probability and thus have difficulty integrating multiple ideas into their solutions. Indeed, previous work has shown that many students have difficulty in using both of these types of calculations ( Smith, 1988 ; Smith and Knight, 2012 ).
Gel/pedigree.
Students who answered consistently incorrectly (000 group) most frequently displayed difficulty in reading the gel to identify the number of allele copies and then connecting this information to the pedigree. In this course, students were taught that, although gels are not always quantitative, one can use the thickness of bands on a DNA gel to determine the relative amounts of DNA present in a sample. Despite being taught this convention, students still had difficulty applying the concept of both allele number (e.g., only one X chromosome allele for a male) and amount of DNA (e.g., a thicker band representing two of the same alleles for an individual). Thus, students need more practice understanding the concept of interpreting information on gels.
Nondisjunction.
In nondisjunction questions, students who consistently answered incorrectly (000 group) had a diversity of misunderstandings about meiosis, with three errors being most common. The nondisjunction questions explicitly asked students to specify a phase in meiosis, if any, that was affected. However, students often failed to consider in which meiotic division, I or II, an error could occur, or they expressed uncertainty about differentiating between the two phases of meiosis. Students also struggled with identifying when during meiosis homologous versus sister chromatids separate; they sometimes attempted to identify the type of chromosome that was failing to separate or to state when each would normally separate, but they were often incorrect. The third error students made represented a general misunderstanding of meiosis in which students incorrectly identified the number of each chromosome that should be present in a gamete, or students assumed an atypical event, such as multiple rounds of replication, must have occurred to produce a gamete with one extra chromosome. Previous work on this topic also found that students demonstrate many errors when depicting meiosis, including incorrect chromosome alignment during metaphase ( Wright and Newman, 2011 ; Newman et al. , 2012 ).
As with previous studies that report on the testing effect (e.g., Adesope et al. , 2017 ; Butler, 2010 ), we found that practice was associated with later assessment performance. Regardless of practice Q1 performance and GCA pretest performance, student success in practice predicted students’ longer-term performance, both practice related and practice unrelated, on their instructor-generated final exam and GCA scores in a course. We also showed that those students who completed both practice assignments performed better than students who did not complete any practice assignments, controlling for GCA pretest performance. Because we could not randomize students into practice or no-practice conditions, we caution that, even though we used the GCA pretest as a proxy for incoming ability, there are likely many other factors influencing these students’ performance. Other factors shown to relate to success include student motivation, interest, and metacognition (e.g., Pintrich and de Groot, 1990 ; Schiefele et al. , 1992 ; Young and Fry, 2008 ).
Limitations
Our study addressed four critical content areas in genetics with which we know students struggle. However, students likely have additional or different difficulties on other genetics content. In addition, these questions had only a single correct answer and thus may have been limited in their ability to test student problem-solving skills. In the future, we would like to examine more ill-defined questions with multiple possible solutions ( National Research Council, 2012 ).
While we anticipated that most students would take the option to receive a hint, only 68% of students did so. To provide an accurate representation of the influence of a hint, we had to limit our analyses to those who chose to take a hint. As seen in Stull and colleagues’ ( 2012 ) work on molecular model use and as suggested by our data examining use of the content language reflected in the hint, not all students are likely to use hints, even when hints are easily available. However, it would be interesting to know why students who choose not to take a hint make that decision and whether this decision is based on high confidence or fear that the hint may confuse them.
We also could not test directly whether students who took a hint performed better than those who did not take a hint in longer-term performance, as the only way to measure this is to randomize the students who do and do not receive a hint. We chose not to take this approach, because we felt it was important for student success to give everyone the same access to information.
Implications for Instruction
This study suggests that, after learning a topic in class, a subset of students who initially give incorrect answers to problems on these topics can improve after receiving a single content idea that may fill a knowledge gap. Some students may generally understand how to solve these problems but lack one or two pieces of information; providing the missing piece allows them to apply their knowledge and solve the problem. For these students, reviewing certain pieces of genetics content, which we describe in this study, may be enough to help them solve such problems correctly. Furthermore, we suggest emphasizing the importance of practicing, as this study showed that success at the end of practice predicts longer-term performance in a class, regardless of initial understanding of genetics topics. Even if a student initially struggles with an answer, this “productive failure” can be beneficial to the student’s learning ( Kapur and Bielaczyc, 2012 ). Students who continue to struggle despite content hints likely lack content knowledge as well as problem-solving skills. We plan to further examine such deficits in how students solve problems in order to provide suggestions that are focused on the logical steps and metacognitive processes necessary for solving problems. Such instruction may be most beneficial after students have an initial chance to practice problems, so that they have a chance to challenge themselves before receiving hints.
Supplementary Material
Acknowledgments.
This work was supported by the National Science Foundation (DUE 1711348). We thank Oscar Whitney for assistance with initial development and testing of questions and Ashton Wiens and Felix Jimenez for assistance with statistical analyses. We are also grateful to Paula Lemons, Stephanie Gardner, and Laura Novick for their advice on the project and to all of the students who participated in this study.
- Adesope O. O., Trevisan D. A., Sundararajan N. (2017). Rethinking the use of tests: A meta-analysis of practice testing . Review of Educational Research , ( 3 ), 659–701. 10.3102/0034654316689306 [ CrossRef ] [ Google Scholar ]
- Aleven V., McLaren B., Roll I., Koedinger K. (2006). Toward meta-cognitive tutoring: A model of help-seeking with a cognitive tutor . International Journal of Artificial Intelligence in Education , , 101–130. [ Google Scholar ]
- Aleven V., Stahl E., Schworm S., Fischer F., Wallace R. (2003). Help seeking and help design in interactive learning environments . Review of Educational Research , ( 3 ), 277–320. 10.3102/00346543073003277 [ CrossRef ] [ Google Scholar ]
- Alexander P. A., Judy J. E. (1988). The interaction of domain-specific and strategic knowledge in academic performance . Review of Educational Research , ( 4 ), 375–404. 10.3102/00346543058004375 [ CrossRef ] [ Google Scholar ]
- Alexander P. A., Pate P. E., Kulikowich J. M., Farrell D. M., Wright N. L. (1989). Domain-specific and strategic knowledge: Effects of training on students of differing ages or competence levels . Learning and Individual Differences , ( 3 ), 283–325. 10.1016/1041-6080(89)90014-9 [ CrossRef ] [ Google Scholar ]
- American Association for the Advancement of Science. (2011). Vision and change in undergraduate biology education: A call to action . Washington, DC. [ Google Scholar ]
- Bassok M., Novick L. R. (2012). Problem solving . In Holyoak K. J., Morrison R. G. (Eds.), Oxford handbook of thinking and reasoning (pp. 413–432). New York: Oxford University Press. [ Google Scholar ]
- Bloom B. S., Engelhart M. D., Furst E. J., Hill W. M., Krathwohl D. R. (1956). Taxonomy of educational objectives: The classification of educational goals . New York: David McKay. [ Google Scholar ]
- Bransford J. D., Johnson M. K. (1972). Contextual prerequisites for understanding: Some investigations of comprehension and recall . Journal of Verbal Learning and Verbal Behavior , ( 6 ), 717–726. 10.1016/S0022-5371(72)80006-9 [ CrossRef ] [ Google Scholar ]
- Butler A. C. (2010). Repeated testing produces superior transfer of learning relative to repeated studying . Journal of Experimental Psychology. Learning, Memory, and Cognition , ( 5 ), 1118–1133. 10.1037/a0019902 [ PubMed ] [ CrossRef ] [ Google Scholar ]
- Chi M. T. H. (1981). Knowledge development and memory performance . In Friedman M. P., Das J. P., O’Connor N. (Eds.), Intelligence and learning (pp. 221–229). Boston: Springer US; 10.1007/978-1-4684-1083-9_20 [ CrossRef ] [ Google Scholar ]
- Chi M. T. H., Feltovich P. J., Glaser R. (1981). Categorization and representation of physics problems by experts and novices . Cognitive Science , ( 2 ), 121–152. 10.1207/s15516709cog0502_2 [ CrossRef ] [ Google Scholar ]
- Corbett A., Kauffman L., Maclaren B., Wagner A., Jones E. (2010). A Cognitive Tutor for genetics problem solving: Learning gains and student modeling . Journal of Educational Computing Research , ( 2 ), 219–239. [ Google Scholar ]
- Dooling D. J., Lachman R. (1971). Effects of comprehension on retention of prose . Journal of Experimental Psychology , , 216. [ Google Scholar ]
- Gick M. L., Holyoak K. J. (1980). Analogical problem solving . Cognitive Psychology , ( 3 ), 306–355. 10.1016/0010-0285(80)90013-4 [ CrossRef ] [ Google Scholar ]
- Kapur M., Bielaczyc K. (2012). Designing for productive failure . Journal of the Learning Sciences , ( 1 ), 45–83. 10.1080/10508406.2011.591717 [ CrossRef ] [ Google Scholar ]
- Landis J. R., Koch G. G. (1977). The measurement of observer agreement for categorical data . Biometrics , ( 1 ), 159–174. [ PubMed ] [ Google Scholar ]
- Meijer J., Veenman M. V. J., van Hout-Wolters B. H. A. M. (2006). Metacognitive activities in text-studying and problem-solving: Development of a taxonomy . Educational Research and Evaluation , ( 3 ), 209–237. 10.1080/13803610500479991 [ CrossRef ] [ Google Scholar ]
- Mevarech Z. R., Amrany C. (2008). Immediate and delayed effects of meta-cognitive instruction on regulation of cognition and mathematics achievement . Metacognition and Learning , ( 2 ), 147–157. 10.1007/s11409-008-9023-3 [ CrossRef ] [ Google Scholar ]
- National Research Council. (2012). Discipline-based education research: Understanding and improving learning in undergraduate science and engineering . Washington, DC: National Academies Press; 10.17226/13362 [ CrossRef ] [ Google Scholar ]
- Newman D. L., Catavero C. M., Wright L. K. (2012). Students fail to transfer knowledge of chromosome structure to topics pertaining to cell division . CBE—Life Sciences Education , ( 4 ), 425–436. 10.1187/cbe.12-01-0003 [ PMC free article ] [ PubMed ] [ CrossRef ] [ Google Scholar ]
- Novick L. R., Bassok M. (2005). Problem solving . In Holyoak K. J., Morrison R. G. (Eds.), The Cambridge handbook of thinking and reasoning (pp. 321–349). New York: Cambridge University Press. [ Google Scholar ]
- Pintrich P. R., de Groot E. V. (1990). Motivational and self-regulated learning components of classroom academic performance . Journal of Educational Psychology , ( 1 ), 33–40. 10.1037/0022-0663.82.1.33 [ CrossRef ] [ Google Scholar ]
- Pol H. J., Harskamp E. G., Suhre C. J. M., Goedhart M. J. (2008). The effect of hints and model answers in a student-controlled problem-solving program for secondary physics education . Journal of Science Education and Technology , ( 4 ), 410–425. 10.1007/s10956-008-9110-x [ CrossRef ] [ Google Scholar ]
- Prevost L. B., Lemons P. P. (2016). Step by step: Biology undergraduates’ problem-solving procedures during multiple-choice assessment . CBE—Life Sciences Education , ( 4 ), ar71 10.1187/cbe.15-12-0255 [ PMC free article ] [ PubMed ] [ CrossRef ] [ Google Scholar ]
- Renkl A., Atkinson R. K. (2010). Learning from worked-out examples and problem solving . In Plass J. L., Moreno R., Brünken R. (Eds.), Cognitive load theory (pp. 91–108). New York: Cambridge University Press. [ Google Scholar ]
- Roediger H. L., Karpicke J. D. (2006). Test-enhanced learning: Taking memory tests improves long-term retention . Psychological Science , ( 3 ), 249–255. 10.1111/j.1467-9280.2006.01693.x [ PubMed ] [ CrossRef ] [ Google Scholar ]
- Schiefele U., Krapp A., Winteler A. (1992). Interest as a predictor of academic achievement: A meta-analysis of research . In Renninger K. A., Hidi S., Krapp A. (Eds.), The role of interest in learning and development (pp. 183–212). Hillsdale, NJ: Erlbaum. [ Google Scholar ]
- Smith J. I., Combs E. D., Nagami P. H., Alto V. M., Goh H. G., Gourdet M. A. A., … Tanner K. D. (2013). Development of the biology card sorting task to measure conceptual expertise in biology . CBE—Life Sciences Education , ( 4 ), 628–644. 10.1187/cbe.13-05-0096 [ PMC free article ] [ PubMed ] [ CrossRef ] [ Google Scholar ]
- Smith M. K., Knight J. K. (2012). Using the Genetics Concept Assessment to document persistent conceptual difficulties in undergraduate genetics courses . Genetics , ( 1 ), 21–32. 10.1534/genetics.111.137810 [ PMC free article ] [ PubMed ] [ CrossRef ] [ Google Scholar ]
- Smith M. K., Wood W. B., Knight J. K. (2008). The Genetics Concept Assessment: A new concept inventory for gauging student understanding of genetics . CBE—Life Sciences Education , ( 4 ), 422–430. [ PMC free article ] [ PubMed ] [ Google Scholar ]
- Smith M. U. (1988). Successful and unsuccessful problem solving in classical genetic pedigrees . Journal of Research in Science Teaching , ( 6 ), 411–433. 10.1002/tea.3660250602 [ CrossRef ] [ Google Scholar ]
- Smith M. U., Good R. (1984). Problem solving and classical genetics: Successful versus unsuccessful performance . Journal of Research in Science Teaching , ( 9 ), 895–912. 10.1002/tea.3660210905 [ CrossRef ] [ Google Scholar ]
- Stull A. T., Hegarty M., Dixon B., Stieff M. (2012). Representational translation with concrete models in organic chemistry . Cognition and Instruction , ( 4 ), 404–434. 10.1080/07370008.2012.719956 [ CrossRef ] [ Google Scholar ]
- Sweller J., Cooper G. A. (1985). The use of worked examples as a substitute for problem solving in learning algebra . Cognition and Instruction , ( 1 ), 59–89. 10.1207/s1532690xci0201_3 [ CrossRef ] [ Google Scholar ]
- Wright L. K., Newman D. L. (2011). An interactive modeling lesson increases students’ understanding of ploidy during meiosis . Biochemistry and Molecular Biology Education , ( 5 ), 344–351. 10.1002/bmb.20523 [ PubMed ] [ CrossRef ] [ Google Scholar ]
- Young A., Fry J. D. (2008). Metacognitive awareness and academic achievement in college students . Journal of the Scholarship of Teaching and Learning , ( 2 ), 1–10. [ Google Scholar ]
Change Password
Your password must have 8 characters or more and contain 3 of the following:.
- a lower case character,
- an upper case character,
- a special character
Password Changed Successfully
Your password has been changed
- Sign in / Register
Request Username
Can't sign in? Forgot your username?
Enter your email address below and we will send you your username
If the address matches an existing account you will receive an email with instructions to retrieve your username
Successful Problem Solving in Genetics Varies Based on Question Content
- Jennifer S. Avena
- Betsy B. McIntosh
- Oscar N. Whitney
- Ashton Wiens
- Jennifer K. Knight
Department of Molecular, Cellular, and Developmental Biology
School of Education, University of Colorado Boulder, Boulder, CO 80309
Search for more papers by this author
Department of Applied Mathematics, University of Colorado Boulder, Boulder, CO 80309
*Address correspondence to: Jennifer Knight ( E-mail Address: [email protected] ).
Problem solving is a critical skill in many disciplines but is often a challenge for students to learn. To examine the processes both students and experts undertake to solve constructed-response problems in genetics, we collected the written step-by-step procedures individuals used to solve problems in four different content areas. We developed a set of codes to describe each cognitive and metacognitive process and then used these codes to describe more than 1800 student and 149 expert answers. We found that students used some processes differently depending on the content of the question, but reasoning was consistently predictive of successful problem solving across all content areas. We also confirmed previous findings that the metacognitive processes of planning and checking were more common in expert answers than student answers. We provide suggestions for instructors on how to highlight key procedures based on each specific genetics content area that can help students learn the skill of problem solving.
INTRODUCTION
The science skills of designing and interpreting experiments, constructing arguments, and solving complex problems have been repeatedly called out as critical for undergraduate biology students to master ( American Association for the Advancement of Science, 2011 ). Yet each of these skills remains elusive for many students, particularly when the skill requires integrating and evaluating multiple pieces of information ( Novick and Bassok, 2005 ; Bassok and Novick, 2012 ; National Research Council, 2012 ). In this paper, we focus on describing the steps students and experts take while solving genetics problems and determining whether the use of certain processes increases the likelihood of success.
The general process of solving a problem has been described as building a mental model in which prior knowledge can be used to represent ways of thinking through a problem state ( Johnson-Laird, 2010 ). Processes used in problem solving have historically been broken down into two components: those that use domain-general knowledge and those that use domain-specific knowledge. Domain-general knowledge is defined as information that can be used to solve a problem in any field, including such strategies as rereading and identifying what a question is asking ( Alexander and Judy, 1988 ; Prevost and Lemons, 2016 ). Although such steps are important, they are unlikely to be the primary determinants of success when specific content knowledge is required. Domain-specific problem solving, on the other hand, is a theoretical framework that considers one’s discipline-specific knowledge and processes used to solve a problem (e.g., Prevost and Lemons, 2016 ). Domain-specific knowledge includes declarative (knowledge of content), procedural (how to utilize certain strategies), and conditional knowledge (when and why to utilize certain strategies) as they relate to a specific discipline ( Alexander and Judy, 1988 ; Schraw and Dennison, 1994 ; Prevost and Lemons, 2016 ).
Previous studies on problem solving within a discipline have emphasized the importance of domain-specific declarative and conditional knowledge, as students need to understand and be able to apply relevant content knowledge to successfully solve problems ( Alexander et al. , 1989 ; Alexander and Judy, 1988 ; Prevost and Lemons, 2016 ). Our prior work ( Avena and Knight 2019 ) also supported this necessity. After students solved a genetics problem within a content area, they were offered a content hint on a subsequent content-matched question. We found that content hints improved performance overall for students who initially did not understand a concept. In characterizing the students’ responses, we found that the students who benefited from the hint typically used the content language of the hint in their solution. However, we also found that some students who continued to struggle included the content language of the hint but did not use the information in their problem solutions. For example, in solving problems on predicted recombination frequency for linked genes, an incorrect solution might use the correct terms of map units and/or recombination frequency but not actually use map units to solve the problem. Thus, these findings suggest that declarative knowledge is necessary but not sufficient for complex problem solving and also emphasize the importance of procedural knowledge, which includes the “logic” of generating a solution ( Avena and Knight, 2019 ). By definition, procedural knowledge uses both cognitive processes, such as providing reasoning for a claim or executing a task, and metacognitive processes, such as planning how to solve a problem and checking (i.e., evaluating) one’s work (e.g., Kuhn and Udell, 2003 ; Meijer et al. , 2006 ; Tanner, 2012 ). We explore these processes in more detail below.
Cognitive Processing: Reasoning
Generating reasoning requires using one’s knowledge to search for and explain an appropriate set of ideas to support or refute a given model ( Johnson-Laird, 2010 ), so reasoning is likely to be a critical component of solving problems. Toulmin’s original scheme for building a scientific argument ( Toulmin, 1958 ) included generating a claim, identifying supporting evidence, and then using reasoning (warrant) to connect the evidence to the claim. Several studies have demonstrated a positive relationship between general reasoning “ability” ( Lawson, 1978 ), defined as the ability to construct logical links between evidence and conclusions using conceptual principles, and performance ( Cavallo, 1996 ; Cavallo et al. , 2004 ; Johnson and Lawson, 1998 ). As elaborated in more recent literature, there are many specific subcategories of reasoning. Students commonly use memorized patterns or formulas to solve problems: this approach is considered algorithmic and could be used to provide logic for a problem ( Jonsson et al. , 2014 ; Nyachwaya et al. , 2014 ). Such algorithmic reasoning may be used with or without conveying an understanding of how an algorithm is used ( Frey et al. , 2020 ). When an algorithm is not appropriate (or not used) in describing one’s reasoning, but instead the solver provides a generalized explanation of underlying connections, this is sometimes referred to as “explanatory” or “causal” reasoning ( Russ et al. , 2008 ). Distinct from causal reasoning is the domain-specific form of mechanistic reasoning, in which a mechanism of action of a biological principle is elaborated ( Russ et al. , 2008 ; Southard et al. , 2016 ). Another common form of reasoning is quantitative reasoning, which can also be described as statistical or, in other specialized situations, graph-construction reasoning (e.g., Deane et al. , 2016 ; Angra and Gardner, 2018 ). The detailed studies of these specific subcategories of reasoning have usually involved extensive interviews with students and/or very specific guidelines that prompt the use of a particular type of reasoning. Those who have explored students’ unprompted general use of reasoning have found that few students naturally use reasoning to support their ideas ( Zohar and Nemet, 2002 ; James and Willoughby, 2011 ; Schen, 2012 ; Knight et al. , 2015 ; Paine and Knight, 2020 ). However, with explicit training to integrate their knowledge into mental models ( Kuhn and Udell, 2003 ; Osborne, 2010 ) or with repeated cueing from instructors ( Russ et al. , 2008 ; Knight et al. , 2015 ), students can learn to generate more frequent, specific, and robust reasoning.
Metacognitive Processing
Successfully generating possible solutions to problems likely also involves metacognitive thinking . Metacognition is often separated into two components: metacognitive knowledge (knowledge about one’s own understanding and learning) and metacognitive regulation (the ability to change one’s approach to learning; Flavell, 1979 ; Jacobs and Paris, 1987 ; Schraw and Moshman, 1995 ). Metacognitive regulation is usually defined as including such processes as planning, monitoring one’s progress, and evaluating or checking an answer ( Flavell, 1979 ; Jacobs and Paris, 1987 ; Schraw and Moshman, 1995 ; Tanner, 2012 ). Several studies have shown that helping students use metacognitive strategies can benefit learning. For example, encouraging the planning of a possible solution beforehand and checking one’s work afterward helps students generate correct answers during problem solving (e.g., Mevarech and Amrany, 2008 ; McDonnell and Mullally, 2016 ; Stanton et al. , 2015 ). However, especially compared with experts, students rarely use metacognitive processes, despite their value ( Smith and Good, 1984 ; Smith, 1988 ). Experts spend more time orienting, planning, and gathering information before solving a problem than do students, suggesting that experts can link processes that facilitate generating a solution with their underlying content knowledge ( Atman et al. , 2007 ; Peffer and Ramezani, 2019 ). Experts also check their problem-solving steps and solutions before committing to an answer, steps not always seen in student responses ( Smith and Good, 1984 ; Smith, 1988 ). Ultimately, prior work suggests that, even when students understand content and employ appropriate cognitive processes, they may still struggle to solve problems that require reflective and regulative skills.
Theoretical Framework: Approaches to Learning
Developing domain-specific conceptual knowledge requires integrating prior knowledge and new disciplinary knowledge ( Schraw and Dennison, 1994 ). In generating conceptual knowledge, students construct mental models in which they link concepts together to generate a deeper understanding ( Johnson-Laird, 2001 ). These mental constructions involve imagining possible relationships and generating deductions and can be externalized into drawn or written models for communicating ideas ( Chin and Brown, 2000 ; Bennett et al. , 2020 ). Mental models can also trigger students to explain their ideas to themselves (self-explanation), which can also help them solve problems ( Chi et al. , 1989 ).
As our goal is to make visible how students grapple with their knowledge during problem solving, we fit this study into the approaches to learning framework (AtL: Chin and Brown, 2000 ). This framework, derived from detailed interviews of middle-school students solving chemistry problems, defines five elements of how students approach learning and suggests that these components promote deeper learning. Three of these elements are identifiable in the current study: engaging in explanations (employing reasoning through understanding and describing relationships and mechanisms), using generative thinking (application of prior knowledge and analogical transfer), and engaging in metacognitive activity (monitoring progress and modifying approaches). The remaining two elements: question asking (focusing on facts or on understanding) and depth of approaching tasks (taking a deep or a surface approach to learning: Biggs, 1987 ) could not be addressed in our study. However, previous studies showed that students who engage in a deep approach to learning also relate new information to prior knowledge and engage in reasoning (explanations), generate theories for how things work (generative thinking), and reflect on their understanding (metacognitive activity). In contrast, those who engage in surface approaches focus more on memorized, isolated facts than on constructing mental or actual models, demonstrating an absence of the three elements described by this framework. Biggs (1987) also previously provided evidence that intrinsically motivated learners tended to use a deep approach, while those who were extrinsically motivated (e.g., by grades), tended to use a surface approach. Because solving complex problems is, at its core, about how students engage in the learning process, these AtL components helped us frame how students’ learning is revealed by their own descriptions of their thinking processes.
Characterizing Problem-Solving Processes
Thus far, a handful of studies have investigated the processes adult students use in solving biology problems, and how these processes might influence their ability to develop reasonable answers ( Smith and Good, 1984 ; Smith, 1988 ; Nehm, 2010 ; Nehm and Ridgway, 2011 ; Novick and Catley, 2013 ; Prevost and Lemons, 2016 ; Sung et al. , 2020 ). In one study, Prevost and Lemons (2016) collected and analyzed students’ written documentation of their problem-solving procedures when answering multiple-choice questions. Students were taught to document their step-by-step thinking as they answered multiple-choice exam questions that ranged from Bloom’s levels 2 to 4 (understand to analyze; Bloom et al. , 1956 ), describing the steps they took to answer each question. The authors’ qualitative analyses of students’ documented problem solving showed that students frequently used domain-general test-taking skills, such as comparing the language of different multiple-choice distractors. However, students who correctly answered questions tended to use more domain-specific procedures that required knowledge of the discipline, such as analyzing visual representations and making predictions, than unsuccessful students. When students solved problems that required the higher-order cognitive skills of application and analysis, they also used more of these specific procedures than when solving lower-level questions. Another recent study explored how students solved exam questions on the genetic topics of recombination and nondisjunction through in-depth clinical interviews ( Sung et al. , 2020 ). These authors described two approaches that are not conceptual: using algorithms to bypass conceptual thinking and using non–biology specific test-taking strategies (e.g., length of answer, specificity of terminology). They also showed that students sometimes alternate between using an algorithm and a conceptual strategy, defaulting to the algorithm when they do not understand the underlying biological concept.
Research Question 1. How do experts and students differ in their description of problem-solving processes, using a much larger sample size than found in the previous literature (e.g., Chi et al. , 1981 ; Smith and Good, 1984 ; Smith, 1988 ; Atman et al. , 2007 ; Peffer and Ramezani, 2019 ).
Research Question 2. Are certain problem-solving processes more likely to be used in correct than in incorrect student answers?
Research Question 3. Do problem-solving processes differ based on content and are certain combinations of problem-solving processes associated with correct student answers for each content area?
Mixed-Methods Approach
This study used a mixed-methods approach, combining both qualitative and quantitative research methods and analysis to understand a phenomenon more deeply ( Johnson et al. , 2007 ). Our goal was to make student thinking visible by collecting written documentation of student approaches to solving problems (qualitative data), in addition to capturing answer correctness (quantitative data), and integrating these together in our analyses. The student responses serve as a rich and detailed data set that can be interpreted using the qualitative process of assigning themes or codes to student writing ( Hammer and Berland, 2014 ). In a qualitative study, the results of the coding process are unpacked using examples and detailed descriptions to communicate the findings. In this study, we share such qualitative results but also convert the coded results into numerical representations to demonstrate patterns and trends captured in the data. This is particularly useful in a large-scale study, because the output can be analyzed statistically to allow comparisons between categories of student answers and different content areas.
Students in this study were enrolled in an introductory-level undergraduate genetics course for biology majors at the University of Colorado in Spring 2017 ( n = 416). This course is the second in a two-course introductory series, with the first course being Introduction to Cell and Molecular Biology. The students were majority white, 60% female, and 63% were in their first or second year. Ninety percent of the students were majoring in biology or a biology-related field (neuroscience, integrative physiology, biochemistry, biomedical engineering). Of the students enrolled in the course, 295 students consented to be included in the study; some of the student responses have been previously described in the prior study ( Avena and Knight, 2019 ). We recruited experts from the Society for the Advancement of Biology Education Research Listserv by inviting graduate students, postdoctoral fellows, and faculty to complete an anonymous online survey consisting of the same questions that students answered. Of the responses received, we analyzed responses from 52 experts. Due to the anonymous nature of the survey, we did not collect descriptive data about the experts.
Problem Solving
As part of normal course work, students were offered two practice assignments covering four content areas related to each of two course exams (also described in Avena and Knight, 2019 ). Students could answer up to nine questions in blocks of three questions each, in randomized order, for three of the four content areas. Expert participants answered a series of four questions, one in each of the four content areas. All questions were offered online using the survey platform Qualtrics. All participants were asked to document their problem-solving processes as they completed the questions (as in Prevost and Lemons 2016 ), and they were provided with written instructions and an example in the online platform only (see Supplemental Material); no instructions were provided in class, and no explicit discussion of types of problem-solving processes to use were provided in class throughout the semester. Students could receive extra credit up to ∼1% of the course point total, obtaining two-thirds credit for explaining their answer and an additional one-third if they answered correctly. All students who completed the assignment received credit regardless of their consent to participate in the research.
We used questions developed for a prior study ( Avena and Knight, 2019 ) on four challenging genetics topics: calculation of the probability of inheritance across multiple generations (Probability), prediction of the cause of an incorrect chromosome number after meiosis (Nondisjunction), interpretation of a gel and pedigree to determine inheritance patterns (Gel/Pedigree), and prediction of the probability of an offspring’s genotype using linked genes (Recombination; see example in Figure 1 ; all questions presented in Supplemental Material). These content areas have previously been shown to be challenging based on student performance ( Smith et al. , 2008 ; Smith and Knight, 2012 ; Avena and Knight, 2019 ). Each content area contained three isomorphic questions that addressed the same underlying concept, targeted higher-order cognitive processes ( Bloom et al. , 1956 ), and contained the same amount of information with a visual ( Avena and Knight, 2019 ). Each question had a single correct answer and was coded as correct (1) or incorrect (0). For each problem-solving assignment, we randomized 1) the order of the three questions within each content area for each student and 2) the order in which each content area was presented. During each set of three isomorphic questions, while solving one of the isomorphic problems, students also had the option to receive a “content hint,” a single most commonly misunderstood fact for each content area. We do not discuss the effects of the content hints in this paper (instead, see Avena and Knight, 2019 ).
FIGURE 1. Sample problem for students from the Gel/Pedigree content area. Problems in each content area contain a written prompt and an illustrated image, as shown in this example.
Process Coding
Students may engage in processes that they do not document in writing, but we are limited to analyzing only what they do provide in their written step-by-step descriptions. For simplicity, throughout this paper, a “process” is a thought documented by the participant that is coded as a particular process. When we refer to “failure” to use a process, we mean that a participant did not describe this thought process in the answer. Our initial analysis of student processes used a selection of codes from Prevost and Lemons (2016) and Toulmin’s ( 1958 ) original codes of Claim and Reason. We note that all the problems we used can potentially be solved using algorithms, memorized patterns previously discussed and practiced in the class, which may have limited the reasoning students supplied. Because of the complexity of identifying different types of reasoning, we did not further subcategorize the reasoning category in the scheme we present, as this is beyond the scope of this paper. We used an emergent coding process ( Saldana, 2015 ) to identify additional and different processes, including both cognitive and metacognitive actions. Thus, our problem-solving processes (PsP) coding scheme captures the thinking that students document while solving genetics problems (see individual process codes in Table 1 ). We used HyperRESEARCH software (ResearchWare, Inc.) to code each individual’s documented step-by-step processes. A step was typically a sentence and sometimes contained multiple ideas. Each step was given one or more codes, with the exception of reasoning supporting a final conclusion (see Table 2 for examples of coded responses). Each individual process code captures when the student describes that process, regardless of whether the statement is correct or incorrect. Four raters (J.K.K., J.S.A., O.N.W., B.B.M.) coded a total of 24 student answers over three rounds of coding and discussion to reach consensus and identify a final coding scheme. Following agreement on the codes, an additional 12 answers were coded by the four raters to determine interrater agreement. Specifically, in these 12 answers, there were 150 instances in which a code for a step was provided by one or more raters. For each of these 150 instances, we identified the number of raters who agreed. We then calculated a final interrater agreement of 83% by dividing the total number of raters who agreed for all 150 instances (i.e., 524) by the total number of possible raters to agree for four raters in 150 instances (i.e., 600). We excluded answers in which students did not describe their problem-solving steps and those in which students primarily or exclusively used domain-general processes (i.e., individual process codes within the General strategy category in Table 1 ) or made claims without any other supporting codes. The latter two exclusion criteria were used because such responses lacked sufficient description to identify the thought processes. The final data set included a total of 1853 answers from 295 students and 149 answers from 52 experts. We used only correct answers from experts to serve as a comparison to student answers, excluding an additional 29 expert answers that were incorrect.
aExamples of student responses are to a variety of content areas and have been edited for clarity. Each individual process code captures the student’s description, regardless of whether the statement is correct or incorrect.
aThe responses above are all solutions to the question in Figure 1 .
After initial coding and analyses, we identified that student use of drawing was differentially associated with correctness based on content area. Thus, to further characterize drawing use, two raters (J.S.A. and J.K.K.) explored incorrect student answers from Probability and Recombination. One rater examined 33 student answers to identify an initial characterization, and then two raters reviewed a subset of answers to agree upon a final scheme. Each rater then individually categorized a portion of the student answers, and the final interrater agreement on 10 student answers was 90%. Interrater agreement was calculated as described earlier, with each answer serving as one instance, so we divided the total number of raters agreeing for each answer (i.e., 18) by the total possible number of raters agreeing (i.e., 20).
Statistical Analyses
The unit of analysis for all models considered is an individual answer to a problem. We investigate three variations of linear models, specified below. The response variable in all cases is binary (presence/absence of process or correct/incorrect answer). Thus, the models are generalized linear models, and, more specifically, logistic regression models. Because our data contain repeated measures in the form of multiple answers per student, we specifically use generalized linear mixed models (GLMM) to include a random effect on the intercept term in all models, grouped by participant identifier ( Gelman and Hill, 2006 ; Theobald, 2018 ). This component of the model accounts for variability in the baseline outcome between participants. In our case, we can model each student’s baseline probability of answering a problem correctly or each participant’s baseline probability of using a given process (e.g., one student may use Reason more frequently than another student). Accounting for this variation yields better estimates of the fixed effects in the models.

The fitted models give some, but not all, pairwise comparisons among predictor groups. We conducted pairwise post hoc comparisons (e.g., expert vs. correct student, expert vs. incorrect student, correct student vs. incorrect student, or among the four content areas) to draw inferences about the differences among all groups. In particular, we performed Tukey pairwise honestly significant difference (HSD) tests for all pairs of groups, comparing estimated marginal means (estimated using the fitted model) on the logit scale. Using estimated marginal means corrects for unbalanced group sample sizes, and using the Tukey HSD test provides adjusted p values, facilitating comparison to a significance level of α = 0.05.
To ease reproducibility, we use “formula” notation conventionally used in R to specify the models we employ in this paper, which has the following general form: outcome = fixed effect + (1 | group). The random effects component is specified within parentheses, with the random effect on the left of the vertical bar and the grouping variable on the right.
Process present = Expert/Student answer status + (1| ID)
Process present = Content area + (1| ID)
where “Process present” is the response variable as described for model 1; “Content area” is the fixed effect: Factor-level grouping: Probability (1)/Nondisjunction (2)/Gel-Pedigree (3)/Recombination (4); and “(1|ID)” is the random effect as described for model 1.
Student answer correctness = Process 1 + Process 2 + … + Process X + (1| ID)
where “Student answer correctness” is the response variable: incorrect (0)/correct (1); “Process 1 + Process 2 + … + Process X” is the list of process factors entered into the model as the fixed effect: absent (0)/present (1); and “(1|ID)” is the random effect as described for models 1 and 2. We identified which components were associated with correctness by seeing which predictor coefficients remained non-zero in a representative lasso model. We identified a representative model for each content area by first identifying the lasso penalty with the lowest Akaike information criterion (AIC) to reduce variance and then identifying a lasso penalty with a similar AIC that could be used across all content areas. Because a penalty parameter of 25 and the penalty parameter with the lowest AIC for each content area had similar AIC values, we consistently used a penalty parameter of 25. Note that when the penalty parameter is set to zero, the GLMM model is recovered. On the other hand, when the penalty parameter is very large, no predictors are included in the model. Thus, the selected penalty parameter forced many, but not all, coefficients to 0, giving a single representative model for each content area.
All models and tests were performed in R (v. 3.5.1). We used the lme4 package in R ( Bates et al. , 2015 ) for models 1 and 2, and estimation of parameters was performed using residual maximum likelihood. For model 3, we used the glmmLasso package, and the model was fit using the default EM-type estimate. Post hoc pairwise comparisons were performed using the emmeans package.
Human Subjects Approval
Human research was approved by the University of Colorado Institutional Review Board (protocols 16-0511 and 15-0380).
The PsP Coding Scheme Helps Describe Written Cognitive and Metacognitive Processes
We developed a detailed set of codes, which we call the PsP scheme to characterize how individuals describe their solutions to complex genetics problems. Table 1 shows the 18 unique processes along with descriptions and examples for each. With the support of previous literature, we grouped the individual processes into seven strategies, also shown in Table 1 . All strategies characterized in this study were domain specific except the General category, which is domain general. We categorized a set of processes as Orientation based on a previously published taxonomy for think-aloud interviews ( Meijer et al. , 2006 ) and on information management processes from the Metacognitive Awareness Inventory ( Schraw and Dennison, 1994 ). Orienting processes include: Notice (identifying important information in the problem), Recall (activating prior knowledge without applying it), Identify Similarity (among question types), and Identify Concept (the “type” of problem). Orientation processes are relatively surface level, in that information is observed and noted, but not acted on. The Metacognition category includes the three common elements of planning (Plan), monitoring (Assess Difficulty), and evaluating (Check) cited in the metacognitive literature (e.g., Schraw and Moshman, 1995 ; Tanner, 2012 ). The Execution strategy includes actions taken to explicitly solve the problem, including Use Information (apply information related to the problem), Integrate (i.e., linking together two visual representations provided to solve the problem or linking a student’s own drawing to information in the problem), Draw, and Calculate. The Use Information category is distinguished from Recall by a student applying a piece of information (Use Information) rather than just remembering a fact without directly using it in the problem solution (Recall). Students may Recall and then Use Information, just Recall, or just Use Information. If a student used the Integrate process, Use Information was not also coded (i.e., Integrate supersedes Use Information). The Reasoning strategy includes just one general process of Reason, which we define as providing an explanation or rationale for a claim, as previously described in Knight et al. (2013) , Lawson (2010) , and Toulmin (1958) . The Conclusion strategy includes Eliminate and Claim, processes that provide types of responses to address the final answer. The single process within the Error strategy category, Misinterpret, characterizes steps in which students misunderstand the question stem. Finally, the General category includes the codes Clarify, State the Process, and Restate, all of which are generic statements of execution, representing processes that are domain general ( Alexander and Judy, 1988 ; Prevost and Lemons, 2016 ).
To help visualize the series of steps students took and how these steps differed across answers and content areas, we provide detailed examples in Tables 2 and 3 . In Table 2 , we provide three examples of similar-length documented processes to the same Gel/Pedigree problem ( Figure 1 ) from a correct expert, a correct student, and an incorrect student. Note the multiple uses of planning and reasoning in the expert answer, multiple uses of reasoning in the correct student answer, and the absence of both such processes in the incorrect student answer. The reasoning used in each case provides a logical explanation for the claim, which either immediately precedes or follows the reasoning statement. For example, in the second incident of Claim and Reason for Eliot, “because otherwise Zach could not be unaffected” is a logical explanation for the claim “it has to be dominant.” Similarly, for Cassie’s Claim and Reason code, “If both parents are heterozygous for the disease” is a logical explanation for the claim “it is probably inherited in a dominant manner.” Table 3 provides additional examples of correct student answers to the remaining three content areas. Note that for Probability and Recombination questions, the Reason process often explains why a certain genotype or probability is assigned (e.g., “otherwise all or none of the children would have the disease” explains why “Both parents of H and J must be Dd” in Li’s Probability answer) or how a probability is calculated, for example, “using the multiplication rule” (Li’s Probability explanation) or “multiply that by the 100% chance of getting ‘af’ from parent 2” (Preston’s Recombination explanation). In Nondisjunction problems, a student may claim that a nondisjunction occurred in a certain stage of meiosis (the Claim) because it produces certain gamete genotypes consistent with such an error (the Reason), as seen in Gabrielle’s answer.
aResponses edited slightly for clarity. See Table 2 for a correct student documented solution to the Gel/Pedigree problem.
Across All Content Areas, Expert Answers Are More Likely Than Student Answers to Contain Orientation, Metacognition, and Execution Processes
For each category of answers (expert, correct student, and incorrect student), we calculated the overall percent of answers that contained each process and compared these frequencies. Note that, in all cases, frequency represents the presence of a process in an answer, not a count of all uses of that process in an answer. The raw frequency of each process is provided in Table 4 , columns 2–4. To determine statistical significance, we used GLMM to account for individual variability in process use. The predicted likelihood of each process per group and pairwise comparisons between groups from this analysis is provided in Table 4 , columns 5–10. These comparisons show that expert answers were significantly more likely than student answers to contain the processes of Identify concept, Recall, Plan, Check, and Use Information ( Table 4 and Supplemental Table S1). The answers in Table 2 represent some of the typical trends identified for each group. For example, expert Eliot uses both Plan and Check, but these metacognitive processes are not used by either student, Cassie (correct answer) or Ian (incorrect answer).
aPairwise comparison: incorrect students to correct students (i–c), incorrect students to correct experts (i–e), correct students to correct experts (c–e). NA, no comparison made due to predicted probability of 0 in at least one group. *** p < 0.001; ** p < 0.01; * p < 0.05; ns: p > 0.05. See Supplemental Table S1 for standard error of coefficient estimates. Interpretation example: 82.05% and 92.36% of incorrect and correct student answers, respectively, contained Reason. The GLMM, after accounting for individual variability, predicts the probability of an incorrect student using Reason to be 91.80%, while the probability of a correct student using Reason is 96.68%.
Across All Content Areas, Correct Student Answers Are More Likely Than Incorrect Answers to Contain the Processes of Reason and Eliminate
Students most commonly used the processes Use Information, Reason, and Claim, each present in at least 50% of both correct and incorrect student answers ( Table 4 ). The processes Notice, Recall, Calculate, and Clarify were present in 20–50% of both correct and incorrect student answers ( Table 4 ). In comparing correct and incorrect student answers across all content areas, we found that Integrate, Reason, Eliminate, and Clarify were more likely used in correct compared with incorrect answers ( Table 4 ). As illustrated in Table 2 , the problem-solving processes in Cassie’s correct answer include: reasoning for a claim of dominant inheritance and eliminating when ruling out the possibility of an X-linked mode of inheritance. However, in describing the incorrect answer, Ian fails to document use of either of these processes.
Process Use Varies by Question Content
To determine whether student answers contain different processes depending on the content of the problem, we separated answers, regardless of correctness, by content area. We then excluded some processes: we did not analyze the Error and General codes, as well as Claim, which was seen in virtually every answer across content areas. We also excluded the very rarely seen processes of Identify Similarity and Identify Concept, which were present in 5% or fewer of both incorrect and correct student answers. For the remaining 11 processes, we found that each content area elicited different frequencies of use, as shown in Table 5 and Supplemental Table S2. Some processes were nearly absent in a content area: Calculate was rarely seen in answers to Nondisjunction and Gel/Pedigree questions and Eliminate was rarely seen in answers to Probability and Recombination questions. Furthermore, in answering Probability questions, students were more likely to use the processes Plan and Use Information than in any other content area. Recall was most likely in Recombination and least likely in Gel/Pedigree. Examples of student answers showing some of these trends are shown in Table 3 .
aAll student answers (correct and incorrect) are reported. Processes excluded from analyses include Claim, those within the Error and General strategies, processes that were present in 5% or fewer of both incorrect and correct student answers. Pairwise comparisons between: Probability (P), Recombination (R), Nondisjunction (N), and Gel/Pedigree (G). NA: no comparison made due to prevalence of 0% in at least one group. *** p < 0.001; ** p < 0.01; * p < 0.05; ns: p > 0.05. See Supplemental Table S2 for standard errors of coefficient estimates. Interpretation example: In Probability questions, 94.43% of answers contain Reason, while in Nondisjunction, 84.69% of answers contain Reason. Based on GLMM estimates to account for individual variability in process use, a question in the Probability content area had a 97.52% chance of using Reason, and a question in the Nondisjunction content area had an 92.88% chance of using this process.
The Combination of Processes Linked to Correctness Differs by Content Area
Performance varied by content area. Students performed best on Nondisjunction problems (75% correct), followed by Gel/Pedigree (73%), Probability (54%), and then Recombination (45%). Table 6 shows the raw data of process prevalence for correct and incorrect student answers in each of the four content areas. To examine the combination of problem-solving processes associated with correct student answers for each content area, we used a representative GLMM model with a lasso penalty. This type of analysis measures the predictive value of a process on answer correctness, returning a coefficient value. The presence of a factor with a higher positive coefficient increases the probability of answering correctly more than a factor with a lower positive coefficient. With each additional positive factor in the model, the likelihood of answering correctly increases in an additive manner ( Table 7 and Supplemental Table S3). To interpret these values, we show the probability estimates (%) for each process, which represent the probability that an answer will be correct in the presence of one or more processes ( Table 7 ). The strength of association of the process with correctness, measured by positive coefficient size, is listed in descending order. Thus, for each content area, the process with the strongest positive association to a correct answer is listed first. A process with a negative coefficient (a negative association with correctness) is listed last, and models with negative associations are highlighted in gray in Table 7 . An example of how to interpret the GLMM model is as follows. For the content area of Probability, Calculate (strongest association with correctness), Use Information, and Reason (weakest association with correctness) in combination are positively associated with correctness; Draw is the only negative predictor of correctness. For this content area, the intercept indicates a 7.31% likelihood of answering correctly in the absence of any of the processes tested. If an answer contains Calculate only, there is a 40.19% chance the answer will be correct. If an answer contains both Calculate and Use Information, there is a 58.60% chance the answer will be correct, and if the answer contains the three processes of Calculate, Use Information, and Reason combined, there is a 67.56% chance the answer will be correct. If Draw is present in addition to these three processes, the chance the answer will be correct slightly decreases to 66.40%. For Recombination, the processes of Calculate, Recall, Use Information, Reason, and Plan in combination are associated with correctness, and Draw and Assess Difficulty are negatively associated with correctness. For Nondisjunction, the processes of Eliminate, Draw, and Reason in combination are associated with correctness. For Gel/Pedigree, only the process of Reason was associated with correctness. The examples of correct student answers for each content area, as shown in Tables 2 and 3 , were selected to include each of the positively associated processes described.
aAll student answers (correct and incorrect) are reported. Processes excluded from analyses include Claim, those within the Error and General strategies, processes that were present in 5% or fewer of both correct and incorrect student answers.
aBased on a representative GLMM model with a lasso penalty predicting answer correctness with a moderate penalty parameter (lambda = 25). The intercept represents the likelihood of a correct answer in the absence of all processes initially entered into the model: Notice, Plan, Recall, Check, Assess Difficulty, Use Information, Integrate, Draw, Calculate, Reasoning, Eliminate. Shaded rows indicate the inclusion of negative predictors in combination with positive predictors. Probabilities were calculated using the inverse logit of the sum of the combination of log odds coefficient estimates and the intercept from Supplemental Table S3.
To identify why drawing may be detrimental for Probability and Recombination problems, we further characterized how students described their process of Draw in incorrect answers from these two content areas. We identified two categories: Inaccurate drawing and Inappropriate drawing application. Table 8 provides descriptions and student examples for each category. For Probability problems, 49% of the incorrect student answers that used Draw were Inaccurate, as they identified incorrect genotypes or probabilities while drawing a Punnett square. Thirty-one percent of the answers contained Inappropriate drawing applications such as drawing a Punnett square for each generation of a multiple-generation pedigree rather than multiplying probabilities. Five percent of the answers displayed both Inaccurate and Inappropriate drawing ( Figure 2 ). For Recombination, 83% of incorrect student answers using Draw used an Inappropriate drawing application, typically treating linked genes as if they were unlinked by drawing a Punnett square to calculate probability. Ten percent of answers used both Inappropriate and Inaccurate drawing ( Figure 2 ).
FIGURE 2. Drawing is commonly inaccurate or inappropriate in incorrect student answers for Probability and Recombination. Drawing categorization from student answers that used Draw and answered incorrectly for content areas of (A) Probability ( n = 55) and (B) Recombination ( n = 71). Each category is mutually exclusive, so those that have both Inaccurate drawing/Inappropriate drawing are not in the individual use categories. “No drawing error” indicates neither inaccurate nor inappropriate drawings were described. “Cannot determine” indicates not enough information was provided in the students’ written answer to assign a drawing use category.
In this study, we identified and characterized the various processes that a large sample of students and experts used to document their answers to complex genetics problems. Overall, although their frequency of use differed, experts and students used the same set of problem-solving strategies. Experts were more likely to use orienting and metacognitive strategies than students, confirming prior findings on expert–novice differences (e.g., Chi et al. , 1981 ; Smith and Good, 1984 ; Smith, 1988 ; Atman et al. , 2007 ; Smith et al. , 2013 ; McDonnell and Mullally, 2016 ; Peffer and Ramezani, 2019 ). For students, we also identified which strategies were most associated with correct answers. The use of reasoning was consistently associated with correct answers across all content areas combined as well as for each individual content area. Students used other processes more or less frequently depending on the content of the question, and the combination of processes associated with correct answers also varied by content area.
Domain-Specific Problem Solving
We found that most processes students used (i.e., all but those in the General category) were domain specific, relating directly to genetics content. Prevost and Lemons (2016) , who examined students’ process of solving multiple-choice biology problems, found that domain-general processes were more common in answers to lower-order than higher-order questions. They also found that using more domain-specific processes was associated with correctness. In our study, students solved only higher-order problems that asked them to apply or analyze information. Students also had to construct their responses to each problem, rather than selecting from multiple predetermined answer options. These two factors may explain the prevalence of domain-specific processes in the current study, which allowed us to investigate further the types of domain-specific processes that lead to correct answers.
Metacognitive Activity: Orienting and Metacognitive Processes Are Described by Experts but Not Consistently by Students
Our results support several previous findings from the literature comparing the problem-solving tactics of experts and students: experts are more likely to describe orienting and metacognitive problem-solving strategies than students, including planning solutions, checking work, and identifying the concept of the problem.
While some students used planning in their correct answers, experts solving the same problems were more likely to do so. Prior studies of solutions to complex problems in both engineering and science contexts found that experts more often used the orienting/planning behavior of gathering appropriate information compared with novices ( Atman et al. , 2007 ; Peffer and Ramezani, 2019 ). Experts likely have engaged in authentic scientific investigations of their own, and planning is more likely when the problem to be solved is more complex (e.g., Atman et al. , 2007 ), so experts are likely more familiar with and see value in planning ahead before pursuing a certain problem-solving approach.
Experts were much more likely than students to describe their use of checking work, as also shown in previous work ( Smith and Good, 1984 ; Smith, 1988 ; McDonnell and Mullally, 2016 ). McDonnell and Mullally (2016) found greater levels of unprompted checking after students experienced modeling of explicitly checking prompts and were given points for demonstrating checking. These researchers also noted that when students reviewed their work, they usually only checked some problem components, not all. Incomplete checking was associated with incorrect answers, while complete checking was associated with correct answers. In the current study, we did not assess the completeness of checking, and therefore may have missed an opportunity to correlate checking with correctness. However, if most students were generally checking their answers in a superficial way (i.e., only checking one step in the problem-solving process versus checking all steps), this could explain why there were no differences in the presence of checking between incorrect and correct student answers. In contrast to our study, Prevost and Lemons (2016) found checking was the most common domain-specific procedure used by students when answering both lower- and higher-order multiple-choice biology questions. The multiple-choice format may prompt checking, as the answers have already been provided in the scenario. In addition, while that study assessed answers to graded exam questions, we examined answers to extra-credit assignments. Thus, a lack of motivation may have influenced whether the students in the current study reported checking their answers.
Identifying the Concept of a Problem.
Although this strategy was relatively uncommon even among experts, they were more likely than students to describe identifying the concept of a problem in their solutions. This is consistent with previous research showing that nonexperts use superficial features to solve problems ( Chi et al. , 1981 ; Smith and Good, 1984 ; Smith et al. , 2013 ), a tactic also associated with incorrect solutions ( Smith and Good, 1984 ). The process of identifying relevant core concepts in a problem allows experts to identify the appropriate strategies and knowledge needed for any given problem ( Chi et al. , 1981 ). Thus, we suggest that providing students with opportunities to recognize the core concepts of different problems, and thus the similarity of their solutions, could be beneficial for learning successful problem solving.
Engaging in Explanations: Using Reasoning Is Consistently Associated with Correct Answers
Our findings suggest that, although reasoning is frequently used by both correct and incorrect students, it is strongly associated with correct student answers across all content areas. Correct answers were more likely than incorrect answers to use reasoning; furthermore, reasoning was associated with a correct answer for each of the four content areas we explored. This supports previous work showing that reasoning ability in general is associated with overall biology performance ( Cavallo, 1996 ; Johnson and Lawson, 1998 ). Students who use reasoning may be demonstrating their ability to think logically and sequentially connect ideas, essentially building an argument for why their answers make sense. In fact, teaching the skill of argumentation helps students learn to use evidence to provide a reason for a claim, as well as to rebut others’ claims ( Toulmin, 1958 ; Osborne, 2010 ), and can improve their performance on genetics concepts ( Zohar and Nemet, 2002 ). Thus, the genetics students in the current study who were able to explain the rationale behind each of their problem-solving steps are likely to have built a conceptual understanding of the topic that allowed them to construct logical rationales for their answers.
In the future, think-aloud interviews should be used to more closely examine the types of reasoning students use. Students may be more motivated and better able to explain their rationales verbally, or with a combination of drawn and verbal descriptions, than they are inclined to do when typing their answers in a writing-only situation. Interviewers can also ask follow-up questions, confirming student explanations and ideas, something that cannot be obtained from written explanations. In addition, the problems used in this study were near-transfer problems, similar to those that students previously solved during class. Such problems can often be solved using an algorithmic approach, as also recently described by Frey et al. (2020) in chemistry. Future studies could identify whether and when students use more complex approaches such as causal reasoning (providing connections between ideas) or mechanistic reasoning (explaining the biological mechanism as part of making causal connections ( Russ et al. , 2008 ; Southard et al. , 2016 ) in addition to or instead of algorithmic reasoning.
Students Use Different Processes to Answer Questions in Different Content Areas
Overall, students answered 60% of the questions correctly. Some content areas were more challenging than others: Recombination was the most difficult, followed by Probability, then Gel/Pedigree and Nondisjunction (see also Avena and Knight, 2019 ). While our results do not indicate that a certain combination of processes are both necessary and sufficient to solve a problem correctly, they can be useful to instructors wishing to guide students in their strategy use when considering their solutions to certain types of problems. In the following section, we discuss the processes that were specifically associated with correctness in student answers for each content area.
Probability.
Solving a Probability question requires calculation, while many other types of problems do not. To solve the questions in this study, students needed to consider multiple generations from two families to calculate the likelihood of independent events occurring by using the product rule. Smith (1988) found that both successful and unsuccessful students often find this challenging. Our previous work also found that failing to use the product rule, or using it incorrectly, was the second most common error in incorrect student answers ( Avena and Knight, 2019 ). Correctly solving probability problems likely also requires a conceptual understanding of the reasoning behind each calculation (e.g., Deane et al. , 2016 ). This type of reasoning, specific to the mathematical components of a problem, is referred to as statistical reasoning, a suggested competency for biology students ( American Association for the Advancement of Science, 2011 ). The code of Reason includes reasoning about other aspects of the problem (e.g., determining genotypes; see Table 3 ) in addition to reasoning related to calculations. While reasoning was prevalent in both incorrect and correct answers to Probability problems, using reasoning still provided an additional 9% likelihood of answering correctly for students who had also used calculating and applying information in their answers.
Generally, calculation alone was not sufficient to answer a Probability question correctly. When students applied information to solving the specific problem (captured with the Use Information code), such as determining genotypes within the pedigree or assigning a probability, their likelihood of generating a correct answer was 40%. This only increased to 59% if they also used Calculate (see Table 7 ). We previously found that the most common content error in these types of probability problems was mis-assigning a genotype or probability due to incorrectly using information in the pedigree; this error was commonly seen in combination with a product rule error ( Avena and Knight, 2019 ). This correlates with our current findings on the importance of applying procedural knowledge: both Use Information and Calculate, under the AtL element of generating knowledge, contribute to correct problem-solving.
Recombination.
Both the Probability and Recombination questions are fundamentally about calculating probabilities; thus, not surprisingly, Calculate is also associated with correct answers to Recombination questions. Determining map units and determining the frequency of one possible genotype among possible gametes both require calculation. Use of Recall in addition to Calculate increases the likelihood of answering correctly from 18 to 39%. This may be due to the complexity of some of the terms in these problems. As shown previously, incorrect answers to Recombination questions often fail to use map units in their solution ( Avena and Knight, 2019 ). Appropriately using map units thus likely requires remembering that the map unit designation is representative of the probability of recombination and then applying this definition to the problem. When students Used Information, along with Calculate and Recall, their likelihood of answering correctly increased to 63%.
Reasoning and planning also contribute to correct answers in this content area. In their solutions, students needed to consider the genotypes of the offspring and both parents to solve the problem. The multistep nature of the problem may give students the opportunity to plan their possible approaches, either at the very beginning of the problem and/or as they walk through these steps. This was seen in Preston’s solution ( Table 3 ), in which the student sequentially made a short-term plan and then immediately used information in the problem to carry out that plan.
Drawing: A Potentially Misused Strategy in Probability and Recombination Solutions.
Only one process, Drawing, was negatively associated with correct answers in solutions to both Probability and Recombination questions. Drawing is generally considered beneficial in problem solving across disciplines, as it allows students to generate a representation of the problem space and/or of their thinking (e.g., Mason and Singh, 2010 ; Quillin and Thomas, 2015 ; Heideman et al. , 2017 ). However, when students generate inaccurate drawings or use a drawing methodology inappropriately, they are unlikely to reach a correct answer. In a study examining complex meiosis questions, Kindfield (1993) found that students with more incorrect responses provided drawings with features not necessary to solving the problem. In our current study, we found that the helpfulness of a drawing depends on its quality and the appropriateness or context of its use.
When answering Recombination problems, many students described drawing a Punnett square and then calculating the inheritance as if the linked genes were actually genes on separate chromosomes. In doing so, students revealed a misunderstanding of when and why to appropriately use a Punnett square as well as their lack of understanding that the frequency of recombination is connected to the frequency of gametes. Because we have also shown that planning is beneficial in solving Recombination problems, we suggest that instructors emphasize that students first plan to look for certain characteristics in a problem, such as linked versus unlinked genes, to identify how to proceed. For example, noting that genes are linked would suggest not using a Punnett square when solving the problem. Similarly, in Probability questions, students must realize that uncertainty in genotypes over multiple generations of a family can be resolved by multiplying probabilities together rather than by making multiple possible Punnett squares for the outcome of a single individual. These findings connect to the AtL elements of generative thinking and taking a deep approach: drawing can be a generative behavior, but students must also be thinking about the underlying context of the problem rather than a memorized fact.
Nondisjunction.
In Nondisjunction problems, students were asked to predict the cause of an error in chromosome number. Our model for processes associated with correctness in nondisjunction problems ( Table 7 ) suggested that the likelihood of answering correctly in the absence of several processes was 70%. This may explain the higher percent of correct answers in this content area (75%) compared with other content areas. Nonetheless, three processes were shown to help students answer correctly. The process Eliminate, even though used relatively infrequently (10%), provides a benefit. Using elimination when there are a finite number of obvious solutions is a reasonable strategy, and one previously shown to be successful ( Smith and Good, 1984 ). Ideally, this strategy would be coupled with drawing the steps of meiosis and then reasoning about which separation errors could not explain the answer. Drawing was associated with correct answers in this content area, though it was neither required nor sufficient. Instead of drawing, some students may have used a memorized series of steps in their solutions. This is referred to as an “algorithmic” explanation, in which a memorized pattern is used to solve the problem. For example, such a line of explanation may go as follows: “beginning from a diploid cell heterozygous for a certain gene, two of the same alleles being present in one gamete indicates a nondisjunction in meiosis II.” Such algorithms can be applied without a conceptual understanding ( Jonsson et al. , 2014 ; Nyachwaya et al. , 2014 ), and thus students may inaccurately apply them without fully understanding or being able to visualize what is occurring during a nondisjunction event ( Smith and Good, 1984 ; Nyachwaya et al. , 2014 ). Using a drawing may help provide a basis for analytic reasoning, providing logical links between ideas and claims that are thoughtful and deliberate ( Alter et al. , 2007 ). Indeed, in Kindfield’s study ( 1993 ), in which participants (experts and students) were asked to complete complex meiosis questions, they found that those with more accurate models of meiosis used their drawings to assist in their reasoning process. Kindfield (1993) suggested that these drawings allowed for additional working memory space, thus supporting an accurate problem-solving process.
Gel/Pedigree.
Unlike other content areas, the only process associated with correctness in the Gel/Pedigree model was Reasoning, which provided a greater contribution to correct solutions than in any other content area. In these problems, students are asked to find the most likely mode of inheritance given both a pedigree of a family and a DNA gel that shows representations of alleles for each family member. The two visuals, along with the text of the problem, provide students an opportunity to provide logical explanations at many points in the problem. Students use reasoning to support intermediate claims as they think through possible solutions, and again for their final claims, or for why they eliminate an option. Almost half of both correct and incorrect student answers to these questions integrated features from both the gel and pedigree to answer the problem. Even though many correct and incorrect answers integrate, correct answers also reason. We suggest that the presence of two visual aids prompts students to integrate information from both, thus potentially increasing the likelihood of using reasoning.
Limitations
In this study, we captured the problem-solving processes of a large sample of students by asking them to write their step-by-step processes as part of an online assignment. In so doing, we may not have captured the entirety of a student’s thought process. For example, students may have felt time pressure to complete an assignment, may have experienced fatigue after answering multiple questions on the same topic, or simply may not have documented everything they were thinking. Students may also have been less likely to indicate they were engaging in drawing, as they were answering questions using an online text platform; exploring drawing in more detail in the future would require interviews or the collection of drawings as a component of the problem-solving assignment. Additionally, students may not have felt that all the steps they engaged in were worth explaining in words; this may be particularly true for metacognitive processes. Students are not likely accustomed to expressing their metacognitive processes or admitting uncertainty or confusion during assessment situations. However, even given these limitations, we have captured some of the primary components of student thinking during problem solving.
In addition, our expert–student comparison may be biased, as experts had different reasons than students for participating in the study. The experts likely did so because they wanted to be helpful and found it interesting. Students, on the other hand, had very different motivations, such as using the problems for practice in order to perform well on the next exam and/or to get extra credit. Although it is likely not possible to put experts and students in the same affective state while they are solving problems, it is worth realizing that the frequencies of processes they use could reflect their different states while answering the questions.
Finally, the questions in the assignments provided to students were similar to those seen previously during in-class work. The low prevalence of metacognitive processes in their solutions could be due to students’ perception that they have already solved similar questions. This may prevent them from articulating their plans or from checking their work. More complex, far-transfer problems would likely elicit different patterns of processes for successful problem solving.
SUGGESTIONS FOR INSTRUCTION
Calculating: In questions regarding probability, students will need to be familiar with mathematical representations and calculations. Practicing probabilistic thinking is critical.
Drawing: Capturing thought processes with a drawing can help visualize the problem space and can be used to generate supportive reasoning for one’s thinking (e.g., a drawing of the stages of meiosis). However, a cautionary note: drawing can lead to unsuccessful problem solving when used in an inappropriate context, such as a Punnett square when considering linked genes or using multiple Punnett squares when other rules should be used, such as multiplication of probabilities from multiple generations.
Eliminating: In questions with clear alternate final answers, eliminating answers, preferably while explaining one’s reasons, is particularly useful.
Practicing metacognition: Although there were few significant differences in metacognitive processes between correct and incorrect student answers, we still suggest that planning and checking are valuable across content areas, as demonstrated by the more frequent use of these processes by experts.
In summary, we suggest that instructors not only emphasize key pieces of challenging content for each given topic, but also consistently demonstrate possible problem-solving strategies, provide many opportunities for students to practice thinking about how to solve problems, and encourage students to explain to themselves and others why each of their steps makes sense.
ACKNOWLEDGMENTS
This work was supported by the National Science Foundation (DUE 1711348). We are grateful to Paula Lemons, Stephanie Gardner, and Laura Novick for their guidance and suggestions on this project. Special thanks also to the many students and experts who shared their thinking while solving genetics problems.
- American Association for the Advancement of Science . ( 2011 ). Vision and change in undergraduate biology education: A call to action . Washington, DC. Google Scholar
- Bassok, M., & Novick, L. R. ( 2012 ). Problem solving . In Holyoak, K. J.Morrison, R. G. (Eds.), Oxford handbook of thinking and reasoning (pp. 413–432). New York, NY: Oxford University Press. Google Scholar
- Biggs, J. B. ( 1987 ). Student approaches to learning and studying. Research monograph . Hawthorn, Australia: Australian Council for Educational Research. Google Scholar
- Bloom, B. S., Krathwohl, D. R., & Masia, B. B. ( 1956 ). Taxonomy of Educational Objectives: The Classification of Educational Goals . New York, NY: David McKay. Google Scholar
- Gelman, A., & Hill, J. ( 2006 ). Data analysis using regression and multilevel/hierarchical models . Cambridge, England: Cambridge University Press. Google Scholar
- Groll, A. ( 2017 ). glmmLasso: Variable selection for generalized linear mixed models by L1-penalized estimation . R package version , 1 (1), 25. Google Scholar
- Kindfield, A. C. H. ( 1993 ). Biology diagrams: Tools to think with . Journal of the Learning Sciences , 3 (1), 1–36. Google Scholar
- Lemke, J. L. ( 1990 ). Talking science: Language, learning, and values . Norwood, NJ: Ablex Publishing. Retrieved July 30, 2020, from http://eric.ed.gov/?id=ED362379 Google Scholar
- McDonnell, L., & Mullally, M. ( 2016 ). Teaching students how to check their work while solving problems in genetics . Journal of College Science Teaching , 46 (1), 68. Google Scholar
- Novick, L. R., & Bassok, M. ( 2005 ). Problem Solving . In Holyoak, K. J.Morrison, R. G. (Eds.), The Cambridge handbook of thinking and reasoning (pp. 321–349). New York. NY: Cambridge University Press. Google Scholar
- Osborne, J. ( 2010 ). Arguing to learn in science: The role of collaborative, critical discourse . Science , 328 , 463–466. Medline , Google Scholar
- Saldana, J. ( 2015 ). The coding manual for qualitative researchers . Los Angeles, CA: Sage. Google Scholar
- Schen, M. ( 2012 , March 25). Assessment of argumentation skills through individual written instruments and lab reports in introductory biology . Paper presented at: Annual Meeting of the National Association for Research in Science Teaching (Indianapolis, IN) . Google Scholar
- Smith, M. K., & Knight, J. K. ( 2012 ). Using the Genetics Concept Assessment to document persistent conceptual difficulties in undergraduate genetics courses . Genetics , 191 , 21–32. Medline , Google Scholar
- Smith, M. K., Wood, W. B., & Knight, J. K. ( 2008 ). The Genetics Concept Assessment: A new concept inventory for gauging student understanding of genetics . CBE—Life Sciences Education , 7 (4), 422–430. Link , Google Scholar
- Toulmin, S. ( 1958 ). The uses of argument . Cambridge: Cambridge University Press. Google Scholar
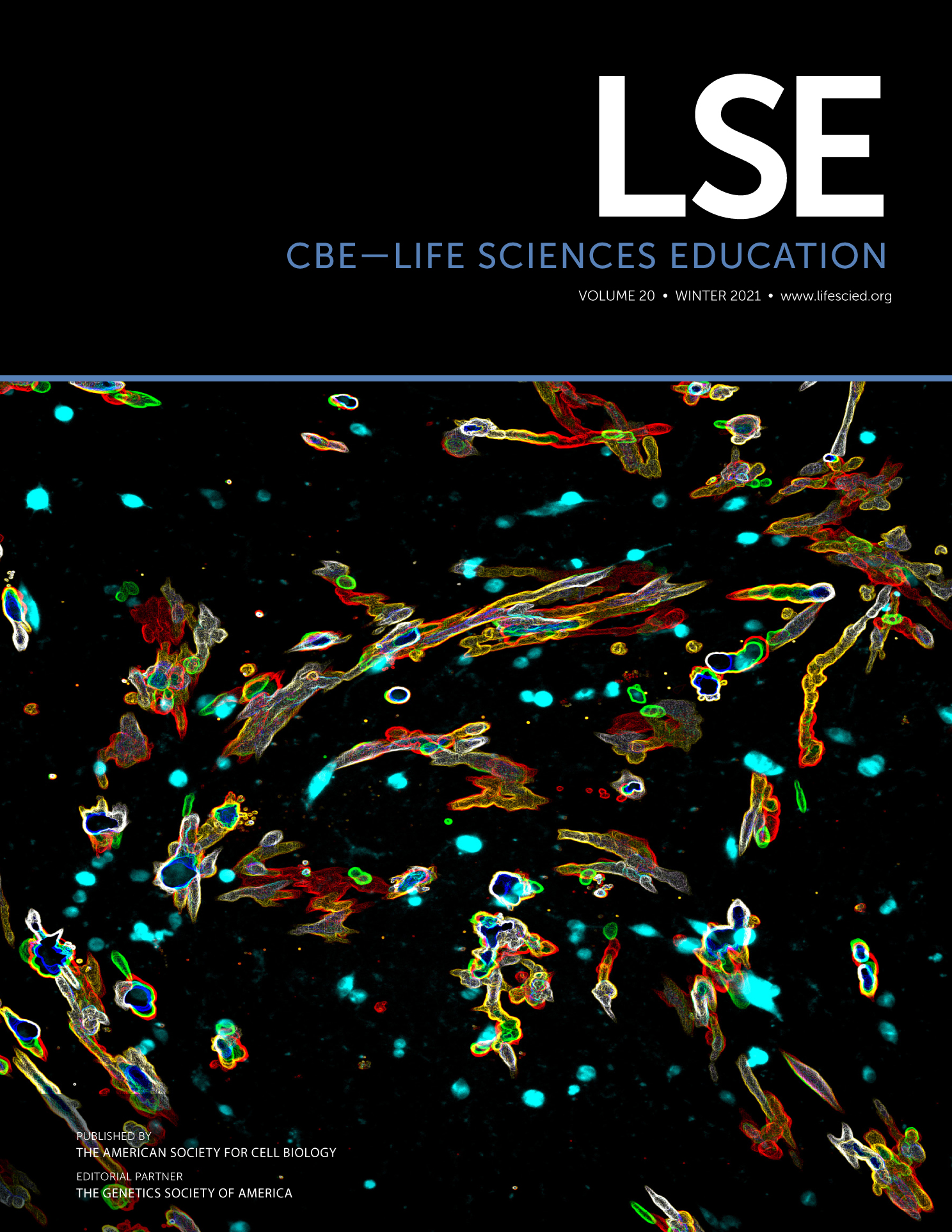
Submitted: 21 January 2021 Revised: 16 July 2021 Accepted: 22 July 2021
© 2021 J. S. Avena et al. CBE—Life Sciences Education © 2021 The American Society for Cell Biology. This article is distributed by The American Society for Cell Biology under license from the author(s). It is available to the public under an Attribution–Noncommercial–Share Alike 3.0 Unported Creative Commons License (http://creativecommons.org/licenses/by-nc-sa/3.0).

AP® Biology
Hardy-weinberg equation: ap® biology crash course.
- The Albert Team
- Last Updated On: March 1, 2022
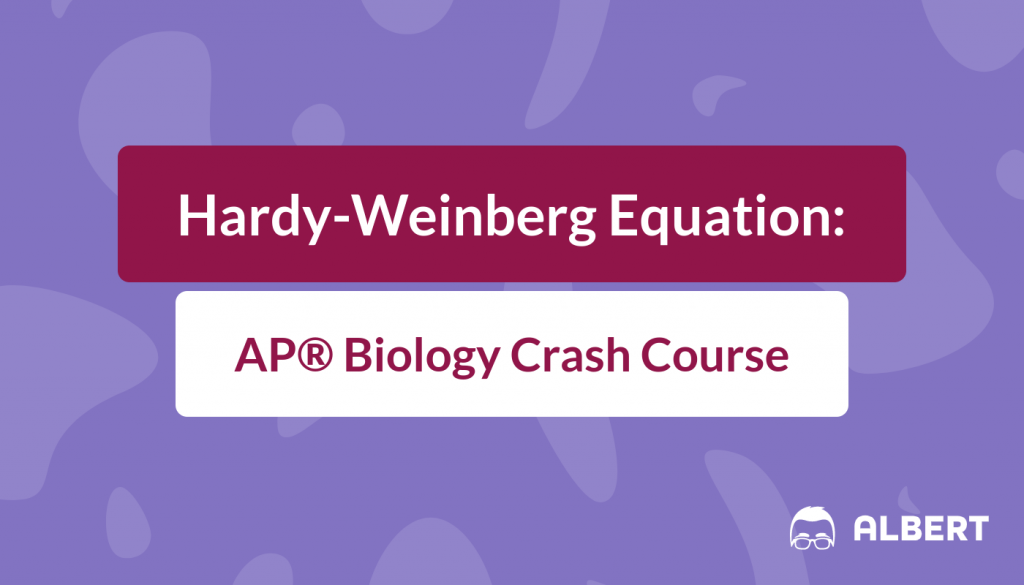
On the AP® Biology exam, you may be asked to predict the frequency of specific genetic traits in a given population. To determine this frequency, we use what is known as the Hardy-Weinberg equation . This equation is a mathematical expression that illustrates the relationship between the frequencies of all genotypes present in the population in question.
Before we get into exactly how the Hardy-Weinberg equation is used to solve genetic word problems on the AP® Biology test, let’s take a look at some of the important elements that play into gene expression and genetic calculations (you’ll be introduced to the actual algebra later on in this crash course).
Phenotypes and Genotypes
A phenotype is a visible, outward-appearing physical characteristic—like freckles, for example. The genotype , one the other hand, is the genetic basis of that physical characteristic. For our freckles example, the specific combination of dominant and recessive alleles that is expressed as either freckled or non-freckled skin would be considered the genotype.
It is important to note here that many human traits are not simply governed by a single gene, and some traits that were previously assumed to be basic single-gene traits (tongue rolling, for example) may even be affected by non-genetic environmental factors. For this crash course, however, we are assuming a simple, single-gene basis for our examples.
In general, there are three kinds of genotypes: homozygous dominant , homozygous recessive , and heterozygous dominant.
A homozygous dominant genotype is one in which both alleles are dominant ( homo = same). Likewise, a homozygous recessive genotype is one in which both alleles are recessive. Finally, a heterozygous dominant genotype is one that contains both a dominant and a recessive gene ( hetero = different). Because a heterozygous dominant genotype includes a dominant gene, it will be expressed as the dominant phenotype in a homozygous dominant individual.
Because freckles are a dominant trait in our example, it would be impossible to tell just from looking whether the freckled person’s genotype is homozygous dominant or heterozygous dominant. Similarly, genetic diseases and disorders can be absent in a phenotype (with the individual presenting as healthy and asymptomatic) but be present in the recessive form in that person’s genotype. In these cases, that person is considered a carrier of the disease.
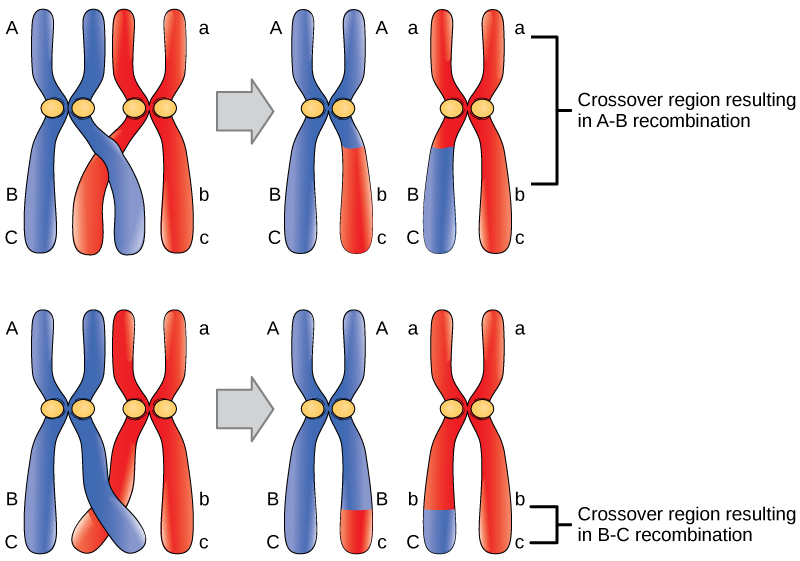
One simple way to mentally visualize how alleles work is to imagine them as socks. In this analogy, let’s say a red sock represents the recessive allele for non-freckled skin and a black sock represents the dominant allele for freckles. A recessive red sock is always tucked into the other sock, regardless of whether it is a dominant black sock or a recessive red sock. If both socks are red, it doesn’t matter which sock is tucked into the other; in either case, the sock showing on the outside will be a recessive red one. If both socks are black, the situation is the same; the sock showing on the outside will always be black. If there is one red sock and one black sock, however, the recessive red sock will be tucked inside the black one, and the sock that shows will be the dominant black sock.
It’s the same with the actual alleles: if both alleles are recessive non-freckle alleles, the result will be a person without freckles. If both alleles are dominant freckle alleles, the result will be a person with freckles. If one allele is dominant and one allele is recessive, the recessive non-freckle allele will be metaphorically “tucked” inside the other, resulting in a person with freckles.
Representing Genotypes in Genetic Problems
Genotypes in genetic problems are represented by a pair of letters with each letter either capitalized or in lowercase. A capital letter represents a dominant allele while a lowercase letter represents a recessive allele.
For our freckles example, let’s use the letter “f.” A capital “F” represents the presence of the dominant allele for freckles, while a lowercase “f” represents the presence of the recessive allele for non-freckled skin. The three possible genotypes, then, would be represented as follows:
- Homozygous dominant = FF
- Homozygous recessive = ff
- Heterozygous dominant = Ff
The Hardy-Weinberg Equation
The Hardy-Weinberg equation states that the frequency at which a specific genotype occurs can be expressed as a ratio of the genotype in question to the total number of alleles in the population.
Algebraically, the equation is expressed as:
The terms of this equation are defined as follows:
- p = the frequency of the dominant allele in a population
- q = the frequency of the recessive allele in a population
- 2pq = the frequency of the heterozygous dominant genotype
- p^2 = the frequency of homozygous dominant genotype
- q^2 = the frequency of homozygous recessive genotype
Because the set of all alleles in the population is made up of one part dominant alleles and one part recessive alleles, the sum of p and q will always equal 1 .
If we have a group of 10 people with 20 total alleles ( 2 alleles per person), and we are told that the frequency of the dominant allele is 6 out of 20 , we can say that p = \frac{6}{20} , or 0.3 .
Since we know that p + q = 1 , we can also say that 0.3 + q = 1 .
Finally, we solve for q by subtracting 0.3 from 1 , leaving 0.7 .
Now, we know that the ratio of dominant alleles is 0.3 , and the ratio of recessive alleles is 0.7 . In other words, 30\% of the alleles in the population are dominant and 70\% are recessive.
Although allele and genotype frequencies can technically be expressed as fractions, decimals, or percentages, you should always represent p and q values as decimals on the AP® Biology exam.
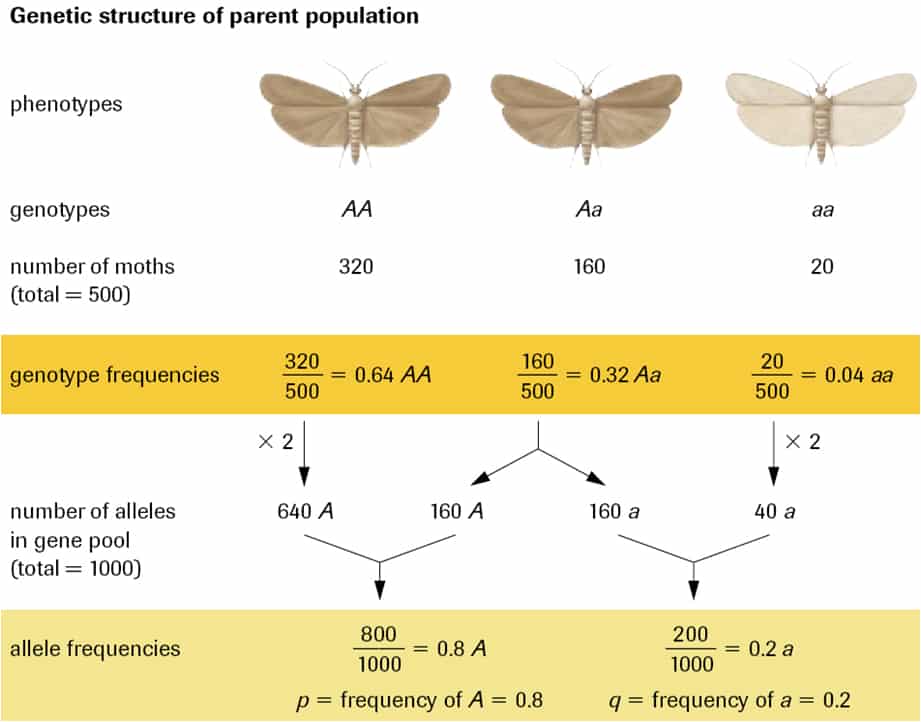
Genotype Practice Problem
Let’s try a full genotype problem in which we use the Hardy-Weinberg equation to calculate the frequency of a specific genotype. The equation may look intimidating, but don’t get too nervous about your algebra skills—the math involved is much simpler than it might seem at first glance.
Let’s say that 60\% of humans do not have freckles. Because freckles are a dominant trait, this means that only those with the homozygous recessive genotype will not have them. Therefore, our homozygous recessive frequency—or q^2 —is 0.6 . How can we determine the frequency of the heterozygous dominant genotype in the human population?
First, we start with the basic Hardy-Weinberg equation, adding in our known q^2 value of 0.6 .
Next, we determine the value of q by taking the square root of q^2 .
Using the knowledge that p + q = 1 , we can then subtract to solve for p .
Recall that we are solving for the frequency of the heterozygous dominant genotype, which is represented by the term 2pq in the Hardy-Weinberg equation. Since we already know the values of p and q , we can now calculate the frequency of this genotype.
At the conclusion of all our number-crunching, we find that the frequency of the heterozygous dominant genotype for freckles in this population is 0.35 , or 35\% . Again, remember that for the AP® Biology exam, this frequency should be written as the decimal 0.35 .
Necessary Assumptions
It is important to note that the Hardy-Weinberg equation operates under the following assumptions:
- The population contains only diploid organisms that reproduce sexually.
- Generations do not overlap and mating occurs randomly.
- The population size is infinitely large.
- Allele frequencies are roughly equal between the sexes.
- There is no mutation, migration, or selection occurring in the population.
Because populations in reality cannot meet all of these assumptions, the Hardy-Weinberg equation won’t necessarily provide the true frequencies of genotypes in real-world applications. Instead, the equation simply predicts theoretical genotype frequencies for single-gene traits.
What have we learned?
- A phenotype is a visible physical trait, while a genotype is a non-visible genetic code.
- There are three kinds of genotypes: homozygous dominant , homozygous recessive , and heterozygous dominant .
- The Hardy-Weinberg equation is p^2 + 2pq + q^2 =1 and is used to determine the frequency of genotypes in a given population.
- To solve for the frequency of a specific genotype, start by replacing the appropriate terms with the known values you’ve been given. From there, it’s Algebra 101.
So long as you are given sufficient information to determine at least one of the terms of the Hardy-Weinberg equation, you should now be prepared to predict the frequencies of all three genotypes as well as those of the dominant and recessive alleles. Try coming up with your own hypothetical genotype problems for even more AP® Biology practice !
Need help preparing for your AP® Biology exam?
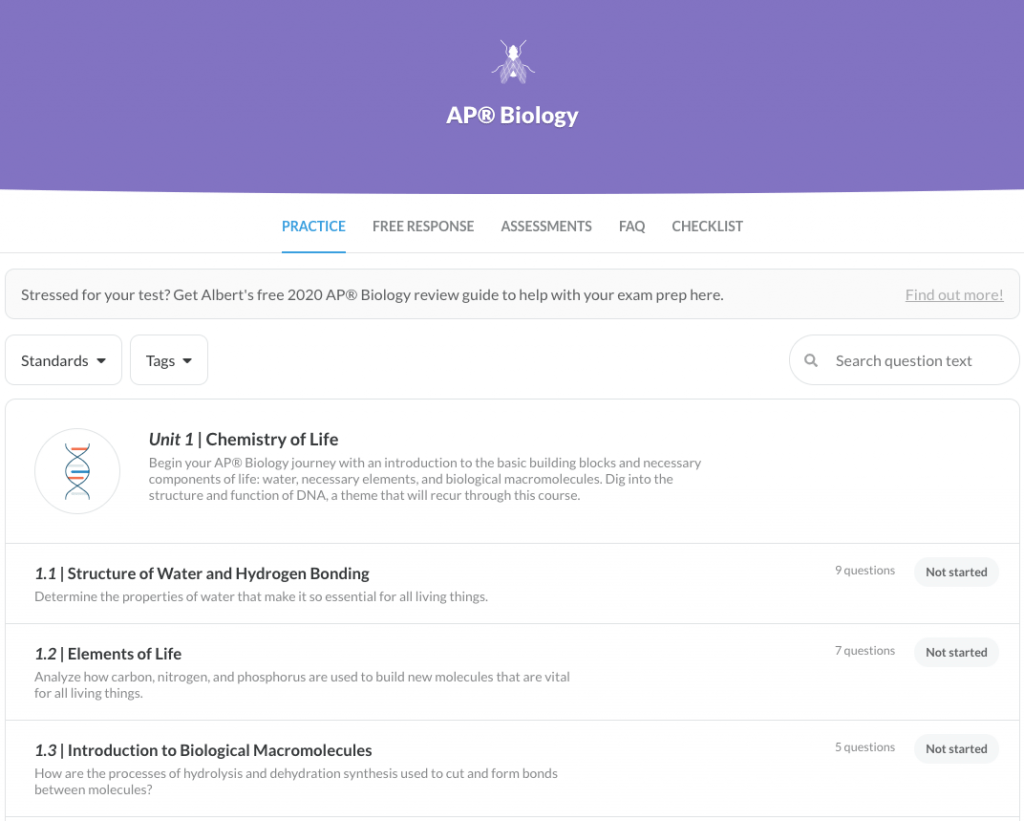
Albert has hundreds of AP® Biology practice questions, free response, and full-length practice tests to try out.
Interested in a school license?
Popular posts.

AP® Score Calculators
Simulate how different MCQ and FRQ scores translate into AP® scores

AP® Review Guides
The ultimate review guides for AP® subjects to help you plan and structure your prep.

Core Subject Review Guides
Review the most important topics in Physics and Algebra 1 .
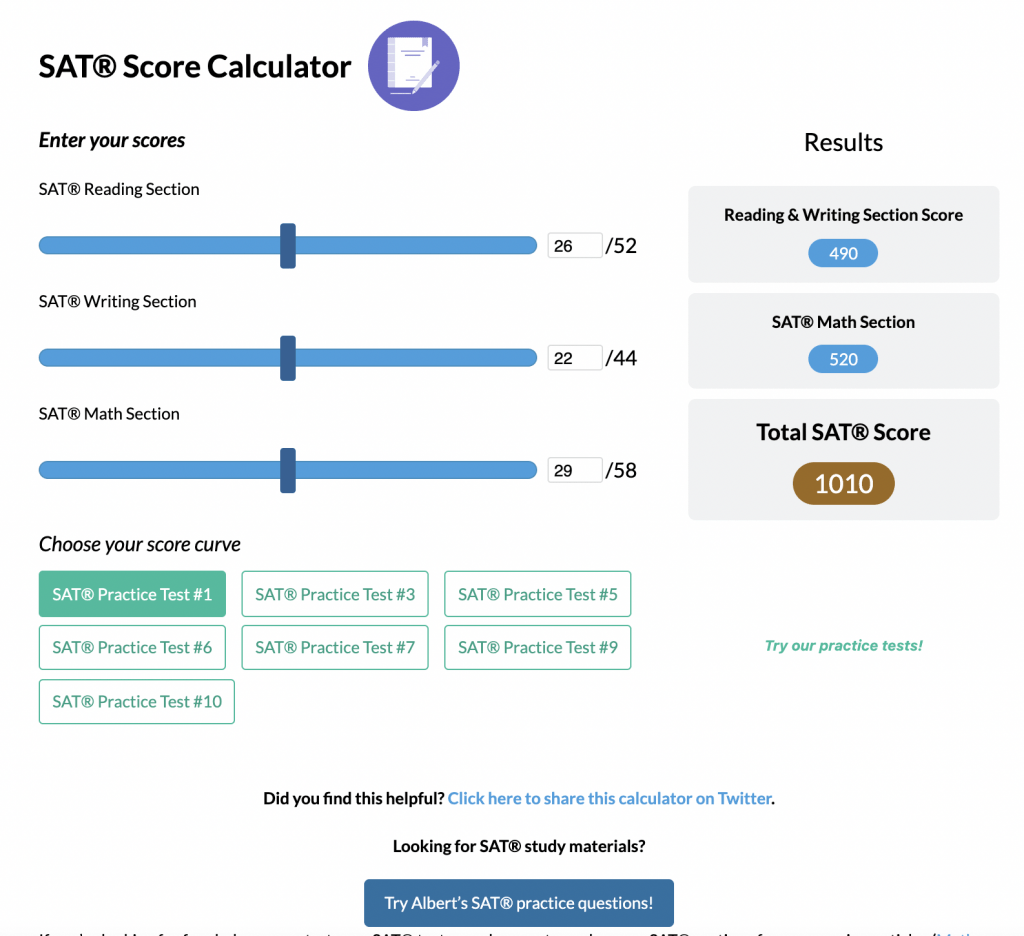
SAT® Score Calculator
See how scores on each section impacts your overall SAT® score

ACT® Score Calculator
See how scores on each section impacts your overall ACT® score
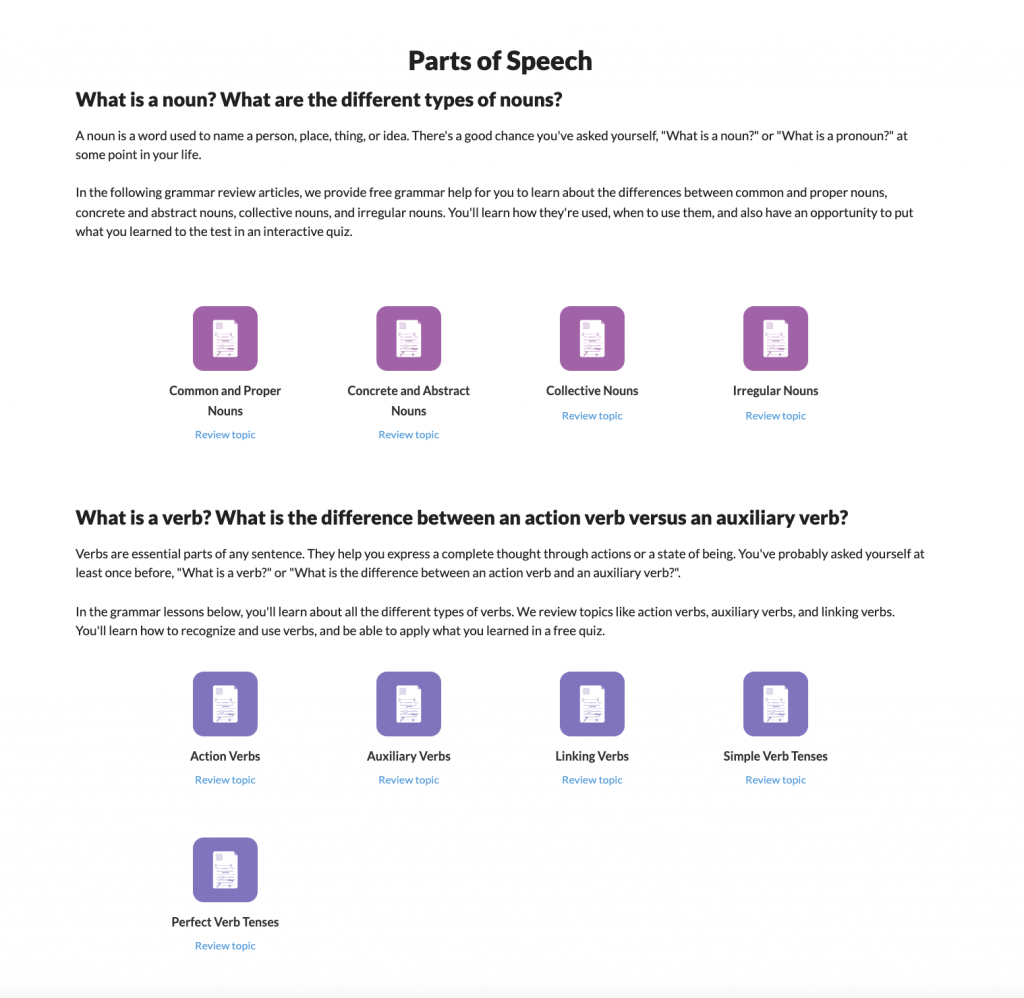
Grammar Review Hub
Comprehensive review of grammar skills

AP® Posters
Download updated posters summarizing the main topics and structure for each AP® exam.

A .gov website belongs to an official government organization in the United States.
A lock ( ) or https:// means you've safely connected to the .gov website. Share sensitive information only on official, secure websites.
- Genetics Basics
- Family Health History
- About Cascade Testing
Genetic Disorders
What to know.
Genetic disorders are health problems that happen because of some type of abnormality in a person's genetic material. There are several types of genetic disorders. Some disorders are caused by a genetic change (mutation) in a single gene; some are caused by an abnormality in one of the chromosomes; and some are complex, involving numerous genes and influences from environmental factors.
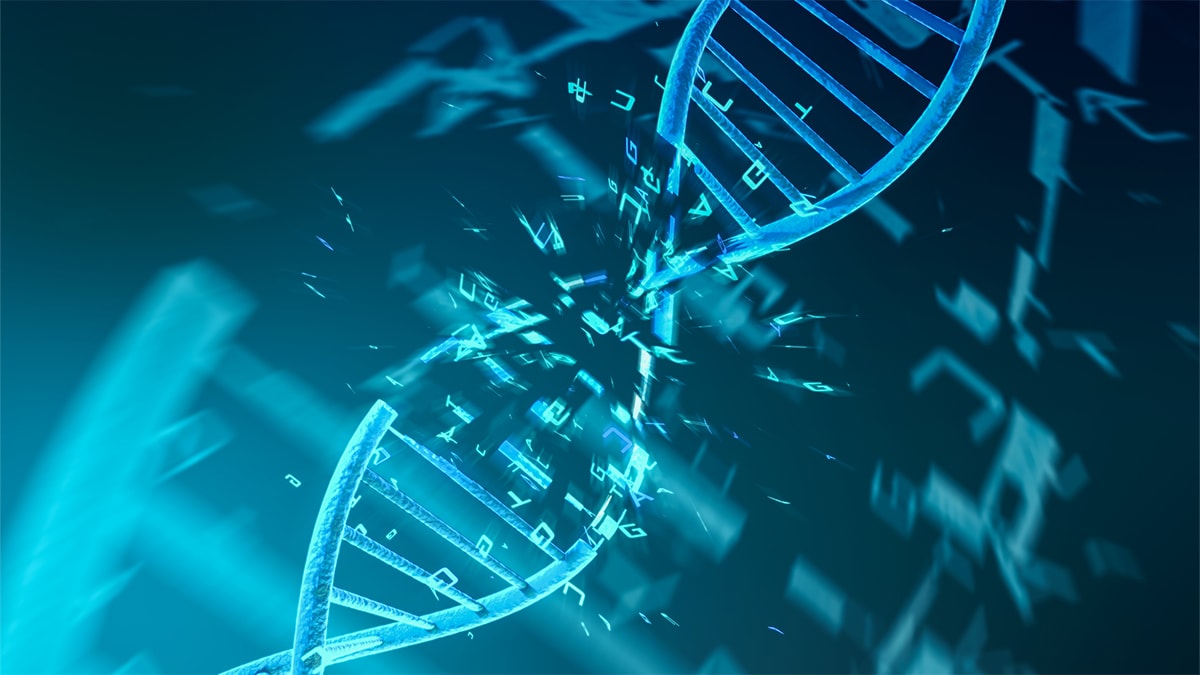
Genetic disorders are health problems that happen because of some type of abnormality in a person's genetic material. There are several types of genetic disorders. In some cases, a genetic change in a single gene can cause someone to have a disease or condition. In other cases, the gene does not have a genetic change, but a person has more or fewer copies of the gene than most people, and this causes a disease or condition. Some diseases or conditions occur when a person does not have the same number of chromosomes as most people or has part of a chromosome that is missing, extra, or not in the right place.
Most genetic disorders happen due to the combination of many genetic changes acting together with a person's behaviors and environment. These are sometimes called complex conditions.
A detailed description of the basic concepts of genetics can be found here .
Single gene disorders
DNA contains the instructions for making your body work. DNA is made up of two strands that wind around each other. Each DNA strand includes chemicals called nitrogen bases—T (thymine), A (adenine), C (cytosine), and G (guanine)—that make up the DNA code. Genes are specific sections of DNA that have instructions for making proteins. Proteins make up most of the parts of your body and make your body work the right way.
Some diseases and conditions happen when a person has a genetic change (sometimes called a mutation) in one of their genes. These types of diseases are called single gene disorders. Sometimes, what happens is that one of the DNA bases is changed. For example, part of a gene that usually has the sequence TAC is changed to the sequence TTC. This can change the way the gene works, for example, by changing the protein that is made. In other cases, one or more of the bases in the DNA sequence are missing altogether, or there are extra bases.
Genetic changes can be passed down to a child from their parents. When this happens, the disease or condition is called hereditary or inherited. Or the changes can happen for the first time in the process of making the sperm or egg or early in development, so the child will have the genetic change but the parents will not.
DNA, genes, and chromosomes
Single gene disorders that affect a gene on one of the 22 autosomal chromosome pairs are called autosomal disorders. Disorders that affect the sex chromosome are called X-linked disorders. Disorders are further described according to whether the affected genetic change is dominant or recessive.
For some diseases and conditions, everyone who inherits the genetic change will have the disease or condition, but how serious it is can vary from person to person. In other cases, people who have the genetic change will be more likely to develop the disease or condition, but some of them will never develop it.
Autosomal dominant
With autosomal dominant diseases or conditions, a person only needs a genetic change in one copy of the gene to have the disease. If one parent has an autosomal dominant disease or condition, each child has a 50% (1 in 2) chance of inheriting the genetic change that causes the condition.
Examples of autosomal dominant conditions include hereditary breast and ovarian cancer caused by genetic changes (mutations) to the BRCA1 and BRCA2 genes ; Lynch syndrome ; and familial hypercholesterolemia .
Autosomal recessive
With autosomal recessive diseases or conditions, a person needs a genetic change in both copies of the gene to have the disease or condition. While a person with a genetic change in only one copy of the gene will not have the disease or condition, they can still pass the genetic change down to their children. These parents are sometimes called "carriers" of the disease because they "carry" the genetic change that causes the disease or condition but do not have the disease themselves.
A parent who is a carrier of a disease has a 50% (1 in 2) chance of passing the gene with the genetic change on to each of their children. If both parents are carriers of the disease, each child has a 25% (1 in 4) chance of inheriting two genes with the genetic change and thus of having the disease. Carrier screening looks for autosomal recessive genetic changes in parents to see if they could have a child with the disease or condition.
Examples of autosomal recessive disorders are sickle cell disease and cystic fibrosis .
Females have two X chromosomes, and males have one X chromosome and one Y chromosome. Each daughter gets an X from her mother and an X from her father. Each son gets an X from his mother and a Y from his father.
Some diseases or conditions happen when a gene on the X chromosome has a genetic change. Because males only have one copy of all the genes on the X chromosome, they are much more likely to be affected by X-linked genetic disorders than females. A female with a genetic change on only one of her two X chromosomes may not have the disease or condition at all. However, in some cases, females with the genetic change on one of their X chromosomes can have the disease or condition, but it is often a milder form of the disease than usually occurs in males.
Because males inherit an X chromosome from their mother, a female with a genetic change on one copy of the gene has a 50% (1 in 2) chance of passing the genetic change on to each of her sons. Her sons could have the disease or condition even though she does not.
Examples of X-linked conditions include fragile X syndrome , Duchenne muscular dystrophy , and hereditary hemophilia .
Chromosomal abnormalities
Different number of chromosomes.
People usually have 23 pairs of chromosomes. But sometimes a person is born with a different number. Having an extra chromosome is called trisomy. Missing a chromosome is called monosomy.
For example, people with Down syndrome have an extra copy of chromosome 21. This extra copy changes the body's and brain's normal development and causes intellectual and physical problems for the person. Some disorders are caused by having a different number of sex chromosomes. For example, people with Turner syndrome usually have only one sex chromosome, an X. Women with Turner syndrome can have problems with growth and heart defects.
Changes in chromosomes
Sometimes chromosomes are incomplete or shaped differently than usual. Missing a small part of a chromosome is called a deletion. A translocation is when part of one chromosome has moved to another chromosome. An inversion is when part of a chromosome has been flipped over.
For example, people with Williams syndrome are missing a small part of chromosome 7. This deletion can result in intellectual disability and a distinctive facial appearance and personality.

Complex conditions
Complex disorders are caused by genetic changes in many different genes working together with environmental factors. Environmental factors include exposures and behaviors such as air pollution, smoking, alcohol use, the amount of exercise a person gets, or the foods they eat. Having a family health history of a complex condition can make you more likely to have that condition yourself. However, genetic testing would not be recommended because there is not a single genetic change causing the condition that could be found by genetic testing.
Most chronic diseases, such as most cases of heart disease , cancer , diabetes , osteoporosis , and asthma , are complex disorders. So are most cases of developmental disabilities, such as autism spectrum disorder and attention deficit / hyperactivity disorder (ADHD) , and mental health conditions, such as depression and schizophrenia .
The vast amount of genetic information available has allowed researchers to develop methods to study which types of genetic changes are found more often in people with a given disease or condition. This allows researchers to estimate a person's risk for a particular disorder based on which genetic changes they have. This estimate is known as the polygenic risk score.
Some important issues need to be considered before polygenic risk scores can be routinely used in health care and public health. Studies are looking at how useful polygenic risk scores are in real-life clinical practice. Information on how each gene change affects disease risk comes from population-level genetic studies. Addressing diversity in development of polygenic risk scores is important, because polygenic risk scores developed from studies in one population (for example, people of Northern European ancestry) might not work as well for other populations (for example, people of West African ancestry). Also, how each gene change affects the polygenic risk score varies from study to study.
Once polygenic risk scores are ready to be used routinely in clinical practice, public health efforts will be needed to address issues such as access, insurance coverage, and sharing of results across health systems.
Genomics and Your Health
Learn more about genomics and its importance for your health
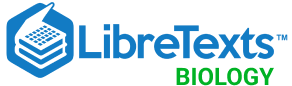
- school Campus Bookshelves
- menu_book Bookshelves
- perm_media Learning Objects
- login Login
- how_to_reg Request Instructor Account
- hub Instructor Commons
Margin Size
- Download Page (PDF)
- Download Full Book (PDF)
- Periodic Table
- Physics Constants
- Scientific Calculator
- Reference & Cite
- Tools expand_more
- Readability
selected template will load here
This action is not available.
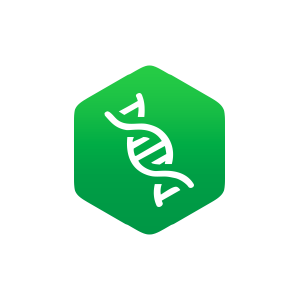
1.7: Probabilities in genetics
- Last updated
- Save as PDF
- Page ID 73824
\( \newcommand{\vecs}[1]{\overset { \scriptstyle \rightharpoonup} {\mathbf{#1}} } \)
\( \newcommand{\vecd}[1]{\overset{-\!-\!\rightharpoonup}{\vphantom{a}\smash {#1}}} \)
\( \newcommand{\id}{\mathrm{id}}\) \( \newcommand{\Span}{\mathrm{span}}\)
( \newcommand{\kernel}{\mathrm{null}\,}\) \( \newcommand{\range}{\mathrm{range}\,}\)
\( \newcommand{\RealPart}{\mathrm{Re}}\) \( \newcommand{\ImaginaryPart}{\mathrm{Im}}\)
\( \newcommand{\Argument}{\mathrm{Arg}}\) \( \newcommand{\norm}[1]{\| #1 \|}\)
\( \newcommand{\inner}[2]{\langle #1, #2 \rangle}\)
\( \newcommand{\Span}{\mathrm{span}}\)
\( \newcommand{\id}{\mathrm{id}}\)
\( \newcommand{\kernel}{\mathrm{null}\,}\)
\( \newcommand{\range}{\mathrm{range}\,}\)
\( \newcommand{\RealPart}{\mathrm{Re}}\)
\( \newcommand{\ImaginaryPart}{\mathrm{Im}}\)
\( \newcommand{\Argument}{\mathrm{Arg}}\)
\( \newcommand{\norm}[1]{\| #1 \|}\)
\( \newcommand{\Span}{\mathrm{span}}\) \( \newcommand{\AA}{\unicode[.8,0]{x212B}}\)
\( \newcommand{\vectorA}[1]{\vec{#1}} % arrow\)
\( \newcommand{\vectorAt}[1]{\vec{\text{#1}}} % arrow\)
\( \newcommand{\vectorB}[1]{\overset { \scriptstyle \rightharpoonup} {\mathbf{#1}} } \)
\( \newcommand{\vectorC}[1]{\textbf{#1}} \)
\( \newcommand{\vectorD}[1]{\overrightarrow{#1}} \)
\( \newcommand{\vectorDt}[1]{\overrightarrow{\text{#1}}} \)
\( \newcommand{\vectE}[1]{\overset{-\!-\!\rightharpoonup}{\vphantom{a}\smash{\mathbf {#1}}}} \)
Introduction
The Punnett square is a valuable tool, but it's not ideal for every genetics problem. For instance, suppose you were asked to calculate the frequency of the recessive class not for an Aa x Aa cross, not for an AaBb x AaBb cross, but for an AaBbCcDdEe x AaBbCcDdEe cross. If you wanted to solve that question using a Punnett square, you could do it – but you'd need to complete a Punnett square with 1024 boxes. Probably not what you want to draw during an exam, or any other time, if you can help it!
The five-gene problem above becomes less intimidating once you realize that a Punnett square is just a visual way of representing probability calculations. Although it’s a great tool when you’re working with one or two genes, it can become slow and cumbersome as the number goes up. At some point, it becomes quicker (and less error-prone) to simply do the probability calculations by themselves, without the visual representation of a clunky Punnett square. In all cases, the calculations and the square provide the same information, but by having both tools in your belt, you can be prepared to handle a wider range of problems in a more efficient way.
In this article, we’ll review some probability basics, including how to calculate the probability of two independent events both occurring (event X and event Y) or the probability of either of two mutually exclusive events occurring (event X or event Y). We’ll then see how these calculations can be applied to genetics problems, and, in particular, how they can help you solve problems involving relatively large numbers of genes.
In this problem, we’re supposed to find the frequency of the recessive class among the offspring of an AaBbCcDdEe x AaBbCcDdEe cross – that is, the frequency of aabbccddee individuals. How do we get an aabbccddee individual? There’s only one way for that to happen: both parents must contribute an abcde gamete.
What, then, is the probability that one of the parents will make an abcde gamete? Both parents are heterozygous for all five genes, so there’s a 1/2 chance of getting the recessive (lowercase) allele for any one gene. To get our desired gamete, we need all five genes in recessive form ( a and b and c and d and e ). This is a case where we can apply the product rule , which states that the probability of event X and event Y happening is the product of their individual probabilities (probability of X times probability of Y), assuming that X and Y are independent events. Thus, the overall probability of one parent producing an abcde gamete is:
Probability of abcde gamete = (probability of a ) x (probability of b ) x (probability of c ) x (probability of d ) x (probability of e )
\(P(abcde)=P(a)\cdot P(b)\cdot P(c)\cdot P(d)\cdot P(e)\)
\(P(abcde)=(1/2)\cdot (1/2)\cdot (1/2)\cdot (1/2)\cdot (1/2)=(1/2)^5=1/32\)
If that’s the probability of one parent making an abcde gamete, what’s the likelihood of both parents doing so? Again, we can apply the "and" rule (product rule), since we need both parent 1 and parent 2 to make an abcde gamete in order to get our target recessive homozygote. Thus, the overall probability is:
Probability of aabbccddee individual = (probability of parent 1 making an abcde gamete) x (probability of parent 2 making an abcde gamete)
\(P(aabbccddee)=P(abcde_\text{parent A})\cdot P(abcde_\text{parent B})\)
\(P(aabbccddee)=(1/32)\cdot (1/32)=1/1024\)
That’s our overall probability for a recessive homozygote for all five genes.
The 1/1024 probability corresponds to 1 box out of the 1024 boxes of the Punnett square you’d have to draw to represent this cross. The probability calculation is the same calculation we’d implicitly do by drawing the Punnett square, just faster and with fewer chances for mistakes.
Probability basics
Probabilities are mathematical measures of likelihood. In other words, they’re a way of quantifying (giving a specific, numerical value to) how likely something is to happen. A probability of 1 for an event means that it is guaranteed to happen, while a probability of 0 for an event means that it is guaranteed not to happen. A simple example of probability is having a 1/2 chance of getting heads when you flip a coin, as Sal explains in this intro to probability video.
Probabilities can be either empirical, meaning that they are calculated from real-life observations, or theoretical, meaning that they are predicted using a set of rules or assumptions.
- The empirical probability of an event is calculated by counting the number of times that event occurs and dividing it by the total number of times that event could have occurred. For instance, if the event you were looking for was a wrinkled pea seed, and you saw it 1,850 times out of the 7,324 total seeds you examined, the empirical probability of getting a wrinkled seed would be 1,850/7,324 = 0.253, or very close to 1 in 4 seeds.
- The theoretical probability of an event is calculated based on information about the rules and circumstances that produce the event. It reflects the number of times an event is expected to occur relative to the number of times it could possibly occur. For instance, if you had a pea plant heterozygous for a seed shape gene ( Rr ) and let it self-fertilize, you could use the rules of probability and your knowledge of genetics to predict that 1 out of every 4 offspring would get two recessive alleles ( rr ) and appear wrinkled, corresponding to a 0.25 (1/4) probability. We’ll talk more below about how to apply the rules of probability in this case.
In general, the larger the number of data points that are used to calculate an empirical probability, such as shapes of individual pea seeds, the more closely it will approach the theoretical probability.
The product rule
One probability rule that's very useful in genetics is the product rule , which states that the probability of two (or more) independent events occurring together can be calculated by multiplying the individual probabilities of the events. For example, if you roll a six-sided die once, you have a 1/6 chance of getting a six. If you roll two dice at once, your chance of getting two sixes is: (probability of a six on die 1) x (probability of a six on die 2) = (1/6) ⋅ (1/6) = 1/36.
In general, you can think of the product rule as the “and” rule: if both event X and event Y must happen in order for a certain outcome to occur, and if X and Y are independent of each other (don’t affect each other’s likelihood), then you can use the product rule to calculate the probability of the outcome by multiplying the probabilities of X and Y.
We can use the product rule to predict frequencies of fertilization events. For instance, consider a cross between two heterozygous ( Aa ) individuals. What are the odds of getting an aa individual in the next generation? The only way to get an aa individual is if the mother contributes an a gamete and the father contributes an a gamete. Each parent has a 1/2 chance of making an a gamete. Thus, the chance of an aa offspring is: (probability of mother contributing a ) x (probability of father contributing a ) = (1/2) ⋅ (1/2) = 1/4.
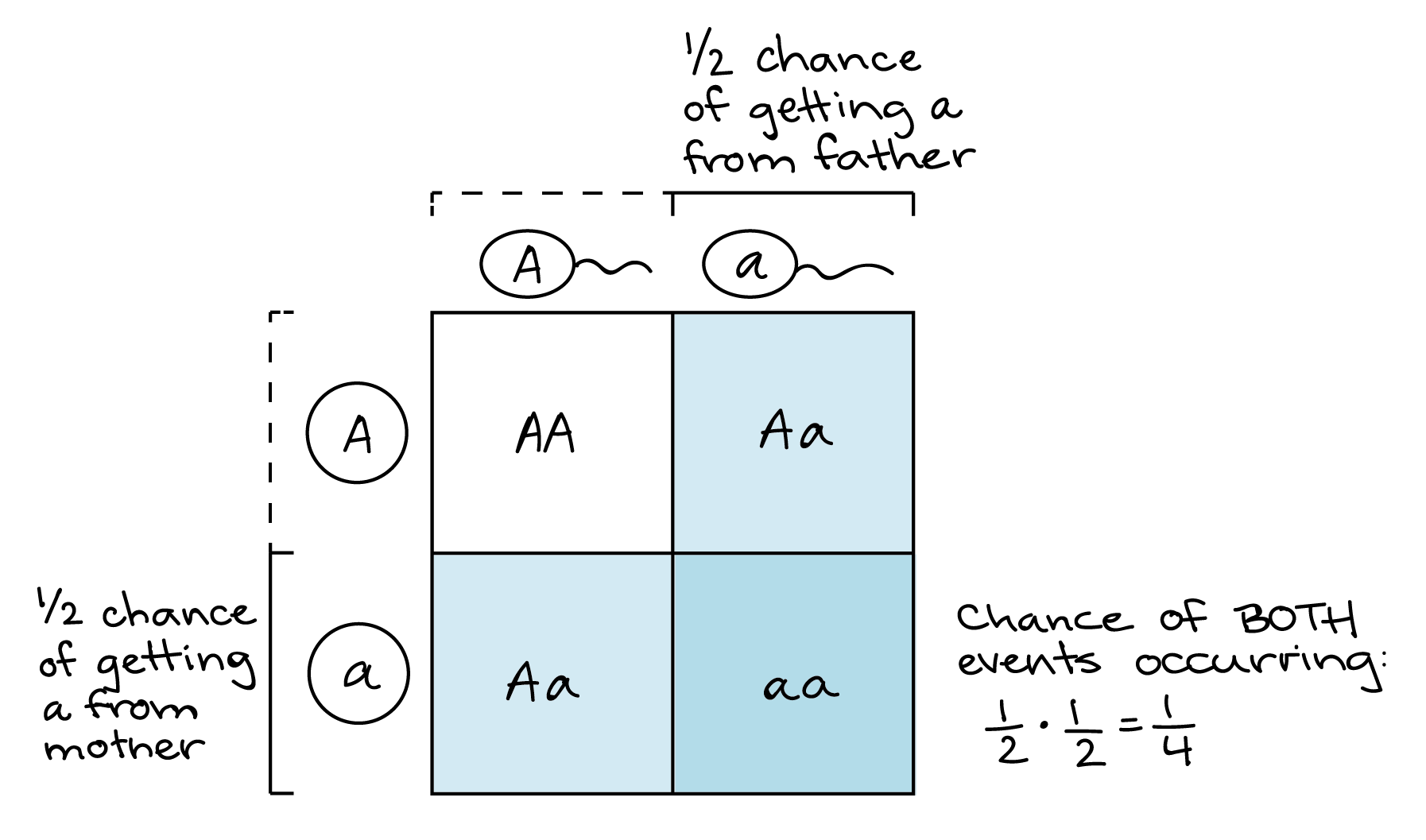
This is the same result you’d get with a Punnett square, and actually the same logical process as well—something that took me years to realize! The only difference is that, in the Punnett square, we'd do the calculation visually: we'd represent the 1/2 probability of an a gamete from each parent as one out of two columns (for the father) and one out of two rows (for the mother). The 1-square intersect of the column and row (out of the 4 total squares of the table) represents the 1/4 chance of getting an a from both parents.
The sum rule of probability
In some genetics problems, you may need to calculate the probability that any one of several events will occur. In this case, you’ll need to apply another rule of probability, the sum rule. According to the sum rule , the probability that any of several mutually exclusive events will occur is equal to the sum of the events’ individual probabilities.
For example, if you roll a six-sided die, you have a 1/6 chance of getting any given number, but you can only get one number per roll. You could never get both a one and a six at the same time; these outcomes are mutually exclusive. Thus, the chances of getting either a one or a six are: (probability of getting a 1) + (probability of getting a 6) = (1/6) + (1/6) = 1/3.
You can think of the sum rule as the “or” rule: if an outcome requires that either event X or event Y occur, and if X and Y are mutually exclusive (if only one or the other can occur in a given case), then the probability of the outcome can be calculated by adding the probabilities of X and Y.
As an example, let's use the sum rule to predict the fraction of offspring from an Aa x Aa cross that will have the dominant phenotype ( AA or Aa genotype). In this cross, there are three events that can lead to a dominant phenotype:
- Two A gametes meet (giving AA genotype), or
- A gamete from Mom meets a gamete from Dad (giving Aa genotype), or
- a gamete from Mom meets A gamete from Dad (giving Aa genotype)
In any one fertilization event, only one of these three possibilities can occur (they are mutually exclusive).
Since this is an “or” situation where the events are mutually exclusive, we can apply the sum rule. Using the product rule as we did above, we can find that each individual event has a probability of 1/4. So, the probability of offspring with a dominant phenotype is: (probability of A from Mom and A from Dad) + (probability of A from Mom and a from Dad) + (probability of a from Mom and A from Dad) = (1/4) + (1/4) + (1/4) = 3/4.
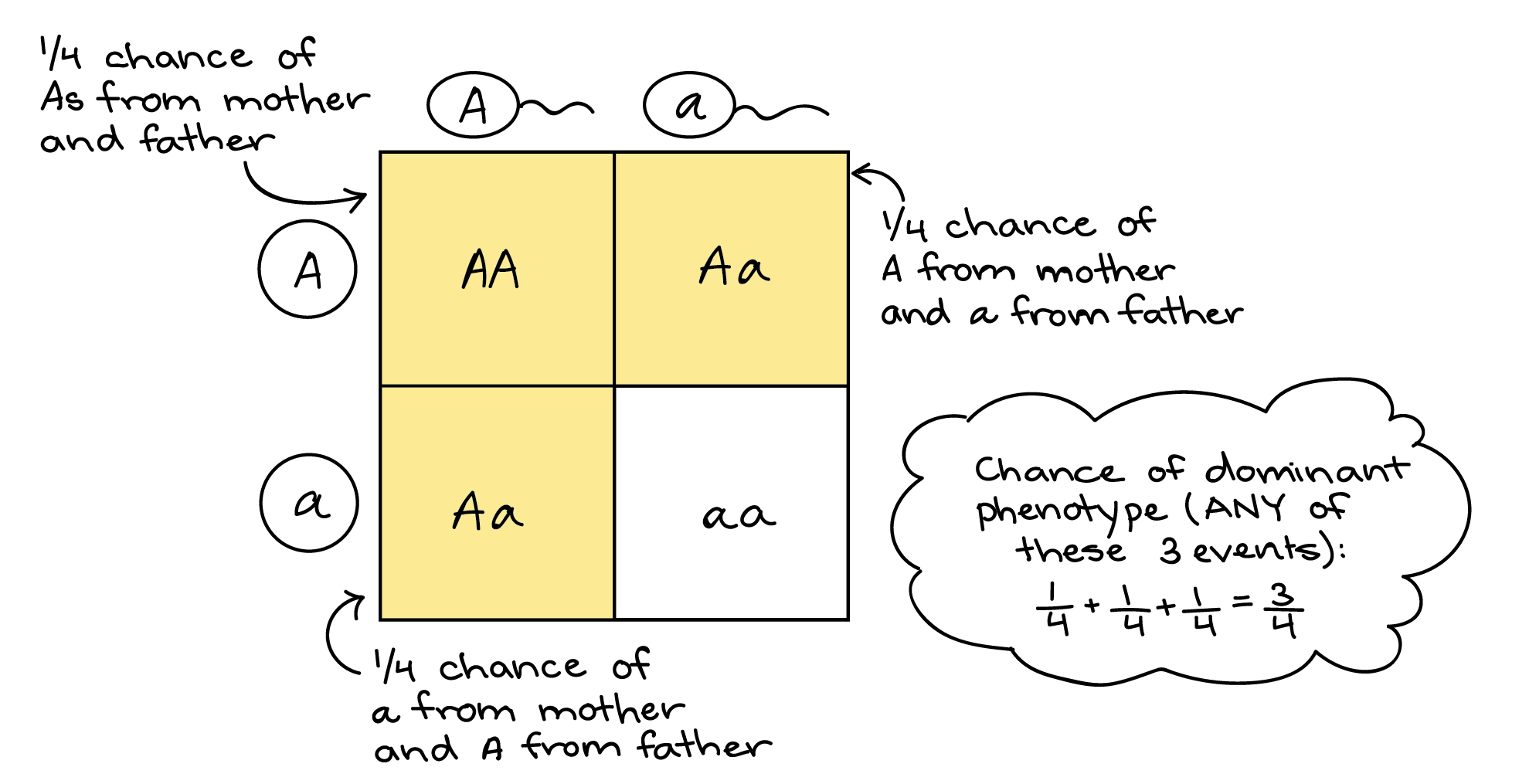
Once again, this is the same result we’d get with a Punnett square. One out of the four boxes of the Punnett square holds the dominant homozygote, AA . Two more boxes represent heterozygotes, one with a maternal A and a paternal a , the other with the opposite combination. Each box is 1 out of the 4 boxes in the whole Punnett square, and since the boxes don't overlap (they’re mutually exclusive), we can add them up (1/4 + 1/4 + 1/4 = 3/4) to get the probability of offspring with the dominant phenotype.
The product rule and the sum rule
Applying probability rules to dihybrid crosses.
Direct calculation of probabilities doesn’t have much advantage over Punnett squares for single-gene inheritance scenarios. (In fact, if you prefer to learn visually, you may find direct calculation trickier rather than easier.) Where probabilities shine, though, is when you’re looking at the behavior of two, or even more, genes.
For instance, let’s imagine that we breed two dogs with the genotype BbCc , where dominant allele B specifies black coat color (versus b , yellow coat color) and dominant allele C specifies straight fur (versus c , curly fur). Assuming that the two genes assort independently and are not sex-linked, how can we predict the number of BbCc puppies among the offspring?
One approach is to draw a 16-square Punnett square. For a cross involving two genes, a Punnett square is still a good strategy. Alternatively, we can use a shortcut technique involving four-square Punnett squares and a little application of the product rule. In this technique, we break the overall question down into two smaller questions, each relating to a different genetic event:
- What’s the probability of getting a Bb genotype?
- What’s the probability of getting an Cc genotype?
In order for a puppy to have a BbCc genotype, both of these events must take place: the puppy must receive Bb alleles, and it must receive Cc alleles. The two events are independent because the genes assort independently (don't affect one another's inheritance). So, once we calculate the probability of each genetic event, we can multiply these probabilities using the product rule to get the probability of the genotype of interest ( BbCc ).
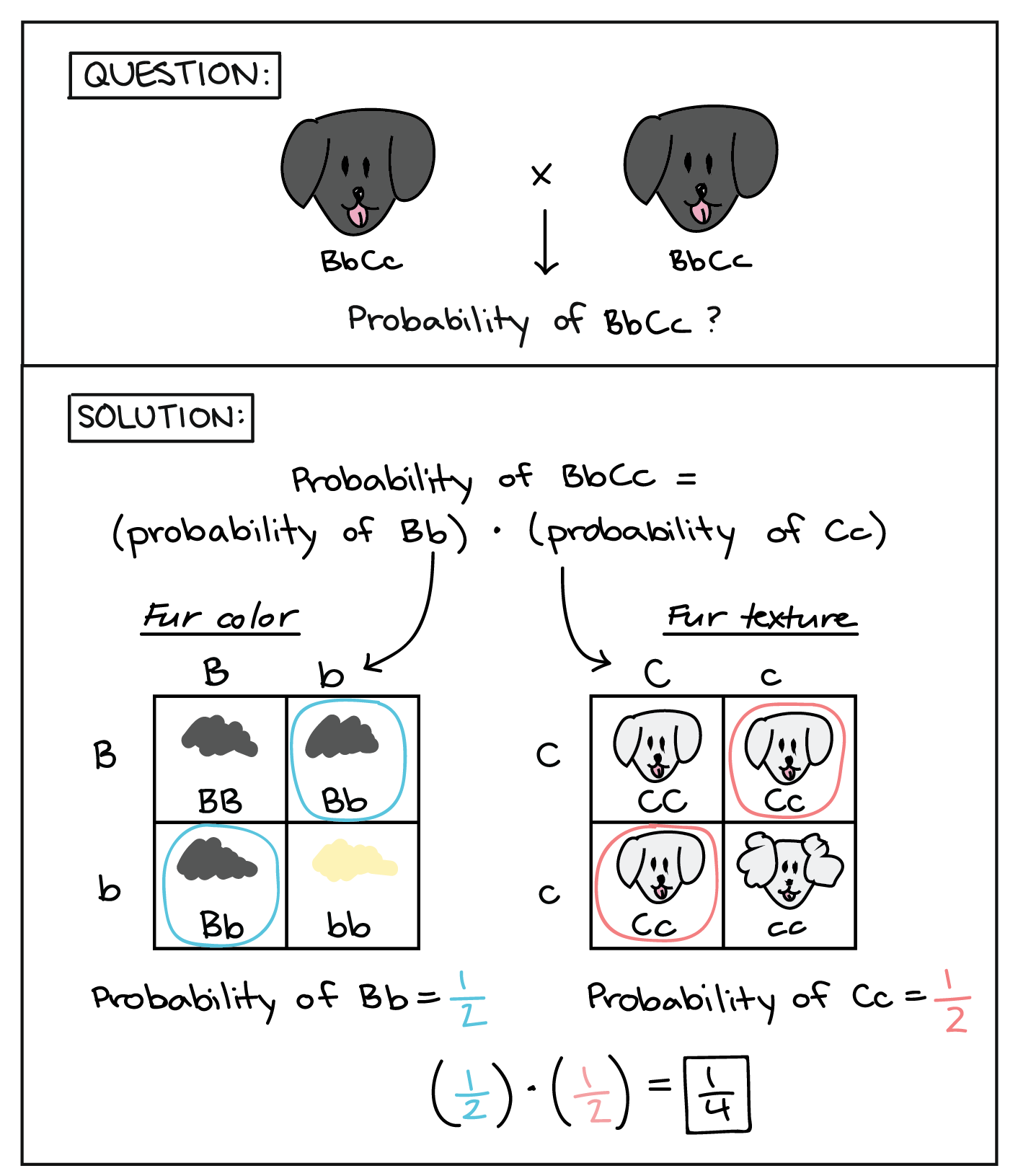
To calculate the probability of getting a Bb genotype, we can draw a 4-square Punnett square using the parents' alleles for the coat color gene only, as shown above. Using the Punnett square, you can see that the probability of the Bb genotype is 1/2. (Alternatively, we could have calculated the probability of Bb using the product rule for gamete contributions from the two parents and the sum rule for the two gamete combinations that give Bb .) Using a similar Punnett square for the parents' fur texture alleles, the probability of getting an Cc genotype is also 1/2. To get the overall probability of the BbCc genotype, we can simply multiply the two probabilities, giving an overall probability of 1/4.
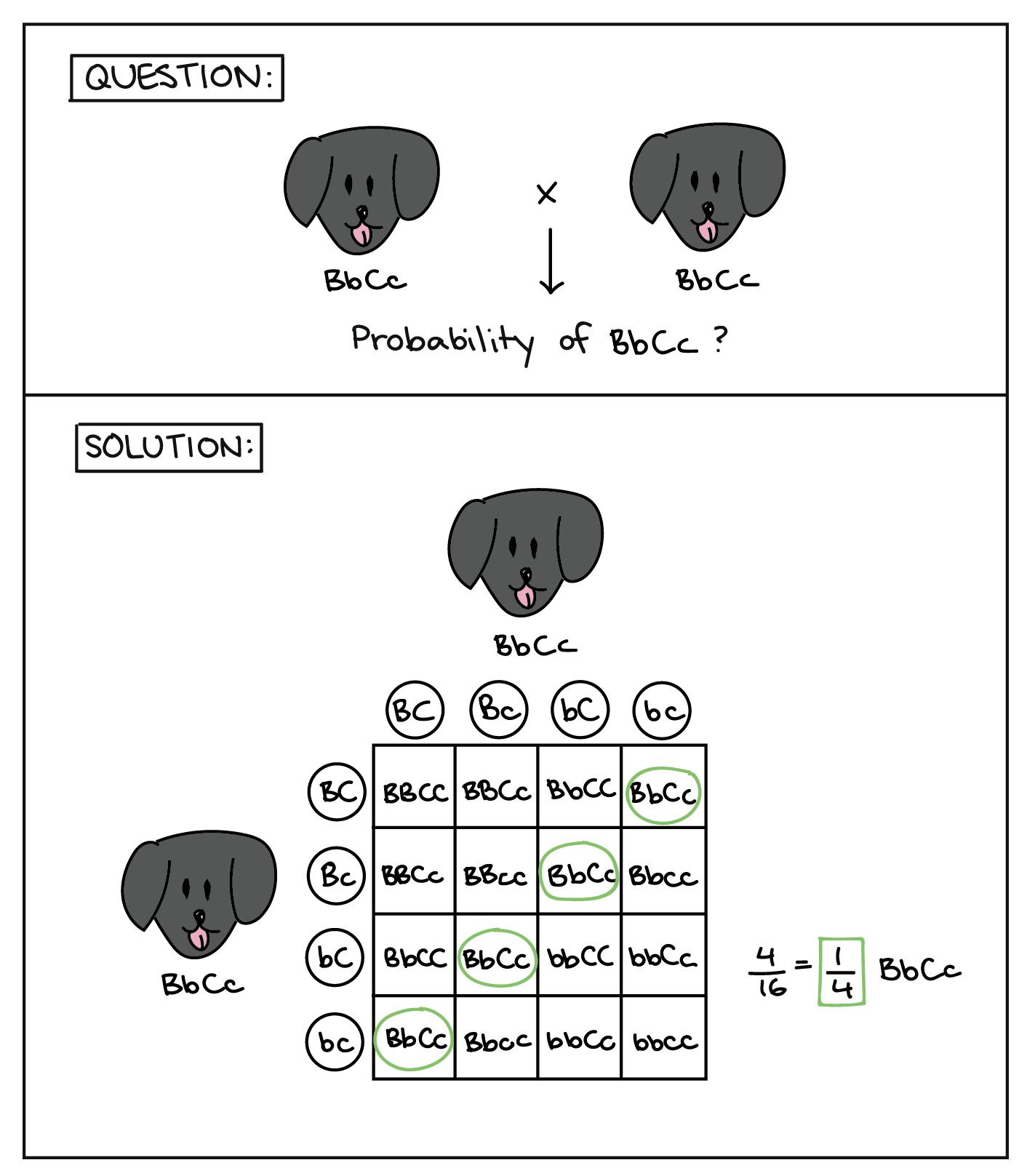
You can also use this technique to predict phenotype frequencies. Give it a try in the practice question below!
Check your understanding
Query \(\PageIndex{1}\)
We can break the question down into two smaller questions:
- What fraction of offspring will have black coat color?
- What fraction of offspring will have straight fur?
Since black coat color and straight fur are dominant traits, all BB and Bb puppies will have black coats, and all CC and Cc puppies will have straight fur, corresponding to 3/4 of puppies in each case. (You can draw out the individual Punnett squares for the color and texture genes to confirm these frequencies.)
To get the probability of a puppy having both black coat color and straight fur, you can multiply the probabilities of these two independent events: \((3/4)\cdot(3/4)=9/16\).
9/16 of the puppies will have black coats and straight fur.
Beyond dihybrid crosses
The probability method is most powerful (and helpful) in cases involving a large number of genes.
For instance, imagine a cross between two individuals with various alleles of four unlinked genes: AaBbCCdd x AabbCcDd . Suppose you wanted to figure out the probability of getting offspring with the dominant phenotype for all four traits. Fortunately, you can apply the exact same logic as in the case of the dihybrid crosses above. To have the dominant phenotype for all four traits, and organism must have: one or more copies of the dominant allele A and one or more copies of dominant allele B and one or more copies of the dominant allele C and one or more copies of the dominant allele D .
Since the genes are unlinked, these are four independent events, so we can calculate a probability for each and then multiply the probabilities to get the probability of the overall outcome.
- The probability of getting one or more copies of the dominant A allele is 3/4. (Draw a Punnett square for Aa x Aa to confirm for yourself that 3 out of the 4 squares are either AA or Aa .)
- The probability of getting one or more copies of the dominant B allele is 1/2. (Draw a Punnett square for Bb x bb : you’ll find that half the offspring are Bb , and the other half bb .)
- The probability of getting one or more copies of the dominant C allele is 1. (If one of the parents is homozygous CC , there’s no way to get offspring without a C allele!)
- The probability of getting one or more copies of the dominant D allele is 1/2, as for B . (Half the offspring will be Dd , and the other half will be dd .)
To get the overall probability of offspring with the dominant phenotype for all four genes, we can multiply the probabilities of the four independent events: \((3/4)\cdot(1/2)\cdot(1)\cdot(1/2)=3/16\).
Query \(\PageIndex{2}\)
It’s not possible to get a quadruple homozygous recessive individual out of this cross. That’s because the probability of getting two recessive c alleles is zero. The first parent has only dominant alleles for this gene, ensuring that each of the offspring will receive at least one dominant C allele (and thus cannot display the recessive phenotype).
How does the zero probability of a cc genotype figure in mathematically? To get the overall probability of the aabbccdd genotype, we'd have to multiply the probabilities of the desired genotypes for the other three genes ( aa , 1/4; bb , 1/2; and dd , 1/2) by the zero corresponding to the cc genotype, giving an overall probability of zero.
\(P(aabbccdd)=P(aa) \cdot P(bb) \cdot P(cc) \cdot P(dd)\)
\(P(aabbccdd)=(1/4)\cdot(1/2)\cdot(0)\cdot(1/2)=0\)
The probability of getting an individual with a recessive phenotype for all four genes is 0.
Contributors and Attributions
Khan Academy (CC BY-NC-SA 3.0; All Khan Academy content is available for free at www.khanacademy.org )
Attribution:
This article is a modified derivative of the following articles:
- “ Mendel’s experiments and the laws of probability ,” by OpenStax College, Biology ( CC BY 3.0 ). Download the original article for free at http://cnx.org/contents/[email protected] .
- “ Laws of inheritance ,” by OpenStax College, Biology ( CC BY 3.0 ). Download the original article for free at http://cnx.org/contents/[email protected] .
The modified article is licensed under a CC BY-NC-SA 4.0 license.
Additional references:
Griffiths, A. J. F., Miller, J. H., Suzuki, D. T., Lewontin, R. C., and Gelbart, W. M. (2000). Using genetic ratios. In An introduction to genetic analysis (7th ed.). New York, NY: W. H. Freeman. Retrieved from http://www.ncbi.nlm.nih.gov/books/NBK21812/ .
Purves, W. K., Sadava, D., Orians, G. H., and Heller, H. C. (2003). Punnett squares or probability calculations: A choice of methods. In Life: The science of biology (7th ed., pp. 195-196). Sunderland, MA: Sinauer Associates.
Reece, J. B., Urry, L. A., Cain, M. L., Wasserman, S. A., Minorsky, P. V., and Jackson, R. B. (2011). Mendel and the gene idea. In Campbell Biology (10th ed., pp. 267-291). San Francisco, CA: Pearson.
Raven, P. H., Johnson, G. B., Mason, K. A., Losos, J. B., and Singer, S. R. (2014). Patterns of inheritance. In Biology (10th ed., AP ed., pp. 221-238). New York, NY: McGraw-Hill.
Staroscik, A. (2015). Punnett square calculator. In SciencePrimer.com . Retrieved from http://scienceprimer.com/punnett-square-calculator .
The Adapa Project. (2014, August 13). What are the laws of segregation and independent assortment and why are they so important? In BioBook . Retrieved from https://adapaproject.org/bbk_temp/tiki-index.php?page=Leaf%3A+What+are+the+laws+of+segregation+and+independent+assortment+and+why+are+they+so+important%3F .
Four problems that biotechnology can help solve
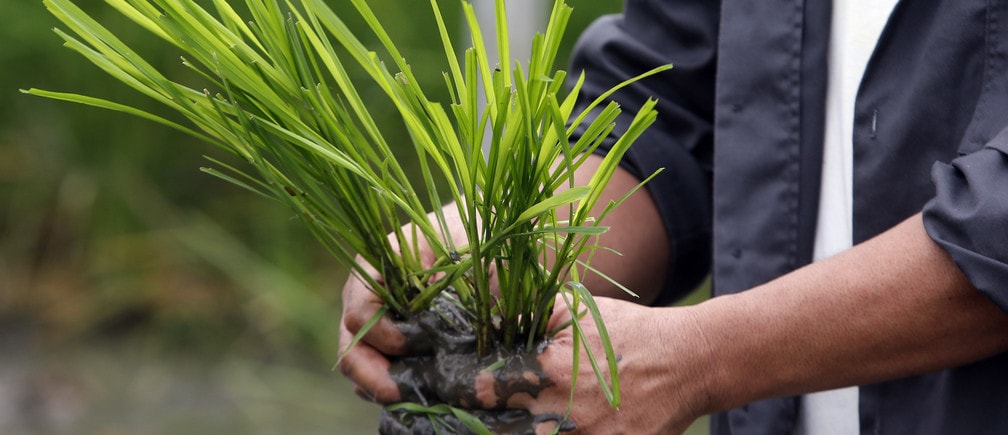
Image: REUTERS/Erik De Castro
.chakra .wef-1c7l3mo{-webkit-transition:all 0.15s ease-out;transition:all 0.15s ease-out;cursor:pointer;-webkit-text-decoration:none;text-decoration:none;outline:none;color:inherit;}.chakra .wef-1c7l3mo:hover,.chakra .wef-1c7l3mo[data-hover]{-webkit-text-decoration:underline;text-decoration:underline;}.chakra .wef-1c7l3mo:focus,.chakra .wef-1c7l3mo[data-focus]{box-shadow:0 0 0 3px rgba(168,203,251,0.5);} Patrick Nee
Christopher dacunha.
.chakra .wef-9dduvl{margin-top:16px;margin-bottom:16px;line-height:1.388;font-size:1.25rem;}@media screen and (min-width:56.5rem){.chakra .wef-9dduvl{font-size:1.125rem;}} Explore and monitor how .chakra .wef-15eoq1r{margin-top:16px;margin-bottom:16px;line-height:1.388;font-size:1.25rem;color:#F7DB5E;}@media screen and (min-width:56.5rem){.chakra .wef-15eoq1r{font-size:1.125rem;}} Future of the Environment is affecting economies, industries and global issues
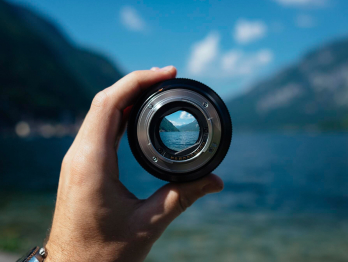
.chakra .wef-1nk5u5d{margin-top:16px;margin-bottom:16px;line-height:1.388;color:#2846F8;font-size:1.25rem;}@media screen and (min-width:56.5rem){.chakra .wef-1nk5u5d{font-size:1.125rem;}} Get involved with our crowdsourced digital platform to deliver impact at scale
Stay up to date:, future of global health and healthcare.
Many of the problems facing humanity are the same recurring challenges that man has tackled for centuries. Hunger, disease, the need for raw materials, and pollution have limited humanity since prehistoric times. However, throughout history the development of new technologies has enabled dramatic improvements in our quality of life.
Modern molecular biotechnology, or the application of our knowledge of the genome to engineer organisms with beneficial traits, enables new solutions to today’s challenges. Today, the Fourth Industrial Revolution, which adds the tools of molecular biotechnology to humanity’s toolbox, promises similar improvements in wellbeing as those that were delivered by previous technological innovations. Utilizing every available technology is crucial as we strive as a species to support higher populations with fewer resources.
But public fear of biotechnology, in spite of the tremendous advances it has already provided, may prevent these innovations from having the impact they promise. The biotechnology industry must substantially increase its efforts to educate and engage the public to ensure that biotechnology truly lives up to its potential.
1. Feeding the next billion
Industrial farming and food production have prompted dramatic shifts in the world economy, and fewer than 5% of developed countries’ populations now work in agriculture. But the World Bank predicts that we will need to produce yet another 50% more food by 2050 while climate change may reduce productivity by 25%.
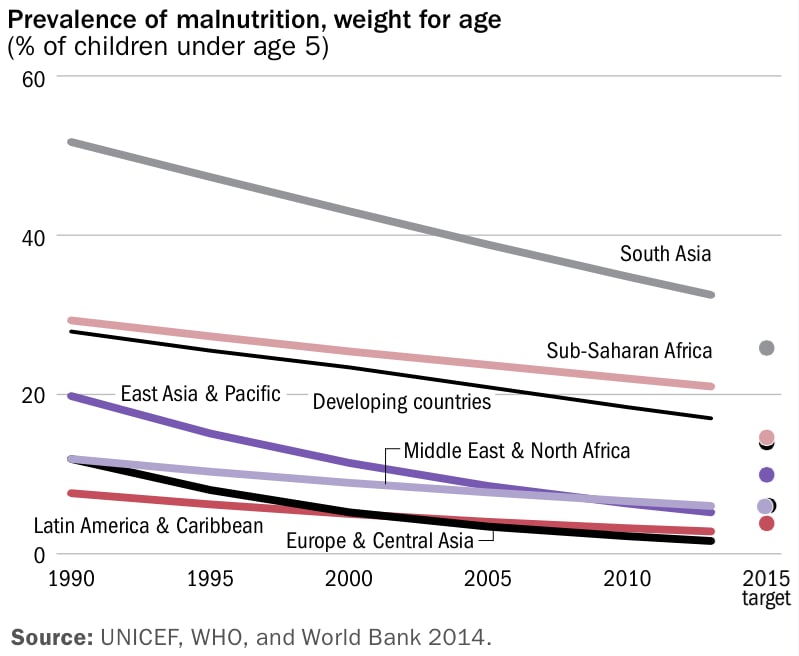
Simply dedicating more land to agriculture is one potential solution, but may result in food production far from the areas of greatest need. Increases in productivity per acre, drought resistant crops, and decreases in the need for chemical fertilizers would all go far to sustainably achieving the food production the world will need, reducing pressure to transform lush forests into agricultural land.
Biotechnology companies such as Indigo Agriculture are employing microbes which can make crops more productive and tolerant of environmental stress, helping to feed the next billion people. In addition, drought resistant crops are being developed by Pioneer, Syngenta, and Monsanto. Foods can also deliver enhanced nutrition, such as Golden Rice with additional vitamin A from the International Rice Research Institute.
2. Tackling disease
Some of the first applications of genetic engineering were in the pharmaceutical industry, helping to treat medical conditions and diseases. Insulin, synthesized with biotechnology, avoided the use of insulin isolated from pigs, to which some patients are allergic. Other treatments created by biotechnology include interferon therapy to trigger one’s immune system, human growth hormone, and the hepatitis B vaccine.
Yet, in spite of this tremendous progress in modern medicine, today we face scary prospects, including the spread of the Zika virus and the rise of antibiotic-resistant bacteria. Biotechnology offers some of the most promising and targeted ways to find solutions to these threats. For example, the British company Oxitec, a subsidiary of Intrexon Corporation, offers a technology to control the spread of a single species of insect, Aedes aegypti, the primary vector for dengue, chikungunya and Zika virus outbreaks around the world. And many researchers are investigating the use of CRISPR/Cas gene-editing technology as a new method of controlling antibiotic-resistant microorganisms.
3. Cleaning up pollution
Glacial records have shown us that, as long as 2,500 years ago, roman-era metal production was a source of global pollution. The streets of London and other cities were polluted by coal and wood fires, as well as by the horses used as transportation. Today, our pollution challenges appear more subtle, but surely technologies will also turn them into anachronisms.
One company, PIARCS, PBC has a new biotechnology to resolve phosphorous in wastewater treatment plants, our own company Universal Bio Mining is developing enzymes capable of degrading chemical residue of petroleum production in the oil sands industry, and Carbios of France is developing a technique to recycle the ubiquitous PET plastic used in our disposable packaging.
4. Harnessing scarce natural resources
The availability of natural resources has always been a constraint and a source of international tension. As easy to reach and process metal deposits are depleted, the mining industry must double the amount of earth it removes from the ground every eight years. In another example, clean fresh water is expected to become one of the greatest sources of international conflict during the 21st century, as people battle over the control of rivers and underground water sources.
Here, again, biotechnology offers new tools to soften or resolve these challenges. Our company, Universal Bio Mining, is developing new processes to extract copper and gold from ores that are currently uneconomic, and start-up companies such as CustoMem from Imperial College of London have created water filters utilizing proteins to filter polluted waters.
The future of biotech
Advances in technology have dramatically increased the rates at which these new biotechnologies can be developed, while at the same time, reducing the cost of development. Gene sequencing and synthesis technologies have dropped precipitously in price, allowing innovators to develop their inventions much faster, and at a lower cost.
However, challenges remain. Regulatory roadblocks make it difficult for small biotech innovators to set up laboratories, and the path to regulatory approval for some technologies remains unclear, discouraging investment.
But most importantly, public fear of these new tools often prevents them from turning into commercial products. For example, the Golden Rice product, announced in 2000 to reduce Vitamin A deficiency that kills 600,000 children per year, has yet to be grown commercially.
The challenges facing humanity remain significant and society simply cannot let these potential solutions be ignored.
The biotechnology industry must continue to educate the public, regulators, and other industries about the potential of the sector. This means actively participating in the development of regulatory processes for these evolving technologies, inviting conversations with all stakeholders, and ensuring the public understands both the technology and the benefits that it delivers.
With time, the public will ask, “Why hasn’t biotechnology solved this problem yet?”
For more information on the Technology Pioneers 2016, visit our website .
Don't miss any update on this topic
Create a free account and access your personalized content collection with our latest publications and analyses.
License and Republishing
World Economic Forum articles may be republished in accordance with the Creative Commons Attribution-NonCommercial-NoDerivatives 4.0 International Public License, and in accordance with our Terms of Use.
The views expressed in this article are those of the author alone and not the World Economic Forum.
Related topics:
The agenda .chakra .wef-n7bacu{margin-top:16px;margin-bottom:16px;line-height:1.388;font-weight:400;} weekly.
A weekly update of the most important issues driving the global agenda
.chakra .wef-1dtnjt5{display:-webkit-box;display:-webkit-flex;display:-ms-flexbox;display:flex;-webkit-align-items:center;-webkit-box-align:center;-ms-flex-align:center;align-items:center;-webkit-flex-wrap:wrap;-ms-flex-wrap:wrap;flex-wrap:wrap;} More on Nature and Biodiversity .chakra .wef-17xejub{-webkit-flex:1;-ms-flex:1;flex:1;justify-self:stretch;-webkit-align-self:stretch;-ms-flex-item-align:stretch;align-self:stretch;} .chakra .wef-nr1rr4{display:-webkit-inline-box;display:-webkit-inline-flex;display:-ms-inline-flexbox;display:inline-flex;white-space:normal;vertical-align:middle;text-transform:uppercase;font-size:0.75rem;border-radius:0.25rem;font-weight:700;-webkit-align-items:center;-webkit-box-align:center;-ms-flex-align:center;align-items:center;line-height:1.2;-webkit-letter-spacing:1.25px;-moz-letter-spacing:1.25px;-ms-letter-spacing:1.25px;letter-spacing:1.25px;background:none;padding:0px;color:#B3B3B3;-webkit-box-decoration-break:clone;box-decoration-break:clone;-webkit-box-decoration-break:clone;}@media screen and (min-width:37.5rem){.chakra .wef-nr1rr4{font-size:0.875rem;}}@media screen and (min-width:56.5rem){.chakra .wef-nr1rr4{font-size:1rem;}} See all
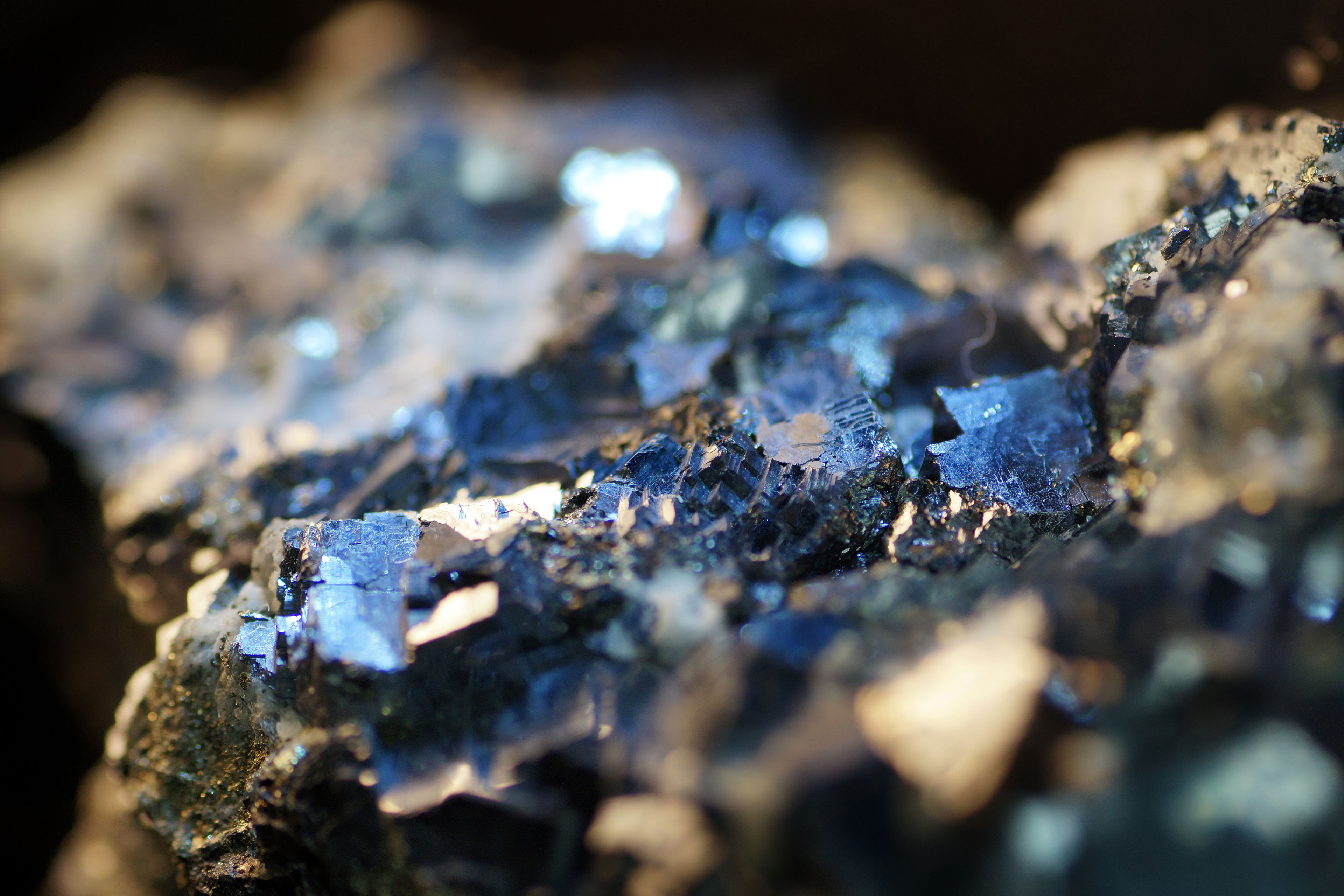
Critical minerals demand has doubled in the past five years – here are some solutions to the supply crunch
Emma Charlton
May 16, 2024
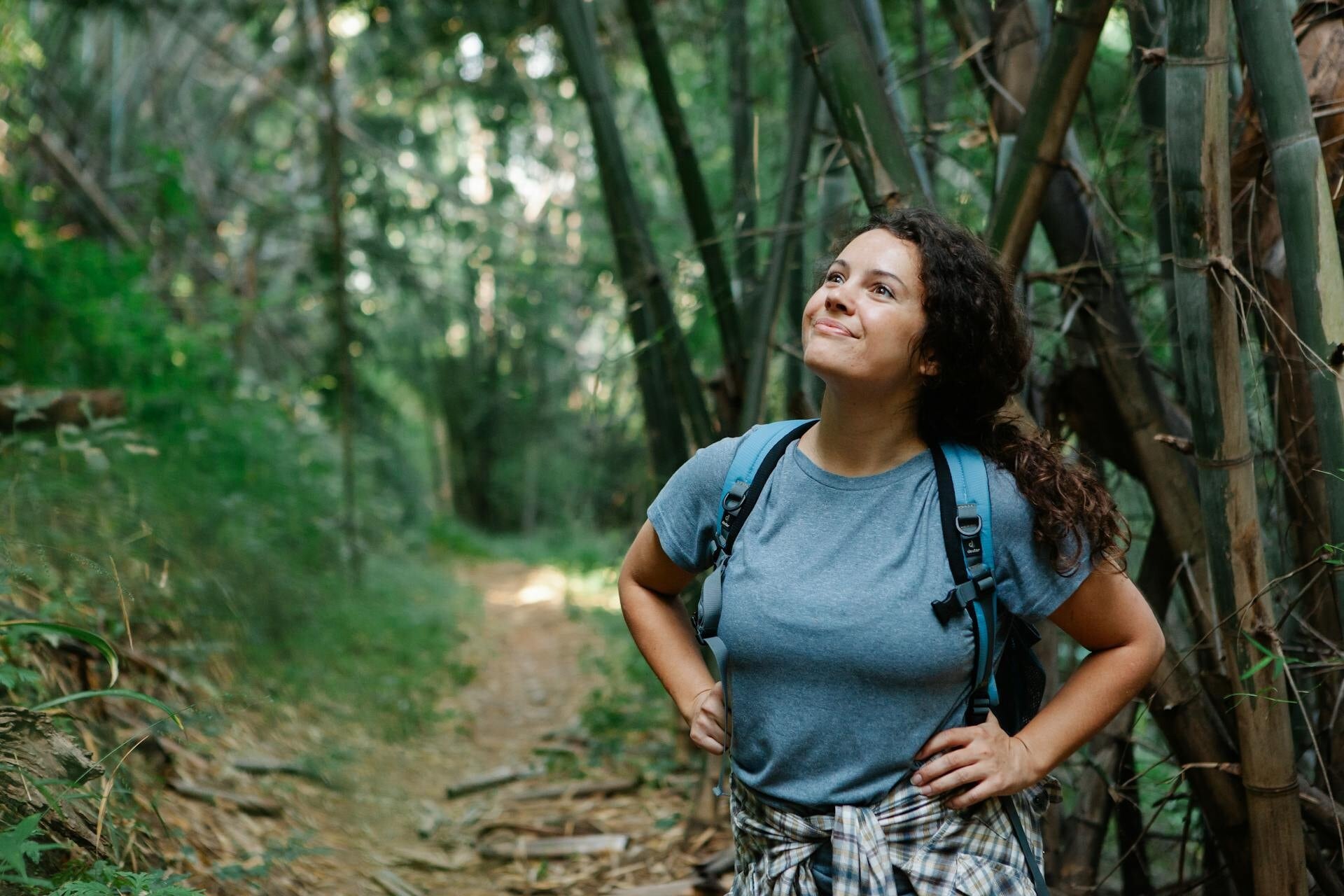
The fascinating link between biodiversity and mental wellbeing
Andrea Mechelli
May 15, 2024

These Japanese volunteers are planting seagrass to fight climate change
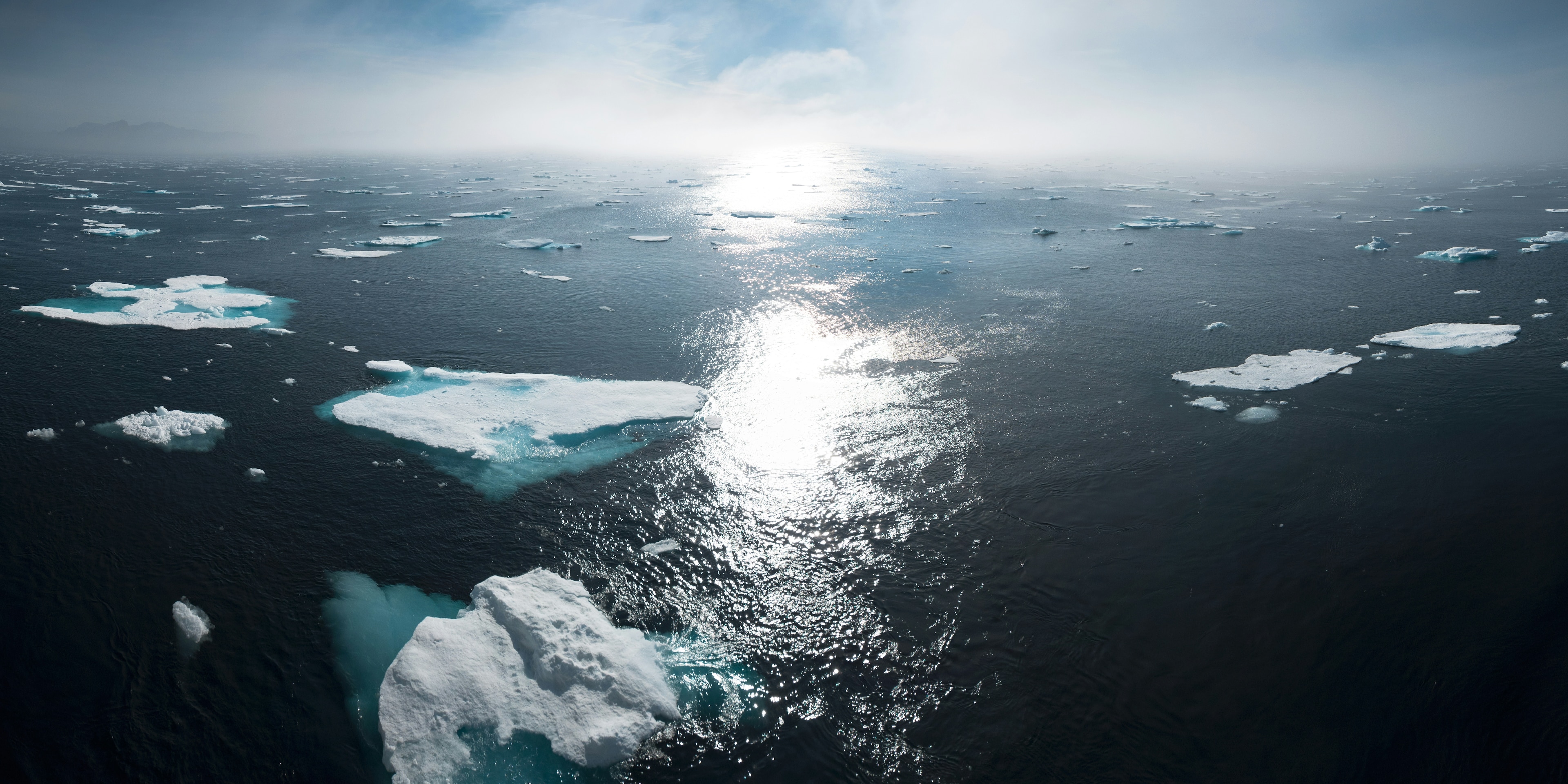
Scientists expect global heating to exceed 1.5°C, and other nature and climate stories you need to read this week
May 13, 2024

Scientists have found 700 species in a Cambodian mangrove forest
Funding the green technology innovation pipeline: Lessons from China
May 8, 2024
Thank you for visiting nature.com. You are using a browser version with limited support for CSS. To obtain the best experience, we recommend you use a more up to date browser (or turn off compatibility mode in Internet Explorer). In the meantime, to ensure continued support, we are displaying the site without styles and JavaScript.
- View all journals
- Explore content
- About the journal
- Publish with us
- Sign up for alerts
- Perspective
- Published: 13 May 2024
Integrating population genetics, stem cell biology and cellular genomics to study complex human diseases
- Nona Farbehi ORCID: orcid.org/0000-0001-8461-236X 1 , 2 , 3 na1 ,
- Drew R. Neavin ORCID: orcid.org/0000-0002-1783-6491 1 na1 ,
- Anna S. E. Cuomo 1 , 4 ,
- Lorenz Studer ORCID: orcid.org/0000-0003-0741-7987 3 , 5 ,
- Daniel G. MacArthur 4 , 6 &
- Joseph E. Powell ORCID: orcid.org/0000-0002-5070-4124 1 , 3 , 7
Nature Genetics volume 56 , pages 758–766 ( 2024 ) Cite this article
2041 Accesses
14 Altmetric
Metrics details
- Population genetics
- Transcriptomics
Human pluripotent stem (hPS) cells can, in theory, be differentiated into any cell type, making them a powerful in vitro model for human biology. Recent technological advances have facilitated large-scale hPS cell studies that allow investigation of the genetic regulation of molecular phenotypes and their contribution to high-order phenotypes such as human disease. Integrating hPS cells with single-cell sequencing makes identifying context-dependent genetic effects during cell development or upon experimental manipulation possible. Here we discuss how the intersection of stem cell biology, population genetics and cellular genomics can help resolve the functional consequences of human genetic variation. We examine the critical challenges of integrating these fields and approaches to scaling them cost-effectively and practically. We highlight two areas of human biology that can particularly benefit from population-scale hPS cell studies, elucidating mechanisms underlying complex disease risk loci and evaluating relationships between common genetic variation and pharmacotherapeutic phenotypes.
This is a preview of subscription content, access via your institution
Access options
Access Nature and 54 other Nature Portfolio journals
Get Nature+, our best-value online-access subscription
24,99 € / 30 days
cancel any time
Subscribe to this journal
Receive 12 print issues and online access
195,33 € per year
only 16,28 € per issue
Buy this article
- Purchase on Springer Link
- Instant access to full article PDF
Prices may be subject to local taxes which are calculated during checkout
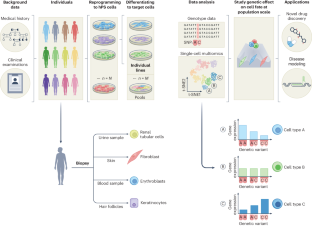
Similar content being viewed by others
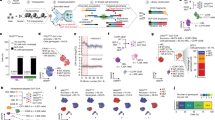
Mapping genotypes to chromatin accessibility profiles in single cells
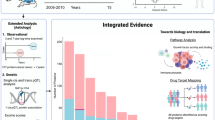
Identifying proteomic risk factors for cancer using prospective and exome analyses of 1463 circulating proteins and risk of 19 cancers in the UK Biobank
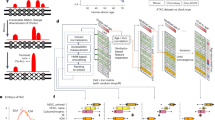
Tracking single-cell evolution using clock-like chromatin accessibility loci
Thomson, J. A. Embryonic stem cell lines derived from human blastocysts. Science https://doi.org/10.1126/science.282.5391.1145 (1998).
Takahashi, K. & Yamanaka, S. Induction of pluripotent stem cells from mouse embryonic and adult fibroblast cultures by defined factors. Cell 126 , 663–676 (2006).
Article CAS PubMed Google Scholar
Takahashi, K. et al. Induction of pluripotent stem cells from adult human fibroblasts by defined factors. Cell 131 , 861–872 (2007).
Liu, G., David, B. T., Trawczynski, M. & Fessler, R. G. Advances in pluripotent stem cells: history, mechanisms, technologies, and applications. Stem Cell Rev. Rep. 16 , 3–32 (2020).
Article PubMed Google Scholar
Efrat, S. Epigenetic memory: lessons from iPS cells derived from human β cells. Front. Endocrinol. 11 , 614234 (2020).
Article Google Scholar
Anderson, R. H. & Francis, K. R. Modeling rare diseases with induced pluripotent stem cell technology. Mol. Cell. Probes 40 , 52–59 (2018).
Article CAS PubMed PubMed Central Google Scholar
Spitalieri, P., Talarico, V. R., Murdocca, M., Novelli, G. & Sangiuolo, F. Human induced pluripotent stem cells for monogenic disease modelling and therapy. World J. Stem Cells 8 , 118–135 (2016).
Article PubMed PubMed Central Google Scholar
Passier, R., Orlova, V. & Mummery, C. Complex tissue and disease modeling using hiPSCs. Cell Stem Cell 18 , 309–321 (2016).
Warren, C. R., Jaquish, C. E. & Cowan, C. A. The NextGen genetic association studies consortium: a foray into in vitro population genetics. Cell Stem Cell 20 , 431–433 (2017).
Visscher, P. M., Brown, M. A., McCarthy, M. I. & Yang, J. Five years of GWAS discovery. Am. J. Hum. Genet. 90 , 7–24 (2012).
Tak, Y. G. & Farnham, P. J. Making sense of GWAS: using epigenomics and genome engineering to understand the functional relevance of SNPs in non-coding regions of the human genome. Epigenetics Chromatin 8 , 57 (2015).
Umans, B. D., Battle, A. & Gilad, Y. Where are the disease-associated eQTLs? Trends Genet. 37 , 109–124 (2021).
Yazar, S. et al. Single-cell eQTL mapping identifies cell type–specific genetic control of autoimmune disease. Science 376 , eabf3041 (2022).
Jerber, J. et al. Population-scale single-cell RNA-seq profiling across dopaminergic neuron differentiation. Nat. Genet. 53 , 304–312 (2021).
Neavin, D. et al. Single cell eQTL analysis identifies cell type-specific genetic control of gene expression in fibroblasts and reprogrammed induced pluripotent stem cells. Genome Biol. 22 , 76 (2021).
Cuomo, A. S. E. et al. Single-cell RNA-sequencing of differentiating iPS cells reveals dynamic genetic effects on gene expression. Nat. Commun. 11 , 810 (2020).
Warren, C. R. et al. Induced pluripotent stem cell differentiation enables functional validation of GWAS variants in metabolic disease. Cell Stem Cell 20 , 547–557 (2017).
Kishore, S. et al. A non-coding disease modifier of pancreatic agenesis identified by genetic correction in a patient-derived iPSC line. Cell Stem Cell 27 , 137–146 (2020).
Magdy, T. et al. RARG variant predictive of doxorubicin-induced cardiotoxicity identifies a cardioprotective therapy. Cell Stem Cell 28 , 2076–2089 (2021).
Bourgeois, S. et al. Towards a functional cure for diabetes using stem cell-derived beta cells: are we there yet? Cells 10 , 191 (2021).
Sharma, A., Sances, S., Workman, M. J. & Svendsen, C. N. Multi-lineage human iPSC-derived platforms for disease modeling and drug discovery. Cell Stem Cell 26 , 309–329 (2020).
Volpato, V. & Webber, C. Addressing variability in iPSC-derived models of human disease: guidelines to promote reproducibility. Dis. Model. Mech. 13 , dmm042317 (2020).
Banovich, N. E. et al. Impact of regulatory variation across human iPSCs and differentiated cells. Genome Res. 28 , 122–131 (2018).
Kilpinen, H. et al. Common genetic variation drives molecular heterogeneity in human iPSCs. Nature 546 , 370–375 (2017).
Panopoulos, A. D. et al. iPSCORE: a resource of 222 iPSC lines enabling functional characterization of genetic variation across a variety of cell types. Stem Cell Rep. 8 , 1086–1100 (2017).
Article CAS Google Scholar
Chen, G., Ning, B. & Shi, T. Single-cell RNA-seq technologies and related computational data analysis. Front. Genet. 10 , 317 (2019).
Elorbany, R. et al. Single-cell sequencing reveals lineage-specific dynamic genetic regulation of gene expression during human cardiomyocyte differentiation. PLoS Genet. 18 , e1009666 (2022).
Ward, M. C., Banovich, N. E., Sarkar, A., Stephens, M. & Gilad, Y. Dynamic effects of genetic variation on gene expression revealed following hypoxic stress in cardiomyocytes. eLife 10 , e57345 (2021).
Shi, Z.-D. et al. Genome editing in hPSCs reveals GATA6 haploinsufficiency and a genetic interaction with GATA4 in human pancreatic development. Cell Stem Cell 20 , 675–688 (2017).
Strober, B. J. et al. Dynamic genetic regulation of gene expression during cellular differentiation. Science 364 , 1287–1290 (2019).
González, F. et al. An iCRISPR platform for rapid, multiplexable, and inducible genome editing in human pluripotent stem cells. Cell Stem Cell 15 , 215–226 (2014).
Barbeira, A. N. et al. Exploiting the GTEx resources to decipher the mechanisms at GWAS loci. Genome Biol. 22 , 49 (2021).
Hamazaki, T., El Rouby, N., Fredette, N. C., Santostefano, K. E. & Terada, N. Concise review: induced pluripotent stem cell research in the era of precision medicine. Stem Cells 35 , 545–550 (2017).
Cuomo, A. S. E. et al. CellRegMap: a statistical framework for mapping context-specific regulatory variants using scRNA-seq. Mol. Syst. Biol. 18 , e10663 (2022).
Cuomo, A. S. E., Nathan, A., Raychaudhuri, S., MacArthur, D. G. & Powell, J. E. Single-cell genomics meets human genetics. Nat. Rev. Genet. 24 , 535–549 (2023).
Mirauta, B. A. et al. Population-scale proteome variation in human induced pluripotent stem cells. eLife 9 , e57390 (2020).
Findley, A. S. et al. Functional dynamic genetic effects on gene regulation are specific to particular cell types and environmental conditions. eLife 10 , e67077 (2021).
Kimura, M. et al. En masse organoid phenotyping informs metabolic-associated genetic susceptibility to NASH. Cell https://doi.org/10.1016/j.cell.2022.09.031 (2022).
Llufrio, E. M., Wang, L., Naser, F. J. & Patti, G. J. Sorting cells alters their redox state and cellular metabolome. Redox Biol. 16 , 381–387 (2018).
Shen, S. et al. Integrating single-cell genomics pipelines to discover mechanisms of stem cell differentiation. Trends Mol. Med. https://doi.org/10.1016/j.molmed.2021.09.006 (2021).
van der Wijst, M. et al. The single-cell eQTLGen consortium. eLife 9 , e52155 (2020).
Soskic, B. et al. Immune disease risk variants regulate gene expression dynamics during CD4 + T cell activation. Nat. Genet. 54 , 817–826 (2022).
Daniszewski, M. et al. Retinal ganglion cell-specific genetic regulation in primary open-angle glaucoma. Cell Genomics 2 , 100142 (2022).
Senabouth, A. et al. Transcriptomic and proteomic retinal pigment epithelium signatures of age-related macular degeneration. Nat. Commun. 13 , 4233 (2022).
Benaglio, P. et al. Mapping genetic effects on cell type-specific chromatin accessibility and annotating complex immune trait variants using single nucleus ATAC-seq in peripheral blood. PLoS Genet. 19 , e1010759 (2023).
Baysoy, A., Bai, Z., Satija, R. & Fan, R. The technological landscape and applications of single-cell multi-omics. Nat. Rev. Mol. Cell Biol. 24 , 695–713 (2023).
Weinshilboum, R. M. & Wang, L. Pharmacogenomics: precision medicine and drug response. Mayo Clin. Proc. 92 , 1711–1722 (2017).
Pirmohamed, M. Personalized pharmacogenomics: predicting efficacy and adverse drug reactions. Annu. Rev. Genom. Hum. Genet. 15 , 349–370 (2014).
Nelson, M. R. et al. The support of human genetic evidence for approved drug indications. Nat. Genet. 47 , 856–860 (2015).
Hay, M., Thomas, D. W., Craighead, J. L., Economides, C. & Rosenthal, J. Clinical development success rates for investigational drugs. Nat. Biotechnol. 32 , 40–51 (2014).
Holmgren, G. et al. Long-term chronic toxicity testing using human pluripotent stem cell-derived hepatocytes. Drug Metab. Dispos. 42 , 1401–1406 (2014).
Kim, J.-H., Kang, M., Jung, J.-H., Lee, S.-J. & Hong, S.-H. Human pluripotent stem cell-derived alveolar epithelial cells as a tool to assess cytotoxicity of particulate matter and cigarette smoke extract. Dev. Reprod. 26 , 155–163 (2022).
Sharma, A. et al. High-throughput screening of tyrosine kinase inhibitor cardiotoxicity with human induced pluripotent stem cells. Sci. Transl. Med. 9 , eaaf2584 (2017).
Han, Y. et al. Identification of SARS-CoV-2 inhibitors using lung and colonic organoids. Nature 589 , 270–275 (2021).
Lam, C. K. & Wu, J. C. Clinical trial in a dish: using patient-derived induced pluripotent stem cells to identify risks of drug-induced cardiotoxicity. Arterioscler. Thromb. Vasc. Biol. 41 , 1019–1031 (2021).
Iwata, R. et al. Mitochondria metabolism sets the species-specific tempo of neuronal development. Science 379 , eabn4705 (2023).
Miller, J. D. et al. Human iPSC-based modeling of late-onset disease via progerin-induced aging. Cell Stem Cell 13 , 691–705 (2013).
Hergenreder, E. et al. Combined small-molecule treatment accelerates maturation of human pluripotent stem cell-derived neurons. Nat. Biotechnol. https://doi.org/10.1038/s41587-023-02031-z (2024).
Fowler, J. L., Ang, L. T. & Loh, K. M. A critical look: challenges in differentiating human pluripotent stem cells into desired cell types and organoids. Wiley Interdiscip. Rev. Dev. Biol. 9 , e368 (2020).
Jiang, S., Feng, W., Chang, C. & Li, G. Modeling human heart development and congenital defects using organoids: how close are we? J. Cardiovasc. Dev. Dis. 9 , 125 (2022).
CAS PubMed PubMed Central Google Scholar
Tremmel, D. M. et al. Validating expression of beta cell maturation-associated genes in human pancreas development. Front. Cell Dev. Biol. 11 , 1103719 (2023).
Washer, S. J. et al. Single-cell transcriptomics defines an improved, validated monoculture protocol for differentiation of human iPSC to microglia. Sci. Rep. 12 , 19454 (2022).
Wolf, F. A., Angerer, P. & Theis, F. J. SCANPY: large-scale single-cell gene expression data analysis. Genome Biol. 19 , 15 (2018).
Wilson, S. B. et al. DevKidCC allows for robust classification and direct comparisons of kidney organoid datasets. Genome Med. 14 , 19 (2022).
Subramanian, A. et al. Single cell census of human kidney organoids shows reproducibility and diminished off-target cells after transplantation. Nat. Commun. 10 , 5462 (2019).
Kammers, K. et al. Gene and protein expression in human megakaryocytes derived from induced pluripotent stem cells. J. Thromb. Haemost. 19 , 1783–1799 (2021).
De Sousa, P. A. et al. Rapid establishment of the European Bank for induced Pluripotent Stem Cells (EBiSC)—the Hot Start experience. Stem Cell Res. 20 , 105–114 (2017).
Morrison, M. et al. StemBANCC: governing access to material and data in a large stem cell research consortium. Stem Cell Rev. Rep. 11 , 681–687 (2015).
The GTEx Consortium The GTEx Consortium atlas of genetic regulatory effects across human tissues. Science 369 , 1318–1330 (2020).
Article PubMed Central Google Scholar
Mitchell, J. M., Nemesh, J., Ghosh, S. & Handsaker, R. E. Mapping genetic effects on cellular phenotypes with ‘cell villages’. Preprint at bioRxiv https://doi.org/10.1101/2020.06.29.174383 (2020).
Neavin, D. R. et al. A village in a dish model system for population-scale hiPSC studies. Nat. Commun. 14 , 3240 (2023).
Kang, H. M. et al. Multiplexed droplet single-cell RNA-sequencing using natural genetic variation. Nat. Biotechnol. 36 , 89–94 (2018).
Wells, M. F. et al. Natural variation in gene expression and viral susceptibility revealed by neural progenitor cell villages. Cell Stem Cell 30 , 312–332 (2023).
Neavin, D. et al. Demuxafy : improvement in droplet assignment by integrating multiple single-cell demultiplexing and doublet detection methods. Genome Biol. 25 , 94 (2024).
Xu, J. et al. Genotype-free demultiplexing of pooled single-cell RNA-seq. Genome Biol. 20 , 290 (2019).
Heaton, H. et al. Souporcell: robust clustering of single-cell RNA-seq data by genotype without reference genotypes. Nat. Methods 17 , 615–620 (2020).
Huang, Y., McCarthy, D. J. & Stegle, O. Vireo: Bayesian demultiplexing of pooled single-cell RNA-seq data without genotype reference. Genome Biol. 20 , 273 (2019).
Hindson, B. J. et al. High-throughput droplet digital PCR system for absolute quantitation of DNA copy number. Anal. Chem. 83 , 8604–8610 (2011).
Dong, X. et al. powerEQTL: an R package and shiny application for sample size and power calculation of bulk tissue and single-cell eQTL analysis. Bioinformatics https://doi.org/10.1093/bioinformatics/btab385 (2021).
Schmid, K. T. et al. scPower accelerates and optimizes the design of multi-sample single cell transcriptomic studies. Nat. Commun. 12 , 6625 (2021).
Camp, J. G., Platt, R. & Treutlein, B. Mapping human cell phenotypes to genotypes with single-cell genomics. Science 365 , 1401–1405 (2019).
Datlinger, P. et al. Pooled CRISPR screening with single-cell transcriptome readout. Nat. Methods 14 , 297–301 (2017).
Dixit, A. et al. Perturb-Seq: dissecting molecular circuits with scalable single-cell RNA profiling of pooled genetic screens. Cell 167 , 1853–1866 (2016).
Rubin, A. J. et al. Coupled single-cell CRISPR screening and epigenomic profiling reveals causal gene regulatory networks. Cell 176 , 361–376 (2019).
Schraivogel, D. et al. Targeted Perturb-seq enables genome-scale genetic screens in single cells. Nat. Methods 17 , 629–635 (2020).
Download references
Acknowledgements
Figures were generated with BioRender.com and further developed by A. Garcia, a scientific illustrator from Bio-Graphics. This research was supported by a National Health and Medical Research Council (NHMRC) Investigator grant (J.E.P., 1175781), research grants from the Australian Research Council (ARC) Special Research Initiative in Stem Cell Science, an ARC Discovery Project (190100825), an EMBO Postdoctoral Fellowship (A.S.E.C.) and an Aligning Science Across Parkinson’s Grant (J.E.P., N.F., D.R.N. and L.S.). J.E.P. is supported by a Fok Family Fellowship.
Author information
These authors contributed equally: Nona Farbehi, Drew R. Neavin.
Authors and Affiliations
Garvan Weizmann Center for Cellular Genomics, Garvan Institute of Medical Research, Sydney, New South Wales, Australia
Nona Farbehi, Drew R. Neavin, Anna S. E. Cuomo & Joseph E. Powell
Graduate School of Biomedical Engineering, University of New South Wales, Sydney, New South Wales, Australia
Nona Farbehi
Aligning Science Across Parkinson’s Collaborative Research Network, Chevy Chase, MD, USA
Nona Farbehi, Lorenz Studer & Joseph E. Powell
Centre for Population Genomics, Garvan Institute of Medical Research, University of New South Wales, Sydney, New South Wales, Australia
Anna S. E. Cuomo & Daniel G. MacArthur
The Center for Stem Cell Biology and Developmental Biology Program, Sloan-Kettering Institute for Cancer Research, New York, NY, USA
Lorenz Studer
Centre for Population Genomics, Murdoch Children’s Research Institute, Melbourne, Victoria, Australia
Daniel G. MacArthur
UNSW Cellular Genomics Futures Institute, University of New South Wales, Sydney, New South Wales, Australia
Joseph E. Powell
You can also search for this author in PubMed Google Scholar
Contributions
All authors conceived the topic and wrote and revised the manuscript.
Corresponding author
Correspondence to Joseph E. Powell .
Ethics declarations
Competing interests.
D.G.M. is a founder with equity in Goldfinch Bio, is a paid advisor to GSK, Insitro, Third Rock Ventures and Foresite Labs, and has received research support from AbbVie, Astellas, Biogen, BioMarin, Eisai, Merck, Pfizer and Sanofi-Genzyme; none of these activities is related to the work presented here. J.E.P. is a founder with equity in Celltellus Laboratory and has received research support from Illumina. The other authors declare no conflict of interest.
Peer review
Peer review information.
Nature Genetics thanks Kelly Frazer, Gosia Trynka and the other, anonymous, reviewer(s) for their contribution to the peer review of this work.
Additional information
Publisher’s note Springer Nature remains neutral with regard to jurisdictional claims in published maps and institutional affiliations.
Supplementary information
Supplementary information.
Supplementary Table 1.
Rights and permissions
Springer Nature or its licensor (e.g. a society or other partner) holds exclusive rights to this article under a publishing agreement with the author(s) or other rightsholder(s); author self-archiving of the accepted manuscript version of this article is solely governed by the terms of such publishing agreement and applicable law.
Reprints and permissions
About this article
Cite this article.
Farbehi, N., Neavin, D.R., Cuomo, A.S.E. et al. Integrating population genetics, stem cell biology and cellular genomics to study complex human diseases. Nat Genet 56 , 758–766 (2024). https://doi.org/10.1038/s41588-024-01731-9
Download citation
Received : 24 January 2023
Accepted : 20 March 2024
Published : 13 May 2024
Issue Date : May 2024
DOI : https://doi.org/10.1038/s41588-024-01731-9
Share this article
Anyone you share the following link with will be able to read this content:
Sorry, a shareable link is not currently available for this article.
Provided by the Springer Nature SharedIt content-sharing initiative
Quick links
- Explore articles by subject
- Guide to authors
- Editorial policies
Sign up for the Nature Briefing newsletter — what matters in science, free to your inbox daily.

When & How to Solve Problems with Genetic Algorithms
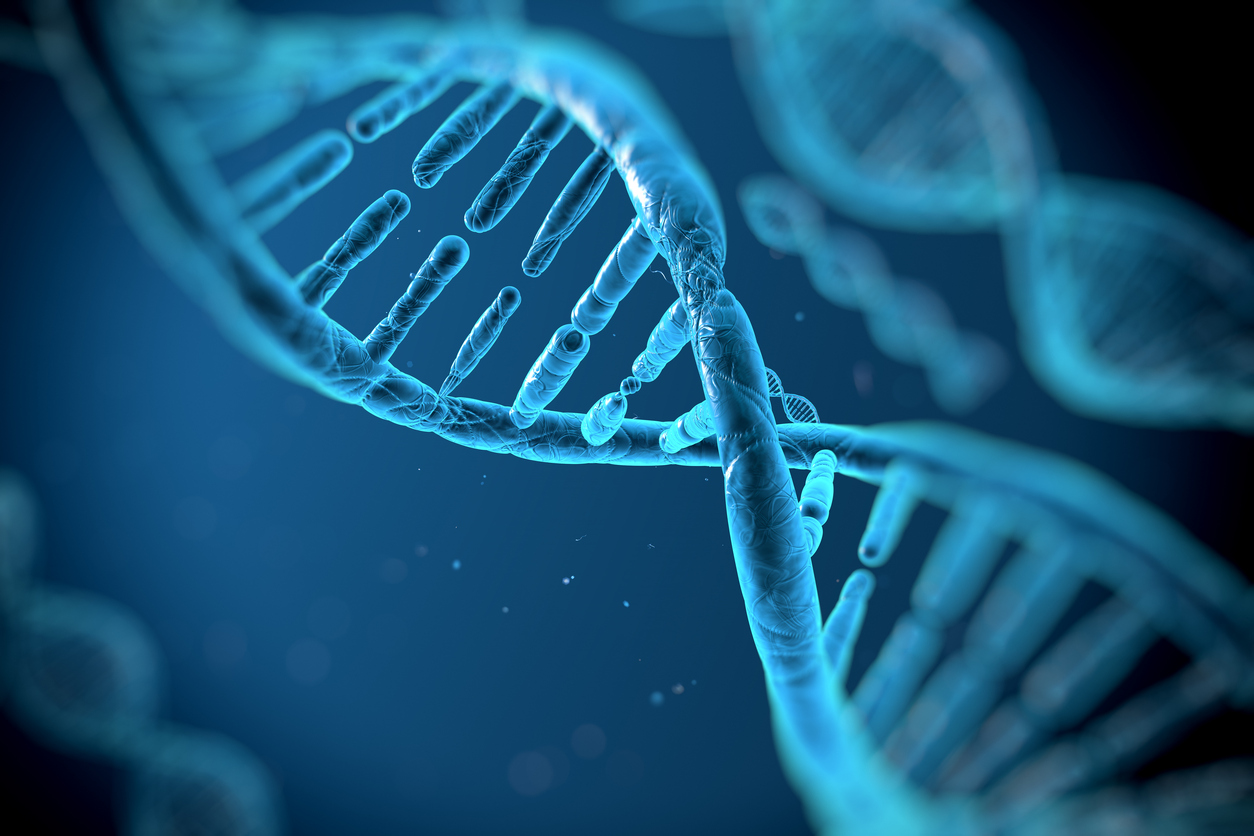
Article summary
Basic steps, when to use genetic algorithms.
- For Example...
Genetic algorithms are a class of algorithms designed to explore a large search space and find optimal solutions by mimicking evolution and natural selection. Potential solutions are randomly found, evaluated, and bred with one another in hopes of producing better solutions.
The process of using genetic algorithms goes like this:
- Determine the problem and goal
- Break down the solution to bite-sized properties (genomes)
- Build a population by randomizing said properties
- Evaluate each unit in the population
- Selectively breed (pick genomes from each parent)
- Rinse and repeat
GAs are not good for all kinds of problems. They’re best for problems where there is a clear way to evaluate fitness. If your search space is not well constrained or your evaluation process is computationally expensive, GAs may not find solutions in a sane amount of time. In my experience, they’re most helpful when there is a decent algorithm in place, but the “knobs” just need to be tweaked.
For Example…
On a recent project, we had an algorithm that we knew would work, but we needed to find the best possible settings for the tune-able parameters.
We were using pressure data from inside a testing lung to parse out breath waves and calculate derived values such as breath rate, tidal volumes, and peak pressures. We had many recordings of pressure data and knew the expected results. The goal was simple: find the set of variables that would get us as close as possible to the expected results. Our properties were a handful of settings for our existing algorithm: low/high thresholds, smoothing factors, averaging window sizes, etc.
A simpler illustration
To walk through how it works, let’s use something a little simpler: a cannon.
Like the old Cannon Fodder game, we have a cannon that has two variables: powder and elevation. Our goal is to shoot as far as possible. (Let’s pretend we don’t know about physics and don’t know that 45 degrees is the answer.)
Our goal : max distance Our genomes : powder (0-100) P and elevation (-90-90 degrees) E
Initial population
We start by generating a sample population and evaluating its members. Normally, this sample would be much larger, but for our example, we’ll keep it small:
Given these results, we can use an elitist strategy to select the top X percent of the population to “reproduce.” Once selected, we use crossover/recombination to blend the best of the population.
Crossover and mutation
Our “Elites” include:
You may use more than two “parents,” but to keep things simple, I’ll just use two. We mix and match values from the parents (just like in biology). We also mutate a percentage of the values to introduce some randomness into our genomes.
The amount of mutation can greatly affect your results and should be tweaked based on your domain and the problem you are trying to solve. To keep our gene pool from becoming too focused, we’ll also include a couple of non-elites from the previous generation.
After crossover:
With non-elites for diversity:
After mutation:
Keeping the non-elites and mutating some of the values will keep us from reaching local optima.
Are we done yet?
Now, we just repeat this process until we’re done. But, how do we know we’re done? GAs are usually terminated by:
- Fixed number of generations
- Fixed amount of processing time
- Optimal/good enough solution is found
- Good ol’ manual killing
For our example here, the algorithm will trend toward a full powder shot at 45 degrees, and we’ll have our clear winner.
Depending on runtime, problem, and domain, any of these terminators are acceptable. For our breath parsing from pressure samples, we used a manual intervention. If possible, I recommend saving off your populations so you can resume long-running simulations.
In the end, using a GA helped us get our algorithm within tolerances fairly quickly. When algorithms changed or new genomes were discovered, we simply added the genome and started over again with a few of our saved elite values. GAs aren’t a perfect fit for a lot of problems, but they’re definitely a fun and interesting tool to have in the toolbox.
Related Posts
Embracing mainline development: beyond feature branches, chatgpt and the value of a computer science education, inspired by nature: an introduction to genetic algorithms, keep up with our latest posts..
We’ll send our latest tips, learnings, and case studies from the Atomic braintrust on a monthly basis.
Tell Us About Your Project
We’d love to talk with you about your next great software project. Fill out this form and we’ll get back to you within two business days.
Artificial Intelligence—Genetic Algorithm
- First Online: 19 May 2024
Cite this chapter
- Wei Weng 2
In the realm of artificial intelligence (AI), genetic algorithm (GA) is a powerful problem-solving technique rooted in the principles of natural evolution. This chapter equips learners with the understanding and tools to use GA in addressing complex challenges. The chapter opens by highlighting the types of problems for which this evolutionary approach is particularly adept. Venturing into the anatomy of GA, the chapter presents the detailed flow of GA that begins with initial population creation and goes through evaluation, selection, crossover, and mutation. It delves into the mechanics and details of each step, offering insights into how GA mimics the process of natural evolution to converge toward optimal solutions. Detailed examples illustrate step by step how GA is implemented to solve a complex problem. The chapter concludes with an engaging exercise that invites learners to apply their newfound knowledge to solve a problem. This hands-on opportunity will empower learners to transcend theory and experiment with application. By mastering GA, learners will gain the ability to navigate the evolutionary landscape of AI.
This is a preview of subscription content, log in via an institution to check access.
Access this chapter
- Available as PDF
- Read on any device
- Instant download
- Own it forever
- Available as EPUB and PDF
- Compact, lightweight edition
- Dispatched in 3 to 5 business days
- Free shipping worldwide - see info
Tax calculation will be finalised at checkout
Purchases are for personal use only
Institutional subscriptions
Mitchell M (1998) An introduction to genetic algorithms. MIT Press, Cambridge, Massachusetts, US
Book Google Scholar
Obitko M (1998) Introduction to genetic algorithms. https://www.obitko.com/tutorials/genetic-algorithms/about.php
Download references
Author information
Authors and affiliations.
Institute of Liberal Arts and Science, Kanazawa University, Kanazawa, Ishikawa, Japan
You can also search for this author in PubMed Google Scholar
Corresponding author
Correspondence to Wei Weng .
Rights and permissions
Reprints and permissions
Copyright information
© 2024 The Author(s), under exclusive license to Springer Nature Singapore Pte Ltd.
About this chapter
Weng, W. (2024). Artificial Intelligence—Genetic Algorithm. In: A Beginner’s Guide to Informatics and Artificial Intelligence. Springer, Singapore. https://doi.org/10.1007/978-981-97-1477-3_5
Download citation
DOI : https://doi.org/10.1007/978-981-97-1477-3_5
Published : 19 May 2024
Publisher Name : Springer, Singapore
Print ISBN : 978-981-97-1476-6
Online ISBN : 978-981-97-1477-3
eBook Packages : Computer Science Computer Science (R0)
Share this chapter
Anyone you share the following link with will be able to read this content:
Sorry, a shareable link is not currently available for this article.
Provided by the Springer Nature SharedIt content-sharing initiative
- Publish with us
Policies and ethics
- Find a journal
- Track your research
Subscribe or renew today
Every print subscription comes with full digital access
Science News
Why using genetic genealogy to solve crimes could pose problems.
Some worry that authorities could violate people’s rights using the method

DNA DETECTION Police are using DNA in new ways in crime investigations: to probe family trees in public genealogy databases for suspects. The technique, called genetic genealogy, raises privacy concerns.
Couperfield/Shutterstock
Share this:
By Tina Hesman Saey
June 7, 2018 at 2:00 pm
Police are using a new type of DNA sleuthing, called genetic genealogy. Already the technique has caught murder and rape suspects in California and Washington. While solving the cases has given cause for celebration, the tactics used in catching the alleged culprits have many privacy and civil rights experts worried.
Closing the Golden State Killer case ( SN Online: 4/29/18 ) and the previously unsolved double murder of a young Canadian couple ( SN Online: 5/23/18 ) involved probing a public online database of people’s DNA and family-tree information called GEDmatch.
In a May 29 opinion piece published in the Annals of Internal Medicine, bioethicist Christine Grady and colleagues argue that police should be more transparent about how they use forensic DNA searches . Meanwhile, law professor Natalie Ram and colleagues go even further in an essay in the June 8 Science , writing that eroding limits on the use of crime-solving technology “threatens our collective civil liberties and opens the door to socially and politically unacceptable genetic surveillance.”
Here are a few key points in the debate:
Why are police using GEDmatch instead of DNA testing companies?
If police wanted to use 23andMe or AncestryDNA to help solve crimes, they would need a clean saliva sample from a potential suspect to send to the company for testing and analysis. But crime scene DNA doesn’t come in that form, so police can’t send DNA to the companies for testing. That’s not the case with the DNA analysis service GEDmatch, to which customers can upload raw DNA data received from testing companies.
“That means the police or law enforcement investigators are equally able, if they have enough DNA to create a sufficiently complete genetic sequence, to upload that to GEDmatch” to find potential suspects, says Ram, of the University of Baltimore School of Law.
Companies like 23andMe and Ancestry also require consent from the person the DNA belongs to before the companies will do testing. That’s, obviously, not possible with crime scene DNA. However, GEDmatch in May changed its terms of service “to explicitly embrace the use of their service by law enforcement,” Ram says.
Could these searches lead to people being investigated needlessly?
Before police tracked the Golden State Killer suspect through GEDmatch, investigators subpoenaed Family Tree DNA for information about a customer whose Y chromosome partially matched DNA from one of the crime scenes. Police then used that information to order a man in an Oregon nursing home to give a DNA sample. He was not a match.
“Just having DNA match something at the crime scene doesn’t mean the person committed the crime,” says Grady, who heads the National Institutes of Health Clinical Center’s Department of Bioethics in Bethesda, Md. “It just means that they were there, or something that they used was there.” Police still have to prove the suspect committed the crime.
If DNA tests are helping to catch criminals, shouldn’t we be all for it?
“Catching criminals is great,” Ram says. “But privacy is also important. Police could solve more crime if they, for instance, could go rifle through anybody’s home at any time for no reason.” But such searches are illegal, because they violate people’s rights to privacy and security against unreasonable search and seizure.
Some people have suggested this type of search could be limited to solving very serious crimes and cold cases. But such limits have been tried before, Ram notes. Over the past decade, some U.S. states have begun allowing the use of police DNA databases for “familial searches.” Such searches may implicate close relatives of people in the police database as suspects in a crime. “States that embraced that technology initially said, ‘we’re only going to use this for really, really serious crimes.’ ” But then Colorado in 2009 convicted its first suspect with this technique — for “a burglary where someone broke into a car and stole about a dollar and a half in change,” Ram says.
Many people say DNA data should be treated differently than other personal information. “There are things that are unique about genetic data. Most importantly, that it has implications for others,” Grady says. People strive to protect medical information, but medical records reveal things about only an individual. DNA reveals things about relatives, too.
“If I give up my genetic data, you can learn things about people who are related to me that you can’t learn from my cholesterol or my blood pressure, or even my psych history,” Grady says. There should be some safeguards, she adds. “No law enforcement agencies have rules or standards about how they use this kind of information.”
Do any laws protect against this type of search?
Because GEDmatch is a public database, privacy protections that govern private companies probably don’t apply. “If people voluntarily put their data on GEDmatch, they’ve given it away,” Grady says. It’s like they’ve cut their hair and left the hair on the floor. There’s no protection for that.” Other laws governing the privacy of genetic and medical information don’t apply genealogical DNA data deposited in public databases.
But Grady believes that could soon change. “I suspect there will be people entertaining new laws in light of the recent uses.”
More Stories from Science News on Genetics
Here’s why some pigeons do backflips.

A genetic parasite may explain why humans and other apes lack tails

Ancient viruses helped speedy nerves evolve

Newfound immune cells are responsible for long-lasting allergies

Geneticist Krystal Tsosie advocates for Indigenous data sovereignty

How ancient herders rewrote northern Europeans’ genetic story

Fetuses make a protein that causes morning sickness in pregnancy

Why Huntington’s disease may take so long to develop
Subscribers, enter your e-mail address for full access to the Science News archives and digital editions.
Not a subscriber? Become one now .
Opinion | Letters: Country’s problems can’t be solved…
Share this:.
- Click to share on Facebook (Opens in new window)
- Click to share on X (Opens in new window)
Daily e-Edition
Evening e-Edition
- Readers React
Breaking News
Opinion | lehigh county man fatally shot wife, then himself, coroner says, opinion | letters: country’s problems can’t be solved through the use of force.

Criminal history wasn’t needed in report on couple’s death
An elderly couple were fatally hit by a car Friday night and their names were not released until Tuesday’s edition of local news. Was it really necessary to say that the victim was found guilty of a crime in 2008 and prior to that in 1990? What does that have to do with the accident? How about if they have any family and this is what is mentioned about the accident?
Denise Williams
Lower Macungie Township
Sheer force not the answer to solving country’s problems
A recent letter to the editor recounted a list of problems the nation faces and suggested they’re all the result of weak leadership. But the alternative, Donald Trump, though he has a forceful personality, knows very little about how anything in government works. If you need confirmation of the last part of that sentence, read the commentaries of some of his top advisers when he was president — Rex Tillerson, John Kelly, John Bolton, William Barr, etc. — and about the difficulty they had getting him to understand any kind of complexity, or even some fairly basic principles. A plumber or electrician, faced with a complex problem, wouldn’t get very far trying to solve it with strength alone; he would need to understand what’s going on. The problems facing the nation today won’t be helped by a sledgehammer. I’ll take Biden any day of the week over the alternative. I haven’t been happy with every one of his moves — there’s never been a politician about whom I could say that — but he has several landmark achievements in his presidency already, and he’s worked credibly, if incrementally, on most of the issues before us now.
Quality is important emphasis in Rick Anderson interview
Some thoughts on Rick Anderson’s two-hour interview, “St. Luke’s CEO talks local health care” (April 21). Anderson is to be congratulated on his nearly 39-year run as St. Luke’s CEO. He successfully navigated many turbulent waters, and is in good company: Warren Buffett chaired Berkshire-Hathaway for over 50 years and Thomas Watson chaired IBM for 42 years.
I like Anderson’s recurring emphasis on quality — highly important in health care — although the article said nothing about the metrics St. Luke’s uses to measure quality. Nor was the managerial role of physicians at St. Luke’s addressed (it is physicians who swear to uphold the Hippocratic oath).
Physicians’ private entrepreneurial practices are gradually being absorbed by hospital networks and doctors become employees. To what extent are professional managers and bean counters supplanting physicians’ expertise in health care decisions?
The planned merger/acquisition of Jefferson Health in Philadelphia and Lehigh Valley Health Network reminds me of the Philadelphia Electric Co. (PECO) proposal to take over our local PPL electric utility some 20 years ago. Public concern in the Lehigh Valley eventually put the kibosh on that proposal.
I wish Mr. Anderson well and hope that his “quality” theme continues to improve health care outcomes and strengthens physicians’ role in St. Luke’s health care decisions.
James Largay
Upper Saucon Township
Help pay decent wage for child care workers
Quality child care teachers are a primary component of the quality of a child care program. My children love their teacher and that relationship is central to fostering their love for learning. I’m one of the fortunate families who are able to have their children in high quality child care. Unfortunately, in Pennsylvania less than half of children in child care are enrolled in a high quality program.
Thousands of families are struggling to find child care because of the staffing crisis. Many classrooms are closed and waitlists are the norm. In fact, Sonya Sue’s Daycare, where my children go, has multiple children on the waiting list. Child care programs are simply unable to compete with rising wages and benefits now being offered by companies requiring less specialized skill.
To ensure my children and children across Pennsylvania are able to find the high-quality care and education they deserve, our lawmakers must prioritize funding to help programs pay teachers a livable wage.
Samantha Shive
Stuttering Foundation has resources available
May 12-18 is National Stuttering Awareness Week. One in every 100 people stutter. That’s 80 million people worldwide. Yet stuttering is often misunderstood and even laughed at.
To address the many myths surrounding this complex disorder, the Stuttering Foundation has compiled a list of “75 Must-Use Resources for the Stuttering Community” — available for free on at StutteringHelp.org.
The foundation’s most popular content represents a mix of time-tested favorites and newer innovations:
* Drawings and letters from kids featuring submissions from children around the world and published in our magazine
* “Answers for Employers” brochure answering frequent questions from employers received by the foundation each year
* “Self-Therapy for the Stutterer” e-book written by founder Malcolm Fraser in 1978
* List of famous people who stutter continuously updated with many celebrities, sports heroes, writers and world leaders.
* A podcast, now in its sixth season with more than 100,000 listeners.
* “Advice to Those Who Stutter” audio book, drafted in 1972 and updated by 28 therapists who stutter themselves.
* I Stutter ID card, helping people identify themselves in a nonverbal way.
All resources are available at StutteringHelp.org.
Jane Fraser
The writer is the president of The Stuttering Foundation.
Mackenzie’s help earned him a vote for Congress
On Nov. 5, Ryan Mackenzie will have my vote for U.S. Congress. Like most people, I didn’t used to think a lot about identity theft. It was something I’d heard about, but I never thought it would happen to me. Then, like millions of Americans, I discovered that I had become a victim. This was a scary time for me. When I got a bill for an account that was opened fraudulently, it couldn’t be canceled. I wasn’t sure where to turn, so I tried the offices of Susan Wild and some of my other representatives. Unfortunately, no one seemed to know how to help me, and I felt alone and frustrated. That’s when I ran into state Rep. Ryan Mackenzie at a local event. He wasn’t even my state representative, but when I mentioned my problem, he promised to take care of it. About a week later, everything was taken care of, and I am thankful to him and his office for helping me. I’ll always be grateful to Ryan Mackenzie for stepping up to help when no one else would. That’s why he will have my vote for U.S. Congress.
Mona Chibber
Whitehall Township
The Morning Call encourages community dialogue on important issues. Submit a letter to the editor at [email protected] .
More in Opinion

SUBSCRIBER ONLY
Opinion | browse political cartoons for the week of may 20.

Opinion | Your View: Fixing the way Washington regulates manufacturers would benefit them and the Lehigh Valley

Opinion | Letters to the Editor: Alley houses plan for Bethlehem will create more problems than it will solve

Opinion | Your View: Honor the efforts of women, Black Americans during World War II
- Skip to main content
- Keyboard shortcuts for audio player
TED Radio Hour
- Subscribe to Breaking News Alerts
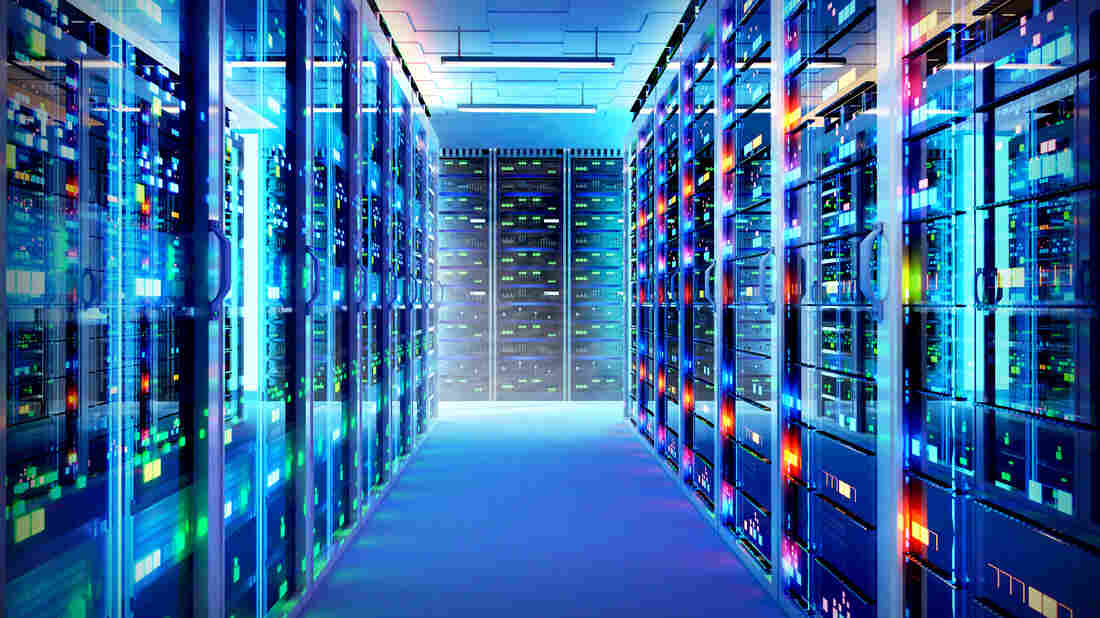
Our tech has a climate problem: How we solve it
AI, EVs and satellites can help fight the climate crisis. But they, too, have an environmental cost. This hour, TED speakers examine how we can use each innovation without making the problem worse.
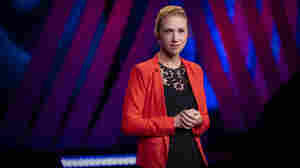
ChatGPT vs. the climate: The hidden environmental costs of AI
by Manoush Zomorodi , James Delahoussaye , Sanaz Meshkinpour
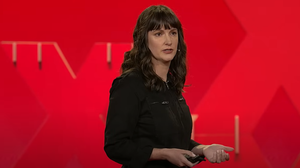
Short-term loss for long-term gain? The ethical dilemma at the heart of EVs
by Manoush Zomorodi , Matthew Cloutier , Sanaz Meshkinpour , Kirk Siegler

Satellites can monitor climate emissions... but space junk puts them at risk
by Manoush Zomorodi , Harsha Nahata , Sanaz Meshkinpour
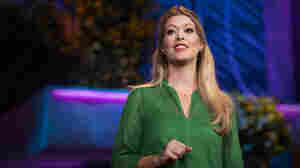
Wind energy can be unpredictable. AI can help
by Manoush Zomorodi , Rachel Faulkner White , Sanaz Meshkinpour
- See TED Radio Hour sponsors and promo codes

IMAGES
VIDEO
COMMENTS
Solutions to Genetics Problems This chapter is much more than a solution set for the genetics problems. Here you will find details concerning the assumptions made, the approaches taken, the predictions that are reasonable, and strategies that you can use to solve any genetics problem. The value of this chapter depends on you.
Management may include more frequent cancer screening or preventive (prophylactic) surgery to remove the tissues at highest risk of becoming cancerous. Genetic disorders may cause such severe health problems that they are incompatible with life. In the most severe cases, these conditions may cause a miscarriage of an affected embryo or fetus.
The Punnett square is a valuable tool, but it's not ideal for every genetics problem. For instance, suppose you were asked to calculate the frequency of the recessive class not for an Aa x Aa cross, not for an AaBb x AaBb cross, but for an AaBbCcDdEe x AaBbCcDdEe cross. If you wanted to solve that question using a Punnett square, you could do it - but you'd need to complete a Punnett square ...
When solving a genetics problem, you are calculating probabilities. The probability of a particular event is the "chance" that event will occur. It's a prediction. Probabilities are expressed as decimals. Probability values range from 0 to 1.0. A probability of 1.0 is a certainty - it's equivalent to a chance of 100%.
Solving Genetic ProblemsWhat is a Genetic Problem?A genetic problem is a type examination question that involves both a knowledge of Mendel's experiments, an...
Two rules of probability are used in solving genetics problems: the rule of multiplication and the rule of addition. ... One approach is to ask the class if they would use elephants to study genetics. The disadvantages of using elephants actually highlight the advantages of using peas, corn, fruit flies, or mice for genetics studies: short life ...
In some cases, the answer is yes. Genes that are sufficiently close together on a chromosome will tend to "stick together," and the versions (alleles) of those genes that are together on a chromosome will tend to be inherited as a pair more often than not. This phenomenon is called genetic linkage.
Abstract. Problem solving is an integral part of doing science, yet it is challenging for students in many disciplines to learn. We explored student success in solving genetics problems in several genetics content areas using sets of three consecutive questions for each content area. To promote improvement, we provided students the choice to ...
Problem solving is a critical skill in many disciplines but is often a challenge for students to learn. To examine the processes both students and experts undertake to solve constructed-response problems in genetics, we collected the written step-by-step procedures individuals used to solve problems in four different content areas.
The Punnett square is a diagram that is used to predict an outcome of a particular cross or breeding experiment. It is named after Reginald C. Punnett, who d...
Mendel's work suggested that just two alleles existed for each gene. Today, we know that's not always, or even usually, the case! Although individual humans (and all diploid organisms) can only have two alleles for a given gene, multiple alleles may exist in a population level, and different individuals in the population may have different pairs of these alleles.
The Hardy-Weinberg equation is. p 2 + 2 p q + q 2 = 1. p^2 + 2pq + q^2 =1 p2 +2pq +q2 = 1 and is used to determine the frequency of genotypes in a given population. To solve for the frequency of a specific genotype, start by replacing the appropriate terms with the known values you've been given. From there, it's Algebra 101.
Genetic disorders are health problems that happen because of some type of abnormality in a person's genetic material. There are several types of genetic disorders. In some cases, a genetic change in a single gene can cause someone to have a disease or condition. In other cases, the gene does not have a genetic change, but a person has more or ...
BSC 2012. BSC 2011. MENDELIAN GENETICS PROBLEMS. The following problems are provided to develop your skill and test your understanding of solving problems in the patterns of inheritance. They will be most helpful if you solve them on your own. However, you should seek help if you find you cannot answer a problem.
The product rule. One probability rule that's very useful in genetics is the product rule, which states that the probability of two (or more) independent events occurring together can be calculated by multiplying the individual probabilities of the events. For example, if you roll a six-sided die once, you have a 1/6 chance of getting a six.
solving "word problems." Several years ago I developed a format to help students work though solving a "word problem." The format has proven to be very helpful. The format involves setting up a chart and filling in the "genetics shorthand" as one reads the question. I also have formats for solving chi square problems, mapping/gene ...
And many researchers are investigating the use of CRISPR/Cas gene-editing technology as a new method of controlling antibiotic-resistant microorganisms. 3. Cleaning up pollution. Glacial records have shown us that, as long as 2,500 years ago, roman-era metal production was a source of global pollution.
In this article, I am going to explain how genetic algorithm (GA) works by solving a very simple optimization problem. The idea of this note is to understand the concept of the algorithm by solving an optimization problem step by step. Let us estimate the optimal values of a and b using GA which satisfy below expression.
Solving non-linear problems with genetic algorithms (Part 2) In this series of articles, discover how genetic algorithms (also called evolutionary algorithms) can help you solve… towardsdatascience.com
hPS cells have primarily been used to study rare diseases 6,7, most of which have been associated with a few genetic loci, typically located in protein-coding genome regions. hPS cells have been ...
Genetic algorithms are often applied as an approach to solve global optimization problems. As a general rule of thumb genetic algorithms might be useful in problem domains that have a complex fitness landscape as mixing, i.e., mutation in combination with crossover, is designed to move the population away from local optima that a traditional ...
Basic Steps. The process of using genetic algorithms goes like this: Determine the problem and goal. Break down the solution to bite-sized properties (genomes) Build a population by randomizing said properties. Evaluate each unit in the population. Selectively breed (pick genomes from each parent) Rinse and repeat.
Genetic algorithm (GA) is a biologically inspired search method used to find high-quality solutions for complex problems. As previously introduced in Chap. 4, exhaustive search is often impractical for solving NP-hard optimization problems, and GA is designed to quickly identify good solutions.GA can effectively tackle a large variety of NP-hard optimization problems, including the single ...
June 7, 2018 at 2:00 pm. Police are using a new type of DNA sleuthing, called genetic genealogy. Already the technique has caught murder and rape suspects in California and Washington. While ...
Intelligence, a blend of genetics and environment, centers on problem-solving abilities and is best enhanced through learning and formal education.
They are commonly used to generate high-quality solutions for optimization problems and search problems. Genetic algorithms simulate the process of natural selection which means those species that can adapt to changes in their environment can survive and reproduce and go to the next generation. In simple words, they simulate "survival of the ...
"People tackling very huge issues that you don't even realize 1) is a problem that could be tackled with design, and 2), they're almost your age and they're doing it somehow. That was very important to see," she said. "People really think that you can solve anything."
Genetic testing underpins vital branches of medical science. Our research shows the question of who can assess a person's genomic data directly influences public trust in future genomic research ...
A plumber or electrician, faced with a complex problem, wouldn't get very far trying to solve it with strength alone; he would need to understand what's going on. The problems facing the ...
AI, EVs and satellites can help fight the climate crisis. But they, too, have an environmental cost. This hour, TED speakers examine how we can use each innovation without making the problem worse.