(Stanford users can avoid this Captcha by logging in.)
- Send to text email RefWorks EndNote printer

Case study methodology in higher education
Available online.
- IGI Global Ebooks
More options
- Find it at other libraries via WorldCat
- Contributors
Description
Creators/contributors, contents/summary.
- Chapter 1. Case study: defining and differentiating among types of case studies
- Chapter 2. Types of case studies
- Chapter 3. Using case studies in the higher education classroom: case studies in higher education what's the big idea?
- Chapter 4. Case study methodology: an analysis of effective methods in business cases
- Chapter 5. The types of case studies in research and career-based endeavors
- Chapter 6. Writing the case study
- Chapter 7. Student-authored case studies: the case of an educational leadership course in Kazakhstan
- Chapter 8. Developing a case writing club to create local cases in a private university in Puebla, Mexico: examples from academia
- Chapter 9. Teaching with case studies in higher education
- Chapter 10. Learner perception of using case study method as a teaching method in higher education
- Chapter 11. Practicing what we preach: a case study of the implementation of a complex conceptual framework
- Chapter 12. The utility of interdisciplinary case study: research and education in the arts and sciences
- Chapter 13. The good teacher in the good school: case studies to improve education and training of future Italian teachers
- Chapter 14. "Asking the woman question" in case study research
- Chapter 15. Challenges of a case study.
Bibliographic information
Browse related items.

- Stanford Home
- Maps & Directions
- Search Stanford
- Emergency Info
- Terms of Use
- Non-Discrimination
- Accessibility
© Stanford University , Stanford , California 94305 .
Online ordering is currently unavailable due to technical issues. We apologise for any delays responding to customers while we resolve this. For further updates please visit our website: https://www.cambridge.org/news-and-insights/technical-incident . Due to planned maintenance there will be periods of time where the website may be unavailable. We apologise for any inconvenience.
Case Study Research Principles and Practices
- Library eCollections
Description
Case Study Research: Principles and Practices provides a general understanding of the case study method as well as specific tools for its successful implementation. These tools are applicable in a variety of fields including anthropology, business and management, communications, economics, education, medicine, political science, psychology, social work, and sociology. Topics include: a survey of case study approaches; a methodologically tractable definition of 'case study'; strategies for case selection, including random sampling and other algorithmic approaches; quantitative and qualitative modes of…
- Add to bookmarks
- Download flyer
- Add bookmark
- Cambridge Spiral eReader
Key features
- The only book of its kind to break down traditional boundaries between qualitative and quantitative, experimental and nonexperimental methods
- An excellent teaching tool for students in all fields where the case study method is prominent, such as business, anthropology, economics, education and medicine
- Thoroughly revised and updated throughout to take account of recent developments and up-to-date studies
About the book
- DOI https://doi.org/10.1017/9781316848593
- Series Strategies for Social Inquiry
- Subjects Politics and International Relations, Qualitative Research Methods, Research Methods In Politics, Research Methods In Sociology and Criminology, Research Methods in Political Science, Research Methods in Sociology and Criminology, Sociology
- Publication date: 05 January 2017
- ISBN: 9781107181267
- Dimensions (mm): 228 x 152 mm
- Weight: 0.78kg
- Contains: 5 b/w illus. 35 tables
- Page extent: 362 pages
- Availability: Available
- ISBN: 9781316632505
- Weight: 0.64kg
- Publication date: 01 February 2019
- ISBN: 9781316848593
Access options
Review the options below to login to check your access.
Personal login
Log in with your Cambridge Higher Education account to check access.
Purchase options
There are no purchase options available for this title.
Have an access code?
To redeem an access code, please log in with your personal login.
If you believe you should have access to this content, please contact your institutional librarian or consult our FAQ page for further information about accessing our content.
John Gerring is Professor of Government at the University of Texas, Austin. He is the author of Party Ideologies in America, 1828–1996 (Cambridge, 1998), A Centripetal Theory of Democratic Governance (Cambridge, 2008), Concepts and Method: Giovanni Sartori and his Legacy (with David Collier, 2009), Social Science Methodology: A Unified Framework, 2nd edition (Cambridge, 2012), and Applied Social Science Methodology (with Dino Christenson, Cambridge, forthcoming), along with numerous articles.
Case Study Methodology in Higher Education
- Related Documents
Does case‐study methodology lack rigour? The need for quality criteria for sound case‐study research, as illustrated by a recent case in secondary and higher education
The development of prospective music teachers’ harmonic hearing at higher education establishments.
The development of harmonic hearing is an essential component of the system of music teachers’ training. The paper is concerned with the type of a case study which deals with the study of professional groups (a bigger and more diffusive group of 14 students). The process of training music teachers at sol-fa classes in a higher education establishment is analyzed and described. The sol-fa classes are oriented towards the development of harmonic hearing. The purpose of the study is to develop a technology of conducting sol-fa classes oriented towards developing students’ – the prospective music teachers’ – harmonic hearing on the basis of a case-study methodology; to present the developed material and ways of its application in the training process; to analyze and summarize the results of the research done. The research results show that the criteria relating to practical music making of students, such as polyphonic singing and intoning intervals and chords, and also those relating to creativeness, such as improvisation and composing the accompaniment for the melody, have been the most effective ones.
How Doba Business School Adopted its Business Model due to Coronavirus Pandemic?
The coronavirus pandemic (pandemic) is posing difficult and unpredictable challenges for Higher Education Institutions (HEIs). They have to adapt their business models (BMs), traditional working mechanisms and the way they transfer their knowledge to their students. The aim of this paper is to show how HEI of applied sciences overcame these challenges, and how they adapted its BM. The case study methodology was used. Based on the case of DOBA Business School Maribor, Slovenia, authors present how it reacted to the changed business and education circumstances. Discussion and concluding remarks stress out the lessons Doba Business School has learned from the pandemic so far and how these insights can help other HEIs to change their BMs in order to cope better in these challenging times.
Internationalization in Canadian Higher Education: A Case Study of the Gap between Official Discourses and On-the-Ground Realities
This case study about one university’s internationalization initiative, known as North Goes South, provides a nuanced and finely grained understanding of what internationalization looks like in practice. The study was guided by a desire to probe the perceived impact of a Canadian–East African internationalization initiative on students, faculty, and Tanzanian community members. The article begins with a brief review of the literature on internationalization and higher education in Canada. The rationale for using a case-study methodology is presented, along with the background and context of the case. Following an outline of the research methods, the study results are reviewed to show the complex and contradictory ways in which this internationalization initiative played out in one higher education setting, pointing to the gap between official discourses of internationalization and on-the-ground realities.
Academic Advisors in Australian Higher Education: Perceptions, Role Identities, and Recommendations
Previous research has evidenced the importance of student and staff interactions as critical functions to support student success at university. Increasingly, academic advising units support these interactions. However, while common throughout North American contexts, little is known about the implementation of such units internationally. In this paper, we use a case study methodology to discuss the introduction of an academic advising team at an Australian university to explore how staff adjusted to these new roles and their reflections on how others perceived them. We use reflective diaries submitted by the advisors (n = 11) to analyze how their role identities formed over time and suggested recommendations for supporting teams in the future.
The Types of Case Studies in Research and Career-Based Endeavors
This chapter focuses on the overarching components of the case study methodology in the context of research and career-based teaching and organizational learning settings. More specifically, this chapter, presented in several distinct sections, provides a description of the various types of case studies that can be selected for research purposes as well as for use as a teaching tool for career professionals, higher education faculty, and others interested in employing this type methodology. This chapter is intended to serve as a foundation to the subsequent text in this book pertaining to the detailed descriptions and elements of the case study serving as either a research design or a function of the teaching and learning process in academic and career-based settings. Providing a rich initial presentation of the types and qualities of the case study research design, this chapter will launch additional structure for the later chapters to offer a deeper understanding for the reader.
Aspire UWA: A case study of widening access in Higher Education
Widening university access to students from low socio-economic status (LSES) and non-traditional backgrounds has been a key equity objective for Australian universities, particularly since the 2008 Review into Australian Higher Education (Bradley, Noonan, Nugent & Scales, 2008). Aspire UWA is an equity pathway that aims to inform aspirations and build academic attainment through direct involvement with students who are the “most able least likely” to access the benefits of higher education (Harris, 2010, p. 7). Through forming long-term partnerships with 63 secondary schools across Western Australia (WA), Aspire UWA has grown since 2009 to engage over 10,000 students annually. Its learning framework is designed to deliver age-appropriate activities to inspire and inform students from Years 7-12 to achieve their educational goals. This paper adopts a case study methodology to explore the Aspire UWA approach, the specific operation of Aspire UWA and the efficacy of the program.
Case studies, make‐your‐case studies, and case stories: a critique of case‐study methodology in sustainability in higher education
Supporting the development of program leaders in higher education: an action research case study.
The higher education (HE) landscape continues to grow in complexity; thus, there is a need to improve the understanding of leadership in this context. This action research (AR) study was undertaken in a multi-disciplinary context of an Australian university to develop and evaluate an action learning (AL) project promoting leadership practice. An overview of AL is provided to situate the case study methodology and to demonstrate how it is used to develop leadership capabilities and benefit ‘team learning’. The findings support the development of AR programs for leaders in the ever-changing environment of HE. The need for an understanding of what leadership is, the development of a learning community and the articulation of the learning processes are seen as essential to support leaders in their development. Leaders not only need to be reflective but also require a safe and trusting environment to support their quest for career progression, grants and awards.
Export Citation Format
Share document.

Case Study Methodology in Higher Education
- Author(s) Annette Baron
- Publisher Information Science Reference
Print ISBN 9781522594291, 1522594299
Etext isbn 9781522594321, 1522594329.
- Copyright 2019
- Available from $ 195.00 USD SKU: 9781522594321
The world’s #1 eTextbook reader for students. VitalSource is the leading provider of online textbooks and course materials. More than 15 million users have used our Bookshelf platform over the past year to improve their learning experience and outcomes. With anytime, anywhere access and built-in tools like highlighters, flashcards, and study groups, it’s easy to see why so many students are going digital with Bookshelf.
Over 2.7 million titles available from more than 1,000 publishers
Over 65,000 customer reviews with an average rating of 9.5
Over 5 billion digital pages viewed over the past 12 months
Over 7,000 institutions using Bookshelf across 241 countries
Case Study Methodology in Higher Education is written by Annette Baron and published by Information Science Reference. The Digital and eTextbook ISBNs for Case Study Methodology in Higher Education are 9781522594321, 1522594329 and the print ISBNs are 9781522594291, 1522594299. Save up to 80% versus print by going digital with VitalSource. Additional ISBNs for this eTextbook include 9781522594314.
Academia.edu no longer supports Internet Explorer.
To browse Academia.edu and the wider internet faster and more securely, please take a few seconds to upgrade your browser .
Enter the email address you signed up with and we'll email you a reset link.
- We're Hiring!
- Help Center
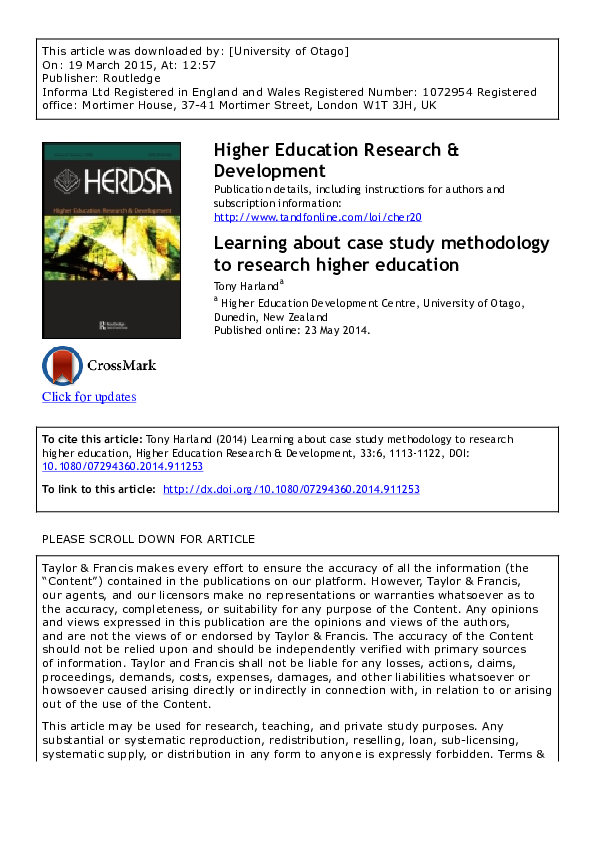
Learning about case study methodology to research higher education

2014, Higher Education Research & Development
Related Papers
johnpatrick gonzales
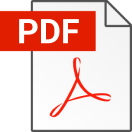
Paula Dawidowicz
Unlike theoretical scholars who seek knowledge to expand humanity’s (or their) understanding of a topic, scholar practitioners seek knowledge that can be applied to understand change or create change in a specific phenomenon. Although many of the same research methods can be used by both groups of educational scholars, and although research designs are determined in large part by the research questions being asked, several research methods can prove most useful for scholar practitioners examining learning environments. One, the case study, stands out as perhaps the most versatile and researcher-friendly, though. A case study, bounded by specific location and topic parameters, can allow solid evaluation of the workings of a program or teaching method. It can also allow consideration of specific needs to address an educational situation. This article briefly discusses the nature and purpose of different types of research, then focuses on the nature and usefulness of the case study met...
Nina Oktavia
Case study is believed as the widely used kind of research to view phenomena, despite of some critics on it concerning mostly on its data reliability, validity and subjectivity. This article therefore discusses some aspects of case study which are considered important to be recognized by novice researchers, especially about the way how to design and how to make sure the quality and reliability of the case. In addition, the case studying educational research also becomes the focus to be discussed, completed with some examples, to be able to open our mind to the plenty opportunities for case study in education.
South African Journal of Higher Education
Peter Rule , Robert J Balfour
ABSTRACT The Project Postgraduate Educational Research (PPER) data indicate that case study is by far the most popular methodology among South African education masters and doctorate students in the period 1995-2004. This paper reflects on the reasons for the preference for case study by considering epistemological and contextual factors. It unpacks the links between the methodological choice of case study, on the one hand, and the thematic focus and sectoral location of theses, on the other. While acknowledging the strengths of case study methodology regarding manageability, depth and experiential texture, the paper argues that the predominance of case study limits the applicability of research to wider questions of policy and implementation, and thus contributes to the hiddenness of students’ voices in public discourse around education. It suggests ways of enhancing the wider relevance of case studies through multiple case designs, sequential case studies, mixed method designs and synoptic reviews.
Anju Chhetri
This article presents the case study as a type of qualitative research. Its aim is to give a detailed description of a case study-its definition, some classifications, and several advantages and disadvantages-in order to provide a better understanding of this widely used type of qualitative approac h. In comparison to other types of qualitative research, case studies have been little understood both from a methodological point of view, where disagreements exist about whether case studies should be considered a research method or a research type, and from a content point of view, where there are ambiguities regarding what should be considered a case or research subject. A great emphasis is placed on the disadvantages of case studies, where we try to refute some of the criticisms concerning case studies, particularly in comparison to quantitative research approaches.
Nurse researcher
Camille Cronin
To describe how case study research (CSR) was used inductively as an all-encompassing theoretical framework to examine learning in the workplace. Case study research is a method with strong philosophical underpinnings which provides a framework for exploratory research in real-life settings ( Yin 2009 ). A study of five students' experiences of learning in healthcare environments to explore real-life contexts over the course of two years. The study was rich with empirical data, offering a pragmatic framework for learning in the workplace. Observations, interviews and documents were used. As a result of using CSR, the findings were analysed systematically and rigorously. Using multiple methods verified data and strengthened the significance of the findings. This paper focuses on the strengths of using the CSR methodology. CSR embraces qualitative research methods in a rigorous and systematic manner. CSR has been applied to one example of research, addressing each step of the rese...
Jean Pasquero
… Teaching and Learning Research Programme, First …
David Gosling , Vaneeta D'Andrea
Paul Ashwin
Loading Preview
Sorry, preview is currently unavailable. You can download the paper by clicking the button above.
RELATED PAPERS
INTERNATIONAL JOURNAL OF MANAGEMENT & INFORMATION TECHNOLOGY
Damber Kharka
ethiopia-ed.net
Kathleen Armour
Higher Education Pedagogies
Frances O'Brien
Ilse Schrittesser
RELATED TOPICS
- We're Hiring!
- Help Center
- Find new research papers in:
- Health Sciences
- Earth Sciences
- Cognitive Science
- Mathematics
- Computer Science
- Academia ©2024
- Applied Philosophy
Case studies, make-your-case studies, and case stories: A critique of case-study methodology in sustainability in higher education
- February 2004
- This person is not on ResearchGate, or hasn't claimed this research yet.

- Wageningen UR & Gothenburg University
Discover the world's research
- 25+ million members
- 160+ million publication pages
- 2.3+ billion citations

- Glen Cousquer
- Emily Norris

- J BEHAV HEALTH SER R

- Jillian Zitars
- Deborah Scharf
- Szerkesztők Márkus Edina
- Bucher Eszter
- Boros Julianna

- Mohammad A. Alsharif
- Jacob Ofori-Darko

- Aline Cristiane Rocha Lacerda
- Maria das Graças Cleophas

- Amanda Isaac

- Cathy Lembke

- P. A.DE ALMEIDA

- Philip Cassell
- Sharan B. Merriam
- Wilfred Carr

- Stephen Gough
- Etienne Wenger
- Stephen M. Corey
- Recruit researchers
- Join for free
- Login Email Tip: Most researchers use their institutional email address as their ResearchGate login Password Forgot password? Keep me logged in Log in or Continue with Google Welcome back! Please log in. Email · Hint Tip: Most researchers use their institutional email address as their ResearchGate login Password Forgot password? Keep me logged in Log in or Continue with Google No account? Sign up
Case Study Research Method in Psychology
Saul Mcleod, PhD
Editor-in-Chief for Simply Psychology
BSc (Hons) Psychology, MRes, PhD, University of Manchester
Saul Mcleod, PhD., is a qualified psychology teacher with over 18 years of experience in further and higher education. He has been published in peer-reviewed journals, including the Journal of Clinical Psychology.
Learn about our Editorial Process
Olivia Guy-Evans, MSc
Associate Editor for Simply Psychology
BSc (Hons) Psychology, MSc Psychology of Education
Olivia Guy-Evans is a writer and associate editor for Simply Psychology. She has previously worked in healthcare and educational sectors.
On This Page:
Case studies are in-depth investigations of a person, group, event, or community. Typically, data is gathered from various sources using several methods (e.g., observations & interviews).
The case study research method originated in clinical medicine (the case history, i.e., the patient’s personal history). In psychology, case studies are often confined to the study of a particular individual.
The information is mainly biographical and relates to events in the individual’s past (i.e., retrospective), as well as to significant events that are currently occurring in his or her everyday life.
The case study is not a research method, but researchers select methods of data collection and analysis that will generate material suitable for case studies.
Freud (1909a, 1909b) conducted very detailed investigations into the private lives of his patients in an attempt to both understand and help them overcome their illnesses.
This makes it clear that the case study is a method that should only be used by a psychologist, therapist, or psychiatrist, i.e., someone with a professional qualification.
There is an ethical issue of competence. Only someone qualified to diagnose and treat a person can conduct a formal case study relating to atypical (i.e., abnormal) behavior or atypical development.
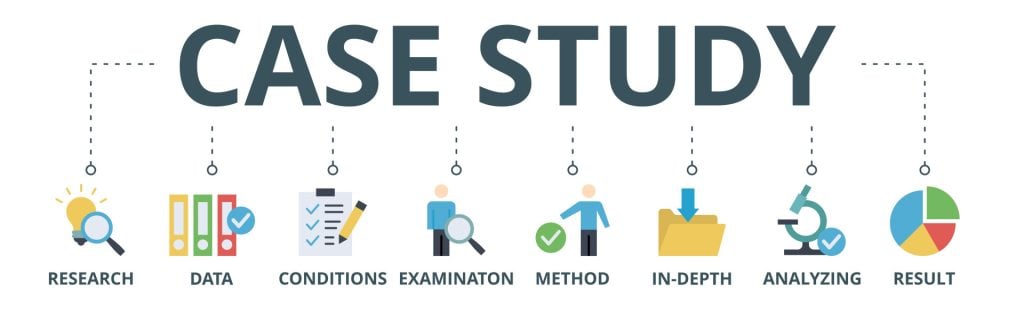
Famous Case Studies
- Anna O – One of the most famous case studies, documenting psychoanalyst Josef Breuer’s treatment of “Anna O” (real name Bertha Pappenheim) for hysteria in the late 1800s using early psychoanalytic theory.
- Little Hans – A child psychoanalysis case study published by Sigmund Freud in 1909 analyzing his five-year-old patient Herbert Graf’s house phobia as related to the Oedipus complex.
- Bruce/Brenda – Gender identity case of the boy (Bruce) whose botched circumcision led psychologist John Money to advise gender reassignment and raise him as a girl (Brenda) in the 1960s.
- Genie Wiley – Linguistics/psychological development case of the victim of extreme isolation abuse who was studied in 1970s California for effects of early language deprivation on acquiring speech later in life.
- Phineas Gage – One of the most famous neuropsychology case studies analyzes personality changes in railroad worker Phineas Gage after an 1848 brain injury involving a tamping iron piercing his skull.
Clinical Case Studies
- Studying the effectiveness of psychotherapy approaches with an individual patient
- Assessing and treating mental illnesses like depression, anxiety disorders, PTSD
- Neuropsychological cases investigating brain injuries or disorders
Child Psychology Case Studies
- Studying psychological development from birth through adolescence
- Cases of learning disabilities, autism spectrum disorders, ADHD
- Effects of trauma, abuse, deprivation on development
Types of Case Studies
- Explanatory case studies : Used to explore causation in order to find underlying principles. Helpful for doing qualitative analysis to explain presumed causal links.
- Exploratory case studies : Used to explore situations where an intervention being evaluated has no clear set of outcomes. It helps define questions and hypotheses for future research.
- Descriptive case studies : Describe an intervention or phenomenon and the real-life context in which it occurred. It is helpful for illustrating certain topics within an evaluation.
- Multiple-case studies : Used to explore differences between cases and replicate findings across cases. Helpful for comparing and contrasting specific cases.
- Intrinsic : Used to gain a better understanding of a particular case. Helpful for capturing the complexity of a single case.
- Collective : Used to explore a general phenomenon using multiple case studies. Helpful for jointly studying a group of cases in order to inquire into the phenomenon.
Where Do You Find Data for a Case Study?
There are several places to find data for a case study. The key is to gather data from multiple sources to get a complete picture of the case and corroborate facts or findings through triangulation of evidence. Most of this information is likely qualitative (i.e., verbal description rather than measurement), but the psychologist might also collect numerical data.
1. Primary sources
- Interviews – Interviewing key people related to the case to get their perspectives and insights. The interview is an extremely effective procedure for obtaining information about an individual, and it may be used to collect comments from the person’s friends, parents, employer, workmates, and others who have a good knowledge of the person, as well as to obtain facts from the person him or herself.
- Observations – Observing behaviors, interactions, processes, etc., related to the case as they unfold in real-time.
- Documents & Records – Reviewing private documents, diaries, public records, correspondence, meeting minutes, etc., relevant to the case.
2. Secondary sources
- News/Media – News coverage of events related to the case study.
- Academic articles – Journal articles, dissertations etc. that discuss the case.
- Government reports – Official data and records related to the case context.
- Books/films – Books, documentaries or films discussing the case.
3. Archival records
Searching historical archives, museum collections and databases to find relevant documents, visual/audio records related to the case history and context.
Public archives like newspapers, organizational records, photographic collections could all include potentially relevant pieces of information to shed light on attitudes, cultural perspectives, common practices and historical contexts related to psychology.
4. Organizational records
Organizational records offer the advantage of often having large datasets collected over time that can reveal or confirm psychological insights.
Of course, privacy and ethical concerns regarding confidential data must be navigated carefully.
However, with proper protocols, organizational records can provide invaluable context and empirical depth to qualitative case studies exploring the intersection of psychology and organizations.
- Organizational/industrial psychology research : Organizational records like employee surveys, turnover/retention data, policies, incident reports etc. may provide insight into topics like job satisfaction, workplace culture and dynamics, leadership issues, employee behaviors etc.
- Clinical psychology : Therapists/hospitals may grant access to anonymized medical records to study aspects like assessments, diagnoses, treatment plans etc. This could shed light on clinical practices.
- School psychology : Studies could utilize anonymized student records like test scores, grades, disciplinary issues, and counseling referrals to study child development, learning barriers, effectiveness of support programs, and more.
How do I Write a Case Study in Psychology?
Follow specified case study guidelines provided by a journal or your psychology tutor. General components of clinical case studies include: background, symptoms, assessments, diagnosis, treatment, and outcomes. Interpreting the information means the researcher decides what to include or leave out. A good case study should always clarify which information is the factual description and which is an inference or the researcher’s opinion.
1. Introduction
- Provide background on the case context and why it is of interest, presenting background information like demographics, relevant history, and presenting problem.
- Compare briefly to similar published cases if applicable. Clearly state the focus/importance of the case.
2. Case Presentation
- Describe the presenting problem in detail, including symptoms, duration,and impact on daily life.
- Include client demographics like age and gender, information about social relationships, and mental health history.
- Describe all physical, emotional, and/or sensory symptoms reported by the client.
- Use patient quotes to describe the initial complaint verbatim. Follow with full-sentence summaries of relevant history details gathered, including key components that led to a working diagnosis.
- Summarize clinical exam results, namely orthopedic/neurological tests, imaging, lab tests, etc. Note actual results rather than subjective conclusions. Provide images if clearly reproducible/anonymized.
- Clearly state the working diagnosis or clinical impression before transitioning to management.
3. Management and Outcome
- Indicate the total duration of care and number of treatments given over what timeframe. Use specific names/descriptions for any therapies/interventions applied.
- Present the results of the intervention,including any quantitative or qualitative data collected.
- For outcomes, utilize visual analog scales for pain, medication usage logs, etc., if possible. Include patient self-reports of improvement/worsening of symptoms. Note the reason for discharge/end of care.
4. Discussion
- Analyze the case, exploring contributing factors, limitations of the study, and connections to existing research.
- Analyze the effectiveness of the intervention,considering factors like participant adherence, limitations of the study, and potential alternative explanations for the results.
- Identify any questions raised in the case analysis and relate insights to established theories and current research if applicable. Avoid definitive claims about physiological explanations.
- Offer clinical implications, and suggest future research directions.
5. Additional Items
- Thank specific assistants for writing support only. No patient acknowledgments.
- References should directly support any key claims or quotes included.
- Use tables/figures/images only if substantially informative. Include permissions and legends/explanatory notes.
- Provides detailed (rich qualitative) information.
- Provides insight for further research.
- Permitting investigation of otherwise impractical (or unethical) situations.
Case studies allow a researcher to investigate a topic in far more detail than might be possible if they were trying to deal with a large number of research participants (nomothetic approach) with the aim of ‘averaging’.
Because of their in-depth, multi-sided approach, case studies often shed light on aspects of human thinking and behavior that would be unethical or impractical to study in other ways.
Research that only looks into the measurable aspects of human behavior is not likely to give us insights into the subjective dimension of experience, which is important to psychoanalytic and humanistic psychologists.
Case studies are often used in exploratory research. They can help us generate new ideas (that might be tested by other methods). They are an important way of illustrating theories and can help show how different aspects of a person’s life are related to each other.
The method is, therefore, important for psychologists who adopt a holistic point of view (i.e., humanistic psychologists ).
Limitations
- Lacking scientific rigor and providing little basis for generalization of results to the wider population.
- Researchers’ own subjective feelings may influence the case study (researcher bias).
- Difficult to replicate.
- Time-consuming and expensive.
- The volume of data, together with the time restrictions in place, impacted the depth of analysis that was possible within the available resources.
Because a case study deals with only one person/event/group, we can never be sure if the case study investigated is representative of the wider body of “similar” instances. This means the conclusions drawn from a particular case may not be transferable to other settings.
Because case studies are based on the analysis of qualitative (i.e., descriptive) data , a lot depends on the psychologist’s interpretation of the information she has acquired.
This means that there is a lot of scope for Anna O , and it could be that the subjective opinions of the psychologist intrude in the assessment of what the data means.
For example, Freud has been criticized for producing case studies in which the information was sometimes distorted to fit particular behavioral theories (e.g., Little Hans ).
This is also true of Money’s interpretation of the Bruce/Brenda case study (Diamond, 1997) when he ignored evidence that went against his theory.
Breuer, J., & Freud, S. (1895). Studies on hysteria . Standard Edition 2: London.
Curtiss, S. (1981). Genie: The case of a modern wild child .
Diamond, M., & Sigmundson, K. (1997). Sex Reassignment at Birth: Long-term Review and Clinical Implications. Archives of Pediatrics & Adolescent Medicine , 151(3), 298-304
Freud, S. (1909a). Analysis of a phobia of a five year old boy. In The Pelican Freud Library (1977), Vol 8, Case Histories 1, pages 169-306
Freud, S. (1909b). Bemerkungen über einen Fall von Zwangsneurose (Der “Rattenmann”). Jb. psychoanal. psychopathol. Forsch ., I, p. 357-421; GW, VII, p. 379-463; Notes upon a case of obsessional neurosis, SE , 10: 151-318.
Harlow J. M. (1848). Passage of an iron rod through the head. Boston Medical and Surgical Journal, 39 , 389–393.
Harlow, J. M. (1868). Recovery from the Passage of an Iron Bar through the Head . Publications of the Massachusetts Medical Society. 2 (3), 327-347.
Money, J., & Ehrhardt, A. A. (1972). Man & Woman, Boy & Girl : The Differentiation and Dimorphism of Gender Identity from Conception to Maturity. Baltimore, Maryland: Johns Hopkins University Press.
Money, J., & Tucker, P. (1975). Sexual signatures: On being a man or a woman.
Further Information
- Case Study Approach
- Case Study Method
- Enhancing the Quality of Case Studies in Health Services Research
- “We do things together” A case study of “couplehood” in dementia
- Using mixed methods for evaluating an integrative approach to cancer care: a case study

Related Articles
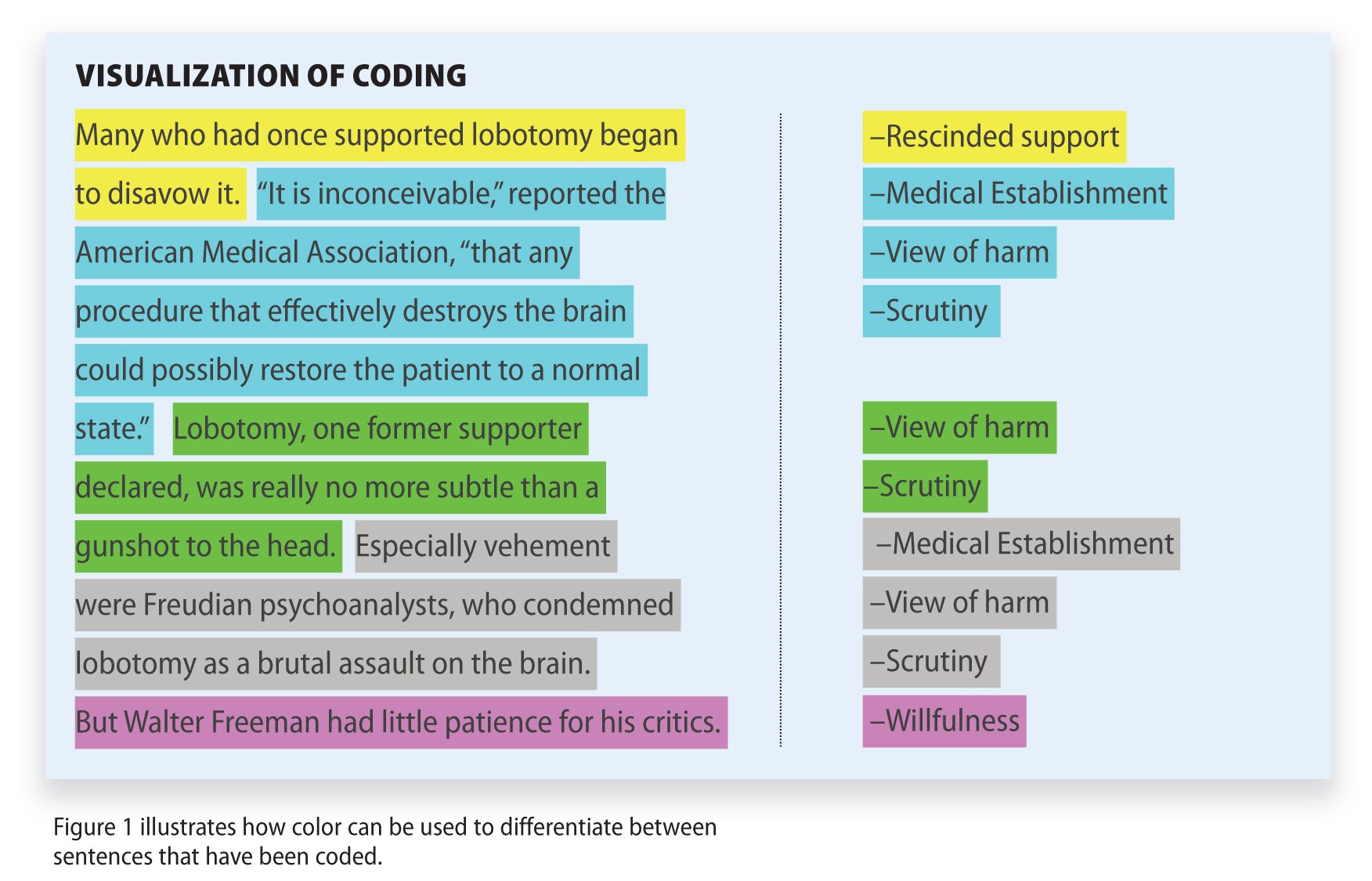
Research Methodology
Qualitative Data Coding
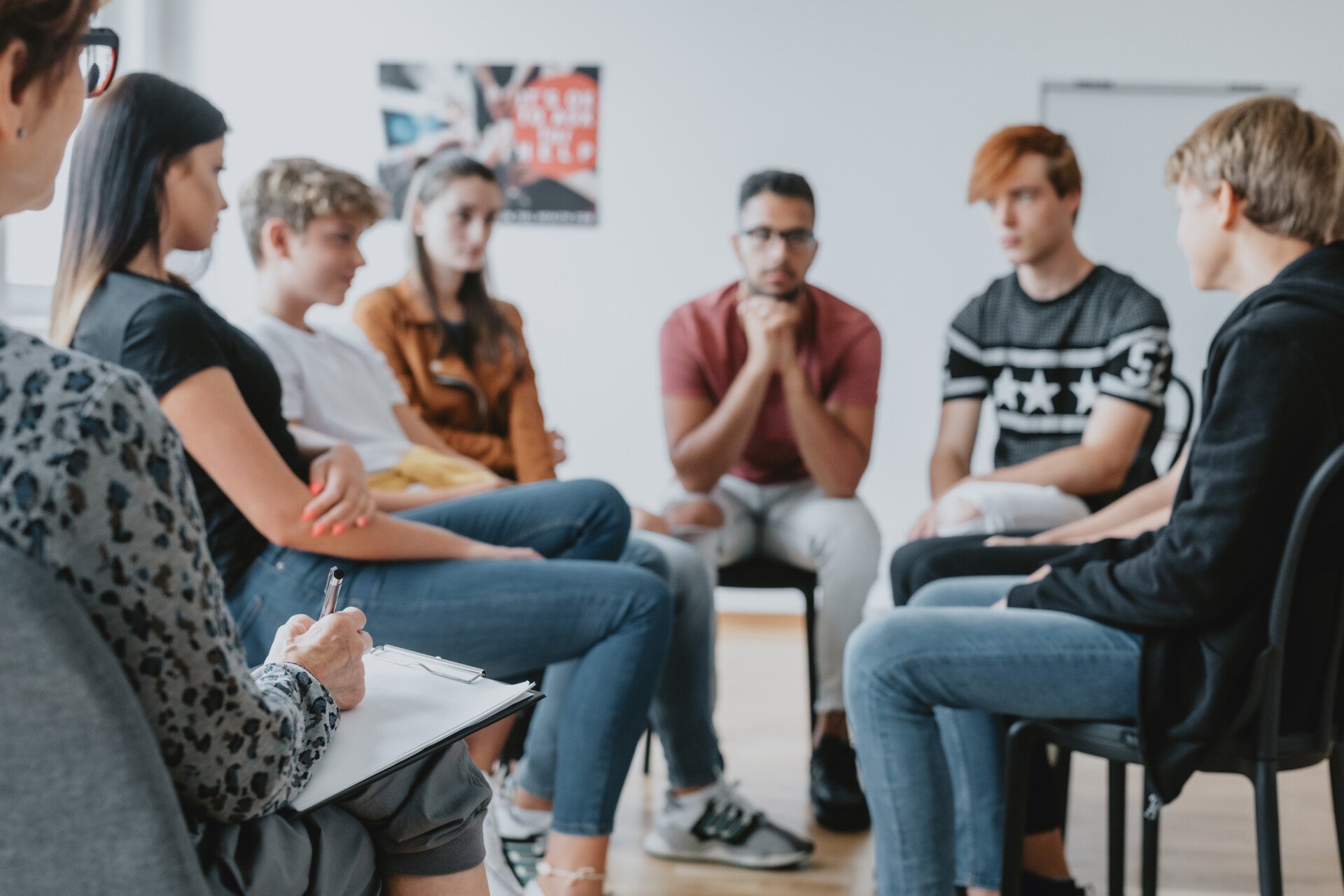
What Is a Focus Group?
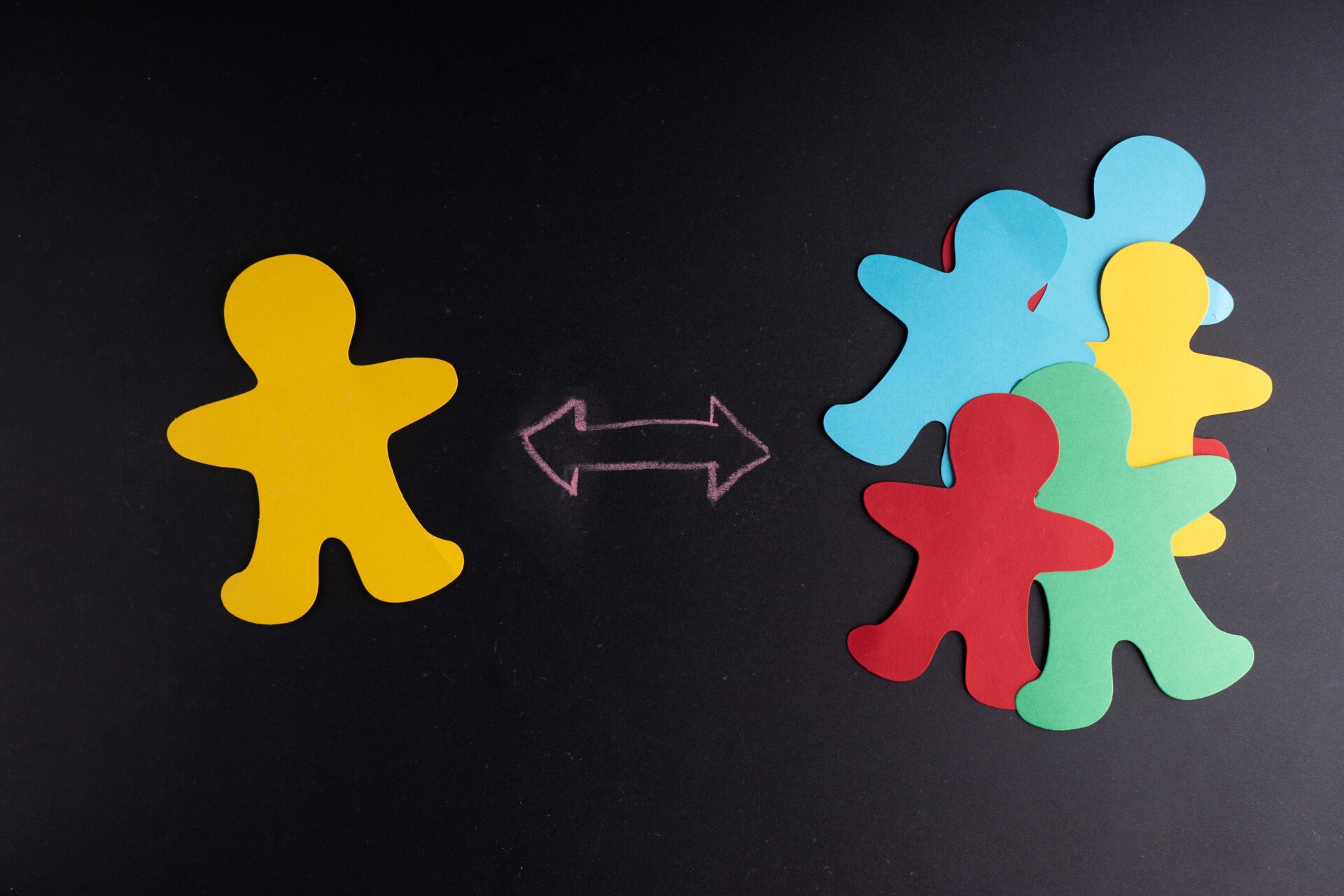
Cross-Cultural Research Methodology In Psychology
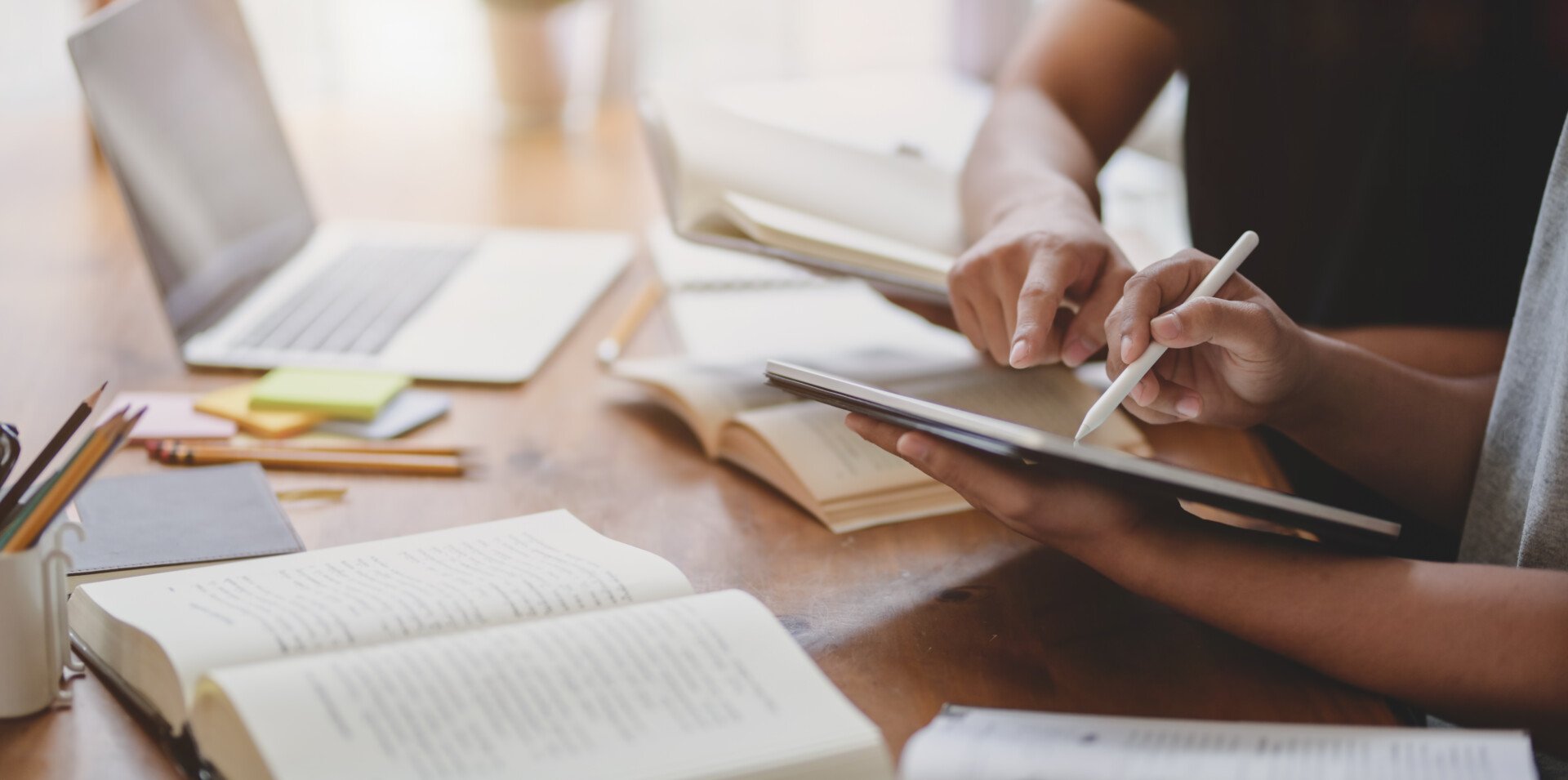
What Is Internal Validity In Research?
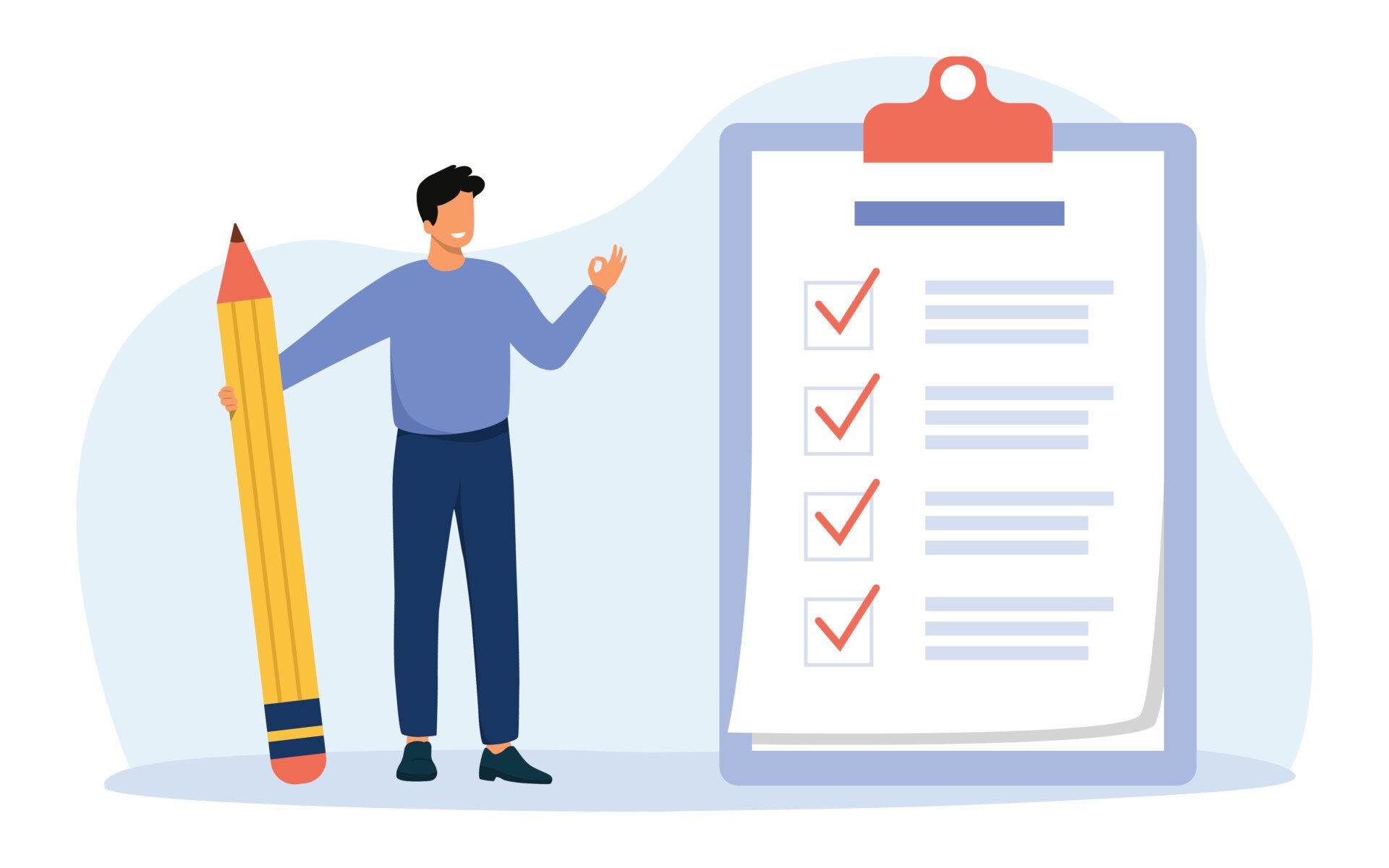
Research Methodology , Statistics
What Is Face Validity In Research? Importance & How To Measure
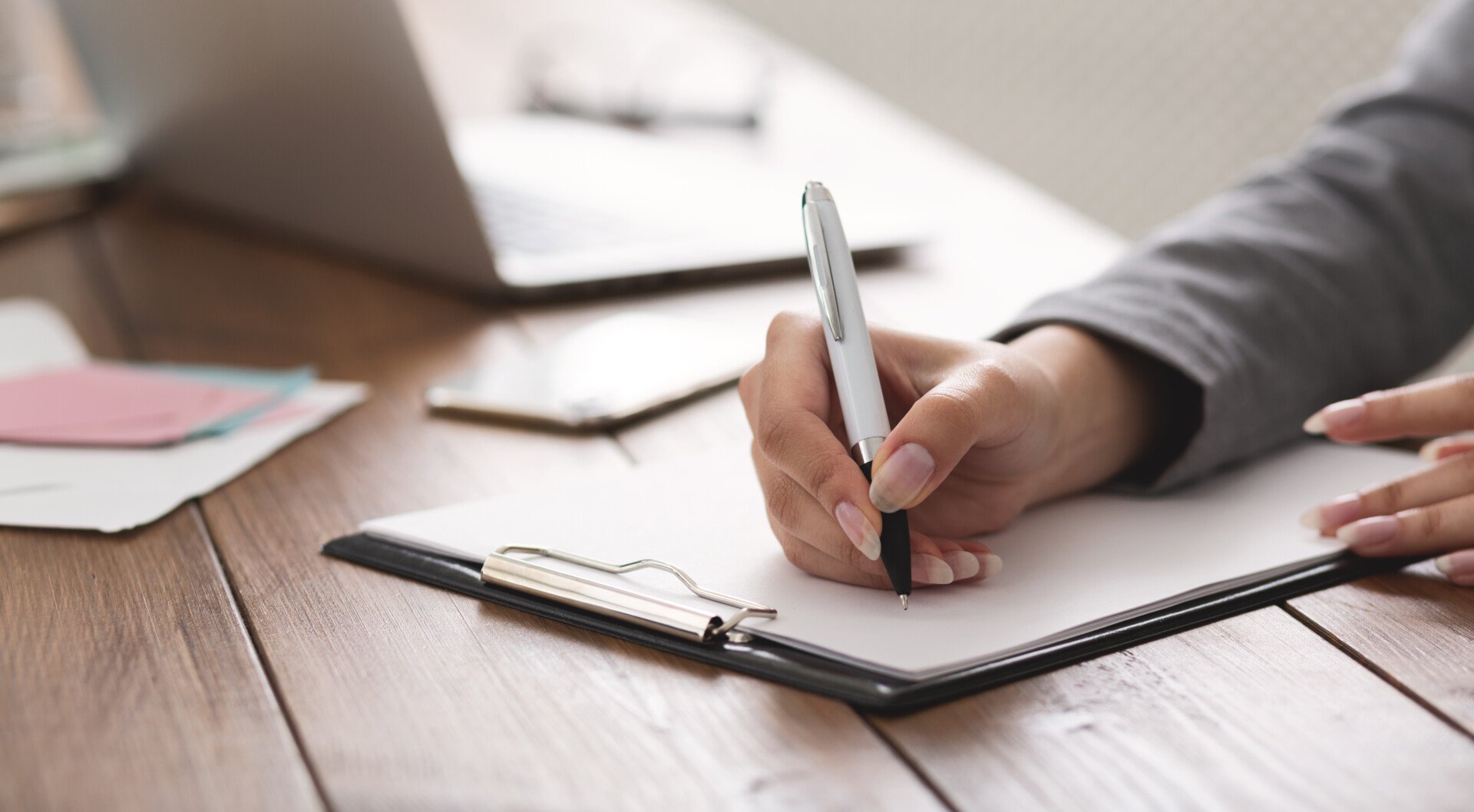
Criterion Validity: Definition & Examples
- Student Success
- Academic Life
Program Innovations: Promoting Success in Student Research
To enhance the student experience and increase access to experiential learning, colleges and universities have gotten creative with undergraduate research experiences.
By Ashley Mowreader
You have / 5 articles left. Sign up for a free account or log in.
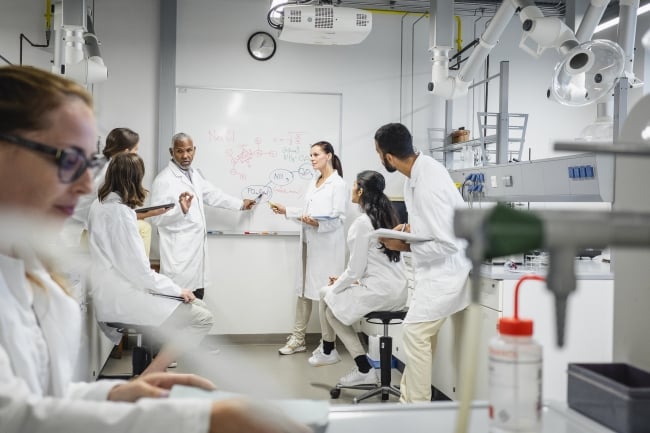
Undergraduate research can provide students with hands-on experience in a lab environment, as well as help them determine career opportunities they might not otherwise consider.
sanjeri/E+/Getty Images Plus
Undergraduate research opportunities are one way to provide experiential learning in many disciplines, introducing learners to research methods under the supervision of a faculty member and providing experience for a résumé.
A 2021 study from the University of Central Florida found student researchers are more likely to have higher grade point averages and graduation rates, and higher matriculation into graduate school, as well as life skills such as analyzing literature critically, observing and collecting data and communication.
However, not every student has equal access to undergraduate research opportunities. The study found non-STEM students, transfer students and part-time students are less likely to participate in research compared to their peers.
To increase student participation in undergraduate research and boost skill development among student researchers, institutions have created innovative models of work. Here are seven examples.
Survey Says
Around three in 10 students say they are required to participate in undergraduate research, according to a winter 2023 Student Voice survey from Inside Higher Ed , and a slightly smaller number (27 percent) believe that undergraduate research should be required in their program.
Four-year students are more likely to say undergraduate research is required in their program (32 percent) compared to their two-year peers (15 percent).
First-year research opportunities—University of Missouri
Career exposure in the first year can help students feel confident about their paths throughout college and provide a head start in building their résumés.
Mizzou is known for its Interdisciplinary Plant Group that hosts research scholars exploring innovations in plant biology and a first-year program that provides young researchers a leg up in their work, giving them research time with more experienced researchers and mentorship.
FRIPS, short for Freshman Research in Plants , supports 10 to 15 students annually, who work alongside a faculty member and their research group on plant biology. Students also meet regularly with their FRIPS scholars cohort and gain professional development training.
Each student’s work is funded by grant dollars from the National Science Foundation (NSF).
Graduates of FRIPS often go on to become Goldwater Scholars and NSF graduate research fellows. The program also creates a place of belonging and community for new students to the university.
Underrepresented minority students—Davidson College
Some students face systemic disadvantages in participating in co-curricular experiences because they may lack the social capital or be unfamiliar with the norms of higher ed to identify and participate in a faculty-led research experience.
At Davidson College in North Carolina, rising sophomores can participate in a four- to six-week summer intensive research fellowship program called RISE (Research in Science Experience) . This program is designed for students from historically marginalized groups including low-income and first-generation students.
The goal of RISE is to equip students to take on larger, more intensive academic-year and summer experiences for later in their college career. Each student receives $2,500 in scholarships and funds to cover on-campus housing, which the college arranges for all participants.
Jacquelline Nyakunu , a rising junior at Davidson, spent the summer with chemistry professor Cindy Hauser researching hookah, studying the smoking of shisha and the chemical composition of the tobacco. Nyakunu wrote in a blog post that the experience taught her about her passion for chemistry, built her research skills and solidified her career path as a pre-medicine student.
Editors’ Picks
- Can University of the Arts Be Saved?
- ‘Unprecedented Steps’: Board Pulls Plug on Columbia Law Review Website
- Faculty Power on the Line in Kentucky
Transfer students—University of California, Los Angeles
Transferring into a new institution can be a challenge for many students, and finding ways to get plugged in and connected to one’s field of interest can be just as hard.
UCLA offers an initiative exclusively for transfer students to both promote their academic success at the university and expose them to undergraduate research opportunities, the Transfer Research Entry Program (TREP).
To participate, each student must be an incoming transfer student with at least two years remaining at UCLA and be considering a career in research. Participants attend a one-week virtual bootcamp about research, which covers careers in research and how to write a cover letter and curriculum vitae. The program also provides networking opportunities for transfer students and academic survival skills for the transition to UCLA.
There’s no obligation to take a research role after the bootcamp, but students are encouraged to do so and given guidance on how to find their areas of interest, the application and interview process as well.
Financial support—University of Texas at El Paso
Financial need can be a barrier to participation for some students. The University of Texas at El Paso is a Hispanic Serving Institution (84 percent Hispanic), commuter school with a large population of Pell-eligible students (60 percent) and first-generation learners. Many students are working to support themselves and their families, explains Lourdes E. Echegoyen, director of the Campus Office of Undergraduate Research Initiatives.
As a result, UTEP staff realized a need to provide financial assistance through employment to give students high-impact activities.
University staff have identified grant funding from federal agencies, including the National Science Foundation, the National Institutes of Health, the Department of Education, and private foundations.
Students can receive financial support through stipends or tuition scholarships. The university’s student employment program also provides employment positions for undergraduate researchers across disciplines.
“Generally, full time students are supported to conduct research during the academic year from 10 to 19 hours per week—depending on the program—thus allowing students to remain on campus and be mentored as research trainees,” Echegoyen says.
UTEP leaders have seen the benefits of undergraduate research on retention and persistence among students, with one program focused on biomedical research having a 98 percent retention rate among students across four years, compared to a 37 percent retention rate among their peers who did not participate.&
Community partnerships—Roosevelt University
During the COVID-19 pandemic, Roosevelt University in Chicago partnered with The Field Museum to digitize and analyze data collected at the museum. Visitors had measured specimens of liverworts, but the data needed to be sorted and inaccurate measurements eliminated from the set to be most useful to scientists.
Students wrote code to screen and clean the data, helping set the researchers up for success and teaching students firsthand about research processes in a remote setting.
Career development—Elon University
At Elon in North Carolina, returning students can participate in undergraduate research over the summer in between academic terms, funded by the university. While career readiness is a natural component of research experiences, leaders at the university wanted to bolster student skills beyond the laboratory, says Eric Hall, professor of exercise and director of undergraduate research at Elon.
Now, student researchers attend regular professional-development workshops that inform and establish career competencies. The workshops are co-led by other campus partners, including the writing center, career services, the fellowships office and librarians, Hall says.
For the 2024 session, workshops include a session on LinkedIn on how the fellowships office can support student goals, professional writing for graduate school and industry, and navigating academic publishing.
The new initiative is still being evaluated, with formal data collection underway, but anecdotal evidence from post-assessments shows students enjoy and learn from the experiences.
Research in the classroom—California State Polytechnic University, Pomona
Cal Poly Pomona leaders wanted to expose more learners to undergraduate research, understanding that first-generation, Pell-eligible or historically underserved students have lower access to research opportunities, says Winny Dong, faculty director for the office of undergraduate research.
Rather than asking students to squeeze an additional responsibility into their schedules, faculty members brought research to the classroom, embedding experiences into required general education courses.
The initiative makes it so all students are exposed to research and required to participate in some capacity, helping build their skills and pique interest for those who may consider a career in research.

Supporting Students With Disabilities in Degree Attainment
Students with disabilities in higher education are less likely to graduate and land full-time employment after colleg
Share This Article
More from academic life.
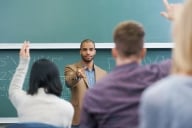
Report: Grade Inflation Highlighted in Developmental Course Placement
A new study from ACT finds students who completed high school during the COVID-19 pandemic were placed in development

Positive Partnership: Streamlining and Speeding Up Transfer Completion
A partnership between George Mason University and Northern Virginia Community College promotes timely degree completi
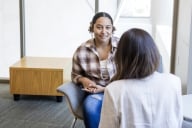
Alternate Angles of Higher Ed Equity: Seven Insights Gained From European Universities
Setting aside affordability in the conversation about equity allows for a broader range of insight that could benefit
- Become a Member
- Sign up for Newsletters
- Learning & Assessment
- Diversity & Equity
- Career Development
- Labor & Unionization
- Shared Governance
- Academic Freedom
- Books & Publishing
- Financial Aid
- Residential Life
- Free Speech
- Physical & Mental Health
- Race & Ethnicity
- Sex & Gender
- Socioeconomics
- Traditional-Age
- Adult & Post-Traditional
- Teaching & Learning
- Artificial Intelligence
- Digital Publishing
- Data Analytics
- Administrative Tech
- Alternative Credentials
- Financial Health
- Cost-Cutting
- Revenue Strategies
- Academic Programs
- Physical Campuses
- Mergers & Collaboration
- Fundraising
- Research Universities
- Regional Public Universities
- Community Colleges
- Private Nonprofit Colleges
- Minority-Serving Institutions
- Religious Colleges
- Women's Colleges
- Specialized Colleges
- For-Profit Colleges
- Executive Leadership
- Trustees & Regents
- State Oversight
- Accreditation
- Politics & Elections
- Supreme Court
- Student Aid Policy
- Science & Research Policy
- State Policy
- Colleges & Localities
- Employee Satisfaction
- Remote & Flexible Work
- Staff Issues
- Study Abroad
- International Students in U.S.
- U.S. Colleges in the World
- Intellectual Affairs
- Seeking a Faculty Job
- Advancing in the Faculty
- Seeking an Administrative Job
- Advancing as an Administrator
- Beyond Transfer
- Call to Action
- Confessions of a Community College Dean
- Higher Ed Gamma
- Higher Ed Policy
- Just Explain It to Me!
- Just Visiting
- Law, Policy—and IT?
- Leadership & StratEDgy
- Leadership in Higher Education
- Learning Innovation
- Online: Trending Now
- Resident Scholar
- University of Venus
- Student Voice
- Health & Wellness
- The College Experience
- Life After College
- Academic Minute
- Weekly Wisdom
- Reports & Data
- Quick Takes
- Advertising & Marketing
- Consulting Services
- Data & Insights
- Hiring & Jobs
- Event Partnerships
4 /5 Articles remaining this month.
Sign up for a free account or log in.
- Create Free Account
Search with any image
Unsupported image file format.
Image file size is too large..
Drag an image here

- Education & Teaching
- Schools & Teaching
Sorry, there was a problem.

Download the free Kindle app and start reading Kindle books instantly on your smartphone, tablet, or computer - no Kindle device required .
Read instantly on your browser with Kindle for Web.
Using your mobile phone camera - scan the code below and download the Kindle app.


Image Unavailable

- To view this video download Flash Player
Case Study Methodology in Higher Education (Advances in Higher Education and Professional Development) 1st Edition
- ISBN-10 1522594299
- ISBN-13 978-1522594291
- Edition 1st
- Publisher Information Science Reference
- Publication date June 28, 2019
- Language English
- Dimensions 7 x 0.94 x 10 inches
- Print length 355 pages
- See all details
Product details
- Publisher : Information Science Reference; 1st edition (June 28, 2019)
- Language : English
- Hardcover : 355 pages
- ISBN-10 : 1522594299
- ISBN-13 : 978-1522594291
- Item Weight : 1.4 pounds
- Dimensions : 7 x 0.94 x 10 inches
Customer reviews
5 star | 0% | |
4 star | 0% | |
3 star | 0% | |
2 star | 0% | |
1 star | 0% |
Customer Reviews, including Product Star Ratings help customers to learn more about the product and decide whether it is the right product for them.
To calculate the overall star rating and percentage breakdown by star, we don’t use a simple average. Instead, our system considers things like how recent a review is and if the reviewer bought the item on Amazon. It also analyzed reviews to verify trustworthiness.
No customer reviews
- About Amazon
- Investor Relations
- Amazon Devices
- Amazon Science
- Sell products on Amazon
- Sell on Amazon Business
- Sell apps on Amazon
- Become an Affiliate
- Advertise Your Products
- Self-Publish with Us
- Host an Amazon Hub
- › See More Make Money with Us
- Amazon Business Card
- Shop with Points
- Reload Your Balance
- Amazon Currency Converter
- Amazon and COVID-19
- Your Account
- Your Orders
- Shipping Rates & Policies
- Returns & Replacements
- Manage Your Content and Devices
- Amazon Assistant
- Conditions of Use
- Privacy Notice
- Consumer Health Data Privacy Disclosure
- Your Ads Privacy Choices
How technology is shaping learning in higher education
About the authors.
This article is a collaborative effort by Claudio Brasca, Charag Krishnan , Varun Marya , Katie Owen, Joshua Sirois, and Shyla Ziade, representing views from McKinsey’s Education Practice.
The COVID-19 pandemic forced a shift to remote learning overnight for most higher-education students, starting in the spring of 2020. To complement video lectures and engage students in the virtual classroom, educators adopted technologies that enabled more interactivity and hybrid models of online and in-person activities. These tools changed learning, teaching, and assessment in ways that may persist after the pandemic. Investors have taken note. Edtech start-ups raised record amounts of venture capital in 2020 and 2021, and market valuations for bigger players soared.
A study conducted by McKinsey in 2021 found that to engage most effectively with students, higher-education institutions can focus on eight dimensions of the learning experience. In this article, we describe the findings of a study of the learning technologies that can enable aspects of several of those eight dimensions (see sidebar “Eight dimensions of the online learning experience”).
Eight dimensions of the online learning experience
Leading online higher-education institutions focus on eight key dimensions of the learning experience across three overarching principles.
Seamless journey
Clear education road map: “My online program provides a road map to achieve my life goals and helps me structure my day to day to achieve steady progress.”
Seamless connections: “I have one-click access to classes and learning resources in the virtual learning platform through my laptop or my phone.”
Engaging teaching approach
Range of learning formats: “My program offers a menu of engaging courses with both self-guided and real-time classes, and lots of interaction with instructors and peers.”
Captivating experiences: “I learn from the best professors and experts. My classes are high quality, with up-to-date content.”
Adaptive learning: “I access a personalized platform that helps me practice exercises and exams and gives immediate feedback without having to wait for the course teacher.”
Real-world skills application: “My online program helps me get hands-on practice using exciting virtual tools to solve real-world problems.”
Caring network
Timely support: “I am not alone in my learning journey and have adequate 24/7 support for academic and nonacademic issues.”
Strong community: “I feel part of an academic community and I’m able to make friends online.”
In November 2021, McKinsey surveyed 600 faculty members and 800 students from public and private nonprofit colleges and universities in the United States, including minority-serving institutions, about the use and impact of eight different classroom learning technologies (Exhibit 1). (For more on the learning technologies analyzed in this research, see sidebar “Descriptions of the eight learning technologies.”) To supplement the survey, we interviewed industry experts and higher-education professionals who make decisions about classroom technology use. We discovered which learning tools and approaches have seen the highest uptake, how students and educators view them, the barriers to higher adoption, how institutions have successfully adopted innovative technologies, and the notable impacts on learning (for details about our methodology, see sidebar “About the research”).
Double-digit growth in adoption and positive perceptions
Descriptions of the eight learning technologies.
- Classroom interactions: These are software platforms that allow students to ask questions, make comments, respond to polls, and attend breakout discussions in real time, among other features. They are downloadable and accessible from phones, computers, and tablets, relevant to all subject areas, and useful for remote and in-person learning.
- Classroom exercises: These platforms gamify learning with fun, low-stakes competitions, pose problems to solve during online classes, allow students to challenge peers to quizzes, and promote engagement with badges and awards. They are relevant to all subject areas.
- Connectivity and community building: A broad range of informal, opt-in tools, these allow students to engage with one another and instructors and participate in the learning community. They also include apps that give students 24/7 asynchronous access to lectures, expanded course materials, and notes with enhanced search and retrieval functionality.
- Group work: These tools let students collaborate in and out of class via breakout/study rooms, group preparation for exams and quizzes, and streamlined file sharing.
- Augmented reality/virtual reality (AR/VR): Interactive simulations immerse learners in course content, such as advanced lab simulations for hard sciences, medical simulations for nursing, and virtual exhibit tours for the liberal arts. AR can be offered with proprietary software on most mobile or laptop devices. VR requires special headsets, proprietary software, and adequate classroom space for simultaneous use.
- AI adaptive course delivery: Cloud-based, AI-powered software adapts course content to a student’s knowledge level and abilities. These are fully customizable by instructors and available in many subject areas, including business, humanities, and sciences.
- Machine learning–powered teaching assistants: Also known as chatbot programs, machine learning–powered teaching assistants answer student questions and explain course content outside of class. These can auto-create, deliver, and grade assignments and exams, saving instructors’ time; they are downloadable from mobile app stores and can be accessed on personal devices.
- Student progress monitoring: These tools let instructors monitor academic progress, content mastery, and engagement. Custom alerts and reports identify at-risk learners and help instructors tailor the content or their teaching style for greater effectiveness. This capability is often included with subscriptions to adaptive learning platforms.
Survey respondents reported a 19 percent average increase in overall use of these learning technologies since the start of the COVID-19 pandemic. Technologies that enable connectivity and community building, such as social media–inspired discussion platforms and virtual study groups, saw the biggest uptick in use—49 percent—followed by group work tools, which grew by 29 percent (Exhibit 2). These technologies likely fill the void left by the lack of in-person experiences more effectively than individual-focused learning tools such as augmented reality and virtual reality (AR/VR). Classroom interaction technologies such as real-time chatting, polling, and breakout room discussions were the most widely used tools before the pandemic and remain so; 67 percent of survey respondents said they currently use these tools in the classroom.
About the research
In November 2021, McKinsey surveyed 634 faculty members and 818 students from public, private, and minority-serving colleges and universities over a ten-day period. The survey included only students and faculty who had some remote- or online-learning experience with any of the eight featured technologies. Respondents were 63 percent female, 35 percent male, and 2 percent other gender identities; 69 percent White, 18 percent Black or African American, 8 percent Asian, and 4 percent other ethnicities; and represented every US region. The survey asked respondents about their:
- experiences with technology in the classroom pre-COVID-19;
- experiences with technology in the classroom since the start of the COVID-19 pandemic; and
- desire for future learning experiences in relation to technology.
The shift to more interactive and diverse learning models will likely continue. One industry expert told us, “The pandemic pushed the need for a new learning experience online. It recentered institutions to think about how they’ll teach moving forward and has brought synchronous and hybrid learning into focus.” Consequently, many US colleges and universities are actively investing to scale up their online and hybrid program offerings .
Differences in adoption by type of institution observed in the research
- Historically Black colleges and universities (HBCUs) and tribal colleges and universities made the most use of classroom interactions and group work tools (55 percent) and the least use of tools for monitoring student progress (15 percent).
- Private institutions used classroom interaction technologies (84 percent) more than public institutions (63 percent).
- Public institutions, often associated with larger student populations and course sizes, employed group work and connectivity and community-building tools more often than private institutions.
- The use of AI teaching-assistant technologies increased significantly more at public institutions (30 percent) than at private institutions (9 percent), though overall usage remained comparatively higher at private institutions.
- The use of tools for monitoring student progress increased by 14 percent at private institutions, versus no growth at public institutions.
Some technologies lag behind in adoption. Tools enabling student progress monitoring, AR/VR, machine learning–powered teaching assistants (TAs), AI adaptive course delivery, and classroom exercises are currently used by less than half of survey respondents. Anecdotal evidence suggests that technologies such as AR/VR require a substantial investment in equipment and may be difficult to use at scale in classes with high enrollment. Our survey also revealed utilization disparities based on size. Small public institutions use machine learning–powered TAs, AR/VR, and technologies for monitoring student progress at double or more the rates of medium and large public institutions, perhaps because smaller, specialized schools can make more targeted and cost-effective investments. We also found that medium and large public institutions made greater use of connectivity and community-building tools than small public institutions (57 to 59 percent compared with 45 percent, respectively). Although the uptake of AI-powered tools was slower, higher-education experts we interviewed predict their use will increase; they allow faculty to tailor courses to each student’s progress, reduce their workload, and improve student engagement at scale (see sidebar “Differences in adoption by type of institution observed in the research”).
While many colleges and universities are interested in using more technologies to support student learning, the top three barriers indicated are lack of awareness, inadequate deployment capabilities, and cost (Exhibit 3).
Students want entertaining and efficient tools
More than 60 percent of students said that all the classroom learning technologies they’ve used since COVID-19 began had improved their learning and grades (Exhibit 4). However, two technologies earned higher marks than the rest for boosting academic performance: 80 percent of students cited classroom exercises, and 71 percent cited machine learning–powered teaching assistants.
Although AR/VR is not yet widely used, 37 percent of students said they are “most excited” about its potential in the classroom. While 88 percent of students believe AR/VR will make learning more entertaining, just 5 percent said they think it will improve their ability to learn or master content (Exhibit 5). Industry experts confirmed that while there is significant enthusiasm for AR/VR, its ability to improve learning outcomes is uncertain. Some data look promising. For example, in a recent pilot study, 1 “Immersive biology in the Alien Zoo: A Dreamscape Learn software product,” Dreamscape Learn, accessed October 2021. students who used a VR tool to complete coursework for an introductory biology class improved their subject mastery by an average of two letter grades.
Faculty embrace new tools but would benefit from more technical support and training
Faculty gave learning tools even higher marks than students did, for ease of use, engagement, access to course resources, and instructor connectivity. They also expressed greater excitement than students did for the future use of technologies. For example, while more than 30 percent of students expressed excitement for AR/VR and classroom interactions, more than 60 percent of faculty were excited about those, as well as machine learning–powered teaching assistants and AI adaptive technology.
Eighty-one percent or more of faculty said they feel the eight learning technology tools are a good investment of time and effort relative to the value they provide (Exhibit 6). Expert interviews suggest that employing learning technologies can be a strain on faculty members, but those we surveyed said this strain is worthwhile.
While faculty surveyed were enthusiastic about new technologies, experts we interviewed stressed some underlying challenges. For example, digital-literacy gaps have been more pronounced since the pandemic because it forced the near-universal adoption of some technology solutions, deepening a divide that was unnoticed when adoption was sporadic. More tech-savvy instructors are comfortable with interaction-engagement-focused solutions, while staff who are less familiar with these tools prefer content display and delivery-focused technologies.
According to experts we interviewed, learning new tools and features can bring on general fatigue. An associate vice president of e-learning at one university told us that faculty there found designing and executing a pilot study of VR for a computer science class difficult. “It’s a completely new way of instruction. . . . I imagine that the faculty using it now will not use it again in the spring.” Technical support and training help. A chief academic officer of e-learning who oversaw the introduction of virtual simulations for nursing and radiography students said that faculty holdouts were permitted to opt out but not to delay the program. “We structured it in a ‘we’re doing this together’ way. People who didn’t want to do it left, but we got a lot of support from vendors and training, which made it easy to implement simulations.”
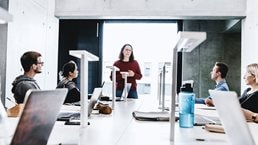
Reimagining higher education in the United States
Takeaways from our research.
Despite the growing pains of digitizing the classroom learning experience, faculty and students believe there is a lot more they can gain. Faculty members are optimistic about the benefits, and students expect learning to stay entertaining and efficient. While adoption levels saw double-digit growth during the pandemic, many classrooms have yet to experience all the technologies. For institutions considering the investment, or those that have already started, there are several takeaways to keep in mind.
- It’s important for administration leaders, IT, and faculty to agree on what they want to accomplish by using a particular learning technology. Case studies and expert interviews suggest institutions that seek alignment from all their stakeholders before implementing new technologies are more successful. Is the primary objective student engagement and motivation? Better academic performance? Faculty satisfaction and retention? Once objectives are set, IT staff and faculty can collaborate more effectively in choosing the best technology and initiating programs.
- Factor in student access to technology before deployment. As education technology use grows, the digital divide for students puts access to education at risk. While all the institution types we surveyed use learning technologies in the classroom, they do so to varying degrees. For example, 55 percent of respondents from historically Black colleges and universities and tribal colleges and universities use classroom interaction tools. This is lower than public institutions’ overall utilization rate of 64 percent and private institutions’ utilization rate of 84 percent. Similarly, 15 percent of respondents from historically Black colleges and universities and tribal colleges and universities use tools for monitoring student progress, while the overall utilization rate for both public and private institutions is 25 percent.
- High-quality support eases adoption for students and faculty. Institutions that have successfully deployed new learning technologies provided technical support and training for students and guidance for faculty on how to adapt their course content and delivery. For example, institutions could include self-service resources, standardize tools for adoption, or provide stipend opportunities for faculty who attend technical training courses. One chief academic officer told us, “The adoption of platforms at the individual faculty level can be very difficult. Ease of use is still very dependent upon your IT support representative and how they will go to bat to support you.”
- Agree on impact metrics and start measuring in advance of deployment. Higher-education institutions often don’t have the means to measure the impact of their investment in learning technologies, yet it’s essential for maximizing returns. Attributing student outcomes to a specific technology can be complex due to the number of variables involved in academic performance. However, prior to investing in learning technologies, the institution and its faculty members can align on a core set of metrics to quantify and measure their impact. One approach is to measure a broad set of success indicators, such as tool usage, user satisfaction, letter grades, and DFW rates (the percentage of students who receive a D, F, or Withdraw) each term. The success indicators can then be correlated by modality—online versus hybrid versus in-class—to determine the impact of specific tools. Some universities have offered faculty grants of up to $20,000 for running pilot programs that assess whether tools are achieving high-priority objectives. “If implemented properly, at the right place, and with the right buy-in, education technology solutions are absolutely valuable and have a clear ROI,” a senior vice president of academic affairs and chief technology officer told us.
In an earlier article , we looked at the broader changes in higher education that have been prompted by the pandemic. But perhaps none has advanced as quickly as the adoption of digital learning tools. Faculty and students see substantial benefits, and adoption rates are a long way from saturation, so we can expect uptake to continue. Institutions that want to know how they stand in learning tech adoption can measure their rates and benchmark them against the averages in this article and use those comparisons to help them decide where they want to catch up or get ahead.
Claudio Brasca is a partner in McKinsey’s Bay Area office, where Varun Marya is a senior partner; Charag Krishnan is a partner in the New Jersey office; Katie Owen is an associate partner in the St. Louis office, where Joshua Sirois is a consultant; and Shyla Ziade is a consultant in the Denver office.
The authors wish to thank Paul Kim, chief technology officer and associate dean at Stanford School of Education, and Ryan Golden for their contributions to this article.
Explore a career with us
Related articles.

Setting a new bar for online higher education
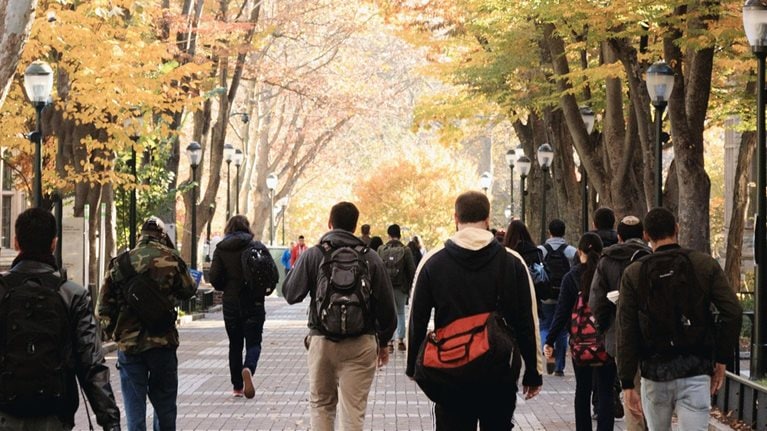
How to transform higher-education institutions for the long term
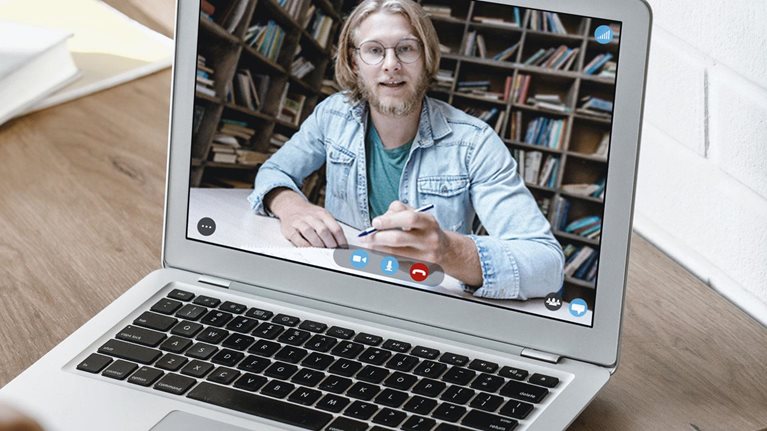
Scaling online education: Five lessons for colleges
- Search Menu
- Sign in through your institution
- Advance Articles
- Author Guidelines
- Open Access Options
- Self-Archiving Policy
- About International Journal of Low-Carbon Technologies
- Editorial Board
- Advertising and Corporate Services
- Journals Career Network
- Dispatch Dates
- Journals on Oxford Academic
- Books on Oxford Academic

Article Contents
1 introduction, 2 case study: shunde polytechnic, 3 methodology, 4 results and discussion, 5 conclusions, acknowledgements, author contributions.
- < Previous
Study on the effects of low-carbon education on the carbon emissions of college students: a case study in Guangdong Province

- Article contents
- Figures & tables
- Supplementary Data
Zhang Junting, Lyu Shun, Chen Zefeng, Study on the effects of low-carbon education on the carbon emissions of college students: a case study in Guangdong Province, International Journal of Low-Carbon Technologies , Volume 19, 2024, Pages 1425–1431, https://doi.org/10.1093/ijlct/ctae097
- Permissions Icon Permissions
College students are the main force in low-carbon society and social governance, and therefore, their awareness of low-carbon is of vital importance. This article indicates a case study about the effects of low-carbon education on the carbon emissions of college students. In 2019, low-carbon education was promoted on the campus of Shunde Polytechnic and questionnaire survey and interview method were used to study the carbon emissions and low-carbon behaviors of college students after 3 years. Based on the results, the carbon emissions of college students in the aspects of food and daily use accounted for 53.8% and 40.8%, respectively, of the total carbon emissions. In the aspect of food, wine and staple foods accounted for a higher proportion. Their per capital carbon emissions were 291.536 kg and 454.651 kg, respectively. The main contributor to daily use was laptops, which emitted 530.351 kg of carbon per capital. The carbon emissions of clothing, housing, and transportation were relatively low. It is found that low-carbon facilities and measures and low-carbon living environments in colleges have a very positive effect on the reduction of carbon emissions of college students. But the disunity between knowing and doing is still common among college students. Therefore, further measures must be taken to improve the situation.
Over the past decades, China’s economy has achieved rapid development, accompanied by the increasing consumption of fossil energy such as oil and coal. In this process, the ecological environment has suffered serious damage, such as global warming, sea level rise, and many other hazards [ 1 ]. These problems are contrary to the direction of realizing sustainable economic and social development.
According to the latest analysis data from the International Energy Agency (IEA), China has become the largest carbon emitter. In 2021, China’s carbon dioxide emissions exceeded 12.9 billion tons, accounting for 33% of the global total and twice that of the USA. According to the China Energy Big Data Report (2022), China’s coal consumption in 2021 was 4.87 billion tons, an increase of 4% year-on-year, and coal accounted for 47.7% of the total energy consumption, which is related to the coal-dominated energy consumption structure in China [ 2 ]. In the long run, the traditional energy production and consumption structure is not sustainable, and the damage caused to the ecological environment is irreversible. In this case, many countries begin to attach importance to low-carbon economies and use various fiscal and tax policies to promote the development of low-carbon economies. The development of low-carbon economy has become a new direction for China’s economic transformation [ 3 ].
In light of its economic development, the Chinese government has proposed to build and improve a green, low-carbon, and circular economic system and achieve the commitment of “carbon peak” by 2030 and “carbon neutrality” by 2060 at an early date. In October 2021, the Chinese government proposed to establish a green and low carbon development economic system and promote the transformation of economic and social development to green and low carbon, which is the long-term strategy to realize sustainable development [ 4 ].
College students are important driving forces for environmental protection and sustainable development. Their understanding of the environment will determine the development trend of social environment and ecology in the future. Improving their environmental literacy has become a strategic task for the whole society. As a special group, college students have strong plasticity and influence [ 5 ]. On the one hand, there are still high carbon behaviors in college students’ living habits, but based on their plasticity. It is feasible to study and analyze college students’ behaviors and give them correct guidance. On the other hand, the behavioral concept of college students can produce a demonstration effect, which has a strong social radiation effect. Therefore, improving the low-carbon literacy of college students is an important aspect of accelerating the formation of low-carbon environmental protection lifestyle and consumption mode in the public, which is conducive to the formation of a low energy, low consumption, and low-expenditure lifestyle in the whole society and promoting the construction of ecological civilization [ 6 ].
Low-carbon work has gradually shifted from the macro perspective to individual lifestyle carbon emissions. Shui and Dowlatabadi [ 7 ] propose the consumer lifestyle model law to explore the impact of consumer lifestyle on the environment in 2005. In 2006, Britain’s environment minister proposed a personal carbon cap [ 8 ]. Rahman et al. [ 9 ] introduced the Carbon Footprint Calculator app in 2009 as a basis for dynamically tracking individual carbon footprints. In 2009, Riddell et al . [ 10 ] estimated that college students each produced nearly 4 t carbon emissions per year. In 2013, Larsen et al. [ 11 ] calculated the carbon footprint of the Norwegian University of Science and Technology by applying the environment extended I/O model, showing that the average student contribution to carbon emissions was 4.6 t/a.
The current research on college students’ carbon emissions mainly focuses on four aspects: (i) The analysis of college students’ carbon footprint and the research on emission reduction measures. Yixin et al. ’s research [ 12 ] shows that the carbon emissions of college students in Beijing have different proportions in different aspects of clothing, food, housing, and transportation; (ii) Research on low carbon lifestyle of college students. Zeguang et al . [ 13 ] studied the cognition and practice of low carbon consumption among college students and pointed out that college students should establish a correct consumption concept, put forward a series of measures to practice low carbon lifestyle and (iii) explore the relevant influencing factors of college students’ low-carbon consumption behavior. Ma Xiaoxu’s research [ 14 ] shows that environmental responsibility awareness, environmental impact concern, and low carbon concern have a significant impact on college students’ low carbon consumption intention; (iv) Research on college students’ low-carbon life education. Xin and Zhendong’s research [ 15 ] shows that low-carbon life education aims to cultivate low-carbon values, develop ecological morality, establish a correct view of nature, and disseminate low-carbon ideas, popularize low-carbon knowledge, develop low-carbon behavior content, and cultivate group and individual autonomy and consciousness of low-carbon life. In a word, the current research on college students’ carbon emissions shows multiangle and multilevel development, which has guiding significance for the research in this article.
In this article, a college in Guangdong, China was taken as a case study, which lasted for 3 years (from 2019 to 2022). In this study, the students’ carbon emission behavior was firstly investigated and suggestions for carbon reduction at campus were given, and the college adopted several measures of low-carbon education to improve the students’ carbon emission behavior, then an investigation was conducted again to know the students carbon footprints. By comparing the results before and after college’s activity, the effects of low-carbon education could be fully understood, and effective suggestion could also be provided. The investigation and research in this article not only grasp an important aspect of college students’ culture, moral quality, and sense of mission but also have important academic value and extensive social significance.
Shunde Polytechnic is a public college in Guangdong Province which is located in the south China. According to the statistical data for the year 2022, the campus covers an area of 1.2 million m 2 , with a construction area of more than 600 000 m 2 . It has 11 secondary colleges and 53 majors, with a total of 16 000 full-time students, 2781 adult education students, and 1086 faculty and staff members.
Figure 1 shows the CO 2 emission of university students from different countries compared to other countries. The Chinese students have much smaller CO 2 emission. But there is still space for CO 2 emission reduction among the Chinese university students. In order to better understand the students’ carbon footprints, a survey has been conducted in Shunde Polytechnic to investigate the students’ energy behavior in 2019. Figure 2 shows the composition of students’ energy consumption. It can be seen that students consume more electricity in dorms and research building, and the electricity in these two places can easily be affected by students’ behavior.
![case study methodology in higher education The CO2 emission of university students from different countries [16].](https://oup.silverchair-cdn.com/oup/backfile/Content_public/Journal/ijlct/19/10.1093_ijlct_ctae097/2/m_ctae097f1.jpeg?Expires=1720806303&Signature=Yj5-jF7jHt8FirDs7rJd1h4qb06mIC7bWRVm1u3y~3Xau-OGJeF3~0l4Z2VZXWXsU~P9WmLC4qbCwP2Z6xLGvcFR9YlbqVKtSRKbOYvLwF2dAn1ESIklCgNVbBiKFcJcp8UmMD4U~xnG4WS9xqy0aGcFa~jwA4yuROWDFeI1gbTptTzU5abdZmejq-GJp5u02I1429nvunvGQH3lTcuVugcCK83hji7UV8Fc8rrMYhZCWtyyWqlInlHsWghvxurGhcBb7m3eYK5ZNmO7wgw1sH2cRHaiSAgPgk1OmmhK4aVEGzrSsLdDztxQlxdzBoVpgYwoONe~o91yRciDDnNMQA__&Key-Pair-Id=APKAIE5G5CRDK6RD3PGA)
The CO 2 emission of university students from different countries [ 16 ].

The composition of students’ energy consumption in 2019.
To further reduce the CO 2 emission of students at Shunde Polytechnic, the college proposed several low-carbon education measures, e.g. low-carbon seminar, poster, low-carbon behavior evaluation, etc. After 3 years of adjustment, a carbon footprint investigation was conducted again to obtain an in-depth understanding on the effects of low-carbon education on the carbon footprint of college students, and corresponding measures for further carbon reduction were proposed based on the research.
3.1 Carbon emission calculation
At present, there are two authoritative methods for the calculation of carbon emissions, one is from a macro perspective, and the other is from a micro perspective. For the calculation from the macro perspective, the guidance document is mainly based on the ‘National Greenhouse Gas Inventory’ guidelines issued by the United Nations. In the calculation of carbon emissions, it is necessary to first classify carbon emission sources and establish a subset until all carbon emission sources are covered. For the calculation from the micro perspective, the most critical step is to calculate the carbon emission factor of different products, obtain the unit carbon emission of the product, and obtain the total carbon emissions. In this article, the calculation method from the bottom to the top microlevel is used to determine the carbon emission factors and calculate the total carbon emission. The expression is as follows:
where CE is the carbon emissions of a certain lifestyle; A x means the activity level of one detail in the lifestyle; and EF x refers to the carbon emission factor of one detail in the lifestyle. The carbon emission factors of different items are shown in Table 1 .
Carbon emission factors [ 17 ] .
. | . | . | . |
---|---|---|---|
Housing | Water | 0.93 | kgCO /m |
Electricity | 0.612 | kgCO /kWh | |
Clothing | Cloths | 5.7 | kgCO /piece |
Washing powder | 0.72 | kgCO /kg | |
Transportation | Buses | 0.0045 | kgCO /min |
Taxis | 0.15 | kgCO /min | |
Electric motor | 0.0535 | kgCO /min | |
Bicycle | 0.0113 | kgCO /min | |
Food | Rice | 2.7 | kgCO /kg |
Wheaten food | 1.25 | kgCO /kg | |
Beef and mutton | 1.3657 | kgCO /kg | |
Pork | 1.1892 | kgCO /kg | |
Fruits | 0.0498 | kgCO /kg | |
Vegetables | 0.0274 | kgCO /kg | |
Milk | 0.4256 | kgCO /kg | |
Eggs | 0.9026 | kgCO /kg | |
Alcohol | 1.1293 | kgCO /kg | |
Cigarette | 0.96 | kgCO /package | |
Daily use | Plastic bags | 0.0001 | kgCO /each |
Disposable cutlery | 0.023 | kgCO /set | |
Paper products | 3.5 | kgCO /kg | |
Laptops | 482 | kgCO /each |
. | . | . | . |
---|---|---|---|
Housing | Water | 0.93 | kgCO /m |
Electricity | 0.612 | kgCO /kWh | |
Clothing | Cloths | 5.7 | kgCO /piece |
Washing powder | 0.72 | kgCO /kg | |
Transportation | Buses | 0.0045 | kgCO /min |
Taxis | 0.15 | kgCO /min | |
Electric motor | 0.0535 | kgCO /min | |
Bicycle | 0.0113 | kgCO /min | |
Food | Rice | 2.7 | kgCO /kg |
Wheaten food | 1.25 | kgCO /kg | |
Beef and mutton | 1.3657 | kgCO /kg | |
Pork | 1.1892 | kgCO /kg | |
Fruits | 0.0498 | kgCO /kg | |
Vegetables | 0.0274 | kgCO /kg | |
Milk | 0.4256 | kgCO /kg | |
Eggs | 0.9026 | kgCO /kg | |
Alcohol | 1.1293 | kgCO /kg | |
Cigarette | 0.96 | kgCO /package | |
Daily use | Plastic bags | 0.0001 | kgCO /each |
Disposable cutlery | 0.023 | kgCO /set | |
Paper products | 3.5 | kgCO /kg | |
Laptops | 482 | kgCO /each |
3.2 Data collection
This research follows three basic principles: the combination of theoretical analysis and practice, the combination of qualitative analysis and quantitative research, and interdisciplinary research. In terms of specific methods of data collection, it mainly adopts the literature analysis method, questionnaire survey method, and interview method.
(1) Literature analysis
The literature analysis method is a theoretical method of modern science and technology research, that deeply consults, analyzes, and sorts out the scientific data of the literature of related things and uses it to find the physical nature and scientific attributes of related things. In terms of this research, literature related to carbon emission assessment, low carbon environmental protection education for college students, and low carbon environmental protection social governance was collected and sorted out, and some relevant literature was reviewed.
(2) Questionnaire survey
Questionnaire survey is a research method that collects relevant information by asking questions in written form. Students from a college in Guangdong, China were selected as key respondents and totally 1200 students were investigated randomly by questionnaires and 1097 valid questionnaires were collected. Considering the impact of age and gender on the research results, the age and gender of the respondents are both generally equally distributed.
The purpose of the questionnaire survey was to understand the low-carbon environmental awareness and behavior of college students, and the survey object was mainly college students. The students who participated in the survey were freshman, sophomore, junior, and senior, accounting for 22.17%, 23.22%, 33.48%, and 21.13%, respectively. Among the students who participated in this questionnaire survey, male students accounted for 49.5% of the total number, while female students accounted for 50.5%. From this data, it can be seen that the students participating in the survey come from different genders and grades, and the questionnaire had certain rationality.
The questionnaire mainly consisted of two parts. The first part was about carbon emissions of college students. From the aspects of clothing, food, housing, transportation, daily use, and environmental protection behavior, the differences in carbon emission behavior of college students were analyzed from four aspects: consumption characteristics, individual characteristics, consciousness characteristics and behavior characteristics. The main influencing factors of carbon emission were found. The detailed contents information of this part of questionnaire is shown in Table 2 . The second part of the questionnaire was about environmental protection awareness, attitude to low-carbon living, low-carbon education in college, and importance of low-carbon education for social governance. The main aim of this part was to obtain an in-depth understanding of students’ environmental protection behavior.
(3) Interview
Detailed contents of different aspects in the questionnaire .
. | . |
---|---|
Clothing | The purchase of clothing, washing powder, and washing liquid in each school year |
Food | Staple food, complementary food, fruit, milk, alcohol, and tobacco |
Housing | Daily water and electricity use |
Transportation | The time and means of transportation used in daily trip |
Daily use | Daily use of electronic equipment, disposable chopsticks, express delivery, paper products, and plastic bags |
. | . |
---|---|
Clothing | The purchase of clothing, washing powder, and washing liquid in each school year |
Food | Staple food, complementary food, fruit, milk, alcohol, and tobacco |
Housing | Daily water and electricity use |
Transportation | The time and means of transportation used in daily trip |
Daily use | Daily use of electronic equipment, disposable chopsticks, express delivery, paper products, and plastic bags |
The interview method mainly refers to a method of collecting other relevant resources and literature in a purposeful, reasonable, and systematic way, in which the investigator conducts oral conversations with other respondents and asks them about their feelings or views about the phenomenon in a specific time or situation. In this actual research, the authors first prepared an interview outline on the basis of fully understanding the research content, and then conducted in-depth interviews with college students, college teachers, and social personnel. The interview could help the authors to gain a well understanding of the status of low-carbon education among college students from a more comprehensive perspective and help us further understand the importance of low carbon education for the development of students and social governance. Finally, the interview results are helpful to provide reasonable suggestions for further improvement of low-carbon education for college students.
4.1 Preliminary statistics of questionnaire survey
Data observed from the questionnaire were firstly collected and analyzed. It can be observed from the data that from the aspect of water usage, the number of college students taking a shower less than 100 minutes per week accounted for 66.5%, 100–200 minutes accounted for 26.8%, and more than 200 minutes accounted for 6.7%. Most students used water for a reasonable time, but there were some students who used water for a long time, resulting in a waste of resources. The electricity in the dormitory was limited by the objective conditions of the dormitory, which were basically limited to lighting electricity and low-power electrical appliances, and the most important high-power electrical facilities were air conditioners. In this respect, carbon emissions have not changed significantly from before.
According to public statistics, the carbon emissions of private transportation in China show an increasing trend year by year. Among public transportation, taxis account for the largest proportion of carbon emissions. The survey found that more than 86.2% of college students chose low-carbon emission transportation modes, such as buses and bicycles, when traveling. Due to the reasons of COVID-19, most college students only had activities near the college, and even if they need to travel, the travel time was short. In terms of the choice of travelling tools, most college students were more inclined to choose public transportation, so the carbon emissions caused by traveling are relatively small.
In terms of the use of disposable tableware, 55.6% of the students used disposable tableware between 0 and 20 times per week, and the carbon emissions generated by disposable tableware accounted for 48.3% of the survey group. More than 42.2% of students used disposable tableware more than 20 times a week, accounting for a high proportion, indicating that there was a large space for improving students’ low-carbon awareness.
4.2 Carbon emission structure analysis
According to the data obtained from the survey, the selection of main activity data in the analysis is introduced: The quantity of clothing purchased and the laundry behavior will have a great impact on the carbon emissions of clothing. In the aspect of clothing, the excessive carbon emission was obvious, so the quantity of clothing and the amount of laundry detergent were selected as the main factors affecting clothing. The amount of food has an important impact on carbon emissions. In terms of food, staple food, auxiliary food, and daily consumption of fruit, milk, wine, and tobacco were selected as the measurement criteria. Water resources are widely used in the life of college students, and the resulting carbon emissions cannot be underestimated. Therefore, the water that college students frequently use in daily life was selected for consideration. For daily consumption products, express delivery, plastic bags, disposable chopsticks, laptops, and paper products that had a greater impact on carbon emissions were selected for analysis. In terms of transportation, the variables of subway, bus, taxi, electric vehicle, and bicycle were selected for analysis. Through the above analysis, the numerical characteristics of carbon emissions from students’ life in Shunde Polytechnic can be found, and the distribution of carbon emissions from the investigated objects can be better reflected.
According to the analysis results for each variable, it can be seen that the distribution basically conforms to the sampling requirements. The total carbon emission of college students at Shunde Polytechnic was 18201.72 kg, of which the per capital carbon emission of clothing, food, housing, transportation, and daily use were 819.08, 9592.31, 91.01, 418.64, and 7280.69 kg, respectively.
The proportions of carbon emissions from different aspects of college students are shown in Fig. 3 . It can be seen that the life carbon emissions of college students at Shunde Polytechnic are mainly concentrated in the aspects of food and daily use, with the total proportion of about 94%. Carbon emissions from food occupy the first place in all aspects of life. The shares of carbon emissions from different food types in colleges are shown in Fig. 4 , among them, vegetables, eggs, fruits, milk, meat, tobacco, drinks, staple food, and other carbon emissions accounted for 0.19%, 2.16%, 0.32%, 1.86%, 21.44%, 1.07%, 28.49%, and 44.47% of the food carbon emissions, respectively. Alcohol and staple foods accounted for 72.96% of food’s total carbon emissions. It can be seen that the unreasonable diet structure of students in Shunde Polytechnic was very serious, and there were generally bad habits such as picky eating and drinking drinks. In this aspect, there is a large space for carbon emission reduction. From the perspective of per capital carbon emissions of specific behaviors, carbon emissions from clothing purchased during the school year accounted for a relatively large proportion in the aspect of clothing, whose per capital carbon emissions are 40.597 kg. In terms of food, drinks and staple foods occupied a relatively large proportion of carbon emissions (291.536 kg and 454.651 kg, respectively). In the daily use aspect, which accounted for a relatively large of carbon emissions was the laptop with a per capital carbon emissions as high as 530.351 kg, plastic bags released less carbon emissions with a per capital carbon emissions of only 0.0002 kg. It can be seen that most of the college students have a healthy and good living habits. In terms of transportation, the carbon emission is relatively small due to the influence of COVID-19 in recent years. The proportion of carbon emissions from food and daily use is closely related to the higher education environment received by college students, but there is still a large part of the room for emission reduction.

Carbon emission of college students from different aspects.

The shares of carbon emissions from different food types in colleges.
In terms of environmental behavior, students at Shunde Polytechnic are analyzed in four aspects: waste clothing disposal method, daily traveling method, meal surplus, and disposable container use. Among them, 15.4% of students chose to discard their old clothing, 23.4% chose to donate them, 5.6% chose others, and 55.6% chose to leave them idle. Among the daily travel modes, 82.4% of college students choose walking, 7.1% choose electric vehicles, 6.8% choose bicycles, 1.4% choose buses, and nearly 1.0% choose taxis, rail transit, and motor vehicles. 57.9% of students have little surplus, 37.9% have little surplus, and 4.2% have a lot of surplus. In terms of takeaway containers, 61.2% of college students used plastic bags, 27.9% used disposable plastic cutlery, 0.9% used paper bags, and 10.0% used self-catering cutlery.
According to the results of relevant analysis, 68.1% of college students in Foshan choose to discard or idle old clothing, indicating that college students still have not completed the effective use of clothing in the disposal of old clothing. In terms of daily travel, 89.2% of college students chose walking and cycling to travel, indicating that the students possess low-carbon behavior in traveling. In terms of meal leftovers, 95.8% of college students have little leftovers, and their overall behavior is good. In terms of disposable container, 89.1% of college students choose to use plastic bags and disposable plastic tableware. The main reason is that most students give priority to the convenience of take-out food, resulting in the lack of environmental protection behavior.
4.3 Low-carbon awareness analysis
In the following research, the authors investigated the low-carbon awareness of college students by questionnaires, and the main results are presented and discussed in this part.
In the respondents, 42% of the students think that individuals play a very large role in low-carbon process, while 48% of the students think individuals play a relatively large role. However, there are still 10% of the students think that individuals play no role in low-carbon process. As to whether they pay attention to the news about environmental protection and carbon reduction, 26% of the students answered often, 62% chose occasionally, and 12% never pay attention to the news about environmental protection. This shows that college students’ attention to low-carbon issues needs to be strengthened.
About the understanding level of the concept of low carbon and environmental protection, 58.60% of the students knew roughly the meaning and content, 25.15% of the students were familiar with the meaning and content, 12.12% of the students had heard but did not know the meaning, and 4.13% of the students had never heard. Only nearly 25% of students are familiar with the content and meaning of ‘low-carbon and environmental protection’, which is worth our deep thought. The college, government, and relevant groups should strengthen the publicity of the concept of ‘low-carbon environmental protection’ in colleges. Only by letting more people know this knowledge, we can achieve low-carbon environmental protection better.
What are the main channels through which college students learn about the concept of low carbon and environmental protection? According to the survey data, it is mainly through the Internet, accounting for 79.8%. In fact, this channel also reflects the contemporary college students contact with the Internet for lots of time. Most of the time is with computers and mobile phones. 55.6% of college students learn about it through TV and radio. The rest of the students learn mainly through newspapers, magazines, and other channels. Therefore, if colleges and relevant government departments want to increase the spread of the concept of low carbon and environmental protection among college students, releasing this knowledge on the Internet can be a good means of publicity.
In terms of students’ attitude toward low-carbon and environmental protection, we designed ‘Do you think the promotion and practice of low carbon and environmental protection are closely related to college students?’. According to the statistics, 66.70% of the students think it is relevant, college students are the main force in environmental protection; 9.09% of the students think it is irrelevant and think it is the government’s business; 24.21% of the students are not clear. It can be seen from this set of data that most students hold a positive attitude toward it, believing that the promotion of ‘low carbon and environmental protection’ is related to themselves. For those students whose attitude is not clear or negative, they should be guided positively and improve their understanding.
4.4 Interview analysis
To understand the status of low-carbon education in colleges, several college students and tutors were interviewed. The main aim of the interview was to collect the low-carbon activities and measures in colleges and suggestions from college students and tutors.
In terms of low-carbon activities and measures in colleges, about 55% interviewees thought it was not enough. The colleges put more attention on professional education, but less on low-carbon education as the main job of colleges is to solve the employment problem of students. Based on the interview of tutors, they were not forced to add low-carbon education to their courses because they had heavy teaching tasks. Besides, only little college associations are about low-carbon environmental protections, thus the opportunities for tutors and students to participate in low-carbon environmental activities are very limited, resulting in limited low-carbon propagandizing and popularizing.
Low-carbon living environment in colleges should be further built. For example, several students mentioned that they had limited methods to dispose waste cloths, based on previous questionnaire. 68.1% of college students choose to discard or idle old clothing. Based on the interview, the interviewees indicated that the handling facilities were not enough, and they had no way to sell or donate their clothes even they wished to. In the canteens, disposable plastic food container, cutlery, and plastic bags usually severed to students due to the severe take-out demand, but unfortunately, most of the students would not bring their own food containers. Furthermore, the canteens usually interfere with students’ meal choices very little, even the students waste much food, they do not need to take responsibility for your actions.
Last but not least, the disunity of knowing and doing is very common among college students. For example, nearly all the students know that the disposable cutlery has negative effects on the low-carbon process and environmental protection, 61.2% of college students will use plastic bags and 27.9% use disposable plastic cutlery because of the convenience and low cost of disposable cutlery. When questioned ‘would you support the ban on using disposable cutlery and plastic bags in the college canteen’, only 56% of the interviewees support it, which showed that there is still a distance between having environmental awareness and actually taking action to protect the environment.
4.5 Suggestions
Based on the research, several suggestions are proposed for the promotion of low-carbon education in colleges.
Firstly, we will promote the improvement of college students’ environmental literacy and normalize environmental science popularization. Specifically, measures can be taken from the following two aspects. Firstly, national environmental protection education, the annual ‘World Environment Day’, ‘National Tree Planting Week’, and other special activity days are used to organize various forms of activities to promote environmental protection. Secondly, it is necessary to create an environment protection atmosphere in the whole society and to guide young people to take an active part in environmental protection activities such as planting trees. Besides, it is urgent to improve and strengthen the organization of large-scale voluntary activities, as well as the training, incentive, guarantee, feedback, and supervision mechanism of volunteers.
Secondly, various activities should be taken to enhance public environmental awareness. First, public courses on environmental protection should be offered. Colleges and universities should set up courses related to ecological and environmental protection to improve the cognitive ability of environmental protection of college students and actively guide them to establish scientific ecological and environmental awareness. Second, various forms of environmental protection activities should be organized. By organizing environmental protection activities, college students can understand the importance of keeping the environment clean and maintaining the balance of the ecological environment and develop the good habit of protecting the environment. Thirdly, research on environmental protection should be encouraged. Colleges and universities can organize students to carry out research on environmental protection topics, so that students can ask questions, decide the direction of research, collect and sort out data, draw conclusions, and take social actions in the process of implementing project plans. By doing environmental protection research, the students’ environmental awareness could be improved.
Thirdly, students themselves should learn more about low-carbon life and eliminate the behavior of comparison and waste. From the perspective of the school, it is necessary to create a good low-carbon environment, provide students with low-carbon life as far as possible, and integrate low-carbon into their daily life.
Based on the collection and collation of 1097 valid questionnaires, this article conducted an in-depth exploration of different aspects of college students’ carbon emissions in Shunde Polytechnic. Based on the research, the following conclusions can be drawn:
(1) The carbon emissions of college students in the aspects of food and daily use accounted for 53.8% and 40.8%, relatively in the total carbon emissions. In the aspect of food, wine and staple food accounted for a higher proportion. Their per capital carbon emissions are 291. 536 kg and 454.651 kg, respectively. The main contributor to daily use is laptops, which emit 530.351 kg of carbon per capital.
(2) The carbon emissions of clothing, housing, and transportation are relatively low, respectively, accounting for 3.3%, 0.3%, and 1.8% of the total carbon emissions.
(3) It is found that the low-carbon facilities and measures and low-carbon living environments in colleges have a very positive effect on the reduction of carbon footprint of college students. But the disunity of knowing and doing is still common among college students. Therefore, further measures must be taken to improve the situation.
The authors would appreciate The Plan of Philosophy and Social Science of Guangdong Province 2023 Annual Discipline Co-Constructive Project (Project No. GD23XGL121), 2023 Young Innovative Talent Category of Colleges and Universities in Guangdong Province (Project No. 2023WQNCX255), Special Funds for the Cultivation of 2023 Guangdong College Students’ Scientific and Technological Innovation. (‘Climbing Program’ Special Funds Project No.pdjh2023b1077).
Junting Zhang (Formal analysis [equal], Investigation [equal], Methodology [equal]), Shun Lyu (CRediT contribution not specified), Shun Lyu (Conceptualization [lead], Funding acquisition [lead], Resources [lead], Supervision [lead]), Chen Zefeng (Data curation [equal], Software [equal], Validation [equal]).
None declared.
Xiang Y . Evaluating parallelized support vector regression and nearest neighbor regression with different input variations for estimating daily global solar radiation of the humid subtropical region in China . Int J Low-Carbon Technol 2023 ; 18 : 95 – 110 . https://doi.org/10.1093/ijlct/ctad005 .
Google Scholar
Yuekun L . Fiscal and taxation policy research on promoting low carbon economy development in Liaoning Province . Shenyang, China : Shenyang University , 2022 .
Google Preview
Shi H , Chai J , Quanying L . et al. The impact of China's low-carbon transition on economy, society and energy in 2030 based on CO2 emissions drivers . Energy 2022 ; 239 :122336. https://doi.org/10.1016/j.energy.2021.122336 .
Jin C , Lv Z , Li Z . et al. Green finance, renewable energy and carbon neutrality in OECD countries . Renew Energy 2023 ; 211 : 279 – 84 . https://doi.org/10.1016/j.renene.2023.04.105 .
Liu J , Tian J , Lyu W . et al. The impact of COVID-19 on reducing carbon emissions: from the angle of international student mobility . Appl Energy 2022 ; 317 :119136. https://doi.org/10.1016/j.apenergy.2022.119136 .
Si H , Li N , Duan X . et al. Understanding the public's willingness to participate in the carbon generalized system of preferences (CGSP): an innovative mechanism to drive low-carbon behavior in China . Sustain Prod Consump 2023 ; 38 : 1 – 12 . https://doi.org/10.1016/j.spc.2023.03.016 .
Shui B , Dowlatabadi H . Consumer lifestyle approach to US energy use and the related CO emissions . Energy Policy 2005 ; 33 : 197 – 208 . https://doi.org/10.1016/S0301-4215(03)00210-6 .
Paterson M , Stripple J . My space: governing individuals’carbon emissions . Soc Space 2010 ; 28 : 341 – 62 . https://doi.org/10.1068/d4109 .
Rahman F , Ahamed SI , Hoque E . et al. UCFC-ubiquitous personal carbon footprint calculation platform. Chicago, IL, USA . In: International Conference on Green Computing IEEE Computer Society 2010 , pp. 425 – 32 .
Riddell W , Bhatia KK , Parisi M . et al. Assessing carbon dioxide emissions from energy use at a university . Int J Sustain High Educ 2009 ; 10 : 266 – 78 . https://doi.org/10.1108/14676370910972576 .
Larsen HN , Pettersen J , Solli C . et al. Investigating the carbon footprint of a university-the case of NTNU . J Clean Prod 2013 ; 48 : 39 – 47 . https://doi.org/10.1016/j.jclepro.2011.10.007 .
Liu Y , Wang J , Fu B . et al. Influence of college students’ life style on carbon emission in Beijing . J Arid Land Resour Environ 2019 ; 32 : 31 – 6 .
Zeguang Z , Xiumei D . Study on low-carbon consumption strategy of college students . China Adult Educ 2013 ; 13 : 60 – 2 .
Xiaoxu M . Influencing factors of low-carbon consumption behavior of college students based on structural equation model-a case study in Yangzhou University . Consum Econ 2015 ; 31 : 87 – 91 .
Xin Z , Zhendong D . Analysis on the connotation and content of low-carbon life education for college students . Heilongjiang Res Higher Educ 2014 ; 5 : 26 – 8 .
Jiao P , Xiaoming A , Xin L . et al. Structural characteristics of college student’ carbon emission in Hebei Province and the path of carbon emission reduction . J Arid Land Res Environ 2023 ; 37 : 8 – 14 .
Li X , Tan H , Rackes A . Carbon footprint analysis of student behavior for a sustainable university campus in China . J Clean Prod 2015 ; 106 : 97 – 108 . https://doi.org/10.1016/j.jclepro.2014.11.084 .
Month: | Total Views: |
---|---|
June 2024 | 10 |
Email alerts
Citing articles via, affiliations.
- Online ISSN 1748-1325
- Print ISSN 1748-1317
- Copyright © 2024 Oxford University Press
- About Oxford Academic
- Publish journals with us
- University press partners
- What we publish
- New features
- Open access
- Institutional account management
- Rights and permissions
- Get help with access
- Accessibility
- Advertising
- Media enquiries
- Oxford University Press
- Oxford Languages
- University of Oxford
Oxford University Press is a department of the University of Oxford. It furthers the University's objective of excellence in research, scholarship, and education by publishing worldwide
- Copyright © 2024 Oxford University Press
- Cookie settings
- Cookie policy
- Privacy policy
- Legal notice
This Feature Is Available To Subscribers Only
Sign In or Create an Account
This PDF is available to Subscribers Only
For full access to this pdf, sign in to an existing account, or purchase an annual subscription.
- Research article
- Open access
- Published: 03 June 2024
From concerns to benefits: a comprehensive study of ChatGPT usage in education
- Hyeon Jo ORCID: orcid.org/0000-0001-7442-4736 1
International Journal of Educational Technology in Higher Education volume 21 , Article number: 35 ( 2024 ) Cite this article
606 Accesses
5 Altmetric
Metrics details
Artificial Intelligence (AI) chatbots are increasingly becoming integral components of the digital learning ecosystem. As AI technologies continue to evolve, it is crucial to understand the factors influencing their adoption and use among students in higher education. This study is undertaken against this backdrop to explore the behavioral determinants associated with the use of the AI Chatbot, ChatGPT, among university students. The investigation delves into the role of ChatGPT’s self-learning capabilities and their influence on students’ knowledge acquisition and application, subsequently affecting the individual impact. It further elucidates the correlation of chatbot personalization with novelty value and benefits, underscoring their importance in shaping students’ behavioral intentions. Notably, individual impact is revealed to have a positive association with perceived benefits and behavioral intention. The study also brings to light potential barriers to AI chatbot adoption, identifying privacy concerns, technophobia, and guilt feelings as significant detractors from behavioral intention. However, despite these impediments, innovativeness emerges as a positive influencer, enhancing behavioral intention and actual behavior. This comprehensive exploration of the multifaceted influences on student behavior in the context of AI chatbot utilization provides a robust foundation for future research. It also offers invaluable insights for AI chatbot developers and educators, aiding them in crafting more effective strategies for AI integration in educational settings.
Introduction
In the realm of advanced language models, OpenAI’s Generative Pre-trained Transformer (GPT) has emerged as a groundbreaking tool that has begun to make significant strides in numerous disciplines (Biswas, 2023a , b ; Firat, 2023 ; Kalla, 2023 ). Education, in particular, has become a fertile ground for this innovation (Kasneci et al., 2023 ). A standout instance is ChatGPT, the latest iteration of this transformative technology, which has been rapidly adopted by a large segment of university students across the globe (Rudolph et al., 2023 ). At its core, ChatGPT is armed with the ability to produce cohesive, contextually appropriate responses predicated on preceding dialogues, providing an interactive medium akin to human conversation (King & ChatGPT, 2023 ). This interactive capacity of ChatGPT has the potential to significantly restructure the educational landscape, altering the way students absorb, interact, and engage with academic content (Fauzi et al., 2023 ). Despite the burgeoning intrigue surrounding this technology and its applications, the comprehensive examination of determinants shaping students’ behavior in the adoption and usage of ChatGPT remains a largely uncharted territory. A thorough, systematic understanding of these determinants, both facilitative and inhibitive, is essential for the more effective deployment and acceptance of such a tool in educational settings, thereby contributing to its ultimate success and efficacy. The current study seeks to shed light on this crucial aspect, aiming to provide a nuanced comprehension of the array of factors that influence university students’ behavioral intentions and patterns towards utilizing ChatGPT for their educational pursuits.
ChatGPT is replete with remarkable features courtesy of its underlying technologies: machine learning (Rospigliosi, 2023 ) and natural language processing (Maddigan & Susnjak, 2023 ). Its capabilities span across a broad spectrum, from completing texts, answering questions, to even spawning original content. In the landscape of education, these functionalities morph into a powerful apparatus that could revolutionize traditional learning modalities. Similar to a human tutor, students can converse with ChatGPT—asking questions, eliciting explanations, or diving into profound discussions about their study materials. Beyond its conversational abilities, ChatGPT’s capacity to learn from past interactions enables it to refine its responses over time, catering more accurately to the user’s distinct needs and preferences (McGee, 2023 ). This attribute of continuous learning and adaptation paves the way for a more personalized and efficient learning experience, offering custom-made academic assistance (Aljanabi, 2023 ). This intricate combination of characteristics arguably makes ChatGPT a potent tool in the educational sphere, worthy of systematic examination to optimize its potential benefits and mitigate any challenges.
ChatGPT’s unique features manifest a range of enabling factors that can significantly influence its adoption among university students. One such factor is the self-learning attribute that empowers the AI to progressively enhance its performance (Rijsdijk et al., 2007 ). This feature aligns with the ongoing learning journey of students, potentially fostering a symbiotic learning environment. Another pivotal factor is the scope for knowledge acquisition and application. As ChatGPT assists in knowledge acquisition, students can simultaneously apply their newly garnered knowledge, potentially bolstering their academic performance. Personalization of the AI forms another significant enabling factor, ensuring that the interactions are fine-tuned to each student’s distinctive needs, thereby promoting a more individual-centered learning experience. Lastly, the novelty value that ChatGPT brings to the educational realm can stimulate student engagement, making learning an exciting and enjoyable process. Unraveling the influence of these factors on students’ behavior toward ChatGPT could yield valuable insights, paving the way for the tool’s effective implementation in education.
Alongside the positive influencers, some potential detractors could hinder the adoption of ChatGPT among university students. Among these, privacy concerns stand out as paramount (Lund & Wang, 2023 ; McCallum, 2023 ). With data breaches becoming increasingly common, students might be apprehensive about sharing their academic queries and discussions with an AI tool. Technophobia is another potential inhibitor, as not all students might be comfortable interacting with an advanced AI model. Moreover, some students might experience guilt feelings while using ChatGPT, equating its assistance to a form of ‘cheating’ (Anders, 2023 ). Evaluating these potential inhibitors is crucial to forming a comprehensive understanding of the factors that shape students’ behavior towards ChatGPT. This balanced approach could help identify ways to alleviate these concerns and foster wider adoption of this promising educational tool.
Despite the growing adoption of AI tools like ChatGPT, there is a notable gap in the literature pertaining to how these tools influence behavior in an educational context. Moreover, existing studies often fail to account for both enabling and inhibiting factors. Therefore, this paper endeavors to fill this gap by offering a more balanced and comprehensive exploration. The primary objective of this study is to empirically examine the determinants—both enablers and inhibitors—that influence university students’ behavioral intentions and actual behavior towards using ChatGPT. By doing so, this study seeks to make a valuable contribution to the academic discourse surrounding the use of AI in education, while also offering practical implications for its more effective implementation in educational settings.
The rest of this paper is structured as follows: the next section reviews the relevant literature and formulates the research hypotheses. The subsequent section outlines the research methodology, followed by a presentation and discussion of the results. Finally, the paper concludes with the implications for theory and practice, and suggestions for future research.
Theoretical background and hypothesis development
This study is grounded in several theoretical frameworks that inform the understanding of AI-based language models, like ChatGPT, and their impact on individual impact, benefits, behavioral intention, and behavior.
Figure 1 displays the research mode. The model asserts that the AI’s self-learning capability influences knowledge acquisition and application, which in turn impact individual users. Personalization of the AI and the novelty value it offers are also predicted to have substantial effects on the perceived benefits, influencing the behavioral intention to use AI, culminating in actual behavior. The model also takes into account potential negative influences such as perceived risk, technophobia, and feelings of guilt on the behavioral intention to use the AI. The last two constructs in the model, behavioral intention and innovativeness, are anticipated to influence the actual behavior of the AI.
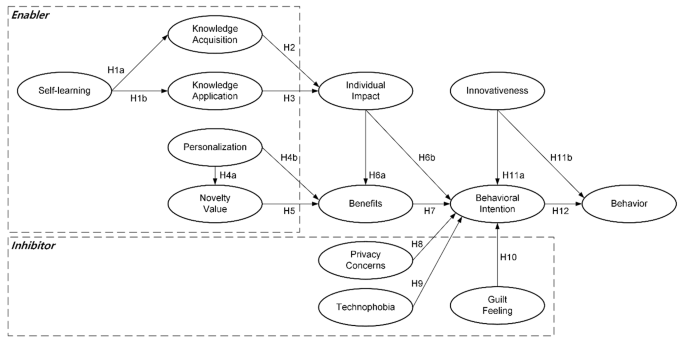
Research Model
Self-determination theory (SDT)
The SDT is indeed a critical theoretical foundation in this study. The theory, developed by Ryan and Deci ( 2000 ), posits that when tasks are intrinsically motivating and individuals perceive competence in these tasks, it leads to improved performance, persistence, and creativity. This theoretical framework supports the idea that the self-learning capability of AI, like ChatGPT, is vital in fostering intrinsic motivation among its users. Specifically, the self-learning aspect allows ChatGPT to tailor its interactions according to the individual user’s needs, thereby creating a more engaging and personally relevant learning environment. This can enhance users’ perception of their competence, resulting in more effective knowledge acquisition and application (H1).
Further, by promoting effective knowledge acquisition and application, the self-learning capability of ChatGPT can significantly impact individuals positively (H2, H3). That is, students can use the knowledge gained from interactions with ChatGPT to improve their academic performance, deepen their understanding of various subjects, and even stimulate their creativity. This aligns with the SDT’s emphasis on competence perception as a driver of enhanced performance and creativity.
Therefore, in line with the SDT, this study hypothesizes that the self-learning capability of ChatGPT, which promotes intrinsic motivation and perceived competence, can lead to improved knowledge acquisition and application, and consequently, a positive individual impact. This theoretical foundation helps to validate the first three hypotheses of the study and underscores the significance of intrinsic motivation and perceived competence in leveraging the educational potential of AI tools like ChatGPT.
- Self-learning
Self-learning is considered as an inherent capability of ChatGPT, the AI tool (Rijsdijk et al., 2007 ). This unique ability of ChatGPT allows it to conduct continuous learning, improve itself, and solve problems based on big data analysis. The concept of self-learning in AI involves the system’s ability to learn and improve from experience without being explicitly programmed (Bishop & Nasrabadi, 2006 ). Such an AI-based tool, like ChatGPT, can evolve its capabilities over time, making it a highly efficient tool for knowledge acquisition and application among students (Berland et al., 2014 ). Berland et al. ( 2014 ) pointed out that self-learning in AI can provide personalized learning experiences, thereby helping students to acquire and retain knowledge more effectively. This is due to the AI’s ability to adapt its responses based on past interactions and data, allowing for more targeted and relevant knowledge acquisition. For knowledge application, the self-learning capability of AI tools like ChatGPT can play a significant role. D’mello and Graesser ( 2013 ) argued that such AI systems could enhance learners’ ability to apply acquired knowledge, as they can simulate a variety of scenarios and provide immediate feedback. This real-time, contextual problem-solving can further strengthen knowledge application skills in students. Consequently, recognizing the potential of self-learning in AI, such as in ChatGPT, to improve both knowledge acquisition and application among university students, this study suggests the following hypotheses.
H1a. Self-learning has a positive effect on knowledge acquisition.
H1b. Self-learning has a positive effect on knowledge application.
Knowledge Acquisition
Knowledge acquisition is the process in which new information is obtained and subsequently expanded upon when additional insights are gained. A research by Nonaka ( 1994 ) emphasized the role of knowledge acquisition in enhancing organizational productivity. Another study by Alavi and Leidner ( 2001 ) suggested that knowledge acquisition can positively influence individual performance by improving the efficiency and effectiveness of task execution. Several scholars have shown the significance of knowledge acquisition on perceived usefulness in the context of learning (Al-Emran et al., 2018 ; Al-Emran & Teo, 2020 ). Given the capabilities of AI chatbots like ChatGPT in providing users with immediate, accurate, and personalized information, it’s likely that knowledge acquisition from using such a tool could lead to improved task performance or individual impact. Therefore, this study proposes the following hypothesis:
H2. Knowledge acquisition has a positive effect on individual impact.
Knowledge application
Knowledge application, as defined by Al-Emran et al. ( 2020 ), implies the facilitation of individuals to effortlessly utilize knowledge techniques via efficient storage and retrieval systems. With AI-driven chatbots like ChatGPT, this application process can be significantly enhanced. The positive influence of effective knowledge application on individual outcomes has also been corroborated by previous research (Al-Emran & Teo, 2020 ; Alavi & Leidner, 2001 ; Heisig, 2009 ). Therefore, this study suggests the following hypothesis:
H3. Knowledge application has a positive effect on individual impact.
- Personalization
The study’s conceptual framework is further informed by personalization (Maghsudi et al., 2021 ; Yu et al., 2017 ), underscoring the importance of personalized experiences in a learning environment. Literature on personalization advocates that designing experiences tailored to individual needs and preferences enhances the user’s engagement and satisfaction (Desaid, 2020 ; Jo, 2022 ). In the context of AI, personalization refers to the ability of the system, in this case, ChatGPT, to adapt its interactions based on the unique requirements and patterns of the individual user. This capacity to customize interactions could make the learning process more relevant and stimulating for the students, thereby potentially increasing the novelty value they derive from using ChatGPT (H4a).
Furthermore, the personalization theory also suggests that a personalized learning environment can lead to increased perceived benefits, as it allows students to learn at their own pace, focus on areas of interest or difficulty, and receive feedback that is specific to their learning progress (H4b). This aligns with the results of the study, which found a significant positive relationship between personalization and perceived benefits.
Personalization refers to the technology’s ability to tailor interactions based on the user’s preferences, behaviors, and individual characteristics, providing a unique and individualized user experience (Wirtz et al., 2018 ). This capacity has been found to significantly enhance users’ perception of value in their interactions with the technology (Chen et al., 2022 ). Previous research has indicated that the personalization capabilities of AI chatbots, such as ChatGPT, can enhance this novelty value, as the individualized user experience provides a unique and fresh engagement every time (Haleem et al., 2022 ; Koubaa et al., 2023 ). Research has highlighted that personalized interactions with AI chatbots can lead to increased perceived benefits, such as improved efficiency, productivity, and task performance (Makice, 2009 ). Personalization enables the technology to cater to the individual user’s needs effectively, thereby enhancing their perception of the benefits derived from using it (Wirtz et al., 2018 ). Thus, this study proposes the following hypotheses:
H4a. Personalization has a positive effect on novelty value.
H4b. Personalization has a positive effect on benefits.
Diffusion of Innovation
The diffusion of innovations theory offers a valuable lens through which to understand the adoption of new technologies (Rogers, 2010 ). The theory suggests that the diffusion and adoption of an innovation in a social system are influenced by five key factors: the innovation’s relative advantage, compatibility, complexity, trialability, and observability.
One essential aspect of the innovation, which resonates strongly with this study, is its relative advantage – the degree to which an innovation is perceived as being better than the idea it supersedes. This relative advantage often takes the form of novelty value, especially in the early stages of an innovation’s diffusion process. In this context, ChatGPT, with its self-learning capability, personalization, and interactive conversation style, can be seen as a novel approach to traditional learning methods. This novelty can make the learning process more engaging, exciting, and effective, thereby increasing the perceived benefits for students.
Rogers’ theory also highlights that these perceived benefits can significantly influence an individual’s intention to adopt the innovation. The more an individual perceives the benefits of the innovation to outweigh the costs, the more likely they are to adopt it. In the context of this study, the novelty value of using ChatGPT contributes to the perceived benefits (H5), which in turn influences the intention to use it (H7). This underscores the importance of managing the perceived novelty value of AI tools like ChatGPT in a learning environment, as it can be a crucial determinant of their adoption and use. Furthermore, Rogers’ theory stresses the importance of compatibility and simplicity of the innovation. These attributes align with the concepts of personalization and self-learning capabilities of ChatGPT, highlighting that an AI tool that aligns with users’ needs and is easy to use is more likely to be adopted.
Novelty value
Novelty value refers to the unique and new experience that users perceive when interacting with the technology (Moussawi et al., 2021 ). This sense of novelty has been associated with increased interest, engagement, and satisfaction, contributing to a more valuable and enriching user experience (Hund et al., 2021 ). In this context, the novelty value provided by AI artifacts can enhance the perceived value of using the technology (Jo, 2022 ). This relationship has been documented in prior research, showing that the novelty of technology use can lead to increased perceived benefits, possibly due to heightened user interest, engagement, and satisfaction (Huang & Benyoucef, 2013 ). Therefore, this study suggests the following hypothesis:
H5. Novelty value has a positive effect on benefits.
Technology Acceptance Model (TAM)
The TAM has been widely employed to explain user acceptance and usage of technology. TAM posits that two particular perceptions, perceived usefulness (the degree to which a person believes that using a particular system would enhance their job performance) and perceived ease of use (the degree to which a person believes that using a particular system would be free of effort), determine an individual’s attitude toward using the system, which in turn influences their behavioral intention to use it, and ultimately their actual system use.
In the context of this study, TAM offers valuable insight into how individual impact, perceived as the influence of using ChatGPT on a student’s academic performance and outcomes, can affect perceived benefits and behavioral intention (H6a, H6b). Students are more likely to use ChatGPT if they perceive it to have a positive impact on their learning outcomes. This perceived impact can enhance the perceived benefits of using ChatGPT, which can then influence their behavioral intention to use it. This aligns with Davis’s proposition that perceived usefulness positively influences behavioral intention to use a technology (Davis, 1989 ).
Moreover, the relationship between perceived benefits and behavioral intention (H7) is also consistent with TAM. According to Davis ( 1989 ), when users perceive a system as beneficial, they are more likely to form positive intentions to use it. Thus, the more students perceive the benefits of using ChatGPT, such as improved learning outcomes, personalized learning experiences, and increased engagement, the stronger their intention to use ChatGPT.
Individual impact
Individual impact refers to the perceived improvements in task performance and productivity brought about by the use of an IT such as ChatGPT (Aparicio et al., 2017 ). The perceived usefulness, which is closely related to individual impact, has a positive effect on behavioral intention to use a technology (Davis, 1989 ). In the literature, numerous studies have demonstrated that when users perceive a technology to be beneficial and impactful on their personal efficiency or productivity, they are more likely to have a positive behavioral intention towards using it (Gatzioufa & Saprikis, 2022 ; Kelly et al., 2022 ; Venkatesh et al., 2012 ). Similarly, these perceived improvements or benefits can also increase the perceived value of the technology, contributing to its overall appeal (Kim & Benbasat, 2006 ). Therefore, building upon this premise, this study proposes the following hypotheses.
H6a. Individual impact has a positive effect on benefits.
H6b. Individual impact has a positive effect on behavioral intention.
The concept of benefits is the perceived advantage or gain a user experiences from the use of the IT (Al-Fraihat et al., 2020 ). This study employed the individual impact and benefits separately. The rationale for considering individual impact and benefits as separate constructs in this research stems from the subtle differences in their underlying meanings and implications in the context of using AI chatbots like ChatGPT. While they might appear closely related, treating them separately can provide a more nuanced understanding of user experiences and perceptions. Individual impact, as defined in this research, pertains to the direct effects of using ChatGPT on the user’s performance and productivity. It encapsulates the tangible, immediate changes that occur in a user’s work efficiency and task completion speed as a result of using the AI chatbot. Therefore, individual impact serves as a measure of performance enhancement, indicating the ‘output’ side of the user’s interaction with ChatGPT. On the other hand, benefits, as gauged by the proposed survey items, are more encompassing. They extend beyond the immediate task-related effects to include the overall advantages perceived by the users. This could range from acquiring new knowledge to achieving personal or educational goals. Benefits, in essence, capture the ‘outcome’ aspect of using ChatGPT, reflecting a broader range of positive effects that contribute to user satisfaction and perceived value. By differentiating between individual impact and benefits, this research can provide a more detailed and comprehensive analysis of users’ experiences with ChatGPT.
The benefit as a determinant of behavioral intention is firmly established within the extant literature on technology acceptance and adoption. the theory of reasoned action (TRA) posits that the perception of benefits or value is directly linked to behavioral intentions (Fishbein & Ajzen, 1975 ). Similarly, the TAM proposes that perceived usefulness significantly influences behavioral intention to use a technology (Davis, 1989 ). Recent studies further confirm this relationship, which highlighted that the perceived benefit of a technology strongly predicts users’ intentions to use it (Cao et al., 2021 ; Chao, 2019 ; Yang & Wibowo, 2022 ). Given the consistent findings supporting this relationship, the following hypothesis is proposed:
H7. Benefits has a positive effect on behavioral intention.
Privacy theory and Technostress
The privacy paradox theory, formulated by Barnes ( 2006 ), provides insight into the intriguing contradiction that exists between individuals’ expressed concerns about privacy and their actual online behavior. Despite voicing concerns about their privacy, many individuals continue to disclose personal information or engage in activities that may compromise their privacy. However, in the context of AI technologies like ChatGPT, this study posits that privacy concerns may have a more pronounced impact. Particularly in an educational context, where sensitive academic information may be shared, privacy concerns can act as a deterrent, negatively influencing the behavioral intention to use the technology (H8).
On the other hand, the technostress model by Tarafdar et al. ( 2007 ) further illuminates our understanding of technology-induced stress and its impact on behavioral intention. This model proposes that individuals can experience different forms of technostress, including technophobia, which refers to the fear or anxiety experienced by some individuals when faced with new technologies. Technophobia can be particularly prevalent among individuals who lack familiarity or comfort with emerging technologies. In the context of this study, technophobia can potentially inhibit students from adopting and using AI tools like ChatGPT, thus negatively impacting their behavioral intention (H9).
Together, the privacy paradox theory and the technostress model provide a theoretical basis for understanding the potential inhibitors that could affect university students’ behavioral intention to use ChatGPT. By considering these theories, this study offers a more comprehensive perspective on the factors that can influence the adoption and use of AI technologies in an educational setting.
Privacy concerns
The concept of privacy concern refers to an individual’s anxiety or worry about the potential misuse or improper handling of personal data (Barth & de Jong, 2017 ). Privacy concern has been widely studied in the context of technology use, with a substantial body of research suggesting it is a major determinant of user behavior and intention (de Cosmo et al., 2021 ; Lutz & Tamò-Larrieux, 2021 ; Zhu et al., 2022 ). In the era of big data and pervasive digital technology, privacy concerns have increasingly become a pivotal factor shaping user behaviors (Smith et al., 2011 ). A host of empirical studies suggest that privacy concerns have a negative impact on behavioral intention. For example, Dinev and Hart ( 2006 ) observed that online privacy concerns negatively influence intention to use Internet services. Similarly, Li (2012) found that privacy concerns negatively affect users’ intention to adopt mobile apps. In the context of AI chatbots like ChatGPT, this concern could be even more pronounced, given the bot’s capacity for self-learning and data processing. Therefore, following the pattern observed in previous research, this study suggests the following hypothesis:
H8. Privacy concern has a negative effect on behavioral intention.
Technophobia
Technophobia is typically described as a personal fear or anxiety towards interacting with advanced technology, including AI systems such as ChatGPT (Ivanov & Webster, 2017 ). People suffering from technophobia often exhibit avoidance behavior when it comes to using new technology (Khasawneh, 2022 ). Several studies have empirically supported the negative relationship between technophobia and behavioral intention. For instance, Marakas et al. ( 1998 ) demonstrated that technophobia significantly reduces users’ behavioral intention to use a new IT system. Further, Selwyn (2018) found that technophobia can lead to avoidance behaviors and resistance towards adopting and using new technology, thus negatively influencing behavioral intention. Some users may fear losing control to these systems or fear making mistakes while using them, leading to a decline in their intention to use AI systems like ChatGPT. In consideration of these factors and in line with previous studies, this study posits the following hypothesis:
H9. Technophobia has a negative effect on behavioral intention.
Affect theory
The affect theory, proposed by Lazarus ( 1991 ), offers a comprehensive framework for understanding how emotions influence behavior, including technology use. According to this theory, emotional responses are elicited by the cognitive appraisal of a given situation and these emotional responses, in turn, have a significant impact on individuals’ behavior.
Applying this theory to the context of this study, it can be suggested that the use of AI technologies like ChatGPT can elicit a range of emotional responses among students. One particular emotion that is of interest in this study is guilt feeling. Guilt is typically experienced when individuals perceive that they have violated a moral standard. In the case of using AI technologies for learning, some students might perceive this as a form of ‘cheating’ or unfair advantage, thus leading to feelings of guilt.
This study hypothesizes that these guilt feelings can negatively influence students’ behavioral intention to use ChatGPT (H10). That is, students who experience guilt feelings related to using ChatGPT might be less inclined to use this tool for their learning. This underscores the importance of considering emotional factors, in addition to cognitive and behavioral factors, when exploring the determinants of technology use in an educational setting.
By integrating affect theory into the analysis, this study contributes to a more holistic understanding of the factors influencing the adoption and use of AI technologies in education. It also highlights the need for strategies that address potential guilt feelings among students to promote more positive attitudes and intentions towards using these technologies for learning.
Guilt feeling
Guilt feeling as a psychological state has been discussed extensively in consumer behavior research, often in the context of regret after certain consumption decisions (Khan et al., 2005 ; Zeelenberg & Pieters, 2007 ). Recently, guilt feeling has been associated with technology use, particularly when users perceive their technology use as excessive or inappropriate (Masood et al., 2022 ). For instance, guilt feeling could emerge among students when they feel they excessively rely on AI tools, such as ChatGPT, for completing assignments instead of their own effort and intellectual work. In line with the negative state relief model (Cialdini et al., 1973 ), individuals experiencing guilt feelings tend to take actions to alleviate these feelings. If university students feel guilty for using ChatGPT in their assignments, they might reduce their future use of this tool, thereby negatively impacting their behavioral intention to use ChatGPT. This finding is consistent with the results of a study conducted by Masood et al. ( 2022 ), which found that feelings of guilt resulting from excessive use of social networking sites predicted discontinuation. Thus, in the light of previous research suggesting a potential link between guilt feelings and behavioral intentions related to technology use, this study proposes the following hypothesis:
H10. Guilt feeling has a negative effect on behavioral intention.
- Innovativeness
Lastly, the diffusion of innovation theory (Rogers, 2010 ) lends further support to the hypotheses within the model (H12a, H12b), positing a positive influence of innovativeness on both behavioral intention and behavior. Innovativeness is defined as an individual’s predisposition to adopt new ideas, processes, or products earlier than other members of a social system (Rogers, 1983 ). It plays a critical role in technology adoption research, with prior studies highlighting the positive effects of innovativeness on both behavioral intention and actual behavior. For instance, Agarwal and Prasad ( 1998 ) found that personal innovativeness in the domain of information technology significantly influenced individuals’ intention to use a new technology. Similarly, Varma Citrin et al. ( 2000 ) discovered that consumer innovativeness positively affected both intention to use and actual use of self-service technologies. In the context of AI chatbots like ChatGPT, it is reasonable to propose that innovative university students, open to trying new technologies and applications, would exhibit stronger intentions to use ChatGPT and indeed, more likely to use it in practice. Hence, this study advances the following hypotheses:
H11a. Innovativeness has a positive effect on behavioral intention.
H11b. Innovativeness has a positive effect on behavior.
Theory of planned behavior (TPB)
The TPB, as proposed by Ajzen ( 1991 ), is a widely recognized psychological framework for understanding human behavior in various contexts, including technology adoption (Huang, 2023 ; Meng & Choi, 2019 ; Song & Jo, 2023 ). At the heart of TPB is the principle that individuals’ behavioral intentions are the most immediate and critical determinant of their actual behavior. This principle is predicated on the assumption that individuals are rational actors who make systematic use of the information available to them when deciding their course of action.
In the context of this study, the TPB supports the hypothesis that behavioral intention, influenced by various enabling and inhibiting factors, can significantly predict the actual use of ChatGPT among university students (H11). That is, students who have a stronger intention to use ChatGPT, either due to perceived benefits or other positive factors, are more likely to engage in actual usage behavior. Conversely, those with weaker intentions, perhaps due to privacy concerns or feelings of guilt, are less likely to use ChatGPT.
This hypothesis, grounded in TPB, is instrumental in linking the various enabling and inhibiting factors to the actual use of ChatGPT. It helps to consolidate the theoretical framework of the study, enabling a more holistic understanding of the behavioral dynamics at play. Moreover, it underscores the importance of cultivating positive behavioral intentions among students to encourage the actual use of AI technologies in education.
By integrating the TPB into the analysis, this study not only aligns with established psychological theories of behavior but also expands the application of these theories in the context of AI technology adoption in education.
- Behavioral intention
The relationship between behavioral intention and actual behavior is a fundamental principle in various behavior prediction models, most notably the TPB by Ajzen ( 1991 ). The TPB posits that behavioral intention is the most proximal determinant of actual behavior. The assumption is that individuals act in accordance with their intentions, given that they have sufficient control over the behavior. Empirical studies have provided substantial evidence to support this link (Gatzioufa & Saprikis, 2022 ; Sun & Jeyaraj, 2013 ). In the context of ChatGPT usage among university students, it is therefore expected that higher intentions to use ChatGPT would translate into actual usage behavior. Therefore, drawing on existing literature and theoretical models, this study suggests the following hypothesis:
H12. Behavioral intention has a positive effect on behavior.
Methodology
The instrument for this study was carefully crafted, leveraging a structured questionnaire to assess the influential factors in university students’ behavioral intentions and actual use of ChatGPT. Items in the questionnaire were adopted and adapted from the existing literature, ensuring their validity and relevance in examining the constructs of interest. Each item in the questionnaire was evaluated using a seven-point Likert scale, with 1 representing ‘strongly disagree’ and 7 denoting ‘strongly agree’. This provided a nuanced measurement of the participants’ perceptions and intentions. The survey questions were meticulously designed based on established theories and previous studies in the field, ensuring relevance to our research objectives.
Table A1 outlines a comprehensive list of constructs and their associated items used in the study, detailing how each construct is measured through specific statements. The constructs include self-learning, knowledge acquisition, knowledge application, personalization, novelty value, individual impact, benefits, privacy concern, technophobia, guilt feeling, innovativeness, behavioral intention, and behavior. Each item is attributed to sources from recent research, evidencing the rigorous grounding of these measures in existing literature. For instance, self-learning items are drawn from Chen et al. ( 2022 ), indicating the AI’s capability to enhance its functions through learning. Knowledge acquisition and application items reflect the user’s ability to gain and use knowledge via ChatGPT, sourced from Al-Emran et al. ( 2018 ) and Al-Sharafi et al. ( 2022 ), respectively. Personalization items, derived from Chen et al. ( 2022 ), measure ChatGPT’s ability to tailor responses to individual users. The novelty value construct, sourced from Hasan et al. ( 2021 ), captures the unique experience of using ChatGPT. Individual impact and benefits items, referenced from various authors, assess the practical advantages of using ChatGPT in educational settings. Privacy concern and technophobia items address potential user apprehensions, while guilt feeling items, a novel addition from Masood et al. ( 2022 ), explore emotional reactions to using AI in education. Innovativeness items, cited from Agarwal and Prasad ( 1998 ), examine user openness to new technologies. Behavioral intention and behavior constructs, finally, measure users’ future use intentions and current use patterns, demonstrating the study’s comprehensive approach to understanding AI chatbot utilization among university students.
The survey items underwent a rigorous validation process involving academic experts and a pilot study with a subset of the target population to refine their clarity and applicability. Prior to the actual distribution of the survey, a pretest was carried out with a select group of students to ensure the clarity and comprehensibility of the items. Feedback from this pretest was taken into account, and minor revisions were made to enhance the clarity of certain questions. The refined questionnaire was then disseminated to a broader group of university students, with the collected responses forming the primary data for this study. These responses were meticulously analyzed to understand the role of various factors on students’ behavioral intention and their actual usage of ChatGPT.
Questionnaire Design and Data Collection
The questionnaire was structured in two sections. The first section collected demographic information about the respondents, including their age, gender, and field of study, to capture the heterogeneity of the sample. The second section was dedicated to evaluating the constructs related to the study, each measured using multiple items.
Once the final version of the questionnaire was prepared, it was disseminated among university students. The data for this study was collected via a Google survey form. To reach a broad range of university students, the survey was disseminated through multiple channels. First, it was sent out to a network of professors at several universities. These professors then distributed the survey form to their students. During the survey phase, we explained the purpose of our study to professors across the country, asking for their assistance in distributing the survey if their students were actively using ChatGPT and were willing to participate. Additionally, some professors further shared the survey with their professional networks, expanding our reach. This approach was selected to ensure a diverse and representative sample of students from various disciplines and academic levels. Approximately 400 students were initially approached through this network. This method was chosen over others to leverage the existing academic networks, ensuring a broad yet relevant participant base for our study, reflecting diverse experiences with ChatGPT across different universities. In addition to professors, the survey was also shared within various university communities, such as student groups and clubs. This allowed the study to reach students who might not have been reached through the professors’ networks. Finally, the survey was distributed within ChatGPT community groups. This was especially important as it enabled the study to capture the perspectives of students who were already familiar with or using ChatGPT, thereby enriching the study’s insights into the factors affecting students’ behavioral intentions towards using AI tools like ChatGPT. Responses were collected over a period of several weeks, with a total of 273 responses received. The sample size of this study, 273, is considered adequate and appropriate given the number of latent variables and observation variables in the model. The literature suggests that a higher sample size is always better in SEM-based studies due to its effect on statistical power (Hair et al., 2021 ). In this case, the ratio of sample size to the number of latent variables (approximately 21:1) is within acceptable limits. Moreover, the sample size also exceeds the minimum thresholds suggested by other guidelines. For instance, Hair et al. ( 2021 ) suggested a minimum sample size of 10 times the number of items in the scale with the most items. Considering that the scale with the most items in this study contains 3 items, the sample size of 273 is well above the minimum threshold. The data, then, were analyzed.
Table 1 presents sample details. The gender split among respondents was nearly even, with 123 males (45.1%) and 150 females (54.9%), ensuring diverse gender perspectives. Age-wise, respondents ranged from under 20 to over 23 years. The largest groups were those under 20 (42.9%) and over 23 (37.4%), with 21- and 22-year-olds constituting 9.2% and 10.6%, respectively. Most respondents (89.4%) were undergraduates, with postgraduates making up 10.6%, reflecting the primary academic users of AI tools like ChatGPT. In terms of study majors, Business (44.3%) was predominant, followed by Humanities and Social Sciences (22.7%), and Science (22.0%). Arts, Sports, and Military majors each represented 5.5%. The analysis of descriptive statistics was conducted using the pivot table feature in Excel.
The theoretical framework for this study was evaluated utilizing the Partial Least Squares (PLS) approach via SmartPLS 4 software. The PLS method is a prevalent choice within the Information Systems/Information Technology domain, as substantiated by (Hair et al., 2014 ). One of the principal benefits of PLS lies in its fewer constraints concerning sample size distribution and residuals relative to covariance-based structural equation techniques such as LISREL and AMOS, as highlighted by (Hair et al., 2021 ). Our analysis employed a three-step strategy, encompassing common method bias (CMB), the measurement model, and the structural model.
Common Method Bias (CMB)
CMB refers to the spurious effect that can occur when both predictor and criterion variables are collected from the same source at the same time. It can potentially inflate or deflate the observed relationships between the constructs, leading to biased results. Thus, addressing the issue of CMB is critical to ensuring the validity of the study’s findings. In this study, we took several procedural and statistical steps to address the potential issue of CMB. Procedurally, we ensured the anonymity of the respondents and the confidentiality of their responses. We also used clear and simple language in the survey to minimize any ambiguity in the questions.
Statistically, we employed the Harman’s single factor test, which is a commonly used method to detect CMB (Podsakoff et al., 2003 ). The basic premise of this test is that if a single factor emerges from a factor analysis of all the items in the questionnaire or one general factor accounts for the majority of the covariance among the measures, then common method variance is a problem. In our case, the first of included factors explained 33.88% of the total variance, suggesting that CMB is not a substantial concern in this study. Additionally, we used the marker variable technique, which involves selecting a variable that is theoretically unrelated to other variables in the study and checking for its correlation with them. If significant correlations exist, they might be due to common method variance. Our marker variable showed negligible correlations with the study variables, further indicating that CMB is unlikely to have significantly influenced our results. In conclusion, both procedural and statistical checks suggest that CMB is not a major issue in this study, and the relationships identified between the constructs can be interpreted with confidence.
Measurement model
The measurement model in this study was assessed by examining the reliability, convergent validity, and discriminant validity of the constructs. Reliability was assessed using composite reliability (CR) and Cronbach’s alpha (CA). The values for both CR and CA should exceed the threshold of 0.7, indicating acceptable reliability. In our study, all constructs exceeded this threshold, demonstrating good reliability. Convergent Validity is the extent to which a measure correlates positively with alternate measures of the same construct. It was assessed using the average variance extracted (AVE) and the factor loadings of the indicators. All AVE values should be greater than 0.5, and all factor loadings should be significant and exceed 0.7. In our study, all constructs demonstrated satisfactory convergent validity as they met these criteria. Table 2 describes the test results of reliability and validity.
Discriminant validity refers to the extent to which a construct is truly distinct from other constructs. This was examined using the Fornell-Larcker criterion and the heterotrait-monotrait ratio (HTMT). According to the Fornell-Larcker criterion, the square root of the AVE of each construct should be greater than its highest correlation with any other construct. Table 3 shows the results of Fornell-Larcker criterion.
Table 4 displays the HTMT matrix. Notably, knowledge acquisition and knowledge application showed a 0.954 HTMT value, above the 0.85 norm. Despite exceeding the threshold, these values suggest a moderate overlap. Although related, the constructs are distinct: knowledge acquisition involves generating new knowledge, while knowledge application focuses on immediate knowledge integration. Given their different roles in AI-assisted learning, and supported by literature allowing higher HTMT values within the same network, both constructs are retained in our study. Future research should further investigate their differentiation.
Structural model
A Structural Equation Modeling (SEM) analysis was carried out to scrutinize the hypothesized interconnections among the constructs using Partial Least Squares (PLS). For hypothesis testing and path coefficient determination, this study employed a bootstrapping procedure, setting the subsample size at 5000. Overall, the structural model describes approximately 38.0% of the variability in behavior. Figure 2 ; Table 5 details of the significance test results of SEM.
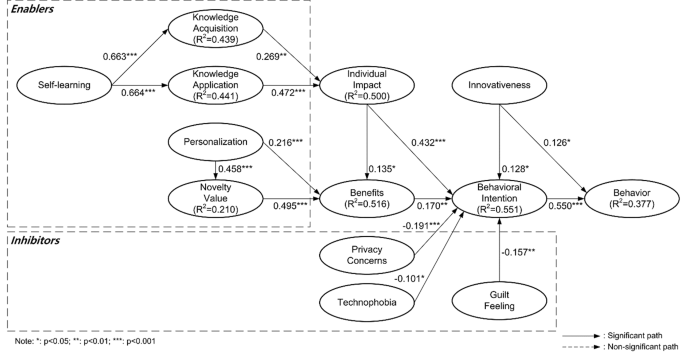
The Structural Model with Path Coefficients
The results of this study confirmed a positive correlation between self-learning of ChatGPT and knowledge acquisition and application, consistent with prior research on AI-driven learning tools (H1a, H1b) (Jarrahi et al., 2023 ). The results mean that as students engage with ChatGPT, they acquire new knowledge, which is then processed and incorporated into their existing knowledge base. Also, interacting with ChatGPT not only helps students gain new knowledge but also aids them in applying this knowledge in various scenarios, consequently resulting in a higher individual impact. The ability of AI to leverage vast data and improve problem-solving provides a fertile ground for enhanced learning. Yet, the novelty of this finding in the context of AI like ChatGPT calls for more studies to solidify this understanding.
Additionally, the results revealed that knowledge acquisition and application significantly influenced individual impact (H2, H3), mirroring the findings of Arpaci et al., (2020), Al-Emran et al. ( 2018 ), Al-Emran and Teo ( 2020 ), and Alavi and Leidner ( 2001 ). The findings suggest that as learners more actively gain knowledge from ChatGPT, they experience improvements in their productivity, task completion speed, and overall performance. Further, as learners effectively utilize the knowledge acquired from ChatGPT, they can better achieve their goals and enhance their work capabilities. This reinforces the importance of AI tools in achieving task completion and boosting productivity. Nevertheless, the variance in individual impact explained by these two factors indicates that other elements may also be at play.
Personalization was found to significantly correlate with novelty value and benefits (H4a, H4b), supporting Kapoor et al.‘s assertion of personalized experiences driving perceived value (Kapoor & Vij, 2018 ). As well the results are keeping with the observations in previous works (Haleem et al., 2022 ; Koubaa et al., 2023 ). The analysis suggests that the tailored experience delivered by ChatGPT is perceived as novel by the users. As users find these tailored experiences new and intriguing, the novelty value of the AI tool is enhanced. Similarly, as the AI tool continuously learns and adapts to individual users’ needs, it helps learners achieve their learning objectives more effectively and efficiently. This aligns with the perception of increased benefits, as users recognize the tool’s contribution to their learning outcomes. However, the degree of this impact varies, suggesting that personalization’s impact may be contingent on other factors that need exploration.
The positive effect of novelty value on perceived benefits (H5) found in this study aligns with diffusion theory (Rogers, 2010 ) and past study on novelty of AI (Jo, 2022 ). The unique and different learning experience that ChatGPT offers might stimulate users’ curiosity and interest in learning. It could make the learning process more enjoyable, keeping users engaged and motivated, which, in turn, enhances their learning outcomes and the perceived benefits from using the chatbot. Yet, the relative strength of this relationship indicates that novelty may not be the only driver of perceived benefits.
Regarding the relationship between individual impact and benefits, and behavioral intention (H6a, H6b), our findings are in line with the TAM (Davis, 1989 ), further solidifying its relevance in the AI context. These findings imply that when users perceive a higher individual impact from using ChatGPT (i.e., improved efficiency, productivity, or task accomplishment), they also perceive more benefits and express stronger intentions to continue using it. Moreover, the immediate positive effects of using ChatGPT, such as accomplishing tasks more quickly or increasing productivity, directly contribute to users’ willingness to continue using it. Yet, the relative effect sizes suggest that while individual impact affects both outcomes, its influence is stronger on behavioral intention, a facet worthy of further examination.
In line with prior studies (de Cosmo et al., 2021 ; Lutz & Tamò-Larrieux, 2021 ; Zhu et al., 2022 ), privacy concern was negatively associated with behavioral intention (H8). The results coincide with the privacy calculus theory (Dienlin & Metzger, 2016 ; Dinev & Hart, 2006 ), which suggests that when privacy concerns outweigh the perceived benefits, it can deter future usage intention. In congruent with previous findings (Marakas et al., 1998 ; Selwyn, 2013 ), this study found technophobia negatively impacting behavioral intention (H9), adding a novel dimension to our understanding of AI acceptance. This result highlights the need for easy-to-use, user-friendly interfaces and perhaps educational programs to mitigate fear and promote user confidence when engaging with AI chatbots. Similarly, the guilt feeling affecting behavioral intention (H10) provides a new angle for exploration in AI usage context.
Lastly, the results confirmed the strong effect of behavioral intention on actual behavior (H11), as suggested by (Ajzen, 1991 ). Also, innovativeness positively affected behavioral intention and behavior (H12a, H12b), adding to the growing literature on innovation adoption (Rogers, 2010 ). In the context of using AI chatbots, such as ChatGPT, students who are more innovative (who have higher levels of innovativeness) demonstrated a stronger intention to use and an actual higher usage of the chatbot. This could be interpreted to mean that these students are more inclined to explore and make use of new technologies in their learning processes, which in turn influences their behavior in a positive manner. Furthermore, the positive correlation between innovativeness and behavioral intention may also be linked to the tendency of innovative individuals to perceive less risk in trying out new technologies. This lack of perceived risk, coupled with their natural proclivity towards novelty, may increase their intention to utilize AI chatbots. However, the precise mechanisms through which innovativeness operates in the AI context warrant further study.
Theoretical contributions
The current study, in its quest to uncover the relationships between AI chatbot variables and their effects on university students, has made several valuable theoretical contributions to the existing body of literature on AI and education. Prior to this research, much of the literature focused on the use of AI chatbots in commercial and customer service settings, leaving the educational context relatively unexplored (Ashfaq et al., 2020 ; Chung et al., 2020 ; Pillai & Sivathanu, 2020 ). This study has filled this gap by focusing specifically on university students and their interaction with AI chatbots, thereby providing new insights and extending the knowledge boundary of the AI field to encompass the educational sector.
Additionally, this study’s emphasis on the self-learning capabilities of ChatGPT as a significant determinant of knowledge acquisition and application among students is a significant contribution to the existing body of literature. Previous research primarily centered on the chatbot’s features and functionalities (Haleem et al., 2022 ; Hocutt et al., 2022 ), leaving the learning capabilities of these AI systems underexplored. By focusing on the self-learning feature of ChatGPT, this study has expanded the discourse on AI capabilities and their impact on knowledge dissemination in the educational context.
This research significantly contributes to the understanding of innovativeness’s impact on behavioral intention and behavior in AI chatbot usage. While prior studies focused mainly on business contexts (BARIŞ, 2020 ; Heo & Lee, 2018 ; Selamat & Windasari, 2021 ), applying these insights to AI chatbots is relatively new, marking this study as a pioneer. Innovativeness as a determinant in this realm is novel for several reasons. First, it shifts focus from technology attributes to user characteristics, highlighting individual differences in technology adoption often overshadowed by technocentric views. Second, our study provides empirical evidence that innovativeness positively influences both behavioral intention and behavior, challenging previous assertions that limited its influence to early adoption stages (Agarwal & Prasad, 1998 ). By applying innovativeness in an AI chatbot context, with its unique interactional dynamics, the research broadens the theoretical construct of innovativeness, emphasizing the relevance of individual traits in emerging technology usage. Furthermore, this study’s integration of innovativeness into the research model encourages future research to consider other personal traits affecting technology usage, promoting a more comprehensive, user-centric approach to technology adoption. Hence, exploring innovativeness in AI chatbot usage offers significant theoretical insights and opens avenues for future research in technology usage behaviors.
This research makes a notable theoretical contribution by exploring the negative effects of privacy concern, technophobia, and guilt feeling on behavioral intention towards AI chatbot use, areas often overlooked in favor of positive influences (Ashfaq et al. 2020 ; Huang, 2021 ; Nguyen et al., 2021 ; Rafiq et al. 2022 ). Privacy concern, consistent with prior studies (Dinev & Hart, 2006 ), is critically examined in AI chatbots, where data privacy is paramount due to the potential for extensive personal data sharing. This study extends understanding by focusing on AI chatbots, a context where privacy concerns have heightened relevance. Technophobia’s impact, previously explored in technology adoption (Khasawneh, 2022 ; Kotze et al., 2016 ; Koul & Eydgahi, 2020 ), is uniquely applied to AI chatbots, shedding light on how fear and anxiety towards advanced technology can affect usage intentions. This perspective enriches existing knowledge by situating technophobia within the realm of cutting-edge AI technologies. Guilt feeling, a relatively unexplored concept in technology use, especially in AI chatbots, is also investigated. This study reveals how guilt, potentially stemming from AI reliance for learning or work, can deter usage intention, thus addressing a significant gap in technology adoption research.
This research notably contributes to theory by examining the effects of innovativeness on behavioral intention and behavior in AI chatbot usage, a relatively unexplored area, especially outside of business contexts (BARIŞ, 2020 ; Heo & Lee, 2018 ; Selamat & Windasari, 2021 ). This study stands out as one of the first to apply these concepts to AI chatbots, marking a novel approach in several ways. Firstly, it shifts the focus from technology attributes to user characteristics, emphasizing individual differences in technology adoption—a perspective often overshadowed by a technocentric focus. This approach diverges from traditional technology acceptance models, spotlighting the user’s innovativeness. Secondly, by demonstrating empirically that innovativeness positively affects both behavioral intention and behavior, this research underscores the dynamic role of this personal trait in driving technology usage. Furthermore, the study broadens the theoretical construct of innovativeness by applying it to the unique interactional dynamics of AI chatbots, thereby highlighting the relevance of individual traits in using emerging technologies. Lastly, integrating innovativeness into the research model sets a precedent for future studies to include other personal traits or user characteristics that may influence technology usage behavior. This approach promises a more holistic, user-centric understanding of technology adoption and use. Overall, exploring the impact of innovativeness in AI chatbot usage provides valuable theoretical insights and opens avenues for future research, enhancing our understanding of technology adoption in this rapidly evolving field.
The research significantly enhances theoretical understanding by substantiating the relationships between constructs like knowledge acquisition and application, individual impact, and benefits in the AI chatbot context. Previously, educational and organizational literature recognized the importance of knowledge acquisition and application for performance (Al-Emran et al., 2018 ; Al-Emran & Teo, 2020 ; Bhatt, 2001 ; Grant, 1996 ; Heisig, 2009 ). This study extends these principles to AI chatbot usage, underscoring their critical role in effective technology utilization. The study’s innovation lies in demonstrating how these cognitive processes interact with the individual impact construct. This interaction offers a comprehensive view of a user’s learning journey within the AI chatbot environment. Furthermore, the research goes beyond by illustrating that user-perceived benefits are influenced by both AI chatbot personalization and individual impact, the latter being a synergistic result of knowledge acquisition and application. This interplay provides a nuanced view of the factors contributing to perceived benefits, deepening our theoretical grasp of what renders AI chatbot usage beneficial. By presenting a complex, interconnected model of AI chatbot usage, this research contributes to a more thorough understanding of the dynamics involved. It encourages a multi-dimensional approach in examining AI chatbots’ adoption and usage factors. The findings also prompt further scholarly inquiry into how these relationships vary with context, AI chatbot type, or user characteristics. Thus, this study lays a foundation for future research, aiming to enrich the comprehension of AI chatbot usage dynamics.
Lastly, this study’s comprehensive research model, encompassing multiple constructs and their interrelationships, serves as a robust theoretical framework for future research in AI chatbot usage in educational settings. The findings of this study, highlighting the significance of various constructs and their relationships, provide a roadmap for scholars, guiding future research to better understand the dynamics of AI chatbot usage and its effects on students’ learning experiences. Furthermore, our research model takes a holistic approach by combining both positive and negative predictors of behavioral intention. This approach can offer a more comprehensive understanding of the intricate factors influencing AI chatbot use among university students.
Managerial implications
The findings of this study have numerous practical implications, particularly for educators, students, and developers of AI chatbot technologies.
Firstly, the results underscore the potential benefits of employing AI chatbots, like ChatGPT, as supplementary learning tools in educational settings. Given the significant positive effect of ChatGPT’s self-learning capabilities on knowledge acquisition and application among students, educators could consider integrating such AI chatbots into their teaching methods (Essel et al., 2022 ; Mohd Rahim et al., 2022 ; Vázquez-Cano et al., 2021 ). The integration of AI chatbots could take various forms, such as using chatbots to provide additional learning resources, facilitate interactive learning exercises, or offer personalized tutoring. Given the increasingly widespread use of online and blended learning methods in education (Crawford et al., 2020 ), the potential of AI chatbots to enhance students’ learning experiences should not be overlooked.
Secondly, this study’s findings may serve as a guide for students in maximizing their learning outcomes while using AI chatbots. Understanding that the self-learning capabilities of chatbots significantly enhance knowledge acquisition and application, students could be more inclined to utilize these tools for learning (Al-Sharafi et al., 2022 ). Furthermore, recognizing that personalization contributes positively to novelty value and benefits might motivate students to engage more deeply with personalized learning experiences offered by AI chatbots.
Thirdly, the present study’s results offer crucial insights into key features that users find valuable in an AI chatbot within an educational context. The demonstrated significance of self-learning capabilities, personalization, and novelty value indicates distinct areas that developers can focus on to amplify user experience and educational outcomes. Understanding the paramount importance of self-learning capabilities can shape the development strategies of AI chatbots. For instance, developers can focus on improving the ability of AI chatbots like ChatGPT to learn from user interactions, thereby enhancing the quality of responses over time. It would require advanced machine learning algorithms to grasp the context of user inquiries accurately, ensuring a consistent learning experience for the users. As noted by (Komiak & Benbasat, 2006 ), the AI’s ability to learn and adapt based on user input and data over time can lead to improved user satisfaction and ultimately, enhanced learning outcomes. Personalization is another pivotal feature that developers need to consider. This could involve customizing chatbot responses according to the user’s level of understanding, learning pace, and areas of interest. Personalization also extends to recognizing users’ specific learning goals and offering resources or guidance to help them achieve these goals. This feature can increase the value that users derive from their interactions with the chatbot (Jo, 2022 ). Lastly, the novelty value of AI chatbots in educational contexts cannot be underestimated. Developers can capitalize on this by introducing innovative features and interaction modes that keep users engaged and make learning exciting. Such features could include gamified learning activities, integration with other learning resources, and more interactive and responsive dialogue systems. It is well established in literature that perceived novelty can positively impact users’ attitudes and behaviors towards a new technology (Hasan et al., 2021 ; Jo, 2022 ).
Fourthly, the study’s findings related to the negative impact of privacy concerns, technophobia, and guilt feelings on behavioral intentions provide crucial insights for AI chatbot developers and educational institutions. Privacy concerns form a significant barrier to the acceptance of AI technologies in various fields, including education (Belen Saglam et al., 2021 ; Ischen et al., 2020 ; Manikonda et al., 2018 ). The concerns typically arise from the vast amounts of personal data AI systems gather, analyze, and store (Kokolakis, 2017 ). In response, developers can strengthen the privacy features of AI chatbots, clearly communicate their data handling practices to users, and ensure compliance with stringent data protection regulations. For instance, incorporating robust encryption methods, anonymization techniques, and allowing users to control the data they share can allay privacy concerns. Educational institutions can also provide guidance on safe and responsible use of AI technologies to further assuage these concerns. Technophobia, or the fear of technology, can also hinder the acceptance and use of AI chatbots. To address this, developers can design user-friendly interfaces, provide extensive user support, and gradually introduce advanced features to avoid overwhelming users. Moreover, educational institutions can play a vital role in mitigating technophobia by offering appropriate training and support to help students become comfortable with using these technologies. It has been suggested that familiarity and understanding significantly reduce technophobia (Khasawneh, 2018a ; Xi et al., 2022 ). Guilt feeling, as revealed in this study, is another factor that negatively influences behavioral intention. This can occur when users feel guilty about relying heavily on AI chatbots for learning or assignments. To address this, educational institutions should foster an environment that encourages balanced use of AI tools. This could involve providing guidelines on ethical AI use, setting boundaries on AI tool usage in assignments, and integrating AI tools as supplemental rather than primary learning resources.
Finally, the significant impact of perceived benefits and individual impact on behavioral intentions underscores the importance of demonstrating the tangible benefits of AI chatbot use in education to users. Making these benefits clear to users could encourage greater adoption and more effective use of AI chatbots in educational settings.
Limitation and Future Research
While this study makes several important contributions to our understanding of AI chatbots’ use in an educational context, it also opens up avenues for further investigation. The study’s primary limitation is its focus on university students, which may limit the generalizability of the findings. Future studies could explore different demographics, such as younger students, adult learners, or professionals engaging in continuous education, to provide a broader understanding of AI chatbot utilization in diverse learning contexts. The scope of the research could also be extended to include various types of AI chatbot technologies. As AI continues to advance, chatbots are becoming more diverse in their capabilities and functionalities. Hence, research considering different kinds of AI chatbots, their specific features, and their effects on learning outcomes could provide further insight. Moreover, this study primarily focuses on individual impacts and user perceptions. Future research could also look into institutional perspectives and the macro-level impacts of AI chatbot adoption in education. For instance, studies could investigate how the implementation of AI chatbots affects teaching strategies, curriculum development, and institutional resource allocation. Lastly, it would be insightful to conduct longitudinal studies to understand the long-term effects of AI chatbot usage on student performance and attitudes towards AI technologies. This approach could reveal trends and impacts that may not be immediately apparent in cross-sectional studies.
Data availability
The data used in this study are available from the corresponding author upon reasonable request.
Agarwal, R., & Prasad, J. (1998). A conceptual and operational definition of personal innovativeness in the domain of Information Technology. Information Systems Research , 9 (2), 204–215.
Article Google Scholar
Ajzen, I. (1991). The theory of Planned Behavior. Organizational Behavior and Human Decision Processes , 50 (2), 179–211. https://doi.org/10.1016/0749-5978(91)90020-T .
Al-Emran, M., & Teo, T. (2020). Do Knowledge Acquisition and Knowledge sharing really affect E-Learning adoption? An empirical study. Education and Information Technologies , 25 (3), 1983–1998.
Al-Emran, M., Mezhuyev, V., & Kamaludin, A. (2018). Students’ perceptions towards the integration of knowledge management processes in M-Learning systems: A preliminary study. International Journal of Engineering Education , 34 (2), 371–380.
Google Scholar
Al-Emran, M., Arpaci, I., & Salloum, S. A. (2020). An empirical examination of continuous intention to Use M-Learning: An Integrated Model. Education and Information Technologies , 25 (4), 2899–2918. https://doi.org/10.1007/s10639-019-10094-2 .
Al-Fraihat, D., Joy, M., & Sinclair, J. (2020). Evaluating E-Learning systems Success: An empirical study. Computers in Human Behavior , 102 , 67–86.
Al-Sharafi, M. A., Al-Emran, M., Iranmanesh, M., Al-Qaysi, N., Iahad, N. A., & Arpaci, I. (2022). Understanding the impact of Knowledge Management factors on the sustainable use of Ai-Based chatbots for Educational purposes using a Hybrid Sem-Ann Approach. Interactive Learning Environments , 1–20.
Alavi, M., & Leidner, D. E. (2001). Knowledge Management and Knowledge Management systems: Conceptual foundations and Research Issues. MIS Quarterly , 107–136.
Aljanabi, M. (2023). Chatgpt: Future directions and open possibilities. Mesopotamian Journal of Cybersecurity , 2023 , 16–17.
Anders, B. A. (2023). Is using Chatgpt Cheating, Plagiarism, both, neither, or Forward thinking? Patterns , 4 (3).
Aparicio, M., Bacao, F., & Oliveira, T. (2017). Grit in the path to E-Learning success. Computers in Human Behavior , 66 , 388–399.
Ashfaq, M., Yun, J., Yu, S., & Loureiro, S. M. C. (2020). I, Chatbot: Modeling the determinants of users’ satisfaction and continuance intention of Ai-Powered Service agents. Telematics and Informatics , 54 , 101473.
BARIŞ, A. (2020). A New Business Marketing Tool: Chatbot. GSI Journals Serie B: Advancements in Business and Economics , 3 (1), 31–46.
Barnes, S. B. (2006). A Privacy Paradox: Social Networking in the United States. first monday .
Barth, S., & de Jong, M. D. T. (2017). The privacy Paradox – investigating discrepancies between expressed privacy concerns and actual online behavior – a systematic literature review. Telematics and Informatics , 34 (7), 1038–1058. https://doi.org/10.1016/j.tele.2017.04.013 .
Belen Saglam, R., Nurse, J. R., & Hodges, D. (2021). Privacy Concerns in Chatbot Interactions: When to Trust and When to Worry. HCI International 2021-Posters: 23rd HCI International Conference, HCII 2021, Virtual Event, July 24–29, 2021, Proceedings, Part II 23.
Berland, M., Baker, R. S., & Blikstein, P. (2014). Educational Data Mining and Learning analytics: Applications to Constructionist Research. Technology Knowledge and Learning , 19 , 205–220.
Bhatt, G. D. (2001). Knowledge Management in Organizations: Examining the Interaction between technologies, techniques, and people. Journal of Knowledge Management .
Bishop, C. M., & Nasrabadi, N. M. (2006). Pattern Recognition and Machine Learning (Vol. 4). Springer.
Biswas, S. S. (2023a). Potential use of Chat Gpt in global warming. Annals of Biomedical Engineering , 51 (6), 1126–1127.
Biswas, S. S. (2023b). Role of Chat Gpt in Public Health. Annals of Biomedical Engineering , 1–2.
Brill, T. M., Munoz, L., & Miller, R. J. (2019). Siri, Alexa, and other Digital assistants: A study of customer satisfaction with Artificial Intelligence Applications. Journal of Marketing Management , 35 (15–16), 1401–1436.
Cao, G., Duan, Y., Edwards, J. S., & Dwivedi, Y. K. (2021). Understanding managers’ attitudes and behavioral intentions towards using Artificial Intelligence for Organizational decision-making. Technovation , 106 , 102312.
Chao, C. M. (2019). Factors determining the behavioral intention to Use Mobile Learning: An application and extension of the Utaut Model. Frontiers in Psychology , 10 , 1652.
Chen, Q., Gong, Y., Lu, Y., & Tang, J. (2022). Classifying and measuring the Service Quality of Ai Chatbot in Frontline Service. Journal of Business Research , 145 , 552–568.
Chung, M., Ko, E., Joung, H., & Kim, S. J. (2020). Chatbot E-Service and customer satisfaction regarding luxury brands. Journal of Business Research , 117 , 587–595.
Cialdini, R. B., Darby, B. L., & Vincent, J. E. (1973). Transgression and altruism: A case for Hedonism. Journal of Experimental Social Psychology , 9 (6), 502–516.
Crawford, J., Butler-Henderson, K., Rudolph, J., Malkawi, B., Glowatz, M., Burton, R., Magni, P., & Lam, S. (2020). Covid-19: 20 countries’ higher education Intra-period Digital pedagogy responses. Journal of Applied Learning & Teaching , 3 (1), 1–20.
D’mello, S., & Graesser, A. (2013). Autotutor and Affective Autotutor: Learning by talking with cognitively and emotionally Intelligent computers that talk back. ACM Transactions on Interactive Intelligent Systems (TiiS) , 2 (4), 1–39.
Davis, F. D. (1989). Perceived usefulness, perceived ease of Use, and user Acceptance of Information Technology. MIS Quarterly , 13 (3), 319–340.
de Cosmo, L. M., Piper, L., & Di Vittorio, A. (2021). The role of attitude toward chatbots and privacy concern on the relationship between attitude toward Mobile Advertising and behavioral intent to Use Chatbots. Italian Journal of Marketing , 2021 (1), 83–102. https://doi.org/10.1007/s43039-021-00020-1 .
Desaid, D. (2020). A study of personalization effect . on Users Satisfaction with E Commerce Websites.
Dienlin, T., & Metzger, M. J. (2016). An extended privacy Calculus model for Snss: Analyzing Self-Disclosure and Self-Withdrawal in a Representative U.S. Sample. Journal of Computer-Mediated Communication , 21 (5), 368–383. https://doi.org/10.1111/jcc4.12163 .
Dinev, T., & Hart, P. (2006). An extended privacy Calculus model for E-Commerce transactions. Information Systems Research , 17 (1), 61–80.
Essel, H. B., Vlachopoulos, D., Tachie-Menson, A., Johnson, E. E., & Baah, P. K. (2022). The impact of a virtual teaching Assistant (Chatbot) on students’ learning in Ghanaian higher education. International Journal of Educational Technology in Higher Education , 19 (1), 1–19.
Fauzi, F., Tuhuteru, L., Sampe, F., Ausat, A. M. A., & Hatta, H. R. (2023). Analysing the role of Chatgpt in improving Student Productivity in Higher Education. Journal on Education , 5 (4), 14886–14891.
Firat, M. (2023). How Chat Gpt Can Transform Autodidactic Experiences and Open Education. Department of Distance Education, Open Education Faculty, Anadolu Unive .
Fishbein, M., & Ajzen, I. (1975). Belief, attitude, intention, and Behavior: An introduction to theory and research . Addison-Wesley.
Gatzioufa, P., & Saprikis, V. (2022). A Literature Review on Users’ Behavioral Intention toward Chatbots’ Adoption. Applied Computing and Informatics , ahead-of-print (ahead-of-print). https://doi.org/10.1108/ACI-01-2022-0021 .
Grant, R. M. (1996). Toward a knowledge-based theory of the firm. Strategic Management Journal , 17 (S2), 109–122.
Hair, J. F., Sarstedt, M., Hopkins, L., & Kuppelwieser, V. G. (2014). Partial least squares structural equation modeling (Pls-Sem): An Emerging Tool in Business Research. European Business Review , 26 (2), 106–121.
Hair, J. F., Hult, G. T. M., Ringle, C. M., & Sarstedt, M. (2021). A primer on partial least squares structural equation modeling (Pls-Sem) . Sage.
Haleem, A., Javaid, M., & Singh, R. P. (2022). An era of Chatgpt as a significant futuristic support Tool: A study on features, abilities, and challenges. BenchCouncil Transactions on Benchmarks Standards and Evaluations , 2 (4), 100089. https://doi.org/10.1016/j.tbench.2023.100089 .
Hasan, R., Shams, R., & Rahman, M. (2021). Consumer Trust and Perceived Risk for Voice-controlled Artificial Intelligence: The case of Siri. Journal of Business Research , 131 , 591–597.
Heisig, P. (2009). Harmonisation of Knowledge management–comparing 160 km frameworks around the Globe. Journal of Knowledge Management , 13 (4), 4–31.
Heo, M., & Lee, K. J. (2018). Chatbot as a New Business Communication Tool: The case of Naver Talktalk. Business Communication Research and Practice , 1 (1), 41–45.
Hocutt, D. L., Ranade, N., & Verhulsdonck, G. (2022). Localizing content: The roles of Technical & Professional communicators and Machine Learning in Personalized Chatbot responses. Technical Communication , 69 (4), 114.
Huang, Y. C. (2023). Integrated concepts of the Utaut and Tpb in virtual reality behavioral intention. Journal of Retailing and Consumer Services , 70 , 103127.
Huang, Z., & Benyoucef, M. (2013). From E-Commerce to Social Commerce: A close look at design features. Electronic Commerce Research and Applications , 12 (4), 246–259.
Huang, C. Y., Yang, M. C., & Huang, C. Y. (2021). An empirical study on factors influencing consumer adoption intention of an Ai-Powered Chatbot for Health and Weight Management. International Journal of Performability Engineering , 17 (5), 422–432.
Hund, A., Wagner, H. T., Beimborn, D., & Weitzel, T. (2021). Digital Innovation: Review and Novel Perspective. The Journal of Strategic Information Systems , 30 (4), 101695. https://doi.org/10.1016/j.jsis.2021.101695 .
Ischen, C., Araujo, T., Voorveld, H., van Noort, G., & Smit, E. (2020). Privacy Concerns in Chatbot Interactions. Chatbot Research and Design: Third International Workshop, CONVERSATIONS 2019, Amsterdam, The Netherlands, November 19–20, 2019, Revised Selected Papers 3.
Ivanov, S. H., & Webster, C. (2017). Adoption of Robots, Artificial Intelligence and Service Automation by Travel, Tourism and Hospitality Companies–a Cost-Benefit Analysis. Artificial Intelligence and Service Automation by Travel, Tourism and Hospitality Companies–A Cost-Benefit Analysis .
Jarrahi, M. H., Askay, D., Eshraghi, A., & Smith, P. (2023). Artificial Intelligence and Knowledge Management: A Partnership between Human and Ai. Business Horizons , 66 (1), 87–99. https://doi.org/10.1016/j.bushor.2022.03.002 .
Jo, H. (2022). Continuance intention to Use Artificial Intelligence Personal Assistant: Type, gender, and use experience. Heliyon , 8 (9), e10662. https://doi.org/10.1016/j.heliyon.2022.e10662 .
Kalla, D., & Smith, N. (2023). Study and Analysis of Chat Gpt and its impact on different fields of Study. International Journal of Innovative Science and Research Technology , 8 (3).
Kapoor, A. P., & Vij, M. (2018). Technology at the dinner table: Ordering Food Online through Mobile apps. Journal of Retailing and Consumer Services , 43 , 342–351.
Kasneci, E., Sessler, K., Küchemann, S., Bannert, M., Dementieva, D., Fischer, F., Gasser, U., Groh, G., Günnemann, S., Hüllermeier, E., Krusche, S., Kutyniok, G., Michaeli, T., Nerdel, C., Pfeffer, J., Poquet, O., Sailer, M., Schmidt, A., Seidel, T., & Kasneci, G. (2023). Chatgpt for Good? On opportunities and challenges of large Language models for Education. Learning and Individual Differences , 103 , 102274. https://doi.org/10.1016/j.lindif.2023.102274 .
Kelly, S., Kaye, S. A., & Oviedo-Trespalacios, O. (2022). A Multi-Industry Analysis of the Future Use of Ai Chatbots. Human Behavior and Emerging Technologies , 2022 .
Khan, U., Dhar, R., & Wertenbroch, K. (2005). A behavioral decision theory perspective on hedonic and utilitarian choice. Inside consumption (pp. 166–187). Routledge.
Khasawneh, O. Y. (2018a). Technophobia without boarders: The influence of Technophobia and Emotional Intelligence on Technology Acceptance and the moderating influence of Organizational Climate. Computers in Human Behavior , 88 , 210–218. https://doi.org/10.1016/j.chb.2018.07.007 .
Khasawneh, O. Y. (2018b). Technophobia: Examining its hidden factors and defining it. Technology in Society , 54 , 93–100.
Khasawneh, O. Y. (2022). Technophobia: How students’ Technophobia impacts their Technology Acceptance in an online class. International Journal of Human–Computer Interaction , 1–10. https://doi.org/10.1080/10447318.2022.2085398 .
Kim, D., & Benbasat, I. (2006). The effects of Trust-assuring arguments on Consumer Trust in Internet stores: Application of toulmin’s model of Argumentation. Information Systems Research , 17 (3), 286–300.
King, M. R., & ChatGPT. (2023). A conversation on Artificial Intelligence, Chatbots, and Plagiarism in Higher Education. Cellular and Molecular Bioengineering , 16 (1), 1–2.
Kokolakis, S. (2017). Privacy attitudes and privacy behaviour: A review of current research on the privacy Paradox Phenomenon. Computers & Security , 64 , 122–134.
Komiak, S. Y., & Benbasat, I. (2006). The effects of personalization and familiarity on Trust and Adoption of Recommendation agents. MIS Quarterly , 941–960.
Kotze, T. G., Anderson, O., & Summerfield, K. (2016). Technophobia: Gender differences in the adoption of high-technology Consumer products. South African Journal of Business Management , 47 (1), 21–28.
Koubaa, A., Boulila, W., Ghouti, L., Alzahem, A., & Latif, S. (2023). Exploring Chatgpt Capabilities and Limitations: A Critical Review of the Nlp Game Changer . https://doi.org/10.20944/preprints202303.0438.v1 .
Koul, S., & Eydgahi, A. (2020). The Impact of Social Influence, Technophobia, and Perceived Safety on Autonomous Vehicle Technology Adoption. Periodica Polytechnica Transportation Engineering , 48 (2), 133–142.
Lazarus, R. S. (1991). Emotion and adaptation . Oxford University Press.
Lund, B. D., & Wang, T. (2023). Chatting about Chatgpt: How may ai and gpt impact academia and libraries? Library Hi Tech News.
Lutz, C., & Tamò-Larrieux, A. (2021). Do Privacy Concerns About Social Robots Affect Use Intentions? Evidence from an Experimental Vignette Study [Original Research]. Frontiers in Robotics and AI , 8 . https://doi.org/10.3389/frobt.2021.627958 .
Maddigan, P., & Susnjak, T. (2023). Chat2vis: Generating Data Visualisations Via Natural Language Using Chatgpt, Codex and Gpt-3 Large Language Models. Ieee Access .
Maghsudi, S., Lan, A., Xu, J., & van Der Schaar, M. (2021). Personalized education in the Artificial Intelligence Era: What to expect Next. IEEE Signal Processing Magazine , 38 (3), 37–50.
Makice, K. (2009). Twitter Api: Up and running: Learn how to build applications with the Twitter Api . O’Reilly Media, Inc.
Manikonda, L., Deotale, A., & Kambhampati, S. (2018). What’s up with Privacy? User Preferences and Privacy Concerns in Intelligent Personal Assistants. Proceedings of the 2018 AAAI/ACM Conference on AI, Ethics, and Society.
Marakas, G. M., Yi, M. Y., & Johnson, R. D. (1998). The Multilevel and Multifaceted Character of Computer Self-Efficacy: Toward clarification of the Construct and an Integrative Framework for Research. Information Systems Research , 9 (2), 126–163.
Masood, A., Luqman, A., Feng, Y., & Shahzad, F. (2022). Untangling the adverse effect of Sns stressors on Academic Performance and its impact on students’ social media discontinuation intention: The moderating role of guilt. SAGE Open , 12 (1), 21582440221079905. https://doi.org/10.1177/21582440221079905 .
McCallum, S. (2023). Chatgpt Banned in Italy over Privacy Concerns . BBC. Retrieved May 1 from https://www.bbc.com/news/technology-65139406 .
McGee, R. W. (2023). Is Chat Gpt Biased against Conservatives? An Empirical Study. An Empirical Study (February 15, 2023) .
McLean, G., & Osei-Frimpong, K. (2019). Hey Alexa… examine the variables influencing the Use of Artificial Intelligent in-home Voice assistants. Computers in Human Behavior , 99 , 28–37.
Meng, B., & Choi, K. (2019). Tourists’ intention to use location-based services (lbs). International Journal of Contemporary Hospitality Management , 31 (8), 3097–3115. https://doi.org/10.1108/IJCHM-09-2018-0734 .
Mohd Rahim, N. I., Iahad, A., Yusof, N., A. F., & Al-Sharafi, A., M (2022). Ai-Based Chatbots Adoption Model for higher-education institutions: A hybrid pls-sem-neural Network Modelling Approach. Sustainability , 14 (19), 12726.
Moussawi, S., Koufaris, M., & Benbunan-Fich, R. (2021). How perceptions of intelligence and anthropomorphism affect adoption of Personal Intelligent agents. Electronic Markets , 31 (2), 343–364. https://doi.org/10.1007/s12525-020-00411-w .
Nguyen, D. M., Chiu, Y. T. H., & Le, H. D. (2021). Determinants of Continuance Intention Towards Banks’ Chatbot Services in Vietnam: A Necessity for Sustainable Development. Sustainability , 13 (14), 7625. https://www.mdpi.com/2071-1050/13/14/7625 .
Nonaka, I. (1994). A dynamic theory of Organizational Knowledge Creation. Organization Science , 5 (1), 14–37.
Pillai, R., & Sivathanu, B. (2020). Adoption of Ai-Based chatbots for hospitality and tourism. International Journal of Contemporary Hospitality Management , 32 (10), 3199–3226. https://doi.org/10.1108/IJCHM-04-2020-0259 .
Podsakoff, P. M., MacKenzie, M., Scott, B., Lee, J. Y., & Podsakoff, N. P. (2003). Common method biases in behavioral research: A critical review of the literature and recommended remedies. Journal of Applied Psychology , 885 (879). 10.1037.
Rafiq, F., Dogra, N., Adil, M., & Wu, J. Z. (2022). Examining Consumer’s intention to adopt Ai-Chatbots in Tourism using partial least squares structural equation modeling method. Mathematics , 10 (13), 2190.
Rijsdijk, S. A., Hultink, E. J., & Diamantopoulos, A. (2007). Product Intelligence: Its conceptualization, measurement and impact on consumer satisfaction. Journal of the Academy of Marketing Science , 35 , 340–356.
Rogers, E. (1983). Diffusion of Innovations, 3rd Editio Ed. In: New York.
Rogers, E. M. (2010). Diffusion of innovations . Simon and Schuster.
Rospigliosi, P. (2023). Artificial Intelligence in Teaching and Learning: What questions should we ask of Chatgpt? In (Vol. 31, pp. 1–3). Taylor & Francis.
Rudolph, J., Tan, S., & Tan, S. (2023). Chatgpt: Bullshit Spewer or the end of traditional assessments in higher education? Journal of Applied Learning and Teaching , 6 (1).
Ryan, R. M., & Deci, E. L. (2000). Self-determination theory and the facilitation of intrinsic motivation, Social Development, and well-being. American Psychologist , 55 (1), 68.
Selamat, M. A., & Windasari, N. A. (2021). Chatbot for smes: Integrating customer and business owner perspectives. Technology in Society , 66 , 101685.
Selwyn, N. (2013). Discourses of Digital Disruption in Education: A Critical Analysis. In Fifth International Roundtable on Discourse Analysis, City University Hong Kong, May 23–25, 2013 (pp. 1–28).
Smith, H. J., Dinev, T., & Xu, H. (2011). Information Privacy Research: An Interdisciplinary Review. MIS Quarterly , 989–1015.
Song, H. G., & Jo, H. (2023). Understanding the Continuance Intention of Omnichannel: Combining Tam and Tpb. Sustainability , 15 (4), 3039.
Sun, Y., & Jeyaraj, A. (2013). Information Technology Adoption and Continuance: A longitudinal study of individuals’ behavioral intentions. Information & Management , 50 (7), 457–465.
Tarafdar, M., Tu, Q., Ragu-Nathan, B. S., & Ragu-Nathan, T. (2007). The impact of technostress on role stress and Productivity. Journal of Management Information Systems , 24 (1), 301–328.
Urbach, N., Smolnik, S., & Riempp, G. (2010). An empirical investigation of employee Portal Success. The Journal of Strategic Information Systems , 19 (3), 184–206. https://doi.org/10.1016/j.jsis.2010.06.002 .
Varma Citrin, A., Sprott, D. E., Silverman, S. N., & Stem, D. E. (2000). Adoption of internet shopping: The role of consumer innovativeness. Industrial Management & Data Systems , 100 (7), 294–300. https://doi.org/10.1108/02635570010304806 .
Vázquez-Cano, E., Mengual-Andrés, S., & López-Meneses, E. (2021). Chatbot to Improve Learning Punctuation in Spanish and to Enhance Open and Flexible Learning environments. International Journal of Educational Technology in Higher Education , 18 (1), 33. https://doi.org/10.1186/s41239-021-00269-8 .
Venkatesh, V., Thong, J. Y. L., & Xu, X. (2012). Consumer Acceptance and Use of Information Technology: Extending the Unified Theory of Acceptance and Use of Technology. MIS Quarterly , 36 (1), 157–178. https://doi.org/10.2307/41410412 .
Wirtz, J., Patterson, P. G., Kunz, W. H., Gruber, T., Lu, V. N., Paluch, S., & Martins, A. (2018). Brave New World: Service Robots in the Frontline. Journal of Service Management , 29 (5), 907–931. https://doi.org/10.1108/JOSM-04-2018-0119 .
Xi, W., Zhang, X., & Ayalon, L. (2022). When less intergenerational closeness helps: The influence of intergenerational physical proximity and technology attributes on Technophobia among older adults. Computers in Human Behavior , 131 , 107234. https://doi.org/10.1016/j.chb.2022.107234 .
Yang, R., & Wibowo, S. (2022). User Trust in Artificial Intelligence: A comprehensive conceptual Framework. Electronic Markets , 32 (4), 2053–2077. https://doi.org/10.1007/s12525-022-00592-6 .
Yu, H., Miao, C., Leung, C., & White, T. J. (2017). Towards Ai-Powered personalization in Mooc Learning. Npj Science of Learning , 2 (1), 15.
Zeelenberg, M., & Pieters, R. (2007). A theory of Regret Regulation 1.0. Journal of Consumer Psychology , 17 (1), 3–18.
Zhu, X., Cao, Q., & Liu, C. (2022). Mechanism of Platform Interaction on Social Media Users&Rsquo Intention to Disclose Privacy: A Case Study of Tiktok App. Information , 13 (10), 461. https://www.mdpi.com/2078-2489/13/10/461 .
Download references
Acknowledgements
The author received no financial support for the research, authorship, and/or publication of this article.
Author information
Authors and affiliations.
Headquarters, HJ Institute of Technology and Management, Jungdong-ro 71 39, Bucheon-si, Gyeonggi-do, 14721, Republic of Korea
You can also search for this author in PubMed Google Scholar
Corresponding author
Correspondence to Hyeon Jo .
Ethics declarations
Declaration of generative ai and ai-assisted technologies in the writing process.
During the preparation of this work the author(s) used ChatGPT in order to improve language and readability. After using this tool/service, the author(s) reviewed and edited the content as needed and take(s) full responsibility for the content of the publication.
Additional information
Publisher’s note.
Springer Nature remains neutral with regard to jurisdictional claims in published maps and institutional affiliations.
Rights and permissions
Open Access This article is licensed under a Creative Commons Attribution 4.0 International License, which permits use, sharing, adaptation, distribution and reproduction in any medium or format, as long as you give appropriate credit to the original author(s) and the source, provide a link to the Creative Commons licence, and indicate if changes were made. The images or other third party material in this article are included in the article’s Creative Commons licence, unless indicated otherwise in a credit line to the material. If material is not included in the article’s Creative Commons licence and your intended use is not permitted by statutory regulation or exceeds the permitted use, you will need to obtain permission directly from the copyright holder. To view a copy of this licence, visit http://creativecommons.org/licenses/by/4.0/ .
Reprints and permissions
About this article
Cite this article.
Jo, H. From concerns to benefits: a comprehensive study of ChatGPT usage in education. Int J Educ Technol High Educ 21 , 35 (2024). https://doi.org/10.1186/s41239-024-00471-4
Download citation
Received : 05 February 2024
Accepted : 25 April 2024
Published : 03 June 2024
DOI : https://doi.org/10.1186/s41239-024-00471-4
Share this article
Anyone you share the following link with will be able to read this content:
Sorry, a shareable link is not currently available for this article.
Provided by the Springer Nature SharedIt content-sharing initiative
- Artificial Intelligence

IMAGES
VIDEO
COMMENTS
Case Study Methodology in Higher Education is an essential research publication that focuses on the history and theories relating to case study methodology including techniques for writing case studies and utilizing them in university settings to prepare students for real-life career-related scenarios. This publication features a wide range of ...
"Case Study Methodology in Higher Education" is an essential research publication that focuses on the history and theories relating to case study methodology including techniques for writing case studies and utilizing them in university settings to prepare students for real-life career-related scenarios. This publication features a wide range ...
Types of Case Studies Different scholars have suggested different typologies for case studies. Stake (1995) suggests three types of case study based on case selection: the intrinsic case study, where the case is given, rather than chosen, as commonly occurs in program evaluation; the instrumental case study, where the case is selected for its ability to contribute to a general understanding of a
Case study provides a sound methodology that can reflect the variability of research contexts and enables a flexible approach to address a wide range of research questions that are relevant to the evolving nature of WIL. Keywords: Case study, higher education, work-integrated learning, qualitative research
"This book focuses on the history and theories relating to case study methodology. It also examines the implications of utilizing case studies in university settings and explores how case studies prepare professionals for real-life career-related scenarios"--Read more.
This chapter describes the history of case study teaching, types of cases, and experimental data supporting their effectiveness. It also describes a model for comparing the efficacy of the various case study methods.
Taking the results of this study with the concept of constructive alignment (Biggs, 1996; Hrivnak, 2019), we can apply the findings of higher education research to the design of case method courses and of management education courses that use other pedagogies. Again, the R-SPQ-2F could be used to evaluate changes made as a result of this approach.
Case Study Methodology in Higher Education is an essential research publication that focuses on the history and theories relating to case study methodology including techniques for writing case studies and utilizing them in university settings to prepare students for real-life career-related scenarios. This publication features a wide range of ...
Case Study Research: Principles and Practices provides a general understanding of the case study method as well as specific tools for its successful implementation. These tools are applicable in a variety of fields including anthropology, business and management, communications, economics, education, medicine, political science, psychology ...
The types of case studies / Laurie Wellner, Kathleen Friedman -- Teaching with case studies in higher education / Lynne Orr, Linda Weekley -- Practicing what we preach : a case study of the implementation of a complex conceptual framework / Stephen Kroeger, Susan Gregson, Jonathan Breiner, Anna DeJarnette, Michelle Duda, Christopher Atchison -- Student-authored case studies : the case of an ...
Study Methodology . Teachers Training. The development of harmonic hearing is an essential component of the system of music teachers' training. The paper is concerned with the type of a case study which deals with the study of professional groups (a bigger and more diffusive group of 14 students). The process of training music teachers at sol ...
This study indicates that the case method is more effective than a lecture-based course, when evaluated in terms of approaches to learning, an internationally accepted measure of course quality in higher education. In particular, the case method promotes a deep approach (learning with intent and strategies to understand) and discourages a ...
The main aim of the present study was to design, implement, and assess an experience based on the case study methodology, approached from an interdisciplinary perspective.
Case Study Methodology in Higher Education is written by Annette Baron and published by Information Science Reference. The Digital and eTextbook ISBNs for Case Study Methodology in Higher Education are 9781522594321, 1522594329 and the print ISBNs are 9781522594291, 1522594299. Save up to 80% versus print by going digital with VitalSource.
In this paper, I draw on 10 years' experience of teaching research methods in higher education. I teach on a one-year part-time course that aims to help academics change their practice and contribute to the theories of teaching in higher education through publication of their work. ... The preferred research method is case study and there are ...
Case study is a 'method of learning' (Flyvbjerg, 2006) and writing for publication drives this to a higher standard. Qualitative research and case study, like all research, has to fulfill its purposes and these need to be worked out by the researcher as a guide to aligning the research process with outcomes. Case study should be judged on ...
Within the ongoing debate on the roles and tensions between teaching and research in higher education, this paper explores the extent to which the pedagogical case study method can contribute to ...
In this paper, I draw on 10 years' experience of teaching research methods in higher education. I teach on a one-year part-time course that aims to help academics change their practice and contribute to the theories of teaching in higher education through publication of their work. ... The preferred research method is case study and there are ...
Journal Higher Education Studies in Higher Education Teaching in Higher Education Higher Education Research & Development Total Editorial location Case study Conceptual study Total number of articles Europe Europe Europe Australasia 344 238 176 146 181 62 111 101 525 300 287 247 904 455 1359 Note: An article is defined as a case study if the ...
Search for: HOME; OUR MISSION. About; ACCESS MODELS. Institutional. Library Provisioned Textbooks
In higher education, case studies can be utilized to have students put themselves into problems faced by a protagonist and, by doing so, address academic or career-related issues. Working through ... Case Study Methodology in Higher Education is an essential research publication that focuses
Case-study methods in sustainability in higher education vary according to the researcher's purpose in conducting the case. Frequently, the researcher is an outside
The case study research method originated in clinical medicine (the case history, i.e., the patient's personal history). ... Saul Mcleod, PhD., is a qualified psychology teacher with over 18 years of experience in further and higher education. He has been published in peer-reviewed journals, including the Journal of Clinical Psychology ...
To enhance the student experience and increase access to experiential learning, colleges and universities have gotten creative with undergraduate research experiences. Undergraduate research opportunities are one way to provide experiential learning in many disciplines, introducing learners to research methods under the supervision of a faculty member and providing experience for a résumé.
Case Study Methodology in Higher Education is an essential research publication that focuses on the history and theories relating to case study methodology including techniques for writing case studies and utilizing them in university settings to prepare students for real-life career-related scenarios. This publication features a wide range of ...
The target population encompasses all students enrolled in nationally recognized higher education study programs during the survey period, year 2019. ... Method: principal-component factors ... Lukas K., Wilson R. (2014). Belonging in the first year: A creative discipline cohort case study. The International Journal of the First Year in Higher ...
Methods. This multiple-case study was funded, in part, by an NSF grant focused on supporting teachers to understand and teach engineering education within elementary contexts. This research seeks to understand nascent conceptualizations that undergraduate preservice elementary teachers have in relation to similar undergraduates focused on ...
Investors have taken note. Edtech start-ups raised record amounts of venture capital in 2020 and 2021, and market valuations for bigger players soared. A study conducted by McKinsey in 2021 found that to engage most effectively with students, higher-education institutions can focus on eight dimensions of the learning experience. In this article ...
This article indicates a case study about the effects of low-carbon education on the carbon emissions of college students. In 2019, low-carbon education was promoted on the campus of Shunde Polytechnic and questionnaire survey and interview method were used to study the carbon emissions and low-carbon behaviors of college students after 3 years.
Artificial Intelligence (AI) chatbots are increasingly becoming integral components of the digital learning ecosystem. As AI technologies continue to evolve, it is crucial to understand the factors influencing their adoption and use among students in higher education. This study is undertaken against this backdrop to explore the behavioral determinants associated with the use of the AI Chatbot ...