- Open access
- Published: 07 December 2020

Synergistic health effects of air pollution, temperature, and pollen exposure: a systematic review of epidemiological evidence
- Susan C. Anenberg ORCID: orcid.org/0000-0002-9668-603X 1 ,
- Shannon Haines 1 , 2 ,
- Elizabeth Wang 1 ,
- Nicholas Nassikas 3 &
- Patrick L. Kinney 4
Environmental Health volume 19 , Article number: 130 ( 2020 ) Cite this article
16k Accesses
92 Citations
14 Altmetric
Metrics details
Exposure to heat, air pollution, and pollen are associated with health outcomes, including cardiovascular and respiratory disease. Studies assessing the health impacts of climate change have considered increased exposure to these risk factors separately, though they may be increasing simultaneously for some populations and may act synergistically on health.
Our objective is to systematically review epidemiological evidence for interactive effects of multiple exposures to heat, air pollution, and pollen on human health.
We systematically searched electronic literature databases (last search, April 29, 2019) for studies reporting quantitative measurements of associations between at least two of the exposures and mortality from any cause and cardiovascular and respiratory morbidity and mortality specifically. Following the Navigation Guide systematic review methodology, we evaluated the risk of bias of individual studies and the overall quality and strength of evidence.
We found 56 studies that met the inclusion criteria. Of these, six measured air pollution, heat, and pollen; 39 measured air pollution and heat; 10 measured air pollution and pollen; and one measured heat and pollen. Nearly all studies were at risk of bias from exposure assessment error. However, consistent exposure-response across studies led us to conclude that there is overall moderate quality and sufficient evidence for synergistic effects of heat and air pollution. We concluded that there is overall low quality and limited evidence for synergistic effects from simultaneous exposure to (1) air pollution, pollen, and heat; and (2) air pollution and pollen. With only one study, we were unable to assess the evidence for synergistic effects of heat and pollen.
Conclusions
If synergistic effects between heat and air pollution are confirmed with additional research, the health impacts from climate change-driven increases in air pollution and heat exposure may be larger than previously estimated in studies that consider these risk factors individually.
Peer Review reports
Climate change is expected to increase exposure to environmental health risk factors, including extreme temperatures, air pollution, and aeroallergens [ 1 , 2 , 3 , 4 , 5 ]. These environmental health risk factors are associated with a range of health outcomes, including cardiovascular and respiratory disease [ 5 ]. Changes in these risk factors will be spatially heterogeneous, depending on local emission sources, meteorology, vegetation type and distribution, and other factors. As these risk factors do not exist in isolation, populations may experience simultaneous increases in exposure to heat, air pollutants, and pollen. Understanding whether these environmental health risk factors have synergistic effects on health outcomes can inform future climate change health risk assessments. The objective of this paper is therefore to determine whether the current state of the epidemiological evidence supports the presence of synergistic effects between heat, air pollutants, and pollen on human health outcomes.
Both average and extreme temperatures are expected to increase with climate change [ 5 ]. These changes may compromise the body’s ability to regulate temperature leading to a range of health outcomes, including heat exhaustion, heatstroke, and hyperthermia [ 6 ]. Exposure to extreme heat events can worsen cardiovascular and respiratory diseases, as well as other chronic conditions, such as cerebrovascular disease, diabetes, and kidney disease [ 7 , 8 ]. The mechanisms by which heat exacerbates respiratory disease are not well understood. In respiratory diseases such as asthma and chronic obstructive lung disease, inflammation plays a central role in the pathogenesis and exacerbation of the disease. Heat increases systemic and pulmonary inflammation as a consequence of thermoregulation – the attempt by the body to maintain a temperature within a safe range [ 9 ]. A second mechanism by which heat affects chronic lung disease may be related to impairment in breathing patterns meant to compensate for elevations in body temperature [ 10 , 11 ]. Heat induces cardiovascular disorders through multiple mechanisms including cell damage, inflammation, and blood clotting [ 12 ]. For mortality, epidemiological studies have linked even small increases in daily mean or maximum temperatures with increases in premature death. Applying these epidemiological exposure-response relationships to climate model simulations of future temperature, studies have attributed tens of thousands of premature deaths to increasing temperatures in the United States by mid-century [ 13 ]. The most vulnerable population subgroups to heat include older adults, children, people working outdoors, and economically disadvantaged communities [ 7 ], as well as end stage renal disease patients [ 14 ]. While climate adaptation measures can lessen some of the health impacts, climate change-related temperature increases are expected to be an important health risk factor in the U.S. and globally in the future.
Air pollution exposures may also increase with climate change through various pathways, including increased frequency of stagnation events that prohibit atmospheric venting, enhanced photochemical production of secondary pollutants (e.g. tropospheric ozone and some components of fine particulate matter, PM 2.5 ), and increasing “natural” gaseous and particulate emissions influenced by warmer and drier conditions (e.g. wildfire smoke, airborne soil dust, and ozone and PM 2.5 formation from biogenic volatile organic compounds) [ 2 ]. As a result, simulations of future air quality under various climate change scenarios indicate a likely “climate penalty” for ozone, making it harder to attain ambient air quality standards even with the same level of anthropogenic emission controls in place [ 15 , 16 ]. The literature is more mixed for the effects of climate change on PM 2.5 given the varied and often counteracting effects of climate on PM 2.5 components and precursor emissions, as well as atmospheric transport and loss. Recent studies suggest a potentially large influence of wildfire smoke and airborne soil dust on PM 2.5 concentrations [ 17 ]. Air pollution exposure can have large implications for human health, particularly heart and lung disease and mortality, through various mechanisms. Exposure to air pollutants, such as PM 2.5 and ozone, increases oxidative stress leading to pulmonary and systemic inflammation and increased permeability of the lung lining (airway epithelium), increased airway hyperresponsiveness in asthmatics, and decreases in lung function in healthy patients and patients with chronic lung disease [ 17 , 18 ]. Development and worsening of cardiovascular disease in response to air pollution exposure likely occurs along pathways that include systemic inflammation, alterations in coagulation, dysfunction in the lining of blood vessels (endothelial dysfunction), and progression of atherosclerosis [ 19 ]. Following these pathways, air pollution is associated with increased respiratory and cardiovascular mortality. Given the large body of epidemiological literature providing strong evidence for associations between PM 2.5 and premature mortality from cardiovascular disease, respiratory disease, and lung cancer, and between ozone and respiratory mortality, even small increases in pollution levels in the future can have profound influences on human health outcomes [ 17 , 20 ].
Climate change is also expected to affect the start, duration, and intensity of the pollen season, with changes differing by region [ 21 ]. Climate change and rising greenhouse gas concentrations are correlated with aeroallergens in a number of ways, including increased and faster plant growth, increased pollen production by plants, increased allergenic proteins contained in pollen, earlier start time of plant growth, and longer plant seasons [ 22 ]. Meteorological conditions, including precipitation, atmospheric temperature, humidity, and wind speed, can alter the concentrations of plant pollens, which can then influence the occurrence of allergic diseases [ 23 ]. Inhalation of pollen grains causes disruption of the immune system within the lungs and increases the susceptibility of individuals to respiratory viral infections [ 24 ]. These breakdowns in immune system defenses following exposure to pollen are seen not only in patients with underlying allergies, but also in healthy individuals. In asthmatics, exposure to pollen activates an array of immune cells resulting in bronchoconstriction and increased permeability of airway epithelium [ 25 ]. There are few studies that have examined the link between aeroallergen exposure and cardiovascular disease; however, airborne pollen may be a risk factor for myocardial infarction [ 26 ]. The mechanism may be related to pollen triggering mast cell activation and histamine release leading to coronary artery spasm or plaque rupture. With the pervasiveness of allergies and allergic asthma among diverse populations throughout the U.S. and the world, climate-related changes in aeroallergen exposure may have widespread impacts on allergic rhinitis and asthma emergency department visits, both of which place a heavy burden on the U.S. healthcare system.
There is substantial literature on respiratory and cardiovascular outcomes related to the isolated exposure to heat, air pollution, or pollen [ 12 , 27 , 28 , 29 ]. However, fewer studies examine potential synergies or mechanisms behind interactions among these environmental risk factors. There is evidence that air pollutants can bind to pollen grains, precipitating faster release of allergens, increasing allergen absorption in the lungs, and potentiating the allergenicity of pollen, however this is mostly supported in in vitro and animal studies and the clinical significance on a population level is less certain [ 17 , 30 , 31 ]. Prior studies suggest a joint effect of air pollution and heat on health outcomes such as mortality and respiratory morbidity [ 32 ]. Many disease states, including heart and lung disease, share a common pathway in which exposure to heat, air pollution, and pollen causes systemic and organ-specific inflammation and cellular damage [ 9 , 17 , 28 , 33 ].
Previous studies assessing the potential health impacts of future climate change have considered heat, air pollution, and pollen exposure individually and have not accounted for potential synergistic effects [ 7 , 34 , 35 , 36 , 37 , 38 , 39 , 40 ]. For example, the comprehensive Climate Change Impacts and Risk Analysis project for the U.S. includes estimates of future increases in heat-related mortality, ozone-related mortality, and asthma emergency department visits attributable to aeroallergens, with substantial increases simulated for moderate and severe climate scenarios [ 4 , 41 ]. Each of these risk factors was considered separately when estimating future health impacts. If there are synergistic effects between these exposures, using single-hazard approaches may underestimate the health impacts of heat, air pollution, and pollen exposures under climate change.
Here, we conduct a systematic literature review of epidemiological studies to determine whether simultaneous exposure to heat, air pollution, and pollen (or a subset of these risk factors) synergistically increases the risk of mortality from any cause and mortality and morbidity of cardiovascular and respiratory disease specifically. We focus on these three risk factors as they share common attributes – they are conditions of the ambient air and have been found to affect respiratory and cardiovascular health. Other risk factors associated with climate change may also affect these health systems, but we consider the body of literature to be too nascent to support a more inclusive systematic review. Results of our review may be useful to more comprehensively characterize future public health disease burdens under climate change scenarios.
Search strategy, study selection, and data extraction
We conducted a systematic literature review using the Navigation Guide, a methodology for evaluating environmental evidence based on methods used in the clinical sciences [ 42 ]. The objective of this systematic review is to assess whether there are interactions between exposure to criteria air pollutants, extreme heat, and pollen, or a subset of these three risk factors, on cardiovascular or respiratory outcomes in human populations. Criteria air pollutants include ground-level ozone (O 3 ), carbon monoxide (CO), nitrogen dioxide (NO 2 ), lead, particulate matter (PM), and sulfur dioxide (SO 2 ).
We define the “Population”, “Exposure”, “Comparator”, and “Outcomes” (PECO) statement as:
Population: Any human population of any age in any location.
Exposure : Areas where populations are simultaneously exposed to a) criteria air pollutants and extreme heat; b) criteria air pollutants and pollen; c) pollen and extreme heat; or d) all three risk factors.
Comparator: Areas where these simultaneous exposures are not occurring.
Outcome: Cardiovascular and respiratory diseases or mortality.
We searched the databases PubMed, ProQuest, and Scopus with the search terms “air pollution”, “air quality”, “air pollutants”, “pollen”, “aeroallergens”, “temperature”, “heat”, “dust”, “NO 2 ”, “SO 2 ”, “particulate matter”, “ozone”, “multipollutant” for exposures, and the terms “cardiovascular”, “respiratory”, “mortality”, “asthma”, and “allergies” for outcomes (Table S 1 ). We conducted a first search on April 22, 2019 and an updated search with more search terms on April 29, 2019. We found additional articles through hand searching the references of fully screened articles.
We included original studies that measured at least two of the exposures (heat, air pollution, and pollen) and at least one of the health outcomes (cardiovascular or respiratory disease or mortality), without limiting by publication date. We excluded studies that were not published in English, did not study a human population, did not measure at least two of the exposures, did not report quantitative results for exposure-response relationships, or did not describe interactions between the exposures. We screened for reference duplicates using Mendeley Desktop. When it was not clear whether studies met the inclusion criteria or not, two reviewers discussed each study and came to a joint decision on inclusion or exclusion.
Data extraction and risk of bias for each included study
Two authors independently extracted data and analyzed risk of bias for each included study. A third author reviewed all studies to resolve discrepancies between the two independent reviewers’ risk of bias ratings. We evaluated risk of bias for each of our included studies using the Cochrane Collaboration’s “Risk of Bias” tool and the Agency for Healthcare Research and Quality’s domains [ 43 ]. The domains we evaluated included study design, exposure assessment (air pollution), exposure assessment (temperature), exposure assessment (pollen), detection of outcome, reporting, and conflict of interest. Study design was rated as “low” risk of bias if it was a cohort, case crossover, or time series design. To be rated as “low” risk of bias for air pollution exposure assessment, the study must have measured at least two criteria pollutants and must have measured them in a way that represented individual exposure. To be rated as “low” risk of bias for pollen exposure assessment, the study had to use a method that measured pollen exposure at an individual level. To be rated as “low” risk of bias for temperature, studies had to use data from meteorological surveillance networks; we did not judge a lack of individual exposure measurement to introduce high risk of bias for temperature since temperature is less spatially heterogeneous compared with air pollution. To be rated as “low” risk of bias for detection of health outcome, the study had to use the International Classification of Diseases (ICD) to classify the health outcome category. To be rated as “low” risk of bias for reporting, the study had to report all outcomes that were assessed. To be rated as “low” risk of bias for conflict of interest, the study had to acknowledge that there was no conflict of interest. The possible ratings for the studies for each domain were “low”, “probably low”, “probably high”, or “high” risk of bias. We used the “probably low” and “probably high” categories when not enough information was given to definitively assign “low” and “high” ratings.
Quality and strength of evidence across studies
To evaluate the quality and strength of evidence across all studies, we used the Grading of Recommendations Assessment, Development and Evaluation (GRADE) systematic review approach [ 44 ]. We stratified papers by the following categories of multiple exposures: 1) heat, air pollution, and pollen; 2) heat and air pollution; 3) air pollution and pollen; and 4) heat and pollen.
To evaluate the quality of the evidence across all studies, we upgraded and downgraded studies according to several criteria. Downgrading factors included serious risk of bias, serious indirectness in the studies such that evidence is not directly comparable to our PECO statement criteria, serious inconsistency in effect estimates across studies, serious imprecision due to small sample size and/or small outcome count, and likely publication bias resulting in an over or underestimate of true effects from exposure. Downgrading for serious risk of bias by − 1 occurred if there were instances of an unclear limitation in the evidence and by − 2 if there were instances of serious limitations or very serious limitations during the assessments. Downgrading for inconsistency by − 1 occurred if there were minimal or no overlap of confidence intervals and by − 2 if there was wide variance of point estimates across studies. Downgrading for indirectness by − 1 was applied if there were large differences in study population and by − 2 if there were large differences and if surrogate outcomes were applied. Downgrading for imprecision by − 1 occurred if there was a small sample size or small outcome count and by − 2 if there was both.
Upgrading factors included large magnitude of effect such that confounding alone could not explain the association, consistent dose-response gradient across studies, all plausible confounding would reduce a demonstrated effect, and all possible confounding would suggest a spurious effect when the actual results show no effect. After considering the upgrading and downgrading factors, the studies were then given a rating of “low quality”, “moderate quality”, or “high quality.” Possible ratings were 0, meaning no change from initial quality rating, − 1 or − 2, meaning downgrades in quality rating, and + 1 and + 2, meaning upgrades in quality rating. Upgrading for large magnitude of effect by + 1 occurred with the effect estimate was large such as a relative risk of 2 or higher and by + 2 if there was a very large effect estimate such as a relative risk of 5 or higher. Upgrading for dose-response by + 1 was applied if there was observation that there was a dose response gradient between increased exposure and increased outcomes and by + 2 if there was a rapid and large absolute increase in outcomes as dose increased. Upgrading for effect of plausible confounding by + 1 was applied if the plausible confounders were adjusted for in the analysis.
We evaluated the strength of evidence across all studies based on quality of the evidence, direction of effect estimates, confidence in effect estimates, and other attributes [ 45 ]. To the extent possible, we discuss these ratings according to categories of health outcomes (e.g. all-cause mortality, cardiovascular disease, and respiratory disease). The ratings for strength of the evidence are: “evidence of lack of association” (studies show no adverse effect), “inadequate evidence” (studies permit no conclusion about an effect), “limited evidence” (studies suggest an effect but only in a single or limited number of studies), and “sufficient evidence” (studies indicate a causal relationship between exposure and effect). We followed the more detailed definitions of each strength rating given by Johnson et al. [ 46 ].
Our search retrieved 1730 unique records, and we added 16 papers identified through other sources (Fig. 1 ). We screened 605 papers after removing duplicates and assessed the full text of 406 articles for eligibility. We excluded 350 articles because they did not describe interactions between the exposures or did not describe the outcome measures. Ultimately, we included 56 studies that met our eligibility criteria. Table 1 includes descriptions of each study.
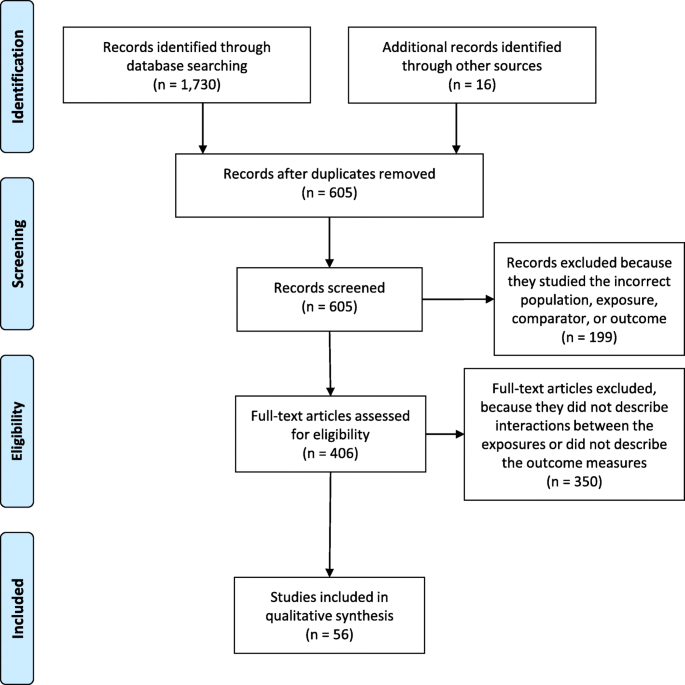
PRISMA Diagram showing the number of studies included and excluded at each step
Of these 56 studies, six measured air pollution, heat, and pollen; 39 measured air pollution and heat; 10 measured air pollution and pollen; and one measured heat and pollen. Forty-six studies were a time series design, three were cohort studies, one was a cross sectional design, one was a nested case control design, and five were a case-crossover design. Data collection in these studies ranged from 1987 to 2010 and publication date ranged from 2002 to 2018. The qualifying studies ranged widely in air pollutants and pollen types measured, metrics used for each exposure type (e.g. averaging times, time lags), and health outcomes (including asthma and hay fever symptoms, cardiovascular and respiratory emergency department visits and hospitalizations, cause-specific mortality, and all-cause mortality).
Risk of bias determinations and rationale for each study can be found in Tables S 2 through S 57 . Almost all of the studies were rated as “low” or “probably low” risk of bias for study design, detection of outcome, reporting, and conflict of interest (Fig. 2 ). Risk of bias for exposure assessment varied across the studies. For air pollution and pollen, we rated many studies as having a “probably high” risk due to a lack of exposure measurement at an individual level, as they used exposure assessment techniques such as central site monitors that are broadly representative of regional air pollution levels but may not represent individual exposure well. Several of these studies only used one central site monitor, which we judged could potentially introduce bias since pollution levels vary spatially within geographic areas such as cities. For temperature, studies were generally rated as having a “low” or “probably low” risk of bias since data were sourced from meteorological monitoring networks and temperature is less spatially heterogeneous compared with air pollution.
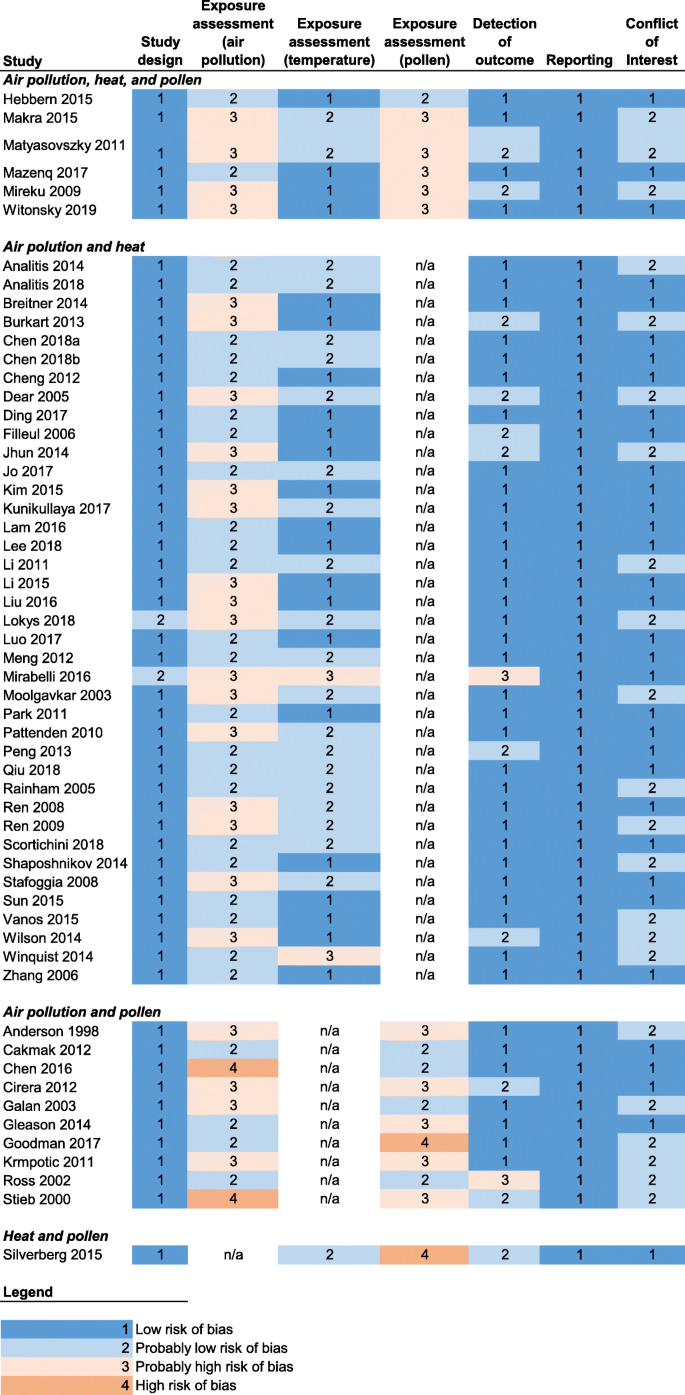
Final risk of bias evaluation for each study
We next assessed the quality and strength of the evidence across the studies. We found six studies that examined potential interactive effects between simultaneous exposure to all three risk factors: air pollutants, pollen, and heat (Table 1 ). The studies were conducted in Canada, France, Hungary, and the U.S. and all focused on respiratory hospitalizations and emergency department visits (all except one focused specifically on asthma). The studies used widely different methods for categorizing temperature exposure, including spatial synoptic classification [ 47 , 48 ], seasonal analysis [ 52 ], and interday temperature change [ 51 ]. Generally, the studies were individually rated as low risk of bias for most categories, including study design, detection of outcome, reporting, and conflict of interest. However, we judged some to be at probably high risk of bias for exposure assessment for both air pollutants and pollen. The findings across the studies were inconsistent, with some studies reporting interactive effects of all three or some combination of the exposures [ 47 , 48 , 49 , 52 ], while others reported independent effects that were unaffected by controlling for the other risk factors [ 51 ] or were inconclusive when considering simultaneous exposure to all three risk factors [ 50 ].
Overall, we rated the quality of the evidence for synergistic respiratory effects between air pollution, heat, and pollen as “low” since studies were inconsistent in finding significant evidence of interactive effects and studies that reported positive associations of interactions had minimal magnitudes (Table 2 ). We rated the overall strength of the evidence as “limited” since synergistic effects between heat, air pollution, and pollen were observed in some studies, but these findings were not consistent across studies.
We found 39 articles that examined potential interactive effects between exposure to air pollutants and heat (Table 1 ). These studies were carried out in Europe, the U.S., Canada, Russia, Taiwan, South Korea, India, Hong Kong, and China. Most were conducted in urban areas. A majority of the studies (29) included health endpoints that were not disease-specific, such as all-cause and non-accidental mortality. A smaller subset of 12 studies considered respiratory disease specifically (some focusing on asthma specifically) and 11 considered cardiovascular disease specifically (we have included migraine in this category as a potential indicator of cardiovascular disease, Adelborg et al. [ 103 ]). Most studies included multiple criteria pollutants – most often ozone and PM 10 , though some only included ozone, and some also included PM 2.5 , PM 2.5–10 , NO 2 , SO 2 , and CO. The temperature metric differed between studies and included daily mean, minimum and/or maximum.
Of these 39 studies addressing synergistic effects between air pollution and heat, 19 reported interactive effects between heat and air pollution exposure on health outcomes studied. Out of these studies, 15 of 29 studies examined health outcomes that were not disease-specific (e.g. all-cause mortality, hospital admissions) and found synergistic effects [ 53 , 54 , 55 , 57 , 58 , 60 , 61 , 66 , 68 , 71 , 73 , 74 , 75 , 76 , 77 ], four of 12 studies found synergistic effects for respiratory health outcomes [ 55 , 57 , 59 , 84 ], and eight of 11 studies found synergistic effects for cardiovascular health outcomes [ 54 , 55 , 57 , 58 , 59 , 88 , 90 , 91 ]. Here, we are not distinguishing between mortality and morbidity for respiratory and cardiovascular health outcomes. Generally, the studies found synergistic effects from simultaneous exposure to extremely high temperatures and air pollution, with a potentially additional role of relative humidity. A method of weather classification that incorporated humidity used in some of the papers was spatial synoptic classification (SSC), which is described as a “semi-automated statistical approach designed to classify complex daily weather conditions into one of six distinct categories, or a transitional category” and uses values of temperature, dew point, u and v components of wind, cloud cover, and sea level pressure [ 47 , 48 , 73 , 78 ]. A strength of this group of studies was the large datasets of pollutant levels and meteorology, including from the National, Morbidity, Mortality, and Air Pollution Study (NMMAPS) in the United States [ 61 , 65 , 67 , 90 , 91 ] and the Ultrafine Particles and Health Study Group in Europe [ 61 , 62 ]. Compared with the other categories in our review, air pollution and heat studies covered the broadest geographic area and included the largest number of people in the studies.
The evidence was strongest for synergistic effects between heat and exposure to either ozone and PM 2.5 . For ozone, 11 of 29 studies reported synergistic effects with heat [ 53 , 54 , 55 , 60 , 61 , 71 , 73 , 74 , 84 , 90 , 91 ]. These effects were found among inter quartile temperature analysis, seasonal analysis, and heatwave analysis in the studies. Effects were found for all-cause mortality, non-accidental mortality, cardiovascular mortality, and morbidity outcomes. High levels of ozone and high temperatures tended to be reported together and the strongest effects on outcomes were found at the highest exposures. We also found evidence for synergistic effects between heat and particulate matter, with 10 of 27 studies reporting synergistic effects [ 53 , 54 , 60 , 61 , 66 , 73 , 74 , 75 , 76 , 88 ]. These effects were found among inter quartile temperature analysis, seasonal analysis, and heatwave analysis in the studies. Effects were found for all-cause mortality, non-accidental mortality, and morbidity outcomes. A potential interactive effect between heat and particulate matter is further supported by Mazenq et al. [ 50 ], who found that temperature and particulate matter were linked but pollen was not.
While most studies assessing synergistic effects between air pollution and temperature focused on heat, several examined effects of cold [ 55 , 56 , 58 , 59 , 60 , 61 , 62 , 67 , 70 , 73 , 77 , 79 , 80 , 83 , 84 , 86 , 87 , 88 ]. Generally, stronger results were found in warmer seasons when compared to cold seasons. Zhang et al. [ 80 ] was the only study in our review that found that synergy between ozone and the cold season was stronger than for the warm season.
We upgraded the overall quality of the evidence of synergistic effects between air pollution and heat because of the relatively consistent finding of significant exposure-response relationships showing interactive effects (Table 3 ). The consistent findings of interactive effects between air pollutants and heat held for all three health outcome categories considered: health outcomes that were not disease-specific (e.g. all-cause mortality), respiratory disease, and cardiovascular disease, though more studies found interactive effects for non-cause-specific endpoints and for cardiovascular disease than for respiratory disease. This result may highlight the need for more studies focusing not only on respiratory disease, but also on other diseases. These factors led us to rate the overall quality of the evidence as “Moderate” and the overall strength of the evidence as “Sufficient.”
We found 10 studies that assessed potential interactive effects between exposure to air pollution and pollen (Table 1 ). These studies were conducted in Europe, Canada, Australia, and the U.S. Studies included a variety of pollen types and air pollutants, with little consistency between them. Health outcomes considered were all respiratory morbidity (mostly hospital admissions and emergency department visits), with the exception of one that focused on cardiopulmonary emergency department visits [ 101 ].
The studies in this category were inconsistent in their study designs and findings. For example, Anderson et al. [ 92 ] concluded that there was no evidence for synergy between air pollutants and pollen, with the exception of SO 2 and grass pollen in children during the warm season. Chen et al. [ 94 ] also found little evidence of interactions between air pollutants and pollen but did find that several of the air pollution and pollen exposures were stronger in the cool season than in the warm season. In contrast, Goodman et al. [ 98 ] found that, in most populations, adjusting for outdoor pollen generally attenuated relative risk of hospital admissions for both ozone and PM 2.5 . Ross et al. [ 100 ] found the association between ozone and asthma medication use was increased after adjusting for aeroallergens. Cakmak et al. [ 93 ] found that there were synergistic effects on asthma hospitalization between tree pollen and increasing PM 2.5 , and between weed pollen and PM 10 .
Given that the 10 studies included inconsistent pollen types and air pollutants, with inconsistent results, we were unable to draw strong conclusions for this category. Overall, we rated the quality of the evidence as “Low” and the strength of the evidence as “Limited.” We did not upgrade the quality of the evidence since the studies reported inconsistent findings, and since studies that did find synergistic effects reported effect estimates that had low magnitudes (Table 4 ).
Our search only found one study that examined interactions between heat and pollen [ 102 ]. This study explored climate factors and pollen count impacts on pediatric hay fever prevalence among 91,642 children across the U.S. Hay fever prevalence was shown to increase with the second, third, and fourth quartile mean annual temperature and mean total pollen counts. This study was particularly strong given the large size and national representation of the included population. However, with only one study, we did not draw conclusions regarding the quality and strength of evidence for interactive effects between heat and pollen.
We conducted a systematic literature review of human population health studies to examine the evidence for synergistic effects from simultaneous exposure to air pollution, pollen, and heat, or a subset of these three risk factors. We found limited evidence for synergistic respiratory effects of air pollution, pollen, and heat; sufficient evidence for synergistic all-cause mortality, cardiovascular, and respiratory effects of air pollution and heat (particularly for ozone and particulate matter); and limited evidence for synergistic respiratory effects of air pollution and pollen. We were unable to assess evidence for pollen and heat because only one paper came up in our searches.
Overall, there was a substantially larger body of literature examining interactive effects between air pollution and heat, compared with those that included pollen as an exposure of interest. The evidence for interactive effects between air pollution and heat is further strengthened by large datasets of pollutant levels and meteorological data, including from the National, Morbidity, Mortality, and Air Pollution Study (NMMAPS) in the U.S. and the Ultrafine Particles and Health Study Group in Europe. An additional strength across all categories was that a majority of the studies had a low risk of bias for study design, with many of them using a time series design.
Though there were some strengths in the literature, we also found serious weaknesses that precluded our ability to draw strong conclusions as to the existence of interactive health effects from simultaneous exposure to these risk factors. Limitations included that all of the studies we found were short-term studies that were unable to address effects of long-term exposure. We found no cohort studies that could properly attribute exposure at an individual level and account for health outcomes that may take years to manifest. In addition, exposure measurements and metrics for air pollutants, pollen, and temperature were inconsistent and not standardized between the studies. Judging the potential bias from exposure measurement for air pollution, temperature, and pollen is difficult with only limited information available in the papers. For example, some papers did not report the number of monitoring stations used to assign exposures or the length of time for which the exposure data were collected. Recent studies of air pollution have begun using more sophisticated methods to assign exposure, such as models that use satellite remote sensing or land use variables that provide greater spatial coverage compared with ground monitors such as those run by government monitoring networks [ 104 , 105 , 106 ]. For pollen, the studies in this review all used pollen count as the exposure metric, which may not account for pollen potency [ 23 ]. Another limitation is that many studies were missing information about confounders that were considered, which could influence the magnitude of the associations they found. Finally, while we restricted our review to studies that looked at interaction between two of the three hazards, several studies may have treated these risk factors as mediators or effect modifiers. Future research should explore the role of these issues. Additional research should also explore effects of these risk factors on additional health outcomes, such as birth outcomes, as well as vulnerable populations, including children, the elderly, pregnant women, and people with genetic predisposition to cardiovascular and respiratory disease.
We included only heat, air pollution, and pollen in this review, as they are all conditions of the ambient air for which we judged there to be enough epidemiological literature to assess. Other important environmental drivers of disease related to the ambient air that we did not include here are occupational exposures; different types of air pollutant mixtures (including from different combustion sources and different composition of particulate matter); and exposure to airborne bacteria, viruses, molds, and fungus. In reality, people are exposed to a complex set of risk factors that remain poorly defined and explored in the literature. In addition, the chronic diseases considered affected by these risk factors are multi-factorial with heavy influence from genetic and lifestyle (e.g. diet, exercise) factors. Our literature review highlights the importance of including environmental factors in epidemiological and risk assessment studies, even if strong conclusions cannot yet be drawn from the current set of available studies.
In this systematic literature review of epidemiological studies, we found evidence for synergistic effects of heat and air pollutants (particularly for ozone and particulate matter), but not for the combination of heat, air pollution, and pollen together or of air pollution and pollen or heat and pollen. Our findings support consideration of combined effects of heat and air pollution in assessing health impacts from these risk factors in the present day and in the future as climate change progresses. However, the literature is too nascent to support inclusion of interactive effects between air pollution and pollen or heat and pollen in risk assessments. Future research should continue to explore potential interactive effects of environmental exposures on human health, as people are often exposed to multiple environmental risk factors simultaneously. This is a rapidly evolving field of study, and our review and conclusions should be updated to include new evidence as it becomes available. If new evidence supports our conclusion that heat and air pollution exposure act synergistically on human health, the health impacts from climate change-driven increases in air pollution and heat exposure may be larger than previously estimated in studies that consider these risk factors individually.
Availability of data and materials
All data are available within the article and supplemental material.
Abbreviations
Carbon monoxide
Grading of Recommendations Assessment, Development and Evaluation
Nitrogen dioxide
Population, Exposure, Control, Outcome
Fine particulate matter
Coarse particulate matter
Sulfur dioxide
Fiore AM, Naik V, Leibensperger EM. Air quality and climate connections. J Air Waste Manage Assoc. 2015 Jun 3;65(6):645–85.
Article CAS Google Scholar
Kinney PL. Climate change, air quality, and human health. Am J Prev Med. 2008 Nov;35(5):459–67.
Article Google Scholar
Kinney PL. Interactions of climate change, air pollution, and human health. Curr Envir Health Rpt. 2018 Mar;5(1):179–86.
U.S. Environmental Protection Agency. Multi-Model Framework for Quantitative Sectoral Impacts Analysis: A Technical Report for the Fourth National Climate Assessment [Internet]. U.S. Environmental Protection Agency; 2017 [cited 2017 Nov 8]. Available from: https://cfpub.epa.gov/si/si_public_record_Report.cfm?dirEntryId=335095 .
US Global Change Research Program. The Impacts of Climate Change on Human Health in the United States: A Scientific Assessment [Internet]. U.S. Global Change Research Program (USGCRP); 2016. Available from: https://doi.org/10.7930/J0R49NQX .
McGeehin MA, Mirabelli M. The potential impacts of climate variability and change on temperature-related morbidity and mortality in the United States. Environ Health Perspect. 2001 May;109(suppl 2):185–9.
Melillo JM, Richmond T (T. C), Yohe GW. Climate Change Impacts in the United States: The Third National Climate Assessment [Internet]. U.S. Global Change Research Program; 2014 [cited 2020 Nov 18]. Available from: https://nca2014.globalchange.gov/downloads .
Xu R, Zhao Q, Coelho MSZS, Saldiva PHN, Zoungas S, Huxley RR, et al. Association between heat exposure and hospitalization for diabetes in Brazil during 2000–2015: a Nationwide case-crossover study. Environ Health Perspect. 2019 Nov;127(11):117005.
Anderson GB, Dominici F, Wang Y, McCormack MC, Bell ML, Peng RD. Heat-related emergency hospitalizations for respiratory diseases in the Medicare population. Am J Respir Crit Care Med. 2013 May 15;187(10):1098–103.
Mannino DM. The natural history of chronic obstructive pulmonary disease. Eur Respir J. 2006 Mar 1;27(3):627–43.
White MD. Components and mechanisms of thermal hyperpnea. J Appl Physiol. 2006 Aug;101(2):655–63.
Liu C, Yavar Z, Sun Q. Cardiovascular response to thermoregulatory challenges. Am J Phys Heart Circ Phys. 2015 Dec 1;309(11):H1793–812.
CAS Google Scholar
Petkova E, Bader D, Anderson G, Horton R, Knowlton K, Kinney P. Heat-related mortality in a warming climate: projections for 12 U.S. cities. IJERPH. 2014 Oct 31;11(11):11371–83.
Remigio RV, Jiang C, Raimann J, Kotanko P, Usvyat L, Maddux FW, et al. Association of Extreme Heat Events with Hospital Admission or mortality among patients with end-stage renal disease. JAMA Netw Open. 2019 Aug 9;2(8):e198904.
Reidmiller DR, Avery CW, Easterling DR, Kunkel KE, Lewis KLM, Maycock TK, et al. Impacts, Risks, and Adaptation in the United States: The Fourth National Climate Assessment, Volume II [Internet]. U.S. Global Change Research Program; 2018 [cited 2020 Nov 18]. Available from: https://nca2018.globalchange.gov/ .
Wu S, Mickley LJ, Leibensperger EM, Jacob DJ, Rind D, Streets DG. Effects of 2000–2050 global change on ozone air quality in the United States. J Geophys Res. 2008;113(D6):D06302.
Google Scholar
Integrated Science Assessment (ISA) for Particulate Matter (Final Report, Dec 2019) [Internet]. Washington, DC, U.S.: U.S. Environmental Protection Agency; 2019 p. 1967. Report No.: EPA/600/R−19/188, 2019. Available from: https://cfpub.epa.gov/ncea/isa/recordisplay.cfm?deid=347534 .
Integrated Science Assessment (ISA) for Ozone and Related Photochemical Oxidants (Final Report, Feb 2013) [Internet]. Washington, DC, U.S.: U.S. Environmental Protection Agency; 2013 p. 1251. Report No.: EPA/600/R-10/076F, 2013. Available from: https://cfpub.epa.gov/ncea/isa/recordisplay.cfm?deid=247492 .
Cosselman KE, Navas-Acien A, Kaufman JD. Environmental factors in cardiovascular disease. Nat Rev Cardiol. 2015 Nov;12(11):627–42.
Garcia-Menendez F, Saari RK, Monier E, Selin NE. U.S. air quality and health benefits from avoided climate change under greenhouse gas mitigation. Environ Sci Technol. 2015 Jul 7;49(13):7580–8.
Ziska LH, Beggs PJ. Anthropogenic climate change and allergen exposure: the role of plant biology. J Allergy Clin Immunol. 2012 Jan;129(1):27–32.
D’Amato G, Rottem M, Dahl R, Blaiss M, Ridolo E, Cecchi L, et al. Climate change, migration, and allergic respiratory diseases: an update for the allergist. World Allergy Organ J. 2011 Jul;4(7):120–5.
D’Amato G, Holgate ST, Pawankar R, Ledford DK, Cecchi L, Al-Ahmad M, et al. Meteorological conditions, climate change, new emerging factors, and asthma and related allergic disorders. A statement of the World Allergy Organization. World Allergy Organization J. 2015;8:25.
Gilles S, Blume C, Wimmer M, Damialis A, Meulenbroek L, Gökkaya M, et al. Pollen exposure weakens innate defense against respiratory viruses. Allergy. 2020 Mar;75(3):576–87.
Weinberger SE, Cockrill BA, Mandel J. Principles of pulmonary medicine. 7th ed. Philadelphia, PA: Elsevier; 2019.
Weichenthal S, Lavigne E, Villeneuve PJ, Reeves F. Airborne pollen concentrations and emergency room visits for myocardial infarction: a multicity case-crossover study in Ontario, Canada. Am J Epidemiol. 2016;183(7):613–21.
Peel JL, Tolbert PE, Klein M, Metzger KB, Flanders WD, Todd K, et al. Ambient Air Pollution and Respiratory Emergency Department Visits. Epidemiology. 2005;16(2):164–74.
Rajagopalan S, Al-Kindi SG, Brook RD. Air pollution and cardiovascular disease. J Am Coll Cardiol. 2018 Oct;72(17):2054–70.
Soneja S, Jiang C, Fisher J, Upperman CR, Mitchell C, Sapkota A. Exposure to extreme heat and precipitation events associated with increased risk of hospitalization for asthma in Maryland, USA. Environ Health. 2016 Dec;15(1):57.
Baldacci S, Maio S, Cerrai S, Sarno G, Baïz N, Simoni M, et al. Allergy and asthma: effects of the exposure to particulate matter and biological allergens. Respir Med. 2015 Sep;109(9):1089–104.
Sedghy F, Varasteh A-R, Sankian M, Moghadam M. Interaction between air pollutants and pollen grains: the role on the rising trend in allergy. Rep Biochem Mol Biol. 2018 Apr;6(2):219–24.
De Sario M, Katsouyanni K, Michelozzi P. Climate change, extreme weather events, air pollution and respiratory health in Europe. Eur Respir J. 2013 Sep;42(3):826–43.
Cayrol C, Duval A, Schmitt P, Roga S, Camus M, Stella A, et al. Environmental allergens induce allergic inflammation through proteolytic maturation of IL-33. Nat Immunol. 2018 Apr;19(4):375–85.
Anenberg SC, Weinberger KR, Henry R, Neumann JE, Crimmins A, Fann N, et al. Impacts of oak pollen on allergic asthma in the United States and potential influence of future climate change. GeoHealth. 2017;1(3):80–92.
Achakulwisut P, Mickley LJ, Anenberg SC. Drought-sensitivity of fine dust in the US southwest: implications for air quality and public health under future climate change. Environ Res Lett. 2018 May 1;13(5):054025.
Achakulwisut P, Anenberg SC, Neumann JE, Penn SL, Weiss N, Crimmins A, et al. Effects of increasing aridity on ambient dust and public health in the U.S. southwest under climate change. GeoHealth. 2019;3(5):127–44.
Fann N, Nolte CG, Dolwick P, Spero TL, Brown AC, Phillips S, et al. The geographic distribution and economic value of climate change-related ozone health impacts in the United States in 2030. J Air Waste Manage Assoc. 2015 May 4;65(5):570–80.
Ford B, Val Martin M, Zelasky SE, Fischer EV, Anenberg SC, Heald CL, et al. Future fire Impacts on smoke concentrations, visibility, and health in the contiguous United States. GeoHealth. 2018 Aug;2(8):229–47.
Li T, Horton RM, Kinney PL. Projections of seasonal patterns in temperature- related deaths for Manhattan, New York. Nature Clim Change. 2013;3(8):717–21.
Neumann JE, Anenberg SC, Weinberger KR, Amend M, Gulati S, Crimmins A, et al. Estimates of present and future asthma emergency department visits associated with exposure to oak, birch, and grass pollen in the United States. GeoHealth. 2019 Jan;3(1):11–27.
Martinich J, Crimmins A. Climate damages and adaptation potential across diverse sectors of the United States. Nat Clim Chang. 2019 May;9(5):397–404.
Woodruff TJ, Sutton P. The navigation guide work group. An evidence-based medicine methodology to bridge the gap between clinical and environmental health sciences. Health Aff. 2011 May;30(5):931–7.
Higgins JPT, Green S. Cochrane handbook for systematic reviews of interventions. [Internet]. 5.1.0. Hoboken, N.J.: Wiley; 2011 [cited 2020 Nov 17]. 672 p. Available from: http://public.ebookcentral.proquest.com/choice/publicfullrecord.aspx?p=4041340 .
Dijkers M. Introducing GRADE: a systematic approach to rating evidence in systematic reviews and to guideline development [Internet]. Austin, TX, USA: Center on Knowledge Translation for Disability and Rehabilitation Research; 2013 p. 9. Report No.: 5.1.0. Available from: https://ktdrr.org/products/update/v1n5/dijkers_grade_ktupdatev1n5.pdf .
Schünemann H, Brożek J, Guyatt G, Oxman A. GRADE Handbook [Internet]. 2013. Available from: https://gdt.gradepro.org/app/handbook/handbook.html .
Johnson PI, Sutton P, Atchley DS, Koustas E, Lam J, Sen S, et al. The navigation guide—evidence-based medicine meets environmental health: systematic review of human evidence for PFOA effects on fetal growth. Environ Health Perspect. 2014 Oct;122(10):1028–39.
Hebbern C, Cakmak S. Synoptic weather types and aeroallergens modify the effect of air pollution on hospitalisations for asthma hospitalisations in Canadian cities. Environ Pollut. 2015 Sep;204:9–16.
Makra L, Puskás J, Matyasovszky I, Csépe Z, Lelovics E, Bálint B, et al. Weather elements, chemical air pollutants and airborne pollen influencing asthma emergency room visits in Szeged, Hungary: performance of two objective weather classifications. Int J Biometeorol. 2015 Sep;59(9):1269–89.
Matyasovszky I, Makra L, Bálint B, Guba Z, Sümeghy Z. Multivariate analysis of respiratory problems and their connection with meteorological parameters and the main biological and chemical air pollutants. Atmos Environ. 2011 Aug;45(25):4152–9.
Mazenq J, Dubus J-C, Gaudart J, Charpin D, Nougairede A, Viudes G, et al. Air pollution and children’s asthma-related emergency hospital visits in southeastern France. Eur J Pediatr. 2017 Jun;176(6):705–11.
Mireku N, Wang Y, Ager J, Reddy RC, Baptist AP. Changes in weather and the effects on pediatric asthma exacerbations. Ann Allergy Asthma Immunol. 2009 Sep;103(3):220–4.
Witonsky J, Abraham R, Toh J, Desai T, Shum M, Rosenstreich D, et al. The association of environmental, meteorological, and pollen count variables with asthma-related emergency department visits and hospitalizations in the Bronx. J Asthma. 2019 Sep 2;56(9):927–37.
Analitis A, Michelozzi P, D’Ippoliti D. de’Donato F, Menne B, Matthies F, et al. effects of heat waves on mortality: effect modification and confounding by air pollutants. Epidemiology. 2014 Jan;25(1):15–22.
Analitis A, de’ Donato F, Scortichini M, Lanki T, Basagana X, Ballester F, et al. Synergistic Effects of Ambient Temperature and Air Pollution on Health in Europe: Results from the PHASE Project. IJERPH. 2018;15(9):1856.
Breitner S, Wolf K, Devlin RB, Diaz-Sanchez D, Peters A, Schneider A. Short-term effects of air temperature on mortality and effect modification by air pollution in three cities of Bavaria, Germany: a time-series analysis. Sci Total Environ. 2014 Jul;485–486:49–61.
Cheng Y, Kan H. Effect of the interaction between outdoor air pollution and extreme temperature on daily mortality in Shanghai. China Journal of Epidemiology. 2012;22(1):28–36.
Li G, Zhou M, Cai Y, Zhang Y, Pan X. Does temperature enhance acute mortality effects of ambient particle pollution in Tianjin City, China. Science of The Total Environment. 2011 Apr;409(10):1811–7.
Li L, Yang J, Guo C, Chen P-Y, Ou C-Q, Guo Y. Particulate matter modifies the magnitude and time course of the non-linear temperature-mortality association. Environ Pollut. 2015 Jan;196:423–30.
Lokys HL, Junk J, Krein A. Short-term effects of air quality and thermal stress on non-accidental morbidity—a multivariate meta-analysis comparing indices to single measures. Int J Biometeorol. 2018 Jan;62(1):17–27.
Burkart K, Canário P, Breitner S, Schneider A, Scherber K, Andrade H, et al. Interactive short-term effects of equivalent temperature and air pollution on human mortality in Berlin and Lisbon. Environ Pollut. 2013 Dec;183:54–63.
Chen K, Wolf K, Breitner S, Gasparrini A, Stafoggia M, Samoli E, et al. Two-way effect modifications of air pollution and air temperature on total natural and cardiovascular mortality in eight European urban areas. Environ Int. 2018 Jul;116:186–96.
Chen K, Wolf K, Hampel R, Stafoggia M, Breitner S, Cyrys J, et al. Does temperature-confounding control influence the modifying effect of air temperature in ozone–mortality associations?: Environmental Epidemiology. 2018 Mar;2(1):e008.
Dear K, Ranmuthugala G, Kjellström T, Skinner C, Hanigan I. Effects of temperature and ozone on daily mortality during the august 2003 heat wave in France. Archives of Environmental & Occupational Health. 2005 Jul;60(4):205–12.
Filleul L, Cassadou S, Médina S, Fabres P, Lefranc A, Eilstein D, et al. The relation between temperature, ozone, and mortality in nine French cities during the heat wave of 2003. Environ Health Perspect. 2006 Sep;114(9):1344–7.
Jhun I, Fann N, Zanobetti A, Hubbell B. Effect modification of ozone-related mortality risks by temperature in 97 US cities. Environ Int. 2014 Dec;73:128–34.
Kim SE, Lim Y-H, Kim H. Temperature modifies the association between particulate air pollution and mortality: a multi-city study in South Korea. Sci Total Environ. 2015 Aug;524–525:376–83.
Liu T, Zeng W, Lin H, Rutherford S, Xiao J, Li X, et al. Tempo-spatial variations of ambient ozone-mortality associations in the USA: results from the NMMAPS data. IJERPH. 2016 Aug 26;13(9):851.
Meng X, Zhang Y, Zhao Z, Duan X, Xu X, Kan H. Temperature modifies the acute effect of particulate air pollution on mortality in eight Chinese cities. Sci Total Environ. 2012 Oct;435–436:215–21.
Moolgavkar SH. Air pollution and daily mortality in two U.S. counties: season-specific analyses and exposure-response relationships. Inhal Toxicol. 2003 Jan;15(9):877–907.
Park AK, Hong YC, Kim H. Effect of changes in season and temperature on mortality associated with air pollution in Seoul, Korea. J Epidemiol Community Health. 2011 Apr 1;65(4):368–75.
Pattenden S, Armstrong B, Milojevic A, Heal MR, Chalabi Z, Doherty R, et al. Ozone, heat and mortality: acute effects in 15 British conurbations. Occup Environ Med. 2010 Oct 1;67(10):699–707.
Peng RD, Samoli E, Pham L, Dominici F, Touloumi G, Ramsay T, et al. Acute effects of ambient ozone on mortality in Europe and North America: results from the APHENA study. Air Qual Atmos Health. 2013 Jun 1;6(2):445–53.
Rainham DGC, Smoyer-Tomic KE, Sheridan SC, Burnett RT. Synoptic weather patterns and modification of the association between air pollution and human mortality. Int J Environ Health Res. 2005 Oct;15(5):347–60.
Scortichini M, De Sario M, de Donato F, Davoli M, Michelozzi P, Stafoggia M. Short-Term Effects of Heat on Mortality and Effect Modification by Air Pollution in 25 Italian Cities. IJERPH. 2018;15(8):1771.
Shaposhnikov D, Revich B, Bellander T, Bedada GB, Bottai M, Kharkova T, et al. Mortality Related to Air Pollution with the Moscow Heat Wave and Wildfire of 2010. Epidemiology. 2014;25(3):359–64.
Stafoggia M, Schwartz J, Forastiere F, Perucci CA. Does temperature modify the association between air pollution and mortality? A multicity case-crossover analysis in Italy. Am J Epidemiol. 2008 Jun 15;167(12):1476–85.
Sun S, Cao P, Chan K-P, Tsang H, Wong C-M, Thach T-Q. Temperature as a modifier of the effects of fine particulate matter on acute mortality in Hong Kong. Environ Pollut. 2015 Oct;205:357–64.
Vanos JK, Cakmak S, Kalkstein LS, Yagouti A. Association of weather and air pollution interactions on daily mortality in 12 Canadian cities. Air Qual Atmos Health. 2015 Jun;8(3):307–20.
Wilson A, Rappold AG, Neas LM, Reich BJ. Modeling the effect of temperature on ozone-related mortality. Ann Appl Stat. 2014 Sep;8(3):1728–49.
Zhang Y, Huang W, London SJ, Song G, Chen G, Jiang L, et al. Ozone and daily mortality in Shanghai, China. Environmental Health Perspectives. 2006 Aug;114(8):1227–32.
Ding P-H, Wang G-S, Guo Y-L, Chang S-C, Wan G-H. Urban air pollution and meteorological factors affect emergency department visits of elderly patients with chronic obstructive pulmonary disease in Taiwan. Environ Pollut. 2017 May;224:751–8.
Jo E-J, Lee W-S, Jo H-Y, Kim C-H, Eom J-S, Mok J-H, et al. Effects of particulate matter on respiratory disease and the impact of meteorological factors in Busan. Korea Respiratory Medicine. 2017 Mar;124:79–87.
Kunikullaya KU, Vijayaraghava A, Asha P, Kunnavil R, MuraliMohan BV. Meteorological parameters and pollutants on asthma exacerbation in Bangalore, India – an ecological retrospective time-series study. Journal of Basic and Clinical Physiology and Pharmacology [Internet]. 2017 Jan 1 [cited 2020 Nov 17];28(2). Available from: https://www.degruyter.com/doi/10.1515/jbcpp-2016-0074 .
Lam HC, Li AM, Chan EY, Goggins WB. The short-term association between asthma hospitalisations, ambient temperature, other meteorological factors and air pollutants in Hong Kong: a time-series study. Thorax. 2016 Dec;71(12):1097–109.
Mirabelli MC, Vaidyanathan A, Flanders WD, Qin X, Outdoor PM GP. Ambient Air Temperature, and Asthma Symptoms in the Past 14 Days among Adults with Active Asthma. Environ Health Perspect. 2016 Dec;124(12):1882–90.
Qiu H, Tan K, Long F, Wang L, Yu H, Deng R, et al. The burden of COPD morbidity attributable to the interaction between ambient air pollution and temperature in Chengdu, China. IJERPH. 2018 Mar 11;15(3):492.
Winquist A, Kirrane E, Klein M, Strickland M, Darrow LA, Sarnat SE, et al. Joint Effects of Ambient Air Pollutants on Pediatric Asthma Emergency Department Visits in Atlanta, 1998–2004. Epidemiology. 2014;25(5):666–73.
Lee H, Myung W, Cheong H-K, Yi S-M, Hong Y-C, Cho S-I, et al. Ambient air pollution exposure and risk of migraine: synergistic effect with high temperature. Environ Int. 2018 Dec;121:383–91.
Luo K, Li R, Wang Z, Zhang R, Xu Q. Effect modification of the association between temperature variability and daily cardiovascular mortality by air pollutants in three Chinese cities. Environ Pollut. 2017 Nov;230:989–99.
Ren C, Williams GM, Morawska L, Mengersen K, Tong S. Ozone modifies associations between temperature and cardiovascular mortality: analysis of the NMMAPS data. Occup Environ Med. 2008 Apr 1;65(4):255–60.
Ren C, Williams GM, Mengersen K, Morawska L, Tong S. Temperature enhanced effects of ozone on cardiovascular mortality in 95 large US communities, 1987–2000: assessment using the NMMAPS data. Archives of Environmental & Occupational Health. 2009 Oct 19;64(3):177–84.
Anderson HR, de Leon AP, Bland JM, Bower JS, Emberlin J, Strachan DP. Air pollution, pollens, and daily admissions for asthma in London 1987-92. Thorax. 1998 Oct 1;53(10):842–8.
Cakmak S, Dales RE, Coates F. Does air pollution increase the effect of aeroallergens on hospitalization for asthma? J Allergy Clin Immunol. 2012 Jan;129(1):228–31.
Chen K, Glonek G, Hansen A, Williams S, Tuke J, Salter A, et al. The effects of air pollution on asthma hospital admissions in Adelaide, South Australia, 2003-2013: time-series and case-crossover analyses. Clin Exp Allergy. 2016 Nov;46(11):1416–30.
Cirera L, García-Marcos L, Giménez J, Moreno-Grau S, Tobías A, Pérez-Fernández V, et al. Daily effects of air pollutants and pollen types on asthma and COPD hospital emergency visits in the industrial and Mediterranean Spanish city of Cartagena. Allergol Immunopathol. 2012 Jul;40(4):231–7.
Galán I, Tobías A, Banegas JR, Aránguez E. Short-term effects of air pollution on daily asthma emergency room admissions. Eur Respir J. 2003 Nov;22(5):802–8.
Gleason JA, Bielory L, Fagliano JA. Associations between ozone, PM2.5, and four pollen types on emergency department pediatric asthma events during the warm season in New Jersey: a case-crossover study. Environ Res. 2014 Jul;132:421–9.
Goodman JE, Loftus CT, Liu X, Zu K. Impact of respiratory infections, outdoor pollen, and socioeconomic status on associations between air pollutants and pediatric asthma hospital admissions. Larcombe A, editor. PLoS ONE. 2017;12(7):e0180522.
Krmpotic D, Luzar-Stiffler V, Rakusic N, Stipic Markovic A, Hrga I, Pavlovic M. Effects of traffic air pollution and hornbeam pollen on adult asthma hospitalizations in Zagreb. Int Arch Allergy Immunol. 2011;156(1):62–8.
Ross MA, Persky VW, Scheff PA, Chung J, Curtis L, Ramakrishnan V, et al. Effect of ozone and aeroallergens on the respiratory health of asthmatics. Archives of Environmental Health: An International Journal. 2002 Nov;57(6):568–78.
Stieb DM, Beveridge RC, Brook JR, Smith-Doiron M, Burnett RT, Dales RE, et al. Air pollution, aeroallergens and cardiorespiratory emergency department visits in Saint John, Canada. J Expo Sci Environ Epidemiol. 2000 Oct;10(5):461–77.
Silverberg JI, Braunstein M, Lee-Wong M. Association between climate factors, pollen counts, and childhood hay fever prevalence in the United States. Journal of Allergy and Clinical Immunology. 2015;135(2):463–9 e5.
Adelborg K, Szépligeti SK, Holland-Bill L, Ehrenstein V, Horváth-Puhó E, Henderson VW, et al. Migraine and risk of cardiovascular diseases: Danish population based matched cohort study. BMJ. 2018 Jan;31:k96.
Jerrett M, Turner MC, Beckerman BS, Pope CA, van Donkelaar A, Martin RV, et al. Comparing the health effects of ambient particulate matter estimated using ground-based versus remote sensing exposure estimates. Environ Health Perspect. 2017 Apr;125(4):552–9.
Vodonos A, Awad YA, Schwartz J. The concentration-response between long-term PM2.5 exposure and mortality; a meta-regression approach. Environ Res. 2018 Oct;166:677–89.
Di Q, Wang Y, Zanobetti A, Wang Y, Koutrakis P, Choirat C, et al. Air pollution and mortality in the Medicare population. N Engl J Med. 2017 Jun 29;376(26):2513–22.
Download references
Acknowledgements
We acknowledge helpful insights from Henry Roman and Jason Sacks and research assistance from Nick Pendleton.
This research was partly funded by the U.S. Environmental Protection Agency under contract EP-D − 14-031 via a subcontract from Industrial Economics, Inc and by a grant from the Wellcome Trust (No. 216075/Z/19/Z). The U.S. EPA did not have a substantive role in any aspect of this paper, including in conception, analysis, or drafting. This paper has not been reviewed by the U.S. EPA and does not necessarily reflect the views of the agency.
Author information
Authors and affiliations.
Milken Institute School of Public Health, George Washington University, 950 New Hampshire Ave NW, Washington, DC, 20052, USA
Susan C. Anenberg, Shannon Haines & Elizabeth Wang
Now at: American Lung Association, Springfield, IL, USA
Shannon Haines
Department of Pulmonary, Critical Care, and Sleep Medicine, Brown University Alpert Medical School, Providence, RI, 02903, USA
Nicholas Nassikas
School of Public Health, Boston University, Boston, MA, USA
Patrick L. Kinney
You can also search for this author in PubMed Google Scholar
Contributions
S.C.A. conceived of the study, oversaw the analysis, and was responsible for drafting the manuscript. S.H. conducted the literature review, evaluated risk of bias and strength and quality of the evidence, and wrote much of the manuscript. E.W. evaluated risk of bias. N.N. and P.K. reviewed the analysis and contributed to the manuscript writing. The author(s) read and approved the final manuscript.
Corresponding author
Correspondence to Susan C. Anenberg .
Ethics declarations
Ethics approval and consent to participate.
Not applicable.
Consent for publication
Competing interests.
The authors declare that they have no competing interests.
Additional information
Publisher’s note.
Springer Nature remains neutral with regard to jurisdictional claims in published maps and institutional affiliations.
Supplementary Information
Additional file 1., rights and permissions.
Open Access This article is licensed under a Creative Commons Attribution 4.0 International License, which permits use, sharing, adaptation, distribution and reproduction in any medium or format, as long as you give appropriate credit to the original author(s) and the source, provide a link to the Creative Commons licence, and indicate if changes were made. The images or other third party material in this article are included in the article's Creative Commons licence, unless indicated otherwise in a credit line to the material. If material is not included in the article's Creative Commons licence and your intended use is not permitted by statutory regulation or exceeds the permitted use, you will need to obtain permission directly from the copyright holder. To view a copy of this licence, visit http://creativecommons.org/licenses/by/4.0/ . The Creative Commons Public Domain Dedication waiver ( http://creativecommons.org/publicdomain/zero/1.0/ ) applies to the data made available in this article, unless otherwise stated in a credit line to the data.
Reprints and permissions
About this article
Cite this article.
Anenberg, S.C., Haines, S., Wang, E. et al. Synergistic health effects of air pollution, temperature, and pollen exposure: a systematic review of epidemiological evidence. Environ Health 19 , 130 (2020). https://doi.org/10.1186/s12940-020-00681-z
Download citation
Received : 25 August 2020
Accepted : 30 November 2020
Published : 07 December 2020
DOI : https://doi.org/10.1186/s12940-020-00681-z
Share this article
Anyone you share the following link with will be able to read this content:
Sorry, a shareable link is not currently available for this article.
Provided by the Springer Nature SharedIt content-sharing initiative
- Air pollution
- Temperature
- Systematic review
Environmental Health
ISSN: 1476-069X
- General enquiries: [email protected]
Thank you for visiting nature.com. You are using a browser version with limited support for CSS. To obtain the best experience, we recommend you use a more up to date browser (or turn off compatibility mode in Internet Explorer). In the meantime, to ensure continued support, we are displaying the site without styles and JavaScript.
- View all journals
- My Account Login
- Explore content
- About the journal
- Publish with us
- Sign up for alerts
- Open access
- Published: 04 July 2022
A systematic review and meta-analysis on the association between ambient air pollution and pulmonary tuberculosis
- Christian Akem Dimala 1 , 2 &
- Benjamin Momo Kadia 3 , 4
Scientific Reports volume 12 , Article number: 11282 ( 2022 ) Cite this article
2967 Accesses
12 Citations
5 Altmetric
Metrics details
- Environmental sciences
- Health care
- Medical research
- Risk factors
There is inconclusive evidence on the association between ambient air pollution and pulmonary tuberculosis (PTB) incidence, tuberculosis-related hospital admission and mortality. This review aimed to assess the extent to which selected air pollutants are associated to PTB incidence, hospital admissions and mortality. This was a systematic review of studies published in English from January 1st, 1946, through May 31st, 2022, that quantitatively assessed the association between PM 2.5 , PM 10 , NO 2 , SO 2 , CO, O 3 and the incidence of, hospital admission or death from PTB. Medline, Embase, Scopus and The Cochrane Library were searched. Extracted data from eligible studies were analysed using STATA software. Random-effect meta-analysis was used to derive pooled adjusted risk and odds ratios. A total of 24 studies (10 time-series, 5 ecologic, 5 cohort, 2 case–control, 1 case cross-over, 1 cross-sectional) mainly from Asian countries were eligible and involved a total of 437,255 tuberculosis cases. For every 10 μg/m 3 increment in air pollutant concentration, there was a significant association between exposure to PM 2.5 (pooled aRR = 1.12, 95% CI: 1.06–1.19, p < 0.001, N = 6); PM 10 (pooled aRR = 1.06, 95% CI: 1.01–1.12, p = 0.022, N = 8); SO 2 (pooled aRR = 1.08, 95% CI: 1.04–1.12, p < 0.001, N = 9); and the incidence of PTB. There was no association between exposure to CO (pooled aRR = 1.04, 95% CI: 0.98–1.11, p = 0.211, N = 4); NO 2 (pooled aRR = 1.08, 95% CI: 0.99–1.17, p = 0.057, N = 7); O 3 (pooled aRR = 1.00, 95% CI: 0.99–1.02, p = 0.910, N = 6) and the incidence of PTB. There was no association between the investigated air pollutants and mortality or hospital admissions due to PTB. Overall quality of evidence was graded as low (GRADE approach). Exposure to PM 2.5 , PM 10 and SO 2 air pollutants was found to be associated with an increased incidence of PTB, while exposure to CO, NO 2 and O 3 was not. There was no observed association between exposure to these air pollutants and hospital admission or mortality due to PTB. The quality of the evidence generated, however, remains low. Addressing the tuberculosis epidemic by 2030 as per the 4th Sustainable Development Goal may require a more rigorous exploration of this association.
Similar content being viewed by others
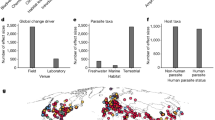
A meta-analysis on global change drivers and the risk of infectious disease
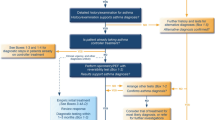
Key recommendations for primary care from the 2022 Global Initiative for Asthma (GINA) update
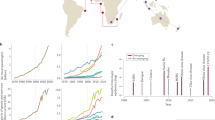
Infectious disease in an era of global change
Introduction.
Pulmonary tuberculosis (PTB), a bacterial infection of the lungs caused by Mycobacterium tuberculosis is one of the top 10 causes of death worldwide and the leading cause of death from a single infectious agent 1 . PTB remains a global health emergency despite the significant progress that has been made worldwide in its control over the past two and a half decades 2 . Much still needs to be done to end the tuberculosis epidemic by 2030 3 as per the World Health Organisation’s (WHO) 4th sustainable development goal (SDG). This includes addressing important predisposing factors to tuberculosis infection such as smoking, diabetes, human immunodeficiency virus (HIV) and social determinants of health such as poverty, malnutrition, poor ventilation and over-crowding among others 1 , 4 . A multi-faceted and multi-sectorial approach to tuberculosis prevention, case identification, management and control of its health and social determinants is therefore required 4 , 5 .
Air pollution, currently on several global health agendas, has rapidly become a global problem with the increasing global urbanisation, transportation-related emissions, and increased energy consumption. Air pollution could therefore be an important factor to address on the journey to ending tuberculosis as there are growing concerns of its association to increased tuberculosis-related hospital admissions and deaths 6 , 7 .
There is a well-known association between different air pollutants and cardio-respiratory diseases 8 , 9 , 10 , 11 , 12 , 13 , 14 , 15 . However, there is still no conclusive evidence of an association between PTB and outdoor air pollution despite its well-known association to indoor pollution from activities such as smoking and biomass fuel burning 16 , 17 , 18 The review by Popovic et al. indicated a possible association between PM 2.5 and PTB outcomes (incidence, hospital admissions and mortality) and reported the contrasting findings from several earlier studies on the association between PM 10 , NO 2 , and SO 2 and PTB 19 , but did not synthesise these findings to determine to what extent these air pollutants are associated to PTB. Also, several studies have been published on this subject after the review by Popovic et al. This review therefore had as objectives to determine if there is an association between the selected air pollutants (PM 2.5 , PM 10 , NO 2 , SO 2 , CO, O 3 ) and PTB incidence, hospital admissions and mortality, and to what extent, by systematically reviewing and quantitatively combining published evidence on this topic.
This was a systematic literature review and meta-analysis of articles published in English from January 1st, 1946, through May 31st, 2022, that quantitatively assessed the association between ambient air pollution and PTB. The study protocol for this review was registered with the international prospective register of systematic reviews (PROSPERO) with trial registration number CRD42020165888 and has been published 20 . This review was reported according to The RepOrting standards for Systematic Evidence Syntheses (ROSES) for systematic review 21 as presented in Additional file 1 .
Deviations from the protocol
There were no deviations from the published study protocol.
Search for articles
A comprehensive search strategy (Additional file 2 ) combining medical subject headings (MeSH) and free-text searches for the appropriate keywords was developed by the authors and used to search the databases: Medline, Embase, Scopus and The Cochrane Library. The keywords ‘air pollution’, ‘carbon monoxide’, ‘nitrogen dioxide’, ‘sulphur dioxide’, ‘ozone’, and ‘particulate matter’ were combined with the keywords ‘tuberculosis’, ‘incidence’, ‘mortality’, ‘hospital admission’ and their respective synonyms, using the Boolean operator ‘AND’ in the search strategy. The search was run by the principal investigator (CAD), all searches were limited to the language English and grey literature search was not conducted given the lack of relevant studies from preliminary searches of the grey literature. Search dates of interest were January 1st, 1946, through May 31st, 2022. The search language was in English, and all the database searches were done on the same day, June 5th, 2022. The search was run twice to ensure replicability of results and the same results were obtained with each search run.
Article screening and study eligibility criteria
Screening process.
Articles returned by the search were saved on Zotero Version 5.0 reference management software and duplicates of the studies were manually removed by the principal investigator (CAD) with the assistance of the reference management software. More articles were added to the search output by the principal investigator by reviewing the reference list of relevant articles. The titles and abstracts of all the remaining articles were then independently screened for eligibility according to the set eligibility criteria by each of the two independent reviewers (CAD and BMK). The full texts of all the articles retained after the title and abstract screen, were then independently reviewed by the same two independent reviewers (CAD and BMK) for eligibility and inclusion to the analysis. The two independent reviewers compared their findings at the end of both the title and abstract screening and the full text review stages of the article selection process to ensure concordance in their final selection. There were no reviewer disagreements at all stages of the study selection process and no third reviewer to settle discordances as had been planned in the study protocol, was therefore needed due to concordance in the findings of the two independent reviewers.
Eligibility criteria
The following criteria were used during the article selection process to determine the eligible studies.
The following studies were included:
Population: Studies focused on adults aged 18 and above with PTB
Exposure: Studies that reported direct measurements on any of the air pollutants; carbon monoxide (CO), nitrogen dioxide (NO 2 ), sulphur dioxide (SO 2 ), ozone (O 3 ), particulate matter ≤ 2.5 µm (PM 2.5 ) and/or particulate matter ≤ 10 µm (PM 10 ) in any country, region, city or locality;
Outcomes: Studies that reported measures of association on the risk of PTB incidence, hospital admission and/or mortality from PTB;
Study design/Other: Cross-sectional, case–control, cohorts, case-crossover, ecological and time-series studies that reported on the association between ambient air pollution and PTB.
The following studies were excluded:
Population: Studies that reported on respiratory diseases other than PTB
Exposure: Studies that reported on other forms of air pollution such as indoor air pollution
Outcomes: Studies that reported outcomes related to PTB in combination with other respiratory diseases. Studies that reported on measures of effect/association other than risk ratios and odds ratios or that provided data from which these measures could not be calculated.
Other: Conference abstracts, editorials, letters, opinion papers, unpublished studies, same studies published in different journals with the same or a different title.
Study validity assessment
Assessment of study quality of each included study was done by both independent reviewers (CAD and BMK) using the respective Study Quality Assessment Tools of the National Health Institute/National Heart, Lung and Blood Institute (NHI/NHLBI) 22 depending on their study designs. There was no discordance in the overall rating of the quality of the eligible studies. Study quality indicators were included in the meta-regression.
The overall quality of the evidence provided by the studies with regards to the primary outcome of interest was assessed and graded as very low, low, moderate or high, using the Grading of Recommendations Assessment, Development and Evaluation (GRADE) 23 .
Data coding and extraction strategy
Data on the publication details, study methods and outcomes of interest were extracted from the eligible studies into a Microsoft excel office 365 data extraction sheet (Additional file 3 ) by the principal investigator (CAD) and independently rechecked by a second reviewer (BMK) for accuracy. The following data were extracted: First author, year of publication, study location, study design, socio-demographic and clinical characteristics of study participants, study duration, number of tuberculosis cases and new tuberculosis cases, annual incidences of tuberculosis, mean and median concentration data on air pollutants of interest (CO, NO 2 , SO 2 , O 3 , PM 2.5 and PM 10 ), data on incidence, hospital admission and mortality from tuberculosis, including measures of effect/association (risk ratios, odds ratios and percentage change in the incidence of PTB) and their respective confidence intervals, and confounders reported by the respective studies and if studies adjusted for confounders or not. PM 2.5 and PM 10 air pollutants were measured in µg/m 3 and NO 2 , SO 2 and O 3 in parts per billion (ppb) and CO in parts per million (ppm). For studies that reported air pollutant concentrations in units other than the above, the Air Pollution Information System 24 , was used to convert air pollutant concentrations to appropriate units, taking into consideration the average yearly temperatures reported for the various cities or countries. The average annual outdoor temperature obtained from public sources was used for studies that did not report them. In studies where several measures of effect were reported for different quintiles or levels of exposure to air pollutants, the largest numerical estimates of the measures of effect were considered, to quantify the maximum extent of the association of air pollutants to PTB. When protective effects were observed among the measures of effect, the lowest numerical measures of effect were used. Default measures of effect reported by the studies were considered. Adjusted measures of effect were chosen over crude measures, and both single-pollutant models and multi-pollutant models were reported as appropriate. All data was transferred to STATA version 14.0 statistical software for analysis.
Potential effect modifiers/reasons for heterogeneity
Between-study heterogeneity was anticipated given the differences in study designs, settings, duration, sample sizes, and population characteristics based on review of existing literature.
Data synthesis and presentation
Meta-analyses were done through random effects models to account for the possibility of between-study heterogeneity. Risk ratios and odds ratios on the incidence of PTB following exposure to the selected air pollutants, and their respective confidence intervals from the various studies, were log-transformed, and the corresponding standard errors derived. Pooled summary estimates for the respective log-transformed measures of association were computed and presented on forest plots. Studies were pooled according to their study designs with ecologic studies and studies that used time-series analysis pooled together, separate from cohort and case–control studies. Heterogeneity between studies was assessed using the Cochrane’s Q test, and the I 2 test statistic was reported as a measure of the extent of this heterogeneity. The Begg’s and Egger’s statistical tests were used for the statistical assessments of publication bias and small study effect 25 , 26 . All statistical tests and plots were done on STATA version 14.0 statistical software.
Ethics approval and consent to participate
This systematic review does not require ethical approval as it entails a synthesis of data collected from several primary studies. No primary data collection from patients will be done for this systematic review.
Review descriptive statistics
Figure 1 summarises the study selection process.
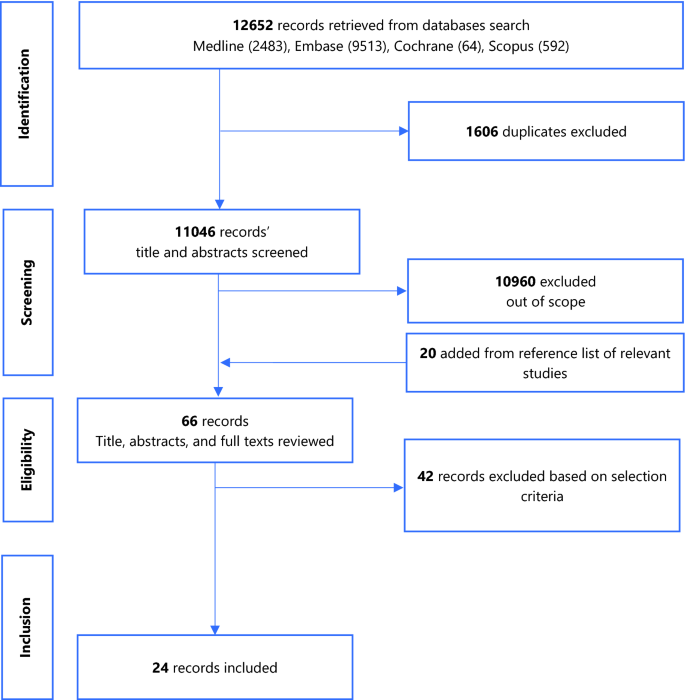
PRISMA flow chart.
A total of 12,652 records were returned by the search. Following removal of duplicates, screening of titles and abstracts, addition of studies from the reference list of relevant studies, full-text reviews, 24 eligible studies were retained. Figure 1 summarises the PRISMA flow chart of the study selection process. The studies excluded following full-text review and the reasons for exclusion are presented in Additional file 4 .
Narrative synthesis including study validity assessment
Most studies were from Asian countries and a total of 437,255 tuberculosis cases were reported across the 22 studies that reported the number of tuberculosis cases over their study periods (1996–2019) 7 , 27 , 28 , 29 , 30 , 31 , 32 , 33 , 34 , 35 , 36 , 37 , 38 , 39 , 40 , 41 , 42 , 43 , 44 , 45 , 46 . Of the 24 studies included in the review, 10 were time series, 5 were cohort studies (3 retrospective, 2 prospective), 5 were ecologic, 2 were case–control studies (1 nested, 1 retrospective), 1 was a retrospective case cross-over and 1 was cross-sectional. Average male participation was at 64.9% (N = 13 studies) 7 , 27 , 29 , 30 , 31 , 32 , 33 , 34 , 35 , 37 , 40 , 43 , 46 , mean age of 46.3 years (N = 7 studies) 27 , 30 , 31 , 32 , 37 , 40 , 43 and average annual tuberculosis incidence was 45.3 per 100,000 population (N = 10 studies) 7 , 27 , 29 , 32 , 35 , 36 , 45 , 46 , 47 , 48 . Study and participant characteristics are summarised on Table 1 .
The average of the annual mean concentrations of the various air pollutants are presented on Table 2 .
Twelve studies were of good quality, eleven of fair quality and one of poor quality (Additional file 6 ). The overall quality of evidence for the association of all 6 air pollutants to the incidence of PTB was graded as low based on the study limitations affecting generalisability of the findings, and some inconsistency across the studies due to the significantly elevated between-study heterogeneity (Additional file 7 ).
Data synthesis
Association between air pollutants and pulmonary tuberculosis incidence.
There was a significant association between exposure to PM 2.5 and incidence of pulmonary tuberculosis (PTB), pooled adjusted RR = 1.12 (95% CI: 1.06–1.19), p < 0.001, N = 6, I 2 = 72.4% 7 , 29 , 38 , 39 , 43 , 49 . There was no evidence of publication bias (Begg’s test, p = 0.133 and Egger’s test, p = 0.203). Begg’s test, p = 1. Likewise, Xiong et al. 46 reported an association (RR = 3.10, 95% CI: 1.10–8.79) for a 50 µg/m 3 increase in PM 2.5 concentration. The study by Lai et al. 32 (RR = 1.39, 95% CI: 0.95–2.03) which was cohort in design did not find a significant association. Jassal et al. 28 reported an odds ratio of 25.3 (95% CI: 3.38–29.1).
There was a significant association between exposure to PM 10 and incidence of PTB, pooled adjusted RR = 1.06 (95% CI: 1.01–1.12), p = 0.022, N = 8, I 2 = 97.6% (Begg’s test, p = 0.536 and Egger’s test, p = 0.204) 7 , 29 , 35 , 39 , 40 , 43 , 44 , 49 . The studies by Lai et al. 32 (HR = 0.95, 95% CI: 0.78–1.17) and Hwang et al. 27 (male RR = 1.00, 95% CI: 0.96–1.05 and female RR = 1.01, 95% CI: 0.98–1.05) did not find a significant association. Likewise, the pooled adjusted OR was 1.03 (95% CI: 1.01–1.04), p = 0.001, N = 3, I 2 = 0% (Begg’s test, p = 1 and Egger’s test, p = 0.211) (Fig. 2 ) 31 , 34 , 37 .
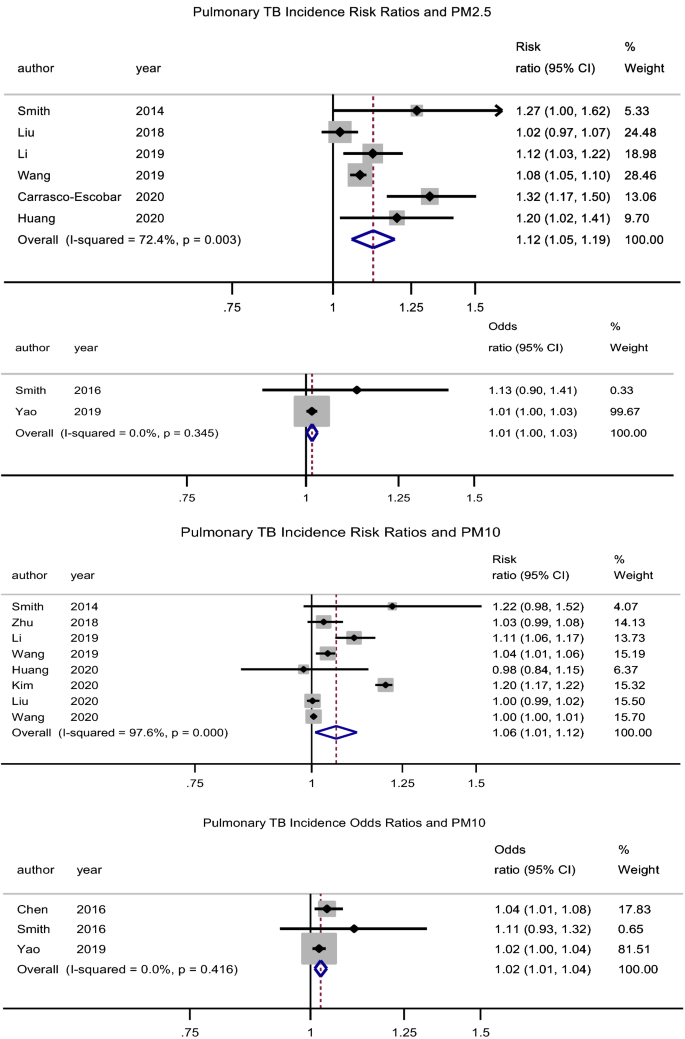
Forest plot showing the individual and pooled risk ratios and odds ratios for pulmonary tuberculosis incidence for PM 2.5 and PM 10 . The dashed line on the Forest plot represents the overall pooled estimate. The grey squares and horizontal lines represent the vaccine acceptance rate of each study and their 95% confidence intervals. The size of the grey square represents the weight contributed by each study in the meta-analysis. The diamond represents the pooled vaccine acceptance rate and its 95% confidence intervals.
There was no significant association between exposure to CO and the incidence of PTB, pooled adjusted RR = 1.04 (95% CI: 0.98–1.11), p = 0.211, N = 4, I 2 = 87.4% (Begg’s test, p = 0.734 and Egger’s test, p = 0.355) 39 , 43 , 47 , 49 . The studies by Lai et al. 32 (HR = 1.89, 95% CI: 0.78–4.58) and Hwang et al. 27 (male RR = 0.99, 95% CI: 0.95–1.03 and female RR = 1.01, 95% CI: 0.98–1.04) had similar findings. The pooled adjusted OR was 1.22 (95% CI: 0.84–1.76), p = 0.305, N = 3, I 2 = 78.5% (Begg’s test, p = 1 and Egger’s test, p = 0.364) (Fig. 3 ) 31 , 34 , 37 . However, Xiong et al. 46 (RR = 1.436, 95% CI: 1.004–2.053) reported a significant association for a 100 µg/m 3 increase in CO concentration.
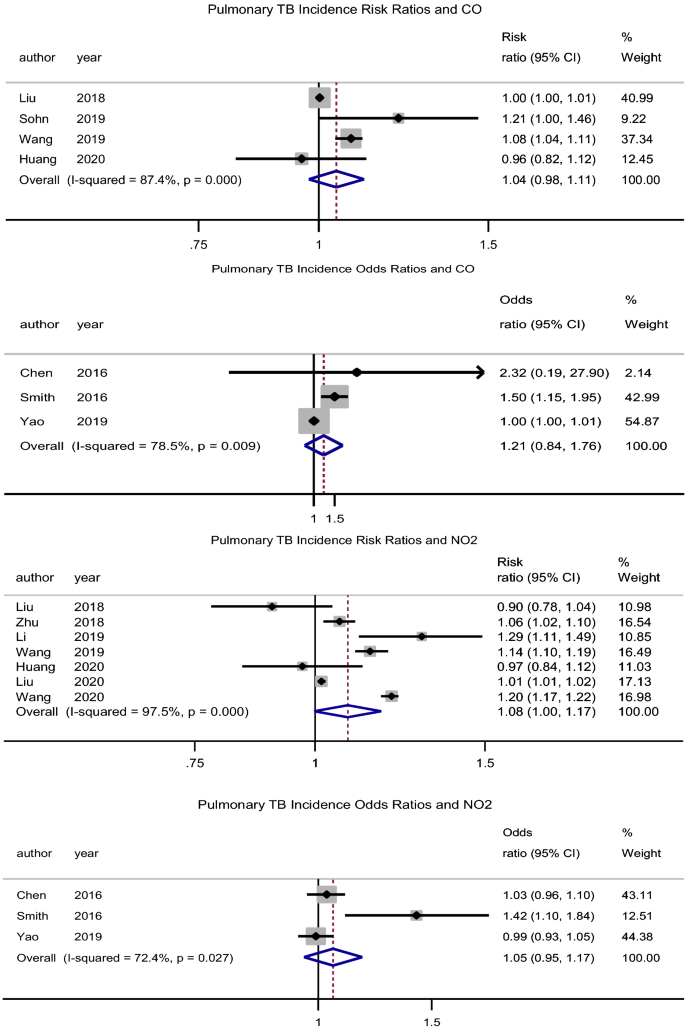
Forest plot showing the individual and pooled risk ratios and odds ratios for pulmonary tuberculosis incidence for CO and NO 2 . The dashed line on the Forest plot represents the overall pooled estimate. The grey squares and horizontal lines represent the odds ratios of each study and their 95% confidence intervals. The size of the grey square represents the weight contributed by each study in the meta-analysis. The diamond represents the pooled odds ratio and its 95% confidence intervals.
There was no association between exposure to NO 2 and the incidence of PTB, pooled adjusted RR = 1.08 (95% CI: 0.99–1.17), p = 0.057, N = 7, I 2 = 98% (Begg’s test, p = 1 and Egger’s test, p = 0.437) (Fig. 3 ) 7 , 35 , 39 , 40 , 43 , 48 , 49 . Lai et al. 32 (HR = 1.33, 95% CI: 1.04–1.70) found a significant association, while Hwang et al. 27 (male RR = 1.00, 95% CI: 0.96–1.05 and female RR = 1.01, 95% CI: 0.98–1.05) did not. Likewise, the pooled adjusted OR was 1.05 (95% CI: 0.95–1.17), p = 0.322, N = 3, I 2 = 72.4% (Begg’s test, p = 0.296 and Egger’s test, p = 0.145) (Fig. 3 ) 31 , 34 , 37 . However, Xiong et al. 46 (RR = 1.8, 95% CI: 1.11–2.91) reported a significant association for a 5 µg/m 3 increase in NO 2 concentration.
There was an association between exposure to SO 2 and incidence of PTB, pooled adjusted RR = 1.08 (95% CI: 1.04–1.12), p < 0.001, N = 9, I 2 = 94.4% (Begg’s test, p = 0.517 and Egger’s test, p = 0.356) (Fig. 4 ) 7 , 35 , 39 , 40 , 43 , 44 , 47 , 48 , 49 . Hwang et al. 27 (male RR = 1.07, 95% CI: 1.03–1.12 and female RR = 1.02, 95% CI: 0.98–1.07) reported similar findings in males. Likewise, Xiong et al. 46 reported an association (RR = 1.62, 95% CI: 1.12–2.33) for a 5 µg/m 3 increase in SO 2 concentration.
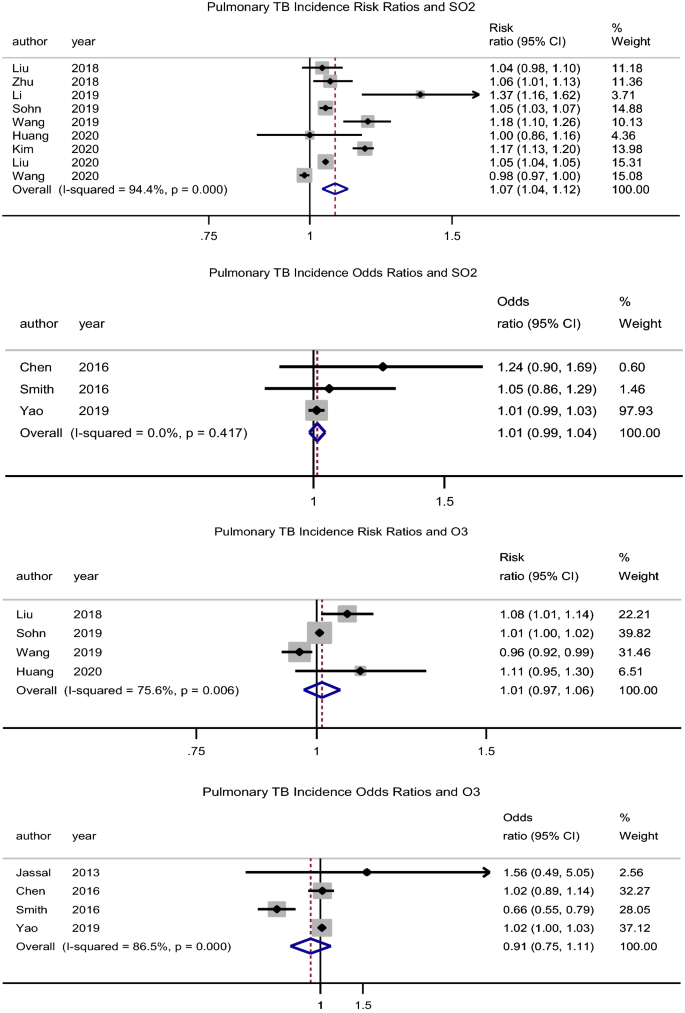
Forest plot showing the individual and pooled risk ratios and odds ratios for pulmonary tuberculosis incidence for SO 2 and O 3 . The dashed line on the Forest plot represents the overall pooled estimate. The grey squares and horizontal lines represent the odds ratios of each study and their 95% confidence intervals. The size of the grey square represents the weight contributed by each study in the meta-analysis. The diamond represents the pooled odds ratio and its 95% confidence intervals.
There was no significant association between O 3 exposure and incidence of PTB, pooled adjusted RR = 1.01 (95% CI: 0.97–1.06), p = 0.560, N = 4, I 2 = 75.6% (Begg’s test, p = 0.734 and Egger’s test, p = 0.734) (Fig. 4 ) 39 , 43 , 47 , 49 . While Hwang et al. 27 had similar findings (male RR = 0.99, 95% CI: 0.94–1.03 and female RR = 1.01, 95% CI: 0.97–1.05), Lai et al. 32 rather found a protective effect (HR = 0.69, 95% CI: 0.49–0.98). Xiong et al. 46 reported an association (RR = 0.96, 95% CI: 0.93–1.0) for a 5 µg/m 3 increase in O 3 concentration.
Table 3 summarises the percentage change in the number of PTB cases for the respective changes in air pollutant concentrations.
Association between air pollutants and hospital admissions and mortality due to pulmonary tuberculosis
Two studies reported a significant association between PM 2.5 and PTB mortality; OR = 1.46 (95% CI: 1.15–1.85) 33 and percentage change in cases of 0.08% (95% CI: 0.06–0.09) 45 . There was no significant association between CO, SO 2 , and O 3 and PTB mortality 47 (Table 4 ). Likewise, there was no significant association between PM 10 , CO, SO 2 , O 3 and hospital admission 30 , 47 . NO 2 was associated with hospital admission due to PTB, OR: 1.21 (95% CI: 1.10–1.33) (Table 4 ).
Subgroup analysis and meta-regression
Studies were categorised according to their duration (less than 5 years and 5 years or more), location (Asia and others), number of PTB cases (less than 5000 and 5000 or more) and study quality (good and fair/poor). None of these study characteristics could explain the observed heterogeneity across studies, except for study location with regards to exposure to PM 2.5 air pollutant. There was a higher risk of PTB incidence with PM 2.5 exposure in studies conducted out of Asia (Additional file 5 ).
Exposure to PM 2.5 , PM 10 and SO 2 air pollutants was found to be associated with an increased incidence of PTB, while exposure to CO, NO 2 and O 3 was not. There was no observed association between exposure to these air pollutants and hospital admission or mortality due to PTB. The findings of this review are particularly relevant given the increasing global concentrations and exposure to some air pollutants such as SO 2 and PM 2.5 over the past decades 50 , 51 . Public health strategies aimed at ending the tuberculosis epidemic would therefore have to work alongside interventions aimed at improving overall air quality and addressing air pollution 51 .
Air pollutants including O 3 and NO 2 mainly originate from volatile organic compounds, combustion processes including heating, power generation, the engines of vehicles and ships and also from industry emissions 52 . SO 2 originates from the burning of fossil fuels for power generation and the smelting of sulfur-containing mineral ores 52 . PM 2.5 and PM 10 which consist of particles of organic and inorganic substances are typically suspended in the air 52 . Air pollutants have been previously associated with the development of cardio-respiratory diseases in both children and adults 8 , 9 , 11 . Traffic-related pollution and several air pollutants such as O 3 , NO 2 , PM 2.5 and PM 10 , have not only been associated with exacerbations of asthma and chronic obstructive pulmonary disease, but have also been implicated in the development of these conditions especially in childhood 11 , 53 , 54 . Air pollutants are known to increase the risk of infection when inhaled as they dampen the natural defence barriers of the respiratory tract, inhibit muco-ciliary clearance, inhibit macrophages and initiate a chronic inflammatory response with the release of pro-inflammatory mediators 55 , 56 . In a similar way, exposure to particulate matter for example has immunomodulatory effects on antimycobacterial activity through impaired expression of important cytokines and chemokines which are important in controlling mycobacterial infections 57 , 58 . This reduced antimycobacterial host immune response predisposes to tuberculosis infection.
Measures and policies in various sectors such as the transport, housing and industry sectors are known to reduce air pollutions, including; prioritising walking and cycling in cities, using low-emission vehicles; using clean technologies that reduce industrial emissions; improving access to clean household energy for heating, lighting and cooking; making cities more green; using low-emission fuels and combustion-free power sources, among others 52 . In 2015, the WHO member states adopted a resolution for enhanced global response to the adverse health effects of air pollution, and the WHO has been overseeing this response through; the production of air quality guidelines and exposure limits to these air pollutants 52 .
Even though the studies by Ge et al. 59 and Xu et al. 60 reported a possible association between short-term exposure to SO 2 , our review did not assess outpatient PTB visits as an outcome. This is therefore a subject amenable to further exploration.
The studies in this review were conducted over a 24-year period and we did not observe a particular change or variation in the trend of the reported associations between exposure to the air pollutants and PTB incidence over time across the older and newer studies. Close to four fifth of the studies in our review were conducted in Asia and up to half of the studies were in China, which could affect the generalisability of the findings of this review, however, China is still a high-burden country for tuberculosis 61 , 62 . The observed between-study heterogeneity highlights the need for more uniform study designs and methods in future studies aiming to assess this association.
Interpretation of the findings from this review should take into consideration some limitations. This review did not assess the contribution of indoor air pollution and other comorbidities to the increased risk of PTB incidence, hospital admission and mortality. The different study designs and methodologies affected the types of confounders that could be adjusted for in the different studies and therefore introducing inconsistency in the adjustment of confounders across studies. This review, therefore, focused on the strongest reported associations between air pollutant exposure and PTB incidence rather than on the duration of exposure to the air pollutants.
Exposure to PM 2.5 , PM 10 , NO 2 and SO 2 air pollutants was found to be associated with an increased incidence of PTB, while exposure to CO and O 3 was not. These findings of this study and the overall quality of the evidence highlight the need for more rigorous exploration of this association.
Data availability
The datasets used and/or analysed during the current study available from the corresponding author on reasonable request.
World Health Organization. Global Tuberculosis Report 2019 (World Health Organization, 2019).
Google Scholar
GBD Tuberculosis Collaborators. Global, regional, and national burden of tuberculosis, 1990–2016: Results from the global burden of diseases, injuries, and risk factors 2016 study. Lancet Infect Dis. 18 (12), 1329–1349 (2018).
Article Google Scholar
United Nations. Sustainable Development Goals. Goal 3: Ensure healthy lives and promote well-being for all at all ages [Internet]. https://www.un.org/sustainabledevelopment/health/ [cited 2020 Jan 17].
Hargreaves, J. R. et al. The social determinants of tuberculosis: From evidence to action. Am. J. Public Health 101 (4), 654–662 (2011).
Article PubMed PubMed Central Google Scholar
Grobusch, M. P. & Kapata, N. Global burden of tuberculosis: Where we are and what to do. Lancet Infect. Dis. 18 (12), 1291–1293 (2018).
Article PubMed Google Scholar
Rajaei, E. et al. Outdoor air pollution affects tuberculosis development based on geographical information system modeling. Biomed. Biotechnol. Res. J. BBRJ 2 (1), 39 (2018).
Li, Z. et al. Long-term effect of exposure to ambient air pollution on the risk of active tuberculosis. Int. J. Infect. Dis. 1 (87), 177–184 (2019).
Article CAS Google Scholar
Requia, W. J. et al. Global association of air pollution and cardiorespiratory diseases: A systematic review, meta-analysis, and investigation of modifier variables. Am. J. Public Health 108 (S2), S123–S130 (2017).
Atkinson, R. W., Kang, S., Anderson, H. R., Mills, I. C. & Walton, H. A. Epidemiological time series studies of PM2.5 and daily mortality and hospital admissions: A systematic review and meta-analysis. Thorax 69 (7), 660–665 (2014).
Article CAS PubMed Google Scholar
Ab Manan, N., Noor Aizuddin, A. & Hod, R. Effect of air pollution and hospital admission: A systematic review. Ann. Glob. Health 84 (4), 670–678 (2018).
Kelly, F. J. & Fussell, J. C. Air pollution and airway disease. Clin. Exp. Allergy J. Br. Soc. Allergy Clin. Immunol. 41 (8), 1059–1071 (2011).
Moore, E. et al. Global associations between air pollutants and chronic obstructive pulmonary disease hospitalizations. A systematic review. Ann. Am. Thorac. Soc. 13 (10), 1814–1827 (2016).
PubMed PubMed Central Google Scholar
Song, Q., Christiani, D. C., Wang, X. & Ren, J. The global contribution of outdoor air pollution to the incidence, prevalence, mortality and hospital admission for chronic obstructive pulmonary disease: A systematic review and meta-analysis. Int. J. Environ. Res. Public Health 11 (11), 11822–11832 (2014).
Schwartz, J. PM10 ozone, and hospital admissions for the elderly in Minneapolis-St. Paul, Minnesota. Arch. Environ. Health Int. J. 49 (5), 366–374 (1994).
Dockery, D. W. et al. Effects of inhalable particles on respiratory health of children. Am. Rev. Respir. Dis. 139 (3), 587–594 (1989).
Sumpter, C. & Chandramohan, D. Systematic review and meta-analysis of the associations between indoor air pollution and tuberculosis. Trop. Med. Int. Health 18 (1), 101–108 (2013).
Kolappan, C. & Subramani, R. Association between biomass fuel and pulmonary tuberculosis: A nested case–control study. Thorax 64 (8), 705–708 (2009).
Kurmi, O. P., Sadhra, C. S., Ayres, J. G. & Sadhra, S. S. Tuberculosis risk from exposure to solid fuel smoke: A systematic review and meta-analysis. J. Epidemiol. Community Health 68 (12), 1112–1118 (2014).
Popovic, I. et al. A systematic literature review and critical appraisal of epidemiological studies on outdoor air pollution and tuberculosis outcomes. Environ. Res. 170 , 33–45 (2019).
Dimala, C. A., Kadia, B. M. & Hansell, A. The association between ambient air pollution and pulmonary tuberculosis: A systematic review protocol. Environ. Evid. 9 (1), 29 (2020).
Haddaway, N. R., Macura, B., Whaley, P. & Pullin, A. S. ROSES RepOrting standards for Systematic Evidence Syntheses: Pro forma, flow-diagram and descriptive summary of the plan and conduct of environmental systematic reviews and systematic maps. Environ. Evid. 7 (1), 7 (2018).
Study Quality Assessment Tools—NHLBI, NIH [Internet]. https://www.nhlbi.nih.gov/health-pro/guidelines/in-develop/cardiovascular-risk-reduction/tools [cited 2017 Nov 2].
What is GRADE?—BMJ Best Practice [Internet]. https://bestpractice.bmj.com/info/toolkit/learn-ebm/what-is-grade/ [cited 2020 Jan 17].
Unit Conversion | Air Pollution Information System [Internet]. http://www.apis.ac.uk/unit-conversion [cited 2020 Feb 10].
Begg, C. B. & Mazumdar, M. Operating characteristics of a rank correlation test for publication bias. Biometrics 50 (4), 1088–1101 (1994).
Article CAS PubMed MATH Google Scholar
Egger, M., Smith, G. D., Schneider, M. & Minder, C. Bias in meta-analysis detected by a simple, graphical test. BMJ 315 (7109), 629–634 (1997).
Article CAS PubMed PubMed Central Google Scholar
Hwang, S. et al. Impact of outdoor air pollution on the incidence of tuberculosis in the Seoul metropolitan area, South Korea. Korean J. Intern. Med. 29 (2), 183–190 (2014).
Jassal, M. S., Bakman, I. & Jones, B. Correlation of ambient pollution levels and heavily-trafficked roadway proximity on the prevalence of smear-positive tuberculosis. Public Health 127 (3), 268–274 (2013).
Smith, G. S., Schoenbach, V. J., Richardson, D. B. & Gammon, M. D. Particulate air pollution and susceptibility to the development of pulmonary tuberculosis disease in North Carolina: An ecological study. Int. J. Environ. Health Res. 24 (2), 103–112 (2014).
Álvaro-Meca, A., Díaz, A., de Miguel, D. J., Resino, R. & Resino, S. Environmental factors related to pulmonary tuberculosis in HIV-infected patients in the combined antiretroviral therapy (cART) era. PLoS One 11 (11), e0165944 (2016).
Article PubMed PubMed Central CAS Google Scholar
Chen, K. Y. et al. Particulate matter is associated with sputum culture conversion in patients with culture-positive tuberculosis. Ther. Clin. Risk Manag. 12 , 41 (2016).
Lai, T. C. et al. Ambient air pollution and risk of tuberculosis: A cohort study. Occup. Environ. Med. 73 (1), 56–61 (2016).
Peng, Z., Liu, C., Xu, B., Kan, H. & Wang, W. Long-term exposure to ambient air pollution and mortality in a Chinese tuberculosis cohort. Sci. Total Environ. 15 (580), 1483–1488 (2017).
Article ADS CAS Google Scholar
Smith Geneé, S. et al. Air pollution and pulmonary tuberculosis: A nested case-control study among members of a Northern California Health Plan. Environ. Health Perspect. 124 (6), 761–768 (2016).
Zhu, S. et al. Ambient air pollutants are associated with newly diagnosed tuberculosis: A time-series study in Chengdu, China. Sci Total Environ. 631–632 , 47–55 (2018).
Article ADS PubMed CAS Google Scholar
Joob, B. & Wiwanitkit, V. Incidence of pulmonary tuberculosis and particulate matter 2.5 pollutant level: The association analysis for 2019 air pollution Crisis, Bangkok, Thailand. Biomed. Biotechnol. Res. J. 3 (2), 126–126 (2019).
Yao, L. et al. Ambient air pollution exposures and risk of drug-resistant tuberculosis. Environ. Int. 124 , 161–169 (2019).
Article PubMed CAS Google Scholar
Carrasco-Escobar, G., Schwalb, A., Tello-Lizarraga, K., Vega-Guerovich, P. & Ugarte-Gil, C. Spatio-temporal co-occurrence of hotspots of tuberculosis, poverty and air pollution in Lima, Peru. Infect. Dis. Poverty 9 (1), 32 (2020).
Huang, K. et al. Association between short-term exposure to ambient air pollutants and the risk of tuberculosis outpatient visits: A time-series study in Hefei, China. Environ. Res. 184 , 109343 (2020).
Wang, W. et al. Epidemiological characteristics of tuberculosis and effects of meteorological factors and air pollutants on tuberculosis in Shijiazhuang, China: A distribution lag non-linear analysis. Environ. Res. 22 , 110310 (2020).
Yang, J. et al. A study on the relationship between air pollution and pulmonary tuberculosis based on the general additive model in Wulumuqi, China. Int. J. Infect. Dis. 1 (96), 42–47 (2020).
You, S., Tong, Y. W., Neoh, K. G., Dai, Y. & Wang, C. H. On the association between outdoor PM2.5 concentration and the seasonality of tuberculosis for Beijing and Hong Kong. Environ. Pollut. Barking Essex 2016 (218), 1170–1179 (1987).
Liu, Y., Cui, L. L., Hou, L. J., Yu, C. B., Tao, N. N., Liu, J. Y. et al . Ambient air pollution exposures and newly diagnosed pulmonary tuberculosis in Jinan, China: A time series study. Sci. Rep. [ Internet ] 8 (1). https://www.scopus.com/inward/record.uri?eid=2-s2.0-85057222564&doi=10.1038%2fs41598-018-35411-6&partnerID=40&md5=688c7ce37da23c36a007e125ddddadbc (2018).
Kim, H., Yu, S. & Choi, H. Effects of particulate air pollution on tuberculosis development in seven major cities of Korea from 2010 to 2016: Methodological considerations involving long-term exposure and time lag. Epidemiol. Health 42 , e2020012 (2020).
Liu, Y. et al. Effect of ambient air pollution on tuberculosis risks and mortality in Shandong, China: A multi-city modeling study of the short- and long-term effects of pollutants. Environ. Sci. Pollut. Res. Int. 28 (22), 27757–27768 (2021).
Xiong, Y. et al. Association of daily exposure to air pollutants with the risk of tuberculosis in Xuhui District of Shanghai, China. Int. J. Environ. Res. Public Health 19 (10), 6085 (2022).
Sohn, M. et al. Association of social deprivation and outdoor air pollution with pulmonary tuberculosis in spatiotemporal analysis. Int. J. Environ. Health Res. 29 (6), 657–667 (2019).
Liu, F., Zhang, Z., Chen, H. & Nie, S. Associations of ambient air pollutants with regional pulmonary tuberculosis incidence in the central Chinese province of Hubei: A Bayesian spatial-temporal analysis. Environ. Health 19 (1), 51 (2020).
Wang, H., Tian, C., Wang, W. & Luo, X. Temporal cross-correlations between ambient air pollutants and seasonality of tuberculosis: A time-series analysis. Int. J. Environ. Res. Public Health [ Internet ] 16 (9). https://www.scopus.com/inward/record.uri?eid=2-s2.0-85065795216&doi=10.3390%2fijerph16091585&partnerID=40&md5=6b4fa1102eabb1f409e8770c89f3a35f (2019).
Butt, E. W. et al. Global and regional trends in particulate air pollution and attributable health burden over the past 50 years. Environ. Res. Lett. 12 (10), 104017 (2017).
Shaddick, G., Thomas, M. L., Mudu, P., Ruggeri, G. & Gumy, S. Half the world’s population are exposed to increasing air pollution. Npj Clim. Atmos. Sci. 3 (1), 1–5 (2020).
Ambient (outdoor) air pollution [Internet]. https://www.who.int/news-room/fact-sheets/detail/ambient-(outdoor)-air-quality-and-health [cited 2022 Jun 11].
McConnell, R. et al. Asthma in exercising children exposed to ozone: A cohort study. Lancet Lond. Engl. 359 (9304), 386–391 (2002).
Gauderman, W. J. et al. The effect of air pollution on lung development from 10 to 18 years of age. N. Engl. J. Med. 351 (11), 1057–1067 (2004).
Gasser, M. et al. Toxic effects of brake wear particles on epithelial lung cells in vitro. Part Fibre Toxicol. 6 (1), 30 (2009).
Behndig, A. F. et al. Airway antioxidant and inflammatory responses to diesel exhaust exposure in healthy humans. Eur. Respir. J. 27 (2), 359–365 (2006).
Article ADS CAS PubMed Google Scholar
Rivas, C. E., Cantarella, P., Sarkar, S., Rockafellow, M., Osornio Vargas, A. R., Torres, M. et al . Particulate air pollution matter (PM) modifies innate immunity of type II pneumocytes (A549) against mycobacterium tuberculosis. Am. J. Respir. Crit. Care Med . [ Internet ]. 189 , Meeting Abstracts. https://doi.org/10.1164/ajrccm-conference.2014.189.1_MeetingAbstracts.A2489 (2014).
Sarkar, S. et al. Suppression of the NF-κB pathway by diesel exhaust particles impairs human antimycobacterial immunity. J. Immunol. 188 (6), 2778–2793 (2012).
Ge, E. et al. Ambient sulfur dioxide levels associated with reduced risk of initial outpatient visits for tuberculosis: A population based time series analysis. Environ. Pollut. 228 , 408–415 (2017).
Xu, M. et al. Association of air pollution with the risk of initial outpatient visits for tuberculosis in Wuhan, China. Occup. Environ. Med. 76 (8), 560–566 (2019).
Tuberculosis (TB) [Internet]. https://www.who.int/news-room/fact-sheets/detail/tuberculosis [cited 2021 Apr 3].
Tuberculosis China [Internet]. https://www.who.int/westernpacific/health-topics/tuberculosis [cited 2021 Apr 3].
Download references
Author information
Authors and affiliations.
Health and Human Development (2HD) Research Network, Douala, Cameroon
Christian Akem Dimala
Department of Medicine, Reading Hospital, Tower Health System, West Reading, PA, USA
Health Education and Research Organisation (HERO) Cameroon, Buea, Cameroon
Benjamin Momo Kadia
Department of Clinical Sciences, Liverpool School of Tropical Medicine, Liverpool, UK
You can also search for this author in PubMed Google Scholar
Contributions
C.A.D. conceived and designed the experiments. C.A.D. and B.M.K. conducted the experiments. C.A.D. produced the manuscript. B.M.K. reviewed the manuscript. All authors approved the final copy of the manuscript.
Corresponding author
Correspondence to Benjamin Momo Kadia .
Ethics declarations
Competing interests.
The authors declare no competing interests.
Additional information
Publisher's note.
Springer Nature remains neutral with regard to jurisdictional claims in published maps and institutional affiliations.
Supplementary Information
Supplementary information 1., supplementary information 2., supplementary information 3., supplementary information 4., supplementary information 5., supplementary information 6., supplementary information 7., supplementary information 8., rights and permissions.
Open Access This article is licensed under a Creative Commons Attribution 4.0 International License, which permits use, sharing, adaptation, distribution and reproduction in any medium or format, as long as you give appropriate credit to the original author(s) and the source, provide a link to the Creative Commons licence, and indicate if changes were made. The images or other third party material in this article are included in the article's Creative Commons licence, unless indicated otherwise in a credit line to the material. If material is not included in the article's Creative Commons licence and your intended use is not permitted by statutory regulation or exceeds the permitted use, you will need to obtain permission directly from the copyright holder. To view a copy of this licence, visit http://creativecommons.org/licenses/by/4.0/ .
Reprints and permissions
About this article
Cite this article.
Dimala, C.A., Kadia, B.M. A systematic review and meta-analysis on the association between ambient air pollution and pulmonary tuberculosis. Sci Rep 12 , 11282 (2022). https://doi.org/10.1038/s41598-022-15443-9
Download citation
Received : 21 December 2021
Accepted : 23 June 2022
Published : 04 July 2022
DOI : https://doi.org/10.1038/s41598-022-15443-9
Share this article
Anyone you share the following link with will be able to read this content:
Sorry, a shareable link is not currently available for this article.
Provided by the Springer Nature SharedIt content-sharing initiative
This article is cited by
The effects of meteorological factors and air pollutants on the incidence of tuberculosis in people living with hiv/aids in subtropical guangxi, china.
- Fengyi Wang
- Zongxiang Yuan
BMC Public Health (2024)
By submitting a comment you agree to abide by our Terms and Community Guidelines . If you find something abusive or that does not comply with our terms or guidelines please flag it as inappropriate.
Quick links
- Explore articles by subject
- Guide to authors
- Editorial policies
Sign up for the Nature Briefing newsletter — what matters in science, free to your inbox daily.

Click through the PLOS taxonomy to find articles in your field.
For more information about PLOS Subject Areas, click here .
Loading metrics
Open Access
Peer-reviewed
Research Article
The effect of air-pollution and weather exposure on mortality and hospital admission and implications for further research: A systematic scoping review
Roles Conceptualization, Data curation, Formal analysis, Investigation, Methodology, Project administration, Resources, Visualization, Writing – original draft, Writing – review & editing
* E-mail: [email protected]
Affiliation School of Geography and Sustainable Development, University of St Andrews, Scotland, United Kingdom

Roles Conceptualization, Funding acquisition, Supervision, Writing – review & editing
Affiliation School of Medicine, University of St Andrews, Scotland, United Kingdom
Roles Methodology, Validation
Affiliation Department of Landscape Design and Ecosystem Management, American University of Beirut, Beirut, Lebanon
Roles Conceptualization, Funding acquisition, Investigation, Supervision, Writing – review & editing
- Mary Abed Al Ahad,
- Frank Sullivan,
- Urška Demšar,
- Maya Melhem,
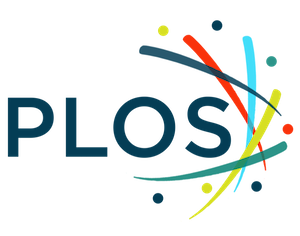
- Published: October 29, 2020
- https://doi.org/10.1371/journal.pone.0241415
- Peer Review
- Reader Comments
Air-pollution and weather exposure beyond certain thresholds have serious effects on public health. Yet, there is lack of information on wider aspects including the role of some effect modifiers and the interaction between air-pollution and weather. This article aims at a comprehensive review and narrative summary of literature on the association of air-pollution and weather with mortality and hospital admissions; and to highlight literature gaps that require further research.
We conducted a scoping literature review. The search on two databases (PubMed and Web-of-Science) from 2012 to 2020 using three conceptual categories of “environmental factors”, “health outcomes”, and “Geographical region” revealed a total of 951 records. The narrative synthesis included all original studies with time-series, cohort, or case cross-over design; with ambient air-pollution and/or weather exposure; and mortality and/or hospital admission outcomes.
The final review included 112 articles from which 70 involved mortality, 30 hospital admission, and 12 studies included both outcomes. Air-pollution was shown to act consistently as risk factor for all-causes, cardiovascular, respiratory, cerebrovascular and cancer mortality and hospital admissions. Hot and cold temperature was a risk factor for wide range of cardiovascular, respiratory, and psychiatric illness; yet, in few studies, the increase in temperature reduced the risk of hospital admissions for pulmonary embolism, angina pectoris, chest, and ischemic heart diseases. The role of effect modification in the included studies was investigated in terms of gender, age, and season but not in terms of ethnicity.
Air-pollution and weather exposure beyond certain thresholds affect human health negatively. Effect modification of important socio-demographics such as ethnicity and the interaction between air-pollution and weather is often missed in the literature. Our findings highlight the need of further research in the area of health behaviour and mortality in relation to air-pollution and weather, to guide effective environmental health precautionary measures planning.
Citation: Abed Al Ahad M, Sullivan F, Demšar U, Melhem M, Kulu H (2020) The effect of air-pollution and weather exposure on mortality and hospital admission and implications for further research: A systematic scoping review. PLoS ONE 15(10): e0241415. https://doi.org/10.1371/journal.pone.0241415
Editor: Chon-Lin Lee, National Sun Yat-sen University, TAIWAN
Received: June 23, 2020; Accepted: October 15, 2020; Published: October 29, 2020
Copyright: © 2020 Abed Al Ahad et al. This is an open access article distributed under the terms of the Creative Commons Attribution License , which permits unrestricted use, distribution, and reproduction in any medium, provided the original author and source are credited.
Data Availability: All relevant data are within the paper and its Supporting Information files.
Funding: This review is part of a PhD project that is funded by the St Leonard’s PhD scholarship, University of St Andrews, Scotland, United Kingdom. The open access publication fees were funded by the University of St Andrews Libraries, Scotland, United Kingdom.
Competing interests: The authors declare that they have no conflict of interest.
Introduction
Air-pollution and weather exposure beyond region-specific thresholds have serious effects on the public health [ 1 , 2 ]. Worldwide, population growth, increased urbanization, economic and industrial growth, intense energy consumption, high usage of transportation vehicles, improved living standards, and changing lifestyles and consumption patterns for at least the last 100 years have resulted in increased emissions of air pollutants including greenhouse gases; and fluctuations in ambient temperature and other weather variables [ 3 , 4 ].
Ambient air-pollution consists of a range of pollutants including particulate matters with diameters of less than 10 μm (PM10) and less than 2.5 μm (PM2.5), nitrogen oxides (NOx) including nitrogen dioxide (NO2), Sulphur dioxide (SO2), Carbon monoxide (CO), and Ozone (O3) that have been associated with a range of different acute and chronic health conditions [ 5 , 6 ].
Weather exposure in terms of changing temperature, relative humidity, rainfall and other weather patterns can cause a wide range of acute illness and result in deaths especially among vulnerable populations who lack adequate physiological and behavioural responses to weather fluctuations [ 7 , 8 ]. Age (elderly and children vs adults), sex, socioeconomic factors (poverty, education, and ethnicity among others), pre-existing chronic diseases, use of certain medications, and environmental conditions such as the absence of central heating increase individual’s susceptibility to environmental exposures [ 1 , 9 , 10 ]. Research has shown that hospital admissions and mortality increase when weather exposure exceed certain thresholds with lags up to 20 days [ 11 – 14 ].
Most of the literature has shown positive correlations of air-pollution and/or exposure to weather variables beyond region-specific thresholds with all-cause and cause-specific mortality and/or hospital admission especially related to respiratory and cardiovascular diseases [ 14 – 21 ]. Though, there is a lack of information on wider aspects including the role of some effect modifiers such as ethnicity and the interaction between air-pollution and weather factors. Literature has shown that ethnic minorities often live in more disadvantaged, highly populated urban communities with poor housing conditions and higher levels of air pollution exposure [ 22 – 24 ]. This results in poorer health and higher risk for chronic health problems with time. Similar to ethnicity, the interaction between air-pollution and weather variables in relation to health outcomes is often missed in the literature despite its importance in minimizing biased estimations. Air pollutants are highly reactive, and their formation is either catalysed or slowed down based on the existing weather conditions. For example, the presence of sunlight catalyses the formation of ozone pollutant resulting in higher ozone concentrations during the summer [ 25 ].
In this context, a thorough literature review is needed to map the available literature and highlight areas that require further research and investigation. Not to mention that further understanding of the effect of air-pollution and weather exposure on mortality and hospital admission is needed to achieve better environmental and health system planning, organization, resources allocation, and interventions. This article aims to provide a comprehensive review and narrative summary (not numerical estimate) of literature on the association of air-pollution and weather with mortality and hospital admissions; and to shed the light on areas that require further research. As far as we are aware, this is the first literature review examining the effect of multiple exposures (air-pollution and weather) on multiple outcomes (mortality and hospital admissions). We chose to focus our scoping literature review on countries that are part of the single European Union (EU) market (Austria, Belgium, Bulgaria, Croatia, Republic of Cyprus, Czech Republic, Denmark, Estonia, Finland, France, Germany, Greece, Hungary, Ireland, Italy, Latvia, Lithuania, Luxembourg, Malta, Netherlands, Poland, Portugal, Romania, Slovakia, Slovenia, Spain and Sweden, Norway, and Switzerland) and United Kingdom (UK) because these countries exhibit similar socio-economic, environmental, and health policies; minimizing the contextual differences in the effect of air-pollution and weather on mortality and hospital admission. Literature examining the effect of air-pollution and/or weather on mortality and hospital admissions in countries outside the EU and UK will be used for comparison purposes.
Materials and methods
Search strategy and database sources.
To ensure methodological reliability, we carried out our scoping literature review according to the “Preferred Reporting Items for Systematic Reviews and Meta-Analyses for scoping reviews” (PRISMA-ScR) guidelines ( S1 Checklist ) [ 26 ].
A literature search was performed on the 6 th of February 2020 using “PubMed” and “Web of Science” database sources that cover health, medical, and environmental literature. We attempted to assess the effects of air-pollution and weather events on mortality and hospital admission in Europe by searching original research articles published in peer-reviewed journals in the last 8 years (between 06/02/2012 and 06/02/2020 inclusive). We chose to review research published in the last 8 years because in March 2007, the European Union (EU) Heads of State and Government endorsed an “integrated climate change and energy strategy” that will come into action post the expiry of Kyoto Protocol targets in 2012 and that aims to combat climate change and weather fluctuations and cut air-pollution emissions to 30% below the 1990 levels [ 27 ].
Our search strategy was divided into three conceptual categories: “environmental factors”, “health outcomes”, and “Geographical region”. The “Environmental factors” refers to air-pollution, including PM10, PM2.5, NO2, SO2, CO, and O3 air pollutants and to weather variables, including air temperature, rainfall, wind, relative humidity, and vapour pressure. The “health outcomes” include hospital admissions and mortality and the “Geographical region” refers to the EU countries and UK. For each conceptual category, a set of “MeSH” and “All Fields” terms joined by the Boolean operator “OR” were developed. Later, the three conceptual categories’ search terms were joined using the Boolean operator “AND”. Our search strategy excluded the “influenza infections”, as these are considered confounders rather than outcomes for air-pollution and weather exposure. For more details about the search codes used to navigate PubMed and Web of Science search engines, please refer to S1 Table .
To minimize finding irrelevant literature, our search was limited to the following categories in the “Web of Science” search engine: environmental sciences, public environmental occupational health, medicine general internal, environmental studies, multidisciplinary sciences, geosciences multidisciplinary, respiratory system, geography physical, geography, cardiac cardiovascular systems, urban studies, healthcare sciences services, peripheral vascular disease, medicine research experimental, emergency medicine, critical care medicine, health policy services, primary healthcare, social sciences biomedical, and demography. Grey literature, non-English language articles, conference abstracts, books, reports, masters and PhD dissertations, and unpublished studies were excluded from this review.
Inclusion and exclusion criteria
To determine the studies that would be included in this scoping review, a set of inclusion and exclusion criteria were developed for the procedure of title, keyword, and abstract screening.
The inclusion criteria involved original quantitative research studies conducted in the EU and UK; that included at least one analysis where mortality and/or hospital admission was the outcome and where one or more of the following exposures were investigated: 1) ambient air pollutants including PM10, PM2.5, CO, NO2/NOx, SO2, and O3; 2) weather exposures including temperature, rainfall, wind, humidity, and pressure; and 3) extreme weather events including heat waves, cold spells, and droughts. Due to the large amount of literature on this topic and to allow comparable results between the studies, this review was limited to cohort, time-series, and case-crossover/self-controlled quantitative study designs where hazard ratios (HR), relative risks (RR), odd ratios (OR), or percentage increase were reported for quantifying the factors associated with mortality and hospital admission. These three study designs allow a temporal follow up to evaluate the effect of time varying exposures (air-pollution and weather) on the mortality and hospital admission health outcomes.
The exclusion criteria included the following:
- Methodological studies
- Original data studies that investigated the effect of ambient air-pollution and/or weather on mortality and/or hospital admission in countries outside the EU market and UK
- Articles studying the effect of indoor air-pollution on mortality and hospital admission
- Studies examining air-pollution and weather exposure on animals and plants
- Studies on occupational air-pollution exposure
- Non-English language articles
- Mortality and/or hospital admission projections and forecasting studies
- Protocol and letter to editor papers
- Qualitative research studies
- All types of literature reviews including but not limited to narrative, scoping, and systematic literature reviews
Screening and data abstraction
Our search strategy revealed 487 articles from the “PubMed” database and 517 articles from the “Web of Science” database. These articles were exported to the citation manager software “Endnote” where 53 duplicates were identified and removed resulting in a total of 951 articles ( Fig 1 ). Using the titles, key words, and abstracts, the 951 articles were screened for relevance according to the inclusion and exclusion criteria, explained in the previous section only by first author (MA). To ensure a rigorous and reliable application of the inclusion and exclusion criteria in the screening process, a second researcher (MM) screened independently a sample of 20% of the titles and abstracts of the 951 identified records. Disagreements between the two researchers were resolved through discussion until consensus was reached. All the studies that met the inclusion criteria (n = 149 articles) were retrieved for full text screening by MA. Following the full text screening phase, an additional 37 articles were excluded by MA resulting in a total of 112 articles to be included in the final narrative synthesis ( Fig 1 ).
- PPT PowerPoint slide
- PNG larger image
- TIFF original image
https://doi.org/10.1371/journal.pone.0241415.g001
For narrative synthesis, the following information was retrieved from the 112 articles:
- Study design
- Location of the study population
- The outcome of interest
- Sample size
- Exposure variables
- The confounders adjusted for
- The assessed exposure time and the lags considered
- The exposure assessment method
- The statistical/modelling approach
- The relative risks (RR)/incident relative risks (IRR)/odd ratios (OR)/hazard ratios (HR) with their respective confidence intervals or the percentage increase that quantify the association between the outcome of interest (mortality and/or hospital admission) and the exposures (air-pollution and/or weather events).
Ethical approval
Not applicable for this scoping literature review as it only includes descriptive narrative analysis of 112 published articles.
A total of 112 studies ( S2 Table ) were included in the final narrative review from which 70 involved the mortality outcome, 30 the hospital admission outcome, and 12 studies included both health outcomes ( Table 1 ). Most of the studies used the time-series study design (n = 74, 66%) with Poisson models for data analysis, while minority of the reviewed studies employed the case-crossover design (n = 19, 17%) with conditional logistic regression for data analysis, and the cohort design (n = 18, 16%) with Cox hazard regression for data analysis ( Table 1 ).
https://doi.org/10.1371/journal.pone.0241415.t001
Most of the studies examined all-cause, cardiovascular and respiratory disease mortality and hospital admission outcomes while some studies tried to focus more directly on certain types of specific diseases such as psychiatric disorders including mania and depression, pulmonary embolism, myocardial infarction, stroke, ischemic heart disease, arrhythmias, atrial fibrillation, heart failure, cerebrovascular disease, chronic obstructive pulmonary disease (COPD), lung cancer, and diabetes ( Table 1 ).
Table 1 shows the descriptive statistics of the included articles. S2 Table summarise the characteristics of the included studies in more details by the type of investigated health outcome. S3 Table demonstrate the included article’s reported associations in terms of coefficients with 95% confidence intervals between air-pollution and/or weather exposure and mortality and/or hospital admission outcomes.
The effect of air-pollution on mortality and hospital admission
In this review, six air pollutants (PM2.5, PM10, O3, CO, SO2, and NO2/NOx) were identified as causes of increased rates of mortality and hospital admissions. Each pollutant affects a range of diseases, most commonly, cardiovascular, respiratory, and cerebrovascular diseases. Some of the health effects can be immediate while others might appear after several days of initial exposure ( Fig 2 ).
https://doi.org/10.1371/journal.pone.0241415.g002
The effect of particulate matter pollutants on mortality and hospital admission.
Particulate matter is a heterogeneous mixtures of liquid droplets and solid particles suspended in the air that can result either from natural resources (windblown Saharan and non-Saharan dust, volcano ashes, forest fires, pollen, etc…) or from man-made activities including industrial processes, transportation vehicle smoke, burning of fossil fuels, extensive energy usage, combustion processes, and grinding and mining industries [ 28 ]. Due to its size, mass composition, and chemical components, particulate matter with larger diameter (PM10) will be deposited in nasal cavities and upper airways while particulate matter with smaller diameter (PM2.5) may penetrate more deeply the respiratory system reaching the alveoli and blood stream, carrying with them various toxic substances [ 29 ]. This in turn will cause health problems in humans such as asthma, irregular heartbeat, nonfatal heart attacks, decreased lung function, coughing and difficulty breathing symptoms [ 30 ].
Our review showed that PM10 air-pollution is positively associated with a range of cardiovascular and respiratory diseases mortality and hospital admission outcomes ( Fig 3A and S3 Table ). Fischer et al. (2015) showed an elevated hazard of 1.06 (95% CI = 1.04 to 1.08) for cardiovascular disease mortality for every 10 μg/m3 increase in PM10 pollution in the Netherlands [ 31 ]. Likewise, PM10 pollution acted as a risk factor for respiratory diseases mortality (HR = 1.11, 95%CI = 1.08 to 1.15; RR = 1.056, 95%CI = 1.043 to 1.069) [ 21 , 32 ] and hospital admission (%increase = 0.69, 95% CI = 0.20 to 1.19) [ 20 ].
https://doi.org/10.1371/journal.pone.0241415.g003
Air-pollution with PM2.5 exhibited a similar effect on human health as that of PM10 ( Fig 3B and S3 Table ). Nevertheless, PM2.5 was shown to have a greater risk on human health as compared to PM10 due to its smaller diameter size allowing more deep penetration into the respiratory system [ 33 ]. In France, Sanyal et al. (2018) showed an increased risk of 1.11 and 1.02 for all-cause hospital admission and moratality respectively per 10 μg/m3 increase in PM2.5 pollutant [ 32 ].
The effect of ozone pollution on mortality and hospital admission.
Contrary to particulate matter pollution, the effect of ozone on mortality and hospital admission did not show a consistent effect. In some studies, ozone acted as a protective factor agianst mortality and hospital admission, while in other studies it showed increased risk or no association with mortality and hospital admissions ( Fig 3C and S3 Table ). This is related to the fact that ozone is a highly reactive pollutant and its formation is related to the presence of sunlight [ 25 ]. In a cohort study conducted by Carey et al. (2013) in England, ozone acted as a protective factor agianst all-cause mortality (HR = 0.96, 95%CI = 0.93 to 0.98), cardiovascular mortality (HR = 0.96, 95%CI = 0.94 to 0.98), respiratory mortality (HR = 0.93, 95%CI = 0.90 to 0.96), and lung cancer mortality (HR = 0.94, 95%CI = 0.90 to 0.98) [ 21 ]. However, ozone acted as a risk factor in some of the reviewed studies leading up to 2% increase in all-cause mortality per interquartile range increase of ozone concentration [ 34 – 37 ].
The effect of nitrogen oxides pollution on mortality and hospital admission.
Similar to other air pollutants, this review showed that exposure to nitrogen dioxide and nitrogen oxides pollution can cause many types of diseases resulting in increased risk for all-cause mortality and hospital admission [ 25 , 32 , 38 , 39 ] ( Fig 3D and S3 Table ). A study conducted in Belgium showed a 3.5% increase in cardiovascular hospital admission as well as 4.5% and 4.9% increase in ischemic stroke and haemorrhagic stroke hospital admissions respectively for each 10 μg/m3 increase in NO2 [ 40 ].
The effect of sulphur dioxide pollution on mortality and hospital admission.
Sulphur dioxide air-pollution is mainly caused from industrial processes and power plants that involve burning of fossil fuel. Exposure to SO2 pollution can cause mild health effects including eyes, nose, and throat irritations as well as severe health effects such as bronchial spasms and deaths due to respiratory insufficiency [ 41 ].
The effect of sulphur dioxide (SO2) on mortality and hospital admission was investigated in only 12 out of the 112 reviewed studies. Exposure to SO2 air-pollution was found to increase the risk for all-cause, cardiovascular, and respiratory mortality [ 21 , 39 , 42 ] ( Fig 3E and S3 Table ).
The effect of carbon monoxide pollution on mortality and hospital admission.
Carbon monoxide results from incomplete combustion of fossil fuels. Carbon monoxide is dangerous for human beings since it possess the ability to bind to haemoglobin resulting in reduction of the red blood cells to carry oxygen to cells [ 41 ].
Only 10 out of the 112 reviewed studies investigated the association of carbon monoxide (CO) with mortality and hospital admission. The majority of these studies showed that carbon monoxide exposure can cause a number of cardiovascular and respiratory health problems ( Fig 3F and S3 Table ). Exposure to carbon monoxide pollution resulted in increased odds for pulmonary embolism hospital admission [ 43 ]. Additionally, Renzi et al. (2017) showed that all-cause mortality increases by 0.12% for every 1 mg/m3 increase in CO [ 39 ]. On the contrary, carbon monoxide acted as a protective factor against chest disease hospital admission among patients with sickle cell anaemia in one of the reviewed studies [ 44 ]. This association was explained by the fact that carbon monoxide can bind to haemoglobin which enhances the affinity of other binding sites for oxygen in addition to reducing vasoconstriction and inflammation; suggesting a beneficial effect rather than risk factor for patients with sickle cell disease [ 44 ].
The effect of air temperature on mortality and hospital admission
Exposure to hot or cold temperature beyond region-specific thresholds exhibits a range of direct and indirect effects on human health. The direct effects include hyperthermia or heat stress during hot temperature exposures and hypothermia and ischemic stroke during cold temperature exposures [ 45 ]. Besides the direct effects, small fluctuations in temperature across time can result in indirect effects on the respiratory and cardiovascular systems of the body [ 45 ].
Most of the reviewed articles that studied the effect of weather exposure on mortality and hospital admission focused on air temperature exposure with lags ranging from 0 days up to 5 weeks for cold temperatures and from 0 days up to 25 days for hot temperatures. The reviewed studies examined the effect of cold temperature, hot temperature, and air temperature increase on a range of diseases, most commonly, cardiovascular, respiratory, and psychiatric disorders. Table 2 below shows the definitions of “cold temperature”, “hot temperature”, and “air temperature increase” classifications derived from the reviewed studies.
https://doi.org/10.1371/journal.pone.0241415.t002
Cold temperature acted as a risk factor for several types of mortality and hospital admission outcomes ( Fig 4A and S3 Table ). Nevertheless, cold temperature was a protective factor only in one of the reviwed studies for all-cause mortality at lag 0 (RR = 0.99, 95%CI = 0.985 to 0.995); yet cold temperature acted as a risk factor for all-cause mortality in the same study at lag of 14 days with a relative risk of 1.003 emphasizing the delayed effect of cold temperature on mortality [ 46 ].
https://doi.org/10.1371/journal.pone.0241415.g004
Similar to cold temperature, hot temperature also acted as a risk factor in most of the reviewed studies for a number of mortality and hospital admission outcomes ( Fig 4B and S3 Table ). On the other hand, hot temperatures were associated with reductions in hospital admission rates for ischemic heart disease (RR = 0.74, 95%CI = 0.55 to 0.99) in a study conducted by Bijelovic et al. (2017) and for all-cause hospital admissions (RR = 0.961, 95% CI = 0.956 to 0.967) and cardiovascular hospital admissions (RR = 0.975, 95% CI = 0.957 to 0.993) in a study conducted by Monteiro et al. (2013) [ 47 , 48 ].
Some studies examined the effect of increasing temperature across the whole year on mortality and hospital admission. More than half of these studies showed a significant positive association between the increasing temperature and the mortality and hospital admission outcomes ( Fig 4C and S3 Table ).
The effect of other weather exposures on mortality and hospital admission
Similar to the temperature, weather exposures that include humidity, rainfall, sunshine, snowcover, air pressure, daylight, wind speed and wind direction with lags ranging from 0 up to 7 days were found to affect a range of diseases, most commonly, cardiovascular, respiratory, and psychiatric disorders ( S3 Table ).
Weather variables that showed significant positive assciations with hospital admission included: a rainfall effect on psychiatric hospital admission [ 49 ], sunshine and daylight effects on hospital trauma [ 50 ] and psychaitric admissions [ 51 ], wind speed effects on chest disease hospital admission [ 44 ], and air pressure effects on mania and depression hospital admission [ 49 ].
It is worth mentioning that sunshine showed inconsistency in its effect on psychaitric hospital admission, acting as a risk factor in a Danish study [ 52 ] while acting as a protective factor in a study conducted in Ireland [ 49 ].
The adjustments and effect modifications for the association of air-pollution and weather exposure with mortality and hospital admission
Most of the reviewed studies stratified and adjusted their analysis by age and gender [ 25 , 40 , 51 , 53 – 61 ]. Socio-economic deprivation, education attainment, income level, marital status, and occupational class were considered as confounders or effect modifiers in some of the reviewed studies [ 25 , 58 , 62 – 66 ]. However, only one study considered ethnicity to act as an effect modifier in the association between all-cause mortality and “summer smog” days defined as having maximum temperature of 25°C and PM10 pollutant oncentration of 50 μg/m3 [ 62 ]. And only two studies investigating the effect of air-pollution on all-cause and cardiovascular mortality in England adjusted for ethnicity in their multivariate regresison models [ 67 , 68 ].
Some of the studies that examined the effect of air-pollution on mortality and hospital admission accounted for air temperature effect in their analysis [ 35 , 40 , 46 ]. Likewise, some of the reviewed articles that studied the association of weather exposure to mortality and hospital admission considered the effect of air-pollution in their analysis [ 34 , 65 , 69 – 72 ].
Other variables considered to affect the relationship of air-pollution and/or weather exposure with mortality and/or hospital admission included: weekend and holiday effect, population decrease during the summer, influenza epidemics, season, day of the week, and tobacco smoke [ 25 , 40 , 46 , 54 , 56 , 73 – 79 ].
In this scoping review of 112 articles, we aimed to examine the effect of (1) air pollution, (2) temperature, and (3) other weather exposures on mortality and hospital admission outcomes.
The first part of the review showed that air-pollution acted consistently as a risk factor for all-cause, cardiovascular, respiratory, cerebrovascular and cancer mortality and hospital admission in the EU and UK which is in line with the findings of studies conducted in other regions of the world [ 80 – 84 ]. For instance, elevated risks of cardiovascular and respiratory diseases mortality were reported in Istanbul-Turkey for every 10 μg/m3 increase in PM10, SO2 and NO2 pollutants [ 85 ]. An exception was ozone (O3) air-pollution which showed inconsistent association with mortality and hospital admission. Two explanations were offered in the literature for the negative association between health outcomes and ozone pollution. The first explanation is related to the fact that ozone is a highly seasonal pollutant since its formation is catalysed by sunlight rendering higher ozone concentrations in the summer as compared to winter season. Thus, ozone effect on health outcomes should be analysed by accounting for the season effect [ 21 ]. In the continental United States, a 49% higher risk in all-cause mortality was shown for every 10 ppb increase in ozone during the warm-season [ 86 ]. The second explanation is related to the high reactivity of ozone leading to the formation of other pollutants such as NO2 and particulate matter. Therefore, ozone is negatively correlated with other air pollutants and its effect on health outcomes should be analysed as a combined effect of O3 and NO2 (known as Ox effect) [ 25 ].
Additionally, our scoping review showed that the effect of particulate matter (PM10 and PM2.5) pollution on mortality and hospital admission is more studied in the literature as compared to the other air pollutants. This could be related to the more pronounced effects of particulate matter exposure on health which is corroborated by many studies across the world [ 81 , 87 ]. Despite the fact that PM10 particles are deposited in the nasal cavities and upper airways, PM2.5 may penetrate deep into the lung tissues (reaching the alveoli and bloodstream) and irritate the respiratory airways causing various respiratory and cardiovascular problems [ 29 , 30 , 88 ].
Similar to air pollution, the second part of this review showed that hot and cold temperature exposures beyond region-specific thresholds are risk factors for a wide range of respiratory, cardiovascular (including: ischemic heart disease, myocardial infarction, pulmonary embolism, stroke, heart failure, and COPD), and psychiatric (including: mania and depression) illness in the EU and UK. These findings are corroborated by a wide body of literature from across the world [ 89 – 97 ]. In India, cold temperatures below 13.8°C were associated with increased risk of 6.3% for all-cause mortality, 27.2% for stroke mortality, 9.7% for ischemic heart disease mortality, and 6.5% for respiratory diseases mortality [ 92 ]. In Istanbul-Turkey, 23 days of exposure to hot temperature above 22.8°C was associated with a total of 419 excess deaths [ 90 ]. In Korea, hot temperature days of 25°C compared to 15°C were significantly associated with a 4.5% increase in cardiovascular hospitalizations [ 98 ].
It is worth to point out that the effect of cold temperature on health is more delayed (up to 5 weeks) in comparison to the more immediate effects of hot temperature (up to 25 days). Similar study in Northeast-Asia showed a delayed risk of cold temperature on mortality after 5 to 11 days, yet a more immediate effect of hot temperature on mortality after 1 to 3 days in each of Taiwan, Korea, and Japan countries [ 91 ].
Although exposure to hot or cold temperature can affect the health negatively, our scoping review showed that in few studies, the increase in temperature reduced the risk of hospital admissions for some types of cardiovascular diseases; mainly for pulmonary embolism, angina pectoris, chest, and ischemic heart diseases. This could be explained by the fact that hot temperature can cause immediate increase in cardiovascular mortality rates; whereby many cases might pass directly to the death state without passing through the hospital admission state resulting in lower hospital admission rates [ 47 ].
The third part of this scoping review presented the studies that examined the effects of other weather exposures such as relative humidity, barometric pressure, rainfall, and wind speed on mortality and hospital admission outcomes. These weather exposures were found to affect significantly only hospital admission. No significant effect was noted with respect to the mortality outcome. The weather exposures acted as a risk factor for psychiatric disorders (including depression and Mania), chest disease, and trauma hospital admissions. This was corroborated by evidence from countries outside the EU as well [ 99 – 101 ]. Yet in some of the reviewed studies, weather exposures acted as a protective factor for some types of psychiatric and cardiovascular disorders. The significant negative association between ischemic heart disease hospital admission and humidity in one of the reviewed studies was explained by the fact that people in general and the elderly specifically reduce their activities during high humidity and temperature periods. This is mainly due to the lack of the body’s ability to perspire, which in turn reduces their risk of cardiovascular complications [ 48 ]. As for the protective effect of some weather exposures on psychiatric hospital admissions, similar findings were presented in Iran; with a negative association between barometric pressure and schizophrenia hospital admissions and rainy days and bipolar hospital admissions [ 101 ].
In addition to the association of air pollution and weather exposure with mortality and hospital admission outcomes, our review aimed to present the individual, socio-economic, and environmental factors that play an important role in modifying the latter association. The effect modifiers identified in this scoping review included: pre-existing health conditions, age, gender, educational attainment, wealth or income or socio-economic deprivation, occupation, marital status, tobacco smoking, season, day of the week, holidays, and influenza epidemics.
Individuals with pre-existing chronic health conditions face increased susceptibility toward air-pollution and weather exposure related mortality and hospital admission [ 52 , 54 , 78 , 102 ].
Older people are more vulnerable to the health effects associated with air-pollution, hot or cold temperatures, and other weather variables [ 54 , 56 , 103 – 105 ]. This is due to the physiological degeneration of the human body with increasing age. Aging affects the normal function of the body organs resulting in many chronic cardiovascular, urinary, and respiratory health conditions. This reduces the ability of older people to adapt to increased concentrations of air pollutants and changing weather conditions [ 103 , 105 , 106 ]. Moreover, old age people have lower immunity and antioxidant defence as compared to young people placing them at a higher risk [ 107 ]. Many older people also have reduced mobility and mental abilities which delay their access to healthcare leading to severe health complications and death [ 108 ].
As for gender, our review revealed inconsistency regarding its modification effect on the association between air-pollution and weather exposure and mortality and hospital admission health outcomes. Nevertheless, most of the reviewed studies have found that females have higher risks of mortality and/or hospital admission after exposure to air-pollution and/or weather fluctuations beyond region-specific thresholds including hot and cold temperatures [ 9 , 47 , 57 , 58 , 65 , 78 , 109 – 112 ]. Whereas some studies found higher risks of mortality and/or hospital admissions among males in relation to air-pollution and/or weather exposure [ 25 , 40 , 42 , 59 , 70 , 113 , 114 ]. One explanation for this might be due to the physiological differences between males and females. Females have smaller lung size, yet higher airways reactivity making them more susceptible to air-pollution health effects as compared to males [ 42 , 115 ]. Likewise, higher pulse rates and smaller heart size relative to the human body in females as compared to males render females more vulnerable to the health effects of air-pollution and hot or cold temperature exposures [ 115 ]. Moreover, females exhibit more fluctuations in hormone levels due to pregnancy, menstrual cycle and menopause periods which may place them at a higher health risk upon exposure to air-pollution and weather variations [ 115 ]. The different lifestyle, socio-economic position, and occupation type between males and females may also lead to different levels and duration of air-pollution and weather exposure [ 62 , 109 , 116 – 118 ]. However, it is worth mentioning that the effect modification of gender in the association of air-pollution and weather exposure with mortality and hospital admission outcomes is believed to be confounded by age since in many of the reviewed studies, higher risks were found among old aged females (age>65 years old) [ 47 , 57 , 61 , 78 , 112 ] and old aged males (age >70 years old) [ 114 ]. This confounding effect could be reduced either by assessing the combined effect modification of age and gender through an interaction term or by stratifying the analysis according to both the age groups and gender.
Wealth and socio-economic deprivation were also considered by some of the reviewed studies as an effect modifier in the relationship of air-pollution and weather with mortality and hospital admission. In general, the absence of wealth and presence of socio-economic deprivation increase the risk of exposure to air-pollution and weather variations resulting in elevated mortality and hospital admission rates in Europe [ 62 , 66 , 76 ] and in other parts of the world including New Zealand [ 22 ], United States of America [ 119 ], and Chile [ 120 ].
Educational attainment was also considered by some of the reviewed studies as an effect modifier, with higher risks detected among individuals with lower educational attainment [ 25 , 63 , 64 , 121 , 122 ]. Despite the consideration of age, gender, education, and wealth effect in the association of air-pollution and weather with mortality and hospital admissions in Europe, our scoping review revealed the lack of investigation into the role of other important socio-demographics such as ethnicity. Research has extensively shown that ethnic minorities live in more disadvantaged communities and have lower socio-economic status as well as poor housing conditions. This results in higher risk for chronic health problems associated with higher exposure on one hand and with lower access to quality healthcare on the other hand [ 22 – 24 ].
Finally, it is worth to note that most of the reviewed studies with a time-series or case-crossover design adjusted their analysis for the season effect [ 40 , 46 , 52 , 54 , 60 , 71 , 73 , 74 , 123 , 124 ]. It is well established that air-pollution, temperature, and other weather variables vary with seasons [ 125 – 127 ]. Not to mention that the emission, formation, and dispersion of air pollutants is affected by seasonal weather variations which in turn affects the individual exposure levels [ 128 ]. Outdoor activities and daily habits (eg. Window ventilation of houses) might also vary depending on the season which reflect changes in the level and duration of individual exposure to air-pollution and weather changes [ 129 ].
Despite the value of this scoping literature review, it has some limitations. First, the employed search strategy was limited to original articles published in peer reviewed journals which might have led to the omission of unpublished work or articles that were published in non-indexed journals. Nevertheless, our search strategy involved navigation through two databases which enables a good catch of major published studies addressing the effect of air-pollution and weather exposure on mortality and hospital admission. Second, limiting our inclusion criteria only to English language articles might have resulted in missing some research written in other languages. However, as most of the literature worldwide is published in the English language, we believe that no major papers have been excluded. Third, this review was limited only to quantitative research which would have led to missing out other type of important research including opinion research pieces and letters to editor as well as qualitative research studies. Opinion research pieces and letters to editor provide a critical appraisal/discussion for the findings of original studies which warrant future research development. Qualitative studies provide an overview about the effect of air-pollution or weather variations on human health from the perspective of lay people rather than relying only on objective census/statistics numbers as in quantitative research. Forth, due to resources limitations, title and abstract screening as well as data abstraction were done only by one researcher (MA). Nevertheless, a second researcher performed title and abstract screening for a random sample of 20% of the retrieved records. Given the high consensus between the two researchers, we are confident of the exact application of the inclusion and exclusion criteria. Our goal from this literature review was not to produce a numerical estimate but rather to give a narrative summary on the effect of air-pollution/weather on mortality/hospital admission. Hence, missing some studies would not be a major concern for this scoping review.
Literature gaps and implications for future research
This scoping review helped us to identify literature gaps that require further research.
First, this review revealed the extensive research carried out to determine the effect of air-pollution on human health. Yet, due to the high correlation between air pollutants and the issue of collinearity in multivariate models, most of the studies examined the effect of single pollutants on mortality and hospital admission outcomes. Nevertheless, the issue of correlation between air pollutants is highly contextual and it depends on the study settings including the season and the specific geographical area. Hence, future researchers should try to examine the effect of multi-pollutants on mortality and/or hospital admission in one model, where strong correlations between the air pollutants are absent.
Second, the majority of studies examined the direct effects of air-pollution and weather exposure on mortality and hospital admission without considering the role of certain effect modifiers. The examined effect modifiers considered mostly by the literature involve age, gender, education, socio-economic deprivation, and season. Therefore, there is a lack of evidence regarding the modifying effect of other individual factors such as previous disease conditions and ethnicity which affect the person’s health vulnerability. Indeed, future research is needed to find out the reasons behind elevated individual’s susceptibility to the detrimental effects of air-pollution and weather variations in certain groups of population.
Third, our review showed that most of the studies either investigated the effect of air-pollution or the effect of weather on mortality and hospital admission. The formation and dispersion of air pollutants depends highly on the existing atmospheric conditions such as temperature, humidity, and wind speed [ 130 ]. Therefore, future studies should consider examining the effect of both, weather conditions and air-pollution, on human health through interaction terms or adjustments in the analysis models.
Fourth, although extensive research has been performed to study the effect of particulate matter and nitrogen oxides pollution on human health, there was a lack of research with respect to other air pollutants including carbon monoxide, ozone, and sulphur dioxide. This might be due to the absence of rigorous and reliable measurements of these pollutants or due to the complexity of analysing the effect of these pollutants.
Fifth, literature is more focused on examining the effect of temperature on mortality and hospital admission, placing less emphasis on other weather exposures. Hence, future research should shift the focus toward other weather exposures such as wind speed, rainfall, humidity, snow cover, daylight, and air pressure.
Sixth, there was a lack of research examining the effect of air-pollution and weather on hospital admission. Mortality was the major outcome in most of the reviewed studies due to the ease of data access governed by less ethical considerations. Additionally, analysis is more straightforward given that it occurs only once in an individual’s life. Thus, it is recommended for future research to consider the impact of air-pollution and weather variables on hospital admission on its own and in combination with mortality through multistate modelling.
Finally, the majority of studies in this field employ the time-series design which uses aggregated mortality and hospital admission data linked to environmental exposures at the local authorities or municipalities level. Research that uses aggregated data neglect the physiological and socio-economic differences among individuals. Additionally, assigning air-pollution and weather exposure based on wide geographies overlook the small geographical exposure differences biasing the drawn estimates. Therefore, there is a need for cohort research studies that utilize individual level data linked to air-pollution and weather exposure at small geographical spatial resolution (eg. Postcodes).
In summary, our scoping review showed that air-pollution and weather exposure beyond certain thresholds lead to various impacts on human health, most commonly cardiovascular and respiratory problems, resulting in increased rates of mortality and hospital admission. Yet, further research is needed given that the effect modification of important socio-demographics such as ethnicity and the interaction between air-pollution and weather is often missed in the literature. Understanding this should give enough evidence to the policy makers to plan and act accordingly aiming to reduce the effects of air pollution and weather variations on the public health. Additionally, research should focus on projecting future health behaviour and mortality patterns in relation to air pollution and weather variations, in order to guide effective environmental and health precautionary measures planning.
Supporting information
S1 checklist. prisma checklist followed for this systematic scoping review data searching, screening, and abstraction..
https://doi.org/10.1371/journal.pone.0241415.s001
S1 Table. The search codes used in PubMed and Web of Science databases for this scoping literature review.
https://doi.org/10.1371/journal.pone.0241415.s002
S2 Table. A detailed summary of the characteristics of the 112 studies included in this scoping review by the type of investigated health outcome.
https://doi.org/10.1371/journal.pone.0241415.s003
S3 Table. A detailed summary of the reported associations between air pollution and/or weather exposures and mortality and/or hospital admission outcomes in the 112 reviewed studies in terms of coefficients and 95% confidence intervals.
https://doi.org/10.1371/journal.pone.0241415.s004
Acknowledgments
The authors would like to thank the University of St Andrews Library services for helping in developing the search codes used in this scoping literature review.
- View Article
- PubMed/NCBI
- Google Scholar
- 3. World-Bank. Cities and Climate Change: An Urgent Agenda. Washington DC: The World Bank; 2010.
- 5. WHO. Ambient (outdoor) air pollution: World health organization (WHO); 2018 [Available from: https://www.who.int/news-room/fact-sheets/detail/ambient-(outdoor)-air-quality-and-health
- 27. European-Commission. EU action against climate change: Leading global action to 2020 and beyond Luxembourg: Office for Official Publications of the European Communities; 2009. Contract No.: KH-78-09-725-EN-C.
- 41. Samet J, Jaakkola J. The epidemiologic approach In Air pollution and health (Holgate ST, Samet JM, Koren HS and Maynard RL, Eds.). Academic Press, London; 1999.
- Research article
- Open access
- Published: 28 November 2017
A systematic review of data mining and machine learning for air pollution epidemiology
- Colin Bellinger ORCID: orcid.org/0000-0002-3567-7834 1 ,
- Mohomed Shazan Mohomed Jabbar 1 ,
- Osmar Zaïane 1 &
- Alvaro Osornio-Vargas 2
BMC Public Health volume 17 , Article number: 907 ( 2017 ) Cite this article
27k Accesses
152 Citations
12 Altmetric
Metrics details
Data measuring airborne pollutants, public health and environmental factors are increasingly being stored and merged. These big datasets offer great potential, but also challenge traditional epidemiological methods. This has motivated the exploration of alternative methods to make predictions, find patterns and extract information. To this end, data mining and machine learning algorithms are increasingly being applied to air pollution epidemiology.
We conducted a systematic literature review on the application of data mining and machine learning methods in air pollution epidemiology. We carried out our search process in PubMed, the MEDLINE database and Google Scholar. Research articles applying data mining and machine learning methods to air pollution epidemiology were queried and reviewed.
Our search queries resulted in 400 research articles. Our fine-grained analysis employed our inclusion/exclusion criteria to reduce the results to 47 articles, which we separate into three primary areas of interest: 1) source apportionment; 2) forecasting/prediction of air pollution/quality or exposure; and 3) generating hypotheses. Early applications had a preference for artificial neural networks. In more recent work, decision trees, support vector machines, k-means clustering and the APRIORI algorithm have been widely applied. Our survey shows that the majority of the research has been conducted in Europe, China and the USA, and that data mining is becoming an increasingly common tool in environmental health. For potential new directions, we have identified that deep learning and geo-spacial pattern mining are two burgeoning areas of data mining that have good potential for future applications in air pollution epidemiology.
Conclusions
We carried out a systematic review identifying the current trends, challenges and new directions to explore in the application of data mining methods to air pollution epidemiology. This work shows that data mining is increasingly being applied in air pollution epidemiology.
The potential to support air pollution epidemiology continues to grow with advancements in data mining related to temporal and geo-spacial mining, and deep learning. This is further supported by new sensors and storage mediums that enable larger, better quality data. This suggests that many more fruitful applications can be expected in the future.
Peer Review reports
The decreasing costs of remote sensors for measuring airborne agents, along with the increasing availability of environmental and clinical data, has led to an explosion in the number of pollution datasets available for analysis. These datasets often have a very large number of samples and tend to have a significant number of variables with mixed degrees of dependencies. These big datasets come with complexity that renders it difficult to rely on traditional epidemiological or environmental health models to analyze them. As a result, new methods of analysis are required in order to advance our understanding of the data. Data mining and machine learning methods from computing science present a wide array of scalable and reliable methods that have performed well on similar problems in other domains. This has inspired a burgeoning field of research within Environmental Health aimed at the adoption of data mining methods to analyze modern, big datasets in air pollution epidemiology inefficient and effective ways.
Data mining is the computational process that is often applied to analyze large datasets, discover patterns, extract actionable knowledge and predict outcomes of future or unknown events. Methods used in this process come from a combination of computational disciplines including Artificial Intelligence, Statistics, Mathematics, Machine Learning, and Database Systems. Apart from the core methods used to carry out the analysis, the process of data mining can involve various preprocessing steps prior to executing the mining algorithm. In addition, a post-processing stage is typically employed to visualize the results of the analysis (i.e. recognized patterns or retrieved information) in an intuitive and easy-to-communicate manner. In this review, we limit our scope to focus on core data analysis techniques as they have been applied to the field of air pollution epidemiology and reported within the air pollution epidemiology literature.
In a broad sense, there are two major paradigms of algorithms: prediction and knowledge discovery. Within these, there are four sub-categories: 1) Classification and regression, 2) Clustering, 3) Association Rule Mining, and 4) Outlier/Anomaly Detection. In addition, there are some relatively new and exciting areas of data analysis, such as spatial data mining and graph data mining, that have been made possible via the building blocks of data mining methods.
According to the best of our knowledge, there are no studies that investigate the depth and breadth of the application of data mining methods within air pollution epidemiology. With this in mind, we perform an investigation to identify which data mining methods have been applied, and to which areas of air pollution epidemiology they have been applied to. Our goal is to point domain researchers to preexisting data mining applications in their areas, and related areas, as well as advance their understanding of the potential of data mining and inspire them to explore further research avenues.
Methodology and paradigms of data mining algorithms
Data mining algorithms are particularly beneficial on complex datasets with a large number of variables and samples. With respect to knowledge discovery, they add insight into high-dimensional problems where traditional statistical methods often fail. Similarly, machine learning algorithm can induce accurate predictor functions from complex, high-dimensional datasets where statistical and mathematical methods, such as regression, can be prone to inaccuracies and be difficult to apply due to their underlying assumptions.
Considerations for applying data mining
In order to implement a successful data mining solution, the user must analyze and formalize their objective. The problem objective guides the user to the appropriate paradigm of learning algorithm. If the objective is to identify hidden groups in data or identify associations between key variables in the data, the users are interested in knowledge discovery and will want to select a clustering or association mining algorithm. Alternatively, the objective might be to induce a predictive model that can classify samples as belonging to a particular category, such as poor air quality, or a real-valued outcome, such as the air quality index.
A large and growing number of algorithms belong to the prediction paradigm and the knowledge discovery paradigm. How to choose between the methods within each paradigm is a topic in its own right. To assist practitioners that are new to the application of machine learning algorithms, Domingos discusses the some of the key considerations in [ 1 ].
When making this decision, the user should consider the complexity of the problem and the amount of data available. A simple, linear classifier, for example, will be ineffective on a complex non-linear classification problem. A large volume of data will facilitate the use of advanced learning algorithms, such as deep artificial neural networks [ 2 ], however, it also forces users to consider questions related to storage, memory and training time.
In general, it is widely understood that there is no silver bullet when it comes to learning algorithms. From an application perspective, a good practice is to select a small, diverse set of algorithms from the paradigm of relevant methods, test them individually and select the one that best meets the performance objectives. Alternatively, grouping a diverse set of models to form an ensemble of predictors has been demonstrated to be an effective solution in theory and practice [ 3 ]. Within the surveyed literature, for example, [ 4 ] applied an ensemble formed of neural networks, support vector machines, Gaussian processes, decision trees and random forests.
Once a set of potential algorithms has been selected, the models that are induced by each algorithm over the available dataset must be evaluated in order to select the one model, or ensemble, that is most likely to preform the best on the prediction task in the future. This is an area of research that is presented in Error Estimation for Pattern Recognition [ 5 ] and Evaluating Learning Algorithms [ 6 ].
The paradigms of learning that have most widely been applied in air pollution epidemiology can be categorized as prediction-based or knowledge discovery methods.
Value prediction
Value prediction is a common and widely applicable area of data mining in which the objective is to take in a set of variables related to an instance drawn from an underlying sample population and predict the corresponding value. Depending on the nature of the application, the user will choose either a data mining algorithm that makes categorical predictions (a classifier) or numeric predictions (similar to regression.) Typical classification algorithms include decision trees, Bayesian classifiers, support vector machines and multilayer perceptrons. Artificial neural networks, support vector regression and regression trees are typical data mining methods for performing numeric predictions. The standard approach to select the most appropriate method for a given problem, such as a classification problem, is to perform repeated trials with multiple classifier algorithms and select the approach that performs the best on the learning problem.
More formally, prediction algorithms are typically induced through a process of supervised learning. The objective is, thus, to make predictions y about instances x of the target problem. For this, a parametrized function \(\mathcal {F}: x \rightarrow y\) is induced. The prediction problem can be one of discrete value prediction, such as classifying breast cancer, or continuous value prediction, much like regression.
In order to perform supervised learning, a dataset X of examples, such as patient information, and corresponding values (or labels) Y , are compiled and used for model induction. Each row of X is a feature vector x =( x 1 , x 2 ,..., x n ). The features x i are equivalent to the data variables in a statistical context. The label set, Y ∈ { y 1 , y 2 ,... y n }, specifies the value that each corresponding instance x i takes. In discrete prediction tasks, the class labels are typically mutually exclusive but do not necessarily have to be [ 7 ]. For continuous values prediction, the value space typically involves real numbers, \(Y \in \mathcal {R}\) , but can also apply to integers, \(Y \in \mathcal {I}\) .
Decision trees, Bayesian methods, support vector machines and artificial neural networks are the most common supervised learning algorithms. We provide a brief overview and direct the reader to [ 8 ] for a detailed description of these algorithms.
Decision trees are simple, but an often effective form of learning classifiers, regressors, and rules. The induction process applies a divide-and-conquer strategy which partitions the data space based on the feature values. Decision trees are often preferred over the more sophisticated models that we discuss below in fields such as medicine because the decisions leading to their predictions can be understood by humans. A very simple example of the interoperability comes from a hypothetical flu classifier which makes predictions { FEVER = TRUE ∧ HEADACHE = TRUE ∧ COUGH = TRUE → FLU = TRUE }.
The standard tree induction algorithms are CART, ID3 and C4.5 [ 9 – 11 ]. Decision trees are induced in a top-down manner by recursively selecting a feature that best divides the training instances according to their labels. A notion of purity known as information that is measured in units of bits is commonly used to measure purity in the determination of the best feature f i at the current level l i . Branches from level l i to level l i −1 are then created; one branch is made for each potential value of f i . The training set is partitioned based on the branches from l i to l i −1 and the process is repeated for each node in level l i −1 . The recursive process stops when the leaves only contain instances from a single class. It should be noted, however, that a form of pruning must be applied to the tree to avoid overfitting.
Artificial neural networks are a powerful form of learning algorithm with a long tradition in pattern recognition and machine learning. Their foundation comes from mathematical attempts at replicating information processing in biological systems [ 12 ]. In modern applications, however, they deviate significantly from the roots of their biological inspiration.
With modern memory and processing power, there is a great potential for complex artificial neural network architectures such as convolutional networks and recurrent network that have seen recent success in deep learning [ 2 ]. The standard architecture, however, is a feedforward network known as a multilayer perceptron. The name refers to the fact that the network is a directed graph that is typically composed of three or more layers. The nodes in the first layer are connected to the nodes in the second layer and so on. The first layer is the input layer. This is where the feature vector x enters the network. It is passed successively through the layers of the network until it reaches the final layer, the output layer. The layers between the input and the output layers are known as hidden layers. Each hidden layer is composed of a user-specified number of hidden units (the nodes in the directed graph).
For each unit i of each hidden layer l , the value of the unit \(h^{(l)}_{i}\) is calculated as the values of the units connected to \(h^{(l)}_{i}\) from the layer below as:
where i is the number of units in the previous layer, j is the specifies the unit in the current layer, ω ji is the parametrized weights connecting layer l −1 to the current layer, l , for unit j , and b l is the bias applied to the current layer.
An activation function is applied to hidden value \(\mathbf {h}^{(l)}_{j}\) . The choice of a non-linear activation, such as sigmoid , enables the model to learn a non-linear representation of the data. However, regularized linear units have recently been found to be useful in the hidden layers [ 13 ].
Multilayer perceptrons are typically trained via back-propagation with gradient descent. This involves updating the weights of the network over multiple iterations of the training set. This is a non-convex optimization process, and thus, training may get stuck in local minima. In practice, however, the models have been found to be very effective.
Support vector machines (SVM) are a powerful method for solving classification and regression problems based on the calculation of the maximum margin hyperplane [ 14 , 15 ]. For non-linear SVM, the data is mapped to a higher dimensional space via a user-specified kernel, such as a polynomial kernel or a radial basis function. The maximal margin hyperplane is implicitly found in this higher dimensional space, the result of which can be a non-linear decision boundary in the original space. A key property of SVM is that model induction is a convex optimization problem. As a result, any local minima is also a global minima.
The maximum margin classifier is of the form y ( x )= w T θ ( x )+ b , where x is a query instance, w is the maximum margin hyperplane, θ is a kernel function, and b is an offset.
The maximum margin hyperplane is solved via:
where x n and y n are the training instances and labels. Directly solving this optimization problem is very complex, however, it can be converted to a simpler, but equivalent problem using the Lagrangian dual which is solvable via quadratic programming. Finally, for kernels satisfying the property k ( x i , x j )= θ ( x i )· θ ( x j ) the kernel trick is used to avoid performing the computations in the kernel-space.
Knowledge discovery
Clustering algorithms are a form of knowledge discovery performed via unsupervised learning. They group the instances of a dataset X into k clusters based on an algorithm specific notion of similarity. The process is termed unsupervised because the algorithms do not use a label set for learning. As a result, the process is one of knowledge discovery that infers the groupings from the data.
Similar to classification and regression, a wide variety of clustering algorithms have been developed. Selecting the right algorithm is domain dependent. Nonetheless, the k-means algorithm remains one of the most prominent clustering techniques. It is often preferred for its simplicity and theoretical foundation.
K-means employs an iterative process of updating the cluster centres that repeats until convergence. The k in k-means refers to the user-specified number of clusters. Initially, the k centres are set at random. Subsequently, each instance in X is assigned to the cluster of its nearest centre. The k centres are then updated to be at the centre of their assigned group. Convergence occurs when the centres stop moving.
In spite of its popularity, k-means has some well-known weaknesses, such as susceptibility to outliers. The Density-based clustering algorithm DBSCAN is an alternative method designed to account for noisy instances and outliers. In addition, one can manufacture scenarios in which k-means will fail to define good clusters under certain conditions.
Hierarchical clustering is a form of distance-based clustering that creates hierarchies of clusters. The clusters are either built agglomeratively or divisively. The former commences by assuming each instance of X belongs to its own cluster and builds up the hierarchy by successively merging clusters. Alternatively, the divisive approach starts with all instances in one big cluster and recursively splits the clusters into smaller clusters down the tree. This form of clustering is very effective for visualizing the groupings and different levels of granularity.
Association rules are similar to the rules extracted from decision trees and produced by rule-based classifiers. The key difference is that in association rule mining, the notion of class categories is not utilized in the rule induction process.
In association rule mining, a dataset X is given in which the rows are instances and the columns are the feature, F ∈ { f 1 , f 2 ,..., f n }, that quantify the instances. In medical domains, the features could be has _ cough ∈ { yes , no }, fever _ level ∈ { none, low, medium, high }, has _ headache ∈ { yes, no }, etc .
Through association rule mining we aim to generate a set of interesting rules from X of the form A → B , where A ⊂ F and B ⊆ F . In contrast, rule-based classifiers learn rules of the form A → B , where A ⊂ F and B ∈ Y ; here, Y is the set of possible class labels.
Given the definition of an association rule, any unique combination of the features, F , can appear on the left side and the right side of the implication. As a result, an enormous number of rules can be generated. Many of these, perhaps the majority, would be uninteresting according to any reasonable assessment. Thus, the rules must be filtered or pruned, as to only keep the valuable rules. Individually assessing each rule in a brute-force manner is prohibitive, and thus, more efficient methods of rule induction have been developed.
The APRIORI algorithm is the most common technique of association mining [ 16 ]. The key to their strategy is the employment of an iterative process that builds up frequent item sets and association rules from their simplest form (one-item sets) to the complex (two-item sets, three-item sets,..., n-item sets). An example of a one-item set and a two-item set from our medical domain is has _ cough = yes , and has _ cough = yes ∧ fever _ level = none . The items are deemed to be frequent if they have more than a user-specified number of necessary occurrences s in the dataset.
The algorithm gains its efficiency from the realization that if a one-item set, such as has _ cough = yes , is not frequent in the dataset, then no two-item set including the one-item set, such as has _ cough = yes ∧ fever _ level = none , can be frequent. Therefore, the algorithm can ignore all higher-order rules involving has _ cough = yes . In general, the algorithm commences by finding all frequent one-itemsets and then finds candidate two-items sets from the frequent one-item sets. The two-itemsets that are frequent are kept, and the process repeats until some point, k , is reached where no k -itemsets are frequent.
In the last stage, all of the frequent itemsets are used to form association rules. The frequent item set A 1 ∧ A 2 ∧ A 3 , for example, generates A 1 → A 2 ∧ A 3 , A 1 ∧ A 2 → A 3 , etc . A similar bottom-up methodology is applied here to efficiently generate rules that meet the minimum frequency requirement.
We have undertaken this survey in a systematic manner guided by the work of Kitchenham in [ 17 ] and the PRISMA standards [ 18 ]. Accordingly, the strategy for conducting this survey is detailed in the following sub-sections. In addition, we have taken motivation for the organization of this survey from a related survey on dengue disease surveillance [ 19 ].
Research questions
The primary research questions considered in this survey are:
R1 To what degree has data mining been applied in air pollution epidemiology?
R2 Are there any hotbeds of this research area?
R3 To which sub-fields of air pollution epidemiology has data mining been applied?
R4 Which data mining methods have been applied?
R5 What are the limitations of the current work?
R6 What potentially fruitful directions remain unexplored?
With respect to R1, we searched the relevant epidemiological literature for research employing data mining techniques. We did not place any bounds on the dates, however, it is clear that the active period is relatively small. Moreover, there is an upward trend in the frequency as the benefits of data mining become more widely known, and tools that lower the barriers to use are made available.
Following from R1, R2 considered if the existing research is uniformly spread around the countries and institutions of the world, or if particular countries and institutions have a more keen focus on researching this area.
To address R3, we filtered through the identified articles to find any reasonable sub-categorization of the epidemiological work in terms of the application areas. This process revealed three categories of epidemiological studies of air pollution in the literature involving data mining.
In R4, we looked to see which paradigms, and which algorithms, have been applied in the air pollution epidemiology literature. From this vantage point, we found that four classes of methods have been applied.
For research question R5, we considered if, given the objectives, the data and/or the mining algorithms applied had any limitations. Given our backgrounds in data mining, we were particularly focused on the data used, algorithms applied and the processes by which the methods were evaluated.
Finally, in R6 we considered the reasonable next steps. Once again, our consideration here took a data mining perspective. To this end, we were interested in identifying new ways of using the existing data and cutting edge data mining algorithms that should be tested within this research domain.
Search process
We performed a temporally unbounded search for articles listed in the PubMed database 1 , the Public Library of Science (PLOS) 2 and Google Scholar 3 . This includes articles published up to the time of writing in October 2017.
The articles reported herein result from a three-part search procedure. This involved: a ) a query-based search to produce a long list of potential articles designed and conducted by CB and MSMJ, b ) a fine-grained manual evaluation of the long-listed articles by one author performed by CB and MSMJ, and c ) identified articles were reviewed by the remaining authors (AOV and OZ). The queries applied to the database and with the number of articles returned are reported in Table 1 .
We excluded articles that did not go through a peer review process in recognized biomedical publication, and articles that did not apply one or more data mining algorithms. Many environmental health articles, for example, mention, and/or discuss, the potential for data mining but did not applying data mining methods. Articles that discuss data mining in the future work were returned by our queries, but are not appropriate for inclusion in our survey.
Data extraction and synthesis
The following information was extracted from each of the selected articles:
The source (journal or conference) and full reference.
A summary of the objective of the study.
The air pollutants of interest in the study.
The data mining method applied to achieve the objective.
A summary of the findings of the study.
This information was extracted by CB and MSMJ and validated by AOV and OZ. Any disagreements were handled via discussion and common consensus. After the raw details of the articles were tabulated, data synthesis was performed. In addition, AOV extracted information about each article related to the biomedical objectives.
Data synthesis involved analyzing the objectives, data mining methods, and the target pollutants in order to identify categories to effectively group the various studies. This exercise was performed by CB and reviewed by the remaining authors. Our goal in the categorization was to identify a hierarchy of categories that provided a sketch of the research landscape. In addition, the purpose was to facilitate quick and easy locating of the studies that are related to the reader’s area of interest. The identified categories are listed below:
Physical Area
Outdoor (Rural, Urban and General 4 )
Forecasting and Prediction
Source Apportionment
Hypothesis Generation
Data Mining Method
Classification
Association Mining
Aspects of data mining in air pollution epidemiology
Environmental setting: overview.
In this section we discuss the target areas of interest (the environmental setting). We have separated these into indoor, outdoor and general. Indoor refers the studies focused on indoor air pollution, such as air pollutants measured within the home or workplace. Outdoor refers to studies interested in outdoor air pollution, such as air pollution measured at a specific intersection or the dispersion of pollutants across an area of interest. It can be further separated into urban, metropolitan and rural. Given that the current breadth of research is still relatively sparse, we focus on the top level of abstraction in this article. We note, however, that a large portion of the research in the outdoor category has been applied to urban and/or metropolitan settings. This is, perhaps, not surprising given that the high population density in metropolitan areas can lead to high impact research. Nonetheless, it suggests rural environments as a potential direction for future work.
The general category covers research that applies data mining methods to study the health impacts of combinations of chemicals common in air pollution. These studies were typically conducted in laboratory settings rather than in the field (or relying on data collected from the field). Table 2 includes a categorized list of articles in relation to their environmental settings.
Categorized study objectives: overview
We grouped the selected articles into the following general study objectives: forecasting and prediction, source apportionment and hypothesis generation. A large percentage of the articles identified in our survey dealt with forecasting or predicting pollution levels based on various climatic and/or pollutant values. These studies considered: a ) forecasting future pollution levels at a specific location given some specific data for that location, b ) forecasting current pollution levels at a specific site given some regional data, and c ) forecasting the geo-spatial distribution of air quality or the spread of pollutants.
Closely related are the studies that were designed to predict increases in sickness or hospitalization from climatic and pollution measurements or to classify sickness in individuals given an air quality or pollution assessment.
Studies classified into the source apportionment category aimed to trace a given decrease in air quality or increase in a given pollutant back to its emission source given a set of pollutant and climatic variables.
Finally, a large number of articles performed hypothesis generation. These studies take in the wide variety of data available about the evolution of air pollution at a specific location, its spread across a region or the globe, health indicator variables, etc ., and use data mining algorithms to identify hidden associations between the variables. These associations are used to test existing assumptions and generate new ones. Exemplary associations might indicate that a certain chemical combination X,Y,Z is associated with increased volume in the emergency department at a hospital of interest, or that climatic conditions W,R combined with heavy seaport traffic, lead to a decrease in the air quality index. These associations can serve to motivate focused trials to study the discovered relationship in depth.
Table 3 includes the articles in a list sorted according to the objectives of the research. It is worth noting that a given article may have more than one objective, and thus, may appear multiple times in the table.
Summary statistics
Prisma results.
The summary statistics recording the numbers of articles returned from our search process, excluded, and included are presented in the PRISMA flow chart in Fig. 1 . Our initial search returned 400 articles. In addition to these, one article ([ 20 ]) was suggested during the review process. After the initial screening and eligibility assessment, 47 articles were included in this survey.
PRISMA flow diagram. Overview of the PRISMA results from our search process
Regional and temporal overview
We have found that eighteen of the studies were from Europe and the UK, sixteen were from the USA, ten were from China, and four were from other Asian countries. The detailed breakdown of this is provided in Fig. 2 . The papers were published between 2000 and October 20, 2017. Figure 3 illustrates a strong upward trend in recent years. We believe this to be owing to better access to data and computing power, along with a growing awareness and access to data mining tools that are accessible to users outside of the data mining community. These tools include the Weka data mining software, which enables users to directly apply data mining algorithms to their data through Java interfaces or a graphical user interface [ 21 ].
Publications Per Country. The number of publications per country identified a predominance in the filed by European countries, the USA and China
Publications Per Year. Number of articles per year between January 2000 and October 2017. We identified an apparent tendency of an increased number of publications on data mining and epidemiology in recent years
Study objectives
The summary statistics for the study objectives are as follows: sixty percent of the study objectives were to forecast or predict epidemiological values/outcomes, such as the AQI or increases in emergency room visits. Thirty percent performed hypothesis generation. This included objectives, such as learning from the data, in which combinations of variables are associated with increases in hospitalization, and understanding which combination of meteorological variables are associated with a degradation in air quality due to emissions from neighbouring cities. Finally, ten percent of the studies focused on source apportionment.
Data mining paradigm
We identified that classification, regression, clustering and association mining algorithms have been applied. Classification and regression relate to prediction and forecasting objective, whereas clustering and association mining generally apply to hypothesis generation and source apportionment.
Table 4 includes the articles in a list sorted according to the objectives of the research. Data mining methods for performing numeric predictions, such as regression and classification, were most widely applied. This area encompassed 59% of the research. Clustering algorithms were applied in 26% of the work, and 15% of the articles employed association mining.
Detailed analysis
Source apportionment.
Table 5 summarizes source apportionment studies employing data mining techniques. These studies explore the impact of chemical emissions and other airborne agents in conjunction with climatological factors [ 22 – 24 ]. They focus on apportioning particular airborne pollutants to potential sources, such as industrial sites, regions and major intersections. These studies have mainly focused on outdoor and urban air pollution as it is the most widely known issue. In particular, principal component analysis (PCA) has been applied to identify correlations and the importance of particular meteorological parameters, traffic, fuel fired equipment and industries in causing air pollution [ 23 – 25 ]. Alternative approaches have utilized clustering-based solutions with correlation analysis to accomplish the task of source apportionment [ 22 , 23 ].
Strengths : The work presented in [ 23 ] proposes to perform enhanced source apportionment and classification. The authors claim that the key to achieving this is in the use of clustering algorithms developed for data mining. The advantage of these is that they are intended for rich, high-dimensional datasets that may include outliers. These factors can be problematic for conventional methods of source apportionment, such as principle component analysis and positive matrix factorization.
Once again, we have identified that the clarity with which the authors present the problem, and then juxtapose the limitation of conventional methods with the potential of data mining approaches, to be a very strong point in this paper. In addition, we appreciate that the authors have gone beyond simply applying standard clustering algorithms, and rather, employed their domain knowledge in order to refine the method in order to develop a superior clustering algorithm for the domain. The authors describe their algorithm, how to set the threshold parameter and the data pre-processing in detail. Crucially, this makes the proposed solution easily implementable by others.
Forecasting and prediction
Tables 5 and 6 summarizes 18 studies which applied machine learning techniques. We observed that investigators are primarily interested in predicting a) the distribution of ambient pollutant concentrations or related measures such as the air quality index (AQI), b) human exposure or c) risk of a health outcome.
According to the above points, the first category consists of 15 studies which either focus on predicting the distribution of particular air pollutants or predicting the quality of air in general. Twenty-seven percent of the above 15 studies (i.e. 4) focus on predicting or forecasting the air quality or air pollution in general. Sixty-six percent of the studies (i.e. 10) are interested in well-known specific air pollutants such as nitrogen oxides (NOx), particulate matter (PM), sulfur dioxide (SO2), carbon monoxide (CO), ozone (O3) and Volatile Organic Compounds VOCs. An interesting, and potentially fruitful, data source utilized by some of the studies that focus on air quality prediction comes from social media posts; social media offers a very rich source of information, which is not typically utilized in scientific analyses of this type [ 26 , 27 ].
Fifty percent of the studies focusing on specific air pollutants use artificial neural networks. Other well-known data mining techniques used include decision trees and support vector machines. In addition, some studies have used ensemble models which are composed of multiple models. The final outcome is determined based on the consensus of the outcome of each model, or some other method of arbitration. Ensemble models have been demonstrated to outperform the base classifiers from which they are composed in a variety of settings. They can be applied to both discrete and continuous value prediction [ 28 , 29 ].
The second category, namely human exposure, consists of 4 studies. These studies focus on identifying regions or exposures, predicting the activities of humans to help understand the exposure better and quantifying the exposure levels.
Interestingly, some of the studies also focus on building better infrastructure to collect data, or on ways to improve the quality of the collected data. This investment can be interpreted as a level of confidence in the application of data mining, and its potential to help shape future research and understanding. The interest in more fundamental problems like data collection and the accuracy of the collected data, in addition to a single focus on building a model based on the available data is very important. Work based on primary questions as such these will ensure high-quality datasets are available in the future, and thus, that better data mining and machine learning models will be possible.
Strengths : The authors in [ 30 ] propose a hybrid system that incorporates a variety of machine learning methods to produce more accurate forecasts and evaluations of air pollution. The authors note that data driven approaches are often more accurate and less complex than model-based approaches, such as chemical transport models, for predicting air quality. Data mining and machine learning-based approaches are data driven methods that are recognized as being powerful forecasting tools. This motivates them as a good choice for the authors. Although we do not see this as being as strong as the previous motivations for applying data mining and machine learning, it is certainly a sufficient reason to consider machine learning.
Hypothesis generation
We observed that many studies (i.e. above 60% of the studies that we have considered) have predominantly applied association rule mining—a primary class of data mining techniques—to generate new hypotheses regarding potential connections between air pollution and adverse health conditions.
From the identified articles, we observed that respiratory disease is an adverse health outcome of interest in these studies. Many studies focusing on respiratory disease are interested in finding out any potential connection between the disease and particulate matter or other airborne pollutants such as SO2 and NOx.
Our results demonstrated that there is a growing interest in generating new hypotheses explaining the connection between a combination of air pollutants and a particular adverse health impact. In [ 31 ], for exampled, the authors used the Bayesian Kernel Machine Regression (BKMR) method, which was recently introduced by epidemiologists. This illustrates the benefit of applying data mining methods to modern epidemiological datasets.
Strengths : The authors in [ 20 ] are interested in generating hypotheses about the joint effect of multiple airborne chemicals on pediatric asthma. Their work demonstrates that classification and regression trees can be used to overcome the challenge presented by multiple chemical interactions when identifying complex joint effects.
We have identified this as a noteworthy paper because the authors are studying a problem that is difficult to solve using conventional epidemiological methods. The paper is strengthened by the fact that the authors clearly justify the ML/DM solution to the problem. In addition, the authors explain why they selected the specific ML algorithm. Finally, this work is an excellent example of how ML/DM algorithms can be augmented and combined with knowledge and practices from the target domain in order to make an accurate and appropriate joint methodology. In particular, the authors demonstrate a refinement to the standard CART algorithm to control for confounding variables. This is important to note because in some applications, data mining practitioners can lose sight of useful, and often necessary, domain knowledge, which hampers the final results. Table 7 summarizes 15 studies which applied data mining techniques to generate new hypotheses to better understand the relationship between air pollution and health.
Challenges and limitations
We have identified a few reoccurring challenges in the surveyed papers. A major theme revolves around data. Many articles, for example, report results from data collected over a short period of time, and from one, or only a few, locations [ 32 , 33 ]. As a result, the findings cannot necessarily be generalized to new locations. This is particularly the case for prediction models trained on local data.
Most real-world data requires preprocessing to combine data sources, remove noise and properly structure the data. The necessities of this may be challenging for domain practitioners. Moreover, certain decisions that must be made during preprocessing can have an impact on the effectiveness of the trained model. Decision trees and association mining algorithms, for example, take categorical variable inputs, whilst continuous variables are common in epidemiology and atmospheric science. Thus, variables, such as temperature, must be converted to discrete categories (low, medium, high) for example [ 33 ]. In many cases, the ideal split points may be unclear. In general, the current literature does not focus on how to best preprocess air pollution epidemiological datasets.
Given the volume of social media data, and the fact that the vast majority of it is irrelevant to the data mining objective, it often has to be filtered. In [ 27 ], for example, keyword filtering is applied to gather relevant micro-blogs from Sina Weibo. How exactly to filter, or process the data, is an open question. A potential new direction here is to apply feature selection or feature extraction [ 2 ].
As noted by [ 34 ], it is important to recognize the limits of your data. Issues, such as granularity and representativeness, can limit what can be discovered from the data. Likewise, when generating association rules to predict outcomes, such as hospitalization or an increase in respiratory disease from weather and pollution data, the training data may not account for all relevant factors. In [ 35 ], it is noted that their data does not account for the accumulative nature of health outcomes.
Other challenges in applying data mining methods include the selection of user-specified parameters for the algorithms. Choosing the ideal number of clusters, for example, is important for performance of clustering algorithms [ 22 ]. In addition, metrics must be used that are appropriate for the target domain. In some cases, suitable evaluation metrics may not exist within the data mining literature, in which case new metrics may be required [ 36 ].
Finally, in many cases practitioners prefer data mining models that produce predictions in a manner that can be easily analyzed and understood [ 32 , 36 ]. This limits the choice of algorithms to rule learners and decision trees, and thus, many of the strongest algorithms are omitted. Perhaps, research focused on making the predictions of artificial neural networks and support vector machines more interpretable could be helpful for the health sciences community [ 37 ].
From an application perspective, we found the discussion of data mining related choices to be limited. Whilst the majority of the articles surveyed contain sufficient details about the algorithms implemented, readers could benefit from a similar level of detail in regards to other key design and implementation decisions. With few exceptions, such as [ 38 ], most of the surveyed articles report results for a single data mining algorithm. Readers would benefit from understanding how and why the specific choice of algorithm was made. As we noted in the overview section on Paradigms of Data Mining Algorithms, it is standard within the data mining community to run trials using a diverse set of algorithms. We often missed a discussion of details regarding which other algorithms were considered, and how they were evaluated. Finally, details regarding how the software was implemented and which data mining packages were used would be valuable to other readers from the air pollution epidemiology community.

Future directions
Deep learning.
Traditional artificial neural networks have proven to be accurate predictors for classification and regression problems. Within this survey, we have found them to be used for predicting global ground level PM 2.5 [ 4 ], predicting air pollution indicators and modeling personal exposure [ 39 ]. In recent years, however, deep learning has elevated the potential for learning with artificial neural networks to new heights. Thus, deep learning methods may also be very fruitful within air pollution epidemiology.
Deep learning is based on standard artificial neural network algorithms but utilizes much larger and deeper networks trained on big datasets. The training process in conjunction with the depth of the networks enables the learning of data abstractions at the different depths. This is found to disentangle complex features. Deep learning methods have been highly effective in areas such as image classification, speech recognition, and other complex problems [ 2 ].
Model selection
Model selection and evaluation are very important aspects of applying machine learning algorithms to real-world applications. However, they often receive less attention than the machine learning algorithms themselves. It is important to consider the breadth of techniques when developing applications in data mining in order to select the right approach for the domain.
For a given machine learning algorithm, model selection refers to the choosing of a parameterized version of the model based on the training data. The key is to select a model that will perform well on unseen data in the future. Once a parametrized model has been selected, the evaluation process provides an estimate of how the model will perform during future application. Some common evaluation metrics are accuracy, root mean square error (RMSE), f-measure and the area under the ROC curve (AUC).
Cross-validation
In the surveyed literature, various forms of cross validation have been applied [ 24 , 26 , 38 ]. In addition to these, various other methods can be applied, each of which has strengths and weaknesses. It is important to select a method that is appropriate for your target domain. Evaluation metrics estimate performance in different ways, and thus, it is important to choose one that is consistent with the target domain. The details of model selection and evaluation are thoroughly discussed in [ 6 ].
- Association mining
Our results demonstrated that much of the research that applied hypothesis generation utilize association mining. These studies typically relied on frequency to identify the associations. It is worth pointing out some alternatives, particularly for scientific domains. Statistical significance test-based methods, for example, have been developed to offer a better assessment of the quality of the association [ 40 , 41 ]. These could be of great benefit to future applications in air pollution epidemiology.
Class imbalance
In a related context, [ 38 ] noted the potential impact of class imbalance, or skewed class distributions, on the performance of machine learning algorithms. Class imbalance is said to occur when one class is significantly less likely, or less frequent, in the training set, than the other class. A detailed discussion of the impacts and potential solutions to class imbalance is undertaken in [ 42 ]. Given that we are often interested in less frequent, or even rare, events in air pollution epidemiology, methods developed for imbalanced learning may have great potential here.
Recent progress in technology and corresponding decreases in the price of computing power has made if possible to measure and store a wide variety of environmental health variables and form them into big datasets. Moreover, social media and other on-line resources provide an entirely new perspective from which to conduct environmental health analyses. These big datasets come with complexities that render it difficult to rely on traditional epidemiological or environmental health models to analyze them. To this end, data mining methods offer great potential to advance our understanding of the causes and impacts of air pollution.
From our survey, we have found a strong increase in the number of articles reporting to apply data mining methods to air pollution epidemiology. We attribute this to the increasing availability of large datasets and computing power, along with the growing awareness of the potential benefits of data mining. In spite of this trend and the potential benefit within the field, to the best of our knowledge, a survey of the existing state-of-the-art has not been performed.
To fill this void, we undertook a study to explore the extent to which data mining has been applied to air pollution epidemiology. This survey is intended for practitioners and researchers alike. We aim to point domain researchers to existing data mining applications within their respective areas, and related areas, as well as advance their understanding of the potential of data mining and inspire them to explore further research avenues.
Our survey illustrates that a wide variety of data mining algorithms have been applied to various sub-fields of air pollution epidemiology. Machine learning algorithms, for example, have been applied both as classifiers and regressors in forecasting and prediction problems. Clustering algorithms, such as K-Means and hierarchical clustering have been applied to knowledge discovery and source appropriation. In addition, a great number of studies have applied association mining for hypothesis generation.
1 see https://www.ncbi.nlm.nih.gov/pubmed
2 https://www.plos.org
3 https://scholar.google.ca
4 General refers to the study of air pollutants not specific to a certain setting.
Domingos P. A few useful things to know about machine learning. Commun ACM. 2012; 55(10):78–87.
Article Google Scholar
LeCun Y, Bengio Y, Hinton G. Deep learning. Nature. 2015; 521(7553):436–44.
Article CAS PubMed Google Scholar
Dietterich TG, et al. Ensemble methods in machine learning. Multiple Classif Syst. 2000; 1857:1–15.
Lary DJ, Faruque FS, Malakar N, Moore A, Roscoe B, Adams ZL, Eggelston Y. Estimating the global abundance of ground level presence of particulate matter (pm2. 5). Geospatial Health. 2014; 8(3):611–30.
Neto UMB, Dougherty ER. Error estimation for pattern recognition. Hoboken: John Wiley & Sons; 2015.
Book Google Scholar
Japkowicz N, Shah M. Evaluating Learning Algorithms: a Classification Perspective. Cambridge: Cambridge University Press; 2011.
Bellinger C, Amid A, Japkowicz N, Victor H. Multi-label classification of anemia patients. In: Proceedings of the IEEE 14th International Conference on Machine Learning and Applications (ICMLA).2015. p. 825–30.
Bishop C. Pattern Recognition and Machine Learning (Information Science and Statistics). 1613-9011. Cambridge: Springer; 2006.
Google Scholar
Breiman L, Friedman J, Stone CJ, Olshen RA. Classification and Regression Trees. Chicago: CRC Press; 1984.
Quinlan JR. Induction of decision trees. Mach Learn. 1986; 1(1):81–106.
Quinlan JR. C4. 5: Programs for Machine Learning. San Francisco: Morgan Kaufmann; 1993.
Rumelhart DE, Hinton GE, Williams RJ. Learning internal representations by error propagation. Parallel Distributed Processing: Exploration of the Micro-structure of Cognition. 1986; 1:1–34.
Maas AL, Hannun AY, Ng AY. Rectifier nonlinearities improve neural network acoustic models. In: Proceedings of the International Conference on Machine Learning.2013.
Vapnik V. The Nature of Statistical Learning Theory. New York: Springer; 1995.
Schölkopf B, Smola AJ. Learning with kernels. Cambridge: MIT Press; 2002.
Agrawal R, Srikant R. Fast algorithms for mining association rules. In: Proceedings of the 20th International Conference on Very Large Data Bases (VLDB).1994. p. 487–99.
Kitchenham B. Procedure for undertaking systematic reviews. Technical report, Computer Science Department, Keele University (TRISE-0401) and National ICT Australia Ltd (0400011T. 1), Joint Technical Report. 2004.
Moher D, Liberati A, Tetzlaff J, Altman D. Preferred reporting items for systematic reviews and meta-analyses: the prisma statement. J Clin Epidemiol. 2009; 62(10):1006–12.
Article PubMed Google Scholar
Runge-Ranzinger S, Horstick O, Marx M, Kroeger A. What does dengue disease surveillance contribute to predicting and detecting outbreaks and describing trends?Trop Med Int Health. 2008; 13(8):1022–41.
Gass K, Klein M, Chang HH, Flanders WD, Strickland MJ. Classification and regression trees for epidemiologic research: an air pollution example. Environ Health. 2014; 13(1):17.
Article PubMed PubMed Central Google Scholar
Hall M, Frank E, Holmes G, Pfahringer B, Reutemann P, Witten IH. The weka data mining software: An update. SIGKDD Explor Newsl. 2009; 11(1):10–18.
Chen HW, Tsai CT, She CW, Lin YC, Chiang CF. Exploring the background features of acidic and basic air pollutants around an industrial complex using data mining approach. Chemosphere. 2010; 81(10):1358–67.
Chen M, Wang P, Chen Q, Wu J, Chen X. A clustering algorithm for sample data based on environmental pollution characteristics. Atmos Environ. 2015; 107:194–203.
Article CAS Google Scholar
Singh KP, Gupta S, Rai P. Identifying pollution sources and predicting urban air quality using ensemble learning methods. Atmos Environ. 2013; 80:426–37.
Thurston GD, Spengler JD. A quantitative assessment of source contributions to inhalable particulate matter pollution in metropolitan boston. Atmos Environ (1967). 1985; 19(1):9–25.
Jiang W, Wang Y, Tsou MH, Fu X. Using social media to detect outdoor air pollution and monitor air quality index (aqi): a geo-targeted spatiotemporal analysis framework with sina weibo (chinese twitter). PloS ONE. 2015; 10(10):0141185.
Wang S, Paul MJ, Dredze M. Social media as a sensor of air quality and public response in china. J Med Internet Res. 2015; 17(3):22.
Freund Y, Schapire RE. Experiments with a new boosting algorithm. In: Proceedings of the Thirteenth International Conference on Machine Learning: 1996. p. 148–56.
Friedman JH. Greedy function approximation: a gradient boosting machine. Ann Stat. 2001; 29(5):1189–232.
Xu Y, Yang W, Wang J. Air quality early-warning system for cities in china. Atmos Environ. 2017; 148:239–57.
Bobb JF, Valeri L, Henn BC, Christiani DC, Wright RO, Mazumdar M, Godleski JJ, Coull BA. Bayesian kernel machine regression for estimating the health effects of multi-pollutant mixtures. Biostatistics. 2014; 16(3):058.
Payus C, Sulaiman N, Shahani M, Bakar AA. Association rules of data mining application for respiratory illness by air pollution database. Int J Basic Appl Sci. 2013; 13(3):11–16.
Kukkonen J, Partanen L, Karppinen A, Ruuskanen J, Junninen H, Kolehmainen M, Niska H, Dorling S, Chatterton T, Foxall R, et al. Extensive evaluation of neural network models for the prediction of no 2 and pm 10 concentrations, compared with a deterministic modelling system and measurements in central helsinki. Atmos Environ. 2003; 37(32):4539–550.
Reid CE, Jerrett M, Petersen ML, Pfister GG, Morefield PE, Tager IB, Raffuse SM, Balmes JR. Spatiotemporal prediction of fine particulate matter during the 2008 northern california wildfires using machine learning. Environ Sci Technol. 2015; 49(6):3887–96.
Zhu W, Wang J, Zhang W, Sun D. Short-term effects of air pollution on lower respiratory diseases and forecasting by the group method of data handling. Atmos Environ. 2012; 51:29–38.
Toti G, Vilalta R, Lindner P, Lefer B, Macias C, Price D. Analysis of correlation between pediatric asthma exacerbation and exposure to pollutant mixtures with association rule mining. Artif Intell Med. 2016; 74:44–52.
Setiono R, Liu H. Understanding neural networks via rule extraction. In: Proceedings of the International Joint Conference of Artificial Intelligence (IJCAI).1995. p. 480–5.
Pandey G, Zhang B, Jian L. Predicting submicron air pollution indicators: a machine learning approach. Environ Sci Process Impacts. 2013; 15(5):996–1005.
Aquilina NJ, Delgado-Saborit JM, Gauci AP, Baker S, Meddings C, Harrison RM. Comparative modeling approaches for personal exposure to particle-associated pah. Environ Sci Technol. 2010; 44(24):9370–6.
Hämäläinen W. Statapriori: an efficient algorithm for searching statistically significant association rules. Knowl Inform Syst. 2010; 23(3):373–99.
Hämäläinen W. Kingfisher: an efficient algorithm for searching for both positive and negative dependency rules with statistical significance measures. Knowl Inform Syst. 2012; 32(2):383–414.
He H, Ma Y. Imbalanced Learning: Foundations, Algorithms, and Applications. Piscataway: John Wiley & Sons; 2013.
Min KD, Kwon HJ, Kim K, Kim SY. Air pollution monitoring design for epidemiological application in a densely populated city. Int J Environ Res Public Health. 2017; 14(7):686.
Article PubMed Central Google Scholar
Keller JP, Drton M, Larson T, Kaufman JD, Sandler DP, Szpiro AA. Covariate-adaptive clustering of exposures for air pollution epidemiology cohorts. Ann Appl Stat. 2017; 11(1):93.
Li D, Liu J, Zhang J, Gui H, Du P, Yu T, Wang J, Lu Y, Liu W, Cheng Y. Identification of long-range transport pathways and potential sources of pm2.5 and pm10 in beijing from 2014 to 2015. J Environ Sci. 2017; 56:214–29.
Stingone JA, Pandey OP, Claudio L, Pandey G. Using machine learning to identify air pollution exposure profiles associated with early cognitive skills among u.s. children. Environ Pollut. 2017; 230:730–40.
Liu BC, Binaykia A, Chang PC, Tiwari MK, Tsao CC. Urban air quality forecasting based on multi-dimensional collaborative support vector regression (svr): A case study of beijing-tianjin-shijiazhuang. PLOS ONE. 2017; 12(7):1–17.
Mirto M, Fiore S, Conte L, Bruno LV, Aloisio G. A spatial data analysis infrastructure for environmental health research. In: Proceedings of the International Conference on High Performance Computing and Simulation (HPCS): 2016. p. 435–42.
Lewis AC, Lee JD, Edwards PM, Shaw MD, Evans MJ, Moller SJ, Smith KR, Buckley JW, Ellis M, Gillot SR, et al. Evaluating the performance of low cost chemical sensors for air pollution research. Faraday Discuss. 2016; 189:85–103.
Reid CE, Jerrett M, Tager IB, Petersen ML, Mann JK, Balmes JR. Differential respiratory health effects from the 2008 northern california wildfires: a spatiotemporal approach. Environ Res. 2016; 150:227–35.
Fernández-Camacho R, Cabeza IB, Aroba J, Gómez-Bravo F, Rodríguez S, de la Rosa J. Assessment of ultra-fine particles and noise measurements using fuzzy logic and data mining techniques. Sci Total Environ. 2015; 512:103–13.
Lary D, Lary T, Sattler B. Using machine learning to estimate global pm2. 5 for environmental health studies. Environ Health Insights. 2015; 9(Suppl 1):41.
CAS PubMed PubMed Central Google Scholar
Qin S, Liu F, Wang C, Song Y, Qu J. Spatial-temporal analysis and projection of extreme particulate matter (pm 10 and pm 2.5) levels using association rules: A case study of the jing-jin-ji region, china. Atmos Environ. 2015; 120:339–50.
Chen J, Chen H, Zheng G, Pan JZ, Wu H, Zhang N. Big smog meets web science: smog disaster analysis based on social media and device data on the web. In: Proceedings of the 23rd International Conference on World Wide Web. ACM: 2014. p. 505–10. http://www.sciencedirect.com/science/article/pii/S1352231015303484 .
Dias D, Tchepel O. Modelling of human exposure to air pollution in the urban environment: a gps-based approach. Environ Sci Pollut Res. 2014; 21(5):3558–71.
Philibert A, Loyce C, Makowski D. Prediction of n 2 o emission from local information with random forest. Environ Pollut. 2013; 177:156–63.
Padula AM, Mortimer K, Hubbard A, Lurmann F, Jerrett M, Tager IB. Exposure to traffic-related air pollution during pregnancy and term low birth weight: estimation of causal associations in a semiparametric model. Am J Epidemiol. 2012; 176(9):148.
Ma Y, Richards M, Ghanem M, Guo Y, Hassard J. Air pollution monitoring and mining based on sensor grid in london. Sensors. 2008; 8(6):3601–623.
Article CAS PubMed PubMed Central Google Scholar
Niska H, Hiltunen T, Karppinen A, Ruuskanen J, Kolehmainen M. Evolving the neural network model for forecasting air pollution time series. Eng Appl Artif Intell. 2004; 17(2):159–67.
Kolehmainen M, Martikainen H, Ruuskanen J. Neural networks and periodic components used in air quality forecasting. Atmos Environ. 2001; 35(5):815–25.
Challoner A, Pilla F, Gill L. Prediction of indoor air exposure from outdoor air quality using an artificial neural network model for inner city commercial buildings. Int J Environ Res Public health. 2015; 12(12):15233–53.
Pegoretti S, Verdi L. Machine learning for the analysis of indoor radon distribution, compared with ordinary kriging. Radiat Prot Dosim. 2009; 137(3-4):324–8.
Bell SM, Edwards SW. Identification and prioritization of relationships between environmental stressors and adverse human health impacts. Environ Health Perspec. 2015; 123(11):1193–9.
Bell SM, Edwards SW. Building associations between markers of environmental stressors and adverse human health impacts using frequent itemset mining. In: Proceedings of the SIAM International Conference on Data Mining: 2014. p. 551–9.
Hu M, Li W, Li L, Houston D, Wu J. Refining time-activity classification of human subjects using the global positioning system. PloS ONE. 2016; 11(2):0148875.
Beckerman BS, Jerrett M, Martin RV, van Donkelaar A, Ross Z, Burnett RT. Application of the deletion/substitution/addition algorithm to selecting land use regression models for interpolating air pollution measurements in california. Atmospheric Environ. 2013; 77:172–7.
Dominici F, Peng RD, Bell ML, Pham L, McDermott A, Zeger SL, Samet JM. Fine particulate air pollution and hospital admission for cardiovascular and respiratory diseases. Jama. 2006; 295(10):1127–34.
Corani G. Air quality prediction in milan: feed-forward neural networks, pruned neural networks and lazy learning. Ecol Model. 2005; 185(2):513–29.
Ghanem M, Guo Y, Hassard J, Osmond M, Richards M. Sensor grids for air pollution monitoring. In: Proc. 3rd UK e-Science All Hands Meeting.Nottingham: 2004.
Download references
Acknowledgments
The authors are grateful for the financial support of CIHR/NSERC.
This work was supported by a Collaborative Health Research Grant for CIHR/NSERC, and the Alberta Institute for Machine Intelligence (AMII).
Availability of data and materials
The datasets generated or analyzed during our survey are available from the corresponding author upon reasonable request.
Author information
Authors and affiliations.
Department of Computing Science, University of Alberta, Edmonton, Canada
Colin Bellinger, Mohomed Shazan Mohomed Jabbar & Osmar Zaïane
Department of Paediatrics, University of Alberta, Edmonto, Canada
Alvaro Osornio-Vargas
You can also search for this author in PubMed Google Scholar
Contributions
CB and MSMJ performed the literature review and gather the data used in this survey. CB and MSMJ performed the initial analysis and paper verification. All authors took part in the final analysis of the articles selected for inclusion in this survey. AOV examined and summarized the objectives of the surveyed articles from a biomedical perspective. Based on this analysis, OZ, CB and MSMJ designed the outline and structure of the survey. CB and MSMJ wrote the paper. All authors read and approved the final manuscript.
Corresponding author
Correspondence to Colin Bellinger .
Ethics declarations
Ethics approval and consent to participate.
Not applicable.
Consent for publication
Competing interests.
The authors declare that they have no competing interests.
Publisher’s Note
Springer Nature remains neutral with regard to jurisdictional claims in published maps and institutional affiliations.
Rights and permissions
Open Access This article is distributed under the terms of the Creative Commons Attribution 4.0 International License( http://creativecommons.org/licenses/by/4.0/ ), which permits unrestricted use, distribution, and reproduction in any medium, provided you give appropriate credit to the original author(s) and the source, provide a link to the Creative Commons license, and indicate if changes were made. The Creative Commons Public Domain Dedication waiver( http://creativecommons.org/publicdomain/zero/1.0/ ) applies to the data made available in this article, unless otherwise stated.
Reprints and permissions
About this article
Cite this article.
Bellinger, C., Mohomed Jabbar, M., Zaïane, O. et al. A systematic review of data mining and machine learning for air pollution epidemiology. BMC Public Health 17 , 907 (2017). https://doi.org/10.1186/s12889-017-4914-3
Download citation
Received : 26 April 2017
Accepted : 14 November 2017
Published : 28 November 2017
DOI : https://doi.org/10.1186/s12889-017-4914-3
Share this article
Anyone you share the following link with will be able to read this content:
Sorry, a shareable link is not currently available for this article.
Provided by the Springer Nature SharedIt content-sharing initiative
- Epidemiology
- Air pollution
- Data mining
- Machine learning
BMC Public Health
ISSN: 1471-2458
- General enquiries: [email protected]
Advertisement
Urban air pollution control policies and strategies: a systematic review
- Research article
- Published: 08 October 2021
- Volume 19 , pages 1911–1940, ( 2021 )
Cite this article
- Ahmad Jonidi Jafari 1 , 2 ,
- Esmail Charkhloo ORCID: orcid.org/0000-0002-7988-7753 1 , 2 &
- Hasan Pasalari 1 , 2
2309 Accesses
26 Citations
4 Altmetric
Explore all metrics
A wide range of policies, strategies, and interventions have been implemented to improve air quality all over the world. This systematic review comprehensively appraises the policies and strategies on air pollutants controls enacted in different countries, worldwide. Three databases, Web of Science, PubMed and Scopus, were used for the search. After screening, a total of 114 eligible manuscripts were selected from 2219 documents for further analysis. Selected articles were divided into two categories: (1) articles focusing on introducing the policies and strategies enacted for controlling air pollution in different countries, and (2) articles which focused on different policies and strategies to control one or more specific pollutants. In the former one, urban air pollution control strategies and policies were divided into four categories, namely, general strategies and policies, transportation, energy, and industry. In case of latter category, policies and strategies focused on controlling six pollutants (PM, SO 2 , NO 2 , VOC S , O 3 and photochemical smog). The results indicated that, the most common policies and strategies enacted in most countries are pertinent to the transportation sector. Changing energy sources, in particular elimination or limited use of solid fuels, was reported as an effective action by governments to reduce air pollution. Overall, most policies enacted by governments can be divided into three general categories: (a) incentive policies such as implementing a free public transportation program to use fewer private cars, (b) supportive policies such as paying subsidies to change household fuels, and (c) punitive policies such as collecting tolls for cars to enter the congestion charging areas. Depending on the circumstances, these policies are implemented alone or jointly. In addition to the acceptance of international agreements to reduce air pollution by governments, greater use of renewable energy, clean fuels, and low-pollution or no-pollution vehicles such as electric vehicles play an important role in reducing air pollution.
This is a preview of subscription content, log in via an institution to check access.
Access this article
Price includes VAT (Russian Federation)
Instant access to the full article PDF.
Rent this article via DeepDyve
Institutional subscriptions
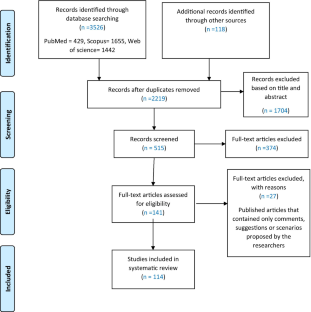
Similar content being viewed by others
How can urban policies improve air quality and help mitigate global climate change: a systematic mapping review, addressing equity in interventions to reduce air pollution in urban areas: a systematic review.
European Best Practices to Mitigate Air Pollution: A Review
Paoletti E, Schaub M, Matyssek R, Wieser G, Augustaitis A, Bastrup-Birk A, et al. Advances of air pollution science: from forest decline to multiple-stress effects on forest ecosystem services. Environ Pollut. 2010;158(6):1986–9.
CAS Google Scholar
LI ZD, Data E, De Dai IY. Japan-china comparative analysis on measures against sulfur dioxides pollution. IEE Japan, Report. 1999.
WHO. Ambient (outdoor) air quality and health 2018. https://www.who.int/news-room/fact-sheets/detail/ambient-(outdoor)-air-quality-and-health . Accessed 2 June 2021
Neidell MJ. Air pollution, health, and socio-economic status: the effect of outdoor air quality on childhood asthma. J Health Econ. 2004;23(6):1209–36.
Google Scholar
Nœss Ø, Piro FN, Nafstad P, Smith GD, Leyland AH. Air pollution, social deprivation, and mortality: a multilevel cohort study. Epidemiology. 2007;18:686–94.
Hoek G, Brunekreef B, Goldbohm S, Fischer P, van den Brandt PA. Association between mortality and indicators of traffic-related air pollution in the Netherlands: a cohort study. Lancet. 2002;360(9341):1203–9.
Bell ML, Ebisu K. Environmental inequality in exposures to airborne particulate matter components in the United States. Environ Health Perspect. 2012;120(12):1699–704.
Chi GC, Hajat A, Bird CE, Cullen MR, Griffin BA, Miller KA, et al. Individual and neighborhood socioeconomic status and the association between air pollution and cardiovascular disease. Environ Health Perspect. 2016;124(12):1840–7.
Deguen S, Zmirou-Navier D. Social inequalities resulting from health risks related to ambient air quality—a European review. Eur J Public Health. 2010;20(1):27–35.
Evans GW, Kantrowitz E. Socioeconomic status and health: the potential role of environmental risk exposure. Annu Rev Public Health. 2002;23(1):303–31.
Afroz R, Hassan MN, Ibrahim NA. Review of air pollution and health impacts in Malaysia. Environ Res. 2003;92(2):71–7.
Anderson HR. Air pollution and mortality: a history. Atmos Environ. 2009;43(1):142–52.
Brunekreef B, Holgate ST. Air pollution and health. Lancet. 2002;360(9341):1233–42.
Lv Y, Huang G, Li Y, Yang Z, Sun W. A two-stage inexact joint-probabilistic programming method for air quality management under uncertainty. J Environ Manag. 2011;92(3):813–26.
Sokhi RS, Mao H, Srimath ST, Fan S, Kitwiroon N, Luhana L, et al. An integrated multi-model approach for air quality assessment: development and evaluation of the OSCAR air quality assessment system. Environ Model Softw. 2008;23(3):268–81.
Sunyer J, Saez M, Murillo C, Castellsague J, Martinez F, Antó JM. Air pollution and emergency room admissions for chronic obstructive pulmonary disease: a 5-year study. Am J Epidemiol. 1993;137(7):701–5.
Svartengren M, Strand V, Bylin G, Jarup L, Pershagen G. Short-term exposure to air pollution in a road tunnel enhances the asthmatic response to allergen. Eur Respir J. 2000;15(4):716–24.
Anjaneyulu M, Harikrishna M, Chenchuobulu S. Modeling ambient carbon monoxide pollutant due to road traffic. World Acad Sci Eng Technol. 2006;17:103–6.
Badami MG. Transport and urban air pollution in India. Environ Manag. 2005;36(2):195–204.
Mashelkar R, Biswas D, Krishnan N, Mathur O, Natarajan R, Niyati K, et al. Report of the expert committee on auto fuel policy. Ministry of Petroleum and Natural Gas, Government of India, New Delhi. 2002.
Molina L, Kolb C, Foy Bd, Lamb B, Brune W, Jimenez J, et al. Air quality in North America’s most populous city–overview of the MCMA-2003 campaign. Atmos Chem Phys. 2007;7(10):2447–73.
Molina L, Molina MJ. Air quality in the Mexico Megacity: an integrated assessment. Dordrecht: Springer Science & Business Media; 2002.
Singh A, Gupta H, Gupta K, Singh P, Gupta V, Sharma R. A comparative study of air pollution in Indian cities. Bull Environ Contam Toxicol. 2007;78(5):411–6.
Wang H, Fu L, Zhou Y, Du X, Ge W. Trends in vehicular emissions in China’s mega cities from 1995 to 2005. Environ Pollut. 2010;158(2):394–400.
Zhou T, Sun J, Yu H. Temporal and spatial patterns of China’s main air pollutants: years 2014 and 2015. Atmosphere. 2017;8(8):137.
Bell ML, Davis DL, Gouveia N, Borja-Aburto VH, Cifuentes LA. The avoidable health effects of air pollution in three Latin American cities: Santiago, Sao Paulo, and Mexico City. Environ Res. 2006;100(3):431–40.
Quraishi TA. Discussion on air pollution abatement strategies for residential solid-fuel burning appliances. Int J Environ Stud. 1988;31(1):19–37.
Stern AC, Professor E. History of air pollution legislation in the United States. J Air Pollut Control Assoc. 1982;32(1):44–61.
Horowitz CA. Paris agreement. Int Leg Mater. 2016;55(4):740–55.
Rogelj J, Den Elzen M, Höhne N, Fransen T, Fekete H, Winkler H, et al. Paris agreement climate proposals need a boost to keep warming well below 2 C. Nature. 2016;534(7609):631–9.
(EC) EC. Council directive 1999/30/EC of 22 april 1999 relating to limit values for sulphur dioxide, nitrogen dioxide and oxides of nitrogen, particulate matter and lead in ambient air. Available online: https://eur-lex.europa.eu/legal-content/EN/TXT/?uri=CELEX:31999L0030 . Accessed on 24 Mar 2016.
(UNECE). 1979 Convention on long-rangetransboundary air pollution. Available online: https://www.unece.org/fileadmin/DAM/env/lrtap/full%20text/1979.CLRTAP.e.pdf . Accessed on 25 Oct 2016.
Hardie RW, Thayer GR, Barrera-Roldán A. Development of a methodology for evaluating air pollution options for improving the air quality in Mexico City. Sci Total Environ. 1995;169(1–3):295–301.
Sousa Santos G, Sundvor I, Vogt M, Grythe H, Haug TW, Høiskar BA, et al. Evaluation of traffic control measures in Oslo region and its effect on current air quality policies in Norway. Transp Policy. 2020;99:251–61.
Moher D, Liberati A, Tetzlaff J, Altman DG, Group P. Preferred reporting items for systematic reviews and meta-analyses: the PRISMA statement. PLoS Med. 2009;6(7):e1000097.
Wu Y, Wang RJ, Zhou Y, Lin BH, Fu LX, He KB, et al. On-road vehicle emission control in Beijing: past, present, and future. Environ Sci Technol. 2011;45(1):147–53.
Zhou Y, Fu LX, Cheng LL. Characterization of in-use light-duty gasoline vehicle emissions by remote sensing in Beijing: impact of recent control measures. J Air Waste Manag Assoc. 2007;57(9):1071–7.
Hao JM, Wang LT, Li L, Hu JN, Yu XC. Air pollutants contribution and control strategies of energy-use related sources in Beijing. Sci China Ser D-Earth Sci. 2005;48:138–46.
Guo SH, Chen LQ. Can urban rail transit systems alleviate air pollution? Empirical evidence from Beijing. Growth Chang. 2019;50(1):130–44.
Yang W, Yu CY, Yuan W, Wu XY, Zhang W, Wang XJ. High-resolution vehicle emission inventory and emission control policy scenario analysis, a case in the Beijing-Tianjin-Hebei (BTH) region. China J Clean Prod. 2018;203:530–9.
Xing J, Zhang FF, Zhou Y, Wang SX, Ding D, Jang C, et al. Least-cost control strategy optimization for air quality attainment of Beijing-Tianjin-Hebei region in China. J Environ Manag. 2019;245:95–104.
Xie HJ, Liu MF, Jiang W. Balanced development and industrialization and urbanization serving as a strategy for pollution agglomeration control. Ekoloji. 2019;28(107):2431–41.
Song XW, Hao YP, Zhu XD. Air pollutant emissions from vehicles and their abatement scenarios: a case study of Chengdu-Chongqing urban agglomeration, China. Sustainability. 2019;11(22):6503.
Wei W, Li P, Wang H, Song M. Quantifying the effects of air pollution control policies: a case of Shanxi province in China. Atmos Pollut Res. 2018;9(3):429–38.
Qiu ZW, Li XX, Hao YZ, Deng SX. Potential of diesel emissions reduction strategies in Xi’an. China Clean Technol Environ Policy. 2016;18(8):2717–24.
Chen X, Yuan ZR. Environmentally friendly traffic control strategy—a case study in Xi’an city. J Clean Prod. 2020. https://doi.org/10.1016/j.jclepro.2019.119397 .
Article Google Scholar
Ahlers AL, Shen YD. Breathe easy? Local nuances of authoritarian environmentalism in China’s battle against air pollution. China Q. 2018;234:299–319.
Li W, Shao L, Wang W, Li H, Wang X, Li Y, et al. Air quality improvement in response to intensified control strategies in Beijing during 2013–2019. Sci Total Environ. 2020;744:140776.
Cui L, Zhou J, Peng X, Ruan S, Zhang Y. Analyses of air pollution control measures and co-benefits in the heavily air-polluted Jinan city of China, 2013–2017. Sci Rep. 2020. https://doi.org/10.1038/s41598-020-62475-0 .
Jin Y, Andersson H, Zhang S. Air pollution control policies in China: a retrospective and prospects. Int J Environ Res Public Health. 2016;13(12):1219.
Wang SX, Hao JM. Air quality management in China: issues, challenges, and options. J Environ Sci. 2012;24(1):2–13.
Zhan J, Wang M, Liu Y, Feng C, Gan T, Li L, et al. Impact of the ‘13th five-year plan’ policy on air quality in Pearl River Delta, China: a case study of Haizhu district in Guangzhou city using WRF-Chem. Appl Sci. 2020;10(15):5276.
Yang X, Teng F. The air quality co-benefit of coal control strategy in China. Resour Conserv Recycl. 2018;129:373–82.
Jiang X, Li G, Fu W. Government environmental governance, structural adjustment and air quality: a quasi-natural experiment based on the three-year action plan to win the blue sky defense war. J Environ Manag. 2021. https://doi.org/10.1016/j.jenvman.2020.111470 .
Tsai WT. Current status of air toxics management and its strategies for controlling emissions in Taiwan. Toxics. 2016. https://doi.org/10.3390/toxics4020008 .
Shaw D, Hung MF. Evolution and evaluation of air pollution control policy in Taiwan. Environ Econ Policy Stud. 2001;4(3):141–66.
Kuo PH, Ni PC, Keats A, Tsuang BJ, Lan YY, Lin MD, et al. Retrospective assessment of air quality management practices in Taiwan. Atmos Environ. 2009;43(25):3925–34.
Rusco FW, Walls WD. Vehicular emissions and control policies in Hong Kong. Contemp Econ Policy. 1995;13(1):50–61.
Cui L, Wang XL, Ho KF, Gao Y, Liu C, Ho SSH, et al. Decrease of VOC emissions from vehicular emissions’ in Hong Kong from 2003 to 2015: results from a tunnel, study. Atmos Environ. 2018;177:64–74.
Yao DW, Lyu XP, Murray F, Morawska L, Yu W, Wang JY, et al. Continuous effectiveness of replacing catalytic converters on liquified petroleum gas-fueled vehicles in Hong Kong. Sci Total Environ. 2019;648:830–8.
Phuntsho S, Kanitpong K. Vehicle emission control strategies and public opinion in Bhutan. Transp Res Rec. 2010;2157:103–10.
Amann M, Purohit P, Bhanarkar AD, Bertok I, Borken-Kleefeld J, Cofala J, et al. Managing future air quality in megacities: a case study for Delhi. Atmos Environ. 2017;161:99–111.
Dholakia HH, Purohit P, Rao S, Garg A. Impact of current policies on future air quality and health outcomes in Delhi. India Atmos Environ. 2013;75:241–8.
Majumdar D, Purohit P, Bhanarkar AD, Rao PS, Rafaj P, Amann M, et al. Managing future air quality in megacities: emission inventory and scenario analysis for the Kolkata Metropolitan City, India. Atmos Environ. 2020. https://doi.org/10.1016/j.atmosenv.2019.117135 .
Peng W, Dai H, Guo H, Purohit P, Urpelainen J, Wagner F, et al. The critical role of policy enforcement in achieving health, air quality, and climate benefits from India’s clean electricity transition. Environ Sci Technol. 2020;54(19):11720–31.
Chelani AB, Devotta S. Air quality assessment in Delhi: before and after CNG as fuel. Environ Monit Assess. 2007;125(1–3):257–63.
Kathuria V. Vehicular pollution control in Delhi. Transp Res Part D-Transp Environ. 2002;7(5):373–87.
Goyal P, Gulia S, Goyal SK, Kumar R. Assessment of the effectiveness of policy interventions for air quality control regions in Delhi city. Environ Sci Pollut Res. 2019;26(30):30967–79.
Nishioka S. Traffic pollution: control policy and research trend. Transp Res Part A. 1989;23(1):73–81.
Botta E, Yamasaki S. “Policies, regulatory framework and enforcement for air quality management: The case of Japan”. 2020; OECD Environment Working Papers, No. 156, OECD Publishing, Paris, https://doi.org/10.1787/b2de0bc1-en .
Rutherford D, Ortolano L. Air quality impacts of Tokyo’s on-road diesel emission regulations. Transp Res Part D: Transp Environ. 2008;13(4):239–54.
Asadollah-Fardi G. Air quality management in Tehran. Kitakyushu Initiative Seminar on Urban Air Quality Management, Bangkok, Thailand, February. Accessed on the internet. 2008. https://www.unescap.org/esd/environment/kitakyushu/urban_air/city_report/Tehran.pdf .
Hirota K. Comparative studies on vehicle related policies for air pollution reduction in ten Asian countries. Sustainability. 2010;2(1):145–62.
Irving P, Moncrieff I. New Zealand traffic and local air quality. Sci Total Environ. 2004;334:299–306.
Johnston FH, Hanigan IC, Henderson SB, Morgan GG. Evaluation of interventions to reduce air pollution from biomass smoke on mortality in Launceston, Australia: retrospective analysis of daily mortality, 1994–2007. BMJ. 2013. https://doi.org/10.1136/bmj.e8446 .
Lareau TJ. Alternate stationary source air pollution control policies: a welfare analysis. Public Finance Rev. 1981;9(3):281–307.
Judd BR. Decision analysis of auto emission control. Socioecon Plann Sci. 1977;11(3):123–30.
Norco JE, Cohen AS. Modeling emission control strategies: the ‘London Law’ in Chicago. VDI VERLAG-GMBH. 1973:D38-D40.
Mandelker DR, Taub F. Constitutional limitations on emission quotas as an air pollution control strategy. Ecol Law Q. 1979;8(2):269–302.
Guldmann JM. Modeling the location of greenbelts as a means for air quality control. Socioecon Plann Sci. 1983;17(4):217–24.
Fraas A, McGartland A. Alternative fuels for pollution control: an empirical evaluation of benefits and costs. Contemp Econ Policy. 1990;8(1):62–74.
Scott FA Jr, Berger MC, Blomquist GC. Impacts of air pollution control strategies in Kentucky. Growth Chang. 1988;19(2):40–55.
Gaut NE, Gladden LW, Newman E. Dynamic emission controls: a cost effective strategy for air quality control. ISA Trans. 1974;13(4):277–90.
Lloyd AC, Lents JM, Green C, Nemeth P. Air quality management in Los Angeles: perspectives on past and future emission control strategies. J Air Pollut Control Assoc. 1989;39(5):696–703.
Lloyd AC. California clean air initiatives—the role of fuel cells. J Power Sour. 1992;37(1–2):241–53.
Bruhl RJ, Linder SH, Sexton K. Case study of municipal air pollution policies: Houston’s air toxic control strategy under the white administration, 2004–2009. Environ Sci Technol. 2013;47(9):4022–8.
Collins CO, Scott SL. Air pollution in the Valley of Mexico. Geogr Rev. 1993;83(2):119–33.
Davis LW. The effect of driving restrictions on air quality in Mexico City. J Polit Econ. 2008;116(1):38–81.
Streit GE, Guzman F. Mexico City air quality: progress of an international collaborative project to define air quality management options. Atmos Environ. 1996;30(5):723–33.
Carvalho VSB, Freitas ED, Martins LD, Martins JA, Mazzoli CR, Andrade MDF. Air quality status and trends over the Metropolitan Area of São Paulo, Brazil as a result of emission control policies. Environ Sci Policy. 2015;47:68–79.
Dente B, Lewanski R. Administrative networks and implementation effectiveness—industrial air-pollution control policy in Italy. Policy Stud J. 1982;11(1):116–29.
Cesaroni G, Boogaard H, Jonkers S, Porta D, Badaloni C, Cattani G, et al. Health benefits of traffic-related air pollution reduction in different socioeconomic groups: the effect of low-emission zoning in Rome. Occup Environ Med. 2012;69(2):133–9.
Weber E. Air pollution control strategy in the Federal Republic of Germany. J Air Pollut Control Assoc. 1981;31(1):24–30.
Beck P. Air pollution control policy in the Federal Republic of Germany as a strategy for dealing with environmental risks. Environ Int. 1984;10(5–6):463–73.
Jiang W, Boltze M, Groer S, Scheuvens D. Impacts of low emission zones in Germany on air pollution levels. Transp Res Procedia. 2017;25:3370–82.
Qadir R, Abbaszade G, Schnelle-Kreis J, Chow J, Zimmermann R. Concentrations and source contributions of particulate organic matter before and after implementation of a low emission zone in Munich, Germany. Environ Pollut. 2013;175:158–67.
Hutchinson EJ, Pearson PJ. An evaluation of the environmental and health effects of vehicle exhaust catalysts in the UK. Environ Health Perspect. 2004;112(2):132–41.
Carnell E, Vieno M, Vardoulakis S, Beck R, Heaviside C, Tomlinson S, et al. Modelling public health improvements as a result of air pollution control policies in the UK over four decades—1970 to 2010. Environ Res Lett. 2019;14(7):074001.
Ellison RB, Greaves SP, Hensher DA. Five years of London’s low emission zone: effects on vehicle fleet composition and air quality. Transp Res Part D: Transp Environ. 2013;23:25–33.
Burr ML, Karani G, Davies B, Holmes B, Williams K. Effects on respiratory health of a reduction in air pollution from vehicle exhaust emissions. Occup Environ Med. 2004;61(3):212–8.
Panteliadis P, Strak M, Hoek G, Weijers E, van der Zee S, Dijkema M. Implementation of a low emission zone and evaluation of effects on air quality by long-term monitoring. Atmos Environ. 2014;86:113–9.
Plinke E, Atak M, Haasis HD, Rentz O. Emission control strategies for turkey in view of an integration into the European community. Int J Energy Res. 1992;16(3):223–39.
Atimtay AT, Incecik S, editors. Air pollution problem in Istanbul, Turkey and strategical efforts in air quality management. Actes du colloque «13th Clean Air Congress», Londres; 2004.
Toman M, Cofała J, Bates R. Alternative standards and instruments for air pollution control in Poland. Environ Resour Econ. 1994;4(5):401–17.
Adamson S, Bates R, Laslett R, Pototschnig A. Energy use, air pollution, and environmental policy in Krakow: Can economic incentives really help? World Bank Technical Paper.1996:11-52.
Aunan K, Pátzay G, Aaheim HA, Seip HM. Health and environmental benefits from air pollution reductions in Hungary. Sci Total Environ. 1998;212(2–3):245–68.
Clancy L, Goodman P, Sinclair H, Dockery DW. Effect of air-pollution control on death rates in Dublin, Ireland: an intervention study. Lancet. 2002;360(9341):1210–4.
Dockery DW, Rich DQ, Goodman PG, Clancy L, Ohman-Strickland P, George P, et al. Effect of air pollution control on mortality and hospital admissions in Ireland. Res Rep (Health Eff Inst). 2013;176:3–109.
Stathopoulos A, Argyrakos G. Control strategies for reducing environmental pollution from road traffic. Sci Total Environ. 1993;134(1–3):315–24.
Mavrotas G, Ziomas IC, Diakouaki D. A combined MOIP–MCDA approach to building and screening atmospheric pollution control strategies in urban regions. Environ Manag. 2006;38(1):149–60.
Naiker Y, Diab R, Zunckel M, Hayes ET. Introduction of local air quality management in South Africa: overview and challenges. Environ Sci Policy. 2012;17:62–71.
Chávez CA, Stranlund JK, Gómez W. Controlling urban air pollution caused by households: uncertainty, prices, and income. J Environ Manag. 2011;92(10):2746–53.
Gramsch E, Le Nir G, Araya M, Rubio MA, Moreno F, Oyola P. Influence of large changes in public transportation (Transantiago) on the black carbon pollution near streets. Atmos Environ. 2013;65:153–63.
Ghorani-Azam A, Riahi-Zanjani B, Balali-Mood M. Effects of air pollution on human health and practical measures for prevention in Iran. J Res Med Sci. 2016. https://doi.org/10.4103/1735-1995.189646 .
Zhang XG, Fung JCH, Zhang YM, Lau AKH, Leung KKM, Huang W. Assessing PM25 emissions in 2020: the impacts of integrated emission control policies in China. Environ Pollut. 2020;263:114575.
Fekete H, Kuramochi T, Roelfsema M, den Elzen M, Forsell N, Höhne N, et al. A review of successful climate change mitigation policies in major emitting economies and the potential of global replication. Renew Sustain Energy Rev. 2021;137:110602.
Lozano A, Granados F, Guzmán A. Impacts of modifications on urban road infrastructure and traffic management: a case study. Procedia Soc Behav Sci. 2014;162:368–77.
Rojas-Rueda D, de Nazelle A, Teixidó O, Nieuwenhuijsen MJ. Replacing car trips by increasing bike and public transport in the greater Barcelona metropolitan area: a health impact assessment study. Environ Int. 2012;49:100–9.
Hesketh R, Jones L, Hinrichs-Krapels S, Kirk A, Johnson S. Air quality improvement initiatives in other cities. 2017. https://www.kcl.ac.uk/sspp/policy-institute/%20publications/air-quality-improvement-initiatives-in-other-cities.pdf .
Jiang Y, Zhou X, Xu Q. Scenario analysis-based decision and coordination in supply chain management with production and transportation scheduling. Symmetry. 2019;11(2):160.
Ko A, Woo Y, Jang J, Jung Y, Pyo Y, Jo H, et al. Complementary effects between NO oxidation of DPF and NO2 decomposition of SCR in light-duty diesel engine. J Ind Eng Chem. 2019;80:160–70.
García-Contreras R, Soriano JA, Fernández-Yáñez P, Sánchez-Rodríguez L, Mata C, Gómez A, et al. Impact of regulated pollutant emissions of euro 6d-temp light-duty diesel vehicles under real driving conditions. J Clean Prod. 2021;286:124927.
Hao J, Fu L, He K, Wu Y. Urban vehicular pollution control. Beijing: China Environmental Science Press; 2001.
Hao J, Hu J, Fu L. Controlling vehicular emissions in Beijing during the last decade. Transp Res Part A: Policy Pract. 2006;40(8):639–51.
Liu H, He K, He D, Fu L, Zhou Y, Walsh MP, et al. Analysis of the impacts of fuel sulfur on vehicle emissions in China. Fuel. 2008;87(13–14):3147–54.
Hawkins TR, Gausen OM, Strømman AH. Environmental impacts of hybrid and electric vehicles—a review. Int J Life Cycle Assess. 2012;17(8):997–1014.
Holman C, Harrison R, Querol X. Review of the efficacy of low emission zones to improve urban air quality in European cities. Atmos Environ. 2015;111:161–9.
Cyrys J, Peters A, Wichmann H-E. Umweltzone München-Eine erste Bilanz. Umweltmedizin in Forschung und Praxis. 2009;14(3):127–32.
Jones AM, Harrison RM, Barratt B, Fuller G. A large reduction in airborne particle number concentrations at the time of the introduction of “sulphur free” diesel and the London low emission zone. Atmos Environ. 2012;50:129–38.
Santos G, Behrendt H, Teytelboym A. Part II: policy instruments for sustainable road transport. Res Transp Econ. 2010;28(1):46–91.
Greene W, BJ T. Institutional and regulatory approaches to control residential wood burning emissions. Proc. Conf. Wood Combust. Environ. Assess. 162-174, EPA 600/9-81-029 (U.S. Environmental Protection Agency, Washington D.C., 1981).
Zhao B, Wang S, Wang J, Fu JS, Liu T, Xu J, et al. Impact of national NOx and SO2 control policies on particulate matter pollution in China. Atmos Environ. 2013;77:453–63.
Daneshpajooh N, Arhami M, Azoji H. PM dispersion during stable winter episodes in Tehran and effect of governmental emission regulations. Atmos Pollut Res. 2020;11(8):1316–28.
Mediavilla-Sahagun A, ApSimon HM. Urban scale integrated assessment for London: which emission reduction strategies are more effective in attaining prescribed PM10 air quality standards by 2005? Environ Model Softw. 2006;21(4):501–13.
Ding AJ, Huang X, Nie W, Chi XG, Xu Z, Zheng LF, et al. Significant reduction of PM2.5 in eastern China due to regional-scale emission control: evidence from SORPES in 2011–2018. Atmos Chem Phys. 2019;19(18):11791–801.
Gao M, Liu Z, Zheng B, Ji D, Sherman P, Song S, et al. China’s emission control strategies have suppressed unfavorable influences of climate on wintertime PM 2.5 concentrations in Beijing since 2002. Atmos Chem Phys. 2020;20(3):1497–505.
Albuquerque TTD, West J, Andrade MD, Ynoue RY, Andreao WL, dos Santos FS, et al. Analysis of PM2.5 concentrations under pollutant emission control strategies in the metropolitan area of Sao Paulo, Brazil. Environ Sci Pollut Res. 2019;26(32):33216–27.
Kumar P, Gulia S, Harrison RM, Khare M. The influence of odd–even car trial on fine and coarse particles in Delhi. Environ Pollut. 2017;225:20–30.
Xu H, Ho SSH, Cao J, Guinot B, Kan H, Shen Z, et al. A 10-year observation of PM 2.5-bound nickel in Xi’an, China: effects of source control on its trend and associated health risks. Sci Rep. 2017. https://doi.org/10.1038/srep41132 .
Raufer RK. Particulate and lead air pollution control in Cairo: benefits valuation and cost-effective control strategies. Nat Res Forum. 1997;21(3):209–19.
Cyrys J, Peters A, Soentgen J, Wichmann H-E. Low emission zones reduce PM10 mass concentrations and diesel soot in German cities. J Air Waste Manag Assoc. 2014;64(4):481–7.
Trnka D. Policies, regulatory framework and enforcement for air quality management: the case of Korea. OECD: Paris, France, 2020; Available online: https://www.oecd.org/officialdocuments/publicdisplaydocumentpdf/?cote=ENV/WKP(2020)5&docLanguage=En .
Kleeman MJ, Cass GR. Effect of emissions control strategies on the size and composition distribution of urban particulate air pollution. Environ Sci Technol. 1999;33(1):177–89.
Kanada M, Fujita T, Fujii M, Ohnishi S. The long-term impacts of air pollution control policy: historical links between municipal actions and industrial energy efficiency in Kawasaki City, Japan. J Clean Prod. 2013;58:92–101.
Ikeda Y, Yasuda R, Nakaminami H, Tanaka M, Lee C, Higashino H. A mixed-integer optimization technique for developing a sulfur emission control strategy for China. Water Air Soil Pollut. 2001;130(1–4):265–70.
Xu Y, Masui T. Local air pollutant emission reduction and ancillary carbon benefits of SO2 control policies: application of AIM/CGE model to China. Eur J Oper Res. 2009;198(1):315–25.
Wang XM, Liu H, Pang JM, Carmichael G, He KB, Fan Q, et al. Reductions in sulfur pollution in the Pearl River Delta region, China: assessing the effectiveness of emission controls. Atmos Environ. 2013;76:113–24.
MacDonald BI. Alternative strategies for control of sulfur dioxide emissions. J Air Pollut Control Assoc. 1975;25(5):525–8.
Ruane MF, Gruhl J, Schweppe FC. Supplementary control systems—a demonstration. IEEE Trans Power Appar Syst. 1976;95(1):309–17.
Shao M, Zhang Y, Raufer R. Control strategies for vehicular NOx emissions in Guangzhou, China. Nat Resour Forum. 2001;25(2):157–66.
Shon Z-H, Kim K-H. Impact of emission control strategy on NO2 in urban areas of Korea. Atmos Environ. 2011;45(3):808–12.
Cui L, Li HW, Huang Y, Zhang Z, Lee SC, Blake DR, et al. The characteristics and sources of roadside VOCs in Hong Kong: effect of the LPG catalytic converter replacement programme. Sci Total Environ. 2021. https://doi.org/10.1016/j.scitotenv.2020.143811 .
Shin HJ, Kim JC, Lee SJ, Kim YP. Evaluation of the optimum volatile organic compounds control strategy considering the formation of ozone and secondary organic aerosol in Seoul. Korea Environ Sci Pollut Res. 2013;20(3):1468–81.
Fujita EM, Campbell DE, Stockwell WR, Lawson DR. Past and future ozone trends in California’s South Coast Air Basin: reconciliation of ambient measurements with past and projected emission inventories. J Air Waste Manag Assoc. 2013;63(1):54–69.
Streets D, Chang Y-S, Tompkins M, Ghim Y, Carter L. Efficient regional ozone control strategies for the eastern United States. J Environ Manag. 2001;61(4):345–65.
Pratapas JM, Calcagni J. Ozone control strategies in the United States. Environ Int. 1983;9(6):529–38.
Heninger BT, Shah FA. Control of stationary and mobile source air pollution: reducing emissions of hydrocarbons for ozone abatement in Connecticut. Land Econ. 1998;74(4):497–513.
Cohan DS, Tian D, Hu YT, Russell AG. Control strategy optimization for attainment and exposure mitigation: case study for ozone in Macon, Georgia. Environ Manag. 2006;38(3):451–62.
Yu X, Yuan ZB, Fung JCH, Xue J, Li Y, Zheng JY, et al. Ozone changes in response to the heavy-duty diesel truck control in the Pearl River Delta. Atmos Environ. 2014;88:269–74.
Castell N, Mantilla E, Stein AF, Salvador R, Millan M. Simulation and evaluation of control strategies for ozone reduction in a complex terrain in Southwestern Spain. Environ Model Assess. 2011;16(6):565–76.
Tong P, Zhang Q, Lin H, Jian X, Wang X. Simulation of the impact of the emergency control measures on the reduction of air pollutants: a case study of APEC blue. Environ Monit Assess. 2020. https://doi.org/10.1007/s10661-019-8056-1 .
Haagen Smit AJ. Abatement strategy for photochemical smog. Adv Chem Ser. 1972;113:169–90.
Katsouyanni K, Touloumi G, Spix C, Schwartz J, Balducci F, Medina S, et al. Short term effects of ambient sulphur dioxide and particulate matter on mortality in 12 European cities: results from time series data from the APHEA project. BMJ. 1997;314(7095):1658.
Wang X, Bi X, Sheng G, Fu J. Chemical composition and sources of PM10 and PM2.5 aerosols in Guangzhou, China. Environ Monit Assess. 2006;119(1):425–39.
Behera SN, Sharma M, Nayak P, Shukla SP, Gargava P. An approach for evaluation of proposed air pollution control strategy to reduce levels of nitrogen oxides in an urban environment. J Environ Plann Manag. 2014;57(4):467–94.
Lal S, Patil R. Monitoring of atmospheric behaviour of NOx from vehicular traffic. Environ Monit Assess. 2001;68(1):37–50.
van Aardenne JA, Carmichael GR, Levy H II, Streets D, Hordijk L. Anthropogenic NOx emissions in Asia in the period 1990–2020. Atmos Environ. 1999;33(4):633–46.
Krishnamurthy A, Adebayo B, Gelles T, Rownaghi A, Rezaei F. Abatement of gaseous volatile organic compounds: a process perspective. Catal Today. 2020;350:100–19.
Kleinman L, Lee YN, Springston SR, Nunnermacker L, Zhou X, Brown R, et al. Ozone formation at a rural site in the southeastern United States. J Geophys Res: Atmos. 1994;99(D2):3469–82.
Liu S, Trainer M, Fehsenfeld F, Parrish D, Williams E, Fahey DW, et al. Ozone production in the rural troposphere and the implications for regional and global ozone distributions. J Geophys Res: Atmos. 1987;92(D4):4191–207.
Sillman S. The relation between ozone, NOx and hydrocarbons in urban and polluted rural environments. Dev Environ Sci. 2002;1:339–85.
Raifman M, Lambert KF, Levy JI, Kinney PL. Mortality implications of increased active mobility for a proposed regional transportation emission cap-and-invest program. J Urban Health. 2021. https://doi.org/10.1289/isee.2021.O-SY-064 .
Download references
Acknowledgements
The authors thank the Research Center for Environmental Health Technology, Iran University of Medical Sciences for the provision of financial support [Grant No.99-3-61-19814]. (Ethics Code: IR.IUMS.REC.1399.1364).
Author information
Authors and affiliations.
Research Center for Environmental Health Technology, Iran University of Medical Sciences, Tehran, Iran
Ahmad Jonidi Jafari, Esmail Charkhloo & Hasan Pasalari
Department of Environmental Health Engineering, School of Public Health, Iran University of Medical Sciences, Tehran, Iran
You can also search for this author in PubMed Google Scholar
Corresponding author
Correspondence to Esmail Charkhloo .
Ethics declarations
Conflicts of interest.
The authors formally declare no conflict(s) of interest.
Additional information
Publisher's note.
Springer Nature remains neutral with regard to jurisdictional claims in published maps and institutional affiliations.
Rights and permissions
Reprints and permissions
About this article
Jonidi Jafari, A., Charkhloo, E. & Pasalari, H. Urban air pollution control policies and strategies: a systematic review. J Environ Health Sci Engineer 19 , 1911–1940 (2021). https://doi.org/10.1007/s40201-021-00744-4
Download citation
Received : 28 August 2021
Accepted : 20 September 2021
Published : 08 October 2021
Issue Date : December 2021
DOI : https://doi.org/10.1007/s40201-021-00744-4
Share this article
Anyone you share the following link with will be able to read this content:
Sorry, a shareable link is not currently available for this article.
Provided by the Springer Nature SharedIt content-sharing initiative
- Air quality
- Intervention
- Air pollutants
- Find a journal
- Publish with us
- Track your research

An official website of the United States government
The .gov means it’s official. Federal government websites often end in .gov or .mil. Before sharing sensitive information, make sure you’re on a federal government site.
The site is secure. The https:// ensures that you are connecting to the official website and that any information you provide is encrypted and transmitted securely.
- Publications
- Account settings
Preview improvements coming to the PMC website in October 2024. Learn More or Try it out now .
- Advanced Search
- Journal List
- Wiley Open Access Collection

Ambient air pollution and cardiovascular diseases: An umbrella review of systematic reviews and meta‐analyses
Jeroen de bont.
1 Institute of Environmental Medicine, Karolinska Institutet, Stockholm Sweden
Suganthi Jaganathan
2 Centre for Environmental Health, Public Health Foundation of India, Delhi‐NCR India
3 Centre for Chronic Disease Control, New Delhi India
Marcus Dahlquist
Åsa persson, massimo stafoggia.
4 Department of Epidemiology, Lazio Region Health Service, Rome Italy
Petter Ljungman
5 Department of Cardiology, Danderyd University Hospital, Danderyd Sweden
Associated Data
Table S2 : Search strategy in PubMed, EMBASE and Web of Science (WOS).
Table S3 : Descriptive information of the included reviews.
Table S4 . Meta‐analytic estimates: short‐term exposures to air pollution on adverse cardiovascular outcomes (effect estimates are specified as % increase in RR/HR/OR or beta (linear) for 10 µg/m 3 increase of air pollution).
Table S5 . Meta‐analytic estimates: long‐term exposures to air pollution on adverse cardiovascular outcomes (effect estimates are specified as % increase in RR/HR/OR or beta (linear) for 10 µg/m3 increase of air pollution).
Table S6 . Systematic review results: short‐term exposures to air pollution and adverse cardiovascular outcomes.
Table S7 . Systematic review results: long‐term exposures to air pollution and adverse cardiovascular outcomes.
Figure S1 : Effect estimates of the association between short‐term exposure to PM 10 and cardiovascular outcomes.
Figure S2 : Effect estimates of the association between long‐term exposure to PM 10 and cardiovascular outcomes.
The available evidence on the effects of ambient air pollution on cardiovascular diseases (CVDs) has increased substantially. In this umbrella review, we summarized the current epidemiological evidence from systematic reviews and meta‐analyses linking ambient air pollution and CVDs, with a focus on geographical differences and vulnerable subpopulations. We performed a search strategy through multiple databases including articles between 2010 and 31 January 2021. We performed a quality assessment and evaluated the strength of evidence. Of the 56 included reviews, the most studied outcomes were stroke (22 reviews), all‐cause CVD mortality, and morbidity (19). The strongest evidence was found between higher short‐ and long‐term ambient air pollution exposure and all‐cause CVD mortality and morbidity, stroke, blood pressure, and ischemic heart diseases (IHD). Short‐term exposures to particulate matter <2.5 μm (PM 2.5 ), <10 μm (PM 10 ), and nitrogen oxides (NO x ) were consistently associated with increased risks of hypertension and triggering of myocardial infarction (MI), and stroke (fatal and nonfatal). Long‐term exposures of PM 2.5 were largely associated with increased risk of atherosclerosis, incident MI, hypertension, and incident stroke and stroke mortality. Few reviews evaluated other CVD outcomes including arrhythmias, atrial fibrillation, or heart failure but they generally reported positive statistical associations. Stronger associations were found in Asian countries and vulnerable subpopulations, especially among the elderly, cardiac patients, and people with higher weight status. Consistent with experimental data, this comprehensive umbrella review found strong evidence that higher levels of ambient air pollution increase the risk of CVDs, especially all‐cause CVD mortality, stroke, and IHD. These results emphasize the importance of reducing the alarming levels of air pollution across the globe, especially in Asia, and among vulnerable subpopulations.

Introduction
Ambient air pollution is considered the foremost environmental risk factor of mortality and morbidity worldwide [ 1 ]. Recent evidence from the Global Burden of Disease (GBD) study estimated that ambient air pollution caused 4.2 million deaths (7.6% of total global mortality) and 103.1 million disability‐adjusted life‐years (DALYs) (4.2% of global DALYs) in 2015 [ 1 ]. More specifically, ambient air pollution has been associated with adverse respiratory diseases, decreased lung function, cancer, obesity, diabetes, and cardiovascular diseases (CVD) among adults [ 2 , 3 , 4 ]. In fact, the GBD in 2015 reported that air pollutants accounted for 19% of all cardiovascular deaths [ 1 ].
Air pollution is a complex mixture of particles and gases whose physical and chemical composition, origin, and toxicity differ spatially and temporally [ 5 , 6 ]. Air pollutants in the solid phase are usually classified according to their size—PM 2.5 are particulate matter (PM) less than 2.5 μm in diameter, PM 10 are less than 10 μm, PM coarse are between 2.5 and 10 μm, and ultrafine particles (UFP) are less than 0.1 μm. Smaller sizes of PM have been associated with more adverse effects on human health as they can penetrate more deeply into the respiratory tract [ 2 ]. Particles may have substantially different chemical and physical properties and emissions can vary between locations and over time [ 6 ]. In urban areas, PM 2.5 and UFP are directly generated by combustion processes in vehicles, industry and energy production, and from domestic heating, whereas PM 10 and PM coarse are composed largely of crustal material, sea salt, and biological materials [ 5 , 6 ] (Fig. 1 ). Motor vehicles and industrial plants are also important sources of gaseous air pollutants, such as oxides of nitrogen (NO x ) derived from combustion processes of burning fossil fuels at high temperatures. Nitrogen dioxide (NO 2 ) is the secondary pollutant of nitrogen oxides that reacts rapidly with oxygen to form NO 2 . In nonurban or rural areas, NO 2 is usually at its lowest levels as it is consumed in secondary processes involving gaseous precursors to generate fine particles. Overall, there seems to be no safe threshold for air pollution levels. Even in countries with low levels of air pollution, there is evidence of associations with cardiovascular health [ 7 ]. Interestingly, irrespective of the body of evidence available, air pollution guidelines vary substantially across the globe (Fig. 1 ). The lowest annual thresholds of PM 2.5 are the Australian air quality guidelines (8 μg/m 3 ), whereas China and India have much higher thresholds (35 and 40 μg/m 3 , respectively). In many countries, especially in low‐and‐middle‐income countries (LMICs), the health effects of long‐term exposure to air pollution are yet to be studied in detail and desired thresholds for air quality are routinely violated [ 8 ]. On 22 September 2021, the World Health Organization released revised air quality guidelines based on newer evidence and recommended more stringent guidelines with annual thresholds for PM 2.5 of 5 μg/m 3 (a reduction from earlier guidelines of 10 μg/m 3 ), further highlighting the urgency to improve air quality across the globe.

Graph showing population‐weighted annual PM 2.5 (where PM is particulate matter) averages (2019) and air quality standards (Note: presenting the two most polluted and two least polluted countries in each region) [ 8 ].
The available evidence for CVD effects of air pollution has increased substantially during the last decade [ 9 , 10 ]. Current reviews have been limited to specific aspects of air pollution and CVD. To date, we lack an umbrella review that has synthesized all the available evidence from systematic reviews and meta‐analysis investigating the effect of PM and NOx on multiple cardiovascular outcomes including short and long‐term studies. Additionally, the levels of ambient air pollution have increased rapidly in LMICs during the last decades, but there are very few studies from such high concentration areas. Hence, more studies in LMICs are needed owing to substantial differences between pollutant sources, composition, spatial‐temporal variability, population characteristics, and access to health care compared with high‐income countries. The effects of air pollution are variable for different population subgroups, owing to age, gender, socio‐economic status, and occupation [ 11 ].
In this umbrella review, we aimed to summarize the current epidemiological evidence from systematic reviews and meta‐analyses linking ambient air pollution (PM and NO x ) and multiple CVDs. We additionally tried to review evidence of heterogenous effects that exist based on geography and among vulnerable subpopulations.
Search strategy, inclusion, and exclusion of reviews
We developed the umbrella review in accordance with the reporting guidance in the Preferred Reporting Items for Systematic Reviews and Meta‐Analyses Protocols (PRISMA‐P) (Table S1 ). The protocol was registered in the Prospero database (ID: CRD42021250209). We performed a comprehensive search in PubMed, EMBASE and Web of Science for articles published between 1 January 2010 and 31 January 2021, limited to articles published in English (Table S2 ). The search strategy identified systematic reviews and meta‐analyses of observational studies in humans of different study designs (cohort, case‐crossover, cross‐sectional, and time‐series) evaluating the short‐ and long‐term effect of ambient air pollution on multiple CVDs. We focused on the following exposures: PM (PM 10 , PM 2.5 , PM coarse , elemental carbon [EC], and UFP) and nitrogen oxides (NO x and NO 2 ); and the following CVD outcomes: all‐cause CVD mortality and morbidity, ischemic heart disease (IHD; in this review, we considered coronary heart disease as part of IHD), myocardial infarction (MI), atherosclerosis, arterial stiffness, blood pressure and hypertension, heart failure, stroke and cerebrovascular diseases, arrhythmias, atrial fibrillation, and cardiac arrest. We excluded reviews that did not specify the following: a research question, search strategy, the databases used, inclusion/exclusion criteria, screening methods, quality assessment of included studies’ quality, and information about data synthesis. We excluded reviews related to ozone, sulphur dioxide, carbon monoxide, indoor air pollution, occupational exposures, transboundary haze/desert storms, intervention studies, and experimental studies. We further excluded nonepidemiological reviews/narrative reviews and reviews that focussed on other outcomes, including metabolic disorders, respiratory conditions, congenital heart diseases, venous thrombosis, and coagulation markers. Two researchers (S.J. and J.D.B.) screened independently the titles and abstracts and conducted full‐text screening for potential inclusion. Any disagreement was resolved in consensus with the rest of the team (M.D., Å.P., M.S., and P.L.).
The data were extracted in duplicate (S.J. and J.D.B.) and reverted to the original article in case of disagreements. The following characteristics were extracted: citation details, type of review, participation details, study setting and context, date range of database searching, number of included studies (types of study and country of origin), main exposures, main outcomes, main results, results pertaining to LMICs, and vulnerable populations (Table S3 ). For meta‐analytical reviews, we additionally extracted the summary meta‐analytic estimate and corresponding 95% confidence interval (CI), and heterogeneity measure. The effect estimates and CI were converted to percentage excess risk of odds ratio (OR)/relative risk (RR)/hazard ratio (HR; % excess risk = [OR/HR/RR − 1] × 100) and were harmonized to a 10 μg/m 3 increase of air pollution to enable comparison. For systematic reviews, we summarized the number of studies that found statistically significant associations and the direction of the association estimates.
Quality assessment
To assess the methodological quality of the included systematic reviews and meta‐analyses, we considered the critical domains of the AMSTAR‐2 (A MeaSurement Tool to Assess Systematic Reviews) checklist [ 12 ]. The AMSTAR‐2 quality assessment tool has a 16‐item or domain checklist. Seven of these items were considered critical: (1) protocol registered before starting the review, (2) adequacy of the literature search, (3) justification for excluding individual studies, (4) risk of bias from individual studies included in the review, (5) appropriateness of meta‐analytical methods, (6) consideration of risk of bias when interpreting the results of the review, and (7) assessment of presence and likely impact of publication bias. The quality was rated as low (when only 1 critical domain was met), moderate (between 2 and 5 critical domains were met), and high (6 or all critical domains were met). To evaluate the strength of evidence, we calculated a percentage by dividing the number of meta‐analyses reporting positively significant associations among the total numbers of meta‐analyses for each specific CVD outcome and temporality. Then, we classified the strength of evidence in the following categories: (1) sufficient, evidence is sufficiently conclusive (>75% of the meta‐analyses have shown a statistical and positive significant association); (2) limited, positive associations but not conclusive (>50% to ≤75% of the meta‐analyses have shown statistically significant associations); (3) inadequate, positive association reported in less than 50% of meta‐analyses (>25% to ≤50%); (4) insufficient, no association reported in one or more meta‐analyses (0% to ≤25%); and (5) no studies, no studies have been conducted.
Descriptive results
We identified a total of 1112 publications across the three different databases (Fig. 2 ). After screening titles and abstracts, we excluded publications for duplicates ( N = 375) and irrelevant ( N = 654) publications and identified a total of 83 publications eligible for full‐text reading. We further excluded reviews that were not a systematic review and/or meta‐analysis (13 publications), discussed confounding or effect modification by other exposures rather than the exposure of our interest (4), were not in the scope of our umbrella review (4), were incomplete systematic reviews (4), were reviews lacking epidemiological results (1), or were not in English (1). We finally included a total of 10 systematic reviews and 46 meta‐analyses in this umbrella review (Fig. 2 and Table S3 ). The most common outcomes were stroke (22 reviews) and all‐cause CVD mortality and morbidity (21) and less common outcomes were cardiac arrest (3) and arterial stiffness (1) (Table 1 ). A total of 44 reviews focused on short‐term effects and 33 on long‐term effects, and 52 and 26 reviews focused on the effect of PM and nitrogen oxides, respectively (Table 1 ).

Flowchart of the included reviews.
Summary of included reviews by CVD outcomes, exposure temporality, air pollutants, and percentage of studies in LMICs
Abbreviations: CVD, cardiovascular disease; LMICs, low‐and‐middle‐income countries; NO x , nitrogen oxides; PM, particulate matter.
All‐cause CVD mortality and morbidity
In total, four systematic reviews and 17 meta‐analyses were published between 2010 and 2020 and evaluated the short‐ and long‐term exposures of ambient air pollution on all‐cause CVD mortality and morbidity [ 13 , 14 , 15 , 16 , 17 , 18 , 19 , 20 , 21 , 22 , 23 , 24 , 25 , 26 , 27 , 28 , 29 , 30 , 31 , 32 , 33 ]. From the meta‐analyses, increased short‐term exposure to PM 2.5 (3 meta‐analytic estimates out of 3), PM 10 (2/2), and NO x (5/5) were all statistically significantly associated with all‐cause CVD mortality (Figs 3 , ,4, 4 , and S1 ). Percent excess risk per 10 μg/m 3 increase ranged from 0.64 (0.39; 0.97) to 1.00 (1.00; 2.00) for PM 2.5 , from 0.39 (0.26; 0.53) to 0.49 (0.35, 0.63) for PM 10 , and from 0.88 (0.63; 1.13) to 1.62 (1.18; 2.06) for NO x . From the meta‐analyses reporting all‐cause CVD morbidity (hospital admissions), there were statistically significant positive associations for short‐term exposure to PM 2.5 (1/2), PM 10 (2/2), and NO x (3/4) (Figs 3 , ,4, 4 , and S1 ). In the systematic reviews, all effect estimates from the individual studies were associated with an increased CVD mortality (16 out of 18 individual studies) and morbidity (8/11) for PM 2.5 , but some were not statistically significant (Table S6 ).

Effect estimates of the association between short‐term exposure to PM 2.5 and CVDs. Note: we selected the main effect estimate from the meta‐analyses if multiple effect estimates were available for each CVD outcome in the same meta‐analysis. Effect estimates are estimated per 10 μg/m 3 range increase in PM 2.5. Abbreviations: BP, blood pressure; CeVD, cerebrovascular diseases; CVD, cardiovascular diseases; ICD, implantable cardioverter defibrillator; IHD, ischemic heart diseases; MI, myocardial infarction; PM, particulate matter. * Beta coefficient (linear regression) for change in systolic and diastolic values per increase of PM 2.5 .

Effect estimates of the association between short‐term exposure to NO x /NO 2 and CVDs. Note: we selected the main effect estimate from the meta‐analyses if multiple effect estimates were available for each CVD outcome in the same meta‐analysis. Effect estimates are estimated per 10 μg/m 3 range increase in NO x /NO 2. Abbreviations: BP, blood pressure; CeVD, cerebrovascular diseases; CVD, cardiovascular diseases; IHD, ischemic heart diseases; MI, myocardial infarction. * Beta coefficient (linear regression) for change in systolic and diastolic values per increase of NO x /NO 2 .
Long‐term exposure to PM 2.5 (7/7) and NO x (4/4) was consistently associated with all‐cause CVD mortality, but to a lesser extent for PM 10 (2/4) (Figs 5 , ,6, 6 , and S5 ). Percent excess risk per 10 μg/m 3 increase ranged from 11.00 (7.00; 14.00) to 20.00 (9.00; 31.00) for PM 2.5 and from 3.00 (2.00; 5.00) to 23.00 (15.00; 31.00) for NO x (Figs 5 , ,6, 6 , and S2 ). Two meta‐analysis reviews evaluated the association between the air pollutants and all‐cause CVD incidence with meta‐analytical effect estimates indicating higher risks, but mostly not statistically significant. A similar consistent pattern with significant positive associations was also observed in the systematic reviews although based on a few number of studies (Table S7 ).

Effect estimates of the association between long‐term exposure to PM 2.5 and CVDs. Note: we selected the main effect estimate from the meta‐analyses if multiple effect estimates were available for each CVD outcome in the same meta‐analysis. Effect estimates are estimated per 10 μg/m 3 range increase in PM 2.5. Abbreviations: ABI, ankle‐brachial index, BP, blood pressure; CAC, coronary artery calcification; CeVD, cerebrovascular diseases; CIMT, carotid intima‐media thickness test; CVD, cardiovascular diseases; IHD, ischemic heart diseases; MI, myocardial infarction; PM, particulate matter. * Beta coefficient (linear regression) for change in systolic, diastolic, CIMT, CAC, or ABI values per increase of PM 2.5 .

Effect estimates of the association between long‐term exposure to NO x /NO 2 and CVDs. Note: we selected the main effect estimate from the meta‐analyses if multiple effect estimates were available for each CVD outcome in the same meta‐analysis. Effect estimates are estimated per 10 μg/m 3 range increase in NO x /NO 2 . Abbreviations: BP, blood pressure; CVD, cardiovascular diseases; IHD, ischemic heart diseases. * Beta coefficient (linear regression) for change in systolic or diastolic values per increase of NO x /NO 2 .
Ischemic heart disease and myocardial infarction
A total of 10 and seven meta‐analyses evaluated the short‐ and long‐term exposure to PM and NO x on IHD and MI, respectively [ 13 , 16 , 20 , 22 , 24 , 25 , 28 , 29 , 30 , 33 , 34 , 35 , 36 , 37 , 38 , 39 , 40 ]. For both IHD and MI, two systematic reviews were included.
The short‐term exposure to PM 2.5 (3/3) and NO x (3/5) was associated with IHD mortality and hospital admission. Percent excess risk per 10 μg/m 3 increase ranged from 2.50 (1.50; 3.60) to 3.36 (0.68; 6.10) for PM 2.5 and from 0.88 (0.52; 1.20) to 1.42 (−0.04; 2.90) for NO x (Figs 3 and 4 ). For PM 10 , one out of two meta‐analyses found a statistically significant association with increased IHD mortality/hospital admission. In the systematic reviews, most of the individual reviews concluded that air pollutants were associated with increased IHD mortality (Table S6 ), especially in the Zhang and Routledge [ 25 ] review that focused only on studies conducted in China (Table S3 ). Regarding the long‐term exposures, nine out of 10 meta‐analytic estimates found that increased levels of PM 2.5 (4/4), PM 10 (2/3), and NO x (2/2) were associated with increased risk of IHD mortality (Figs 5 , ,6, 6 , and S2 ). Higher long‐term exposures to PM 2.5 (3/3), PM 10 (1/3), and NO x (2/3) were also associated with incident IHD.
Focussing on reviews of air pollution and MI, short‐term exposures to PM 2.5 (4/4), PM 10 (4/4), and NO x (1/1) were associated with increased triggering of MI mortality and hospital admissions (Figs 3 , ,4, 4 , and S1 ). Percent excess risk per 10 μg/m 3 increase ranged from 1.20 (1.00; 1.50) to 2.40 (0.70; 4.10) for PM 2.5 , from 0.50 (0.10; 0.80) to 1.10 (0.60; 1.60) for PM 10 , and 1.10 (0.60; 1.60) for NO x . Two out of three meta‐analyses reported a statistically significant association with increased long‐term exposure to PM 2.5 and increased risk of MI mortality/incidence (Figs 5 , ,6, 6 , and S2 ). No meta‐analyses evaluated the effect of long‐term effect of PM 10 and NO x on MI. From the systematic reviews, no conclusive results could be drawn as only a few individual studies were included (between 1 and 3 studies, Table S7 ).
Atherosclerosis and arterial stiffness
No systematic reviews or meta‐analyses evaluated the short‐term effects of air pollution on atherosclerosis. One systematic review and three meta‐analyses examined the association between long‐term exposure to PM (PM 2.5 and PM 10 ) and atherosclerosis, but no reviews evaluated the long‐term effect of NO x [ 41 , 42 , 43 , 44 ]. The most common measurements to assess atherosclerosis were carotid intima‐media thickness (CIMT), followed by coronary artery calcification (CAC), arterial calcification, and ankle‐brachial index (ABI). Two out of three meta‐analytical estimates demonstrated statistically significant associations between PM 2.5 and CIMT ranging from 2.09 mm (0.04; 4.48) to 16.79 mm (4.95; 28.63) increased CIMT per year for every 10 μg/m 3 increase in PM 2.5 (Fig. 5 ). No associations were found between PM 10 and CIMT. In addition, in one meta‐analysis, PM 2.5 was associated with CAC but not with ABI. In one systematic review [ 44 ], the individual observational studies demonstrated that increased levels of PM were statistically associated with CIMT (6 out of 9 individual studies), CIMT progression (4/4), CAC prevalence (4/7), and CAC progression (1/2) (Table S7 ).
One systematic review evaluated both short‐ and long‐term exposure to PM 2.5 , PM 10 , and NO x on arterial stiffness (Tables S6 and S7 ) [ 45 ]. They reported that increased levels of PM (PM 2.5 and PM 10 ) and NO x were associated with increased arterial stiffness and pulse wave reflection, but no statistically significant association was observed for brachial‐ankle pulse wave velocity.
Blood pressure and hypertension
A total of 10 reviews (6 meta‐analyses) examined the short and long‐term effect of PM (PM 2.5 , PM 10 , black carbon/EC, UFP, and PM coarse ) on blood pressure and hypertension, and only three studies additionally explored the association with NO x [ 19 , 20 , 24 , 27 , 46 , 47 , 48 , 49 , 50 , 51 ]. In the short‐term exposure studies, higher exposures to PM 2.5 (2 meta‐analytic estimates out of 2), PM 10 (1/1), and NO x (1/1) were associated with higher diastolic blood pressure, whereas only PM 2.5 (2/2) was also associated with higher systolic blood pressure (Figs 3 , ,4, 4 , and S1 ). The increase of mmHg in diastolic blood pressure per 10 μg/m 3 increase of PM 2.5 ranged from 0.15 mmHg (0.01; 0.19) to 0.52 mmHg (0.25; 0.79). Further, increased short‐term exposure to PM 2.5 (2/2), PM 10 (3/3), and NO x (2/3) was associated with an increased risk of hypertension. In the systematic reviews, short‐term exposure to EC was not consistently associated with blood pressure, whereas another review found a consistently small positive association between short‐term exposure to UFP and systolic and diastolic blood pressure (Table S6 ).
Similar to the short‐term effects, a meta‐analysis of long‐term exposures demonstrated significant associations between higher PM 2.5 (1/2), PM 10 (1/1), and NO 2 (1/1) and increased diastolic blood pressure but not systolic blood pressure (Figs 5 , ,6, 6 , and S2 ). In addition, long‐term exposure to PM 2.5 was associated with incident hypertension in all included meta‐analyses (3/3). The percentage risk of incident hypertension ranged from 5.00 (1.00; 9.00) to 7.00 (1.00; 14.00) per 10 μg/m 3 increase in PM 2.5 (Fig. 5 ). PM 10 (1/2) and NO x (1/2) were less consistently associated with incident hypertension. The meta‐analytic excess risk was even larger in a study that focused on the long‐term risk of PM 2.5 on hypertension in women (per cent excess risk per 10 μg/m 3 increase in PM 2.5 = 23.00 [8.00; 40.00]). In the systematic reviews, one review reported that 14 out of 19 included studies found a positive association between EC and systolic and diastolic blood pressure, and another found in two studies a positive association between PM 10 and hypertension in LMICs (Table S7 ).
Heart failure
Five meta‐analyses studied the association between short‐ and long‐term exposure to air pollution on heart failure [ 13 , 20 , 22 , 24 , 52 ]. The short‐term exposures to PM 2.5 (2 meta‐analytic estimates out of 2), PM 10 (1/1), and NO x (2/2) were associated with heart failure mortality and hospital admissions (Figs 3 , ,4, 4 , and S1 ). Percent excess risk per 10 μg/m 3 ranged from 2.12 (1.42; 2.82) to 4.39 (1.35; 7.53) for PM 2.5 , 2.12 (1.42; 2.82) for PM 10 , and from 0.90 (0.66; 1.14) to 2.41 (−0.01; 2.86) for NO x . In contrast, the one meta‐analysis [ 24 ] that evaluated the long‐term effect of PM 2.5 , PM 10 , and NO x on heart failure demonstrated uninformative associations with very large CIs (Figs 5 , ,6, 6 , and S2 ).
In total, two systematic reviews and 20 meta‐analyses evaluated the short‐ and long‐term effects of ambient air pollution on stroke mortality and morbidity, resulting in the most studied CVD outcome during our research period [ 13 , 16 , 20 , 22 , 23 , 24 , 25 , 28 , 29 , 30 , 31 , 33 , 53 , 54 , 55 , 56 , 57 , 58 , 59 , 60 , 61 , 62 ]. Increased short‐term exposure to PM 2.5 (5 meta‐analytic estimates out of 5), PM 10 (2/2), and NO x (5/6) was statistically associated with increased stroke mortality (Figs 3 , ,4, 4 , and S1 ). Regarding stroke hospital admission, statistically significant associations were found for PM 2.5 (4/7), PM 10 (4/4), and NO x (5/5). Similar results were found in a systematic review (Table S6 ). All pollutants were associated with increased cerebrovascular mortality and, to a large extent, associated with cerebrovascular hospital admission. Regarding specific types of strokes, one meta‐analysis found that NO x was associated with hospital admissions for hemorrhagic and ischemic stroke, but no clear associations were found between short‐term exposures to PM 2.5 and PM 10 and specific stroke types (Figs 3 , ,4, 4 , and S1 ).
Long‐term exposure to PM 2.5 was consistently associated with increased stroke mortality (5/5) and stroke incidence (7/7) in meta‐analyses (Fig. 6 ) and one systematic review (Table S7 ). The percent excess risk for long‐term exposure to PM 2.5 per 10 μg/m 3 increase ranged from 11.00 (4.00; 18.00) to 26.56 (1.54; 57.75) for increased stroke mortality and from 11.00 (5.00; 17.00) to 14.00 (8.00; 21.00) for increased stroke incidence. No associations were observed between the long‐term effects of PM 10 and NO x , and stroke mortality and incidence (Fig. 6 / S2 ).
Arrhythmias, atrial fibrillation, and cardiac arrest
Two systematic reviews and four meta‐analyses evaluated the short‐term effect of PM 2.5 , PM 10 , and NO x on arrhythmias (all subtypes grouped together) [ 13 , 20 , 22 , 34 , 47 , 63 ]. No reviews on the long‐term effects of air pollution and arrhythmias were found. PM 2.5 (2 meta‐analytic estimates out of 2), PM 10 (1/1), and NO 2 (1/1) were associated with increased hospital admission for arrhythmia, and only NO x was additionally associated with increased mortality due to arrhythmias (Figs 3 , ,4, 4 , and S1 ). Among the systematic reviews, the included studies showed consistent trends of positive estimates between PM and arrhythmias but did not reach statistical significance (Table S6 ). No reviews on the long‐term effects of air pollution and arrhythmias were found.
There were four meta‐analyses that evaluated the short‐ and long‐term effect of air pollution on atrial fibrillation [ 24 , 64 , 65 , 66 ]. Increased short‐term exposure to PM 2.5 (2/2), PM 10 (1/1), and NO 2 (2/2) was associated with increased atrial fibrillation (Figs 3 , ,4, 4 , and S1 ). Another meta‐analysis [ 65 ] found that a 10 μg/m 3 increase in PM 2.5 was associated with a 24.00% (0.00; 53.00) excess risk of atrial fibrillation in patients with an implantable cardioverter‐defibrillator (Fig. 3 ). In addition, increased long‐term exposure to PM 2.5 (1/2), PM 10 (1/2), and NO 2 (2/2) was inconsistently associated with increased atrial fibrillation (Figs 5 , ,6, 6 , and S2 ).
One systematic review and two meta‐analyses studied the association between short‐term exposure to air pollutants and out‐of‐hospital cardiac arrest (OHCA) [ 26 , 67 , 68 ]. In one meta‐analysis, short‐term exposure to PM 2.5 , PM 10 , and NO 2 was associated with increased OHCA. The other meta‐analysis focussed only on ambulance dispatch data and found similar results in direction and magnitude, but these were not statistically significant (Figs 3 , ,4, 4 , and S1 ). Finally, in the systematic review, consistent results were found between short‐term exposure to PM 2.5 and PM 10 on OHCA, but not for NO x (Table S6 ).
Strength of evidence
Overall, using our a priori definition, we found sufficient strength of evidence for both short‐ and long‐term exposure to PM and increased risk of CVD mortality, IHD, and MI, and for short‐term PM exposure and increased risk of stroke, blood pressure, heart failure, and arrhythmias (Table 2 ). There was limited evidence supporting an associated risk between long‐term exposure to PM and atherosclerosis or arterial stiffness outcomes and for incident stroke. There was inadequate evidence to support associations between long‐term PM exposure and blood pressure, hypertension, and arrhythmias. Similar to PM, there was sufficient evidence in support of associations between NO x and CVD mortality or morbidity for both short‐ and long‐term exposure. Sufficient evidence also supported associations between short‐term exposure to NO x and stroke and arrhythmias, and long‐term exposure to NO x and IHD and MI. The strength of evidence for NO x is limited or inadequate for blood pressure or hypertension for bothering short and long term. The strength of evidence, however, should be interpreted with caution, as some of the outcome categories (heart failure, atherosclerosis, and arrhythmias) were included in very few reviews/meta‐analyses.
Strength of evidence of included reviews and meta‐analysis
Abbreviations: CVD, cardiovascular disease; NO x , nitrogen oxides; PM, particulate matter.
Geographic differences and vulnerable populations
A total of 26 out of the 56 reviews mentioned the presence of different associations of ambient air pollution and CVD outcomes according to geographical locations and vulnerable populations (Table 1 ).
Among the included reviews and meta‐analyses, a majority of the evidence came from high‐income countries. Very few outcomes had a considerable number of primary studies conducted in LMICs, as described in Table 1 . The risk of air pollution exposure for CVD outcomes was likely to be stronger in Asian countries, with higher levels of air pollution compared to North America and Europe (7 out of 9 mentioned higher meta‐analytic estimates), although heterogeneity was higher. In addition, two other meta‐analyses also found stronger associations in more heavily polluted areas around the world (2/2).
Elderly people (4/4), cardiac patients (2/2), and subgroups with higher body mass index status (2/2) were more susceptible to the adverse effects of air pollution. Inconsistent differences were observed across sex (4/7 found higher estimates among women), smoking status (only 1/4 find stronger associations among smokers), and more socio‐economically deprived people (1 out of 2). There were also other reviews suggesting that women in their menopause (1 review), non‐White population (1), and people with diabetes (1) are vulnerable subgroups.
This umbrella review summarized the epidemiological evidence of short‐ and long‐term exposure to PM and NO x and risk of multiple CVDs and indicated, overall, that exposure to increased levels of ambient air pollution was consistently associated with several important adverse cardiovascular health outcomes. We noted the largest amount of evidence for ambient air pollution and all‐cause CVD mortality, all‐cause CVD hospital admission, IHD, blood pressure, and stroke. Short‐term exposure to PM and NO x was consistently associated with MI, hypertension, and stroke mortality and stroke morbidity. By and large, long‐term exposure to PM 2.5 was associated with increased incidence of MI, atherosclerosis, incident hypertension, incident stroke, and stroke mortality. Few reviews focussed on heart failure, arrhythmias, and atrial fibrillation but most of them reported statistically significant positive associations. The reviews further suggested a stronger impact of air pollution on CVD outcomes in LMICs, especially in Asia where higher levels of air pollution are observed, and among specific population subgroups like the elderly, patients with preexisting CVD, and people with higher body mass index status.
Mechanistic support for CVD effects of air pollution exposure from animal and experimental studies
Studies evaluating the mechanisms underlying the effects of air pollution on CVD have increased substantially during the last decade. The most common initial pathways are (1) oxidative stress and inflammation, (2) autonomic nervous imbalance, and (3) direct particle translocation. These pathways may activate secondary pathways such as endothelial dysfunction, thrombotic pathways, activation of a hypothalamic and pituitary‐adrenal axis (HPA), and epigenomic changes (Fig. 7 ). The pathways are distinct and may occur at different time points and parts of the body, but they are highly interconnected with effects that may converge at some point to increase the risk of CVD outcomes [ 69 ]. In addition, many pathways share similar CVD intermediate endpoints, leading in varying degrees to arrhythmogenesis, increased blood pressure, atherosclerosis development, or increased arterial stiffness. These may in turn increase the risk of developing distinct CVD clinical events, including cardiac arrest, IHD, heart failure, stroke, and finally, CVD mortality. The relevant order or duration of air pollution exposure may activate different pathways at various stages. Some pathways may be activated after short‐term exposures (e.g., autonomic imbalance), and others may be promoted by long‐term exposure (e.g., atherosclerosis). Overall, the influence on the pathways will depend on the type of pollutant, dose and duration of exposure, specific cardiovascular endpoints, and individual health status [ 70 ].

Potential biological pathways between air pollution and cardiovascular diseases.
Controlled human exposures studies and toxicological studies have demonstrated that increased levels of air pollution may increase the sensitivity of the heart to ischemic stress through different pathways [ 9 , 10 , 71 ]. During the last decades, experimental studies have shown that short‐ and long‐term inhalation of PM 2.5 provokes oxidative stress and systemic inflammation [ 9 , 10 ]. Oxidative stress is a state where higher levels of free radicals, reactive oxygen species (ROS), and reactive nitrogen species are accumulated in different parts of the body, including the lungs, vascular bed, and even at a local cellular/tissue level. The disruption of redox signaling and excess ROS is suggested to increase adverse biological effects (e.g., lipid/protein/deoxyribonucleic acid [DNA] oxidation and initiation of proinflammatory cascades) and may alter cardiac and vascular function through the disruption of important redox‐sensitive signaling pathways, the depletion of vasodilators and antioxidants, the perturbation of cellular mechanisms, and the oxidation of proteins and lipids [ 10 , 69 ]. For example, PM 2.5 was shown to reduce antioxidant capacity in mice and decrease the contractility of cardiomyocytes [ 72 ]. Inflammation and oxidative stress are closely interconnected and both lead to a cascade of molecular processes that further affect vascular function and hemostasis [ 10 , 37 ]. These experimental studies have demonstrated that an increase of PM was associated with increases in both cellular and biochemical markers of pulmonary and systemic inflammation, including interleukin‐6 and C‐reactive protein, among others. The release of cytokines can alter hemostasis and increase the risk of thrombosis [ 10 , 73 ]. Nitrogen oxides have demonstrated a similar response to that of PM in both animal models and humans, starting with pulmonary inflammation and migration of inflammatory markers causing systemic inflammation and oxidative stress, which can result in activation of cells lining the blood vessels [ 9 ]. However, the evidence supporting the biological plausibility of NO x is still considered to be limited and inconsistent [ 9 ]. Overall, oxidative stress and systemic inflammation potentially induce several pathological responses (e.g., endothelial dysfunction, vasoconstriction, coagulation) that may increase the risk of IHD and MI, and other CVD outcomes [ 9 , 10 , 24 , 56 ]. Indeed, in controlled human exposure and toxicological studies, short‐term exposure to air pollutants has been demonstrated to increase blood viscosity and initiate thrombotic responses and hypercoagulability [ 9 , 10 , 37 , 74 ]. PM also causes impaired flow‐mediated dilation, vasoconstriction, temporary coronary occlusion, and ischemia in animal and human experimental studies, all suggested as potential mechanisms leading to cardiovascular morbidity including IHD and MI [ 8 ]. Further corroborating this, experimental human and animal model studies suggest progression and changes in atherosclerotic plaques and atherothrombotic events such as MI and ischemic stroke on exposure to air pollution [ 75 ].
The pathways between long‐term exposure to air pollution and, specifically, atherosclerosis can be explained by an interplay between plasma lipoproteins, inflammation, endothelial activation, neutrophil attraction to the endothelium, extravasation, and lipid uptake [ 10 ]. Animal studies in mice lacking apolipoprotein necessary for the clearance of fats and cholesterol found that air pollution accelerates atherosclerosis [ 10 , 76 ]. Another animal study provided evidence for PM 2.5 ‐mediated effects on atherosclerotic plaque progression in a genetically susceptible mouse model [ 77 ]. In addition, animal and human studies have observed that short‐ and long‐term exposure to air pollution can provoke persistent effects on vascular function, increasing arterial stiffness, impaired conduit artery flow‐mediated dilatation, arteriolar dysfunction, and retinal artery changes [ 78 ]. In human experiments, controlled short‐term exposures to PM 2.5 were associated with decreased brachial artery flow, increased vasoconstrictor endothelin‐1 in blood, and increased levels of vascular endothelial growth factor as a marker for vascular damage [ 42 , 79 – 81 ]. Long‐term exposure to air pollution may provoke the depletion of endothelial progenitor cells, explaining vascular remodeling effects [ 82 , 83 ]. Consistent with these vascular effects, a high concentration of PM has further been associated with increased plaque formation and decreased plaque stability in animal and human studies. Overall, short‐term changes in the endothelial function may provoke changes in vascular tone and stiffness, whereas long‐term changes may lead to increased cardiac afterload, diastolic dysfunction, alteration in coronary flow reserve, and left ventricular hypertrophy and fibrosis [ 9 , 10 ].
As has been previously mentioned, air pollution has been shown to induce inflammation, oxidative stress, and vascular effects such as endothelial dysfunction and arterial stiffness, all known contributors to hypertension. Additionally, daily changes in air pollution may trigger short‐term effects on blood pressure and hypertension by altering the autonomic nervous system (ANS) [ 10 , 48 ]. Inhalation of particles may stimulate the sympathetic nervous system, increasing the circulating levels of vasoconstrictors, elevating blood pressure, and decreasing the flow to the heart [ 9 , 10 ]. A study conducted by Wold et al. [ 72 ] found that, relative to controls, mice exposed long term to PM 2.5 had a statistically significant increase in systolic and diastolic blood pressure and mean arterial pressure, while pulse pressure decreased relative to controls. Although less studied, some toxicological evidence suggests that NO 2 may increase arterial stiffness, increase markers for CVD stability and progression and dyslipidemia, decrease heart‐rate variability (HRV), and reduce heart rate, but whether this reflects an independent effect of NO 2 is still not clearly distinguished in the available body of epidemiologic and toxicological evidence [ 9 ].
Emerging evidence suggests that prenatal and postnatal PM 2.5 exposure can result in heart failure later in life [ 84 ]. A study conducted by Gorr et al. in 2014 exposed female mice to PM 2.5 during pregnancy and found an association with reduced left ventricular fractional shortening with greater ventricular systolic diameter, reduced ejection fraction, and other indicators of cardiac dysfunction when compared to control mice during a postnatal time [ 85 ]. A similar study confirmed these findings of ventricular dysfunction as well as prolonged increased action potentials in isolated cardiomyocytes [ 86 ]. Another mice model study observed associations between short‐term exposures to UFP and decreased levels in isovolumic developed pressure and contractility compared with filtered air controls [ 87 ]. In addition, a controlled human study in both exercising heart‐failure patients and control patients found an association between increased levels of diesel exhaust with decreased left ventricular stroke volume (i.e., the amount of blood the left ventricle pumps per beat) [ 88 ]. These studies have confirmed that exposure to air pollution was sufficient to produce heart failure with anatomical evidence (dilated cardiomyopathy with ventricular volume changes, and ventricular wall thickening), functional measures (impaired pressure‐volume loops and deficits in contraction length), and cellular manifestation (delayed calcium reuptake during relaxation and reduced response to B‐adrenergic stimulation, increased cardiac collagen deposition) [ 85 ].
Animal studies provide evidence for ANS pathway alterations and resulting stroke [ 9 , 10 ]. Solid particles like PM can induce cerebral ischemia‐like phenotypes. Air pollutants caused features of stroke‐like neuronal loss and activation of microglia and astrocytes [ 89 ]. In rodent models, PM of nanoscale caused additive effects of air pollution and ischemic stroke on cerebral damage [ 90 ]. In vivo and in vitro models have provided evidence to suggest that air pollution would exacerbate stroke, with oxidative stress being an anticipated mechanism [ 91 ]. Oxidative stress was proposed to play a contributing role in the disruption of the blood–brain barrier after inhalation of vehicle exhausts [ 92 , 93 , 94 ]. Various types of PM can induce cellular‐molecular changes characteristic of the response to stroke (e.g., alterations in N ‐methyl‐ d ‐aspartate channel activity, dopaminergic and glutamatergic disruption) and are associated with inflammation and oxidative stress [ 89 ]. Direct exposure of diesel exhaust particles (DEP) to isolated brain capillaries increased oxidative stress and inflammation and may alter blood–brain barrier permeability [ 92 ]. While there is debate as to whether inhaled particles can access the brain in sufficient numbers and penetrate throughout cerebral tissue, results from in vitro findings offer a means by which inhalation of (the smallest ultrafine) particles could confer increased susceptibility to the consequences of a stroke [ 95 ].
Animal and experimental studies have demonstrated multiple pathways by which air pollution can lead to arrhythmias, atrial fibrillation, and cardiac arrests such as ANS alterations, oxidative stress, and inflammation [ 9 , 10 ]. Changes in the ANS contribute to abnormalities or worsening of arrhythmia measured in toxicological studies [ 96 , 97 ]. ANS alteration may increase heart rate parameters and atrial pressure, which can trigger ventricular arrhythmias, and atrial fibrillation increases the risk of related CVD events such as heart failure and stroke [ 9 , 10 ]. Alternatively, effects may be mediated through direct particle translocation across the blood–brain barrier, with the potential to modify autonomic nervous regulation and dysregulate heart rate, HRV, and blood pressure [ 10 , 78 ]. A human autopsy study demonstrated the presence of combustion‐derived magnetite particles in both the brain and the heart but identifying the precise contribution of pollutant translocation is still very difficult [ 98 ]. Pulmonary instillation of DEP in rats increased the incidence of cardiac arrhythmia and the extent of MI [ 99 ].
Other suggested pathways
Recent studies suggest that air pollution may affect HPA activation and may constitute an important pathway for CVD risk [ 69 ]. Hypothalamic activation may increase brown adipose dysfunction, white adipose inflammation, and insulin resistance, whereas adrenal axis activation may increase glucocorticoids [ 100 , 101 ]. Other pathways could be related to epigenomic changes. Some studies suggested novel associations between long‐term ambient air pollution exposure and site‐specific DNA methylation in purified monocytes. Inconsistent evidence on long‐term NO 2 exposure and genome‐wide DNA methylation needs to be studied further. Human observational studies have suggested that PM exposure may determine loss of methylation in blood DNA, potentially reflecting activation of proinflammatory states in blood leukocytes [ 102 , 103 , 104 ].
Collectively, animal, experimental, and epidemiological studies provide evidence for CVD effects of PM and NO x exposure through a number of known pathways for CVD development and death.
Geographic differences and vulnerable population
Results from our umbrella review are very similar to what was observed in the GBD in 2019, where it was noted that Asia had higher effect estimates for CVD mortality and hypertension among the global population [ 105 ]. Notably, countries with higher air pollution levels like India and China also have higher absolute numbers for DALYs compared to countries with lower pollution levels [ 106 ]. It was also noted that DALYs attributable to CVDs and ambient air pollution decreased among high‐income countries compared to other countries. The dose‐response function is steeper among more polluted areas, but this relationship seems to be nonlinear, and there is no lower concentration threshold that can be considered safe for the population [ 107 ]. Overall, the source and composition of pollutants vary vastly among and within countries; thus, an assessment of the impact of air pollution on CVD in any given exposure setting is dependent on using dose‐response functions relevant to the context of the population exposure [ 69 ].
In this umbrella review, we observed that vulnerable population groups like the elderly, cardiac patients, and adults with higher body mass index are at increased risk for air pollution–related cardiovascular health effects. The elderly population may be more susceptible as a function of the gradual decline of biological and physiological processes of the aging process, reducing their resilience to air pollution exposure. Populations with pre‐existing cardiovascular conditions may be predisposed to a higher risk for exacerbations of underlying disease processes. People with a higher body mass index may have a higher risk of cardiovascular events following ambient air pollution exposure, due to their altered state of blood coagulability and metabolism. We were unable to draw conclusions from this umbrella review on other potentially vulnerable groups such as those of different ethnicity, socioeconomic status, and gender differences mostly based on inadequate data availability.
Limitations and strength
The general strength of this umbrella review is that it provides a comprehensive overview of the effect of ambient air pollution on multiple cardiovascular outcomes. We were able to include a wide range of air pollutants and CVD outcomes, and we focused both on short‐ and long‐term exposures. We further harmonized all the effect estimates across the different meta‐analyses to increase comparability. However, some of the limitations should be considered. First, high heterogeneity was observed among the different meta‐analyses, which may weaken arguments for causality, although almost all estimates showed significant effects in the same direction. Second, the results of the umbrella review are limited to the evidence summarized in the meta‐analyses, which are prone to publication bias. However, most meta‐analyses did not find publication bias, but they cannot be fully ruled out. Third, we only included published systematic reviews and meta‐analyses until January 2021 and thus the most recent primary studies are likely not included in these reviews. This is especially relevant for new developing fields such as atrial fibrillation. Fourth, the quality of the meta‐analyses varied considerably with different search approaches and different effect estimates obtained from different study designs. However, we evaluated the quality of the reviews and most of them had an acceptable or high quality.
Research gaps and future research
Although there is increasing evidence to support that air pollution has an important effect on cardiovascular health, there are still gaps that must be addressed in future research studies. Most of the evidence is collected for PM 2.5 while other size fractions and components of PM are less well understood and would benefit from more research since toxicity might differ, as well as their source emissions. More research on multipollutant models, although methodologically challenging due to collinearity, might disentangle individual pollutant effects as well as explore the potential interaction between pollutants. This would better inform targeted interventions. From our review, we also observed that there is a need for more research on outcomes like heart failure, atherosclerosis, and specific arrhythmias such as atrial fibrillation, as well as more studies on NO x . Air quality should attend to safeguarding the health of vulnerable populations and research identifying these subpopulations is important to inform effective environmental health policy. In addition, it is clear that CVD effects of high levels of air pollution in LMICs warrant further research especially since these countries carry an enormous share of the burden of disease, have different source emissions than high‐income countries, and also potentially include other vulnerable populations based on housing conditions, concomitant diseases, nutritional status, and, for example, the additional burden of indoor air pollution from biomass burning. In the wake of the global COVID‐19 pandemic with large numbers of individuals with long‐term sequelae and possible lung injury and effects on immunity, we posit that it will be important to follow up on the potential consequences of air pollution exposure in these populations. Finally, specific air pollution mitigation programs are urgently needed to tackle adverse cardiovascular events, focusing on extremely polluted areas and vulnerable populations [ 5 , 9 , 10 , 108 , 109 ] and innovative designs evaluating the efficacy of interventions in reducing levels of air pollution and their effect on clinical CVD are warranted.
This comprehensive umbrella review provides strong evidence that increased levels of ambient air pollution increase the risk of CVDs and CVD mortality, especially from stroke and IHD. The large and consistent evidence of these reviews emphasizes the importance of developing interventions to reduce the alarming levels of air pollution across the globe, especially in Asia and among vulnerable subpopulations.
Conflict of interest
There is no conflict of interest to declare.
This review was funded by an honorarium from JIM. J. D. B. was supported by funding from the Swedish Research Council for Sustainable Development FORMAS, S. J. was supported by India GEOHealth Hub (U01TW010097), and P. L. was supported by the Strategic Research Area Epidemiology at Karolinska Institutet.
Author contributions
Jeroen de Bont: conceptualization; data curation; formal analyses; methodology; visualization; validation; writing – original draft (co‐lead); writing – review and editing (equal). Suganthi Jaganathan: conceptualization; data curation; formal analyses; methodology; visualization; validation; writing – original draft (co‐lead); writing – review and editing (equal). Marcus Dahlquist: conceptualization; writing – review and editing (equal). Åsa Persson: conceptualization; writing – review and editing (equal). Massimo Stafoggia: conceptualization; writing – review and editing (equal). Petter Ljungman: conceptualization; supervision; validation; writing – review and editing (equal).
Supporting information
Table S1 . Preferred Reporting Items for Systematic Review and Meta‐analysis (PRISMA‐P) guidelines of 2020.
Acknowledgment
The authors would like to acknowledge Gun Brit Knutssøn, KI Library, for assistance in the literature search.
de Bont J, Jaganathan S, Dahlquist M, Persson A, Stafoggia M, Ljungman P. Ambient air pollution and cardiovascular diseases: an umbrella review of systematic reviews and meta‐analyses . J Intern Med . 2022; 291 :779–800. [ PMC free article ] [ PubMed ] [ Google Scholar ]
Jeroen de Bont and Suganthi Jaganathan shared first authorship.
The impact of air quality on tourism: a systematic literature review
Journal of Tourism Futures
ISSN : 2055-5911
Article publication date: 27 March 2020
Issue publication date: 4 June 2021
Tourism may have important positive and negative economic, socio-cultural and environmental impacts. However, cultural and natural resources are also the base to the development of competitive destinations and changes in these resources can have an important impact on tourism development. Despite the considerable literature regarding the impacts of tourism, a limited number of studies examine the impact of the environment on tourism, specifically the impact of air quality (AQ). Therefore, this paper aims to review what is known about the impact of AQ on tourism demand, analysing the different methods and approaches used, as well as the results obtained.
Design/methodology/approach
A systematic literature review method was used to examine the state of the art in this topic and identify research gaps and new research directions. Only 26 papers were identified that examine the impact of AQ on tourism demand.
The majority of the studies were carried out in China and investigate the impact of AQ on tourism from the perspective of tourism demand. Both global (tourism demand) and individual (tourist perceptions) approaches have been used to investigate the impact of AQ on tourism.
Originality/value
This is the first systematic literature review on the impact of outdoor AQ on tourism demand. Moreover, this paper analyses the methods and approaches that have been used in the literature to examine the impact of outdoor AQ on tourism demand. The paper ends with a discussion on the identified research gaps concerning the influence of AQ on tourism development.
- Air quality
- Air pollution
- Systematic literature review
Eusébio, C. , Carneiro, M.J. , Madaleno, M. , Robaina, M. , Rodrigues, V. , Russo, M. , Relvas, H. , Gama, C. , Lopes, M. , Seixas, V. , Borrego, C. and Monteiro, A. (2021), "The impact of air quality on tourism: a systematic literature review", Journal of Tourism Futures , Vol. 7 No. 1, pp. 111-130. https://doi.org/10.1108/JTF-06-2019-0049
Emerald Publishing Limited
Copyright © 2020, Celeste Eusébio, Maria João Carneiro, Mara Madaleno, Margarita Robaina, Vítor Rodrigues, Michael Russo, Hélder Relvas, Carla Gama, Myriam Lopes, Vania Seixas, Carlos Borrego and Alexandra Monteiro
Published in Journal of Tourism Futures . Published by Emerald Publishing Limited. This article is published under the Creative Commons Attribution (CC BY 4.0) license. Anyone may reproduce, distribute, translate and create derivative works of this article (for both commercial and non-commercial purposes), subject to full attribution to the original publication and authors. The full terms of this license may be seen at http://creativecommons.org/licences/by/4.0/legalcode
1. Introduction
In the present era, one of the major motivations for travelling is to avoid the usual environment and seek a site with a pleasant location for releasing psychological stress and pressure ( Ritchie and Crouch, 2003 ). Therefore, environmental quality must be taken into account as an important factor in the decision-making process of potential tourists, as it can have a significant effect on the competitiveness of tourism destinations ( Zhang et al. , 2015 ; Becken et al. , 2017 ). However, the environment in some large tourism destination countries such as Egypt, China and India, is deteriorating with the progressive urbanization and industrialization. Beyond the “traditional” environmental problems such as garbage disposal and water pollution, the problem of haze pollution has risen in recent years, and air quality (AQ) has become a universal concern ( Chen et al. , 2017 ), now being incorporated into the wider category of environmental quality.
Ambient AQ should attract more academic attention than thermal comfort and the aesthetic dimension of the physical environment, as AQ is closely related to health risks ( Costa et al. , 2014 ). However, the existing literature places relatively low attention on the effects of AQ on tourism demand and the competitiveness of tourism destinations. Overall, the impacts of environmental and climate change issues on tourism have previously been discussed in several studies but few of them have focussed directly on the impacts of air pollution on the competitiveness of a tourism destination ( Saenz-de-Miera and Rosselló, 2013 ; Rossello-Nadal, 2014 ; Sajjad et al. , 2014 ). AQ also pertains to physical comfort, which is crucial to tourist experiences and has become a severe concern for human health. Medical evidence suggests that short and long-term exposures to ambient air pollution can engender a wide variety of acute and chronic health problems ( Seaton et al. , 1995 ; WHO, 2017). Moreover, AQ also affects aesthetic enjoyment, with people’s perception of reduced visibility, which is affected by the presence of particles and haze, which is being increasingly researched ( Rizzi et al. , 2014 ).
The objective of this paper is to review what is known and has been published, about the impact of outdoor AQ on tourism demand, using a systematic literature review method. To date, there is no literature review study on this topic. It is of note that the impact of tourism on AQ was also not addressed. This literature review aims to identify the geographical context, research methodologies used, researched subjects of the papers and the findings on the impact of AQ on tourism. This approach was adopted to provide contributions to guide future research, regarding which models should be used and what kind of data needs to be collected.
The paper is organized in various sections. In Section 2, the methodology used to select and analyse the papers is presented. In Section 3, the findings are reported, specifically the distribution of papers over time, among journals, subject areas, authorship and citations, the geographical contexts of the studies and the research methods used (data collection and data analysis methods), as well as the key results found. Finally, in Section 4, the main conclusions are summarized, research gaps are identified and some research questions are proposed to overcome these gaps.
2. Methodology
Several types of methodologies have been used in previous articles to conduct a literature review (e.g. systematic literature review, structured literature review, bibliometric analysis and systematic literature network analysis) ( Centobelli and Ndou, 2019 ; Comerio and Strozzi, 2019 ). The differences among these methodologies are mainly related to the research protocol used. In this paper, a systematic literature review is applied and the research protocol used encompasses two stages. First, a protocol was defined and put into practice to select the articles that will be analysed. Second, a content analysis of the articles selected was undertaken.
2.1 Articles selection
The protocol used to select the papers is reported in Figure 1 .
As presented in Figure 1 , the identification of the studies was carried out through a search on the Scopus database during January 2019, using the following search code – (“AQ” or “atmospheric emissions” or “air pollutants”) and (“tourism” or “visitor” or “tourist”) – in the article title, abstract and keywords without any restriction of time or subject. This database has been used in several previous studies ( Centobelli and Ndou, 2019 ; Comerio and Strozzi, 2019 ; Dann et al. , 2019 ) and is one of the largest databases of peer-reviewed literature (scientific journals, books and conference proceedings). A total of 488 records were obtained. Further, to include both scientific literature and “grey” literature, articles, reviews, articles in press and conference proceedings were selected. As a consequence of this first screening, the sample of records reduced to 383. Further, only documents written in English were selected. Papers written in other languages were excluded, given the complexities associated with translation. Based on this screening, 26 records were excluded.
Manual screening of the abstract titles and the abstracts of the 357 records was carried out by two authors (one specialized in AQ and the other in tourism) to verify if each paper was relevant to be included in this research. When the title and the abstract were not conclusive, the whole article was analysed. Concerning the inclusion criteria, it was decided to include both conceptual and empirical studies (qualitative and quantitative), where the influence of outdoor AQ on tourism demand of a tourism destination was analysed. Therefore, papers regarding the environmental impact of tourism on AQ and studies concerning indoor AQ of tourism attractions and facilities were excluded from this systematic literature review. A great number of documents only mention the topics of AQ and tourism in a superficial way, without an analysis of the impact of outdoor AQ on tourism demand. Consequently, only 20 papers were selected. To increase the sample of papers selected, two complementary searches were carried out on the Google Scholar database and Online Knowledge Library (b-on). From these analyses, only one additional paper was added. Further, the references of the 21 papers identified were analysed and 5 more papers were included in the sample. Consequently, a total of 26 papers were selected to be analysed in the second stage of this systematic literature review. This reduced number of papers clearly shows that this topic has been almost neglected in the literature, despite the great increase that the tourism industry has registered in the past few years and the increase in well-being and health concerns.
2.2 Articles analysis
The distribution over time;
Distribution by journal, scientific area and authorship; and
The distribution by the geographical context where the study was conducted.
The research methods (data collection and data analysis methods);
The dimensions of AQ and tourism demand investigated; and
The results obtained concerning the impact of outdoor AQ on the dimensions of tourism demand analysed.
This content analysis was complemented with an investigation of word frequencies (in title, abstract and keywords), using the Nvivo software, to identify, through word clouds, the terms most frequently used in this research field.
3. Findings
3.1 distribution of the papers over time.
A total of 26 papers were analysed. There seems to be a growing interest on the impact of outdoor AQ on tourism demand among researchers, as most of these papers were published in the past decade (88 per cent), mainly in 2017 and 2018 (12 and 42 per cent of the total set of papers were published in these years, respectively) ( Figure 2 ).
3.2 Journals, authorship and citations
There is a great variety among the authors of these papers. Almost all (98 per cent) wrote one paper and only Rob Law is the author of two. The papers are published in journals and conference proceedings of several areas, which reveals the relevance of this topic to different fields. However, there is a higher prevalence of papers in journals or proceedings from the field of “tourism, leisure and hospitality management” (38 per cent), with a notable contribution of “environmental science” (19 per cent), also having some papers published in journals or proceedings in the areas of “geography, planning and development” (8 per cent) and “health” or “medicine” (8 per cent) ( Table I ). It is important to notice that journals or proceedings can be related to more than one field, for example, environment and health. Papers have, on average, 7.2 citations in Scopus and 11.0 citations in Google Scholar. However, there is a high variation regarding citations, with many of the most recent papers (representing 19 per cent of the total) not yet having any citations in Scopus or Google Scholar, while 23 per cent have more than 20 citations in Scopus or in Google Scholar ( Table I ).
3.3 Geographical context
In terms of geographical areas, Figure 3 reveals that the spatial distribution of the published work addressing the impact of AQ on tourism is mainly focussed over the China and Southeast Asia regions.
China emerges as the country where most studies were conducted (about 42 per cent of the total), mainly in its capital (Beijing) with five specific studies focussed on this city (and not included in the six studies for China as a country shown in Figure 2 ). When other Asian countries are considered, the number of studies carried out in this region represents 58 per cent of the total studies analysed. Moreover, 16 per cent of the published studies were undertaken in the USA. A limited number of studies were conducted in European countries.
3.4 Most frequently used terms
The word clouds were generated through the qualitative analysis software NVivo 12, which allowed us to develop a word frequency query. To do so, this task separately analysed the word frequency in specific items of the mentioned papers, namely, the title, keywords and abstract. By default, the software automatically excludes the so-called “stop words”, which refer to conjunctions and/or prepositions and similar, which helped to substantially reduce the number of meaningless words. In each case, the words with a frequency equal to “1” were excluded, mainly because they may not be meaningful to the study objectives. Additionally, the following expressions, matching with the search terms used in the initial protocol, were also excluded: “AQ”, “atmospheric emissions”, “air pollutants”, “tourism”, “visitor” and “tourist”. To obtain more accurate results, the words were grouped according to the option “stemmed words”, which groups similar words such as “significant” and “significantly”.
Originally, the analysis generated 930 words in the case of the abstracts, 142 for the titles and 118 for keywords. By applying the above-mentioned filter, the final result was a total of 432, 31 and 38 words, respectively. Based on each list of frequencies, the software created the following word clouds ( Figure 4 ). Focussing on the top five of the most significant words, and as observed in Figure 4 , only the expressions “pollution” and “environmental” are common in the analysed lists. The words “impact”, “China” and “perceptions” also appear with high frequency in, at least, two of the three lists of frequencies and “parks” or “haze”, reflecting the common topics between the several considered studies.
3.5 Research methods
Most papers selected for this systematic review include empirical studies, only one is a literature review ( Zajchowski et al. , 2018 ). The literature review carried out by Zajchowski et al. (2018) differs from the one in the present paper, as it only focusses on the social and psychological effects of degraded AQ in and around parks and protected areas, and only analyses papers published in journals. In contrast, the analysis carried out in the present paper focussed, as already mentioned, on destinations, excluding specific attractions, but encompassed a wider range of documents, including papers published in conference proceedings. Only three papers – Hill et al. (2000) , Keiser et al. (2018) and Zhang et al. (2015) – are cited in the two literature reviews.
In this section, the methods used in the papers that include an empirical study to collect and analyse the data are described. It was considered useful to include a summary of the methodologies adopted to examine the influence of outdoor AQ on tourism. This would be helpful in future research to identify potential methodologies that may be used to assess this influence.
3.5.1 Type of data and data collection methods.
In terms of the type of data used, the observed sample of articles reviewed is well distributed between primary and secondary data ( Table II ). A total of 56 per cent of our sample used primary data. From these, 11 articles use questionnaires to explore the relationship between AQ and tourism. Only one study relies on interviews directed to tourists and two rely on experiments to extract primary data. The data sources used to collect secondary data correspond to diverse sources, which can be divided into quantitative data and online reviews. In total, 14 studies collect data from quantitative national and international sources to explore the AQ-tourism relationship and only two of the reviewed articles consider tourists’ online reviews as secondary source data. Moreover, some studies simultaneously use primary and secondary data sources to drive reliable conclusions, merging experiments or questionnaires with secondary data. At least Hipp and Ogunseitan (2011) , Jun-hui (2018) and Pant et al. (2018) simultaneously use primary and secondary data. To study visitors’ perceptions of AQ or to infer about the impact of air pollution on tourism, the joint use of questionnaires and evaluations collected from well-known travelling opinion surveys (such as in TripAdvisor; Saura et al. , 2018 ) can be particularly efficient and practical.
3.5.2 Data analysis methods.
Table IV presents a summary of the methodologies used by the authors considered in this literature review regarding the impacts of AQ on tourism. Most of the studies analysed use a quantitative analysis (92 per cent), while only 8 per cent (two studies) rely on qualitative analysis.
In terms of econometric methodologies, simple data analysis is the preferred method considering that it is complex/difficult to collect a long time series of data using questionnaires to build hypotheses and then test them. Even so, the content analysis could be another alternative when using qualitative data, but authors have only recently started using it ( Wu et al. , 2018 ; Saura et al. , 2018 ). When using quantitative data, Table IV shows that the most applied methods are those of ANOVA, linear regression analysis and panel data models. Simple descriptive statistics are used in 80 per cent of the studies analysed, where correlation analysis and t -tests are also commonly applied to explore data properties. The use of χ 2 tests and reliability analysis is less common.
More complex econometric models are used when authors use quantitative secondary data ( Tables II and III). These models allow having both a clearer picture of the quantitative impact of AQ on tourism and economic growth, as well as development at national and regional levels. The analysis suggests that using secondary data panel models and time series models (like VAR) allows for a more complete analysis of the relationship between AQ and tourism. Content analysis, which has not been used, can be used together with other methods to perform a more in-depth analysis of the influence of AQ on the tourism demand.
capture both static and dynamic interdependencies;
treat the links across units in an unrestricted fashion;
easily incorporate time variations in the coefficients and in the variance of the shocks;
account for cross sectional dynamic heterogeneities; and
use Granger causality and capture more complex correlation analysis.
Moreover, more qualitative studies should be carried out in this field to examine the influence of AQ on the behaviour and experiences of tourists. Table IV evidences the limitations, thus, far in terms of methodology analysis and provides valuable future research directions.
3.6 Variables used
3.6.1 dimensions of air quality..
The variables used in the various studies to measure AQ and its impact on tourism differ, essentially, between studies that examine the impact of AQ on visitors and studies that analyse the impact of AQ on global tourism demand. In the first group, a great number of studies use the perception of AQ and the perception of haze pollution ( Table IV ). In a smaller number of studies, visitor perception of the environmental risks, atmospheric contaminations, ozone depletion, fog and smog are analysed. Additionally, other studies use measurements of variables related to AQ rather than visitor perception such as the pollution standard index (PSI), days of haze, visibility, particulate matter (PM) and black carbon.
Articles that analyse the impacts of AQ on global tourism demand are no longer based on perception but quantitative AQ variables. Most studies are based on indices of air pollution (or AQ). There is another subset of studies that are based on emission variables (e.g. CO 2 , waste gases, methane, nitrous oxide and ozone). Moreover, there is a dispersion of studies that use other variables such as visibility, PM concentrations and haze.
3.6.2 Dimensions of tourism demand.
Individual visitors.
Global tourism demand.
In the first group, most of the studies examine the travel intention and destination choice. Moreover, the effect of AQ on tourism has also been analysed through the well-being and quality of life (QOL) variables (including physical and psychological health). A limited number of studies examined the impact of AQ on tourism experience, destination image, visitors’ satisfaction and type of activities carried out during a trip. In the second group, the studies focus on global tourism demand, mainly on the size of tourism flows (e.g. tourist arrivals, number of visitors, departures, international tourism receipts and expenditures) ( Table V ). However, several dimensions of tourism demand have been neglected in these studies such as the impact of AQ on destination competitiveness, emotions, memorability and post-travel behaviours.
3.7 Impact of air quality on tourism
Papers that analyse the impact of AQ on individual visitors.
Papers that examine the impact of AQ on global tourism demand.
3.7.1 Impact on individual visitors.
Travel behaviour, travel intention and destination choice;
Well-being and QOL;
Visitors’ satisfaction; and
Destination image.
3.7.1.1 Impact on travel behaviour, travel intention and destination choice.
A deep analysis of the results reveals that good AQ is likely to have a positive influence on travel behaviour, travel intention and destination choice ( Bohm and Pfister, 2011 ; Becken et al. , 2017 ; Hill et al. , 2000 ; Jun-hui, 2018 ; Law and Cheung, 2009 ; Zhang et al. , 2015 ; Zhu, 2018 ). This corroborates part of the findings of the literature review undertaken by Zajchowski et al. (2018) on the effects of good AQ on human behaviour. However, the variables representing AQ, the methodologies adopted and the tourism markets and destinations considered, vary across the studies. Zhu (2018) , Zhang et al. (2015) and Hill et al. (2000) analyse haze effects on tourism in Beijing, while Zhu (2018) examines how the haze affects the travel wishes and decisions of Beijing residents. The results show that for the majority of the residents, hazy weather has an impact on their willingness to travel and means of travel. More than 80 per cent of the respondents are not satisfied with travelling during fog and haze days. Moreover, differences in terms of age were identified, with young people (under the age of 20) being more reluctant to travel on haze days. Zhang et al. (2015) also analyse the potential impacts of haze pollution on the tourism industry of Beijing, through a questionnaire applied to potential tourists of this tourism destination. The results obtained reveal that haze pollution affects tourist choice of destinations and departure time. Differences are noticed among visitors with different travel purposes, with visitors travelling for sightseeing and leisure revealing a much higher concern regarding haze pollution than those travelling for business or visiting friends and relatives. Leading to visibility conditions that depend on haze affecting the likelihood of visiting the destinations in the future. Hill et al. (2000) analysed this topic for the White Mountain National Forest, NH (USA) and concluded that as visibility decreases, the probability of accepting visibility conditions tends to decrease and also that potential visitors planning a visit to the White Mountains in the future would be less likely to visit this protected area if the visibility conditions got worse. Finally, Jun-hui (2018) examines tourist perception of fog and haze in Xi’an (a world famous historical and cultural city in China). In this study, the tourists were categorized into three segments (“blunt type”, “normal type” and “sensitive type”) according to their perceptions of the fog and haze. Differences were obtained in terms of sociodemographic profiles and travel behaviour (before and during the trip). Tourists may not perceive the AQ conditions of the location they are visiting until they reach their home countries and feel the difference.
Other authors focussed on the tourist environmental risk perception and on the influence of this perception on present and future visits. Bohm and Pfister (2011) analyse traveller environmental risk perception in two tourism destinations with different environmental problems as follows: Australia (with ozone depletion) and Bangkok (with severe air pollution). Results show that travellers usually perceive lower risks than non-travellers, and that the decision to travel to environmentally afflicted destinations is related to people’s anticipated emotional response. Becken et al. (2017) also reveals that, to American and Australian citizens, feelings towards the air (affective risk perceptions) also have a negative impact on intentions to visit China. Law and Cheung (2009) concluded this when analysing international visitor perception of AQ (both indoor and outdoor) in Hong Kong. Their findings reveal that the respondents generally do not perceive the AQ in Hong Kong as a concern when they select this country as a tourism destination, but after their visit they considered the AQ in Hong Kong worse than in their home countries. Moreover, the results also reveal that many of the respondents were willing to pay an additional departure tax to fund improvements in AQ.
3.7.1.2 Impact on well-being and quality of life.
One set of studies ( Bohm and Pfister, 2011 ; Hipp and Ogunseitan, 2011 ; Pant et al. , 2018 ; Sato et al. , 2016 ) analyses the impact of AQ on well-being and QOL. Environmental problems experienced during the trip may affect travellers’ assessment of their QOL, as stated by Bohm and Pfister (2011) in a study based on Germans who travelled to environmentally afflicted destinations (Australia and Bangkok) and of Germans who did not travel. A study conducted in the California beaches by Hipp and Ogunseitan (2011) , using objective and subjective measures of AQ, highlights the important impact of AQ on some aspects of visitors’ QOL, through ordinal logistic models. The perceived AQ, measured using a scale from “very unhealthy” to “very healthy”, has a significant positive influence on the perception of psychological restorativeness as a whole and four dimensions of this construct – fascination, coherence, compatibility and legibility. Moreover, visitors are much more likely to perceive a higher psychological restorativeness in days considered as healthy AQ, taking into account an objective measurement of ground-level ozone concentrations. This is noticed for restorativeness as a whole and to its five dimensions considered in the study – being away, fascination, coherence, compatibility and legibility.
The impact of the exposure to air pollutants perceived by tourists and their potential health risks was also a focus in some studies such as Pant et al. (2018) and Sato et al. (2016) . The first study was carried out in Vietnam, concluding that the exposure of a tourist to air pollutants in Vietnam is lower than in cities in India and China. The second study was carried in China (Beijing) and confirmed the impact of short-term exposure to high concentrations of PM (PM 2.5 and PM 10) on tourist health, specifically on the cough reflex threshold, urge-to-cough and pulmonary function. Authors remark that a higher risk can exist in the case of unhealthy and health fragile groups (e.g. elderly, children and people with asthma or other pulmonary diseases).
3.7.1.3 Impact on visitors’ satisfaction
Only four studies ( Agarwal et al. , 2018 ; Peng and Xiao, 2018 ; Saura et al. , 2018 ; Wu et al. , 2018 ) examine the impact of AQ on visitor satisfaction. Factors influencing Chinese satisfaction with international self-drive holidays were analysed in Wu et al. (2018) . AQ is one of the factors analysed. Findings show that for the Chinese drive tourists, AQ has a positive and significant effect on their overall travel satisfaction. Moreover, Peng and Xiao (2018) also observed that in the case of domestic tourists of Beijing, the perception of experience risk produced by smog could cause travel dissatisfaction. Furthermore, Agarwal et al. (2018) examine whether hotel review scores provided by guests travelling in Singapore and Hong Kong in three online platforms – TripAdvisor.com , Agoda.com and Expedia.com – are influenced by haze episodes. Serious haze episodes showed to have a negative impact on online review scores, which reveals a decrease on guest satisfaction levels. Saura et al. (2018) analysed tweets of 25 Spanish hotels on social media, with the aim of investigating the experience of hotel guests. The authors try to identify environmental factors among comments grouped into negative and positive, according to guest feelings. Environmental factors related to atmospheric contamination emerge with considerable frequency, among negative and positive tweets. This means that these features are relevant for hotel guests and can trigger positive feelings when people perceive a good AQ. On the other hand, when contamination exists and there are risks of asthma and other breathing problems, negative feelings emerge.
3.7.1.4 Impact on destination image.
Despite that, theoretically, AQ is a relevant attribute to the competitiveness of tourism destinations, the research in this field is very scarce. Only the studies carried out by Becken et al. (2017) and Peng and Xiao (2018) investigate the impact of AQ on destination image. The model developed by Becken et al. (2017) is tested among Americans and Australians, and results reveal that feelings towards the air (affective risk perceptions) have a negative impact on both the cognitive and affective image of China. However, no significant differences are detected on feelings towards the risk of AQ between American and Australian citizens. Moreover, concerning China domestic tourists, the majority agree that smog has a negative influence on Beijing’s image, as concluded by Peng and Xiao (2018) .
3.7.2 Impact on global tourism demand.
There are also studies that address the impact of AQ on tourism but at the macro level. That is, not analysing the individual decisions of the tourist, but looking at the tourism industry of a country or region or a specific tourism attraction (such as a natural park). A consensual conclusion is that air pollution reduces the number of tourists ( Anaman and Looi, 2000 ; Deng et al. , 2017 ; Wang et al. , 2018 ; Sajjad et al. , 2014 ; Zhou et al. , 2018 ; Liu et al. , 2019 ; Keiser et al. , 2018 ; Yan et al. , 2019 ; Chen et al. , 2017 ). For instance, Anaman and Looi (2000) , using two different methodologies, estimated that 1997 and 1998 haze-related air pollution in Brunei caused about 3.75 or 28.70 per cent reduction in the number of tourists and that the total direct economic loss suffered by the tourism industry was estimated to be about B$1m to B$8m (respectively, for each methodology). Furthermore, these negative economic losses and negative impacts on tourist demand are very reliant on the phases of business cycles ( Chen et al. , 2017 ). Another example of clear evidence of negative impacts of bad AQ on tourists, as well as on human health, is the study of Keiser et al. (2018) , for the USA national parks. They found a strong negative relationship between ozone concentrations and park visitation.
The impact of AQ on tourism can vary when different pollutants are analysed. For instance, Liu et al. (2019) concluded that carbon dioxide has no significant impact on tourism while PM 2.5 has a significant negative impact. The reason for this result may be that the effect of PM 2.5 on air is that its more perceptible compared to CO 2 (colourless and odourless). Yan et al. (2019) concluded that SO 2 has the largest impact on tourism demand, followed by PM 2.5, NO 2 and PM 10, while CO (carbon monoxide) and O 3 seem to have little influence.
Another interesting result comes from Zhou et al. (2018) , who reveal that the negative impact on tourism is higher when pollution increases. For low levels of pollution, there are almost no perceived impacts, but as AQ further deteriorates, tourists become more alert to its adverse effect, and thus, the negative impact of air pollution occurs.
The impacts of AQ can also be different between domestic and international tourists ( Liu et al. ,2019 ), overnight visitors and one day visitors ( Poudyal et al. , 2013 ), as well as visitors and local residents ( Yan et al. , 2019 ). Liu et al. (2019) concluded that for China, domestic tourists are more sensitive than international tourists. The reason may be that international visitors are not able to perceive the AQ of China or have little or no information about this condition before travelling to the country. Poudyal et al. (2013) estimated elasticity for a national park in the USA, revealing that a programme aiming to improve the average visibility by 10 per cent (5.5 km) from the current level could result in an increase of about one million annual recreational visits. However, the increase would be higher for overnight visitors than for day visitors because the elasticity of tourism demand with respect to visibility was higher in overnight visitors. Yan et al. (2019) also estimated that the effect of pollutants is at least four times smaller on visitors than on local residents.
Time is also an important variable, as pollution worsens, the impact of pollution will last for a longer period and the degree of the impact in each time period will be more severe ( Zhou et al. , 2018 ). Some authors identify a delay effect in the impacts of AQ on tourism demand (five days in the case of Wang et al. (2018) and one month in the case of Zhou et al. (2018) . Poudyal et al. (2013) concluded that park visitations in a given month are significantly affected by the cumulative effect of the visibility condition in both the current and proceeding months.
Some authors have already indicated that the study of the impact of air pollution on tourism variables may be enriched with the introduction of some control variables such as tourism resource endowments, namely, the level of transport infrastructure, open-up degree, accommodation facilities ( Deng et al. , 2017 ) or disposable income level ( Wang et al. , 2018 ). Deng et al. (2017) also conclude that air pollution in nearby regions also affects local tourism, being the spillover effect (indirect effect) even larger than the direct effect.
4. Conclusions
A systematic review was performed to study the subject of impacts of AQ on tourism demand, analysing the different methods and approaches used to assess these impacts and the results obtained. A total of 26 papers were identified, with the majority published in the past decade (88 per cent) and quite restricted/focussed to China and Southeast Asia regions.
The reduced number of studies analysing the impact of AQ on tourism and the diversity of dimensions of AQ or tourism demand considered in these studies, make it difficult to draw conclusions on this kind of impact. However, some trends are observable. Both research on global tourism demand and on individual perceptions reveal that AQ tends to have a positive influence on tourism demand, with decreases in AQ leading to decreases in tourism flows or to a lower likelihood of visiting certain destinations.
The studies reviewed confirm that nobody would like to travel to those places where the environment is severely polluted. Compared with other environmental pollution such as water and soil pollution, air pollution is much more visible and can be perceived more easily by the public, which justifies the high impact that it may have on tourism in a given destination. Special attention should be devoted to avoiding high levels of pollution and bad conditions regarding AQ, as the impact of AQ on tourism tends to be higher when AQ is significantly reduced.
The results and conclusions of the present study can contribute to tourism management in similar destinations around the world. Hereby, more attention should continue to be devoted to inspecting the influences of any changes in atmospheric conditions on the demand for tourism, as extreme weather events are becoming more intense and more frequent.
Although some research studies have examined the negative impacts of air pollution on tourism, this important area of inquiry is still in its early stages and many critical issues have yet to be analysed and discussed. The most notable issues are how AQ influences the seasonality of tourism demand, tourism experience, attractiveness and competitiveness of tourism industry; and how it influences the magnitude and nature of the economic impacts of tourism industry. There is further lack of studies, which relate AQ impacts to population exposure (pollutant concentration multiplied by people and time). Therefore, more secondary data related to regional and national impacts of tourism activities has to be included into the analysis of the relationship between AQ and tourism. As previously mentioned, China and Asiatic countries have been extensively analysed under this issue. However, little is known about other regions and nations around the world where AQ levels are low or are significantly affected by high levels of air pollution.
Besides that, there is still a limited number of studies examining the role of AQ in the attractiveness and competitiveness of tourism destinations, and the question about the effects of AQ over tourism destination choices remains unanswered. Then, very little concern is devoted to good AQ and how the AQ index can become an important destination attribute influencing the attractiveness of tourism destinations.
Despite being a systematic literature review, this study has limitations that need to be addressed. The first is that the studies analysed, i.e. those focussed on the effects of outdoor AQ on tourism, are very scarce. This partially results from the option to only include studies focussed on outdoor AQ. As AQ is extremely linked to physical comfort and may have impacts on the tourist experience, future research should include studies on indoor AQ, providing the opportunity to strengthen the sample and to establish some comparisons between two different, but in a certain way, related matters.
Another limitation is the fact that most of the studies were focussed on Asian countries, with very few being conducted in other regions of the world. Although being an uncontrolled issue, there is an opportunity for future studies to be developed, helping to understand if the findings obtained in Asian countries are aligned and coherent with other regions, namely, in a context where environmental awareness is a growing phenomenon.
Finally, the option to only include papers in English can also be pointed out as a limitation, namely, because they may not be representative of all the evidence on the topic in analysis. Therefore, future research should be extended to included literature published in other languages.
Considering the great need of carrying out more studies, especially in other regions of the world beyond Asian countries, to ensure a sustainable development of tourism in the future, a wide plethora of methodological approaches may be adopted. As already mentioned, secondary data should be further explored. Moreover, mixed methodological approaches, including both quantitative and qualitative methods, should be privileged in further research to obtain a deeper knowledge of how AQ affects tourism demand and, consequently, the competitiveness of tourism destinations. Considering the environmental changes that have been occurring and that will take place in the future, longitudinal studies are also highly relevant to assess how the impact of AQ on tourism demand is changing and what kind of strategies should be designed to ensure sustainable development. The ongoing Portuguese research project ARTUR ( http://projeto-artur.web.ua.pt ) will address some of these concerns and its outcomes may help to increase the knowledge on both the environmental and tourism sectors, promoting both AQ protection and tourism sustainability.
Acknowledgements
Thanks are due for the financial support to FCT/MCTES through national funds, and the co-funding by the FEDER, within the PT2020 Partnership Agreement and Compete 2020, for the ARTUR project (POCI-01-0145-FEDER-029374) and CESAM (UID/AMB/50017 – POCI- 01-0145-FEDER-007638).
PRISMA flow diagram of the article selection process
Number of papers published by year of publication
Geographical location of the study areas focussed and covered by the reference
Word frequency represented by word clouds according to abstracts, titles and keywords
Information on the publication, authorship and citations (continues)
Methods of data analysis
Dimensions of AQ
Dimension of tourism demand
Agarwal , S. , Wang , L. and Yang , Y. ( 2018 ), “ Blessing in disguise? Environmental shocks and performance enhancement ”, ShanghaiTech SEM Working Paper No. 2018-012 , November . available at: http://ssrn.com/abstract=1330752 (accessed 24 January 2019 ).
Anaman , K. and Looi , C. ( 2000 ), “ Economic impact of haze-related air pollution on the tourism industry in Brunei Darussalam ”, Economic Analysis and Policy , Vol. 30 No. 2 , pp. 133 - 143 .
Becken , S. , Jin , X. , Zhang , C. and Gao , J. ( 2017 ), “ Urban air pollution in China: destination image and risk perceptions ”, Journal of Sustainable Tourism , Vol. 25 No. 1 , pp. 130 - 147 .
Bohm , G. and Pfister , H.-R. ( 2011 ), “ Tourism in the face of environmental risks: sunbathing under the ozone hole, and strolling through polluted air ”, Scandinavian Journal of Hospitality and Tourism , Vol. 11 No. 3 , pp. 250 - 267 .
Centobelli , P. and Ndou , V. ( 2019 ), “ Managing customer knowledge through the use of big data analytics in tourism research ”, Current Issues in Tourism , Vol. 1 , pp. 1 - 22 .
Chen , C.-M. , Lin , Y.-L. and Hsu , C.-L. ( 2017 ), “ Does air pollution drive away tourists? A case study of the sun moon lake national scenic area, Taiwan ”, Transportation Research Part D: Transport and Environment , Vol. 53 , pp. 398 - 402 .
Comerio , N. and Strozzi , F. ( 2019 ), “ Tourism and its economic impact: a literature review using bibliometric tools ”, Tourism Economics , Vol. 25 No. 1 , pp. 109 - 131 .
Costa , S. , Ferreira , J. , Silveira , C. , Costa , C. , Lopes , D. , Relvas , H. , Borrego , C. , Roebeling , P. , Miranda , A.I. and Paulo Teixeira , J. ( 2014 ), “ Integrating health on air quality assessment – review report on health risks of two major European outdoor air pollutants: PM and NO 2 ”, Journal of Toxicology and Environmental Health Part B Critical Review , Vol. 17 No. 6 , pp. 307 - 340 .
Dann , D. , Teubner , T. and Weinhardt , C. ( 2019 ), “ Poster child and guinea pig – insights from a structured literature review on Airbnb ”, International Journal of Contemporary Hospitality Management , Vol. 31 No. 1 , pp. 427 - 473 .
Deng , T. , Xin , L. and Mulan , M. ( 2017 ), “ Evaluating impact of air pollution on China’s inbound tourism industry: a spatial econometric approach ”, Asia Pacific Journal of Tourism Research , Vol. 22 No. 7 , pp. 771 - 780 .
Hill , L.B. , Halstead , J.M. , Stevens , T.H. and Kimball , K.D. ( 2000 ), “ Visitor perceptions and valuation of visibility in the great Gulf wilderness, New Hampshire ”, USDA Forest Service Proceedings , Vol. 5 , pp. 304 - 311 .
Hipp , J.A. and Ogunseitan , O.A. ( 2011 ), “ Effect of environmental conditions on perceived psychological restorativeness of coastal parks ”, Journal of Environmental Psychology , Vol. 31 No. 4 , pp. 421 - 429 .
Jun-Hui , Y. ( 2018 ), “ Research on the tourists’ type and behavior based on the fog and haze perception: taking Xi'an as a case ”, in Weng , C.H. & Weerasinghe , R. (Eds), Proceedings of 3rd International Conference on Advances in Energy and Environment Research , Vol. 53 , Les Ulis: E3S Web of Conferences .
Keiser , D. , Lade , G. and Rudik , I. ( 2018 ), “ Air pollution and visitation at US national parks ”, Science Advances , Vol. 4 No. 7 , eaat1613 .
Law , R. and Cheung , C. ( 2009 ), “ Air quality in Hong Kong: a study of the perception of international visitors ”, Journal of Sustainable Tourism , Vol. 15 No. 4 , pp. 390 - 401 .
Liu , J. , Pan , H. and Zheng , S. ( 2019 ), “ Tourism development, environment and policies: differences between domestic and international tourists ”, Sustainability , Vol. 11 No. 5 , pp. 1390 - 1405 .
Nejati , M. , Mohamed , B. and Omar , S.I. ( 2015 ), “ The influence of perceived environmental impacts of tourism on the perceived importance of sustainable tourism ”, e-Review of Tourism Research , Vol. 12 Nos 1/2 , pp. 99 - 114 .
Pant , P. , Huynh , W. and Peltier , R. ( 2018 ), “ Exposure to air pollutants in Vietnam: assessing potential risk for tourists ”, Journal of Environmental Sciences , Vol. 73 , pp. 147 - 154 .
Peng , J. and Xiao , H. ( 2018 ), “ How does smog influence domestic tourism in China? A case study of Beijing ”, Asia Pacific Journal of Tourism Research , Vol. 23 No. 12 , pp. 1115 - 1128 .
Poudyal , N.C. , Paudel , B. and Green , G.T. ( 2013 ), “ Estimating the impact of impaired visibility on the demand for visits to national parks ”, Tourism Economics , Vol. 19 No. 2 , pp. 433 - 452 .
Ritchie , J.R.B. and Crouch , G.I. ( 2003 ), The Competitive Destination? A Sustainable Tourism Perspective , CABI Publishing , Cambridge, MA .
Rizzi , L.I. , De La Maza , C. , Cifuentes , L.A. and Gómez , J. ( 2014 ), “ Valuing air quality impacts using stated choice analysis: trading off visibility against morbidity effects ”, Journal of Environmental Management , Vol. 146 , pp. 470 - 480 .
Rossello-Nadal , J. ( 2014 ), “ How to evaluate the effects of climate change on tourism ”, Tourism Management , Vol. 42 , pp. 334 - 340 .
Saenz-de-Miera , O. and Rosselló , J. ( 2013 ), “ Tropospheric ozone, air pollution and tourism: a case study of Mallorca ”, Journal of Sustainable Tourism , Vol. 21 No. 8 , pp. 1232 - 1243 .
Sajjad , F. , Noreen , U. and Zaman , K. ( 2014 ), “ Climate change and air pollution jointly creating nightmare for tourism industry ”, Environmental Science and Pollution Research , Vol. 21 No. 21 , pp. 12403 - 12418 .
Sato , R. , Gui , P. , Ito , K. and Kohzuki Ebihara , S. ( 2016 ), “ effect of Short-term exposure to high particulate levels on cough reflex sensitivity in healthy tourists: a pilot study ”, The Open Respiratory Medicine Journal , Vol. 10 No. 1 , pp. 96 - 104 .
Saura , J.R. , Palos-Sanchez , P. and Martin , M.A.R. ( 2018 ), “ Attitudes expressed in online comments about environmental factors in the tourism sector: an exploratory study ”, International Journal of Environmental Research and Public Health , Vol. 15 No. 3 , p. 553 .
Seaton , A. , Godden , D. , MacNee , W. and Donaldson , K. ( 1995 ), “ Particulate air pollution and acute health effects ”, Lancet (London, England)) , Vol. 345 No. 8943 , pp. 176 - 178 .
Wang , L. , Fang , B. and Law , R. ( 2018 ), “ Effect of air quality in the place of origin on outbound tourism demand: disposable income as a moderator ”, Tourism Management , Vol. 68 , pp. 152 - 161 .
World Health Organization [WHO] ( 2017 ), World Health Statistics 2018: monitoring Health for the SDGs, Sustainable Development Goals , World Health Organization , Geneva .
Wu , M.-Y. , Pearce , P.L. and Li , Q. ( 2018 ), “ Chinese behind the wheel: factors affecting their satisfaction with international self-drive holidays ”, Journal of Destination Marketing & Management , Vol. 9 , pp. 12 - 19 .
Yan , L. , Duarte , F. , Wand , D. , Zheng , S. and Ratti , C. ( 2019 ), “ Exploring the effect of air pollution on social activity in China using geotagged social media check-in data ”, Cities , Vol. 91 , pp. 116 - 125 .
Zajchowski , C.A.B. , Brownlee , M.T.J. and Rose , J. ( 2018 ), “ Air quality and the visitor experience in parks and protected areas ”, Tourism Geographies , Vol. 1 , doi: 10.1080/14616688.2018.1522546 .
Zhang , A. , Zhong , L. , Xu , Y. , Wang , H. and Dang , L. ( 2015 ), “ Tourists’ perception of haze pollution and the potential impacts on travel: reshaping the features of tourism seasonality in Beijing, China ”, Sustainability , Vol. 7 No. 3 , pp. 2397 - 2414 .
Zhou , B. , Qu , H. , Du , X. and Liu , F. ( 2018 ), “ Air quality and inbound tourism in China ”, Tourism Analysis , Vol. 23 No. 1 , pp. 159 - 164 .
Zhu , R. ( 2018 ), “ Analysis on the impact of haze on Beijing residents’ traveling intention and Decision-Making ”, IOP Conference Series: Materials Science and Engineering , Vol. 394 .
Further reading
Law , R. and Cheung , C. ( 1998 ), “ Prospects of the Hong Kong tourism industry ”, Hospitality Review , Vol. 16 No. 2 , pp. 39 - 51 .
Saenz-de-Miera , O. and Rosselló , J. ( 2014 ), “ Modeling tourism impacts on air pollution: the case study of PM10 in Mallorca ”, Tourism Management , Vol. 40 , pp. 273 - 281 .
Corresponding author
About the authors.
Celeste Eusébio is based at GOVCOPP, Department of Economics, Management, Industrial Engineering and Tourism, University of Aveiro, Aveiro, Portugal. She is an Assistant Professor of Tourism and a researcher at the GOVCOPP Research Unit at the University of Aveiro (UA) (Portugal). She holds a degree in Tourism Management and Planning from the UA (1995), a Master in Economics from the University of Coimbra (1998) and a doctoral degree in tourism, also from the UA (2006). Her research interests include tourism economics, tourism and sustainable development, tourism forecasts and consumer behaviour in tourism.
Maria João Carneiro is based at GOVCOPP, Department of Economics, Management, Industrial Engineering and Tourism, University of Aveiro, Aveiro, Portugal. She is Assistant Professor of Tourism at the University of Aveiro (UA) (in Portugal) and researcher at the Governance, Competitiveness and Public Policy (GOVCOPP) research unit at this University. She holds a degree in Tourism Management and Planning from UA, an MBA from New University of Lisbon and a PhD in tourism from UA. She has published papers in several journals and conference proceedings, and has also presented several works in conferences and seminars. Her research interests are competitiveness of tourism destinations, consumer behaviour in tourism and destination marketing.
Mara Madaleno is based at GOVCOPP, Department of Economics, Management, Industrial Engineering and Tourism, University of Aveiro, Aveiro, Portugal. She is Assistant Professor at the University of Aveiro (UA). Member of the Research Unit on Governance, Competitiveness and Public Policies (GOVCOPP), currently lectures Finance and Economics at the undergraduate, graduate MSc and PhD levels at the Department of Economics, Management, Industrial Engineering and Tourism. She is a co-author of scientific articles in peer-reviewed reference journals, books and book chapters in the areas of energy economics, finance and economics. Director of the Master in Economics (branches of Finance and Company Economics) at the DEGEIT and vice-director of the master in sustainable energy systems, both in UA.
Margarita Robaina is based at GOVCOPP, Department of Economics, Management, Industrial Engineering and Tourism, University of Aveiro, Aveiro, Portugal. She holds a PhD in Economics, a Master’s in Business Economics and a degree in Economics from the University of Aveiro (UA). She is a member of the Governance, Competitiveness and Public Policy Research Unit, in the Competitiveness, Innovation and Sustainability group. Its areas of interest are Energy Economics, Environmental and Natural Resources Economics and Energy and Environmental Policy. She is an Assistant Professor in the Department of Economics, Management, Industrial Engineering and Tourism at the UA since 2011 and is currently a member of the Executive Committee of the Department. Margarita has several publications with scientific arbitration and has participated in several conferences and projects.
Vítor Rodrigues is based at GOVCOPP, Department of Economics, Management, Industrial Engineering and Tourism, University of Aveiro, Aveiro, Portugal. He is a research fellow at the Department of Economics, Management, Industrial Engineering and Tourism, University of Aveiro (UA). With a Master in Tourism Management and Planning and a degree in Tourism from the UA, his research work has been focussed on Chinese Outbound Tourism, Meetings Industry and Tourism Governance, counting several scientific works as author and co-author.
Michael Russo is based at CESAM, Department of Environment and Planning, University of Aveiro, Aveiro, Portugal. He is a research fellow at the Department of Environment and Planning, University of Aveiro. With a BSc in Environmental Engineering (2014) and a MSc in sustainable energy systems (2016), he is a member of the GEMAC research group working in emissions modelling and regional air quality modelling.
Hélder Relvas is based at CESAM, Department of Environment and Planning, University of Aveiro, Aveiro, Portugal. He holds a PhD in Environmental Sciences and Engineering. The focus of his research work is air quality management using neural networks and Integrated Assessment Models to develop plans and measures to improve air quality. He is already author of more than 10 scientific papers and have participated in several national projects and international research projects.
Carla Gama is based at CESAM, Department of Environment and Planning, University of Aveiro, Aveiro, Portugal. She has a PhD in Environmental Sciences and Engineering. The focus of her research work is on the long-range transport of mineral dust from North Africa, its composition and deposition over the ocean. She has been participating in European and National research projects and collaborating with the Portuguese Environmental Agency regarding the air quality forecast system over Portugal.
Myriam Lopes is based at CESAM, Department of Environment and Planning, University of Aveiro, Aveiro, Portugal. She is an Assistant Professor of Environmental Engineering at the University of Aveiro (UA). She holds a PhD in Environmental Science from the UA (2007), a Master in Atmospheric pollution (2003) and a degree in Environmental Engineering. She is a researcher and vice-coordinator of CESAM – Centre for Environment and Marine Studies – associated laboratory. Her researcher topics cover air quality management and climate change mitigation and adaptation strategies, with emphasis on urbanized areas, including heath impacts, urban metabolism and sustainability. She was involved in several national and international research projects, as well as technical consultancy projects for society.
Vânia Seixas is based at CESAM, Department of Environment and Planning, University of Aveiro, Aveiro, Portugal. She holds a PhD Student Programme on Environmental Sciences and Engineering, Licensed degree in Environmental and Natural Resources Engineering (pre-Bologna treaty) at the University of Trás-os-Montes and Alto Douro (UTAD) in 2008. Master’s degree in Environmental Engineering in 2014. Participation in more than 10 technical-scientific events; Presented four oral communications, two of them in international congresses. Co-author of one book published by Principia Editora and of four technic-scientific reports. Is also co-author, as first author, of two scientific articles in indexed proceedings. Actuality she has a scholarship at the University of Aveiro.
Carlos Borrego is based at CESAM, Department of Environment and Planning, University of Aveiro, Aveiro, Portugal. He is Emeritus Full Professor of Environmental Engineering at the University of Aveiro (UA). During more than 40 years working on environmental problems, he is representing Portugal in international scientific organizations, in committees for research and evaluation of environmental strategy and sustainable development. He was Minister of Environment (XI and XII Governments), Vice-Rector for Research in the UA, Director of the Department of Environment and Planning, National Delegate to the EU Research and Innovation Framework Programs and Director of IDAD-Institute for Environment and Development, the interface unit of University of Aveiro for cooperation with the Society.
Alexandra Monteiro is based at CESAM, Department of Environment and Planning, University of Aveiro, Aveiro, Portugal. She holds a PhD in Environmental Science from the University of Aveiro (UA) (2007), a Master in Atmospheric pollution (2003) and a degree in Chemical Engineering, from the University of Porto in 1996. She was Assistant Professor since 2008 and is presently a Principal Researcher at UA, belonging to the CESAM – Centre for Environment and Marine Studies – associated laboratory. She has more than 60 SCI papers and her main research interests include gaseous and aerosol emission pollutants, in particular shipping emissions, air quality modelling and its impacts on climate and human health.
Related articles
We’re listening — tell us what you think, something didn’t work….
Report bugs here
All feedback is valuable
Please share your general feedback
Join us on our journey
Platform update page.
Visit emeraldpublishing.com/platformupdate to discover the latest news and updates
Questions & More Information
Answers to the most commonly asked questions here
A systematic review of air pollution as a risk factor for cardiovascular disease in South Asia: limited evidence from India and Pakistan
Affiliations.
- 1 Institute of Public Health, University of Heidelberg, Heidelberg, Germany. Electronic address: [email protected].
- 2 Institute of Public Health, University of Heidelberg, Heidelberg, Germany.
- 3 Federal Postgraduate Medical Institute, Lahore, Pakistan.
- PMID: 24064368
- DOI: 10.1016/j.ijheh.2013.08.003
Cardiovascular diseases (CVD) are major contributors to mortality and morbidity in South Asia. Chronic exposure to air pollution is an important risk factor for cardiovascular diseases, although the majority of studies to date have been conducted in developed countries. Both indoor and outdoor air pollution are growing problems in developing countries in South Asia yet the impact on rising rates of CVD in these regions has largely been ignored. We aimed to assess the evidence available regarding air pollution effects on CVD and CVD risk factors in lower income countries in South Asia. A literature search was conducted in PubMed and Web of Science. Our inclusion criteria included peer-reviewed, original, empirical articles published in English between the years 1990 and 2012, conducted in the World Bank South Asia region (Afghanistan, Bangladesh, Bhutan, India, Maldives, Nepal, Pakistan and Sri Lanka). This resulted in 30 articles. Nine articles met our inclusion criteria and were assessed for this systematic review. Most of the studies were cross-sectional and examined measured particulate matter effects on CVD outcomes and indicators. We observed a bias as nearly all of the studies were from India. Hypertension and CVD deaths were positively associated with higher particulate matter levels. Biomarkers of oxidative stress such as increased levels of P-selection expressing platelets, depleted superoxide dismutase and reactive oxygen species generation as well as elevated levels of inflammatory-related C-reactive protein, interleukin-6 and interleukin-8 were also positively associated with biomass use or elevated particulate matter levels. An important outcome of this investigation was the evidence suggesting important air pollution effects regarding CVD risk in South Asia. However, too few studies have been conducted. There is as an urgent need for longer term investigations using robust measures of air pollution with different population groups that include a wider range of air pollutants and outcomes, including early indicators of CVD. These regions are facing burdens from increasing urbanization, air pollution and populations, generally weaker health infrastructure, aging populations and increased incidence of non-communicable diseases, included CVD. The extent to which the problem of air pollution and CVD will impact these countries will depend largely on the information available to inform policy and programs, which are still lacking, political will as well as social and economic development.
Keywords: Biomarkers; Cardiovascular disease; Indoor air pollution; Inflammation; Outdoor air pollution; Particulate matter; South Asia.
Copyright © 2013 Elsevier GmbH. All rights reserved.
Publication types
- Research Support, Non-U.S. Gov't
- Systematic Review
- Air Pollutants / adverse effects*
- Air Pollution / adverse effects*
- Asia, Western
- Cardiovascular Diseases / etiology*
- Inflammation Mediators
- Oxidative Stress
- Particulate Matter / adverse effects*
- Risk Factors
- Air Pollutants
- Particulate Matter

IMAGES
VIDEO
COMMENTS
This review (178 published articles) is the first to systematically examine the psychological (affective, cognitive, behavioral), economic, and social effects of air pollution beyond its physiological and environmental effects. Affectively, air pollution decreases happiness and life satisfaction, and increases annoyance, anxiety, mental disorders, self-harm, and suicide.
Objective: To analyze the state of the art on the effects of air pollution on human health through a mapping review of existing systematic reviews and meta-analyses (SRs and MAs). Methods: The systematic mapping review was based on the recommendations for this type of scientific approach in environmental sciences. The search was performed using ...
The current work provides the most systematic assessment of the current literature on ambient air pollution and health. Multi-pollutant preventive approaches are required to enhance the comprehensiveness of the impacts of air pollution on human health. ... A systematic review of air pollution and incidence of out-of-hospital cardiac arrest. J ...
Indoor air pollution from unprocessed solid fuel use and pneumonia risk in children aged under five years: a systematic review and meta-analysis. Bull World Health Organ . (2008) 86 :390-4. 10.2471/BLT.07.044529 [ PMC free article ] [ PubMed ] [ CrossRef ] [ Google Scholar ]
We provide a comprehensive and updated systematic review and meta-analysis of the association between air pollution exposure and depression, searching PubMed, Embase, and Web of Sciences for relevant articles published up to May 2021, and eventually including 39 studies.
Ambient air pollution is the leading environmental risk factor for disease globally. Air pollutants can increase the risk of some respiratory infections, but their effects on tuberculosis (TB) are unclear. In this systematic literature review, we aimed to assess epidemiological studies on the associ …
Global population growth and increasing pollution levels are directly related. The effect does not just apply to outdoor spaces. Likewise, the low indoor air quality is also having a negative impact on the health of the building residents. According to the World Health Organization, indoor air pollution is a leading cause of 1.6 million premature deaths annually. Tackling this public health ...
Search strategy, study selection, and data extraction. We conducted a systematic literature review using the Navigation Guide, a methodology for evaluating environmental evidence based on methods used in the clinical sciences [].The objective of this systematic review is to assess whether there are interactions between exposure to criteria air pollutants, extreme heat, and pollen, or a subset ...
This systematic review summarises and evaluates the literature investigating associations between exposure to air pollution and general population cognition, which has important implications for health, social and economic inequalities, and human productivity. The engines MEDLINE, Embase Classic+Embase, APA PsycInfo, and SCOPUS were searched up to May 2022. Our inclusion criteria focus on the ...
Abstract. A wide range of policies, strategies, and interventions have been implemented to improve air quality all over the world. This systematic review comprehensively appraises the policies and strategies on air pollutants controls enacted in different countries, worldwide. Three databases, Web of Science, PubMed and Scopus, were used for ...
With the continuous acceleration of urbanization, air pollution has become an increasingly serious threat to public health. Strengthening the detection and control of pollutants has become a focal point in current society. In light of the increasing amount of literature in the field of air pollution control with every passing year, numerous reviews have been compiled; however, only a limited ...
The aim of this study is to provide an updated systematic literature review of peer-reviewed epidemiological studies that examined the health impacts of outdoor air pollution in Australia, including short- and long-term exposure. Following PRISMA guidelines, we conducted a systematic literature review. Broad search terms were applied to two ...
Air pollution, currently on several global health agendas, has rapidly become a global problem with the increasing global urbanisation, transportation-related emissions, and increased energy consumption. ... This was a systematic literature review and meta-analysis of articles published in English from January 1st, 1946, through May 31st, 2022 ...
A systematic literature review and critical appraisal of epidemiological studies on outdoor air pollution and tuberculosis outcomes. Environ. Res. 170 , 33-45 (2019).
(1) Background: There is increasing awareness that the quality of the indoor environment affects our health and well-being. Indoor air quality (IAQ) in particular has an impact on multiple health outcomes, including respiratory and cardiovascular illness, allergic symptoms, cancers, and premature mortality. (2) Methods: We carried out a global systematic literature review on indoor exposure to ...
This is the first systematic mapping review carried out on the effects of air pollution on health, considering only systematic reviews and meta-analysis. The current mapping review includes relevant SRs and MAs to provide a more complete picture of the work performed thus far in one of the most important fields of research.
Background Air-pollution and weather exposure beyond certain thresholds have serious effects on public health. Yet, there is lack of information on wider aspects including the role of some effect modifiers and the interaction between air-pollution and weather. This article aims at a comprehensive review and narrative summary of literature on the association of air-pollution and weather with ...
We conducted a systematic literature review on the application of data mining and machine learning methods in air pollution epidemiology. We carried out our search process in PubMed, the MEDLINE database and Google Scholar. Research articles applying data mining and machine learning methods to air pollution epidemiology were queried and reviewed.
Search strategy. The present systematic review was performed according to standard methods the Preferred Reporting Items for Systematic Review and Meta-Analysis (PRISMA) [].A comprehensive screening approach to find the urban air pollution control strategies and policies published in peer-reviewed journal was performed up to 19 January 2021 in three scientific databases: PubMed, Web of Science ...
Infant mortality is a widely reported indicator of population health and a leading public health concern. In this systematic review and meta-analysis, we review the available literature for epidemiologic evidence of the association between short-term air pollution exposure and infant mortality. Relevant publications were identified through PubMed and Web of Science databases using ...
The available evidence on the effects of ambient air pollution on cardiovascular diseases (CVDs) has increased substantially. In this umbrella review, we summarized the current epidemiological evidence from systematic reviews and meta‐analyses linking ambient air pollution and CVDs, with a focus on geographical differences and vulnerable subpopulations.
IoT-based applications may be considered as reliable and cheap alternatives for indoor and outdoor pollution monitoring, and the synthesis of existing research, knowledge gaps, associated challenges, and future recommendations is made. Global population growth and increasing pollution levels are directly related. The effect does not just apply to outdoor spaces. Likewise, the low indoor air ...
The adequate assessment and management of indoor air quality in healthcare facilities is of utmost importance for patient safety and occupational health purposes. This study aims to identify the recent trends of research on the topic through a systematic literature review following the preferred reporting items for systematic reviews and meta-analyses (PRISMA) methodology. A total of 171 ...
This systematic review (Lam et al., 2016) was the first systematic review and metaanalysis of the literature on the association between autism spectrum disorder (ASD) and air pollution and ultimately concluded that there was "limited evidence of toxicity." While there was a relatively rich database of human evidence, we found several ...
The objective of this paper is to review what is known and has been published, about the impact of outdoor AQ on tourism demand, using a systematic literature review method. To date, there is no literature review study on this topic. It is of note that the impact of tourism on AQ was also not addressed.
However, the impact of air pollution on body weight at a population level remains inconclusive. This systematic review and meta-analysis will estimate the association of ambient air pollution with obesity, distribution of ectopic adipose tissue, and the incidence and prevalence of non-alcoholic fatty liver disease among adults.
Research has indicated that certain environmental exposures may increase the risk of unprovoked seizures and new onset epilepsy. This study aimed to synthesize the literature that has estimated the associations between short- and long-term exposure to outdoor air and noise pollution and the risk of unprovoked seizures and new onset epilepsy.
A systematic review of air pollution as a risk factor for cardiovascular disease in South Asia: limited evidence from India and Pakistan Int J Hyg Environ Health. 2014 Mar ... A literature search was conducted in PubMed and Web of Science. Our inclusion criteria included peer-reviewed, original, empirical articles published in English between ...
Air pollution has a ubiquitous impact on ecosystem functioning through myriad processes, including the acidification and eutrophication of soil and water, deposition of heavy metals and direct (and indirect) effects on flora and fauna. Describing the impacts of air pollution on organisms in the field is difficult because levels of exposure do not occur in a uniform manner across space and time ...
A systematic literature review and critical appraisal of epidemiological studies on outdoor air pollution and tuberculosis outcomes. ... Childhood tuberculosis and exposure to indoor air pollution: a systematic review and meta-analysis. Int. J. Tuberc. Lung Dis, 19 (5) (2015), pp. 596-602, 10.5588/ijtld.14.0686.