Thank you for visiting nature.com. You are using a browser version with limited support for CSS. To obtain the best experience, we recommend you use a more up to date browser (or turn off compatibility mode in Internet Explorer). In the meantime, to ensure continued support, we are displaying the site without styles and JavaScript.
- View all journals
- My Account Login
- Explore content
- About the journal
- Publish with us
- Sign up for alerts
- Review Article
- Open access
- Published: 15 January 2018

Impact of remote patient monitoring on clinical outcomes: an updated meta-analysis of randomized controlled trials
- Benjamin Noah 1 , 2 ,
- Michelle S. Keller 1 , 2 , 3 ,
- Sasan Mosadeghi 4 ,
- Libby Stein 1 , 2 ,
- Sunny Johl 1 , 2 ,
- Sean Delshad 1 , 2 ,
- Vartan C. Tashjian 1 , 2 , 5 ,
- Daniel Lew 1 , 2 , 5 ,
- James T. Kwan 1 , 2 ,
- Alma Jusufagic 1 , 2 , 3 &
- Brennan M. R. Spiegel 1 , 2 , 3 , 5 , 6
npj Digital Medicine volume 1 , Article number: 20172 ( 2018 ) Cite this article
53k Accesses
140 Citations
655 Altmetric
Metrics details
- Disease prevention
- Health services
- Weight management
An Author Correction to this article was published on 09 April 2018
This article has been updated
Despite growing interest in remote patient monitoring, limited evidence exists to substantiate claims of its ability to improve outcomes. Our aim was to evaluate randomized controlled trials (RCTs) that assess the effects of using wearable biosensors (e.g. activity trackers) for remote patient monitoring on clinical outcomes. We expanded upon prior reviews by assessing effectiveness across indications and presenting quantitative summary data. We searched for articles from January 2000 to October 2016 in PubMed, reviewed 4,348 titles, selected 777 for abstract review, and 64 for full text review. A total of 27 RCTs from 13 different countries focused on a range of clinical outcomes and were retained for final analysis; of these, we identified 16 high-quality studies. We estimated a difference-in-differences random effects meta-analysis on select outcomes. We weighted the studies by sample size and used 95% confidence intervals (CI) around point estimates. Difference-in-difference point estimation revealed no statistically significant impact of remote patient monitoring on any of six reported clinical outcomes, including body mass index (−0.73; 95% CI: −1.84, 0.38), weight (−1.29; −3.06, 0.48), waist circumference (−2.41; −5.16, 0.34), body fat percentage (0.11; −1.56, 1.34), systolic blood pressure (−2.62; −5.31, 0.06), and diastolic blood pressure (−0.99; −2.73, 0.74). Studies were highly heterogeneous in their design, device type, and outcomes. Interventions based on health behavior models and personalized coaching were most successful. We found substantial gaps in the evidence base that should be considered before implementation of remote patient monitoring in the clinical setting.
Similar content being viewed by others
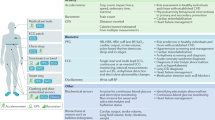
Smart wearable devices in cardiovascular care: where we are and how to move forward
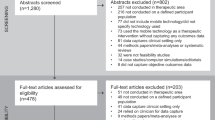
A systematic review of feasibility studies promoting the use of mobile technologies in clinical research
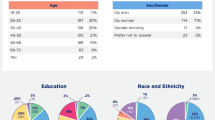
Wearable fitness tracker use in federally qualified health center patients: strategies to improve the health of all of us using digital health devices
Introduction.
Wearable biosensors are non-invasive devices used to acquire, transmit, process, store, and retrieve health-related data. 1 Biosensors have been integrated into a variety of platforms, including watches, wristbands, skin patches, shoes, belts, textiles, and smartphones. 2 , 3 Patients have the option to share data obtained by biosensors with their providers or social networks to support clinical treatment decisions and disease self-management. 4
The ability of wearable biosensors to passively capture and track continuous health data gives promise to the field of health informatics, which has recently become an area of interest for its potential to advance precision medicine. 1 The concept of leveraging technological innovations to enhance care delivery has many names in the healthcare lexicon. The terms digital health, mobile health, mHealth, wireless health, Health 2.0, eHealth, quantified self, self-tracking, telehealth, telemedicine, precision medicine, personalized medicine, and connected health are among those that are often used synonymously. 5 A 2005 systematic review uncovered over 50 unique and disparate definitions for the term e-health in the literature. 6 A similar 2007 study found 104 individual definitions for the term telemedicine. 7 For the purpose of this study, we employ the term remote patient monitoring (RPM) and define it as the use of a non-invasive, wearable device that automatically transmits data to a web portal or mobile app for patient self-monitoring and/or health provider assessment and clinical decision-making.
The literature on RPM reveals enthusiasm over its promises to improve patient outcomes, reduce healthcare utilization, decrease costs, provide abundant data for research, and increase physician satisfaction. 2 , 3 , 8 Non-invasive biosensors that allow for RPM offer patients and clinicians real-time data that has the potential to improve the timeliness of care, boost treatment adherence, and drive improved health outcomes. 4 , 9 The passive gathering of data may also permit clinicians to focus their efforts on diagnosing, educating, and treating patients, theoretically improving productivity and efficiency of the care provided. 8 However, despite anecdotal reports of RPM efficacy and growing interest in these new health technologies by researchers, providers, and patients alike, little empirical evidence exists to substantiate claims of its ability to improve clinical outcomes, and our research indicates many patients are not yet interested in or willing to share RPM data with their physicians. 4 A recently published systematic review by Vegesna et al. summarized the state of RPM but provided only a qualitative overview of the literature. 10 In this review, we provide a quantitative analysis of RPM studies to provide clinicians, patients, and health system leaders with a clear view of the effectiveness of RPM on clinical outcomes. Specifically, our study questions were as follows: How effective are RPM devices and associated interventions in changing important clinical outcomes of interest to patients and their clinicians? Which elements of RPM interventions lead to a higher likelihood of success in affecting clinically meaningful outcomes?
We sought to identify randomized controlled trials (RCTs) that assess the effects of using non-invasive, wearable biosensors for RPM on clinical outcomes. Understanding precisely in which contexts biosensors can improve health outcomes is important in guiding research pathways and increasing the effectiveness and quality of care.
Study selection and data collection
We identified 4348 titles for review (Fig. 1 ). Of these, we selected 777 for abstract review and 64 for full-text review. A total of 27 studies were retained for final analysis. 11 , 12 , 13 , 14 , 15 , 16 , 17 , 18 , 19 , 20 , 21 , 22 , 23 , 24 , 25 , 26 , 27 , 28 , 29 , 30 , 31 , 32 , 33 , 34 , 35 All studies were RCTs published in peer-reviewed journals.
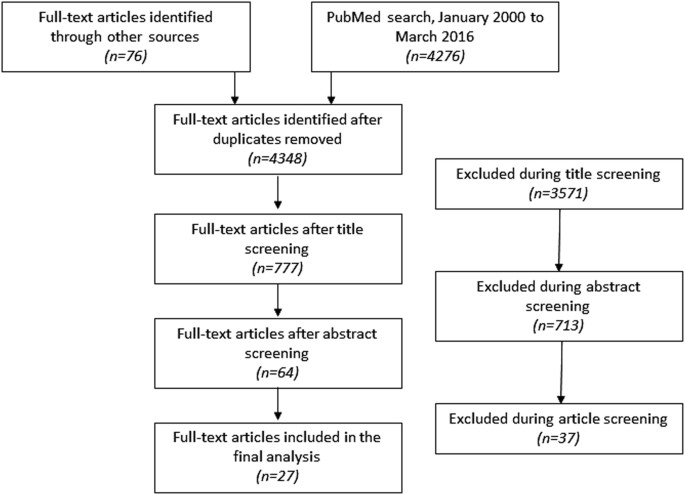
PRISMA flow diagram of the process used in study selection
Quantitative identifiers
Study details.
The 27 studies analyzed had an average study duration of 7.8 months. The study periods ranged from 7 days to 29 months (Table 1 ). The average sample size was 239 patients and ranged from 40 to 1437 patients. Sixteen studies were determined to be of high quality, with a Jadad score equal to 3. Eleven studies were determined to be of low quality. The mean Jadad score for all 27 studies identified in this review was 2.44 (Table 1 ). Since it is often not feasible to double-blind interventions with wearable devices, the maximum Jadad score in these trials was 3.
Study outcomes
Eleven studies examined patient populations with cardiovascular disease, including heart failure, arrhythmias, and hypertension. Six studies evaluated patients with pulmonary diseases, including emphysema, asthma, and sleep apnea. Six trials examined overweight or obese patients or tested interventions aimed at increasing physical activity to prevent weight gain. The remaining studies focused on chronic pain, stroke, and Parkinson’s disease (Table 2 ).
Devices and interventions
RPM devices employed in these studies included blood pressure monitors, ambulatory electrocardiograms, cardiac event recorders, positive airway pressure machines, electronic weight scales, physical activity trackers and accelerometers, spirometers, and pulse oximeters. The control arms of most studies offered education along with standard care but without RPM; however, eight studies used other, similar devices. One study included various types of behavioral economics incentives (either donations to charity or cash incentives) in addition to the biosensors. 36
Twenty-two study interventions contained a feedback loop with a care provider, such as a physician or nurse, who analyzed patient data and communicated back with the patient to modify treatment regimens, improve adherence, or consult. Only five study interventions contained a feedback loop where a care provider was not involved. In those instances, patients logged onto a web portal or mobile app to self-monitor their measurements and view a synthesis of their personal health data.
Qualitative review of high-quality studies
We examined the interventions, theoretical frameworks, and outcomes of the 16 high-quality studies by outcome or disease focus to determine if there were common intervention elements that resulted in greater effects on health and resource outcomes.
Remote patient monitoring for high-acuity patients: chronic obstructive pulmonary disease and heart failure
Five high-quality studies compared RPM with usual care for high-acuity patients with chronic obstructive pulmonary disease (COPD) or heart failure. 11 , 16 , 25 , 31 , 35 In Chau et al., 40 participants with a previous hospitalization and diagnosed with moderate or severe COPD were randomized to usual care or a telecare device kit that provided patient feedback and was monitored by a community nurse. 11 Although several participants experienced technical problems using the device kit, participants expressed greater engagement in self-management of their COPD overall. Nonetheless, the study found no positive effects in any of the primary outcomes when compared to usual care. As the authors note, the study was underpowered and had a short follow-up period of 2 months. In De San Miguel et al., the intervention was similar: 80 participants received telehealth equipment that monitored vital signs daily and was observed by a telehealth nurse. 25 Patients in the intervention group experienced reductions in hospitalizations, emergency department visits, and length of stay, but none of the reductions were statistically significant when compared to the control group. Even so, the costs savings were $2931 per person, suggesting that a study with more power could potentially see significant cost and utilization savings. Dinesen et al. used a similar study design: 111 participants with COPD were randomized to receive telecare kits or usual care. 31 The study found reductions in hospital admissions and lower costs of admissions in the intervention group, but only the mean hospital admission rate was statistically significant. Likewise, Pedone et al. followed 99 participants with COPD randomized to RPM or usual care, and found that the number of exacerbations and exacerbation-related hospitalizations dropped in the intervention group, but neither result was significant. 35 The BEAT-HF trial by Ong et al. followed 1437 participants hospitalized with heart failure who were randomized to RPM or usual care. 16 Centralized nurses actively monitored the RPM data. The researchers found no differences in 180-day all-cause readmissions between the two groups. Four studies demonstrate the promise of RPM for COPD-related hospitalizations and costs; longer follow-up periods and larger sample sizes are needed to determine the full effect of RPM on COPD outcomes. The use of measures such as the Patient Activation Measure 37 in future studies could identify whether factors such as engagement and self-efficacy are important moderators of healthcare outcomes. More evidence is needed to determine whether heart failure is amenable to RPM; these patients may require more intensive follow-up care and may not be the ideal target population for RPM.
Remote patient monitoring for chronic disease: hypertension
Two high-quality studies focused on hypertension. 15 , 23 Kim et al. examined 374 patients randomized to (1) home blood pressure monitoring, (2) remote monitoring using a wireless blood pressure cuff with clinician follow-up, or (3) remote monitoring without clinician follow-up. 23 There were no differences observed in the primary endpoint, sitting systolic blood pressure, in the three groups. However, subjects over 55 years old with remote monitoring (with or without clinician follow-up) experienced significant decreases in the adjusted mean sitting systolic blood pressure when compared to the control group. These results indicate that for a select group of patients, RPM could be effective in hypertension treatment. Logan et al. provided home blood pressure telemonitoring with self-care messages on a smartphone after each reading for patients in the intervention group. 15 Messages were tailored based on care pathways defined by running averages of blood pressure measurements. Physicians were alerted if patients’ blood pressure crossed specific pre-set thresholds and regular feedback was provided to patients and clinicians. Systolic blood pressure decreased in the intervention group; however, self-care smartphone-based support also appeared to worsen depression scores. These studies illustrate that tailored RPM interventions based on care pathways can effectively reduce blood pressure for select groups of patients, but researchers should examine adverse consequences such as depression and other patient-reported outcomes when designing interventions that include continuous monitoring.
Remote patient monitoring for rehabilitation: stroke, Parkinson’s disease, low back pain, and hand function
Four high-quality studies focused on providing feedback regarding various aspects of mobility rehabilitation, including stroke or Parkinson’s disease rehabilitation, low back pain physiotherapy, or hand function physical therapy. 20 , 24 , 27 , 28 Dorsch et al. recruited 135 participants with stroke of any type from 16 rehabilitation centers in 11 countries. 24 All participants wore wireless ankle tri-axial accelerometers while performing conventional rehabilitation exercises; intervention participants received and reviewed augmented feedback with therapists who used the wireless device data, while the control group received standardized verbal feedback from therapists. The researchers found no significant difference in the average daily time spent walking between groups throughout the duration of the trial. The researchers theorized that because participants walked such short amounts of time per day (a mean daily time of eight minutes in the severe group and 12 minutes in the moderate group), there was insufficient time to use the data provided by the wireless devices.
In Ginis et al., 40 participants with Parkinson’s disease undergoing gait training were randomized to home visits from the researcher who provided training on using a smartphone application and ankle-based wireless devices that offered positive and corrective feedback on gait, or an active control, in which they received personalized gait feedback from the same researcher during home visits. 20 Both groups improved on the primary outcomes (single- and dual-task gait speed), but patients using the app and wireless devices improved significantly more on balance and experienced less deterioration over the six-week period.
Kent et al. randomized 112 participants in eight clinics between wearing active wireless motion sensors placed along the spine, and placebo sensors while receiving physical therapy and guideline-based care. 28 Participants received six to eight physical therapy treatment sessions over 10 weeks and were followed for a year. Patients in the intervention group experienced significantly less pain and improved function compared to the control group. Designed as a pilot study, Piga et al. assigned 20 patients with systemic sclerosis or rheumatoid arthritis to use a self-managed hand kinesiotherapy protocol assisted by an RPM device. 27 The device provides both visual and audio feedback on strength-, mobility-, and dexterity-based therapy. The control group received the kinesiotherapy protocol alone. Both groups improved over time, but there was no statistically significant difference in primary outcomes between the two groups. The researchers found, however, that measured adherence to the home-based RPM therapy was very high (90%). These four studies demonstrate mixed results on the use of RPM in rehabilitation but suggest potential insights. First, RPM is most useful in settings where there are clearly defined opportunities to use the data to change clinical care. For example, in the study examining stroke rehabilitation, participants did not walk enough throughout the day to effectively use the feedback. The study examining Parkinson’s disease rehabilitation, however, provided ample opportunities for participants to use the feedback over a six-week period, and participants saw important changes in clinical outcomes. Second, adherence to home-based rehabilitation therapy might be an important process outcome that could be included in future studies. Finally, using placebo sensors such as those used in Kent et al. is an important way to increase the validity and reliability of these studies.
Remote patient monitoring for increasing physical activity: overweightness and obesity
Five studies examined whether RPM could increase physical activity and combined activity monitors with a variety of behavioral interventions, including text messaging, personalized coaching, group-based behavior therapy, or cash- or charity-based incentives. In Wang et al., 67 participants who were overweight or obese were randomly assigned to wear a Fitbit One activity tracker alone or to wear the activity tracker combined with receiving physical activity prompts three times a day via text messages. 32 The researchers found that both groups wearing the Fitbit devices saw a small increase in moderate-to-vigorous physical activity. Participants receiving the automatic text message prompts saw a small additional increase in activity that lasted only one week. Shuger et al. randomized 197 overweight or obese participants between four groups: (1) a control group that received a self-directed weight loss program via a manual, (2) a group that participated in a group-based behavioral weight loss program, (3) a group that received an armband (the SenseWear Armband) that monitored energy balance, daily energy expenditure, and energy intake, and (4) a group that received the armband and the group-based behavioral program. 19 The group receiving the armband and group-based behavioral health intervention was the only one that achieved significant weight loss at nine months compared to the control group. Finkelstein et al. employed a behavioral economics study design, randomizing 800 participants from 13 companies in Singapore to one of four groups: (1) the control group, (2) Fitbit Zip activity tracker alone, (3) Fitbit Zip plus charity incentives, or (4) Fitbit Zip plus cash incentives. 36 At 12 months, the Fitbit-only group and the Fitbit plus charity incentives group outperformed the control group and the Fitbit plus cash incentive group. The group receiving cash incentives saw a reduction in moderate-to-vigorous physical activity when compared to the control group.
Four hundred and seventy-one overweight and obese participants in Jakicic et al. received a low-calorie diet, prescription for physical activity, text message prompts, group counseling sessions, telephone counseling sessions, and access to materials on a website; the enhanced intervention group also received an activity tracker (FIT Core) that displayed data via the device interface or a website. 38 The group that used the activity tracker experienced a lower amount of weight loss compared to the non-tracker group. Finally, in Wijsman et al., 235 participants aged 60–70 years without diabetes were randomized to the intervention group or a waitlist control group. 17 Participants in the intervention group received a commercially available physical activity program (Philips DirectLife) based on the stages of change and I-change health behavior change models. The program includes an accelerometer-based activity tracker, a personal website, and a personal e-coach who provides support via email. After 13 weeks, daily physical activity increased significantly, and weight, waist circumference, and fat mass decreased significantly more in the intervention group compared to the control group. The results from these four different physical activity studies propose plausible directions into how and whether activity trackers can motivate behavior change. Cash incentives proved to be less effective than charity incentives, and automated, non-personalized text messages were also unproductive. Successful interventions combined RPM with several evidence-based components, including personalized coaching or group-based programs, or were grounded in validated behavior change models.
Data analysis
For the meta-analysis, we created six groups of outcomes that had three or more studies, including: body mass index (BMI), weight, waist circumference, body fat percentage, systolic blood pressure, and diastolic blood pressure. There were no groupings found among the binary variables, so they were not included in the meta-analysis. In total, the meta-analysis included eight of the 27 studies.
Body mass index (BMI)
Four studies 17 , 19 , 33 , 38 reported baseline and final outcome data for both intervention and control groups for BMI. The total aggregated calculation included 455 control patients and 616 intervention patients (Fig. 2 ). The meta-analysis yielded a mean difference point estimate of −0.73 (95% confidence interval: [−1.84, 0.38]), indicating no statistically significant difference between the experimental and control arms at the 95% confidence level with respect to whether RPM-based interventions resulted in a change in BMI. The I 2 statistic was 92% (95% Confidence Interval: [83%, 96%]), illustrating a high degree of heterogeneity.

Point estimates of the mean difference for each study (green squares) and the corresponding 95% Confidence Intervals (horizontal black lines) are shown, with the size of the green square representing the relative weight of the study. The black diamond represents the overall pooled estimate, with the tips of the diamond representing the 95% Confidence Intervals
Six studies 17 , 19 , 30 , 33 , 36 , 38 reported data for both intervention and control groups for weight. The meta-analysis calculation was based on 824 control patients and 1392 intervention patients (Fig. 3 ). The meta-analysis yielded a mean difference point estimate of −1.29 (95% Confidence Interval: [−3.06, 0.48]), indicating no statistically significant difference. The I 2 statistic was 92% (95% Confidence Interval: [85%, 96%]), illustrating a high degree of heterogeneity.
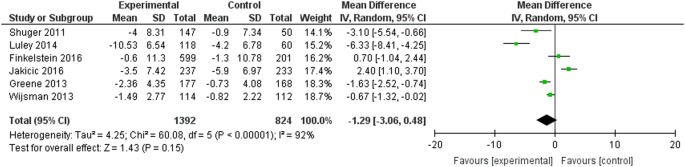
Point estimates of the mean difference for each study (green squares) and the corresponding 95% Confidence Intervals (horizontal lines) are shown, with the size of the green square representing the relative weight of the study. The black diamond represents the overall pooled estimate, with the tips of the diamond representing the 95% Confidence Intervals
Waist circumference
Three studies 17 , 19 , 33 reported data for both intervention and control groups for waist circumference, with a total of 222 control patients and 379 intervention patients ( Fig. 4 ) . The meta-analysis yielded a mean difference point estimate of −2.41 (95% Confidence Interval: [−5.16, 0.34]), indicating no statistically significant difference. The I 2 statistic was 84% (95% [51%, 95%]), illustrating a moderate to high degree of heterogeneity.

Body fat percentage
Three studies 17 , 19 , 38 reported data for both intervention and control groups for body fat percentage. There were a total of 395 control patients and 498 intervention patients ( Fig. 5 ) . The meta-analysis yielded a mean difference point estimate of 0.11 (95% Confidence Interval: [−1.56, 1.34]), indicating no statistically significant difference. The I 2 statistic was 86% (95% [59%, 95%]), illustrating a moderate to high degree of heterogeneity.

Point estimates of the mean difference for each study (green squares) and the corresponding 95% confidence intervals (horizontal lines) are shown, with the size of the green square representing the relative weight of the study. The black diamond represents the overall pooled estimate, with the tips of the diamond representing the 95% Confidence Intervals
Systolic blood pressure
Five studies 15 , 17 , 19 , 23 , 33 , 36 reported data for both intervention and control groups for systolic blood pressure, with a total of 548 control patients and 1135 intervention patients (Fig. 6 ). The meta-analysis yielded a mean difference point estimate of −0.99 (95% Confidence Interval: [−2.73, 0.74]), indicating no statistically significant difference. The I 2 statistic was 44% (95% [0%, 81%]), illustrating an unknown degree of heterogeneity.
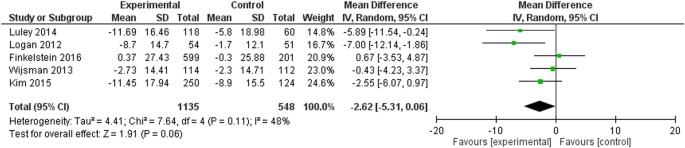
Diastolic blood pressure
Four studies 15 , 17 , 23 , 33 reported data for both intervention and control groups for diastolic blood pressure, with a total of 347 control patients and 536 intervention patients (Fig. 7 ). The meta-analysis yielded a mean difference point estimate of −0.74 (95% Confidence Interval: [−2.34, 0.86]), indicating no statistically significant difference. The I 2 statistic was 28% (95% [0%, 73%]), illustrating an unknown degree of heterogeneity.

Based on our systematic review and examination of high-quality studies on RPM, we found that remote patient monitoring showed early promise in improving outcomes for patients with select conditions, including obstructive pulmonary disease, Parkinson’s disease, hypertension, and low back pain. Interventions aimed at increasing physical activity and weight loss using various activity trackers showed mixed results: cash incentives and automated text messages were ineffective, whereas interventions based on validated health behavior models, care pathways, and tailored coaching were the most successful. However, even within these interventions, certain populations appeared to benefit more from RPM than others. For example, only adults over 55 years of age saw benefits from RPM in one hypertension study. Future studies should be powered to analyze sub-populations to better understand when and for whom RPM is most effective.
For the meta-analyses, we examined six different outcomes (BMI, weight, waist circumference, body fat percentage, systolic blood pressure, and diastolic blood pressure), and found no statistically significant differences between the use of RPM devices and controls with regard to any of these outcomes. However, we were limited by high heterogeneity and scarcity of high-quality studies. The high degree of heterogeneity is likely due to differences in the types of devices used, follow-up periods, and the types of controls in each study. In summary, our results indicate that while some RPM interventions may prove to be promising in changing clinical outcomes in the future, there are still large gaps in the evidence base. Of note, we found that many currently available consumer products have not yet been tested in RCTs with clinically meaningful outcomes. Although some consumer-facing digital health products may be effective for promoting behavior change, there is currently a dearth of evidence that these devices achieve health benefits; more research is needed in this field. Patients, clinicians, and health system leaders should proceed with caution before implementing and using RPM to reliably change clinical outcomes.
Future research should identify and remedy potential barriers to RPM effectiveness on clinical outcomes. For example, factorial design trials should evaluate variants of an RPM intervention in terms of frequency, duration, intensity, and timing. We also found that there are few large-scale clinical trials demonstrating a clinically meaningful impact on patient outcomes. Only one study identified in this review had a sample size of more than 1000 patients; most studies included fewer than 200 patients. Additionally, most studies had relatively short follow-up periods. Given that many of these studies were described as pilot studies, it is clear that the field of RPM is relatively new and evolving. Larger studies with multiple intervention groups will be able to better distinguish which components are most effective and whether behavior change can be sustained over time using RPM.
Future studies would also highly benefit from a mixed-methods approach in which both patients and clinicians are interviewed. Adding a qualitative component would give researchers insight into which RPM elements best engage and motivate patients, nurses, allied health workers, and physicians. Behavior change is complex; understanding how and if specific devices and device-related interventions and incentives motivate health behavior change is an important area that is still not well understood. For example, previous studies have found that most devices result in only short-term changes in behavior and motivation. 39 Activity trackers have been found to change behavior for only approximately three to six months. 40 Studies in this review found that cash incentives performed worse than charity incentives, illustrating that incentivizing individuals is complex and nuanced. Gaining a better understanding of how individuals interface with these health-related technologies will assist in developing evidence-based devices that have the potential to change behavior over longer periods of time.
One of the challenges of this review was the relatively broad survey into the effectiveness of RPM on clinical outcomes. This broad approach allowed us to examine the similarities among interventions targeted at different conditions, but also made it difficult to combine results among studies using different devices and associated interventions. Additional limitations of this study include the use of one primary database, PubMed, to identify articles. However, we examined review articles to identify potential studies that may be listed in other databases. Additionally, the study question focused solely on non-invasive wearable devices and excluded invasive devices such as glucose sensors, on which there have been many studies. The scope of this study included only RCTs with clinically meaningful outcomes. These rigorous search criteria excluded studies without controls or randomization. While non-randomized studies may nonetheless inform the field of RPM, given the risk of selection bias inherent in non-randomized trials, we determined it was optimal to restrict the inclusion criteria to RCTs in this meta-analysis of controlled trials.
An inherent shortcoming of most wearable device studies is difficulty in following double-blind procedures; the intervention arms necessarily include patient engagement or, at minimum, placement of the device on the patient’s body, which can be difficult to blind. Some studies have used devices that were turned off or were non-functional to reduce a potential placebo or Hawthorne effect, 41 but given the data feedback loop integrated into many of these devices, it is extremely difficult to blind the provider receiving the data, which may impact results. Nonetheless, this shortcoming would tend to benefit the active intervention, making it more likely to show a difference in an unblinded study.
For RPM interventions to impact healthcare, they will need to impact outcomes that matter to patients. Examples include patient-reported health related quality of life (HRQOL), symptom severity, satisfaction with care, resource utilization, hospitalizations, readmissions, and survival. There is little data investigating the impact of RPM on these outcome measures. It may strengthen the interventions if they are developed directly in partnership with end-users—i.e. patients themselves. Further research might also emphasize how to personalize RPM interventions, as described by Joseph Kvedar and others. 42 , 43 , 44 This approach seeks to optimize applications and sensors within a biopsychosocial framework. 44 By using validated behavior-based models from the psychological and public health literature that integrate a variety of data from time of day to step counts, to the local weather, to levels of depression or anxiety, these tailored applications aim to generate contextually appropriate, highly tailored messages to patients at the right time and right place. 42 , 43 , 44 , 45 This approach might combine the most successful elements of the effective interventions in this review, including personalized coaching and feedback, in a more cost-effective manner. Additionally, given the pronounced challenges in changing health-related behaviors, incorporating well-researched theoretical frameworks into interventions, such as the Health Belief Model, 46 the Stages of Change Model, 47 or Theory of Reasoned Action/Planned Behavior, 48 may be ultimately more successful than merely improving the technical aspects of RPM.
Study identification
We performed a systematic review of PubMed from January 2000 to October 2016 to identify RCTs that assessed clinical outcomes related to the use of non-invasive wearable biosensors versus a control condition. The subject headings and key words incorporated into the search strategy included:
(“biosensing techniques”[MeSH Terms] OR “Remote sensing technology”[MeSH] OR “remote sensing”[text word] OR “On body sensor”[text word] OR Biosensor*[text word] OR “Wearable device”[text word] OR “Constant health monitoring”[text word] OR “Wireless technology”[text word] OR “wearable sensor”[text word] OR “wearable”[text word] OR “medical sensor”[text word] OR “Body Sensor”[text word] OR “Passive monitor”[text word] OR “wireless monitor”[text word] OR “monitoring device”[text word] OR “wireless sensor”[text word]) AND (hasabstract[text] OR English[lang]) AND (“Clinical Trial “[Publication Type] OR “Randomized Controlled Trial “[Publication Type] OR “randomized”[tiab] OR “placebo”[tiab] OR “therapy”[sh] OR randomly[tiab] OR trial[tiab] OR groups[tiab]) NOT (“animals”[MeSH] NOT “humans”[MeSH]).
After an initial review of our search yield, we added the following subject headings and key words:
(“Remote monitoring”[text word] OR “Remote patient monitoring”[text word] OR “self-monitoring”[text word] OR “self-tracking”[text word] OR “remote tracking”[text word] OR “home monitoring”[text word] OR “wireless monitoring”[text word] OR “online monitoring”[text word] OR “online tracking”[text word] OR “telemonitoring”[text word] OR “ambulatory monitoring”[text word]) AND (“e-health”[text word] OR “m-health”[text word] OR “mobile”[text word] OR “mobile health”[text word] OR “telehealth”[text word] OR “telemedicine”[text word] OR “digital health”[text word] OR “digital medicine”[text word] OR ((“smartphone”[MeSH Terms] OR “smartphone”[All Fields]) AND text[All Fields] AND word[All Fields]) OR “social network”[text word] OR “Web based”[text word] OR “online portal”[text word] OR “internet based”[text word] OR “cell phone”[text word] OR “mobile phone”[text word]).
Additionally, we consulted references from a previous systematic review. 10
Study selection and data extraction
We assessed all titles for relevance and rejected titles if they fulfilled pre-specified exclusion criteria (Table 3 ). Eight trained investigators independently screened titles in pairs of two. We calculated Fleiss’ Kappa, a measure of the degree of consistency between two or more raters to ensure high inter-rater reliability, and aimed for a kappa higher than 0.85. 49 For studies identified in the second review process, a second independent review was performed. Differences regarding inclusion and exclusion criteria were resolved through consensus. We followed a similar method to review abstracts for all studies that passed the title screening stage, and included any study that met all of the abstract inclusion criteria (Table 3 ).
Data abstraction and data management
Each study was jointly abstracted for data by two reviewers and the results were entered into a standardized abstraction form. For each study, the reviewers extracted data about the targeted disease state, device type, control intervention, clinically relevant outcomes, type of feedback loop, descriptive information of subjects, and study design. For the analysis, we examined only continuous variables.
For continuous variables, we used a difference-in-differences model to assess relative change between the baseline measure and final measure for control and treatment groups. If a study did not provide baseline data, we emailed the respective authors and requested the data. If we did not receive a reply or the authors did not have baseline data, we excluded the study from this analysis.
We standardized all studies to provide the change from baseline mean and standard deviation for both the experimental and control arms. If a study reported only standard errors, p -values, or confidence intervals, we converted these to standard deviations (see Appendix). If a study did not provide a standard deviation or any of the three statistics mentioned above, we contacted the primary author, as explained above, and excluded the study from this analysis if they could not provide that information. Many of the identified studies used more than one experimental arm; we followed methods from Cochrane to combine the two groups into one larger group (see Appendix). 50 We directionally corrected all signs and adjusted any differences in units of calculation (i.e. lbs vs. kg).
Given the heterogeneity of the interventions and outcomes, we grouped the outcome variables into separate groups for analysis (e.g. cholesterol, blood pressure). This process was jointly completed by two reviewers, with any disagreements discussed with a third-party arbiter.
Statistical analyses
We used Review Manager (Review Manager [RevMan] Version 5.3. Copenhagen: The Nordic Cochrane Centre, The Cochrane Collaboration, 2014) to conduct a difference-in-differences random effects analysis. We used a difference-in-differences random effects analysis to help control for the many differences in the studies and to limit heterogeneity. We weighted the studies by sample size and used 95% confidence intervals around our point estimates. We also assessed for heterogeneity using the I 2 statistic and calculated the 95% confidence intervals using the standard methods described by Higgins et al. 51 We did not perform tests for funnel plot asymmetry to examine publication bias given that this type of analysis is not recommended for meta-analyses with fewer than 10 studies. 52
Strength of the body of evidence
We assigned a score for methodological quality by applying the Jadad scale, 53 a commonly used instrument for measuring the quality of randomized controlled trials. The score awards points for appropriate randomization, presence of concealed allocation, adequacy of double blinding, appropriateness of blinding technique, and documentation of withdrawals and dropouts. The score ranges from 0 to 5, where a score of ≥3 denotes “high quality” based on the original validation studies. We measured inter-rater agreement for each step with a k statistic, and adopted a threshold of ≥0.7 as the definition for acceptable agreement. Disagreements were adjudicated by discussion and consensus between the two primary reviewers and a third-party arbiter.
Data availability
The data used in this study was manually abstracted from the 27 studies identified in the systematic review. The meta-analysis used data from 8 of those 27 studies, which are referenced at the relevant points in the paper.
Change history
09 april 2018.
A correction to this article has been published and is linked from the HTML version of this article.
Andreu-Perez, J., Leff, D. R., Ip, H. M. & Yang, G. Z. From wearable sensors to smart implants—toward pervasive and personalized healthcare. IEEE Trans. Biomed. Eng. 62 , 2750–2762 (2015).
Article PubMed Google Scholar
Ajami, S. & Teimouri, F. Features and application of wearable biosensors in medical care. J. Res. Med. Sci. 20 , 1208–1215 (2015).
Article PubMed PubMed Central CAS Google Scholar
Steinhubl, S. R., Muse, E. D. & Topol, E. J. The emerging field of mobile health. Sci. Transl. Med. 7 , 283rv283 (2015).
Article Google Scholar
Pevnick, J. M., Fuller, G., Duncan, R. & Spiegel, B. M. R. A large-scale initiative inviting patients to share personal fitness tracker data with their providers: initial results. PLoS ONE 11 , e0165908 (2016).
Atallah, L., Lo, B. & Yang, G. Z. Can pervasive sensing address current challenges in global healthcare? J. Epidemiol. Glob. Health 2 , 1–13 (2012).
Banaee, H., Ahmed, M. U. & Loutfi, A. Data mining for wearable sensors in health monitoring systems: a review of recent trends and challenges. Sens. 13 , 17472–17500 (2013).
Article CAS Google Scholar
Dobkin, B. H. & Dorsch, A. The promise of mHealth: daily activity monitoring and outcome assessments by wearable sensors. Neurorehabil. Neural Repair. 25 , 788–798 (2011).
Article PubMed PubMed Central Google Scholar
Oh, H., Rizo, C., Enkin, M. & Jadad, A. What is eHealth (3): a systematic review of published definitions. J. Med. Internet Res. 7 , e1 (2005).
Sood, S. et al. What is telemedicine? A collection of 104 peer-reviewed perspectives and theoretical underpinnings. Telemed. J. E. Health 13 , 573–590 (2007).
Vegesna, A., Tran, M., Angelaccio, M. & Arcona, S. Remote patient monitoring via non-invasive digital technologies: a systematic review. Telemed. J. E. Health 23 , 3–17 (2017).
Chau, J. P. et al. A feasibility study to investigate the acceptability and potential effectiveness of a telecare service for older people with chronic obstructive pulmonary disease. Int. J. Med. Inform. 81 , 674–682 (2012).
Bloss, C. S. et al. A prospective randomized trial examining health care utilization in individuals using multiple smartphone-enabled biosensors. PeerJ . 4 , e1554 (2016).
Scalvini, S. et al. Cardiac event recording yields more diagnoses than 24-hour Holter monitoring in patients with palpitations. J. Telemed. Telecare. 11 , 14–16 (2005).
Ryan, D. et al. Clinical and cost effectiveness of mobile phone supported self monitoring of asthma: multicentre randomised controlled trial. BMJ 344 , e1756 (2012).
Logan, A. G. et al. Effect of home blood pressure telemonitoring with self-care support on uncontrolled systolic hypertension in diabetics. Hypertension 60 , 51–57 (2012).
Article PubMed CAS Google Scholar
Ong, M. K. et al. Effectiveness of remote patient monitoring after discharge of hospitalized patients with heart failure: the better effectiveness after transition-heart failure (BEAT-HF) randomized clinical trial. JAMA Intern. Med. 176 , 310–318 (2016).
Wijsman, C. A. et al. Effects of a web-based intervention on physical activity and metabolism in older adults: randomized controlled trial. J. Med. Internet Res. 15 , e233 (2013).
Pedone, C., Rossi, F. F., Cecere, A., Costanzo, L. & Antonelli Incalzi, R. Efficacy of a physician-led multiparametric telemonitoring system in very old adults with heart failure. J. Am. Geriatr. Soc. 63 , 1175–1180 (2015).
Shuger, S. L. et al. Electronic feedback in a diet- and physical activity-based lifestyle intervention for weight loss: a randomized controlled trial. Int. J. Behav. Nutr. Phys. Act. 8 , 41 (2011).
Ginis, P. et al. Feasibility and effects of home-based smartphone-delivered automated feedback training for gait in people with Parkinson’s disease: a pilot randomized controlled trial. Park. Relat. Disord. 22 , 28–34 (2016).
Lee, Y. H. et al. Impact of home-based exercise training with wireless monitoring on patients with acute coronary syndrome undergoing percutaneous coronary intervention. J. Korean Med. Sci. 28 , 564–568 (2013).
Tan, B. Y., Ho, K. L., Ching, C. K. & Teo, W. S. Novel electrogram device with web-based service centre for ambulatory ECG monitoring. Singap. Med. J. 51 , 565–569 (2010).
CAS Google Scholar
Kim, Y. N., Shin, D. G., Park, S. & Lee, C. H. Randomized clinical trial to assess the effectiveness of remote patient monitoring and physician care in reducing office blood pressure. Hypertens. Res. 38 , 491–497 (2015).
Dorsch, A. K., Thomas, S., Xu, X., Kaiser, W. & Dobkin, B. H. SIRRACT: an international randomized clinical trial of activity feedback during inpatient stroke rehabilitation enabled by wireless sensing. Neurorehabil. Neural Repair. 29 , 407–415 (2015).
De San Miguel, K., Smith, J. & Lewin, G. Telehealth remote monitoring for community-dwelling older adults with chronic obstructive pulmonary disease. Telemed. J. E. Health 19 , 652–657 (2013).
Woodend, A. K. et al. Telehome monitoring in patients with cardiac disease who are at high risk of readmission. Heart Lung. 37 , 36–45 (2008).
Piga, M. et al. Telemedicine applied to kinesiotherapy for hand dysfunction in patients with systemic sclerosis and rheumatoid arthritis: recovery of movement and telemonitoring technology. J. Rheumatol. 41 , 1324–1333 (2014).
Kent, P., Laird, R. & Haines, T. The effect of changing movement and posture using motion-sensor biofeedback, versus guidelines-based care, on the clinical outcomes of people with sub-acute or chronic low back pain-a multicentre, cluster-randomised, placebo-controlled, pilot trial. BMC Musculoskelet. Disord. 16 , 131 (2015).
Fox, N. et al. The impact of a telemedicine monitoring system on positive airway pressure adherence in patients with obstructive sleep apnea: a randomized controlled trial. Sleep 35 , 477–481 (2012).
Greene, J., Sacks, R., Piniewski, B., Kil, D. & Hahn, J. S. The impact of an online social network with wireless monitoring devices on physical activity and weight loss. J. Prim. Care Community Health 4 , 189–194 (2013).
Dinesen, B. et al. Using preventive home monitoring to reduce hospital admission rates and reduce costs: a case study of telehealth among chronic obstructive pulmonary disease patients. J. Telemed. Telecare. 18 , 221–225 (2012).
Wang, J. B. et al. Wearable sensor/device (Fitbit One) and SMS text-messaging prompts to increase physical activity in overweight and obese adults: a randomized controlled trial. Telemed. J. E. Health 21 , 782–792 (2015).
Luley, C. et al. Weight loss by telemonitoring of nutrition and physical activity in patients with metabolic syndrome for 1 year. J. Am. Coll. Nutr. 33 , 363–374 (2014).
Dansky, K. H., Vasey, J. & Bowles, K. Impact of telehealth on clinical outcomes in patients with heart failure. Clin. Nurs. Res. 17 , 182–199 (2008).
Pedone, C., Chiurco, D., Scarlata, S. & Incalzi, R. A. Efficacy of multiparametric telemonitoring on respiratory outcomes in elderly people with COPD: a randomized controlled trial. BMC Health Serv. Res. 13 , 82 (2013).
Finkelstein, E. A. et al. Effectiveness of activity trackers with and without incentives to increase physical activity (TRIPPA): a randomised controlled trial. Lancet Diabetes Endocrinol . https://doi.org/10.1016/S2213-8587(16)30284-4 (2016).
Hibbard, J. H., Stockard, J., Mahoney, E. R. & Tusler, M. Development of the patient activation measure (PAM): conceptualizing and measuring activation in patients and consumers. Health Serv. Res. 39 , 1005–1026 (2004).
Jakicic, J. M. et al. Effect of wearable technology combined with a lifestyle intervention on long-term weight loss: the IDEA randomized clinical trial. JAMA 316 , 1161–1171 (2016).
Klasnja, P., Consolvo, S. & Pratt, W. In Proc. SIGCHI Conference on Human Factors Computing Systems. 3063–3072 (ACM, Vancouver, BC, Canada, 2011).
Shih, P. C., Han, K., Poole, E. S., Rosson, M. B. & Carroll, J. M. Use and adoption challenges of wearable activity trackers. In iConf. Proc . (iSchools, Newport Beach, California, 2015).
McCambridge, J., Witton, J. & Elbourne, D. R. Systematic review of the Hawthorne effect: new concepts are needed to study research participation effects. J. Clin. Epidemiol. 67 , 267–277 (2014).
Agboola, S. et al. Pain management in cancer patients using a mobile app: study design of a randomized controlled trial. JMIR Res. Protoc. 3 , e76 (2014).
Agboola, S. et al. Improving outcomes in cancer patients on oral anti-cancer medications using a novel mobile phone-based intervention: study design of a randomized controlled trial. JMIR Res. Protoc. 3 , e79 (2014).
Kvedar, J., Coye, M. J. & Everett, W. Connected health: a review of technologies and strategies to improve patient care with telemedicine and telehealth. Health Aff. 33 , 194–199 (2014).
Agboola, S., Palacholla, R. S., Centi, A., Kvedar, J. & Jethwani, K. A multimodal mHealth intervention (FeatForward) to improve physical activity behavior in patients with high cardiometabolic risk factors: rationale and protocol for a randomized controlled trial. JMIR Res. Protoc . 5 (2016).
Rosenstock, I. M. The health belief model and preventive health behavior. Health Educ. Monogr. 2 , 354–386 (1974).
Prochaska, J. O., DiClemente, C. C. & Norcross, J. C. In search of how people change. Appl. Addict. Behav. Am. Psychol. 47 , 1102–1114 (1992).
Ajzen, I. The theory of planned behavior. Organ. Behav. Hum. Decis. Process. 50 , 179–211 (1991).
McHugh, M. L. Interrater reliability: the kappa statistic. Biochem. Med. 22 , 276–282 (2012).
Higgins, J. P. & Green, S. Cochrane Handbook for Systematic Reviews of Interventions. (The Cochrane Collaboration, 2011).
Higgins, J. P. & Thompson, S. G. Quantifying heterogeneity in a meta-analysis. Stat. Med. 21 , 1539–1558 (2002).
Sterne, J. A. C. et al. Recommendations for examining and interpreting funnel plot asymmetry in meta-analyses of randomised controlled trials. BMJ . 343 (2011).
Jadad, A. R. et al. Assessing the quality of reports of randomized clinical trials: is blinding necessary? Control. Clin. Trials 17 , 1–12 (1996).
Download references
Author information
Authors and affiliations.
Division of Health Services Research, Cedars-Sinai Medical Center, Los Angeles, CA, USA
Benjamin Noah, Michelle S. Keller, Libby Stein, Sunny Johl, Sean Delshad, Vartan C. Tashjian, Daniel Lew, James T. Kwan, Alma Jusufagic & Brennan M. R. Spiegel
Cedars-Sinai Center for Outcomes Research and Education (CS-CORE), Los Angeles, CA, USA
Department of Health Policy and Management, UCLA Fielding School of Public Health, Los Angeles, CA, USA
Michelle S. Keller, Alma Jusufagic & Brennan M. R. Spiegel
Department of Medicine, University of Arizona, College of Medicine Tucson, Tucson, AZ, USA
Sasan Mosadeghi
Cedars-Sinai Medical Center, Los Angeles, CA, USA
Vartan C. Tashjian, Daniel Lew & Brennan M. R. Spiegel
American Journal of Gastroenterology, Bethesda, USA
Brennan M. R. Spiegel
You can also search for this author in PubMed Google Scholar
Contributions
B.N. abstracted the data, ran the analysis, and wrote the results. M.S.K. summarized the high-quality studies in the results section. M.S.K., A.J., and B.M.S. assisted in writing and editing the manuscript. M.S.K., S.M., L.S., S.J., S.D., V.C.T., D.L., and J.T.K. conducted the literature search, and systematic review. All authors contributed to and have approved the final manuscript.
Corresponding author
Correspondence to Brennan M. R. Spiegel .
Ethics declarations
Competing interests.
The authors declare no competing financial interests.
Additional information
Publisher's note: Springer Nature remains neutral with regard to jurisdictional claims in published maps and institutional affiliations.
Change history: The original version of this Article had an incorrect Article number of 2 and an incorrect Publication year of 2017. These errors have now been corrected in the PDF and HTML versions of the Article.
Rights and permissions
Open Access This article is licensed under a Creative Commons Attribution 4.0 International License, which permits use, sharing, adaptation, distribution and reproduction in any medium or format, as long as you give appropriate credit to the original author(s) and the source, provide a link to the Creative Commons license, and indicate if changes were made. The images or other third party material in this article are included in the article’s Creative Commons license, unless indicated otherwise in a credit line to the material. If material is not included in the article’s Creative Commons license and your intended use is not permitted by statutory regulation or exceeds the permitted use, you will need to obtain permission directly from the copyright holder. To view a copy of this license, visit http://creativecommons.org/licenses/by/4.0/ .
Reprints and permissions
About this article
Cite this article.
Noah, B., Keller, M.S., Mosadeghi, S. et al. Impact of remote patient monitoring on clinical outcomes: an updated meta-analysis of randomized controlled trials. npj Digital Med 1 , 20172 (2018). https://doi.org/10.1038/s41746-017-0002-4
Download citation
Received : 11 May 2017
Revised : 28 August 2017
Accepted : 31 August 2017
Published : 15 January 2018
DOI : https://doi.org/10.1038/s41746-017-0002-4
Share this article
Anyone you share the following link with will be able to read this content:
Sorry, a shareable link is not currently available for this article.
Provided by the Springer Nature SharedIt content-sharing initiative
This article is cited by
Post-hospitalization remote monitoring for patients with heart failure or chronic obstructive pulmonary disease in an accountable care organization.
- Samantha Harris
- Kayla Paynter
- Patrick G. Lyons
BMC Health Services Research (2024)
Feasibility and acceptability of C-PRIME: A health promotion intervention for family caregivers of patients with colorectal cancer
- Lisa M. Gudenkauf
- Brian D. Gonzalez
Supportive Care in Cancer (2024)
Association of Remote Patient Monitoring with Mortality and Healthcare Utilization in Hypertensive Patients: a Medicare Claims–Based Study
- Mahip Acharya
- Corey J. Hayes
Journal of General Internal Medicine (2024)
Transforming ophthalmology in the digital century—new care models with added value for patients
- Peter M. Maloca
- Martin K. Schmid
Synchronized wearables for the detection of haemodynamic states via electrocardiography and multispectral photoplethysmography
- Daniel Franklin
- Andreas Tzavelis
- John A. Rogers
Nature Biomedical Engineering (2023)
Quick links
- Explore articles by subject
- Guide to authors
- Editorial policies
Sign up for the Nature Briefing newsletter — what matters in science, free to your inbox daily.


- SUGGESTED TOPICS
- The Magazine
- Newsletters
- Managing Yourself
- Managing Teams
- Work-life Balance
- The Big Idea
- Data & Visuals
- Reading Lists
- Case Selections
- HBR Learning
- Topic Feeds
- Account Settings
- Email Preferences
Monitoring Employees Makes Them More Likely to Break Rules
- Chase Thiel,
- Julena M. Bonner,
- David Welsh,
- Niharika Garud

Researchers found that when workers know they’re being surveilled, they often feel less responsible for their own conduct.
As remote work becomes the norm, more and more companies have begun tracking employees through desktop monitoring, video surveillance, and other digital tools. These systems are designed to reduce rule-breaking — and yet new research suggests that in some cases, they can seriously backfire. Specifically, the authors found across two studies that monitored employees were substantially more likely to break rules, including engaging in behaviors such as cheating on a test, stealing equipment, and purposely working at a slow pace. They further found that this effect was driven by a shift in employees’ sense of agency and personal responsibility: Monitoring employees led them to subconsciously feel less responsibility for their own conduct, ultimately making them more likely to act in ways that they would otherwise consider immoral. However, when employees feel that they are being treated fairly, the authors found that they are less likely to suffer a drop in agency and are thus less likely to lose their sense of moral responsibility in response to monitoring. As such, the authors suggest that in cases where monitoring is necessary, employers should take steps to enhance perceptions of justice and thus preserve employees’ sense of agency.
In April 2020, global demand for employee monitoring software more than doubled . Online searches for “how to monitor employees working from home” increased by 1,705%, and sales for systems that track workers’ activity via desktop monitoring, keystroke tracking, video surveillance, GPS location tracking, and other digital tools went through the roof. Some of these systems purport to use employee data to improve wellbeing — for example, Microsoft is developing a system that would use smart watches to collect data on employees’ blood pressure and heart rate, producing personalized “anxiety scores” to inform wellness recommendations. But the vast majority of employee monitoring tools are focused on tracking performance, increasing productivity, and deterring rule-breaking.

- CT Chase Thiel is the Bill Daniels Chair of Business Ethics and an associate professor of management at the University of Wyoming’s College of Business. His research examines causes of organizational misconduct through a behavioral lens, characteristics of moral people, and the role of leaders in the creation and maintenance of ethical workplaces.
- JB Julena M. Bonner is an Associate Professor of management in the Marketing and Strategy Department of the Jon M. Huntsman School of Business at Utah State University. She received her PhD in Management from Oklahoma State University. Her research interests include behavioral ethics, ethical leadership, moral emotions, and workplace deviance. See her faculty page here .
- JB John Bush is an Assistant Professor of Management in the College of Business at the University of Central Florida. His research focuses on employee ethicality and performance in organizations.
- David Welsh is an associate professor in the Department of Management and Entrepreneurship at Arizona State University’s W.P. Carey School of Business. He holds a Ph.D. in Management from the University of Arizona. His research focuses primarily on issues related to unethical behavior in the workplace. See his faculty page here .
- NG Niharika Garud is an associate professor in the Department of Management and Marketing at University of Melbourne’s Faculty of Business & Economics. Her research focuses primarily on understanding management of people, performance, and innovation in organizations. See her faculty page here .
Partner Center
This website may not work correctly because your browser is out of date. Please update your browser .
A case study focuses on a particular unit - a person, a site, a project. It often uses a combination of quantitative and qualitative data.
Case studies can be particularly useful for understanding how different elements fit together and how different elements (implementation, context and other factors) have produced the observed impacts.
There are different types of case studies, which can be used for different purposes in evaluation. The GAO (Government Accountability Office) has described six different types of case study:
1. Illustrative : This is descriptive in character and intended to add realism and in-depth examples to other information about a program or policy. (These are often used to complement quantitative data by providing examples of the overall findings).
2. Exploratory : This is also descriptive but is aimed at generating hypotheses for later investigation rather than simply providing illustration.
3. Critical instance : This examines a single instance of unique interest, or serves as a critical test of an assertion about a program, problem or strategy.
4. Program implementation . This investigates operations, often at several sites, and often with reference to a set of norms or standards about implementation processes.
5. Program effects . This examines the causal links between the program and observed effects (outputs, outcomes or impacts, depending on the timing of the evaluation) and usually involves multisite, multimethod evaluations.
6. Cumulative . This brings together findings from many case studies to answer evaluative questions.
The following guides are particularly recommended because they distinguish between the research design (case study) and the type of data (qualitative or quantitative), and provide guidance on selecting cases, addressing causal inference, and generalizing from cases.
This guide from the US General Accounting Office outlines good practice in case study evaluation and establishes a set of principles for applying case studies to evaluations.
This paper, authored by Edith D. Balbach for the California Department of Health Services is designed to help evaluators decide whether to use a case study evaluation approach.
This guide, written by Linda G. Morra and Amy C. Friedlander for the World Bank, provides guidance and advice on the use of case studies.
Expand to view all resources related to 'Case study'
- Broadening the range of designs and methods for impact evaluations
- Case studies in action
- Case study evaluations - US General Accounting Office
- Case study evaluations - World Bank
- Comparative case studies
- Dealing with paradox – Stories and lessons from the first three years of consortium-building
- Designing and facilitating creative conversations & learning activities
- Estudo de caso: a avaliação externa de um programa
- Evaluation tools
- Evaluations that make a difference
- Methods for monitoring and evaluation
- Reflections on innovation, assessment and social change processes: A SPARC case study, India
- Toward a listening bank: A review of best practices and the efficacy of beneficiary assessment
- UNICEF webinar: Comparative case studies
- Using case studies to do program evaluation
'Case study' is referenced in:
- Week 32: Better use of case studies in evaluation
Back to top
© 2022 BetterEvaluation. All right reserved.
The Monitoring and Evaluation Toolkit
This section asks:
What is a case study?
- What are the different types of case study ?
- What are the advantages and disadvantages of a case study ?
- How to Use Case Studies as part of your Monitoring & Evaluation?

There are many different text books and websites explaining the use of case studies and this section draws heavily on those of Lamar University and the NCBI (worked examples), as well as on the author’s own extensive research experience.
If you are monitoring/ evaluating a project, you may already have obtained general information about your target school, village, hospital or farming community. But the information you have is broad and imprecise. It may contain a lot of statistics but may not give you a feel for what is really going on in that village, school, hospital or farming community.
Case studies can provide this depth. They focus on a particular person, patient, village, group within a community or other sub-set of a wider group. They can be used to illustrate wider trends or to show that the case you are examining is broadly similar to other cases or really quite different.
In other words, a case study examines a person, place, event, phenomenon, or other type of subject of analysis in order to extrapolate key themes and results that help predict future trends, illuminate previously hidden issues that can be applied to practice, and/or provide a means for understanding an important research problem with greater clarity.
A case study paper usually examines a single subject of analysis, but case study papers can also be designed as a comparative investigation that shows relationships between two or among more than two subjects. The methods used to study a case can rest within a quantitative, qualitative, or a mixture of the two.

Different types of case study
There are many types of case study. Drawing on the work of Lamar University and the NCBI , some of the best-known types are set out below.
It is best not to worry too much about the nuances that differentiate types of case study. The key is to recognise that the case study is a detailed illustration of how your project or programme has worked or failed to work on an individual, hospital, school, target community or other group/ economic sector.
- Explanatory case studies aim to answer ‘how’ or ’why’ questions with little control on behalf of researcher over occurrence of events. This type of case studies focus on phenomena within the contexts of real-life situations. Example: “An investigation into the reasons of the global financial and economic crisis of 2008 – 2010.”
- Descriptive case studies aim to analyze the sequence of interpersonal events after a certain amount of time has passed. Studies in business research belonging to this category usually describe culture or sub-culture, and they attempt to discover the key phenomena. Example: Impact of increasing levels of funding for prosthetic limbs on the employment opportunities of amputees. A case study of the West Point community of Monrovia (Liberia).
- Exploratory case studies aim to find answers to the questions of ‘what’ or ‘who’. Exploratory case study data collection method is often accompanied by additional data collection method(s) such as interviews, questionnaires, experiments etc. Example: “A study into differences of local community governance practices between a town in francophone Cameroon and a similar-sized town in anglophone Cameroon.”
- Critical instance : This examines a single instance of unique interest, or serves as a critical test of an assertion about a programme, problem or strategy. The focus might be on the economic or human cost of a tsunami or volcanic eruption in a particular area.
- Representative : This relates to case which is typical in nature and representative of other cases that you might examine. An example might be a mother, with a part-time job and four children, living in a community where this is the norm
- Deviant : This refers to a case which is out of line with others. Deviant cases can be particularly interesting and often attract greater attention from analysts. A patient with immunity to a particular virus is worth studying as that study might provide clues to a possible cure to that virus
- Prototypical : This involves a case which is ahead of the curve in some way and has the capacity to set a trend. A particular African town or city may be a free bicyle loan scheme and the experiences of that town might suggest a future path to be followed by other towns and regions.
- Most similar cases : Here you are looking at more than one case and you have selected two cases which have a preponderance of features in common. You might for example be looking at two schools, each of which teaches boys aged from 11-15 and each of which charges similar fees. They are located in the same country but are in different regions where the local authorities devote different levels of resource to secondary school education. You may have a project in each of these areas and you may wish to explain why your project has been more successful in one than the other.
- Most dissimilar cases : these are cases which are, in most key respects, very different and where you might expect to find different outcomes. You might for example select a class of top-ranking pupils and compare it with a class of bottom-ranking puils. This could help to bring out the factors that contribute to or detract from academic success.
Advantages and Disadvantages of Case Study Method
- It helps explain how and why a phenomenon has occurred, thereby going beyond numerical data
- It allows the integration of qualitative and quantitative data collection and analysis methods
- It provides rich (or ‘thick) detail and is well suited to capturing complexities of real-life situations and the challenges facing real people
- Case studies (sometimes illustrated with quotations from beneficiairies/ stakeholder and with photographs) are often included as boxes in project reports and evaluations, thereby adding adding a human dimension to an otherwise dry description and data.
- Case studies may offer you an opportunity to gather evidence that challenges prevailing assumptions about a research problem and provide a new set of recommendations applied to practice that have not been tested previously.
Disadvantages
- Case studies may be marked by a lack of rigour (e.g. a study may not be sufficiently in-depth or a single case study may not be sufficient)
- Single case studies may offer very little basis for generalisations of findings and conclusions.
- Case studies often tend to be success stories (so they may involve a degree of bias).
Where to next?
Click here to return to the top of the page, here to return to step 3 (Data checking) and here to see a short worked example of a metrics-based evaluation.
This site uses cookies to optimize functionality and give you the best possible experience. If you continue to navigate this website beyond this page, cookies will be placed on your browser. To learn more about cookies, click here .
Search form
202-624-3270
- Mission and Vision
- Board of Directors
- Leadership Council
- Policy Principles & Priorities
- Policy Steering Committee
- Stakeholders
- All Resources
- Health Equity & Access
- Improving the Patient Experience
- Digital Care
- Privacy & Cybersecurity
- Member Benefits
- Become a Member
- Get Involved
- Our Members
- Executives in Focus
- Upcoming Events
- Past Events
- News Releases
- Member News
- Join Our Mailing List
Case Study: Remote Patient Monitoring
Interoperability.

One top-three integrated delivery network (IDN) was facing a challenge: it was unable to manage the growing number of patients with chronic conditions. Past remote patient monitoring (RPM) programs lived outside the EHR in onerous web-based dashboards because integrating data into flowsheets and existing workflows came with exorbitant costs. To meet the various needs of stakeholders, the IDN partnered with Validic to implement a cost-effective approach to RPM.
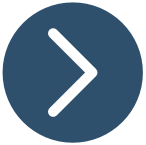
Academia.edu no longer supports Internet Explorer.
To browse Academia.edu and the wider internet faster and more securely, please take a few seconds to upgrade your browser .
Enter the email address you signed up with and we'll email you a reset link.
- We're Hiring!
- Help Center
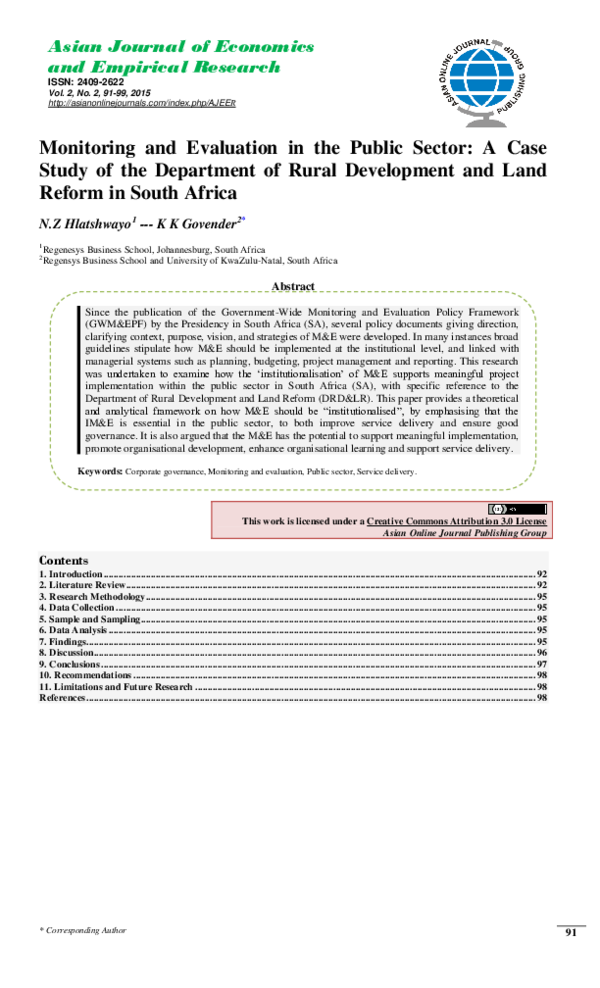
Monitoring and Evaluation in the Public Sector: A Case Study of the Department of Rural Development and Land Reform in South Africa

Since the publication of the Government-Wide Monitoring and Evaluation Policy Framework (GWM&EPF) by the Presidency in South Africa (SA), several policy documents giving direction, clarifying context, purpose, vision, and strategies of M&E were developed. In many instances broad guidelines stipulate how M&E should be implemented at the institutional level, and linked with managerial systems such as planning, budgeting, project management and reporting. This research was undertaken to examine how the „institutionalisation‟ of M&E supports meaningful project implementation within the public sector in South Africa (SA), with specific reference to the Department of Rural Development and Land Reform (DRD&LR). This paper provides a theoretical and analytical framework on how M&E should be “institutionalised”, by emphasising that the IM&E is essential in the public sector, to both improve service delivery and ensure good governance. It is also argued that the M&E has the potential to support meaningful implementation, promote organisational development, enhance organisational learning and support service delivery.
Related Papers
James Malesela
The global context provides an important platform for governments to build and sustain their M&E systems by adopting the best practices and lessons. Monitoring and evaluation (M&E) in South African government has gradually been recognised as a mechanism to enhance good governance. The advent of framework for the government-wide M&E inculcates a culture of reflection and importance of keeping track of the policy, programme or project implementation. M&E form an indispensable part of public management and administrative tools accessible for managers to improve the business processes of the institution. M&E therefore provides a significant panacea for the growing pressure on the institutions to enhance good governance. The principles of good governance comprise accountability, transparency, rule of law, public participation, responsiveness and effectiveness. These principles correlate precisely with the values governing public administration enshrined in the Constitution of the Republic of South Africa, 1996. They serve as standards and indicators to monitor and measure performance. The relevance of monitoring, evaluation and good governance in Public Administration is inevitable. M&E cuts across the generic administrative and managerial functions of public administration while good governance demonstrates/exhibits the outcome of functional M&E.
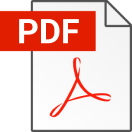
Niringiye Ignatius
Philipp Krause
Roan Neethling , daniel meyer
The 1994 democratic rule and Constitution of 1996 shaped the way in which service delivery would be transformed in South Africa. This was done by developing new structures and policies that would ultimately attempt to create equity and fairness in the provision of services within the municipal sphere to all residents. This article analyses the perceptions of business owners regarding the creation of an enabling environment and service delivery within one of the best performing municipalities in Gauteng: the Midvaal Local Municipal area. A total of 50 business owners were interviewed by means of a quantitative questionnaire. Data were statistically analysed by using descriptive data as well as a chi-square cross tabulation. The results revealed that the general perception of service delivery is above acceptable levels. However, in some categories the business owners were less satisfied regarding land use and zoning process and regulations. Overall, the business owners felt that the local government was creating an enabling environment for business to prosper. No significant statistical difference was found regarding perceptions of service delivery and the enabling environment, between small and large businesses in the study area. This type of analysis provides the foundation for improved service delivery and policy development and allows for future comparative analysis research into local government. The research has also placed the relationship between good governance, service delivery and the creation of an enabling environment in the spotlight.
Zwelibanzi Mpehle
Gerrit Van der Waldt
Paschal ResearchTrainers
Lebogang L Nawa
The institutionalisation of cultural policy has, to date, become an effective tool for cultureled development in some parts of the world. South Africa is yet to fully embrace this phenomenon in its developmental matrix. While the government has introduced certain strategies, such as the Integrated Development Plan (IDP), to coordinate its post-apartheid development imperatives across all of its spheres, role players, such as politicians, town planners and developers, continue to carry on with their subjective approaches to development, without culture as the mediator. This perpetuates the fragmentation of spatial landscapes and infrastructure networks in these areas along racial and cultural lines. This article suggests that South Africa may benefit from formulating local, cultural policies for the revitalisation of decaying cities into new integrated, liveable and vibrant residential, business and sporting environs.
The principal question this study aims to answer is why and how a left-of-centre government not hobbled by heavy external leverage, with developmental state precedents, potentially positive macroeconomic fundamentals, and well-developed alternative policies for housing and urban reconstruction came to settle on a conservative housing policy founded on ‘precepts of the pre-democratic period’. Arguably, this policy is even more conservative than World Bank strictures and paradigms, whose advice the incoming democratic government ‘normally ignored’ and ‘tacitly rejected’. The study, which spans the period from the early 1990s to 2007, commences from the premise that housing is an expression and component of a society’s wider development agenda and is bound up with daily routines of the ordering and institutionalisation of social existence and social reproduction. It proposes an answer that resides in the mechanics and modalities of post-apartheid state construction and its associated techniques and technologies of societal penetration and regime legitimisation. The vagaries and vicissitudes of post-Cold War statecraft, the weight of history and legacy, strategic blundering, and the absence of a cognitive map and compass to guide post-apartheid statecraft, collectively contribute to past and present defects and deformities of our two decade-old developmentalism, writ large in our human settlements. Alternatives to the technocratic market developmentalism of our current housing praxis spotlight empowering shelter outcomes but were bastardised. This is not unrelated to the toxicity of mixing conservative governmentalities (neoliberal macroeconomic precepts, modernist planning orientations, supply-side citizenship and technocratic projections of state) with ‘ambiguated’ counter-governmentalities (self-empowerment, self-responsibilisation, the aestheticisation of poverty and heroic narratives about the poor). Underscored in the study is the contention that state developmentalism and civil society developmentalism rise and fall together, pivoting on (savvy) reconnection of economics and politics (the vertical axis of governance) and state and society (the horizontal axis). Without robust reconfiguration and recalibration of axes, the revamped or, more appropriately, reconditioned housing policy – Breaking New Ground – struggles to navigate the limitations of the First Decade settlement state shelter delivery regime and the Second Decade’s (weak) developmental state etho-politics. The prospects for success are contingent on structurally rewiring inherited and contemporary contacts and circuits of power, influence and money in order to tilt resource and institutional balances in favour of the poor. Present pasts and present futures, both here and abroad, offer resources for more transformative statecraft and sustainable human settlements, but only if we are prepared to challenge the underlying economic and political interests that to date have, and continue to, preclude such policies. History, experience and contemporary record show there are alternatives – another possible and necessary world – via small and large steps, millimetres and centimetres, trial and error.
RELATED PAPERS
UCT Master's Dissertation Series no. 5
Pieter U Pretorius
Myo Naing , Anne Mc Lennan
Jacob Fatile
Masters Degree Thesis
Stephen Baguma
Zukiswa Kota , Monica Hendricks , Eric Matambo
David Schaub-Jones
IOSR Journals
Kobus Muller , daniel meyer , Malcolm Wallis , Jacobus S Wessels , Liezel Lues , Xolile Thani , Thandi Matsiliza , melody brauns , Frederik M Uys
Rick de Satgé
Sonia Bakweleng Malapane
chris landsberg
Shikha Vyas-Doorgapersad
Inge Amundsen
Fanie Cloete
Carmen Alpin
Andrea Juan
Globalizations 12 (2)
The Future of Evaluation
Laila El Baradei , Nermine Wally , Doha Abdelhamid
Anil Kanjee , Yusuf Sayed
Tam O'Neil
African Evaluation Journal
Development Bank of Southern AfricaOperations …
Cherrel Africa
Francois B van Schalkwyk , Stefaan Verhulst , Glenn Maail , Piovesan Federico , Silvana Fumega
BRIDGE, IDS, Brighton
Bridget Byrne
Liz David-Barrett
Dr C L Pieterse
Ross R Worthington
Thabang Mpande
Victoria Awiti
Tristan Gorgens
University of Sussex. Doctoral thesis
Blanca Lopez
Robert Cameron
RELATED TOPICS
- We're Hiring!
- Help Center
- Find new research papers in:
- Health Sciences
- Earth Sciences
- Cognitive Science
- Mathematics
- Computer Science
- Academia ©2024

An official website of the United States government
The .gov means it’s official. Federal government websites often end in .gov or .mil. Before sharing sensitive information, make sure you’re on a federal government site.
The site is secure. The https:// ensures that you are connecting to the official website and that any information you provide is encrypted and transmitted securely.
- Publications
- Account settings
Preview improvements coming to the PMC website in October 2024. Learn More or Try it out now .
- Advanced Search
- Journal List
- J Healthc Inform Res
- v.2(1-2); 2018 Jun

Machine Learning and Mobile Health Monitoring Platforms: A Case Study on Research and Implementation Challenges
Omar boursalie.
1 School of Biomedical Engineering, McMaster University, Hamilton, ON Canada
Reza Samavi
2 Department of Computing and Software, McMaster University, Hamilton, ON Canada
3 eHealth Graduate Program, McMaster University, Hamilton, ON Canada
Thomas E. Doyle
4 Department of Electrical and Computer Engineering, McMaster University, Hamilton, ON Canada
Machine learning-based patient monitoring systems are generally deployed on remote servers for analyzing heterogeneous data. While recent advances in mobile technology provide new opportunities to deploy such systems directly on mobile devices, the development and deployment challenges are not being extensively studied by the research community. In this paper, we systematically investigate challenges associated with each stage of the development and deployment of a machine learning-based patient monitoring system on a mobile device. For each class of challenges, we provide a number of recommendations that can be used by the researchers, system designers, and developers working on mobile-based predictive and monitoring systems. The results of our investigation show that when developers are dealing with mobile platforms, they must evaluate the predictive systems based on its classification and computational performance. Accordingly, we propose a new machine learning training and deployment methodology specifically tailored for mobile platforms that incorporates metrics beyond traditional classifier performance.
Introduction
Chronic diseases such as cardiovascular disease (CVD) are an increasing burden for global health-care systems as the population ages [ 1 ]. As a result, there is growing interest in developing remote patient monitoring (RPM) systems to assist health professionals in the management of chronic diseases by analyzing immense data collected from wearable sensors and health record data. Generally, the analysis is completed using machine learning algorithms (MLA) [ 2 , 3 ] resided on remote servers that can handle expensive computational operations. Advances in mobile technology provide new opportunities to deploy MLAs locally on mobile devices lowering transmission expenses and allowing the system to work without any interruption when the network connection is poor or non-existent. However, transferring the data analysis from a remote server to a mobile device introduces its own set of challenges. While there is a wealth of research studies focusing on using machine learning algorithms for remote patient monitoring systems (e.g., CVD [ 4 ], respiratory [ 5 ], diabetes [ 6 ]), the characteristics of the implementation environment (such as required computational power, the network bandwidth and the power consumption to train and/or test the algorithm) and its impact on classification performance is rarely investigated.
In this paper, we systematically study the impacts of the design decisions, made during a mobile RPM system development, on the system’s classification and computational performance. We adapt the Yin’s case study methodology [ 7 ] to investigate the challenges we faced in the design, implementation and deployment of the multi-source mobile analytic RPM system, M4CVD (Mobile Machine Learning Model for Monitoring Cardiovascular Disease) [ 8 ]. Four classes of challenges for developing a mobile monitoring system are investigated: data collection, data processing, machine learning and system deployment. We also present our recommendations for addressing the main challenge for each development stage. As part of our recommendations, we propose a novel training and deployment methodology for MLAs on mobile platforms that incorporates additional metrics beyond classification performance.
The paper’s contributions and structure are as follows: Section 2 provides an overview of the research model and case study methodology used in this paper. In Section 3 , we describe the implementation procedure and challenges we encountered during system development. In Section 4 we present our recommendations for addressing the main challenges identified at each development stage. Section 5 describes the related research. We conclude in Section 6 .
Research Method
Early remote patient monitoring systems were signal acquisition platforms that continuously transmitted physiological data from a single sensor to a remote server for analysis. Increasingly, monitoring systems are using machine learning algorithms to automatically analyze the collected data which have been shown to increase prediction accuracy with less strict assumptions compared to statistical methods [ 5 ]. Regardless of the algorithm, the most common approach used in the development of a machine learning-based monitoring system is shown in Fig. 1 a. First, the training data is collected and manually labeled. Next, preprocessing, feature extraction and data fusion techniques are selected to transform the input data into a set of features suitable as inputs to the classifier. Finally, the machine learning algorithm is trained and tested. Most monitoring systems data processing and analysis stages are developed and deployed on remote servers since both stages have a complexity order of approximately O ( n ) 3 [ 9 ].

An overview of a current and b our proposed methodology for developing a MLA-based remote monitoring system. New components are in bold. Stages in white are on a remote sever while stages in gray are on a mobile device
In this research, we investigate how the complexity described above can be managed when the mobile platform is considered as an additional dimension on a remote monitoring system design. We group the challenges we encountered according to Fig. 1 a. As part of our methodology we are interested in extending the model described in Fig. 1 a to answer the following queries: (1) What are the challenges of monitoring heterogeneous data sources? (2) What are the computational requirements of a monitoring system on a mobile device? (3) How can the computational requirements of a mobile platform be incorporated into the training, testing, and deployment of machine learning algorithms? (4) What are the trade-offs between classifier accuracy and mobile computational performance?
Following the case study methodology [ 7 ], we systematically encoded our observation, challenges and design decisions made at every stage of system development shown in Fig. 1 a. Our objective was to investigate the main challenges for each development stage. We identified four general decision milestones faced during the development of the mobile-based RPM system with cascading effects on system performance: (1) training data labeling method, (2) data fusion technique, (3) classifier selection, and (4) adapting classifier requirements based on current computational environment. For each milestone a number of alternatives were studied by creating a set of sister RPM systems and evaluating each system in terms of its classification and computational performance.
In Section 3 we discuss the challenges we encountered grouped in terms of the development stages shown in Fig. 1 a. We also explore how the design decisions made during system development impacts the model’s classification and mobile computational performance. The challenges we identified are solely based on our experience developing M4CVD. However, from related studies we identified that challenges in model training [ 3 , 10 ] and deployment [ 11 ] are generic to developing any MLA-based mobile systems.
Based on our findings, in Section 4 we propose a series of recommendations for addressing the four main decision milestones shown in Fig. 1 a. As part of our recommendations, we extend Fig. 1 a by proposing a new training and deployment methodology for MLAs on mobile platforms as shown in Fig. 1 b. First, we investigate two methods to label training data automatically. Next, we present a comparative analysis of two data fusion techniques for combining heterogeneous data. Third, we propose a novel training methodology for mobile-based MLAs. Currently, classifier training and testing are completed on a remote server. We propose conducting the classifier testing on a mobile device to create accuracy-computational profiles for each candidate model. Our proposed method allows developers to study the trade-offs between a candidate classifier’s accuracy and computational requirements to improve system efficiency. Finally, we propose deploying multiple models with various accuracy-computational profiles to the mobile device. The system can then dynamically select the best model to use based on real-time computational resource availability.
RPM Development
In this section, we describe the system development process and identify the challenges we encountered for each stage in Fig. 1 a. In Section 3.1 we discuss the data collection stage. Next, we discuss the data processing stage in Section 3.2 . Section 3.3 presents a comparative analysis of two machine learning algorithms: 1) Support vector machine (SVM) and 2) Multilayer perceptron (MLP). In Section 3.4 we describe the deployment environment and evaluate the RPM system’s mobile computational requirements.
Data Collection
The first step in data collection is to determine the monitoring system’s input sources. Monitoring systems are increasingly analyzing data from a variety of heterogeneous sensors such as ECG and blood pressure (BP) devices to monitor a patient’s physiological deterioration [ 12 ]; interested readers are referred to [ 9 ] for a review on wearable technology. In addition, the growing accessibility of electronic health records using mobile devices [ 4 ] provides new opportunities for monitoring systems to analyze sensor physiological data within the context of a patient’s clinical data. The next data collection step is to collect the training data. Currently, the data collection step is conducted internally to give researchers full control over their training dataset composition. However, creating a training set containing heterogeneous data suitable for our study is a very challenging and time-consuming task. Instead, we decided to share our experience using the Multiparameter Intelligent Monitoring in Intensive Care II (MIMIC-II) database [ 13 ] to develop our system. We selected the MIMIC-II database because it contains both a physiological and clinical database of anonymized intensive care unit (ICU) patients [ 13 ]. Patients with heart disease were identified in the MIMIC-II database as those with a primary International Classification of Diseases (ICD-9) code between 390 − 459 [ 14 ]. In total, 502 heart disease patients with matched physiological and clinical records were identified in the MIMIC-II database. The breakdown of low- and high-risk patients is shown in Table 1 . The techniques for labeling the data are presented in Section 4.1 . A two-sample t test was used to compare continuous variables (e.g., age) while a chi-square test was to compare categorical variables (e.g., gender) between the low and high-risk groups with a p value less than α = 0.05 deemed significant. Our results show that age, weight, systolic and diastolic blood pressure were different between low and high-risk groups. Subsequently, machine learning algorithms may be able to separate the classes by constructing a hypersurface. Using an open source dataset decreases system development and allows researcher access to larger and more diverse training sets.
Training data baseline characteristics
DRG diagnosis-related group [ 15 ], SAPS I simplified acute physiology score I [ 16 ]
Italicized p values are clinically significant ( α < 0.05)
Working with wearable sensors, health records, and published datasets not created specifically for our study have its own set of challenges which we summarize in Table 2 . First, the quality of both wearable sensors and health records remains a challenge when developing monitoring systems. Despite recent advances, the quality of consumer devices remains too low for medical applications [ 17 ]. Similarly, health record data is mostly unstructured and must be converted into a data format suitable for automated analysis [ 18 ]. For example, important clinical data (e.g. patient habits) are currently stored as narrative notes that cannot be natively processed by a computer, thus requiring the development of context-specific natural language processing techniques. Next, there is a need to develop communication protocols to allow devices from multiple vendors to communicate with the monitoring system [ 19 ]. There are also technical, security, and privacy challenges for integrating an external monitoring platform with a hospital health record system. Third, most researchers currently only publish their studies final feature set (e.g., UCI [ 20 ]) which have limited use outside the scope of the original study. In addition, the data quality of online repositories may prevent the analysis of the data using machine learning or signal processing. For example, Physionet’s current guidelines [ 21 ] only set the minimum requirements to ensure a physiological dataset’s compatibility with the waveform viewers which is not suitable for all research applications. There is a need to develop standards for online repositories to enable future signal processing and machine learning applications. Overall, the biggest challenge in the data collection stage was labeling the training examples so they can be analyzed using supervised machine learning algorithms. Labeling the training set (e.g., low or high disease severity) is usually completed manually by a medical expert which can be very time consuming [ 22 ] and limits the size of the training set. In Section 4.1 , we investigate two methods for automatically labeling severity of a patient being at risk of cardiovascular disease.
Data collection challenges
Data Processing
The heterogeneous data must be processed into a set of features suitable for analysis using MLAs. Our data processing stage consists of: 1) Wearable sensor preprocessing, 2) Health record imputation, and 3) Feature extraction. First, sensor preprocessing is used to improve the quality of physiological signals which suffer from noise and motion artifact [ 23 ]. Specifically, the ECG signal undergoes four preprocessing steps: filtering [ 24 ], detrending, ECG signal quality assessment [ 25 ] and R peak detection [ 26 ]. Next, imputation methods are used to deal with the missing and incomplete data in health records [ 27 ]. For example, in our training database 33% of health records were missing data on patient height. We used regression imputation where patients with known age, weight, and height [ 28 ] were used to construct a 2 n d order height imputation model. The final data processing stage is feature extraction for converting continuous physiological signals into discrete values. Our feature extraction stage primarily focused on extracting time, heart rate variability [ 29 ], and frequency features [ 30 ] from 5 minute ECG signals in the MIMIC-II physiological database. No additional feature extraction for BP recordings and health records was necessary because they already contain the features of interest. After reviewing the literature, we identified twenty-four prospective features extracted from ECG, BP, and health records that are used for monitoring CVD. Eleven features (Table 3 ) were successfully implemented and validated for further study.
The 11 features from ECG and BP sensors and health records monitored by M4CVD. C continuous features, D discrete feature
The process for selecting the final feature set is rarely discussed in the literature beyond the use of feature selection algorithms [ 31 ]. However, in our experience the primary feature selection criteria is not a feature’s contribution to model accuracy but rather identifying features that can be successfully extracted and validated. While the data processing challenges summarized in Table 4 are context-specific it is important to discuss these challenges to serve as a guide for future developers of data processing libraries and monitoring systems. First, proposed ECG preprocessing libraries are mostly tested on gold standard datasets [ 32 ] which have less noise and motion artifacts compared to wearable sensor data. There is a need for a gold standard database of ECG recordings from wearable sensors. Second, selecting the proper health record imputation method is a challenge because each method introduces their own level of uncertainty [ 33 ]. Third, the ECG recordings in the MIMIC-II database underwent signal decimation destroying the ECG signal’s frequency component. As a result, both the ECG detection libraries [ 21 ] and the frequency domain feature were not successfully validated. It is outside the scope of this paper to improve the automatic peak detection methods. Only features extracted from the R peak (heart rate, R-R interval heart rate variability, SDNN, rMSSD and pNN50) [ 29 ] were included in the final feature set. The training dataset also rarely included information on patient habits (e.g. smoking and exercise) which were excluded from study. Finally, a common challenge working with heterogeneous data is selecting the data fusion technique to combine the data for analysis using MLAs. In Section 4.2 we present a comparative analysis of two data fusion techniques for combining data from wearable sensor and health records.
Data processing challenges
Machine Learning
The third step as shown in Fig. 1 a was the design and training of the SVM and MLP to predict low or high disease severity. Both classification algorithms are popular in the medical domain [ 23 ] due to their ability to map features to higher dimensional space: the SVM using kernel functions while the MLP uses hidden layers [ 9 ]. Interested readers are referred to [ 34 ] and [ 35 ] for a detailed explanation on the SVM and MLP respectively. Both classifiers were trained and tested on the dataset of 502 patient records containing 11 features extracted from wearable sensors and health records. The LibSVM machine learning library [ 36 ] and MATLAB’s neural network toolbox was used to implement the SVM and MLP respectively. The SVM was trained using 10-fold cross-validation (CV) training with 70% of the dataset for training and 30% for testing. For MLP training the dataset was divided into 80% training and 20% testing sets with 25% of the training data used as the validation set (The cross-validation results are presented in Section 4.3 ). Then, the best SVM and MLP configurations were tested using a Monte Carlo simulation where each algorithm was trained and tested 1000 times on a random subset of training examples. No patient record was used in both the training and testing set during the same simulation run. The Monte Carlo results and mean receiver-operator curves (ROC) [ 37 ] for each classifier are shown in Table 5 and Fig. 2 respectively. Both models achieved stable and reusable parameter configurations. Our results show that the SVM had the best overall performance. The SVM appears to generalize consistently across simulation runs as the SVM always finds the global minima solution. On the other hand, the MLP update it’s weights and bias individually so it is more sensitive to the variability within each feature. Based on classifier accuracy we would recommend the SVM for CVD severity classification. The best SVM and MLP were then deployed to a mobile environment for further testing as discussed in Section 3.4 .

ROC curve for severity estimation. The mean of 1000 experiments have been shown for each classifier
M4CVD Performance for SVM and MLP. The mean of 1000 experiments is shown
Our results are promising since they do exceed those of current early-warning system which monitor physiological indicators [ 38 ]. The early-warning system was implemented in twelve hospitals over a six month period and identified 30% (95/611) of patients who were subsequently admitted to the ICU. Nevertheless, existing algorithms are designed for analyzing homogeneous data from a single data source. As a result, there a number of challenges (Table 6 ) using machine learning for analyzing heterogeneous data on a mobile device. First, there is a need for new algorithms that can analyze heterogeneous datasets [ 39 ]. Such algorithms will need to deal with structured, semi-structured, and unstructured data simultaneously [ 40 ]. Second, deployed MLAs cannot incorporate new data without expert supervision. Third, the main challenge we identified is that the current classifier training methodology focuses on determining the model configurations that maximizes the model’s classification performance (e.g., accuracy). In Section 4.3 we propose a new training methodology for machine learning that evaluates a model using classification performance and mobile computational complexity. Finally, many RPM systems we reviewed (Section 5 ) were only evaluated using accuracy which can lead to suboptimal solutions [ 41 ]. Researchers should also report precision and recall or F1 scores when discussing classifier performance.
Machine learning challenges
Deployment and Hardware Evaluation
In this paper, the development and deployment of M4CVD was done on different target hardware. We used a 64-bit Windows 7 laptop with a 2.2 GHz Intel i7 CPU and 12 GB RAM using MATLAB 2014A for developing the monitoring system. The final system was then deployed in C+ + to a Linux Raspberry Pi 2 Model B (RASPI), a single board computer (Quad-core, ARMv7, 900 MHz CPU, 1 GB RAM) with similar performance to the low-cost 2014 Motorola Moto G.
Table 7 shows the computational requirements for the input, data processing, and deployed classifier modules. Our initial hypothesis was that machine learning models present a considerable burden for low resource devices because they have a complexity order of approximately O ( n ) 3 [ 9 ]. Surprisingly, our results show that the analysis stage required among the lowest computational resources in terms of execution time and current consumption. Instead, the signal acquisition and data processing modules were major computational bottlenecks in our mobile monitoring system. The most computationally expensive components in our system were the ECG quality assessment and R peak detection stages due to a large amount of raw physiological data processed. Interestingly, Table 7 shows that the support vector machine and multilayer perceptron had very different computational requirements despite their similar classification performances. The SVM took 70x longer and required 2X the current compared to the multilayer perceptron. The different computational requirement appears to be a result of how each model classifies new data after deployment. The SVM constantly maps each input data vector into higher dimensional space using the kernel function which can be computationally expensive. On the other hand, once deployed the MLP is a series of equations requiring less computational resources. Overall, our results demonstrate that the MLA’s complexity was not a barrier for adoption on a mobile device. In fact, our findings suggest that many RPM systems already run the most computationally expensive modules (data collection and processing) locally. We recommend the MLP for deployment in a mobile monitoring system because the MLP has similar classifier performance and superior mobile computational performance compared to the support vector machine.
Hardware consumption for acquisition, data processing and deployed classifier modules on Raspberry Pi 2
The highest value for each metric is in italics
Deploying a monitoring system to a mobile device and evaluating the system’s computational performance is a non-trivial and time-consuming task (Table 8 ). First, there is a need for preprocessing and machine learning libraries that are optimized for deployment on a mobile device. For example, the support vector machine can be implemented using fixed-point arithmetic which is less computationally expensive [ 42 ]. Next, developers should consider both accuracy and computational power when selecting the preprocessing techniques, features, and classifier for their monitoring systems. Third, popular MLA libraries [ 43 , 44 ] assume that model training and deployment occurs in the same computational environment. Future libraries should support training and deployment to different platforms natively. The next generation of RPM systems will be deployed entirely on mobile devices with little communication with remote servers. However, the main challenge with existing mobile RPM systems such as M4CVD is that they have constant computational requirements regardless of the current usage environment. In Section 4.4 , we propose a methodology to allow a monitoring system to adapt their classification module based on user preferences and the current system condition. However, evaluating the computational requirements for mobile systems requires its own experimental procedure and setup which extends classifier training and system development time.
Deployment and mobile computational requirement challenges
Recommendations
In this section we propose a system development methodology (Fig. 1 b) that addresses the four main decision points identified in this paper: 1) training data labeling method, 2) heterogeneous data fusion, 3) optimizing machine learning classifiers for a mobile environment, and 4) adapting MLA based on current computational requirements. In Section 4.1 we investigate using automatic techniques to label our training set. Section 4.2 compares two data fusion techniques (feature and decision-level fusion) for combining heterogeneous data sources. Note that our recommendations for automatic data labeling and heterogeneous data fusion are based on our experience developing M4CVD and are domain specific. We also propose a machine learning training methodology that considers both classification performance and computational cost during cross-validated training in Section 4.3 . In Section 4.4 we propose a deployment methodology for dynamically selecting the best classifier based on the current computational resources available on a mobile device. Our recommendations for extending the MLA training and deployment methodology can be used when developing classifiers for any mobile application.
In this section, we investigate two methods to automatically label the disease severity of the 502 patient records used to train M4CVD: 1) Simplified Acute Physiology Score I (SAPS) [ 16 ] and 2) Diagnosis Related Group (DRG) [ 15 ]. SAPS is an intensive care unit (ICU) patient severity scoring system. DRG is a USA hospital payment classification system that measures the relative amount of resources used to treat the patient which we use as an indicator for patient severity. Both metrics are calculated by health professionals during the patient’s hospital stay and stored in the MIMIC-II database.
Once the SAPS and DRG scores were retrieved for each patient record, the next step was to separate the training examples into low and high severity classes using the automatic prioritization of ICU patients method proposed by [ 45 , 46 ]. High-risk patients were defined as those whose severity score was above the calculated median scores. Overall, 54 and 51% of patient examples were labeled high severity based on their SAPS and DRG score respectively. Table 9 compares the classification results for each labeling technique across a subset of the classifier configurations tested. Our results show that both models could be trained to distinguish between low and high-risk patients using data labeled automatically by the SAPS or DRG metrics. The support vector machine had higher classification performance using the SAPS while the multilayer perception showed improved performance using the DRG labels.
Comparison of SAPS I and DRG automatic labeling techniques for cross-validation (k = 10) training
Automatic labeling offers several advantages. First, automated labeling enables developers to build models using larger datasets compared to datasets that are labeled manually. Next, automatic labeling is a method for incorporating pre-existing medical knowledge into MLAs. Third, automatic labeling reduces system development time. Automatic labeling can serve as a preprocessing step to evaluate the distribution of a dataset and identify the best data subset for manual expert labeling. However, automatic labeling can be domain specific and time-consuming to develop. In addition, an important area to investigate is the agreement between labels generated by automated techniques and human experts. Finally, automatic labeling may not always be available. An alternative labeling method is unsupervised learning [ 47 ] which is a class of algorithms used to discover hidden patterns or groupings from unlabeled datasets. Interested readers are referred to [ 48 ] for a detailed explanation on unsupervised learning.
A data fusion stage is increasingly used in monitoring systems to combine heterogeneous data into a single higher dimension feature vector. Multiple data fusion techniques have been used in the literature; interested readers are referred to [ 49 ] for a full review. However, as far as we know a comparison between fusion methods on the same monitoring system has not been presented. In this section we compared two data fusion techniques on a mobile device: (1) feature-level and (2) decision-level fusion [ 50 , 51 ]. While our comparison in this section is domain specific, our recommendations can serve as a starting point for researchers developing systems that combine data from heterogeneous sources.
Feature-level fusion is the simple concatenation of heterogeneous features into a single input vector [ 52 ]. However, each extracted feature has their own numeric ranges which present a challenge. During training features with large physiological ranges may be assigned more weight regardless of the importance of the feature to classification accuracy [ 53 ]. The range bias can be removed by normalizing all features to a range of (0,1). Feature-level fusion can be very powerful because it allows us to correlate features across data sources and is not computationally expensive. However, feature-level fusion requires a large training dataset in order to apply feature selection algorithms [ 31 ]. On the other hand, decision-level fusion allows us to incorporate medical knowledge directly into our model. Before concatenation, each feature is first evaluated individually to make a local decision. The classifier then makes a high-level decision by analyzing all the local decisions [ 52 ]. In this paper, healthy and unhealthy ranges set by The Canadian Heart and Stroke Foundation [ 54 ] were used for each local decision (Table 10 ). Each feature was assigned a category corresponding to each its range of healthy and unhealthy values (e.g., 1–4) and normalized to remove range bias. Features without healthy and unhealthy ranges (e.g., age) were normalized.
Decision-level data fusion local decision ranges for each feature
Both feature and decision-level fusion were tested across all classifier training configurations, a subset of results is shown in Table 11 . Interestingly, both models showed improved performance using feature-level fusion that did not incorporate any a priori medical knowledge. Our results demonstrate the risk of injecting designers’ bias into the model using decision-level fusion. For example, the physiological ranges used in Table 10 are based on the overall healthy population. However, our training set on average has higher mean values for each feature compared to the overall population because the patients have CVD. As a result, the local decision assigns many of the training patient’s features as medium or high risk (few low risk) reducing the classifier’s sensitivity. On the other hand, when no decision-level fusion is conducted the machine learning algorithm determines for itself the relative importance of each input feature individually without the need for expert input. The MLP considers each feature importance by updating each weight and bias individually through back-propagation [ 55 ]. Both feature and decision-level fusion were not computationally expensive but decision-level fusion does introduce additional computational overhead.
Comparison of feature and decision-level data fusion techniques for cross-validation (k = 10) training
Currently, the objective of training MLAs is to determine the best architecture (e.g., kernel and learning function) and user-defined parameters (e.g., C, gamma, number of neurons) that maximize the model’s classification performance. Our proposed methodology extends MLA training to evaluate each model configuration’s classification performance (e.g., accuracy) and mobile computational requirements. First, each configuration is trained and tested using the traditional cross-validation technique. For example, Fig. 3 a shows the traditional cross-validation accuracy results for the SVM presented in Section 3.3 . Next, each model is deployed to the target mobile device and evaluated in terms of current consumption, execution time, CPU and memory usage. As a work in progress, we evaluated the SVM training and computational testing on a Windows 7 laptop with 2.2 GHz Intel i7 CPU and 12 GB RAM using MATLAB 2014A. Finally, a cross-validation graph showing how the performance metrics change with different model configurations was generated. Figure 3 b demonstrates how the SVM’s configuration effects the model’s execution time. Developers can use Fig. 3 to study the trade-offs between a classifier’s accuracy and efficiency. For example, examining Fig. 3 the highest classifier accuracy was 65.3% and took 1.1 ms to run. However, the developer may decide that a 5% decrease in accuracy (65.3% down to 60%) is an acceptable trade-off to save 36% in execution time (1.1 ms down to 0.7 ms) increasing the monitoring systems’ operation time. The optimal model is now the one that balances both accuracy and execution time.

The proposed cross-validation procedure examines both accuracy ( a ) and normalized execution time ( b ) to identify the best overall SVM classifier
Our proposed training methodology provides developers a better indicator of their classifiers overall performance. The proposed methodology can be used when developing classifiers for any mobile application as our method extends the MLA training procedure. However, our methodology will increase the model’s training time compared to traditional cross-validation training since every candidate model is deployed and tested on the mobile device. In addition, our proposed methodology would require the development of an automated procedure to deploy the classifier to the mobile device and evaluate its computational performance.
The final stage in Fig. 1 b is deploying the classifier to the mobile device. However, once deployed existing monitoring systems cannot adapt their model’s computational resources based on real-time resource availability. A potential solution is to deploy multiple classifiers with various accuracy-computational profiles to the mobile device. Our study shows that multiple classifiers can be stored on a mobile device due to each model’s small storage requirement (SVM- 68 KB, MLP- 20 KB). Figure 4 shows our proposed model for selecting the best classifier. Figure 4 a shows the normalized run times for 100 SVMs. In this paper, we assume the model with the shortest run time also has the lowest resource requirements. The user selects the minimally acceptable runtime they will accept (yellow plane) and Fig. 4 b shows the maximum normalized accuracy the system can achieve under the user constraints. In this case, our model shows that there is no trade-off between accuracy and execution time until 0.5 normalized run time after which decreasing the classifier’s execution time reduces its classification accuracy. Interestingly, we only need to deploy three of the 100 SVMs to capture the full range of accuracy and computational trade-offs corresponding to the main inflection points in Fig. 4 b. Our proposed model will further increase the efficiency of classifiers running on mobile and low resource devices.

The proposed deployment model allows the user to select the trade-off between the SVM’s computational usage ( a ) and accuracy ( b )
The proposed methodology for dynamically selecting a MLA’s configuration can be used when deploying any classifier into a mobile environment. In addition, the proposed methodology can be automated as many mobile systems provide access to the device’s current computational status (e.g., CPU, RAM, battery life). For example, if the monitoring system’s battery life goes below 10% the system can automatically switch to the most efficient classifier to extend the system’s operation time. The proposed model allows the user to visualize the trade-off between system accuracy and execution time.
Related Work
In this section, we review existing remote monitoring systems in terms of their data collection (Section 5.1 ), processing (Section 5.2 ) and analysis (Section 5.3 ) modules.
Early RPM proposals measured only a single physiological signal, primarily ECG [ 56 ] and activity level [ 17 , 42 ]. Increasingly, RPM systems are monitoring multiple physiological signals using wearable devices [ 6 , 57 ] or ICU monitors [ 58 , 59 ]. However, most monitoring systems we reviewed used the local device for signal acquisition only, despite mobile phones having the computational power to support MLAs [ 60 ]. Existing systems also do not integrate with electronic health record repositories despite their growing accessibility on mobile devices [ 4 ]. Instead, existing systems only collect and display basic clinical data to the health professionals [ 61 ]. In addition, the majority of the papers we reviewed [ 59 , 62 – 64 ] had their own internal data collection stage or used an open-sourced database [ 65 ]. However, most training records are annotated manually by experts [ 3 , 63 , 65 , 66 ]. As a result, the size of training sets in existing studies has been small ranging in size from only a few dozen [ 62 , 63 ] to a few hundred [ 59 , 64 ] patients. Existing studies on monitoring systems have focused on describing each system’s implementation and accuracy. In this paper, we explored the challenges of developing the acquisition, processing and analysis stages for a monitoring system that analyzed heterogeneous data on a mobile device. We also investigated the use of hospital severity metrics to label a large training set automatically.
The data processing stage consists of preprocessing, feature extraction and data fusion to combine heterogeneous data. Most physiological preprocessing modules involve low/high pass filtering [ 56 , 67 ], signal amplification [ 68 ] and basic feature detection (e.g., R peak [ 67 ]). The features extracted from the preprocessed signals have varied considerably between RPM systems [ 23 ] depending on the combination of features that best maximize each system’s accuracy. In current systems, the feature extraction stage has occurred primarily on remote servers [ 2 , 3 , 65 , 69 ] but is increasingly being completed on low resource devices [ 10 , 60 ]. While developing preprocessing and feature extraction techniques remains an active area of research [ 25 , 69 ], the computational requirements for these stages on low resource devices have not been investigated in depth [ 23 ]. In this paper, we evaluated the computational requirements for M4CVD’s preprocessing and feature extraction stage. Surprisingly, our results show that the preprocessing stage was the most computationally demanding component of our system.
Multi-sensor monitoring systems have traditionally analyzed [ 2 ] and displayed [ 70 ] each sensor stream independently. Recently, RPM systems have begun to use data fusion techniques to combine data from multiple sources for analysis [ 49 ]. Feature-level fusion is the most common data fusion technique used in monitoring systems [ 2 , 59 , 71 ]. Decision-level fusion has also been used to detect abnormal physiological signals [ 64 ] and label sensor data with the patient’s current activity level [ 63 ]. However, existing surveys on sensor fusion techniques [ 49 ] do not compare the effectiveness of different techniques using the same RPM system. In this paper, we compared the classification performance and computational requirements for both feature and decision-level fusion in the same monitoring system.
Machine learning algorithms are increasingly being used in the medical field for screening, diagnosis, treatment, prognosis, monitoring and disease management [ 72 ]. In monitoring systems MLAs are primarily used for novelty detection [ 2 , 69 ] and severity classification [ 3 , 64 , 65 ] applications. The main limitation of these systems is that the data analysis occurs on remote servers requiring continuous data transmission. Increasing mobile computational power provides new opportunities to deploy MLAs directly on the low resource device. For example, HeartToGo [ 60 ] used MLAs deployed on a mobile device to classify ECG signals with an accuracy of 90%. However, HeartToGo only monitors a single wearable sensor. Another example is the CHRONIOUS platform [ 10 ], a mobile RPM system for patients suffering from chronic obstructive pulmonary and kidney disease which achieves an accuracy of 95% [ 10 , 73 ].
Multiple studies have conducted a comparative analysis of MLAs [ 3 , 5 ]. Overall, the SVM has slightly better performance compared to the MLP in monitoring patient severity. For example, Clifton et al. [ 2 ] used ICU monitors to analyze patient respiratory rate, HR, and BP to detect periods of signal abnormality. The SVM performed best out of the five classifiers tested with an accuracy of 95%. Another comparative analysis was conducted during the development of the CHRONIOUS system [ 10 ] where both the SVM and MLP achieved a similar accuracy of 89% and 87.5% respectively. Existing comparative analyses have focused on evaluating a system’s classification accuracy. However, a key difference between mobile and remote server-based systems is the limited computational resources available on the mobile device. Understanding the system’s resource requirements is a key metric to assess the systems overall usability and to identify areas of improvement. Despite this importance, only a few studies have investigated their system’s resource requirements in-depth [ 11 , 68 , 74 ]. In this paper, we have evaluated the SVM and MLP in terms of both their classification performance and execution time. We have also proposed a novel training and deployment methodology for MLAs operating on mobile devices.
Conclusion, Limitations, and Future Work
Advances in mobile technology provide new opportunities to analyze collected data directly on low and even ultra-low resource devices. However, our findings show that there are specific challenges when monitoring systems are being developed for mobile platforms. In this paper, we presented a case study to systematically investigate the challenges we faced in the design, implementation, and deployment of a mobile monitoring system. Based on our findings, we developed recommendations for each development stage which can be used as guidelines by future researchers, system designers, and developers working on mobile-based monitoring systems. While most of our recommendations are stage-specific, our proposal to evaluate classifiers based on accuracy and computational performance is applicable throughout the development process. For example, MLA features could be evaluated based on their contribution to both model accuracy and computational overhead. The work presented in this paper contributes towards the goal of personalized predictive monitoring.
Our study also exhibits some limitations. First, our recommendations are domain specific and do not account for the data collection, processing and analysis techniques used for monitoring other chronic diseases such as respiratory disease and diabetes. In addition, the implementation challenges for the communication, security and privacy modules for a monitoring system on a mobile device were not investigated in this paper.
In view of these results, our next step is to generalize our methodology by investigating other MLA-based mobile systems. Future work will also focus on developing feature selection and training methodologies that consider both classifier accuracy and mobile computational requirements during the optimization of machine learning algorithms. The training methodology will require heuristic algorithms to automatically find satisfactory solutions in the model configuration search space. We are also investigating MLAs that can incorporate new data without constant expert supervision. Finally, we will consider testing the monitoring system using other classifiers such as random forest trees and multi-class MLAs.
Acknowledgments
Support from the McMaster School of Biomedical Engineering, McMaster Science & Research Board (SERB), Vector Institute for Artificial Intelligence, and Natural Sciences & Engineering Research Council of Canada (NSERC) is acknowledged.
Compliance with Ethical Standards
The authors declare that they have no conflict of interest.
MACHINEMETRICS BLOG
Machine vs. production monitoring: the road to production..., 2024 product releases: an inside look, introducing batch connect machines, introducing condition monitoring workflows.
Ready to empower your shop floor?
- MachineMetrics
- Product Updates
- Data Science
- Lean Manufacturing
Machine Monitoring
IoT in Manufacturing: Top Use Cases and Case Studies
Updated May 17, 2021
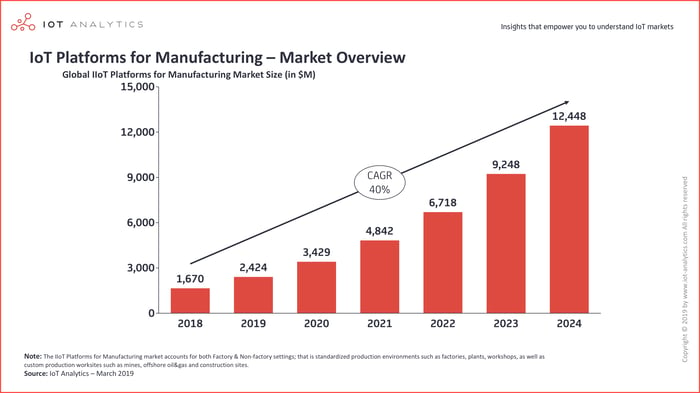
Within this article, we’ll be discussing practical IoT applications in manufacturing and use cases of industrial IoT technology in manufacturing
What is IoT?
What is iiot, the benefits of iot in manufacturing.
IoT represents a digital transformation in manufacturing processes and business operations. Using it alongside an advanced machine data platform can be transformational. And there are many benefits of IoT in manufacturing:
Process Optimization
Inventory Management
Predictive Maintenance
IoT in Manufacturing Use Cases [+Case Studies]
Remote monitoring.
Learn more about remote monitoring for machine builders and OEMs.
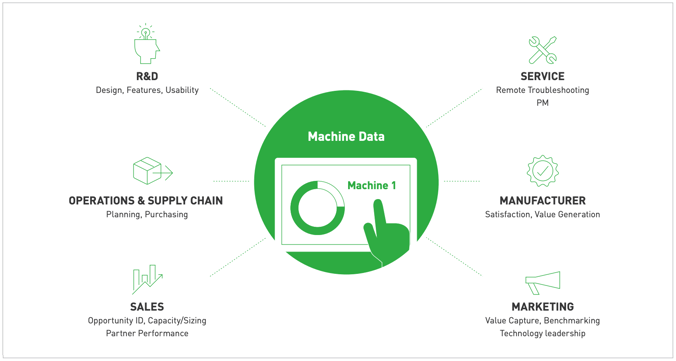
Supply Chain Management and Optimization
- Real-time tracking of assets and products
- Automation of warehouse tasks
- Digitized paperwork management
- Forecasting accuracy improvement
- Greater control of inventory

Digital Twins
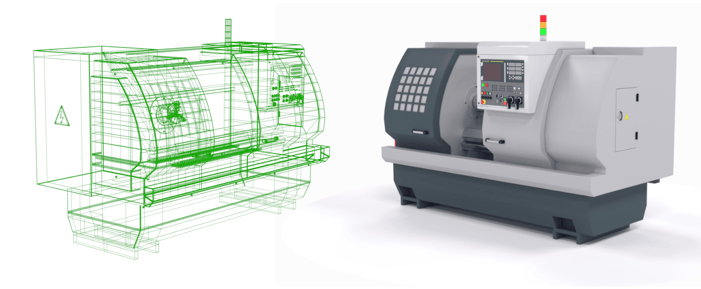
Real-Time Machine Monitoring
Production visibility.
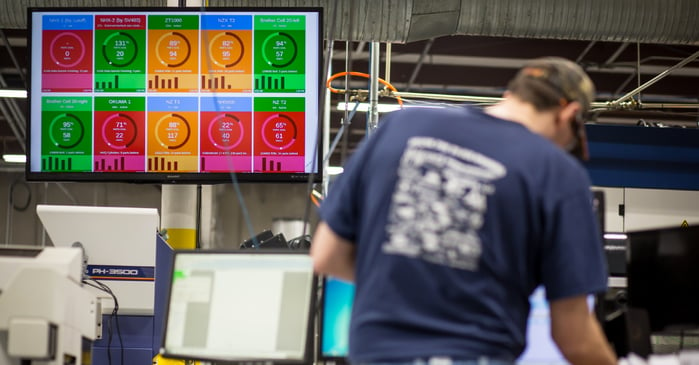
Integrating Systems
Compiling kpis.
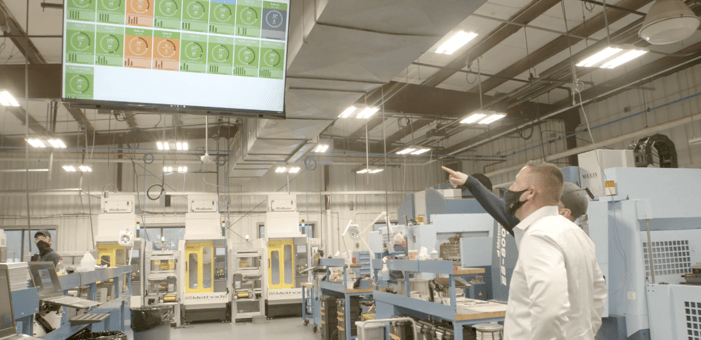
Asset Utilization
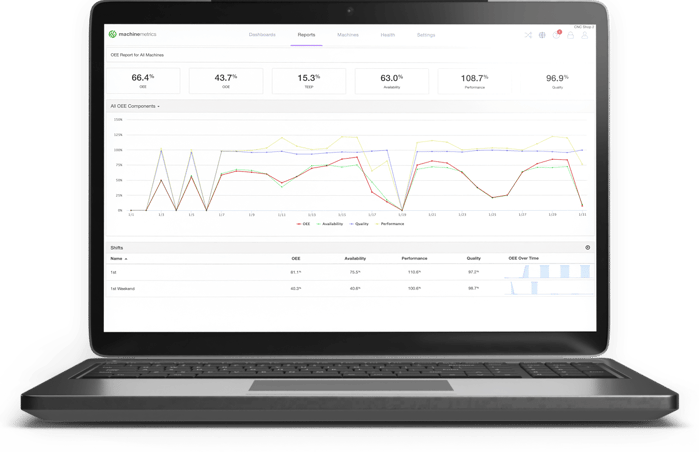
Difficulties of Adopting IoT in Manufactur ing
1. large investments are required and the roi is questionable, 2. concerns about data security, 3. employees who aren’t qualified, 4. integration with operational technology and older systems, how to use iot and machine data for remote operations, boosting your operational efficiency with iiot, subscribe to our mailing list, related posts, read the latest.
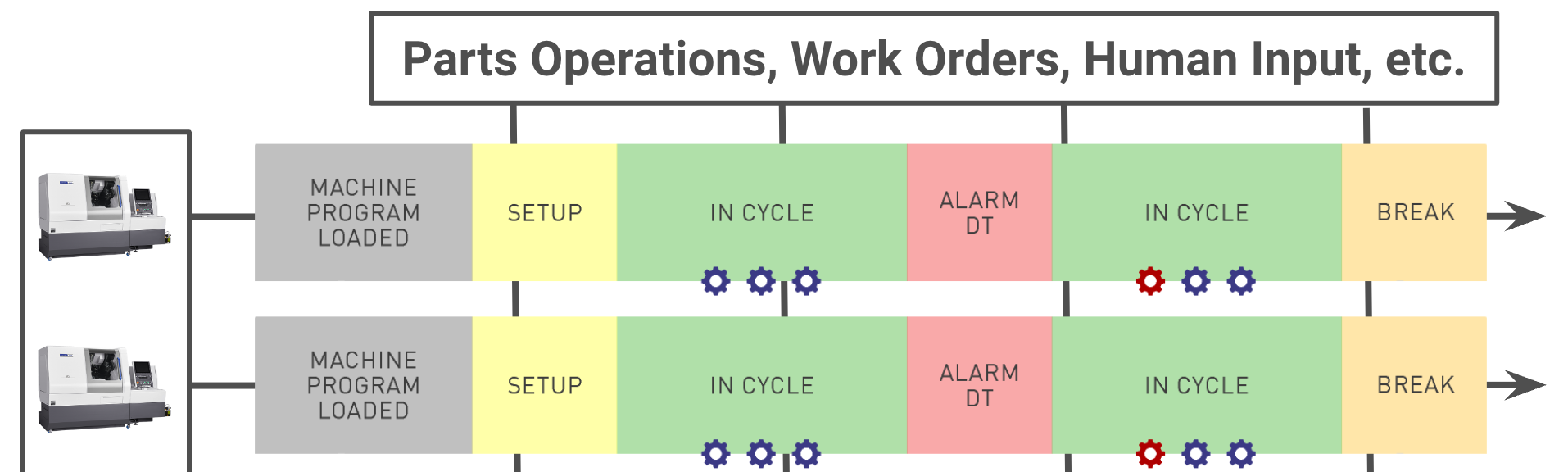
Lists by Topic
- MachineMetrics (382)
- Lean Manufacturing (72)
- Industry 4.0 (50)
- Manufacturing data (23)
- Manufacturing News (20)
- Data Science (15)
- data collection (14)
- Product Updates (12)
- Machine Monitoring (10)
- manufacturing analytics (10)
- Connected Factory (9)
- Smart Manufacturing (9)
- industrial iot (9)
- CNC Machines (8)
- Productivity (8)
- Downtime (7)
- Industrial Automation (7)
- Tool Monitoring (7)
- Big Data (6)
- Data Visualisation (5)
- Edge Computing (5)
- Maintenance (5)
- Process Optimization (5)
- Shop Floor (5)
- digital manufacturing (5)
- Automotive (4)
- Machine Learning (4)
- Quality Assurance (4)
- Aerospace and Defense (3)
- Contract Manufacturing (3)
- Data Cleaning (3)
- Death of MES (3)
- Heavy Machinery Manufacturing (3)
- MES software (3)
- Medical Device Manufacturing (3)
- OEE Software (3)
- Oil and Gas Manufacturing (3)
- capacity (3)
- continuous improvement (3)
- inustrial IOT (3)
- preventative maintenance (3)
- security (3)
- 8 wastes (2)
- Condition Monitoring (2)
- Dashboards (2)
- Internet of things (2)
- Production Monitoring (2)
- Strategic Partnerships (2)
- management (2)
- press release (2)
- real-time analytics (2)
- Digital Transformation (1)
- Downtime Categorization (1)
- FANUC FOCAS (1)
- Line Balancing (1)
- Machine Tool Distributors (1)
- Machinery (1)
- Manufacturing Innovation (1)
- Production Scheduling (1)
- Quality Control (1)
- Supply Chain (1)
- Turnkey contracts (1)
- coaching (1)
- elon musk (1)
- employee engagement (1)
- link roundup (1)
- manufacturing (1)
- manufacturing software (1)
- networks (1)
- release notes (1)
844-822-0664
Easthampton Office
116 Pleasant St, Suite 332, Easthampton, MA 01027
Edge Platform
Cloud Platform
APIs & Applications
Production Monitoring
Condition Monitoring
For Machine Builders and Distributors
For Developers
Aerospace & Defense
Contract Manufacturers
Heavy Machinery
Medical devices
Oil & Gas
Precision Metalworking
ERP Integration
Metal Stamping & Fabrication
Tool, Die & Mold CNC
ROI Calculator
Waste Calculator
Connectivity Hub
Partner Program
Privacy Policy
Data Processing Addendum
Service Level Agreement
Website Terms
- Log in | Sign up
- Use Kaa for free
- Kaa IoT Platform
- Kaa IoT Gateway
- Node-RED Hosting
- Kaa Cloud Migration
- Edge-to-Cloud IoT Development
- IoT Data Analytics
- IoT Services
- Webinars & Tutorials
- Customer stories
- IoT Knowledge Base
- Agriculture
- Smart Energy
- Smart Retail
- Consumer Electronics
- Industrial IoT
- Sport & Fitness
- Smart Metering
- Smart Building
- Fleet Management
- Air Quality Monitoring
Machine Condition Monitoring: A Detailed Guide & Case Study
An average large plant loses 25 hours a month to unplanned downtime, according to The True Cost of Downtime report by Siemens. It’s more than a full day’s production. Machine breakdowns cost billions in lost revenue and repairs for manufacturers.
What does the IIoT (Industrial Internet of Things) have to offer? Let’s take a look at machine condition monitoring. It’s a proactive approach that relies on real-time sensor data to predict faults, including bearing issues, excess heat, and gear tooth surface wear.
In this article, we'll explore the efficiency of machine condition monitoring, compare it to traditional methods, and delve into its operational stages. Also, we will introduce our machine condition monitoring solution in collaboration with SIA Connect .
What’s machine condition monitoring?
Machine condition monitoring, also known as condition-based monitoring, is a maintenance approach that anticipates machine health and safety. It relies on real-time machine sensor data to predict potential issues.
Condition monitoring techniques extend across diverse equipment types, including rotating machinery, auxiliary systems, and components like compressors, pumps, motors, and presses. This comprehensive approach considers various factors, including efficiency, wear and tear, performance indicators, usage statistics, and maintenance records.
Which faults can be identified through machine condition monitoring?
- Bearing issues
- Excess heat
- Shaft unbalance
- Gear tooth surface wear
- Load misalignment
- Stator eccentricity
- Other critical machine failures
In conclusion, machine condition monitoring lets companies use real-time data from their assets to track and improve the performance and maintenance of their machines. Through this approach, companies can ensure that their machinery won't break unexpectedly and need excessive maintenance.
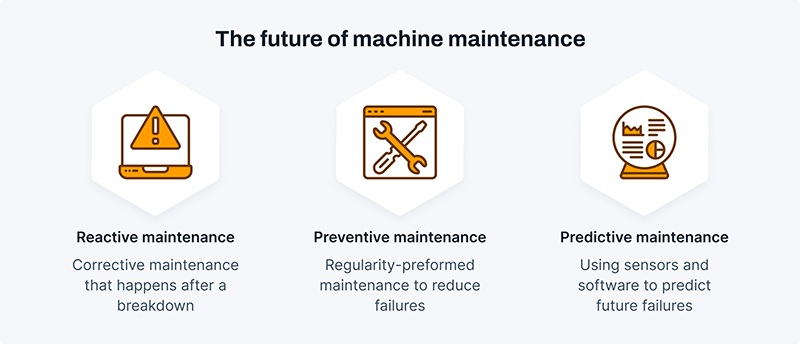
Adding condition monitoring is a key part of creating a strong, condition-based maintenance strategy or a predictive maintenance approach for industrial machinery and equipment. Therefore, manufacturers can support maintenance tasks for equipment at the right time, instead of maintaining it too much or waiting for it to break down.
Traditional machine monitoring vs. condition-based monitoring
Most manufacturing companies, on the other hand, continue to use traditional strategies like reactive or calendar-based maintenance. The primary barrier to machine condition monitoring adoption is the need for more machine condition data, which limits manufacturers' options. They can maintain equipment on a schedule or wait for failures. These ineffective solutions lead to significant waste, such as needless maintenance costs and extended periods of machine downtime.
Of course, traditional machine monitoring offers valuable machine-uptime data for quick reactions to downtime events. Condition-based monitoring, on the other hand, gives you more in-depth machine analytics. The limitations of the traditional approach impede the ability to diagnose problems accurately and adjust processes to optimize asset performance. The consequence is a reliance on either costly preventative measures or reactive maintenance, lacking a true understanding of the underlying issues.
Also, simple tracking for machine downtime forces manufacturers to either do expensive, pointless preventative maintenance or rely on reactive maintenance, which fixes the problem without really understanding what caused it until it happens again. The interesting thing is that over-maintenance, which usually happens because of a strategy based on a calendar, can be just as wasteful as unplanned downtime because it costs money and takes time.
How does machine condition monitoring work?
Condition monitoring has evolved significantly from the days of manually feeling vibrations with a wooden stick. Digital technology and the Internet have propelled it forward. Today, real-time monitoring enables engineers to schedule maintenance precisely when needed.
Modern machine condition monitoring operates in three key stages.
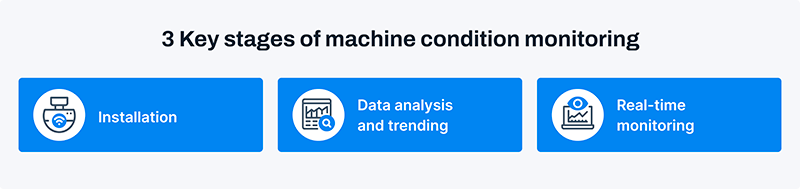
- 1 Installation The first step is the installation of IoT monitoring sensors on operational assets, including rotating machinery (turbines, compressors, pumps, motors, fans) and stationary assets (boilers, heat exchangers). Sensors are strategically placed on your equipment, capturing data measurements for vibration, temperature, and other metrics.
- 2 Data analysis and trending The installation of the monitoring system allows you to start measuring the performance of your machinery. Prior to anything else, you need to take some baseline measurements. Once you have this, you can use it as a benchmark to ensure that your equipment is running at peak efficiency.
- 3 Real-time monitoring The system continuously analyzes data, triggering alerts when abnormalities indicate potential issues. The system can now monitor your systems using sensors and condition monitoring software that will evaluate performance and provide diagnostics. The system can also send out an alert when an operational abnormality is detected and assess the data to determine if immediate action is required or if the machine can operate for a while longer while maintenance is scheduled. This allows for targeted maintenance before breakdowns occur.
Why is machine condition monitoring efficient?
The global machine condition monitoring market is expected to reach $4.7 billion by 2029, growing at a compound annual growth rate of 8.3% from 2024, according to the MarketsandMarkets study . The main driver behind machine condition monitoring adoption is a rising inclination towards wireless communication.
The advent of wireless communication technology allows the deployment of small, easy-to-install sensors in areas where fixed cabling is impractical. This innovation enables high-frequency condition monitoring data acquisition, overcoming challenges related to location, cable length, and installation space availability, ultimately facilitating remote machinery monitoring.
For many industries, machine breakdowns are a costly reality. Unwanted pauses cause a halt in production, revenue losses, and may even lead to safety risks. Yet, these hurdles can be addressed with machine condition monitoring.
Condition monitoring is more than just checking if the machine is 'ON' or 'OFF'. It enables proactive maintenance. The sensors linked to your gear gather machine health data in real-time. You can tell how well your machinery works by measuring relevant indicators like temperature, vibration, and performance.
Machine condition management isn't just about fancy technology. It's about empowering informed decision-making. Operators, managers, and maintenance teams gain deeper insights into machine performance, making better choices that enhance overall production and profitability.
Think of machine condition management as the happy medium between outdated calendar-based schedules and reactive panic mode. It's mature, data-driven maintenance that extracts the most value from your equipment, ensuring a healthier, more efficient, and ultimately more prosperous operation.
As we've seen, condition-based monitoring isn't all about high-tech devices. It's about bringing actual gains to your operations. The specific benefits you can get from this proactive approach are worth going over in more detail.
Benefits of machine monitoring
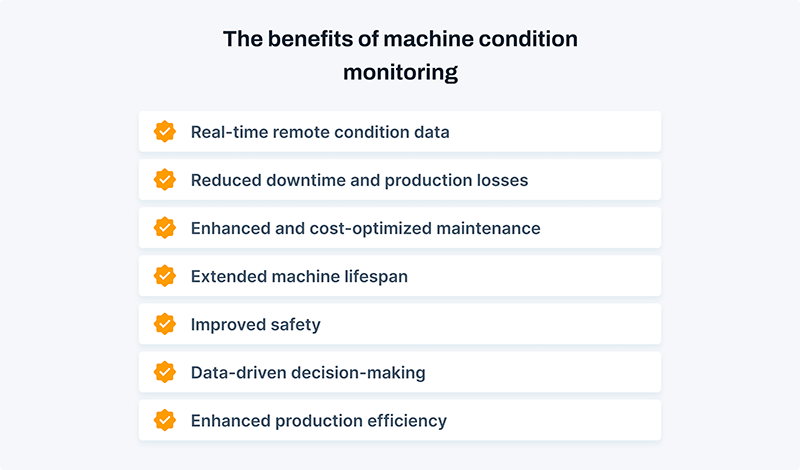
Real-time remote condition data
Machine condition monitoring isn't just about a single snapshot of equipment health. The true power lies in seeing the bigger story. It's a continuous dialogue, revealing your machinery's inner workings through real-time data streams. Tracking multiple conditions simultaneously across diverse machines unlocks data-driven insights on overall machinery health and performance.
IoT analytics can display this data in real time with push notifications, allowing engineers and operators to visualize previously invisible aspects of the condition on the shop floor. In addition, the true worth of a machine monitoring program is realized when multiple conditions can be monitored at once.
By giving a comprehensive picture of asset health over time, the collected data helps you identify the source of issues and avoid needless failures. Having this supplementary information will allow engineers to make well-informed decisions regarding their machinery and any necessary maintenance. It will also empower you to swiftly adapt to real-world conditions and plan maintenance strategically.
When a change in running levels is detected across the production chain, operators can assess the source of the problem and take appropriate action. In addition to setting health and performance thresholds, you can adjust them according to how machines are used in your operations. This allows you to plan maintenance schedules accordingly.
Your machines might not require maintenance as often as recommended. Alternatively, consider a scenario in a hot, humid facility where machines degrade faster, demanding more frequent maintenance. Condition monitoring empowers you to align schedules with actual performance, optimizing uptime and minimizing unnecessary costs.
Reduced downtime and production losses
Machine condition monitoring involves the continuous or periodic assessment of the health and performance of industrial machinery. By using various sensors and data analysis techniques, it can detect potential issues, abnormalities, or signs of wear and tear at an early stage. This early detection is critical because it allows maintenance teams to address problems before they escalate into major failures.
With the data collected through condition monitoring, predictive maintenance strategies can be implemented. Rather than following a fixed schedule for maintenance, which may lead to unnecessary downtime and production interruptions, machine condition monitoring allows maintenance activities to be scheduled based on the actual condition of the equipment. This proactive approach ensures that maintenance is performed when needed, reducing unexpected breakdowns.
By addressing potential issues before they lead to equipment failure, machine condition monitoring helps minimize unplanned downtime. Unexpected breakdowns can be disruptive to production schedules and can result in significant financial losses. By preventing these breakdowns, businesses can maintain a more consistent and reliable production flow.
Continuous monitoring allows for the optimization of equipment performance. By identifying and addressing inefficiencies or malfunctions promptly, the machinery can operate at its optimal level. This optimization contributes to maintaining a steady and reliable production process, reducing the likelihood of interruptions that can result in production losses.
Machine condition monitoring positively impacts overall equipment effectiveness (OEE), which is a metric that assesses how well equipment is utilized in terms of availability, performance, and quality. By improving the reliability and efficiency of machines, you increase OEE, which leads to higher productivity and reduced losses.
Ultimately, the reduction in downtime and production losses translates into significant cost savings for the business. Investing in machine condition monitoring technologies and practices not only enhances operational efficiency but also leads to substantial savings. It serves as a compelling justification for businesses to embrace condition-based monitoring.
Enhanced and cost-optimized maintenance
Maintenance costs, seemingly modest per asset, accumulate significantly across numerous plant-wide assets. Just a 10% reduction through condition monitoring will significantly impact overall plant profitability. This strategic planning tool enhances insight into asset management, enabling proactive maintenance before functional failures.
Opting for a 'fix as it breaks' approach may seem cost-effective initially, but it proves more expensive in the long run. Condition monitoring enhances maintenance efficiency by pinpointing faults, eliminating the need for engineers to inspect operational components while identifying issues. This not only accelerates maintenance but also cuts expenses associated with paying engineers for unproductive time, presenting a dual benefit of time and cost savings.
Extended machine lifespan
Condition monitoring not only detects anomalies but also significantly extends the lifespan of machinery. Continuous monitoring of parameters, especially those with the potential to cause severe damage, allows for preemptive assessment and repair. By addressing issues before they escalate into downtime events or cause long-term, costly damage, condition monitoring acts as a proactive safeguard for equipment longevity.
Condition-based monitoring approach not only prevents catastrophic failures but also ensures optimal performance over an extended period. Ultimately, the investment in condition monitoring translates into a prolonged equipment lifespan, contributing to overall operational efficiency and cost-effectiveness.
Improved safety
Ensuring workplace safety is paramount, especially in highly automated factories. Depending solely on handheld devices for machine health monitoring exposes workers to avoidable risks. Periodic breakdowns due to maintenance lapses further heighten employee vulnerability to hazardous conditions and potential environmental disasters.
Implementing condition monitoring is a proactive strategy. By anticipating and addressing issues before they lead to failure, it safeguards employee safety and promotes secure work practices. Owners can plan maintenance interventions in advance, minimizing risks to employees working in proximity to the machinery.
In essence, improved safety through condition monitoring not only protects the workforce but also fosters a secure and sustainable operational environment.
Data-driven decision-making
Condition monitoring boosts decision-making based on data from your machinery. You can easily access a constantly updated data repository on machinery performance and health. This wealth of information proves invaluable for formulating and analyzing key performance indicators and making informed choices based on current and historical data. It also helps optimize operating parameters and allocate resources effectively to ensure machines consistently operate at peak performance levels.
Condition-based approach empowers you to optimize operating parameters and maximize efficiency and output while minimizing wear and tear. Also, you can track key performance indicators with granularity, pinpointing areas for improvement and monitoring the impact of adjustments.
Enhanced production efficiency
Condition monitoring can also improve production efficiency by pinpointing areas for improvement. Keeping track of which parts are running poorly allows you to focus efficiency improvement efforts on those specific parts. Thus, you can improve the overall capabilities of your equipment.
Condition monitoring can also help leaders make important decisions, which improves overall production efficiency. Using condition monitoring data as a guide, this strategic approach reduces downtime and increases machinery lifetime value. Thus, you get a balance between efficient resource utilization and sustained product quality.
Machine condition monitoring solution by SIA Connect & Kaa IoT platform
Unplanned downtime due to equipment failure can cripple operations, leading to lost revenue and expensive repairs. SIA Connect and the Kaa IoT platform join forces to deliver a powerful solution that tackles this challenge head-on – a real-time machine condition monitoring system designed to keep your critical equipment running smoothly.
The integrated system seamlessly connects sensors like accelerometers to the SIA Connect gateway , capturing continuous vibration data and other relevant parameters in real-time. This data then seamlessly flows to the secure Kaa Cloud, your centralized hub for comprehensive insights on machinery health and performance. On the Kaa dashboard, operators can define routines for detecting anomalies and get notified.
The condition monitoring solution provides a real-time frequency monitoring system for critical machinery to perform maintenance at the right time and prevent unexpected downtime and machine breakages.
Let's take a look at the use case that will benefit from the machine condition monitoring solution by Kaa and SIA Connect. Consider the critical components of an offshore wind turbine: the generator, transmission, and main bearings. Real-time surveillance of their temperature and vibration can be a game-changer. A slight shift in vibration frequency might indicate shaft misalignment, while a temperature rise in a bearing could signal inadequate lubrication.
If these anomalies are not corrected in time, they can have fatal consequences. A shaft misalignment can cause severe damage to the nacelle of a wind turbine, and if the turbine is located offshore, the time and cost related to the failure are significant.
Benefits of the solution
- Cost efficient edge-to-cloud solution The integrated solution serves as a cost-efficient edge-to-cloud system, effectively utilizing resources while providing comprehensive monitoring capabilities. By optimizing the data transmission process from edge devices to the cloud through the Kaa IoT Platform, operational costs are minimized without compromising on the breadth of monitoring.
- Flexibility to add multiple sensors and communication protocols An inherent strength of the solution lies in its flexibility to accommodate multiple sensors and communication protocols. This adaptability ensures that the monitoring system can be tailored to suit diverse industrial environments and specific machinery requirements. It allows organizations to seamlessly incorporate the latest sensor technologies and communication methods.
- Simplicity in configuring sensor setup as well as notification rules The user-friendly nature of the solution is highlighted by its simplicity in configuring sensor setups and notification rules. This ease of configuration is crucial for operators and maintenance personnel, enabling them to efficiently set up the monitoring system without the need for extensive training. The intuitive interface streamlines the process, enhancing overall usability.
- Scalability in the verticals (number of sensors) and the horizontals (number of sites) Scalability is a key feature that sets the solution apart. It can scale vertically by accommodating varying numbers of sensors, ensuring that organizations can expand or adjust their monitoring infrastructure based on the evolving needs of their machinery. Furthermore, the solution is horizontally scalable, supporting integration across multiple sites. This flexibility allows seamless deployment across diverse industrial landscapes.
Final words
In conclusion, machine condition monitoring is a powerful tool for optimizing your industrial operations. It transcends outdated calendar-based schedules and reactive panic mode, replacing them with informed, data-driven maintenance.
The benefits of machine condition monitoring are undeniable, but implementing it can be daunting. This is where SIA Connect and the Kaa IoT platform come in, offering a seamless, cost-effective solution that makes condition-based monitoring accessible to manufacturers. The given solution provides a real-time frequency monitoring system that prevents costly downtime and breakages.
With KaaIoT and SIA Connect, you can:
- Monitor critical equipment in real-time
- Identify potential issues early
- Schedule maintenance proactively
- Prevent costly breakdowns
- Improve your bottom line
Ready to unlock the power of machine condition monitoring and take your operations to the next level? Get in touch with us to learn more about how KaaIoT can help with your IIoT endeavors.
Feel the benefits of Kaa Cloud on yourself
Related stories.
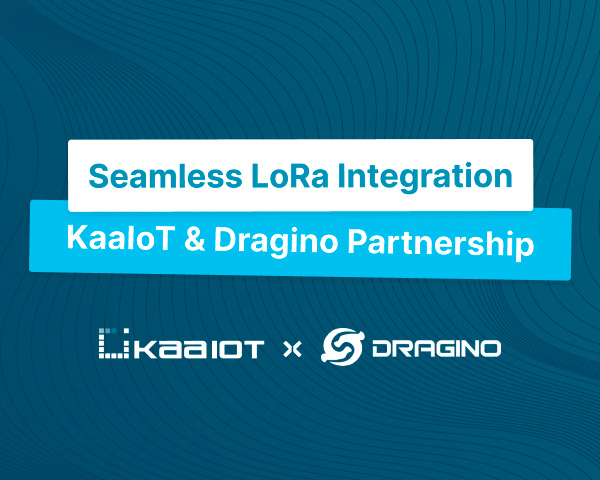
- 04 June 2024
Seamless LoRa Integration: KaaIoT & Dragino Partnership
KaaIoT announces a partnership with Dragino, a hardware provider.
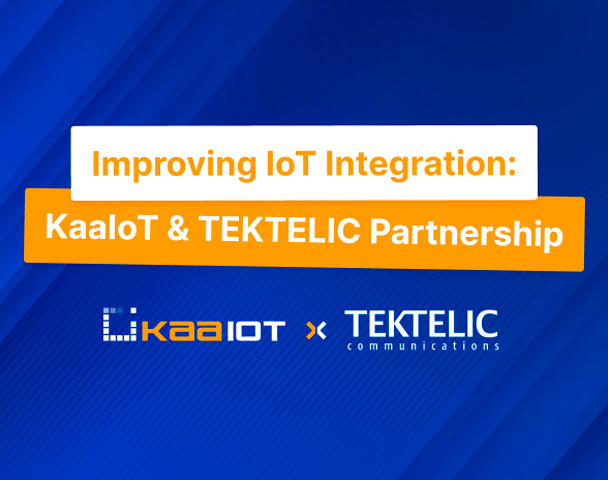
- 16 Apr 2024
Improving IoT Integration: KaaIoT & TEKTELIC Partnership
KaaIoT & TEKTELIC partner to simplify IoT development with pre-integrated hardware & software solutions....
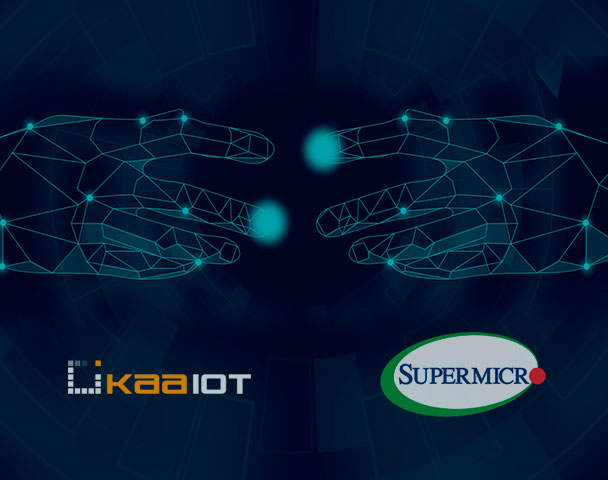
- 02 Apr 2024
KaaIoT & Supermicro Collaborate to Provide AI-Powered IoT Solutions for the Edge
KaaIoT and Supermicro offer a combined solution for AI-powered IoT applications at the network's edge...
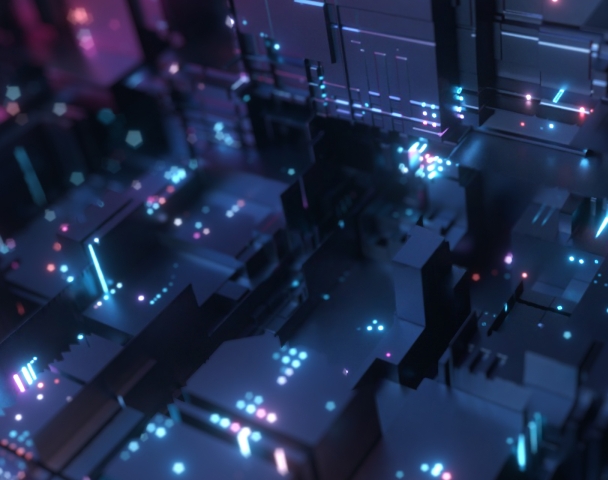
- 28 Jul 2023
Understanding IoT Device Management: Comprehensive Guide
Unlock insights into challenges, best practices & solutions for seamless IoT device management with our in-depth guide.
System Dynamics Modeling for Smartphone-Based Healthcare Tools: Case Study on ECG Monitoring
Ieee account.
- Change Username/Password
- Update Address
Purchase Details
- Payment Options
- Order History
- View Purchased Documents
Profile Information
- Communications Preferences
- Profession and Education
- Technical Interests
- US & Canada: +1 800 678 4333
- Worldwide: +1 732 981 0060
- Contact & Support
- About IEEE Xplore
- Accessibility
- Terms of Use
- Nondiscrimination Policy
- Privacy & Opting Out of Cookies
A not-for-profit organization, IEEE is the world's largest technical professional organization dedicated to advancing technology for the benefit of humanity. © Copyright 2024 IEEE - All rights reserved. Use of this web site signifies your agreement to the terms and conditions.
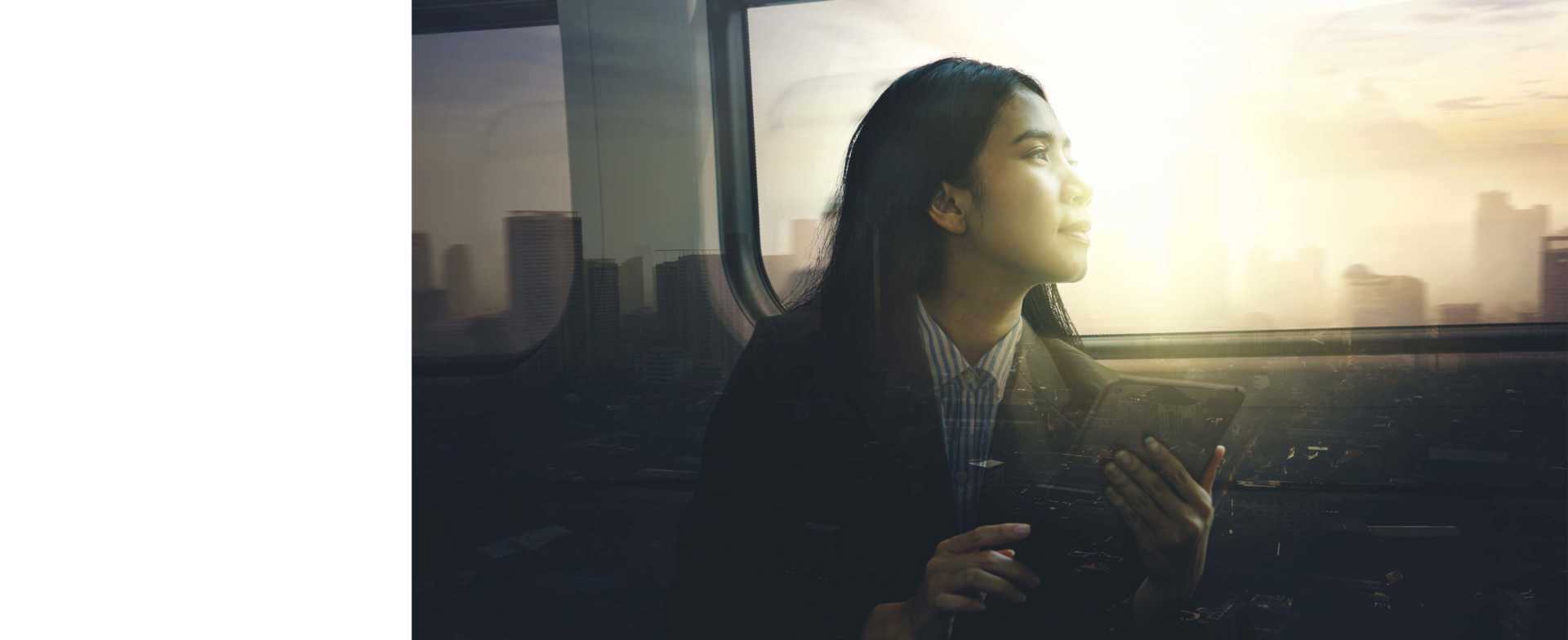
Fraud Detection & Transaction Monitoring Case Studies
Read our payment fraud detection success stories
INETCO case studies
Explore our customer success stories to see how inetco has helped these companies improve payment security, transaction monitoring, and enhance banking experience., transaction monitoring case studies.

How BECU Enhances Member Experience with Real-time ATM Transaction Intelligence
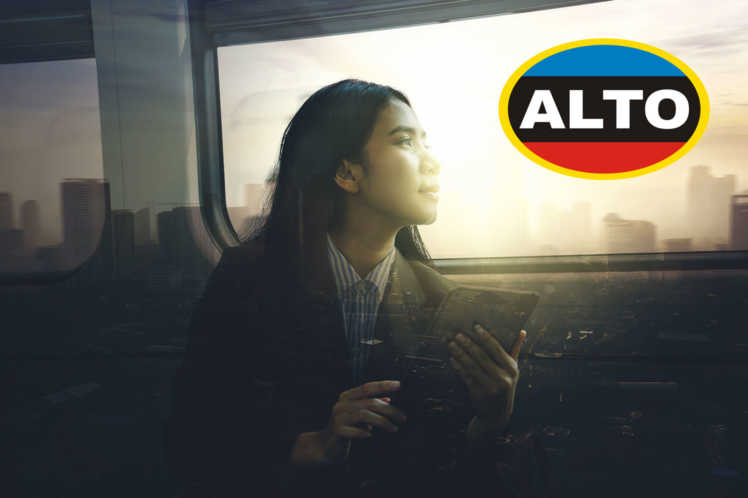
How PT. ALTO Network Provides World-Class Payment Transaction Security
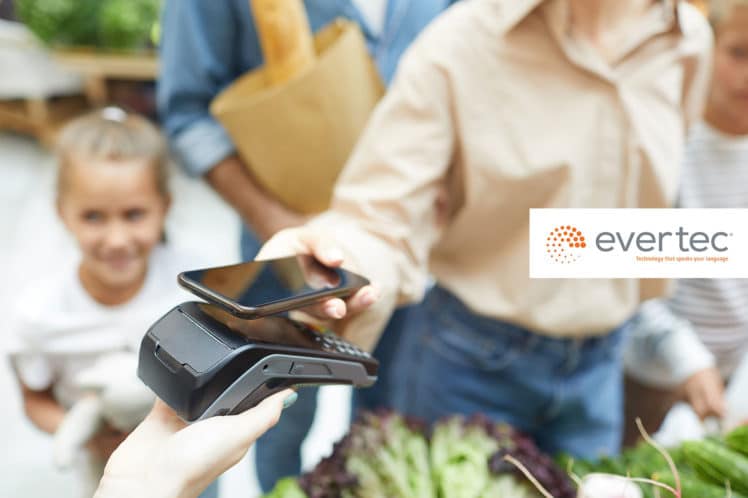
How EVERTEC Costa Rica, S.A. Improves Customer Service Levels and Proactive Problem Resolution with Real-time End-to-End Transaction Monitoring
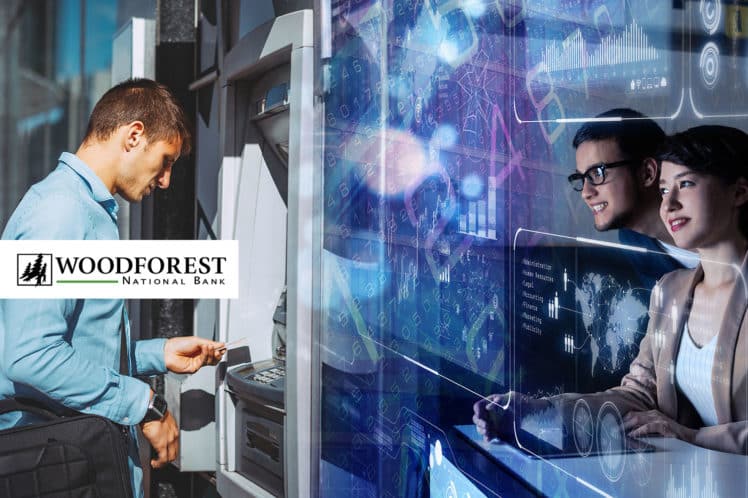
How Woodforest National Bank Improves Customer Experience, ATM Management and Branch Profitability with Real-time Transaction Monitoring and Analytics
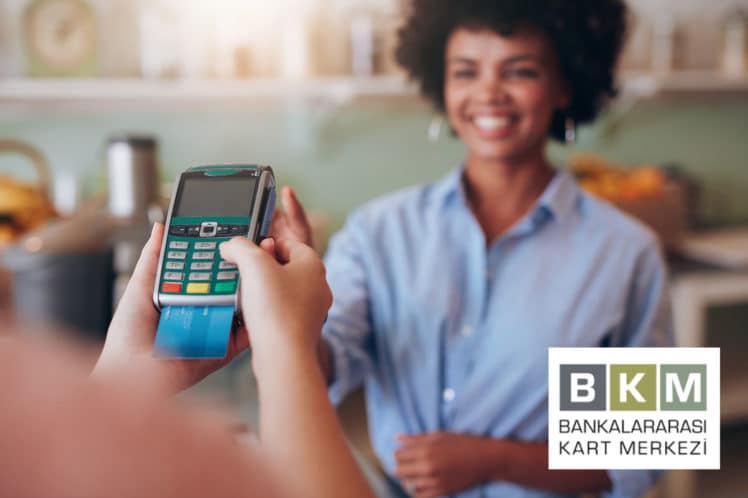
How BKM Improved Service Level Delivery through End-to-End Transaction Visibility
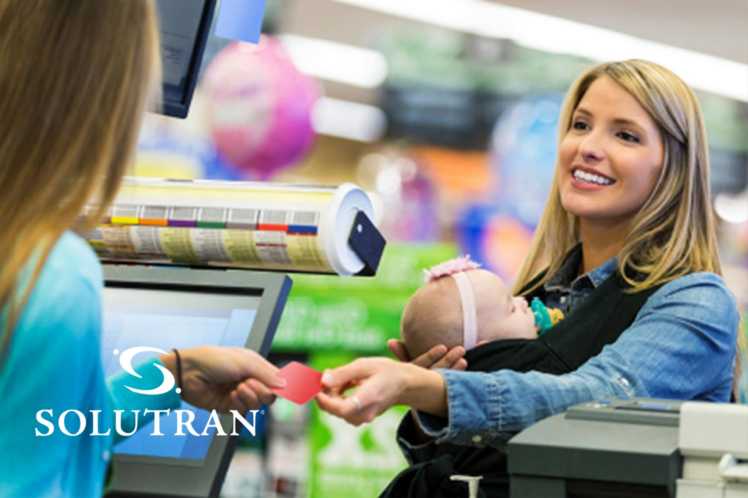
Payment Processing Case Study: How Solutran® Deploys a New Electronic Payment Processing Platform
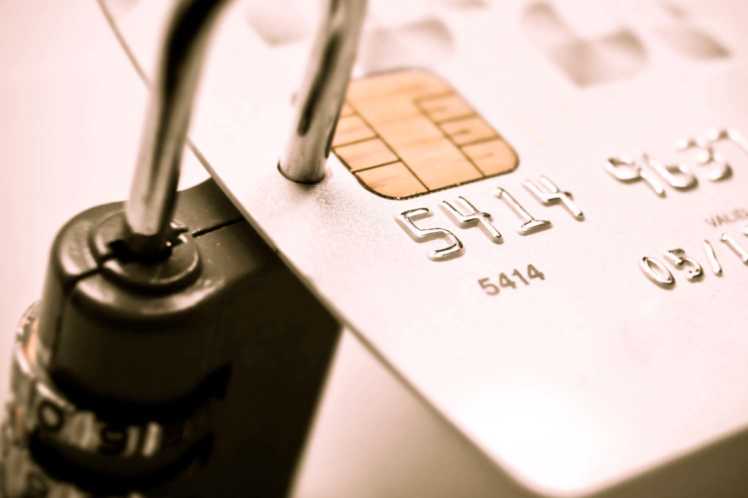
How a Canadian Credit Union Meets Interac® Transaction Monitoring Compliance
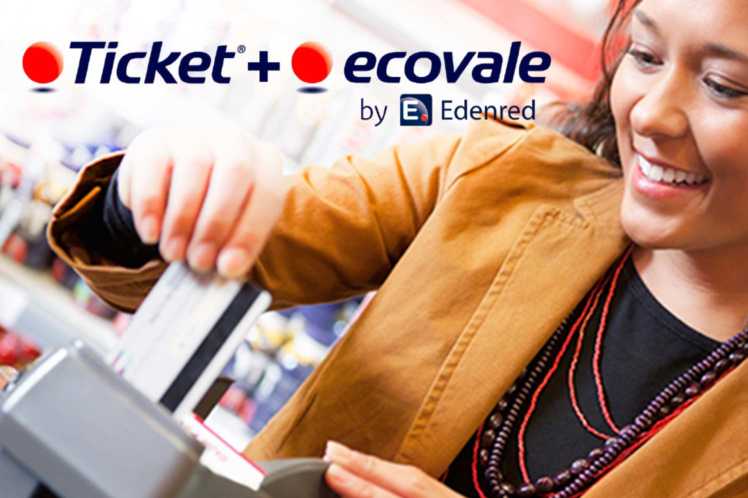
How Edenred México Safely Expands their Prepaid Corporate Service Solutions with Real-time Payments Monitoring
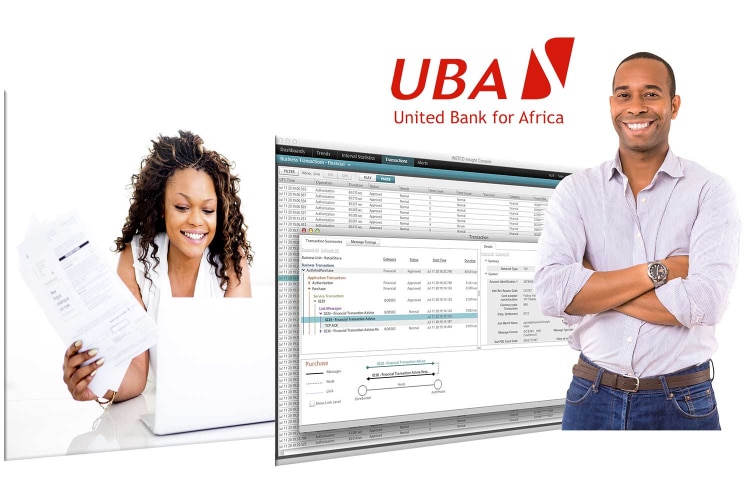
Omnichannel Banking Case Study: How UBA Guarantees Delivery of a Seamless Experience
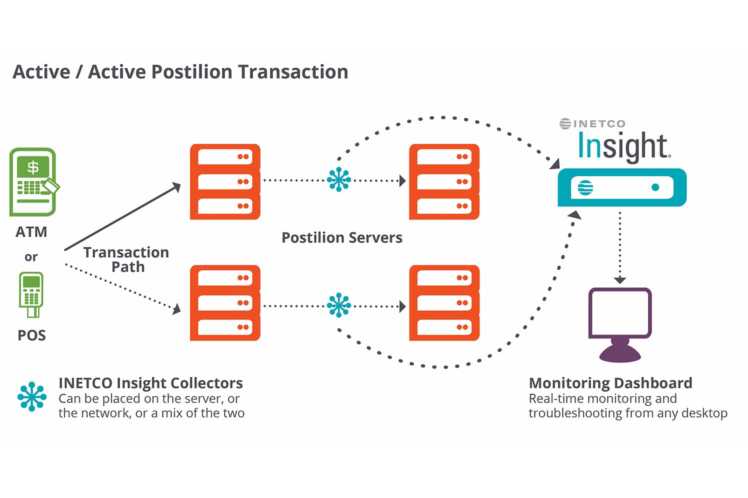
How a Global Card Network Provider Reduced Transaction Time-outs by 75%
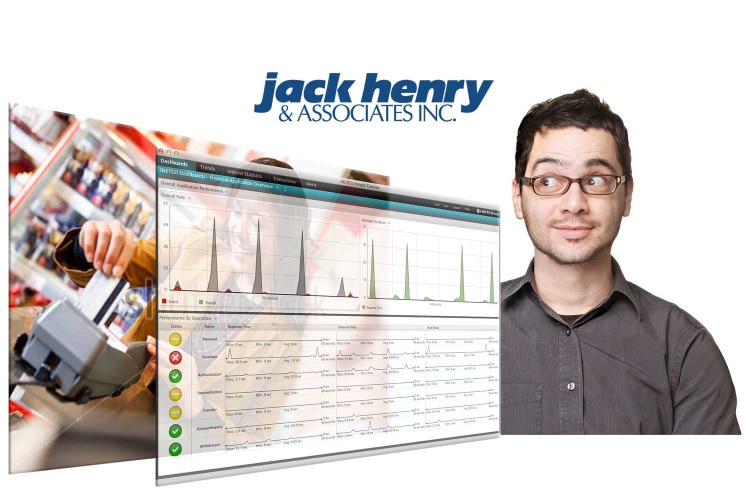
Jack Henry & Associates, Inc.® - Enhancing customer service reliability
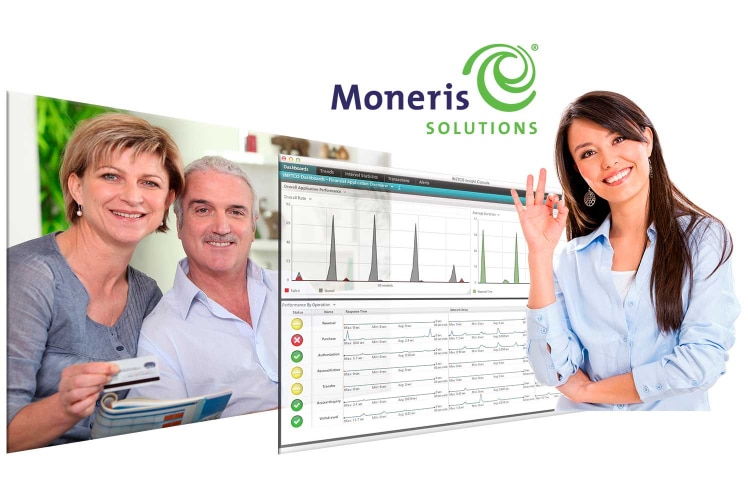
Moneris Solutions – Monitoring payment applications in over 350,000 merchant locations
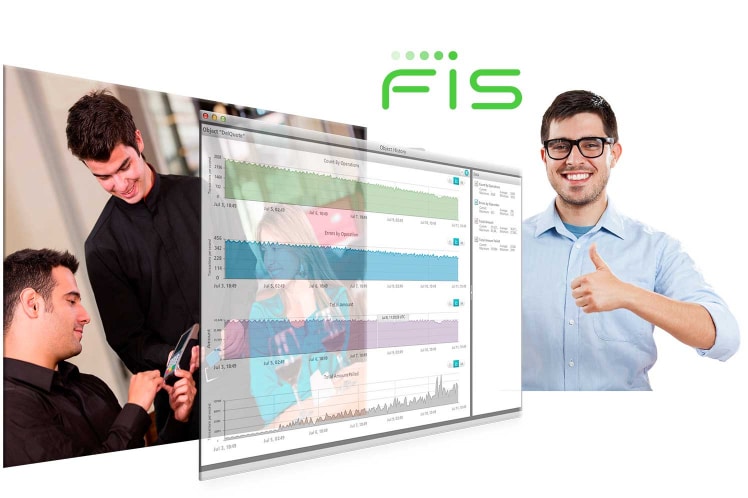
FIS – Resolving transaction performance issues 60% faster
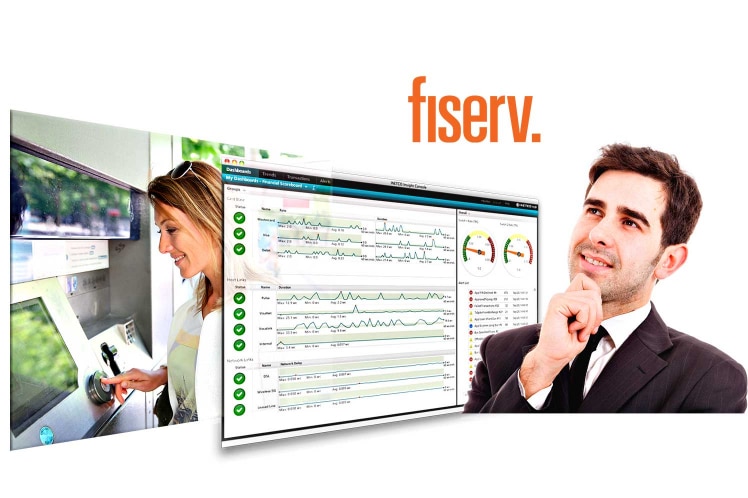
EFT Channels Case Study – Open Solutions Canada (Fiserv)
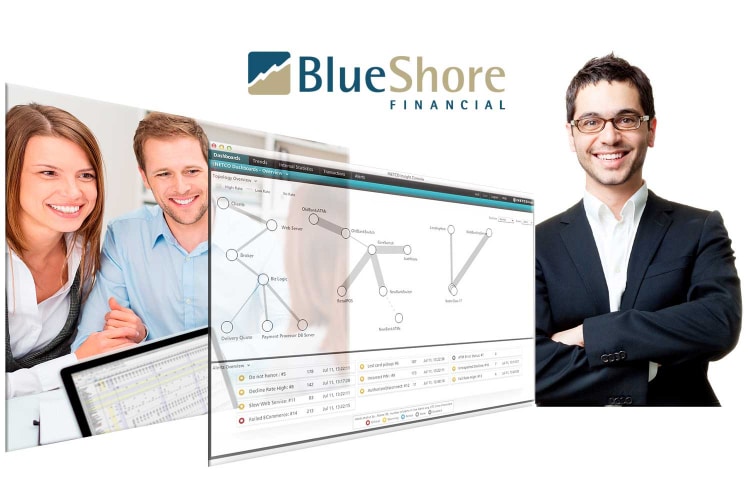
BlueShore Financial – Integrating a new core banking system into a real-time ATM and POS environment
Fraud prevention case studies.
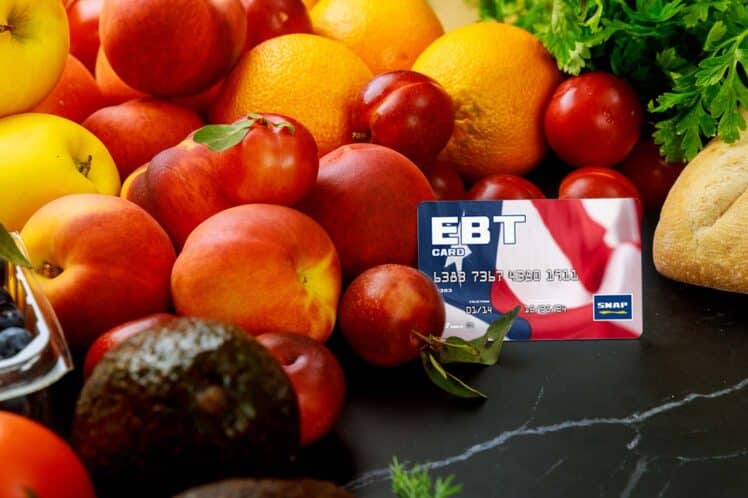
EBT SNAP Fraud: A Growing Multi-Billion Dollar Problem
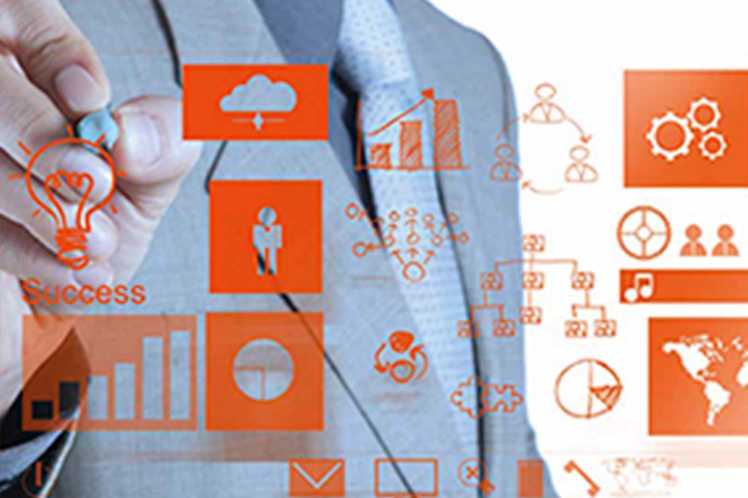
ATM Fraud Detection Case Study: How a Major FI in Africa Improved Early Warning Fraud Detection
Fraud analytics case studies.
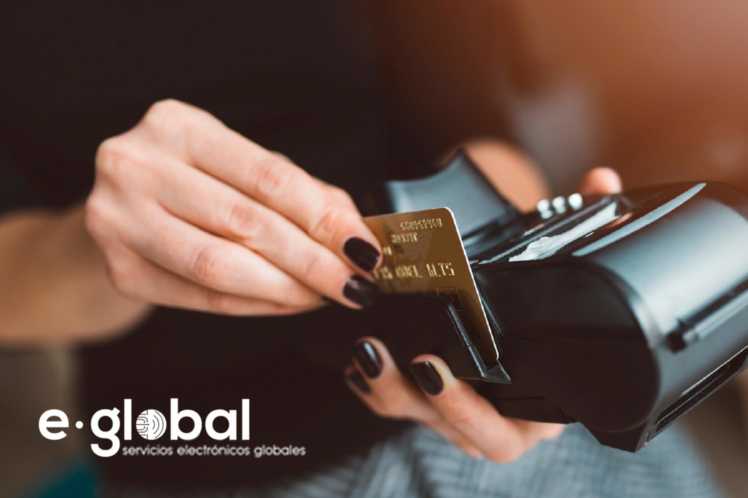
Fraud Analytics Case Study: How E-Global Speeds Up Fraud Analysis
Interested in exploring inetco solutions for your environment.
A risk-based monitoring approach to source data monitoring and documenting monitoring findings
Affiliations.
- 1 Utah Data Coordinating Center, University of Utah, Salt Lake City, UT 84108, USA. Electronic address: [email protected].
- 2 Utah Data Coordinating Center, University of Utah, Salt Lake City, UT 84108, USA.
- PMID: 38810931
- DOI: 10.1016/j.cct.2024.107581
Background: Clinical trial monitoring is evolving from labor-intensive to targeted approaches. The traditional 100% Source Data Monitoring (SDM) approach fails to prioritize data by significance, diverting attention from critical elements. Despite regulatory guidance on Risk-Based Monitoring (RBM), its widespread implementation has been slow.
Methods: Our study teams assess the study's overall risk, document heightened and critical risks, and create a study-specific risk-based monitoring plan, integrating SDM and Central Data Monitoring (CDM). SDM combines a fixed list of pre-identified variables and a list of randomly identified variables to monitor. Identifying variables follows a two-step approach: first, a random sample of participants is selected, second, a random set of variables for each participant selected is identified. Sampling weights prioritize critical variables. Regular team meetings are held to discuss and compile significant findings into a Study Monitoring Report.
Results: We present a random SDM sample and a Study Monitoring Report. The random SDM output includes a look-up table for selected database elements. The report provides a holistic view of the study issues and overall health.
Conclusions: The proposed random sampling method is used to monitor a representative set of critical variables, while the Study Monitoring Report is written to summarize significant monitoring findings and data trends. The report allows the sponsor to assess the current status of the study and data effectively. Communicating and sharing emerging insights facilitates timely adjustments of future monitoring activities, optimizing efficiencies, and study outcomes.
Keywords: Central data monitoring; Risk assessment risk management; Risk-based monitoring; Source data monitoring; Statistical sampling; Study monitoring report.
Copyright © 2024. Published by Elsevier Inc.
The state of AI in early 2024: Gen AI adoption spikes and starts to generate value
If 2023 was the year the world discovered generative AI (gen AI) , 2024 is the year organizations truly began using—and deriving business value from—this new technology. In the latest McKinsey Global Survey on AI, 65 percent of respondents report that their organizations are regularly using gen AI, nearly double the percentage from our previous survey just ten months ago. Respondents’ expectations for gen AI’s impact remain as high as they were last year , with three-quarters predicting that gen AI will lead to significant or disruptive change in their industries in the years ahead.
About the authors
This article is a collaborative effort by Alex Singla , Alexander Sukharevsky , Lareina Yee , and Michael Chui , with Bryce Hall , representing views from QuantumBlack, AI by McKinsey, and McKinsey Digital.
Organizations are already seeing material benefits from gen AI use, reporting both cost decreases and revenue jumps in the business units deploying the technology. The survey also provides insights into the kinds of risks presented by gen AI—most notably, inaccuracy—as well as the emerging practices of top performers to mitigate those challenges and capture value.
AI adoption surges
Interest in generative AI has also brightened the spotlight on a broader set of AI capabilities. For the past six years, AI adoption by respondents’ organizations has hovered at about 50 percent. This year, the survey finds that adoption has jumped to 72 percent (Exhibit 1). And the interest is truly global in scope. Our 2023 survey found that AI adoption did not reach 66 percent in any region; however, this year more than two-thirds of respondents in nearly every region say their organizations are using AI. 1 Organizations based in Central and South America are the exception, with 58 percent of respondents working for organizations based in Central and South America reporting AI adoption. Looking by industry, the biggest increase in adoption can be found in professional services. 2 Includes respondents working for organizations focused on human resources, legal services, management consulting, market research, R&D, tax preparation, and training.
Also, responses suggest that companies are now using AI in more parts of the business. Half of respondents say their organizations have adopted AI in two or more business functions, up from less than a third of respondents in 2023 (Exhibit 2).
Gen AI adoption is most common in the functions where it can create the most value
Most respondents now report that their organizations—and they as individuals—are using gen AI. Sixty-five percent of respondents say their organizations are regularly using gen AI in at least one business function, up from one-third last year. The average organization using gen AI is doing so in two functions, most often in marketing and sales and in product and service development—two functions in which previous research determined that gen AI adoption could generate the most value 3 “ The economic potential of generative AI: The next productivity frontier ,” McKinsey, June 14, 2023. —as well as in IT (Exhibit 3). The biggest increase from 2023 is found in marketing and sales, where reported adoption has more than doubled. Yet across functions, only two use cases, both within marketing and sales, are reported by 15 percent or more of respondents.
Gen AI also is weaving its way into respondents’ personal lives. Compared with 2023, respondents are much more likely to be using gen AI at work and even more likely to be using gen AI both at work and in their personal lives (Exhibit 4). The survey finds upticks in gen AI use across all regions, with the largest increases in Asia–Pacific and Greater China. Respondents at the highest seniority levels, meanwhile, show larger jumps in the use of gen Al tools for work and outside of work compared with their midlevel-management peers. Looking at specific industries, respondents working in energy and materials and in professional services report the largest increase in gen AI use.
Investments in gen AI and analytical AI are beginning to create value
The latest survey also shows how different industries are budgeting for gen AI. Responses suggest that, in many industries, organizations are about equally as likely to be investing more than 5 percent of their digital budgets in gen AI as they are in nongenerative, analytical-AI solutions (Exhibit 5). Yet in most industries, larger shares of respondents report that their organizations spend more than 20 percent on analytical AI than on gen AI. Looking ahead, most respondents—67 percent—expect their organizations to invest more in AI over the next three years.
Where are those investments paying off? For the first time, our latest survey explored the value created by gen AI use by business function. The function in which the largest share of respondents report seeing cost decreases is human resources. Respondents most commonly report meaningful revenue increases (of more than 5 percent) in supply chain and inventory management (Exhibit 6). For analytical AI, respondents most often report seeing cost benefits in service operations—in line with what we found last year —as well as meaningful revenue increases from AI use in marketing and sales.
Inaccuracy: The most recognized and experienced risk of gen AI use
As businesses begin to see the benefits of gen AI, they’re also recognizing the diverse risks associated with the technology. These can range from data management risks such as data privacy, bias, or intellectual property (IP) infringement to model management risks, which tend to focus on inaccurate output or lack of explainability. A third big risk category is security and incorrect use.
Respondents to the latest survey are more likely than they were last year to say their organizations consider inaccuracy and IP infringement to be relevant to their use of gen AI, and about half continue to view cybersecurity as a risk (Exhibit 7).
Conversely, respondents are less likely than they were last year to say their organizations consider workforce and labor displacement to be relevant risks and are not increasing efforts to mitigate them.
In fact, inaccuracy— which can affect use cases across the gen AI value chain , ranging from customer journeys and summarization to coding and creative content—is the only risk that respondents are significantly more likely than last year to say their organizations are actively working to mitigate.
Some organizations have already experienced negative consequences from the use of gen AI, with 44 percent of respondents saying their organizations have experienced at least one consequence (Exhibit 8). Respondents most often report inaccuracy as a risk that has affected their organizations, followed by cybersecurity and explainability.
Our previous research has found that there are several elements of governance that can help in scaling gen AI use responsibly, yet few respondents report having these risk-related practices in place. 4 “ Implementing generative AI with speed and safety ,” McKinsey Quarterly , March 13, 2024. For example, just 18 percent say their organizations have an enterprise-wide council or board with the authority to make decisions involving responsible AI governance, and only one-third say gen AI risk awareness and risk mitigation controls are required skill sets for technical talent.
Bringing gen AI capabilities to bear
The latest survey also sought to understand how, and how quickly, organizations are deploying these new gen AI tools. We have found three archetypes for implementing gen AI solutions : takers use off-the-shelf, publicly available solutions; shapers customize those tools with proprietary data and systems; and makers develop their own foundation models from scratch. 5 “ Technology’s generational moment with generative AI: A CIO and CTO guide ,” McKinsey, July 11, 2023. Across most industries, the survey results suggest that organizations are finding off-the-shelf offerings applicable to their business needs—though many are pursuing opportunities to customize models or even develop their own (Exhibit 9). About half of reported gen AI uses within respondents’ business functions are utilizing off-the-shelf, publicly available models or tools, with little or no customization. Respondents in energy and materials, technology, and media and telecommunications are more likely to report significant customization or tuning of publicly available models or developing their own proprietary models to address specific business needs.
Respondents most often report that their organizations required one to four months from the start of a project to put gen AI into production, though the time it takes varies by business function (Exhibit 10). It also depends upon the approach for acquiring those capabilities. Not surprisingly, reported uses of highly customized or proprietary models are 1.5 times more likely than off-the-shelf, publicly available models to take five months or more to implement.
Gen AI high performers are excelling despite facing challenges
Gen AI is a new technology, and organizations are still early in the journey of pursuing its opportunities and scaling it across functions. So it’s little surprise that only a small subset of respondents (46 out of 876) report that a meaningful share of their organizations’ EBIT can be attributed to their deployment of gen AI. Still, these gen AI leaders are worth examining closely. These, after all, are the early movers, who already attribute more than 10 percent of their organizations’ EBIT to their use of gen AI. Forty-two percent of these high performers say more than 20 percent of their EBIT is attributable to their use of nongenerative, analytical AI, and they span industries and regions—though most are at organizations with less than $1 billion in annual revenue. The AI-related practices at these organizations can offer guidance to those looking to create value from gen AI adoption at their own organizations.
To start, gen AI high performers are using gen AI in more business functions—an average of three functions, while others average two. They, like other organizations, are most likely to use gen AI in marketing and sales and product or service development, but they’re much more likely than others to use gen AI solutions in risk, legal, and compliance; in strategy and corporate finance; and in supply chain and inventory management. They’re more than three times as likely as others to be using gen AI in activities ranging from processing of accounting documents and risk assessment to R&D testing and pricing and promotions. While, overall, about half of reported gen AI applications within business functions are utilizing publicly available models or tools, gen AI high performers are less likely to use those off-the-shelf options than to either implement significantly customized versions of those tools or to develop their own proprietary foundation models.
What else are these high performers doing differently? For one thing, they are paying more attention to gen-AI-related risks. Perhaps because they are further along on their journeys, they are more likely than others to say their organizations have experienced every negative consequence from gen AI we asked about, from cybersecurity and personal privacy to explainability and IP infringement. Given that, they are more likely than others to report that their organizations consider those risks, as well as regulatory compliance, environmental impacts, and political stability, to be relevant to their gen AI use, and they say they take steps to mitigate more risks than others do.
Gen AI high performers are also much more likely to say their organizations follow a set of risk-related best practices (Exhibit 11). For example, they are nearly twice as likely as others to involve the legal function and embed risk reviews early on in the development of gen AI solutions—that is, to “ shift left .” They’re also much more likely than others to employ a wide range of other best practices, from strategy-related practices to those related to scaling.
In addition to experiencing the risks of gen AI adoption, high performers have encountered other challenges that can serve as warnings to others (Exhibit 12). Seventy percent say they have experienced difficulties with data, including defining processes for data governance, developing the ability to quickly integrate data into AI models, and an insufficient amount of training data, highlighting the essential role that data play in capturing value. High performers are also more likely than others to report experiencing challenges with their operating models, such as implementing agile ways of working and effective sprint performance management.
About the research
The online survey was in the field from February 22 to March 5, 2024, and garnered responses from 1,363 participants representing the full range of regions, industries, company sizes, functional specialties, and tenures. Of those respondents, 981 said their organizations had adopted AI in at least one business function, and 878 said their organizations were regularly using gen AI in at least one function. To adjust for differences in response rates, the data are weighted by the contribution of each respondent’s nation to global GDP.
Alex Singla and Alexander Sukharevsky are global coleaders of QuantumBlack, AI by McKinsey, and senior partners in McKinsey’s Chicago and London offices, respectively; Lareina Yee is a senior partner in the Bay Area office, where Michael Chui , a McKinsey Global Institute partner, is a partner; and Bryce Hall is an associate partner in the Washington, DC, office.
They wish to thank Kaitlin Noe, Larry Kanter, Mallika Jhamb, and Shinjini Srivastava for their contributions to this work.
This article was edited by Heather Hanselman, a senior editor in McKinsey’s Atlanta office.
Explore a career with us
Related articles.

Moving past gen AI’s honeymoon phase: Seven hard truths for CIOs to get from pilot to scale
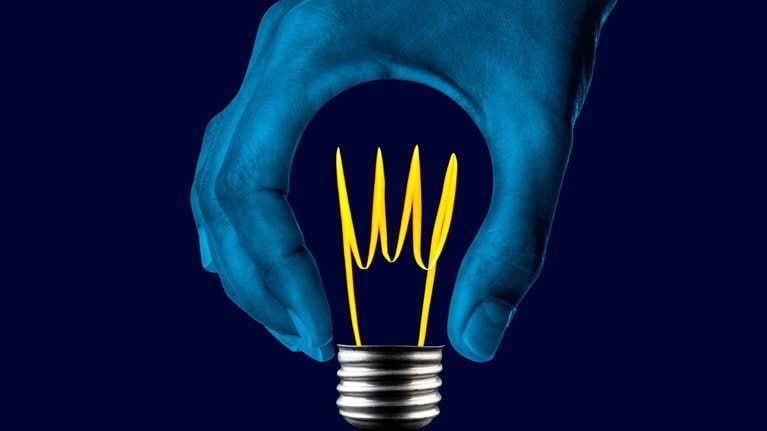
A generative AI reset: Rewiring to turn potential into value in 2024

Implementing generative AI with speed and safety
Temporal and spatial evolution simulation and attribution analysis of vegetation photosynthesis over the past 21 years based on satellite SIF data: a case study from Asia
- Published: 06 June 2024
- Volume 196 , article number 597 , ( 2024 )
Cite this article
- Haixiang Si 1 ,
- Ruiyan Wang 1 , 2 &
- Xiaoteng Li 1
Photosynthesis in vegetation is one of the key processes in maintaining regional ecological balance and climate stability, and it is of significant importance for understanding the health of regional ecosystems and addressing climate change. Based on 2001–2021 Global OCO-2 Solar-Induced Fluorescence (GOSIF) dataset, this study analyzed spatiotemporal variations in Asian vegetation photosynthesis and its response to climate and human activities. Results show the following: (1) From 2001 to 2021, the overall photosynthetic activity of vegetation in the Asian region has shown an upward trend, exhibiting a stable distribution pattern with higher values in the eastern and southern regions and lower values in the central, western, and northern regions. In specific regions such as the Turgen Plateau in northwestern Kazakhstan, Cambodia, Laos, and northeastern Syria, photosynthesis significantly declined. (2) Meteorological factors influencing photosynthesis exhibit differences based on latitude and vertical zones. In low-latitude regions, temperature is the primary driver, while in mid-latitude areas, solar radiation and precipitation are crucial. High-latitude regions are primarily influenced by temperature, and high-altitude areas depend on precipitation and solar radiation. (3) Human activities (56.44%) have a slightly greater impact on the dynamics of Asian vegetation photosynthesis compared to climate change (43.56%). This research deepens our comprehension of the mechanisms behind the fluctuations in Asian vegetation photosynthesis, offering valuable perspectives for initiatives in environmental conservation, sustainability, and climate research.
This is a preview of subscription content, log in via an institution to check access.
Access this article
Price includes VAT (Russian Federation)
Instant access to the full article PDF.
Rent this article via DeepDyve
Institutional subscriptions
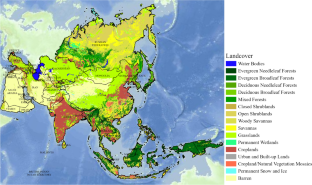
Data availability
No datasets were generated or analysed during the current study.
Andrade, A. M. D., Michel, R. F. M., Bremer, U. F., Schaefer, C. E. G. R., & Simões, J. C. (2018). Relationship between solar radiation and surface distribution of vegetation in Fildes Peninsula and Ardley Island, Maritime Antarctica. International Journal of Remote Sensing, 39 (8), 2238–2254. https://doi.org/10.1080/01431161.2017.1420937
Article Google Scholar
Ascensão, F., Fahrig, L., Clevenger, A. P., Corlett, R. T., Jaeger, J. A. G., Laurance, W. F., & Pereira, H. M. (2018). Environmental challenges for the Belt and Road Initiative. Nature Sustainability, 1 (5), 206–209. https://doi.org/10.1038/s41893-018-0059-3
Bai, X., Zhang, S., Li, C., Xiong, L., Song, F., Du, C., et al. (2023). A carbon-neutrality-capacity index for evaluating carbon sink contributions. Environmental Science and Ecotechnology, 15 , 100237. https://doi.org/10.1016/j.ese.2023.100237
Article CAS Google Scholar
Bashir, B., Cao, C., Naeem, S., ZamaniJoharestani, M., Bo, X., Afzal, H., et al. (2020). Spatio-temporal vegetation dynamic and persistence under climatic and anthropogenic factors. Remote Sensing, 12 (16), 2612. https://doi.org/10.3390/rs12162612
Cooper, M., & Messina, C. D. (2023). Breeding crops for drought-affected environments and improved climate resilience. The Plant Cell, 35 (1), 162–186. https://doi.org/10.1093/plcell/koac321
Dan, S., Li, H., Ping, L., & De, X. (2013). Effects of climate change on vegetation in desert steppe Inner Mongolia. Natural Resources, 04 (04), 319–322. https://doi.org/10.4236/nr.2013.44038
Estoque, R. C., Ooba, M., Avitabile, V., Hijioka, Y., DasGupta, R., Togawa, T., & Murayama, Y. (2019). The future of Southeast Asia’s forests. Nature Communications, 10 (1), 1829. https://doi.org/10.1038/s41467-019-09646-4
Fu, L., Cao, Y., Kuang, S.-Y., & Guo, H. (2021). Index for climate change adaptation in China and its application. Advances in Climate Change Research, 12 (5), 723–733. https://doi.org/10.1016/j.accre.2021.06.006
Ge, W., Deng, L., Wang, F., & Han, J. (2021). Quantifying the contributions of human activities and climate change to vegetation net primary productivity dynamics in China from 2001 to 2016. Science of the Total Environment, 773 , 145648. https://doi.org/10.1016/j.scitotenv.2021.145648
Green, J. K., Konings, A. G., Alemohammad, S. H., Berry, J., Entekhabi, D., Kolassa, J., et al. (2017). Regionally strong feedbacks between the atmosphere and terrestrial biosphere. Nature Geoscience, 10 (6), 410–414. https://doi.org/10.1038/ngeo2957
Hussain, S., Ulhassan, Z., Brestic, M., Zivcak, M., Zhou, W., Allakhverdiev, S. I., et al. (2021). Photosynthesis research under climate change. Photosynthesis Research, 150 (1–3), 5–19. https://doi.org/10.1007/s11120-021-00861-z
Kashyap, R., Kuttippurath, J., & Kumar, P. (2023). Browning of vegetation in efficient carbon sink regions of India during the past two decades is driven by climate change and anthropogenic intrusions. Journal of Environmental Management, 336 , 117655. https://doi.org/10.1016/j.jenvman.2023.117655
Li, X., & Xiao, J. (2019). A global, 0.05-degree product of solar-induced chlorophyll fluorescence derived from OCO-2, MODIS, and reanalysis data. Remote Sensing, 11 (5), 517. https://doi.org/10.3390/rs11050517
Li, A., Wu, J., & Huang, J. (2012). Distinguishing between human-induced and climate-driven vegetation changes: A critical application of RESTREND in inner Mongolia. Landscape Ecology, 27 (7), 969–982. https://doi.org/10.1007/s10980-012-9751-2
Li, P., Qian, H., Howard, K. W. F., & Wu, J. (2015). Building a new and sustainable “Silk Road economic belt.” Environmental Earth Sciences, 74 (10), 7267–7270. https://doi.org/10.1007/s12665-015-4739-2
Li, G., Chen, W., Zhang, X., Bi, P., Yang, Z., Shi, X., & Wang, Z. (2022). Spatiotemporal dynamics of vegetation in China from 1981 to 2100 from the perspective of hydrothermal factor analysis. Environmental Science and Pollution Research, 29 (10), 14219–14230. https://doi.org/10.1007/s11356-021-16664-7
Liu, X., Zhu, Z., Yu, M., & Liu, X. (2021). Drought-induced productivity and economic losses in grasslands from Inner Mongolia vary across vegetation types. Regional Environmental Change, 21 (2), 59. https://doi.org/10.1007/s10113-021-01789-9
Lu, C., Hou, M., Liu, Z., Li, H., & Lu, C. (2021). Variation characteristic of NDVI and its response to climate change in the middle and upper reaches of Yellow River Basin, China. IEEE Journal of Selected Topics in Applied Earth Observations and Remote Sensing, 14 , 8484–8496. https://doi.org/10.1109/JSTARS.2021.3105897
Ma, P., Zhao, J., Zhang, H., Zhang, L., & Luo, T. (2023). Increased precipitation leads to earlier green-up and later senescence in Tibetan alpine grassland regardless of warming. Science of the Total Environment, 871 , 162000. https://doi.org/10.1016/j.scitotenv.2023.162000
Meroni, M., Rossini, M., Picchi, V., Panigada, C., Cogliati, S., Nali, C., & Colombo, R. (2008). Assessing steady-state fluorescence and PRI from hyperspectral proximal sensing as early indicators of plant stress: The case of ozone exposure. Sensors, 8 (3), 1740–1754. https://doi.org/10.3390/s8031740
Misal, H., Hoare, V. H. C., & Miles, V. (2022). Responding to the climate crisis – Taking action on the IPCC 6th Assessment Report . Weather, 77 (4), 149–150. https://doi.org/10.1002/wea.4162
Mohammat, A., Wang, X., Xu, X., Peng, L., Yang, Y., Zhang, X., et al. (2013). Drought and spring cooling induced recent decrease in vegetation growth in Inner Asia. Agricultural and Forest Meteorology, 178–179 , 21–30. https://doi.org/10.1016/j.agrformet.2012.09.014
Mohammed, G. H., Colombo, R., Middleton, E. M., Rascher, U., van der Tol, C., Nedbal, L., et al. (2019). Remote sensing of solar-induced chlorophyll fluorescence (SIF) in vegetation: 50 years of progress. Remote Sensing of Environment, 231 , 111177. https://doi.org/10.1016/j.rse.2019.04.030
Nakamura, Y., Krestov, P. V., & Omelko, A. M. (2007). Bioclimate and zonal vegetation in Northeast Asia: First approximation to an integrated study. Phytocoenologia, 37 (3–4), 443–470. https://doi.org/10.1127/0340-269X/2007/0037-0443
Nanzad, L., Zhang, J., Tuvdendorj, B., Yang, S., Rinzin, S., Prodhan, F. A., & Sharma, T. P. P. (2021). Assessment of drought impact on net primary productivity in the terrestrial ecosystems of Mongolia from 2003 to 2018. Remote Sensing, 13 (13), 2522. https://doi.org/10.3390/rs13132522
Paul-Limoges, E., Damm, A., Hueni, A., Liebisch, F., Eugster, W., Schaepman, M. E., & Buchmann, N. (2018). Effect of environmental conditions on sun-induced fluorescence in a mixed forest and a cropland. Remote Sensing of Environment, 219 , 310–323. https://doi.org/10.1016/j.rse.2018.10.018
Piao, S., Cui, M., Chen, A., Wang, X., Ciais, P., Liu, J., & Tang, Y. (2011). Altitude and temperature dependence of change in the spring vegetation green-up date from 1982 to 2006 in the Qinghai-Xizang Plateau. Agricultural and Forest Meteorology, 151 (12), 1599–1608. https://doi.org/10.1016/j.agrformet.2011.06.016
Piao, S., Yue, C., Ding, J., & Guo, Z. (2022). Perspectives on the role of terrestrial ecosystems in the ‘carbon neutrality’ strategy. Science China Earth Sciences, 65 (6), 1178–1186. https://doi.org/10.1007/s11430-022-9926-6
Porcar-Castell, A., Tyystjärvi, E., Atherton, J., van der Tol, C., Flexas, J., Pfündel, E. E., et al. (2014). Linking chlorophyll a fluorescence to photosynthesis for remote sensing applications: Mechanisms and challenges. Journal of Experimental Botany, 65 (15), 4065–4095. https://doi.org/10.1093/jxb/eru191
Reichstein, M., Ciais, P., Papale, D., Valentini, R., Running, S., Viovy, N., et al. (2007). Reduction of ecosystem productivity and respiration during the European summer 2003 climate anomaly: A joint flux tower, remote sensing and modelling analysis. Global Change Biology, 13 (3), 634–651. https://doi.org/10.1111/j.1365-2486.2006.01224.x
Seddon, A. W. R., Macias-Fauria, M., Long, P. R., Benz, D., & Willis, K. J. (2016). Sensitivity of global terrestrial ecosystems to climate variability. Nature, 531 (7593), 229–232. https://doi.org/10.1038/nature16986
Song, L., Guanter, L., Guan, K., You, L., Huete, A., Ju, W., & Zhang, Y. (2018). Satellite sun-induced chlorophyll fluorescence detects early response of winter wheat to heat stress in the Indian Indo-Gangetic Plains. Global Change Biology, 24 (9), 4023–4037. https://doi.org/10.1111/gcb.14302
Srivastava, S., Mehta, L., & Naess, L. O. (2022). Increased attention to water is key to adaptation. Nature Climate Change, 12 (2), 113–114. https://doi.org/10.1038/s41558-022-01277-w
Stavi, I. (2023). Urgent reduction in greenhouse gas emissions is needed to avoid irreversible tipping points: Time is running out. All Earth, 35 (1), 38–45. https://doi.org/10.1080/27669645.2023.2178127
Su, Y., Yang, X., Gentine, P., Maignan, F., Shang, J., & Ciais, P. (2022). Observed strong atmospheric water constraints on forest photosynthesis using eddy covariance and satellite-based data across the Northern Hemisphere. International Journal of Applied Earth Observation and Geoinformation, 110 , 102808. https://doi.org/10.1016/j.jag.2022.102808
Tewari, V. P., Verma, R. K., & Von Gadow, K. (2017). Climate change effects in the Western Himalayan ecosystems of India: Evidence and strategies. Forest Ecosystems, 4 (1), 13. https://doi.org/10.1186/s40663-017-0100-4
Umuhoza, J., Jiapaer, G., Tao, Y., Jiang, L., Zhang, L., Gasirabo, A., et al. (2023). Analysis of fluctuations in vegetation dynamic over Africa using satellite data of solar-induced chlorophyll fluorescence. Ecological Indicators, 146 , 109846. https://doi.org/10.1016/j.ecolind.2022.109846
van Leeuwen, W. J. D., Orr, B. J., Marsh, S. E., & Herrmann, S. M. (2006). Multi-sensor NDVI data continuity: Uncertainties and implications for vegetation monitoring applications. Remote Sensing of Environment, 100 (1), 67–81. https://doi.org/10.1016/j.rse.2005.10.002
Wang, X., Wang, T., Xu, J., Shen, Z., Yang, Y., Chen, A., et al. (2022). Enhanced habitat loss of the Himalayan endemic flora driven by warming-forced upslope tree expansion. Nature Ecology & Evolution, 6 (7), 890–899. https://doi.org/10.1038/s41559-022-01774-3
Wang, Y., Liu, J., Wennberg, P. O., He, L., Bonal, D., Köhler, P., et al. (2023). Elucidating climatic drivers of photosynthesis by tropical forests. Global Change Biology, 29 (17), 4811–4825. https://doi.org/10.1111/gcb.16837
Wei, Z. F., Huang, Q. Y., & Zhang, R. (2019). Dynamics of vegetation coverage and response to climate change in China-South Asia-Southeast Asia during 1982–2013. Applied Ecology and Environmental Research, 17 (2), 2865–2879. https://doi.org/10.15666/aeer/1702_28652879
Wu, K., Chen, J., Yang, H., Yang, Y., & Hu, Z. (2023). Spatiotemporal variations in the sensitivity of vegetation growth to typical climate factors on the Qinghai-Tibet Plateau. Remote Sens, 15 , 2355. https://doi.org/10.3390/rs15092355
Zhang, Y., Gentine, P., Luo, X., Lian, X., Liu, Y., Zhou, S., et al. (2022). Increasing sensitivity of dryland vegetation greenness to precipitation due to rising atmospheric CO2. Nature Communications, 13 (1), 4875. https://doi.org/10.1038/s41467-022-32631-3
Zhang, R., Zhou, Y., Hu, T., Sun, W., Zhang, S., Wu, J., & Wang, H. (2023). Detecting the spatiotemporal variation of vegetation phenology in northeastern China based on MODIS NDVI and solar-induced chlorophyll fluorescence dataset. Sustainability, 15 (7), 6012. https://doi.org/10.3390/su15076012
Zhong, R., Wang, P., Mao, G., Chen, A., & Liu, J. (2021). Spatiotemporal variation of enhanced vegetation index in the Amazon Basin and its response to climate change. Physics and Chemistry of the Earth, Parts A/B/C, 123 , 103024. https://doi.org/10.1016/j.pce.2021.103024
Zhou, L., Tian, Y., Myneni, R. B., Ciais, P., Saatchi, S., Liu, Y. Y., et al. (2014). Widespread decline of Congo rainforest greenness in the past decade. Nature, 509 (7498), 86–90. https://doi.org/10.1038/nature13265
Zhu, L., Sun, S., Li, Y., Liu, X., & Hu, K. (2023). Effects of climate change and anthropogenic activity on the vegetation greening in the Liaohe River Basin of northeastern China. Ecological Indicators, 148 , 110105. https://doi.org/10.1016/j.ecolind.2023.110105
Download references
This research was funded by the Shandong Province Graduate Education Quality Improvement Plan (SDYAL21044) and the Funds of the Natural Science Foundation of Shandong Province (ZR2020MD003).
Author information
Authors and affiliations.
College of Resources and Environment, Shandong Agricultural University, Tai’an, 271018, China
Haixiang Si, Ruiyan Wang & Xiaoteng Li
National Engineering Research Center for Efficient Utilization of Soil and Fertilizer Resources, Shandong Agricultural University, Tai’an, 271018, China
Ruiyan Wang
You can also search for this author in PubMed Google Scholar
Contributions
Haixiang Si: conceptualization, methodology, validation, writing—original draft, writing—review and editing, formal analysis, visualization.
Ruiyan Wang: conceptualization, methodology, writing—original draft, formal analysis, visualization, writing—review and editing, supervision, project administration, funding acquisition.
Xiaoteng Li: writing—original draft, writing—review and editing, formal analysis, visualization.
All authors have read and agreed to the published version of the manuscript.
Corresponding author
Correspondence to Ruiyan Wang .
Ethics declarations
Ethical approval.
Not applicable, as this work did not involve humans or animals in any way, but only atmospheric dust.
Competing interests
The authors declare no competing interests.
Additional information
Publisher's note.
Springer Nature remains neutral with regard to jurisdictional claims in published maps and institutional affiliations.
Rights and permissions
Springer Nature or its licensor (e.g. a society or other partner) holds exclusive rights to this article under a publishing agreement with the author(s) or other rightsholder(s); author self-archiving of the accepted manuscript version of this article is solely governed by the terms of such publishing agreement and applicable law.
Reprints and permissions
About this article
Si, H., Wang, R. & Li, X. Temporal and spatial evolution simulation and attribution analysis of vegetation photosynthesis over the past 21 years based on satellite SIF data: a case study from Asia. Environ Monit Assess 196 , 597 (2024). https://doi.org/10.1007/s10661-024-12755-3
Download citation
Received : 29 January 2024
Accepted : 25 May 2024
Published : 06 June 2024
DOI : https://doi.org/10.1007/s10661-024-12755-3
Share this article
Anyone you share the following link with will be able to read this content:
Sorry, a shareable link is not currently available for this article.
Provided by the Springer Nature SharedIt content-sharing initiative
- Vegetation photosynthesis
- Solar-induced chlorophyll fluorescence
- Spatiotemporal variations
- Climate change
- Find a journal
- Publish with us
- Track your research

An official website of the United States government
Here’s how you know
Official websites use .gov A .gov website belongs to an official government organization in the United States.
Secure .gov websites use HTTPS A lock ( Lock A locked padlock ) or https:// means you’ve safely connected to the .gov website. Share sensitive information only on official, secure websites.
https://www.nist.gov/publications/chemical-contamination-and-bivalve-health-milwaukee-estuary-integrated-assessment-case
Chemical Contamination and Bivalve Health in the Milwaukee Estuary: An Integrated Assessment Case Study (2017 - 2018)
Download paper, additional citation formats.
- Google Scholar
If you have any questions about this publication or are having problems accessing it, please contact [email protected] .

IMAGES
VIDEO
COMMENTS
Two high-quality studies focused on hypertension. 15,23 Kim et al. examined 374 patients randomized to (1) home blood pressure monitoring, (2) remote monitoring using a wireless blood pressure ...
This book presents a disciplined, qualitative exploration of case study methods by drawing from naturalistic, holistic, ethnographic, phenomenological and biographic research methods.
Using Case Studies. to doProgram. Evaluation. valuation of any kind is designed to document what happened in a program. Evaluation should show: 1) what actually occurred, 2) whether it had an impact, expected or unexpected, and 3) what links exist between a program and its observed impacts.
In April 2020, global demand for employee monitoring software more than doubled.Online searches for "how to monitor employees working from home" increased by 1,705%, and sales for systems that ...
Progress monitoring, a type of formative assessment (i.e., frequent evaluation), is often used to evaluate student learning. Though there are a number of methods for monitoring a student's progress, the most widely used is curriculum-based measurement (CBM), the type that will be discussed in this case study set. Progress monitoring:
A case study focuses on a particular unit - a person, a site, a project. It often uses a combination of quantitative and qualitative data. ... Evaluation Initiative, a global network of organizations and experts supporting country governments to strengthen monitoring, evaluation, and the use of evidence in their countries. The GEI focuses ...
Operational Implementation of Remote Patient Monitoring Within a Large Ambulatory Health System: Multimethod Qualitative Case Study ... Testa PA. Scaling virtual health at the epicentre of coronavirus disease 2019: a case study from NYU Langone Health. J Telemed Telecare. 2022; 28 (3):224-229. doi: 10.1177/1357633X20941395. https://journals ...
What is a case study? There are many different text books and websites explaining the use of case studies and this section draws heavily on those of Lamar University and the NCBI (worked examples), as well as on the author's own extensive research experience.. If you are monitoring/ evaluating a project, you may already have obtained general information about your target school, village ...
Daily Updates of the Latest Projects & Documents. This paper comprises a collection of case studies on the design and implementation of monitoring and ongoing evaluation systems in rural development projects. The case .
Case Study: Remote Patient Monitoring. One top-three integrated delivery network (IDN) was facing a challenge: it was unable to manage the growing number of patients with chronic conditions. Past remote patient monitoring (RPM) programs lived outside the EHR in onerous web-based dashboards because integrating data into flowsheets and existing ...
Summary. Southern California's air pollution endangers citizen health. Government CIOs can learn how South Coast AQMD improved the quality of life for 17 million citizens with an app and open data portal that provide real-time air quality information based on AI predictive modeling of IoT data.
The case studies in this chapter describe experiences from countries in monitoring and evaluation of national adolescent health plans and strategies, and youth involvement in such efforts. Process evaluation of PLAN-A intervention (Peer-Led physical Activity iNtervention for Adolescent girls) in the United Kingdom
Since the publication of the Government-Wide Monitoring and Evaluation Policy Framework (GWM&EPF) by the Presidency in South Africa ... a linear-snowball sampling technique was considered appropriate for this purpose (Babbie, 2002). By using a case study, the researchers took a broad over-view of the emergence of M&E as a „movement" to ...
We adapt the Yin's case study methodology to investigate the challenges we faced in the design, implementation and deployment of the multi-source mobile analytic RPM system, M4CVD (Mobile Machine Learning Model for Monitoring Cardiovascular Disease) . Four classes of challenges for developing a mobile monitoring system are investigated: data ...
In a case study, the minimum redundancy maximum relevance (mRMR) method was proposed and tested to use for tool condition monitoring (Fernandes et al., 2019). The algorithm reduced the feature space from 47 to 32 by ranking the features by their relevance to the objective and penalizing those that are redundant.
Remote monitoring and maintenance are important for improving the performance of production systems. However, existing studies on this topic usually focus on the monitoring and maintenance of the working conditions of the equipment and pay relatively less attention to the processing craft and processing quality. In addition, as far as we know, there are relatively few industrial case studies ...
Initiatives by The Joint Commission 1 and Department of Health & Human Services 2 have brought increased attention to the topic of monitoring of patients on opioids and galvanized hospitals, including Johns Hopkins Hospital, to pursue continuous vital sign monitoring programs. The hospital's philosophy is that "failure-to-rescue" events (i.e., when a patient dies from a medical ...
Remote monitoring is a great use case for leaders with industrial assets out in the field, such as machine builders. With IoT-connected assets and IoT sensors on an industrial internet, you can monitor equipment usage and health in order to assess performance and deploy service should there be any problems. ... Case Study: Fastenal Uses Real ...
Predictive monitoring. The high school in this case study aims to predict the end-of-year grades of its students. This enables the school to receive early warnings on exceptional students. In this section, we will thus consider predictive monitoring of student performance. 4.2.1. Multilevel predictive monitoring.
Machine Condition Monitoring: A Detailed Guide & Case Study. Tech. / Feb 12, 2024. An average large plant loses 25 hours a month to unplanned downtime, according to The True Cost of Downtime report by Siemens. It's more than a full day's production. Machine breakdowns cost billions in lost revenue and repairs for manufacturers.
A SD model for smartphone-based healthcare monitoring is introduced. Specifically, smartphone-based heart monitoring using electrocardiogram (ECG) is studied in this article from a SD point of view. The model includes factors such as patient wellbeing and care, cost, convenience, user friendliness, in addition to other embedded ECG system ...
Transaction monitoring case studies. Case Study. How BECU Enhances Member Experience with Real-time ATM Transaction Intelligence. Read more. Case Study. How PT. ALTO Network Provides World-Class Payment Transaction Security.
Digital trace data presents an opportunity for promptly monitoring shifts in migrant populations. This contribution aims to determine whether the number of European migrants in the United Kingdom (UK) declined between March 2019 and March 2020, using weekly estimates derived from the Facebook Advertising Platform.
PDF | On May 1, 2006, Palena Neale and others published Monitoring and Evaluation-1 PREPARING A CASE STUDY: A Guide for Designing and Conducting a Case Study for Evaluation Input Monitoring and ...
Therefore, frequent monitoring of liver enzymes should be conducted to minimize the risk of severe PIPC/TAZ-induced abnormal liver enzyme levels in patients with low hepatic functional reserve. ... Minagawa K, Hayakawa T, Takahashi Y, Asai S. Signal detection of potential hepatotoxic drugs: case-control study using both a spontaneous reporting ...
Results: We present a random SDM sample and a Study Monitoring Report. The random SDM output includes a look-up table for selected database elements. The report provides a holistic view of the study issues and overall health. Conclusions: The proposed random sampling method is used to monitor a representative set of critical variables, while ...
If 2023 was the year the world discovered generative AI (gen AI), 2024 is the year organizations truly began using—and deriving business value from—this new technology.In the latest McKinsey Global Survey on AI, 65 percent of respondents report that their organizations are regularly using gen AI, nearly double the percentage from our previous survey just ten months ago.
Study area. Asia is a region located in the eastern part of the Eurasian continent, and it is the largest and most populous continent in the world (Fig. 1).The majority of Asia's landmass is situated in the eastern and northern hemispheres, bordered by the Pacific Ocean to the east, the Arctic Ocean to the north, and the Indian Ocean to the south.
Case Study: Shaking Table Experiment The proposed SG-EnMCMC method has been successfully implemented in a shaking table experiment conducted on a 3-storey reinforced concrete structure. The prototype of the test specimen featured a frame in the transverse direction (frame direction) and a frame-wall interacting system in the longitudinal ...
Chemical Contamination and Bivalve Health in the Milwaukee Estuary: An Integrated Assessment Case Study (2017 - 2018) Published. June 3, 2024. Author(s) Pawel Jaruga, Neil Fuller, Kimani Kimbrough, Michael Edwards, Eric Davenport, Amy Ringwood, Ed Johnson. Abstract The Milwaukee Estuary is a highly urbanized and industrialized watershed that ...