- Subject List
- Take a Tour
- For Authors
- Subscriber Services
- Publications
- African American Studies
- African Studies
- American Literature
- Anthropology
- Architecture Planning and Preservation
- Art History
- Atlantic History
- Biblical Studies
- British and Irish Literature
- Childhood Studies
- Chinese Studies
- Cinema and Media Studies
- Communication
- Criminology
- Environmental Science
- Evolutionary Biology
- International Law
- International Relations
- Islamic Studies
- Jewish Studies
- Latin American Studies
- Latino Studies
- Linguistics
- Literary and Critical Theory
- Medieval Studies
- Military History
- Political Science
- Public Health
- Renaissance and Reformation
- Social Work
- Urban Studies
- Victorian Literature
- Browse All Subjects

How to Subscribe
- Free Trials
In This Article Expand or collapse the "in this article" section Training and Development
Introduction, general overviews.
- Reference Works
- Instructional Systems Design
- Needs Assessment
- Training Methods
- Pre-training Interventions
- Training Media
- Training Teams
- Training Evaluation
- Learner Characteristics
- Learning Context
- Employee Development
- Macroperspectives
Related Articles Expand or collapse the "related articles" section about
About related articles close popup.
Lorem Ipsum Sit Dolor Amet
Vestibulum ante ipsum primis in faucibus orci luctus et ultrices posuere cubilia Curae; Aliquam ligula odio, euismod ut aliquam et, vestibulum nec risus. Nulla viverra, arcu et iaculis consequat, justo diam ornare tellus, semper ultrices tellus nunc eu tellus.
- Alternative Work Arrangements
- Career Studies
- Career Transitions and Job Mobility
- Global Human Resources
- Goal Setting
- Human Resource Management
- Organization Culture
Other Subject Areas
Forthcoming articles expand or collapse the "forthcoming articles" section.
- Cooperation-Competition (Coopetition)
- Diversity and Firm Performance
- Organization Design
- Find more forthcoming articles...
- Export Citations
- Share This Facebook LinkedIn Twitter
Training and Development by Kenneth G. Brown LAST REVIEWED: 26 October 2015 LAST MODIFIED: 26 October 2015 DOI: 10.1093/obo/9780199846740-0013
Training and development is the study of how structured experiences help employees gain work-related knowledge, skill, and attitudes. It is like many other topics in management in that it is inherently multidisciplinary in nature. At its core is the psychological study of learning and transfer. A variety of disciplines offer insights into this topic, including, but not limited to, industrial and organizational psychology, educational psychology, human resource development, organizational development, industrial and labor relations, strategic management, and labor economics. The focus of this bibliography is primarily psychological with an emphasis on theory and practice that examines training processes and the learning outcomes they seek to influence. Nevertheless, literature from other perspectives will be introduced on a variety of topics within this area of study.
These articles and chapters provide background for the study of training and development, particularly as studied by management scholars with backgrounds in human resource management, organizational behavior, human resource development, and industrial and organizational psychology. Kraiger 2003 examines training from three different perspectives. Aguinis and Kraiger 2009 provides a narrative review of ten years of research on training and employee development, focusing on the many benefits of providing structured learning experiences to employees. Brown and Sitzmann 2011 also reviews the literature and emphasizes research on the processes that are required to ensure that training benefits emerge. Arthur, et al. 2003 meta-analyzes the literature on training effectiveness. Russ-Eft 2002 proposes a typology of training designs. Salas, et al. 2012 offers recommendations for evidence-based training practice. Noe, et al 2014 examines training in a broader context, relative to the roles of informal learning and knowledge transfer.
Aguinis, Herman, and Kurt Kraiger. “Benefits of Training and Development for Individuals and Teams, Organizations, and Society.” Annual Review of Psychology 60.1 (January 2009): 451–474.
DOI: 10.1146/annurev.psych.60.110707.163505
A comprehensive review of training and development literature from 1999 to 2009 with an emphasis on the benefits that training offers across multiple levels of analysis.
Arthur, Winfred A., Jr., Winston Bennett Jr., Pamela S. Edens, and Suzanne T. Bell. “Effectiveness of Training in Organizations: A Meta-analysis of Design and Evaluation Features.” Journal of Applied Psychology 88.2 (April 2003): 234–245.
DOI: 10.1037/0021-9010.88.2.234
Offers a comprehensive meta-analysis of the relationships among training design and evaluation features and various training effectiveness outcomes (reaction, learning, behavior, and results).
Brown, Kenneth G., and Traci Sitzmann. “Training and Employee Development for Improved Performance.” In APA Handbook of Industrial and Organizational Psychology . Vol. 2, Selecting and Developing Members for the Organization . Edited by Sheldon Zedeck, 469–503. Washington, DC: American Psychological Association, 2011.
DOI: 10.1037/12170-000
A comprehensive review of training and development in work organizations with an emphasis on the processes necessary for training to be effective for improving individual and team performance.
Kraiger, Kurt. “Perspectives on Training and Development.” In Handbook of Psychology . Vol. 12. Edited by Irving B. Weiner and Walter C. Borman, Daniel R. Ilgen, and Richard J. KIlimoski, 171–192. Hoboken, NJ: John Wiley, 2003.
DOI: 10.1002/0471264385
Reviews training literature from three perspectives: instruction, learning, and organizational change.
Noe, Raymond A., Alena D. M. Clarke, and Howard J. Klein. “Learning in the Twenty-first-century Workplace.” Annual Review of Organizational Psychology and Organizational Behavior 1 (2014): 245–275.
DOI: 10.1146/annurev-orgpsych-031413-091321
A review that places training and development in a broader context with other learning-related interventions and practices such as informal learning and knowledge sharing. The chapter explains factors that facilitate learning in organizations.
Russ-Eft, Darlene. “A Typology of Training Design and Work Environment Factors Affecting Workplace Learning and Transfer.” Human Resource Development Review 1 (March 2002): 45–65.
DOI: 10.1177/1534484302011003
Presents a typology summarizing elements of training and work environments that foster transfer of training.
Salas, Eduardo, Scott I. Tannenbaum, Kurt Kraiger, and Kimberly A. Smith-Jentsch. “The Science of Training and Development in Organizations: What Matters in Practice.” Psychological Science in the Public Interest 13.2 (2012): 74–101.
DOI: 10.1177/1529100612436661
Reviews meta-analytic evidence and offers evidence-based recommendations for maximizing training effectiveness.
back to top
Users without a subscription are not able to see the full content on this page. Please subscribe or login .
Oxford Bibliographies Online is available by subscription and perpetual access to institutions. For more information or to contact an Oxford Sales Representative click here .
- About Management »
- Meet the Editorial Board »
- Abusive Supervision
- Adverse Impact and Equal Employment Opportunity Analytics
- Alliance Portfolios
- Applied Political Risk Analysis
- Approaches to Social Responsibility
- Assessment Centers: Theory, Practice and Research
- Attributions
- Authentic Leadership
- Bayesian Statistics
- Behavior, Organizational
- Behavioral Approach to Leadership
- Behavioral Theory of the Firm
- Between Organizations, Social Networks in and
- Brokerage in Networks
- Business and Human Rights
- Certified B Corporations and Benefit Corporations
- Charismatic and Innovative Team Leadership By and For Mill...
- Charismatic and Transformational Leadership
- Compensation, Rewards, Remuneration
- Competitive Dynamics
- Competitive Heterogeneity
- Competitive Intensity
- Computational Modeling
- Conditional Reasoning
- Conflict Management
- Considerate Leadership
- Corporate Philanthropy
- Corporate Social Performance
- Corporate Venture Capital
- Counterproductive Work Behavior (CWB)
- Cross-Cultural Communication
- Cross-Cultural Management
- Cultural Intelligence
- Culture, Organization
- Data Analytic Methods
- Decision Making
- Dynamic Capabilities
- Emotional Labor
- Employee Aging
- Employee Engagement
- Employee Ownership
- Employee Voice
- Empowerment, Psychological
- Entrepreneurial Firms
- Entrepreneurial Orientation
- Entrepreneurship
- Entrepreneurship, Corporate
- Entrepreneurship, Women’s
- Equal Employment Opportunity
- Faking in Personnel Selection
- Family Business, Managing
- Financial Markets in Organization Theory and Economic Soci...
- Findings, Reporting Research
- Firm Bribery
- First-Mover Advantage
- Fit, Person-Environment
- Forecasting
- Founding Teams
- Global Leadership
- Global Talent Management
- Grounded Theory
- Hofstedes Cultural Dimensions
- Human Capital Resource Pipelines
- Human Resource Management, Strategic
- Human Resources, Global
- Human Rights
- Humanitarian Work Psychology
- Humility in Management
- Impression Management at Work
- Influence Strategies/Tactics in the Workplace
- Information Economics
- Innovative Behavior
- Intelligence, Emotional
- International Economic Development and SMEs
- International Economic Systems
- International Strategic Alliances
- Job Analysis and Competency Modeling
- Job Crafting
- Job Satisfaction
- Judgment and Decision Making in Teams
- Knowledge Sharing and Collaboration within and across Firm...
- Leader-Member Exchange
- Leadership Development
- Leadership Development and Organizational Change, Coaching...
- Leadership, Ethical
- Leadership, Global and Comparative
- Leadership, Strategic
- Learning by Doing in Organizational Activities
- Management History
- Management In Antiquity
- Managerial and Organizational Cognition
- Managerial Discretion
- Meaningful Work
- Multinational Corporations and Emerging Markets
- Neo-institutional Theory
- Neuroscience, Organizational
- New Ventures
- Organization Design, Global
- Organization Development and Change
- Organization Research, Ethnography in
- Organization Theory
- Organizational Adaptation
- Organizational Ambidexterity
- Organizational Behavior, Emotions in
- Organizational Citizenship Behaviors (OCBs)
- Organizational Climate
- Organizational Control
- Organizational Corruption
- Organizational Hybridity
- Organizational Identity
- Organizational Justice
- Organizational Legitimacy
- Organizational Networks
- Organizational Paradox
- Organizational Performance, Personality Theory and
- Organizational Responsibility
- Organizational Surveys, Driving Change Through
- Organizations, Big Data in
- Organizations, Gender in
- Organizations, Identity Work in
- Organizations, Political Ideology in
- Organizations, Social Identity Processes in
- Overqualification
- Paternalistic Leadership
- Pay for Skills, Knowledge, and Competencies
- People Analytics
- Performance Appraisal
- Performance Feedback Theory
- Planning And Goal Setting
- Proactive Work Behavior
- Psychological Contracts
- Psychological Safety
- Real Options Theory
- Recruitment
- Regional Entrepreneurship
- Reputation, Organizational Image and
- Research, Ethics in
- Research, Longitudinal
- Research Methods
- Research Methods, Qualitative
- Resource Redeployment
- Resource-Dependence Theory
- Response Surface Analysis, Polynomial Regression and
- Role of Time in Organizational Studies
- Safety, Work Place
- Selection, Applicant Reactions to
- Self-Determination Theory for Work Motivation
- Self-Efficacy
- Self-Fulfilling Prophecy In Management
- Self-Management and Personal Agency
- Sensemaking in and around Organizations
- Service Management
- Shared Team Leadership
- Social Cognitive Theory
- Social Evaluation: Status and Reputation
- Social Movement Theory
- Social Ties and Network Structure
- Socialization
- Sports Settings in Management Research
- Stakeholders
- Status in Organizations
- Strategic Alliances
- Strategic Human Capital
- Strategy and Cognition
- Strategy Implementation
- Structural Contingency Theory/Information Processing Theor...
- Team Composition
- Team Conflict
- Team Design Characteristics
- Team Learning
- Team Mental Models
- Team Newcomers
- Team Performance
- Team Processes
- Teams, Global
- Technology and Innovation Management
- Technology, Organizational Assessment and
- the Workplace, Millennials in
- Theory X and Theory Y
- Time and Motion Studies
- Training and Development
- Trust in Organizational Contexts
- Unobtrusive Measures
- Virtual Teams
- Whistle-Blowing
- Work and Family: An Organizational Science Overview
- Work Contexts, Nonverbal Communication in
- Work, Mindfulness at
- Workplace Aggression and Violence
- Workplace Coaching
- Workplace Commitment
- Workplace Gossip
- Workplace Meetings
- Workplace, Spiritual Leadership in the
- World War II, Management Research during
- Privacy Policy
- Cookie Policy
- Legal Notice
- Accessibility
Powered by:
- [66.249.64.20|109.248.223.228]
- 109.248.223.228
Talent development: a systematic literature review of empirical studies
Purpose The purpose of this study is to identify and examine findings from empirical research regarding organizations’ talent development (TD) strategies, taking into consideration the countries in which the studies were conducted and the TD-approach organizations adopted, and recognize the positive outcomes of TD implementation, as well as potential issues and challenges. Design/methodology/approach This systematic literature review used Garrard’s matrix method to organize the review of publications. It identified 31 empirical articles from the total of 551 publications. Findings The findings indicate that a majority of the studies were conducted in countries other than the USA and that they were all published recently, after 2007. The results show that organizations have mostly applied organizational development interventions at the individual level for developing talented employees, followed by formal training and development. Additionally, managerial issues were identified as the most common issue on the way of implementing TD interventions. Research limitations/implications Trying to define TD as a discrete concept from HRD could be considered as both differentiating the current literature review and a limitation. Originality/value This article is among the first to identify TD interventions through a systematic literature review and provides a model of TD’s intervention antecedents and outcomes for the follow-up empirical works.
- Related Documents
How is process mining technology used by organizations? A systematic literature review of empirical studies
Purpose The purpose of this paper is to review empirical studies on process mining in order to understand its use by organizations. The paper further aims to outline future research opportunities. Design/methodology/approach The authors propose a classification model that combines core conceptual elements of process mining with prior models from technology classification from the enterprise resource planning and business intelligence field. The model incorporates an organizational usage, a system-orientation and service nature, adding a focus on physical services. The application is based on a systematic literature review of 144 research papers. Findings The results show that, thus far, the literature has been chiefly concerned with realization of single business process management systems in single organizations. The authors conclude that cross-system or cross-organizational process mining is underrepresented in the ISR, as is the analysis of physical services. Practical implications Process mining researchers have paid little attention to utilizing complex use cases and mining mixed physical-digital services. Practitioners should work closely with academics to overcome these knowledge gaps. Only then will process mining be on the cusp of becoming a technology that allows new insights into customer processes by supplying business operations with valuable and detailed information. Originality/value Despite the scientific interest in process mining, particularly scant attention has been given by researchers to investigating its use in relatively complex scenarios, e.g., cross-system and cross-organizational process mining. Furthermore, coverage on the use of process mining from a service perspective is limited, which fails to reflect the marketing and business context of most contemporary organizations, wherein the importance of such scenarios is widely acknowledged. The small number of studies encountered may be due to a lack of knowledge about the potential of such scenarios as well as successful examples, a situation the authors seek to remedy with this study.
Entrepreneurship in rural hospitality and tourism. A systematic literature review of past achievements and future promises
Purpose Entrepreneurship in the rural hospitality and tourism sector (RHT) has received wide attention in the past decade. However, a systematic review on this topic is currently lacking. This study aims to track the progress of the RHT and entrepreneurship literature by examining the various thematic research areas, identifying the research gaps and forecasting avenues of future research on the topic. Design/methodology/approach This paper catalogs and synthesizes the body of literature from the year 2000–2020 using a systematic literature review methodology. After discussing a brief history of RHT and entrepreneurship, the current study presents a review of 101 research articles. Findings The review highlights that RHT and entrepreneurship have received relatively limited attention from entrepreneurship journals. The content analysis revealed different gaps and limitations in the understanding of entrepreneurship in RHT, including a predominance of qualitative studies with limited theoretically-grounded and generalizable empirical studies. Furthermore, a high concentration of studies is from European countries. Six main thematic research areas were identified, namely, barriers and enablers, the roles of an entrepreneur, women in RHT, influencers of firm performance, innovation and value creation and methodological commonalities. The review also advances an RHT entrepreneurship ecosystem framework to summarize the findings. Originality/value Six promising research avenues are outlined based on the six themes identified. The suggested research questions draw from allied literature on small and medium businesses, innovation, women entrepreneurship and institutions to encourage the interdisciplinary cross-pollination of ideas. The findings are summarized in a novel research framework.
Successful business intelligence implementation: a systematic literature review
Purpose The purpose of this paper is to present a systematic literature review to determine the factors that relate to successful business intelligence (BI) system implementation. Design/methodology/approach The study has a collection of literature that highlights potential references in relation to factors for system implementation in relation to BI. There is the employment of “content analysis”, given that the study purpose is the achievement of deep understanding of the variety of factors of implementation that other researchers have previously identified. Findings An initial investigation of 38 empirical studies on the implementation of BI led to ten factors being compiled. Difficulties in implementation were found to exist in relation to the operationalisation of large numbers of factors within organisations. The implementation factors were analysed and then sorted into a descending order based upon their frequency of occurrence. Research limitations/implications The research is limited to consider BI implementation factors. Moreover, literature is collected from selected databases and journals from 1998 to 2018. Practical implications Researchers of BI may, within the future, develop models for the measurement of the implementation level of BI within industries along with the sustaining of them. Moreover, work-based learning industries can benefit by adopting the results of this study for the effective implementation of BI. The implementation factors can be seen as key constructs upon which there may be the undertaking of more statistical analyses. Originality/value The original output from this research can help researchers’ in the future in enhancing identification of studies that are relevant for the review of literature for their research.
The measurement of flow and social flow at work: a 30-year systematic review of the literature
Purpose The purpose of this paper is to review three decades of the literature on flow measurement and propose issues to advance research on the measurement of social flow at work. Design/methodology/approach In a systematic literature review, the authors analyzed 143 articles published in the first three decades (1983–2013) of scholarly publications on flow measurement, of which 84 articles used scales to measure flow and 16 articles used scales to measure flow at work. Findings The main findings are: flow is frequently measured in association with other constructs or by means of proxies; flow measurement is highly dependent on a study’s purposes and context; flow is mostly studied at the level of the individual and, when studied beyond the individual, the measurement of flow in groups is simplified as an aggregation of individual-level measures; and social flow at work is an underresearched construct that nevertheless impacts organizations in important ways, thus deserving a specific research agenda. Research limitations/implications The first limitation refers to the databases included in the review. There is always the possibility that important works were ignored. Another limitation is that the coding procedure was highly dependent on the authors’ discretion, as it did not include independent coding and formal assessment of agreement among coders. But the greatest limitation may refer to our very perspectives on flow, flow measurement and social flow at work, as they are highly attached to current models instead of seeing the issues with different lenses. This limitation is also present in the literature. Practical implications Reviewing three decades of scholarly publications on how flow has been measured contributes to organizations in their planning for person-job fit. The measurement of flow can reveal if and when flow correlates with personal characteristics and organizational events, thus serving to inform initiatives on personnel development, acculturation and job design. However, considering that flow as a social phenomenon has been conceived in superficial terms, that a vast number of empirical studies were developed with non-professional subjects, and that flow measurement involves significant adaptations to each situation, organizations are thus advised to be careful in adopting extant instruments. Originality/value This study provides a rich account on how flow measurement has been addressed in the scholarly literature, and it calls attention to research opportunities on social flow at work.
Examining organizations’ continued use of open source technologies
Purpose Our knowledge of why organizations continue to use open source software (OSS) infrastructure technologies is relatively limited, and existing models appear inadequate to explain this continuance phenomenon given that they are set at the individual level and also do not take into account the unique characteristics of OSS. The paper aims to discuss these issues. Design/methodology/approach Using an institutional perspective, this paper posits that coercive (business value of IT) and normative (open source ideology (IDEO)) factors may be credited with sustaining the continued use of OSS technologies. The study argues that organizations that subscribe to IDEO are more likely to continue using OSS technologies. Survey data are collected from organizations that have implemented an OSS infrastructure technology and a moderated multiple regression analysis is performed to test the proposed hypotheses. Findings In addition to the business value provided by OSS technologies, adherence to IDEO also impacts decisions to continue using those technologies. The results suggest that once an OSS is implemented in an organization, IDEO can enhance organizations’ intentions to continue using such technologies, directly, as well as indirectly, by amplifying the impact of the perceived business value of the technology. Originality/value Much of extant literature on continued use focuses on end-user technologies. This paper is one of the first to focus on infrastructure technologies and examine organizations’ intentions to continue using those technologies by developing a parsimonious theory-driven model for examining organizations’ continued use intentions toward infrastructure IT. Additionally, much of open source research to date has been inwardly focused, and this paper is one of few empirical studies to focus on the demand or consumption side of OSS technologies.
A Systematic Literature Review of Organizational Factors Influencing 21st-Century Skills
Skills, such as critical thinking, creativity, communication, problem solving, collaboration, operational skills, and information management, have become increasingly important for 21st-century employees. These skills are often referred to as 21st-century skills and influence how employees handle novel situations. They are indispensable in an economy where the knowledge and skills of employees are seen as a measure for economic potential. This systematic literature review summarizes the current academic knowledge about organizational factors that influence 21st-century skills on an individual level. A search was performed in three databases. The factors found can be sub-divided into three main categories, namely leader characteristics, job characteristics, and organizational characteristics. Transformational leadership was the factor most mentioned in the literature found. Most research found during the search was done on the level of organizational output, exposing a clear gap concerning organizational factors that influence the skill-level of individual employees. These findings can have substantive implications when looking to improve employee skills by altering organizational determinants, by enabling targeted actions to improve these skills for the individual employee.
The disruption of blockchain in auditing – a systematic literature review and an agenda for future research
PurposeThis paper presents a systematic literature review, including content and bibliometric analyses, of the impact of blockchain technology (BT) in auditing, to identify trends, research areas and construct an agenda for future research.Design/methodology/approachThe authors include studies from 2010 to 2020 in their structured literature review (SLR), using accounting journals on the Scopus database, which yielded 40 articles with blockchain and auditing at its core.FindingsOne of the contributions of the authors’ analyses is to group the prior research, and therefore also the agenda for future research, into three main research areas: (1) Blockchain as a tool for auditing professionals to improve business information systems to save time and prevent fraud; (2) Smart contracts enabling Audit 4.0 efficiency, reporting, disclosure and transparency; (3) Cryptocurrency and initial coin offerings (ICOs) as a springboard for corporate governance and new venture financing. The authors’ findings have several important implications for practice and theory.Practical implicationsThe results of this study emphasise that (1) the disruption of blockchain in auditing is in a nascent phase and there is a need for compelling empirical studies and potential for the involvement of practitioners; (2) there may be a need to reconsider audit procedures especially suited for digitalisation and BT adoption; (3) standards, guidelines and training are required to pivot towards and confront the challenge BT will represent for auditing; and (4) there are two sides to the BT coin for auditing, enthusiasm about the potential and risk upon implementation. These practical implications can also be seen as a template for future research in a quest to align theory and practice.Originality/valueThe authors’ SLR facilitates the identification of research areas and implications, forming a useful baseline for practitioners, professionals and academics, as they draft the state of the art on the disruption of blockchain in auditing, highlighting how BT is changing auditing activities and traditions.
Academic entrepreneurship intentions: a systematic literature review
PurposeThe objective of this research is to have an up-to-date and comprehensive assessment of the current knowledge regarding the variables that encourage the individuals, within the academic community, to get involved in knowledge exploitation activities. It is influenced by the observation that there is a need for more systematic scrutiny of micro-level processes to deepen our understanding of academic entrepreneurship (Balven et al., 2018; Wright and Phan, 2018). The study proposes to answer to ‘What are the drivers of academic entrepreneurial intentions?’ and ‘What are the emerging topics for future research?’Design/methodology/approachThe paper follows a Systematic Literature Review process (Tranfield et al., 2003) and adopts a four-step process format from previous literature reviews within the entrepreneurship context (Miller et al., 2018). From the results within Scopus and Web of Science databases, this research selected, evaluated, summarised and synthesised 66 relevant papers.FindingsThis study provides a factor-listed representation of the individual, organisational and institutional variables that should be considered in the strategies defined by the university. Moreover, the study concludes that the push factors behind the intentions are multiple, context-dependent, hierarchy-dependent, heterogeneous and, at the same time, dependent on each other and against each other. Lastly, the study contributes to academic entrepreneurship literature, especially entrepreneurial intention literature, which has recently received more researchers' attention.Originality/valueThe study corroborates that the individual factors, directly and indirectly via Theory of Planned Behaviour, strongly impact the academics' intentions. While the focus of the papers under review was an in-depth analysis of a selected group of factors, this SLR sought to compile the factors that were identified and provide a broader picture of all those factors to be considered by the university management. It contributes to the identification and clustering of the drivers that encourage academics to engage in knowledge valorisation activities, differentiating them by activity. For the practitioners, this list can be used by university managers, TTOs and department managers, and policymakers to guide questionnaires or interviews to analyse their academics' intentions and adequately support its academic engagement strategy. Lastly, this study also suggests worthwhile avenues for future research.
Individual and situational influences on injurious assaults against the police
Purpose Violence against the police represents an ongoing and serious problem in the USA. In 2014, over 48,000 law enforcement officers assaulted while on duty. Although over one in four of these resulted in injury, little is known about the conditions under which injury is likely to occur. The purpose of this paper is to provide an assessment of the individual and situational factors that predict injurious assaults against law enforcement. Design/methodology/approach Using logistic regression, the current study analyzes data from the 2012 National Incident-Based Reporting System (NIBRS) on all assaults against the police (n=8,987) in order to understand, within a routine activities theoretical framework, how individual-level characteristics (i.e. officer and offender characteristics) and situational influences (i.e. assignment type, activity type, and location) predict the likelihood that an assault will result in injury. Findings Overall, findings suggest support for a routine activities theory of violence against the police. Initiating an arrest, one-officer vehicle type, and incidents occurring on highways/roads were all more likely to result in injurious assaults against the police. Other predictors of injury include officer and offender demographics as well as the time the incident took place. Research limitations/implications This research was unable to control for some factors that may influence the likelihood of injury such as wearing body armor. Additionally, NIBRS data are not nationally representative, which limits the generalizability of the findings. Originality/value This is one of the first papers to use national data to examine the individual and situational factors that predict injurious assaults against law enforcement.

Scandals of abuse: policy responses in intellectual disabilities
Purpose – The purpose of this paper is to compare two scandals related to the care of individuals with intellectual and developmental disabilities (I/DD) in the USA and the UK. Design/methodology/approach – A descriptive case study methodology was used to conduct an in-depth qualitative analysis of the two scandals to examine the process of scandal development, and to survey the policy response against policy trends and theories of abuse in each case. The two cases were systematically analysed against a theoretical framework derived from Bonnie and Wallace (2003) theoretical framework for understanding abuse based on its sociocultural context, the social embeddedness of organisations providing care, and the individual level characteristics and interactions of subjects and carers. Findings – In both cases the process of scandal construction was comparable, and each case offered confirmatory support to extant theories of abuse, and to wider policy trends within I/DD. Research limitations/implications – The study examines only the short-term policy responses to the scandals in two countries, based on published material only. Originality/value – This paper contributes an international comparison of the similarities and differences in the social construction of scandal and the policy responses to abuse and neglect of a vulnerable population using systematic analytical frameworks.
Export Citation Format
Share document.
Academia.edu no longer supports Internet Explorer.
To browse Academia.edu and the wider internet faster and more securely, please take a few seconds to upgrade your browser .
Enter the email address you signed up with and we'll email you a reset link.
- We're Hiring!
- Help Center
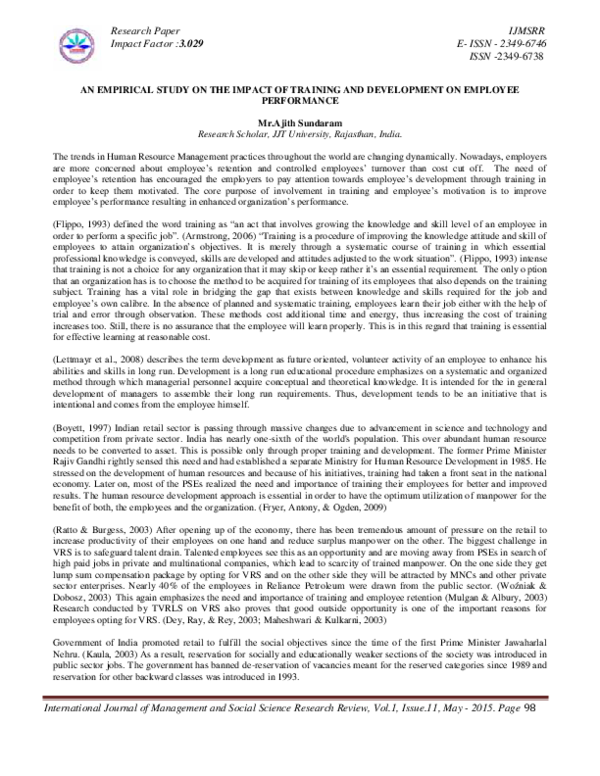
AN EMPIRICAL STUDY ON THE IMPACT OF TRAINING AND DEVELOPMENT ON EMPLOYEE PERFORMANCE

The trends in Human Resource Management practices throughout the world are changing dynamically. Nowadays, employers are more concerned about employee's retention and controlled employees' turnover than cost cut off. The need of employee's retention has encouraged the employers to pay attention towards employee's development through training in order to keep them motivated. The core purpose of involvement in training and employee's motivation is to improve employee's performance resulting in enhanced organization's performance. (Flippo, 1993) defined the word training as " an act that involves growing the knowledge and skill level of an employee in order to perform a specific job ". (Armstrong, 2006) " Training is a procedure of improving the knowledge attitude and skill of employees to attain organization's objectives. It is merely through a systematic course of training in which essential professional knowledge is conveyed, skills are developed and attitudes adjusted to the work situation ". (Flippo, 1993) intense that training is not a choice for any organization that it may skip or keep rather it's an essential requirement. The only o ption that an organization has is to choose the method to be acquired for training of its employees that also depends on the training subject. Training has a vital role in bridging the gap that exists between knowledge and skills required for the job and employee's own calibre. In the absence of planned and systematic training, employees learn their job either with the help of trial and error through observation. These methods cost additional time and energy, thus increasing the cost of training increases too. Still, there is no assurance that the employee will learn properly. This is in this regard that training is essential for effective learning at reasonable cost. (Lettmayr et al., 2008) describes the term development as future oriented, volunteer activity of an employee to enhance his abilities and skills in long run. Development is a long run educational procedure emphasizes on a systematic and organized method through which managerial personnel acquire conceptual and theoretical knowledge. It is intended for the in general development of managers to assemble their long run requirements. Thus, development tends to be an initiative that is intentional and comes from the employee himself. (Boyett, 1997) Indian retail sector is passing through massive changes due to advancement in science and technology and competition from private sector. India has nearly one-sixth of the world's population. This over abundant human resource needs to be converted to asset. This is possible only through proper training and development. The former Prime Minister Rajiv Gandhi rightly sensed this need and had established a separate Ministry for Human Resource Development in 1985. He stressed on the development of human resources and because of his initiatives, training had taken a front seat in the national economy. Later on, most of the PSEs realized the need and importance of training their employees for better and improved results. The human resource development approach is essential in order to have the optimum utilization of manpower for the benefit of both, the employees and the organization. (Fryer, Antony, & Ogden, 2009) (Ratto & Burgess, 2003) After opening up of the economy, there has been tremendous amount of pressure on the retail to increase productivity of their employees on one hand and reduce surplus manpower on the other. The biggest challenge in VRS is to safeguard talent drain. Talented employees see this as an opportunity and are moving away from PSEs in search of high paid jobs in private and multinational companies, which lead to scarcity of trained manpower. On the one side they get lump sum compensation package by opting for VRS and on the other side they will be attracted by MNCs and other private sector enterprises. Nearly 40% of the employees in Reliance Petroleum were drawn from the public sector. (Woźniak & Dobosz, 2003) This again emphasizes the need and importance of training and employee retention (Mulgan & Albury, 2003) Research conducted by TVRLS on VRS also proves that good outside opportunity is one of the important reasons for employees opting for VRS. (Dey, Government of India promoted retail to fulfill the social objectives since the time of the first Prime Minister Jawaharlal Nehru. (Kaula, 2003) As a result, reservation for socially and educationally weaker sections of the society was introduced in public sector jobs. The government has banned de-reservation of vacancies meant for the reserved categories since 1989 and reservation for other backward classes was introduced in 1993.
Related Papers
Venkata Sandeep
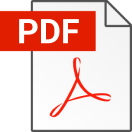
Bharath Kumar
IJRMS Journal
In this competitive world, training plays an important role in the competent and challenging format of business. Training is the nerve that synthesized the smooth functioning of the organization and enhancing the quality of work life of the employees. Development is a process that leads to qualitative as well as quantitative advancements in the organization, especially at the managerial level, it is less considered with physical skills and is more concerned with knowledge, values, attitudes and behavior in addition to specific skills. The purpose of this paper is to measure the impact of training and development on employee's performance and productivity. This paper is quantitative in nature. Data for the paper have been collected through primary source that are from questionnaires surveys of Odisha Cement Limited, Odisha. The study result implies that there is a positive impact of training and development in the improvement of productivity and employee's performance of OCL.
Chief E D I T O R IJRISAT
Training is the art of facilitating the knowledge and skills of an employee to marvel a job. Training moulds the employee's knowledge, skills, aptitude, behaviour and attitude towards the requirements of the job and organization. Training bridges the gap between job requirements and employee specifications but not all employees would be motivated intrinsically to undergo training giving room for the external motivation. The Indian retail industry has presently emerged as one of the most dynamic and fast paced industries. It accounts for over 10 per cent of the country's gross domestic product (GDP). This is an in depth study about the employees working in retail sector in the Ghaziabad district of Uttar Pradesh, India. This study will help the retail industries to know the inner feeling of the employees and thereby train them accordingly. The employer can also implement and customize new techniques to keep the momentum of the staff at good rhythm thereby ensuring smooth and efficient working of the industry. This study will also help to know the common motivational factor that urges the employees to learn swiftly and efficiently thereby helping the companies to save time, resources and get the best work from their employees.
Nigerian Chapter of Arabian Journal of Business and Management Review
Zeeshan Haider , Naqvi Hamad , Emir Čičkušić
Tolulope J Ogunleye
Overtime, study had shown that to be relevant in any field of work there is need for continuous learning through training and development. The study is aimed at finding out the need for employees training and development in an organization. The need for improvement to change the phenomenon of low productivity and poor service delivery attributed to the employee’s in-adequate experience, calls for investigation on how effective training and development of employee can facilitate improved corporate performance using the banking industry as a field of discuss.. The study concluded that training and development brings about career growth for the employees and bankers thus the study recommended that all organization must do induction training at entry point into the banking sector.
Rasmita Behera
In this competitive world, training plays an important role in the competent and challenging format of business. Training is the nerve that synthesized the smooth functioning of the organization and enhancing the quality of work life of the employees. Development is a process that leads to qualitative as well as quantitative advancements in the organization, especially at the managerial level, it is less considered with physical skills and is more concerned with knowledge, values, attitudes and behavior in addition to specific skills. The purpose of this paper is to measure the impact of training and development on employee's performance and productivity. This paper is quantitative in nature. Data for the paper have been collected through primary source that are from questionnaires surveys of Odisha Cement Limited, Odisha. The study result implies that there is a positive impact of training and development in the improvement of productivity and employee's performance of OCL.
sri muthaylamma thalli
In the present era of globalisation, there is an imperative need for employee with extraordinary skills which adds potential to perform better. Human beings are naturally gifted with the potential to learn. It is up to us to leverage this inherent potential and achieve what we are destined to. There has also been rapid transformation in organisation structure and work practices. These changes would definitely have a significant impact for training and development of the work place. As training occupies a top spot in the HR agenda for many organisations, but the impact on the bottom line does not fall in line with expectations. The answer lies in better structure, sharp vision on the end goals and a tighter focus on the cardinal rules of the game. This paper deals with the present scenario and issues in the Indian context. It analyses how these issues if resolved can lead to better productivity.
International Journal of Advanced Research (IJAR)
IJAR Indexing
The Banking sector undergoes a sea level changes with respect to the world?s economic situation irrespective of the economical status of the country. The challenges it faces shapes the way it behaves in the last few decades. The Banks in general have been well taken forward by the Private sector banks which set the destiny of its own compared to the public sector banks. The Reserve Bank of India or Central Banks in many other countries testifies the same the contribution made by them. The reason behind the sea level change or the challenges cannot be handled by Machine, Money or Materials but it is the Men the prime factor would take care of it, meaning they have been updating along with the changes and challenges it faces. The challenges are very similar like, up-gradation in the technology, people?s behavioral change, policy change worldwide, competition and fast changing facts and figures are all the prime contributors for the challenges in the private banking sector. Mumbai is not just one of the states in India where the banks has to function as similar to other places, it I one of the most happening gauged among the top states of cities in the world, therefore the global implication will fall on the Banking players in the state especially private banks, who are considerably fast runners. The challenges have been tackled by the human Resource, named, Employees were provided with the considerable training in two phases, viz., (i) In general and (ii) At a Global standard. The references have considerably contributed to justify and the need for the re-training and re-skilling among the employees of the private banks on all the technical and other areas where the skills to be sharpened. The result of the training well develops the employees professionally along with the organization and the economy as a whole.
African Journal of Management and Business Research
Afropolitan Journals
Employees are the major assets of any organisation. The best thing that can happen to any organisation is for it to have a well-trained workforce; the active role they play towards a company’s success cannot be underestimated. And, as a result, equipping these unique assets by way of training becomes imperative in order to maximise job performances and also positioning them to take on the challenges of today’s competitive business climates. However, extensive researches have been conducted in the area of human resource management, the same cannot be said on employee training especially as it concerns most developing countries. Training and development is a critical process, which seeks to improve the performance of workers in organisations. Training, according to Michael Armstrong (2012) is the use of systematic and planned instructions activities to promote learning. Development would include both training to increase skills in performing a specific job; a total growth of an employee on the job, and education. Moreover, the efficiency and effectiveness of training and development depends on having people with the right skills, attitude and capabilities in order to reach individuals and organisational goals effectively. However, the findings from sampled companies over the years, revealed that working conditions and lack of workable resources affect the HR training and development of employees. It is recommended that certain areas be improved, that is, management support; total commitment of management, sufficient fund, provision of feedback to employees and the conducting of employee training on a continuous basis, which invariably would improve employee’s performance in organisations if properly implemented.
RELATED PAPERS
Caries Research
C. van Loveren
Jeremy Delaney
Iheringia Serie Botanica
Liane Dorneles
Journal of Econometrics
Bolanle Adejumo
Marly Baretta
Revista de Ensino, Educação e Ciências Humanas
Anelisa Esteves
Journal of Molecular Liquids
Hussein Gharibi
Catheterization and Cardiovascular Interventions
gian paolo anzola
Letters in Applied Microbiology
Lucas Correia
Anna Nekaris
Journal of Biotechnology
International Journal of Current Microbiology and Applied Sciences
Dr. PORDHIYA K I S H O R K U M A R ISHWARLAL
Trends in Microbiology
Steven Heaton
MicrobiologyOpen
Cynthia Whitchurch
Estuaries and Coasts
Andrea Woolfolk
Bulletin of Science, Technology & Society
Carl Mitcham
International Journal of Engineering Trends and Technology
Tasaduk Hussain
jhkghjf hfdgedfg
The Prostate
Seong-Tae Kim
Journal of Analytical and Applied Pyrolysis
willem windig
Fronteiras: Journal of Social, Technological and Environmental Science
paulo martinez
arXiv (Cornell University)
Margit Rösler
IEEE Transactions on Robotics
Anthony Maciejewski
Cardiopulmonary Physical Therapy Journal
Nielsen David
RELATED TOPICS
- We're Hiring!
- Help Center
- Find new research papers in:
- Health Sciences
- Earth Sciences
- Cognitive Science
- Mathematics
- Computer Science
- Academia ©2024
MBA Knowledge Base
Business • Management • Technology
Home » Research Literature Reviews » Literature Review – Employee Training and Development
Literature Review – Employee Training and Development
Introduction.
Human resources are considered by many to be the most important asset of an organization, yet very few employers are able to harness the full potential from their employees (Radcliffe, 2005). Human resource is a productive resource consisting of the talents and skills of human beings that contribute to the production of goods and services (Kelly, 2001). Lado and Wilson (1994) define human resource system as a set of distinct but interrelated activities, functions, and processes that are directed at attracting, developing, and maintaining a firm’s human resources. According to Gomez-Mejia, Luis R., David B. Balkin and Robert L. Cardy, (2008), it is the process of ensuring that the organization has the right kind of people in the right places at the right time. The objective of Human Resources is to maximize the return on investment from the organization’s human capital and minimize financial risk. It is the responsibility of human resource managers to conduct these activities in an effective, legal, fair, and consistent manner (Huselid, 1995).
Employee Training and Development
Training and development is a subsystem of an organization that emanate from two independent yet interdependent words training and development. Training is often interpreted as the activity when an expert and learner work together to effectively transfer information from the expert to the learner (to enhance a learner’s knowledge, attitudes or skills) so the learner can better perform a current task or job. Training activity is both focused upon, and evaluated against, the job that an individual currently holds (Learner R., 1986). On the other hand development is often viewed as a broad, ongoing multi-faceted set of activities (training activities among them) to bring someone or an organization up to another threshold of performance. This development often includes a wide variety of methods, e.g., orienting about a role, training in a wide variety of areas, ongoing training on the job, coaching, mentoring and forms of self-development. Some view development as a life-long goal and experience. Development focuses upon the activities that the organization employing the individual, or that the individual is part of, may partake in the future, and is almost impossible to evaluate (Nadler Leonard, 1984).
Training and development ensures that randomness is reduced and learning or behavioral change takes place in structured format. In the field of human resource management , training and development is the field concerned with organizational activity aimed at bettering the performance of individuals and groups in organizational settings. It has been known by several names, including employee development, human resource development , and learning and development (Harrison Rosemary, 2005).
As the generator of new knowledge, employee training and development is placed within a broader strategic context of human resources management , i.e. global organizational management, as a planned staff education and development, both individual and group, with the goal to benefit both the organization and employees. To preserve its obtained positions and increase competitive advantage , the organization needs to be able to create new knowledge , and not only to rely solely on utilization of the existing (Vemic, 2007). Thus, the continuous employee training and development has a significant role in the development of individual and organizational performance . The strategic procedure of employee training and development needs to encourage creativity, ensure inventiveness and shape the entire organizational knowledge that provides the organization with uniqueness and differentiates it from the others.
The Value of Training and Development
According to Beardwell & Holden (1997) human resource management has emerged as a set of prescriptions for managing people at work. Its central claim is that by matching the size and skills of the workforce to the productive requirements of the organization, and by raising the quality of individual employee contributions to production, organizations can make significant improvements on their performance.
The environment of an organization refers to the sum total of the factors or variables that may influence the present and future survival of an organization (Armstrong, 1998). The factors may be internal or external to the organization. Cascio W. F, (1995), uses the terms societal environment to define the varying trends and general forces that do not relate directly to the company but could impact indirectly on the company at some point in time. Four of these forces are identified as economic, technological, legal and political and socio-cultural and demographic forces. The second type of environment is the task environment that comprises elements directly influencing the operations and strategy of the organization. These may include the labour market, trade unions, competition and product markets comprising customers, suppliers and creditors. The task environment elements are directly linked to the company and are influenced by the societal environment.
However, variables in the task, competitive or operative environment as they are variously referred to, affect organizations in a specific industry and it is possible to control them to some extent. As such, environmental change, whether remote or task, disrupts the equilibrium that exists between the organization’s strategy and structure, necessitating adjustment to change. Pfeffer (1998) proposes that there is evidence demonstrating that effectively managed people can produce substantially enhanced economic performance. Pfeffer extracted from various studies, related literature, and personal observation and experience a set of seven dimensions that seem to characterize most if not all of the systems producing profits through people. He named them the seven practices of successful organizations and they are: employment security, selective hiring of new personnel, self-managed teams and decentralization of decision making as the basic principles of organizational design, comparatively high compensation contingent on organizational performance , extensive training, reduced status distinctions and barriers, including dress, language, office arrangements, and wage differences across levels, and extensive sharing of financial and performance information throughout the organization.
Effect of Training and Development on Employee Productivity
McGhee (1997) stated that an organization should commit its resources to a training activity only if, in the best judgment of managers, the training can be expected to achieve some results other than modifying employee behavior. It must support some organizational goals , such as more efficient production or distribution of goods and services, product operating costs, improved quality or more efficient personal relations is the modification of employees behavior affected through training should be aimed at supporting organization objectives.
Effect of Training and Development on Employee Motivation
Motivation is concerned with the factors that influence people to behave in certain ways. Arnold etal (1991), have listed the components as being, direction-what a person is trying to do, effort- how hard a person is trying to and persistence- how long a person keeps on trying. Motivating other people is about getting them to move in the direction you want them to go in order to achieve a result, well motivated people are those with clearly defined goals who take action that they expect will achieve those goals. Motivation at work can take place in two ways. First, people can motivate themselves by seeking, finding and carrying out that which satisfies their needs or at least leads them to expect that their goals will be achieved. Secondly, management can motivate people through such methods as pay, promotion, praise and training (Synderman 1957). The organization as a whole can provide the context within which high levels of motivation can be achieved training the employees in areas of their job performance.
Effect of Training and Development on Competitive Advantage
Competitive advantage is the essence of competitive strategy . It encompasses those capabilities, resources, relationships, and decisions, which permits an organization to capitalize on opportunities in the marketplace and to avoid threats to its desired position, (Lengnick-Hall 1990). Boxall and Purcell (1992) suggest that ‘human resource advantage can be traced to better people employed in organizations with better processes.’ This echoes the resource based view of the firm, which states that ‘distinctive human resource practices help to create the unique competences that determine how firms compete’ (Capelli and Crocker- Hefter, 1996). Intellectual capital is the source of competitive advantage for organizations. The challenge is to ensure that firms have the ability to find, assimilate, compensate, and retain human capital in shape of talented individual who can drive a global organization that both responsive to its customer and ‘the burgeoning opportunities of technology’ (Armstrong, 2005)
Effect of Training and Development on Customer Relations
William Edward Deming , one of the quality Gurus defines quality as a predictable degree of uniformity and dependability at low costs and suitable to the market, he advises that an organization should focus on the improvement of the process as the system rather than the work is the cause of production variation (Gale 1994). Many service organizations have embraced this approach of quality assurance by checking on the systems and processes used to deliver the end product to the consumer. Essentially this checks on; pre-sale activities which encompass the advice and guidance given to a prospective client, customer communications ( how well the customers are informed of the products and services, whether there are any consultancy services provided to help the customers assess their needs and any help line available for ease of access to information on products), the speed of handling a client’s transactions and processing of claims, the speed of handling customers calls and the number of calls abandoned or not answered, on the selling point of Products/Services a customer would be interested to know about the opening hours of the organization, the convenience of the location and such issues (Gale 1994). This is only possible when employees are well trained and developed to ensure sustainability of the same.
- Armstrong, M (1998): Human Resource Management: Strategy and Action, Irwin, Boston
- Betcherman, G., K. McMullen and K. Davidman (1998), Training for the New Economy: A Synthesis Report, Canadian Policy Research Network, Ottawa, pp. 117
- Cascio, W. F. (1995). Whither industrial and organizational psychology in a changing world of work?American Psychologist, 50, 928—939
- Harrison Rosemary (2005). Learning and Development.CIPD Publishing. pp. 5
- Huselid, M. A. (1995) The impact of human resource management practices on turnover, productivity and corporate financial performance, Academy of Management Journal, 38(3), 635-672
- Kelly D, (2001), Dual Perceptions of HRD: Issues for Policy: SME’s, Other Constituencies, and the Contested Definitions of Human Resource Development,
- Lado, A., & Wilson, M. (1994) Human resource systems and sustained competitive advantage: A competency-based perspective, Academy of Management Journal, 19(4), 699-727
- Learner, R. (1986).Concepts and Theories of Human Development (2nd ed.). New York: Random House).
- Nadler, Leonard (1984). The Handbook of Human Resource Development (Glossary). New York: John Wiley & Sons.
- Pfeffer J., (1998), The Human Equation; Building Profits by Putting People First, HBS press, Boston
- Tessema, M. and Soeters, J. (2006) Challenges and prospects of HRM in developing countries: testing the HRM-performance link in Eritrean civil service, International Journal of Human Resource Management, 17(1), 86 -105
Related posts:
- Different Employee Training and Development Methods
- Literature Review – Organizational Learning
- Literature Review about E-Banking In India
- Case Study of Dell: Employee Training and Development
- Role of Case Studies in Employee Training and Development
- Literature Review – Quality Management Systems
- Literature Review – Social Media Marketing Strategies
- Literature Review – Credit Derivatives
- How to Write a Good Literature Review
- The Literature Review in Research
Leave a Reply Cancel reply
Your email address will not be published. Required fields are marked *

IMAGES
VIDEO
COMMENTS
©2018 IJRAR August 2018, Volume 5, Issue 3 www.ijrar.org (E-ISSN 2348-1269, P- ISSN 2349-5138) IJRAR190A012 International Journal of Research and Analytical Reviews (IJRAR) www.ijrar.org 49 Review of Literature: Bramley and Kitson (1994) discussed four level of training evaluation.The first is the trainee's reaction to
A comprehensive review of training and development literature from 1999 to 2009 with an emphasis on the benefits that training offers across multiple levels of analysis. Arthur, Winfred A., Jr., Winston Bennett Jr., Pamela S. Edens, and Suzanne T. Bell. "Effectiveness of Training in Organizations: A Meta-analysis of Design and Evaluation ...
Key words: Training and Development, Evaluation and effectiveness of training, Employee's attitude, Satisfaction of employees 1. Introduction This paper enunciates the importance, need of review of literature and the related review of studies to the topic. Management Education in India is of a comparatively recent origin.
The human resource development strategy involves higher education. In this manner, the business course plans to furnish understudies with the abilities required in the realm of work..
Literature review. 2.1. Training. Training is consists of an organization's planned efforts to help employees ... training and development is the organization's strategic instrument to improve employee performance by acquiring and equipping employees with the cutting-edge skills and knowledge along with the right organization attitude by the ...
This article provides a review of the training and development literature since the year 2000. We review the literature focusing on the benefits of training and development for individuals and teams, organizations, and society. We adopt a multidisciplinary, multilevel, and global perspective to demonstrate that training and development activities in work organizations can produce important ...
3. Development training should be afforded to all levels and/or positions. 4. Training and development is important for job growth. 5. Training and development is important for potential advancement. If you have not had training, please skip Questions 6-12. 6. I receive updated training which is required for my position. 7.
Background: The need for competence training and development in health management and leadership workforces has been emphasised. However, evidence of the outcomes and impact of such training and development has not been systematically assessed. The aim of this review is to synthesise the available evidence of the outcomes and impact of training and development in relation to the competence of ...
Talent development: a systematic literature review of empirical studies. Purpose The purpose of this study is to identify and examine findings from empirical research regarding organizations' talent development (TD) strategies, taking into consideration the countries in which the studies were conducted and the TD-approach organizations ...
A literature review on training and development and quality of work life. Pallavi P. Kulkarni. Published 1 April 2013. Business, Education, Psychology. The authors suggest that training and development is a process leading to qualitative as well as quantitative advancements in an organization, especially at the managerial level.
Framework on the Effe ctiveness of Training & Development - "Review Of Literature" , International Journal of Mechanical Engineering and Technology, 9(7), 2018, pp. 932 - 943.
Literature Review: Training And Development: According to the Michel Armstrong, "Training is systematic development of the knowledge, skills and attitudes required by an individual to perform adequately a given task or job". (Source: A Handbook of Human Resource Management Practice, Kogan Page, 8th Ed.,2001) According
The development of information and communication technologies has changed our way of working, emphasizing the need for individuals to develop collaborative skills. The aim of the present systematic review was to examine the extent to which these skills can be developed through the use of digital tools. A search of seven electronic databases ...
The literature review of HRM-related JIT articles suggests that HRM practices in the areas of staffing, training and development, compensation, and employee retention can be used to develop an ...
Furthermore, the study seeks to investigate the meaning of training and development, identify the importance of employee performance and also, the study seeks to explore the relationship between training and development and employees' performance. This study will be conducted through the use of secondary data in form of a literature review.
LITERATURE REVIEW ON TRAINING AND DEVELOPMENT IN WORK SETTING . Abstract . This study reviews the existing literature in training and development, which considered as essential practices in Human Resource Management (HRM); moreover, they constitute a necessary investment and a significant component of the organizations' budgets.
LITERATURE REVIEW: TRAINING AND DEVELOPMENT: According to the Michel Armstrong, "Training is systematic development of the knowledge, skills and attitudes required by an individual to perform adequately a given task or job". (Source: A Handbook of Human Resource Management Practice, Kogan Page, 8th Ed.,2001) According to the Edwin B Flippo ...
After conducting a vast literature review the following hypothesis was developed for the purpose of this study completion. Ho: Training & Development positively affects Perceived Employee Performance. H1: Training & Development negatively affects Perceived Employee Performance. Standard deviation measures the level/ amount of dispersion for the ...
Effect of Training and Development on Employee Motivation. Motivation is concerned with the factors that influence people to behave in certain ways. Arnold etal (1991), have listed the components as being, direction-what a person is trying to do, effort- how hard a person is trying to and persistence- how long a person keeps on trying.
T & D program make the organization result oriented and enable it to face technological, political and environmental changes. Karim et al (2019) was of the opinion that Training and Development is ...
Joint leadership development training; capacity building program Awareness of the importance and diversification of UG and AL in universities ... Fischer M., Mitra M., Ledger J., Behrens S. (2019). Leadership development in higher education: A literature review and implications for programme redesign. Higher Education Quarterly, 73, 218-234 ...
233578771 Literature Review on Employee Training and Development - Free download as Word Doc (.doc / .docx), PDF File (.pdf), Text File (.txt) or read online for free. The document discusses training and development in organizations. It defines training as imparting job-specific skills to help employees perform their current roles better, while development prepares employees for future roles.
Framework on the Effe ctiveness of Training & Development - "Review Of Literature" , International Journal of Mechanical Engineering and Technology, 9(7), 2018, pp. 932 - 943.
The initial broad search returned 8,420 publications which were filtered and reviewed in-depth to fit the aim of the study, produced only 21 relevant publications from the years 2000-2021.FindingsThe four identified themes of obstacles to SC in developing countries are as follows: construction professional training and education, clients ...
LITERATURE REVIEW ON TRAINING AND DEVELOPMENT IN WORK SETTING. February 2023. BAU Journal - Society Culture and Human Behavior 4 (2) DOI: 10.54729/2789-8296.1136. Authors: Abdul Beydoun. Beirut ...
Ahmed et al. (2014) examined the impact of training and development on employee performance. while focusing a case study from different banking sector of north Punjab. The dimensions used. in this ...