This site uses cookies to optimize functionality and give you the best possible experience. If you continue to navigate this website beyond this page, cookies will be placed on your browser. To learn more about cookies, click here .

From economic wealth to well-being: exploring the importance of happiness economy for sustainable development through systematic literature review
- Open access
- Published: 23 May 2024
Cite this article
You have full access to this open access article
- Shruti Agrawal ORCID: orcid.org/0000-0002-1620-9429 1 , 5 ,
- Nidhi Sharma 1 , 5 ,
- Karambir Singh Dhayal ORCID: orcid.org/0000-0002-0000-4330 2 &
- Luca Esposito ORCID: orcid.org/0000-0001-5983-6898 3 , 4
575 Accesses
Explore all metrics
The pursuit of happiness has been an essential goal of individuals and countries throughout history. In the past few years, researchers and academicians have developed a huge interest in the notion of a ‘happiness economy’ that aims to prioritize subjective well-being and life satisfaction over traditional economic indicators such as Gross Domestic Product (GDP). Over the past few years, many countries have adopted a happiness and well-being-oriented framework to re-design the welfare policies and assess environmental, social, economic, and sustainable progress. Such a policy framework focuses on human and planetary well-being instead of material growth and income. The present study offers a comprehensive summary of the existing studies on the subject, exploring how a happiness economy framework can help achieve sustainable development. For this purpose, a systematic literature review (SLR) summarised 257 research publications from 1995 to 2023. The review yielded five major thematic clusters, namely- (i) Going beyond GDP: Transition towards happiness economy, (ii) Rethinking growth for sustainability and ecological regeneration, (iii) Beyond money and happiness policy, (iv) Health, human capital and wellbeing and (v) Policy push for happiness economy. Furthermore, the study proposes future research directions to help researchers and policymakers build a happiness economy framework.
Similar content being viewed by others
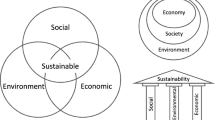
Three pillars of sustainability: in search of conceptual origins

The costs and benefits of environmental sustainability
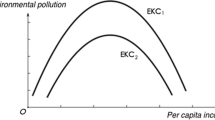
Impact of green finance on economic development and environmental quality: a study based on provincial panel data from China
Avoid common mistakes on your manuscript.
1 Introduction
Happiness is considered the ultimate goal of human beings (Ikeda, 2010 ; Lama, 2012 ). All economic, social, environmental and political human activities are aligned towards achieving this goal. This fundamental pursuit of human life introduces a new scope of research, namely the ‘happiness economy’ (Agrawal and Sharma 2023 ). The happiness economy is an emerging economic domain wherein many countries are working to envision and implement a happiness-oriented framework by expanding how they measure economic success, which includes wellbeing and sustainability (Cook and Davíðsdóttir 2021 ; Forgeard et al., 2011 ). The investigation of happiness, life-satisfaction and subjective well-being has witnessed increasing research interest across the disciplines- from psychology, philosophy, psychiatry, and cognitive neuroscience to sociology, economics and management (Diener 1984 ; Hallberg and Kullenberg, 2019 ).
In the post-Covid era, the world seeks an enormous transformation shift in the public system (Costanza 2020 ). However, public authorities need more time to realize such needs. To experience the ‘policy transformation’ within the coming few years, we require a paradigm shift that helps warm peoples’ hearts and minds. The new economic paradigm can penetrate the policy processes in advanced economies and every part of the world affected by the epidemic with the support of intellectuals, researchers, entrepreneurs and professionals.
OECD ( 2016 ) proposed a well-being economy framework to measure living conditions and people’s well-being. In 2020, developed countries like Finland, New Zealand, Iceland, Scotland and Wales have become members of the Wellbeing Economy Government (WEGo) (Abrar 2021 ). Since then, the network of government and international authorities across the globe has gained a quick momentum concerning an increasing tendency about a growing tendency to concentrate governmental decisions around human well-being rather than wealth and economic growth (Coscieme et al. 2019 ; Costanza et al. 2020 ).
In light of these circumstances, the purpose of this article is to describe the concept of a “happiness economy” or one that seeks to give everyone fair possibilities for growth, a sense of social inclusion, and stability that can support human resilience (Coyne and Boettke 2006 ). It provides a promising route towards improved social well-being and environmental health and is oriented towards serving individuals and communities (Skul’skaya & Shirokova, 2010 ). Moreover, the happiness economy paradigm is a transition from material production and consumption of commodities and services as the only means to economic development towards embracing a considerable variety of economic, social, environmental and subjective well-being dynamics that are considered fundamental contributors to human happiness (Atkinson et al., 2012 ; King et al., 2014 ; Agrawal and Sharma 2023 ). In following so, it reflects the ‘beyond growth’ approach that empathizes with the revised concept of growth, which is not centred around an increase in income or material production; instead it is grounded in the philosophy of achieving greater happiness for more people (Fioramonti et al. 2019a ).
Whereas the other critiques of economic growth emphasize contraction, frugality and deprivation, the happiness economy relies on a cumulative approach of humanity, hope and well-being, with a perceptive to build a ‘forward-looking’ narrative of ways for humans to live a happy and motivated life by inspiring the cumulative actions and encouraging policy-reforms in the measuring growth of an economy (Stucke 2013 ). Agrawal et al. ( 2023a , b ) explore the domain of happiness economics through a review of the various trends coupled with the future directions and highlight why it needs to be supported for a well-managed economic system and a happy society.
In this paper, we define a “happiness economy as an economy that aims to achieve the well-being of individuals in a nation, promoting human happiness, environmental up-gradation, and sustainability. Alternatively, as an economy where the wellbeing of people counts more than the goals of production and income”. Moreover, we have examined the existing body of research on the happiness economy and analyzed the emerging research themes related to rethinking the conventional approach to economic growth. We conclude by discussing how the happiness economy concept has been accepted so far and realizing its importance by triggering policy reforms at the societal level, by outlining potential future directions that might be included into the current national post-growth policies.
Various researchers and experts in the field of happiness economy support the idea that there is a lack of thorough studies related to the concept, definitions, and themes of the happiness economy model in the nations. This gap has motivated us to conduct a SLR in order to identify the evolution in the domain of happiness economy and to identify the emerging themes in this context. Therefore, this present study seeks to offer a holistic outline of the emerging research area of the happiness economy and helps to understand how the happiness economy can accelerate sustainable development. With the following research questions, this study seeks to give an all-encompassing review of this subject.
What is the annual publication trend in this domain and the most contributing authors, journals, countries etc?
Which themes and upcoming research areas are present in this field?
What directions will the happiness economics study field go in the future?
The SCOPUS database was used to achieve the above research objectives. We have selected 257 articles for examination by hand-selecting the pertinent keywords and going over each one. In the methods section, a thorough explanation of the procedures for gathering, reviewing, and selecting documents is provided.
The remainder of this paper is structured as follows; A thorough survey of the literature on the happiness economy is provided in Sect. 2 . The research approach employed in the study is presented in Sect. 3 . A thorough data analysis of the research findings is given in Sect. 4 . After discussing the results in Sect. 5 , Sect. 6 suggests areas for further research in this field. The study is summarised with a conclusion in Sect. 7 . Section 8 outlines the study’s limitation.
2 Literature review
The supporters of conventional economic growth proclaim that the material production of goods and services and consumption is vital to enhancing one’s living standards. The statement is true to some degree, mainly in countries of enormous deprivation. Some studies have found significantly less correlation between growth and happiness after fulfilling minimum threshold needs (Easterlin 1995 ; Kahneman and Krueger, 2006 ; Inglehart et al., 2008 ). These studies recommend that rather than concentrating solely on economic growth, governmental policy should give priority to non-economic aspects of human existence above a particular income level. According to some researchers, it is challenging to distinguish between the use and emissions of natural resources and economic growth (absolute decoupling) because of the interdependence between socioeconomic conditions and their biophysical basis (Wiedenhofer et al. 2020 ; Wang and Su, 2019 ; Wu et al., 2018 ). However, a shred of increasing evidence shows that it could be possible for humans to maintain a quality of life and a decent standard of living inside the ecological frontier of the environment, given that a contemporary perspective on the production and use of materials are adopted in conjunction with more fair wealth distribution (Millward-Hopkins et al. 2020 ; Bengtsson et al., 2018 ; Ni et al., 2022 ).
The scholarly discourse and institutional framework on the relationship between happiness and economic progress are synthesised in the happiness economy (Frey and Gallus 2012 ; Sohn, 2010 ; Clark et al., 2016 ; Easterlin, 2015 ; Su et al., 2022 ). From a happiness economy perspective, extreme materialism is unsustainable as it significantly impacts natural resources and hinders social coherence and individuals psychological and physical well-being (Fioramonti et al. 2022a ). Additionally, inequalities within countries have grown, while psychological suffering has increased, especially during accelerated growth (Vicente 2020 ; Galbraith, 2009 ). The modern world is witnessing anxiety, depression, wars, reduction of empathy, climate change, pandemics, loss of social bonds and other psychological disorders (Brahmi et al., 2022 ; Santini et al., 2015 ).
It has been scientifically proven that cordial human relations, care-based activity, voluntary activities and the living environment immensely impact a person’s health and societal well-being (Bowler et al. 2010 ; Keniger et al., 2013 ). Ecological economists demonstrated that free ecosystem services have enhanced human well-being (Fang et al. 2022 ). Social epidemiologists have long argued that an increase in inequalities has a negative influence on society while providing equality tends to improve significant objective ways of well-being, from healthier communities to happier communities, declining hate and crime and enhancing social cohesion, productivity, unity and mutual trust (Aiyar and Ebeke 2020 ; Ferriss, 2010 ).
From moving beyond materialistic growth, the happiness economy promotes, appreciates, and protects the environmental, societal, and human capital contributions that lead to cummalative well-being. In a happiness economy framework, a multidimensional approach is needed to evaluate the level of development based on the environmental parameters, health outcomes, as well as public trust, hope, value-creating education and social bonds (Agrawal and Sharma 2023 ; Bayani et al. 2023 ; Lavrov, 2010 ). Such factors have consistently been excluded from any traditional concept or assessment of economic growth. As a result, countries have promoted more industrial activities that deteriorate the authentic ways of human well-being and, hence, the foundations of economic progress.
An excess of production can create a detrimental effect on climate and people’s health, thereby creating a negative externality for society (Fioramonti et al. 2022b ). Moderation of output may be more efficient and desirable than hyper/over-production, as the former can reduce negative environmental externalities (e.g. waste, climate change) and create positive externalities (e.g. employment of the local resources and community) (Kim et al. 2019 ; Kinman and Jones, 2008 ). Moreover, people can also be productive in other contexts outside of the workplace, such as as volunteers, business owners, artists, friends, or members of the community (Fioramonti et al. 2022a ).
Various scholars and scientific research have established that the essential contributions to happiness in one’s life are made by natural surroundings, green and blue spaces, eco-friendly environment, healthy social relations, spirituality, good health, responsible consumption and value-creating education (Helliwell et al. 2021 ; Francart et al., 2018 ; Armstrong et al., 2016 ; Gilead, 2016 ; Giannetti et al., 2015 ). Unfortunately, existing conventional growth theories have ignored all these significant contributions. For example, GDP considers natural ecosystems as economically helpful only up until they are mined and their products are traded (Carrero et al. 2020 ). The non-market benefits they generate, such as natural fertilization, soil regeneration, climate regulation, clean air and maintenance of biodiversity, are entirely ignored (Boyd 2007 ; Hirschauer et al., 2014). The quality time people spend with their families and communities for leisure, educating future generations and making a healthy communal harmony is regarded meaningless, even in the event that they are important to enhance people’s well-being and, hence, to assist any dimension of economic engagement (Griep et al. 2015 ; Agrawal et al., 2020 ). Similarly, if an economy is focusing on people’s healthy lifestyle (for example, by providing comfortable working hours, improving work-life balance, emphasizing mental health, focusing on healthy food, reducing pollution, and promoting sustainable consumption), it is not considered in sync with the growth paradigm (Roy 2021 ; Scrieciu et al., 2013; Shrivastava and Zsolnai 2022 ; Lauzon et al., 2023 ).
Among the latest reviews, Bayani et al. ( 2023 ) highlight that the economics of happiness helps reduce the country’s financial crime by providing a livelihood that reduces financial delinquency. Chen ( 2023 ) highlights that smart city performance enhances urban happiness by adopting green spaces, reusing and recycling products, and controlling pollution. The study by (Agrawal and Sharma 2023 ) proposed a conceptual framework for a happiness economy to achieve sustainability by going beyond GDP. Similarly, Fioramonti et al. ( 2019b ) explored going beyond GDP for a transition towards a happy and well-being economy. The article by Laurent et al. ( 2022 ) has intensively reviewed the well-being indicators in Rome and proposed a conceptual framework for it.
Table 1 provides a thorough summary of the prior review studies about the happiness economy and its contribution to public policy and sustainable development.
3 Research methodology
In the current study, we have adopted an integrative review approach of SLR and bibliometric analysis of the academic literature to get a detailed knowledge of the study, which could also help propose future research avenues. The existing scientific production’s qualitative and quantitative context must be incorporated for a conclusive decision. The study by Meredith ( 1993 ) defines that SLR enables an “integrating several different works on the same topic, summarising the common elements, contrasting the differences, and extending the work in some fashion”. In the present study, the “Preferred Reporting Items for Systematic Reviews and Meta-Analyses” (PRISMA) is applied to perform the SLR to follow systematic and transparent steps for the research methodology, as shown in Fig. 1 . The PRISMA technique includes the identification, screening, eligibility, and exclusion criteria parts of the review process.
Additionally, examples of the data abstraction and analysis processes are provided (Mengist et al. 2020 ; Moher et al., 2015 ). The four main phases of the PRISMA process are eligibility, identification, screening, and data abstraction and analysis. Because the PRISMA technique employs sequential steps to accomplish the study’s purpose, it benefits SLR research. Moreover, the bibliometric analysis helps summarise the existing literature’s bibliographic data and determine the emerging condition of the intellectual structure and developing tendencies in the specified research domain (Dervis 2019 ).
3.1 Identification
The step to conduct the PRISMA is the identification of the relevant keywords to initiate the search for material. Next, search strings for the digital library’s search services are created using the selected keywords. The basic search query is for digital library article titles, keywords, and abstracts. Next, a Boolean AND or OR operator is used to generate the search string (Boolean combinations of the operators may also be used).
There are different search databases to conduct the review studies, such as Scopus, Sage, Web of Science, IEEE, and Google Scholar. Among all the available search databases, we have used the Scopus database to identify the articles; since 84% of the material on Web of Science (WoS) overlaps with Scopus, very few authors have addressed the benefits of adopting Scopus over WoS (Mongeon and Paul-Hus 2016 ). Scopus is widely used by academicians and researchers for quantitative analysis (Donthu et al. 2021 ). It is the biggest database of scientific research and contains citations and abstracts from peer-reviewed publications consisting of journal research articles, books and conference articles (Farooque et al., 2019 ; Dhayal et al., 2022 ; Brahmi et al., 2022 ). The following search term was used: (TITLE-ABS-KEY (“happiness economy” OR “economics of happiness” OR “happiness in economy” OR “economy of happiness” OR “economy of wellbeing” OR “wellbeing economy” OR “wellbeing in economy” OR “beyond growth”). This process yields 380 artciles in the initial phase.
3.2 Screening
The second phase is completed by all identified articles from the Scopus database obtained from the search string in the identification phase. The publications are either included or excluded throughout the screening process based on the standards established by the authors and with the aid of particular databases. Exclusion and inclusion criteria are shown during the screening phase to identify pertinent articles for the systematic review procedure. The timeline of this study’s selected articles is from 1995 to 2023. The first article related to the research domain was published in 1995. The second criterion for the inclusion includes the types of documents. In the present research, the authors have regarded only peer-reviewed journals and review articles. Other types of articles, such as books, book chapters, conference articles, notes, and editorials, are excluded to maintain the quality of the review. The third inclusion and exclusion criterion is based on language. All the non-English language documents are excluded to avoid translation confusion; hence, only the English language articles are considered for the final review. After the screening process, 297 articles are obtained.
3.3 Eligibility
Articles are manually selected or excluded depending on specific criteria specified by the authors during the eligibility process. During the elimination process, the authors excluded the articles that did not fit into the scope of review after manual screening of the articles. Two hundred fifty-seven articles were selected after the eligibility procedure. These selected articles are carefully reviewed for the study by reviewing the titles, abstracts, and standards from earlier screening processes.
3.4 Data abstraction and analysis
Analysis and abstraction of data are part of the fourth step. Finally, 257 papers were taken into account for final review. After that, the studies are culled to identify pertinent themes and subthemes for the current investigation by thoroughly reviewing each article’s text. An integrative review is a form of study that combines mixed, qualitative, and quantitative research procedures. It is carried out as shown in Fig. 1 . R-studio Bibliometrix and VOSviewer version 1.6.18 were used to evaluate the final study dataset corpus of 257 articles. Since the Bibliometrix software package is a free-source tool programmed in the R language. It is proficient of conducting comprehensive scientific mapping. It also contains several graphical and statistical features with flexible and frequent updates (Agrawal et al. 2023a , b ).
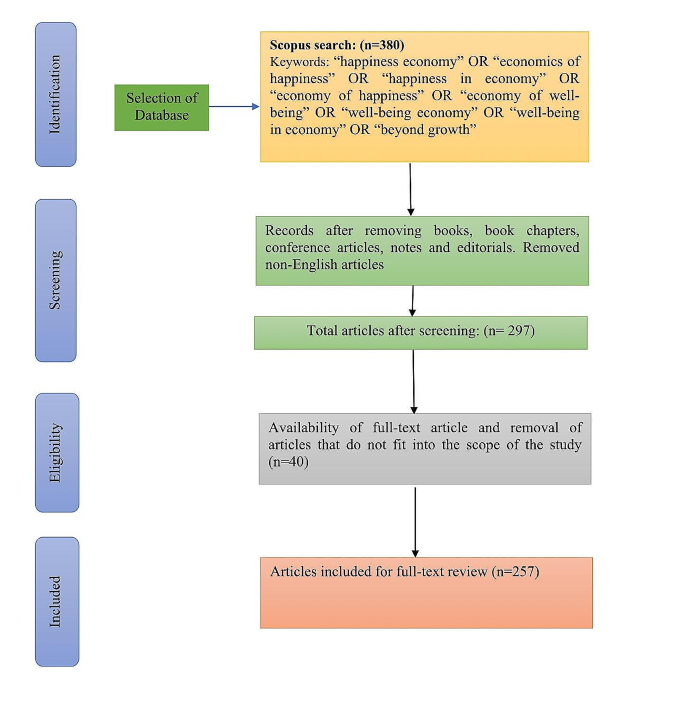
Extraction of articles and selection process
This section provides an answer to the first research question, RQ1, by indicating the main information of corpus data, research publication trends, influential prolific authors, journals, countries and most used keywords, etc. (Refer to Tables 2 , 3 and 4 ) and (Refer to Figs. 2 , 3 , 4 , 5 and 6 ).
4.1 Bibliometric analysis
Table 2 shows the relevant information gathered from the publication-related details. It presents the cognitive knowledge of the research area, for instance, details about authors, annual average publication, average citations and collaboration index. By observing the rate of document publishing, the study illustrates how much has already been done and how much remains to be investigated.
The annual publication trend is shown in Fig. 2 . It is reflected that the first article related to happiness in an economy was released in the year 1995 when (Bowling 1995 ) published the article “What things are important in people’s lives? A survey of the public’s judgements to inform scales of health related quality of life” where the article discussed “quality of life” and “happiness” as an essential component of a healthy life. Oswald ( 1997 ) brought the concept of happiness and economics together and raised questions such as “Does money buy happiness?” or “Do you think your children’s lives will be better than your own?”. Eventually, the gross national product of the past year and the coming year’s exchange rate was no longer the concern; instead, happiness as the sublime moment became more accurate (Schyns 1998 ; Easterlin, 2001; Frey and Stutzer, 2005 ). Post-2013, we can see exponential growth in the publication trend, and the reason behind the growth is the report published by the “ Stiglitz-Sen-Fitoussi” Commission, which has identified limitations of GDP and questioned the metric of wealth, economic and societal progress. The affirmed questions have gained the attention of researchers and organizations, and thus, they have explored the alternatives to GDP. As a result, the “Organization for Economic Co-operation and Development” (OECD) have proposed a wellbeing framework. Some research work has significantly impacted that time, contributing to the immense growth in this research area (Sangha et al. 2015 ; Spruk and Kešeljević, 2015 ; Nunes et al., 2016 ).
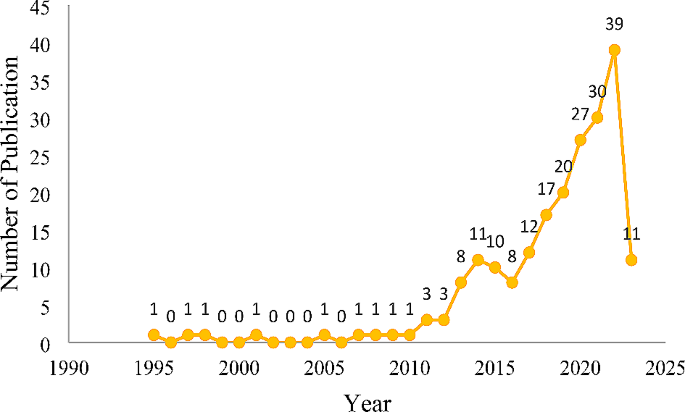
Publication trend
Table 3 shows the top prolific journals concerning the topmost publications in the domain of happiness economy for the corpus of 257 articles, namely “International Journal of Environmental Research and Public Health”, “Ecological Economics”, “Ecological Indicators”, “Sustainability” and “Journal of Cleaner Production” with 5, 4, 4,4 and 4 articles respectively (Refer to Table 4 ). Moreover, the most influential journals with maximum citations are “Nature Human Behavior”, “Quality of Life Research”, “Journal of Applied Behavior Analysis”, “Journal of Cleaner Production” and “Ecological Economics”, with 219, 205, 186, 154 and 142 citations, respectively. “Journal of Cleaner Production” and “Ecological Economics” are highly prolific and the most influential journals in the happiness economy research domain.
Table 4 shows the most influential authors. Baños, R.M. and Botella, C. are the two most contributing authors with maximum publications. For the maximum number of citations, Zheng G. and Coscieme L. are the topmost authors for their research work. The nations were sorted according to the quantity of publications, and Fig. 3 showed where the top ten countries with the highest number of publications are listed originated. It can be seen from the figure that the United Stated has contributed the maximum publications, 66, followed by the United Kingdom with 41 articles, followed by Germany with 32 articles. It is worth noting that emerging nation such as India and China have also made significant contributions.
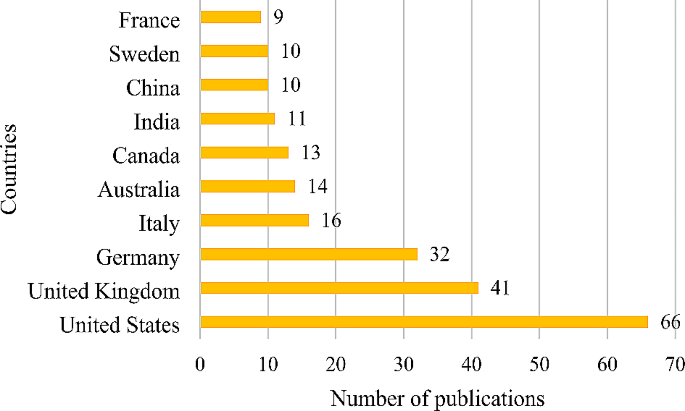
Top ten contributing countries
Figure 4 shows semantic network analysis in which the relationships between words in individual texts are performed. In the present study, we have identified word frequency distributions and the co-occurrences of the authors’ keywords in this study. We employed co-word analysis to find repeated keywords or terms in the title, abstract, or body of a text. In Fig. 5 , the circle’s colour represents a particular cluster, and the circle’s radius indicates how frequently the words occur. The size of a keyword’s node indicates how frequently that keyword appears. The arcs connecting the nodes represent their co-occurrence in the same publication. The greater the distance between two nodes, the more often the two terms co-occur. It can be seen that “happiness” is linked with “growth” and “life satisfaction”. The nodes of “green economy”, “ecological economics”, and “climate change” are in a separate cluster that shows they are emerging areas, and future studies can explore the relationship between happiness economy with these keywords.
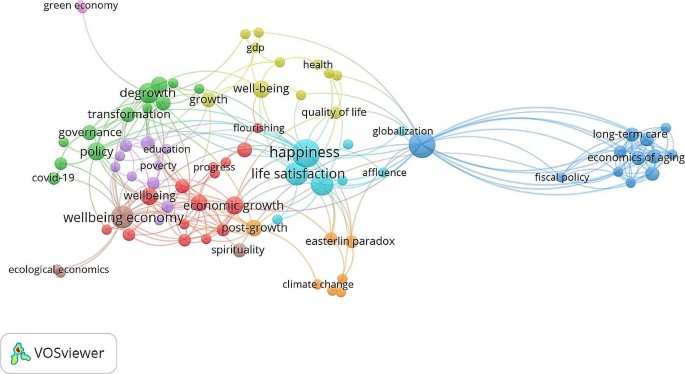
Co-ocurrance of author’s keyword (Author’s compilation)
4.2 Thematic map analysis through R studio
The thematic analysis map, as shown in Fig. 5 , displays, beneath the author’s keywords, the visualisation of four distinct topic typologies produced via a biblioshiny interface. The thematic map shows nine themes/clusters under four quadrants segregated in “Callon’s centrality” and “density value”. The degree of interconnectedness between networks is determined by Callon’s centrality, while Callon’s density determines the internal strength of networks. (Chen et al. 2019 ). The rectangular boxes in Fig. 5 represent the subthemes under each topic or cluster that are either directly or indirectly connected to the major themes, based on the available research. In the upper-right quadrant, four themes have appeared, namely “circular economy”, “well-being economy”, “depression”, and “sustainable development”, they fall under the category of motor themes since they are extremely pertinent to the research field, highly repetitious, and well-developed. When compared to other issues with internal linkages but few exterior relations, “urban population” in the upper-left quadrant is seen as a niche concern since it is not as significant. This cluster may have affected the urban population’s happiness (Knickel et al. 2021 ). “Social innovation” is categorised as an emerging or declining subject with low centrality and density, meaning it is peripheral and undeveloped. It is positioned in the lower-left quadrant. Last but not least, the transversal and fundamental themes “happiness economy”, “subjective well-being”, and “climate change” in the lower-right quadrant are seen to be crucial to the happiness economy study field but are still in the early stages of development. As a result, future research must place greater emphasis on the quantitative and qualitative growth of the study area in light of the key themes that have been identified.
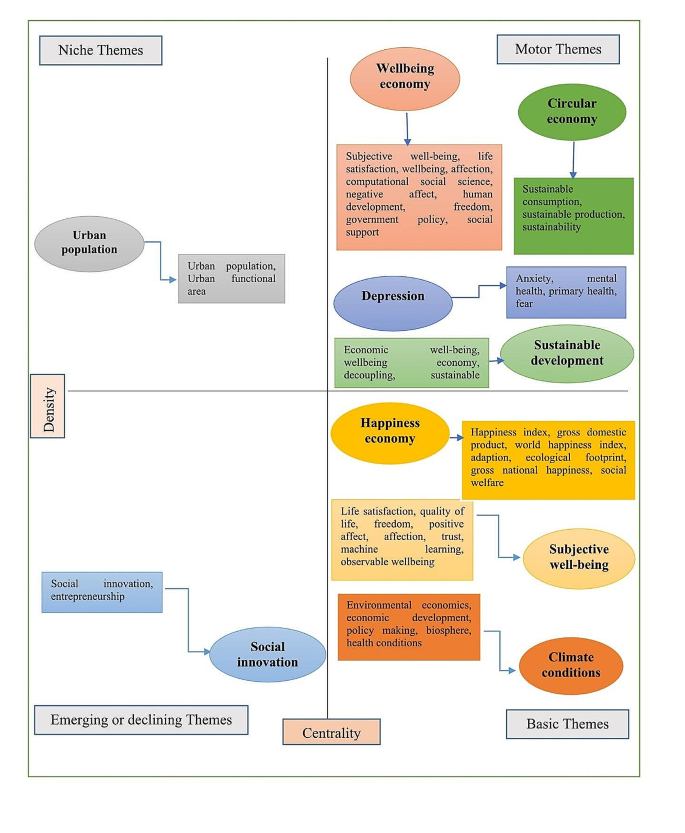
Thematic map analysis
4.3 Science mapping through cluster analysis
In the study, science mapping was conducted to examine the interrelationship between the research domains that could be intellectual (Aria and Cuccurullo 2017 ; Donthu et al. 2021 ). It includes various techniques, such as co-authorship analysis, co-occurrence analysis, bibliographic coupling, etc. We have used R-Studio for the study’s temporal analysis by cluster analysis. To answer RQ2, the authors have performed a qualitative examination of the emerging cluster themes through the science mapping of the existing research corpus of 257 articles by performing bibliographic coupling of documents. Bibliographic coupling analysis helps identify clusters reflecting the most recent research themes in the happiness economy field to illuminate the field’s current areas of interest.
The visual presentation of science mapping relied on VoSviewer version 1.6.18 (refer to Fig. 6 ). Five significant clusters emerged in this research domain (refer to Table 5 ). Going beyond GDP: Transition towards happiness economy, rethinking growth for sustainability and ecological regeneration, beyond money and happiness policy, health, human capital and wellbeing and Policy-Push for happiness economy. A thorough examination identified cluster analyzes has also assists us in identifying potential future research proposals. (Franceschet 2009 )
4.4 Cluster 1: Going beyond GDP: transition towards happiness economy
It depicts from the green colour circles and nodes, where seven research articles were identified with a common theme of beyond GDP that can be seen in Fig. 6 . Cook and Davíðsdóttir ( 2021 ) investigated the linkages between the alternative measure of the beyond growth approach such as a well-being economy prespective and the SDGs. They proposed a conceptual model of a well-being economy consisting of four capital assets interrelated with SDGs that promote well-being goals and domains. To extend the concept of going beyond GDP, various economic well-being indicators are being aligned with the different economic, environmental, and social dimensions to target the set goals of SDG. It is found that the “Genuine Progress Indicator” (GPI) is consider as the most extensive method that covers the fourteen targets among the seventeen’s SDG’s. Cook et al. ( 2022 ) consider SDGs to represent the classical, neoclassical and growth-based economy model and as an emerging paradigm for a well-being economy. The significance of GDP is more recognized within the goals of sustainable development.
GPI is considered an alternative indicator of economic well-being. On this basis, excess consumption of high-quality energy will expand macro-economic activity, which GDP measures. For such, a conceptual exploration of the study is conducted on how pursuing “Sustainable Energy Development” (SED) that can increase the GPI results. As the study’s outcome, according to the GPI, SED will have a significant advantage in implementing energy and environment policy and will also contribute to the advancement of social and economic well-being. Coscieme et al. ( 2020a ) explored the connection between the unconditional growth of GDP and SDG. The author considered that policy coherence for sustainable development should lessen the damaging effects of cyclic manufacturing on the ecosystem. Thus, the services considered free of charge in the GDP model should be valued as a component of society. Generally, such services include ecosystem services and a myriad of “economic” functions like rainfall and carbon sequestration. To work for SDG 8, defined by the “United Nations Sustainable Development Goals” (UNSDGs), a higher GDP growth rate would eventually make it more difficult to achieve environmental targets and lessen inequality. Various guidelines were proposed to select alternative variables for SDG-8 to enhance coherence among all the SDG and other policies for sustainability.
Fioramonti et al. ( 2019a ) state their focus is to go beyond GDP toward a well-being economy rather than material output with the help of convergence reforms in policies and economic shifts. To achieve the SDG through protecting the environment, promoting equality, equitable development and sharing economy. The authors have developed the Sustainable Well-Being Index (SWBI) to consolidate the “Beyond GDP” streams as a metric of well-being matched with the objectives to achieve SDG. The indicators of well-being for an economy have enough possibility to connect current transformations in the economic policies and the economy that, generally, GDP is unable to capture.
Fioramonti et al. ( 2022a ) investigate the critical features of the Wellbeing Economy (WE), including its various parameters like work, technology, and productivity. Posting a WE framework that works for mainstream post-growth policy at the national and international levels was the study’s primary goal. The authors have focused on building a society that promotes well-being that should be empowering, adaptable, and integrative. A well-being economic model should develop new tools and indicators to monitor all ecological and human well-being contributors. A multidimensional approach including critical components for a well-being economy was proposed that creates value to re-focus on economic, societal, personal, and natural aspects. Rubio-Mozos et al. ( 2019 ) conducted in-depth interviews with Fourth Sector business leaders, entrepreneurs, and academicians to investigate the function of small and medium-sized businesses and the pressing need to update the economic model using a new measure in line with UN2030. They have proposed a network from “limits to growth” to a “sustainable well-being economy”.
4.5 Cluster 2: Rethinking growth for sustainability and ecological regeneration
Figure 6 depicts it from blue circles and nodes, wherein four papers were identified. Knickel et al. ( 2021 ) proposed an analytical approach by collecting the data from 11 European areas to examine the existing conditions, difficulties, and anticipated routes forward. The goal of the study is to define the many ideas of a sustainable well-being economy and territorial development plans that adhere to the fundamental characteristics of a well-being economy. A transition from a conventional economic viewpoint to a broader view of sustainable well-being is centred on regional development plans and shifting rural-urban interactions.
Pillay ( 2020 ) investigates the new theories of de-growth, ecosocialism, well-being and happiness economy to break the barriers of traditional economic debates by investigating ways to commercialise and subjugate the state to a society in line with non-human nature. The significant indicator of Gross National Happiness (GNH) is an alternative working indicator of development; thus, the Chinese wall between Buddha and Marx has been built. They questioned the perspective of Buddha and Marx, whether they were harmonized or became a counter-hegemonic movement. In order to determine if the happiness principle is grounded in spiritual values and aligns with the counter-hegemonic ecosocialist movement, the author examined the ecosocialist perspective. Shrivastava and Zsolnai ( 2022 ) have investigated the theoretical and practical ramifications of creative organisations for well-being rooted in the drive for a well-being economy. Wellbeing and happiness-focused economic frameworks are emerging primarily in developed countries. This new policy framework also abolishes GDP-based economic growth and prioritizes individual well-being and ecological regeneration. To understand its application and interpretation, Van Niekerk ( 2019 ) develops a conceptual framework and theoretical analysis of inclusive economics. It contributes to developing a new paradigm for economic growth, both theoretically and practically.
4.6 Cluster 3: ‘Beyond money’ and happiness policy
It depicts pink circles and nodes, wherein five articles were identified, as shown in Fig. 6 . According to Diener and Seligman ( 2004a ) economic indicators are critical in the early phases of economic growth when meeting basic requirements is the primary focus. However, as society becomes wealthier, an individual’s well-being becomes less dependent on money and more on social interactions and job satisfaction. Individuals reporting high well-being outperform those reporting low well-being in terms of income and performance. A national well-being index is required to evaluate well-being variables and shape policies systematically. Diener and Seligman ( 2018 ) propounded the ‘Beyond Money’ concept in 2004. In response to the shortcomings of GDP and economic measures, other quality-of-life indicators, such as health and education, have been created. The national account of well-being has been proposed as a common path to provide societies with an overall quality of life metric. While measuring the subjective well-being of people, the authors reasoned a societal indicator of the quality of life. In this article, the authors have proposed an economy of well-being model by combining subjective and objective measures to convince policymakers and academicians to enact policies that enhance human welfare. The well-being economy includes quality of life indicators and life satisfaction, subjective well-being and happiness.
Frey and Stutzer ( 2000 ) perceived the microeconomic well-being variables in countries. In the study, survey data was used from 6000 individuals in Switzerland and showed that the individuals are happier in developed democracies and institutions (government federalization). They analyzed the reported subjective well-being data to determine the function of federal and democratic institutions on an individual’s satisfaction with life. The study found a negative relationship between income and unemployment. Three criteria have been employed in the study to determine happiness: demographic and psychological traits, macro- and microeconomic factors, and constitutional circumstances. Thus, a new pair of determinants reflects happiness’s effect on individuals’ income, unemployment, inflation and income growth.
Happiness policy, according to Frey and Gallus ( 2013b ), is an intrinsic aspect of the democratic process in which various opinions are collected and examined. “Happiness policy” is far more critical than continuing a goal such as increasing national income and instead considered an official policy goal. The article focuses on how politicians behave differently when they believe that achieving happiness is the primary objective of policy. Frey et al. ( 2014 ) explored the three critical areas of happiness, which are positive and negative shocks on happiness, choice of comparison and its extent to derive the theoretical propositions that can be investigated in future research. It discussed the areas where a more novel and comprehensive theoretical framework is needed: comparison, adaptation, and happiness policy. Wolfgramm et al. ( 2020 ) derived a value-driven transformation framework in Māori economics of wellbeing. It contributes to a multilevel and comprehensive review of Māori economics and well-being. The framework is adopted to advance the policies and implement economies of well-being.
4.7 Cluster 4: Health, human capital and wellbeing
It is depicted as a red colour circle and nodes in Fig. 6 , and only three papers on empirical investigations were found. Laurent et al. ( 2022 ) investigated the Health-Environment Nexus report published by the “Wellbeing Economy Alliance”. In place of increased production and consumption, they suggested a comprehensive framework for human health and the environment that includes six essential paths. The six key pathways are well-being energy, sustainable food, health care, education, social cooperation and health-environment nexus. The proposed variables yield the co-benefits for the climate, health and sustainable economy. Steer clear of the false perception of trade-offs, such as balancing the economy against the environment or the need to save lives. McKinnon and Kennedy ( 2021 ) focuses on community economics of well-being that benefits entrepreneurs and employees. They investigated the interactions of four social enterprises that work for their employees inside and within the broader community. Cylus et al. ( 2020 ) proposed the opportunities and challenges in adopting the model of happiness or well-being in an economy as an alternative measure of GDP. Orekhov et al. ( 2020 ) proposed the derivation of happiness from the World Happiness Index (WHI) data to estimate the regression model for developed countries.
4.8 Cluster 5: Policy-push for happiness economy
It is depicted as an orange circle and nodes in Fig. 6 , and only five papers on empirical and review investigations were found. Oehler-Șincai et al. ( 2023 ) proposed the conceptual and practical perspective of household-income-labour dynamics for policy formulation. It discusses the measurement of well-being as a representation of various policies focusing on health, productivity, and longevity. It focuses on the role of policy in building the subjective and objective dimensions of well-being, defines the correlation between well-being, employment policies, and governance, is inclined to the well-being performance of various countries, and underscores present risks that jeopardize well-being. Musa et al. ( 2018 ) have developed a “community happiness index” by incorporating the four aspects of sustainability—economic, social, environmental, and urban governance—as well as the other sustainability domains, such as human well-being and eco-environmental well-being. From then onwards, community happiness and sustainable urban development emerged. Chernyahivska et al. ( 2020 ) developed strategies to raise the standard of living for people in countries undergoing economic transition by using the quality of life index. The methods uncovered are enhancing employment opportunities and uplifting the international labour market in urban and rural areas, prioritizing human capital, eliminating gender inequality, focusing on improving the individual’s health, and enhancing social protection. Zheng et al. ( 2019 ) investigated the livelihood and well-being index of the population that makes liveable conditions and city construction in society based on people’s happiness index. The structure of a liveable city should be emphasised on sustainable development. The growth strategy in urban areas is an essential aspect of building a liveable city. Frey and Gallus ( 2013a ) criticised the National Happiness Index as a policy goal in a country because it cannot be measured and thus fails to measure the true happiness of people. To measure real happiness, the government should establish living conditions that enable individuals to become happy. The rule of law and human rights must support the process.
The structure of a liveable city should be emphasized in sustainable development. The growth strategy in urban areas is an essential aspect of building a liveable city. Frey and Gallus ( 2013a ) criticized the National Happiness Index as a policy goal in a country because it cannot be measured and thus fails to identify the true individuals happiness. To measure real happiness, the government should establish living conditions that enable individuals to be happy. The process needs to be supported by human rights and the rule of law.
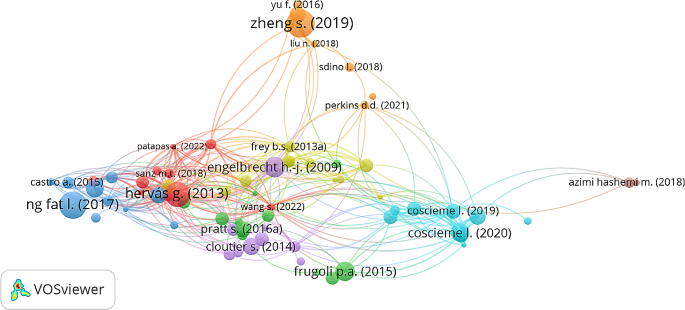
Visualization of cluster analysis
5 Discussion of findings
Concerns like the improved quality-of-life and a decent standard of living within the ecological frontier of the environment have various effects on individuals overall well-being and life satisfaction. The ‘beyond growth’ approach empathized with the revised concept of growth, which is based on the idea of maximising happiness for a larger number of people rather than being driven by a desire for financial wealth or production. In that aspect, the notion of happiness economy is designed that prioritizes serving both people and the environment over the other. This present article has focused on the beyond growth approach and towards a new economic paradigm by doing bibliometric and visual analysis on the dataset that was obtained from Scopus, helping to determine which nations, publications, and authors were most significant in this field of study.
In this field of study, developed nations have made significant contributions as compared to the developing nations. In total, 59 countries have made the substantial contribution to the beyond growth approach literature an some of them have proposed their respective national well-being economy framwework. Among 59 countries the United States and the United Kingdom have been crucial to the publishing. With the exception of five of the top 10 nations, Europe contributes the most to scientific research. The existing research shows the inclination of developed and developing countries to build a new economic paradigm that goes beyond growth by prioritizing the happiness level at individual as well as at collective level.
The most prolific journals in this research domain are the “International Journal of Environmental Research” and “Public Health” with the total publication of 5 and 4. The top two cited journals were the “ Nature Human Behavior” with 219 citations and the “Quality of Life Research” with 205 citations. Due to various economic and non-economic factors, these journals struggled to strike a balance between scientific accuracy and timeliness, and it became vital to spread accurate and logical knowledge. For, example, discussing the relationship between inequality and well-being, exploring the challenges and opportunites of happiness economy in different countries, assessing the role of health in all policies to support the transition to the well-being economy. Visualization of semantic network analysis of co-ocurrance of authors keywords from the VOSviewer showed the future research scope to explore the association between happiness economy along with green economy, climate change, spirituality and sustainability. However, in the thematic mapping, the motor themes denotes the themes that are well-developed and repetative in research, such as, well-being economy, depression, sustainable development and circular economy. The basic themes depicts the developing and transveral themes such as happiness economy, subjective well-being and climate condition. As a result, future research must place greater emphasis on the theoretical and practical expansion of the research field in view of the determined major subjects.
The present study have performed the cluster analysis to identify the emerging research themes in this domain through VOSviewer that helps to analyze the network of published documents. Based on published papers, the author can analyse the interconnected network structure with the use of cluster analysis. We have identified the top five clusters from the study. Each cluster denote the specific and defined theme of the research in this domain. In cluster 1, the majorly of the authors are working in the area of going beyond GDP and transition towards happiness economy, which consists of empirical and review studies. Cluster 2 represents that authors are exploring the relationship between rethinking growth for sustainability and ecological regeneration to evaluate the transition from a conventional economic thought to a broader view of sustainable well-being which is centred on regional development plans and shifting rural-urban interactions. In cluster 3, the authors are exploring the beyond money and happiness policy themes and identified the shortcomings of GDP and economic measures, other quality-of-life indicators, such as health and education. They have proposed the well-being index to evaluate the well-being variables and shape socio-economic policies systematically. The authors have proposed an economy of well-being model by combining subjective and objective measures to convince policymakers and academicians to enact policies that enhance human welfare. The well-being economy includes quality of life indicators and life satisfaction, subjective well-being and happiness. In cluster 4, the authors are working of related theme of Health, human capital and wellbeing, whereby they have put up a comprehensive framework for health and the environment that includes several important avenues for prioritising human and ecological well-being over increased production and consumption. In cluster 5, the authors have suggested the policy-push for happiness economy in which they have identified the conceptual and practical perspective of household-income-labour dynamics for policy formulation. Majorly of the authors in this clutster have focused on the role of policy in building the subjective and objective dimensions of well-being, defines the correlation between well-being, employment policies, and governance, is inclined to the well-being performance of various countries, and underscores present risks that jeopardize well-being. Hence, the present study will give academics, researchers, and policymakers a thorough understanding of the productivity, features, key factors, and research outcomes in this field of study.
6 Scope for future research avenues
The emergence of a happiness economy will transform society’s traditional welfare measure. Such changes will generate more reliable and practical means to measure the well-being or welfare of an economy. After a rigorous analysis of the existing literature, we have proposed the scope for future research in Table 6 .
7 Conclusion
In 2015, the United Nations proposed the pathbreaking and ambitious seventeen “Sustainable Development Goals” (SDGs) for countries to steer their policies toward achieving them by 2030. In reality, economic growth remains central to the agenda for SDGs, demonstrating the absence of a ground-breaking and inspirational vision that might genuinely place people and their happiness at the core of a new paradigm for development. As this research has reflect, there are various evidence that the happiness economy strategy is well-suited to permeate policies geared towards sustainable development. In this context, ‘happiness’ may be a strong concept that ensures the post-2030 growth will resonate with the socioeconomic and environmental traits of everyone around the world while motivating public policies for happiness.
The current research has emphasized the many dynamics of the happiness economy by using a bibliometric analytic study of 257 articles. We have concluded that the happiness economy is an emerging area that includes different dimensions of happiness, such as ecological regeneration, circular economy, sustainability, sustainable well-being, economic well-being, subjective well-being, and well-being economy. In addition to taking into consideration the advantages and disadvantages of human participation in the market, a happiness-based economic system would offer new metrics to assess all contributions to human and planetary well-being. In terms of theoretical ramifications, we suggest that future scholars concentrate on fusing the welfare and happiness theory with economic policy. As countries are predisposed to generate disharmony and imbalance, maximizing societal well-being now entails expanding sustainable development. Since the happiness economy is still a relatively novel field, it offers numerous potential research opportunities.
8 Limitations
Similar to every other research, this one has significant restrictions as well. We are primarily concerned that all our data were extracted from the Scopus database. Furthermore, future research can utilize other software like BibExcel and Gephi to expound novel variables and linkages. Given the research limitations, this article still provides insightful and relevant direction to policymakers, scholars, and those intrigued by the idea of happiness and well-being in mainstream economics.
The study offers scope for future research in connecting the happiness economy framework with different SDGs. Future studies can also carry empirical research towards creating a universally acceptable ‘happiness economy index’ with human and planetary well-being at its core.
Data availability
Data not used in this article.
Abrar, R.: Building the transition together: WEAll’s perspective on creating a Wellbeing Economy. Well-Being Transition. 157–180 (2021). https://doi.org/10.1007/978-3-030-67860-9_9/COVER
Agrawal, R., Agrawal, S., Samadhiya, A., Kumar, A., Luthra, S., Jain, V.: Adoption of green finance and green innovation for achieving circularity: An exploratory review and future directions. Geosci. Front. 101669 (2023a). https://doi.org/10.1016/J.GSF.2023.101669
Agrawal, S., Sharma, N., Singh, M.: Employing CBPR to understand the well-being of higher education students during covid-19 lockdown in India. SSRN Electron. J. (2020). https://doi.org/10.2139/ssrn.3628458
Agrawal, S., Sharma, N.: Beyond GDP: A movement toward happiness economy to achieve sustainability. Sustain. Green. Future. 95–114 (2023). https://doi.org/10.1007/978-3-031-24942-6_5
Agrawal, S., Sharma, N., Bruni, M.E., Iazzolino, G.: Happiness economics: Discovering future research trends through a systematic literature review. J. Clean. Prod. 416 , 137860 (2023b). https://doi.org/10.1016/j.jclepro.2023.137860
Article Google Scholar
Aiyar, S., Ebeke, C.: Inequality of opportunity, inequality of income and economic growth. World Dev. 136 , 105115 (2020). https://doi.org/10.1016/J.WORLDDEV.2020.105115
Armstrong, C.M.J., Connell, K.Y.H., Lang, C., Ruppert-Stroescu, M., LeHew, M.L.A.: Educating for sustainable fashion: using clothing acquisition abstinence to explore sustainable consumption and life beyond growth. J Consum Policy. 39 (4), 417–439 (2016). https://doi.org/10.1007/s10603-016-9330-z
Approaches to Improving the Quality of Life: How to Enhance the Quality of Life - Abbott L. Ferriss - Google Books . (n.d.). Retrieved April 25, from (2023). https://books.google.co.in/books?hl=en&lr=&id=9AKdtNzGsGcC&oi=fnd&pg=PR8&dq=equality+tends+to+improve+major+objective+ways+of+wellbeing,+from+healthier+communities+to+happier+communities,+from+declining+hate+and+crime+and+to+improved+social+cohesion,+productivity,+unity+and+interpersonal+trust&ots=pZ5kbKdqrC&sig=vfwoVTo2Aur-nV9J9HNF4rbF74o&redir_esc=y#v=onepage&q&f=false
Aria, M., Cuccurullo, C.: Bibliometrix: An R-tool for comprehensive science mapping analysis. J. Informetrics. 11 (4), 959–975 (2017). https://doi.org/10.1016/J.JOI.2017.08.007
Atkinson, S., Fuller, S., Painter, J.: Wellbeing and place, pp. 1–14. Ashgate Publishing (2012). https://researchers.mq.edu.au/en/publications/wellbeing-and-place
Bengtsson, M., Alfredsson, E., Cohen, M., Lorek, S., Schroeder, P.: Transforming systems of consumption and production for achieving the sustainable development goals: moving beyond efficiency. Sustain. Sci. 13 (6), 1533–1547 (2018). https://doi.org/10.1007/s11625-018-0582-1
Bayani, E., Ahadi, F., Beigi, J.: The preventive impact of Happiness Economy on Financial Delinquency. Political Sociol. Iran. 5 (11), 4651–4670 (2023). https://doi.org/10.30510/PSI.2022.349645.3666
Better Life Initiative: Measuring Well-Being and Progress - OECD . (n.d.). Retrieved December 8, from (2022). https://www.oecd.org/wise/better-life-initiative.htm
Bowler, D.E., Buyung-Ali, L.M., Knight, T.M., Pullin, A.S.: A systematic review of evidence for the added benefits to health of exposure to natural environments. BMC Public. Health. 10 (1), 1–10 (2010). https://doi.org/10.1186/1471-2458-10-456/TABLES/1
Bowling, A.: What things are important in people’s lives? A survey of the public’s judgements to inform scales of health related quality of life. Soc. Sci. Med. 41 (10), 1447–1462 (1995). https://doi.org/10.1016/0277-9536(95)00113-L
Boyd, J.: Nonmarket benefits of nature: What should be counted in green GDP? Ecol. Econ. 61 (4), 716–723 (2007). https://doi.org/10.1016/J.ECOLECON.2006.06.016
Brahmi, M., Aldieri, L., Dhayal, K.S., Agrawal, S.: Education 4.0: can it be a component of the sustainable well-being of students? pp. 215–230 (2022). https://doi.org/10.4018/978-1-6684-4981-3.ch014
Carrero, G.C., Fearnside, P.M., Valle, D. R., de Alves, S., C: Deforestation trajectories on a Development Frontier in the Brazilian Amazon: 35 years of settlement colonization, policy and economic shifts, and Land Accumulation. Environ. Manage. 2020. 66:6 (6), 966–984 (2020). https://doi.org/10.1007/S00267-020-01354-W 66
Chen, C.W.: Can smart cities bring happiness to promote sustainable development? Contexts and clues of subjective well-being and urban livability. Developments Built Environ. 13 , 100108 (2023). https://doi.org/10.1016/J.DIBE.2022.100108
Chen, X., Lun, Y., Yan, J., Hao, T., Weng, H.: Discovering thematic change and evolution of utilizing social media for healthcare research. BMC Med. Inf. Decis. Mak. 19 (2), 39–53 (2019). https://doi.org/10.1186/S12911-019-0757-4/FIGURES/10
Chernyahivska, V.V., Bilyk, O.I., Charkina, A.O., Zhayvoronok, I., Farynovych, I.V.: Strategy for improving the quality of life in countries with economies in transition. Int. J. Manag. 11 (4), 523–531 (2020).
Clark, A.E., Flèche, S., Senik, C.: Economic growth evens out happiness: evidence from six surveys. Rev Income Wealth. 62 (3), 405–419 (2016). https://doi.org/10.1111/roiw.12190
Construction strategies and evaluation models of livable city based on the happiness index | IEEE Conference Publication | IEEE Xplore . (n.d.). Retrieved April 1, from (2023). https://ieeexplore.ieee.org/abstract/document/6640911
Cook, D., Davíðsdóttir, B.: An appraisal of interlinkages between macro-economic indicators of economic well-being and the sustainable development goals. Ecol. Econ. 184 (2021). https://doi.org/10.1016/j.ecolecon.2021.106996
Cook, D., Davíðsdóttir, B., Gunnarsdóttir, I.: A conceptual exploration of how the pursuit of sustainable Energy Development is implicit in the genuine Progress Indicator. Energies. 15 (6) (2022). https://doi.org/10.3390/en15062129
Coscieme, L., Sutton, P., Mortensen, L.F., Kubiszewski, I., Costanza, R., Trebeck, K., Pulselli, F.M., Giannetti, B.F., Fioramonti, L.: Overcoming the myths of mainstream economics to enable a newwellbeing economy. Sustain. (Switzerland). 11 (16) (2019). https://doi.org/10.3390/su11164374
Coscieme, L., Mortensen, L.F., Anderson, S., Ward, J., Donohue, I., Sutton, P.C.: Going beyond gross domestic product as an indicator to bring coherence to the Sustainable Development Goals. J. Clean. Prod. 248 , 119232 (2020). https://doi.org/10.1016/J.JCLEPRO.2019.119232
Coscieme, L., Mortensen, L.F., Anderson, S., Ward, J., Donohue, I., Sutton, P.C.: Going beyond gross domestic product as an indicator to bring coherence to the Sustainable Development Goals. J. Clean. Prod. 248 (2020a). https://doi.org/10.1016/j.jclepro.2019.119232
Costanza, R.: Ecological economics in 2049: Getting beyond the argument culture to the world we all want. Ecol. Econ. 168 , 106484 (2020). https://doi.org/10.1016/J.ECOLECON.2019.106484
Costanza, R., Caniglia, E., Fioramonti, L., Kubiszewski, I., Lewis, H., Lovins, H., McGlade, J., Mortensen, L.F., Philipsen, D., Pickett, K.E., Ragnarsdottir, K.V., Roberts, D.: Toward a Sustainable Wellbeing Economy. Solutions: For a Sustainable and Desirable Future . (2020). https://openresearch-repository.anu.edu.au/handle/1885/205271
Coyne, C.J., Boettke, P.J.: Economics and Happiness Research: Insights from Austrian and Public Choice Economics. Happiness Public. Policy. 89–105 (2006). https://doi.org/10.1057/9780230288027_5
Cylus, J., Smith, P.C., Smith, P.C.: The economy of wellbeing: What is it and what are the implications for health? BMJ. 369 (2020). https://doi.org/10.1136/bmj.m1874
Dervis, H.: Bibliometric analysis using bibliometrix an R package. J. Scientometr. Res. 8 (3), 156–160 (2019). https://doi.org/10.5530/JSCIRES.8.3.32
Dhayal, K.S., Brahmi, M., Agrawal, S., Aldieri, L., Vinci, C.P.: A paradigm shift in education systems due to COVID-19, pp. 157–166 (2022)
Diener, E.: Subjective well-being. Psychol. Bull. 95 (3), 542–575. (1984). https://doi.org/10.1037/0033-2909.95.3.542. https://psycnet.apa.org/record/1984-23116-001
Diener, E., Seligman, M.E.P.: Beyond money: Toward an economy of well-being. Psychological Science in the Public Interest, Supplement , 5 (1). (2004a). https://www.scopus.com/inward/record.uri?eid=2-s2.0-3142774261&partnerID=40&md5=e86b2c930837502a9ce9cbd057c0df82
Diener, E., Seligman, M.E.P.: Beyond money: Toward an economy of well-being. Psychol. Sci. Public. Interest. 5 (1), 1–31 (2004b). https://doi.org/10.1111/j.0963-7214.2004.00501001.x
Diener, E., Seligman, M.E.P.: Beyond money: Progress on an economy of well-being. Perspect. Psychol. Sci. 13 (2), 171–175 (2018). https://doi.org/10.1177/1745691616689467
Dolan, P., Peasgood, T., White, M.: Do we really know what makes us happy? A review of the economic literature on the factors associated with subjective well-being. J. Econ. Psychol. 29 (1), 94–122 (2008). https://doi.org/10.1016/J.JOEP.2007.09.001
Donthu, N., Kumar, S., Mukherjee, D., Pandey, N., Lim, W.M.: How to conduct a bibliometric analysis: An overview and guidelines. J. Bus. Res. 133 , 285–296 (2021). https://doi.org/10.1016/j.jbusres.2021.04.070
Easterlin, R.A.: Will raising the incomes of all increase the happiness of all? J. Econ. Behav. Organ. 27 (1), 35–47 (1995). https://doi.org/10.1016/0167-2681(95)00003-B
Easterlin, R.A.: Happiness and economic growth - the evidence. In: Global Handbook of Quality of Life, pp. 283–299. Springer, Netherlands (2015). https://doi.org/10.1007/978-94-017-9178-6_12
Fang, Z., Wang, H., Xue, S., Zhang, F., Wang, Y., Yang, S., Zhou, Q., Cheng, C., Zhong, Y., Yang, Y., Liu, G., Chen, J., Qiu, L., Zhi, Y.: A comprehensive framework for detecting economic growth expenses under ecological economics principles in China. Sustainable Horizons. 4 , 100035 (2022). https://doi.org/10.1016/J.HORIZ.2022.100035
Farooque, M., Zhang, A., Thürer, M., Qu, T., Huisingh, D.: Circular supply chain management: a definition and structured literature review. J. Clean. Prod. 228 , 882–900 (2019). https://doi.org/10.1016/J.JCLEPRO.2019.04.303
Ferriss, A.L.: Approaches to improving the quality of life?: how to enhance the quality of life. 150 (2010)
Fioramonti, L., Coscieme, L., Mortensen, L.F.: From gross domestic product to wellbeing: How alternative indicators can help connect the new economy with the Sustainable Development Goals: Https://Doi.Org/10.1177/2053019619869947 , 6 (3), 207–222. (2019). https://doi.org/10.1177/2053019619869947
Fioramonti, L., Coscieme, L., Mortensen, L.F.: From gross domestic product to wellbeing: How alternative indicators can help connect the new economy with the Sustainable Development Goals. Anthropocene Rev. 6 (3), 207–222 (2019a). https://doi.org/10.1177/2053019619869947
Fioramonti, L., Coscieme, L., Costanza, R., Kubiszewski, I., Trebeck, K., Wallis, S., Roberts, D., Mortensen, L.F., Pickett, K.E., Wilkinson, R., Ragnarsdottír, K.V., McGlade, J., Lovins, H., De Vogli, R.: Wellbeing economy: An effective paradigm to mainstream post-growth policies? Ecol. Econ. 192 , 107261 (2022b). https://doi.org/10.1016/j.ecolecon.2021.107261
Fioramonti, L., Coscieme, L., Costanza, R., Kubiszewski, I., Trebeck, K., Wallis, S., Roberts, D., Mortensen, L.F., Pickett, K.E., Wilkinson, R., Ragnarsdottír, K.V., McGlade, J., Lovins, H., De Vogli, R.: Wellbeing economy: An effective paradigm to mainstream post-growth policies? Ecol. Econ. 192 (2022a). https://doi.org/10.1016/j.ecolecon.2021.107261
Forgeard, M.J.C., Jayawickreme, E., Kern, M.L., Seligman, M.E.P.: Doing the right thing: measuring wellbeing for public policy. Int J Wellbeing. 1 (1), 79–106 (2011). https://doi.org/10.5502/ijw.v1i1.15
Francart, N., Malmqvist, T., Hagbert, P.: Climate target fulfilment in scenarios for a sustainable Swedish built environment beyond growth. Futures. 98 , 1–18 (2018). https://doi.org/10.1016/J.FUTURES.2017.12.001
Franceschet, M.: A cluster analysis of scholar and journal bibliometric indicators. J. Am. Soc. Inform. Sci. Technol. 60 (10), 1950–1964 (2009). https://doi.org/10.1002/ASI.21152
Frey, B.S., Gallus, J.: Happiness policy and economic development. Int. J. Happiness Dev. 1 (1), 102 (2012). https://doi.org/10.1504/IJHD.2012.050835
Frey, B.S., Gallus, J.: Political economy of happiness. Appl. Econ. 45 (30), 4205–4211 (2013a). https://doi.org/10.1080/00036846.2013.778950
Frey, B.S., Gallus, J.: Subjective well-being and policy. Topoi. 32 (2), 207–212 (2013b). https://doi.org/10.1007/S11245-013-9155-1/METRICS
Frey, B.S., Stutzer, A.: Happiness, economy and institutions. Econ. J. 110 (466), 918–938 (2000). https://doi.org/10.1111/1468-0297.00570
Frey, B.S., Stutzer, A.: What can economists learn from Happiness Research? Source: J. Economic Literature. 40 (2), 402–435 (2002). https://doi.org/10.1257/002205102320161320
Frey, B.S., Stutzer, A.: Happiness research: State and prospects. Rev. Soc. Econ. 63 (2), 207–228 (2005). https://doi.org/10.1080/00346760500130366
Frey, B.S., Gallus, J., Steiner, L.: Open issues in happiness research. Int. Rev. Econ. 61 (2), 115–125 (2014). https://doi.org/10.1007/s12232-014-0203-y
Frijters, P., Clark, A.E., Krekel, C., Layard, R.: A happy choice: Wellbeing as the goal of government. Behav. Public. Policy. 4 (2), 126–165 (2020). https://doi.org/10.1017/BPP.2019.39
Galbraith, J.K.: Inequality, unemployment and growth: new measures for old controversies. J. Econ. Inequal. 7 (2), 189–206 (2009). https://doi.org/10.1007/s10888-008-9083-2
Giannetti, B.F., Agostinho, F., Almeida, C.M.V.B., Huisingh, D.: A review of limitations of GDP and alternative indices to monitor human wellbeing and to manage eco-system functionality. J. Clean. Prod. 87 (1), 11–25. (2015). https://doi.org/10.1016/j.jclepro.2014.10.051
Gilead, T.: Education’s role in the economy: towards a new perspective. 47 (4), 457–473. (2016). https://doi.org/10.1080/0305764X.2016.1195790
Griep, Y., Hyde, M., Vantilborgh, T., Bidee, J., De Witte, H., Pepermans, R.: Voluntary work and the relationship with unemployment, health, and well-being: A two-year follow-up study contrasting a materialistic and psychosocial pathway perspective. J. Occup. Health Psychol. 20 (2), 190–204 (2015). https://doi.org/10.1037/A0038342
Hallberg, M., Kullenberg, C.: Happiness studies. Nord. J. Work. Life Stud. 7 (1), 42–50 (2019). https://doi.org/10.5324/NJSTS.V7I1.2530
Helliwell, J., Layard, R., Sachs, J., Neve, J.-E.: World Happiness Report 2021. Happiness and Subjective Well-Being . (2021). https://www.wellbeingintlstudiesrepository.org/hw_happiness/5
Ikeda, D. (2010). A New Humanism: The University Addresses of Daisaku Ikeda - Daisaku Ikeda - Google Books . books.google.co.in/books?hl = en&lr=&id = 17aKDwAAQBAJ&oi = fnd&pg = PP1&ots = gQvBHjJA7P&sig = wVOxQ_XlCIrj39Q08W-kxc_sPjA&redir_esc = y#v = onepage&q&f = false
Inglehart, R., Foa, R., Peterson, C., Welzel, C.: Development, freedom, and rising happiness: a global perspective (1981–2007). 3 (4), 264–285 (2008). https://doi.org/10.1111/j.1745-6924.2008.00078.x
Kahneman, D., Krueger, A.B.: Developments in the measurement of subjective well-being. J. Econ. Perspect. 20 (1), 3–24 (2006). https://doi.org/10.1257/089533006776526030
Keniger, L.E., Gaston, K.J., Irvine, K.N., Fuller, R.A.: What are the benefits of interacting with nature? Int. J. Environ. Res. Public Health. 10 (3), 913–935 (2013). https://doi.org/10.3390/IJERPH10030913
Kinman, G., Jones, F.: A life beyond work? job demands, work-life balance, and wellbeing in UK academics. 17 (1–2), 41–60 (2008). https://doi.org/10.1080/10911350802165478
Kim, K.H., Kang, E., Yun, Y.H.: Public support for health taxes and media regulation of harmful products in South Korea. BMC Public. Health. 19 (1), 1–12 (2019). https://doi.org/10.1186/S12889-019-7044-2/TABLES/5
King, M.F., Renó V.F., Novo, E.M.L.M.: The concept, dimensions and methods of assessment of human well-being within a socioecological context: a literature review. Soc Indic Res. 116 (3), 681–698 (2014). https://doi.org/10.1007/s11205-013-0320-0
Knickel, K., Almeida, A., Galli, F., Hausegger-Nestelberger, K., Goodwin-Hawkins, B., Hrabar, M., Keech, D., Knickel, M., Lehtonen, O., Maye, D., Ruiz-Martinez, I., Šūmane, S., Vulto, H., Wiskerke, J.S.C.: Transitioning towards a sustainable wellbeing economy—implications for rural–urban relations. Land. 10 (5) (2021). https://doi.org/10.3390/land10050512
Kullenberg, C., Nelhans, G.: The happiness turn? Mapping the emergence of happiness studies using cited references. Scientometrics. 103 (2), 615–630 (2015). https://doi.org/10.1007/S11192-015-1536-3/FIGURES/5
Lama, D.: A human approach to world peace: his holiness the Dalai Lama. J. Hum. Values. 18 (2), 91–100 (2012). https://doi.org/10.1177/0971685812454479
Laurent, É., Galli, A., Battaglia, F., Libera Marchiori, D., G., Fioramonti, L.: Toward health-environment policy: Beyond the Rome Declaration. Global Environmental Change , 72 . (2022). https://doi.org/10.1016/j.gloenvcha.2021.102418
Lauzon, C., Stevenson, A., Peel, K., Brinsdon, S.: A “bottom up” health in all policies program: supporting local government wellbeing approaches. Health Promot J Austr. (2023). https://doi.org/10.1002/hpja.712
Lavrov, I.: Prospect as a model of the future in the happiness economy - new normative theory of wellbeing. Published Papers (2010). https://ideas.repec.org/p/rnp/ppaper/che5.html
McKinnon, K., Kennedy, M.: Community economies of wellbeing: How social enterprises contribute to surviving well together. In: Social Enterprise, Health, and Wellbeing: Theory, Methods, and Practice. Taylor and Francis Inc (2021). https://doi.org/10.4324/9781003125976-5
Mengist, W., Soromessa, T., Legese, G.: Method for conducting systematic literature review and meta-analysis for environmental science research. MethodsX. 7 (2020). https://doi.org/10.1016/j.mex.2019.100777
Meredith, J.: Theory building through conceptual methods. Int. J. Oper. Prod. Manage. 13 (5), 3–11 (1993). https://doi.org/10.1108/01443579310028120
Millward-Hopkins, J., Steinberger, J.K., Rao, N.D., Oswald, Y.: Providing decent living with minimum energy: A global scenario. Glob. Environ. Change. 65 , 102168 (2020). https://doi.org/10.1016/J.GLOENVCHA.2020.102168
Moher, D., Shamseer, L., Clarke, M., Ghersi, D., Liberati, A., Petticrew, M., Shekelle, P., Stewart, L.A. Preferred reporting items for systematic review and meta-analysis protocols (PRISMA-P) 2015 statement. Syst. Rev. 4 (1), 1 (2015). https://doi.org/10.1186/2046-4053-4-1
Mongeon, P., Paul-Hus, A.: The journal coverage of web of Science and Scopus: A comparative analysis. Scientometrics. 106 (1), 213–228 (2016). https://doi.org/10.1007/S11192-015-1765-5/FIGURES/6
Musa, H.D., Yacob, M.R., Abdullah, A.M., Ishak, M.Y.: Enhancing subjective well-being through strategic urban planning: Development and application of community happiness index. Sustainable Cities Soc. 38 , 184–194 (2018). https://doi.org/10.1016/J.SCS.2017.12.030
Ni, Z., Yang, J., Razzaq, A.: How do natural resources, digitalization, and institutional governance contribute to ecological sustainability through load capacity factors in highly resource-consuming economies? Resour. Policy. 79 , 103068 (2022). https://doi.org/10.1016/J.RESOURPOL.2022.103068
Nunes, A.R., Lee, K., O’Riordan, T.: The importance of an integrating framework for achieving the sustainable development goals: the example of health and well-being. BMJ Glob Health. 1 (3), e000068 (2016). https://doi.org/10.1136/BMJGH-2016-000068
Ócsai, A.: The future of ecologically conscious business. Palgrave Stud. Sustainable Bus. Association Future Earth. 259–274 (2021). https://doi.org/10.1007/978-3-030-60918-4_7
OECD.: Measuring and assessing well-being in Israel (2016). https://doi.org/10.1787/9789264246034-EN
Oehler-Șincai, I.M.: Well-Being, Quality of Governance, and Employment Policies: International Perspectives. (2023). https://doi.org/10.1080/07360932.2023.2189078
Orekhov, V.D., Prichina, O.S., Loktionova, Y.N., Yanina, O.N., Gusareva, N.B.: Scientific analysis of the happiness index in regard to the human capital development. J. Adv. Res. Dyn. Control Syst. 12 (4 Special Issue), 467–478 (2020). https://doi.org/10.5373/JARDCS/V12SP4/20201512
Oswald, A.J.: Happiness and economic performance. Econ. J. 107 (445), 1815–1831 (1997). https://doi.org/10.1111/J.1468-0297.1997.TB00085.X
Pillay, D.: Happiness, wellbeing and ecosocialism–a radical humanist perspective. Globalizations. 17 (2), 380–396 (2020). https://doi.org/10.1080/14747731.2019.1652470
Roy, M.J.: Towards a ‘Wellbeing Economy’: What Can We Learn from Social Enterprise? 269–284. (2021). https://doi.org/10.1007/978-3-030-68295-8_13
Rubio-Mozos, E., García-Muiña, F.E., Fuentes-Moraleda, L.: Rethinking 21st-century businesses: An approach to fourth sector SMEs in their transition to a sustainable model committed to SDGs. Sustain. (Switzerland). 11 (20) (2019). https://doi.org/10.3390/su11205569
Santini, Z.I., Koyanagi, A., Tyrovolas, S., Mason, C., Haro, J.M.: The association between social relationships and depression: a systematic review. J. Affect. Disord. 175 , 53–65 (2015). https://doi.org/10.1016/J.JAD.2014.12.049
Sangha, K.K., Le Brocque, A., Costanza, R., Cadet-James, Y.: Ecosystems and indigenous well-being: An integrated framework. Global Ecol. Conserv. 4 , 197–206 (2015). https://doi.org/10.1016/J.GECCO.2015.06.008
Schyns, P.: Crossnational differences in happiness: Economic and cultural factors explored. Soc. Indic. Res. 43 (1–2), 3–26 (1998). https://doi.org/10.1023/A:1006814424293/METRICS
Shrivastava, P., Zsolnai, L.: Wellbeing-oriented organizations: Connecting human flourishing with ecological regeneration. Bus. Ethics Environ. Responsib. 31 (2), 386–397 (2022). https://doi.org/10.1111/beer.12421
Skul’skaya, L.V., Shirokova, T.K.: Is it possible to build an economy of happiness? (On the book by E.E. Rumyantseva Economy of Happiness (INFRA-M, Moscow, 2010) [in Russian]). Studies on Russian Economic Development 2010 21:4 , 21 (4), 455–456. (2010). https://doi.org/10.1134/S1075700710040131
Sohn, K.: Considering happiness for economic development: determinants of happiness in Indonesia. SSRN Electronic J. (2010). https://doi.org/10.2139/SSRN.2489785
Spruk, R., Kešeljević, A.: Institutional origins of subjective well-being: estimating the effects of economic freedom on national happiness. J. Happiness Stud. 17 (2), 659–712 (2015). https://doi.org/10.1007/S10902-015-9616-X
Su, Y.S., Lien, D., Yao, Y.: Economic growth and happiness in China: a Bayesian multilevel age-period-cohort analysis based on the CGSS data 2005–2015. Int. Rev. Econ. Finance. 77 , 191–205 (2022). https://doi.org/10.1016/J.IREF.2021.09.018
Stucke, M.E.: Should competition policy promote happiness? Fordham Law Review , 81 (5), 2575–2645. (2013). https://www.scopus.com/inward/record.uri?eid=2-s2.0-84878026480&partnerID=40&md5=66be6f8cd1fe9df6ca216e7724c928b3
van Niekerk, A.: A conceptual framework for inclusive economics. South. Afr. J. Economic Manage. Sci. 22 (1) (2019). https://doi.org/10.4102/sajems.v22i1.2915
Vicente, M.J.V.: How more equal societies reduce stress, restore sanity and improve everyone’s well-being por Richard Wilkinson y Kate Pickett. Sistema: Revista de Ciencias Sociales, ISSN 0210–0223, No 257, 2020, Págs. 135–140 , 257 , 135–140. (2020). https://dialnet.unirioja.es/servlet/articulo?codigo=7999261
Wang, Q., Su, M.: The effects of urbanization and industrialization on decoupling economic growth from carbon emission – a case study of China. Sustain Cities Soc. 51 , 101758 (2019). https://doi.org/10.1016/J.SCS.2019.101758
Wiedenhofer, D., Virág, D., Kalt, G., Plank, B., Streeck, J., Pichler, M., Mayer, A., Krausmann, F., Brockway, P., Schaffartzik, A., Fishman, T., Hausknost, D., Leon-Gruchalski, B., Sousa, T., Creutzig, F., Haberl, H.: A systematic review of the evidence on decoupling of GDP, resource use and GHG emissions, part I: Bibliometric and conceptual mapping. Environ. Res. Lett. 15 (6), 063002 (2020). https://doi.org/10.1088/1748-9326/AB8429
Wolfgramm, R., Spiller, C., Henry, E., Pouwhare, R.: A culturally derived framework of values-driven transformation in Māori economies of well-being (Ngā Hono ōhanga oranga). AlterNative. 16 (1), 18–28 (2020). https://doi.org/10.1177/1177180119885663
Wu, Y., Zhu, Q., Zhu, B.: Decoupling analysis of world economic growth and CO2 emissions: a study comparing developed and developing countries. J. Clean. Prod. 190 , 94–103 (2018) https://doi.org/10.1016/j.jclepro.2018.04.139
Zheng, S., Wang, J., Sun, C., Zhang, X., Kahn, M.E.: Air pollution lowers Chinese urbanites’ expressed happiness on social media. Nat. Hum. Behav. 3 (3), 237–243 (2019). https://doi.org/10.1038/s41562-018-0521-2
Download references
The authors declare that no funds, grants, or other support were received during the preparation of this manuscript.
Open access funding provided by Università degli Studi di Salerno within the CRUI-CARE Agreement.
Author information
Authors and affiliations.
Department of Humanities and Social Sciences, Malaviya National Institute of Technology Jaipur, Malaviya Nagar, J.L.N. Marg, Jaipur, Rajasthan, 302017, India
Shruti Agrawal & Nidhi Sharma
Department of Economics and Finance, Birla Institute of Technology and Science (BITS), Pilani, Rajasthan, India
Karambir Singh Dhayal
Department of Economics and Statistics, University of Salerno, Fisciano, Italy
Luca Esposito
Karelian Institute, University of Eastern Finland, Joensuu, Finland
Erasmus Happiness Economics Research Organisation, Erasmus University, Rotterdam, Netherlands
You can also search for this author in PubMed Google Scholar
Contributions
All authors contributed to the study conception and design. Shruti Agrawal: Conceptualization, Material preparation, Data Collection, Formal analysis, Methodology, Writing - Original Draft, Review and Editing. Nidhi Sharma: Validation, Project Administration, Supervision, and Writing - Review & Editing. Karambir Singh Dhayal: Validation, Formal analysis, Methodology, Writing - Review and Editing. Luca Esposito: Validation, Writing - Review and Editing. The first draft of the manuscript was written by Shruti Agrawal and all authors commented on previous versions of the manuscript. All authors read and approved the final manuscript
Corresponding author
Correspondence to Luca Esposito .
Ethics declarations
Ethical approval.
This material is the authors’ own original work, which has not been previously published elsewhere. The paper is not currently being considered for publication elsewhere. The paper reflects the authors’ own research and analysis in a truthful and complete manner.
Competing interests
The authors declare no conflict of interest.
Additional information
Publisher’s note.
Springer Nature remains neutral with regard to jurisdictional claims in published maps and institutional affiliations.
Rights and permissions
Open Access This article is licensed under a Creative Commons Attribution 4.0 International License, which permits use, sharing, adaptation, distribution and reproduction in any medium or format, as long as you give appropriate credit to the original author(s) and the source, provide a link to the Creative Commons licence, and indicate if changes were made. The images or other third party material in this article are included in the article’s Creative Commons licence, unless indicated otherwise in a credit line to the material. If material is not included in the article’s Creative Commons licence and your intended use is not permitted by statutory regulation or exceeds the permitted use, you will need to obtain permission directly from the copyright holder. To view a copy of this licence, visit http://creativecommons.org/licenses/by/4.0/ .
Reprints and permissions
About this article
Agrawal, S., Sharma, N., Dhayal, K.S. et al. From economic wealth to well-being: exploring the importance of happiness economy for sustainable development through systematic literature review. Qual Quant (2024). https://doi.org/10.1007/s11135-024-01892-z
Download citation
Accepted : 21 April 2024
Published : 23 May 2024
DOI : https://doi.org/10.1007/s11135-024-01892-z
Share this article
Anyone you share the following link with will be able to read this content:
Sorry, a shareable link is not currently available for this article.
Provided by the Springer Nature SharedIt content-sharing initiative
- Happiness economy
- Sustainability
- Find a journal
- Publish with us
- Track your research
Thank you for visiting nature.com. You are using a browser version with limited support for CSS. To obtain the best experience, we recommend you use a more up to date browser (or turn off compatibility mode in Internet Explorer). In the meantime, to ensure continued support, we are displaying the site without styles and JavaScript.
- View all journals
- My Account Login
- Explore content
- About the journal
- Publish with us
- Sign up for alerts
- Open access
- Published: 26 May 2024
A double machine learning model for measuring the impact of the Made in China 2025 strategy on green economic growth
- Jie Yuan 1 &
- Shucheng Liu 2
Scientific Reports volume 14 , Article number: 12026 ( 2024 ) Cite this article
396 Accesses
1 Altmetric
Metrics details
- Environmental economics
- Environmental impact
- Sustainability
The transformation and upgrading of China’s manufacturing industry is supported by smart and green manufacturing, which have great potential to empower the nation’s green development. This study examines the impact of the Made in China 2025 industrial policy on urban green economic growth. This study applies the super-slacks-based measure model to measure cities’ green economic growth, using the double machine learning model, which overcomes the limitations of the linear setting of traditional causal inference models and maintains estimation accuracy under high-dimensional control variables, to conduct an empirical analysis based on panel data of 281 Chinese cities from 2006 to 2021. The results reveal that the Made in China 2025 strategy significantly drives urban green economic growth, and this finding holds after a series of robustness tests. A mechanism analysis indicates that the Made in China 2025 strategy promotes green economic growth through green technology progress, optimizing energy consumption structure, upgrading industrial structure, and strengthening environmental supervision. In addition, the policy has a stronger driving effect for cities with high manufacturing concentration, industrial intelligence, and digital finance development. This study provides valuable theoretical insights and policy implications for government planning to promote high-quality development through industrial policy.
Similar content being viewed by others
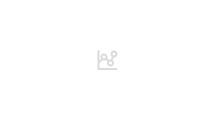
The impact of artificial intelligence on employment: the role of virtual agglomeration
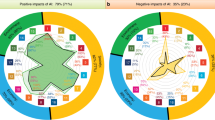
The role of artificial intelligence in achieving the Sustainable Development Goals
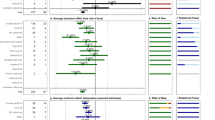
Systematic review and meta-analysis of ex-post evaluations on the effectiveness of carbon pricing
Introduction.
Since China’s reform and opening up, the nation’s economy has experienced rapid growth for more than 40 years. According to the National Bureau of Statistics, China’s per capita GDP has grown from 385 yuan in 1978 to 85,698 yuan in 2022, with an average annual growth rate of 13.2%. However, obtaining this growth miracle has come at considerable social and environmental costs 1 . Current pollution prevention and control systems have not yet fundamentally alleviated the structural and root causes, impairing China’s economic progress toward high-quality development 2 . The report of the 20th National Congress of the Communist Party of China proposed that the future will be focused on promoting the formation of green modes of production and lifestyles and advancing the harmonious coexistence of human beings and nature. This indicates that transforming the mode of economic development is now the focus of the government’s attention, calling for advancing the practices of green growth aimed at energy conservation, emissions reduction, and sustainability while continuously increasing economic output 3 . As a result, identifying approaches to balance economic growth and green environmental protection in the development process and realize green economic growth has become an arduous challenge and a crucially significant concern for China’s high-quality economic development.
An intrinsic driver of urban economic growth, manufacturing is also the most energy-intensive and pollution-emitting industry, and greatly constrains urban green development 4 . China’s manufacturing industry urgently needs to advance the formation of a resource-saving and environmentally friendly industrial structure and manufacturing system through transformation and upgrading to support for green economic growth 5 . As an incentive-based industrial policy that emphasizes an innovation-driven and eco-civilized development path through the development and implementation of an intelligent and green manufacturing system, Made in China 2025 is a significant initiative for promoting the manufacturing industry’s transformation and upgrading, providing solid economic support for green economic growth 6 . To promote the effective implementation of this industrial policy, fully mobilize localities to explore new modes and paths of manufacturing development, and strengthen the urban manufacturing industry’s influential demonstration role in advancing the green transition, the Ministry of Industry and Information Technology of China successively launched 30 Made in China 2025 pilot cities (city clusters) in 2016 and 2017. The Pilot Demonstration Work Program for “Made in China 2025” Cities specified that significant results should be achieved within three to 5 years. After several years of implementation, has the Made in China 2025 pilot policy promoted green economic growth? What are the policy’s mechanisms of action? Are there differences in green economic growth effects in pilot cities based on various urban development characteristics? This study’s theoretical interpretation and empirical examination of the above questions can add to the growing body of related research and provide valuable insights for cities to comprehensively promote the transformation and upgrading of manufacturing industry to advance China’s high-quality development.
This study constructs an analytical framework at the theoretical level to analyze the impact of the Made in China 2025 strategy on urban green economic growth, and uses the double machine learning (ML) model to test its green economic growth effect. The contributions of this study are as follows. First, focusing on the field of urban green development, the study incorporates variables representing the potential economic and environmental effects of the Made in China 2025 policy into a unified framework to systematically examine the impact of the Made in China 2025 pilot policy on the urban green economic growth, providing a novel perspective for assessing the effects of industrial policies. Second, we investigate potential transmission mechanisms of the Made in China 2025 strategy affecting green economic growth from the perspectives of green technology advancement, energy consumption structure optimization, industrial structure upgrading, and environmental supervision strengthening, establishing a useful supplement for related research. Third, leveraging the advantage of ML algorithms in high-dimensional and nonparametric prediction, we apply a double ML model assess the policy effects of the Made in China 2025 strategy to avoid the “curse of dimensionality” and the inherent biases of traditional econometric models, and improve the credibility of our research conclusions.
The remainder of this paper is structured as follows. Section “ Literature review ” presents a literature review. Section “ Policy background and theoretical analysis ” details our theoretical analysis and research hypotheses. Section “ Empirical strategy ” introduces the model setting and variables selection for the study. Section “ Empirical result ” describes the findings of empirical testing and analyzes the results. Section “ Conclusion and policy recommendation ” summarizes our conclusions and associated policy implications.
Literature review
Measurement and influencing factors of green economic growth.
The Green Economy Report, which was published by the United Nations Environment Program in 2011, defined green economy development as facilitating more efficient use of natural resources and sustainable growth than traditional economic models, with a more active role in promoting combined economic development and environmental protection. The Organization for Economic Co-operation and Development defined green economic growth as promoting economic growth while ensuring that natural assets continue to provide environmental resources and services; a concept that is shared by a large number of institutions and scholars 7 , 8 , 9 . A considerable amount of research has assessed green economic growth, primarily using three approaches. First, single-factor indicators, such as sulfur dioxide emissions, carbon dioxide emissions intensity, and other quantified forms; however, this approach neglects the substitution of input factors such as capital and labor for the energy factor, which has certain limitations 5 , 10 . Second, studies have been based on neoclassical economic growth theory, incorporating factors of capital, technology, energy, and the environment, and constructing a green Solow model to measure green total factor productivity (GTFP) 11 , 12 . Third, based on neoclassical economic growth theory, some studies have simultaneously considered desirable and undesirable output, applying Shepard’s distance function, the directional distance function, and data envelopment analysis to measure GTFP 13 , 14 , 15 .
Economic growth is an extremely complex process, and green economic growth is also subject to a combination of multiple complex factors. Scholars have explored the influence mechanisms of green economic growth from perspectives of resource endowment 16 , technological innovation 17 , industrial structure 18 , human capital 19 , financial support 20 , government regulation 21 , and globalization 22 . In the field of policy effect assessment, previous studies have confirmed the green development effects of pilot policies such as innovative cities 23 , Broadband China 24 , smart cities 25 , and low-carbon cities 26 . However, few studies have focused on the impact of Made in China 2025 strategy on urban green economic growth and identified its underlying mechanisms.
The impact of Made in China 2025 strategy
Since the industrial policy of Made in China 2025 was proposed, scholars have predominantly focused on exploring its economic effects on technological innovation 27 , digital transformation 28 , and total factor productivity (TFP) 29 , while the potential environmental effects have been neglected. Chen et al. (2024) 30 found that Made in China 2025 promotes firm innovation through tax incentives, public subsidies, convenient financing, academic collaboration and talent incentives. Xu (2022) 31 point out that Made in China 2025 policy has the potential to substantially improve the green innovation of manufacturing enterprises, which can boost the green transformation and upgrading of China’s manufacturing industry. Li et al. (2024) 32 empirically investigates the positive effect of Made in China 2025 strategy on digital transformation and exploratory innovation in advanced manufacturing firms. Moreover, Liu and Liu (2023) 33 take “Made in China 2025” as an exogenous shock and find that the pilot policy has a positive impact on the high-quality development of enterprises and capital markets. Unfortunately, scholars have only discussed the impact of Made in China 2025 strategy on green development and environmental protection from a theoretical perspective and lack empirical analysis. Li (2018) 27 has compared Germany’s “Industry 4.0” and China’s “Made in China 2025”, and point out that “Made in China 2025” has clear goals, measures and sector focus. Its guiding principles are to enhance industrial capability through innovation-driven manufacturing, optimize the structure of Chinese industry, emphasize quality over quantity, train and attract talent, and achieve green manufacturing and environment. Therefore, it is necessary to systematically explore the impact and mechanism of Made in China 2025 strategy on urban green economic growth from both theoretical and empirical perspectives.
Causal inference based on double ML
The majority of previous studies have used traditional causal inference models to assess policy effects; however, some limitations are inherent to the application of these models. For example, the parallel trend test of the difference-in-differences model has stringent requirements on appropriate sample data; the synthetic control method can construct a virtual control group that conforms to the parallel trend, but it requires that the treatment group does not have the extreme value characteristics, and it is only applicable to “one-to-many” circumstances; and the propensity score matching (PSM) method involves a considerable amount of subjectivity in selecting matching variables. To compensate for the shortcomings of traditional models, scholars have started to explore the application of ML in the field of causal inference 34 , 35 , 36 , and double ML is a typical representative.
Double ML was formalized in 2018 34 , and the relevant research falls into two main categories. The first strand of literature applies double ML to assess causality concerning economic phenomena. Yang et al. (2020) 37 applied double ML using a gradient boosting algorithm to explore the average treatment effect of top-ranked audit firms, verifying its robustness compared with the PSM method. Zhang et al. (2022) 38 used double ML to quantify the impact of nighttime subway services on the nighttime economy, house prices, traffic accidents, and crime following the introduction of nighttime subway services in London in 2016. Farbmacher et al. (2022) 39 combined double ML with mediating effects analysis to assess the causal relationship between health insurance coverage and youth wellness and examine the indirect mechanisms of regular medical checkups, based on a national longitudinal health survey of youth conducted by the US Bureau of Labor Statistics. The second strand of literature has innovated methodological theory based on double ML. Chiang et al. (2022) 40 proposed an improved multidirectional cross-fitting double ML method, obtaining regression results for high-dimensional parameters while estimating robust standard errors for dual clustering, which can effectively adapt to multidirectional clustered sampled data and improve the validity of estimation results. Bodory et al. (2022) 41 combined dynamic analysis with double ML to measure the causal effects of multiple treatment variables over time, using weighted estimation to assess the dynamic treatment effects of specific subsamples, which enriched the dynamic quantitative extension of double ML.
In summary, previous research has conducted some useful investigations regarding the impact of socioeconomic policies on green development, but limited studies have explored the relationship between the Made in China 2025 strategy and green economic growth. This study takes 281 Chinese cities as the research object, and applies the super-slacks-based measure (SBM) model to quantify Chinese cities’ green economic growth from 2006 to 2021. Based on a quasi-natural experiment of Made in China 2025 pilot policy implementation, we use the double ML model to test the impact and transmission mechanisms of the policy on urban green economic growth. We also conduct a heterogeneity analysis of cities based on different levels of manufacturing agglomeration, industrial intelligence, and digital finance. This study applies a novel approach and provides practical insights for research in the field of industrial policy assessment.
Policy background and theoretical analysis
Policy background.
The Made in China 2025 strategy aims to encourage and support local exploration of new paths and models for the transformation and upgrading of the manufacturing industry, and to drive the improvement of manufacturing quality and efficiency in other regions through demonstration effects. According to the Notice of Creating “Made in China 2025” National Demonstration Zones issued by the State Council, municipalities directly under the central government, sub-provincial cities, and prefecture-level cities can apply for the creation of demonstration zones. Cities with proximity and high industrial correlation can jointly apply for urban agglomeration demonstration zones. The Notice clarifies the goals and requirements for creating demonstration zones in areas such as green manufacturing, clean production, and environmental protection. In 2016, Ningbo became the first Made in China 2025 pilot city, and a total of 12 cities and 4 city clusters were included in the list of Made in China 2025 national demonstration zones. In 2018, the State Council issued the Evaluation Guidelines for “Made in China 2025” National Demonstration Zone, which further clarified the evaluation process and indicator system of the demonstration zone. Seven primary indicators and 29 secondary indicators were formulated, including innovation driven, quality first, green development, structural optimization, talent oriented, organizational implementation, and coordinated development of urban agglomerations. This indicator system can evaluate the creation process and overall effectiveness of pilot cities (city clusters), which is beneficial for the promotion of successful experiences and models in demonstration areas.
Advancing green urban development is a complex systematic project that requires structural adjustment and technological and institutional changes in the socioeconomic system 42 . The Made in China 2025 strategy emphasizes the development and application of smart and green manufacturing systems, which can unblock technological bottlenecks in the manufacturing sector in terms of industrial production, energy consumption, and waste emissions, and empower cities to operate in a green manner. In addition, the Made in China 2025 policy established requirements for promoting technological innovation to advance energy saving and environmental protection, improving the rate of green energy use, transforming traditional industries, and strengthening environmental supervision. For pilot cities, green economy development requires the support of a full range of positive factors. Therefore, this study analyzes the mechanisms by which the Made in China 2025 strategy affects urban green economic growth from the four paths of green technology advancement, energy consumption structure optimization, industrial structure upgrading, and environmental supervision strengthening.
Theoretical analysis and research hypotheses
As noted, the Made in China 2025 strategy emphasizes strengthening the development and application of energy-saving and environmental protection technologies to advance cleaner production. Pilot cities are expected to prioritize the driving role of green innovation, promote clustering carriers and innovation platforms for high-tech enterprises, and guide the progress of enterprises’ implementation of green technology. Specifically, pilot cities are encouraged to optimize the innovation environment by increasing scientific and technological investment and financial subsidies in key areas such as smart manufacturing and high-end equipment and strengthening intellectual property protection to incentivize enterprises to conduct green research and development (R&D) activities. These activities subsequently promote the development of green innovation technologies and industrial transformation 43 . Furthermore, since quality human resources are a core aspect of science and technology innovation 44 , pilot cities prioritize the cultivation and attraction of talent to establish a stable human capital guarantee for enterprises’ ongoing green technology innovation, transform and upgrade the manufacturing industry, and advance green urban development. Green technology advances also contribute to urban green economic growth. First, green technology facilitates enterprises’ adoption of improved production equipment and innovation in green production technology, accelerating the change of production mode and driving the transformation from traditional crude production to a green and intensive approach 45 , promoting green urban development. Second, green technology advancement accelerates green innovations such as clean processes, pollution control technologies, and green equipment, and facilitates the effective supply of green products, taking full advantage of the benefits of green innovations 46 and forming a green economic development model to achieve urban green economic growth.
The Made in China 2025 pilot policy endeavors to continuously increase the rate of green and low-carbon energy use and reduce energy consumption. Under target constraints of energy saving and carbon control, pilot cities will accelerate the cultivation of high-tech industries in green environmental protection and high-end equipment manufacturing with advantages of sustainability and low resource inputs 47 to improve the energy consumption structure. Pilot cities also advance new energy sector development by promoting clean energy projects, subsidizing new energy consumption, and supporting green infrastructure construction and other policy measures 48 to optimize the energy consumption structure. Energy consumption structure optimization can have a profound impact on green economy development. Optimization means that available energy tends to be cleaner, which can reduce the manufacturing industry’s dependence on traditional fossil energy and raise the proportion of clean energy 49 , ultimately promoting green urban development. Pilot cities also provide financial subsidies for new energy technology R&D, which promotes the innovation and application of new technologies, energy-saving equipment, efficient resource use, and energy-saving diagnostics, which allow enterprises to save energy and reduce consumption and improve energy use efficiency and TFP 50 , advancing the growth of urban green economy.
At its core, the Made in China 2025 strategy promotes the transformation and upgrading of the manufacturing sector. Pilot cities guide and develop technology-intensive high-tech industries, adjust the proportion of traditional heavy industry, and improve the urban industrial structure. Pilot cities also implement the closure, merger, and transformation of pollution-intensive industries; guide the fission of professional advantages of manufacturing enterprises 51 ; and expand the establishment and development of service-oriented manufacturing and productive service industries to promote the evolution of the industrial structure toward rationalization and high-quality development 52 . Upgrading the industrial structure can also contribute to urban green economic growth. First, industrial structure upgrading promotes the transition from labor- and capital-intensive industries to knowledge- and technology-intensive industries, which optimizes the industrial distribution patterns of energy consumption and pollutant emissions and promotes the transformation of economic growth dynamics and pollutant emissions control, providing a new impetus for cities’ sustainable development 53 . Second, changes in industrial structure and scale can have a profound impact on the type and quantity of pollutant emissions. By introducing high-tech industries, service-oriented manufacturing, and production-oriented service industries, pilot cities can promote the transformation of pollution-intensive industries, promoting the adjustment and optimization of industrial structure and scale 54 to achieve the purpose of driving green urban development.
The Made in China 2025 strategy proposes strengthening green supervision and conducting green evaluations, establishing green development goals for the manufacturing sector in terms of emissions and consumption reduction and water conservation. This requires pilot cities to implement stringent environmental regulatory policies, such as higher energy efficiency and emissions reduction targets and sewage taxes and charges, strict penalties for excess emissions, and project review criteria 55 , which consolidates the effectiveness of green development. Under the framework of environmental authoritarianism, strengthening environmental supervision is a key measure for achieving pollution control and improving environmental quality 56 . Therefore, environmental regulatory enhancement can help cities achieve green development goals. First, according to the Porter hypothesis 57 , strong environmental regulatory policies encourage firms to internalize the external costs of environmental supervision, stimulate technological innovation, and accelerate R&D and application of green technologies. This response helps enterprises improve input–output efficiency, achieve synergy between increasing production and emissions reduction, partially or completely offset the “environmental compliance cost” from environmental supervision, and realize the innovation compensation effect 58 . Second, strict environmental regulations can effectively mitigate the complicity of local governments and enterprises in focusing on economic growth while neglecting environmental protection 59 , urging local governments to constrain enterprises’ emissions, which compels enterprises to conduct technological innovation and pursue low-carbon transformation, promoting urban green economic growth.
Based on the above analysis, we propose the mechanisms that promote green economic growth through Made in China 2025 strategy, as shown in Fig. 1 . The proposed research hypotheses are as follows:
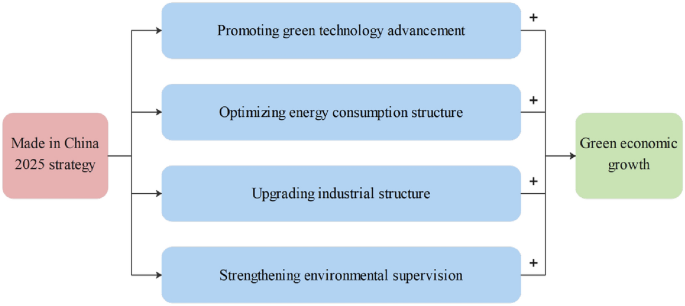
Mechanism analysis of Made in China 2025 strategy and green economic growth.
Hypothesis 1
The Made in China 2025 strategy promotes urban green economic growth.
Hypothesis 2
The Made in China 2025 strategy drives urban green economic growth through four channels: promoting green technology advancement, optimizing energy consumption structure, upgrading industrial structure, and strengthening environmental supervision.
Empirical strategy
Double ml model.
Compared with traditional causal inference models, double ML has unique advantages in variable selection and model estimation, and is also more applicable to the research problem of this study. Green economic growth is a comprehensive indicator of transformative urban growth that is influenced by many socioeconomic factors. To ensure the accuracy of our policy effects estimation, the interference of other factors on urban green economic growth must be controlled as much as possible; however, when introducing high-dimensional control variables, traditional regression models may face the “curse of dimensionality” and multicollinearity, rendering the accuracy of the estimates questionable. Double ML uses ML and regularization algorithms to automatically filter the preselected set of high-dimensional control variables to obtain an effective set of control variables with higher prediction accuracy. This approach avoids the “curse of dimensionality” caused by redundant control variables and mitigates the estimation bias caused by the limited number of primary control variables 39 . Furthermore, nonlinear relationships between variables are the norm in the evolution of economic transition, and ordinary linear regression may suffer from model-setting bias producing estimates that lack robustness. Double ML effectively overcomes the problem of model misspecification by virtue of the advantages of ML algorithms in handling nonlinear data 37 . In addition, based on the idea of instrumental variable functions, two-stage predictive residual regression, and sample split fitting, double ML mitigates the “regularity bias” in ML estimation and ensures unbiased estimates of the treatment coefficients in small samples 60 .
Based on the analysis above, this study uses the double ML model to assess the policy effects of the Made in China 2025 strategy. The partial linear double ML model is constructed as follows:
where i denotes the city, t denotes the year, and Y it represents green economic growth. Policy it represents the policy variable of Made in China 2025, which is set as 1 if the pilot is implemented and 0 otherwise. θ 0 is the treatment coefficient that is the focus of this study. X it denotes the set of high-dimensional control variables, and the ML algorithm is used to estimate the specific functional form \(\hat{g}(X_{it} )\) . U it denotes the error term with a conditional mean of zero.
Direct estimation of Eqs. ( 1 ) and ( 2 ) yields the following estimate of the treatment coefficient:
where n denotes the sample size.
Notably, the double ML model uses a regularization algorithm to estimate the specific functional form \(\hat{g}(X_{it} )\) , which prevents the variance of the estimate from being too large, but inevitably introduces a “regularity bias,” resulting in a biased estimate. To speed up the convergence of the \(\hat{g}(X_{it} )\) directions so that the estimates of the treatment coefficients satisfy unbiasedness with small samples, the following auxiliary regression is constructed:
where \(m(X_{it} )\) is the regression function of the treatment variable on the high-dimensional control variable, using ML algorithms to estimate the specific functional form \(\hat{m}(X_{it} )\) . V it is the error term with a conditional mean of zero.
The specific operation process follows three stages. First, we use the ML algorithm to estimate the auxiliary regression \(\hat{m}(X_{it} )\) and take its residuals \(\hat{V}_{it} = Policy_{it} - \hat{m}(X_{it} )\) . Second, we use the ML algorithm to estimate \(\hat{g}(X_{it} )\) and change the form of the main regression \(Y_{it} - \hat{g}(X_{it} ) = \theta_{0} Policy_{it} + U_{it}\) . Finally, we regress \(\hat{V}_{it}\) as an instrumental variable for Policy it , obtaining unbiased estimates of the treatment coefficients as follows:
Variable selection
- Green economic growth
We apply the super-SBM model to measure urban green economic growth. The super-SBM model is compatible with radial and nonradial characteristics, which avoids inflated results due to ignoring slack variables and deflated results due to ignoring the linear relationships between elements, and can truly reflect relative efficiency 61 . The SBM model reflects the nature of green economic growth more accurately compared with other models, and has been widely adopted by scholars 62 . The expression of the super-SBM model considering undesirable output is as follows:
where x is the input variable; y and z are the desirable and undesirable output variables, respectively; m denotes the number of input indicators; s 1 and s 2 represent the respective number of indicators for desirable and undesirable outputs; k denotes the period of production; i , r , and t are the decision units for the inputs, desirable outputs, and undesirable outputs, respectively; \(s^{ - }\) , \(s^{ + }\) , and \(s^{z - }\) are the respective slack variables for the inputs, desirable outputs, and undesirable outputs; and γ is a vector of weights. A larger \(\rho_{SE}\) value indicates greater efficiency. If \(\rho_{SE}\) = 1, the decision unit is effective; if \(\rho_{SE}\) < 1, the decision unit is relatively ineffective, indicating a loss of efficiency.
Referencing Sarkodie et al. (2023) 63 , the evaluation index system of green economic growth is constructed as shown in Table 1 .

Made in China 2025 pilot policy
The list of Made in China 2025 pilot cities (city clusters) published by the Ministry of Industry and Information Technology of China in 2016 and 2017 is matched with the city-level data to obtain 30 treatment group cities and 251 control group cities. The policy dummy variable of Made in China 2025 is constructed by combining the implementation time of the pilot policies.
Mediating variables
This study also examines the transmission mechanism of the Made in China 2025 strategy affecting urban green economic growth from four perspectives, including green technology advancement, energy consumption structure optimization, industrial structure upgrading, and strengthening of environmental supervision. (1) The number of green patent applications is adopted to reflect green technology advancement. (2) Energy consumption structure is quantified using the share of urban domestic electricity consumption in total energy consumption. (3) The industrial structure upgrading index is calculated using the formula \(\sum\nolimits_{i = 1}^{3} {i \times (GDP_{i} /GDP)}\) , where GDP i denotes the added value of primary, secondary, or tertiary industries. (4) The frequency of words related to the environment in government work reports is the proxy for measuring the intensity of environmental supervision 64 .
Control variables
Double ML can effectively accommodate the case of high-dimensional control variables using regularization algorithms. To control for the effect of other urban characteristics on green economic growth, this study introduces the following 10 control variables. We measure education investment by the ratio of education expenditure to GDP. Technology investment is the ratio of technology expenditure to GDP. The study measures urbanization using the share of urban built-up land in the urban area. Internet penetration is the number of internet users as a share of the total population at the end of the year. We measure resident consumption by the total retail sales of consumer goods per capita. The unemployment rate is the ratio of the number of registered unemployed in urban areas at the end of the year to the total population at the end of the year. Financial scale is the ratio of the balance of deposits and loans of financial institutions at the end of the year to the GDP. Human capital is the natural logarithm of the number of students enrolled in elementary school, general secondary schools, and general tertiary institutions per 10,000 persons. Transportation infrastructure is the natural logarithm of road and rail freight traffic. Finally, openness to the outside world is reflected by the ratio of actual foreign investment to GDP. Quadratic terms for the control variables are also included in the regression analysis to improve the accuracy of the model’s fit. We introduce city and time fixed effects as individual and year dummy variables to avoid missing information on city and time dimensions.
Data sources
This study uses 281 Chinese cities spanning from 2006 to 2021 as the research sample. Data sources include the China City Statistical Yearbook, the China Economic and Social Development Statistics Database, and the EPS Global Statistics Database. We used the average annual growth rate method to fill the gaps for the minimal missing data. To remove the effects of price changes, all data measured in monetary units are deflated using the consumer price index for each province for the 2005 base period. The descriptive statistics of the data are presented in Table 2 .
Empirical result
Baseline results.
The sample split ratio of the double ML model is set to 1:4, and we use the Lasso algorithm to predict and solve the main and auxiliary regressions, presenting the results in Table 3 . Column (1) does not control for fixed effects or control variables, column (2) introduces city and time fixed effects, and columns (3) and (4) add control variables to columns (1) and (2), respectively. The regressions in columns (1) and (2) are highly significant, regardless of whether city and time fixed effects are controlled. Column (4) controls for city fixed effects, time fixed effects, and the primary term of the control variable over the full sample interval, revealing that the regression coefficient of the Made in China 2025 pilot policy on green economic growth is positive and significant at the 1% level, confirming that the Made in China 2025 strategy significantly promotes urban green economic growth. Column (5) further incorporates the quadratic terms of the control variables and the regression coefficients remain significantly positive with little change in values. Therefore, Hypothesis 1 is verified.
Parallel trend test
The prerequisite for the establishment of policy evaluation is that the development status of cities before the pilot policy is introduced is similar. Referring to Liu et al. (2022) 29 , we adopt a parallel trend test to verify the effectiveness of Made in China 2025 pilot policy. Figure 2 shows the result of parallel trend test. None of the coefficient estimates before the Made in China 2025 pilot policy are significant, indicating no significant difference between the level of green economic growth in pilot and nonpilot cities before implementing the policy, which passes the parallel trend test. The coefficient estimates for all periods after the policy implementation are significantly positive, indicating that the Made in China 2025 pilot policy can promote urban green economic growth.
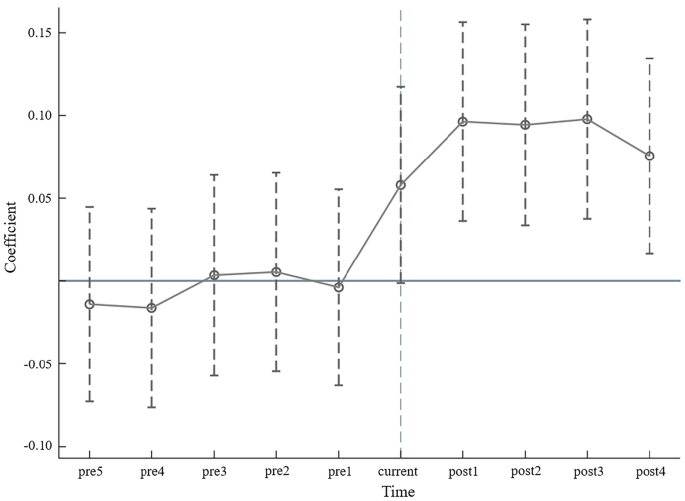
Parallel trend test.
Robustness tests
Replace explained variable.
Referencing Oh and Heshmati (2010) 65 and Tone and Tsutsui (2010) 66 , we use the Malmquist–Luenberger index under global production technology conditions (GML) and an epsilon-based measure (EBM) model to recalculate urban green economic growth. The estimation results in columns (1) and (2) of Table 4 show that the estimated coefficients of the Made in China 2025 pilot policy remain significantly positive after replacing the explanatory variables, validating the robustness of the baseline findings.
Adjusting the research sample
Considering the large gaps in the manufacturing development base between different regions in China, using all cities in the regression analysis may lead to biased estimation 67 . Therefore, we exclude cities in seven provinces with a poor manufacturing development base (Gansu, Qinghai, Ningxia, Xinjiang, Tibet, Yunnan, and Guizhou) and four municipalities with a better development base (Beijing, Tianjin, Shanghai, and Chongqing). The other city samples are retained to rerun the regression analysis, and the results are presented in column (3) of Table 4 . The first batch of pilot cities of the Made in China 2025 strategy was released in 2016, and the second batch of pilot cities was released in 2017. To exclude the effect of point-in-time samples that are far from the time of policy promulgation, the regression is also rerun by restricting the study interval to the three years before and after the promulgation of the policy (2013–2020), and the results are presented in column (4) of Table 4 . The coefficients of the Made in China 2025 pilot policy effect on urban green economic growth decrease after adjusting for the city sample and the time interval, but remain significantly positive at the 1% level. This, once again, verifies the robustness of the benchmark regression results.
Eliminating the impact of potential policies
During the same period of the Made in China 2025 strategy implementation, urban green economy growth may be affected by other relevant policies. To ensure the accuracy of the policy effect estimates, four representative policy categories overlapping with the sample period, including smart cities, low-carbon cities, Broadband China, and innovative cities, were collected and organized. Referencing Zhang and Fan (2023) 25 , dummy variables for these policies are included in the benchmark regression model and the results are presented in Table 5 . The estimated coefficient of the Made in China 2025 pilot policy decreases after controlling for the effects of related policies, but remains significantly positive at the 1% level. This suggests that the positive impact of the Made in China 2025 strategy on urban green economic growth, although overestimated, does not affect the validity of the study’s findings.
Reset double ML model
To avoid the impact of the double ML model imparting bias on the conclusions, we conduct robustness tests by varying the sample splitting ratio, the ML algorithm, and the model estimation form. First, we change the sample split ratio of the double ML model from 1:4 to 3:7 and 1:3. Second, we replace the Lasso ML algorithm with random forest (RF), gradient boosting (GBT), and BP neural network (BNN). Third, we replace the partial linear model based on the dual ML with a more generalized interactive model, using the following main and auxiliary regressions for the analysis:
among them, the meanings of each variable are the same as Eqs. ( 1 ) and ( 2 ).
The estimated coefficients for the treatment effects are obtained from the interactive model as follows:
Table 6 presents the regression results after resetting the double ML model, revealing that the sample split ratio, ML algorithm, and the model estimation form in double ML model did not affect the conclusion that the Made in China 2025 strategy promotes urban green economic growth, and only alters the magnitude of the policy effect, once again validating the robustness of our conclusions.
Difference-in-differences model
To further verify the robustness of the estimation results, we use traditional econometric models for regression. Based on the difference-in-differences (DID) model, a synthetic difference-in-differences (SDID) model is constructed by combining the synthetic control method 68 . It constructs a composite control group with a similar pre-trend to the treatment group by linearly combining several individuals in the control group, and compares it with the treatment group 69 . Table 7 presents the regression results of traditional DID model and SDID model. The estimated coefficient of the Made in China 2025 policy remains significantly positive at the 1% level, which once again verifies the robustness of the study’s findings.
Mechanism verification
This section conducts mechanism verification from four perspectives of green technology advancement, energy consumption structure, industrial structure, and environmental supervision. The positive impacts of the Made in China 2025 strategy on green technology advancement, energy consumption structure optimization, industrial structure upgrading, and strengthening environmental supervision are empirically examined using a dual ML model (see Table A.1 in the Online Appendix for details). Referencing Farbmacher et al. (2022) 39 for causal mediating effect analysis of double ML (see the Appendix for details), we test the transmission mechanism of the Made in China 2025 strategy on green economic growth based on the Lasso algorithm, presenting the results in Table 8 . The findings show that the total effects under different mediating paths are all significantly positive at the 1% level, verifying that the Made in China 2025 strategy positively promotes urban green economic growth.
Mechanism of green technology advancement
The indirect effect of green technological innovation is significantly positive for both the treatment and control groups. After stripping out the path of green technology advancement, the direct effects of the treatment and control groups remain significantly positive, indicating that the increase in the level of green technological innovation brought about by the Made in China 2025 strategy significantly promotes urban green economic growth. The Made in China 2025 strategy proposes to strengthen financial and tax policy support, intellectual property protection, and talent training systems. Through the implementation of policy incentives, pilot cities have fostered the concentration of high-technology enterprises and scientific and technological talent cultivation, exerting a knowledge spillover effect that further promotes green technology advancement. At the same time, policy preferences have stimulated the demand for innovation in energy conservation and emissions reduction, which raises enterprises’ motivation to engage in green innovation activities. Green technology advancement helps cities achieve an intensive development model, bringing multiple dividends such as lower resource consumption, reduced pollution emissions, and improved production efficiency, which subsequently promotes green economic growth.
Mechanism of energy consumption structure
The indirect effect of energy consumption structure is significantly positive for the treatment and control groups, while the direct effect of the Made in China 2025 pilot policy on green economic growth remains significantly positive, indicating that the policy promotes urban green economic growth through energy consumption structure optimization. The policy encourages the introduction of clean energy into production processes, reducing pressure on enterprise performance and the cost of clean energy use, which helps enterprises to reduce traditional energy consumption that is dominated by coal and optimize the energy structure to promote green urban development.
Mechanism of industrial structure
The indirect effects of industrial structure on the treatment and control groups are significantly positive. After stripping out the path of industrial structure upgrading, the direct effects remain significantly positive for both groups, indicating that the Made in China 2025 strategy promotes urban green economic growth through industrial structure optimization. Deepening the restructuring of the manufacturing industry is a strategic task specified in Made in China 2025. Pilot cities focus on transforming and guiding the traditional manufacturing industry toward high-end, intelligent equipment upgrades and digital transformation, driving the regional industrial structure toward rationalization and advancement to achieve rational allocation of resources. Upgrading industrial structure is a prerequisite for cities to advance intensive growth and sustainable development. By assuming the roles of “resource converter” and “pollutant controller,” industrial upgrading can continue to release the dividends of industrial structure, optimize resource allocation, and improve production efficiency, establishing strong support for green economic growth.
Mechanism of environmental supervision
The treatment and control groups of environmental supervision has a positive indirect effect in the process of the Made in China 2025 pilot policy affecting green economic growth that is significant at the 1% level, affirming the transmission path of environmental supervision. The Made in China 2025 strategy states that energy consumption, material consumption, and pollutant emissions per unit of industrial added value in key industries should reach the world’s advanced level by 2025. This requires pilot cities to consolidate and propagate the effectiveness of green development by strengthening environmental supervision while promoting the manufacturing sector’s green development. Strengthening environmental supervision promotes enterprises’ energy saving and emissions reduction through innovative compensation effects, while restraining enterprises’ emissions behaviors by tightening environmental protection policies, promoting environmental legislation, and increasing penalties to advance green urban development. Based on the above analysis, Hypothesis 2 is validated.
Heterogeneity analysis
Heterogeneity of manufacturing agglomeration.
To reduce production and transaction costs and realize economies of scale and scope, the manufacturing industry tends to accelerate its growth through agglomeration, exerting an “oasis effect” 70 . Cities with a high degree of manufacturing agglomeration are prone to scale and knowledge spillover effects, which amplify the agglomeration functions of talent, capital, and technology, strengthening the effectiveness of pilot policies. Based on this, we use the locational entropy of manufacturing employees to measure the degree of urban manufacturing agglomeration in the year (2015) before policy implementation, using the median to divide the full sample of cities into high and low agglomeration groups. Columns (1) and (2) in Table 9 reveal that the Made in China 2025 pilot policy has a stronger effect in promoting green economic growth in cities with high manufacturing concentration compared to those with low concentration. The rationale for this outcome may be that cities with a high concentration of manufacturing industries has large population and developed economy, which is conducive to leveraging agglomeration economies and knowledge spillover effects. Meanwhile, they are able to offer greater policy concessions by virtue of economic scale, public services, infrastructure, and other advantages. These benefits can attract the clustering of productive services and the influx of innovative elements such as R&D talent, accelerating the transformation and upgrading of the manufacturing industry and the integration and advancement of green technologies, empowering the green urban development.
Heterogeneity of industrial intelligence
As a landmark technology for the integration of the new scientific and technological revolution with manufacturing, industrial intelligence is a new approach for advancing the green transformation of manufacturing production methods. Based on this, we use the density of industrial robot installations to measure the level of industrial intelligence in cities in the year (2015) prior to policy implementation 71 , using the median to classify the full sample of cities into high and low level groups. Columns (3) and (4) in Table 9 reveals that the Made in China 2025 pilot policy has a stronger driving effect on the green economic growth of highly industrial intelligent cities. The rationale for this outcome may be that with the accumulation of smart factories, technologies, and equipment, a high degree of industrial intelligence is more likely to leverage the green development effects of pilot policies. For cities where the development of industrial intelligence is in its infancy or has not yet begun, the cost of information and knowledge required for enterprises to undertake technological R&D is higher, reducing the motivation and incentive to conduct innovative activities, diminishing the pilot policy’s contribution to green economic growth.
Heterogeneity of digital finance
As a fusion of traditional finance and information technology, digital finance has a positive impact on the development of the manufacturing industry by virtue of its advantages of low financing thresholds, fast mobile payments, and wide range of services 72 . Cities with a high degree of digital finance development have abundant financial resources and well-developed financial infrastructure that provide enterprises with more complete financial services, with subsequent influence on the effects of pilot policies. We use the Peking University Digital Inclusive Finance Index to measure the level of digital financial development in cities in the year (2015) prior to policy implementation, using the median to divide the full sample of cities into high and low level groups. Columns (5) and (6) in Table 9 reveal that the Made in China 2025 pilot policy has a stronger driving effect on the green economic growth of cities with highly developed digital finance. The rationale for this outcome may be that cities with a high degree of digital finance development can fully leverage the universality of financial resources, provide financial supply for environmentally friendly and technology-intensive enterprises, effectively alleviate the mismatch of financial capital supply, and provide financial security for enterprises to conduct green technology R&D. Digital finance also makes enterprises’ information more transparent through a rich array of data access channels, which strengthens government pollution regulation and public environmental supervision and compels enterprises to engage in green technological innovation to promote green economic growth.
Conclusion and policy recommendation
Conclusions.
This study examines the impact of the Made in China 2025 strategy on urban green economic growth using the double ML model based on panel data for 281 Chinese cities from 2006 to 2021. The relevant research results are threefold. First, the Made in China 2025 strategy significantly promotes urban green economic growth; a conclusion that is supported by a series of robustness tests. Second, regarding mechanisms, the Made in China 2025 strategy promotes urban green economic growth through green technology advancement, energy consumption structure optimization, industrial structure upgrading, and strengthening of environmental supervision. Third, the heterogeneity analysis reveals that the Made in China 2025 strategy has a stronger driving effect on green economic growth for cities with a high concentration of manufacturing and high degrees of industrial intelligence and digital finance.
policy recommendations
We next propose specific policy recommendations based on our findings. First, policymakers should summarize the experience of building pilot cities and create a strategic model to advance the transformation and upgrading of the manufacturing industry to drive green urban development. The Made in China 2025 pilot policy effectively promotes green economic growth and highlights the significance of the transformation and upgrading of the manufacturing industry to empower sustainable urban development. The government should strengthen the model and publicize summaries of successful cases of manufacturing development in pilot cities to promote the experience of manufacturing transformation and upgrading by producing typical samples to guide the transformation of the manufacturing industry to intelligence and greening. Policies should endeavor to optimize the industrial structure and production system of the manufacturing industry to create a solid real economy support for high-quality urban development.
Second, policymakers should explore the multidimensional driving paths of urban green economic growth and actively stimulate the green development dividend of pilot policies by increasing support for enterprise-specific technologies, subsidizing R&D in areas of energy conservation and emissions reduction, consumption reduction and efficiency, recycling and pollution prevention, and promoting the progress of green technologies. The elimination of outdated production capacity must be accelerated and the low-carbon transformation of traditional industries must be targeted, while guiding the clustering of high-tech industries, optimizing cities’ industrial structure, and driving industrial structure upgrading. Policymakers can regulate enterprises’ production practices and enhance the effectiveness of environmental supervision by improving the system of environmental information disclosure and mechanisms of rewards and penalties for pollution discharge. In addition, strategies should consider cities’ own resource endowment, promote large-scale production of new energy, encourage enterprises to increase the proportion of clean energy use, and optimize the structure of energy consumption.
Third, policymakers should engage a combination of urban development characteristics and strategic policy implementation to empower green urban development, actively promoting optimization of manufacturing industry structure, and accelerating the development of high-technology industries under the guidance of policies and the market to promote high-quality development and agglomeration of the manufacturing industry. At the same time, the government should strive to popularize the industrial internet, promote the construction of smart factories and the application of smart equipment, increase investment in R&D to advance industrial intelligence, and actively cultivate new modes and forms of industrial intelligence. In addition, new infrastructure construction must be accelerated, the application of information technology must be strengthened, and digital financial services must be deepened to ease the financing constraints for enterprises conducting R&D on green technologies and to help cities develop in a high-quality manner.
Data availability
The datasets used or analysed during the current study are available from the corresponding author on reasonable request.
Cheng, K. & Liu, S. Does urbanization promote the urban–rural equalization of basic public services? Evidence from prefectural cities in China. Appl. Econ. 56 (29), 3445–3459. https://doi.org/10.1080/00036846.2023.2206625 (2023).
Article Google Scholar
Yin, X. & Xu, Z. An empirical analysis of the coupling and coordinative development of China’s green finance and economic growth. Resour. Policy 75 , 102476. https://doi.org/10.1016/j.resourpol.2021.102476 (2022).
Fernandes, C. I., Veiga, P. M., Ferreira, J. J. M. & Hughes, M. Green growth versus economic growth: Do sustainable technology transfer and innovations lead to an imperfect choice?. Bus. Strateg. Environ. 30 (4), 2021–2037. https://doi.org/10.1002/bse.2730 (2021).
Orsatti, G., Quatraro, F. & Pezzoni, M. The antecedents of green technologies: The role of team-level recombinant capabilities. Res. Policy 49 (3), 103919. https://doi.org/10.1016/j.respol.2019.103919 (2020).
Lin, B. & Zhou, Y. Measuring the green economic growth in China: Influencing factors and policy perspectives. Energy 241 (15), 122518. https://doi.org/10.1016/j.energy.2021.122518 (2022).
Fang, M. & Chang, C. L. Nexus between fiscal imbalances, green fiscal spending, and green economic growth: Empirical findings from E-7 economies. Econ. Change Restruct. 55 , 2423–2443. https://doi.org/10.1007/s10644-022-09392-6 (2022).
Qian, Y., Liu, J. & Forrest, J. Y. L. Impact of financial agglomeration on regional green economic growth: Evidence from China. J. Environ. Plan. Manag. 65 (9), 1611–1636. https://doi.org/10.1080/09640568.2021.1941811 (2022).
Awais, M., Afzal, A., Firdousi, S. & Hasnaoui, A. Is fintech the new path to sustainable resource utilisation and economic development?. Resour. Policy 81 , 103309. https://doi.org/10.1016/j.resourpol.2023.103309 (2023).
Ahmed, E. M. & Elfaki, K. E. Green technological progress implications on long-run sustainable economic growth. J. Knowl. Econ. https://doi.org/10.1007/s13132-023-01268-y (2023).
Shen, F. et al. The effect of economic growth target constraints on green technology innovation. J. Environ. Manag. 292 (15), 112765. https://doi.org/10.1016/j.jenvman.2021.112765 (2021).
Zhao, L. et al. Enhancing green economic recovery through green bonds financing and energy efficiency investments. Econ. Anal. Policy 76 , 488–501. https://doi.org/10.1016/j.eap.2022.08.019 (2022).
Ferreira, J. J. et al. Diverging or converging to a green world? Impact of green growth measures on countries’ economic performance. Environ. Dev. Sustain. https://doi.org/10.1007/s10668-023-02991-x (2023).
Article PubMed PubMed Central Google Scholar
Song, X., Zhou, Y. & Jia, W. How do economic openness and R&D investment affect green economic growth?—Evidence from China. Resour. Conserv. Recycl. 149 , 405–415. https://doi.org/10.1016/j.resconrec.2019.03.050 (2019).
Xu, J., She, S., Gao, P. & Sun, Y. Role of green finance in resource efficiency and green economic growth. Resour. Policy 81 , 103349 (2023).
Zhou, Y., Tian, L. & Yang, X. Schumpeterian endogenous growth model under green innovation and its enculturation effect. Energy Econ. 127 , 107109. https://doi.org/10.1016/j.eneco.2023.107109 (2023).
Luukkanen, J. et al. Resource efficiency and green economic sustainability transition evaluation of green growth productivity gap and governance challenges in Cambodia. Sustain. Dev. 27 (3), 312–320. https://doi.org/10.1002/sd.1902 (2019).
Wang, K., Umar, M., Akram, R. & Caglar, E. Is technological innovation making world “Greener”? An evidence from changing growth story of China. Technol. Forecast. Soc. Change 165 , 120516. https://doi.org/10.1016/j.techfore.2020.120516 (2021).
Talebzadehhosseini, S. & Garibay, I. The interaction effects of technological innovation and path-dependent economic growth on countries overall green growth performance. J. Clean. Prod. 333 (20), 130134. https://doi.org/10.1016/j.jclepro.2021.130134 (2022).
Ge, T., Li, C., Li, J. & Hao, X. Does neighboring green development benefit or suffer from local economic growth targets? Evidence from China. Econ. Modell. 120 , 106149. https://doi.org/10.1016/j.econmod.2022.106149 (2023).
Lin, B. & Zhu, J. Fiscal spending and green economic growth: Evidence from China. Energy Econ. 83 , 264–271. https://doi.org/10.1016/j.eneco.2019.07.010 (2019).
Sohail, M. T., Ullah, S. & Majeed, M. T. Effect of policy uncertainty on green growth in high-polluting economies. J. Clean. Prod. 380 (20), 135043. https://doi.org/10.1016/j.jclepro.2022.135043 (2022).
Sarwar, S. Impact of energy intensity, green economy and blue economy to achieve sustainable economic growth in GCC countries: Does Saudi Vision 2030 matters to GCC countries. Renew. Energy 191 , 30–46. https://doi.org/10.1016/j.renene.2022.03.122 (2022).
Park, J. & Page, G. W. Innovative green economy, urban economic performance and urban environments: An empirical analysis of US cities. Eur. Plann. Stud. 25 (5), 772–789. https://doi.org/10.1080/09654313.2017.1282078 (2017).
Feng, Y., Chen, Z. & Nie, C. The effect of broadband infrastructure construction on urban green innovation: Evidence from a quasi-natural experiment in China. Econ. Anal. Policy 77 , 581–598. https://doi.org/10.1016/j.eap.2022.12.020 (2023).
Zhang, X. & Fan, D. Collaborative emission reduction research on dual-pilot policies of the low-carbon city and smart city from the perspective of multiple innovations. Urban Climate 47 , 101364. https://doi.org/10.1016/j.uclim.2022.101364 (2023).
Cheng, J., Yi, J., Dai, S. & Xiong, Y. Can low-carbon city construction facilitate green growth? Evidence from China’s pilot low-carbon city initiative. J. Clean. Prod. 231 (10), 1158–1170. https://doi.org/10.1016/j.jclepro.2019.05.327 (2019).
Li, L. China’s manufacturing locus in 2025: With a comparison of “Made-in-China 2025” and “Industry 4.0”. Technol. Forecast. Soc. Change 135 , 66–74. https://doi.org/10.1016/j.techfore.2017.05.028 (2018).
Wang, J., Wu, H. & Chen, Y. Made in China 2025 and manufacturing strategy decisions with reverse QFD. Int. J. Prod. Econ. 224 , 107539. https://doi.org/10.1016/j.ijpe.2019.107539 (2020).
Liu, X., Megginson, W. L. & Xia, J. Industrial policy and asset prices: Evidence from the Made in China 2025 policy. J. Bank. Finance 142 , 106554. https://doi.org/10.1016/j.jbankfin.2022.106554 (2022).
Chen, K. et al. How does industrial policy experimentation influence innovation performance? A case of Made in China 2025. Humanit. Soc. Sci. Commun. 11 , 40. https://doi.org/10.1057/s41599-023-02497-x (2024).
Article CAS Google Scholar
Xu, L. Towards green innovation by China’s industrial policy: Evidence from Made in China 2025. Front. Environ. Sci. 10 , 924250. https://doi.org/10.3389/fenvs.2022.924250 (2022).
Li, X., Han, H. & He, H. Advanced manufacturing firms’ digital transformation and exploratory innovation. Appl. Econ. Lett. https://doi.org/10.1080/13504851.2024.2305665 (2024).
Liu, G. & Liu, B. How digital technology improves the high-quality development of enterprises and capital markets: A liquidity perspective. Finance Res. Lett. 53 , 103683 (2023).
Chernozhukov, V. et al. Double/debiased machine learning for treatment and structural parameters. Econom. J. 21 (1), C1–C68. https://doi.org/10.1111/ectj.12097 (2018).
Article MathSciNet Google Scholar
Athey, S., Tibshirani, J. & Wager, S. Generalized random forests. Ann. Stat. 47 (2), 1148–1178. https://doi.org/10.1214/18-AOS1709 (2019).
Knittel, C. R. & Stolper, S. Machine learning about treatment effect heterogeneity: The case of household energy use. AEA Pap. Proc. 111 , 440–444 (2021).
Yang, J., Chuang, H. & Kuan, C. Double machine learning with gradient boosting and its application to the Big N audit quality effect. J. Econom. 216 (1), 268–283. https://doi.org/10.1016/j.jeconom.2020.01.018 (2020).
Zhang, Y., Li, H. & Ren, G. Quantifying the social impacts of the London Night Tube with a double/debiased machine learning based difference-in-differences approach. Transp. Res. Part A Policy Pract. 163 , 288–303. https://doi.org/10.1016/j.tra.2022.07.015 (2022).
Farbmacher, H., Huber, M., Lafférs, L., Langen, H. & Spindler, M. Causal mediation analysis with double machine learning. Econom. J. 25 (2), 277–300. https://doi.org/10.1093/ectj/utac003 (2022).
Chiang, H., Kato, K., Ma, Y. & Sasaki, Y. Multiway cluster robust double/debiased machine learning. J. Bus. Econ. Stat. 40 (3), 1046–1056. https://doi.org/10.1080/07350015.2021.1895815 (2022).
Bodory, H., Huber, M. & Lafférs, L. Evaluating (weighted) dynamic treatment effects by double machine learning. Econom. J. 25 (3), 628–648. https://doi.org/10.1093/ectj/utac018 (2022).
Waheed, R., Sarwar, S. & Alsaggaf, M. I. Relevance of energy, green and blue factors to achieve sustainable economic growth: Empirical study of Saudi Arabia. Technol. Forecast. Soc. Change 187 , 122184. https://doi.org/10.1016/j.techfore.2022.122184 (2023).
Taskin, D., Vardar, G. & Okan, B. Does renewable energy promote green economic growth in OECD countries?. Sustain. Account. Manag. Policy J. 11 (4), 771–798. https://doi.org/10.1108/SAMPJ-04-2019-0192 (2020).
Ding, X. & Liu, X. Renewable energy development and transportation infrastructure matters for green economic growth? Empirical evidence from China. Econ. Anal. Policy 79 , 634–646. https://doi.org/10.1016/j.eap.2023.06.042 (2023).
Ferguson, P. The green economy agenda: Business as usual or transformational discourse?. Environ. Polit. 24 (1), 17–37. https://doi.org/10.1080/09644016.2014.919748 (2015).
Pan, D., Yu, Y., Hong, W. & Chen, S. Does campaign-style environmental regulation induce green economic growth? Evidence from China’s central environmental protection inspection policy. Energy Environ. https://doi.org/10.1177/0958305X231152483 (2023).
Zhang, Q., Qu, Y. & Zhan, L. Great transition and new pattern: Agriculture and rural area green development and its coordinated relationship with economic growth in China. J. Environ. Manag. 344 , 118563. https://doi.org/10.1016/j.jenvman.2023.118563 (2023).
Li, J., Dong, K. & Dong, X. Green energy as a new determinant of green growth in China: The role of green technological innovation. Energy Econ. 114 , 106260. https://doi.org/10.1016/j.eneco.2022.106260 (2022).
Herman, K. S. et al. A critical review of green growth indicators in G7 economies from 1990 to 2019. Sustain. Sci. 18 , 2589–2604. https://doi.org/10.1007/s11625-023-01397-y (2023).
Mura, M., Longo, M., Zanni, S. & Toschi, L. Exploring socio-economic externalities of development scenarios. An analysis of EU regions from 2008 to 2016. J. Environ. Manag. 332 , 117327. https://doi.org/10.1016/j.jenvman.2023.117327 (2023).
Huang, S. Do green financing and industrial structure matter for green economic recovery? Fresh empirical insights from Vietnam. Econ. Anal. Policy 75 , 61–73. https://doi.org/10.1016/j.eap.2022.04.010 (2022).
Li, J., Dong, X. & Dong, K. Is China’s green growth possible? The roles of green trade and green energy. Econ. Res.-Ekonomska Istraživanja 35 (1), 7084–7108. https://doi.org/10.1080/1331677X.2022.2058978 (2022).
Zhang, H. et al. Promoting eco-tourism for the green economic recovery in ASEAN. Econ. Change Restruct. 56 , 2021–2036. https://doi.org/10.1007/s10644-023-09492-x (2023).
Article ADS Google Scholar
Ahmed, F., Kousar, S., Pervaiz, A. & Shabbir, A. Do institutional quality and financial development affect sustainable economic growth? Evidence from South Asian countries. Borsa Istanbul Rev. 22 (1), 189–196. https://doi.org/10.1016/j.bir.2021.03.005 (2022).
Yuan, S., Li, C., Wang, M., Wu, H. & Chang, L. A way toward green economic growth: Role of energy efficiency and fiscal incentive in China. Econ. Anal. Policy 79 , 599–609. https://doi.org/10.1016/j.eap.2023.06.004 (2023).
Capasso, M., Hansen, T., Heiberg, J., Klitkou, A. & Steen, M. Green growth – A synthesis of scientific findings. Technol. Forecast. Soc. Change 146 , 390–402. https://doi.org/10.1016/j.techfore.2019.06.013 (2019).
Wei, X., Ren, H., Ullah, S. & Bozkurt, C. Does environmental entrepreneurship play a role in sustainable green development? Evidence from emerging Asian economies. Econ. Res. Ekonomska Istraživanja 36 (1), 73–85. https://doi.org/10.1080/1331677X.2022.2067887 (2023).
Iqbal, K., Sarfraz, M. & Khurshid,. Exploring the role of information communication technology, trade, and foreign direct investment to promote sustainable economic growth: Evidence from Belt and Road Initiative economies. Sustain. Dev. 31 (3), 1526–1535. https://doi.org/10.1002/sd.2464 (2023).
Li, Y., Zhang, J. & Lyu, Y. Toward inclusive green growth for sustainable development: A new perspective of labor market distortion. Bus. Strategy Environ. 32 (6), 3927–3950. https://doi.org/10.1002/bse.3346 (2023).
Chernozhukov, V. et al. Double/Debiased/Neyman machine learning of treatment effects. Am. Econ. Rev. 107 (5), 261–265. https://doi.org/10.1257/aer.p20171038 (2017).
Chen, C. Super efficiencies or super inefficiencies? Insights from a joint computation model for slacks-based measures in DEA. Eur. J. Op. Res. 226 (2), 258–267. https://doi.org/10.1016/j.ejor.2012.10.031 (2013).
Article ADS MathSciNet Google Scholar
Tone, K., Chang, T. & Wu, C. Handling negative data in slacks-based measure data envelopment analysis models. Eur. J. Op. Res. 282 (3), 926–935 (2020).
Sarkodie, S. A., Owusu, P. A. & Taden, J. Comprehensive green growth indicators across countries and territories. Sci. Data 10 , 413. https://doi.org/10.1038/s41597-023-02319-4 (2023).
Jiang, Z., Wang, Z. & Lan, X. How environmental regulations affect corporate innovation? The coupling mechanism of mandatory rules and voluntary management. Technol. Soc. 65 , 101575 (2021).
Oh, D. H. & Heshmati, A. A sequential Malmquist-Luenberger productivity index: Environmentally sensitive productivity growth considering the progressive nature of technology. Energy Econ. 32 (6), 1345–1355. https://doi.org/10.1016/j.eneco.2010.09.003 (2010).
Tone, K. & Tsutsui, M. An epsilon-based measure of efficiency in DEA - A third pole of technical efficiency. Eur. J. Op. Res. 207 (3), 1554–1563. https://doi.org/10.1016/j.ejor.2010.07.014 (2010).
Lv, C., Song, J. & Lee, C. Can digital finance narrow the regional disparities in the quality of economic growth? Evidence from China. Econ. Anal. Policy 76 , 502–521. https://doi.org/10.1016/j.eap.2022.08.022 (2022).
Arkhangelsky, D., Athey, S., Hirshberg, D. A., Imbens, G. W. & Wager, S. Synthetic difference-in-differences. Am. Econ. Rev. 111 (12), 4088–4118 (2021).
Abadie, A., Diamond, A. & Hainmueller, J. Synthetic control methods for comparative case studies: Estimating the effect of California’s tobacco control program. J. Am. Stat. Assoc. 105 (490), 493–505 (2010).
Article MathSciNet CAS Google Scholar
Fang, J., Tang, X., Xie, R. & Han, F. The effect of manufacturing agglomerations on smog pollution. Struct. Change Econ. Dyn. 54 , 92–101. https://doi.org/10.1016/j.strueco.2020.04.003 (2020).
Yang, S. & Liu, F. Impact of industrial intelligence on green total factor productivity: The indispensability of the environmental system. Ecol. Econ. 216 , 108021. https://doi.org/10.1016/j.ecolecon.2023.108021 (2024).
Zhang, P., Wang, Y., Wang, R. & Wang, T. Digital finance and corporate innovation: Evidence from China. Appl. Econ. 56 (5), 615–638. https://doi.org/10.1080/00036846.2023.2169242 (2024).
Download references
Acknowledgements
This work was supported by the Major Program of National Fund of Philosophy and Social Science of China (20&ZD133).
Author information
Authors and affiliations.
School of Public Finance and Taxation, Zhejiang University of Finance and Economics, Hangzhou, 310018, China
School of Economics, Xiamen University, Xiamen, 361005, China
Shucheng Liu
You can also search for this author in PubMed Google Scholar
Contributions
J.Y.: Methodology, Validation. S.L.: Writing - Reviewing and Editing, Validation, Methodology. All authors have read and agreed to the published version of the manuscript.
Corresponding author
Correspondence to Shucheng Liu .
Ethics declarations
Competing interests.
The authors declare no competing interests.
Additional information
Publisher's note.
Springer Nature remains neutral with regard to jurisdictional claims in published maps and institutional affiliations.
Supplementary Information
Supplementary information., rights and permissions.
Open Access This article is licensed under a Creative Commons Attribution 4.0 International License, which permits use, sharing, adaptation, distribution and reproduction in any medium or format, as long as you give appropriate credit to the original author(s) and the source, provide a link to the Creative Commons licence, and indicate if changes were made. The images or other third party material in this article are included in the article's Creative Commons licence, unless indicated otherwise in a credit line to the material. If material is not included in the article's Creative Commons licence and your intended use is not permitted by statutory regulation or exceeds the permitted use, you will need to obtain permission directly from the copyright holder. To view a copy of this licence, visit http://creativecommons.org/licenses/by/4.0/ .
Reprints and permissions
About this article
Cite this article.
Yuan, J., Liu, S. A double machine learning model for measuring the impact of the Made in China 2025 strategy on green economic growth. Sci Rep 14 , 12026 (2024). https://doi.org/10.1038/s41598-024-62916-0
Download citation
Received : 05 March 2024
Accepted : 22 May 2024
Published : 26 May 2024
DOI : https://doi.org/10.1038/s41598-024-62916-0
Share this article
Anyone you share the following link with will be able to read this content:
Sorry, a shareable link is not currently available for this article.
Provided by the Springer Nature SharedIt content-sharing initiative
- Made in China 2025
- Industrial policy
- Double machine learning
- Causal inference
By submitting a comment you agree to abide by our Terms and Community Guidelines . If you find something abusive or that does not comply with our terms or guidelines please flag it as inappropriate.
Quick links
- Explore articles by subject
- Guide to authors
- Editorial policies
Sign up for the Nature Briefing: Anthropocene newsletter — what matters in anthropocene research, free to your inbox weekly.

- Search Menu
- Sign in through your institution
- Advance articles
- Editor's Choice
- State of the art
- Special and virtual issues
- Author Guidelines
- Submission Site
- Open Access
- About Socio-Economic Review
- About the Society for the Advancement of Socio-Economics
- Editorial Board
- Advertising and Corporate Services
- Self-Archiving Policy
- Dispatch Dates
- Journals on Oxford Academic
- Books on Oxford Academic

Article Contents
1. introduction, 2. theoretical considerations and literature review, 3. data and methods, 5. supplementary analyses, 6. conclusions, acknowledgements, supplementary material.
- < Previous
Explaining the training disadvantage of less-educated workers: the role of labor market allocation in international comparison

- Article contents
- Figures & tables
- Supplementary Data
Carla Hornberg, Jan Paul Heisig, Heike Solga, Explaining the training disadvantage of less-educated workers: the role of labor market allocation in international comparison, Socio-Economic Review , Volume 22, Issue 1, January 2024, Pages 195–222, https://doi.org/10.1093/ser/mwad023
- Permissions Icon Permissions
Less-educated workers have the lowest participation rates in job-related further training across the industrialized world, but the extent of their disadvantage varies. Using data on 28 high- and middle-income countries, we assess different explanations for less-educated workers’ training disadvantage relative to intermediate-educated workers, with a focus on the role of labor market allocation (i.e. job tasks, other job features and firm characteristics). Shapley decompositions reveal a broadly similar pattern for all countries: differences in labor market allocation between less- and intermediate-educated workers are more important for explaining the training gap than differences in individual learning disposition (i.e. cognitive skills and motivation to learn). Our analysis further suggests that the training gap is related to educational and labor market institutions and that labor market allocation processes play a key role in mediating any institutional ‘effects’. Strong conclusions regarding the role of institutions are hampered by the small country-level sample, however.
Research consistently shows that less-educated adults (i.e. those who have not completed upper-secondary education) are severely disadvantaged in the labor market in terms of earnings, job security and career development (e.g. Gebel and Giesecke, 2011 ; Abrassart, 2013 ; Heisig et al. , 2019 ). These disadvantages are compounded by the fact that less-educated adults are less likely to participate in job-related further training than workers with higher levels of education ( Bassanini et al. , 2005 ; OECD, 2019a ). As training opportunities arguably constitute a major ‘job perk’ ( Bills and Hodson, 2007 , p. 261), training inequalities can be considered an important dimension of labor market inequality in their own right. Furthermore, since job-related further training is crucial for acquiring, maintaining and enhancing skills ( Desjardins and Rubenson, 2013 ; Cedefop, 2015 ), a lack of training opportunities likely contributes to less-educated workers’ elevated risks of precarious employment, unemployment and even social exclusion ( Dieckhoff et al. , 2007 ). A reduction of inequalities in further training participation therefore has the potential to strengthen both economic competitiveness and social cohesion ( European Commission, 2000 ). But despite resurgent political interest in equalizing access to continuing skill formation, training inequalities as well as country differences in their extent persist ( Dieckhoff et al. , 2007 ).
In this article, we seek to improve our understanding of less-educated workers’ training disadvantage through a comparative analysis of survey data from the Programme for the International Assessment of Adult Competencies (PIAAC; OECD, 2016 ). We focus on job-related non-formal training (NFT), the predominant form of adult education and training ( Cedefop, 2015 ; Desjardins and Ioannidou, 2020 ). Job-related NFT refers to intentional, job-related training activities that are typically provided in the form of classroom instruction, lectures, theoretical and practical courses, seminars or workshops. It is often certified but does not lead to qualifications recognized by national or sub-national education authorities. 1
Recent work on training participation suggests that labor market allocation plays a decisive role in determining access to job-related NFT: Job- and firm-related characteristics—including job tasks, use of technologies, type of work contract and the firm environment—appear to trump any (residual) influences of socio-demographic or attainment-related worker characteristics such as age, education or work experience ( Schindler et al. , 2011 ; Görlitz and Tamm, 2016 ; Mohr et al. , 2016 ; Saar and Räis, 2017 ; Ehlert, 2020 ). Our contribution departs from these findings and seeks to extend them in three main respects.
First, our focus on the training disadvantage of less-educated workers puts inequalities in training participation at the center of the analysis, whereas most previous studies focus on overall access to training and do not explicitly differentiate by the level of education. By the same token, previous work also fails to provide clear evidence on the contributions of differences in labor market allocation and worker characteristics to training inequalities between educational groups.
Second, our data provide a set of high-quality measures capturing workers’ individual learning disposition , most importantly cognitive skills and overall motivation to learn. We thus account for an alternative explanation of training inequalities that are not modeled adequately in previous work. While this is mostly due to data limitations, it does raise concerns that the decisive role of labor market allocation might be an artifact of omitting these alternative factors.
Third, we take a comparative perspective and thus go beyond existing research on the role of labor market allocation for training participation, which has been largely confined to Germany. This focus on a single country raises obvious questions about generalizability, especially given Germany’s rather unique firm-based vocational education and training (VET) system and its occupation-specific, highly credentialized labor market ( Protsch and Solga, 2016 ; Elbers et al. , 2020 ). We also complement the comparative literature on training participation and skill formation systems more broadly which has paid considerable attention to the role of educational and labor market institutions but has largely abstracted away from underlying mechanisms at the level of individual workers ( Estevez-Abe et al. , 2001 ; Roosmaa and Saar, 2012 ; Desjardins and Rubenson, 2013 ; Saar et al. , 2013 ; Vogtenhuber, 2015 ; Desjardins and Ioannidou, 2020 ). Therefore, we do not know how educational and labor market institutions contribute to country differences in training inequalities: Do they do so by shaping the labor market allocation of less-educated vis-à-vis better-educated workers? Or do they rather operate through cognitive skills and learning dispositions more broadly, an alternative that seems plausible for education system characteristics in particular?
In summary, we seek to answer the following two sets of research questions: (i) Does labor market allocation explain the training disadvantage of less-educated workers? Does the finding that labor market allocation is decisive for training participation generalize to countries other than Germany, and is it robust to controlling for differences in individual learning disposition? (ii) Does the size of less-educated workers’ training disadvantage vary according to educational and labor market institutions? And to what extent can such institutional ‘effects’ be attributed to differences in the labor market allocation of less- and better-educated workers?
Our analysis is based on PIAAC data for 33 523 adults in 28 high- and middle-income countries. PIAAC provides high-quality and internationally comparable data on job-related NFT and key explanatory variables, including job tasks, motivation to learn and general cognitive competencies (numeracy and literacy skills). The latter is based on an extensive assessment component rather than the kinds of self-reports or very short tests included in other surveys. They arguably provide the best internationally comparable data on adult skills so far.
We focus on the training disadvantage of less-educated workers (who did not complete upper-secondary education) relative to intermediate-educated workers (who hold an upper-secondary or non-tertiary post-secondary degree). This focus on low-intermediate differences is motivated by the fact that intermediate-educated workers are the main competitors of less-educated workers when it comes to hiring and training opportunities. Higher education graduates, in contrast, tend to be employed in very different labor market segments ( OECD, 2019a , b ).
Our empirical analysis proceeds in two main steps. First, we provide a Shapley decomposition of the training disadvantage of less-educated workers for each country. Our goal here is to quantify the contribution of differences in labor market allocation and learning disposition to the overall training gap. The second step focuses on country-level regressions that relate the training disadvantage of less-educated workers to several labor market and education systems institutions. We again combine these regressions with Shapley decompositions to investigate whether institutional ‘effects’ can be attributed to differences in the labor market allocation and learning disposition of less-educated relative to intermediate-educated workers.
Our main findings are (i) that the training gap between less- and intermediate-educated workers is very substantial in most countries and (ii) that differences in labor market allocation are an important driver of these training inequalities in all countries (while workers’ learning disposition tends to matter less). We also find (iii) that labor market and educational institutions appear to moderate the magnitude of less-educated training disadvantage, although strong conclusions are hampered by the small country-level sample. Finally, (iv) differences in the labor market allocation of less- and intermediate-educated seem to be a major mechanism underlying the associations between institutional characteristics and training inequalities.
Figure 1 presents our stylized model of the interplay between education, labor market allocation and participation in job-related NFT—and of how it might depend on institutional context. In Section 2.1, we first discuss why we expect labor market allocation to mediate the education-training relationship in all countries ( paths a and b ), before turning to the potential moderating role of educational and labor market institutions in Section 2.2 ( paths 1 and 2 ). Section 2.3 then provides a shorter discussion of workers’ learning disposition as an alternative explanation of training inequalities. Section 2.4 distills the theoretical discussion into two broad empirical expectations.

The education-training relationship and the role of labor market allocation.
Note : Authors’ elaboration.
2.1 The role of labor market allocation
As job-related NFT is mostly employer-sponsored ( Cedefop, 2015 ; Desjardins and Ioannidou, 2020 ), differences in training opportunities and participation are often attributed to differences in employers’ expected returns to training investments ( Acemoglu and Pischke, 1998 ; Dieckhoff et al. , 2007 ). 2 For reasons described below, these expected returns likely depend on the types of work people do (i.e. job tasks), other features of the job and employment relationship (e.g. work hours, type of contract) and various organizational/firm characteristics (e.g. public versus private ownership, firm size). Together, these sets of factors describe what a person does on her job, on what (contractual) terms she does it and where she does it (i.e. the work context). We subsume them under the umbrella term ‘labor market allocation’ because they can be understood as the current endpoints of a dynamic (allocation) process of labor market positioning and matching. For each of the three sets of factors— job tasks , other job characteristics and firm characteristics —we now discuss how and why they are plausibly linked to training opportunities.
Job tasks are constitutive of what it means to do a certain job—in fact, jobs have even been defined as ‘bundles of task’ ( Autor and Handel, 2013 , p. 64). They refer to what people actually do when they perform their work, with standard taxonomies separating job tasks into manual versus cognitive tasks on the one hand and into routine versus non-routine tasks on the other ( Autor et al. , 2003 ). Effective job performance requires that workers possess the skills needed to perform their job tasks, which in turn suggests that job task profiles play a key role in determining access to training opportunities ( Schindler et al. , 2011 ). A potential mechanism linking job tasks to demand for continuing skills investments refers to the pace at which job-relevant skills depreciate. Previous research suggests that skills required to perform abstract (i.e. non-routine cognitive) tasks change more rapidly than those needed for manual tasks ( Arthur et al. , 1998 ; Blechinger and Pfeiffer, 2000 ). From the employer’s perspective, expected returns to training should therefore be higher for workers who perform high shares of non-routine cognitive tasks than for workers who mostly perform routine and/or manual tasks ( Schindler et al. , 2011 ; Görlitz and Tamm, 2016 ; Mohr et al. , 2016 ; Ehlert, 2020 ). As less-educated workers predominantly hold low-skilled jobs ( Bassanini et al. , 2005 ; Nedelkoska and Quintini, 2018 ; Heisig et al. , 2019 ) characterized by higher shares of routine and manual tasks ( Autor et al. , 2003 ; Autor and Handel, 2013 ), job tasks likely mediate the education-training relationship ( path a and b in Figure 1 ).
Besides job tasks, there are a number of other job characteristics that might explain the low training participation of less-educated workers. For example, less-educated workers are more often employed in fixed-term or part-time work, in lower-status occupations and less likely to use computers at work ( path a ; OECD, 2019b ). All of these job characteristics are empirically associated with lower participation in job-related NFT ( Bassanini et al. , 2005 ; Schindler et al. , 2011 ; Görlitz and Tamm, 2016 ; Nedelkoska and Quintini, 2018 ). As with job tasks, these associations can be linked to expected-returns-to-training considerations on the employer’s side ( path b ). For example, both part-time work and fixed-term contracts should reduce the total number of work hours that an employer can expect to receive from an employee after the completion of a training measure.
Similar rationales apply to firm characteristics . Less-educated workers are overrepresented in smaller firms and less training-intensive economic sectors ( path a ). Small firms have fewer financial resources for training investments and usually lack human resources departments to organize them. Furthermore, firms in the growing service economy invest less in training because of higher labor turnover and weaker collective bargaining power than, for example, in the public sector ( path b ; Schindler et al. , 2011 ; Mohr et al. , 2016 ; Wotschack, 2020 ).
2.2 The roles of educational and labor market institutions
The above considerations suggest that differences in the labor market allocation of less-educated vis-à-vis intermediate-educated workers will be an important source of education-related training inequalities in most or even all countries ( Figure 1 , paths a and b ). At the same time, we would expect the magnitude of less-educated workers’ training disadvantage to depend on the national context, particularly in terms of labor market and educational institutions. Given the likely importance of labor market allocation for training inequalities, we would further expect such institutional ‘effects’ to partly operate through two (mutually non-exclusive) channels: First, national institutional factors might strengthen or weaken the relationship between educational attainment and labor market allocation ( Figure 1 , path 1 : institutions as moderators of path a ). Second, they might strengthen or weaken the link between labor market allocation and training participation ( Figure 1 , path 2 : institutions as moderators of path b ). We now discuss each of these paths for a variety of labor markets and educational institutions.
As for possible effects of labor market institutions through path 1 , strict employment protection legislation (EPL) has been argued to nurture a labor market dualism between core workers with permanent contracts and ‘secondary’ workers with temporary contracts (see the overview in Bentolila et al. , 2019 ). Less-educated adults are prototypical secondary workers, suggesting that strict EPL reinforces training inequalities by pushing them into unstable and low-quality ‘dead-end’ jobs ( Biegert, 2019 ). Strong trade unions , in contrast, might weaken the link between formal qualifications and labor market allocation by pursuing a broadly egalitarian agenda that includes quality jobs for less-educated workers, particularly in the context of larger firms ( Brunello, 2001 ; Wotschack, 2020 ).
Concerning educational institutions, stratified education systems with early tracking have been argued to reinforce the link between formal qualifications and actual skills, for example, in the form of larger skills gaps between less- and intermediate-educated adults ( Andersen and van de Werfhorst, 2010 ; Heisig and Solga, 2015 ; Heisig, 2018 ; Heisig et al. , 2019 ). Stronger links between formal qualifications and skills—or greater ‘skills transparency’ ( Andersen and van de Werfhorst, 2010 )—should in turn raise the importance of formal qualifications for labor market positioning. This suggests that less-educated workers in countries with early tracking and high-skill transparency will often be relegated to low-skilled jobs ( Heisig et al. , 2019 ; see also Shavit and Müller, 2000 ; Andersen and van de Werfhorst, 2010 ; Bol and van de Werfhorst, 2013 )—and thus jobs that provide fewer incentives and opportunities for further training (see Section 2.1). A strong vocational orientation of upper-secondary education may further amplify this boundary between skilled and low-skilled jobs: As employers can rely on occupationally trained workers for skilled jobs, less-educated workers (who have not completed certified vocational training) tend to be sorted into low-skilled labor market segments ( Shavit and Müller, 2000 ; Bol et al. , 2019 ; Elbers et al. , 2020 ).
Turning to path 2 in Figure 1 (moderation of path b ), the above institutions might also shape training inequalities by strengthening or weakening the link between labor market allocation and opportunities for job-related NFT. Strict EPL raises hiring and firing costs and therefore provides employers with an incentive to rely on the training of their employees rather than external recruitment. Less-educated workers may benefit less from this than intermediate-educated workers, however. Training opportunities may be focused on core workers, while less-educated adults are more often employed on fixed-term contracts. Strong trade unions , by contrast, might weaken the link between labor market positioning and access to training by negotiating inclusive collective agreements that ensure training opportunities also for workers in low-skilled jobs, especially in larger firms ( Wotschack, 2020 ). In addition, strong unions and collective bargaining might have an indirect effect by lowering wage inequality : Previous research indicates that low wage inequality increases employers’ incentive to invest in their workforces, including those in low-skilled jobs because it allows them to reap a greater portion of the resulting productivity increases ( Acemoglu and Pischke, 1999 ; Bassanini et al. , 2005 ).
Turning to the key features of secondary education systems, early tracking and the skills transparency of educational degrees do not seem to have obvious implications for the link between labor market allocation and training opportunities. As for vocational orientation , the Varieties of Capitalism approach ( Hall and Soskice, 2001 ) highlight that most coordinated market economies (CMEs) are strongly reliant on occupational skills for their high-skill equilibrium. These skills are ensured not only through initial VET but also through continued job-related NFT after labor market entry ( Sorge and Streeck, 2018 ). However, given the dualistic nature of labor markets in many of these countries, these investments in further training might be focused on workers in skilled positions and thereby strengthen rather than weaken the link between labor market allocation and training opportunities.
Taken together, these considerations suggest that less-educated workers’ training disadvantage should be larger in countries with lower union density, stricter EPL, higher wage inequality, greater skills transparency, earlier tracking and a stronger vocational orientation of upper- secondary education.
2.3 The role of workers’ learning disposition
Differences in workers’ learning disposition—that is, in their (cognitive) skills and motivation to learn—are another common explanation for the training disadvantage of less-educated workers ( Becker, 1964 ). Employers might be reluctant to invest in workers that seem to lack the skills or motivation needed for a successful learning experience ( Brunello, 2001 ). For similar reasons, less-educated workers might not expect to gain much from attending a training course ( Acemoglu and Pischke, 1999 ; Siebert, 2006 ). These explanations for training inequalities have an empirical basis in that less-educated adults actually tend to have substantially lower cognitive skills than their higher-educated counterparts ( Heisig and Solga, 2015 ). Their general motivation to learn tends to be lower as well, in part because it is an important predictor of educational attainment in the first place (e.g. Doll, 2010 ).
The role of differences in workers’ disposition might again depend on the national institutional context. For example, Heisig and Solga (2015) show that the skills gap between less- and intermediate-educated workers is larger in countries with early tracking education systems, which suggests that skill differentials will be an important source of training inequalities in these countries. A full discussion of plausible institutional moderation is beyond the scope of this article, however, and we consider the corresponding parts of the analysis as explorative.
That being said, our ability to account for workers’ individual learning disposition—facilitated by the unique assessment component of the PIAAC survey (see Section 3 below)—is important. Confounding of the relationship between labor market allocation and training by differences in learning dispositions (e.g. more learning-motivated workers sorting into more skilled jobs) is a plausible concern that previous studies cannot account for due to data limitations. The potentials of our data should not be overstated, however. Our cross-sectional data do not allow us to model potential feedback effects between labor market allocation and workers’ learning disposition. For example, workers’ levels of cognitive skills might be affected by the nature of their job tasks. In such a scenario, skill differentials would partly be a consequence (and mediator) of differences in labor market allocation, rather than a pure confounder. While our results thus need to be interpreted cautiously, we nonetheless consider them important for assessing the robustness of prior findings on the important role of labor market allocation.
2.4 Empirical expectations
Expectation 1: We expect labor market allocation in terms of job tasks, other job features, and firm characteristics to play an important role in accounting for less-educated workers’ training disadvantage in all countries, above and beyond workers’ learning disposition (i.e. their skills and motivation to learn).
Expectation 2: We expect that country-level associations between (educational/labor market) institutions and the low-intermediate training gap are largely attributable to differences in the labor market allocation of less- relative to intermediate-educated workers. Controlling for labor market allocation at the individual level should thus account for a large portion of any institutional ‘effects’ on the training gap that we may find.
3.1 Data and sample
We use data from PIAAC conducted for 24 countries in 2011/12 and for another nine countries in 2014/15 ( OECD, 2013 , 2016 ). Of these, we exclude Australia and Indonesia because they do not provide public use files, Cyprus and Russia because of low data quality, and Singapore because of missing country-level variables. Our country sample comprises the remaining 28 high- and middle-income countries, most of which are members of the OECD (for a list, see Table 2 below).
Country-specific Shapley decompositions of less-educated workers’ training disadvantage (% points)
Notes: Ordered by size of the demographically adjusted training disadvantage (see Figure 2 ). Negative values indicate lower (adjusted) training participation rates for less-educated workers compared to the intermediate-educated group (percentage point difference). Correspondingly, negative (or positive) values of the explained part indicate that compositional differences in labor market allocation or learning disposition contribute to (reduce) the training disadvantage. Contributions are estimated as the combined average marginal contribution of the sets of labor market allocation/learning disposition measures over all possible elimination sequences leading from the fully adjusted to the demographically adjusted training disadvantage (Shapley decomposition). For subsets, see Figure 3 and Supplementary data , Section B.
P < 0.10,
P < 0.05,
P < 0.01,
P < 0.001 (two-tailed tests).
Source: PIAAC, authors’ calculations.
We restrict the sample to 25- to 54-year-old 3 wage and salary workers who have, (i), not completed upper-secondary education or, (ii), completed upper-secondary (including non-tertiary post-secondary) education but no tertiary-level program. We refer to the former group as ‘less-educated’ and to the latter as ‘intermediate-educated’. Employees with a tertiary-level degree are not part of the analysis as they tend to work in very different labor market segments than less-educated workers. We also drop workers who had worked in their current job for less than a year. 4 This ensures that labor market allocation characteristics at the time of interview match the reference period of the training participation measures, which refer to the 12 months before the interview (see below). Finally, we exclude the so-called ‘literacy-related non-respondents’ ( OECD, 2013 ) and 157 cases with incomplete information on sample-defining variables (i.e. age, highest educational degree, employment status and job tenure).
A total of 33 956 cases meet the above sample restrictions. Of these, 1605 (4.7%) have missing information on at least one variable included in the analysis. Our primary missing data approach is to use multiple imputations through chained equations with 10 imputations, one for each ‘plausible value’ (PV) of the PIAAC numeracy score (see Section 3.2 below). However, we had to drop 433 incomplete cases with missing information on the economic sector or foreign-birth/foreign-language status. This was due to persistent convergence problems with the multinomial logistic regressions required to impute these variables. Our final sample consists of 33 523 cases. 5 Section G in the Supplementary data provides further details on the imputation procedure.
3.2 Individual-level measures
The outcome variable is training participation , measured as attendance of job-related NFT in the 12 months before the interview. Appendix Figure A1 shows that job-related NFT is the dominant form of adult training participation for both less- and intermediate-educated workers. We do not consider training intensity in terms of training hours because this information is not available for all countries and would likely lead to similar results ( Bassanini et al. , 2005 ). 6
Our central independent variable is an indicator for being less educated . We follow standard practice and define this as ‘not having completed upper secondary education’. More technically, less-educated workers have formal qualifications corresponding to levels 0 to 2 in the 1997 revision of the International Standard Classification of Education (ISCED-97). Intermediate-educated workers, the comparison group, have qualifications at ISCED-97 levels 3 and 4.
We operationalize labor market allocation with three sets of variables: job tasks , other job characteristics and firm characteristics . Our measures of job tasks build on Ehlert’s (2020) PIAAC-based study and differentiate between abstract (i.e. non-routine cognitive), routine (i.e. routine cognitive) and manual (i.e. routine and non-routine manual) tasks. We use factor analysis with the principal component method (FA-PC) 7 to measure abstract tasks and routine tasks (see Supplementary data, Section A , for items and factor loadings). To capture manual tasks, we include two measures without dimension reduction through factor analysis: the frequency of working physically and the frequency of using hand and finger accuracy.
The set of other job characteristics includes three variables: computer use at work (yes/no), part-time employment (yes/no) and ISEI scores as an indicator of occupational status (International Socio-Economic Index of Occupational Status; Ganzeboom and Treiman, 1996 ). We use ISEI scores based on one-digit 2008 International Standard Classification of Occupation codes because more fine-grained categories are not available for all countries.
The set of firm characteristics includes firm size in terms of the number of employees (five categories), public versus private firm ownership (dummy variable) and eight broad economic sectors following the International Standard Industrial Classification of All Economic Activities (ISIC; United Nations Statistical Division, 2008 ).
We use two measures to capture differences in the learning disposition of workers. For cognitive skills , we use the PIAAC numeracy proficiency scores. They are based on standardized and internationally comparable test items, a unique feature of the survey. Since the PIAAC testing component is more sophisticated and elaborate than in almost any other population-based survey of adults, its length had to be limited in order not to overburden respondents. PIAAC therefore provides 10 so-called PVs whose variability reflects remaining uncertainty about individual proficiency levels ( OECD, 2013 ). We account for these PVs by running all analyses 10 times and combining the PV-specific estimates according to the appropriate rules ( Little and Rubin, 2002 ; for further details, see Section 3.5 below). To assess workers’ motivation to learn , we again use FA-PC to construct an indicator based on four items that express intrinsic motivation for several learning behaviors, as proposed by Gorges et al. (2016 ; see Supplementary data, Section A , for items and factor loadings).
Table 1 presents an overview of the variables used for the five predictor sets (for descriptive statistics, see Supplementary data, Section A ). In addition to these focal individual-level variables, our analysis uses several straightforward measures of key demographic characteristics such as age or gender (see Section 3.4).
Overview of the five sets of individual-level predictors
3.3 Country-level variables
To measure cross-national differences in education systems , we use the external differentiation index as an indicator of early tracking ( Bol and van de Werfhorst, 2013 ), the percentage of students attending vocational programs in upper-secondary education as a measure of vocational orientation ( Bol and van de Werfhorst, 2013 ) and the skills gap between less- and intermediate-educated adults—calculated from the PIAAC data—as a direct measure of the skills transparency of educational degrees ( Heisig and Solga, 2015 ; Heisig, 2018 ).
Labor market institutions are measured with the OECD indicator of EPL governing the dismissal of workers on regular contracts, union density rates and the ratio of gross earnings at the 50th and 10th percentiles (P50/P10) of full-time employees as an indicator of wage inequality in the lower half of the wage distribution (i.e. where intermediate- and especially less-educated workers are primarily located). 8
Depending on the time of PIAAC data collection (see Section 3.1 above), the country variables refer to 2011/12 or 2014/15, or to the closest year available. More information on the country-level variables, including scores, sources and a correlation matrix, is provided in Appendix Tables A1 and A2 .
3.4 Analytic strategy
This section summarizes the main elements of our empirical strategy. A more detailed, algorithmic description of our approach is provided in Supplementary data, Section H .
A first challenge that we need to address is the different demographic compositions of the two educational groups. While we have no substantive interest in the role of demographic factors, they might nevertheless confound our results. Throughout the analysis, and for each country separately, we therefore reweight intermediate-educated workers to have the same demographic profile as the less-educated group in terms of gender, age, living with partner, having children (aged <13 years), foreign degree and foreign-birth/foreign-language status (for descriptive statistics, see Supplementary data, Section A ). We rely on the entropy balancing method by Hainmueller (2012) to obtain the weights for this compositional adjustment.
Concerning Expectation 1, our goal is to estimate, for each of the 28 countries, (i), the magnitude of the training disadvantage of less-educated workers and, (ii), the extent to which it can be attributed to different aspects of labor market allocation and workers’ learning disposition. Our approach is based on country-specific linear probability models (LPMs) that regress the binary measure of job-related NFT participation on the indicator variable for belonging to the less-educated group and all possible combinations of the five sets of explanatory variables identified above ( Table 1 ). Education coefficients from ‘empty’ LPM specifications that include only the education indicator (and apply the demographic adjustment weights described above) provide estimates of the demographically adjusted training disadvantage. 9 Education coefficients from ‘full’ LPM specifications that include all five sets of explanatory variables provide estimates of the fully adjusted training disadvantage.
The fully adjusted estimate will typically be smaller (in absolute terms) than the demographically adjusted one because group differences in labor market allocation and learning disposition partly account for the training disadvantage of the less educated. To quantify the unique contribution of each of the five sets of explanatory factors, we use a Shapley decomposition ( Shorrocks, 2013 ). In this framework, the contribution of a set of predictors (e.g. job tasks) to the training disadvantage is estimated as the average marginal change in the estimated training gap (i.e. the coefficient on the low education indicator) associated with removing that set of predictors across all possible elimination sequences leading from the full LPM to the empty LPM specification. With five sets of predictors, there are a total of 120 (=5!) elimination sequences.
To assess Expectation 2 on the moderating role of education systems and labor market institutions, we use a two-step approach that connects the country-specific individual-level LPMs described above with linear regressions at the country level. In the first step of the estimation procedure, the LPMs provide us with country-specific estimates of the training disadvantage. In the second step, these estimates then become the dependent variable in a country-level regression with 28 cases. Given this small number of cases, we entered the institutional explanatory variables one at a time. The country-level regressions are fitted by Feasible Generalized Least Squared to account for the fact that the dependent variables are estimated with (sampling) error and therefore heteroscedastic ( Lewis and Linzer, 2005 ). We additionally use HC3 robust standard errors to adjust for any remaining heteroscedasticity ( Long and Ervin, 2000 ). As discussed in Lewis and Linzer (2005) and Heisig et al. (2017) , the two-step approach is a flexible alternative to more widely used mixed-effects (multilevel) models.
If the institutional ‘effects’ on the training disadvantage are attributable to the labor market allocation of less relative to intermediate-educated workers, controlling for the latter in the country-specific (first-step) regressions should lead to a reduction of the coefficients on institutional variables in the country-level (second-step) regressions. For example, if high rates of union density reduce the training disadvantage by reducing education-related inequalities in job tasks, we would expect controlling for job tasks in the first-step regressions to result in a reduction of the union density coefficient in the second-step regression. To explore this possibility, we again conducted Shapley decompositions—where we now decomposed, for each institutional predictor, the difference between the predictor’s coefficient for the demographically adjusted gap and its coefficient for the fully adjusted gap. In so doing, we answer the following question: By how much does removing a set of (individual-level) predictors (e.g. job tasks) from the country-specific (first-step) regressions change the coefficient of an institutional predictor (e.g. union density) in the country-level (second-step) regressions, again averaging across all possible elimination sequences?
3.5 Weighting and variance estimation
Finally, we provide a short summary of our approach to weighting and variance estimation (for further details, see the algorithmic description in the Supplementary data, Section H ). The PIAAC survey comes with sample weights that correct for unequal selection probabilities and selective non-response. We take these weights into account, (i), by applying the unmodified sample weights for less-educated workers and, (ii), by supplying the sample weights as base weights for the reweighting of the socio-demographic composition of intermediate-educated workers through the entropy balancing procedure (see Section 3.4 above).
In addition to the final sample weights, PIAAC provides replicate weights that implement (jackknife) approaches to variance estimation to account for PIAAC’s complex survey design ( OECD, 2016 ). We use these replicate weights to obtain standard errors and confidence intervals for the Shapley-based contributions of the different variable sets in the country-specific regressions. 10 To account for the uncertainty of the imputed missing values and the 10 PVs for workers’ numeracy proficiency, we repeat this procedure for each completed data set and subsequently obtain final point and variance estimates using ‘Rubin’s rules’ ( Little and Rubin, 2002 ).
The above steps account for statistical uncertainty arising from (complex) sampling of individuals within countries and from (imputation) uncertainty in the imputed values and competency scores. When looking at country-level relationships to assess Expectation 2, we additionally need to account for uncertainty introduced by the finite country-level sample. In this step of the analysis, we therefore combine the above procedures with a non-parametric bootstrap with 999 replications. Bootstrap samples are drawn by sampling with replacement from the 28 countries in our analysis.
Figure 2 shows the training disadvantage of less-educated relative to intermediate-educated workers before and after adjustment for differences in labor market allocation (job tasks, other job characteristics and firm characteristics) and workers’ learning disposition (numeracy skills, motivation to learn). While the estimated demographically adjusted training disadvantage (Panel A) is negative in all countries and statistically significant in all but three, its magnitude varies considerably—ranging from −26 percentage points in the Slovak Republic to only −3 percentage points in Japan. 11 The fully adjusted training disadvantage (Panel B) is considerably smaller in all countries and statistically insignificant in most. In a few cases, the point estimate even becomes positive, indicating a training advantage of less-educated workers over observationally similar workers with intermediate levels of education (none of the positive point estimates are statistically significant, however). Estimates for the fully adjusted gap range from −17 percentage points in Lithuania to 9 percentage points in Greece. Labor market allocation and learning disposition combined thus explain large parts of the education-training relationship.

Training disadvantage of less-educated workers relative to intermediate-educated workers in 28 countries (% points).
Notes: Ordered by size of the demographically adjusted training disadvantage. (A) Demographically adjusted gap is adjusted for gender, age, living with partner, having children (age <13 years), foreign degree and foreign-birth/foreign-language status. (B) Fully adjusted training disadvantage additionally accounts for differences in labor market allocation and learning disposition ( Table 1 ). Vertical lines indicate 95% confidence intervals. For country abbreviations and sample sizes, see Table 2 below.
Source: PIAAC, authors’ own calculations.
But what is the role of the individual variable sets? Does labor market allocation really turn out to be important for less-educated workers’ training disadvantage above and beyond individual learning disposition (Expectation 1)? Table 2 provides an answer to this question based on the Shapley approach (see Section 3.4 above). The total explained part is equal to the difference between the demographically adjusted and the fully adjusted gaps presented in Figure 2 . The last two columns of the table decompose the total explained part into the combined contributions of the three sets of the labor market allocation measures (job tasks, other job characteristics and firm characteristics) and of the two learning disposition measures (numeracy skills, motivation to learn). Figure 3 depicts the individual contributions of the five sets of predictors (for the exact values, see Supplementary data, Section B ). Negative values mean that compositional differences with respect to the given set of predictors contribute to less-educated workers’ training disadvantage (i.e. they partly explain the gap). Positive values indicate that compositional differences in a given domain reduce the training disadvantage (i.e. the latter would be even larger in the absence of these compositional differences).
Country-specific Shapley decompositions of less-educated workers’ training disadvantage for the five sets of predictors (% points).
Notes: Ordered by size of the demographically adjusted training disadvantage ( Figure 2 ). Vertical lines indicate 95% confidence intervals based on jackknife replicate weights. Shapley decomposition: contributions of each set are equal to the average marginal contribution to the training disadvantage over all possible elimination sequences leading from the fully adjusted to the demographically adjusted training gap (for variables, see Table 1 ). For exact values, see Supplementary data, Section B .
Table 2 shows that differences in labor market allocation generally make a substantial contribution to less-educated workers’ training disadvantage, above and beyond workers’ learning disposition, in all 28 countries—supporting Expectation 1. In fact, labor market allocation accounts for a larger part of the training gap than workers’ learning disposition in all countries, despite marked institutional differences.
The more detailed decomposition in Figure 3 shows that job characteristics (i.e. computer use, occupational status and part-time employment) tend to be the most important factor in the majority of countries. Only in Lithuania, Slovenia, Austria, Poland, Finland and Belgium differences in job tasks make the largest contribution to the training gap. Firm characteristics are the most important aspect of labor market allocation in only three countries (South Korea, Ireland and Turkey), and there are several countries where they play essentially no role.
Turning to workers’ learning disposition, motivation to learn seems to be at most a minor factor, except in Poland. The contribution of workers’ numeracy skills is small in many countries as well, but there are a few exceptions where these skills do play a substantial and statistically significant role, most importantly Slovak Republic, Germany, and the USA.
We now examine the role of educational and labor market institutions (Expectation 2). Figure 4 graphically summarizes the results (for exact values, see Supplementary data, Section D ). Panel A shows, for each institutional predictor, the coefficient from a country-level regression for the demographically adjusted gap (squares) and from a similar regression for the fully adjusted gap (circles), along with 90% and 95% confidence intervals. Predictors were entered one at a time and are all z -standardized, so coefficient estimates can be interpreted as the predicted percentage point change in the training disadvantage associated with a standard deviation increase in the respective institutional characteristic. Negative (or positive) coefficient estimates imply that the training disadvantage tends to increase (or decrease) as the respective institutional predictor increases.
Country-level regressions of the training disadvantage of less-educated workers and Shapley decomposition results (% points).
Notes: N = 28 countries; for external differentiation N = 26 (w/o Estonia, Lithuania). (A) The coefficient estimates from country-level feasible generalized least squares regressions of the demographically adjusted and fully adjusted training gap on the country-level predictors ( Lewis and Linzer, 2005 ). Country-specific (first-step) regressions for the fully adjusted training gap are reported in the Supplementary data , Section C. Country-level predictors are z-standardized (mean of 0, standard deviation of 1) and entered one at a time (i.e. other country characteristics are not controlled). Thick lines indicate two-sided 90% and thinner extensions indicate two-sided 95% confidence intervals based on heteroscedasticity-consistent (HC3) standard errors ( Long and Ervin, 2000 ). (B) The total difference in coefficient estimates for the demographically adjusted and for the fully adjusted gap and using a Shapley approach to decompose this difference into the contributions of the five sets of individual-level predictors. These contributions are estimated as the average marginal change in the institutional coefficient associated with eliminating a given set of individual-level predictors from the country-specific (first-step) regressions, averaged over all possible elimination sequences leading from the fully adjusted to the demographically adjusted gap. Symbols indicate two-tailed p -values based on a non-parametric cluster bootstrap: + P < 0.10; * P < 0.05. See ‘Data and Methods’ section and Supplementary data , Section H, for further details.
Starting with results for the demographically adjusted training gap and taking the coefficient estimates at face value, a standard deviation increase in union density reduces the training disadvantage of less-educated workers by 1.3 percentage points, while standard deviation increases in EPL and wage inequality raise the training disadvantage by 1.5 and 1.4 percentage points, respectively. Turning to educational institutions, the training disadvantage of less-educated workers increases with the level of skills transparency (2.2 percentage points), external differentiation (2.2 percentage points) and vocational orientation of upper-secondary education (1.4 percentage points). These coefficient estimates generally have the expected sign (see Section 2.2 above), and their magnitude is quite substantial relative to the average demographically adjusted training disadvantage (−15.5 percentage points) and its cross-country standard deviation (5.7 percentage points; see Appendix Table A1 ). However, despite these relatively large effect sizes, only the union density and skills transparency coefficients approach conventional levels of statistical significance ( P < 0.1, as indicated by the 90% confidence intervals, not including zero). When excluding the USA, the most problematic country case according to the outlier analysis in Supplementary data, Section E , both of these coefficients increase in absolute size and become statistically significant at the 5% level (union density: b = 1.5; wage inequality: b = −2.2).
Turning to the fully adjusted training gap, all country-level relationships are weaker (i.e. closer to zero) than for the demographically adjusted training gap and also far from reaching statistical significance. Generally speaking, this indicates that individual-level differences in labor market allocation and learning disposition largely account for any institutional ‘effects’ on the demographically adjusted gap. Panel B in Figure 4 depicts the size of this attenuation and shows that it is statistically significant in four cases: union density, wage inequality, skills transparency and external differentiation (for details on the bootstrapping procedure for assessing statistical significance, see Supplementary data, Section H ).
Which specific individual-level factors play the most important role here? Or to put it differently: Through which individual-level channels do the effects of the institutional predictors operate? To answer these questions, we conducted another set of Shapley decompositions whose results are also reported in Figure 4 , Panel B. We now highlight the main insights from this exercise.
When it comes to labor market institutions, the inequality-reducing effects of higher union density and lower wage inequality appear to stem from less-educated workers’ allocation to more training-active workplaces in terms of firm and other job characteristics (e.g. larger firms, more frequent computer use). For EPL, we find a substantial and statistically significant contribution of job tasks, which appears consistent with the dualization thesis: less-educated workers seem to more often end up in secondary labor market jobs with low skill requirements when EPL is strict.
Turning to educational institutions, any effects of skills transparency and external differentiation on the training disadvantage appear to operate through workers’ skills (which is quite intuitive) but also through job tasks (external differentiation only) and other job characteristics. The coefficient of vocational orientation changes relatively little when differences in labor market allocation and workers’ skills are accounted for, and the detailed decomposition suggests that this is due to offsetting effects of job tasks on one hand and firm characteristics on the other.
Overall, the decomposition results are consistent with Expectation 2 that any effects of educational and labor market institutions on the training disadvantage of less-educated workers operate, to a good extent, through labor market allocation (although, perhaps unsurprisingly, differences in individual learning disposition also seem to play a role in mediating the effects of external differentiation and skills transparency). It bears repeating, however, that these results are based on a small sample and need to be interpreted cautiously. In particular, the associations of the various institutional measures with the demographically adjusted training gap, while meaningful in size, do not attain conventional levels of statistical significance.
We conducted several supplementary analyses to extend the main results and to assess the robustness, especially of the country-level findings. As a first check, we reran our analyses using literacy instead of numeracy proficiency. The results (available upon request) were very similar to the main analyses presented above.
Second, we conducted influence diagnostics for the country-level regressions presented in Figure 4 above. This analysis revealed that the results are not driven by a single country, although—as noted in the previous section—the USA emerged as a somewhat influential case (see Supplementary data, Section E ).
Third, we included two potential confounding factors into our country-level regressions (results available upon request): the share of less-educated among the adult population —because higher shares might decrease less-educated workers’ risk of stigmatization and improve their labor market placement ( Gesthuizen et al. , 2011 )—and the share of unemployed less-educated adults to control for potential cross-national differences in the selectivity of the employed group (our analytic sample). Neither indicator led to substantial changes in the country-level regression results.
Fourth, we explored the country-level correlation between the low-intermediate and the intermediate-tertiary training gap, which turns out to be zero (Pearson’s r of 0.03; see Supplementary data, Section A ). The low-intermediate training gap is thus largely independent of training inequalities at the upper end. This suggests that cross-national variation in the low-intermediate gap is not primarily driven by variation in intermediate-educated workers’ access to training (if this were the case, we should see a negative correlation between the low-intermediate and intermediate-tertiary gaps).
In a final supplementary analysis, we considered a typology-based approach, as commonly used in comparative training research ( Roosmaa and Saar, 2012 ; Desjardins and Rubenson, 2013 ) and the political economy literature more broadly ( Estevez-Abe et al. , 2001 ; Hall and Soskice, 2001 ). More specifically, we explored country variation across a limited set of ‘institutional configurations’ as an alternative to our main analysis, which looks at institutional characteristics one at a time (see Supplementary data, Section F ). We identified configurations by running a cluster analysis on the institutional features considered in our country-level regressions. This inductive approach is more practicable than a theory-guided one because the classification of several countries in our sample is uncertain or contested. A four-cluster solution seemed most appropriate and aligns quite well with standard welfare regime typologies and the Varieties of Capitalism framework ( Hall and Soskice, 2001 ). Country-level regressions revealed that less-educated workers’ (demographically adjusted) training disadvantage is smallest in social-democratic CMEs, largest in conservative CMEs, and somewhere in between in the remaining two groups, European and North American liberal market economies. Shapley decompositions confirmed that labor market allocation remains more important than learning disposition when it comes to explaining cross-national differences in the training gap (here: across clusters). These results (reported in the Supplementary data , Table F3 ) are qualitatively consistent with those in Figure 4 . They also suggest that training disadvantages do not map neatly onto standard political economy classifications in that we find the smallest training inequalities in one type of CMEs (social-democratic CMEs) and the largest in another (conservative CMEs).
Job-related further training is considered important for improving workers’ productivity, employment outcomes and career prospects in an ever-changing world of work. The training disadvantage of less-educated workers thus constitutes an important dimension of labor market inequalities and appears to cement rather than counteract labor market inequalities by the level of formal education. Better understanding the nature and sources of training inequalities is therefore of high interest, both theoretically and policy-wise.
In this article, we have examined the training disadvantage of less-educated relative to intermediate-educated workers across 28 countries, focusing on job-related NFT as the dominant form of continued training in advanced economies. Our analysis generalizes and extends previous results that labor market allocation is decisive in determining workers’ access to training ( Schindler et al. , 2011 ; Görlitz and Tamm, 2016 ; Mohr et al. , 2016 ; Saar and Räis, 2017 ; Ehlert, 2020 ). Despite salient institutional differences, labor market allocation emerges as the key proximate factor shaping the training disadvantage of less-educated workers in all 28 countries under study. In particular, it turns out to be more important for understanding training inequalities than workers’ learning disposition. As for the different dimensions of labor market allocation, what we referred to as ‘other job characteristics’ (computer use, occupational status and part-time employment) emerged as the most important domain, but job tasks (routine, abstract and manual tasks) and firm characteristics (firm size, economic sector, public versus private ownership) are relevant factors, too, with some variation across countries.
Country-level regressions suggest that the magnitude of less-educated workers’ training disadvantage depends on educational and labor market institutions, although strong conclusions are hampered by the limited sample size. That being said, strong unions appear to be associated with smaller training inequalities, while high skills transparency (i.e. a strong association between formal qualifications and actual skills) appears to amplify them, resembling findings for other dimensions of labor market attainment such as occupational status ( Andersen and van de Werfhorst, 2010 ; Gebel and Giesecke, 2011 ; Abrassart, 2013 ; Heisig et al. , 2019 ). Results for other institutional characteristics do not reach statistical significance, although coefficient estimates generally point in the expected direction. Importantly, decomposition results indicate that labor market allocation again is a key channel through which any institutional ‘effects’ operate.
This explanatory salience of labor market allocation carries important insights for the political economy literature on production and skill regimes ( Estevez-Abe et al. , 2001 ; Busemeyer, 2009 ). While this literature points to differences in overall training participation across skill formation regimes, it has paid little attention to training inequalities —especially at the very bottom of the educational ladder, the focus of our study. Our results are consistent with a key tenet of the Varieties of Capitalism literature in that the workplace (i.e. labor market allocation) emerges as the main source of these inequalities. At the same time, our analysis suggests that prominent taxonomies of skill and production regimes are of limited use for understanding how inequalities in adult further training vary across countries. A likely reason is that ‘[adult learning systems] are in general not only less regulated but also less homogeneous than the regular cycle of formal education regarding their institutional structure, function and target groups’ ( Desjardins and Ioannidou, 2020 , p. 146). Thus, our study may inspire the political economy literature to not only focus on initial skill formation but also to deepen the theoretical consideration of further training and of training inequalities between educational groups.
While our study significantly advances our understanding of the factors driving less-educated workers’ training disadvantage, it will not be the final word on the issue. Our cross-sectional, conditioning-on-observables approach does not justify strong causal claims, and the moderate size of our country sample further limits our ability to draw robust conclusions. However, the unique skills assessment component of the PIAAC survey enabled us to control for a potentially important confounder that is not accounted for in most previous studies.
Despite this key strength, our individual-level data also have some weaknesses. A major one is the relative shortage of information on the firm context. We had to rely on respondent reports of some very general firm characteristics (number of employees, broad economic sector and public versus private ownership). The fact that firm characteristics account for a substantial portion of the training disadvantage in at least some countries, despite the relative crudeness of these measures, attests to the importance of the organizational context. Linked employer–employee data providing detailed information on training policies, training infrastructures, recruitment strategies, or the structure of internal labor markets have clear potential to move the literature forward. Unfortunately, such data continue to remain rare—and virtually non-existent when it comes to data sets that are well-suited for cross-national comparisons.
Policy-wise, our findings highlight a vicious cycle: less-educated workers are more likely to be exposed to work situations that require fewer skills investments and provide fewer job-related learning opportunities, which cements and reinforces their poor labor market prospects in the long run. Policies aiming to reduce inequalities in training participation should therefore target training barriers related to the job and firm characteristics—while taking into account country-specific patterns in the relevance of individual labor market allocation characteristics. Promising measures include the reduction of training costs (e.g. through subsidies), provision of information on expected productivity returns, or the promotion of collective agreements in workplaces with limited incentives and infrastructures to train, such as smaller firms.
Examples of job-related NFT are type-writing courses or introductory courses on information technologies. In contrast to NFT, formal training leads to a recognized certificate such as a university degree or a vocational qualification, whereas informal training is also intentional but less organized, occurring in the workplace or the family, for example ( Eurostat, 2006 ).
The following discussion adopts this perspective in that we focus on employer-based explanations for (not) training workers. This is mostly for parsimony, and we do not mean to imply that workers’ agency does not matter. Employer- and worker-focused explanations usually point in the same direction, however, and we cannot disentangle the two sides of the process empirically. For example, longer expected (remaining) tenure with a firm increases incentives to invest in firm-specific skills for both employers and workers.
The age bounds increase cross-national comparability by reducing country differences due to age of labor market entry and retirement.
Further analyses (available upon request) show that less-educated workers are somewhat more likely to be short tenured and that they are also more likely to be employed on a fixed-term/non-standard contract in the 26 countries where this information is available (all except Canada and the USA). This suggests that our estimates of the training gap may be somewhat conservative because fixed-term employees may be less likely to be included in training measures.
Our sample of employed less-educated adults is positively selected in terms of job-related NFT participation (see Appendix, Figure A1 ) or skills (see Online Supplement, Section A). This selectivity indicates that our estimates of training gaps and potential explanations may be somewhat conservative, even though intermediate-educated workers show qualitatively similar selectivity when compared with their non-employed counterparts.
Among the 26 countries that do provide training hours information, training participation rates and average training hours are highly correlated, with (country-level) Pearson correlations of 0.77 for the less-educated and 0.81 for the intermediate-educated group (see Online Supplement, Section A).
With the principal component method, we follow Ehlert (2020) . Robustness checks with other methods, such as the principal factor method (FA-PF) or a principal component analysis, produce almost identical factor scores with Pearson correlations above 0.99.
Due to data limitations, the wage inequality data are not fully comparable and refer to weekly and monthly earnings in 24 countries, to hourly earnings in two countries, and to annual earnings in another two.
For a comparison to the unadjusted training disadvantage (i.e. before accounting for demographic composition), see Online Supplement, Section A.
This means that the entropy balancing procedure is not only carried out for the final sample weights but also for all replicate weights. The resulting demographically-adjusted final sample and replicate weights are then used to calculate final point and variance estimates ( OECD, 2016 ).
For the absolute training participation rates of less- and intermediate-educated workers, see Figure A1 in the Appendix.
A previous version was presented at the 2020 ECSR Online Conference in Amsterdam. We thank all participants, as well as our colleagues at the WZB, in particular Martin Ehlert, Sascha dos Santos and Felix Scholl. We thank the SER editor and the two anonymous reviewers for their thoughtful review and constructive comments.
This research was funded in part by the European Commission for the project ‘Technological inequality—understanding the relation between recent technological innovations and social inequalities (TECHNEQUALITY)’ (Grant Agreement number: 822330—TECHNEQUALITY, H2020-SC6-TRANSFORMATIONS-2018-2019-2020/H2020-SC6-TRANSFORMATIONS-2018).
Supplementary material is available at SOCECO Journal online.
Abrassart A. ( 2013 ) ‘ Cognitive Skills Matter: The Employment Disadvantage of Low-Educated Workers in Comparative Perspective ’, European Sociological Review , 29 , 707 – 719 .
Google Scholar
Acemoglu D. , Pischke J. S. ( 1998 ) ‘ Why Do Firms Train? Theory and Evidence ’, The Quarterly Journal of Economics , 113 , 79 – 119 .
Acemoglu D. , Pischke J. S. ( 1999 ) ‘ Beyond Becker: Training in Imperfect Labour Markets ’, The Economic Journal , 109 , 112 – 142 .
Andersen R. , van de Werfhorst H. G. ( 2010 ) ‘ Education and Occupational Status in 14 Countries ’, The British Journal of Sociology , 61 , 336 – 355 .
Arthur W. Jr. , Bennett W. Jr. , Stanush P. L. , McNelly T. L. ( 1998 ) ‘ Factors That Influence Skill Decay and Retention: A Quantitative Review and Analysis ’, Human Performance , 11 , 57 – 101 .
Autor D. H. , Handel M. J. ( 2013 ) ‘ Putting Tasks to the Test: Human Capital, Job Tasks, and Wages ’, Journal of Labor Economics , 31 , S59 – S96 .
Autor D. H. , Levy F. , Murnane R. J. ( 2003 ) ‘ The Skill Content of Recent Technological Change ’, The Quarterly Journal of Economics , 118 , 1279 – 1333 .
Bassanini A. , Booth A. , Brunello G. , de Paola M. , Leuven E. ( 2005 ) Workplace Training in Europe , IZA Discussion Paper No. 1640, Bonn, Germany, IZA.
Becker G. S. ( 1964 ) Human Capital , New York, NY , Columbia University Press .
Google Preview
Bentolila S. , Dolado J. J. , Jimeno J. F. ( 2019 ) Dual Labour Markets Revisited , CESifo Working Paper No. 7479, Munich, IFO.
Biegert T. ( 2019 ) ‘ Labor Market Institutions, the Insider/Outsider Divide and Social Inequalities in Employment in Affluent Countries ’, Socio-Economic Review , 17 , 255 – 281 .
Bills D. B. , Hodson R. ( 2007 ) ‘ Worker Training: A Review, Critique, and Extension ’, Research in Social Stratification and Mobility , 25 , 258 – 272 .
Blechinger D. , Pfeiffer F. ( 2000 ) ‘Technological Change and Skill Obsolescence: The Case of German Apprenticeship Training’. In Heijke H. , Muysken J. (eds) Education and Training in the Knowledge Based Economy , Houndmills, Basingstoke , Macmillan , pp. 243 – 278 .
Bol T. , Ciocca Eller C. , van de Werfhorst H. G. , DiPrete T. A. ( 2019 ) ‘ School-to-Work Linkages, Educational Mismatches, and Labor Market Outcomes ’, American Sociological Review , 84 , 275 – 307 .
Bol T. , van de Werfhorst H. G. ( 2013 ) ‘ Educational Systems and the Trade-Off between Labor Market Allocation and Equality of Educational Opportunity ’, Comparative Education Review , 57 , 285 – 308 .
Brunello G. ( 2001 ) On the Complementarity between Education and Training in Europe , IZA Discussion Paper No. 309, Bonn, Germany, IZA.
Busemeyer M. R. ( 2009 ) ‘ Asset Specificity, Institutional Complementarities and the Variety of Skill Regimes in Coordinated Market Economies ’, Socio-Economic Review , 7 , 375 – 406 .
Cedefop . ( 2015 ) Job-Related Adult Learning and Continuing Vocational Training in Europe , Cedefop research paper No. 48, Luxembourg, Publications Office of the European Union.
Desjardins R. , Ioannidou A. ( 2020 ) ‘ The Political Economy of Adult Learning Systems ’, Zeitschrift Für Weiterbildungsforschung , 43 , 143 – 168 .
Desjardins R. , Rubenson K. ( 2013 ) ‘ Participation Patterns in Adult Education ’, European Journal of Education , 48 , 262 – 280 .
Dieckhoff M. , Jungblut J. M. , O‘Connell P. J. ( 2007 ) ‘Job-Related Training in Europe’. In Gallie D. (ed) Employment Regimes and the Quality of Work , Oxford , Oxford University Press , pp. 77 – 104 .
Doll B. ( 2010 ) ‘School Dropout’. In Weiner I. B. , Craighead W. E. (eds) The Corsini Encyclopedia of Psychology , Hoboken, NJ , Wiley , pp. 1514 – 1515 .
Ehlert M. ( 2020 ) ‘ No Future, No Training? Explaining Cross-National Variation in the Effect of Job Tasks on Training Participation ’, Kzfss Kölner Zeitschrift Für Soziologie Und Sozialpsychologie , 72 , 483 – 510 .
Elbers B. , Bol T. , DiPrete T. A. ( 2020 ) ‘ Training Regimes and Skill Formation in France and Germany an Analysis of Change between 1970 and 2010 ’, Social Forces , 99 , 1113 – 1114 .
Estevez-Abe M. , Iversen T. , Soskice D. ( 2001 ) ‘Social Protection and the Formation of Skills: A Reinterpretation of the Welfare State’. In Hall P A. , Soskice D. (eds) Varieties of Capitalism , Oxford , Oxford University Press , pp. 145 – 183 .
European Commission . ( 2000 ) Communication from the Commission to the Council, the European Parliament, the Economic and Social Committee and the Committee of Regions: Social Policy Agenda , Brussels , Commission of the European Communities .
Eurostat . ( 2006 ) Classification of Learning Activities–Manual , Luxembourg , Office for Official Publications of the European Union .
Ganzeboom H. B. G. , Treiman D. J. ( 1996 ) ‘ Internationally Comparable Measures of Occupational Status for the 1988 International Standard Classification of Occupations ’, Social Science Research , 25 , 201 – 239 .
Gebel M. , Giesecke J. ( 2011 ) ‘ Labor Market Flexibility and Inequality: The Changing Skill-Based Temporary Employment and Unemployment Risks in Europe ’, Social Forces , 90 , 17 – 39 .
Gesthuizen M. , Solga H. , Kunster R. ( 2011 ) ‘ Context Matters: Economic Marginalisation of Low-Educated Workers in Cross-National Perspective ’, European Sociological Review , 27 , 264 – 280 .
Gorges J. , Maehler D. B. , Koch T. , Offerhaus J. ( 2016 ) ‘ Who Likes to Learn New Things: Measuring Adult Motivation to Learn with PIAAC Data from 21 Countries ’, Large-Scale Assessments in Education , 4 , 9 .
Görlitz K. , Tamm M. ( 2016 ) ‘ Revisiting the Complementarity between Education and Training – the Role of Job Tasks and Firm Effects ’, Education Economics , 24 , 261 – 279 .
Hainmueller J. ( 2012 ) ‘ Entropy Balancing for Causal Effects ’, Political Analysis , 20 , 25 – 46 .
Hall P. A. , Soskice D. ( 2001 ) ‘An Introduction to Varieties of Capitalism’. In Hall P A. , Soskice D. (eds) Varieties of Capitalism , Oxford , Oxford University Press , pp. 1 – 68 .
Heisig J. P. ( 2018 ) ‘ Measuring the Signaling Value of Educational Degrees ’, Large-Scale Assessments in Education , 6 , 9 .
Heisig J. P. , Gesthuizen M. , Solga H. ( 2019 ) ‘ Lack of Skills or Formal Qualifications? New Evidence on Cross-Country Differences in the Labor Market Disadvantage of Less-Educated Adults ’, Social Science Research , 83 , 102314 .
Heisig J. P. , Schaeffer M. , Giesecke J. ( 2017 ) ‘ The Costs of Simplicity: Why Multilevel Models May Benefit from Accounting for Cross-Cluster Differences in the Effects of Controls ’, American Sociological Review , 82 , 796 – 827 .
Heisig J. P. , Solga H. ( 2015 ) ‘ Secondary Education Systems and the General Skills of Less- and Intermediate-Educated Adults ’, Sociology of Education , 88 , 202 – 225 .
Lewis J. B. , Linzer D. A. ( 2005 ) ‘ Estimating Regression Models in Which the Dependent Variable Is Based on Estimates ’, Political Analysis , 13 , 345 – 364 .
Little R. J. A. , Rubin D. B. ( 2002 ) Statistical Analysis with Missing Data , Hoboken, NJ , Wiley .
Long J. S. , Ervin L. H. ( 2000 ) ‘ Using Heteroskedasticity Consistent Standard Errors in the Linear Regression Model ’, The American Statistician , 54 , 217 – 224 .
Mohr S. , Troltsch K. , Gerhards C. ( 2016 ) ‘ Job Tasks and the Participation of Low-Skilled Employees in Employer-Provided Continuing Training in Germany ’, Journal of Education and Work , 29 , 562 – 583 .
Nedelkoska L. , Quintini G. ( 2018 ) Automation, Skills Use and Training , OECD Social, Employment and Migration Working Paper No. 202, Paris, OECD.
OECD . ( 2006 ) Education at a Glance , Paris , OECD .
OECD . ( 2013 ) Skills Outlook 2013: First Results from the Survey of Adult Skills , Paris , OECD .
OECD . ( 2016 ) Technical Report of the Survey of Adult Skills (PIAAC ), 2nd edn, Paris , OECD .
OECD . ( 2019a ) Education at a Glance 2019: OECD Indicators , Paris , OECD .
OECD . ( 2019b ) OECD Employment Outlook 2019 , Paris , OECD .
Protsch P. , Solga H. ( 2016 ) ‘ The Social Stratification of the German VET System ’, Journal of Education and Work , 29 , 637 – 661 .
Roosmaa E. L. , Saar E. ( 2012 ) ‘ Participation in Non-Formal Learning in EU-15 and EU-8 Countries ’, International Journal of Lifelong Education , 31 , 477 – 501 .
Saar E. , Räis M. L. ( 2017 ) ‘ Participation in Job-Related Training in European Countries ’, Journal of Education and Work , 30 , 531 – 551 .
Saar E. , Ure O. B. , Desjardins R. ( 2013 ) ‘ The Role of Diverse Institutions in Framing Adult Learning Systems ’, European Journal of Education , 48 , 213 – 232 .
Schindler S. , Weiss F. , Hubert T. ( 2011 ) ‘ Explaining the Class Gap in Training: The Role of Employment Relations and Job Characteristics ’, International Journal of Lifelong Education , 30 , 213 – 232 .
Shavit Y. , Müller W. ( 2000 ) ‘ Vocational Secondary Education: Where Diversion and Where Safety Net ’, European Societies , 2 , 29 – 50 .
Shorrocks A. F. ( 2013 ) ‘ Decomposition Procedures for Distributional Analysis: A Unified Framework Based on the Shapley Value ’, The Journal of Economic Inequality , 11 , 99 – 126 .
Siebert H. ( 2006 ) Lernmotivation Und Bildungsbeteiligung , Bielefeld, Germany , Bertelsmann .
Sorge A. , Streeck W. ( 2018 ) ‘ Diversified Quality Production: Its Contribution to German Socio-Economic Performance over Time ’, Socio-Economic Review , 16 , 587 – 612 .
United Nations Statistical Division . ( 2008 ) International Standard Industrial Classification of All Economic Activities (ISIC) , New York, NY , United Nations Statistical Division .
Vogtenhuber S. ( 2015 ) ‘ Explaining Country Variation in Employee Training ’, European Sociological Review , 31 , 77 – 90 .
Wotschack P. ( 2020 ) ‘ When Do Companies Train Low-Skilled Workers ’, British Journal of Industrial Relations , 58 , 587 – 616 .

Training participation in adult training (in the last 12 months) of less- and intermediate-educated adults.
Notes: N = 33 523 for the employed sample (Panel A/left column in Panel B), N = 18 512 for the non-employed sample (right column in Panel B). Ordered by country code. Survey weights applied.
Descriptive statistics of the country-level variables used
Notes: Alphabetical order.
Second PIAAC round. Training gap estimates are controlled for socio-demographics by including entropy balancing weights. For the country-level regressions all predictors were z-standardized to have a mean of 0 and a standard deviation of 1.
Sources: 1–2, 6: PIAAC (rounds 1 and 2), authors’ calculations; 3–5, 9–10: OECD online database ( https://stats.oecd.org/ ), measured at time of survey (2011/12 for round 1 and 2014/15 for round 2); 7: Educational Systems Database, version 4 ( Bol and van de Werfhorst, 2013 ); 8: OECD (2006 : Table C2.5) and UNESCO online database ( http://data.uis.unesco.org/ ).
Correlation matrix of country-level variables
Notes: N = 28. For pairwise correlations (7) N = 26 because the index is not available for Estonia and Lithuania, (9) and (10) N = 27 because the shares are not available for Japan.
P < 0.05,
P < 0.01,
P < 0.001.
Source: See Appendix Table A1 .
Supplementary data
Email alerts, citing articles via.
- Contact SASE
- Recommend to your Library
Affiliations
- Online ISSN 1475-147X
- Print ISSN 1475-1461
- Copyright © 2024 Society for the Advancement of Socio-Economics and Oxford University Press
- About Oxford Academic
- Publish journals with us
- University press partners
- What we publish
- New features
- Open access
- Institutional account management
- Rights and permissions
- Get help with access
- Accessibility
- Advertising
- Media enquiries
- Oxford University Press
- Oxford Languages
- University of Oxford
Oxford University Press is a department of the University of Oxford. It furthers the University's objective of excellence in research, scholarship, and education by publishing worldwide
- Copyright © 2024 Oxford University Press
- Cookie settings
- Cookie policy
- Privacy policy
- Legal notice
This Feature Is Available To Subscribers Only
Sign In or Create an Account
This PDF is available to Subscribers Only
For full access to this pdf, sign in to an existing account, or purchase an annual subscription.
This website uses cookies.
By clicking the "Accept" button or continuing to browse our site, you agree to first-party and session-only cookies being stored on your device to enhance site navigation and analyze site performance and traffic. For more information on our use of cookies, please see our Privacy Policy .
Journal of Economic Literature
- JEL December 2021
Vol. 59 No. 4 December 2021
- Download Full Issue PDF (AEA members only)
Find articles in this issue
Front matter, forecasting in the presence of instabilities: how we know whether models predict well and how to improve them, a survey on income inequality in china, mass atrocities and their prevention, ten years of evidence: was fraud a force in the financial crisis, the human tide : a review essay, book review: the great reversal by thomas philippon, "somewhere in the middle you can survive": review of the narrow corridor by daron acemoglu and james robinson, book reviews, annotated listing of new books, jel classification system, doctoral dissertations in economics.

IMAGES
VIDEO
COMMENTS
Journal of Economic Literature. The Journal of Economic Literature (JEL), first published in 1969, is designed to help economists keep abreast of and synthesize the vast flow of literature. Read more about the JEL.
The Journal of Economic Literature (JEL), first published in 1969, is designed to help economists keep abreast of the vast flow of literature.JEL issues contain commissioned, peer-reviewed survey and review articles, book reviews, an annotated bibliography of new books classified by subject matter, and an annual index of dissertations in North American universities.
The Review of Economic Studies remains a highly read and cited publication by researchers, as demonstrated by our latest Impact Factor. To celebrate this continued success, we have curated a free collection of the most cited papers published in recent years. Use this collection to support your current work, or get up to date with important ...
A literature review may be as short as a single sentence or as long as several pages (in which case it is usually presented in a sec-tion of its own headed "Literature Review" or something similar). In many journal articles, the literature review appears as part of the introduction. literature review should do at least four things, the ...
Journal of Economic Literature Vol. 61 No. 4 December 2023 . Download Full Issue PDF (AEA members only) Find articles in this issue ... and Social Class: A Review of Bukodi and Goldthorpe's Social Mobility and Education in Britain. by Kjell G. Salvanes (pp. 1540-78) Book Reviews Ahmed, Faisal Z. Conquests and Rents: A Political Economy of ...
How to Divide When There Isn't Enough: From Aristotle, the Talmud, and Maimonides to the Axiomatics of Resource Allocation. Econometric Society Monographs, no. 62 (pp. 498-500) The Journal of Economic Literature (JEL), first published in 1969, is designed to help economists keep abreast of the vast flow of literature.
In 2021, the Journal of Economic Literature published 26 manuscripts in our Articles section: 14 articles and 12 review essays. This represents an increase of 5 manuscripts compared to 2020. We printed 18 regular book reviews, included 933 book abstracts in the Annotated Listing of New Books in 2021, and we listed 29 pages of PhD dissertation ...
Journal of Economic Literature In 2019, the Journal of Economic Literature published 22 manuscripts in our Articles section: 9 papers and 13 review essays. This represents a decrease of ten manuscripts compared to 2018. The overall page count for the Articles section also decreased in 2019. We printed 27 regular
In this study, we review some of the major works in the literature related to Baker et al.'s (2016) economic policy uncertainty index. The beauty and innovation of Baker et al.'s (2016) index is that most of the factors that affect uncertainty are summed up in one simple index. Moreover, it is publicly available and easy to use.
Journal of Economic Literature In 2017, the Journal of Economic Literature published 36 manuscripts in its Articles sec-tion: 15 papers and 21 review essays. This represents an increase of 8 manuscripts over 2016's total. We also printed 32 regular book reviews. We included 1,723 book annotations in our Annotated Listing of New Books in 2017.
Abstract. This paper provides a comprehensive literature review of the relationship between income inequality and economic growth. In the theoretical literature, we identified various models in which income inequality is linked to economic growth. They include (i) The level of economic development, (ii) The level of technological development ...
The economics of standards pertains to the investigation of economic problems related to standards. It was established as a field of research between 2000 and 2010 and has developed rapidly since. Focusing on the relevant theories, methods, and issues, this article systematically summarizes and evaluates the extant literature on the economics ...
A REVIEW OF ECONOMIC LITERATURE ON FOREIGN DIRECT INVESTMENT David Riker Heather Wickramarachi ECONOMICS WORKING PAPER SERIES Working Paper 2020-04-B U.S. INTERNATIONAL TRADE COMMISSION 500 E Street SW Washington, DC 20436 April 2020 Office of Economics working papers are the result of ongoing professional research of
In recent years, economists have developed new models of endogenous economic growth that consider policy influences on growth and divergent outcomes among countries. . Economic growth : a review of the theoretical and empirical literature
The Journal of Economic Surveys is an international economics journal publishing new ideas in economics, econometrics, economic history and business economics. Abstract The goal of this piece is to survey the developing and rapidly growing literature on the economic consequences of COVID-19 and the governmental responses, and to synthetize the ...
In 2020, the Journal of Economic Literature published 21 manuscripts in our Articles sec-tion: 11 papers, 6 review essays, and 4 sympo-sium contributions. This represents a decrease of one manuscript compared to 2019, but the over-all page count for the Articles section actually increased in 2020. The symposium contributions consisted of two ...
New Horizons in the Economics of Sport. Sports Economics Uncut. New Horizons in the Economics of Sport (pp. 449-450) The Journal of Economic Literature (JEL), first published in 1969, is designed to help economists keep abreast of the vast flow of literature.
A Review of the Theoretical Literature" (Theoretical literature review about the development of economic migration theory from the 1950s to today.) Example literature review #2: "Literature review as a research methodology: An overview and guidelines" ( Methodological literature review about interdisciplinary knowledge acquisition and ...
The present study offers a comprehensive summary of the existing studies on the subject, exploring how a happiness economy framework can help achieve sustainable development. For this purpose, a systematic literature review (SLR) summarised 257 research publications from 1995 to 2023.
Faculty of Economics and Management, University of Szczecin, Szczecin, Poland. Search for more papers by this author. Iwona Bąk, ... The original approach is expressed in the article as a review of literature reviews. As a result of the research conducted using correspondence analysis in the years 2007-2023, 112 publications were isolated ...
Journal of Economic Literature Vol. 60 No. 3 September 2022 . Download Full Issue PDF (AEA members only) Find articles in this issue ... A Review of Barry Eichengreen's The Populist Temptation: Economic Grievance and Political Reaction in the Modern Era. by Konstantin Sonin
Measurement and influencing factors of green economic growth. The Green Economy Report, which was published by the United Nations Environment Program in 2011, defined green economy development as ...
1. Introduction. Research consistently shows that less-educated adults (i.e. those who have not completed upper-secondary education) are severely disadvantaged in the labor market in terms of earnings, job security and career development (e.g. Gebel and Giesecke, 2011; Abrassart, 2013; Heisig et al., 2019).These disadvantages are compounded by the fact that less-educated adults are less likely ...
The PRISMA method and Atlas-ti software are used for thematic analysis. The review highlights important topics such as the development of supply chain management, obstacles in last-mile distribution, the significance of technology, regulatory frameworks, infrastructure improvement, cooperative activities, and the economic and social effects.
A systematic literature review (SLR) was conducted in PubMed and Google Scholar in November 2022. The SLR was performed and reported according to the PRISMA guidelines. Original research studies were included if they reported empirical data on ADCs in ASCs, perioperative areas, and surgical settings.
Journal of Economic Literature Vol. 59 No. 4 December 2021 . Download Full Issue PDF (AEA members only) Find articles in this issue. Title Abstract Author Front Matter (pp. 1-6) ... Book Review: The Great Reversal by Thomas Philippon. by Jan Eeckhout (pp. 1340-60)
Edible landscapes provide a series of benefits for the sustainable development of a city, and therefore, in recent years, their development has become a fashion which has stimulated research. However, there is a need for a comprehensive, integrated, literature review, to increase people's knowledge of edible landscapes for a sustainable future. Therefore, this paper conducts a systematic ...