Operations Management
Browse operations management learning materials including case studies, simulations, and online courses. Introduce core concepts and real-world challenges to create memorable learning experiences for your students.

Browse by Topic
- Capacity Planning
- Demand Planning
- Inventory Management
- Process Analysis
- Process Improvement
- Production Planning
- Project Management
- Quality Management
New! Quick Cases in Operations Management
Quickly immerse students in focused and engaging business dilemmas. No student prep time required.
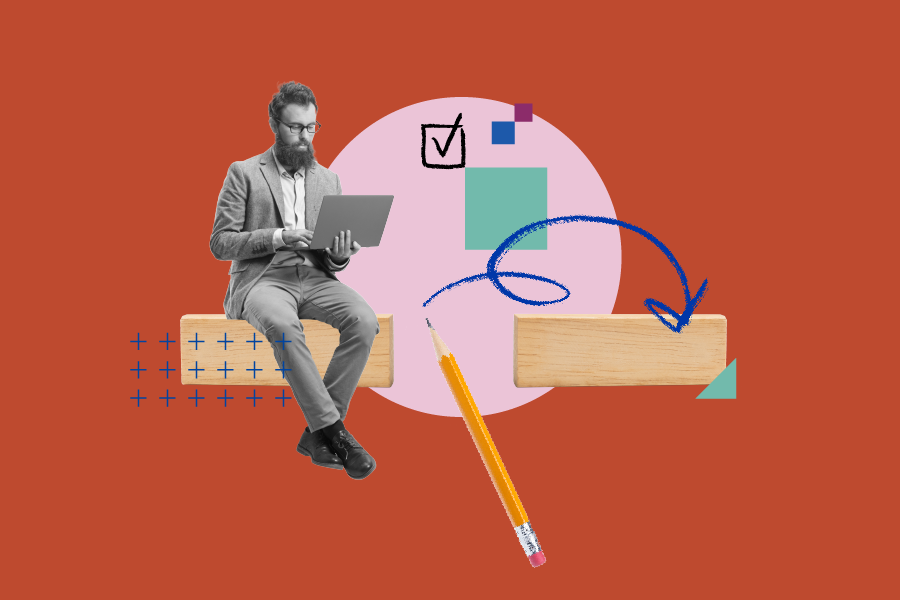
Fundamentals of Case Teaching
Our new, self-paced, online course guides you through the fundamentals for leading successful case discussions at any course level.
New in Operations Management
Explore the latest operations management learning materials
1325 word count
1563 word count
1098 word count
2320 word count
1841 word count
3547 word count
1648 word count
1840 word count
Looking for something specific?
Explore materials that align with your operations management learning objectives
Operations Management Simulations
Give your students hands-on experience making decisions.
Operations Management Cases with Female Protagonists
Explore a collection of operations management cases featuring female protagonists curated by the HBS Gender Initiative.
Operations Management Cases with Protagonists of Color
Discover operations management cases featuring protagonists of color that have been recommended by Harvard Business School faculty.
Foundational Operations Management Readings
Discover readings that cover the fundamental concepts and frameworks that business students must learn about operations management.
Bestsellers in Operations Management
Explore what other educators are using in their operations management courses
Start building your courses today
Register for a free Educator Account and get exclusive access to our entire catalog of learning materials, teaching resources, and online course planning tools.
We use cookies to understand how you use our site and to improve your experience, including personalizing content. Learn More . By continuing to use our site, you accept our use of cookies and revised Privacy Policy .
Smart. Open. Grounded. Inventive. Read our Ideas Made to Matter.
Which program is right for you?
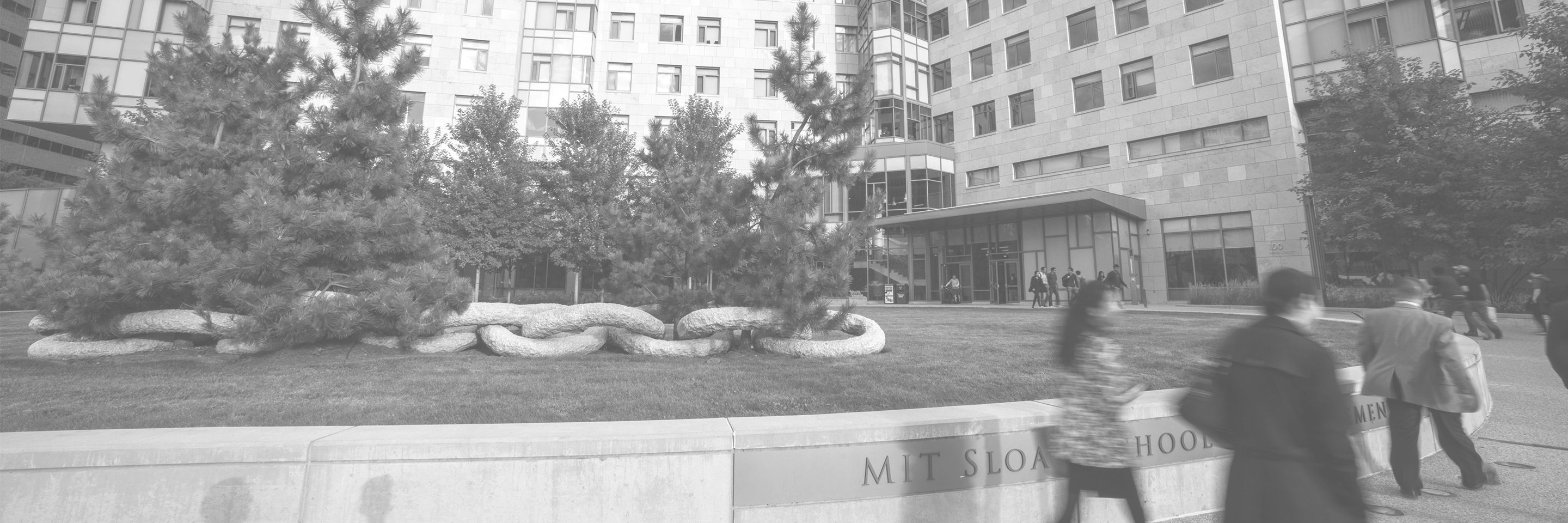
Through intellectual rigor and experiential learning, this full-time, two-year MBA program develops leaders who make a difference in the world.
A rigorous, hands-on program that prepares adaptive problem solvers for premier finance careers.
A 12-month program focused on applying the tools of modern data science, optimization and machine learning to solve real-world business problems.
Earn your MBA and SM in engineering with this transformative two-year program.
Combine an international MBA with a deep dive into management science. A special opportunity for partner and affiliate schools only.
A doctoral program that produces outstanding scholars who are leading in their fields of research.
Bring a business perspective to your technical and quantitative expertise with a bachelor’s degree in management, business analytics, or finance.
A joint program for mid-career professionals that integrates engineering and systems thinking. Earn your master’s degree in engineering and management.
An interdisciplinary program that combines engineering, management, and design, leading to a master’s degree in engineering and management.
Executive Programs
A full-time MBA program for mid-career leaders eager to dedicate one year of discovery for a lifetime of impact.
This 20-month MBA program equips experienced executives to enhance their impact on their organizations and the world.
Non-degree programs for senior executives and high-potential managers.
A non-degree, customizable program for mid-career professionals.
Teaching Resources Library
Operations Management Case Studies
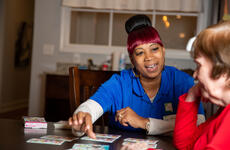
To read this content please select one of the options below:
Please note you do not have access to teaching notes, case research in operations management.
International Journal of Operations & Production Management
ISSN : 0144-3577
Article publication date: 1 February 2002
This paper reviews the use of case study research in operations management for theory development and testing. It draws on the literature on case research in a number of disciplines and uses examples drawn from operations management research. It provides guidelines and a roadmap for operations management researchers wishing to design, develop and conduct case‐based research.
- Operations management
- Methodology
- Case studies
Voss, C. , Tsikriktsis, N. and Frohlich, M. (2002), "Case research in operations management", International Journal of Operations & Production Management , Vol. 22 No. 2, pp. 195-219. https://doi.org/10.1108/01443570210414329
Copyright © 2002, MCB UP Limited
Related articles
We’re listening — tell us what you think, something didn’t work….
Report bugs here
All feedback is valuable
Please share your general feedback
Join us on our journey
Platform update page.
Visit emeraldpublishing.com/platformupdate to discover the latest news and updates
Questions & More Information
Answers to the most commonly asked questions here
Browse Course Material
Course info, instructors.
- Prof. Charles H. Fine
- Prof. Tauhid Zaman
Departments
- Sloan School of Management
As Taught In
- Mathematics
- Social Science
Introduction to Operations Management
Case preparation questions.
The following table contains the preparation questions to be utilized in the “quick” and “deep” case analyses.

You are leaving MIT OpenCourseWare

- About / Contact
- Privacy Policy
- Alphabetical List of Companies
- Business Analysis Topics
Starbucks Operations Management, 10 Decision Areas & Productivity
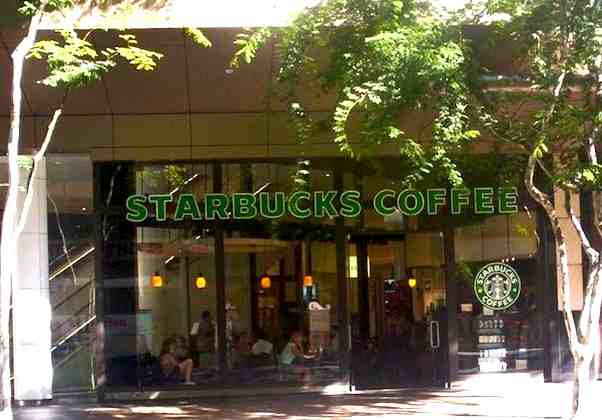
Starbucks Corporation’s operations management (OM) represents business decisions encompassing coffeehouse operations and corporate office activities. These decisions also influence the productivity and operational efficiency of franchisees and licensees. Strategic decisions in operations management direct business development toward the realization of Starbucks’ mission statement and vision statement . However, the diversity of coffee markets worldwide requires the company to apply different approaches to ensure the suitability of operations management to different business environments. Licensed and franchised Starbucks locations flexibly adjust to their local market conditions.
The 10 strategic decisions of operations management facilitate the alignment of all business areas in Starbucks’ organization. The business objectives in these decision areas implement strategies for industry leadership, such as the Coffee and Farmer Equity (C.A.F.E.) program in supply chain management. Effective operations management fortifies the strong brand image and other business strengths discussed in the SWOT analysis of Starbucks .
Starbucks’ Operations Management – 10 Critical Decisions
1. Goods and Services require decisions on the characteristics of business processes to meet the target features and quality of Starbucks products. This decision area of operations management affects other areas of the coffeehouse business. For example, the specifications of Starbucks’ roasted coffee beans establish the cost and quality limits and requirements in corresponding production operations. The coffee company’s emphasis on premium value and premium design means that production operations and productivity measures involve small margins of error to support high quality and value.
This decision area of operations management demonstrates the influence of the coffee industry environment on the company and its target consumers. Food product specifications are made to match social and economic trends, as well as the other external trends discussed in the PESTLE/PESTEL analysis of Starbucks . In addition, distribution channels affect food, beverage, and service design decisions in this area of operations management. For example, the packaging features of Starbucks instant coffees consider the logistics and inventory processes of distribution channels and retailers.
2. Quality Management ensures that business outputs satisfy Starbucks’ quality standards and the quality expectations of customers. Decisions in this area of the coffee company’s operations management aim for policies and processes that meet these standards and expectations. For example, Starbucks sources its coffee beans from farmers who comply with the company’s quality standards. The firm also prefers to buy from farmers certified under the Coffee and Farmer Equity program. Starbucks’ generic competitive strategy and intensive growth strategies are applied to use quality specifications as a selling point.
This critical decision area of operations management also accounts for customer experience in the company’s cafés and online operations. Starbucks’ strategic objective is to maintain consistent quality of service for consistent customer experience in brick-and-mortar and e-commerce environments. Premium service quality is ensured through a warm and friendly organizational culture at Starbucks coffeehouses. This service quality contributes to competitiveness against other coffeehouse firms, like Costa Coffee and Tim Hortons, as well as food-service companies that serve coffee, such as Dunkin’, McDonald’s , Wendy’s , Burger King , and Subway . Thus, Starbucks’ competitive advantage partly depends on this decision area of operations management.
3. Process and Capacity Design contributes to Starbucks’ success. The company’s operations management standardizes processes for efficiency, as observable in its cafés. Also, Starbucks optimizes capacity utilization to meet fluctuations in demand for coffee and food products. For example, processes at the company’s stores are flexible to adjust personnel to spikes in demand during peak hours. In this decision area of operations management, strategic planning at Starbucks aims to maximize productivity and cost-effectiveness through efficiency of workflows and processes.
4. Location Strategy in Starbucks’ operations management for its coffeehouses focuses on urban centers. Most of the company’s locations are in densely populated areas where demand for coffee products is typically high. In some markets, Starbucks uses strategic clustering of cafés in the same area to gain market share and drive competitors away. Strategic effectiveness in this decision area of operations management comes with a suitable marketing strategy to ensure the profitability of these cafés. Starbucks’ marketing mix or 4P helps bring customers to the company’s restaurant locations. Also, the organization of operations in these locations is supported through a suitable corporate structure. Thus, Starbucks’ organizational structure (corporate structure) reflects this location strategy.
5. Layout Design and Strategy for Starbucks cafés address workflow efficiency. The strategic decision in this area of operations management focuses on high productivity and efficiency in the movement of information and resources, including human resources, such as baristas. This layout strategy maximizes Starbucks coffeehouse space utilization with emphasis on premium customer experience, which involves higher prices for a more spacious dining (or drinking) environment. In this decision area of operations management, the company uses customer experience and premium branding to guide layout design and strategy.
6. Human Resources and Job Design have the objective of maintaining stable human resources to support Starbucks’ operational needs. At coffeehouses, the company has teams of baristas. In other parts of the organization, Starbucks has functional positions, like inventory management positions and marketing positions. This decision area of operations management considers human resource management challenges in international business, such as workforce development despite competition with other large food-service firms in the labor market. This area of operations management also integrates Starbucks’ organizational culture (corporate culture) to enhance job satisfaction, combat employee burnout, and support high productivity and operational efficiency.
7. Supply Chain Management focuses on maintaining adequate supply that matches Starbucks’ needs, while accounting for trends in the market. With this strategic objective, operations managers apply diversification in the supply chain for coffee and other ingredients and materials. Starbucks’ diverse set of suppliers ensures a stable supply of coffee beans from farmers in different countries. The company also uses its Coffee and Farmer Equity (C.A.F.E.) program to select and prioritize suppliers based on ethical practices, sustainability, and community impact. Thus, this decision area of operations management integrates ethics and Starbucks’ corporate social responsibility (CSR), ESG, and corporate citizenship into the supply chain. The Five Forces analysis of Starbucks indicates that suppliers have moderate bargaining power in the industry. Decisions in this area of operations management create a balance between the coffee company and its suppliers’ bargaining power, in order to benefit all parties involved.
8. Inventory Management is linked to Starbucks’ supply chain management. The critical decision in this area of operations management focuses on maintaining the adequate availability and movement of inventory to support the coffee company’s production requirements. At restaurants, inventory management involves manual monitoring combined with information technology to support managers and baristas. In supply and distribution hub operations, Starbucks uses automation comprehensively. Such an approach to this decision area of operations management minimizes stockout rates and guarantees adequate supply of food and beverage products and ingredients.
9. Scheduling has the objective of implementing and maintaining schedules that match market demand and Starbucks’ resources, processes, operating capacity, and productivity. In this decision area of operations management, the company applies a combination of fixed and flexible schedules for personnel at corporate offices, coffeehouses, and other facilities. Also, automation is widely used to make scheduling processes efficient and comprehensive, accounting for different market conditions affecting Starbucks locations.
10. Maintenance concerns the availability of resources and operating capacities to support the coffeehouse chain. The strategic objective in this decision area of operations management is to achieve and maintain the high reliability of Starbucks’ resources and capacities, such as for ingredient production processes. The company uses teams of employees and third-party service providers for maintaining facilities and equipment, like machines used for roasting coffee beans. Also, in this area of operations management, Starbucks maintains its human resource capacity through training programs and retention strategies. This approach satisfies the company’s workforce requirements for corporate offices and facilities and supports franchisees and licensees.
Productivity at Starbucks Coffee Company
Operations management at Starbucks uses various productivity criteria, depending on the area of operations under consideration. Some productivity metrics that are applicable to the company’s operations are as follows:
- Average order fulfillment duration (Starbucks coffeehouse productivity)
- Weight of coffee beans processed per time (roasting productivity)
- Average repair duration per equipment type (maintenance productivity)
- Bai, J. (2023). The Starbucks Crisis – External and endogenous pressures of coffee market giants. Frontiers in Business, Economics and Management, 8 (1), 272-275.
- Faeq, D. K. (2022). The importance of employee involvement in work activities to overall productivity. International Journal of Humanities and Education Development (IJHED), 4 (5), 15-26.
- Molnárová, Z., & Reiter, M. (2022). Technology, demand, and productivity: What an industry model tells us about business cycles. Journal of Economic Dynamics and Control, 134 , 104272.
- Reid, R. D., & Sanders, N. R. (2023). Operations Management: An Integrated Approach . John Wiley & Sons.
- Starbucks Corporation – Form 10-K .
- Starbucks Ethical Sourcing of Sustainable Products .
- Starbucks Ethical Sourcing – Coffee .
- Szwarc, E., Bocewicz, G., Golińska-Dawson, P., & Banaszak, Z. (2023). Proactive operations management: Staff allocation with competence maintenance constraints. Sustainability, 15 (3), 1949.
- Copyright by Panmore Institute - All rights reserved.
- This article may not be reproduced, distributed, or mirrored without written permission from Panmore Institute and its author/s.
- Educators, Researchers, and Students: You are permitted to quote or paraphrase parts of this article (not the entire article) for educational or research purposes, as long as the article is properly cited and referenced together with its URL/link.
- Harvard Business School →
- Faculty & Research →
Cases in Operations Management: Analysis and Action
- Format: Print
- Find it at Harvard
About The Authors
W. Earl Sasser
Kim B. Clark
More from the authors.
- October 2021
- Faculty Research
Sam Bernards: A Career in Building Businesses
- January 2021
Karin Vinik at South Lake Hospital (B)-(D)
- December 2019
Karin Vinik at South Lake Hospital (D)
- Sam Bernards: A Career in Building Businesses By: Kim B. Clark and Sarah Eyring
- Karin Vinik at South Lake Hospital (B)-(D) By: Joseph L. Badaracco and Kim B. Clark
- Karin Vinik at South Lake Hospital (D) By: Joseph L. Badaracco and Kim B. Clark
BUS5 147: Service Operations Management
- Getting Started
- Topics and Search Terms
- Articles & Databases
- Company & Financial Information
- Industry & Market Information
- Case Studies
- PESTEL Analysis
Databases from the University Library for Case Studies
- ABI/INFORM This link opens in a new window Enter your search term in the main search box. Scroll down below the search box to find Document Type. Choose "Business Case."
- Business Source Complete This link opens in a new window Enter your search term in the main search box. Scroll down below the search box to find Document Type. Choose "Case Study."
- ScienceDirect This link opens in a new window Enter your search term in the search box marked "Keywords" and click the magnifying glass icon. To the left of your article results, look for the "Article type" heading and choose "Case reports."
- Emerald Insight This link opens in a new window Developed for Business Schools and Management Departments, Emerald Insight is a collection of peer-reviewed management journals -- 100+ full text journals or 75,000+ full text management articles and reviews from the top 300 management journals.
Case Study Analysis by Cengage
Business publisher Cengage has provided an overview of how to analyze a case study.
- Cengage Case Studies: Overview What is case study analysis? A case study presents an account of what happened to a business or industry over a number of years. It chronicles the events that managers had to deal with, such as changes in the competitive environment, and charts the managers' response, which usually involved changing the business- or corporate-level strategy.
- << Previous: Industry & Market Information
- Next: PESTEL Analysis >>
- Last Updated: Jan 8, 2024 11:23 AM
- URL: https://libguides.sjsu.edu/bus147
Loading Results
No Match Found
Operations strategy case studies
Customer operations.
A leading US non-profit health insurer focused on service as a key differentiator. It wanted to gain insight into current operational performance, and develop customer-centric capabilities like self-service and digital competency. PwC's Strategy& was engaged to evaluate and address gaps in customer and member engagement.
Leveraging our health insurance expertise, proprietary market research databases, and best practices to help the client develop its differentiated customer-centric capabilities, we identified quick wins included outsourcing of manual activities, automation of macros/scripting, and standardization of call center work-from-home policies. We delivered a plan to enhance workforce management, consolidate provider data claim, and move to pre-pay policy. Additional recommendations addressed network rationalization, timely issuance of ID cards, and reducing SG&A expenses.
The project identified $25M investment in provider engagement, flexible network design, personalized member service, and real-time enrollment to achieve the desired differentiating capabilities.
Innovation and product development
A global chemicals specialty company with multiple business units and several existing embedded R&D teams was challenged by stagnating growth in difficult market conditions and the client was seeking to reinvigorate the portfolio. The client sought to consolidate R&D capabilities and establish a corporate innovation function to coordinate and drive its long-term R&D agenda and drive growth.
Strategy& was asked to design the innovation operating model, define the collaboration with business units, and develop a concept for R&D partnerships and venturing to drive growth.
We established a target operating model, refocused product innovation into clusters and developed a venturing approach. The client experienced a significant upswing in R&D productivity, new record numbers of patents filed, and breakthroughs innovations in a number of focus areas. Overall, improved R&D coherence led to 13% direct top line growth and 15% EBITDA improvement.
Strategic supply management
A global lighting company with over $5B sales revenue across more than 130 countries was faced with tremendous market disruptions resulting from the transition from traditional lighting to LED. To successfully play in this significantly different market, the company sold off its traditional business and refocused on the technically driven, fast-cycled LED business. To enable this, the client had to adopt new business models. Within this context, the procurement function had to undergo a major transition towards strategic supply management to effectively support the businesses going forward.
Strategy& supported the client in identifying the new requirements resulting from the changed business models, developing the procurement transformation program based on prioritized 4-6 focus areas (e.g. SRM, Supplier and Innovation Scouting), including appropriate KPIs, and designing a comprehensive change management concept and roadmap to ensure engagement and buy-in from the client team.
The transformation delivered significantly improved service levels for the BUs based on nine key strategic supply management capabilities and an adapted operating model with an improved split of roles and responsibilities between corporate headquarters and business units.
Competitive manufacturing
A global product company with $10B sales revenue across more than 130 countries was suffering from a highly complex manufacturing footprint which was not aligned with the client’s main markets. The client was losing sales and profitability due to high order fulfillment cycle times, high manufacturing costs, and low productivity performance in its key operations.
Strategy& designed the global manufacturing footprint strategy based on clearly defined customer and market requirements. As a consequence, the team agreed to realign the operations footprint from 23 to 15 operations by implementing a more balanced global footprint closer to key customers and/or distribution centers.
The transformation delivered shorter order fulfillment cycle times while simultaneously reducing manufacturing costs by up to 10% and improving overall productivity and flexibility. These results led to a gross margin improvement by 5%.

Capital assets
A leading oil field services and equipment company’s financial performance was lagging its peers, and the company had committed to a 3% improvement in North American net margin. Management believed there was an opportunity to improve the effectiveness of their >$1B equipment maintenance spend, but was unclear on where and how to achieve savings.
Strategy& helped the client pinpoint inefficiencies in their maintenance operating model, shifting from a highly reactive and siloed operation to an integrated team using advanced techniques to deliver maintenance when and where needed based on data. The changes were substantial as the client reorganized to break down functional barriers and create a maintenance process focused on customer performance.
Results were impressive — the maintenance transformation program was implemented at the top 80% of locations by revenue, resulting in a ~2% boost to net margins. It also drove a 20% reduction in maintenance cost, 50% reduction in maintenance related downtime, and improved customer service.
General and administrative (G&A) operations
The securities servicing division of a global banking group sought to address business challenges like reduced productivity, sub-optimal operating model for its Center of Excellence (CoE), lack of process standardization, cost escalation, process fragmentation, and duplication. Strategy& was asked to help in accelerating execution and benefits delivery through process optimization, offshoring and redesign of operating model.
Strategy& developed initial hypothesis through a detailed current state analysis, using both quantitative and qualitative tools, and conducted workshops to identify quick win opportunities. We proposed a redesigned operating model for the CoEs, and suggested in-depth implementation plan to drive the changes.
The project identified potential cost saving of $10M per annum and recommended lean FTE allocation across locations. The project also identified opportunities to achieve process efficiency and provided detailed target state structure of the CoE, including team size, shift patterns, and processes performed.
Enterprise-wide operational excellence
A leading tier-1 automotive supplier for the production and processing of rubber, plastics and metal with $680MM. sales revenue faced significant growth rates, but structures, process efficiency and financial performance did not follow accordingly and significant refinancing/cash flow complications evolved.
Strategy& was tasked with reshaping the company starting from product-market-strategy, developing the organizational structure and optimizing the entire process and operations landscape. An overall restructuring concept based on two pillars was developed: 1) Urgent short-term actions focusing on firefighting to ensure customer satisfaction and 2) sustainable long-term measures facilitating the optimization of the company’s footprint, product creation process, sales initiatives as well as lean production initiatives and the definition of an overall production system.
Continued success of these measures was ensured through the implementation of a common reporting structure and escalation process to track progress and define counter measures in case of deviations. The highly successful project identified cost saving initiatives worth more than $135MM. and had the client achieving EBIT margins of 6-8% during the project.

Strategy&'s global footprint
View the complete list of Strategy& worldwide offices
Partner, Strategy& Germany
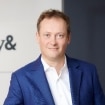
Harald Dutzler
Partner, Strategy& Austria
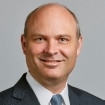
Principal, Strategy& US
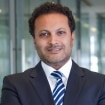
Haroon Sheikh
Senior Executive Advisor, Strategy& Middle East
© 2019 - 2024 PwC. All rights reserved. PwC refers to the PwC network and/or one or more of its member firms, each of which is a separate legal entity. Please see www.pwc.com/structure for further details.
- Privacy statement
- Terms of use
- Cookies info
- About site provider

Case Related Links
Case studies collection.
Business Strategy Marketing Finance Human Resource Management IT and Systems Operations Economics Leadership and Entrepreneurship Project Management Business Ethics Corporate Governance Women Empowerment CSR and Sustainability Law Business Environment Enterprise Risk Management Insurance Innovation Miscellaneous Business Reports Multimedia Case Studies Cases in Other Languages Simplified Case Studies
Short Case Studies
Business Ethics Business Environment Business Strategy Consumer Behavior Human Resource Management Industrial Marketing International Marketing IT and Systems Marketing Communications Marketing Management Miscellaneous Operations Sales and Distribution Management Services Marketing More Short Case Studies >
JavaScript seems to be disabled in your browser. For the best experience on our site, be sure to turn on Javascript in your browser.
We use cookies to make your experience better. To comply with the new e-Privacy directive, we need to ask for your consent to set the cookies. Learn more .
- Compare Products
- Case Collection
- Operations Management
Items 1 - 10 of 14
- You're currently reading page 1
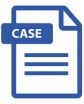
The case is centered around the timeline of the Telangana graduates’ MLC elections 2021, which were held against the backdrop of a known unknown: the COVID-19 pandemic. The electoral officials had to be mindful of the numerous security protocols and complexities involved in implementing the election process in such uncertain times. They had to incorporate additional steps and plan for contingencies to mitigate risks while executing the election process. Halfway through the election planning process, it became clear that the number of voters and candidates was unprecedentedly large. This unexpected development necessitated a revision of the prior plan for conducting the elections. Shashank Goel, Chief Electoral Officer (CEO), and M. Satyavani, Deputy CEO, were architecting the plan for conducting the elections with an unexpectedly large number of voters and candidates under pandemic-induced disruptions. Goel was also reflecting on how to develop contingency plans for these elections, given the uncertainty produced by unforeseen external factors and the associated risks. Although he had the mandate to conduct free and fair elections within the stipulated timelines and was assured that the required resources would be provided, several factors had to be considered. According to the constitutional guidelines for the graduates' MLC elections, qualified and registered graduate voters could cast their vote by ranking candidates preferentially. Paper ballots had to be used because electronic voting machines (EVMs) could not handle preferential voting. The scale and magnitude of the elections necessitated jumbo ballot boxes. To manage the process, the number of polling stations had to be increased, and manpower had to be trained. Further, the presence of healthcare workers to ensure the safety of voters and the deployed staff was imperative. The Telangana CEO’s office had to meet the increased logistical and technical requirements and ensure high voting turnouts while executing the election process.
Postponing the election was not an option for the ECI from the standpoint of the legal code of conduct. The Telangana CEO's office prepared a revised election plan. The project plan was amended to incorporate the need for additional resources and logistical support to execute the election process. As the efforts of the staff were maximized effectively, the elections could be conducted smoothly and transparently although a large number of candidates were in the fray.
Teaching and Learning Objectives:
The key case objectives are to enable students to:
- Appreciate the importance of effective project management, planning, and execution in public administration against the backdrop of uncertainties and complexities.
- Understand the importance of risk identification, risk planning, and prioritization.
- Learn strategies to manage various project risks in a real-life situation.
- Identify the characteristics of effective leadership in times of crisis and the key takeaways from such scenarios
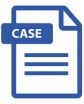
The case is designed to be used in courses on Nonprofit Operations Management, Data Analytics, Six Sigma, and Business Process Excellence/Improvement in MBA or Executive MBA programs. It is suitable for teaching students about the common problem of lower rates of volunteerism in nonprofit organizations. Further, the case study helps present the importance and application of inferential statistics (data analytics) to identify the impact of various factors on the problem (effect). The case is set in early 2021 when Shefali Sharma, the Strategy and Learning Manager with Teach For India (TFI), faced a few challenging questions from a professor at the Indian School of Business (ISB) during her presentation at an industry gathering in Hyderabad, India. Sharma was concerned about the low matriculation rate of TFI fellows, despite the rigorous recruitment, selection, and matriculation (RSM) process. A mere 50-60% matriculation rate was not a commensurate return for an investment of INR 6.5 million and the massive effort put into the RSM process. In 2017, Sharma organized focused informative and experiential events to motivate candidates to join the fellowship, but it was not very clear if these events impacted the TFI matriculation rate. After the industry gathering at ISB, Sharma followed up with the professor to seek his guidance in performing data analytics on the matriculation data. Sharma wondered if inferential data analysis could help her understand which demographic factors and events impact the matriculation rate.
Learning Objective
- Illustrate the importance of inferential statistics as a decision support system in resolving business problems
- Formulating and solving a hypothesis testing problem for attribute (discrete) data
- Visually depicting the flow of work across different stages of a process
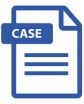
In response to the uncontrollable second wave of COVID-19 in the south Indian state of Telangana in April 2021, a few like-minded social activists in the capital city of Hyderabad came together to establish a 100-bed medical care center to treat COVID-19 patients. The project was named Ashray. Dr. Chinnababu Sunkavalli (popularly known as Chinna) was the project manager of Project Ashray. In addition to the inherent inadequacy of hospital beds to accommodate the growing number of COVID- 19 patients till March 2021, the city faced a sudden spike of infections in April that worsened the situation. Consequently, the occupancy in government and private hospitals in Hyderabad increased by 485% and 311%, respectively, from March to April. According to a prediction model, Chinna knew that hospital beds would be exhausted in several parts of the city in the next few days. The Project Ashray team was concerned about the situation. The team met on April 26, 2021, to schedule the project to establish the medical care center within the next 10 days. The case is suitable for teaching students how to approach the scheduling problem of a time- constrained project systematically. It helps as a pedagogical aid in teaching management concepts such as project visualization, estimating project duration, float, and project laddering or activity splitting, and tools such as network diagrams, critical path method, and crashing. The case exposes students to a real-time problem-solving approach under uncertainty and crises and the critical role of NGOs in supporting the governments. Alongside the Project Management and Operations Management courses, other courses like Managerial decision-making in nonprofit organizations, Health care delivery, and healthcare operations could also find support from this case.
Learning Objectives:
To learn: Time-constrained projects and associated scheduling problems Project visualization using network diagrams Activity sequencing and converting sequential activities to parallel activities Critical path method (early start, early finish, late start, late finish, forward pass, backward pass, and float) to estimate a project's overall duration Project laddering to reduce the project duration wherever possible Project crashing using linear programming
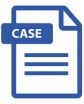
The case goes on to describe the enormous challenges involved in building the 4.94 km long Bogibeel Bridge in the North Eastern Region (NER) of India. When it was finally commissioned in 2018, it was hailed as a marvel of engineering. With two rail lines and a two-lane road over it, the bridge spanned the mighty Brahmaputra river. The Bogibeel Bridge was India's longest and Asia's second-longest road and rail bridge with fully-welded bridge technology that met European codes and welding standards. The interstate connectivity provided by the bridge enabled important socio-economic developments in the NER that included improved logistics and transportation, the growth of medical and educational facilities, higher employment, and the rise of international trade and tourism. While the outcomes of the project were significant, the efforts that went into constructing the Bogibeel Bridge were equally so. This case study is designed to teach the importance of effective risk planning in project management. Further, the case introduces students to earned value analysis and project oversight in managing large projects. The case centers on Indian Railways' need to quickly discover why the Bogibeel project was not going according to plan. The case also serves as a resource to teach public operations management where the focus is on projects and operations that result in socio-economic outcomes.
- Appreciate the importance of risk planning and risk prioritization and learn strategies to manage various project risks
- Understand earned value management (EVM) and the associated metrics and calculations for project evaluation on time and cost schedules.
- Identify social impact outcomes in public/infrastructure projects.
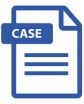
Access to clean water is so critical for development and survival that the United Nations' Sustainable Development Goal number 6 (SDG-6) was to ensure availability and sustained management of water and sanitation. The World Health Organization (WHO) in 2006 estimated that 97 million Indians lacked clean and safe water. Fluoride and total dissolvable solids (TDS) in drinking water were dangerously high at many parts of rural India, with adverse impacts. On the other hand, buying clean drinking water from commercial vendors at market rates was not a realistic alternative, a costly recurring expense that much of India's rural population could not afford. The case tracks the efforts of Huggahalli, head of the technology group of Sri Sathya Sai Seva Organisations (SSSO), to devise a sustainable solution to the drinking water problem in rural India that is low on cost, high on impact. They eventually develop a model that satisfies all these criteria and becomes the basis for a project called Premamrutha Dhaara. Funded by Sri Sathya Sai Central Trust, the project aims to install water purification plants in more than 100 villages spanning six states in India, with the ultimate goal of turning over plant operations to the beneficiary villages and setting up a welfare fund in each village from the revenue generated. Social service projects, particularly in developing countries, have their unique challenges. The case highlights the importance of performing feasibility analysis as part of the project planning in social projects. The case also describes how the financial and operational dimensions of sustainability could lead to a self-sustainable system. The social innovation framework used to deploy the water purification project to achieve broader rural welfare has wider implications for project management, social innovation and change, sustainable operations management, strategic non-profit management, and public policy.
The case offers four possibilities for central objectives:
- To perform feasibility analysis in a Project Management course
- To design a social innovation framework in a Social Innovation and Change course
- To understand the dimensions of self-sustainability in a Sustainable Operations Management course
- To measure social impact in Strategic Non-profit Management and Public Policy courses
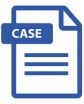
During the Indian general election of 2019, the Nizamabad constituency in Telangana state found itself in an unprecedented situation with a record 185 candidates competing for one seat. Most of these candidates were local farmers who saw the election as a platform for raising awareness about local issues, particularly the perceived lack of government support for guaranteeing minimum support prices for their crops. More than 185 candidates had in fact contested elections from a single constituency in a handful of elections in the past. The Election Commission of India (ECI) had declared them to be "special elections" where it made exceptions to the original election schedule to accommodate the large number of candidates. However, in the 2019 general election, the ECI made no such exceptions, announcing instead that polling in Nizamabad would be conducted as per the original schedule and results would be declared at the same time as the rest of the country. This presented a unique and unexpected challenge for Rajat Kumar, the Telangana Chief Electoral Officer (CEO) and his team. How were they to conduct free and fair and elections within the mandated timeframe with the largest number of electronic voting machines (EVMs) ever deployed to address the will of 185 candidates in a constituency with 1.55 million voters from rural and semi-urban areas? Case A describes the electoral process followed by the world's largest democracy to guarantee free and fair elections. It concludes by posing several situational questions, the answers to which will determine whether the polls in Nizamabad are conducted successfully or not. Case B, which should be revealed after students have had a chance to deliberate on the challenges posed in Case A, describes the decisions and actions taken by Kumar and his team in preparation for the Nizamabad polls and the events that took place on election day and afterward.
To demonstrate how a quantitative approach to decision making can be used in the public policy domain to achieve end goals. To learn how resource allocation decisions can be made by understanding the scale of the problem, the various resource constraints, and the end goals. To discover operational innovations in the face of regulatory and technical constraints and complete the required steps. To understand the multiple steps involved in conducting elections in the Indian context.
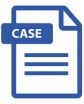
Set in April 2017, this case centers around the digital technology dilemma facing the protagonist Dr. Vimohan, the chief intensivist of Prashant Hospital. The case describes the critical challenges afflicting the intensive care unit (ICU) of the hospital. It then follows Dr. Vimohan as he visits the Bengaluru headquarters of Cloudphysician Healthcare, a Tele-ICU provider. The visit leaves Dr. Vimohan wondering whether he can leverage the Tele-ICU solution to overcome the challenges at Prashant Hospital. He instinctively knew that he would need to use a combination of qualitative and quantitative analysis to resolve this dilemma.
The case study enables critical thinking and decision-making to address the business situation. Assessing the pros and cons of a potential technology solution, examining the readiness of an organization and devising a framework for effective stakeholder and change management are some of the key concepts. Associated tools include cost-benefit analysis, net present value (NPV) analysis, force-field analysis, and change-readiness assessment, in addition to a brief discussion on SWOT analysis.
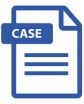
Set in 2016 in Hyderabad, India, the case follows Puvvala Yugandhar, Senior Vice President at Dr. Reddy's Laboratories (DRL), as he decides what to do about an underperforming production policy at their plants. Adopted a decade earlier, the policy, called Replenish to Consumption -Pooled (RTC-P), had not delivered the expected results. Specifically, the plants had been seeing an increase in production switchovers and creeping buffer levels for certain products, which had led to higher holding costs and lost sales for certain products. A senior consultant had suggested that DRL switch to a demand estimation-based policy called Replenish to Anticipation (RTA), which attempted to address the above concerns by segregating production capacity and updating buffer levels using demand estimates. However, Yugandhar, well aware of the challenges of changing production policies, wanted to explore a variant of RTC-P called Replenish to Consumption -Dedicated (RTC-D), which followed the same buffer update rules as RTC-P but maintained dedicated capacities for a subset of products.
By studying and solving the decision problem in the case, students should be able to better appreciate the challenges involved in making long-term operational changes. It gives them an opportunity to: (1) understand how each input might impact the final decision, and (2) how to weigh each of these inputs in arriving at the final decision.
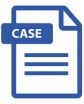
We crafted the case study "Software Acquisition for Employee Engagement at Pilot Mountain Research " for use in Business Marketing, Buyer Behavior, or Operations Management courses in undergraduate, MBA, or Executive Education programs. The Pilot Mountain Market Research (PMMR) case study provides students with the opportunity to examine how buying decisions can be made utilizing online digital tools that are increasingly available to business-to-business (B2B) purchasing managers. To do so, we created fictitious research studies and data to realistically portray the kinds of information that are publicly available to B2B purchasing managers on the Internet today. In this case study, we introduce students to fit analysis, coding quality technical assessment, sentiment analysis, and ratings & reviews analyses. Students are challenged to integrate findings from these diverse analytical tools, combining both qualitative and quantitative data into concrete employee engagement software (EES) purchasing recommendations.
1. Evolving criteria for selecting a software package for organization-wide procurement in a B2B purchase decision context 2. Appreciate increasing digitalization of businesses 3. Understand importance of employee engagement in organizations and what an organization could do to enhance employee engagement among its workforce 4. Understand decision making processes in the context of digitalisation of businesses
- Browse All Articles
- Newsletter Sign-Up
ServiceOperations →
No results found in working knowledge.
- Were any results found in one of the other content buckets on the left?
- Try removing some search filters.
- Use different search filters.
- All Headlines

Top 40 Most Popular Case Studies of 2021
Two cases about Hertz claimed top spots in 2021's Top 40 Most Popular Case Studies
Two cases on the uses of debt and equity at Hertz claimed top spots in the CRDT’s (Case Research and Development Team) 2021 top 40 review of cases.
Hertz (A) took the top spot. The case details the financial structure of the rental car company through the end of 2019. Hertz (B), which ranked third in CRDT’s list, describes the company’s struggles during the early part of the COVID pandemic and its eventual need to enter Chapter 11 bankruptcy.
The success of the Hertz cases was unprecedented for the top 40 list. Usually, cases take a number of years to gain popularity, but the Hertz cases claimed top spots in their first year of release. Hertz (A) also became the first ‘cooked’ case to top the annual review, as all of the other winners had been web-based ‘raw’ cases.
Besides introducing students to the complicated financing required to maintain an enormous fleet of cars, the Hertz cases also expanded the diversity of case protagonists. Kathyrn Marinello was the CEO of Hertz during this period and the CFO, Jamere Jackson is black.
Sandwiched between the two Hertz cases, Coffee 2016, a perennial best seller, finished second. “Glory, Glory, Man United!” a case about an English football team’s IPO made a surprise move to number four. Cases on search fund boards, the future of malls, Norway’s Sovereign Wealth fund, Prodigy Finance, the Mayo Clinic, and Cadbury rounded out the top ten.
Other year-end data for 2021 showed:
- Online “raw” case usage remained steady as compared to 2020 with over 35K users from 170 countries and all 50 U.S. states interacting with 196 cases.
- Fifty four percent of raw case users came from outside the U.S..
- The Yale School of Management (SOM) case study directory pages received over 160K page views from 177 countries with approximately a third originating in India followed by the U.S. and the Philippines.
- Twenty-six of the cases in the list are raw cases.
- A third of the cases feature a woman protagonist.
- Orders for Yale SOM case studies increased by almost 50% compared to 2020.
- The top 40 cases were supervised by 19 different Yale SOM faculty members, several supervising multiple cases.
CRDT compiled the Top 40 list by combining data from its case store, Google Analytics, and other measures of interest and adoption.
All of this year’s Top 40 cases are available for purchase from the Yale Management Media store .
And the Top 40 cases studies of 2021 are:
1. Hertz Global Holdings (A): Uses of Debt and Equity
2. Coffee 2016
3. Hertz Global Holdings (B): Uses of Debt and Equity 2020
4. Glory, Glory Man United!
5. Search Fund Company Boards: How CEOs Can Build Boards to Help Them Thrive
6. The Future of Malls: Was Decline Inevitable?
7. Strategy for Norway's Pension Fund Global
8. Prodigy Finance
9. Design at Mayo
10. Cadbury
11. City Hospital Emergency Room
13. Volkswagen
14. Marina Bay Sands
15. Shake Shack IPO
16. Mastercard
17. Netflix
18. Ant Financial
19. AXA: Creating the New CR Metrics
20. IBM Corporate Service Corps
21. Business Leadership in South Africa's 1994 Reforms
22. Alternative Meat Industry
23. Children's Premier
24. Khalil Tawil and Umi (A)
25. Palm Oil 2016
26. Teach For All: Designing a Global Network
27. What's Next? Search Fund Entrepreneurs Reflect on Life After Exit
28. Searching for a Search Fund Structure: A Student Takes a Tour of Various Options
30. Project Sammaan
31. Commonfund ESG
32. Polaroid
33. Connecticut Green Bank 2018: After the Raid
34. FieldFresh Foods
35. The Alibaba Group
36. 360 State Street: Real Options
37. Herman Miller
38. AgBiome
39. Nathan Cummings Foundation
40. Toyota 2010
Artificial intelligence in strategy
Can machines automate strategy development? The short answer is no. However, there are numerous aspects of strategists’ work where AI and advanced analytics tools can already bring enormous value. Yuval Atsmon is a senior partner who leads the new McKinsey Center for Strategy Innovation, which studies ways new technologies can augment the timeless principles of strategy. In this episode of the Inside the Strategy Room podcast, he explains how artificial intelligence is already transforming strategy and what’s on the horizon. This is an edited transcript of the discussion. For more conversations on the strategy issues that matter, follow the series on your preferred podcast platform .
Joanna Pachner: What does artificial intelligence mean in the context of strategy?
Yuval Atsmon: When people talk about artificial intelligence, they include everything to do with analytics, automation, and data analysis. Marvin Minsky, the pioneer of artificial intelligence research in the 1960s, talked about AI as a “suitcase word”—a term into which you can stuff whatever you want—and that still seems to be the case. We are comfortable with that because we think companies should use all the capabilities of more traditional analysis while increasing automation in strategy that can free up management or analyst time and, gradually, introducing tools that can augment human thinking.
Joanna Pachner: AI has been embraced by many business functions, but strategy seems to be largely immune to its charms. Why do you think that is?
Subscribe to the Inside the Strategy Room podcast
Yuval Atsmon: You’re right about the limited adoption. Only 7 percent of respondents to our survey about the use of AI say they use it in strategy or even financial planning, whereas in areas like marketing, supply chain, and service operations, it’s 25 or 30 percent. One reason adoption is lagging is that strategy is one of the most integrative conceptual practices. When executives think about strategy automation, many are looking too far ahead—at AI capabilities that would decide, in place of the business leader, what the right strategy is. They are missing opportunities to use AI in the building blocks of strategy that could significantly improve outcomes.
I like to use the analogy to virtual assistants. Many of us use Alexa or Siri but very few people use these tools to do more than dictate a text message or shut off the lights. We don’t feel comfortable with the technology’s ability to understand the context in more sophisticated applications. AI in strategy is similar: it’s hard for AI to know everything an executive knows, but it can help executives with certain tasks.
When executives think about strategy automation, many are looking too far ahead—at AI deciding the right strategy. They are missing opportunities to use AI in the building blocks of strategy.
Joanna Pachner: What kind of tasks can AI help strategists execute today?
Yuval Atsmon: We talk about six stages of AI development. The earliest is simple analytics, which we refer to as descriptive intelligence. Companies use dashboards for competitive analysis or to study performance in different parts of the business that are automatically updated. Some have interactive capabilities for refinement and testing.
The second level is diagnostic intelligence, which is the ability to look backward at the business and understand root causes and drivers of performance. The level after that is predictive intelligence: being able to anticipate certain scenarios or options and the value of things in the future based on momentum from the past as well as signals picked in the market. Both diagnostics and prediction are areas that AI can greatly improve today. The tools can augment executives’ analysis and become areas where you develop capabilities. For example, on diagnostic intelligence, you can organize your portfolio into segments to understand granularly where performance is coming from and do it in a much more continuous way than analysts could. You can try 20 different ways in an hour versus deploying one hundred analysts to tackle the problem.
Predictive AI is both more difficult and more risky. Executives shouldn’t fully rely on predictive AI, but it provides another systematic viewpoint in the room. Because strategic decisions have significant consequences, a key consideration is to use AI transparently in the sense of understanding why it is making a certain prediction and what extrapolations it is making from which information. You can then assess if you trust the prediction or not. You can even use AI to track the evolution of the assumptions for that prediction.
Those are the levels available today. The next three levels will take time to develop. There are some early examples of AI advising actions for executives’ consideration that would be value-creating based on the analysis. From there, you go to delegating certain decision authority to AI, with constraints and supervision. Eventually, there is the point where fully autonomous AI analyzes and decides with no human interaction.
Because strategic decisions have significant consequences, you need to understand why AI is making a certain prediction and what extrapolations it’s making from which information.
Joanna Pachner: What kind of businesses or industries could gain the greatest benefits from embracing AI at its current level of sophistication?
Yuval Atsmon: Every business probably has some opportunity to use AI more than it does today. The first thing to look at is the availability of data. Do you have performance data that can be organized in a systematic way? Companies that have deep data on their portfolios down to business line, SKU, inventory, and raw ingredients have the biggest opportunities to use machines to gain granular insights that humans could not.
Companies whose strategies rely on a few big decisions with limited data would get less from AI. Likewise, those facing a lot of volatility and vulnerability to external events would benefit less than companies with controlled and systematic portfolios, although they could deploy AI to better predict those external events and identify what they can and cannot control.
Third, the velocity of decisions matters. Most companies develop strategies every three to five years, which then become annual budgets. If you think about strategy in that way, the role of AI is relatively limited other than potentially accelerating analyses that are inputs into the strategy. However, some companies regularly revisit big decisions they made based on assumptions about the world that may have since changed, affecting the projected ROI of initiatives. Such shifts would affect how you deploy talent and executive time, how you spend money and focus sales efforts, and AI can be valuable in guiding that. The value of AI is even bigger when you can make decisions close to the time of deploying resources, because AI can signal that your previous assumptions have changed from when you made your plan.
Joanna Pachner: Can you provide any examples of companies employing AI to address specific strategic challenges?
Yuval Atsmon: Some of the most innovative users of AI, not coincidentally, are AI- and digital-native companies. Some of these companies have seen massive benefits from AI and have increased its usage in other areas of the business. One mobility player adjusts its financial planning based on pricing patterns it observes in the market. Its business has relatively high flexibility to demand but less so to supply, so the company uses AI to continuously signal back when pricing dynamics are trending in a way that would affect profitability or where demand is rising. This allows the company to quickly react to create more capacity because its profitability is highly sensitive to keeping demand and supply in equilibrium.
Joanna Pachner: Given how quickly things change today, doesn’t AI seem to be more a tactical than a strategic tool, providing time-sensitive input on isolated elements of strategy?
Yuval Atsmon: It’s interesting that you make the distinction between strategic and tactical. Of course, every decision can be broken down into smaller ones, and where AI can be affordably used in strategy today is for building blocks of the strategy. It might feel tactical, but it can make a massive difference. One of the world’s leading investment firms, for example, has started to use AI to scan for certain patterns rather than scanning individual companies directly. AI looks for consumer mobile usage that suggests a company’s technology is catching on quickly, giving the firm an opportunity to invest in that company before others do. That created a significant strategic edge for them, even though the tool itself may be relatively tactical.
Joanna Pachner: McKinsey has written a lot about cognitive biases and social dynamics that can skew decision making. Can AI help with these challenges?
Yuval Atsmon: When we talk to executives about using AI in strategy development, the first reaction we get is, “Those are really big decisions; what if AI gets them wrong?” The first answer is that humans also get them wrong—a lot. [Amos] Tversky, [Daniel] Kahneman, and others have proven that some of those errors are systemic, observable, and predictable. The first thing AI can do is spot situations likely to give rise to biases. For example, imagine that AI is listening in on a strategy session where the CEO proposes something and everyone says “Aye” without debate and discussion. AI could inform the room, “We might have a sunflower bias here,” which could trigger more conversation and remind the CEO that it’s in their own interest to encourage some devil’s advocacy.
We also often see confirmation bias, where people focus their analysis on proving the wisdom of what they already want to do, as opposed to looking for a fact-based reality. Just having AI perform a default analysis that doesn’t aim to satisfy the boss is useful, and the team can then try to understand why that is different than the management hypothesis, triggering a much richer debate.
In terms of social dynamics, agency problems can create conflicts of interest. Every business unit [BU] leader thinks that their BU should get the most resources and will deliver the most value, or at least they feel they should advocate for their business. AI provides a neutral way based on systematic data to manage those debates. It’s also useful for executives with decision authority, since we all know that short-term pressures and the need to make the quarterly and annual numbers lead people to make different decisions on the 31st of December than they do on January 1st or October 1st. Like the story of Ulysses and the sirens, you can use AI to remind you that you wanted something different three months earlier. The CEO still decides; AI can just provide that extra nudge.
Joanna Pachner: It’s like you have Spock next to you, who is dispassionate and purely analytical.
Yuval Atsmon: That is not a bad analogy—for Star Trek fans anyway.
Joanna Pachner: Do you have a favorite application of AI in strategy?
Yuval Atsmon: I have worked a lot on resource allocation, and one of the challenges, which we call the hockey stick phenomenon, is that executives are always overly optimistic about what will happen. They know that resource allocation will inevitably be defined by what you believe about the future, not necessarily by past performance. AI can provide an objective prediction of performance starting from a default momentum case: based on everything that happened in the past and some indicators about the future, what is the forecast of performance if we do nothing? This is before we say, “But I will hire these people and develop this new product and improve my marketing”— things that every executive thinks will help them overdeliver relative to the past. The neutral momentum case, which AI can calculate in a cold, Spock-like manner, can change the dynamics of the resource allocation discussion. It’s a form of predictive intelligence accessible today and while it’s not meant to be definitive, it provides a basis for better decisions.
Joanna Pachner: Do you see access to technology talent as one of the obstacles to the adoption of AI in strategy, especially at large companies?
Yuval Atsmon: I would make a distinction. If you mean machine-learning and data science talent or software engineers who build the digital tools, they are definitely not easy to get. However, companies can increasingly use platforms that provide access to AI tools and require less from individual companies. Also, this domain of strategy is exciting—it’s cutting-edge, so it’s probably easier to get technology talent for that than it might be for manufacturing work.
The bigger challenge, ironically, is finding strategists or people with business expertise to contribute to the effort. You will not solve strategy problems with AI without the involvement of people who understand the customer experience and what you are trying to achieve. Those who know best, like senior executives, don’t have time to be product managers for the AI team. An even bigger constraint is that, in some cases, you are asking people to get involved in an initiative that may make their jobs less important. There could be plenty of opportunities for incorporating AI into existing jobs, but it’s something companies need to reflect on. The best approach may be to create a digital factory where a different team tests and builds AI applications, with oversight from senior stakeholders.
The big challenge is finding strategists to contribute to the AI effort. You are asking people to get involved in an initiative that may make their jobs less important.
Joanna Pachner: Do you think this worry about job security and the potential that AI will automate strategy is realistic?
Yuval Atsmon: The question of whether AI will replace human judgment and put humanity out of its job is a big one that I would leave for other experts.
The pertinent question is shorter-term automation. Because of its complexity, strategy would be one of the later domains to be affected by automation, but we are seeing it in many other domains. However, the trend for more than two hundred years has been that automation creates new jobs, although ones requiring different skills. That doesn’t take away the fear some people have of a machine exposing their mistakes or doing their job better than they do it.
Joanna Pachner: We recently published an article about strategic courage in an age of volatility that talked about three types of edge business leaders need to develop. One of them is an edge in insights. Do you think AI has a role to play in furnishing a proprietary insight edge?
Yuval Atsmon: One of the challenges most strategists face is the overwhelming complexity of the world we operate in—the number of unknowns, the information overload. At one level, it may seem that AI will provide another layer of complexity. In reality, it can be a sharp knife that cuts through some of the clutter. The question to ask is, Can AI simplify my life by giving me sharper, more timely insights more easily?
Joanna Pachner: You have been working in strategy for a long time. What sparked your interest in exploring this intersection of strategy and new technology?
Yuval Atsmon: I have always been intrigued by things at the boundaries of what seems possible. Science fiction writer Arthur C. Clarke’s second law is that to discover the limits of the possible, you have to venture a little past them into the impossible, and I find that particularly alluring in this arena.
AI in strategy is in very nascent stages but could be very consequential for companies and for the profession. For a top executive, strategic decisions are the biggest way to influence the business, other than maybe building the top team, and it is amazing how little technology is leveraged in that process today. It’s conceivable that competitive advantage will increasingly rest in having executives who know how to apply AI well. In some domains, like investment, that is already happening, and the difference in returns can be staggering. I find helping companies be part of that evolution very exciting.
Explore a career with us
Related articles.

Strategic courage in an age of volatility
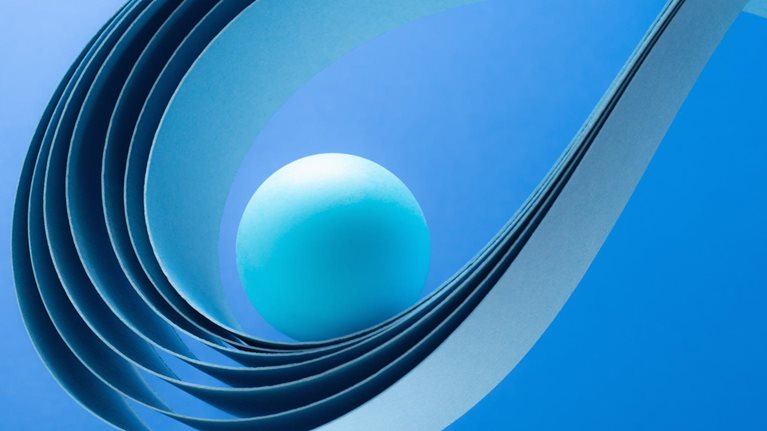
Bias Busters Collection
Machine Learning and image analysis towards improved energy management in Industry 4.0: a practical case study on quality control
- Original Article
- Open access
- Published: 13 May 2024
- Volume 17 , article number 48 , ( 2024 )
Cite this article
You have full access to this open access article
- Mattia Casini 1 ,
- Paolo De Angelis 1 ,
- Marco Porrati 2 ,
- Paolo Vigo 1 ,
- Matteo Fasano 1 ,
- Eliodoro Chiavazzo 1 &
- Luca Bergamasco ORCID: orcid.org/0000-0001-6130-9544 1
155 Accesses
1 Altmetric
Explore all metrics
With the advent of Industry 4.0, Artificial Intelligence (AI) has created a favorable environment for the digitalization of manufacturing and processing, helping industries to automate and optimize operations. In this work, we focus on a practical case study of a brake caliper quality control operation, which is usually accomplished by human inspection and requires a dedicated handling system, with a slow production rate and thus inefficient energy usage. We report on a developed Machine Learning (ML) methodology, based on Deep Convolutional Neural Networks (D-CNNs), to automatically extract information from images, to automate the process. A complete workflow has been developed on the target industrial test case. In order to find the best compromise between accuracy and computational demand of the model, several D-CNNs architectures have been tested. The results show that, a judicious choice of the ML model with a proper training, allows a fast and accurate quality control; thus, the proposed workflow could be implemented for an ML-powered version of the considered problem. This would eventually enable a better management of the available resources, in terms of time consumption and energy usage.
Similar content being viewed by others
Towards Operation Excellence in Automobile Assembly Analysis Using Hybrid Image Processing
Deep Learning Based Algorithms for Welding Edge Points Detection
Artificial Intelligence: Prospect in Mechanical Engineering Field—A Review
Avoid common mistakes on your manuscript.
Introduction
An efficient use of energy resources in industry is key for a sustainable future (Bilgen, 2014 ; Ocampo-Martinez et al., 2019 ). The advent of Industry 4.0, and of Artificial Intelligence, have created a favorable context for the digitalisation of manufacturing processes. In this view, Machine Learning (ML) techniques have the potential for assisting industries in a better and smart usage of the available data, helping to automate and improve operations (Narciso & Martins, 2020 ; Mazzei & Ramjattan, 2022 ). For example, ML tools can be used to analyze sensor data from industrial equipment for predictive maintenance (Carvalho et al., 2019 ; Dalzochio et al., 2020 ), which allows identification of potential failures in advance, and thus to a better planning of maintenance operations with reduced downtime. Similarly, energy consumption optimization (Shen et al., 2020 ; Qin et al., 2020 ) can be achieved via ML-enabled analysis of available consumption data, with consequent adjustments of the operating parameters, schedules, or configurations to minimize energy consumption while maintaining an optimal production efficiency. Energy consumption forecast (Liu et al., 2019 ; Zhang et al., 2018 ) can also be improved, especially in industrial plants relying on renewable energy sources (Bologna et al., 2020 ; Ismail et al., 2021 ), by analysis of historical data on weather patterns and forecast, to optimize the usage of energy resources, avoid energy peaks, and leverage alternative energy sources or storage systems (Li & Zheng, 2016 ; Ribezzo et al., 2022 ; Fasano et al., 2019 ; Trezza et al., 2022 ; Mishra et al., 2023 ). Finally, ML tools can also serve for fault or anomaly detection (Angelopoulos et al., 2019 ; Md et al., 2022 ), which allows prompt corrective actions to optimize energy usage and prevent energy inefficiencies. Within this context, ML techniques for image analysis (Casini et al., 2024 ) are also gaining increasing interest (Chen et al., 2023 ), for their application to e.g. materials design and optimization (Choudhury, 2021 ), quality control (Badmos et al., 2020 ), process monitoring (Ho et al., 2021 ), or detection of machine failures by converting time series data from sensors to 2D images (Wen et al., 2017 ).
Incorporating digitalisation and ML techniques into Industry 4.0 has led to significant energy savings (Maggiore et al., 2021 ; Nota et al., 2020 ). Projects adopting these technologies can achieve an average of 15% to 25% improvement in energy efficiency in the processes where they were implemented (Arana-Landín et al., 2023 ). For instance, in predictive maintenance, ML can reduce energy consumption by optimizing the operation of machinery (Agrawal et al., 2023 ; Pan et al., 2024 ). In process optimization, ML algorithms can improve energy efficiency by 10-20% by analyzing and adjusting machine operations for optimal performance, thereby reducing unnecessary energy usage (Leong et al., 2020 ). Furthermore, the implementation of ML algorithms for optimal control can lead to energy savings of 30%, because these systems can make real-time adjustments to production lines, ensuring that machines operate at peak energy efficiency (Rahul & Chiddarwar, 2023 ).
In automotive manufacturing, ML-driven quality control can lead to energy savings by reducing the need for redoing parts or running inefficient production cycles (Vater et al., 2019 ). In high-volume production environments such as consumer electronics, novel computer-based vision models for automated detection and classification of damaged packages from intact packages can speed up operations and reduce waste (Shahin et al., 2023 ). In heavy industries like steel or chemical manufacturing, ML can optimize the energy consumption of large machinery. By predicting the optimal operating conditions and maintenance schedules, these systems can save energy costs (Mypati et al., 2023 ). Compressed air is one of the most energy-intensive processes in manufacturing. ML can optimize the performance of these systems, potentially leading to energy savings by continuously monitoring and adjusting the air compressors for peak efficiency, avoiding energy losses due to leaks or inefficient operation (Benedetti et al., 2019 ). ML can also contribute to reducing energy consumption and minimizing incorrectly produced parts in polymer processing enterprises (Willenbacher et al., 2021 ).
Here we focus on a practical industrial case study of brake caliper processing. In detail, we focus on the quality control operation, which is typically accomplished by human visual inspection and requires a dedicated handling system. This eventually implies a slower production rate, and inefficient energy usage. We thus propose the integration of an ML-based system to automatically perform the quality control operation, without the need for a dedicated handling system and thus reduced operation time. To this, we rely on ML tools able to analyze and extract information from images, that is, deep convolutional neural networks, D-CNNs (Alzubaidi et al., 2021 ; Chai et al., 2021 ).
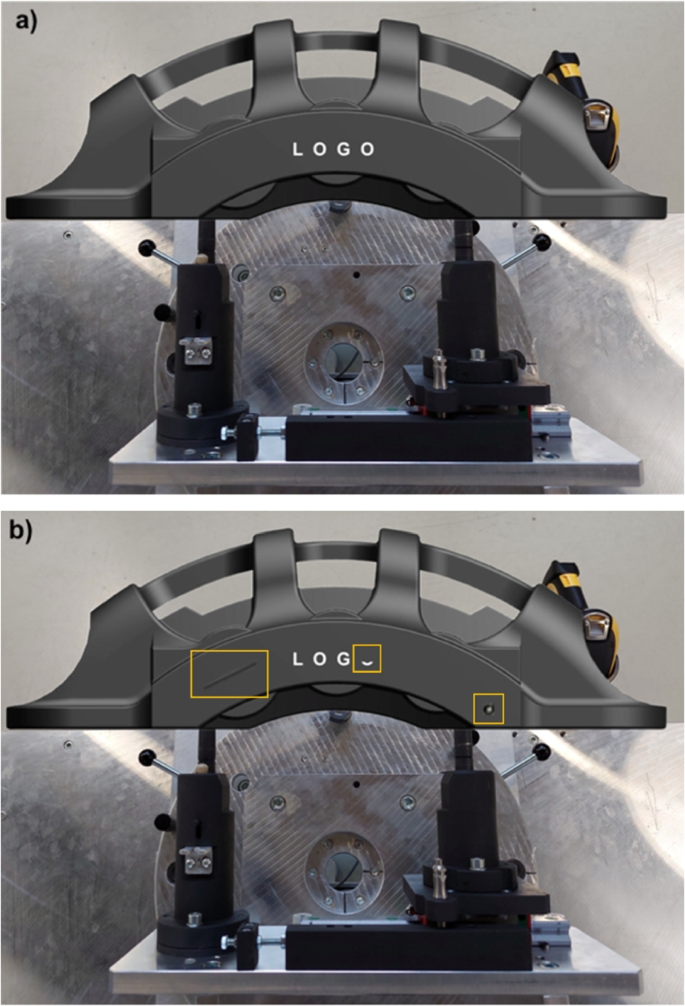
Sample 3D model (GrabCAD ) of the considered brake caliper: (a) part without defects, and (b) part with three sample defects, namely a scratch, a partially missing letter in the logo, and a circular painting defect (shown by the yellow squares, from left to right respectively)
A complete workflow for the purpose has been developed and tested on a real industrial test case. This includes: a dedicated pre-processing of the brake caliper images, their labelling and analysis using two dedicated D-CNN architectures (one for background removal, and one for defect identification), post-processing and analysis of the neural network output. Several different D-CNN architectures have been tested, in order to find the best model in terms of accuracy and computational demand. The results show that, a judicious choice of the ML model with a proper training, allows to obtain fast and accurate recognition of possible defects. The best-performing models, indeed, reach over 98% accuracy on the target criteria for quality control, and take only few seconds to analyze each image. These results make the proposed workflow compliant with the typical industrial expectations; therefore, in perspective, it could be implemented for an ML-powered version of the considered industrial problem. This would eventually allow to achieve better performance of the manufacturing process and, ultimately, a better management of the available resources in terms of time consumption and energy expense.
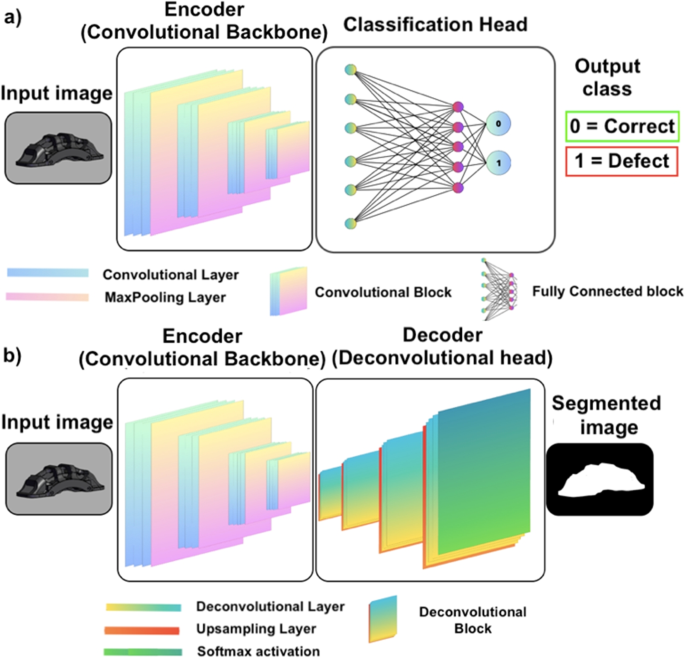
Different neural network architectures: convolutional encoder (a) and encoder-decoder (b)
The industrial quality control process that we target is the visual inspection of manufactured components, to verify the absence of possible defects. Due to industrial confidentiality reasons, a representative open-source 3D geometry (GrabCAD ) of the considered parts, similar to the original one, is shown in Fig. 1 . For illustrative purposes, the clean geometry without defects (Fig. 1 (a)) is compared to the geometry with three possible sample defects, namely: a scratch on the surface of the brake caliper, a partially missing letter in the logo, and a circular painting defect (highlighted by the yellow squares, from left to right respectively, in Fig. 1 (b)). Note that, one or multiple defects may be present on the geometry, and that other types of defects may also be considered.
Within the industrial production line, this quality control is typically time consuming, and requires a dedicated handling system with the associated slow production rate and energy inefficiencies. Thus, we developed a methodology to achieve an ML-powered version of the control process. The method relies on data analysis and, in particular, on information extraction from images of the brake calipers via Deep Convolutional Neural Networks, D-CNNs (Alzubaidi et al., 2021 ). The designed workflow for defect recognition is implemented in the following two steps: 1) removal of the background from the image of the caliper, in order to reduce noise and irrelevant features in the image, ultimately rendering the algorithms more flexible with respect to the background environment; 2) analysis of the geometry of the caliper to identify the different possible defects. These two serial steps are accomplished via two different and dedicated neural networks, whose architecture is discussed in the next section.
Convolutional Neural Networks (CNNs) pertain to a particular class of deep neural networks for information extraction from images. The feature extraction is accomplished via convolution operations; thus, the algorithms receive an image as an input, analyze it across several (deep) neural layers to identify target features, and provide the obtained information as an output (Casini et al., 2024 ). Regarding this latter output, different formats can be retrieved based on the considered architecture of the neural network. For a numerical data output, such as that required to obtain a classification of the content of an image (Bhatt et al., 2021 ), e.g. correct or defective caliper in our case, a typical layout of the network involving a convolutional backbone, and a fully-connected network can be adopted (see Fig. 2 (a)). On the other hand, if the required output is still an image, a more complex architecture with a convolutional backbone (encoder) and a deconvolutional head (decoder) can be used (see Fig. 2 (b)).
As previously introduced, our workflow targets the analysis of the brake calipers in a two-step procedure: first, the removal of the background from the input image (e.g. Fig. 1 ); second, the geometry of the caliper is analyzed and the part is classified as acceptable or not depending on the absence or presence of any defect, respectively. Thus, in the first step of the procedure, a dedicated encoder-decoder network (Minaee et al., 2021 ) is adopted to classify the pixels in the input image as brake or background. The output of this model will then be a new version of the input image, where the background pixels are blacked. This helps the algorithms in the subsequent analysis to achieve a better performance, and to avoid bias due to possible different environments in the input image. In the second step of the workflow, a dedicated encoder architecture is adopted. Here, the previous background-filtered image is fed to the convolutional network, and the geometry of the caliper is analyzed to spot possible defects and thus classify the part as acceptable or not. In this work, both deep learning models are supervised , that is, the algorithms are trained with the help of human-labeled data (LeCun et al., 2015 ). Particularly, the first algorithm for background removal is fed with the original image as well as with a ground truth (i.e. a binary image, also called mask , consisting of black and white pixels) which instructs the algorithm to learn which pixels pertain to the brake and which to the background. This latter task is usually called semantic segmentation in Machine Learning and Deep Learning (Géron, 2022 ). Analogously, the second algorithm is fed with the original image (without the background) along with an associated mask, which serves the neural networks with proper instructions to identify possible defects on the target geometry. The required pre-processing of the input images, as well as their use for training and validation of the developed algorithms, are explained in the next sections.
Image pre-processing
Machine Learning approaches rely on data analysis; thus, the quality of the final results is well known to depend strongly on the amount and quality of the available data for training of the algorithms (Banko & Brill, 2001 ; Chen et al., 2021 ). In our case, the input images should be well-representative for the target analysis and include adequate variability of the possible features to allow the neural networks to produce the correct output. In this view, the original images should include, e.g., different possible backgrounds, a different viewing angle of the considered geometry and a different light exposure (as local light reflections may affect the color of the geometry and thus the analysis). The creation of such a proper dataset for specific cases is not always straightforward; in our case, for example, it would imply a systematic acquisition of a large set of images in many different conditions. This would require, in turn, disposing of all the possible target defects on the real parts, and of an automatic acquisition system, e.g., a robotic arm with an integrated camera. Given that, in our case, the initial dataset could not be generated on real parts, we have chosen to generate a well-balanced dataset of images in silico , that is, based on image renderings of the real geometry. The key idea was that, if the rendered geometry is sufficiently close to a real photograph, the algorithms may be instructed on artificially-generated images and then tested on a few real ones. This approach, if properly automatized, clearly allows to easily produce a large amount of images in all the different conditions required for the analysis.
In a first step, starting from the CAD file of the brake calipers, we worked manually using the open-source software Blender (Blender ), to modify the material properties and achieve a realistic rendering. After that, defects were generated by means of Boolean (subtraction) operations between the geometry of the brake caliper and ad-hoc geometries for each defect. Fine tuning on the generated defects has allowed for a realistic representation of the different defects. Once the results were satisfactory, we developed an automated Python code for the procedures, to generate the renderings in different conditions. The Python code allows to: load a given CAD geometry, change the material properties, set different viewing angles for the geometry, add different types of defects (with given size, rotation and location on the geometry of the brake caliper), add a custom background, change the lighting conditions, render the scene and save it as an image.
In order to make the dataset as varied as possible, we introduced three light sources into the rendering environment: a diffuse natural lighting to simulate daylight conditions, and two additional artificial lights. The intensity of each light source and the viewing angle were then made vary randomly, to mimic different daylight conditions and illuminations of the object. This procedure was designed to provide different situations akin to real use, and to make the model invariant to lighting conditions and camera position. Moreover, to provide additional flexibility to the model, the training dataset of images was virtually expanded using data augmentation (Mumuni & Mumuni, 2022 ), where saturation, brightness and contrast were made randomly vary during training operations. This procedure has allowed to consistently increase the number and variety of the images in the training dataset.
The developed automated pre-processing steps easily allows for batch generation of thousands of different images to be used for training of the neural networks. This possibility is key for proper training of the neural networks, as the variability of the input images allows the models to learn all the possible features and details that may change during real operating conditions.
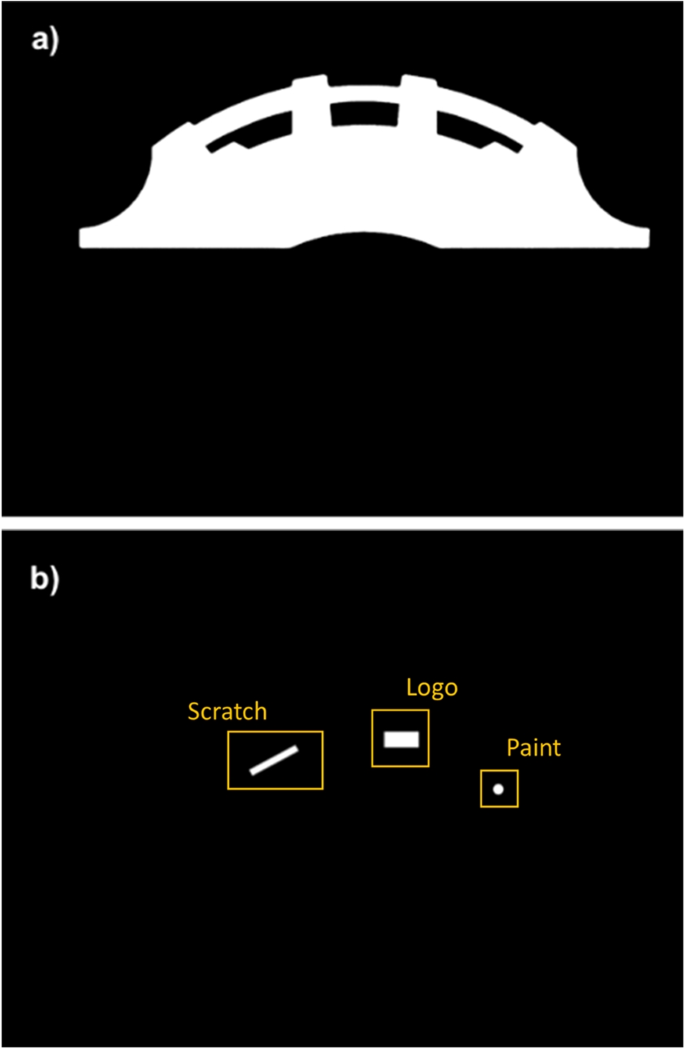
Examples of the ground truth for the two target tasks: background removal (a) and defects recognition (b)
The first tests using such virtual database have shown that, although the generated images were very similar to real photographs, the models were not able to properly recognize the target features in the real images. Thus, in a tentative to get closer to a proper set of real images, we decided to adopt a hybrid dataset, where the virtually generated images were mixed with the available few real ones. However, given that some possible defects were missing in the real images, we also decided to manipulate the images to introduce virtual defects on real images. The obtained dataset finally included more than 4,000 images, where 90% was rendered, and 10% was obtained from real images. To avoid possible bias in the training dataset, defects were present in 50% of the cases in both the rendered and real image sets. Thus, in the overall dataset, the real original images with no defects were 5% of the total.
Along with the code for the rendering and manipulation of the images, dedicated Python routines were developed to generate the corresponding data labelling for the supervised training of the networks, namely the image masks. Particularly, two masks were generated for each input image: one for the background removal operation, and one for the defect identification. In both cases, the masks consist of a binary (i.e. black and white) image where all the pixels of a target feature (i.e. the geometry or defect) are assigned unitary values (white); whereas, all the remaining pixels are blacked (zero values). An example of these masks in relation to the geometry in Fig. 1 is shown in Fig. 3 .
All the generated images were then down-sampled, that is, their resolution was reduced to avoid unnecessary large computational times and (RAM) memory usage while maintaining the required level of detail for training of the neural networks. Finally, the input images and the related masks were split into a mosaic of smaller tiles, to achieve a suitable size for feeding the images to the neural networks with even more reduced requirements on the RAM memory. All the tiles were processed, and the whole image reconstructed at the end of the process to visualize the overall final results.
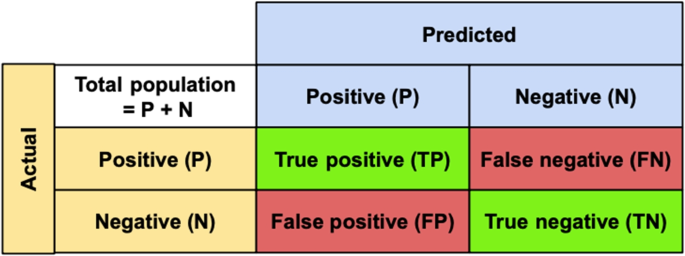
Confusion matrix for accuracy assessment of the neural networks models
Choice of the model
Within the scope of the present application, a wide range of possibly suitable models is available (Chen et al., 2021 ). In general, the choice of the best model for a given problem should be made on a case-by-case basis, considering an acceptable compromise between the achievable accuracy and computational complexity/cost. Too simple models can indeed be very fast in the response yet have a reduced accuracy. On the other hand, more complex models can generally provide more accurate results, although typically requiring larger amounts of data for training, and thus longer computational times and energy expense. Hence, testing has the crucial role to allow identification of the best trade-off between these two extreme cases. A benchmark for model accuracy can generally be defined in terms of a confusion matrix, where the model response is summarized into the following possibilities: True Positives (TP), True Negatives (TN), False Positives (FP) and False Negatives (FN). This concept can be summarized as shown in Fig. 4 . For the background removal, Positive (P) stands for pixels belonging to the brake caliper, while Negative (N) for background pixels. For the defect identification model, Positive (P) stands for non-defective geometry, whereas Negative (N) stands for defective geometries. With respect to these two cases, the True/False statements stand for correct or incorrect identification, respectively. The model accuracy can be therefore assessed as Géron ( 2022 )
Based on this metrics, the accuracy for different models can then be evaluated on a given dataset, where typically 80% of the data is used for training and the remaining 20% for validation. For the defect recognition stage, the following models were tested: VGG-16 (Simonyan & Zisserman, 2014 ), ResNet50, ResNet101, ResNet152 (He et al., 2016 ), Inception V1 (Szegedy et al., 2015 ), Inception V4 and InceptionResNet V2 (Szegedy et al., 2017 ). Details on the assessment procedure for the different models are provided in the Supplementary Information file. For the background removal stage, the DeepLabV3 \(+\) (Chen et al., 2018 ) model was chosen as the first option, and no additional models were tested as it directly provided satisfactory results in terms of accuracy and processing time. This gives preliminary indication that, from the point of view of the task complexity of the problem, the defect identification stage can be more demanding with respect to the background removal operation for the case study at hand. Besides the assessment of the accuracy according to, e.g., the metrics discussed above, additional information can be generally collected, such as too low accuracy (indicating insufficient amount of training data), possible bias of the models on the data (indicating a non-well balanced training dataset), or other specific issues related to missing representative data in the training dataset (Géron, 2022 ). This information helps both to correctly shape the training dataset, and to gather useful indications for the fine tuning of the model after its choice has been made.
Background removal
An initial bias of the model for background removal arose on the color of the original target geometry (red color). The model was indeed identifying possible red spots on the background as part of the target geometry as an unwanted output. To improve the model flexibility, and thus its accuracy on the identification of the background, the training dataset was expanded using data augmentation (Géron, 2022 ). This technique allows to artificially increase the size of the training dataset by applying various transformations to the available images, with the goal to improve the performance and generalization ability of the models. This approach typically involves applying geometric and/or color transformations to the original images; in our case, to account for different viewing angles of the geometry, different light exposures, and different color reflections and shadowing effects. These improvements of the training dataset proved to be effective on the performance for the background removal operation, with a validation accuracy finally ranging above 99% and model response time around 1-2 seconds. An example of the output of this operation for the geometry in Fig. 1 is shown in Fig. 5 .
While the results obtained were satisfactory for the original (red) color of the calipers, we decided to test the model ability to be applied on brake calipers of other colors as well. To this, the model was trained and tested on a grayscale version of the images of the calipers, which allows to completely remove any possible bias of the model on a specific color. In this case, the validation accuracy of the model was still obtained to range above 99%; thus, this approach was found to be particularly interesting to make the model suitable for background removal operation even on images including calipers of different colors.
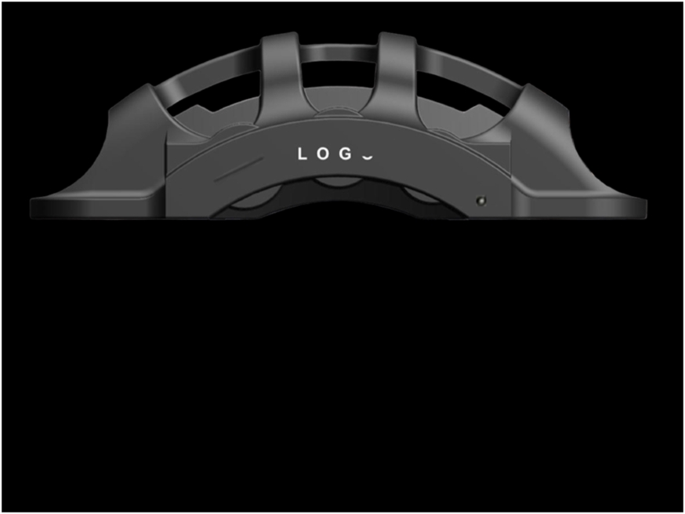
Target geometry after background removal
Defect recognition
An overview of the performance of the tested models for the defect recognition operation on the original geometry of the caliper is reported in Table 1 (see also the Supplementary Information file for more details on the assessment of different models). The results report on the achieved validation accuracy ( \(A_v\) ) and on the number of parameters ( \(N_p\) ), with this latter being the total number of parameters that can be trained for each model (Géron, 2022 ) to determine the output. Here, this quantity is adopted as an indicator of the complexity of each model.
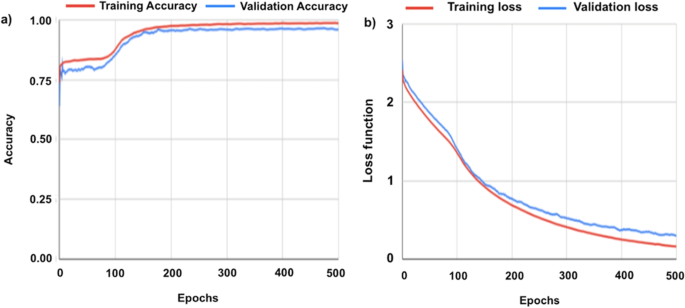
Accuracy (a) and loss function (b) curves for the Resnet101 model during training
As the results in Table 1 show, the VGG-16 model was quite unprecise for our dataset, eventually showing underfitting (Géron, 2022 ). Thus, we decided to opt for the Resnet and Inception families of models. Both these families of models have demonstrated to be suitable for handling our dataset, with slightly less accurate results being provided by the Resnet50 and InceptionV1. The best results were obtained using Resnet101 and InceptionV4, with very high final accuracy and fast processing time (in the order \(\sim \) 1 second). Finally, Resnet152 and InceptionResnetV2 models proved to be slightly too complex or slower for our case; they indeed provided excellent results but taking longer response times (in the order of \(\sim \) 3-5 seconds). The response time is indeed affected by the complexity ( \(N_p\) ) of the model itself, and by the hardware used. In our work, GPUs were used for training and testing all the models, and the hardware conditions were kept the same for all models.
Based on the results obtained, ResNet101 model was chosen as the best solution for our application, in terms of accuracy and reduced complexity. After fine-tuning operations, the accuracy that we obtained with this model reached nearly 99%, both in the validation and test datasets. This latter includes target real images, that the models have never seen before; thus, it can be used for testing of the ability of the models to generalize the information learnt during the training/validation phase.
The trend in the accuracy increase and loss function decrease during training of the Resnet101 model on the original geometry are shown in Fig. 6 (a) and (b), respectively. Particularly, the loss function quantifies the error between the predicted output during training of the model and the actual target values in the dataset. In our case, the loss function is computed using the cross-entropy function and the Adam optimiser (Géron, 2022 ). The error is expected to reduce during the training, which eventually leads to more accurate predictions of the model on previously-unseen data. The combination of accuracy and loss function trends, along with other control parameters, is typically used and monitored to evaluate the training process, and avoid e.g. under- or over-fitting problems (Géron, 2022 ). As Fig. 6 (a) shows, the accuracy experiences a sudden step increase during the very first training phase (epochs, that is, the number of times the complete database is repeatedly scrutinized by the model during its training (Géron, 2022 )). The accuracy then increases in a smooth fashion with the epochs, until an asymptotic value is reached both for training and validation accuracy. These trends in the two accuracy curves can generally be associated with a proper training; indeed, being the two curves close to each other may be interpreted as an absence of under-fitting problems. On the other hand, Fig. 6 (b) shows that the loss function curves are close to each other, with a monotonically-decreasing trend. This can be interpreted as an absence of over-fitting problems, and thus of proper training of the model.
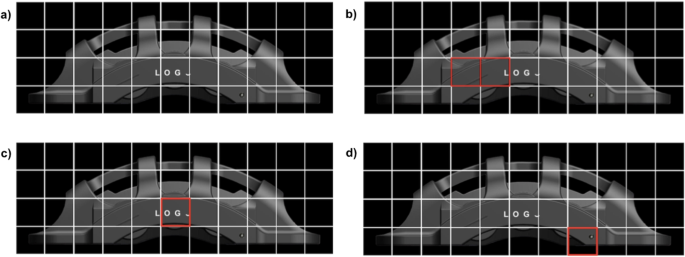
Final results of the analysis on the defect identification: (a) considered input geometry, (b), (c) and (d) identification of a scratch on the surface, partially missing logo, and painting defect respectively (highlighted in the red frames)
Finally, an example output of the overall analysis is shown in Fig. 7 , where the considered input geometry is shown (a), along with the identification of the defects (b), (c) and (d) obtained from the developed protocol. Note that, here the different defects have been separated in several figures for illustrative purposes; however, the analysis yields the identification of defects on one single image. In this work, a binary classification was performed on the considered brake calipers, where the output of the models allows to discriminate between defective or non-defective components based on the presence or absence of any of the considered defects. Note that, fine tuning of this discrimination is ultimately with the user’s requirements. Indeed, the model output yields as the probability (from 0 to 100%) of the possible presence of defects; thus, the discrimination between a defective or non-defective part is ultimately with the user’s choice of the acceptance threshold for the considered part (50% in our case). Therefore, stricter or looser criteria can be readily adopted. Eventually, for particularly complex cases, multiple models may also be used concurrently for the same task, and the final output defined based on a cross-comparison of the results from different models. As a last remark on the proposed procedure, note that here we adopted a binary classification based on the presence or absence of any defect; however, further classification of the different defects could also be implemented, to distinguish among different types of defects (multi-class classification) on the brake calipers.
Energy saving
Illustrative scenarios.
Given that the proposed tools have not yet been implemented and tested within a real industrial production line, we analyze here three perspective scenarios to provide a practical example of the potential for energy savings in an industrial context. To this, we consider three scenarios, which compare traditional human-based control operations and a quality control system enhanced by the proposed Machine Learning (ML) tools. Specifically, here we analyze a generic brake caliper assembly line formed by 14 stations, as outlined in Table 1 in the work by Burduk and Górnicka ( 2017 ). This assembly line features a critical inspection station dedicated to defect detection, around which we construct three distinct scenarios to evaluate the efficacy of traditional human-based control operations versus a quality control system augmented by the proposed ML-based tools, namely:
First Scenario (S1): Human-Based Inspection. The traditional approach involves a human operator responsible for the inspection tasks.
Second Scenario (S2): Hybrid Inspection. This scenario introduces a hybrid inspection system where our proposed ML-based automatic detection tool assists the human inspector. The ML tool analyzes the brake calipers and alerts the human inspector only when it encounters difficulties in identifying defects, specifically when the probability of a defect being present or absent falls below a certain threshold. This collaborative approach aims to combine the precision of ML algorithms with the experience of human inspectors, and can be seen as a possible transition scenario between the human-based and a fully-automated quality control operation.
Third Scenario (S3): Fully Automated Inspection. In the final scenario, we conceive a completely automated defect inspection station powered exclusively by our ML-based detection system. This setup eliminates the need for human intervention, relying entirely on the capabilities of the ML tools to identify defects.
For simplicity, we assume that all the stations are aligned in series without buffers, minimizing unnecessary complications in our estimations. To quantify the beneficial effects of implementing ML-based quality control, we adopt the Overall Equipment Effectiveness (OEE) as the primary metric for the analysis. OEE is a comprehensive measure derived from the product of three critical factors, as outlined by Nota et al. ( 2020 ): Availability (the ratio of operating time with respect to planned production time); Performance (the ratio of actual output with respect to the theoretical maximum output); and Quality (the ratio of the good units with respect to the total units produced). In this section, we will discuss the details of how we calculate each of these factors for the various scenarios.
To calculate Availability ( A ), we consider an 8-hour work shift ( \(t_{shift}\) ) with 30 minutes of breaks ( \(t_{break}\) ) during which we assume production stop (except for the fully automated scenario), and 30 minutes of scheduled downtime ( \(t_{sched}\) ) required for machine cleaning and startup procedures. For unscheduled downtime ( \(t_{unsched}\) ), primarily due to machine breakdowns, we assume an average breakdown probability ( \(\rho _{down}\) ) of 5% for each machine, with an average repair time of one hour per incident ( \(t_{down}\) ). Based on these assumptions, since the Availability represents the ratio of run time ( \(t_{run}\) ) to production time ( \(t_{pt}\) ), it can be calculated using the following formula:
with the unscheduled downtime being computed as follows:
where N is the number of machines in the production line and \(1-\left( 1-\rho _{down}\right) ^{N}\) represents the probability that at least one machine breaks during the work shift. For the sake of simplicity, the \(t_{down}\) is assumed constant regardless of the number of failures.
Table 2 presents the numerical values used to calculate Availability in the three scenarios. In the second scenario, we can observe that integrating the automated station leads to a decrease in the first factor of the OEE analysis, which can be attributed to the additional station for automated quality-control (and the related potential failure). This ultimately increases the estimation of the unscheduled downtime. In the third scenario, the detrimental effect of the additional station compensates the beneficial effect of the automated quality control on reducing the need for pauses during operator breaks; thus, the Availability for the third scenario yields as substantially equivalent to the first one (baseline).
The second factor of OEE, Performance ( P ), assesses the operational efficiency of production equipment relative to its maximum designed speed ( \(t_{line}\) ). This evaluation includes accounting for reductions in cycle speed and minor stoppages, collectively termed as speed losses . These losses are challenging to measure in advance, as performance is typically measured using historical data from the production line. For this analysis, we are constrained to hypothesize a reasonable estimate of 60 seconds of time lost to speed losses ( \(t_{losses}\) ) for each work cycle. Although this assumption may appear strong, it will become evident later that, within the context of this analysis – particularly regarding the impact of automated inspection on energy savings – the Performance (like the Availability) is only marginally influenced by introducing an automated inspection station. To account for the effect of automated inspection on the assembly line speed, we keep the time required by the other 13 stations ( \(t^*_{line}\) ) constant while varying the time allocated for visual inspection ( \(t_{inspect}\) ). According to Burduk and Górnicka ( 2017 ), the total operation time of the production line, excluding inspection, is 1263 seconds, with manual visual inspection taking 38 seconds. For the fully automated third scenario, we assume an inspection time of 5 seconds, which encloses the photo collection, pre-processing, ML-analysis, and post-processing steps. In the second scenario, instead, we add an additional time to the pure automatic case to consider the cases when the confidence of the ML model falls below 90%. We assume this happens once in every 10 inspections, which is a conservative estimate, higher than that we observed during model testing. This results in adding 10% of the human inspection time to the fully automated time. Thus, when \(t_{losses}\) are known, Performance can be expressed as follows:
The calculated values for Performance are presented in Table 3 , and we can note that the modification in inspection time has a negligible impact on this factor since it does not affect the speed loss or, at least to our knowledge, there is no clear evidence to suggest that the introduction of a new inspection station would alter these losses. Moreover, given the specific linear layout of the considered production line, the inspection time change has only a marginal effect on enhancing the production speed. However, this approach could potentially bias our scenario towards always favouring automation. To evaluate this hypothesis, a sensitivity analysis which explores scenarios where the production line operates at a faster pace will be discussed in the next subsection.
The last factor, Quality ( Q ), quantifies the ratio of compliant products out of the total products manufactured, effectively filtering out items that fail to meet the quality standards due to defects. Given the objective of our automated algorithm, we anticipate this factor of the OEE to be significantly enhanced by implementing the ML-based automated inspection station. To estimate it, we assume a constant defect probability for the production line ( \(\rho _{def}\) ) at 5%. Consequently, the number of defective products ( \(N_{def}\) ) during the work shift is calculated as \(N_{unit} \cdot \rho _{def}\) , where \(N_{unit}\) represents the average number of units (brake calipers) assembled on the production line, defined as:
To quantify defective units identified, we consider the inspection accuracy ( \(\rho _{acc}\) ), where for human visual inspection, the typical accuracy is 80% (Sundaram & Zeid, 2023 ), and for the ML-based station, we use the accuracy of our best model, i.e., 99%. Additionally, we account for the probability of the station mistakenly identifying a caliper as with a defect even if it is defect-free, i.e., the false negative rate ( \(\rho _{FN}\) ), defined as
In the absence of any reasonable evidence to justify a bias on one mistake over others, we assume a uniform distribution for both human and automated inspections regarding error preference, i.e. we set \(\rho ^{H}_{FN} = \rho ^{ML}_{FN} = \rho _{FN} = 50\%\) . Thus, the number of final compliant goods ( \(N_{goods}\) ), i.e., the calipers that are identified as quality-compliant, can be calculated as:
where \(N_{detect}\) is the total number of detected defective units, comprising TN (true negatives, i.e. correctly identified defective calipers) and FN (false negatives, i.e. calipers mistakenly identified as defect-free). The Quality factor can then be computed as:
Table 4 summarizes the Quality factor calculation, showcasing the substantial improvement brought by the ML-based inspection station due to its higher accuracy compared to human operators.
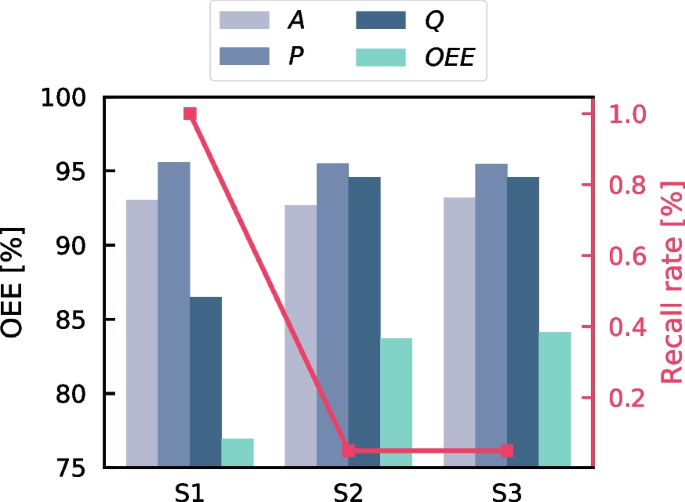
Overall Equipment Effectiveness (OEE) analysis for three scenarios (S1: Human-Based Inspection, S2: Hybrid Inspection, S3: Fully Automated Inspection). The height of the bars represents the percentage of the three factors A : Availability, P : Performance, and Q : Quality, which can be interpreted from the left axis. The green bars indicate the OEE value, derived from the product of these three factors. The red line shows the recall rate, i.e. the probability that a defective product is rejected by the client, with values displayed on the right red axis
Finally, we can determine the Overall Equipment Effectiveness by multiplying the three factors previously computed. Additionally, we can estimate the recall rate ( \(\rho _{R}\) ), which reflects the rate at which a customer might reject products. This is derived from the difference between the total number of defective units, \(N_{def}\) , and the number of units correctly identified as defective, TN , indicating the potential for defective brake calipers that may bypass the inspection process. In Fig. 8 we summarize the outcomes of the three scenarios. It is crucial to note that the scenarios incorporating the automated defect detector, S2 and S3, significantly enhance the Overall Equipment Effectiveness, primarily through substantial improvements in the Quality factor. Among these, the fully automated inspection scenario, S3, emerges as a slightly superior option, thanks to its additional benefit in removing the breaks and increasing the speed of the line. However, given the different assumptions required for this OEE study, we shall interpret these results as illustrative, and considering them primarily as comparative with the baseline scenario only. To analyze the sensitivity of the outlined scenarios on the adopted assumptions, we investigate the influence of the line speed and human accuracy on the results in the next subsection.
Sensitivity analysis
The scenarios described previously are illustrative and based on several simplifying hypotheses. One of such hypotheses is that the production chain layout operates entirely in series, with each station awaiting the arrival of the workpiece from the preceding station, resulting in a relatively slow production rate (1263 seconds). This setup can be quite different from reality, where slower operations can be accelerated by installing additional machines in parallel to balance the workload and enhance productivity. Moreover, we utilized a literature value of 80% for the accuracy of the human visual inspector operator, as reported by Sundaram and Zeid ( 2023 ). However, this accuracy can vary significantly due to factors such as the experience of the inspector and the defect type.
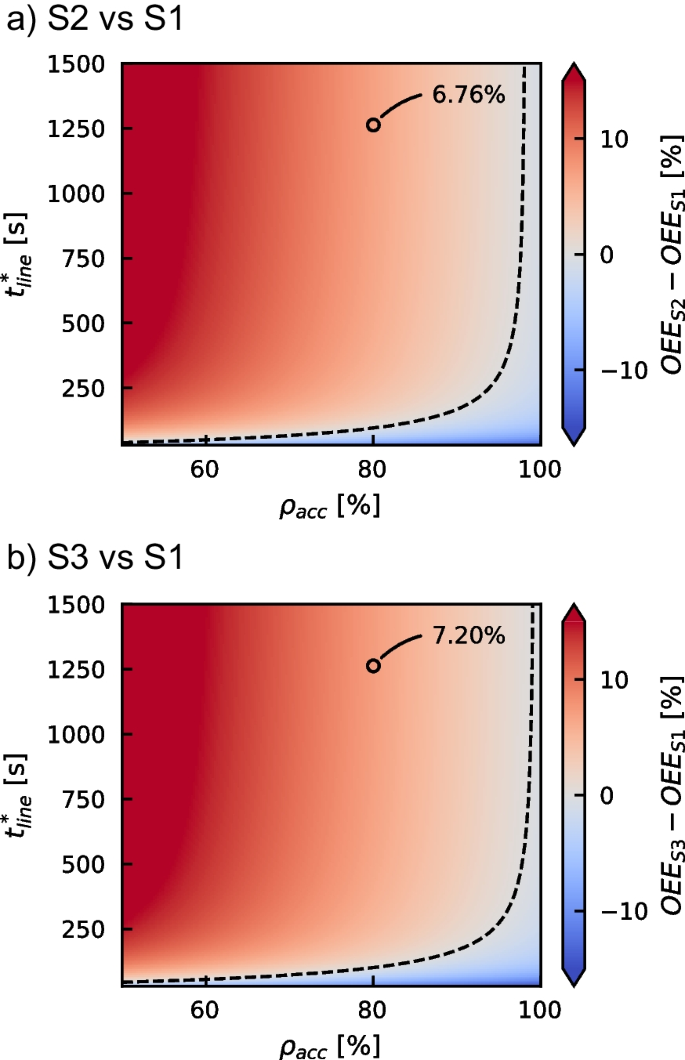
Effect of assembly time for stations (excluding visual inspection), \(t^*_{line}\) , and human inspection accuracy, \(\rho _{acc}\) , on the OEE analysis. (a) The subplot shows the difference between the scenario S2 (Hybrid Inspection) and the baseline scenario S1 (Human Inspection), while subplot (b) displays the difference between scenario S3 (Fully Automated Inspection) and the baseline. The maps indicate in red the values of \(t^*_{line}\) and \(\rho _{acc}\) where the integration of automated inspection stations can significantly improve OEE, and in blue where it may lower the score. The dashed lines denote the breakeven points, and the circled points pinpoint the values of the scenarios used in the “Illustrative scenario” Subsection.
A sensitivity analysis on these two factors was conducted to address these variations. The assembly time of the stations (excluding visual inspection), \(t^*_{line}\) , was varied from 60 s to 1500 s, and the human inspection accuracy, \(\rho _{acc}\) , ranged from 50% (akin to a random guesser) to 100% (representing an ideal visual inspector); meanwhile, the other variables were kept fixed.
The comparison of the OEE enhancement for the two scenarios employing ML-based inspection against the baseline scenario is displayed in the two maps in Fig. 9 . As the figure shows, due to the high accuracy and rapid response of the proposed automated inspection station, the area representing regions where the process may benefit energy savings in the assembly lines (indicated in red shades) is significantly larger than the areas where its introduction could degrade performance (indicated in blue shades). However, it can be also observed that the automated inspection could be superfluous or even detrimental in those scenarios where human accuracy and assembly speed are very high, indicating an already highly accurate workflow. In these cases, and particularly for very fast production lines, short times for quality control can be expected to be key (beyond accuracy) for the optimization.
Finally, it is important to remark that the blue region (areas below the dashed break-even lines) might expand if the accuracy of the neural networks for defect detection is lower when implemented in an real production line. This indicates the necessity for new rounds of active learning and an augment of the ratio of real images in the database, to eventually enhance the performance of the ML model.
Conclusions
Industrial quality control processes on manufactured parts are typically achieved by human visual inspection. This usually requires a dedicated handling system, and generally results in a slower production rate, with the associated non-optimal use of the energy resources. Based on a practical test case for quality control on brake caliper manufacturing, in this work we have reported on a developed workflow for integration of Machine Learning methods to automatize the process. The proposed approach relies on image analysis via Deep Convolutional Neural Networks. These models allow to efficiently extract information from images, thus possibly representing a valuable alternative to human inspection.
The proposed workflow relies on a two-step procedure on the images of the brake calipers: first, the background is removed from the image; second, the geometry is inspected to identify possible defects. These two steps are accomplished thanks to two dedicated neural network models, an encoder-decoder and an encoder network, respectively. Training of these neural networks typically requires a large number of representative images for the problem. Given that, one such database is not always readily available, we have presented and discussed an alternative methodology for the generation of the input database using 3D renderings. While integration of the database with real photographs was required for optimal results, this approach has allowed fast and flexible generation of a large base of representative images. The pre-processing steps required for data feeding to the neural networks and their training has been also discussed.
Several models have been tested and evaluated, and the best one for the considered case identified. The obtained accuracy for defect identification reaches \(\sim \) 99% of the tested cases. Moreover, the response of the models is fast (in the order of few seconds) on each image, which makes them compliant with the most typical industrial expectations.
In order to provide a practical example of possible energy savings when implementing the proposed ML-based methodology for quality control, we have analyzed three perspective industrial scenarios: a baseline scenario, where quality control tasks are performed by a human inspector; a hybrid scenario, where the proposed ML automatic detection tool assists the human inspector; a fully-automated scenario, where we envision a completely automated defect inspection. The results show that the proposed tools may help increasing the Overall Equipment Effectiveness up to \(\sim \) 10% with respect to the considered baseline scenario. However, a sensitivity analysis on the speed of the production line and on the accuracy of the human inspector has also shown that the automated inspection could be superfluous or even detrimental in those cases where human accuracy and assembly speed are very high. In these cases, reducing the time required for quality control can be expected to be the major controlling parameter (beyond accuracy) for optimization.
Overall the results show that, with a proper tuning, these models may represent a valuable resource for integration into production lines, with positive outcomes on the overall effectiveness, and thus ultimately leading to a better use of the energy resources. To this, while the practical implementation of the proposed tools can be expected to require contained investments (e.g. a portable camera, a dedicated workstation and an operator with proper training), in field tests on a real industrial line would be required to confirm the potential of the proposed technology.
Agrawal, R., Majumdar, A., Kumar, A., & Luthra, S. (2023). Integration of artificial intelligence in sustainable manufacturing: Current status and future opportunities. Operations Management Research, 1–22.
Alzubaidi, L., Zhang, J., Humaidi, A. J., Al-Dujaili, A., Duan, Y., Al-Shamma, O., Santamaría, J., Fadhel, M. A., Al-Amidie, M., & Farhan, L. (2021). Review of deep learning: Concepts, cnn architectures, challenges, applications, future directions. Journal of big Data, 8 , 1–74.
Article Google Scholar
Angelopoulos, A., Michailidis, E. T., Nomikos, N., Trakadas, P., Hatziefremidis, A., Voliotis, S., & Zahariadis, T. (2019). Tackling faults in the industry 4.0 era-a survey of machine—learning solutions and key aspects. Sensors, 20 (1), 109.
Arana-Landín, G., Uriarte-Gallastegi, N., Landeta-Manzano, B., & Laskurain-Iturbe, I. (2023). The contribution of lean management—industry 4.0 technologies to improving energy efficiency. Energies, 16 (5), 2124.
Badmos, O., Kopp, A., Bernthaler, T., & Schneider, G. (2020). Image-based defect detection in lithium-ion battery electrode using convolutional neural networks. Journal of Intelligent Manufacturing, 31 , 885–897. https://doi.org/10.1007/s10845-019-01484-x
Banko, M., & Brill, E. (2001). Scaling to very very large corpora for natural language disambiguation. In Proceedings of the 39th annual meeting of the association for computational linguistics (pp. 26–33).
Benedetti, M., Bonfà, F., Introna, V., Santolamazza, A., & Ubertini, S. (2019). Real time energy performance control for industrial compressed air systems: Methodology and applications. Energies, 12 (20), 3935.
Bhatt, D., Patel, C., Talsania, H., Patel, J., Vaghela, R., Pandya, S., Modi, K., & Ghayvat, H. (2021). Cnn variants for computer vision: History, architecture, application, challenges and future scope. Electronics, 10 (20), 2470.
Bilgen, S. (2014). Structure and environmental impact of global energy consumption. Renewable and Sustainable Energy Reviews, 38 , 890–902.
Blender. (2023). Open-source software. https://www.blender.org/ . Accessed 18 Apr 2023.
Bologna, A., Fasano, M., Bergamasco, L., Morciano, M., Bersani, F., Asinari, P., Meucci, L., & Chiavazzo, E. (2020). Techno-economic analysis of a solar thermal plant for large-scale water pasteurization. Applied Sciences, 10 (14), 4771.
Burduk, A., & Górnicka, D. (2017). Reduction of waste through reorganization of the component shipment logistics. Research in Logistics & Production, 7 (2), 77–90. https://doi.org/10.21008/j.2083-4950.2017.7.2.2
Carvalho, T. P., Soares, F. A., Vita, R., Francisco, R., d. P., Basto, J. P., & Alcalá, S. G. (2019). A systematic literature review of machine learning methods applied to predictive maintenance. Computers & Industrial Engineering, 137 , 106024.
Casini, M., De Angelis, P., Chiavazzo, E., & Bergamasco, L. (2024). Current trends on the use of deep learning methods for image analysis in energy applications. Energy and AI, 15 , 100330. https://doi.org/10.1016/j.egyai.2023.100330
Chai, J., Zeng, H., Li, A., & Ngai, E. W. (2021). Deep learning in computer vision: A critical review of emerging techniques and application scenarios. Machine Learning with Applications, 6 , 100134.
Chen, L. C., Zhu, Y., Papandreou, G., Schroff, F., Adam, H. (2018). Encoder-decoder with atrous separable convolution for semantic image segmentation. In Proceedings of the European conference on computer vision (ECCV) (pp. 801–818).
Chen, L., Li, S., Bai, Q., Yang, J., Jiang, S., & Miao, Y. (2021). Review of image classification algorithms based on convolutional neural networks. Remote Sensing, 13 (22), 4712.
Chen, T., Sampath, V., May, M. C., Shan, S., Jorg, O. J., Aguilar Martín, J. J., Stamer, F., Fantoni, G., Tosello, G., & Calaon, M. (2023). Machine learning in manufacturing towards industry 4.0: From ‘for now’to ‘four-know’. Applied Sciences, 13 (3), 1903. https://doi.org/10.3390/app13031903
Choudhury, A. (2021). The role of machine learning algorithms in materials science: A state of art review on industry 4.0. Archives of Computational Methods in Engineering, 28 (5), 3361–3381. https://doi.org/10.1007/s11831-020-09503-4
Dalzochio, J., Kunst, R., Pignaton, E., Binotto, A., Sanyal, S., Favilla, J., & Barbosa, J. (2020). Machine learning and reasoning for predictive maintenance in industry 4.0: Current status and challenges. Computers in Industry, 123 , 103298.
Fasano, M., Bergamasco, L., Lombardo, A., Zanini, M., Chiavazzo, E., & Asinari, P. (2019). Water/ethanol and 13x zeolite pairs for long-term thermal energy storage at ambient pressure. Frontiers in Energy Research, 7 , 148.
Géron, A. (2022). Hands-on machine learning with Scikit-Learn, Keras, and TensorFlow . O’Reilly Media, Inc.
GrabCAD. (2023). Brake caliper 3D model by Mitulkumar Sakariya from the GrabCAD free library (non-commercial public use). https://grabcad.com/library/brake-caliper-19 . Accessed 18 Apr 2023.
He, K., Zhang, X., Ren, S., & Sun, J. (2016). Deep residual learning for image recognition. In Proceedings of the IEEE conference on computer vision and pattern recognition (pp. 770–778).
Ho, S., Zhang, W., Young, W., Buchholz, M., Al Jufout, S., Dajani, K., Bian, L., & Mozumdar, M. (2021). Dlam: Deep learning based real-time porosity prediction for additive manufacturing using thermal images of the melt pool. IEEE Access, 9 , 115100–115114. https://doi.org/10.1109/ACCESS.2021.3105362
Ismail, M. I., Yunus, N. A., & Hashim, H. (2021). Integration of solar heating systems for low-temperature heat demand in food processing industry-a review. Renewable and Sustainable Energy Reviews, 147 , 111192.
LeCun, Y., Bengio, Y., & Hinton, G. (2015). Deep learning. Nature, 521 (7553), 436–444.
Leong, W. D., Teng, S. Y., How, B. S., Ngan, S. L., Abd Rahman, A., Tan, C. P., Ponnambalam, S., & Lam, H. L. (2020). Enhancing the adaptability: Lean and green strategy towards the industry revolution 4.0. Journal of cleaner production, 273 , 122870.
Liu, Z., Wang, X., Zhang, Q., & Huang, C. (2019). Empirical mode decomposition based hybrid ensemble model for electrical energy consumption forecasting of the cement grinding process. Measurement, 138 , 314–324.
Li, G., & Zheng, X. (2016). Thermal energy storage system integration forms for a sustainable future. Renewable and Sustainable Energy Reviews, 62 , 736–757.
Maggiore, S., Realini, A., Zagano, C., & Bazzocchi, F. (2021). Energy efficiency in industry 4.0: Assessing the potential of industry 4.0 to achieve 2030 decarbonisation targets. International Journal of Energy Production and Management, 6 (4), 371–381.
Mazzei, D., & Ramjattan, R. (2022). Machine learning for industry 4.0: A systematic review using deep learning-based topic modelling. Sensors, 22 (22), 8641.
Md, A. Q., Jha, K., Haneef, S., Sivaraman, A. K., & Tee, K. F. (2022). A review on data-driven quality prediction in the production process with machine learning for industry 4.0. Processes, 10 (10), 1966. https://doi.org/10.3390/pr10101966
Minaee, S., Boykov, Y., Porikli, F., Plaza, A., Kehtarnavaz, N., & Terzopoulos, D. (2021). Image segmentation using deep learning: A survey. IEEE transactions on pattern analysis and machine intelligence, 44 (7), 3523–3542.
Google Scholar
Mishra, S., Srivastava, R., Muhammad, A., Amit, A., Chiavazzo, E., Fasano, M., & Asinari, P. (2023). The impact of physicochemical features of carbon electrodes on the capacitive performance of supercapacitors: a machine learning approach. Scientific Reports, 13 (1), 6494. https://doi.org/10.1038/s41598-023-33524-1
Mumuni, A., & Mumuni, F. (2022). Data augmentation: A comprehensive survey of modern approaches. Array, 16 , 100258. https://doi.org/10.1016/j.array.2022.100258
Mypati, O., Mukherjee, A., Mishra, D., Pal, S. K., Chakrabarti, P. P., & Pal, A. (2023). A critical review on applications of artificial intelligence in manufacturing. Artificial Intelligence Review, 56 (Suppl 1), 661–768.
Narciso, D. A., & Martins, F. (2020). Application of machine learning tools for energy efficiency in industry: A review. Energy Reports, 6 , 1181–1199.
Nota, G., Nota, F. D., Peluso, D., & Toro Lazo, A. (2020). Energy efficiency in industry 4.0: The case of batch production processes. Sustainability, 12 (16), 6631. https://doi.org/10.3390/su12166631
Ocampo-Martinez, C., et al. (2019). Energy efficiency in discrete-manufacturing systems: Insights, trends, and control strategies. Journal of Manufacturing Systems, 52 , 131–145.
Pan, Y., Hao, L., He, J., Ding, K., Yu, Q., & Wang, Y. (2024). Deep convolutional neural network based on self-distillation for tool wear recognition. Engineering Applications of Artificial Intelligence, 132 , 107851.
Qin, J., Liu, Y., Grosvenor, R., Lacan, F., & Jiang, Z. (2020). Deep learning-driven particle swarm optimisation for additive manufacturing energy optimisation. Journal of Cleaner Production, 245 , 118702.
Rahul, M., & Chiddarwar, S. S. (2023). Integrating virtual twin and deep neural networks for efficient and energy-aware robotic deburring in industry 4.0. International Journal of Precision Engineering and Manufacturing, 24 (9), 1517–1534.
Ribezzo, A., Falciani, G., Bergamasco, L., Fasano, M., & Chiavazzo, E. (2022). An overview on the use of additives and preparation procedure in phase change materials for thermal energy storage with a focus on long term applications. Journal of Energy Storage, 53 , 105140.
Shahin, M., Chen, F. F., Hosseinzadeh, A., Bouzary, H., & Shahin, A. (2023). Waste reduction via image classification algorithms: Beyond the human eye with an ai-based vision. International Journal of Production Research, 1–19.
Shen, F., Zhao, L., Du, W., Zhong, W., & Qian, F. (2020). Large-scale industrial energy systems optimization under uncertainty: A data-driven robust optimization approach. Applied Energy, 259 , 114199.
Simonyan, K., & Zisserman, A. (2014). Very deep convolutional networks for large-scale image recognition. arXiv preprint arXiv:1409.1556 .
Sundaram, S., & Zeid, A. (2023). Artificial Intelligence-Based Smart Quality Inspection for Manufacturing. Micromachines, 14 (3), 570. https://doi.org/10.3390/mi14030570
Szegedy, C., Ioffe, S., Vanhoucke, V., & Alemi, A. (2017). Inception-v4, inception-resnet and the impact of residual connections on learning. In Proceedings of the AAAI conference on artificial intelligence (vol. 31).
Szegedy, C., Liu, W., Jia, Y., Sermanet, P., Reed, S., Anguelov, D., Erhan, D., Vanhoucke, V., & Rabinovich, A. (2015). Going deeper with convolutions. In Proceedings of the IEEE conference on computer vision and pattern recognition (pp. 1–9).
Trezza, G., Bergamasco, L., Fasano, M., & Chiavazzo, E. (2022). Minimal crystallographic descriptors of sorption properties in hypothetical mofs and role in sequential learning optimization. npj Computational Materials, 8 (1), 123. https://doi.org/10.1038/s41524-022-00806-7
Vater, J., Schamberger, P., Knoll, A., & Winkle, D. (2019). Fault classification and correction based on convolutional neural networks exemplified by laser welding of hairpin windings. In 2019 9th International Electric Drives Production Conference (EDPC) (pp. 1–8). IEEE.
Wen, L., Li, X., Gao, L., & Zhang, Y. (2017). A new convolutional neural network-based data-driven fault diagnosis method. IEEE Transactions on Industrial Electronics, 65 (7), 5990–5998. https://doi.org/10.1109/TIE.2017.2774777
Willenbacher, M., Scholten, J., & Wohlgemuth, V. (2021). Machine learning for optimization of energy and plastic consumption in the production of thermoplastic parts in sme. Sustainability, 13 (12), 6800.
Zhang, X. H., Zhu, Q. X., He, Y. L., & Xu, Y. (2018). Energy modeling using an effective latent variable based functional link learning machine. Energy, 162 , 883–891.
Download references
Acknowledgements
This work has been supported by GEFIT S.p.a.
Open access funding provided by Politecnico di Torino within the CRUI-CARE Agreement.
Author information
Authors and affiliations.
Department of Energy, Politecnico di Torino, Turin, Italy
Mattia Casini, Paolo De Angelis, Paolo Vigo, Matteo Fasano, Eliodoro Chiavazzo & Luca Bergamasco
R &D Department, GEFIT S.p.a., Alessandria, Italy
Marco Porrati
You can also search for this author in PubMed Google Scholar
Corresponding author
Correspondence to Luca Bergamasco .
Ethics declarations
Conflict of interest statement.
The authors declare no competing interests.
Additional information
Publisher's note.
Springer Nature remains neutral with regard to jurisdictional claims in published maps and institutional affiliations.
Supplementary Information
Below is the link to the electronic supplementary material.
Supplementary file 1 (pdf 354 KB)
Rights and permissions.
Open Access This article is licensed under a Creative Commons Attribution 4.0 International License, which permits use, sharing, adaptation, distribution and reproduction in any medium or format, as long as you give appropriate credit to the original author(s) and the source, provide a link to the Creative Commons licence, and indicate if changes were made. The images or other third party material in this article are included in the article’s Creative Commons licence, unless indicated otherwise in a credit line to the material. If material is not included in the article’s Creative Commons licence and your intended use is not permitted by statutory regulation or exceeds the permitted use, you will need to obtain permission directly from the copyright holder. To view a copy of this licence, visit http://creativecommons.org/licenses/by/4.0/ .
Reprints and permissions
About this article
Casini, M., De Angelis, P., Porrati, M. et al. Machine Learning and image analysis towards improved energy management in Industry 4.0: a practical case study on quality control. Energy Efficiency 17 , 48 (2024). https://doi.org/10.1007/s12053-024-10228-7
Download citation
Received : 22 July 2023
Accepted : 28 April 2024
Published : 13 May 2024
DOI : https://doi.org/10.1007/s12053-024-10228-7
Share this article
Anyone you share the following link with will be able to read this content:
Sorry, a shareable link is not currently available for this article.
Provided by the Springer Nature SharedIt content-sharing initiative
- Industry 4.0
- Energy management
- Artificial intelligence
- Machine learning
- Deep learning
- Convolutional neural networks
- Computer vision
- Find a journal
- Publish with us
- Track your research

IMAGES
VIDEO
COMMENTS
A cross-case analysis is an act of comparing and contrasting the patterns emerging from the detailed case write-ups (Benbasat et al., 1987; Eisenhardt, ... it was clear that a significant portion of case studies in operations management are concentrated in a specific geographical area, which includes the United States (n = 12). While in other ...
The 10 Strategic Decision Areas of Operations Management at Walmart. 1. Design of Goods and Services. This decision area of operations management involves the strategic characterization of the retail company's products. In this case, the decision area covers Walmart's goods and services. As a retailer, the company offers retail services.
Operations Management. Browse operations management learning materials including case studies, simulations, and online courses. Introduce core concepts and real-world challenges to create memorable learning experiences for your students.
Netessine S., and R. Shumsky. "Introduction to the Theory and Practice of Yield Management." INFORMS Transactions on Education 3, no. 1 (2002): 34-44. [MSD] Chapter 16. 21 Revenue management II + Break.com case Case. Roels, Guillaume, and Tyler Skowrup. "Break.com." (PDF) UCLA Anderson School of Management Case. UCLA. October 2008. 22
Master of Science in Management Studies. Combine an international MBA with a deep dive into management science. A special opportunity for partner and affiliate schools only. ... Operations Management Case Studies. Teaching Resources Library A Background Note on "Unskilled" Jobs in the United States - Past, Present, and Future
Simply, a 'case' is an instance of something, particularly of the phenomenon under study. In operations management that could include a case of implementing a technology, or a case of changing the design of operations in a facility or a case of changing the way operations are managed. A case could also include a case of failure or of some ...
This article reviews the case study research in the operations management field. In this regard, the paper's key objective is to. represent a general framework to design, develop, and conduct ...
In areas related to operations management, such as com-puter science, the term 'case study' is often used to refer to the performance of a system 'under' certain conditions. This can be the understanding in the context of simulation or optimisation, 2016 informa uK limited, trading as taylor & Francis group.
Journal of Operations Management, 11 (1993) 239-256 Eisevier 239 Conducting case study research in operations management David M. McCutcheon and Jack R. Meredith Department of Operations Management and Information Systems, University of Cincinnati, Cincinnati, OH 4522]-0387, USA (Received 8 October 1990; accepted in revised form 3 March 1993) Abstract Recently, there have been numerous calls ...
Moreover, the case study method is viewed with scepticism by those who consider it to be a weak form of research, one that lacks rigor and objectivity. Here, we offer an introduction to the case study method for OM researchers who may have little background in field based research. We provide an outline of the procedure and cite some excellent ...
Abstract. This paper reviews the use of case study research in operations management for theory development and testing. It draws on the literature on case research in a number of disciplines and uses examples drawn from operations management research. It provides guidelines and a roadmap for operations management researchers wishing to design ...
" MIT Sloan Case. MIT Sloan School of Management. Case: 11-116, January 3, 2012. Construct a process flow diagram of the PATA visit from a patient's perspective. Calculate the capacity and utilization rate at each step in the process. Use capacity analysis tools (build-up diagrams or/and queuing) to decide if and where there is a bottleneck ...
OPERATIONS MANAGEMENT Sustainability and Supply Chain Management HEIZER J A Y RENDER ... Using Software for Productivity Analysis 21 Solved Problems 21 Problems 22 CASE STUDY 24 Uber Technologies, Inc. 24 VIDEO CASE STUDIES 24 Frito-Lay: Operations Management in Manufacturing 24 Hard Rock Cafe: Operations Management in Services 25
Starbucks' Operations Management - 10 Critical Decisions. 1. Goods and Services require decisions on the characteristics of business processes to meet the target features and quality of Starbucks products. This decision area of operations management affects other areas of the coffeehouse business. For example, the specifications of ...
Sasser, W. Earl, Kim B. Clark, David A. Garvin, Margaret B.W. Graham, Ramchandran Jaikumar, and David H. Maister. Cases in Operations Management: Analysis and Action ...
What is case study analysis? A case study presents an account of what happened to a business or industry over a number of years. It chronicles the events that managers had to deal with, such as changes in the competitive environment, and charts the managers' response, which usually involved changing the business- or corporate-level strategy.
Strategy& was tasked with reshaping the company starting from product-market-strategy, developing the organizational structure and optimizing the entire process and operations landscape. An overall restructuring concept based on two pillars was developed: 1) Urgent short-term actions focusing on firefighting to ensure customer satisfaction and ...
Representing a broad range of management subjects, the ICMR Case Collection provides teachers, corporate trainers, and management professionals with a variety of teaching and reference material. The collection consists of Operations case studies and research reports on a wide range of companies and industries - both Indian and international, cases won awards in varies competitions, EFMD Case ...
The case is designed to be used in courses on Nonprofit Operations Management, Data Analytics, Six Sigma, and Business Process Excellence/Improvement in MBA or Executive MBA programs. It is suitable for teaching students about the common problem of lower rates of volunteerism in nonprofit organizations. Further, the case study helps present the ...
A cross-case analysis is an act of comparing and contrasting the patterns emerging from the detailed case write-ups (Benbasat et al., 1987; Eisenhardt, ... it was clear that a significant portion of case studies in operations management are concentrated in a specific geographical area, which includes the United States (n = 12). While in other ...
by Ryan W. Buell, Kamalini Ramdas, and Nazlı Sönmez. Shared service delivery means that customers are served in groups rather than individually. Results from a large-scale study of glaucoma follow-up appointments at a major eye hospital indicate that shared service delivery can significantly improve patients' verbal and non-verbal engagement.
Fifty four percent of raw case users came from outside the U.S.. The Yale School of Management (SOM) case study directory pages received over 160K page views from 177 countries with approximately a third originating in India followed by the U.S. and the Philippines. Twenty-six of the cases in the list are raw cases.
Yuval Atsmon: When people talk about artificial intelligence, they include everything to do with analytics, automation, and data analysis. Marvin Minsky, the pioneer of artificial intelligence research in the 1960s, talked about AI as a "suitcase word"—a term into which you can stuff whatever you want—and that still seems to be the case.
With the advent of Industry 4.0, Artificial Intelligence (AI) has created a favorable environment for the digitalization of manufacturing and processing, helping industries to automate and optimize operations. In this work, we focus on a practical case study of a brake caliper quality control operation, which is usually accomplished by human inspection and requires a dedicated handling system ...
Access the portal of NASS, the official source of agricultural data and statistics in the US, and explore various reports and products.