- Utility Menu


GA4 Tracking Code

fa51e2b1dc8cca8f7467da564e77b5ea
- Make a Gift
- Join Our Email List
- Problem Solving in STEM
Solving problems is a key component of many science, math, and engineering classes. If a goal of a class is for students to emerge with the ability to solve new kinds of problems or to use new problem-solving techniques, then students need numerous opportunities to develop the skills necessary to approach and answer different types of problems. Problem solving during section or class allows students to develop their confidence in these skills under your guidance, better preparing them to succeed on their homework and exams. This page offers advice about strategies for facilitating problem solving during class.
How do I decide which problems to cover in section or class?
In-class problem solving should reinforce the major concepts from the class and provide the opportunity for theoretical concepts to become more concrete. If students have a problem set for homework, then in-class problem solving should prepare students for the types of problems that they will see on their homework. You may wish to include some simpler problems both in the interest of time and to help students gain confidence, but it is ideal if the complexity of at least some of the in-class problems mirrors the level of difficulty of the homework. You may also want to ask your students ahead of time which skills or concepts they find confusing, and include some problems that are directly targeted to their concerns.
You have given your students a problem to solve in class. What are some strategies to work through it?
- Try to give your students a chance to grapple with the problems as much as possible. Offering them the chance to do the problem themselves allows them to learn from their mistakes in the presence of your expertise as their teacher. (If time is limited, they may not be able to get all the way through multi-step problems, in which case it can help to prioritize giving them a chance to tackle the most challenging steps.)
- When you do want to teach by solving the problem yourself at the board, talk through the logic of how you choose to apply certain approaches to solve certain problems. This way you can externalize the type of thinking you hope your students internalize when they solve similar problems themselves.
- Start by setting up the problem on the board (e.g you might write down key variables and equations; draw a figure illustrating the question). Ask students to start solving the problem, either independently or in small groups. As they are working on the problem, walk around to hear what they are saying and see what they are writing down. If several students seem stuck, it might be a good to collect the whole class again to clarify any confusion. After students have made progress, bring the everyone back together and have students guide you as to what to write on the board.
- It can help to first ask students to work on the problem by themselves for a minute, and then get into small groups to work on the problem collaboratively.
- If you have ample board space, have students work in small groups at the board while solving the problem. That way you can monitor their progress by standing back and watching what they put up on the board.
- If you have several problems you would like to have the students practice, but not enough time for everyone to do all of them, you can assign different groups of students to work on different – but related - problems.
When do you want students to work in groups to solve problems?
- Don’t ask students to work in groups for straightforward problems that most students could solve independently in a short amount of time.
- Do have students work in groups for thought-provoking problems, where students will benefit from meaningful collaboration.
- Even in cases where you plan to have students work in groups, it can be useful to give students some time to work on their own before collaborating with others. This ensures that every student engages with the problem and is ready to contribute to a discussion.
What are some benefits of having students work in groups?
- Students bring different strengths, different knowledge, and different ideas for how to solve a problem; collaboration can help students work through problems that are more challenging than they might be able to tackle on their own.
- In working in a group, students might consider multiple ways to approach a problem, thus enriching their repertoire of strategies.
- Students who think they understand the material will gain a deeper understanding by explaining concepts to their peers.
What are some strategies for helping students to form groups?
- Instruct students to work with the person (or people) sitting next to them.
- Count off. (e.g. 1, 2, 3, 4; all the 1’s find each other and form a group, etc)
- Hand out playing cards; students need to find the person with the same number card. (There are many variants to this. For example, you can print pictures of images that go together [rain and umbrella]; each person gets a card and needs to find their partner[s].)
- Based on what you know about the students, assign groups in advance. List the groups on the board.
- Note: Always have students take the time to introduce themselves to each other in a new group.
What should you do while your students are working on problems?
- Walk around and talk to students. Observing their work gives you a sense of what people understand and what they are struggling with. Answer students’ questions, and ask them questions that lead in a productive direction if they are stuck.
- If you discover that many people have the same question—or that someone has a misunderstanding that others might have—you might stop everyone and discuss a key idea with the entire class.
After students work on a problem during class, what are strategies to have them share their answers and their thinking?
- Ask for volunteers to share answers. Depending on the nature of the problem, student might provide answers verbally or by writing on the board. As a variant, for questions where a variety of answers are relevant, ask for at least three volunteers before anyone shares their ideas.
- Use online polling software for students to respond to a multiple-choice question anonymously.
- If students are working in groups, assign reporters ahead of time. For example, the person with the next birthday could be responsible for sharing their group’s work with the class.
- Cold call. To reduce student anxiety about cold calling, it can help to identify students who seem to have the correct answer as you were walking around the class and checking in on their progress solving the assigned problem. You may even want to warn the student ahead of time: "This is a great answer! Do you mind if I call on you when we come back together as a class?"
- Have students write an answer on a notecard that they turn in to you. If your goal is to understand whether students in general solved a problem correctly, the notecards could be submitted anonymously; if you wish to assess individual students’ work, you would want to ask students to put their names on their notecard.
- Use a jigsaw strategy, where you rearrange groups such that each new group is comprised of people who came from different initial groups and had solved different problems. Students now are responsible for teaching the other students in their new group how to solve their problem.
- Have a representative from each group explain their problem to the class.
- Have a representative from each group draw or write the answer on the board.
What happens if a student gives a wrong answer?
- Ask for their reasoning so that you can understand where they went wrong.
- Ask if anyone else has other ideas. You can also ask this sometimes when an answer is right.
- Cultivate an environment where it’s okay to be wrong. Emphasize that you are all learning together, and that you learn through making mistakes.
- Do make sure that you clarify what the correct answer is before moving on.
- Once the correct answer is given, go through some answer-checking techniques that can distinguish between correct and incorrect answers. This can help prepare students to verify their future work.
How can you make your classroom inclusive?
- The goal is that everyone is thinking, talking, and sharing their ideas, and that everyone feels valued and respected. Use a variety of teaching strategies (independent work and group work; allow students to talk to each other before they talk to the class). Create an environment where it is normal to struggle and make mistakes.
- See Kimberly Tanner’s article on strategies to promoste student engagement and cultivate classroom equity.
A few final notes…
- Make sure that you have worked all of the problems and also thought about alternative approaches to solving them.
- Board work matters. You should have a plan beforehand of what you will write on the board, where, when, what needs to be added, and what can be erased when. If students are going to write their answers on the board, you need to also have a plan for making sure that everyone gets to the correct answer. Students will copy what is on the board and use it as their notes for later study, so correct and logical information must be written there.
For more information...
Tipsheet: Problem Solving in STEM Sections
Tanner, K. D. (2013). Structure matters: twenty-one teaching strategies to promote student engagement and cultivate classroom equity . CBE-Life Sciences Education, 12(3), 322-331.
- Designing Your Course
- A Teaching Timeline: From Pre-Term Planning to the Final Exam
- The First Day of Class
- Group Agreements
- Classroom Debate
- Flipped Classrooms
- Leading Discussions
- Polling & Clickers
- Teaching with Cases
- Engaged Scholarship
- Devices in the Classroom
- Beyond the Classroom
- On Professionalism
- Getting Feedback
- Equitable & Inclusive Teaching
- Advising and Mentoring
- Teaching and Your Career
- Teaching Remotely
- Tools and Platforms
- The Science of Learning
- Bok Publications
- Other Resources Around Campus
Change Password
Your password must have 8 characters or more and contain 3 of the following:.
- a lower case character,
- an upper case character,
- a special character
Password Changed Successfully
Your password has been changed
- Sign in / Register
Request Username
Can't sign in? Forgot your username?
Enter your email address below and we will send you your username
If the address matches an existing account you will receive an email with instructions to retrieve your username
Teaching Creativity and Inventive Problem Solving in Science
- Robert L. DeHaan
Division of Educational Studies, Emory University, Atlanta, GA 30322
Search for more papers by this author
Engaging learners in the excitement of science, helping them discover the value of evidence-based reasoning and higher-order cognitive skills, and teaching them to become creative problem solvers have long been goals of science education reformers. But the means to achieve these goals, especially methods to promote creative thinking in scientific problem solving, have not become widely known or used. In this essay, I review the evidence that creativity is not a single hard-to-measure property. The creative process can be explained by reference to increasingly well-understood cognitive skills such as cognitive flexibility and inhibitory control that are widely distributed in the population. I explore the relationship between creativity and the higher-order cognitive skills, review assessment methods, and describe several instructional strategies for enhancing creative problem solving in the college classroom. Evidence suggests that instruction to support the development of creativity requires inquiry-based teaching that includes explicit strategies to promote cognitive flexibility. Students need to be repeatedly reminded and shown how to be creative, to integrate material across subject areas, to question their own assumptions, and to imagine other viewpoints and possibilities. Further research is required to determine whether college students' learning will be enhanced by these measures.
INTRODUCTION
Dr. Dunne paces in front of his section of first-year college students, today not as their Bio 110 teacher but in the role of facilitator in their monthly “invention session.” For this meeting, the topic is stem cell therapy in heart disease. Members of each team of four students have primed themselves on the topic by reading selected articles from accessible sources such as Science, Nature, and Scientific American, and searching the World Wide Web, triangulating for up-to-date, accurate, background information. Each team knows that their first goal is to define a set of problems or limitations to overcome within the topic and to begin to think of possible solutions. Dr. Dunne starts the conversation by reminding the group of the few ground rules: one speaker at a time, listen carefully and have respect for others' ideas, question your own and others' assumptions, focus on alternative paths or solutions, maintain an atmosphere of collaboration and mutual support. He then sparks the discussion by asking one of the teams to describe a problem in need of solution.
Science in the United States is widely credited as a major source of discovery and economic development. According to the 2005 TAP Report produced by a prominent group of corporate leaders, “To maintain our country's competitiveness in the twenty-first century, we must cultivate the skilled scientists and engineers needed to create tomorrow's innovations.” ( www.tap2015.org/about/TAP_report2.pdf ). A panel of scientists, engineers, educators, and policy makers convened by the National Research Council (NRC) concurred with this view, reporting that the vitality of the nation “is derived in large part from the productivity of well-trained people and the steady stream of scientific and technical innovations they produce” ( NRC, 2007 ).
For many decades, science education reformers have promoted the idea that learners should be engaged in the excitement of science; they should be helped to discover the value of evidence-based reasoning and higher-order cognitive skills, and be taught to become innovative problem solvers (for reviews, see DeHaan, 2005 ; Hake, 2005 ; Nelson, 2008 ; Perkins and Wieman, 2008 ). But the means to achieve these goals, especially methods to promote creative thinking in scientific problem solving, are not widely known or used. An invention session such as that led by the fictional Dr. Dunne, described above, may seem fanciful as a means of teaching students to think about science as something more than a body of facts and terms to memorize. In recent years, however, models for promoting creative problem solving were developed for classroom use, as detailed by Treffinger and Isaksen (2005) , and such techniques are often used in the real world of high technology. To promote imaginative thinking, the advertising executive Alex F. Osborn invented brainstorming ( Osborn, 1948 , 1979 ), a technique that has since been successful in stimulating inventiveness among engineers and scientists. Could such strategies be transferred to a class for college students? Could they serve as a supplement to a high-quality, scientific teaching curriculum that helps students learn the facts and conceptual frameworks of science and make progress along the novice–expert continuum? Could brainstorming or other instructional strategies that are specifically designed to promote creativity teach students to be more adaptive in their growing expertise, more innovative in their problem-solving abilities? To begin to answer those questions, we first need to understand what is meant by “creativity.”
What Is Creativity? Big-C versus Mini-C Creativity
How to define creativity is an age-old question. Justice Potter Stewart's famous dictum regarding obscenity “I know it when I see it” has also long been an accepted test of creativity. But this is not an adequate criterion for developing an instructional approach. A scientist colleague of mine recently noted that “Many of us [in the scientific community] rarely give the creative process a second thought, imagining one either ‘has it’ or doesn't.” We often think of inventiveness or creativity in scientific fields as the kind of gift associated with a Michelangelo or Einstein. This is what Kaufman and Beghetto (2008) call big-C creativity, borrowing the term that earlier workers applied to the talents of experts in various fields who were identified as particularly creative by their expert colleagues ( MacKinnon, 1978 ). In this sense, creativity is seen as the ability of individuals to generate new ideas that contribute substantially to an intellectual domain. Howard Gardner defined such a creative person as one who “regularly solves problems, fashions products, or defines new questions in a domain in a way that is initially considered novel but that ultimately comes to be accepted in a particular cultural setting” ( Gardner, 1993 , p. 35).
But there is another level of inventiveness termed by various authors as “little-c” ( Craft, 2000 ) or “mini-c” ( Kaufman and Beghetto, 2008 ) creativity that is widespread among all populations. This would be consistent with the workplace definition of creativity offered by Amabile and her coworkers: “coming up with fresh ideas for changing products, services and processes so as to better achieve the organization's goals” ( Amabile et al. , 2005 ). Mini-c creativity is based on what Craft calls “possibility thinking” ( Craft, 2000 , pp. 3–4), as experienced when a worker suddenly has the insight to visualize a new, improved way to accomplish a task; it is represented by the “aha” moment when a student first sees two previously disparate concepts or facts in a new relationship, an example of what Arthur Koestler identified as bisociation: “perceiving a situation or event in two habitually incompatible associative contexts” ( Koestler, 1964 , p. 95).
In this essay, I maintain that mini-c creativity is not a mysterious, innate endowment of rare individuals. Instead, I argue that creative thinking is a multicomponent process, mediated through social interactions, that can be explained by reference to increasingly well-understood mental abilities such as cognitive flexibility and cognitive control that are widely distributed in the population. Moreover, I explore some of the recent research evidence (though with no effort at a comprehensive literature review) showing that these mental abilities are teachable; like other higher-order cognitive skills (HOCS), they can be enhanced by explicit instruction.
Creativity Is a Multicomponent Process
Efforts to define creativity in psychological terms go back to J. P. Guilford ( Guilford, 1950 ) and E. P. Torrance ( Torrance, 1974 ), both of whom recognized that underlying the construct were other cognitive variables such as ideational fluency, originality of ideas, and sensitivity to missing elements. Many authors since then have extended the argument that a creative act is not a singular event but a process, an interplay among several interactive cognitive and affective elements. In this view, the creative act has two phases, a generative and an exploratory or evaluative phase ( Finke et al. , 1996 ). During the generative process, the creative mind pictures a set of novel mental models as potential solutions to a problem. In the exploratory phase, we evaluate the multiple options and select the best one. Early scholars of creativity, such as J. P. Guilford, characterized the two phases as divergent thinking and convergent thinking ( Guilford, 1950 ). Guilford defined divergent thinking as the ability to produce a broad range of associations to a given stimulus or to arrive at many solutions to a problem (for overviews of the field from different perspectives, see Amabile, 1996 ; Banaji et al. , 2006 ; Sawyer, 2006 ). In neurocognitive terms, divergent thinking is referred to as associative richness ( Gabora, 2002 ; Simonton, 2004 ), which is often measured experimentally by comparing the number of words that an individual generates from memory in response to stimulus words on a word association test. In contrast, convergent thinking refers to the capacity to quickly focus on the one best solution to a problem.
The idea that there are two stages to the creative process is consistent with results from cognition research indicating that there are two distinct modes of thought, associative and analytical ( Neisser, 1963 ; Sloman, 1996 ). In the associative mode, thinking is defocused, suggestive, and intuitive, revealing remote or subtle connections between items that may be correlated, or may not, and are usually not causally related ( Burton, 2008 ). In the analytical mode, thought is focused and evaluative, more conducive to analyzing relationships of cause and effect (for a review of other cognitive aspects of creativity, see Runco, 2004 ). Science educators associate the analytical mode with the upper levels (analysis, synthesis, and evaluation) of Bloom's taxonomy (e.g., Crowe et al. , 2008 ), or with “critical thinking,” the process that underlies the “purposeful, self-regulatory judgment that drives problem-solving and decision-making” ( Quitadamo et al. , 2008 , p. 328). These modes of thinking are under cognitive control through the executive functions of the brain. The core executive functions, which are thought to underlie all planning, problem solving, and reasoning, are defined ( Blair and Razza, 2007 ) as working memory control (mentally holding and retrieving information), cognitive flexibility (considering multiple ideas and seeing different perspectives), and inhibitory control (resisting several thoughts or actions to focus on one). Readers wishing to delve further into the neuroscience of the creative process can refer to the cerebrocerebellar theory of creativity ( Vandervert et al. , 2007 ) in which these mental activities are described neurophysiologically as arising through interactions among different parts of the brain.
The main point from all of these works is that creativity is not some single hard-to-measure property or act. There is ample evidence that the creative process requires both divergent and convergent thinking and that it can be explained by reference to increasingly well-understood underlying mental abilities ( Haring-Smith, 2006 ; Kim, 2006 ; Sawyer, 2006 ; Kaufman and Sternberg, 2007 ) and cognitive processes ( Simonton, 2004 ; Diamond et al. , 2007 ; Vandervert et al. , 2007 ).
Creativity Is Widely Distributed and Occurs in a Social Context
Although it is understandable to speak of an aha moment as a creative act by the person who experiences it, authorities in the field have long recognized (e.g., Simonton, 1975 ) that creative thinking is not so much an individual trait but rather a social phenomenon involving interactions among people within their specific group or cultural settings. “Creativity isn't just a property of individuals, it is also a property of social groups” ( Sawyer, 2006 , p. 305). Indeed, Osborn introduced his brainstorming method because he was convinced that group creativity is always superior to individual creativity. He drew evidence for this conclusion from activities that demand collaborative output, for example, the improvisations of a jazz ensemble. Although each musician is individually creative during a performance, the novelty and inventiveness of each performer's playing is clearly influenced, and often enhanced, by “social and interactional processes” among the musicians ( Sawyer, 2006 , p. 120). Recently, Brophy (2006) offered evidence that for problem solving, the situation may be more nuanced. He confirmed that groups of interacting individuals were better at solving complex, multipart problems than single individuals. However, when dealing with certain kinds of single-issue problems, individual problem solvers produced a greater number of solutions than interacting groups, and those solutions were judged to be more original and useful.
Consistent with the findings of Brophy (2006) , many scholars acknowledge that creative discoveries in the real world such as solving the problems of cutting-edge science—which are usually complex and multipart—are influenced or even stimulated by social interaction among experts. The common image of the lone scientist in the laboratory experiencing a flash of creative inspiration is probably a myth from earlier days. As a case in point, the science historian Mara Beller analyzed the social processes that underlay some of the major discoveries of early twentieth-century quantum physics. Close examination of successive drafts of publications by members of the Copenhagen group revealed a remarkable degree of influence and collaboration among 10 or more colleagues, although many of these papers were published under the name of a single author ( Beller, 1999 ). Sociologists Bruno Latour and Steve Woolgar's study ( Latour and Woolgar, 1986 ) of a neuroendocrinology laboratory at the Salk Institute for Biological Studies make the related point that social interactions among the participating scientists determined to a remarkable degree what discoveries were made and how they were interpreted. In the laboratory, researchers studied the chemical structure of substances released by the brain. By analysis of the Salk scientists' verbalizations of concepts, theories, formulas, and results of their investigations, Latour and Woolgar showed that the structures and interpretations that were agreed upon, that is, the discoveries announced by the laboratory, were mediated by social interactions and power relationships among members of the laboratory group. By studying the discovery process in other fields of the natural sciences, sociologists and anthropologists have provided more cases that further illustrate how social and cultural dimensions affect scientific insights (for a thoughtful review, see Knorr Cetina, 1995 ).
In sum, when an individual experiences an aha moment that feels like a singular creative act, it may rather have resulted from a multicomponent process, under the influence of group interactions and social context. The process that led up to what may be sensed as a sudden insight will probably have included at least three diverse, but testable elements: 1) divergent thinking, including ideational fluency or cognitive flexibility, which is the cognitive executive function that underlies the ability to visualize and accept many ideas related to a problem; 2) convergent thinking or the application of inhibitory control to focus and mentally evaluate ideas; and 3) analogical thinking, the ability to understand a novel idea in terms of one that is already familiar.
LITERATURE REVIEW
What do we know about how to teach creativity.
The possibility of teaching for creative problem solving gained credence in the 1960s with the studies of Jerome Bruner, who argued that children should be encouraged to “treat a task as a problem for which one invents an answer, rather than finding one out there in a book or on the blackboard” ( Bruner, 1965 , pp. 1013–1014). Since that time, educators and psychologists have devised programs of instruction designed to promote creativity and inventiveness in virtually every student population: pre–K, elementary, high school, and college, as well as in disadvantaged students, athletes, and students in a variety of specific disciplines (for review, see Scott et al. , 2004 ). Smith (1998) identified 172 instructional approaches that have been applied at one time or another to develop divergent thinking skills.
Some of the most convincing evidence that elements of creativity can be enhanced by instruction comes from work with young children. Bodrova and Leong (2001) developed the Tools of the Mind (Tools) curriculum to improve all of the three core mental executive functions involved in creative problem solving: cognitive flexibility, working memory, and inhibitory control. In a year-long randomized study of 5-yr-olds from low-income families in 21 preschool classrooms, half of the teachers applied the districts' balanced literacy curriculum (literacy), whereas the experimenters trained the other half to teach the same academic content by using the Tools curriculum ( Diamond et al. , 2007 ). At the end of the year, when the children were tested with a battery of neurocognitive tests including a test for cognitive flexibility ( Durston et al. , 2003 ; Davidson et al. , 2006 ), those exposed to the Tools curriculum outperformed the literacy children by as much as 25% ( Diamond et al. , 2007 ). Although the Tools curriculum and literacy program were similar in academic content and in many other ways, they differed primarily in that Tools teachers spent 80% of their time explicitly reminding the children to think of alternative ways to solve a problem and building their executive function skills.
Teaching older students to be innovative also demands instruction that explicitly promotes creativity but is rigorously content-rich as well. A large body of research on the differences between novice and expert cognition indicates that creative thinking requires at least a minimal level of expertise and fluency within a knowledge domain ( Bransford et al. , 2000 ; Crawford and Brophy, 2006 ). What distinguishes experts from novices, in addition to their deeper knowledge of the subject, is their recognition of patterns in information, their ability to see relationships among disparate facts and concepts, and their capacity for organizing content into conceptual frameworks or schemata ( Bransford et al. , 2000 ; Sawyer, 2005 ).
Such expertise is often lacking in the traditional classroom. For students attempting to grapple with new subject matter, many kinds of problems that are presented in high school or college courses or that arise in the real world can be solved merely by applying newly learned algorithms or procedural knowledge. With practice, problem solving of this kind can become routine and is often considered to represent mastery of a subject, producing what Sternberg refers to as “pseudoexperts” ( Sternberg, 2003 ). But beyond such routine use of content knowledge the instructor's goal must be to produce students who have gained the HOCS needed to apply, analyze, synthesize, and evaluate knowledge ( Crowe et al. , 2008 ). The aim is to produce students who know enough about a field to grasp meaningful patterns of information, who can readily retrieve relevant knowledge from memory, and who can apply such knowledge effectively to novel problems. This condition is referred to as adaptive expertise ( Hatano and Ouro, 2003 ; Schwartz et al. , 2005 ). Instead of applying already mastered procedures, adaptive experts are able to draw on their knowledge to invent or adapt strategies for solving unique or novel problems within a knowledge domain. They are also able, ideally, to transfer conceptual frameworks and schemata from one domain to another (e.g., Schwartz et al. , 2005 ). Such flexible, innovative application of knowledge is what results in inventive or creative solutions to problems ( Crawford and Brophy, 2006 ; Crawford, 2007 ).
Promoting Creative Problem Solving in the College Classroom
In most college courses, instructors teach science primarily through lectures and textbooks that are dominated by facts and algorithmic processing rather than by concepts, principles, and evidence-based ways of thinking. This is despite ample evidence that many students gain little new knowledge from traditional lectures ( Hrepic et al. , 2007 ). Moreover, it is well documented that these methods engender passive learning rather than active engagement, boredom instead of intellectual excitement, and linear thinking rather than cognitive flexibility (e.g., Halpern and Hakel, 2003 ; Nelson, 2008 ; Perkins and Wieman, 2008 ). Cognitive flexibility, as noted, is one of the three core mental executive functions involved in creative problem solving ( Ausubel, 1963 , 2000 ). The capacity to apply ideas creatively in new contexts, referred to as the ability to “transfer” knowledge (see Mestre, 2005 ), requires that learners have opportunities to actively develop their own representations of information to convert it to a usable form. Especially when a knowledge domain is complex and fraught with ill-structured information, as in a typical introductory college biology course, instruction that emphasizes active-learning strategies is demonstrably more effective than traditional linear teaching in reducing failure rates and in promoting learning and transfer (e.g., Freeman et al. , 2007 ). Furthermore, there is already some evidence that inclusion of creativity training as part of a college curriculum can have positive effects. Hunsaker (2005) has reviewed a number of such studies. He cites work by McGregor (2001) , for example, showing that various creativity training programs including brainstorming and creative problem solving increase student scores on tests of creative-thinking abilities.
Model creativity—students develop creativity when instructors model creative thinking and inventiveness.
Repeatedly encourage idea generation—students need to be reminded to generate their own ideas and solutions in an environment free of criticism.
Cross-fertilize ideas—where possible, avoid teaching in subject-area boxes: a math box, a social studies box, etc; students' creative ideas and insights often result from learning to integrate material across subject areas.
Build self-efficacy—all students have the capacity to create and to experience the joy of having new ideas, but they must be helped to believe in their own capacity to be creative.
Constantly question assumptions—make questioning a part of the daily classroom exchange; it is more important for students to learn what questions to ask and how to ask them than to learn the answers.
Imagine other viewpoints—students broaden their perspectives by learning to reflect upon ideas and concepts from different points of view.
How Is Creativity Related to Critical Thinking and the Higher-Order Cognitive Skills?
It is not uncommon to associate creativity and ingenuity with scientific reasoning ( Sawyer, 2005 ; 2006 ). When instructors apply scientific teaching strategies ( Handelsman et al. , 2004 ; DeHaan, 2005 ; Wood, 2009 ) by using instructional methods based on learning research, according to Ebert-May and Hodder ( 2008 ), “we see students actively engaged in the thinking, creativity, rigor, and experimentation we associate with the practice of science—in much the same way we see students learn in the field and in laboratories” (p. 2). Perkins and Wieman (2008) note that “To be successful innovators in science and engineering, students must develop a deep conceptual understanding of the underlying science ideas, an ability to apply these ideas and concepts broadly in different contexts, and a vision to see their relevance and usefulness in real-world applications … An innovator is able to perceive and realize potential connections and opportunities better than others” (pp. 181–182). The results of Scott et al. (2004) suggest that nontraditional courses in science that are based on constructivist principles and that use strategies of scientific teaching to promote the HOCS and enhance content mastery and dexterity in scientific thinking ( Handelsman et al. , 2007 ; Nelson, 2008 ) also should be effective in promoting creativity and cognitive flexibility if students are explicitly guided to learn these skills.
Creativity is an essential element of problem solving ( Mumford et al. , 1991 ; Runco, 2004 ) and of critical thinking ( Abrami et al. , 2008 ). As such, it is common to think of applications of creativity such as inventiveness and ingenuity among the HOCS as defined in Bloom's taxonomy ( Crowe et al. , 2008 ). Thus, it should come as no surprise that creativity, like other elements of the HOCS, can be taught most effectively through inquiry-based instruction, informed by constructivist theory ( Ausubel, 1963 , 2000 ; Duch et al. , 2001 ; Nelson, 2008 ). In a survey of 103 instructors who taught college courses that included creativity instruction, Bull et al. (1995) asked respondents to rate the importance of various course characteristics for enhancing student creativity. Items ranking high on the list were: providing a social climate in which students feels safe, an open classroom environment that promotes tolerance for ambiguity and independence, the use of humor, metaphorical thinking, and problem defining. Many of the responses emphasized the same strategies as those advanced to promote creative problem solving (e.g., Mumford et al. , 1991 ; McFadzean, 2002 ; Treffinger and Isaksen, 2005 ) and critical thinking ( Abrami et al. , 2008 ).
In a careful meta-analysis, Scott et al. (2004) examined 70 instructional interventions designed to enhance and measure creative performance. The results were striking. Courses that stressed techniques such as critical thinking, convergent thinking, and constraint identification produced the largest positive effect sizes. More open techniques that provided less guidance in strategic approaches had less impact on the instructional outcomes. A striking finding was the effectiveness of being explicit; approaches that clearly informed students about the nature of creativity and offered clear strategies for creative thinking were most effective. Approaches such as social modeling, cooperative learning, and case-based (project-based) techniques that required the application of newly acquired knowledge were found to be positively correlated to high effect sizes. The most clear-cut result to emerge from the Scott et al. (2004) study was simply to confirm that creativity instruction can be highly successful in enhancing divergent thinking, problem solving, and imaginative performance. Most importantly, of the various cognitive processes examined, those linked to the generation of new ideas such as problem finding, conceptual combination, and idea generation showed the greatest improvement. The success of creativity instruction, the authors concluded, can be attributed to “developing and providing guidance concerning the application of requisite cognitive capacities … [and] a set of heuristics or strategies for working with already available knowledge” (p. 382).
Many of the scientific teaching practices that have been shown by research to foster content mastery and HOCS, and that are coming more widely into use, also would be consistent with promoting creativity. Wood (2009) has recently reviewed examples of such practices and how to apply them. These include relatively small modifications of the traditional lecture to engender more active learning, such as the use of concept tests and peer instruction ( Mazur, 1996 ), Just-in-Time-Teaching techniques ( Novak et al. , 1999 ), and student response systems known as “clickers” ( Knight and Wood, 2005 ; Crossgrove and Curran, 2008 ), all designed to allow the instructor to frequently and effortlessly elicit and respond to student thinking. Other strategies can transform the lecture hall into a workshop or studio classroom ( Gaffney et al. , 2008 ) where the teaching curriculum may emphasize problem-based (also known as project-based or case-based) learning strategies ( Duch et al. , 2001 ; Ebert-May and Hodder, 2008 ) or “community-based inquiry” in which students engage in research that enhances their critical-thinking skills ( Quitadamo et al. , 2008 ).
Another important approach that could readily subserve explicit creativity instruction is the use of computer-based interactive simulations, or “sims” ( Perkins and Wieman, 2008 ) to facilitate inquiry learning and effective, easy self-assessment. An example in the biological sciences would be Neurons in Action ( http://neuronsinaction.com/home/main ). In such educational environments, students gain conceptual understanding of scientific ideas through interactive engagement with materials (real or virtual), with each other, and with instructors. Following the tenets of scientific teaching, students are encouraged to pose and answer their own questions, to make sense of the materials, and to construct their own understanding. The question I pose here is whether an additional focus—guiding students to meet these challenges in a context that explicitly promotes creativity—would enhance learning and advance students' progress toward adaptive expertise?
Assessment of Creativity
To teach creativity, there must be measurable indicators to judge how much students have gained from instruction. Educational programs intended to teach creativity became popular after the Torrance Tests of Creative Thinking (TTCT) was introduced in the 1960s ( Torrance, 1974 ). But it soon became apparent that there were major problems in devising tests for creativity, both because of the difficulty of defining the construct and because of the number and complexity of elements that underlie it. Tests of intelligence and other personality characteristics on creative individuals revealed a host of related traits such as verbal fluency, metaphorical thinking, flexible decision making, tolerance of ambiguity, willingness to take risks, autonomy, divergent thinking, self-confidence, problem finding, ideational fluency, and belief in oneself as being “creative” ( Barron and Harrington, 1981 ; Tardif and Sternberg, 1988 ; Runco and Nemiro, 1994 ; Snyder et al. , 2004 ). Many of these traits have been the focus of extensive research of recent decades, but, as noted above, creativity is not defined by any one trait; there is now reason to believe that it is the interplay among the cognitive and affective processes that underlie inventiveness and the ability to find novel solutions to a problem.
Although the early creativity researchers recognized that assessing divergent thinking as a measure of creativity required tests for other underlying capacities ( Guilford, 1950 ; Torrance, 1974 ), these workers and their colleagues nonetheless believed that a high score for divergent thinking alone would correlate with real creative output. Unfortunately, no such correlation was shown ( Barron and Harrington, 1981 ). Results produced by many of the instruments initially designed to measure various aspects of creative thinking proved to be highly dependent on the test itself. A review of several hundred early studies showed that an individual's creativity score could be affected by simple test variables, for example, how the verbal pretest instructions were worded ( Barron and Harrington, 1981 , pp. 442–443). Most scholars now agree that divergent thinking, as originally defined, was not an adequate measure of creativity. The process of creative thinking requires a complex combination of elements that include cognitive flexibility, memory control, inhibitory control, and analogical thinking, enabling the mind to free-range and analogize, as well as to focus and test.
More recently, numerous psychometric measures have been developed and empirically tested (see Plucker and Renzulli, 1999 ) that allow more reliable and valid assessment of specific aspects of creativity. For example, the creativity quotient devised by Snyder et al. (2004) tests the ability of individuals to link different ideas and different categories of ideas into a novel synthesis. The Wallach–Kogan creativity test ( Wallach and Kogan, 1965 ) explores the uniqueness of ideas associated with a stimulus. For a more complete list and discussion, see the Creativity Tests website ( www.indiana.edu/∼bobweb/Handout/cretv_6.html ).
The most widely used measure of creativity is the TTCT, which has been modified four times since its original version in 1966 to take into account subsequent research. The TTCT-Verbal and the TTCT-Figural are two versions ( Torrance, 1998 ; see http://ststesting.com/2005giftttct.html ). The TTCT-Verbal consists of five tasks; the “stimulus” for each task is a picture to which the test-taker responds briefly in writing. A sample task that can be viewed from the TTCT Demonstrator website asks, “Suppose that people could transport themselves from place to place with just a wink of the eye or a twitch of the nose. What might be some things that would happen as a result? You have 3 min.” ( www.indiana.edu/∼bobweb/Handout/d3.ttct.htm ).
In the TTCT-Figural, participants are asked to construct a picture from a stimulus in the form of a partial line drawing given on the test sheet (see example below; Figure 1 ). Specific instructions are to “Add lines to the incomplete figures below to make pictures out of them. Try to tell complete stories with your pictures. Give your pictures titles. You have 3 min.” In the introductory materials, test-takers are urged to “… think of a picture or object that no one else will think of. Try to make it tell as complete and as interesting a story as you can …” ( Torrance et al. , 2008 , p. 2).
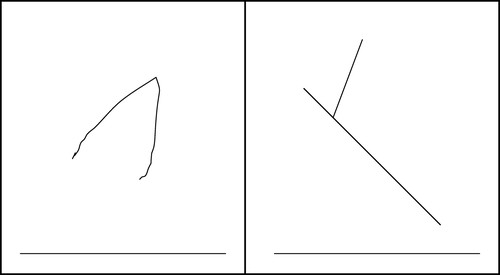
Figure 1. Sample figural test item from the TTCT Demonstrator website ( www.indiana.edu/∼bobweb/Handout/d3.ttct.htm ).
How would an instructor in a biology course judge the creativity of students' responses to such an item? To assist in this task, the TTCT has scoring and norming guides ( Torrance, 1998 ; Torrance et al. , 2008 ) with numerous samples and responses representing different levels of creativity. The guides show sample evaluations based upon specific indicators such as fluency, originality, elaboration (or complexity), unusual visualization, extending or breaking boundaries, humor, and imagery. These examples are easy to use and provide a high degree of validity and generalizability to the tests. The TTCT has been more intensively researched and analyzed than any other creativity instrument, and the norming samples have longitudinal validations and high predictive validity over a wide age range. In addition to global creativity scores, the TTCT is designed to provide outcome measures in various domains and thematic areas to allow for more insightful analysis ( Kaufman and Baer, 2006 ). Kim (2006) has examined the characteristics of the TTCT, including norms, reliability, and validity, and concludes that the test is an accurate measure of creativity. When properly used, it has been shown to be fair in terms of gender, race, community status, and language background. According to Kim (2006) and other authorities in the field ( McIntyre et al. , 2003 ; Scott et al. , 2004 ), Torrance's research and the development of the TTCT have provided groundwork for the idea that creative levels can be measured and then increased through instruction and practice.
SCIENTIFIC TEACHING TO PROMOTE CREATIVITY
How could creativity instruction be integrated into scientific teaching.
Guidelines for designing specific course units that emphasize HOCS by using strategies of scientific teaching are now available from the current literature. As an example, Karen Cloud-Hansen and colleagues ( Cloud-Hansen et al. , 2008 ) describe a course titled, “Ciprofloxacin Resistance in Neisseria gonorrhoeae .” They developed this undergraduate seminar to introduce college freshmen to important concepts in biology within a real-world context and to increase their content knowledge and critical-thinking skills. The centerpiece of the unit is a case study in which teams of students are challenged to take the role of a director of a local public health clinic. One of the county commissioners overseeing the clinic is an epidemiologist who wants to know “how you plan to address the emergence of ciprofloxacin resistance in Neisseria gonorrhoeae ” (p. 304). State budget cuts limit availability of expensive antibiotics and some laboratory tests to patients. Student teams are challenged to 1) develop a plan to address the medical, economic, and political questions such a clinic director would face in dealing with ciprofloxacin-resistant N. gonorrhoeae ; 2) provide scientific data to support their conclusions; and 3) describe their clinic plan in a one- to two-page referenced written report.
Throughout the 3-wk unit, in accordance with the principles of problem-based instruction ( Duch et al. , 2001 ), course instructors encourage students to seek, interpret, and synthesize their own information to the extent possible. Students have access to a variety of instructional formats, and active-learning experiences are incorporated throughout the unit. These activities are interspersed among minilectures and give the students opportunities to apply new information to their existing base of knowledge. The active-learning activities emphasize the key concepts of the minilectures and directly confront common misconceptions about antibiotic resistance, gene expression, and evolution. Weekly classes include question/answer/discussion sessions to address student misconceptions and 20-min minilectures on such topics as antibiotic resistance, evolution, and the central dogma of molecular biology. Students gather information about antibiotic resistance in N. gonorrhoeae , epidemiology of gonorrhea, and treatment options for the disease, and each team is expected to formulate a plan to address ciprofloxacin resistance in N. gonorrhoeae .
In this project, the authors assessed student gains in terms of content knowledge regarding topics covered such as the role of evolution in antibiotic resistance, mechanisms of gene expression, and the role of oncogenes in human disease. They also measured HOCS as gains in problem solving, according to a rubric that assessed self-reported abilities to communicate ideas logically, solve difficult problems about microbiology, propose hypotheses, analyze data, and draw conclusions. Comparing the pre- and posttests, students reported significant learning of scientific content. Among the thinking skill categories, students demonstrated measurable gains in their ability to solve problems about microbiology but the unit seemed to have little impact on their more general perceived problem-solving skills ( Cloud-Hansen et al. , 2008 ).
What would such a class look like with the addition of explicit creativity-promoting approaches? Would the gains in problem-solving abilities have been greater if during the minilectures and other activities, students had been introduced explicitly to elements of creative thinking from the Sternberg and Williams (1998) list described above? Would the students have reported greater gains if their instructors had encouraged idea generation with weekly brainstorming sessions; if they had reminded students to cross-fertilize ideas by integrating material across subject areas; built self-efficacy by helping students believe in their own capacity to be creative; helped students question their own assumptions; and encouraged students to imagine other viewpoints and possibilities? Of most relevance, could the authors have been more explicit in assessing the originality of the student plans? In an experiment that required college students to develop plans of a different, but comparable, type, Osborn and Mumford (2006) created an originality rubric ( Figure 2 ) that could apply equally to assist instructors in judging student plans in any course. With such modifications, would student gains in problem-solving abilities or other HOCS have been greater? Would their plans have been measurably more imaginative?
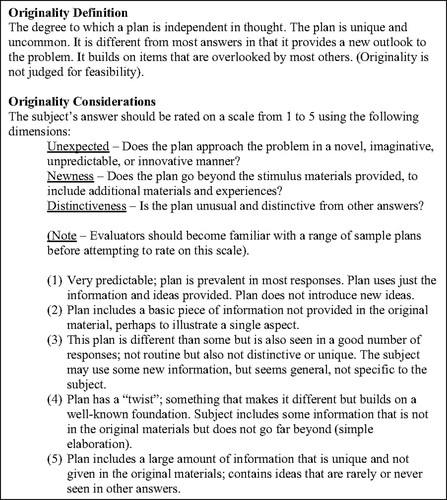
Figure 2. Originality rubric (adapted from Osburn and Mumford, 2006 , p. 183).
Answers to these questions can only be obtained when a course like that described by Cloud-Hansen et al. (2008) is taught with explicit instruction in creativity of the type I described above. But, such answers could be based upon more than subjective impressions of the course instructors. For example, students could be pretested with items from the TTCT-Verbal or TTCT-Figural like those shown. If, during minilectures and at every contact with instructors, students were repeatedly reminded and shown how to be as creative as possible, to integrate material across subject areas, to question their own assumptions and imagine other viewpoints and possibilities, would their scores on TTCT posttest items improve? Would the plans they formulated to address ciprofloxacin resistance become more imaginative?
Recall that in their meta-analysis, Scott et al. (2004) found that explicitly informing students about the nature of creativity and offering strategies for creative thinking were the most effective components of instruction. From their careful examination of 70 experimental studies, they concluded that approaches such as social modeling, cooperative learning, and case-based (project-based) techniques that required the application of newly acquired knowledge were positively correlated with high effect sizes. The study was clear in confirming that explicit creativity instruction can be successful in enhancing divergent thinking and problem solving. Would the same strategies work for courses in ecology and environmental biology, as detailed by Ebert-May and Hodder (2008) , or for a unit elaborated by Knight and Wood (2005) that applies classroom response clickers?
Finally, I return to my opening question with the fictional Dr. Dunne. Could a weekly brainstorming “invention session” included in a course like those described here serve as the site where students are introduced to concepts and strategies of creative problem solving? As frequently applied in schools of engineering ( Paulus and Nijstad, 2003 ), brainstorming provides an opportunity for the instructor to pose a problem and to ask the students to suggest as many solutions as possible in a brief period, thus enhancing ideational fluency. Here, students can be encouraged explicitly to build on the ideas of others and to think flexibly. Would brainstorming enhance students' divergent thinking or creative abilities as measured by TTCT items or an originality rubric? Many studies have demonstrated that group interactions such as brainstorming, under the right conditions, can indeed enhance creativity ( Paulus and Nijstad, 2003 ; Scott et al. , 2004 ), but there is little information from an undergraduate science classroom setting. Intellectual Ventures, a firm founded by Nathan Myhrvold, the creator of Microsoft's Research Division, has gathered groups of engineers and scientists around a table for day-long sessions to brainstorm about a prearranged topic. Here, the method seems to work. Since it was founded in 2000, Intellectual Ventures has filed hundreds of patent applications in more than 30 technology areas, applying the “invention session” strategy ( Gladwell, 2008 ). Currently, the company ranks among the top 50 worldwide in number of patent applications filed annually. Whether such a technique could be applied successfully in a college science course will only be revealed by future research.
- Abrami P. C., Bernard R. M., Borokhovski E., Wadem A., Surkes M. A., Tamim R., Zhang D. ( 2008 ). Instructional interventions affecting critical thinking skills and dispositions: a stage 1 meta-analysis . Rev. Educ. Res 78 , 1102-1134. Google Scholar
- Amabile T. M. ( 1996 ). Creativity in Context , Boulder, CO: Westview Press. Google Scholar
- Amabile T. M., Barsade S. G., Mueller J. S., Staw B. M. ( 2005 ). Affect and creativity at work . Admin. Sci. Q 50 , 367-403. Google Scholar
- Ausubel D. ( 1963 ). The Psychology of Meaningful Verbal Learning , New York: Grune and Stratton. Google Scholar
- Ausubel B. ( 2000 ). The Acquisition and Retention of Knowledge: A Cognitive View , Boston, MA: Kluwer Academic Publishers. Google Scholar
- Banaji S., Burn A., Buckingham D. ( 2006 ). The Rhetorics of Creativity: A Review of the Literature , accessed 29 December 2008 London: Centre for the Study of Children, Youth and Media, www.creativepartnerships.com/data/files/rhetorics-of-creativity-12.pdf . Google Scholar
- Barron F., Harrington D. M. ( 1981 ). Creativity, intelligence and personality . Ann. Rev. Psychol 32 , 439-476. Google Scholar
- Beller M. ( 1999 ). Quantum Dialogue: The Making of a Revolution , Chicago, IL: University of Chicago Press. Google Scholar
- Blair C., Razza R. P. ( 2007 ). Relating effortful control, executive function, and false belief understanding to emerging math and literacy ability in kindergarten . Child Dev 78 , 647-663. Medline , Google Scholar
- Bodrova E., Leong D. J. ( 2001 ). The Tool of the Mind: a case study of implementing the Vygotskian approach In: American Early Childhood and Primary Classrooms , Geneva, Switzerland: UNESCO International Bureau of Education. Google Scholar
- Bransford J. D.Brown A. L.Cocking R. R. ( 2000 ). How People Learn: Brain, Mind, Experience, and School , Washington, DC: National Academies Press. Google Scholar
- Brophy D. R. ( 2006 ). A comparison of individual and group efforts to creatively solve contrasting types of problems . Creativity Res. J 18 , 293-315. Google Scholar
- Bruner J. ( 1965 ). The growth of mind . Am. Psychol 20 , 1007-1017. Medline , Google Scholar
- Bull K. S., Montgomery D., Baloche L. ( 1995 ). Teaching creativity at the college level: a synthesis of curricular components perceived as important by instructors . Creativity Res. J 8 , 83-90. Google Scholar
- Burton R. ( 2008 ). On Being Certain: Believing You Are Right Even When You're Not , New York: St. Martin's Press. Google Scholar
- Cloud-Hanson K. A., Kuehner J. N., Tong L., Miller S., Handelsman J. ( 2008 ). Money, sex and drugs: a case study to teach the genetics of antibiotic resistance . CBE Life Sci. Educ 7 , 302-309. Medline , Google Scholar
- Craft A. ( 2000 ). Teaching Creativity: Philosophy and Practice , New York: Routledge. Google Scholar
- Crawford V. M. ( 2007 ). Adaptive expertise as knowledge building in science teachers' problem solving accessed 1 July 2008 Proceedings of the Second European Cognitive Science Conference Delphi, Greece http://ctl.sri.com/publications/downloads/Crawford_EuroCogSci07Proceedings.pdf . Google Scholar
- Crawford V. M., Brophy S. ( 2006 ). Adaptive Expertise: Theory, Methods, Findings, and Emerging Issues; September 2006 In: accessed 1 July 2008 Menlo Park, CA: SRI International, http://ctl.sri.com/publications/downloads/AESymposiumReportOct06.pdf . Google Scholar
- Crossgrove K., Curran K. L. ( 2008 ). Using clickers in nonmajors- and majors-level biology courses: student opinion, learning, and long-term retention of course material . CBE Life Sci. Educ 7 , 146-154. Link , Google Scholar
- Crowe A., Dirks C., Wenderoth M. P. ( 2008 ). Biology in bloom: implementing Bloom's taxonomy to enhance student learning in biology . CBE Life Sci. Educ 7 , 368-381. Link , Google Scholar
- Davidson M. C., Amso D., Anderson L. C., Diamond A. ( 2006 ). Development of cognitive control and executive functions from 4–13 years: evidence from manipulations of memory, inhibition, and task switching . Neuropsychologia 44 , 2037-2078. Medline , Google Scholar
- DeHaan R. L. ( 2005 ). The impending revolution in undergraduate science education . J. Sci. Educ. Technol 14 , 253-270. Google Scholar
- Diamond A., Barnett W. S., Thomas J., Munro S. ( 2007 ). Preschool program improves cognitive control . Science 318 , 1387-1388. Medline , Google Scholar
- Duch B. J., Groh S. E., Allen D. E. ( 2001 ). The Power of Problem-based Learning , Sterling, VA: Stylus Publishers. Google Scholar
- Durston S., Davidson M. C., Thomas K. M., Worden M. S., Tottenham N., Martinez A., Watts R., Ulug A. M., Caseya B. J. ( 2003 ). Parametric manipulation of conflict and response competition using rapid mixed-trial event-related fMRI . Neuroimage 20 , 2135-2141. Medline , Google Scholar
- Ebert-May D., Hodder J. ( 2008 ). Pathways to Scientific Teaching , Sunderland, MA: Sinauer. Google Scholar
- Finke R. A., Ward T. B., Smith S. M. ( 1996 ). Creative Cognition: Theory, Research and Applications , Boston, MA: MIT Press. Google Scholar
- Freeman S., O'Connor E., Parks J. W., Cunningham M., Hurley D., Haak D., Dirks C., Wenderoth M. P. ( 2007 ). Prescribed active learning increases performance in introductory biology . CBE Life Sci. Educ 6 , 132-139. Link , Google Scholar
- Gabora L. ( 2002 ). Hewett T.Kavanagh E. Cognitive mechanisms underlying the creative process Proceedings of the Fourth International Conference on Creativity and Cognition 2002 October 13–16 Loughborough University, United Kingdom 126-133. Google Scholar
- Gaffney J.D.H., Richards E., Kustusch M. B., Ding L., Beichner R. ( 2008 ). Scaling up education reform . J. Coll. Sci. Teach 37 , 48-53. Google Scholar
- Gardner H. ( 1993 ). Creating Minds: An Anatomy of Creativity Seen through the Lives of Freud, Einstein, Picasso, Stravinsky, Eliot, Graham, and Ghandi In: New York: Harper Collins. Google Scholar
- Gladwell M. ( 2008 ). In the air; who says big ideas are rare? The New Yorker accessed 19 May 2008 www.newyorker.com/reporting/2008/05/12/080512fa_fact_gladwell . Google Scholar
- Guilford J. P. ( 1950 ). Creativity . Am. Psychol 5 , 444-454. Medline , Google Scholar
- Hake R. ( 2005 ). The physics education reform effort: a possible model for higher education . Natl. Teach. Learn. Forum 15 , 1-6. Google Scholar
- Halpern D. E., Hakel M. D. ( 2003 ). Applying the science of learning to the university and beyond . Change 35 , 36-42. Google Scholar
- Handelsman J. ( 2004 ). Scientific teaching . Science 304 , 521-522. Medline , Google Scholar
- Handelsman J, Miller S., Pfund C. ( 2007 ). Scientific Teaching , New York: W. H. Freeman and Co. Google Scholar
- Haring-Smith T. ( 2006 ). Creativity research review: some lessons for higher education. Association of American Colleges and Universities . Peer Rev 8 , 23-27. Google Scholar
- Hatano G., Ouro Y. ( 2003 ). Commentary: reconceptualizing school learning using insight from expertise research . Educ. Res 32 , 26-29. Google Scholar
- Hrepic Z., Zollman D. A., Rebello N. S. ( 2007 ). Comparing students' and experts' understanding of the content of a lecture . J. Sci. Educ. Technol 16 , 213-224. Google Scholar
- Hunsaker S. L. ( 2005 ). Outcomes of creativity training programs . Gifted Child Q 49 , 292-298. Google Scholar
- Kaufman J. C., Baer J. ( 2006 ). Intelligent testing with Torrance . Creativity Res. J 18 , 99-102. Google Scholar
- Kaufman J. C., Beghetto R. A. ( 2008 , Ed. R. L. DeHaanK.M.V. Narayan , Exploring mini-C: creativity across cultures In: Education for Innovation: Implications for India, China and America , Rotterdam, The Netherlands: Sense Publishers, 165-180. Google Scholar
- Kaufman J. C., Sternberg R. J. ( 2007 ). Creativity . Change 39 , 55-58. Google Scholar
- Kim K. H. ( 2006 ). Can we trust creativity tests: a review of the Torrance Tests of Creative Thinking (TTCT) . Creativity Res. J 18 , 3-14. Google Scholar
- Knight J. K., Wood W. B. ( 2005 ). Teaching more by lecturing less . Cell Biol. Educ 4 , 298-310. Link , Google Scholar
- Cetina Knorr K. ( 1995 , Ed. S. JasanoffG. MarkleJ. PetersenT. Pinch , Laboratory studies: the cultural approach to the study of science In: Handbook of Science and Technology Studies , Thousand Oaks, CA: Sage Publications, 140-166. Google Scholar
- Koestler A. ( 1964 ). The Act of Creation , New York: Macmillan. Google Scholar
- Latour B., Woolgar S. ( 1986 ). Laboratory Life: The Construction of Scientific Facts , Princeton, NJ: Princeton University Press. Google Scholar
- MacKinnon D. W. ( 1978 , Ed. D. W. MacKinnon , What makes a person creative? In: In Search of Human Effectiveness , New York: Universe Books, 178-186. Google Scholar
- Martindale C. ( 1999 , Ed. R. J. Sternberg , Biological basis of creativity In: Handbook of Creativity , Cambridge, United Kingdom: Cambridge University Press, 137-152. Google Scholar
- Mazur E. ( 1996 ). Peer Instruction: A User's Manual , Upper Saddle River, NJ: Prentice Hall. Google Scholar
- McFadzean E. ( 2002 ). Developing and supporting creative problem-solving teams: Part 1—a conceptual model . Manage. Decis 40 , 463-475. Google Scholar
- McGregor G. D. ( 2001 ). Creative thinking instruction for a college study skills program: a case study. . Dissert Abstr. Intl 62 , 3293A UMI No. AAT 3027933. Google Scholar
- McIntyre F. S., Hite R. E., Rickard M. K. ( 2003 ). Individual characteristics and creativity in the marketing classroom: exploratory insights . J. Mark. Educ 25 , 143-149. Google Scholar
- Mestre J. P. ( 2005 ). Transfer of Learning: From a Modern Multidisciplinary Perspective , Greenwich, CT: Information Age Publishing. Google Scholar
- Mumford M. D., Mobley M. I., Uhlman C. E., Reiter-Palmon R., Doares L. M. ( 1991 ). Process analytic models of creative capacities . Creativity Res. J 4 , 91-122. Google Scholar
- National Research Council ( 2007 ). Rising Above the Gathering Storm: Energizing and Employing America for a Brighter Economic Future, Committee on Science, Engineering and Public Policy In: Washington, DC: National Academies Press. Google Scholar
- Neisser U. ( 1963 ). The multiplicity of thought . Br. J. Psychol 54 , 1-14. Medline , Google Scholar
- Nelson C. E. ( 2008 ). Teaching evolution (and all of biology) more effectively: strategies for engagement, critical reasoning, and confronting misconceptions Integrative and Comparative Biology Advance Access accessed 15 September 2008 http://icb.oxfordjournals.org/cgi/reprint/icn027v1.pdf . Google Scholar
- Novak G, Gavrin A., Christian W, Patterson E. ( 1999 ). Just-in-Time Teaching: Blending Active Learning with Web Technology , San Francisco, CA: Pearson Benjamin Cummings. Google Scholar
- Osborn A. F. ( 1948 ). Your Creative Power , New York: Scribner. Google Scholar
- Osborn A. F. ( 1979 ). Applied Imagination , New York: Scribner. Google Scholar
- Osburn H. K., Mumford M. D. ( 2006 ). Creativity and planning: training interventions to develop creative problem-solving skills . Creativity Res. J 18 , 173-190. Google Scholar
- Paulus P. B., Nijstad B. A. ( 2003 ). Group Creativity: Innovation through Collaboration , New York: Oxford University Press. Google Scholar
- Perkins K. K., Wieman C. E. ( 2008 , Ed. R. L. DeHaanK.M.V. Narayan , Innovative teaching to promote innovative thinking In: Education for Innovation: Implications for India, China and America , Rotterdam, The Netherlands: Sense Publishers, 181-210. Google Scholar
- Plucker J. A., Renzulli J. S. ( 1999 , Ed. R. J. Sternberg , Psychometric approaches to the study of human creativity In: Handbook of Creativity , Cambridge, United Kingdom: Cambridge University Press, 35-61. Google Scholar
- Quitadamo I. J., Faiola C. L., Johnson J. E., Kurtz M. J. ( 2008 ). Community-based inquiry improves critical thinking in general education biology . CBE Life Sci. Educ 7 , 327-337. Link , Google Scholar
- Runco M. A. ( 2004 ). Creativity . Annu. Rev. Psychol 55 , 657-687. Medline , Google Scholar
- Runco M. A., Nemiro J. ( 1994 ). Problem finding, creativity, and giftedness . Roeper Rev 16 , 235-241. Google Scholar
- Sawyer R. K. ( 2005 ). Educating for Innovation Thinking Skills Creativity accessed 13 August 2008 1 41-48 www.artsci.wustl.edu/∼ksawyer/PDFs/Thinkjournal.pdf . Google Scholar
- Sawyer R. K. ( 2006 ). Explaining Creativity: The Science of Human Innovation , New York: Oxford University Press. Google Scholar
- Schwartz D. L., Bransford J. D., Sears D. ( 2005 , Ed. J. P. Mestre , Efficiency and innovation in transfer In: Transfer of Learning from a Modern Multidisciplinary Perspective , Greenwich, CT: Information Age Publishing, 1-51. Google Scholar
- Scott G., Leritz L. E., Mumford M. D. ( 2004 ). The effectiveness of creativity training: a quantitative review . Creativity Res. J 16 , 361-388. Google Scholar
- Simonton D. K. ( 1975 ). Sociocultural context of individual creativity: a transhistorical time-series analysis . J. Pers. Soc. Psychol 32 , 1119-1133. Medline , Google Scholar
- Simonton D. K. ( 2004 ). Creativity in Science: Chance, Logic, Genius, and Zeitgeist , Oxford, United Kingdom: Cambridge University Press. Google Scholar
- Sloman S. ( 1996 ). The empirical case for two systems of reasoning . Psychol. Bull 9 , 3-22. Google Scholar
- Smith G. F. ( 1998 ). Idea generation techniques: a formulary of active ingredients . J. Creative Behav 32 , 107-134. Google Scholar
- Snyder A., Mitchell J., Bossomaier T., Pallier G. ( 2004 ). The creativity quotient: an objective scoring of ideational fluency . Creativity Res. J 16 , 415-420. Google Scholar
- Sternberg R. J. ( 2003 ). What is an “expert student?” . Educ. Res. 32 , 5-9. Google Scholar
- Sternberg R., Williams W. M. ( 1998 ). Teaching for creativity: two dozen tips accessed 25 March 2008 www.cdl.org/resource-library/articles/teaching_creativity.php . Google Scholar
- Tardif T. Z., Sternberg R. J. ( 1988 , Ed. R. J. Sternberg , What do we know about creativity? In: The Nature of Creativity , New York: Cambridge University Press, 429-440. Google Scholar
- Torrance E. P. ( 1974 ). Norms and Technical Manual for the Torrance Tests of Creative Thinking , Bensenville, IL: Scholastic Testing Service. Google Scholar
- Torrance E. P. ( 1998 ). The Torrance Tests of Creative Thinking Norms—Technical Manual Figural (Streamlined) Forms A and B , Bensenville, IL: Scholastic Testing Service. Google Scholar
- Torrance E. P., Ball O. E., Safter H. T. ( 2008 ). Torrance Tests of Creative Thinking: Streamlined Scoring Guide for Figural Forms A and B , Bensenville, IL: Scholastic Testing Service. Google Scholar
- Treffinger D. J., Isaksen S. G. ( 2005 ). Creative problem solving: the history, development, and implications for gifted education and talent development . Gifted Child Q 49 , 342-357. Google Scholar
- Vandervert L. R., Schimpf P. H., Liu H. ( 2007 ). How working memory and the cerebellum collaborate to produce creativity and innovation . Creativity Res. J 9 , 1-18. Google Scholar
- Wallach M. A., Kogan N. ( 1965 ). Modes of Thinking in Young Children: A Study of the Creativity-Intelligence Distinction , New York: Holt, Rinehart and Winston. Google Scholar
- Wood W. B. ( 2009 ). Innovations in undergraduate biology teaching and why we need them . Annu. Rev. Cell Dev. Biol in press. Medline , Google Scholar
- ChatGPT improves creative problem-solving performance in university students: An experimental study Computers & Education, Vol. 215
- Are we teaching novice instructional designers to be creative? A qualitative case study 16 January 2024 | Instructional Science, Vol. 52, No. 3
- Educators as agents of breadth-biased learning: using social reconstructionism as rationale for embracing media multitasking and enhancing teaching practices in higher education 3 April 2024 | Frontiers in Psychology, Vol. 15
- Searching for creativity: How people search to generate new ideas 1 December 2023 | Journal of the Association for Information Science and Technology, Vol. 75, No. 4
- Fostering creativity in low-engagement students through socratic dialogue: An experiment in an operations class The International Journal of Management Education, Vol. 22, No. 1
- Cognitive flexibility and academic performance: Individual and cross-national patterns among adolescents in 57 countries Personality and Individual Differences, Vol. 217
- What's in a word? Student beliefs and understanding about green chemistry 1 January 2024 | Chemistry Education Research and Practice, Vol. 25, No. 1
- Preservice Physical Education Teachers’ Resistance to Change: The Importance of Occupational Socialization Experiences 26 October 2023 | Trends in Higher Education, Vol. 2, No. 4
- How can we measure metacognition in creative problem-solving? Standardization of the MCPS scale Thinking Skills and Creativity, Vol. 49
- TOWARDS ENHANCING CREATIVITY AND INNOVATION IN EDUCATION SYSTEM FOR YOUTH IN HAIL REGION 1 August 2023 | Advanced Education, Vol. 10, No. 22
- Investigating the impact of innovation competence instruction in higher engineering education 12 June 2023 | European Journal of Engineering Education, Vol. 10
- Regaining creativity in science: insights from conversation 17 May 2023 | Royal Society Open Science, Vol. 10, No. 5
- How transdisciplinary integration, creativity and student motivation interact in three STEAM projects for gifted education? 29 March 2023 | Gifted Education International, Vol. 39, No. 2
- Does creative coursework predict educational, career, and community engagement outcomes for arts alumni? 15 November 2022 | Creativity Research Journal, Vol. 35, No. 2
- Make science disruptive again 27 March 2023 | Nature Biotechnology, Vol. 41, No. 4
- Promoting Creativity in Undergraduate Recreation and Leisure Services Classrooms: An Overview 19 March 2021 | SCHOLE: A Journal of Leisure Studies and Recreation Education, Vol. 38, No. 1
- A Positive Association between Working Memory Capacity and Human Creativity: A Meta-Analytic Evidence 13 January 2023 | Journal of Intelligence, Vol. 11, No. 1
- Students Creativity Through Digital Mind Map 26 July 2023
- Pedagogical and School Practices to Foster Key Competences and Domain-General Literacy 23 August 2023
- Coping with Challenges and Uncertainty in Scientific Research Asia-Pacific Science Education, Vol. 8, No. 2
- Teaching design thinking as a tool to address complex public health challenges in public health students: a case study 12 April 2022 | BMC Medical Education, Vol. 22, No. 1
- ‘Allowing them to dream’: fostering creativity in mathematics undergraduates 26 May 2022 | Journal of Further and Higher Education, Vol. 46, No. 10
- Interaction with metaphors enhances creative potential 1 July 2022 | Journal of Poetry Therapy, Vol. 35, No. 4
- Creative problem solving in knowledge-rich contexts Trends in Cognitive Sciences, Vol. 26, No. 10
- Teaching Protein–Ligand Interactions Using a Case Study on Tau in Alzheimer’s Disease 1 July 2022 | Journal of Chemical Education, Vol. 99, No. 8
- The Effectiveness of Collaborative Learning on Critical Thinking, Creative Thinking, and Metacognitive Skill Ability: Meta-Analysis on Biological Learning 15 July 2022 | European Journal of Educational Research, Vol. volume-11-2022, No. volume-11-issue-3-july-2022
- Student approaches to creative processes when participating in an open-ended project in science 30 June 2022 | International Journal of Science Education, Vol. 44, No. 10
- Arts, Machines, and Creative Education
- Perceived Learning Effectiveness and Student Satisfaction
- A Contribution to Scientific Creativity: A Validation Study Measuring Divergent Problem Solving Ability 3 September 2021 | Creativity Research Journal, Vol. 34, No. 2
- Problem Solving and Digital Transformation: Acquiring Skills through Pretend Play in Kindergarten 28 January 2022 | Education Sciences, Vol. 12, No. 2
- Growing Innovation and Collaboration Through Assessment and Feedback: A Toolkit for Assessing and Developing Students’ Soft Skills in Biological Experimentation 12 May 2022
- The Role of Creativity in Teaching Mathematics Online 1 December 2022
- Breathing Life Into Marketing Scholarship Through Creativity Learning and Teaching
- Mobilizing Research-Based Learning (RBL) in Higher Education
- Trends and opportunities by fostering creativity in science and engineering: a systematic review 2 September 2021 | European Journal of Engineering Education, Vol. 46, No. 6
- The effect of a scientific board game on improving creative problem solving skills Thinking Skills and Creativity, Vol. 41
- Create Teaching Creativity through Training Management, Effectiveness Training, and Teacher Quality in the Covid-19 Pandemic 6 August 2021 | Journal of Ethnic and Cultural Studies, Vol. 8, No. 4
- Creativity and technology in teaching and learning: a literature review of the uneasy space of implementation 11 January 2021 | Educational Technology Research and Development, Vol. 69, No. 4
- Üstün Yetenekli Öğrencilerin Bilimsel Yaratıcılık ve Bilimsel Problem Çözme ile İlgili Öz Değerlendirmeleri 15 July 2021 | Yuzunci Yil Universitesi Egitim Fakultesi Dergisi
- Cultivating creative thinking in engineering student teams: Can a computer‐mediated virtual laboratory help? 23 November 2020 | Journal of Computer Assisted Learning, Vol. 37, No. 2
- Entrepreneurial competencies of undergraduate students: The case of universities in Nigeria The International Journal of Management Education, Vol. 19, No. 1
- Promoting Creativity in General Education Mathematics Courses 5 August 2019 | PRIMUS, Vol. 31, No. 1
- Methodological Considerations for Understanding Students’ Problem Solving Processes and Affective Trajectories During Game-Based Learning: A Data Fusion Approach 3 July 2021
- The main trends in the process of building the creative potential of engineering students 18 June 2021 | E3S Web of Conferences, Vol. 274
- Research on the Present Situation and Countermeasures of Cultivating Graduate Students’ Innovation Ability under Cooperative Innovation Environment Creative Education Studies, Vol. 09, No. 02
- Innovation Centers and the Information Schools: The Influence of LIS Faculty Journal of Education for Library and Information Science, Vol. 61, No. 4
- Biomimetics: teaching the tools of the trade 28 September 2020 | FEBS Open Bio, Vol. 10, No. 11
- STEM academic teachers’ experiences of undertaking authentic assessment-led reform: a mixed method approach 21 March 2019 | Studies in Higher Education, Vol. 45, No. 9
- Problems of forming marketing competencies in the digital economy IOP Conference Series: Materials Science and Engineering, Vol. 940, No. 1
- Culturally Responsive Assessment of Physical Science Skills and Abilities: Development, Field Testing, Implementation, and Results 2 June 2020 | Journal of Advanced Academics, Vol. 31, No. 3
- Culturally Responsive Assessment of Life Science Skills and Abilities: Development, Field Testing, Implementation, and Results 3 June 2020 | Journal of Advanced Academics, Vol. 31, No. 3
- Curriculum Differentiation’s Capacity to Extend Gifted Students in Secondary Mixed-ability Science Classes 27 June 2020 | Talent, Vol. 10, No. 1
- Student Motivation from and Resistance to Active Learning Rooted in Essential Science Practices 23 December 2017 | Research in Science Education, Vol. 50, No. 1
- Integrating Entrepreneurship and Art to Improve Creative Problem Solving in Fisheries Education 27 February 2020 | Fisheries, Vol. 45, No. 2
- Concepts Re-imagined: Relational Signs Beyond Definitional Rigidity 15 August 2020
- Promoting Student Creativity and Inventiveness in Science and Engineering 24 October 2020
- Teaching for Leadership, Innovation, and Creativity
- Problem Çözme Becerileri Eğitim Programının Çocukların Karar Verme Becerileri Üzerindeki Etkisi 30 December 2019 | Erzincan Üniversitesi Eğitim Fakültesi Dergisi, Vol. 21, No. 3
- Mento’s change model in teaching competency-based medical education 27 December 2019 | BMC Medical Education, Vol. 19, No. 1
- The Effects of Individual Preparations on Group Creativity 21 January 2020
- The Value of Creativity for Enhancing Translational Ecologies, Insights, and Discoveries 9 July 2019 | Frontiers in Psychology, Vol. 10
- Using the International Classification of Functioning, Disability, and Health to Guide Students' Clinical Approach to Aging With Pathology Topics in Geriatric Rehabilitation, Vol. 35, No. 3
- Impact of Brainstorming Strategy in Dealing With Knowledge Retention Skill: An Insight Into Special Learners' Needs In Saudi Arabia 1 January 2021 | MIER Journal of Educational Studies Trends & Practices
- The potential of students’ creative disposition as a perspective to develop creative teaching and learning for senior high school biological science 12 March 2019 | Journal of Physics: Conference Series, Vol. 1157
- Evaluating Remote Experiment from a Divergent Thinking Point of View 25 July 2018
- Exploring Creative Education Practices and Implications: A Case study of National Chengchi University, Taiwan 4 April 2022 | Journal of Business and Economic Analysis, Vol. 02, No. 02
- Exploring Creative Education Practices and Implications: A Case study of National Chengchi University, Taiwan 1 January 2020 | Journal of Business and Economic Analysis, Vol. 02, No. 02
- Diverging from the Dogma: A Call to Train Creative Thinkers in Science 14 September 2018 | The Bulletin of the Ecological Society of America, Vol. 100, No. 1
- Comparison of German and Japanese student teachers’ views on creativity in chemistry class 16 May 2018 | Asia-Pacific Science Education, Vol. 4, No. 1
- The use of humour during a collaborative inquiry 27 August 2018 | International Journal of Science Education, Vol. 40, No. 14
- Connecting creative coursework exposure and college student engagement across academic disciplines 29 August 2019 | Gifted and Talented International, Vol. 33, No. 1-2
- Views of German chemistry teachers on creativity in chemistry classes and in general 1 January 2018 | Chemistry Education Research and Practice, Vol. 19, No. 3
- Embedding Critical and Creative Thinking in Chemical Engineering Practice
- Introducing storytelling to educational robotic activities
- Investigating Undergraduates’ Perceptions of Science in Courses Taught Using the CREATE Strategy Journal of Microbiology & Biology Education, Vol. 19, No. 1
- Teachers’ learning on the workshop of STS approach as a way of enhancing inventive thinking skills
- Creativity Development Through Inquiry-Based Learning in Biomedical Sciences
- The right tool for the right task: Structured techniques prove less effective on an ill-defined problem finding task Thinking Skills and Creativity, Vol. 26
- The influential factors and hierarchical structure of college students’ creative capabilities—An empirical study in Taiwan Thinking Skills and Creativity, Vol. 26
- Teacher perceptions of professional role and innovative teaching at elementary schools in Taiwan 10 November 2017 | Educational Research and Reviews, Vol. 12, No. 21
- Evaluation of creative problem-solving abilities in undergraduate structural engineers through interdisciplinary problem-based learning 28 July 2016 | European Journal of Engineering Education, Vol. 42, No. 6
- What Shall I Write Next? 19 September 2017
- Inquiry-based Laboratory Activities on Drugs Analysis for High School Chemistry Learning 3 October 2017 | Journal of Physics: Conference Series, Vol. 895
- BARRIERS TO STUDENTS’ CREATIVE EVALUATION OF UNEXPECTED EXPERIMENTAL FINDINGS 25 June 2017 | Journal of Baltic Science Education, Vol. 16, No. 3
- Kyle J. Frantz ,
- Melissa K. Demetrikopoulos ,
- Shari L. Britner ,
- Laura L. Carruth ,
- Brian A. Williams ,
- John L. Pecore ,
- Robert L. DeHaan , and
- Christopher T. Goode
- Elizabeth Ambos, Monitoring Editor
- A present absence: undergraduate course outlines and the development of student creativity across disciplines 3 October 2016 | Teaching in Higher Education, Vol. 22, No. 2
- Exploring differences in creativity across academic majors for high-ability college students 16 February 2018 | Gifted and Talented International, Vol. 32, No. 1
- Creativity in chemistry class and in general – German student teachers’ views 1 January 2017 | Chemistry Education Research and Practice, Vol. 18, No. 2
- IMPORTANCE OF CREATIVITY IN ENTREPRENEURSHIP 1 January 2017
- Accessing the Finest Minds
- Science and Innovative Thinking for Technical and Organizational Development
- Learning High School Biology in a Social Context Creative Education, Vol. 08, No. 15
- Possibilities and limitations of integrating peer instruction into technical creativity education 6 September 2016 | Instructional Science, Vol. 44, No. 6
- Creative Cognitive Processes in Higher Education 20 November 2014 | The Journal of Creative Behavior, Vol. 50, No. 4
- An Evidence-Based Review of Creative Problem Solving Tools 6 April 2016 | Human Resource Development Review, Vol. 15, No. 2
- Case-based exams for learning and assessment: Experiences in an information systems course
- Case exams for assessing higher order learning: A comparative social media analytics usage exam
- Beyond belief: Structured techniques prove more effective than a placebo intervention in a problem construction task Thinking Skills and Creativity, Vol. 19
- A Belief System at the Core of Learning Science
- Student Research Work and Modeled Situations in Order to Bridge the Gap between Basic Science Concepts and Those from Preventive and Clinical Practice. Meaningful Learning and Informed beneficience Creative Education, Vol. 07, No. 07
- FOSTERING FIFTH GRADERS’ SCIENTIFIC CREATIVITY THROUGH PROBLEM-BASED LEARNING 25 October 2015 | Journal of Baltic Science Education, Vol. 14, No. 5
- Scaffolding for Creative Product Possibilities in a Design-Based STEM Activity 16 November 2014 | Research in Science Education, Vol. 45, No. 5
- Intuition and insight: two concepts that illuminate the tacit in science education 18 June 2015 | Studies in Science Education, Vol. 51, No. 2
- Arts and crafts as adjuncts to STEM education to foster creativity in gifted and talented students 28 March 2015 | Asia Pacific Education Review, Vol. 16, No. 2
- Initiatives Towards an Education for Creativity Procedia - Social and Behavioral Sciences, Vol. 180
- Brian A. Couch ,
- Tanya L. Brown ,
- Tyler J. Schelpat ,
- Mark J. Graham , and
- Jennifer K. Knight
- Michèle Shuster, Monitoring Editor
- Kim Quillin , and
- Stephen Thomas
- Mary Lee Ledbetter, Monitoring Editor
- The Design of IdeaWorks: Applying Social Learning Networks to Support Tertiary Education 21 July 2015
- Video Games and Malevolent Creativity
- Modelling a Laboratory for Ideas as a New Tool for Fostering Engineering Creativity Procedia Engineering, Vol. 100
- “Development of Thinking Skills” Course: Teaching TRIZ in Academic Setting Procedia Engineering, Vol. 131
- Leadership in the Future Experts’ Creativity Development with Scientific Research Activities 4 November 2014
- Developing Deaf Children's Conceptual Understanding and Scientific Argumentation Skills: A Literature Review 3 January 2014 | Deafness & Education International, Vol. 16, No. 3
- Leslie M. Stevens , and
- Sally G. Hoskins
- Nancy Pelaez, Monitoring Editor
- Cortex, Vol. 51
- A Sociotechnological Theory of Discursive Change and Entrepreneurial Capacity: Novelty and Networks SSRN Electronic Journal, Vol. 3
- GEOverse: An Undergraduate Research Journal: Research Dissemination Within and Beyond the Curriculum 1 August 2013
- Learning by Practice, High-Pressure Student Ateliers 2 August 2013
- Relating Inter-Individual Differences in Verbal Creative Thinking to Cerebral Structures: An Optimal Voxel-Based Morphometry Study 5 November 2013 | PLoS ONE, Vol. 8, No. 11
- 21st Century Biology: An Interdisciplinary Approach of Biology, Technology, Engineering and Mathematics Education Procedia - Social and Behavioral Sciences, Vol. 102
- Reclaiming creativity in the era of impact: exploring ideas about creative research in science and engineering Studies in Higher Education, Vol. 38, No. 9
- An Evaluation of Alternative Ways of Computing the Creativity Quotient in a Design School Sample Creativity Research Journal, Vol. 25, No. 3
- A.-M. Hoskinson ,
- M. D. Caballero , and
- J. K. Knight
- Eric Brewe, Monitoring Editor
- Understanding, attitude and environment International Journal for Researcher Development, Vol. 4, No. 1
- Promoting Student Creativity and Inventiveness in Science and Engineering
- Building creative thinking in the classroom: From research to practice International Journal of Educational Research, Vol. 62
- A Demonstration of a Mastery Goal Driven Learning Environment to Foster Creativity in Engineering Design SSRN Electronic Journal, Vol. 111
- The development of creative cognition across adolescence: distinct trajectories for insight and divergent thinking 8 October 2012 | Developmental Science, Vol. 16, No. 1
- A CROSS-NATIONAL STUDY OF PROSPECTIVE ELEMENTARY AND SCIENCE TEACHERS’ CREATIVITY STYLES 10 September 2012 | Journal of Baltic Science Education, Vol. 11, No. 3
- Evaluation of fostering students' creativity in preparing aided recalls for revision courses using electronic revision and recapitulation tools 2.0 Behaviour & Information Technology, Vol. 31, No. 8
- Scientific Creativity: The Missing Ingredient in Slovenian Science Education 15 April 2012 | European Journal of Educational Research, Vol. volume-1-2012, No. volume1-issue2.html
- Could the ‘evolution’ from biology to life sciences prevent ‘extinction’ of the subject field? 6 March 2012 | Suid-Afrikaanse Tydskrif vir Natuurwetenskap en Tegnologie, Vol. 31, No. 1
- Mobile innovations, executive functions, and educational developments in conflict zones: a case study from Palestine 1 October 2011 | Educational Technology Research and Development, Vol. 60, No. 1
- Informing Pedagogy Through the Brain-Targeted Teaching Model Journal of Microbiology & Biology Education, Vol. 13, No. 1
- Teaching Creative Science Thinking Science, Vol. 334, No. 6062
- Sally G. Hoskins ,
- David Lopatto , and
- Leslie M. Stevens
- Diane K. O'Dowd, Monitoring Editor
- Embedding Research-Based Learning Early in the Undergraduate Geography Curriculum Journal of Geography in Higher Education, Vol. 35, No. 3
- Jared L. Taylor ,
- Karen M. Smith ,
- Adrian P. van Stolk , and
- George B. Spiegelman
- Debra Tomanek, Monitoring Editor
- IFAC Proceedings Volumes, Vol. 43, No. 17
- Critical and Creative Thinking Activities for Engaged Learning in Graphics and Visualization Course
- Creativity Development through Inquiry-Based Learning in Biomedical Sciences
Submitted: 31 December 2008 Revised: 14 May 2009 Accepted: 28 May 2009
© 2009 by The American Society for Cell Biology
An IERI – International Educational Research Institute Journal
- Open access
- Published: 10 December 2014
The acquisition of problem solving competence: evidence from 41 countries that math and science education matters
- Ronny Scherer 1 , 2 &
- Jens F Beckmann 3
Large-scale Assessments in Education volume 2 , Article number: 10 ( 2014 ) Cite this article
14k Accesses
16 Citations
1 Altmetric
Metrics details
On the basis of a ‘problem solving as an educational outcome’ point of view, we analyse the contribution of math and science competence to analytical problem-solving competence and link the acquisition of problem solving competence to the coherence between math and science education. We propose the concept of math-science coherence and explore whether society-, curriculum-, and school-related factors confound with its relation to problem solving.
By using the PISA 2003 data set of 41 countries, we apply multilevel regression and confounder analyses to investigate these effects for each country.
Our results show that (1) math and science competence significantly contribute to problem solving across countries; (2) math-science coherence is significantly related to problem solving competence; (3) country-specific characteristics confound this relation; (4) math-science coherence is linked to capability under-utilisation based on science performance but less on math performance.
Conclusions
In sum, low problem solving scores seem a result of an impeded transfer of subjectspecific knowledge and skills (i.e., under-utilisation of science capabilities in the acquisition of problem solving competence), which is characterised by low levels of math-science coherence.
The ability to solve real-world problems and to transfer problem-solving strategies from domain-specific to domain-general contexts and vice versa has been regarded an important competence students should develop during their education in school (Greiff et al. [ 2013 ]; van Merriënboer [ 2013 ]). In the context of large-scale assessments such as the PISA study problem solving competence is defined as the ability to solve cross-disciplinary and real-world problems by applying cognitive skills such as reasoning and logical thinking (Jonassen [ 2011 ]; OECD [ 2004 ]). Since this competence is regarded a desirable educational outcome, especially math and science educators have focused on developing students’ problem solving and reasoning competence in their respective domain-specific contexts (e.g., Kind [ 2013 ]; Kuo et al. [ 2013 ]; Wu and Adams [ 2006 ]). Accordingly, different conceptual frameworks were proposed that describe the cognitive processes of problem solving such as understanding the problem, building adequate representations of the problem, developing hypotheses, conducting experiments, and evaluating the solution (Jonassen [ 2011 ]; OECD [ 2005 ]). In comparing these approaches in math and science, it seems apparent that there is a conceptual overlap between the problem solving models in these two domains. This overlap triggers the question regarding its contribution to the development of students’ cross-curricular problem-solving competence (Abd-El-Khalick et al. [ 2004 ]; Bassok and Holyoak [ 1993 ]; Hiebert et al. [ 1996 ]).
The operationalization and scaling of performance in PISA assessments enables direct contrasting of scores in students’ competences in math and problem solving. Leutner et al. ([ 2012 ]) suggest that discrepancies between math and problem solving scores are indicative of the relative effectiveness of math education (OECD [ 2004 ]). In line with a “Capability-Utilisation Hypothesis”, it is assumed that math scores that negatively deviate from their problem solving counterpart signify an under-utilisation of students’ problem-solving capabilities as indicated by their scores in generic problem solving.
We intend to extend this view in two ways: First, by introducing the concept of math-science coherence we draw the focus on the potential synergistic link between math and science education and its contribution to the acquisition of problem solving competence. Second, the introduction of a Capability Under-Utilisation Index will enable us to extend the focus of the Capability-Utilisation Hypothesis to both, math and science education. The combination of the concept of math-science coherence with the notion of capability-utilisation will help to further explore the facilitating processes involved in the transition of subject-specific knowledge and skills to the acquisition of problem solving competence. These insights are expected to contribute to a better understanding of meaningful strategies to improve and optimize educational systems in different countries.
Theoretical framework
Problem solving as an educational goal.
In the PISA 2003 framework, problem solving is referred to as “an individual’s capacity to use cognitive processes to resolve real, cross-disciplinary situations where the solution path is not immediately obvious” (OECD [ 2004 ], p. 156). This definition is based on the assumption of domain-general skills and strategies that can be employed in various situations and contexts. These skills and strategies involve cognitive processes such as: Understanding and characterizing the problem, representing the problem, solving the problem, reflecting and communicating the problem solution (OECD [ 2003 ]). Problem solving is often regarded a process rather than an educational outcome, particularly in research on the assessment and instruction of problem solving (e.g., Greiff et al. [ 2013 ]; Jonassen [ 2011 ]). This understanding of the construct is based on the assumption that problem solvers need to perform an adaptive sequence of cognitive steps in order to solve a specific problem (Jonassen [ 2011 ]). Although problem solving has also been regarded as a process skill in large-scale assessments such as the PISA 2003 study, these assessments mainly focus on problem solving performance as an outcome that can be used for international comparisons (OECD [ 2004 ]). However, problem solving competence was operationalized as a construct comprised of cognitive processes. In the context of the PISA 2003 study, these processes were referred to as analytical problem solving, which was assessed by static tasks presented in paper-and-pencil format. Analytical problem-solving competence is related to school achievement and the development of higher-order thinking skills (e.g., Baumert et al. [ 2009 ]; OECD [ 2004 ]; Zohar [ 2013 ]). Accordingly, teachers and educators have focused on models of problem solving as guidelines for structuring inquiry-based processes in their subject lessons (Oser and Baeriswyl [ 2001 ]). Van Merriënboer ([ 2013 ]) pointed out that problem solving should not only be regarded a mere instructional method but also as a major educational goal. Recent curricular reforms have therefore shifted towards the development of problem solving abilities in school (Gallagher et al. [ 2012 ]; Koeppen et al. [ 2008 ]). These reforms were coupled with attempts to strengthen the development of transferable skills that can be applied in real-life contexts (Pellegrino and Hilton [ 2012 ]). For instance, in the context of 21 st century skills, researchers and policy makers have agreed on putting emphasis on fostering skills such as critical thinking, digital competence, and problem solving (e.g., Griffin et al. [ 2012 ]). In light of the growing importance of lifelong learning and the increased complexity of work- and real-life problem situations, these skills are now regarded as essential (Griffin et al. [ 2012 ]; OECD [ 2004 ]). Hence, large-scale educational studies such as PISA have shifted towards the assessment and evaluation of problem solving competence as a 21 st century skill.
The PISA frameworks of math and science competence
In large-scale assessments such as the PISA studies, students’ achievement in the domains of science and mathematics play an important role. Moreover, scientific and mathematical literacy are now regarded essential to being a reflective citizen (Bybee [ 2004 ]; OECD [ 2003 ]). Generally, Baumert et al. ([ 2009 ]) have shown that students’ math and science achievements are highly related to domain-general ability constructs such as reasoning or intelligence. In this context, student achievement refers to “the result of domain-specific processes of knowledge acquisition and information processing” (cf. Baumert et al. [ 2009 ], p. 169). This line of argument is reflected in definitions and frameworks of scientific and mathematical literacy, which are conceptualized as domain-specific competences that are hierarchically organized and build upon abilities closely related to problem solving (Brunner et al. [ 2013 ]).
Scientific literacy has been defined within a multidimensional framework, differentiating between three main cognitive processes, namely describing, explaining, and predicting scientific phenomena, understanding scientific investigations, and interpreting scientific evidence and conclusions (OECD [ 2003 ]). In addition, various types of knowledge such as ‘knowledge about the nature of science’ are considered as factors influencing students’ achievements in this domain (Kind [ 2013 ]). We conclude that the concept of scientific literacy encompasses domain-general problem-solving processes, elements of scientific inquiry (Abd-El-Khalick et al. [ 2004 ]; Nentwig et al. [ 2009 ]), and domain-specific knowledge.
The definition of mathematical literacy refers to students’ competence to utilise mathematical modelling and mathematics in problem-solving situations (OECD [ 2003 ]). Here, we can also identify overlaps between cognitive processes involved in mathematical problem solving and problem solving in general: Structuring, mathematizing, processing, interpreting, and validating (Baumert et al. [ 2009 ]; Hiebert et al. [ 1996 ]; Kuo et al. [ 2013 ]; Polya [ 1945 ]). In short, mathematical literacy goes beyond computational skills (Hickendorff [ 2013 ]; Wu and Adams [ 2006 ]) and is conceptually linked to problem solving.
In the PISA 2003 framework, the three constructs of math, science, and problem solving competence overlap conceptually. For instance, solving the math items requires reasoning, which comprises analytical skills and information processing. Given the different dimensions of the scientific literacy framework, the abilities involved in solving the science items are also related to problem solving, since they refer to the application of knowledge and the performance of inquiry processes (OECD [ 2003 ]). This conceptual overlap is empirically supported by high correlations between math and problem solving ( r = .89) and between science and problem solving ( r = .80) obtained for the sample of 41 countries involved in PISA 2003 (OECD [ 2004 ]). The relation between math and science competence was also high ( r = .83). On the one hand, the sizes of the inter-relationships, give rise to the question regarding the uniqueness of each of the competence measures. On the other hand, the high correlations indicate that problem-solving skills are relevant in math and science (Martin et al. [ 2012 ]). Although Baumert et al. ([ 2009 ]) suggest that the domain-specific competences in math and science require skills beyond problem solving (e.g., the application of domain-specific knowledge) we argue from an assessment perspective that the PISA 2003 tests in math, science, and problem solving measure predominantly basic academic skills relatively independent from academic knowledge (see also Bulle [ 2011 ]).
The concept of capability-utilisation
Discrepancies between students’ performance in math/science and problem solving were studied at country level (OECD [ 2004 ]) and were, for example for math and problem solving scores, interpreted in two ways: (1) If students’ perform better in math than in problem solving, they would “have a better grasp of mathematics content […] after accounting for the level of generic problem-solving skills…” (OECD [ 2004 ], p. 55); (2) If students’ estimated problem-solving competence is higher than their estimated math competence, “… this may suggest that students have the potential to achieve better results in mathematics than that reflected in their current performance…” (OECD [ 2004 ], p. 55). Whilst the latter discrepancy constitutes a capability under-utilisation in math, the former suggests challenges in utilising knowledge and skills acquired in domain-specific contexts in domain-unspecific contexts (i.e., transfer problem).
To quantify the degree to which students are able to transfer their problem solving capabilities from domain-specific problems in math or science to cross-curricular problems, we introduce the Capability Under-Utilisation Index (CUUI) as the relative difference between math or science and problem solving scores:
A positive CUUI indicates that the subject-specific education (i.e., math or science) in a country tends to under-utilise its students’ capabilities to problem solve. A negative CUUI indicates that a country’s educational system fails to fully utilise its students’ capabilities to acquire math and science literacy in the development of problem solving. The CUUI reflects the relative discrepancies between the achievement scores in different domains a .
The concept of math-science coherence
In light of the conceptual and empirical discussion on the relationship between math, science, and problem solving competence, we introduce the concept of math-science coherence as follows: First, math-science coherence refers to the set of cognitive processes involved in both subjects and thus represents processes which are related to reasoning and information processing, relatively independent from domain-specific knowledge. Second, math-science coherence reflects the degree to which math and science education is harmonized as a feature of the educational environment in a country. This interpretation is based on the premise that PISA measures students’ competence as educational outcomes (OECD [ 2004 ]). The operationalization of math-science coherence is realized by means of the correlation between math and science scores [ r (M,S)] at the country level. Low math-science coherence indicates that students who are successful in the acquisition of knowledge and skills in math are not necessarily successful in the acquisition of knowledge and skills in science and vice versa.
On the basis of this conceptualization of math-science coherence, we expect a significant and positive relation to problem solving scores, since the conceptual overlap between mathematical and scientific literacy refers to cognitive abilities such as reasoning and information processing that are also required in problem solving (Arts et al. [ 2006 ]; Beckmann [ 2001 ]; Wüstenberg et al. [ 2012 ]). Hence, we assert that math-science coherence facilitates the transfer of knowledge, skills, and insights across subjects resulting in better problem solving performance (OECD [ 2004 ]; Pellegrino and Hilton [ 2012 ]).
We also assume that math-science coherence as well as capability utilisation is linked to characteristics of the educational system of a country. For instance, as Janssen and Geiser ([ 2012 ]) and Blömeke et al. ([ 2011 ]) suggested, the developmental status of a country, measured by the Human Development Index (HDI; UNDP [ 2005 ]), is positively related to students’ academic achievements as well as to teachers’ quality of teaching. Furthermore, the socio-economic status of a country co-determines characteristics of its educational system, which ultimately affects a construct referred to as national intelligence (Lynn and Meisenberg [ 2010 ]). Research also indicated that curricular settings and educational objectives are related to school achievement in general (Bulle [ 2011 ]; Martin et al. [ 2004 ]). Besides these factors, school- and classroom-related characteristics might also confound the relation between math-science coherence and problem solving. For instance, the schools’ autonomy in developing curricula and managing educational resources might facilitate the incorporation of inquiry- and problem-based activities in science lessons (Chiu and Chow [ 2011 ]). These factors have been discussed as being influential to students’ competence development (OECD [ 2004 ], [ 2005 ]). Ewell ([ 2012 ]) implies that cross-national differences in problem solving competence might be related to differences in education and in using appropriate teaching material. These factors potentially confound the relation between math-science coherence and problem solving.
Discrepancies between math and problem solving scores are discussed in relation to quality of education. Although research has found that crossing the borders between STEM subjects positively affects students’ STEM competences (e.g., National Research Council NRC [ 2011 ]), we argue that the PISA analyses have fallen short in explaining cross-country differences in the development of problem solving competence, since they ignored the link between math and science competences and the synergistic effect of learning universally applicable problem-solving skills in diverse subject areas. Hence, we use the concept of math-science coherence to provide a more detailed description of the discrepancies between problem solving and domain-specific competences. In this regard, we argue that the coherence concept indicates the synergistic potential and students’ problem-solving competence the success of transfer.
The present study
The current study is based on the premise that in contrast to math and science competence problem solving competence is not explicitly taught as a subject at school. Problem solving competence, however, is an expected outcome of education (van Merriënboer [ 2013 ]). With the first step in our analyses, we seek to establish whether math and science education are in fact main contributors to the acquisition of problem solving competence. On the basis of this regression hypothesis, we subsequently focus on the question whether there are significant and systematic differences between countries ( Moderation-Hypothesis ). In light of the conceptual overlap due to cognitive processes involved in dealing with math, science and problem solving tasks and the shared item format employed in the assessments, we expect math and science competence scores to substantially predict scores in problem solving competence. Furthermore, since math and science education are differently organized across the 41 countries participating in the PISA 2003 study, differences in the contribution are also expected.
On the basis of these premises, we introduce the concept of math-science coherence, operationalised as the correlation between math and science scores [ r (M,S)], and analyse its relationship to problem solving and the effects of confounders (i.e., country characteristics) as a step of validation. Since math, science, and problem solving competence show a conceptual overlap, we expect problem solving and math-science coherence to be positively related. Countries’ educational systems differ in numerous aspects, their educational structure, and their educational objectives. Countries also differ with regard to the frequency of assessments, the autonomy of schools in setting up curricula and resources, and the educational resources available. Consequently, we expect the relation between math-science coherence and problem solving competence to be confounded by society-, curriculum-, and school-related factors ( Confounding-Hypothesis ).
In a final step, we aim to better understand the mechanisms with which math and science education contributes to the acquisition of problem-solving competence by exploring how math-science coherence, capability utilisation, and problem solving competence are related. We thus provide new insights into factors related to the transfer between students’ domain-specific and cross-curricular knowledge and skills ( Capability-Utilisation Hypothesis ).
In PISA 2003, a total sample of N = 276,165 students (49.4% female) from 41 countries participated. The entire sample was randomly selected by applying a two-step sampling procedure: First, schools were chosen within a country. Second, students were chosen within these schools. This procedure consequently led to a clustered structure of the data set, as students were nested in 10,175 schools. On average, 27 students per school were chosen across schools within countries. Students’ mean age was 15.80 years ( SD = 0.29 years) ranging from 15.17 to 16.25 years.
In the PISA 2003 study, different assessments were used in order to measure students’ competence in math, science, and problem solving. These assessments were administered as paper-and-pencil tests within a multi-matrix design (OECD [ 2005 ]). In this section, the assessments and further constructs are described that served as predictors of the contribution of math and science competence to problem solving at the country level.
Student achievement in math, science, and problem solving
In order to assess students’ competence to solve cross-curricular problems (i.e., analytical problem solving requiring information retrieval and reasoning), students had to work on an analytical problem-solving test. This test comprised a total of 19 items (7 items referred to trouble-shooting, 7 items referred to decision-making, and 5 items referred to system analysis and design; see OECD [ 2004 ]). Items were coded according to the PISA coding scheme, resulting in dichotomous and polytomous scores (OECD [ 2005 ]). Based on these scores, models of item response theory were specified in order to obtain person and item parameters (Leutner et al. [ 2012 ]). The resulting plausible values could be regarded as valid indicators of students’ abilities in problem solving (Wu [ 2005 ]). The problem solving test showed sufficient reliabilities between .71 and .89 for the 41 countries.
To assess mathematical literacy as an indicator of math competence , an 85-items test was administered (for details, refer to OECD [ 2003 ]). Responses were dichotomously or polytomously scored. Again, plausible values were obtained as person ability estimates and reliabilities were good (range: 0.83 – 0.93). In the context of mathematical literacy, students were asked to solve real-world problems by applying appropriate mathematical models. They were prompted to “identify and understand the role mathematics plays in the world, to make well-founded judgements and to use […] mathematics […]” (OECD [ 2003 ], p. 24).
Scientific literacy as a proxy for science competence was assessed by using problems referring to different content areas of science in life, health, and technology. The reliability estimates for the 35 items in this test ranged between .68 and .88. Again, plausible values served as indicators of this competence.
Country-specific characteristics
In our analyses, we incorporated a range of country-specific characteristics that can be subdivided into three main categories. These are: society-related factors, curriculum-related factors, and school-related factors. Country-specific estimates of National Intelligence as derived by Lynn and Meisenberg ([ 2010 ]) as well as the Human Development Index (HDI) were subsumed under society-related factors . The HDI incorporates indicators of a country’s health, education, and living standards (UNDP [ 2005 ]). Both variables are conceptualised as factors that contribute to country-specific differences in academic performance.
Holliday and Holliday ([ 2003 ]) emphasised the role of curricular differences in the understanding of between-country variance in test scores. We incorporated two curriculum-related factors in our analyses. First, we used Bulle’s ([ 2011 ]) classification of curricula into ‘progressive’ and ‘academic’. Bulle ([ 2011 ]) proposed this framework and classified the PISA 2003 countries according to their educational model. In her framework, she distinguishes between ‘academic models’ which are primarily geared towards teaching academic subjects (e.g., Latin, Germanic, and East-Asian countries) and ‘progressive models’ which focus on teaching students’ general competence in diverse contexts (e.g., Anglo-Saxon and Northern countries). In this regard, academic skills refer to the abilities of solving academic-type problems, whereas so called progressive skills are needed in solving real-life problems (Bulle [ 2011 ]). It can be assumed that educational systems that focus on fostering real-life and domain-general competence might be more conducive to successfully tackling the kind of problem solving tasks used in PISA (Kind [ 2013 ]). This classification of educational systems should be seen as the two extreme poles of a continuum rather than as a strict dichotomy. In line with the reflections above, we would argue that academic and progressive skills are not exclusively distinct, since both skills utilise sets of cognitive processes that largely overlap (Klahr and Dunbar [ 1988 ]). The fact that curricular objectives in some countries are shifting (e.g., in Eastern Asia) makes a clear distinction between both models even more difficult. Nonetheless, we will use this form of country-specific categorization based on Bulle’s model in our analyses.
Second, we considered whether countries’ science curricula were ‘integrated’ or ‘not integrated’ (Martin et al. [ 2004 ]). In this context, integration refers to linking multiple science subjects (biology, chemistry, earth science, and physics) to a unifying theme or issue (cf. Drake and Reid [ 2010 ], p. 1).
In terms of school-related factors, we used the PISA 2003 scales of ‘Frequency of assessments in schools’, ‘Schools’ educational resources’, and ‘School autonomy towards resources and curricula’ from the school questionnaire. Based on frequency and rating scales, weighted maximum likelihood estimates (WLE) indicated the degree to which schools performed in these scales (OECD [ 2005 ]).
The country-specific characteristics are summarized in the Table 1 .
The PISA 2003 assessments utilised a randomized incomplete block design to select different test booklets which covered the different content areas of math, science, and problem solving (Brunner et al. [ 2013 ]; OECD [ 2005 ]). The test administration took 120 minutes, and was managed for each participating country separately. It was established that quality standards of the assessment procedure were high.
Statistical analyses
In PISA 2003, different methods of obtaining person estimates with precise standard errors were applied. The most accurate procedure produced five plausible values, which were drawn from a person ability distribution (OECD [ 2005 ]). To avoid missing values in these parameters and to obtain accurate estimates, further background variables were used within the algorithms (Wu [ 2005 ]). The resulting plausible values were subsequently used as indicators of students’ competence in math, science, and problem solving. By applying Rubin’s combination rules (Bouhilila and Sellaouti [ 2013 ]; Enders [ 2010 ]), analyses were replicated with each of the five plausible values and then combined. In this multiple imputation procedure, standard errors were decomposed to the variability across and within the five imputations (Enders [ 2010 ]; OECD [ 2005 ]; Wu [ 2005 ]).
Within the multilevel regression analyses for each country, we specified the student level as level 1 and the school level as level 2. Since PISA 2003 applied a random sampling procedure at the student and the school level, we decided to control for the clustering of data at these two levels (OECD [ 2005 ]). In addition to this two-level procedure, we regarded the 41 countries as multiple groups (fixed effects). This decision was based on our assumption that the countries selected in PISA 2003 did not necessarily represent a sample of a larger population (Martin et al. [ 2012 ]). Moreover, we did not regard the effects of countries as interchangeable, because, given the specific characteristics of education and instruction within countries; we argue that the effects of competences in mathematics and science on problem solving have their own distinct interpretation in each country (Snijders and Bosker [ 2012 ]). The resulting models were compared by taking into account the Akaike’s information criteria ( AIC ), Bayesian information criteria ( BIC ), and the sample-size adjusted BIC . Also, a likelihood ratio test of the log-Likelihood values ( LogL ) was applied (Hox [ 2010 ]).
To test the Moderation-Hypothesis, we first specified a two-level regression model with problem solving scores as outcomes at the student level (level 1), which allowed variance in achievement scores across schools (level 2). In this model, math and science scores predicted problem solving scores at the student level. To account for differences in the probabilities of being selected as a student within the 41 countries and to adjust the standard errors of regression parameters, we used the robust maximum likelihood (MLR) estimator and students’ final weights (see also Brunner et al. [ 2013 ]; OECD [ 2005 ]). All analyses were conducted in Mplus 6.0 by using the TYPE = IMPUTATION option (Muthén and Muthén [ 2010 ]). As Hox ([ 2010 ]) suggested, using multilevel regression models without taking into account the clustering of data in schools often leads to biased estimates, since achievement variables often have substantial variance at the school level. Consequently, we allowed for level-2-variance within the scores.
After having established whether success in math and science education contributes to the development in problem solving competence across the 41 countries, we then tested whether cross-country differences in the unstandardized regression coefficients were statistically significant by using a multi-group regression model, in which the coefficients were constrained to be equal across countries. We compared this model with the freely estimated model.
Finally, we conceptualized the correlation between math and science scores as an indicator of the level of coherence in math and science education in a country. In relation to the Confounding-Hypothesis, we tested country-specific characteristics for their potentially confounding effects on the relation between math-science coherence and problem solving competence. Following the recommendations proposed by (MacKinnon et al. [ 2000 ]), the confounding analysis was conducted in two steps: (1) we estimated two regression equations. In the first equation, problem solving scores across the 41 countries were regressed on math-science coherence. In the second equation, the respective country characteristics were added as further predictors; (2) the difference between the regression coefficients for math-science coherence obtained in either equation represented the magnitude of a potential confounder effect.
Lastly, we tested the Capability-Utilisation Hypothesis by investigating the bivariate correlations among the CUU Indices and math-science coherence.
Regressing problem solving on math and science performance
To test the Moderation-Hypothesis, we specified regression models with students’ problem-solving score as the outcome and math and science scores as predictors for each of the 41 countries. Due to the clustering of data in schools, these models allowed for between-level variance. Intraclass correlations (ICC-1) for math, science, and problem solving performance ranged between .03 and .61 for the school level ( M = .33, SD = .16).
We specified multilevel regression models for each country separately. These results are reported in Table 2 . The regression coefficients for math on problem solving ranged from .53 to .82 with an average of M( β Math ) = .67 ( SD = .06). The average contribution of science towards problem solving was M( β Science ) = .16 ( SD = .09, Min = -.06, Max = .30). The combination of the distributions of both parameters resulted in substantial differences in the variance explanations of the problem solving scores across the 41 countries ( M[R 2 ] = .65, SD = .15, Min = .27, Max = .86). To test whether these differences were statistically significant, we constrained the regression coefficients of math and science competence within the multi-group regression model to be equal across the 41 countries. Compared to the freely estimated model ( LogL = -4,561,273.3, df = 492, AIC = 9,123,530.5, BIC = 9,128,410.7), the restricted model was empirically not preferred LogL = -4,564,877.9, df = 412, AIC = 9,130,579.8, BIC = 9,134,917.6; Δχ 2 [80] = 7,209.2, p < .001. These findings lend evidence for the Moderation-Hypothesis.
From a slightly different perspective, the country-specific amount of variance in problem solving scores that is explained by the variation in math and science performance scores ( R 2 ) is strongly associated with the country’s problem solving score ( r = .77, p < .001), which suggests that the contribution of science and math competence to the acquisition of problem solving competence was significantly lower in low-performing countries.
As shown in Table 2 , the regression weights of math and science were significant for all but two countries. Across countries the regression weight for math tended to be higher than the regression weight for science when predicting problem solving competence. This finding indicates a stronger overlap between students’ competences in mathematics and problem solving on the one hand and similarities between the assessments in both domains on the other hand.
Validating the concept of math-science coherence
In order to validate the concept of math-science coherence, which is operationalised as the correlation between math and science scores [ r (M,S)], we explored its relation to problem solving and country characteristics.
Regarding the regression outcomes shown in Table 2 , it is apparent that math-science coherence varied considerably across countries, ranging from .39 to .88 with an average of M(r) = .70 ( SD = .13). Interestingly, countries’ level of coherence in math-science education was substantially related to their problem solving scores ( r = .76, p < .001). An inspection of Figure 1 reveals that this effect was mainly due to countries that both achieve low problem solving scores and show relatively low levels of math-science coherence (see bottom left quadrant in Figure 1 ), whilst amongst the remaining countries the correlational link between math-science coherence and problem solving score was almost zero ( r = -.08, p = .71) b . This pattern extends the moderation perspective on the presumed dependency of problem solving competence from math and science competences.
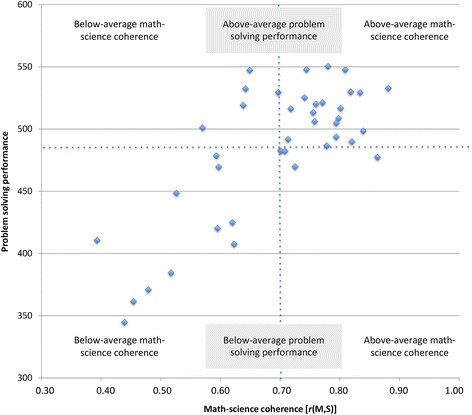
The relation between math-science coherence and problem solving performance across the 41 countries.
As a result of the moderator analysis, we know that countries not only differ in regard to their average problem-solving scores and level of coherence between math and science, countries also differ in the strengths with which math-science coherence predicts problem solving scores. To better understand the conceptual nature of the link between math-science coherence and problem solving, we now attempt to adjust this relationship for potential confounding effects that country-specific characteristics might have. To this end, we employed linear regression and path analysis with students’ problem-solving scores as outcomes, math-science coherence (i.e., r [M,S]) as predictor, and country characteristics as potential confounders.
To establish whether any of the country characteristics had a confounding effect on the link between math-science coherence and problem solving competence, two criteria had to be met: (1) a reduction of the direct effect of math-science coherence on problem solving scores, and (2) testing the difference between the direct effect within the baseline Model M0 and the effect with the confounding Model M1 (Table 3 ).
Regarding the society-related factors, both the countries’ HDI and their national intelligence were confounders with a positive effect. Furthermore, the countries’ integration of the science curriculum was also positively related to the problem solving performance. Finally, the degree of schools’ autonomy towards educational resources and the implementation of curricula and the frequency of assessments were school-related confounders, the former with a positive effect whilst the latter represents a negative confounder. The direct effect of math-science coherence to problem solving decreased and thus indicated that confounding was present (MacKinnon et al. [ 2000 ]).
These findings provide evidence on the Confounding-Hypothesis and support our expectations on the relation between math-science coherence, problem solving, and country characteristics. We regard these results as evidence for the validity of the math-science coherence measure.
Relating math-science coherence to the capability under-utilisation indices
To advance our understanding of the link between math-science coherence and problem solving scores, we tested the Capability-Utilisation Hypothesis. To this end, we explored the relationship between math-science coherence and the CUU Indices for math and science, respectively. For math competence the average Capability Under-Utilisation Index was rather neutral with M CUUI-Math = -0.001 ( SD = 0.02). This suggests that, on average, all countries sufficiently utilise their students’ math capabilities in facilitating the development of problem solving competence (i.e., transfer). It also suggests that math education across participating countries tends to sufficiently utilise generic problem-solving skills (Figure 2 ). The picture is different for science education. Here, the Capability Under-Utilisation Indices and their variation across the participating countries ( M CUUI-Science = -0.01, SD = 0.04) suggest that in a range of countries knowledge and skills taught in science education tend to be under-utilised in the facilitation of the acquisition of problem solving competence (Figure 3 ).
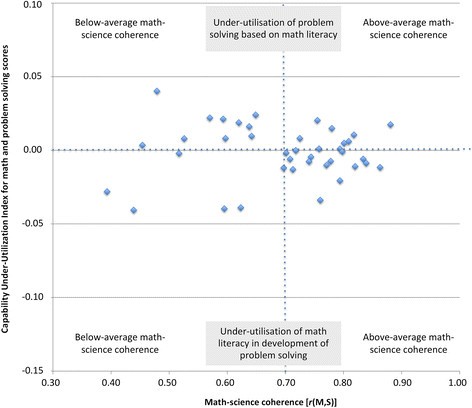
The relation between math-science coherence and the capability under-utilisation index for math and problem solving scores across the 41 countries.
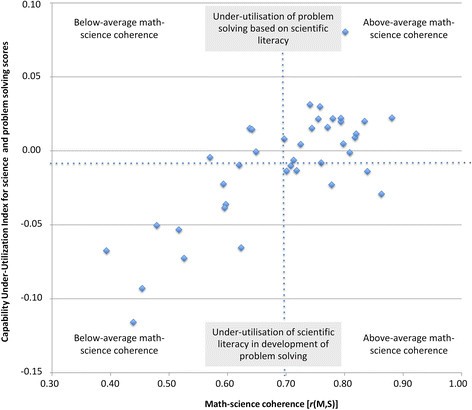
The relation between math-science coherence and the capability under-utilisation index for science and problem solving scores across the 41 countries.
For math competence, the relative difference to problem solving was not related to math-science coherence ( r = .02, p = .89; Figure 2 ). In contrast, the Capability Under-Utilisation Index for science showed a strong positive correlation with math-science coherence ( r = .76, p < .001; Figure 3 ), indicating that low levels of coherence between math and science education were associated with a less effective transfer of domain-specific knowledge and skills to problem solving.
The present study was aimed at investigating the differences in the contribution of math and science competence to problem solving competence across the 41 countries that participated in the PISA 2003 study (Moderation-Hypothesis). To this end, we proposed the concept of math-science coherence and explored its relationship to problem solving competence and how this relationship is confounded by country characteristics (Confounding-Hypothesis). To further extend our understanding of the link between math-science coherence and problem solving, we introduced the concept of capability-utilisation. Testing the Capability-Utilisation Hypothesis enabled us to identify what may contribute to varying levels of math-science coherence and ultimately the development of problem solving competence.
The contribution of math and science competence across countries
Regarding the prediction of problem solving competence, we found that in most countries, math and science competence significantly contributed to students’ performance in analytical problem solving. This finding was expected based on the conceptualizations of mathematical and scientific literacy within the PISA framework referring to shared cognitive processes such as information processing and reasoning (Kind [ 2013 ]; OECD [ 2005 ]), which are regarded as components of problem solving (Bybee [ 2004 ]; Klahr and Dunbar [ 1988 ]; Mayer [ 2010 ]).
It is noteworthy that, for some of the below-average performing countries, science competence did not significantly contribute to the prediction of problem solving competence. It can be speculated that education in these countries is more geared towards math education and modelling processes in mathematical scenarios, whilst the aspect of problem solving in science is less emphasised (Janssen and Geiser [ 2012 ]). The results of multilevel regression analyses supported this interpretation by showing that math competence was a stronger predictor of problem solving competence. On the one hand, this finding could be due to the design of the PISA tests (Adams [ 2005 ]), since math and problem solving items are designed in such a way that modelling real-life problems is required, whereas science items are mostly domain-specific and linked to science knowledge (Nentwig et al. [ 2009 ]; OECD [ 2004 ]). Moreover, one may argue that math and problem solving items allow students to employ different solution strategies, whereas science items offer fewer degrees of freedom for test takers (Nentwig et al. [ 2009 ]). In particular, the shared format of items in math, science, and problem solving may explain an overlap between their cognitive demands. For instance, most of the items were designed in such a way that students had to extract and identify relevant information from given tables or figures in order to solve specific problems. Hence, these items were static and did not require knowledge generation by interaction or exploration but rather the use of given information in problem situations (Wood et al. [ 2009 ]). In contrast to the domain-specific items in math and science, problem solving items did not require the use of prior knowledge in math and science (OECD [ 2004 ]). In addition, some of the math and science items involved cognitive operations that were specific to these domains. For instance, students had to solve a number of math items by applying arithmetic and combinatorial operations (OECD [ 2005 ]). Finally, since items referred to contextual stimuli, which were presented in textual formats, reading ability can be regarded as another, shared demand of solving the items. Furthermore, Rindermann ([ 2007 ]) clearly showed that the shared demands of the achievement tests in large-scale assessments such as PISA were strongly related to students’ general reasoning skills. This finding is in line with the strong relations between math, science, and problem solving competence, found in our study. The interpretation of the overlap between the three competences can also be interpreted from a conceptual point of view. In light of the competence frameworks in PISA, we argue that there are a number of skills that can be found in math, science, and problem solving: information retrieval and processing, knowledge application, and evaluation of results (Griffin et al. [ 2012 ]; OECD [ 2004 ], [ 2005 ]). These skills point out to the importance of reasoning in the three domains (Rindermann [ 2007 ]). Thus, the empirical overlap between math and problem solving can be explained by shared processes of, what Mayer ([ 2010 ]) refers to as, informal reasoning. On the other hand, the stronger effect of math competence could be an effect of the quality of math education. Hiebert et al. ([ 1996 ]) and Kuo et al. ([ 2013 ]) suggested that math education is more based on problem solving skills than other subjects in school (e.g., Polya [ 1945 ]). Science lessons, in contrast, are often not necessarily problem-based, despite the fact that they often start with a set problem. Risch ([ 2010 ]) showed in a cross-national review that science learning was more related to contents and contexts rather than to generic problem-solving skills. These tendencies might lead to a weaker contribution of science education to the development of problem solving competence (Abd-El-Khalick et al. [ 2004 ]).
In sum, we found support on the Moderation-Hypothesis, which assumed systematic differences in the contribution of math and science competence to problem solving competence across the 41 PISA 2003 countries.
The relation to problem solving
In our study, we introduced the concept of math-science coherence, which reflects the degree to which math and science education are harmonized. Since mathematical and scientific literacy show a conceptual overlap, which refers to a set of cognitive processes that are linked to reasoning and information processing (Fensham and Bellocchi [ 2013 ]; Mayer [ 2010 ]), a significant relation between math-science coherence and problem solving was expected. In our analyses, we found a significant and positive effect of math-science coherence on performance scores in problem solving. In this finding we see evidence for the validity of this newly introduced concept of math-science coherence and its focus on the synergistic effect of math and science education on problem solving. The results further suggest that higher levels of coordination between math and science education has beneficial effects on the development of cross-curricular problem-solving competence (as measured within the PISA framework).
Confounding effects of country characteristics
As another step of validating the concept of math-science coherence, we investigated whether country-specific characteristics that are linked to society-, curriculum-, and school-related factors confounded its relation to problem solving. Our results showed that national intelligence, the Human Development Index, the integration of the science curriculum, and schools’ autonomy were positively linked to math-science coherence and problem solving, whilst a schools’ frequency of assessment had a negative confounding effect.
The findings regarding the positive confounders are in line with and also extend a number of studies on cross-country differences in education (e.g., Blömeke et al. [ 2011 ]; Dronkers et al. [ 2014 ]; Janssen and Geiser [ 2012 ]; Risch [ 2010 ]). Ross and Hogaboam-Gray ([ 1998 ]), for instance, found that students benefit from an integrated curriculum, particularly in terms of motivation and the development of their abilities. In the context of our confounder analysis, the integration of the science curriculum as well as the autonomy to allocate resources is expected to positively affect math-science coherence. At the same time, an integrated science curriculum with a coordinated allocation of resources may promote inquiry-based experiments in science courses, which is assumed to be beneficial for the development of problem solving within and across domains. Teaching science as an integrated subject is often regarded a challenge for teachers, particularly when developing conceptual structures in science lessons (Lang and Olson, [ 2000 ]), leading to teaching practices in which cross-curricular competence is rarely taken into account (Mansour [ 2013 ]; van Merriënboer [ 2013 ]).
The negative confounding effect of assessment frequency suggests that high frequencies of assessment, as it presumably applies to both math and science subjects, contribute positively to math-science coherence. However, the intended or unintended engagement in educational activities associated with assessment preparation tends not to be conducive to effectively developing domain-general problem solving competence (see also Neumann et al. [ 2012 ]).
The positive confounder effect of HDI is not surprising as HDI reflects a country’s capability to distribute resources and to enable certain levels of autonomy (Reich et al. [ 2013 ]). To find national intelligence as a positive confounder is also to be expected as the basis for its estimation are often students’ educational outcome measures (e.g., Rindermann [ 2008 ]) and, as discussed earlier, academic achievement measures share the involvement of a set of cognitive processes (Baumert et al. [ 2009 ]; OECD [ 2004 ]).
In summary, the synergistic effect of a coherent math and science education on the development of problem solving competence is substantially linked to characteristics of a country’s educational system with respect to curricula and school organization in the context of its socio-economic capabilities. Math-science coherence, however, also is linked to the extent to which math or science education is able to utilise students’ educational capabilities.
Math-science coherence and capability-utilisation
So far, discrepancies between students’ performance in math and problem solving or science and problem solving have been discussed as indicators of students’ capability utilisation in math or science (Leutner et al. [ 2012 ]; OECD [ 2004 ]). We have extended this perspective by introducing Capability Under-Utilisation Indices for math and science to investigate the effectiveness with which knowledge and skills acquired in the context of math or science education are transferred into cross-curricular problem-solving competence. The Capability Under-Utilisation Indices for math and science reflect a potential quantitative imbalance between math, science, and problem solving performance within a country, whilst the also introduced concept of math-science coherence reflects a potential qualitative imbalance between math and science education.
The results of our analyses suggest that an under-utilisation of problem solving capabilities in the acquisition of science literacy is linked to lower levels of math-science coherence, which ultimately leads to lower scores in problem solving competence. This interpretation finds resonance in Ross and Hogaboam-Gray’s ([ 1998 ]) argumentation for integrating math and science education and supports the attempts of math and science educators to incorporate higher-order thinking skills in teaching STEM subjects (e.g., Gallagher et al. [ 2012 ]; Zohar [ 2013 ]).
In contrast, the CUU Index for math was not related to math-science coherence in our analyses. This might be due to the conceptualizations and assessments of mathematical literacy and problem solving competence. Both constructs share cognitive processes of reasoning and information processing, resulting in quite similar items. Consequently, the transfer from math-related knowledge and skills to cross-curricular problems does not necessarily depend on how math and science education are harmonised, since the conceptual and operational discrepancy between math and problem solving is rather small.
Math and science education do matter to the development of students’ problem-solving skills. This argumentation is based on the assumption that the PISA assessments in math, science, and problem solving are able to measure students’ competence as outcomes, which are directly linked to their education (Bulle [ 2011 ]; Kind [ 2013 ]). In contrast to math and science competence, problem solving competence is not explicitly taught as a subject. Problem solving competence requires the utilisation of knowledge and reasoning skills acquired in specific domains (Pellegrino and Hilton [ 2012 ]). In agreement with Kuhn ([ 2009 ]), we point out that this transfer does not happen automatically but needs to be actively facilitated. In fact, Mayer and Wittrock ([ 2006 ]) stressed that the development of transferable skills such as problem solving competence needs to be fostered within specific domains rather than taught in dedicated, distinct courses. Moreover, they suggested that students should develop a “repertoire of cognitive and metacognitive strategies that can be applied in specific problem-solving situations” (p. 299). Beyond this domain-specific teaching principle, research also proposes to train the transfer of problem solving competence in domains that are closely related (e.g., math and science; Pellegrino and Hilton [ 2012 ]). In light of the effects of aligned curricula (as represented by the concept of math-science coherence), we argue that educational efforts to increase students’ problem solving competence may focus on a coordinated improvement of math and science literacy and fostering problem solving competence within math and science. The emphasis is on coordinated, as the results of our analyses indicated that the coherence between math and science education, as a qualitative characteristic of a country’s educational system, is a strong predictor of problem solving competence. This harmonisation of math and science education may be achieved by better enabling the utilisation of capabilities, especially in science education. Sufficiently high levels of math-science coherence could facilitate the emergence of educational synergisms, which positively affect the development of problem solving competence. In other words, we argue for quantitative changes (i.e., improve science attainment) in order to achieve qualitative changes (i.e., higher levels of curriculum coherence), which are expected to create effective transitions of subject-specific knowledge and skills into subject-unspecific competences to solve real-life problems (Pellegrino and Hilton [ 2012 ]; van Merriënboer [ 2013 ]).
Finally, we encourage research that is concerned with the validation of the proposed indices for different forms of problem solving. In particular, we suggest studying the facilities of the capability-under-utilisation indices for analytical and dynamic problem solving, as assessed in the PISA 2012 study (OECD [ 2014 ]). Due to the different cognitive demands in analytical and dynamic problems (e.g., using existing knowledge vs. generating knowledge; OECD [ 2014 ]), we suspect differences in capability utilisation in math and science. This research could provide further insights into the role of 21 st century skills as educational goals.
a The differences between students’ achievement in mathematics and problem solving, and science and problem solving have to be interpreted relative to the OECD average, since the achievement scales were scaled with a mean of 500 and a standard deviation of 100 for the OECD countries (OECD [ 2004 ], p. 55). Although alternative indices such as country residuals may also be used in cross-country comparisons (e.g., Olsen [ 2005 ]), we decided to use CUU indices, as they reflect the actual differences in achievement scores.
b In addition, we checked whether this result was due to the restricted variances in low-performing countries and found that neither ceiling nor floor effects in the problem solving scores existed. The problem solving scale differentiated sufficiently reliably in the regions below and above the OECD mean of 500.
Abd-El-Khalick F, Boujaoude S, Duschl R, Lederman NG, Mamlok-Naaman R, Hofstein A, Niaz M, Treagust D, Tuan H-L: Inquiry in science education: International perspectives. Science Education 2004, 88: 397–419. doi:10.1002/sce.10118 10.1002/sce.10118
Article Google Scholar
Adams RJ: Reliability as a measurement design effect. Studies in Educational Evaluation 2005, 31: 162–172. doi:10.1016/j.stueduc.2005.05.008 10.1016/j.stueduc.2005.05.008
Arts J, Gijselaers W, Boshuizen H: Understanding managerial problem solving, knowledge use and information processing: Investigating stages from school to the workplace. Contemporary Educational Psychology 2006, 31: 387–410. doi:10.1016/j.cedpsych.2006.05.005 10.1016/j.cedpsych.2006.05.005
Bassok M, Holyoak K: Pragmatic knowledge and conceptual structure: determinants of transfer between quantitative domains. In Transfer on trial: intelligence, cognition, and instruction . Edited by: Detterman DK, Sternberg RJ. Ablex, Norwood, NJ; 1993:68–98.
Google Scholar
Baumert J, Lüdtke O, Trautwein U, Brunner M: Large-scale student assessment studies measure the results of processes of knowledge acquisition: evidence in support of the distinction between intelligence and student achievement. Educational Research Review 2009, 4: 165–176. doi:10.1016/j.edurev.2009.04.002 10.1016/j.edurev.2009.04.002
Beckmann JF: Zur Validierung des Konstrukts des intellektuellen Veränderungspotentials [On the validation of the construct of intellectual change potential] . logos, Berlin; 2001.
Blömeke S, Houang R, Suhl U: TEDS-M: diagnosing teacher knowledge by applying multidimensional item response theory and multiple-group models. IERI Monograph Series: Issues and Methodologies in Large-Scale Assessments 2011, 4: 109–129.
Bouhilila D, Sellaouti F: Multiple imputation using chained equations for missing data in TIMSS: a case study. Large-scale Assessments in Education 2013, 1: 4.
Brunner M, Gogol K, Sonnleitner P, Keller U, Krauss S, Preckel F: Gender differences in the mean level, variability, and profile shape of student achievement: Results from 41 countries. Intelligence 2013, 41: 378–395. doi:10.1016/j.intell.2013.05.009 10.1016/j.intell.2013.05.009
Bulle N: Comparing OECD educational models through the prism of PISA. Comparative Education 2011, 47: 503–521. doi: 10.1080/03050068.2011.555117 10.1080/03050068.2011.555117
Bybee R: Scientific Inquiry and Science Teaching. In Scientific Inquiry and the Nature of Science . Edited by: Flick L, Lederman N. Springer & Kluwers, New York, NY; 2004:1–14. doi:10.1007/978–1-4020–5814–1_1
Chiu M, Chow B: Classroom discipline across forty-One countries: school, economic, and cultural differences. Journal of Cross-Cultural Psychology 2011, 42: 516–533. doi:10.1177/0022022110381115 10.1177/0022022110381115
Drake S, Reid J: Integrated curriculum: Increasing relevance while maintaining accountability. Research into Practice 2010, 28: 1–4.
Dronkers J, Levels M, de Heus M: Migrant pupils’ scientific performance: the influence of educational system features of origin and destination countries. Large-scale Assessments in Education 2014, 2: 3.
Enders C: Applied Missing Data Analysis . The Guilford Press, New York, NY; 2010.
Ewell P: A world of assessment: OECD’s AHELO initiative. Change: The Magazine of Higher Learning 2012, 44: 35–42. doi:10.1080/00091383.2012.706515 10.1080/00091383.2012.706515
Fensham P, Bellocchi A: Higher order thinking in chemistry curriculum and its assessment. Thinking Skills and Creativity 2013, 10: 250–264. doi:10.1016/j.tsc.2013.06.003 10.1016/j.tsc.2013.06.003
Gallagher C, Hipkins R, Zohar A: Positioning thinking within national curriculum and assessment systems: perspectives from Israel, New Zealand and Northern Ireland. Thinking Skills and Creativity 2012, 7: 134–143. doi:10.1016/j.tsc.2012.04.005 10.1016/j.tsc.2012.04.005
Greiff S, Holt D, Funke J: Perspectives on problem solving in educational assessment: analytical, interactive, and collaborative problem solving. The Journal of Problem Solving 2013, 5: 71–91. doi:10.7771/1932–6246.1153 10.7771/1932-6246.1153
Griffin P, Care E, McGaw B: The changing role of education and schools. In Assessment and Teaching of 21st Century Skills . Edited by: Griffin P, McGaw B, Care E. Springer, Dordrecht; 2012:1–15. 10.1007/978-94-007-2324-5_1
Chapter Google Scholar
Hickendorff M: The language factor in elementary mathematics assessments: Computational skills and applied problem solving in a multidimensional IRT framework. Applied Measurement in Education 2013, 26: 253–278. doi:10.1080/08957347.2013.824451 10.1080/08957347.2013.824451
Hiebert J, Carpenter T, Fennema E, Fuson K, Human P, Murray H, Olivier A, Wearne D: Problem Solving as a Basis for Reform in Curriculum and Instruction: The Case of Mathematics. Educational Researcher 1996, 25: 12–21. doi:10.3102/0013189X025004012 10.3102/0013189X025004012
Holliday W, Holliday B: Why using international comparative math and science achievement data from TIMSS is not helpful. The Educational Forum 2003, 67: 250–257. 10.1080/00131720309335038
Hox J: Multilevel Analysis . 2nd edition. Routlegde, New York, NY; 2010.
Janssen A, Geiser C: Cross-cultural differences in spatial abilities and solution strategies: An investigation in Cambodia and Germany. Journal of Cross-Cultural Psychology 2012, 43: 533–557. doi:10.1177/0022022111399646 10.1177/0022022111399646
Jonassen D: Learning to solve problems . Routledge, New York, NY; 2011.
Kind P: Establishing Assessment Scales Using a Novel Disciplinary Rationale for Scientific Reasoning. Journal of Research in Science Teaching 2013, 50: 530–560. doi:10.1002/tea.21086 10.1002/tea.21086
Klahr D, Dunbar K: Dual Space Search during Scientific Reasoning. Cognitive Science 1988, 12: 1–48. doi:10.1207/s15516709cog1201_1 10.1207/s15516709cog1201_1
Koeppen K, Hartig J, Klieme E, Leutner D: Current issues in competence modeling and assessment. Journal of Psychology 2008, 216: 61–73. doi:10.1027/0044–3409.216.2.61
Kuhn D: Do students need to be taught how to reason? Educational Research Review 2009, 4: 1–6. doi:10.1016/j.edurev.2008.11.001 10.1016/j.edurev.2008.11.001
Kuo E, Hull M, Gupta A, Elby A: How Students Blend Conceptual and Formal Mathematical Reasoning in Solving Physics Problems. Science Education 2013, 97: 32–57. doi:10.1002/sce.21043 10.1002/sce.21043
Lang M, Olson J: Integrated science teaching as a challenge for teachers to develop new conceptual structures. Research in Science Education 2000, 30: 213–224. doi:10.1007/BF02461629 10.1007/BF02461629
Leutner D, Fleischer J, Wirth J, Greiff S, Funke J: Analytische und dynamische Problemlösekompetenz im Lichte internationaler Schulleistungsstudien [Analytical and dynamic problem-solvng competence in international large-scale studies]. Psychologische Rundschau 2012, 63: 34–42. doi:10.1026/0033–3042/a000108 10.1026/0033-3042/a000108
Lynn R, Meisenberg G: National IQs calculated and validated for 108 nations. Intelligence 2010, 38: 353–360. doi:10.1016/j.intell.2010.04.007 10.1016/j.intell.2010.04.007
MacKinnon D, Krull J, Lockwood C: Equivalence of the mediation, confounding, and suppression effect. Prevention Science 2000, 1: 173–181. doi:10.1023/A:1026595011371 10.1023/A:1026595011371
Mansour N: Consistencies and inconsistencies between science teachers’ beliefs and practices. International Journal of Science Education 2013, 35: 1230–1275. doi:10.1080/09500693.2012.743196 10.1080/09500693.2012.743196
Martin M, Mullis I, Gonzalez E, Chrostowski S: TIMSS 2003 International Science Report . IEA, Chestnut Hill, MA; 2004.
Martin AJ, Liem GAD, Mok MMC, Xu J: Problem solving and immigrant student mathematics and science achievement: Multination findings from the Programme for International Student Assessment (PISA). Journal of Educational Psychology 2012, 104: 1054–1073. doi:10.1037/a0029152 10.1037/a0029152
Mayer R: Problem solving and reasoning. In International Encyclopedia of Education . 3rd edition. Edited by: Peterson P, Baker E, McGraw B. Elsevier, Oxford; 2010:273–278. doi:10.1016/B978–0-08–044894–7.00487–5 10.1016/B978-0-08-044894-7.00487-5
Mayer R, Wittrock MC: Problem solving. In Handbook of Educational Psychology . 2nd edition. Edited by: Alexander PA, Winne PH. Lawrence Erlbaum, New Jersey; 2006:287–303.
Muthén B, Muthén L: Mplus 6 . Muthén & Muthén, Los Angeles, CA; 2010.
Successful K-12 STEM Education . National Academies Press, Washington, DC; 2011.
Nentwig P, Rönnebeck S, Schöps K, Rumann S, Carstensen C: Performance and levels of contextualization in a selection of OECD countries in PISA 2006. Journal of Research in Science Teaching 2009, 8: 897–908. doi:10.1002/tea.20338 10.1002/tea.20338
Neumann K, Kauertz A, Fischer H: Quality of Instruction in Science Education. In Second International Handbook of Science Education (Part One . Edited by: Fraser B, Tobin K, McRobbie C. Springer, Dordrecht; 2012:247–258.
The PISA 2003 Assessment Frameworks . OECD, Paris; 2003.
Problem solving for tomorrow’s world . OECD, Paris; 2004.
PISA 2003 Technical Report . OECD, Paris; 2005.
PISA 2012 Results : Creative Problem Solving – Students’ Skills in Tackling Real-Life Problems (Vol. V) . OECD, Paris; 2014.
Olsen RV: An exploration of cluster structure in scientific literacy in PISA: Evidence for a Nordic dimension? NorDiNa. ᅟ 2005, 1 (1):81–94.
Oser F, Baeriswyl F: Choreographies of Teaching: Bridging Instruction to Learning. In Handbook of Research on Teaching . 4th edition. Edited by: Richardson V. American Educational Research Association, Washington, DC; 2001:1031–1065.
Pellegrino JW, Hilton ML: Education for Life and Work – Developing Transferable Knowledge and Skills in the 21st Century . The National Academies Press, Washington, DC; 2012.
Polya G: How to solve it: a new aspect of mathematical method . Princeton University Press, Princeton, NJ; 1945.
Reich J, Hein S, Krivulskaya S, Hart L, Gumkowski N, Grigorenko E: Associations between household responsibilities and academic competencies in the context of education accessibility in Zambia. Learning and Individual Differences 2013, 27: 250–257. doi:10.1016/j.lindif.2013.02.005 10.1016/j.lindif.2013.02.005
Rindermann H: The g-factor of international cognitive ability comparisons: The homogeneity of results in PISA, TIMSS, PIRLS and IQ-tests across nations. European Journal of Personality 2007, 21: 661–706. doi:10.1002/per.634
Rindermann H: Relevance of education and intelligence at the national level for the economic welfare of people. Intelligence 2008, 36: 127–142. doi:10.1016/j.intell.2007.02.002 10.1016/j.intell.2007.02.002
Risch B: Teaching Chemistry around the World . Waxmann, Münster; 2010.
Ross J, Hogaboam-Gray A: Integrating mathematics, science, and technology: effects on students. International Journal of Science Education 1998, 20: 1119–1135. doi:10.1080/0950069980200908 10.1080/0950069980200908
Snijders TAB, Bosker RJ: Multilevel Analysis: An Introduction to Basic and Advanced Multilevel Modeling . 2nd edition. Sage Publications, London; 2012.
Human Development Report 2005 . UNDP, New York, NY; 2005.
Van Merriënboer J: Perspectives on problem solving and instruction. Computers & Education 2013, 64: 153–160. doi:10.1016/j.compedu.2012.11.025 10.1016/j.compedu.2012.11.025
Wood RE, Beckmann JF, Birney D: Simulations, learning and real world capabilities. Education Training 2009, 51 (5/6):491–510. doi:10.1108/00400910910987273 10.1108/00400910910987273
Wu M: The role of plausible values in large-scale surveys. Studies in Educational Evaluation 2005, 31: 114–128. doi:10.1016/j.stueduc.2005.05.005 10.1016/j.stueduc.2005.05.005
Wu M, Adams R: Modelling Mathematics Problem Solving Item Responses using a Multidimensional IRT Model. Mathematics Education Research Journal 2006, 18: 93–113. doi:10.1007/BF03217438 10.1007/BF03217438
Wüstenberg S, Greiff S, Funke J: Complex problem solving: More than reasoning? Intelligence 2012, 40: 1–14. doi:10.1016/j.intell.2011.11.003 10.1016/j.intell.2011.11.003
Zohar A: Scaling up higher order thinking in science classrooms: the challenge of bridging the gap between theory, policy and practice. Thinking Skills and Creativity 2013, 10: 168–172. doi:10.1016/j.tsc.2013.08.001 10.1016/j.tsc.2013.08.001
Download references
Author information
Authors and affiliations.
Centre for Educational Measurement, University of Oslo (CEMO), Oslo, Norway
Ronny Scherer
Faculty of Educational Sciences, University of Oslo, Oslo, Norway
School of Education, Durham University, Durham, UK
Jens F Beckmann
You can also search for this author in PubMed Google Scholar
Corresponding author
Correspondence to Ronny Scherer .
Additional information
Competing interests.
The authors declare that they have no competing interests.
Authors’ contributions
RS carried out the analyses, participated in the development of the rationale, and drafted the manuscript. JFB carried out some additional analyses, participated in the development of the rationale, and drafted the manuscript. Both authors read and approved the final manuscript.
Authors’ original submitted files for images
Below are the links to the authors’ original submitted files for images.
Authors’ original file for figure 1
Authors’ original file for figure 2, authors’ original file for figure 3, rights and permissions.
Open Access This article is distributed under the terms of the Creative Commons Attribution 4.0 International License ( https://creativecommons.org/licenses/by/4.0 ), which permits use, duplication, adaptation, distribution, and reproduction in any medium or format, as long as you give appropriate credit to the original author(s) and the source, provide a link to the Creative Commons license, and indicate if changes were made.
Reprints and permissions
About this article
Cite this article.
Scherer, R., Beckmann, J.F. The acquisition of problem solving competence: evidence from 41 countries that math and science education matters. Large-scale Assess Educ 2 , 10 (2014). https://doi.org/10.1186/s40536-014-0010-7
Download citation
Received : 08 June 2014
Accepted : 25 November 2014
Published : 10 December 2014
DOI : https://doi.org/10.1186/s40536-014-0010-7
Share this article
Anyone you share the following link with will be able to read this content:
Sorry, a shareable link is not currently available for this article.
Provided by the Springer Nature SharedIt content-sharing initiative
- Capability under-utilisation
- Math-science coherence
- Math education
- Problem solving competence
- Science education
- Bipolar Disorder
- Therapy Center
- When To See a Therapist
- Types of Therapy
- Best Online Therapy
- Best Couples Therapy
- Best Family Therapy
- Managing Stress
- Sleep and Dreaming
- Understanding Emotions
- Self-Improvement
- Healthy Relationships
- Student Resources
- Personality Types
- Guided Meditations
- Verywell Mind Insights
- 2024 Verywell Mind 25
- Mental Health in the Classroom
- Editorial Process
- Meet Our Review Board
- Crisis Support
Overview of the Problem-Solving Mental Process
Kendra Cherry, MS, is a psychosocial rehabilitation specialist, psychology educator, and author of the "Everything Psychology Book."
:max_bytes(150000):strip_icc():format(webp)/IMG_9791-89504ab694d54b66bbd72cb84ffb860e.jpg)
Rachel Goldman, PhD FTOS, is a licensed psychologist, clinical assistant professor, speaker, wellness expert specializing in eating behaviors, stress management, and health behavior change.
:max_bytes(150000):strip_icc():format(webp)/Rachel-Goldman-1000-a42451caacb6423abecbe6b74e628042.jpg)
- Identify the Problem
- Define the Problem
- Form a Strategy
- Organize Information
- Allocate Resources
- Monitor Progress
- Evaluate the Results
Frequently Asked Questions
Problem-solving is a mental process that involves discovering, analyzing, and solving problems. The ultimate goal of problem-solving is to overcome obstacles and find a solution that best resolves the issue.
The best strategy for solving a problem depends largely on the unique situation. In some cases, people are better off learning everything they can about the issue and then using factual knowledge to come up with a solution. In other instances, creativity and insight are the best options.
It is not necessary to follow problem-solving steps sequentially, It is common to skip steps or even go back through steps multiple times until the desired solution is reached.
In order to correctly solve a problem, it is often important to follow a series of steps. Researchers sometimes refer to this as the problem-solving cycle. While this cycle is portrayed sequentially, people rarely follow a rigid series of steps to find a solution.
The following steps include developing strategies and organizing knowledge.
1. Identifying the Problem
While it may seem like an obvious step, identifying the problem is not always as simple as it sounds. In some cases, people might mistakenly identify the wrong source of a problem, which will make attempts to solve it inefficient or even useless.
Some strategies that you might use to figure out the source of a problem include :
- Asking questions about the problem
- Breaking the problem down into smaller pieces
- Looking at the problem from different perspectives
- Conducting research to figure out what relationships exist between different variables
2. Defining the Problem
After the problem has been identified, it is important to fully define the problem so that it can be solved. You can define a problem by operationally defining each aspect of the problem and setting goals for what aspects of the problem you will address
At this point, you should focus on figuring out which aspects of the problems are facts and which are opinions. State the problem clearly and identify the scope of the solution.
3. Forming a Strategy
After the problem has been identified, it is time to start brainstorming potential solutions. This step usually involves generating as many ideas as possible without judging their quality. Once several possibilities have been generated, they can be evaluated and narrowed down.
The next step is to develop a strategy to solve the problem. The approach used will vary depending upon the situation and the individual's unique preferences. Common problem-solving strategies include heuristics and algorithms.
- Heuristics are mental shortcuts that are often based on solutions that have worked in the past. They can work well if the problem is similar to something you have encountered before and are often the best choice if you need a fast solution.
- Algorithms are step-by-step strategies that are guaranteed to produce a correct result. While this approach is great for accuracy, it can also consume time and resources.
Heuristics are often best used when time is of the essence, while algorithms are a better choice when a decision needs to be as accurate as possible.
4. Organizing Information
Before coming up with a solution, you need to first organize the available information. What do you know about the problem? What do you not know? The more information that is available the better prepared you will be to come up with an accurate solution.
When approaching a problem, it is important to make sure that you have all the data you need. Making a decision without adequate information can lead to biased or inaccurate results.
5. Allocating Resources
Of course, we don't always have unlimited money, time, and other resources to solve a problem. Before you begin to solve a problem, you need to determine how high priority it is.
If it is an important problem, it is probably worth allocating more resources to solving it. If, however, it is a fairly unimportant problem, then you do not want to spend too much of your available resources on coming up with a solution.
At this stage, it is important to consider all of the factors that might affect the problem at hand. This includes looking at the available resources, deadlines that need to be met, and any possible risks involved in each solution. After careful evaluation, a decision can be made about which solution to pursue.
6. Monitoring Progress
After selecting a problem-solving strategy, it is time to put the plan into action and see if it works. This step might involve trying out different solutions to see which one is the most effective.
It is also important to monitor the situation after implementing a solution to ensure that the problem has been solved and that no new problems have arisen as a result of the proposed solution.
Effective problem-solvers tend to monitor their progress as they work towards a solution. If they are not making good progress toward reaching their goal, they will reevaluate their approach or look for new strategies .
7. Evaluating the Results
After a solution has been reached, it is important to evaluate the results to determine if it is the best possible solution to the problem. This evaluation might be immediate, such as checking the results of a math problem to ensure the answer is correct, or it can be delayed, such as evaluating the success of a therapy program after several months of treatment.
Once a problem has been solved, it is important to take some time to reflect on the process that was used and evaluate the results. This will help you to improve your problem-solving skills and become more efficient at solving future problems.
A Word From Verywell
It is important to remember that there are many different problem-solving processes with different steps, and this is just one example. Problem-solving in real-world situations requires a great deal of resourcefulness, flexibility, resilience, and continuous interaction with the environment.
Get Advice From The Verywell Mind Podcast
Hosted by therapist Amy Morin, LCSW, this episode of The Verywell Mind Podcast shares how you can stop dwelling in a negative mindset.
Follow Now : Apple Podcasts / Spotify / Google Podcasts
You can become a better problem solving by:
- Practicing brainstorming and coming up with multiple potential solutions to problems
- Being open-minded and considering all possible options before making a decision
- Breaking down problems into smaller, more manageable pieces
- Asking for help when needed
- Researching different problem-solving techniques and trying out new ones
- Learning from mistakes and using them as opportunities to grow
It's important to communicate openly and honestly with your partner about what's going on. Try to see things from their perspective as well as your own. Work together to find a resolution that works for both of you. Be willing to compromise and accept that there may not be a perfect solution.
Take breaks if things are getting too heated, and come back to the problem when you feel calm and collected. Don't try to fix every problem on your own—consider asking a therapist or counselor for help and insight.
If you've tried everything and there doesn't seem to be a way to fix the problem, you may have to learn to accept it. This can be difficult, but try to focus on the positive aspects of your life and remember that every situation is temporary. Don't dwell on what's going wrong—instead, think about what's going right. Find support by talking to friends or family. Seek professional help if you're having trouble coping.
Davidson JE, Sternberg RJ, editors. The Psychology of Problem Solving . Cambridge University Press; 2003. doi:10.1017/CBO9780511615771
Sarathy V. Real world problem-solving . Front Hum Neurosci . 2018;12:261. Published 2018 Jun 26. doi:10.3389/fnhum.2018.00261
By Kendra Cherry, MSEd Kendra Cherry, MS, is a psychosocial rehabilitation specialist, psychology educator, and author of the "Everything Psychology Book."
How to improve your problem solving skills and build effective problem solving strategies
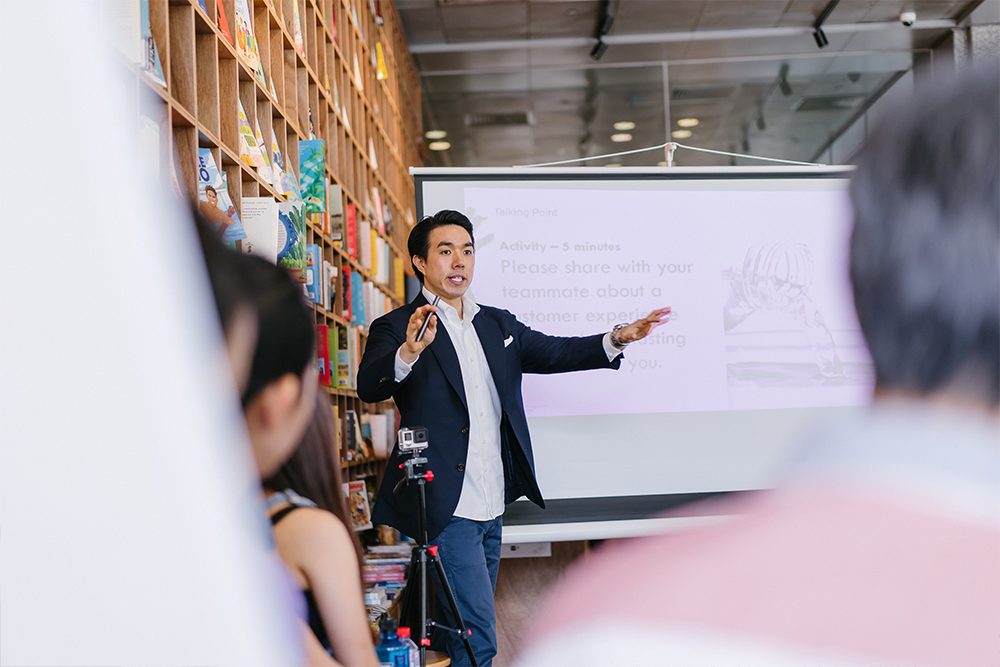
Design your next session with SessionLab
Join the 150,000+ facilitators using SessionLab.
Recommended Articles
A step-by-step guide to planning a workshop, how to create an unforgettable training session in 8 simple steps, 47 useful online tools for workshop planning and meeting facilitation.
Effective problem solving is all about using the right process and following a plan tailored to the issue at hand. Recognizing your team or organization has an issue isn’t enough to come up with effective problem solving strategies.
To truly understand a problem and develop appropriate solutions, you will want to follow a solid process, follow the necessary problem solving steps, and bring all of your problem solving skills to the table.
We’ll first guide you through the seven step problem solving process you and your team can use to effectively solve complex business challenges. We’ll also look at what problem solving strategies you can employ with your team when looking for a way to approach the process. We’ll then discuss the problem solving skills you need to be more effective at solving problems, complete with an activity from the SessionLab library you can use to develop that skill in your team.
Let’s get to it!
What is a problem solving process?
- What are the problem solving steps I need to follow?
Problem solving strategies
What skills do i need to be an effective problem solver, how can i improve my problem solving skills.
Solving problems is like baking a cake. You can go straight into the kitchen without a recipe or the right ingredients and do your best, but the end result is unlikely to be very tasty!
Using a process to bake a cake allows you to use the best ingredients without waste, collect the right tools, account for allergies, decide whether it is a birthday or wedding cake, and then bake efficiently and on time. The result is a better cake that is fit for purpose, tastes better and has created less mess in the kitchen. Also, it should have chocolate sprinkles. Having a step by step process to solve organizational problems allows you to go through each stage methodically and ensure you are trying to solve the right problems and select the most appropriate, effective solutions.
What are the problem solving steps I need to follow?
All problem solving processes go through a number of steps in order to move from identifying a problem to resolving it.
Depending on your problem solving model and who you ask, there can be anything between four and nine problem solving steps you should follow in order to find the right solution. Whatever framework you and your group use, there are some key items that should be addressed in order to have an effective process.
We’ve looked at problem solving processes from sources such as the American Society for Quality and their four step approach , and Mediate ‘s six step process. By reflecting on those and our own problem solving processes, we’ve come up with a sequence of seven problem solving steps we feel best covers everything you need in order to effectively solve problems.
1. Problem identification
The first stage of any problem solving process is to identify the problem or problems you might want to solve. Effective problem solving strategies always begin by allowing a group scope to articulate what they believe the problem to be and then coming to some consensus over which problem they approach first. Problem solving activities used at this stage often have a focus on creating frank, open discussion so that potential problems can be brought to the surface.
2. Problem analysis
Though this step is not a million miles from problem identification, problem analysis deserves to be considered separately. It can often be an overlooked part of the process and is instrumental when it comes to developing effective solutions.
The process of problem analysis means ensuring that the problem you are seeking to solve is the right problem . As part of this stage, you may look deeper and try to find the root cause of a specific problem at a team or organizational level.
Remember that problem solving strategies should not only be focused on putting out fires in the short term but developing long term solutions that deal with the root cause of organizational challenges.
Whatever your approach, analyzing a problem is crucial in being able to select an appropriate solution and the problem solving skills deployed in this stage are beneficial for the rest of the process and ensuring the solutions you create are fit for purpose.
3. Solution generation
Once your group has nailed down the particulars of the problem you wish to solve, you want to encourage a free flow of ideas connecting to solving that problem. This can take the form of problem solving games that encourage creative thinking or problem solving activities designed to produce working prototypes of possible solutions.
The key to ensuring the success of this stage of the problem solving process is to encourage quick, creative thinking and create an open space where all ideas are considered. The best solutions can come from unlikely places and by using problem solving techniques that celebrate invention, you might come up with solution gold.
4. Solution development
No solution is likely to be perfect right out of the gate. It’s important to discuss and develop the solutions your group has come up with over the course of following the previous problem solving steps in order to arrive at the best possible solution. Problem solving games used in this stage involve lots of critical thinking, measuring potential effort and impact, and looking at possible solutions analytically.
During this stage, you will often ask your team to iterate and improve upon your frontrunning solutions and develop them further. Remember that problem solving strategies always benefit from a multitude of voices and opinions, and not to let ego get involved when it comes to choosing which solutions to develop and take further.
Finding the best solution is the goal of all problem solving workshops and here is the place to ensure that your solution is well thought out, sufficiently robust and fit for purpose.
5. Decision making
Nearly there! Once your group has reached consensus and selected a solution that applies to the problem at hand you have some decisions to make. You will want to work on allocating ownership of the project, figure out who will do what, how the success of the solution will be measured and decide the next course of action.
The decision making stage is a part of the problem solving process that can get missed or taken as for granted. Fail to properly allocate roles and plan out how a solution will actually be implemented and it less likely to be successful in solving the problem.
Have clear accountabilities, actions, timeframes, and follow-ups. Make these decisions and set clear next-steps in the problem solving workshop so that everyone is aligned and you can move forward effectively as a group.
Ensuring that you plan for the roll-out of a solution is one of the most important problem solving steps. Without adequate planning or oversight, it can prove impossible to measure success or iterate further if the problem was not solved.
6. Solution implementation
This is what we were waiting for! All problem solving strategies have the end goal of implementing a solution and solving a problem in mind.
Remember that in order for any solution to be successful, you need to help your group through all of the previous problem solving steps thoughtfully. Only then can you ensure that you are solving the right problem but also that you have developed the correct solution and can then successfully implement and measure the impact of that solution.
Project management and communication skills are key here – your solution may need to adjust when out in the wild or you might discover new challenges along the way.
7. Solution evaluation
So you and your team developed a great solution to a problem and have a gut feeling its been solved. Work done, right? Wrong. All problem solving strategies benefit from evaluation, consideration, and feedback. You might find that the solution does not work for everyone, might create new problems, or is potentially so successful that you will want to roll it out to larger teams or as part of other initiatives.
None of that is possible without taking the time to evaluate the success of the solution you developed in your problem solving model and adjust if necessary.
Remember that the problem solving process is often iterative and it can be common to not solve complex issues on the first try. Even when this is the case, you and your team will have generated learning that will be important for future problem solving workshops or in other parts of the organization.
It’s worth underlining how important record keeping is throughout the problem solving process. If a solution didn’t work, you need to have the data and records to see why that was the case. If you go back to the drawing board, notes from the previous workshop can help save time. Data and insight is invaluable at every stage of the problem solving process and this one is no different.
Problem solving workshops made easy
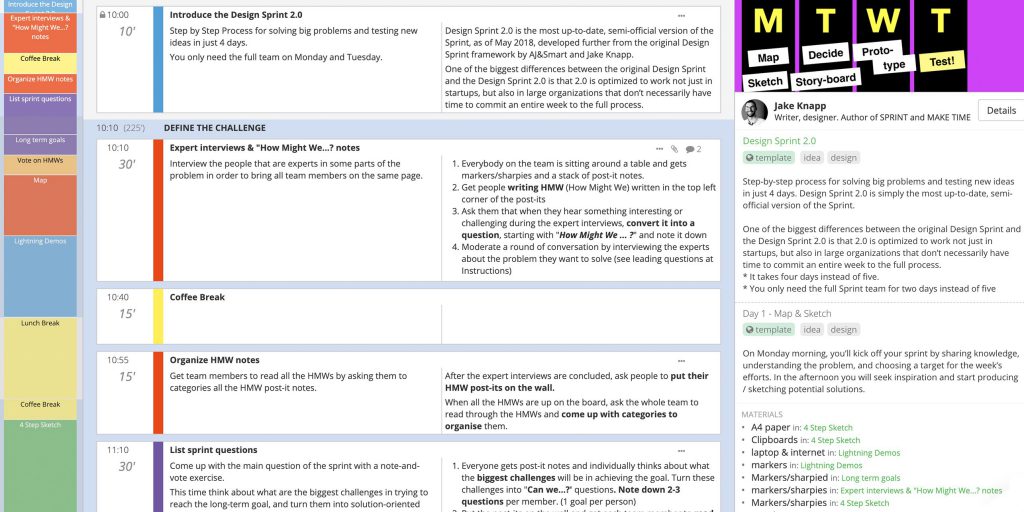
Problem solving strategies are methods of approaching and facilitating the process of problem-solving with a set of techniques , actions, and processes. Different strategies are more effective if you are trying to solve broad problems such as achieving higher growth versus more focused problems like, how do we improve our customer onboarding process?
Broadly, the problem solving steps outlined above should be included in any problem solving strategy though choosing where to focus your time and what approaches should be taken is where they begin to differ. You might find that some strategies ask for the problem identification to be done prior to the session or that everything happens in the course of a one day workshop.
The key similarity is that all good problem solving strategies are structured and designed. Four hours of open discussion is never going to be as productive as a four-hour workshop designed to lead a group through a problem solving process.
Good problem solving strategies are tailored to the team, organization and problem you will be attempting to solve. Here are some example problem solving strategies you can learn from or use to get started.
Use a workshop to lead a team through a group process
Often, the first step to solving problems or organizational challenges is bringing a group together effectively. Most teams have the tools, knowledge, and expertise necessary to solve their challenges – they just need some guidance in how to use leverage those skills and a structure and format that allows people to focus their energies.
Facilitated workshops are one of the most effective ways of solving problems of any scale. By designing and planning your workshop carefully, you can tailor the approach and scope to best fit the needs of your team and organization.
Problem solving workshop
- Creating a bespoke, tailored process
- Tackling problems of any size
- Building in-house workshop ability and encouraging their use
Workshops are an effective strategy for solving problems. By using tried and test facilitation techniques and methods, you can design and deliver a workshop that is perfectly suited to the unique variables of your organization. You may only have the capacity for a half-day workshop and so need a problem solving process to match.
By using our session planner tool and importing methods from our library of 700+ facilitation techniques, you can create the right problem solving workshop for your team. It might be that you want to encourage creative thinking or look at things from a new angle to unblock your groups approach to problem solving. By tailoring your workshop design to the purpose, you can help ensure great results.
One of the main benefits of a workshop is the structured approach to problem solving. Not only does this mean that the workshop itself will be successful, but many of the methods and techniques will help your team improve their working processes outside of the workshop.
We believe that workshops are one of the best tools you can use to improve the way your team works together. Start with a problem solving workshop and then see what team building, culture or design workshops can do for your organization!
Run a design sprint
Great for:
- aligning large, multi-discipline teams
- quickly designing and testing solutions
- tackling large, complex organizational challenges and breaking them down into smaller tasks
By using design thinking principles and methods, a design sprint is a great way of identifying, prioritizing and prototyping solutions to long term challenges that can help solve major organizational problems with quick action and measurable results.
Some familiarity with design thinking is useful, though not integral, and this strategy can really help a team align if there is some discussion around which problems should be approached first.
The stage-based structure of the design sprint is also very useful for teams new to design thinking. The inspiration phase, where you look to competitors that have solved your problem, and the rapid prototyping and testing phases are great for introducing new concepts that will benefit a team in all their future work.
It can be common for teams to look inward for solutions and so looking to the market for solutions you can iterate on can be very productive. Instilling an agile prototyping and testing mindset can also be great when helping teams move forwards – generating and testing solutions quickly can help save time in the long run and is also pretty exciting!
Break problems down into smaller issues
Organizational challenges and problems are often complicated and large scale in nature. Sometimes, trying to resolve such an issue in one swoop is simply unachievable or overwhelming. Try breaking down such problems into smaller issues that you can work on step by step. You may not be able to solve the problem of churning customers off the bat, but you can work with your team to identify smaller effort but high impact elements and work on those first.
This problem solving strategy can help a team generate momentum, prioritize and get some easy wins. It’s also a great strategy to employ with teams who are just beginning to learn how to approach the problem solving process. If you want some insight into a way to employ this strategy, we recommend looking at our design sprint template below!
Use guiding frameworks or try new methodologies
Some problems are best solved by introducing a major shift in perspective or by using new methodologies that encourage your team to think differently.
Props and tools such as Methodkit , which uses a card-based toolkit for facilitation, or Lego Serious Play can be great ways to engage your team and find an inclusive, democratic problem solving strategy. Remember that play and creativity are great tools for achieving change and whatever the challenge, engaging your participants can be very effective where other strategies may have failed.
LEGO Serious Play
- Improving core problem solving skills
- Thinking outside of the box
- Encouraging creative solutions
LEGO Serious Play is a problem solving methodology designed to get participants thinking differently by using 3D models and kinesthetic learning styles. By physically building LEGO models based on questions and exercises, participants are encouraged to think outside of the box and create their own responses.
Collaborate LEGO Serious Play exercises are also used to encourage communication and build problem solving skills in a group. By using this problem solving process, you can often help different kinds of learners and personality types contribute and unblock organizational problems with creative thinking.
Problem solving strategies like LEGO Serious Play are super effective at helping a team solve more skills-based problems such as communication between teams or a lack of creative thinking. Some problems are not suited to LEGO Serious Play and require a different problem solving strategy.
Card Decks and Method Kits
- New facilitators or non-facilitators
- Approaching difficult subjects with a simple, creative framework
- Engaging those with varied learning styles
Card decks and method kids are great tools for those new to facilitation or for whom facilitation is not the primary role. Card decks such as the emotional culture deck can be used for complete workshops and in many cases, can be used right out of the box. Methodkit has a variety of kits designed for scenarios ranging from personal development through to personas and global challenges so you can find the right deck for your particular needs.
Having an easy to use framework that encourages creativity or a new approach can take some of the friction or planning difficulties out of the workshop process and energize a team in any setting. Simplicity is the key with these methods. By ensuring everyone on your team can get involved and engage with the process as quickly as possible can really contribute to the success of your problem solving strategy.
Source external advice
Looking to peers, experts and external facilitators can be a great way of approaching the problem solving process. Your team may not have the necessary expertise, insights of experience to tackle some issues, or you might simply benefit from a fresh perspective. Some problems may require bringing together an entire team, and coaching managers or team members individually might be the right approach. Remember that not all problems are best resolved in the same manner.
If you’re a solo entrepreneur, peer groups, coaches and mentors can also be invaluable at not only solving specific business problems, but in providing a support network for resolving future challenges. One great approach is to join a Mastermind Group and link up with like-minded individuals and all grow together. Remember that however you approach the sourcing of external advice, do so thoughtfully, respectfully and honestly. Reciprocate where you can and prepare to be surprised by just how kind and helpful your peers can be!
Mastermind Group
- Solo entrepreneurs or small teams with low capacity
- Peer learning and gaining outside expertise
- Getting multiple external points of view quickly
Problem solving in large organizations with lots of skilled team members is one thing, but how about if you work for yourself or in a very small team without the capacity to get the most from a design sprint or LEGO Serious Play session?
A mastermind group – sometimes known as a peer advisory board – is where a group of people come together to support one another in their own goals, challenges, and businesses. Each participant comes to the group with their own purpose and the other members of the group will help them create solutions, brainstorm ideas, and support one another.
Mastermind groups are very effective in creating an energized, supportive atmosphere that can deliver meaningful results. Learning from peers from outside of your organization or industry can really help unlock new ways of thinking and drive growth. Access to the experience and skills of your peers can be invaluable in helping fill the gaps in your own ability, particularly in young companies.
A mastermind group is a great solution for solo entrepreneurs, small teams, or for organizations that feel that external expertise or fresh perspectives will be beneficial for them. It is worth noting that Mastermind groups are often only as good as the participants and what they can bring to the group. Participants need to be committed, engaged and understand how to work in this context.
Coaching and mentoring
- Focused learning and development
- Filling skills gaps
- Working on a range of challenges over time
Receiving advice from a business coach or building a mentor/mentee relationship can be an effective way of resolving certain challenges. The one-to-one format of most coaching and mentor relationships can really help solve the challenges those individuals are having and benefit the organization as a result.
A great mentor can be invaluable when it comes to spotting potential problems before they arise and coming to understand a mentee very well has a host of other business benefits. You might run an internal mentorship program to help develop your team’s problem solving skills and strategies or as part of a large learning and development program. External coaches can also be an important part of your problem solving strategy, filling skills gaps for your management team or helping with specific business issues.
Now we’ve explored the problem solving process and the steps you will want to go through in order to have an effective session, let’s look at the skills you and your team need to be more effective problem solvers.
Problem solving skills are highly sought after, whatever industry or team you work in. Organizations are keen to employ people who are able to approach problems thoughtfully and find strong, realistic solutions. Whether you are a facilitator , a team leader or a developer, being an effective problem solver is a skill you’ll want to develop.
Problem solving skills form a whole suite of techniques and approaches that an individual uses to not only identify problems but to discuss them productively before then developing appropriate solutions.
Here are some of the most important problem solving skills everyone from executives to junior staff members should learn. We’ve also included an activity or exercise from the SessionLab library that can help you and your team develop that skill.
If you’re running a workshop or training session to try and improve problem solving skills in your team, try using these methods to supercharge your process!
Active listening
Active listening is one of the most important skills anyone who works with people can possess. In short, active listening is a technique used to not only better understand what is being said by an individual, but also to be more aware of the underlying message the speaker is trying to convey. When it comes to problem solving, active listening is integral for understanding the position of every participant and to clarify the challenges, ideas and solutions they bring to the table.
Some active listening skills include:
- Paying complete attention to the speaker.
- Removing distractions.
- Avoid interruption.
- Taking the time to fully understand before preparing a rebuttal.
- Responding respectfully and appropriately.
- Demonstrate attentiveness and positivity with an open posture, making eye contact with the speaker, smiling and nodding if appropriate. Show that you are listening and encourage them to continue.
- Be aware of and respectful of feelings. Judge the situation and respond appropriately. You can disagree without being disrespectful.
- Observe body language.
- Paraphrase what was said in your own words, either mentally or verbally.
- Remain neutral.
- Reflect and take a moment before responding.
- Ask deeper questions based on what is said and clarify points where necessary.
Active Listening #hyperisland #skills #active listening #remote-friendly This activity supports participants to reflect on a question and generate their own solutions using simple principles of active listening and peer coaching. It’s an excellent introduction to active listening but can also be used with groups that are already familiar with it. Participants work in groups of three and take turns being: “the subject”, the listener, and the observer.
Analytical skills
All problem solving models require strong analytical skills, particularly during the beginning of the process and when it comes to analyzing how solutions have performed.
Analytical skills are primarily focused on performing an effective analysis by collecting, studying and parsing data related to a problem or opportunity.
It often involves spotting patterns, being able to see things from different perspectives and using observable facts and data to make suggestions or produce insight.
Analytical skills are also important at every stage of the problem solving process and by having these skills, you can ensure that any ideas or solutions you create or backed up analytically and have been sufficiently thought out.
Nine Whys #innovation #issue analysis #liberating structures With breathtaking simplicity, you can rapidly clarify for individuals and a group what is essentially important in their work. You can quickly reveal when a compelling purpose is missing in a gathering and avoid moving forward without clarity. When a group discovers an unambiguous shared purpose, more freedom and more responsibility are unleashed. You have laid the foundation for spreading and scaling innovations with fidelity.
Collaboration
Trying to solve problems on your own is difficult. Being able to collaborate effectively, with a free exchange of ideas, to delegate and be a productive member of a team is hugely important to all problem solving strategies.
Remember that whatever your role, collaboration is integral, and in a problem solving process, you are all working together to find the best solution for everyone.
Marshmallow challenge with debriefing #teamwork #team #leadership #collaboration In eighteen minutes, teams must build the tallest free-standing structure out of 20 sticks of spaghetti, one yard of tape, one yard of string, and one marshmallow. The marshmallow needs to be on top. The Marshmallow Challenge was developed by Tom Wujec, who has done the activity with hundreds of groups around the world. Visit the Marshmallow Challenge website for more information. This version has an extra debriefing question added with sample questions focusing on roles within the team.
Communication
Being an effective communicator means being empathetic, clear and succinct, asking the right questions, and demonstrating active listening skills throughout any discussion or meeting.
In a problem solving setting, you need to communicate well in order to progress through each stage of the process effectively. As a team leader, it may also fall to you to facilitate communication between parties who may not see eye to eye. Effective communication also means helping others to express themselves and be heard in a group.
Bus Trip #feedback #communication #appreciation #closing #thiagi #team This is one of my favourite feedback games. I use Bus Trip at the end of a training session or a meeting, and I use it all the time. The game creates a massive amount of energy with lots of smiles, laughs, and sometimes even a teardrop or two.
Creative problem solving skills can be some of the best tools in your arsenal. Thinking creatively, being able to generate lots of ideas and come up with out of the box solutions is useful at every step of the process.
The kinds of problems you will likely discuss in a problem solving workshop are often difficult to solve, and by approaching things in a fresh, creative manner, you can often create more innovative solutions.
Having practical creative skills is also a boon when it comes to problem solving. If you can help create quality design sketches and prototypes in record time, it can help bring a team to alignment more quickly or provide a base for further iteration.
The paper clip method #sharing #creativity #warm up #idea generation #brainstorming The power of brainstorming. A training for project leaders, creativity training, and to catalyse getting new solutions.
Critical thinking
Critical thinking is one of the fundamental problem solving skills you’ll want to develop when working on developing solutions. Critical thinking is the ability to analyze, rationalize and evaluate while being aware of personal bias, outlying factors and remaining open-minded.
Defining and analyzing problems without deploying critical thinking skills can mean you and your team go down the wrong path. Developing solutions to complex issues requires critical thinking too – ensuring your team considers all possibilities and rationally evaluating them.
Agreement-Certainty Matrix #issue analysis #liberating structures #problem solving You can help individuals or groups avoid the frequent mistake of trying to solve a problem with methods that are not adapted to the nature of their challenge. The combination of two questions makes it possible to easily sort challenges into four categories: simple, complicated, complex , and chaotic . A problem is simple when it can be solved reliably with practices that are easy to duplicate. It is complicated when experts are required to devise a sophisticated solution that will yield the desired results predictably. A problem is complex when there are several valid ways to proceed but outcomes are not predictable in detail. Chaotic is when the context is too turbulent to identify a path forward. A loose analogy may be used to describe these differences: simple is like following a recipe, complicated like sending a rocket to the moon, complex like raising a child, and chaotic is like the game “Pin the Tail on the Donkey.” The Liberating Structures Matching Matrix in Chapter 5 can be used as the first step to clarify the nature of a challenge and avoid the mismatches between problems and solutions that are frequently at the root of chronic, recurring problems.
Data analysis
Though it shares lots of space with general analytical skills, data analysis skills are something you want to cultivate in their own right in order to be an effective problem solver.
Being good at data analysis doesn’t just mean being able to find insights from data, but also selecting the appropriate data for a given issue, interpreting it effectively and knowing how to model and present that data. Depending on the problem at hand, it might also include a working knowledge of specific data analysis tools and procedures.
Having a solid grasp of data analysis techniques is useful if you’re leading a problem solving workshop but if you’re not an expert, don’t worry. Bring people into the group who has this skill set and help your team be more effective as a result.
Decision making
All problems need a solution and all solutions require that someone make the decision to implement them. Without strong decision making skills, teams can become bogged down in discussion and less effective as a result.
Making decisions is a key part of the problem solving process. It’s important to remember that decision making is not restricted to the leadership team. Every staff member makes decisions every day and developing these skills ensures that your team is able to solve problems at any scale. Remember that making decisions does not mean leaping to the first solution but weighing up the options and coming to an informed, well thought out solution to any given problem that works for the whole team.
Lightning Decision Jam (LDJ) #action #decision making #problem solving #issue analysis #innovation #design #remote-friendly The problem with anything that requires creative thinking is that it’s easy to get lost—lose focus and fall into the trap of having useless, open-ended, unstructured discussions. Here’s the most effective solution I’ve found: Replace all open, unstructured discussion with a clear process. What to use this exercise for: Anything which requires a group of people to make decisions, solve problems or discuss challenges. It’s always good to frame an LDJ session with a broad topic, here are some examples: The conversion flow of our checkout Our internal design process How we organise events Keeping up with our competition Improving sales flow
Dependability
Most complex organizational problems require multiple people to be involved in delivering the solution. Ensuring that the team and organization can depend on you to take the necessary actions and communicate where necessary is key to ensuring problems are solved effectively.
Being dependable also means working to deadlines and to brief. It is often a matter of creating trust in a team so that everyone can depend on one another to complete the agreed actions in the agreed time frame so that the team can move forward together. Being undependable can create problems of friction and can limit the effectiveness of your solutions so be sure to bear this in mind throughout a project.
Team Purpose & Culture #team #hyperisland #culture #remote-friendly This is an essential process designed to help teams define their purpose (why they exist) and their culture (how they work together to achieve that purpose). Defining these two things will help any team to be more focused and aligned. With support of tangible examples from other companies, the team members work as individuals and a group to codify the way they work together. The goal is a visual manifestation of both the purpose and culture that can be put up in the team’s work space.
Emotional intelligence
Emotional intelligence is an important skill for any successful team member, whether communicating internally or with clients or users. In the problem solving process, emotional intelligence means being attuned to how people are feeling and thinking, communicating effectively and being self-aware of what you bring to a room.
There are often differences of opinion when working through problem solving processes, and it can be easy to let things become impassioned or combative. Developing your emotional intelligence means being empathetic to your colleagues and managing your own emotions throughout the problem and solution process. Be kind, be thoughtful and put your points across care and attention.
Being emotionally intelligent is a skill for life and by deploying it at work, you can not only work efficiently but empathetically. Check out the emotional culture workshop template for more!
Facilitation
As we’ve clarified in our facilitation skills post, facilitation is the art of leading people through processes towards agreed-upon objectives in a manner that encourages participation, ownership, and creativity by all those involved. While facilitation is a set of interrelated skills in itself, the broad definition of facilitation can be invaluable when it comes to problem solving. Leading a team through a problem solving process is made more effective if you improve and utilize facilitation skills – whether you’re a manager, team leader or external stakeholder.
The Six Thinking Hats #creative thinking #meeting facilitation #problem solving #issue resolution #idea generation #conflict resolution The Six Thinking Hats are used by individuals and groups to separate out conflicting styles of thinking. They enable and encourage a group of people to think constructively together in exploring and implementing change, rather than using argument to fight over who is right and who is wrong.
Flexibility
Being flexible is a vital skill when it comes to problem solving. This does not mean immediately bowing to pressure or changing your opinion quickly: instead, being flexible is all about seeing things from new perspectives, receiving new information and factoring it into your thought process.
Flexibility is also important when it comes to rolling out solutions. It might be that other organizational projects have greater priority or require the same resources as your chosen solution. Being flexible means understanding needs and challenges across the team and being open to shifting or arranging your own schedule as necessary. Again, this does not mean immediately making way for other projects. It’s about articulating your own needs, understanding the needs of others and being able to come to a meaningful compromise.
The Creativity Dice #creativity #problem solving #thiagi #issue analysis Too much linear thinking is hazardous to creative problem solving. To be creative, you should approach the problem (or the opportunity) from different points of view. You should leave a thought hanging in mid-air and move to another. This skipping around prevents premature closure and lets your brain incubate one line of thought while you consciously pursue another.
Working in any group can lead to unconscious elements of groupthink or situations in which you may not wish to be entirely honest. Disagreeing with the opinions of the executive team or wishing to save the feelings of a coworker can be tricky to navigate, but being honest is absolutely vital when to comes to developing effective solutions and ensuring your voice is heard.
Remember that being honest does not mean being brutally candid. You can deliver your honest feedback and opinions thoughtfully and without creating friction by using other skills such as emotional intelligence.
Explore your Values #hyperisland #skills #values #remote-friendly Your Values is an exercise for participants to explore what their most important values are. It’s done in an intuitive and rapid way to encourage participants to follow their intuitive feeling rather than over-thinking and finding the “correct” values. It is a good exercise to use to initiate reflection and dialogue around personal values.
Initiative
The problem solving process is multi-faceted and requires different approaches at certain points of the process. Taking initiative to bring problems to the attention of the team, collect data or lead the solution creating process is always valuable. You might even roadtest your own small scale solutions or brainstorm before a session. Taking initiative is particularly effective if you have good deal of knowledge in that area or have ownership of a particular project and want to get things kickstarted.
That said, be sure to remember to honor the process and work in service of the team. If you are asked to own one part of the problem solving process and you don’t complete that task because your initiative leads you to work on something else, that’s not an effective method of solving business challenges.
15% Solutions #action #liberating structures #remote-friendly You can reveal the actions, however small, that everyone can do immediately. At a minimum, these will create momentum, and that may make a BIG difference. 15% Solutions show that there is no reason to wait around, feel powerless, or fearful. They help people pick it up a level. They get individuals and the group to focus on what is within their discretion instead of what they cannot change. With a very simple question, you can flip the conversation to what can be done and find solutions to big problems that are often distributed widely in places not known in advance. Shifting a few grains of sand may trigger a landslide and change the whole landscape.
Impartiality
A particularly useful problem solving skill for product owners or managers is the ability to remain impartial throughout much of the process. In practice, this means treating all points of view and ideas brought forward in a meeting equally and ensuring that your own areas of interest or ownership are not favored over others.
There may be a stage in the process where a decision maker has to weigh the cost and ROI of possible solutions against the company roadmap though even then, ensuring that the decision made is based on merit and not personal opinion.
Empathy map #frame insights #create #design #issue analysis An empathy map is a tool to help a design team to empathize with the people they are designing for. You can make an empathy map for a group of people or for a persona. To be used after doing personas when more insights are needed.
Being a good leader means getting a team aligned, energized and focused around a common goal. In the problem solving process, strong leadership helps ensure that the process is efficient, that any conflicts are resolved and that a team is managed in the direction of success.
It’s common for managers or executives to assume this role in a problem solving workshop, though it’s important that the leader maintains impartiality and does not bulldoze the group in a particular direction. Remember that good leadership means working in service of the purpose and team and ensuring the workshop is a safe space for employees of any level to contribute. Take a look at our leadership games and activities post for more exercises and methods to help improve leadership in your organization.
Leadership Pizza #leadership #team #remote-friendly This leadership development activity offers a self-assessment framework for people to first identify what skills, attributes and attitudes they find important for effective leadership, and then assess their own development and initiate goal setting.
In the context of problem solving, mediation is important in keeping a team engaged, happy and free of conflict. When leading or facilitating a problem solving workshop, you are likely to run into differences of opinion. Depending on the nature of the problem, certain issues may be brought up that are emotive in nature.
Being an effective mediator means helping those people on either side of such a divide are heard, listen to one another and encouraged to find common ground and a resolution. Mediating skills are useful for leaders and managers in many situations and the problem solving process is no different.
Conflict Responses #hyperisland #team #issue resolution A workshop for a team to reflect on past conflicts, and use them to generate guidelines for effective conflict handling. The workshop uses the Thomas-Killman model of conflict responses to frame a reflective discussion. Use it to open up a discussion around conflict with a team.
Planning
Solving organizational problems is much more effective when following a process or problem solving model. Planning skills are vital in order to structure, deliver and follow-through on a problem solving workshop and ensure your solutions are intelligently deployed.
Planning skills include the ability to organize tasks and a team, plan and design the process and take into account any potential challenges. Taking the time to plan carefully can save time and frustration later in the process and is valuable for ensuring a team is positioned for success.
3 Action Steps #hyperisland #action #remote-friendly This is a small-scale strategic planning session that helps groups and individuals to take action toward a desired change. It is often used at the end of a workshop or programme. The group discusses and agrees on a vision, then creates some action steps that will lead them towards that vision. The scope of the challenge is also defined, through discussion of the helpful and harmful factors influencing the group.
Prioritization
As organisations grow, the scale and variation of problems they face multiplies. Your team or is likely to face numerous challenges in different areas and so having the skills to analyze and prioritize becomes very important, particularly for those in leadership roles.
A thorough problem solving process is likely to deliver multiple solutions and you may have several different problems you wish to solve simultaneously. Prioritization is the ability to measure the importance, value, and effectiveness of those possible solutions and choose which to enact and in what order. The process of prioritization is integral in ensuring the biggest challenges are addressed with the most impactful solutions.
Impact and Effort Matrix #gamestorming #decision making #action #remote-friendly In this decision-making exercise, possible actions are mapped based on two factors: effort required to implement and potential impact. Categorizing ideas along these lines is a useful technique in decision making, as it obliges contributors to balance and evaluate suggested actions before committing to them.
Project management
Some problem solving skills are utilized in a workshop or ideation phases, while others come in useful when it comes to decision making. Overseeing an entire problem solving process and ensuring its success requires strong project management skills.
While project management incorporates many of the other skills listed here, it is important to note the distinction of considering all of the factors of a project and managing them successfully. Being able to negotiate with stakeholders, manage tasks, time and people, consider costs and ROI, and tie everything together is massively helpful when going through the problem solving process.
Record keeping
Working out meaningful solutions to organizational challenges is only one part of the process. Thoughtfully documenting and keeping records of each problem solving step for future consultation is important in ensuring efficiency and meaningful change.
For example, some problems may be lower priority than others but can be revisited in the future. If the team has ideated on solutions and found some are not up to the task, record those so you can rule them out and avoiding repeating work. Keeping records of the process also helps you improve and refine your problem solving model next time around!
Personal Kanban #gamestorming #action #agile #project planning Personal Kanban is a tool for organizing your work to be more efficient and productive. It is based on agile methods and principles.
Research skills
Conducting research to support both the identification of problems and the development of appropriate solutions is important for an effective process. Knowing where to go to collect research, how to conduct research efficiently, and identifying pieces of research are relevant are all things a good researcher can do well.
In larger groups, not everyone has to demonstrate this ability in order for a problem solving workshop to be effective. That said, having people with research skills involved in the process, particularly if they have existing area knowledge, can help ensure the solutions that are developed with data that supports their intention. Remember that being able to deliver the results of research efficiently and in a way the team can easily understand is also important. The best data in the world is only as effective as how it is delivered and interpreted.
Customer experience map #ideation #concepts #research #design #issue analysis #remote-friendly Customer experience mapping is a method of documenting and visualizing the experience a customer has as they use the product or service. It also maps out their responses to their experiences. To be used when there is a solution (even in a conceptual stage) that can be analyzed.
Risk management
Managing risk is an often overlooked part of the problem solving process. Solutions are often developed with the intention of reducing exposure to risk or solving issues that create risk but sometimes, great solutions are more experimental in nature and as such, deploying them needs to be carefully considered.
Managing risk means acknowledging that there may be risks associated with more out of the box solutions or trying new things, but that this must be measured against the possible benefits and other organizational factors.
Be informed, get the right data and stakeholders in the room and you can appropriately factor risk into your decision making process.
Decisions, Decisions… #communication #decision making #thiagi #action #issue analysis When it comes to decision-making, why are some of us more prone to take risks while others are risk-averse? One explanation might be the way the decision and options were presented. This exercise, based on Kahneman and Tversky’s classic study , illustrates how the framing effect influences our judgement and our ability to make decisions . The participants are divided into two groups. Both groups are presented with the same problem and two alternative programs for solving them. The two programs both have the same consequences but are presented differently. The debriefing discussion examines how the framing of the program impacted the participant’s decision.
Team-building
No single person is as good at problem solving as a team. Building an effective team and helping them come together around a common purpose is one of the most important problem solving skills, doubly so for leaders. By bringing a team together and helping them work efficiently, you pave the way for team ownership of a problem and the development of effective solutions.
In a problem solving workshop, it can be tempting to jump right into the deep end, though taking the time to break the ice, energize the team and align them with a game or exercise will pay off over the course of the day.
Remember that you will likely go through the problem solving process multiple times over an organization’s lifespan and building a strong team culture will make future problem solving more effective. It’s also great to work with people you know, trust and have fun with. Working on team building in and out of the problem solving process is a hallmark of successful teams that can work together to solve business problems.
9 Dimensions Team Building Activity #ice breaker #teambuilding #team #remote-friendly 9 Dimensions is a powerful activity designed to build relationships and trust among team members. There are 2 variations of this icebreaker. The first version is for teams who want to get to know each other better. The second version is for teams who want to explore how they are working together as a team.
Time management
The problem solving process is designed to lead a team from identifying a problem through to delivering a solution and evaluating its effectiveness. Without effective time management skills or timeboxing of tasks, it can be easy for a team to get bogged down or be inefficient.
By using a problem solving model and carefully designing your workshop, you can allocate time efficiently and trust that the process will deliver the results you need in a good timeframe.
Time management also comes into play when it comes to rolling out solutions, particularly those that are experimental in nature. Having a clear timeframe for implementing and evaluating solutions is vital for ensuring their success and being able to pivot if necessary.
Improving your skills at problem solving is often a career-long pursuit though there are methods you can use to make the learning process more efficient and to supercharge your problem solving skillset.
Remember that the skills you need to be a great problem solver have a large overlap with those skills you need to be effective in any role. Investing time and effort to develop your active listening or critical thinking skills is valuable in any context. Here are 7 ways to improve your problem solving skills.
Share best practices
Remember that your team is an excellent source of skills, wisdom, and techniques and that you should all take advantage of one another where possible. Best practices that one team has for solving problems, conducting research or making decisions should be shared across the organization. If you have in-house staff that have done active listening training or are data analysis pros, have them lead a training session.
Your team is one of your best resources. Create space and internal processes for the sharing of skills so that you can all grow together.
Ask for help and attend training
Once you’ve figured out you have a skills gap, the next step is to take action to fill that skills gap. That might be by asking your superior for training or coaching, or liaising with team members with that skill set. You might even attend specialized training for certain skills – active listening or critical thinking, for example, are business-critical skills that are regularly offered as part of a training scheme.
Whatever method you choose, remember that taking action of some description is necessary for growth. Whether that means practicing, getting help, attending training or doing some background reading, taking active steps to improve your skills is the way to go.
Learn a process
Problem solving can be complicated, particularly when attempting to solve large problems for the first time. Using a problem solving process helps give structure to your problem solving efforts and focus on creating outcomes, rather than worrying about the format.
Tools such as the seven-step problem solving process above are effective because not only do they feature steps that will help a team solve problems, they also develop skills along the way. Each step asks for people to engage with the process using different skills and in doing so, helps the team learn and grow together. Group processes of varying complexity and purpose can also be found in the SessionLab library of facilitation techniques . Using a tried and tested process and really help ease the learning curve for both those leading such a process, as well as those undergoing the purpose.
Effective teams make decisions about where they should and shouldn’t expend additional effort. By using a problem solving process, you can focus on the things that matter, rather than stumbling towards a solution haphazardly.

Create a feedback loop
Some skills gaps are more obvious than others. It’s possible that your perception of your active listening skills differs from those of your colleagues.
It’s valuable to create a system where team members can provide feedback in an ordered and friendly manner so they can all learn from one another. Only by identifying areas of improvement can you then work to improve them.
Remember that feedback systems require oversight and consideration so that they don’t turn into a place to complain about colleagues. Design the system intelligently so that you encourage the creation of learning opportunities, rather than encouraging people to list their pet peeves.
While practice might not make perfect, it does make the problem solving process easier. If you are having trouble with critical thinking, don’t shy away from doing it. Get involved where you can and stretch those muscles as regularly as possible.
Problem solving skills come more naturally to some than to others and that’s okay. Take opportunities to get involved and see where you can practice your skills in situations outside of a workshop context. Try collaborating in other circumstances at work or conduct data analysis on your own projects. You can often develop those skills you need for problem solving simply by doing them. Get involved!
Use expert exercises and methods
Learn from the best. Our library of 700+ facilitation techniques is full of activities and methods that help develop the skills you need to be an effective problem solver. Check out our templates to see how to approach problem solving and other organizational challenges in a structured and intelligent manner.
There is no single approach to improving problem solving skills, but by using the techniques employed by others you can learn from their example and develop processes that have seen proven results.
Try new ways of thinking and change your mindset
Using tried and tested exercises that you know well can help deliver results, but you do run the risk of missing out on the learning opportunities offered by new approaches. As with the problem solving process, changing your mindset can remove blockages and be used to develop your problem solving skills.
Most teams have members with mixed skill sets and specialties. Mix people from different teams and share skills and different points of view. Teach your customer support team how to use design thinking methods or help your developers with conflict resolution techniques. Try switching perspectives with facilitation techniques like Flip It! or by using new problem solving methodologies or models. Give design thinking, liberating structures or lego serious play a try if you want to try a new approach. You will find that framing problems in new ways and using existing skills in new contexts can be hugely useful for personal development and improving your skillset. It’s also a lot of fun to try new things. Give it a go!
Encountering business challenges and needing to find appropriate solutions is not unique to your organization. Lots of very smart people have developed methods, theories and approaches to help develop problem solving skills and create effective solutions. Learn from them!
Books like The Art of Thinking Clearly , Think Smarter, or Thinking Fast, Thinking Slow are great places to start, though it’s also worth looking at blogs related to organizations facing similar problems to yours, or browsing for success stories. Seeing how Dropbox massively increased growth and working backward can help you see the skills or approach you might be lacking to solve that same problem. Learning from others by reading their stories or approaches can be time-consuming but ultimately rewarding.
A tired, distracted mind is not in the best position to learn new skills. It can be tempted to burn the candle at both ends and develop problem solving skills outside of work. Absolutely use your time effectively and take opportunities for self-improvement, though remember that rest is hugely important and that without letting your brain rest, you cannot be at your most effective.
Creating distance between yourself and the problem you might be facing can also be useful. By letting an idea sit, you can find that a better one presents itself or you can develop it further. Take regular breaks when working and create a space for downtime. Remember that working smarter is preferable to working harder and that self-care is important for any effective learning or improvement process.
Want to design better group processes?
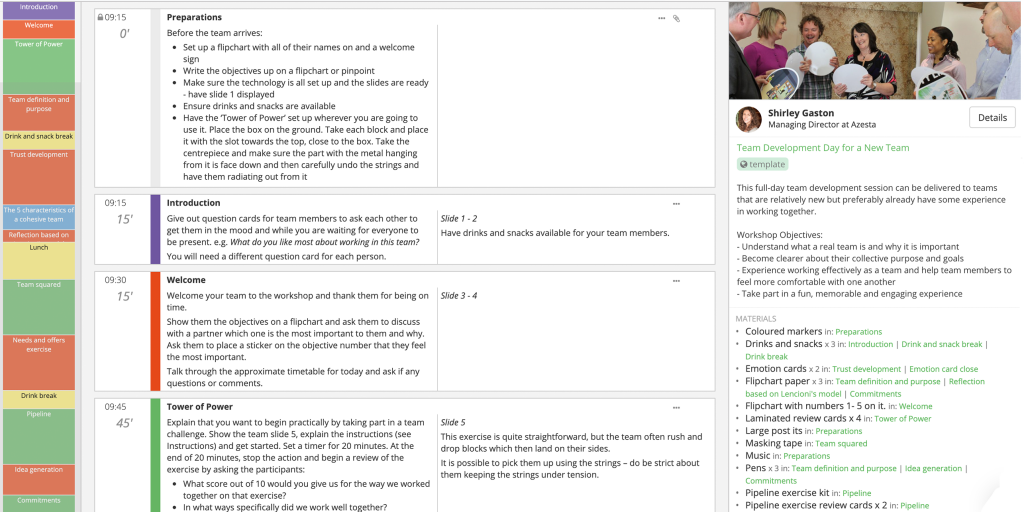
Over to you
Now we’ve explored some of the key problem solving skills and the problem solving steps necessary for an effective process, you’re ready to begin developing more effective solutions and leading problem solving workshops.
Need more inspiration? Check out our post on problem solving activities you can use when guiding a group towards a great solution in your next workshop or meeting. Have questions? Did you have a great problem solving technique you use with your team? Get in touch in the comments below. We’d love to chat!
Leave a Comment Cancel reply
Your email address will not be published. Required fields are marked *
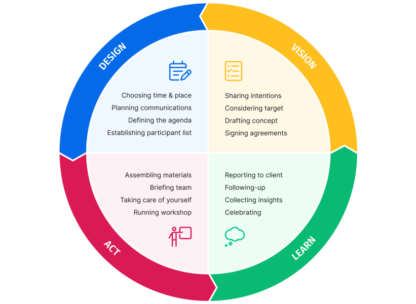
Going from a mere idea to a workshop that delivers results for your clients can feel like a daunting task. In this piece, we will shine a light on all the work behind the scenes and help you learn how to plan a workshop from start to finish. On a good day, facilitation can feel like effortless magic, but that is mostly the result of backstage work, foresight, and a lot of careful planning. Read on to learn a step-by-step approach to breaking the process of planning a workshop into small, manageable chunks. The flow starts with the first meeting with a client to define the purposes of a workshop.…
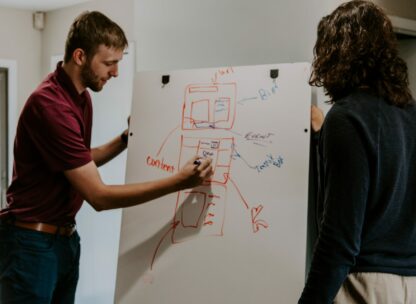
How does learning work? A clever 9-year-old once told me: “I know I am learning something new when I am surprised.” The science of adult learning tells us that, in order to learn new skills (which, unsurprisingly, is harder for adults to do than kids) grown-ups need to first get into a specific headspace. In a business, this approach is often employed in a training session where employees learn new skills or work on professional development. But how do you ensure your training is effective? In this guide, we'll explore how to create an effective training session plan and run engaging training sessions. As team leader, project manager, or consultant,…
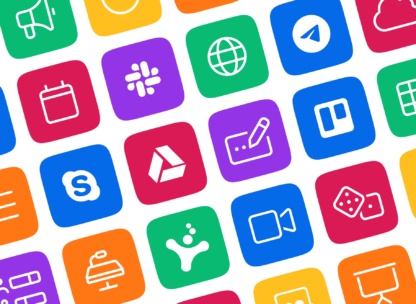
Effective online tools are a necessity for smooth and engaging virtual workshops and meetings. But how do you choose the right ones? Do you sometimes feel that the good old pen and paper or MS Office toolkit and email leaves you struggling to stay on top of managing and delivering your workshop? Fortunately, there are plenty of online tools to make your life easier when you need to facilitate a meeting and lead workshops. In this post, we’ll share our favorite online tools you can use to make your job as a facilitator easier. In fact, there are plenty of free online workshop tools and meeting facilitation software you can…
Design your next workshop with SessionLab
Join the 150,000 facilitators using SessionLab
Sign up for free
How to master the seven-step problem-solving process
In this episode of the McKinsey Podcast , Simon London speaks with Charles Conn, CEO of venture-capital firm Oxford Sciences Innovation, and McKinsey senior partner Hugo Sarrazin about the complexities of different problem-solving strategies.
Podcast transcript
Simon London: Hello, and welcome to this episode of the McKinsey Podcast , with me, Simon London. What’s the number-one skill you need to succeed professionally? Salesmanship, perhaps? Or a facility with statistics? Or maybe the ability to communicate crisply and clearly? Many would argue that at the very top of the list comes problem solving: that is, the ability to think through and come up with an optimal course of action to address any complex challenge—in business, in public policy, or indeed in life.
Looked at this way, it’s no surprise that McKinsey takes problem solving very seriously, testing for it during the recruiting process and then honing it, in McKinsey consultants, through immersion in a structured seven-step method. To discuss the art of problem solving, I sat down in California with McKinsey senior partner Hugo Sarrazin and also with Charles Conn. Charles is a former McKinsey partner, entrepreneur, executive, and coauthor of the book Bulletproof Problem Solving: The One Skill That Changes Everything [John Wiley & Sons, 2018].
Charles and Hugo, welcome to the podcast. Thank you for being here.
Hugo Sarrazin: Our pleasure.
Charles Conn: It’s terrific to be here.
Simon London: Problem solving is a really interesting piece of terminology. It could mean so many different things. I have a son who’s a teenage climber. They talk about solving problems. Climbing is problem solving. Charles, when you talk about problem solving, what are you talking about?
Charles Conn: For me, problem solving is the answer to the question “What should I do?” It’s interesting when there’s uncertainty and complexity, and when it’s meaningful because there are consequences. Your son’s climbing is a perfect example. There are consequences, and it’s complicated, and there’s uncertainty—can he make that grab? I think we can apply that same frame almost at any level. You can think about questions like “What town would I like to live in?” or “Should I put solar panels on my roof?”
You might think that’s a funny thing to apply problem solving to, but in my mind it’s not fundamentally different from business problem solving, which answers the question “What should my strategy be?” Or problem solving at the policy level: “How do we combat climate change?” “Should I support the local school bond?” I think these are all part and parcel of the same type of question, “What should I do?”
I’m a big fan of structured problem solving. By following steps, we can more clearly understand what problem it is we’re solving, what are the components of the problem that we’re solving, which components are the most important ones for us to pay attention to, which analytic techniques we should apply to those, and how we can synthesize what we’ve learned back into a compelling story. That’s all it is, at its heart.
I think sometimes when people think about seven steps, they assume that there’s a rigidity to this. That’s not it at all. It’s actually to give you the scope for creativity, which often doesn’t exist when your problem solving is muddled.
Simon London: You were just talking about the seven-step process. That’s what’s written down in the book, but it’s a very McKinsey process as well. Without getting too deep into the weeds, let’s go through the steps, one by one. You were just talking about problem definition as being a particularly important thing to get right first. That’s the first step. Hugo, tell us about that.
Hugo Sarrazin: It is surprising how often people jump past this step and make a bunch of assumptions. The most powerful thing is to step back and ask the basic questions—“What are we trying to solve? What are the constraints that exist? What are the dependencies?” Let’s make those explicit and really push the thinking and defining. At McKinsey, we spend an enormous amount of time in writing that little statement, and the statement, if you’re a logic purist, is great. You debate. “Is it an ‘or’? Is it an ‘and’? What’s the action verb?” Because all these specific words help you get to the heart of what matters.
Want to subscribe to The McKinsey Podcast ?
Simon London: So this is a concise problem statement.
Hugo Sarrazin: Yeah. It’s not like “Can we grow in Japan?” That’s interesting, but it is “What, specifically, are we trying to uncover in the growth of a product in Japan? Or a segment in Japan? Or a channel in Japan?” When you spend an enormous amount of time, in the first meeting of the different stakeholders, debating this and having different people put forward what they think the problem definition is, you realize that people have completely different views of why they’re here. That, to me, is the most important step.
Charles Conn: I would agree with that. For me, the problem context is critical. When we understand “What are the forces acting upon your decision maker? How quickly is the answer needed? With what precision is the answer needed? Are there areas that are off limits or areas where we would particularly like to find our solution? Is the decision maker open to exploring other areas?” then you not only become more efficient, and move toward what we call the critical path in problem solving, but you also make it so much more likely that you’re not going to waste your time or your decision maker’s time.
How often do especially bright young people run off with half of the idea about what the problem is and start collecting data and start building models—only to discover that they’ve really gone off half-cocked.
Hugo Sarrazin: Yeah.
Charles Conn: And in the wrong direction.
Simon London: OK. So step one—and there is a real art and a structure to it—is define the problem. Step two, Charles?
Charles Conn: My favorite step is step two, which is to use logic trees to disaggregate the problem. Every problem we’re solving has some complexity and some uncertainty in it. The only way that we can really get our team working on the problem is to take the problem apart into logical pieces.
What we find, of course, is that the way to disaggregate the problem often gives you an insight into the answer to the problem quite quickly. I love to do two or three different cuts at it, each one giving a bit of a different insight into what might be going wrong. By doing sensible disaggregations, using logic trees, we can figure out which parts of the problem we should be looking at, and we can assign those different parts to team members.
Simon London: What’s a good example of a logic tree on a sort of ratable problem?
Charles Conn: Maybe the easiest one is the classic profit tree. Almost in every business that I would take a look at, I would start with a profit or return-on-assets tree. In its simplest form, you have the components of revenue, which are price and quantity, and the components of cost, which are cost and quantity. Each of those can be broken out. Cost can be broken into variable cost and fixed cost. The components of price can be broken into what your pricing scheme is. That simple tree often provides insight into what’s going on in a business or what the difference is between that business and the competitors.
If we add the leg, which is “What’s the asset base or investment element?”—so profit divided by assets—then we can ask the question “Is the business using its investments sensibly?” whether that’s in stores or in manufacturing or in transportation assets. I hope we can see just how simple this is, even though we’re describing it in words.
When I went to work with Gordon Moore at the Moore Foundation, the problem that he asked us to look at was “How can we save Pacific salmon?” Now, that sounds like an impossible question, but it was amenable to precisely the same type of disaggregation and allowed us to organize what became a 15-year effort to improve the likelihood of good outcomes for Pacific salmon.
Simon London: Now, is there a danger that your logic tree can be impossibly large? This, I think, brings us onto the third step in the process, which is that you have to prioritize.
Charles Conn: Absolutely. The third step, which we also emphasize, along with good problem definition, is rigorous prioritization—we ask the questions “How important is this lever or this branch of the tree in the overall outcome that we seek to achieve? How much can I move that lever?” Obviously, we try and focus our efforts on ones that have a big impact on the problem and the ones that we have the ability to change. With salmon, ocean conditions turned out to be a big lever, but not one that we could adjust. We focused our attention on fish habitats and fish-harvesting practices, which were big levers that we could affect.
People spend a lot of time arguing about branches that are either not important or that none of us can change. We see it in the public square. When we deal with questions at the policy level—“Should you support the death penalty?” “How do we affect climate change?” “How can we uncover the causes and address homelessness?”—it’s even more important that we’re focusing on levers that are big and movable.
Would you like to learn more about our Strategy & Corporate Finance Practice ?
Simon London: Let’s move swiftly on to step four. You’ve defined your problem, you disaggregate it, you prioritize where you want to analyze—what you want to really look at hard. Then you got to the work plan. Now, what does that mean in practice?
Hugo Sarrazin: Depending on what you’ve prioritized, there are many things you could do. It could be breaking the work among the team members so that people have a clear piece of the work to do. It could be defining the specific analyses that need to get done and executed, and being clear on time lines. There’s always a level-one answer, there’s a level-two answer, there’s a level-three answer. Without being too flippant, I can solve any problem during a good dinner with wine. It won’t have a whole lot of backing.
Simon London: Not going to have a lot of depth to it.
Hugo Sarrazin: No, but it may be useful as a starting point. If the stakes are not that high, that could be OK. If it’s really high stakes, you may need level three and have the whole model validated in three different ways. You need to find a work plan that reflects the level of precision, the time frame you have, and the stakeholders you need to bring along in the exercise.
Charles Conn: I love the way you’ve described that, because, again, some people think of problem solving as a linear thing, but of course what’s critical is that it’s iterative. As you say, you can solve the problem in one day or even one hour.
Charles Conn: We encourage our teams everywhere to do that. We call it the one-day answer or the one-hour answer. In work planning, we’re always iterating. Every time you see a 50-page work plan that stretches out to three months, you know it’s wrong. It will be outmoded very quickly by that learning process that you described. Iterative problem solving is a critical part of this. Sometimes, people think work planning sounds dull, but it isn’t. It’s how we know what’s expected of us and when we need to deliver it and how we’re progressing toward the answer. It’s also the place where we can deal with biases. Bias is a feature of every human decision-making process. If we design our team interactions intelligently, we can avoid the worst sort of biases.
Simon London: Here we’re talking about cognitive biases primarily, right? It’s not that I’m biased against you because of your accent or something. These are the cognitive biases that behavioral sciences have shown we all carry around, things like anchoring, overoptimism—these kinds of things.
Both: Yeah.
Charles Conn: Availability bias is the one that I’m always alert to. You think you’ve seen the problem before, and therefore what’s available is your previous conception of it—and we have to be most careful about that. In any human setting, we also have to be careful about biases that are based on hierarchies, sometimes called sunflower bias. I’m sure, Hugo, with your teams, you make sure that the youngest team members speak first. Not the oldest team members, because it’s easy for people to look at who’s senior and alter their own creative approaches.
Hugo Sarrazin: It’s helpful, at that moment—if someone is asserting a point of view—to ask the question “This was true in what context?” You’re trying to apply something that worked in one context to a different one. That can be deadly if the context has changed, and that’s why organizations struggle to change. You promote all these people because they did something that worked well in the past, and then there’s a disruption in the industry, and they keep doing what got them promoted even though the context has changed.
Simon London: Right. Right.
Hugo Sarrazin: So it’s the same thing in problem solving.
Charles Conn: And it’s why diversity in our teams is so important. It’s one of the best things about the world that we’re in now. We’re likely to have people from different socioeconomic, ethnic, and national backgrounds, each of whom sees problems from a slightly different perspective. It is therefore much more likely that the team will uncover a truly creative and clever approach to problem solving.
Simon London: Let’s move on to step five. You’ve done your work plan. Now you’ve actually got to do the analysis. The thing that strikes me here is that the range of tools that we have at our disposal now, of course, is just huge, particularly with advances in computation, advanced analytics. There’s so many things that you can apply here. Just talk about the analysis stage. How do you pick the right tools?
Charles Conn: For me, the most important thing is that we start with simple heuristics and explanatory statistics before we go off and use the big-gun tools. We need to understand the shape and scope of our problem before we start applying these massive and complex analytical approaches.
Simon London: Would you agree with that?
Hugo Sarrazin: I agree. I think there are so many wonderful heuristics. You need to start there before you go deep into the modeling exercise. There’s an interesting dynamic that’s happening, though. In some cases, for some types of problems, it is even better to set yourself up to maximize your learning. Your problem-solving methodology is test and learn, test and learn, test and learn, and iterate. That is a heuristic in itself, the A/B testing that is used in many parts of the world. So that’s a problem-solving methodology. It’s nothing different. It just uses technology and feedback loops in a fast way. The other one is exploratory data analysis. When you’re dealing with a large-scale problem, and there’s so much data, I can get to the heuristics that Charles was talking about through very clever visualization of data.
You test with your data. You need to set up an environment to do so, but don’t get caught up in neural-network modeling immediately. You’re testing, you’re checking—“Is the data right? Is it sound? Does it make sense?”—before you launch too far.
Simon London: You do hear these ideas—that if you have a big enough data set and enough algorithms, they’re going to find things that you just wouldn’t have spotted, find solutions that maybe you wouldn’t have thought of. Does machine learning sort of revolutionize the problem-solving process? Or are these actually just other tools in the toolbox for structured problem solving?
Charles Conn: It can be revolutionary. There are some areas in which the pattern recognition of large data sets and good algorithms can help us see things that we otherwise couldn’t see. But I do think it’s terribly important we don’t think that this particular technique is a substitute for superb problem solving, starting with good problem definition. Many people use machine learning without understanding algorithms that themselves can have biases built into them. Just as 20 years ago, when we were doing statistical analysis, we knew that we needed good model definition, we still need a good understanding of our algorithms and really good problem definition before we launch off into big data sets and unknown algorithms.
Simon London: Step six. You’ve done your analysis.
Charles Conn: I take six and seven together, and this is the place where young problem solvers often make a mistake. They’ve got their analysis, and they assume that’s the answer, and of course it isn’t the answer. The ability to synthesize the pieces that came out of the analysis and begin to weave those into a story that helps people answer the question “What should I do?” This is back to where we started. If we can’t synthesize, and we can’t tell a story, then our decision maker can’t find the answer to “What should I do?”
Simon London: But, again, these final steps are about motivating people to action, right?
Charles Conn: Yeah.
Simon London: I am slightly torn about the nomenclature of problem solving because it’s on paper, right? Until you motivate people to action, you actually haven’t solved anything.
Charles Conn: I love this question because I think decision-making theory, without a bias to action, is a waste of time. Everything in how I approach this is to help people take action that makes the world better.
Simon London: Hence, these are absolutely critical steps. If you don’t do this well, you’ve just got a bunch of analysis.
Charles Conn: We end up in exactly the same place where we started, which is people speaking across each other, past each other in the public square, rather than actually working together, shoulder to shoulder, to crack these important problems.
Simon London: In the real world, we have a lot of uncertainty—arguably, increasing uncertainty. How do good problem solvers deal with that?
Hugo Sarrazin: At every step of the process. In the problem definition, when you’re defining the context, you need to understand those sources of uncertainty and whether they’re important or not important. It becomes important in the definition of the tree.
You need to think carefully about the branches of the tree that are more certain and less certain as you define them. They don’t have equal weight just because they’ve got equal space on the page. Then, when you’re prioritizing, your prioritization approach may put more emphasis on things that have low probability but huge impact—or, vice versa, may put a lot of priority on things that are very likely and, hopefully, have a reasonable impact. You can introduce that along the way. When you come back to the synthesis, you just need to be nuanced about what you’re understanding, the likelihood.
Often, people lack humility in the way they make their recommendations: “This is the answer.” They’re very precise, and I think we would all be well-served to say, “This is a likely answer under the following sets of conditions” and then make the level of uncertainty clearer, if that is appropriate. It doesn’t mean you’re always in the gray zone; it doesn’t mean you don’t have a point of view. It just means that you can be explicit about the certainty of your answer when you make that recommendation.
Simon London: So it sounds like there is an underlying principle: “Acknowledge and embrace the uncertainty. Don’t pretend that it isn’t there. Be very clear about what the uncertainties are up front, and then build that into every step of the process.”
Hugo Sarrazin: Every step of the process.
Simon London: Yeah. We have just walked through a particular structured methodology for problem solving. But, of course, this is not the only structured methodology for problem solving. One that is also very well-known is design thinking, which comes at things very differently. So, Hugo, I know you have worked with a lot of designers. Just give us a very quick summary. Design thinking—what is it, and how does it relate?
Hugo Sarrazin: It starts with an incredible amount of empathy for the user and uses that to define the problem. It does pause and go out in the wild and spend an enormous amount of time seeing how people interact with objects, seeing the experience they’re getting, seeing the pain points or joy—and uses that to infer and define the problem.
Simon London: Problem definition, but out in the world.
Hugo Sarrazin: With an enormous amount of empathy. There’s a huge emphasis on empathy. Traditional, more classic problem solving is you define the problem based on an understanding of the situation. This one almost presupposes that we don’t know the problem until we go see it. The second thing is you need to come up with multiple scenarios or answers or ideas or concepts, and there’s a lot of divergent thinking initially. That’s slightly different, versus the prioritization, but not for long. Eventually, you need to kind of say, “OK, I’m going to converge again.” Then you go and you bring things back to the customer and get feedback and iterate. Then you rinse and repeat, rinse and repeat. There’s a lot of tactile building, along the way, of prototypes and things like that. It’s very iterative.
Simon London: So, Charles, are these complements or are these alternatives?
Charles Conn: I think they’re entirely complementary, and I think Hugo’s description is perfect. When we do problem definition well in classic problem solving, we are demonstrating the kind of empathy, at the very beginning of our problem, that design thinking asks us to approach. When we ideate—and that’s very similar to the disaggregation, prioritization, and work-planning steps—we do precisely the same thing, and often we use contrasting teams, so that we do have divergent thinking. The best teams allow divergent thinking to bump them off whatever their initial biases in problem solving are. For me, design thinking gives us a constant reminder of creativity, empathy, and the tactile nature of problem solving, but it’s absolutely complementary, not alternative.
Simon London: I think, in a world of cross-functional teams, an interesting question is do people with design-thinking backgrounds really work well together with classical problem solvers? How do you make that chemistry happen?
Hugo Sarrazin: Yeah, it is not easy when people have spent an enormous amount of time seeped in design thinking or user-centric design, whichever word you want to use. If the person who’s applying classic problem-solving methodology is very rigid and mechanical in the way they’re doing it, there could be an enormous amount of tension. If there’s not clarity in the role and not clarity in the process, I think having the two together can be, sometimes, problematic.
The second thing that happens often is that the artifacts the two methodologies try to gravitate toward can be different. Classic problem solving often gravitates toward a model; design thinking migrates toward a prototype. Rather than writing a big deck with all my supporting evidence, they’ll bring an example, a thing, and that feels different. Then you spend your time differently to achieve those two end products, so that’s another source of friction.
Now, I still think it can be an incredibly powerful thing to have the two—if there are the right people with the right mind-set, if there is a team that is explicit about the roles, if we’re clear about the kind of outcomes we are attempting to bring forward. There’s an enormous amount of collaborativeness and respect.
Simon London: But they have to respect each other’s methodology and be prepared to flex, maybe, a little bit, in how this process is going to work.
Hugo Sarrazin: Absolutely.
Simon London: The other area where, it strikes me, there could be a little bit of a different sort of friction is this whole concept of the day-one answer, which is what we were just talking about in classical problem solving. Now, you know that this is probably not going to be your final answer, but that’s how you begin to structure the problem. Whereas I would imagine your design thinkers—no, they’re going off to do their ethnographic research and get out into the field, potentially for a long time, before they come back with at least an initial hypothesis.

Want better strategies? Become a bulletproof problem solver
Hugo Sarrazin: That is a great callout, and that’s another difference. Designers typically will like to soak into the situation and avoid converging too quickly. There’s optionality and exploring different options. There’s a strong belief that keeps the solution space wide enough that you can come up with more radical ideas. If there’s a large design team or many designers on the team, and you come on Friday and say, “What’s our week-one answer?” they’re going to struggle. They’re not going to be comfortable, naturally, to give that answer. It doesn’t mean they don’t have an answer; it’s just not where they are in their thinking process.
Simon London: I think we are, sadly, out of time for today. But Charles and Hugo, thank you so much.
Charles Conn: It was a pleasure to be here, Simon.
Hugo Sarrazin: It was a pleasure. Thank you.
Simon London: And thanks, as always, to you, our listeners, for tuning into this episode of the McKinsey Podcast . If you want to learn more about problem solving, you can find the book, Bulletproof Problem Solving: The One Skill That Changes Everything , online or order it through your local bookstore. To learn more about McKinsey, you can of course find us at McKinsey.com.
Charles Conn is CEO of Oxford Sciences Innovation and an alumnus of McKinsey’s Sydney office. Hugo Sarrazin is a senior partner in the Silicon Valley office, where Simon London, a member of McKinsey Publishing, is also based.
Explore a career with us
Related articles.
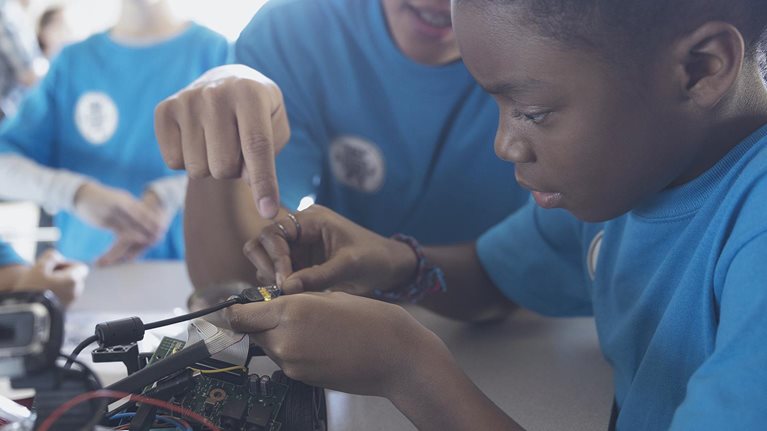
Strategy to beat the odds
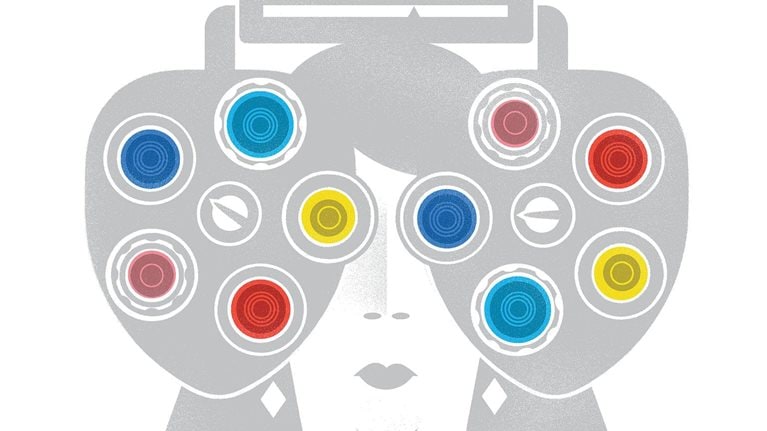
Five routes to more innovative problem solving

- school Campus Bookshelves
- menu_book Bookshelves
- perm_media Learning Objects
- login Login
- how_to_reg Request Instructor Account
- hub Instructor Commons
Margin Size
- Download Page (PDF)
- Download Full Book (PDF)
- Periodic Table
- Physics Constants
- Scientific Calculator
- Reference & Cite
- Tools expand_more
- Readability
selected template will load here
This action is not available.
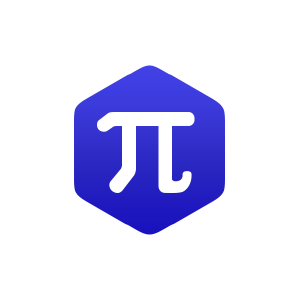
Module 1: Problem Solving Strategies
- Last updated
- Save as PDF
- Page ID 10352
\( \newcommand{\vecs}[1]{\overset { \scriptstyle \rightharpoonup} {\mathbf{#1}} } \)
\( \newcommand{\vecd}[1]{\overset{-\!-\!\rightharpoonup}{\vphantom{a}\smash {#1}}} \)
\( \newcommand{\id}{\mathrm{id}}\) \( \newcommand{\Span}{\mathrm{span}}\)
( \newcommand{\kernel}{\mathrm{null}\,}\) \( \newcommand{\range}{\mathrm{range}\,}\)
\( \newcommand{\RealPart}{\mathrm{Re}}\) \( \newcommand{\ImaginaryPart}{\mathrm{Im}}\)
\( \newcommand{\Argument}{\mathrm{Arg}}\) \( \newcommand{\norm}[1]{\| #1 \|}\)
\( \newcommand{\inner}[2]{\langle #1, #2 \rangle}\)
\( \newcommand{\Span}{\mathrm{span}}\)
\( \newcommand{\id}{\mathrm{id}}\)
\( \newcommand{\kernel}{\mathrm{null}\,}\)
\( \newcommand{\range}{\mathrm{range}\,}\)
\( \newcommand{\RealPart}{\mathrm{Re}}\)
\( \newcommand{\ImaginaryPart}{\mathrm{Im}}\)
\( \newcommand{\Argument}{\mathrm{Arg}}\)
\( \newcommand{\norm}[1]{\| #1 \|}\)
\( \newcommand{\Span}{\mathrm{span}}\) \( \newcommand{\AA}{\unicode[.8,0]{x212B}}\)
\( \newcommand{\vectorA}[1]{\vec{#1}} % arrow\)
\( \newcommand{\vectorAt}[1]{\vec{\text{#1}}} % arrow\)
\( \newcommand{\vectorB}[1]{\overset { \scriptstyle \rightharpoonup} {\mathbf{#1}} } \)
\( \newcommand{\vectorC}[1]{\textbf{#1}} \)
\( \newcommand{\vectorD}[1]{\overrightarrow{#1}} \)
\( \newcommand{\vectorDt}[1]{\overrightarrow{\text{#1}}} \)
\( \newcommand{\vectE}[1]{\overset{-\!-\!\rightharpoonup}{\vphantom{a}\smash{\mathbf {#1}}}} \)
Unlike exercises, there is never a simple recipe for solving a problem. You can get better and better at solving problems, both by building up your background knowledge and by simply practicing. As you solve more problems (and learn how other people solved them), you learn strategies and techniques that can be useful. But no single strategy works every time.
Pólya’s How to Solve It
George Pólya was a great champion in the field of teaching effective problem solving skills. He was born in Hungary in 1887, received his Ph.D. at the University of Budapest, and was a professor at Stanford University (among other universities). He wrote many mathematical papers along with three books, most famously, “How to Solve it.” Pólya died at the age 98 in 1985.1
1. Image of Pólya by Thane Plambeck from Palo Alto, California (Flickr) [CC BY
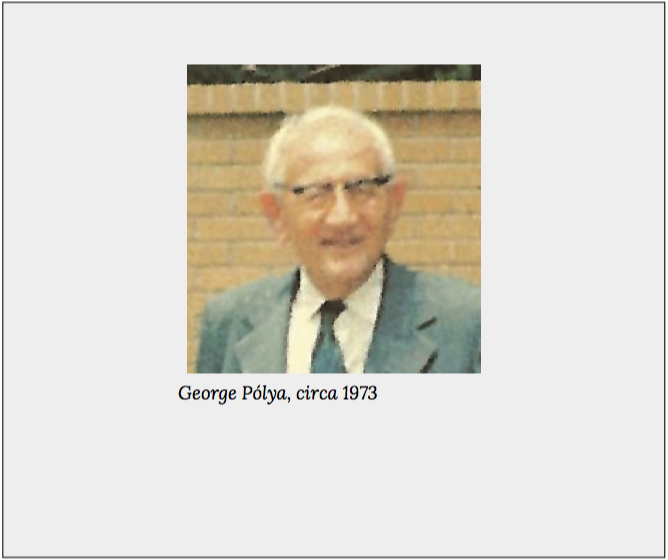
In 1945, Pólya published the short book How to Solve It , which gave a four-step method for solving mathematical problems:
First, you have to understand the problem.
After understanding, then make a plan.
Carry out the plan.
Look back on your work. How could it be better?
This is all well and good, but how do you actually do these steps?!?! Steps 1. and 2. are particularly mysterious! How do you “make a plan?” That is where you need some tools in your toolbox, and some experience to draw upon.
Much has been written since 1945 to explain these steps in more detail, but the truth is that they are more art than science. This is where math becomes a creative endeavor (and where it becomes so much fun). We will articulate some useful problem solving strategies, but no such list will ever be complete. This is really just a start to help you on your way. The best way to become a skilled problem solver is to learn the background material well, and then to solve a lot of problems!
Problem Solving Strategy 1 (Guess and Test)
Make a guess and test to see if it satisfies the demands of the problem. If it doesn't, alter the guess appropriately and check again. Keep doing this until you find a solution.
Mr. Jones has a total of 25 chickens and cows on his farm. How many of each does he have if all together there are 76 feet?
Step 1: Understanding the problem
We are given in the problem that there are 25 chickens and cows.
All together there are 76 feet.
Chickens have 2 feet and cows have 4 feet.
We are trying to determine how many cows and how many chickens Mr. Jones has on his farm.
Step 2: Devise a plan
Going to use Guess and test along with making a tab
Many times the strategy below is used with guess and test.
Make a table and look for a pattern:
Procedure: Make a table reflecting the data in the problem. If done in an orderly way, such a table will often reveal patterns and relationships that suggest how the problem can be solved.
Step 3: Carry out the plan:
Notice we are going in the wrong direction! The total number of feet is decreasing!
Better! The total number of feet are increasing!
Step 4: Looking back:
Check: 12 + 13 = 25 heads
24 + 52 = 76 feet.
We have found the solution to this problem. I could use this strategy when there are a limited number of possible answers and when two items are the same but they have one characteristic that is different.
Videos to watch:
1. Click on this link to see an example of “Guess and Test”
http://www.mathstories.com/strategies.htm
2. Click on this link to see another example of Guess and Test.
http://www.mathinaction.org/problem-solving-strategies.html
Check in question 1:
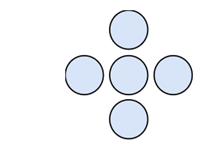
Place the digits 8, 10, 11, 12, and 13 in the circles to make the sums across and vertically equal 31. (5 points)
Check in question 2:
Old McDonald has 250 chickens and goats in the barnyard. Altogether there are 760 feet . How many of each animal does he have? Make sure you use Polya’s 4 problem solving steps. (12 points)
Problem Solving Strategy 2 (Draw a Picture). Some problems are obviously about a geometric situation, and it is clear you want to draw a picture and mark down all of the given information before you try to solve it. But even for a problem that is not geometric thinking visually can help!
Videos to watch demonstrating how to use "Draw a Picture".
1. Click on this link to see an example of “Draw a Picture”
2. Click on this link to see another example of Draw a Picture.
Problem Solving Strategy 3 ( Using a variable to find the sum of a sequence.)
Gauss's strategy for sequences.
last term = fixed number ( n -1) + first term
The fix number is the the amount each term is increasing or decreasing by. "n" is the number of terms you have. You can use this formula to find the last term in the sequence or the number of terms you have in a sequence.
Ex: 2, 5, 8, ... Find the 200th term.
Last term = 3(200-1) +2
Last term is 599.
To find the sum of a sequence: sum = [(first term + last term) (number of terms)]/ 2
Sum = (2 + 599) (200) then divide by 2
Sum = 60,100
Check in question 3: (10 points)
Find the 320 th term of 7, 10, 13, 16 …
Then find the sum of the first 320 terms.
Problem Solving Strategy 4 (Working Backwards)
This is considered a strategy in many schools. If you are given an answer, and the steps that were taken to arrive at that answer, you should be able to determine the starting point.
Videos to watch demonstrating of “Working Backwards”
https://www.youtube.com/watch?v=5FFWTsMEeJw
Karen is thinking of a number. If you double it, and subtract 7, you obtain 11. What is Karen’s number?
1. We start with 11 and work backwards.
2. The opposite of subtraction is addition. We will add 7 to 11. We are now at 18.
3. The opposite of doubling something is dividing by 2. 18/2 = 9
4. This should be our answer. Looking back:
9 x 2 = 18 -7 = 11
5. We have the right answer.
Check in question 4:
Christina is thinking of a number.
If you multiply her number by 93, add 6, and divide by 3, you obtain 436. What is her number? Solve this problem by working backwards. (5 points)
Problem Solving Strategy 5 (Looking for a Pattern)
Definition: A sequence is a pattern involving an ordered arrangement of numbers.
We first need to find a pattern.
Ask yourself as you search for a pattern – are the numbers growing steadily larger? Steadily smaller? How is each number related?
Example 1: 1, 4, 7, 10, 13…
Find the next 2 numbers. The pattern is each number is increasing by 3. The next two numbers would be 16 and 19.
Example 2: 1, 4, 9, 16 … find the next 2 numbers. It looks like each successive number is increase by the next odd number. 1 + 3 = 4.
So the next number would be
25 + 11 = 36
Example 3: 10, 7, 4, 1, -2… find the next 2 numbers.
In this sequence, the numbers are decreasing by 3. So the next 2 numbers would be -2 -3 = -5
-5 – 3 = -8
Example 4: 1, 2, 4, 8 … find the next two numbers.
This example is a little bit harder. The numbers are increasing but not by a constant. Maybe a factor?
So each number is being multiplied by 2.
16 x 2 = 32
1. Click on this link to see an example of “Looking for a Pattern”
2. Click on this link to see another example of Looking for a Pattern.
Problem Solving Strategy 6 (Make a List)
Example 1 : Can perfect squares end in a 2 or a 3?
List all the squares of the numbers 1 to 20.
1 4 9 16 25 36 49 64 81 100 121 144 169 196 225 256 289 324 361 400.
Now look at the number in the ones digits. Notice they are 0, 1, 4, 5, 6, or 9. Notice none of the perfect squares end in 2, 3, 7, or 8. This list suggests that perfect squares cannot end in a 2, 3, 7 or 8.
How many different amounts of money can you have in your pocket if you have only three coins including only dimes and quarters?
Quarter’s dimes
0 3 30 cents
1 2 45 cents
2 1 60 cents
3 0 75 cents
Videos demonstrating "Make a List"
Check in question 5:
How many ways can you make change for 23 cents using only pennies, nickels, and dimes? (10 points)
Problem Solving Strategy 7 (Solve a Simpler Problem)
Geometric Sequences:
How would we find the nth term?
Solve a simpler problem:
1, 3, 9, 27.
1. To get from 1 to 3 what did we do?
2. To get from 3 to 9 what did we do?
Let’s set up a table:
Term Number what did we do
Looking back: How would you find the nth term?
Find the 10 th term of the above sequence.
Let L = the tenth term
Problem Solving Strategy 8 (Process of Elimination)
This strategy can be used when there is only one possible solution.
I’m thinking of a number.
The number is odd.
It is more than 1 but less than 100.
It is greater than 20.
It is less than 5 times 7.
The sum of the digits is 7.
It is evenly divisible by 5.
a. We know it is an odd number between 1 and 100.
b. It is greater than 20 but less than 35
21, 23, 25, 27, 29, 31, 33, 35. These are the possibilities.
c. The sum of the digits is 7
21 (2+1=3) No 23 (2+3 = 5) No 25 (2 + 5= 7) Yes Using the same process we see there are no other numbers that meet this criteria. Also we notice 25 is divisible by 5. By using the strategy elimination, we have found our answer.
Check in question 6: (8 points)
Jose is thinking of a number.
The number is not odd.
The sum of the digits is divisible by 2.
The number is a multiple of 11.
It is greater than 5 times 4.
It is a multiple of 6
It is less than 7 times 8 +23
What is the number?
Click on this link for a quick review of the problem solving strategies.
https://garyhall.org.uk/maths-problem-solving-strategies.html
Problem-Solving Strategies
- First Online: 06 August 2020
Cite this chapter
- Orit Hazzan ORCID: orcid.org/0000-0002-8627-0997 4 ,
- Noa Ragonis ORCID: orcid.org/0000-0002-8163-0199 5 &
- Tami Lapidot 4
1387 Accesses
Problem-solving is generally considered as one of the most important and challenging cognitive activities in everyday as well as in any professional contexts. Specifically, it is one of the central activities performed by computer scientists as well as by computer science learners. However, it is not a uniform or linear process that can be taught as an algorithm to be followed, and the understanding of this individual process is not always clear. Computer science learners often face difficulties in performing two of the main stages of a problem-solving process: problem analysis and solution construction. Therefore, it is important that computer science educators be aware of these difficulties and acquire appropriate pedagogical tools to guide and scaffold learners in learning these skills. This chapter is dedicated to such pedagogical tools. It presents several problem-solving strategies to address in the MTCS course together with appropriate activities that mediate them to the prospective computer science teachers by enabling them to experience the different strategies.
This is a preview of subscription content, log in via an institution to check access.
Access this chapter
- Available as EPUB and PDF
- Read on any device
- Instant download
- Own it forever
- Compact, lightweight edition
- Dispatched in 3 to 5 business days
- Free shipping worldwide - see info
- Durable hardcover edition
Tax calculation will be finalised at checkout
Purchases are for personal use only
Institutional subscriptions
An algorithmic problem is defined by what is given – the initial conditions of the problem and its goals – the desired state, what should be accomplished. An algorithm problem can be solved with a series of actions formulated formally either by pseudo-code or a programming language. See Sect. 12.4.1 .
In advanced computer science classes, it is relevant to mention that in computer science, in addition to the development of problem-solving strategies, special emphasis is placed also on non-solvable problems (see Sect. 12.4.3 ).
Role of Variables in Python: http://www.cs.joensuu.fi/~saja/var_roles/stud_vers/stud_Python_eng.html
Roles of Variables with examples in Scratch: https://www.sisd.net/cms/lib/TX01001452/Centricity/domain/433/cse/1.1.5%20RolesOfVariables_UsedActivity1.2.4.pptx
The Roles of Variables home page ( http://saja.kapsi.fi/var_roles/ ) is a rich resource and contains different kinds of educational resources.
See http://www.cs.joensuu.fi/~saja/var_roles/role_intro.html
See http://www.cs.joensuu.fi/~saja/var_roles/try.html
See http://cs.uef.fi/~pgerdt/RAE/
See http://www.cs.joensuu.fi/~saja/var_roles/why_roles.html
See http://www.cs.joensuu.fi/~saja/var_roles/teaching.html
See http://saja.kapsi.fi/var_roles/literature.html
Ahrendt W, Bubel R, Hahnle R (2009) Integrated and tool-supported teaching of testing, debugging, and verification. In: Gibbons J, Oliveira JN (eds) Proceedings of the 2nd international conference on teaching formal methods (TFM ’09). Springer, Berlin/Heidelberg, pp 125–143
Google Scholar
Alqadi BS, Maletic JI (2017) An empirical study of debugging patterns among novices programmers. In: Proceedings of the 2017 ACM SIGCSE technical symposium on computer science education (SIGCSE ’17). ACM, New York, pp 15–20
Arshad N (2009) Teaching programming and problem solving to CS2 students using think-alouds. SIGCSE Bull 41(1):372–376
Astrachan O, Berry G, Cox L, Mitchener G (1998) Design patterns: an essential component of CS curricula. In: Proceeding of SIGCSE, pp 153–160
Batory D, Sarvela JN, Rauschmayer A (2004) Scaling stepwise refinement. IEEE Trans Softw Eng 30(6):355–371
Bauer A, Popović Z (2017) Collaborative problem solving in an open-ended scientific discovery game. In: Proceedings of the ACM Human-Computer Interaction 1, CSCW, Article 22 (December 2017)
Ben-Ari M, Sajaniemi J (2003) Roles of variables from the perspective of computer science educators. University of Joensuu, Department of Computer Science, Technical Report, Series A-2003–6
Börstler J, Hilburn TB (2016) Team projects in computing education. ACM Trans Comput Educ 16(2), Article 4 (March 2016)
Byckling P, Sajaniemi J (2006) Roles of variables and programming skills improvement. SIGCSE Bull 38(1):413–417
Carver S, McCoy (1988) Learning and transfer of debugging skills: applying task analysis to curriculum design and assessment. In Mayer RE (ed) Teaching and learning computer programming, multiple research perspectives. Lawrence Erlbaum Associates, Inc., Chapter 11
Celepkolu M, Boyer KE (2018) The importance of producing shared code through pair programming. In: Proceedings of the 49th ACM technical symposium on computer science education (SIGCSE ’18). ACM, New York, pp 765–770
Clancy MJ, Linn M C (1999) Patterns and pedagogy. In: Proceedings of the SIGCSE’99, pp 37–42
Cosmides L, Tooby J (1997) Evolutionary psychology: a primer. Retrieved 24 October 2004, from http://www.psych.ucsb.edu/research/cep/primer.html
Dijkstra EW (1976) A discipline of programming. Prentice-Hall, Englewood Cliffs
MATH Google Scholar
East JP, Thomas SR, Wallingford E, Beck W, Drake J (1996) Pattern-based programming instruction. In: Proceedings of ASEE annual conference and exposition, Washington, DC
Ginat D (2003) The greedy trap and learning from mistakes. SIGCSE Bull 35(1):11–15
Ginat D (2004) Algorithmic patterns and the case of the sliding delta. SIGCSE Bull 36(2):29–33
Ginat D (2008) Learning from wrong and creative algorithm design. SIGCSE Bull 40(1):26–30
Ginat D (2009) Interleaved pattern composition and scaffolded learning. In: Proceedings of the 14th Annual ACM SIGCSE Conference on Innovation and Technology in Computer Science Education – ITiCSE ‘09, Paris, France, pp 109–113
Ginat D, Shmalo R (2013) Constructive use of errors in teaching CS1. In: Proceedings of the 44th ACM technical symposium on Computer science education (SIGCSE ’13). ACM, New York, pp 353–358
Hasni TF, Lodhi F (2011) Teaching problem solving effectively. ACM Inroads 2(3):58–62
Hazzan O, Leron U (2006) Why do we resist testing? Syst Des Front Exclus Front Cover Syst Des 3(7):20–23
Johnson DW, Johanson RT (2017) Cooperative learning. Retrieved from: https://2017.congresoinnovacion.educa.aragon.es/documents/48/David_Johnson.pdf
Jonassen DH (2000) Toward a design theory of problem solving. Educ Technol Res Dev 48(4):63–85
Kiesmüller U (2009) Diagnosing learners’ problem-solving strategies using learning environments with algorithmic problems in secondary education. Trans Comput Educ 9(3), Article 17 (September 2009), 26 pages
Laakso MJ, Malmi L, Korhonen A, Rajala T, Kaila E, Salakoski T (2008) Using roles of variables to enhance novice’s debugging work. Issues Informing Sci Inf Technol 5:281–295
Lapidot T, Hazzan O (2005) Song debugging: merging content and pedagogy in computer science education. Inroads SIGCSE Bull 37(4):79–83
Lieberman H (1997) The debugging scandal and what to do about it (special section). Comm ACM 40(4):27–29
Lishinski A, Yadav A, Enbody R, Good J (2016) The influence of problem solving abilities on Students’ performance on different assessment tasks in CS1. In: Proceedings of the 47th ACM technical symposium on computing science education (SIGCSE ’16). ACM, New York, pp 329–334
Muller O (2005) Pattern oriented instruction and the enhancement of analogical reasoning. In: Proceedings of the first International Workshop on Computer Education Research ICER ‘05, Seattle, WA, USA, pp 57–67
Muller O, Haberman B, Averbuch H (2004) (An almost) pedagogical pattern for pattern-based problem solving instruction. In: Proceedings of the 9th Annual SIGCSE Conference on Innovation and Technology in Computer Science. Education, pp 102–106
Muller O, Ginat D, Haberman B (2007) Pattern-oriented instruction and its influence on problem decomposition and solution construction. ACM SIGCSE Bull 39(3):151–155
Murphy L, Lewandowski G, McCauley R, Simon B, Thomas L, Zander C (2008) Debugging: the good, the bad, and the quirky – a qualitative analysis of novices’ strategies. SIGCSE Bull 40(1):163–167
Nagvajara P, Taskin B (2007) Design-for-debug: a vital aspect in education. In: Proceedings of the 2007 IEEE international conference on microelectronic systems education (MSE ’07). IEEE Computer Society, Washington, DC, USA, pp 65–66
Papert S (1980) Mindstorms: children, computers and powerful ideas. Basic Books Inc, New York
Polya G (1957) How to solve it. Doubleday and Co., Inc, Garden City
Polya G (1981) Mathematical discovery on understanding learning and teaching problem solving. Wiley, New York
Popper KR (1992/1959) Logic of scientific discovery. Harper and Row, New York
Proulx VK (2000) Programming patterns and design patterns in the introductory computer science course. Proc SIGCSE 32(1):80–84
Ragonis N (2012) Integrating the teaching of algorithmic patterns into computer science teacher preparation programs. In: Proceedings of the 17th ACM annual conference on Innovation and technology in computer science education (ITiCSE ’12). ACM, New York, pp 339–344
Raman K, Svore KM, Gilad-Bachrach R, Burges CJC (2012) Learning from mistakes: towards a correctable learning algorithm. In: Proceedings of the 21st ACM international conference on information and knowledge management (CIKM ’12). ACM, New York, pp 1930–1934
Reed D (1999) Incorporating problem-solving patterns in CS1. J Comput Sci Edu 13(1):6–13
Reynolds RG, Maletic JI, Porvin SE (1992) Stepwise refinement and problem solving. IEEE Softw 9(5):79–88
Robins A, Rountree J, Rountree N (2003) Learning and teaching programming: a review and discussion. Comput Sci Edu 13(2):137–172
Sajaniemi J (2005) Roles of variables and learning to program. In: Jimoyiannis A (ed) Proceedings of the 3rd Panhellenic conference didactics of informatics, University of Peloponnese, Korinthos, Greece. http://cs.joensuu.fi/~saja/var_roles/abstracts/didinf05.pdf . Accessed 3 July 2010
Santos AL, Sousa J (2017) An exploratory study of how programming instructors illustrate variables and control flow. In: Proceedings of the 17th Koli calling international conference on computing education research (Koli Calling ’17). ACM, New York, pp 173–177
Schoenfeld AH (1983) Episodes and executive decisions in mathematical problem-solving. In: Lesh R, Landaue M (eds) Acquisition of mathematics concepts and processes. Academic Press Inc, New York
Schön DA (1983) The reflective practitioner. BasicBooks
Seta K, Kajino T, Umano M, Ikeda M (2006) An ontology based reflection support system to encourage learning from mistakes. In: Deved V (ed) Proceedings of the 24th IASTED international conference on artificial intelligence and applications (AIA’06). ACTA Press, Anaheim, pp 142–149
Soloway E (1986) Learning to program = learning to construct mechanisms and explanations. CACM 29(1):850–858
Spohrer JG, Soloway E (1986) Analyzing the high frequency bugs in novice programs. In: Soloway E, Iyengar S (eds) Empirical studies of programmers. Ablex, Norwood, pp 230–251
Stoeffler K, Rosen Y, von Davier A (2017) Exploring the measurement of collaborative problem solving using a human-agent educational game. In: Proceedings of the seventh international learning analytics & knowledge conference (LAK ‘17). ACM, New York, pp 570–571
Vainio V, Sajaniemi J (2007) Factors in novice programmers’ poor tracing skills. SIGCSE Bull 39(3):236–240
Vasconcelos J (2007) Basic strategy for algorithmic problem solving. http://www.cs.jhu.edu/~jorgev/cs106/ProblemSolving.html . Accessed 2 June 2010
Vírseda R d V, Orna EP, Berbis E, Guerrero S d L (2011) A logic teaching tool based on tableaux for verification and debugging of algorithms. In: Blackburn P, van Ditmarsch H, Soler-Toscano F, Manzano M (eds) Proceedings of the third international congress conference on tools for teaching logic (TICTTL’11). Springer, Berlin/Heidelberg, pp 239–248
Von Davier AA, Halpin PF (2013) Collaborative problem solving and the assessment of cognitive skills: psychometric considerations. ETS Res Rep Ser 2013(2):1–36
Wallingford E (1996) Toward a first course based on object-oriented patterns. In: Proceedings of the SIGCSE, pp 27–31
Wirth N (1971) Program development by stepwise refinement. CACM 14(4):221–227. http://sunnyday.mit.edu/16.355/wirth-refinement.html . Accessed 13 Nov 2010
Download references
Author information
Authors and affiliations.
Department of Education in Science & Technology, Technion–Israel Institute of Technology, Haifa, Israel
Orit Hazzan & Tami Lapidot
Faculty of Education, Beit Berl College, Doar Beit Berl, Israel
Noa Ragonis
You can also search for this author in PubMed Google Scholar
Rights and permissions
Reprints and permissions
Copyright information
© 2020 Springer Nature Switzerland AG
About this chapter
Hazzan, O., Ragonis, N., Lapidot, T. (2020). Problem-Solving Strategies. In: Guide to Teaching Computer Science. Springer, Cham. https://doi.org/10.1007/978-3-030-39360-1_8
Download citation
DOI : https://doi.org/10.1007/978-3-030-39360-1_8
Published : 06 August 2020
Publisher Name : Springer, Cham
Print ISBN : 978-3-030-39359-5
Online ISBN : 978-3-030-39360-1
eBook Packages : Computer Science Computer Science (R0)
Share this chapter
Anyone you share the following link with will be able to read this content:
Sorry, a shareable link is not currently available for this article.
Provided by the Springer Nature SharedIt content-sharing initiative
- Publish with us
Policies and ethics
- Find a journal
- Track your research
Exploring the Problem Solving Cycle in Computer Science – Strategies, Techniques, and Tools
- Post author By bicycle-u
- Post date 08.12.2023
The world of computer science is built on the foundation of problem solving. Whether it’s finding a solution to a complex algorithm or analyzing data to make informed decisions, the problem solving cycle is at the core of every computer science endeavor.
At its essence, problem solving in computer science involves breaking down a complex problem into smaller, more manageable parts. This allows for a systematic approach to finding a solution by analyzing each part individually. The process typically starts with gathering and understanding the data or information related to the problem at hand.
Once the data is collected, computer scientists use various techniques and algorithms to analyze and explore possible solutions. This involves evaluating different approaches and considering factors such as efficiency, accuracy, and scalability. During this analysis phase, it is crucial to think critically and creatively to come up with innovative solutions.
After a thorough analysis, the next step in the problem solving cycle is designing and implementing a solution. This involves creating a detailed plan of action, selecting the appropriate tools and technologies, and writing the necessary code to bring the solution to life. Attention to detail and precision are key in this stage to ensure that the solution functions as intended.
The final step in the problem solving cycle is evaluating the solution and its effectiveness. This includes testing the solution against different scenarios and data sets to ensure its reliability and performance. If any issues or limitations are discovered, adjustments and optimizations are made to improve the solution.
In conclusion, the problem solving cycle is a fundamental process in computer science, involving analysis, data exploration, algorithm development, solution implementation, and evaluation. It is through this cycle that computer scientists are able to tackle complex problems and create innovative solutions that drive progress in the field of computer science.
Understanding the Importance
In computer science, problem solving is a crucial skill that is at the core of the problem solving cycle. The problem solving cycle is a systematic approach to analyzing and solving problems, involving various stages such as problem identification, analysis, algorithm design, implementation, and evaluation. Understanding the importance of this cycle is essential for any computer scientist or programmer.
Data Analysis and Algorithm Design
The first step in the problem solving cycle is problem identification, which involves recognizing and defining the issue at hand. Once the problem is identified, the next crucial step is data analysis. This involves gathering and examining relevant data to gain insights and understand the problem better. Data analysis helps in identifying patterns, trends, and potential solutions.
After data analysis, the next step is algorithm design. An algorithm is a step-by-step procedure or set of rules to solve a problem. Designing an efficient algorithm is crucial as it determines the effectiveness and efficiency of the solution. A well-designed algorithm takes into consideration the constraints, resources, and desired outcomes while implementing the solution.
Implementation and Evaluation
Once the algorithm is designed, the next step in the problem solving cycle is implementation. This involves translating the algorithm into a computer program using a programming language. The implementation phase requires coding skills and expertise in a specific programming language.
After implementation, the solution needs to be evaluated to ensure that it solves the problem effectively. Evaluation involves testing the program and verifying its correctness and efficiency. This step is critical to identify any errors or issues and to make necessary improvements or adjustments.
In conclusion, understanding the importance of the problem solving cycle in computer science is essential for any computer scientist or programmer. It provides a systematic and structured approach to analyze and solve problems, ensuring efficient and effective solutions. By following the problem solving cycle, computer scientists can develop robust algorithms, implement them in efficient programs, and evaluate their solutions to ensure their correctness and efficiency.
Identifying the Problem
In the problem solving cycle in computer science, the first step is to identify the problem that needs to be solved. This step is crucial because without a clear understanding of the problem, it is impossible to find a solution.
Identification of the problem involves a thorough analysis of the given data and understanding the goals of the task at hand. It requires careful examination of the problem statement and any constraints or limitations that may affect the solution.
During the identification phase, the problem is broken down into smaller, more manageable parts. This can involve breaking the problem down into sub-problems or identifying the different aspects or components that need to be addressed.
Identifying the problem also involves considering the resources and tools available for solving it. This may include considering the specific tools and programming languages that are best suited for the problem at hand.
By properly identifying the problem, computer scientists can ensure that they are focused on the right goals and are better equipped to find an effective and efficient solution. It sets the stage for the rest of the problem solving cycle, including the analysis, design, implementation, and evaluation phases.
Gathering the Necessary Data
Before finding a solution to a computer science problem, it is essential to gather the necessary data. Whether it’s writing a program or developing an algorithm, data serves as the backbone of any solution. Without proper data collection and analysis, the problem-solving process can become inefficient and ineffective.
The Importance of Data
In computer science, data is crucial for a variety of reasons. First and foremost, it provides the information needed to understand and define the problem at hand. By analyzing the available data, developers and programmers can gain insights into the nature of the problem and determine the most efficient approach for solving it.
Additionally, data allows for the evaluation of potential solutions. By collecting and organizing relevant data, it becomes possible to compare different algorithms or strategies and select the most suitable one. Data also helps in tracking progress and measuring the effectiveness of the chosen solution.
Data Gathering Process
The process of gathering data involves several steps. Firstly, it is necessary to identify the type of data needed for the particular problem. This may include numerical values, textual information, or other types of data. It is important to determine the sources of data and assess their reliability.
Once the required data has been identified, it needs to be collected. This can be done through various methods, such as surveys, experiments, observations, or by accessing existing data sets. The collected data should be properly organized, ensuring its accuracy and validity.
Data cleaning and preprocessing are vital steps in the data gathering process. This involves removing any irrelevant or erroneous data and transforming it into a suitable format for analysis. Properly cleaned and preprocessed data will help in generating reliable and meaningful insights.
Data Analysis and Interpretation
After gathering and preprocessing the data, the next step is data analysis and interpretation. This involves applying various statistical and analytical methods to uncover patterns, trends, and relationships within the data. By analyzing the data, programmers can gain valuable insights that can inform the development of an effective solution.
During the data analysis process, it is crucial to remain objective and unbiased. The analysis should be based on sound reasoning and logical thinking. It is also important to communicate the findings effectively, using visualizations or summaries to convey the information to stakeholders or fellow developers.
In conclusion, gathering the necessary data is a fundamental step in solving computer science problems. It provides the foundation for understanding the problem, evaluating potential solutions, and tracking progress. By following a systematic and rigorous approach to data gathering and analysis, developers can ensure that their solutions are efficient, effective, and well-informed.
Analyzing the Data
Once you have collected the necessary data, the next step in the problem-solving cycle is to analyze it. Data analysis is a crucial component of computer science, as it helps us understand the problem at hand and develop effective solutions.
To analyze the data, you need to break it down into manageable pieces and examine each piece closely. This process involves identifying patterns, trends, and outliers that may be present in the data. By doing so, you can gain insights into the problem and make informed decisions about the best course of action.
There are several techniques and tools available for data analysis in computer science. Some common methods include statistical analysis, data visualization, and machine learning algorithms. Each approach has its own strengths and limitations, so it’s essential to choose the most appropriate method for the problem you are solving.
Statistical Analysis
Statistical analysis involves using mathematical models and techniques to analyze data. It helps in identifying correlations, distributions, and other statistical properties of the data. By applying statistical tests, you can determine the significance and validity of your findings.
Data Visualization
Data visualization is the process of presenting data in a visual format, such as charts, graphs, or maps. It allows for a better understanding of complex data sets and facilitates the communication of findings. Through data visualization, patterns and trends can become more apparent, making it easier to derive meaningful insights.
Machine Learning Algorithms
Machine learning algorithms are powerful tools for analyzing large and complex data sets. These algorithms can automatically detect patterns and relationships in the data, leading to the development of predictive models and solutions. By training the algorithm on a labeled dataset, it can learn from the data and make accurate predictions or classifications.
In conclusion, analyzing the data is a critical step in the problem-solving cycle in computer science. It helps us gain a deeper understanding of the problem and develop effective solutions. Whether through statistical analysis, data visualization, or machine learning algorithms, data analysis plays a vital role in transforming raw data into actionable insights.
Exploring Possible Solutions
Once you have gathered data and completed the analysis, the next step in the problem-solving cycle is to explore possible solutions. This is where the true power of computer science comes into play. With the use of algorithms and the application of scientific principles, computer scientists can develop innovative solutions to complex problems.
During this stage, it is important to consider a variety of potential solutions. This involves brainstorming different ideas and considering their feasibility and potential effectiveness. It may be helpful to consult with colleagues or experts in the field to gather additional insights and perspectives.
Developing an Algorithm
One key aspect of exploring possible solutions is the development of an algorithm. An algorithm is a step-by-step set of instructions that outlines a specific process or procedure. In the context of problem solving in computer science, an algorithm provides a clear roadmap for implementing a solution.
The development of an algorithm requires careful thought and consideration. It is important to break down the problem into smaller, manageable steps and clearly define the inputs and outputs of each step. This allows for the creation of a logical and efficient solution.
Evaluating the Solutions
Once you have developed potential solutions and corresponding algorithms, the next step is to evaluate them. This involves analyzing each solution to determine its strengths, weaknesses, and potential impact. Consider factors such as efficiency, scalability, and resource requirements.
It may be helpful to conduct experiments or simulations to further assess the effectiveness of each solution. This can provide valuable insights and data to support the decision-making process.
Ultimately, the goal of exploring possible solutions is to find the most effective and efficient solution to the problem at hand. By leveraging the power of data, analysis, algorithms, and scientific principles, computer scientists can develop innovative solutions that drive progress and solve complex problems in the world of technology.
Evaluating the Options
Once you have identified potential solutions and algorithms for a problem, the next step in the problem-solving cycle in computer science is to evaluate the options. This evaluation process involves analyzing the potential solutions and algorithms based on various criteria to determine the best course of action.
Consider the Problem
Before evaluating the options, it is important to take a step back and consider the problem at hand. Understand the requirements, constraints, and desired outcomes of the problem. This analysis will help guide the evaluation process.
Analyze the Options
Next, it is crucial to analyze each solution or algorithm option individually. Look at factors such as efficiency, accuracy, ease of implementation, and scalability. Consider whether the solution or algorithm meets the specific requirements of the problem, and if it can be applied to related problems in the future.
Additionally, evaluate the potential risks and drawbacks associated with each option. Consider factors such as cost, time, and resources required for implementation. Assess any potential limitations or trade-offs that may impact the overall effectiveness of the solution or algorithm.
Select the Best Option
Based on the analysis, select the best option that aligns with the specific problem-solving goals. This may involve prioritizing certain criteria or making compromises based on the limitations identified during the evaluation process.
Remember that the best option may not always be the most technically complex or advanced solution. Consider the practicality and feasibility of implementation, as well as the potential impact on the overall system or project.
In conclusion, evaluating the options is a critical step in the problem-solving cycle in computer science. By carefully analyzing the potential solutions and algorithms, considering the problem requirements, and considering the limitations and trade-offs, you can select the best option to solve the problem at hand.
Making a Decision
Decision-making is a critical component in the problem-solving process in computer science. Once you have analyzed the problem, identified the relevant data, and generated a potential solution, it is important to evaluate your options and choose the best course of action.
Consider All Factors
When making a decision, it is important to consider all relevant factors. This includes evaluating the potential benefits and drawbacks of each option, as well as understanding any constraints or limitations that may impact your choice.
In computer science, this may involve analyzing the efficiency of different algorithms or considering the scalability of a proposed solution. It is important to take into account both the short-term and long-term impacts of your decision.
Weigh the Options
Once you have considered all the factors, it is important to weigh the options and determine the best approach. This may involve assigning weights or priorities to different factors based on their importance.
Using techniques such as decision matrices or cost-benefit analysis can help you systematically compare and evaluate different options. By quantifying and assessing the potential risks and rewards, you can make a more informed decision.
Remember: Decision-making in computer science is not purely subjective or based on personal preference. It is crucial to use analytical and logical thinking to select the most optimal solution.
In conclusion, making a decision is a crucial step in the problem-solving process in computer science. By considering all relevant factors and weighing the options using logical analysis, you can choose the best possible solution to a given problem.
Implementing the Solution
Once the problem has been analyzed and a solution has been proposed, the next step in the problem-solving cycle in computer science is implementing the solution. This involves turning the proposed solution into an actual computer program or algorithm that can solve the problem.
In order to implement the solution, computer science professionals need to have a strong understanding of various programming languages and data structures. They need to be able to write code that can manipulate and process data in order to solve the problem at hand.
During the implementation phase, the proposed solution is translated into a series of steps or instructions that a computer can understand and execute. This involves breaking down the problem into smaller sub-problems and designing algorithms to solve each sub-problem.
Computer scientists also need to consider the efficiency of their solution during the implementation phase. They need to ensure that the algorithm they design is able to handle large amounts of data and solve the problem in a reasonable amount of time. This often requires optimization techniques and careful consideration of the data structures used.
Once the code has been written and the algorithm has been implemented, it is important to test and debug the solution. This involves running test cases and checking the output to ensure that the program is working correctly. If any errors or bugs are found, they need to be fixed before the solution can be considered complete.
In conclusion, implementing the solution is a crucial step in the problem-solving cycle in computer science. It requires strong programming skills and a deep understanding of algorithms and data structures. By carefully designing and implementing the solution, computer scientists can solve problems efficiently and effectively.
Testing and Debugging
In computer science, testing and debugging are critical steps in the problem-solving cycle. Testing helps ensure that a program or algorithm is functioning correctly, while debugging analyzes and resolves any issues or bugs that may arise.
Testing involves running a program with specific input data to evaluate its output. This process helps verify that the program produces the expected results and handles different scenarios correctly. It is important to test both the normal and edge cases to ensure the program’s reliability.
Debugging is the process of identifying and fixing errors or bugs in a program. When a program does not produce the expected results or crashes, it is necessary to go through the code to find and fix the problem. This can involve analyzing the program’s logic, checking for syntax errors, and using debugging tools to trace the flow of data and identify the source of the issue.
Data analysis plays a crucial role in both testing and debugging. It helps to identify patterns, anomalies, or inconsistencies in the program’s behavior. By analyzing the data, developers can gain insights into potential issues and make informed decisions on how to improve the program’s performance.
In conclusion, testing and debugging are integral parts of the problem-solving cycle in computer science. Through testing and data analysis, developers can verify the correctness of their programs and identify and resolve any issues that may arise. This ensures that the algorithms and programs developed in computer science are robust, reliable, and efficient.
Iterating for Improvement
In computer science, problem solving often involves iterating through multiple cycles of analysis, solution development, and evaluation. This iterative process allows for continuous improvement in finding the most effective solution to a given problem.
The problem solving cycle starts with problem analysis, where the specific problem is identified and its requirements are understood. This step involves examining the problem from various angles and gathering all relevant information.
Once the problem is properly understood, the next step is to develop an algorithm or a step-by-step plan to solve the problem. This algorithm is a set of instructions that, when followed correctly, will lead to the solution.
After the algorithm is developed, it is implemented in a computer program. This step involves translating the algorithm into a programming language that a computer can understand and execute.
Once the program is implemented, it is then tested and evaluated to ensure that it produces the correct solution. This evaluation step is crucial in identifying any errors or inefficiencies in the program and allows for further improvement.
If any issues or problems are found during testing, the cycle iterates, starting from problem analysis again. This iterative process allows for refinement and improvement of the solution until the desired results are achieved.
Iterating for improvement is a fundamental concept in computer science problem solving. By continually analyzing, developing, and evaluating solutions, computer scientists are able to find the most optimal and efficient approaches to solving problems.
Documenting the Process
Documenting the problem-solving process in computer science is an essential step to ensure that the cycle is repeated successfully. The process involves gathering information, analyzing the problem, and designing a solution.
During the analysis phase, it is crucial to identify the specific problem at hand and break it down into smaller components. This allows for a more targeted approach to finding the solution. Additionally, analyzing the data involved in the problem can provide valuable insights and help in designing an effective solution.
Once the analysis is complete, it is important to document the findings. This documentation can take various forms, such as written reports, diagrams, or even code comments. The goal is to create a record that captures the problem, the analysis, and the proposed solution.
Documenting the process serves several purposes. Firstly, it allows for easy communication and collaboration between team members or future developers. By documenting the problem, analysis, and solution, others can easily understand the thought process behind the solution and potentially build upon it.
Secondly, documenting the process provides an opportunity for reflection and improvement. By reviewing the documentation, developers can identify areas where the problem-solving cycle can be strengthened or optimized. This continuous improvement is crucial in the field of computer science, as new challenges and technologies emerge rapidly.
In conclusion, documenting the problem-solving process is an integral part of the computer science cycle. It allows for effective communication, collaboration, and reflection on the solutions devised. By taking the time to document the process, developers can ensure a more efficient and successful problem-solving experience.
Communicating the Solution
Once the problem solving cycle is complete, it is important to effectively communicate the solution. This involves explaining the analysis, data, and steps taken to arrive at the solution.
Analyzing the Problem
During the problem solving cycle, a thorough analysis of the problem is conducted. This includes understanding the problem statement, gathering relevant data, and identifying any constraints or limitations. It is important to clearly communicate this analysis to ensure that others understand the problem at hand.
Presenting the Solution
The next step in communicating the solution is presenting the actual solution. This should include a detailed explanation of the steps taken to solve the problem, as well as any algorithms or data structures used. It is important to provide clear and concise descriptions of the solution, so that others can understand and reproduce the results.
Overall, effective communication of the solution in computer science is essential to ensure that others can understand and replicate the problem solving process. By clearly explaining the analysis, data, and steps taken, the solution can be communicated in a way that promotes understanding and collaboration within the field of computer science.
Reflecting and Learning
Reflecting and learning are crucial steps in the problem solving cycle in computer science. Once a problem has been solved, it is essential to reflect on the entire process and learn from the experience. This allows for continuous improvement and growth in the field of computer science.
During the reflecting phase, one must analyze and evaluate the problem solving process. This involves reviewing the initial problem statement, understanding the constraints and requirements, and assessing the effectiveness of the chosen algorithm and solution. It is important to consider the efficiency and accuracy of the solution, as well as any potential limitations or areas for optimization.
By reflecting on the problem solving cycle, computer scientists can gain valuable insights into their own strengths and weaknesses. They can identify areas where they excelled and areas where improvement is needed. This self-analysis helps in honing problem solving skills and becoming a better problem solver.
Learning from Mistakes
Mistakes are an integral part of the problem solving cycle, and they provide valuable learning opportunities. When a problem is not successfully solved, it is essential to analyze the reasons behind the failure and learn from them. This involves identifying errors in the algorithm or solution, understanding the underlying concepts or principles that were misunderstood, and finding alternative approaches or strategies.
Failure should not be seen as a setback, but rather as an opportunity for growth. By learning from mistakes, computer scientists can improve their problem solving abilities and expand their knowledge and understanding of computer science. It is through these failures and the subsequent learning process that new ideas and innovations are often born.
Continuous Improvement
Reflecting and learning should not be limited to individual problem solving experiences, but should be an ongoing practice. As computer science is a rapidly evolving field, it is crucial to stay updated with new technologies, algorithms, and problem solving techniques. Continuous learning and improvement contribute to staying competitive and relevant in the field.
Computer scientists can engage in continuous improvement by seeking feedback from peers, participating in research and development activities, attending conferences and workshops, and actively seeking new challenges and problem solving opportunities. This dedication to learning and improvement ensures that one’s problem solving skills remain sharp and effective.
In conclusion, reflecting and learning are integral parts of the problem solving cycle in computer science. They enable computer scientists to refine their problem solving abilities, learn from mistakes, and continuously improve their skills and knowledge. By embracing these steps, computer scientists can stay at the forefront of the ever-changing world of computer science and contribute to its advancements.
Applying Problem Solving in Real Life
In computer science, problem solving is not limited to the realm of programming and algorithms. It is a skill that can be applied to various aspects of our daily lives, helping us to solve problems efficiently and effectively. By using the problem-solving cycle and applying the principles of analysis, data, solution, algorithm, and cycle, we can tackle real-life challenges with confidence and success.
The first step in problem-solving is to analyze the problem at hand. This involves breaking it down into smaller, more manageable parts and identifying the key issues or goals. By understanding the problem thoroughly, we can gain insights into its root causes and potential solutions.
For example, let’s say you’re facing a recurring issue in your daily commute – traffic congestion. By analyzing the problem, you may discover that the main causes are a lack of alternative routes and a lack of communication between drivers. This analysis helps you identify potential solutions such as using navigation apps to find alternate routes or promoting carpooling to reduce the number of vehicles on the road.
Gathering and Analyzing Data
Once we have identified the problem, it is important to gather relevant data to support our analysis. This may involve conducting surveys, collecting statistics, or reviewing existing research. By gathering data, we can make informed decisions and prioritize potential solutions based on their impact and feasibility.
Continuing with the traffic congestion example, you may gather data on the average commute time, the number of vehicles on the road, and the impact of carpooling on congestion levels. This data can help you analyze the problem more accurately and determine the most effective solutions.
Generating and Evaluating Solutions
After analyzing the problem and gathering data, the next step is to generate potential solutions. This can be done through brainstorming, researching best practices, or seeking input from experts. It is important to consider multiple options and think outside the box to find innovative and effective solutions.
For our traffic congestion problem, potential solutions can include implementing a smart traffic management system that optimizes traffic flow or investing in public transportation to incentivize people to leave their cars at home. By evaluating each solution’s potential impact, cost, and feasibility, you can make an informed decision on the best course of action.
Implementing and Iterating
Once a solution has been chosen, it is time to implement it in real life. This may involve developing a plan, allocating resources, and executing the solution. It is important to monitor the progress and collect feedback to learn from the implementation and make necessary adjustments.
For example, if the chosen solution to address traffic congestion is implementing a smart traffic management system, you would work with engineers and transportation authorities to develop and deploy the system. Regular evaluation and iteration of the system’s performance would ensure that it is effective and making a positive impact on reducing congestion.
By applying the problem-solving cycle derived from computer science to real-life situations, we can approach challenges with a systematic and analytical mindset. This can help us make better decisions, improve our problem-solving skills, and ultimately achieve more efficient and effective solutions.
Building Problem Solving Skills
In the field of computer science, problem-solving is a fundamental skill that is crucial for success. Whether you are a computer scientist, programmer, or student, developing strong problem-solving skills will greatly benefit your work and studies. It allows you to approach challenges with a logical and systematic approach, leading to efficient and effective problem resolution.
The Problem Solving Cycle
Problem-solving in computer science involves a cyclical process known as the problem-solving cycle. This cycle consists of several stages, including problem identification, data analysis, solution development, implementation, and evaluation. By following this cycle, computer scientists are able to tackle complex problems and arrive at optimal solutions.
Importance of Data Analysis
Data analysis is a critical step in the problem-solving cycle. It involves gathering and examining relevant data to gain insights and identify patterns that can inform the development of a solution. Without proper data analysis, computer scientists may overlook important information or make unfounded assumptions, leading to subpar solutions.
To effectively analyze data, computer scientists can employ various techniques such as data visualization, statistical analysis, and machine learning algorithms. These tools enable them to extract meaningful information from large datasets and make informed decisions during the problem-solving process.
Developing Effective Solutions
Developing effective solutions requires creativity, critical thinking, and logical reasoning. Computer scientists must evaluate multiple approaches, consider various factors, and assess the feasibility of different solutions. They should also consider potential limitations and trade-offs to ensure that the chosen solution addresses the problem effectively.
Furthermore, collaboration and communication skills are vital when building problem-solving skills. Computer scientists often work in teams and need to effectively communicate their ideas, propose solutions, and address any challenges that arise during the problem-solving process. Strong interpersonal skills facilitate collaboration and enhance problem-solving outcomes.
- Mastering programming languages and algorithms
- Staying updated with technological advancements in the field
- Practicing problem solving through coding challenges and projects
- Seeking feedback and learning from mistakes
- Continuing to learn and improve problem-solving skills
By following these strategies, individuals can strengthen their problem-solving abilities and become more effective computer scientists or programmers. Problem-solving is an essential skill in computer science and plays a central role in driving innovation and advancing the field.
Questions and answers:
What is the problem solving cycle in computer science.
The problem solving cycle in computer science refers to a systematic approach that programmers use to solve problems. It involves several steps, including problem definition, algorithm design, implementation, testing, and debugging.
How important is the problem solving cycle in computer science?
The problem solving cycle is extremely important in computer science as it allows programmers to effectively tackle complex problems and develop efficient solutions. It helps in organizing the thought process and ensures that the problem is approached in a logical and systematic manner.
What are the steps involved in the problem solving cycle?
The problem solving cycle typically consists of the following steps: problem definition and analysis, algorithm design, implementation, testing, and debugging. These steps are repeated as necessary until a satisfactory solution is achieved.
Can you explain the problem definition and analysis step in the problem solving cycle?
During the problem definition and analysis step, the programmer identifies and thoroughly understands the problem that needs to be solved. This involves analyzing the requirements, constraints, and possible inputs and outputs. It is important to have a clear understanding of the problem before proceeding to the next steps.
Why is testing and debugging an important step in the problem solving cycle?
Testing and debugging are important steps in the problem solving cycle because they ensure that the implemented solution functions as intended and is free from errors. Through testing, the programmer can identify and fix any issues or bugs in the code, thereby improving the quality and reliability of the solution.
What is the problem-solving cycle in computer science?
The problem-solving cycle in computer science refers to the systematic approach that computer scientists use to solve problems. It involves various steps, including problem analysis, algorithm design, coding, testing, and debugging.
Related posts:
- The Stages of the Problem Solving Cycle in Cognitive Psychology – Understanding, Planning, Execution, Evaluation, and Reflection
- A Comprehensive Guide to the Problem Solving Cycle in Psychology – Strategies, Techniques, and Applications
- The Step-by-Step Problem Solving Cycle for Effective Solutions
- The Importance of Implementing the Problem Solving Cycle in Education to Foster Critical Thinking and Problem-Solving Skills in Students
- The Importance of the Problem Solving Cycle in Business Studies – Strategies for Success
- The Comprehensive Guide to the Problem Solving Cycle in PDF Format
- A Comprehensive Guide on the Problem Solving Cycle – Step-by-Step Approach with Real-Life Example
- The Seven Essential Steps of the Problem Solving Cycle
- Open access
- Published: 11 May 2024
Nursing students’ stressors and coping strategies during their first clinical training: a qualitative study in the United Arab Emirates
- Jacqueline Maria Dias 1 ,
- Muhammad Arsyad Subu 1 ,
- Nabeel Al-Yateem 1 ,
- Fatma Refaat Ahmed 1 ,
- Syed Azizur Rahman 1 , 2 ,
- Mini Sara Abraham 1 ,
- Sareh Mirza Forootan 1 ,
- Farzaneh Ahmad Sarkhosh 1 &
- Fatemeh Javanbakh 1
BMC Nursing volume 23 , Article number: 322 ( 2024 ) Cite this article
500 Accesses
Metrics details
Understanding the stressors and coping strategies of nursing students in their first clinical training is important for improving student performance, helping students develop a professional identity and problem-solving skills, and improving the clinical teaching aspects of the curriculum in nursing programmes. While previous research have examined nurses’ sources of stress and coping styles in the Arab region, there is limited understanding of these stressors and coping strategies of nursing students within the UAE context thereby, highlighting the novelty and significance of the study.
A qualitative study was conducted using semi-structured interviews. Overall 30 students who were undergoing their first clinical placement in Year 2 at the University of Sharjah between May and June 2022 were recruited. All interviews were recorded and transcribed verbatim and analyzed for themes.
During their first clinical training, nursing students are exposed to stress from different sources, including the clinical environment, unfriendly clinical tutors, feelings of disconnection, multiple expectations of clinical staff and patients, and gaps between the curriculum of theory classes and labatories skills and students’ clinical experiences. We extracted three main themes that described students’ stress and use of coping strategies during clinical training: (1) managing expectations; (2) theory-practice gap; and (3) learning to cope. Learning to cope, included two subthemes: positive coping strategies and negative coping strategies.
Conclusions
This qualitative study sheds light from the students viewpoint about the intricate interplay between managing expectations, theory practice gap and learning to cope. Therefore, it is imperative for nursing faculty, clinical agencies and curriculum planners to ensure maximum learning in the clinical by recognizing the significance of the stressors encountered and help students develop positive coping strategies to manage the clinical stressors encountered. Further research is required look at the perspective of clinical stressors from clinical tutors who supervise students during their first clinical practicum.
Peer Review reports
Nursing education programmes aim to provide students with high-quality clinical learning experiences to ensure that nurses can provide safe, direct care to patients [ 1 ]. The nursing baccalaureate programme at the University of Sharjah is a four year program with 137 credits. The programmes has both theoretical and clinical components withs nine clinical courses spread over the four years The first clinical practicum which forms the basis of the study takes place in year 2 semester 2.
Clinical practice experience is an indispensable component of nursing education and links what students learn in the classroom and in skills laboratories to real-life clinical settings [ 2 , 3 , 4 ]. However, a gap exists between theory and practice as the curriculum in the classroom differs from nursing students’ experiences in the clinical nursing practicum [ 5 ]. Clinical nursing training places (or practicums, as they are commonly referred to), provide students with the necessary experiences to ensure that they become proficient in the delivery of patient care [ 6 ]. The clinical practicum takes place in an environment that combines numerous structural, psychological, emotional and organizational elements that influence student learning [ 7 ] and may affect the development of professional nursing competencies, such as compassion, communication and professional identity [ 8 ]. While clinical training is a major component of nursing education curricula, stress related to clinical training is common among students [ 9 ]. Furthermore, the nursing literature indicates that the first exposure to clinical learning is one of the most stressful experiences during undergraduate studies [ 8 , 10 ]. Thus, the clinical component of nursing education is considered more stressful than the theoretical component. Students often view clinical learning, where most learning takes place, as an unsupportive environment [ 11 ]. In addition, they note strained relationships between themselves and clinical preceptors and perceive that the negative attitudes of clinical staff produce stress [ 12 ].
The effects of stress on nursing students often involve a sense of uncertainty, uneasiness, or anxiety. The literature is replete with evidence that nursing students experience a variety of stressors during their clinical practicum, beginning with the first clinical rotation. Nursing is a complex profession that requires continuous interaction with a variety of individuals in a high-stress environment. Stress during clinical learning can have multiple negative consequences, including low academic achievement, elevated levels of burnout, and diminished personal well-being [ 13 , 14 ]. In addition, both theoretical and practical research has demonstrated that increased, continual exposure to stress leads to cognitive deficits, inability to concentrate, lack of memory or recall, misinterpretation of speech, and decreased learning capacity [ 15 ]. Furthermore, stress has been identified as a cause of attrition among nursing students [ 16 ].
Most sources of stress have been categorized as academic, clinical or personal. Each person copes with stress differently [ 17 ], and utilizes deliberate, planned, and psychological efforts to manage stressful demands [ 18 ]. Coping mechanisms are commonly termed adaptation strategies or coping skills. Labrague et al. [ 19 ] noted that students used critical coping strategies to handle stress and suggested that problem solving was the most common coping or adaptation mechanism used by nursing students. Nursing students’ coping strategies affect their physical and psychological well-being and the quality of nursing care they offer. Therefore, identifying the coping strategies that students use to manage stressors is important for early intervention [ 20 ].
Studies on nursing students’ coping strategies have been conducted in various countries. For example, Israeli nursing students were found to adopt a range of coping mechanisms, including talking to friends, engaging in sports, avoiding stress and sadness/misery, and consuming alcohol [ 21 ]. Other studies have examined stress levels among medical students in the Arab region. Chaabane et al. [ 15 ], conducted a systematic review of sudies in Arab countries, including Saudi Arabia, Egypt, Jordan, Iraq, Pakistan, Oman, Palestine and Bahrain, and reported that stress during clinical practicums was prevalent, although it could not be determined whether this was limited to the initial clinical course or occurred throughout clinical training. Stressors highlighted during the clinical period in the systematic review included assignments and workload during clinical practice, a feeling that the requirements of clinical practice exceeded students’ physical and emotional endurance and that their involvement in patient care was limited due to lack of experience. Furthermore, stress can have a direct effect on clinical performance, leading to mental disorders. Tung et al. [ 22 ], reported that the prevalence of depression among nursing students in Arab countries is 28%, which is almost six times greater than the rest of the world [ 22 ]. On the other hand, Saifan et al. [ 5 ], explored the theory-practice gap in the United Arab Emirates and found that clinical stressors could be decreased by preparing students better for clinical education with qualified clinical faculty and supportive preceptors.
The purpose of this study was to identify the stressors experienced by undergraduate nursing students in the United Arab Emirates during their first clinical training and the basic adaptation approaches or coping strategies they used. Recognizing or understanding different coping processes can inform the implementation of corrective measures when students experience clinical stress. The findings of this study may provide valuable information for nursing programmes, nurse educators, and clinical administrators to establish adaptive strategies to reduce stress among students going clinical practicums, particularly stressors from their first clinical training in different healthcare settings.
A qualitative approach was adopted to understand clinical stressors and coping strategies from the perspective of nurses’ lived experience. Qualitative content analysis was employed to obtain rich and detailed information from our qualitative data. Qualitative approaches seek to understand the phenomenon under study from the perspectives of individuals with lived experience [ 23 ]. Qualitative content analysis is an interpretive technique that examines the similarities and differences between and within different areas of text while focusing on the subject [ 24 ]. It is used to examine communication patterns in a repeatable and systematic way [ 25 ] and yields rich and detailed information on the topic under investigation [ 23 ]. It is a method of systematically coding and categorizing information and comprises a process of comprehending, interpreting, and conceptualizing the key meanings from qualitative data [ 26 ].
Setting and participants
This study was conducted after the clinical rotations ended in April 2022, between May and June in the nursing programme at the College of Health Sciences, University of Sharjah, in the United Arab Emirates. The study population comprised undergraduate nursing students who were undergoing their first clinical training and were recruited using purposive sampling. The inclusion criteria for this study were second-year nursing students in the first semester of clinical training who could speak English, were willing to participate in this research, and had no previous clinical work experience. The final sample consisted of 30 students.
Research instrument
The research instrument was a semi structured interview guide. The interview questions were based on an in-depth review of related literature. An intensive search included key words in Google Scholar, PubMed like the terms “nursing clinical stressors”, “nursing students”, and “coping mechanisms”. Once the questions were created, they were validated by two other faculty members who had relevant experience in mental health. A pilot test was conducted with five students and based on their feedback the following research questions, which were addressed in the study.
How would you describe your clinical experiences during your first clinical rotations?
In what ways did you find the first clinical rotation to be stressful?
What factors hindered your clinical training?
How did you cope with the stressors you encountered in clinical training?
Which strategies helped you cope with the clinical stressors you encountered?
Data collection
Semi-structured interviews were chosen as the method for data collection. Semi structured interviews are a well-established approach for gathering data in qualitative research and allow participants to discuss their views, experiences, attitudes, and beliefs in a positive environment [ 27 ]. This approach allows for flexibility in questioning thereby ensuring that key topics related to clinical learning stressors and coping strategies would be explored. Participants were given the opportunity to express their views, experiences, attitudes, and beliefs in a positive environment, encouraging open communication. These semi structured interviews were conducted by one member of the research team (MAS) who had a mental health background, and another member of the research team who attended the interviews as an observer (JMD). Neither of these researchers were involved in teaching the students during their clinical practicum, which helped to minimize bias. The interviews took place at the University of Sharjah, specifically in building M23, providing a familiar and comfortable environment for the participant. Before the interviews were all students who agreed to participate were provided with an explanation of the study’s purpose. The time and location of each interview were arranged. Before the interviews were conducted, all students who provided consent to participate received an explanation of the purpose of the study, and the time and place of each interview were arranged to accommodate the participants’ schedules and preferences. The interviews were conducted after the clinical rotation had ended in April, and after the final grades had been submitted to the coordinator. The timings of the interviews included the month of May and June which ensured that participants have completed their practicum experience and could reflect on the stressors more comprehensively. The interviews were audio-recorded with the participants’ consent, and each interview lasted 25–40 min. The data were collected until saturation was reached for 30 students. Memos and field notes were also recorded as part of the data collection process. These additional data allowed for triangulation to improve the credibility of the interpretations of the data [ 28 ]. Memos included the interviewers’ thoughts and interpretations about the interviews, the research process (including questions and gaps), and the analytic progress used for the research. Field notes were used to record the interviewers’ observations and reflections on the data. These additional data collection methods were important to guide the researchers in the interpretation of the data on the participants’ feelings, perspectives, experiences, attitudes, and beliefs. Finally, member checking was performed to ensure conformability.
Data analysis
The study used the content analysis method proposed by Graneheim and Lundman [ 24 ]. According to Graneheim and Lundman [ 24 ], content analysis is an interpretive technique that examines the similarities and differences between distinct parts of a text. This method allows researchers to determine exact theoretical and operational definitions of words, phrases, and symbols by elucidating their constituent properties [ 29 ]. First, we read the interview transcripts several times to reach an overall understanding of the data. All verbatim transcripts were read several times and discussed among all authors. We merged and used line-by-line coding of words, sentences, and paragraphs relevant to each other in terms of both the content and context of stressors and coping mechanisms. Next, we used data reduction to assess the relationships among themes using tables and diagrams to indicate conceptual patterns. Content related to stress encountered by students was extracted from the transcripts. In a separate document, we integrated and categorized all words and sentences that were related to each other in terms of both content and context. We analyzed all codes and units of meaning and compared them for similarities and differences in the context of this study. Furthermore, the emerging findings were discussed with other members of the researcher team. The final abstractions of meaningful subthemes into themes were discussed and agreed upon by the entire research team. This process resulted in the extraction of three main themes in addition to two subthemes related to stress and coping strategies.
Ethical considerations
The University of Sharjah Research Ethics Committee provided approval to conduct this study (Reference Number: REC 19-12-03-01-S). Before each interview, the goal and study procedures were explained to each participant, and written informed consent was obtained. The participants were informed that participation in the study was voluntary and that they could withdraw from the study at any time. In the event they wanted to withdraw from the study, all information related to the participant would be removed. No participant withdrew from the study. Furthermore, they were informed that their clinical practicum grade would not be affected by their participation in this study. We chose interview locations in Building M23that were private and quiet to ensure that the participants felt at ease and confident in verbalizing their opinions. No participant was paid directly for involvement in this study. In addition, participants were assured that their data would remain anonymous and confidential. Confidentiality means that the information provided by participants was kept private with restrictions on how and when data can be shared with others. The participants were informed that their information would not be duplicated or disseminated without their permission. Anonymity refers to the act of keeping people anonymous with respect to their participation in a research endeavor. No personal identifiers were used in this study, and each participant was assigned a random alpha-numeric code (e.g., P1 for participant 1). All digitally recorded interviews were downloaded to a secure computer protected by the principal investigator with a password. The researchers were the only people with access to the interview material (recordings and transcripts). All sensitive information and materials were kept secure in the principal researcher’s office at the University of Sharjah. The data will be maintained for five years after the study is completed, after which the material will be destroyed (the transcripts will be shredded, and the tapes will be demagnetized).
In total, 30 nursing students who were enrolled in the nursing programme at the Department of Nursing, College of Health Sciences, University of Sharjah, and who were undergoing their first clinical practicum participated in the study. Demographically, 80% ( n = 24) were females and 20% ( n = 6) were male participants. The majority (83%) of study participants ranged in age from 18 to 22 years. 20% ( n = 6) were UAE nationals, 53% ( n = 16) were from Gulf Cooperation Council countries, while 20% ( n = 6) hailed from Africa and 7% ( n = 2) were of South Asian descent. 67% of the respondents lived with their families while 33% lived in the hostel. (Table 1 )
Following the content analysis, we identified three main themes: (1) managing expectations, (2) theory-practice gap and 3)learning to cope. Learning to cope had two subthemes: positive coping strategies and negative coping strategies. An account of each theme is presented along with supporting excerpts for the identified themes. The identified themes provide valuable insight into the stressors encountered by students during their first clinical practicum. These themes will lead to targeted interventions and supportive mechanisms that can be built into the clinical training curriculum to support students during clinical practice.
Theme 1: managing expectations
In our examination of the stressors experienced by nursing students during their first clinical practicum and the coping strategies they employed, we identified the first theme as managing expectations.
The students encountered expectations from various parties, such as clinical staff, patients and patients’ relatives which they had to navigate. They attempted to fulfil their expectations as they progressed through training, which presented a source of stress. The students noted that the hospital staff and patients expected them to know how to perform a variety of tasks upon request, which made the students feel stressed and out of place if they did not know how to perform these tasks. Some participants noted that other nurses in the clinical unit did not allow them to participate in nursing procedures, which was considered an enormous impediment to clinical learning, as noted in the excerpt below:
“…Sometimes the nurses… They will not allow us to do some procedures or things during clinical. And sometimes the patients themselves don’t allow us to do procedures” (P5).
Some of the students noted that they felt they did not belong and felt like foreigners in the clinical unit. Excerpts from the students are presented in the following quotes;
“The clinical environment is so stressful. I don’t feel like I belong. There is too little time to build a rapport with hospital staff or the patient” (P22).
“… you ask the hospital staff for some guidance or the location of equipment, and they tell us to ask our clinical tutor …but she is not around … what should I do? It appears like we do not belong, and the sooner the shift is over, the better” (P18).
“The staff are unfriendly and expect too much from us students… I feel like I don’t belong, or I am wasting their (the hospital staff’s) time. I want to ask questions, but they have loads to do” (P26).
Other students were concerned about potential failure when working with patients during clinical training, which impacted their confidence. They were particularly afraid of failure when performing any clinical procedures.
“At the beginning, I was afraid to do procedures. I thought that maybe the patient would be hurt and that I would not be successful in doing it. I have low self-confidence in doing procedures” (P13).
The call bell rings, and I am told to answer Room No. XXX. The patient wants help to go to the toilet, but she has two IV lines. I don’t know how to transport the patient… should I take her on the wheelchair? My eyes glance around the room for a wheelchair. I am so confused …I tell the patient I will inform the sister at the nursing station. The relative in the room glares at me angrily … “you better hurry up”…Oh, I feel like I don’t belong, as I am not able to help the patient… how will I face the same patient again?” (P12).
Another major stressor mentioned in the narratives was related to communication and interactions with patients who spoke another language, so it was difficult to communicate.
“There was a challenge with my communication with the patients. Sometimes I have communication barriers because they (the patients) are of other nationalities. I had an experience with a patient [who was] Indian, and he couldn’t speak my language. I did not understand his language” (P9).
Thus, a variety of expectations from patients, relatives, hospital staff, and preceptors acted as sources of stress for students during their clinical training.
Theme 2: theory-practice gap
Theory-practice gaps have been identified in previous studies. In our study, there was complete dissonance between theory and actual clinical practice. The clinical procedures or practices nursing students were expected to perform differed from the theory they had covered in their university classes and skills lab. This was described as a theory–practice gap and often resulted in stress and confusion.
“For example …the procedures in the hospital are different. They are different from what we learned or from theory on campus. Or… the preceptors have different techniques than what we learned on campus. So, I was stress[ed] and confused about it” (P11).
Furthermore, some students reported that they did not feel that they received adequate briefing before going to clinical training. A related source of stress was overload because of the volume of clinical coursework and assignments in addition to clinical expectations. Additionally, the students reported that a lack of time and time management were major sources of stress in their first clinical training and impacted their ability to complete the required paperwork and assignments:
“…There is not enough time…also, time management at the hospital…for example, we start at seven a.m., and the handover takes 1 hour to finish. They (the nurses at the hospital) are very slow…They start with bed making and morning care like at 9.45 a.m. Then, we must fill [out] our assessment tool and the NCP (nursing care plan) at 10 a.m. So, 15 only minutes before going to our break. We (the students) cannot manage this time. This condition makes me and my friends very stressed out. -I cannot do my paperwork or assignments; no time, right?” (P10).
“Stressful. There is a lot of work to do in clinical. My experiences are not really good with this course. We have a lot of things to do, so many assignments and clinical procedures to complete” (P16).
The participants noted that the amount of required coursework and number of assignments also presented a challenge during their first clinical training and especially affected their opportunity to learn.
“I need to read the file, know about my patient’s condition and pathophysiology and the rationale for the medications the patient is receiving…These are big stressors for my learning. I think about assignments often. Like, we are just focusing on so many assignments and papers. We need to submit assessments and care plans for clinical cases. We focus our time to complete and finish the papers rather than doing the real clinical procedures, so we lose [the] chance to learn” (P25).
Another participant commented in a similar vein that there was not enough time to perform tasks related to clinical requirements during clinical placement.
“…there is a challenge because we do not have enough time. Always no time for us to submit papers, to complete assessment tools, and some nurses, they don’t help us. I think we need more time to get more experiences and do more procedures, reduce the paperwork that we have to submit. These are challenges …” (P14).
There were expectations that the students should be able to carry out their nursing duties without becoming ill or adversely affected. In addition, many students reported that the clinical environment was completely different from the skills laboratory at the college. Exposure to the clinical setting added to the theory-practice gap, and in some instances, the students fell ill.
One student made the following comment:
“I was assisting a doctor with a dressing, and the sight and smell from the oozing wound was too much for me. I was nauseated. As soon as the dressing was done, I ran to the bathroom and threw up. I asked myself… how will I survive the next 3 years of nursing?” (P14).
Theme 3: learning to cope
The study participants indicated that they used coping mechanisms (both positive and negative) to adapt to and manage the stressors in their first clinical practicum. Important strategies that were reportedly used to cope with stress were time management, good preparation for clinical practice, and positive thinking as well as engaging in physical activity and self-motivation.
“Time management. Yes, it is important. I was encouraging myself. I used time management and prepared myself before going to the clinical site. Also, eating good food like cereal…it helps me very much in the clinic” (P28).
“Oh yeah, for sure positive thinking. In the hospital, I always think positively. Then, after coming home, I get [to] rest and think about positive things that I can do. So, I will think something good [about] these things, and then I will be relieved of stress” (P21).
Other strategies commonly reported by the participants were managing their breathing (e.g., taking deep breaths, breathing slowly), taking breaks to relax, and talking with friends about the problems they encountered.
“I prefer to take deep breaths and breathe slowly and to have a cup of coffee and to talk to my friends about the case or the clinical preceptor and what made me sad so I will feel more relaxed” (P16).
“Maybe I will take my break so I feel relaxed and feel better. After clinical training, I go directly home and take a long shower, going over the day. I will not think about anything bad that happened that day. I just try to think about good things so that I forget the stress” (P27).
“Yes, my first clinical training was not easy. It was difficult and made me stressed out…. I felt that it was a very difficult time for me. I thought about leaving nursing” (P7).
I was not able to offer my prayers. For me, this was distressing because as a Muslim, I pray regularly. Now, my prayer time is pushed to the end of the shift” (P11).
“When I feel stress, I talk to my friends about the case and what made me stressed. Then I will feel more relaxed” (P26).
Self-support or self-motivation through positive self-talk was also used by the students to cope with stress.
“Yes, it is difficult in the first clinical training. When I am stress[ed], I go to the bathroom and stand in the front of the mirror; I talk to myself, and I say, “You can do it,” “you are a great student.” I motivate myself: “You can do it”… Then, I just take breaths slowly several times. This is better than shouting or crying because it makes me tired” (P11).
Other participants used physical activity to manage their stress.
“How do I cope with my stress? Actually, when I get stressed, I will go for a walk on campus” (P4).
“At home, I will go to my room and close the door and start doing my exercises. After that, I feel the negative energy goes out, then I start to calm down… and begin my clinical assignments” (P21).
Both positive and negative coping strategies were utilized by the students. Some participants described using negative coping strategies when they encountered stress during their clinical practice. These negative coping strategies included becoming irritable and angry, eating too much food, drinking too much coffee, and smoking cigarettes.
“…Negative adaptation? Maybe coping. If I am stressed, I get so angry easily. I am irritable all day also…It is negative energy, right? Then, at home, I am also angry. After that, it is good to be alone to think about my problems” (P12).
“Yeah, if I…feel stress or depressed, I will eat a lot of food. Yeah, ineffective, like I will be eating a lot, drinking coffee. Like I said, effective, like I will prepare myself and do breathing, ineffective, I will eat a lot of snacks in between my free time. This is the bad side” (P16).
“…During the first clinical practice? Yes, it was a difficult experience for us…not only me. When stressed, during a break at the hospital, I will drink two or three cups of coffee… Also, I smoke cigarettes… A lot. I can drink six cups [of coffee] a day when I am stressed. After drinking coffee, I feel more relaxed, I finish everything (food) in the refrigerator or whatever I have in the pantry, like chocolates, chips, etc” (P23).
These supporting excerpts for each theme and the analysis offers valuable insights into the specific stressors faced by nursing students during their first clinical practicum. These insights will form the basis for the development of targeted interventions and supportive mechanisms within the clinical training curriculum to better support students’ adjustment and well-being during clinical practice.
Our study identified the stressors students encounter in their first clinical practicum and the coping strategies, both positive and negative, that they employed. Although this study emphasizes the importance of clinical training to prepare nursing students to practice as nurses, it also demonstrates the correlation between stressors and coping strategies.The content analysis of the first theme, managing expectations, paves the way for clinical agencies to realize that the students of today will be the nurses of tomorrow. It is important to provide a welcoming environment where students can develop their identities and learn effectively. Additionally, clinical staff should foster an environment of individualized learning while also assisting students in gaining confidence and competence in their repertoire of nursing skills, including critical thinking, problem solving and communication skills [ 8 , 15 , 19 , 30 ]. Another challenge encountered by the students in our study was that they were prevented from participating in clinical procedures by some nurses or patients. This finding is consistent with previous studies reporting that key challenges for students in clinical learning include a lack of clinical support and poor attitudes among clinical staff and instructors [ 31 ]. Clinical staff with positive attitudes have a positive impact on students’ learning in clinical settings [ 32 ]. The presence, supervision, and guidance of clinical instructors and the assistance of clinical staff are essential motivating components in the clinical learning process and offer positive reinforcement [ 30 , 33 , 34 ]. Conversely, an unsupportive learning environment combined with unwelcoming clinical staff and a lack of sense of belonging negatively impact students’ clinical learning [ 35 ].
The sources of stress identified in this study were consistent with common sources of stress in clinical training reported in previous studies, including the attitudes of some staff, students’ status in their clinical placement and educational factors. Nursing students’ inexperience in the clinical setting and lack of social and emotional experience also resulted in stress and psychological difficulties [ 36 ]. Bhurtun et al. [ 33 ] noted that nursing staff are a major source of stress for students because the students feel like they are constantly being watched and evaluated.
We also found that students were concerned about potential failure when working with patients during their clinical training. Their fear of failure when performing clinical procedures may be attributable to low self-confidence. Previous studies have noted that students were concerned about injuring patients, being blamed or chastised, and failing examinations [ 37 , 38 ]. This was described as feeling “powerless” in a previous study [ 7 , 12 ]. In addition, patients’ attitudes towards “rejecting” nursing students or patients’ refusal of their help were sources of stress among the students in our study and affected their self-confidence. Self-confidence and a sense of belonging are important for nurses’ personal and professional identity, and low self-confidence is a problem for nursing students in clinical learning [ 8 , 39 , 40 ]. Our findings are consistent with a previous study that reported that a lack of self-confidence was a primary source of worry and anxiety for nursing students and affected their communication and intention to leave nursing [ 41 ].
In the second theme, our study suggests that students encounter a theory-practice gap in clinical settings, which creates confusion and presents an additional stressors. Theoretical and clinical training are complementary elements of nursing education [ 40 ], and this combination enables students to gain the knowledge, skills, and attitudes necessary to provide nursing care. This is consistent with the findings of a previous study that reported that inconsistencies between theoretical knowledge and practical experience presented a primary obstacle to the learning process in the clinical context [ 42 ], causing students to lose confidence and become anxious [ 43 ]. Additionally, the second theme, the theory-practice gap, authenticates Safian et al.’s [ 5 ] study of the theory-practice gap that exists United Arab Emirates among nursing students as well as the need for more supportive clinical faculty and the extension of clinical hours. The need for better time availability and time management to complete clinical tasks were also reported by the students in the study. Students indicated that they had insufficient time to complete clinical activities because of the volume of coursework and assignments. Our findings support those of Chaabane et al. [ 15 ]. A study conducted in Saudi Arabia [ 44 ] found that assignments and workload were among the greatest sources of stress for students in clinical settings. Effective time management skills have been linked to academic achievement, stress reduction, increased creativity [ 45 ], and student satisfaction [ 46 ]. Our findings are also consistent with previous studies that reported that a common source of stress among first-year students was the increased classroom workload [ 19 , 47 ]. As clinical assignments and workloads are major stressors for nursing students, it is important to promote activities to help them manage these assignments [ 48 ].
Another major challenge reported by the participants was related to communicating and interacting with other nurses and patients. The UAE nursing workforce and population are largely expatriate and diverse and have different cultural and linguistic backgrounds. Therefore, student nurses encounter difficulty in communication [ 49 ]. This cultural diversity that students encounter in communication with patients during clinical training needs to be addressed by curriculum planners through the offering of language courses and courses on cultural diversity [ 50 ].
Regarding the third and final theme, nursing students in clinical training are unable to avoid stressors and must learn to cope with or adapt to them. Previous research has reported a link between stressors and the coping mechanisms used by nursing students [ 51 , 52 , 53 ]. In particular, the inability to manage stress influences nurses’ performance, physical and mental health, attitude, and role satisfaction [ 54 ]. One such study suggested that nursing students commonly use problem-focused (dealing with the problem), emotion-focused (regulating emotion), and dysfunctional (e.g., venting emotions) stress coping mechanisms to alleviate stress during clinical training [ 15 ]. Labrague et al. [ 51 ] highlighted that nursing students use both active and passive coping techniques to manage stress. The pattern of clinical stress has been observed in several countries worldwide. The current study found that first-year students experienced stress during their first clinical training [ 35 , 41 , 55 ]. The stressors they encountered impacted their overall health and disrupted their clinical learning. Chaabane et al. [ 15 ] reported moderate and high stress levels among nursing students in Bahrain, Egypt, Iraq, Jordan, Oman, Pakistan, Palestine, Saudi Arabia, and Sudan. Another study from Bahrain reported that all nursing students experienced moderate to severe stress in their first clinical placement [ 56 ]. Similarly, nursing students in Spain experienced a moderate level of stress, and this stress was significantly correlated with anxiety [ 30 ]. Therefore, it is imperative that pastoral systems at the university address students’ stress and mental health so that it does not affect their clinical performance. Faculty need to utilize evidence-based interventions to support students so that anxiety-producing situations and attrition are minimized.
In our study, students reported a variety of positive and negative coping mechanisms and strategies they used when they experienced stress during their clinical practice. Positive coping strategies included time management, positive thinking, self-support/motivation, breathing, taking breaks, talking with friends, and physical activity. These findings are consistent with those of a previous study in which healthy coping mechanisms used by students included effective time management, social support, positive reappraisal, and participation in leisure activities [ 57 ]. Our study found that relaxing and talking with friends were stress management strategies commonly used by students. Communication with friends to cope with stress may be considered social support. A previous study also reported that people seek social support to cope with stress [ 58 ]. Some students in our study used physical activity to cope with stress, consistent with the findings of previous research. Stretching exercises can be used to counteract the poor posture and positioning associated with stress and to assist in reducing physical tension. Promoting such exercise among nursing students may assist them in coping with stress in their clinical training [ 59 ].
Our study also showed that when students felt stressed, some adopted negative coping strategies, such as showing anger/irritability, engaging in unhealthy eating habits (e.g., consumption of too much food or coffee), or smoking cigarettes. Previous studies have reported that high levels of perceived stress affect eating habits [ 60 ] and are linked to poor diet quality, increased snacking, and low fruit intake [ 61 ]. Stress in clinical settings has also been linked to sleep problems, substance misuse, and high-risk behaviors’ and plays a major role in student’s decision to continue in their programme.
Implications of the study
The implications of the study results can be grouped at multiple levels including; clinical, educational, and organizational level. A comprehensive approach to addressing the stressors encountered by nursing students during their clinical practicum can be overcome by offering some practical strategies to address the stressors faced by nursing students during their clinical practicum. By integrating study findings into curriculum planning, mentorship programs, and organizational support structures, a supportive and nurturing environment that enhances students’ learning, resilience, and overall success can be envisioned.
Clinical level
Introducing simulation in the skills lab with standardized patients and the use of moulage to demonstrate wounds, ostomies, and purulent dressings enhances students’ practical skills and prepares them for real-world clinical scenarios. Organizing orientation days at clinical facilities helps familiarize students with the clinical environment, identify potential stressors, and introduce interventions to enhance professionalism, social skills, and coping abilities Furthermore, creating a WhatsApp group facilitates communication and collaboration among hospital staff, clinical tutors, nursing faculty, and students, enabling immediate support and problem-solving for clinical situations as they arise, Moreover, involving chief nursing officers of clinical facilities in the Nursing Advisory Group at the Department of Nursing promotes collaboration between academia and clinical practice, ensuring alignment between educational objectives and the needs of the clinical setting [ 62 ].
Educational level
Sharing study findings at conferences (we presented the results of this study at Sigma Theta Tau International in July 2023 in Abu Dhabi, UAE) and journal clubs disseminates knowledge and best practices among educators and clinicians, promoting awareness and implementation of measures to improve students’ learning experiences. Additionally we hold mentorship training sessions annually in January and so we shared with the clinical mentors and preceptors the findings of this study so that they proactively they are equipped with strategies to support students’ coping with stressors during clinical placements.
Organizational level
At the organizational we relooked at the available student support structures, including counseling, faculty advising, and career advice, throughout the nursing program emphasizing the importance of holistic support for students’ well-being and academic success as well as retention in the nursing program. Also, offering language courses as electives recognizes the value of communication skills in nursing practice and provides opportunities for personal and professional development.
For first-year nursing students, clinical stressors are inevitable and must be given proper attention. Recognizing nursing students’ perspectives on the challenges and stressors experienced in clinical training is the first step in overcoming these challenges. In nursing schools, providing an optimal clinical environment as well as increasing supervision and evaluation of students’ practices should be emphasized. Our findings demonstrate that first-year nursing students are exposed to a variety of different stressors. Identifying the stressors, pressures, and obstacles that first-year students encounter in the clinical setting can assist nursing educators in resolving these issues and can contribute to students’ professional development and survival to allow them to remain in the profession. To overcome stressors, students frequently employ problem-solving approaches or coping mechanisms. The majority of nursing students report stress at different levels and use a variety of positive and negative coping techniques to manage stress.
The present results may not be generalizable to other nursing institutions because this study used a purposive sample along with a qualitative approach and was limited to one university in the Middle East. Furthermore, the students self-reported their stress and its causes, which may have introduced reporting bias. The students may also have over or underreported stress or coping mechanisms because of fear of repercussions or personal reasons, even though the confidentiality of their data was ensured. Further studies are needed to evaluate student stressors and coping now that measures have been introduced to support students. Time will tell if these strategies are being used effectively by both students and clinical personnel or if they need to be readdressed. Finally, we need to explore the perceptions of clinical faculty towards supervising students in their first clinical practicum so that clinical stressors can be handled effectively.
Data availability
The data sets are available with the corresponding author upon reasonable request.
Almarwani AM. The effect of integrating a nursing licensure examination preparation course into a nursing program curriculum: a quasi-experimental study. Saudi J Health Sci. 2022;11:184–9.
Article Google Scholar
Horntvedt MT, Nordsteien A, Fermann T, Severinsson E. Strategies for teaching evidence-based practice in nursing education: a thematic literature review. BMC Med Educ. 2018;18:172.
Article PubMed PubMed Central Google Scholar
Larsson M, Sundler AJ, Blomberg K, Bisholt B. The clinical learning environment during clinical practice in postgraduate district nursing students’ education: a cross-sectional study. Nurs Open. 2023;10:879–88.
Article PubMed Google Scholar
Sellberg M, Palmgren PJ, Möller R. A cross-sectional study of clinical learning environments across four undergraduate programs using the undergraduate clinical education environment measure. BMC Med Educ. 2021;21:258.
Saifan A, Devadas B, Mekkawi M, Amoor H, Matizha P, James J, et al. Managing the theory-practice gap in nursing education and practice: hearing the voices of nursing students in the United Arab Emirates. J Nurs Manag. 2021;29:1869–79.
Flott EA, Linden L. The clinical learning environment in nursing education: a concept analysis. J Adv Nurs. 2016;72:501–13.
Kalyani MN, Jamshidi N, Molazem Z, Torabizadeh C, Sharif F. How do nursing students experience the clinical learning environment and respond to their experiences? A qualitative study. BMJ Open. 2019;9:e028052.
Mahasneh D, Shoqirat N, Alsaraireh A, Singh C, Thorpe L. From learning on mannequins to practicing on patients: nursing students’ first-time experience of clinical placement in Jordan. SAGE Open Nurs. 2021;7:23779608211004298.
PubMed PubMed Central Google Scholar
Stubin C. Clinical stress among undergraduate nursing students: perceptions of clinical nursing faculty. Int J Nurs Educ Scholarsh. 2020;17:20190111.
Ahmed WAM. Anxiety and related symptoms among critical care nurses in Albaha, Kingdom of Saudi Arabia. AIMS Med Sci. 2015;2:303–9.
Alhassan. Duke Phillips. 2024.
Ekstedt M, Lindblad M, Löfmark A. Nursing students’ perception of the clinical learning environment and supervision in relation to two different supervision models - a comparative cross-sectional study. BMC Nurs. 2019;18:49.
Bradshaw C, Murphy Tighe S, Doody O. Midwifery students’ experiences of their clinical internship: a qualitative descriptive study. Nurse Educ Today. 2018;68:213–7.
McCarthy B, Trace A, O’Donovan M, O’Regan P, Brady-Nevin C, O’Shea M, et al. Coping with stressful events: a pre-post-test of a psycho-educational intervention for undergraduate nursing and midwifery students. Nurse Educ Today. 2018;61:273–80.
Chaabane S, Chaabna K, Bhagat S, Abraham A, Doraiswamy S, Mamtani R, et al. Perceived stress, stressors, and coping strategies among nursing students in the Middle East and North Africa: an overview of systematic reviews. Syst Rev. 2021;10:136.
Pines EW, Rauschhuber ML, Norgan GH, Cook JD, Canchola L, Richardson C, et al. Stress resiliency, psychological empowerment and conflict management styles among baccalaureate nursing students. J Adv Nurs. 2012;68:1482–93.
Lazarus RS. Coping theory and research: past, present, and future. Psychosom Med. 1993;55:234–47.
Article CAS PubMed Google Scholar
Boyd MA. Essentials of psychiatric nursing. Philadelphia, PA: Wolters Kluwer; 2017.
Google Scholar
Labrague LJ, McEnroe-Petitte DM, Gloe D, Thomas L, Papathanasiou IV, Tsaras K. A literature review on stress and coping strategies in nursing students. J Ment Health. 2017;26:471–80.
Ni C, Lo D, Liu X, Ma J, Xu S, Li L. Chinese female nursing students’ coping strategies, self-esteem and related factors in different years of school. J Nurs Educ Pract. 2012;2:33–41.
Jan LK, Popescu L. Israel’s nursing students’ stress sources and coping strategies during their first clinical experience in hospital wards-a qualitative research. Soc Work Rev / Rev Asistenta Soc. 2014;13:163–88.
Tung YJ, Lo KKH, Ho RCM, Tam WSW. Prevalence of depression among nursing students: a systematic review and meta-analysis. Nurse Educ Today. 2018;63:119–29.
Speziale HS, Streubert HJ, Carpenter DR. Qualitative research in nursing: advancing the humanistic imperative. Philadelphia, PA: Lippincott Williams & Wilkins; 2011.
Graneheim UH, Lundman B. Qualitative content analysis in nursing research: concepts, procedures and measures to achieve trustworthiness. Nurse Educ Today. 2004;24:105–12.
Bryman A. Integrating quantitative and qualitative research: how is it done? Qual Res. 2006;6:97–113.
Holloway I, Wheeler S. Qualitative research in nursing and healthcare. New York, NY: Wiley; 2013.
Richards L, Morse J. A user’s guide to qualitative methods. London, UK: Sage; 2007.
Lincoln Y, Guba EG. The SAGE handbook of qualitative research. Newbury Park, CA: SAGE Publications Inc; 2017.
Park S, Park KS. Family stigma: a concept analysis. Asian Nurs Res. 2014;8:165–71.
Onieva-Zafra MD, Fernández-Muñoz JJ, Fernández-Martínez E, García-Sánchez FJ, Abreu-Sánchez A, Parra-Fernández ML. Anxiety, perceived stress and coping strategies in nursing students: a cross-sectional, correlational, descriptive study. BMC Med Educ. 2020;20:370.
Albloushi M, Ferguson L, Stamler L, Bassendowski S, Hellsten L, Kent-Wilkinson A. Saudi female nursing students experiences of sense of belonging in the clinical settings: a qualitative study. Nurse Educ Pract. 2019;35:69–74.
Arkan B, Ordin Y, Yılmaz D. Undergraduate nursing students’ experience related to their clinical learning environment and factors affecting to their clinical learning process. Nurse Educ Pract. 2018;29:127–32.
Bhurtun HD, Azimirad M, Saaranen T, Turunen H. Stress and coping among nursing students during clinical training: an integrative review. J Nurs Educ. 2019;58:266–72.
Jamshidi N, Molazem Z, Sharif F, Torabizadeh C, Kalyani MN. The challenges of nursing students in the clinical learning environment: a qualitative study. ScientificWorldJournal. 2016;2016:1846178.
Porter SL. First year nursing students’ perceptions of stress and resilience during their initial clinical placement and the introduction of a stress management app: a mixed methods approach. A thesis submitted in partial fulfilment of the requirements of Edinburgh Napier University, for the award of Doctor of Philosophy. 2019. https://www.napier.ac.uk/~/media/worktribe/output-2086663/first-year-nursing-students-perceptions-of-stress-and-resilience-during-their-initial.pdf
Panda S, Dash M, John J, Rath K, Debata A, Swain D, et al. Challenges faced by student nurses and midwives in clinical learning environment - A systematic review and meta-synthesis. Nurse Educ Today. 2021;101:104875.
Ahmadi G, Shahriari M, Keyvanara M, Kohan S. Midwifery students’ experiences of learning clinical skills in Iran: a qualitative study. Int J Med Educ. 2018;9:64–71.
Harrison-White K, Owens J. Nurse link lecturers’ perceptions of the challenges facing student nurses in clinical learning environments: a qualitative study. Nurse Educ Pract. 2018;32:78–83.
Grobecker PA. A sense of belonging and perceived stress among baccalaureate nursing students in clinical placements. Nurse Educ Today. 2016;36:178–83.
Msiska G, Kamanga M, Chilemba E, Msosa A, Munkhondya TE. Sources of stress among undergraduate nursing students during clinical practice: a Malawian perspective. Open J Nurs. 2019;9:1.
Joolaee S, Amiri SRJ, Farahani MA, Varaei S. Iranian nursing students’ preparedness for clinical training: a qualitative study. Nurse Educ Today. 2015;35:e13–7.
Günay U, Kılınç G. The transfer of theoretical knowledge to clinical practice by nursing students and the difficulties they experience: a qualitative study. Nurse Educ Today. 2018;65:81–6.
Farzi S, Shahriari M, Farzi S. Exploring the challenges of clinical education in nursing and strategies to improve it: a qualitative study. J Educ Health Promot. 2018;7:115.
Hamaideh SH, Al-Omari H, Al-Modallal H. Nursing students’ perceived stress and coping behaviors in clinical training in Saudi Arabia. J Ment Health. 2017;26:197–203.
Yaghoobi A, Mohagheghi H, Zade MY, Ganji K, Olfatii N. The effect of time management training on test anxiety and academic achievement motivation among high school students. J Sch Psychol. 2014;3:131–44.
Kebriaei A, Bidgoli MS, Saeedi A. Relationship between use of time management skills and satisfaction with spending time among students of Zahedan University of Medical Sciences. J Med Educ Dev. 2014;6:79–88.
Chen YW, Hung CH. Predictors of Taiwanese baccalaureate nursing students’ physio-psycho-social responses during clinical practicum. Nurse Educ Today. 2014;34:73–7.
Ab Latif R, Mat Nor MZ. Stressors and coping strategies during clinical practice among diploma nursing students. Malays J Med Sci. 2019;26:88–98.
Al-Yateem N, Almarzouqi A, Dias JM, Saifan A, Timmins F. Nursing in the United Arab Emirates: current challenges and opportunities. J Nurs Manag. 2021;29:109–12.
Baraz-Pordanjani S, Memarian R, Vanaki Z. Damaged professional identity as a barrier to Iranian nursing students’ clinical learning: a qualitative study. J Clin Nurs Midwifery. 2014;3:1–15.
Labrague LJ, McEnroe-Petitte DM, Papathanasiou IV, Edet OB, Tsaras K, Leocadio MC, et al. Stress and coping strategies among nursing students: an international study. J Ment Health. 2018;27:402–8.
Madian AAEM, Abdelaziz MM, Ahmed HAE. Level of stress and coping strategies among nursing students at Damanhour University, Egypt. Am J Nurs Res. 2019;7:684–96.
Wu CS, Rong JR, Huang MZ. Factors associated with perceived stress of clinical practice among associate degree nursing students in Taiwan. BMC Nurs. 2021;20:89.
Zhao FF, Lei XL, He W, Gu YH, Li DW. The study of perceived stress, coping strategy and self-efficacy of Chinese undergraduate nursing students in clinical practice. Int J Nurs Pract. 2015;21:401–9.
Bektaş H, Terkes N, Özer Z. Stress and ways of coping among first year nursing students: a Turkish perspective. J Hum Sci. 2018;15:319–30.
John B, Al-Sawad M. Perceived stress in clinical areas and emotional intelligence among baccalaureate nursing students. J Indian Acad Appl Psychol. 2015;41:76–85.
Mapfumo JS, Chitsiko N, Chireshe R. Teaching practice generated stressors and coping mechanisms among student teachers in Zimbabwe. S Afr J Educ. 2012;32:155–66.
Timmins F, Corroon AM, Byrne G, Mooney B. The challenge of contemporary nurse education programmes. Perceived stressors of nursing students: mental health and related lifestyle issues. J Psychiatr Ment Health Nurs. 2011;18:758–66.
Hegberg NJ, Tone EB. Physical activity and stress resilience: considering those at-risk for developing mental health problems. Ment Health Phys Act. 2015;8:1–7.
Shudifat RM, Al-Husban RY. Perceived sources of stress among first-year nursing students in Jordan. J Psychosoc Nurs Ment Health Serv. 2015;53:37–43.
El Ansari W, Adetunji H, Oskrochi R. Food and mental health: relationship between food and perceived stress and depressive symptoms among university students in the United Kingdom. Cent Eur J Public Health. 2014;22:90–7.
Dias JM, Aderibigbe SA, Abraham MS. Undergraduate nursing students’ mentoring experiences in the clinical practicum: the United Arab Emirates (UAE) perspective. J Nurs Manag. 2022;30:4304–13.
Download references
Acknowledgements
The authors are grateful to all second year nursing students who voluntarily participated in the study.
No funding was received. Not applicable.
Author information
Authors and affiliations.
Department of Nursing, College of Health Sciences, University of Sharjah, POBox, Sharjah, 272272, UAE
Jacqueline Maria Dias, Muhammad Arsyad Subu, Nabeel Al-Yateem, Fatma Refaat Ahmed, Syed Azizur Rahman, Mini Sara Abraham, Sareh Mirza Forootan, Farzaneh Ahmad Sarkhosh & Fatemeh Javanbakh
Health Care Management, College of Health Sciences, University of Sharjah, Sharjah, United Arab Emirates
Syed Azizur Rahman
You can also search for this author in PubMed Google Scholar
Contributions
JMD conceptualized the idea and designed the methodology, formal analysis, writing original draft and project supervision and mentoring. MAS prepared the methodology and conducted the qualitative interviews and analyzed the methodology and writing of original draft and project supervision. NY, FRA, SAR, MSA writing review and revising the draft. SMF, FAS, FJ worked with MAS on the formal analysis and prepared the first draft.All authors reviewed the final manuscipt of the article.
Corresponding author
Correspondence to Jacqueline Maria Dias .
Ethics declarations
Ethics approval and consent to participate.
The Research Ethics Committee (REC) under) the Office of the Vice Chancellor for Research and Graduate Studies UOS approved this study (REC 19-12-03-01-S). Additionally, a written consent was obtained from all participants and the process followed the recommended policies and guidelines of the Declaration of Helsinki.
Consent for publication
Not applicable.
Competing interests
Dr Fatma Refaat Ahmed is an editorial board member in BMC Nursing. Other authors do not have any conflict of interest
Additional information
Publisher’s note.
Springer Nature remains neutral with regard to jurisdictional claims in published maps and institutional affiliations.
Electronic supplementary material
Below is the link to the electronic supplementary material.
Supplementary Material 1
Rights and permissions.
Open Access This article is licensed under a Creative Commons Attribution 4.0 International License, which permits use, sharing, adaptation, distribution and reproduction in any medium or format, as long as you give appropriate credit to the original author(s) and the source, provide a link to the Creative Commons licence, and indicate if changes were made. The images or other third party material in this article are included in the article’s Creative Commons licence, unless indicated otherwise in a credit line to the material. If material is not included in the article’s Creative Commons licence and your intended use is not permitted by statutory regulation or exceeds the permitted use, you will need to obtain permission directly from the copyright holder. To view a copy of this licence, visit http://creativecommons.org/licenses/by/4.0/ . The Creative Commons Public Domain Dedication waiver ( http://creativecommons.org/publicdomain/zero/1.0/ ) applies to the data made available in this article, unless otherwise stated in a credit line to the data.
Reprints and permissions
About this article
Cite this article.
Dias, J.M., Subu, M.A., Al-Yateem, N. et al. Nursing students’ stressors and coping strategies during their first clinical training: a qualitative study in the United Arab Emirates. BMC Nurs 23 , 322 (2024). https://doi.org/10.1186/s12912-024-01962-5
Download citation
Received : 06 January 2024
Accepted : 22 April 2024
Published : 11 May 2024
DOI : https://doi.org/10.1186/s12912-024-01962-5
Share this article
Anyone you share the following link with will be able to read this content:
Sorry, a shareable link is not currently available for this article.
Provided by the Springer Nature SharedIt content-sharing initiative
- Clinical practicums
- Coping strategies
- Nursing students
BMC Nursing
ISSN: 1472-6955
- General enquiries: [email protected]
Facility for Rare Isotope Beams
At michigan state university, international research team uses wavefunction matching to solve quantum many-body problems, new approach makes calculations with realistic interactions possible.
FRIB researchers are part of an international research team solving challenging computational problems in quantum physics using a new method called wavefunction matching. The new approach has applications to fields such as nuclear physics, where it is enabling theoretical calculations of atomic nuclei that were previously not possible. The details are published in Nature (“Wavefunction matching for solving quantum many-body problems”) .
Ab initio methods and their computational challenges
An ab initio method describes a complex system by starting from a description of its elementary components and their interactions. For the case of nuclear physics, the elementary components are protons and neutrons. Some key questions that ab initio calculations can help address are the binding energies and properties of atomic nuclei not yet observed and linking nuclear structure to the underlying interactions among protons and neutrons.
Yet, some ab initio methods struggle to produce reliable calculations for systems with complex interactions. One such method is quantum Monte Carlo simulations. In quantum Monte Carlo simulations, quantities are computed using random or stochastic processes. While quantum Monte Carlo simulations can be efficient and powerful, they have a significant weakness: the sign problem. The sign problem develops when positive and negative weight contributions cancel each other out. This cancellation results in inaccurate final predictions. It is often the case that quantum Monte Carlo simulations can be performed for an approximate or simplified interaction, but the corresponding simulations for realistic interactions produce severe sign problems and are therefore not possible.
Using ‘plastic surgery’ to make calculations possible
The new wavefunction-matching approach is designed to solve such computational problems. The research team—from Gaziantep Islam Science and Technology University in Turkey; University of Bonn, Ruhr University Bochum, and Forschungszentrum Jülich in Germany; Institute for Basic Science in South Korea; South China Normal University, Sun Yat-Sen University, and Graduate School of China Academy of Engineering Physics in China; Tbilisi State University in Georgia; CEA Paris-Saclay and Université Paris-Saclay in France; and Mississippi State University and the Facility for Rare Isotope Beams (FRIB) at Michigan State University (MSU)—includes Dean Lee , professor of physics at FRIB and in MSU’s Department of Physics and Astronomy and head of the Theoretical Nuclear Science department at FRIB, and Yuan-Zhuo Ma , postdoctoral research associate at FRIB.
“We are often faced with the situation that we can perform calculations using a simple approximate interaction, but realistic high-fidelity interactions cause severe computational problems,” said Lee. “Wavefunction matching solves this problem by doing plastic surgery. It removes the short-distance part of the high-fidelity interaction, and replaces it with the short-distance part of an easily computable interaction.”
This transformation is done in a way that preserves all of the important properties of the original realistic interaction. Since the new wavefunctions look similar to that of the easily computable interaction, researchers can now perform calculations using the easily computable interaction and apply a standard procedure for handling small corrections called perturbation theory. A team effort
The research team applied this new method to lattice quantum Monte Carlo simulations for light nuclei, medium-mass nuclei, neutron matter, and nuclear matter. Using precise ab initio calculations, the results closely matched real-world data on nuclear properties such as size, structure, and binding energies. Calculations that were once impossible due to the sign problem can now be performed using wavefunction matching.
“It is a fantastic project and an excellent opportunity to work with the brightest nuclear scientist s in FRIB and around the globe,” said Ma. “As a theorist , I'm also very excited about programming and conducting research on the world's most powerful exascale supercomputers, such as Frontier , which allows us to implement wavefunction matching to explore the mysteries of nuclear physics.”
While the research team focused solely on quantum Monte Carlo simulations, wavefunction matching should be useful for many different ab initio approaches, including both classical and quantum computing calculations. The researchers at FRIB worked with collaborators at institutions in China, France, Germany, South Korea, Turkey, and United States.
“The work is the culmination of effort over many years to handle the computational problems associated with realistic high-fidelity nuclear interactions,” said Lee. “It is very satisfying to see that the computational problems are cleanly resolved with this new approach. We are grateful to all of the collaboration members who contributed to this project, in particular, the lead author, Serdar Elhatisari.”
This material is based upon work supported by the U.S. Department of Energy, the U.S. National Science Foundation, the German Research Foundation, the National Natural Science Foundation of China, the Chinese Academy of Sciences President’s International Fellowship Initiative, Volkswagen Stiftung, the European Research Council, the Scientific and Technological Research Council of Turkey, the National Natural Science Foundation of China, the National Security Academic Fund, the Rare Isotope Science Project of the Institute for Basic Science, the National Research Foundation of Korea, the Institute for Basic Science, and the Espace de Structure et de réactions Nucléaires Théorique.
Michigan State University operates the Facility for Rare Isotope Beams (FRIB) as a user facility for the U.S. Department of Energy Office of Science (DOE-SC), supporting the mission of the DOE-SC Office of Nuclear Physics. Hosting what is designed to be the most powerful heavy-ion accelerator, FRIB enables scientists to make discoveries about the properties of rare isotopes in order to better understand the physics of nuclei, nuclear astrophysics, fundamental interactions, and applications for society, including in medicine, homeland security, and industry.
The U.S. Department of Energy Office of Science is the single largest supporter of basic research in the physical sciences in the United States and is working to address some of today’s most pressing challenges. For more information, visit energy.gov/science.

IMAGES
VIDEO
COMMENTS
Problem Solving in STEM. Solving problems is a key component of many science, math, and engineering classes. If a goal of a class is for students to emerge with the ability to solve new kinds of problems or to use new problem-solving techniques, then students need numerous opportunities to develop the skills necessary to approach and answer ...
ABSTRACT. Background: Recent developments in STEM and computer science education put a strong emphasis on twenty-first-century skills, such as solving authentic problems. These skills typically transcend single disciplines. Thus, problem-solving must be seen as a multidisciplinary challenge, and the corresponding practices and processes need to be described using an integrated framework.
Two problem-solving strategies emerge from the data: one emphasizes mental activities related to framing, and the other emphasizes mental activities related to implementation. In the second study, we use a mixed factorial experimental design and mouse-tracking analysis to uncover the causal mechanism that explains the emergence of these two ...
Balancing disciplinary knowledge and practical reasoning in problem solving is needed for meaningful learning. In STEM problem solving, science subject matter with associated practices often appears distant to learners due to its abstract nature. Consequently, learners experience difficulties making meaningful connections between science and their daily experiences. Applying Dewey's idea of ...
Engaging learners in the excitement of science, helping them discover the value of evidence-based reasoning and higher-order cognitive skills, and teaching them to become creative problem solvers have long been goals of science education reformers. But the means to achieve these goals, especially methods to promote creative thinking in scientific problem solving, have not become widely known ...
The traditional teaching of science problem solving involves a considerable amount of drill and practice. Research suggests that these practices do not lead to the development of expert-like problem-solving strategies and that there is little correlation between the number of problems solved (exceeding 1,000 problems in one specific study) and the development of a conceptual understanding.
Problem-solving skills are essential in our daily lives. The video explains different problem-solving methods, including trial and error, algorithm strategy, and heuristics. It also discusses concepts like means-end analysis, working backwards, fixation, and insight. These techniques help us tackle both well-defined and ill-defined problems ...
Science educators with advanced training in curriculum and instruction and science, technology, engineering and math (STEM) learning have a number of inquiry-based strategies at their disposal. They can inspire students to think about real-world problems like scientists do, with the following examples: Solving a water-shortage problem
The problem-solving strategies used in these projects were described briefly with references to the project documents or websites. ... Teaching science problem solving: An overview of experimental work. Journal of Research in Science Teaching, 38(4), 442-468. Article Google Scholar von Hippel, E., & von Kroch, G. (2016). Identifying viable ...
Teaching and learning problem solving in science. Part I: A general strategy. C. T. C. W. Mettes , A. Pilot , H. J. Roossink , and ; H. Kramers-Pals ; ... Three problem-solving instructional strategies and their effect on Nigerian students' attainment in chemistry. Research in Education 1998, 60 (1) ...
The ability to solve real-world problems and to transfer problem-solving strategies from domain-specific to domain-general contexts and vice versa has been regarded an important competence students should develop during their education in school (Greiff et al. []; van Merriënboer []).In the context of large-scale assessments such as the PISA study problem solving competence is defined as the ...
In insight problem-solving, the cognitive processes that help you solve a problem happen outside your conscious awareness. 4. Working backward. Working backward is a problem-solving approach often ...
Problem-solving strategies can live and die on whether people are onboard. Getting some quick wins is a great way of getting people behind the process. ... The science of adult learning tells us that, in order to learn new skills (which, unsurprisingly, is harder for adults to do than kids) grown-ups need to first get into a specific headspace ...
Problem-solving is a vital skill for coping with various challenges in life. This webpage explains the different strategies and obstacles that can affect how you solve problems, and offers tips on how to improve your problem-solving skills. Learn how to identify, analyze, and overcome problems with Verywell Mind.
The purpose of this research was to investigate students’ problem solving strategies (Posamentier & Krulik, 1998) in a problem solving- mathematics classroom, using an open approach as a teaching approach and composed of four phases: 1) posing open-ended problems, 2) students’ self learning, 3) whole class discussion, and 4 ...
This activity initiates the problem-solving process, in which students need to use several cognitive and metacognitive strategies in the shared problem-solving space. Moreover, the students' peer learning strategy was enhanced if they were more willing to study in environments where it was easier to concentrate or find references related to ...
Problem-solving is a mental process that involves discovering, analyzing, and solving problems. The ultimate goal of problem-solving is to overcome obstacles and find a solution that best resolves the issue. The best strategy for solving a problem depends largely on the unique situation. In some cases, people are better off learning everything ...
In military science, problem solving is linked to the concept of "end-states", the conditions or situations which are the aims of the strategy. ... Problem-solving strategies. Problem-solving strategies are steps to overcoming the obstacles to achieving a goal. The iteration of such strategies over the course of solving a problem is the ...
6. Solution implementation. This is what we were waiting for! All problem solving strategies have the end goal of implementing a solution and solving a problem in mind. Remember that in order for any solution to be successful, you need to help your group through all of the previous problem solving steps thoughtfully.
In this episode of the McKinsey Podcast, Simon London speaks with Charles Conn, CEO of venture-capital firm Oxford Sciences Innovation, and McKinsey senior partner Hugo Sarrazin about the complexities of different problem-solving strategies.. Podcast transcript. Simon London: Hello, and welcome to this episode of the McKinsey Podcast, with me, Simon London.
Step 1: Understanding the problem. We are given in the problem that there are 25 chickens and cows. All together there are 76 feet. Chickens have 2 feet and cows have 4 feet. We are trying to determine how many cows and how many chickens Mr. Jones has on his farm. Step 2: Devise a plan.
Learn problem-solving ideas from computer science included core concepts of computational thinking. ... task includes a complex task in which students divide drawing tasks into discrete actions based on their decomposition strategies. Pattern recognition, the second component, is recognizing similarities, repetition, or the unique ...
Abstract. Problem-solving is generally considered as one of the most important and challenging cognitive activities in everyday as well as in any professional contexts. Specifically, it is one of the central activities performed by computer scientists as well as by computer science learners. However, it is not a uniform or linear process that ...
Goals and everyday problem solving: examining the link between age-related goals and problem-solving strategy use.. PubMed. Hoppmann, Christiane A; Coats, Abby Heckman; Blanchard-Fields, Fredda. 2008-07-01. Qualitative interviews on family and financial problems from 332 adolescents, young, middle-aged, and older adults, demonstrated that developmentally relevant goals predicted problem ...
The problem solving cycle is a systematic approach to analyzing and solving problems, involving various stages such as problem identification, analysis, algorithm design, implementation, and evaluation. Understanding the importance of this cycle is essential for any computer scientist or programmer.
Master problem-solving in data science with these six essential strategies, tailored for statistical experts eager to drive innovation through insightful analysis.
General strategies for solving the multi-armed bandit problem. While the objective of the multi-armed bandit problem is always the same — get as much good stuff (whatever it is; money, fish, efficiencies etc.) as possible — the strategy to meet that objective can vary widely depending circumstance.
Nurses and healthcare professionals often face complex challenges that require innovative problem-solving strategies. With the aim of enhancing evidence-based patient care and operational efficiency, the need for a streamlined approach to healthcare project planning and implementation is more critical than ever. Enter the INQUIRE model, a ...
Understanding the stressors and coping strategies of nursing students in their first clinical training is important for improving student performance, helping students develop a professional identity and problem-solving skills, and improving the clinical teaching aspects of the curriculum in nursing programmes. While previous research have examined nurses' sources of stress and coping styles ...
New approach makes calculations with realistic interactions possibleFRIB researchers are part of an international research team solving challenging computational problems in quantum physics using a new method called wavefunction matching. The new approach has applications to fields such as nuclear physics, where it is enabling theoretical calculations of atomic nuclei that were previously not ...