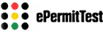
- ePermitTest.com
- Drivers education
- Distracted Driving
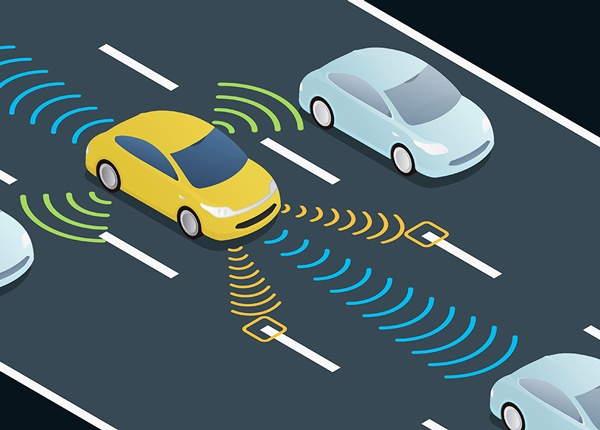

Visual Search Patterns for Safe Driving: Proactive Scanning
When it comes to collecting visual information while driving, there is a right way and a wrong way to go about it. Knowing where to look and how long for can be confusing for new drivers, particularly when there is so much to keep track of inside your car, right in front of the vehicle and 20 seconds ahead of you on the roadway. To drive safely, you need to adopt a systematic and efficient method of visually scanning your environment .
Immediate range
Secondary range, target area range, proactive scanning.
Never allow yourself to stay focused on one point on the roadway while driving. Sure, you need to monitor the situation on the road directly in front of your vehicle, but you also need to monitor the gauges inside your car and the situation on the roadway some distance ahead. Organizing a visual search of the roadway is easier and more effective when we sort the things we need to monitor into ranges and switch our attention between these points at regular intervals.
Everything in the area 4 to 6 seconds ahead of you, including the car’s dashboard, falls into your immediate range . The rear of the car in front of you should mark the far end of your immediate range when you are driving at a safe following distance . All the visual information you receive with this range will tell you how to set your speed and position the vehicle within the lane . Therefore, by the time a point at which you need to stop, turn or merge has entered your immediate range, you should already have started the necessary maneuver.
Beyond your immediate range is the secondary range , which covers everything from the end of the immediate range up to 12 to 15 seconds ahead of your vehicle. Any visual information gathered from your secondary range will help you make decisions about your speed and lane position. At this distance, you may prepare to execute a maneuver, respond to an upcoming situation and communicate with oncoming traffic .
The farthest range you must scan is your target area range (also known as the visual lead area ) which covers the area about 20 to 30 seconds ahead of your vehicle. When directing your attention to the target area range , you should look for all key visual information relating to potential hazards and changes in the roadway environment that may require action as the target area enters your secondary range. You should aim to select a target area as far ahead as possible while maintaining a clear line of sight. This will give you the maximum amount of time to absorb and react to visual information.
While driving, you must always keep your eyes moving between these three ranges so that no important information is neglected. The vehicle immediately in front of you may obstruct your view to some extent, though you can maximize your view of the secondary and target area ranges by maintaining a safe following distance and positioning your vehicle appropriately within the lane. Allow a greater following distance when traveling behind very large vehicles , as they may completely obscure your line of sight and hide other smaller vehicles.
In addition to alternating between the immediate, secondary and target area ranges, you must glance at your mirrors every three to five seconds and visually check the space to the sides of your vehicle. Remember that in doing so, you must not take your attention away from the roadway for more than about half a second at a time.
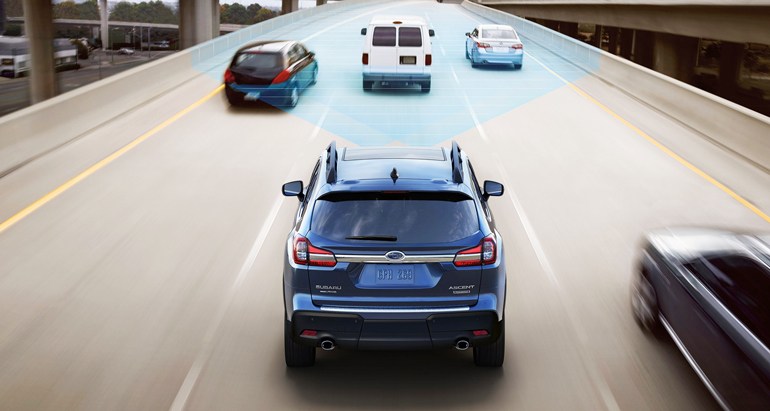
Would you pass a driving test today?
Find out with our free quiz!
Like the article? Give us 5 points!
Click a star to add your vote
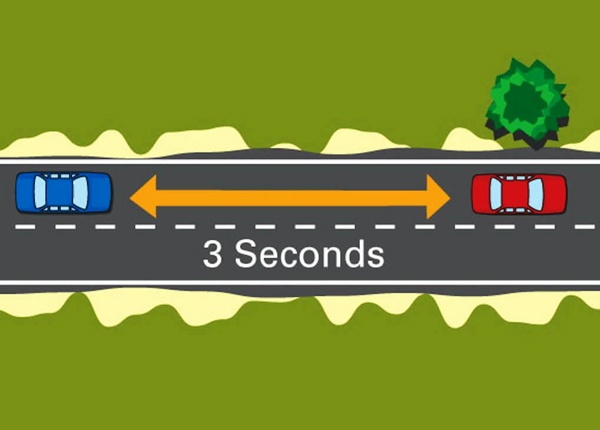
Safe Following Distance
It is impossible to drive safely and attentively without leaving enough space between your vehicle and the car ahead of you. Maintaining an adequate following distance is crucial to maximize your view of the roadway up ahead.
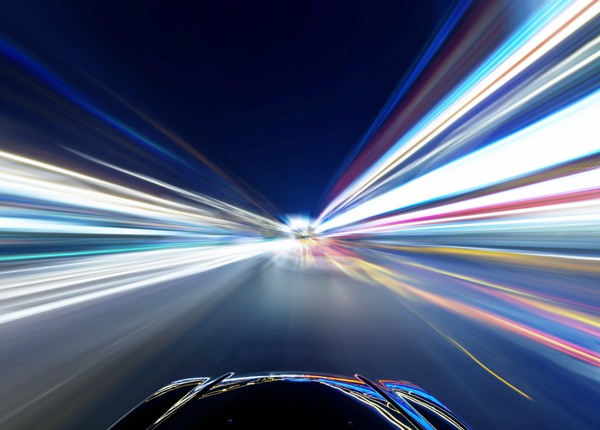
The Effects of Speed
Keeping speed to a minimum is one of the best risk-reducing tactics you can employ as an attentive driver. As the speed you are traveling at increases, so too does the danger you are exposed to and the challenges you face.
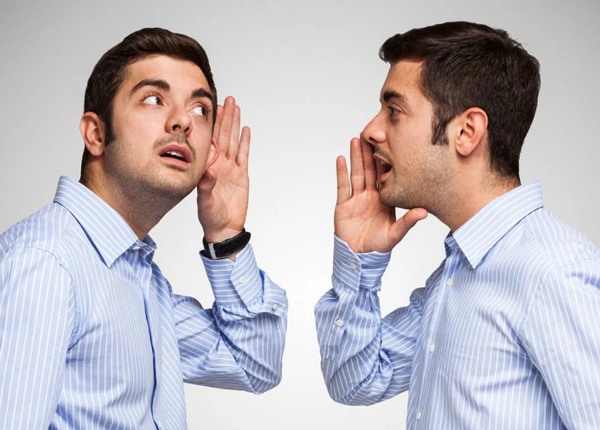
Interacting With Other Drivers
Without effective communication between motorists, it would be impossible to predict the movement of other vehicles and negotiate the roadway safely. Attentive, conscientious motorists think about how their actions will affect other drivers and endeavor to behave considerately, at all times. It is not simply a matter of being polite, it is a matter of safety.
The Importance of Paying Attention
Your ability to fully and consistently focus your attention on the environment around your vehicle is every bit as important as your road rule knowledge and vehicle control skills. Paying attention while driving is an important skill which must not be overlooked while you’re learning to drive.
The Importance of Good Vision
No sense is more important to a driver than vision. As your eyes are responsible for 90% of the information you receive while driving, good vision is essential in making safe and appropriate driving decisions.
Vision Impairments
People with less than 20/40 vision do not qualify for an unrestricted driver’s license in most states. However, there are vast numbers of people with poorer than 20/40 vision who can drive safely and legally under a restricted license, providing they wear corrective glasses or contact lenses. Only in extreme cases of vision impairment or blindness will a person be refused a driving license altogether.
Mental Skills for Driving
The “vision, memory and understanding” trinity allows you to assess and make decisions based on all the information your eyes receive while you’re driving. If you do not receive accurate visual information due to a vision impairment, or do not have relevant memory information stored in your brain to help you make sense of what you have seen, you may not respond to roadway hazards appropriately.
Proprioception and Kinesthesia
While most of the information we receive while driving is visual, our other senses are important too. In addition to sight, our brains collect information about the world around us via hearing, smell, taste and touch.
Visual Targeting
Visual targeting is the practice of focusing your attention on a stationary object which is 12 to 20 seconds ahead of your vehicle. As you move closer to your visual target, you should then select a new fixed object within that 12 to 20-second window, repeating this process continually as you move along the roadway.

- Select State
- Connecticut
- Massachusetts
- Mississippi
- New Hampshire
- North Carolina
- North Dakota
- Pennsylvania
- Rhode Island
- South Carolina
- South Dakota
- Washington D.C.
- West Virginia
- In-Car Driving Lessons
- Traffic School
Practice Permit Test
- DMV Information
Visual Search Strategies
Welcome to our quick & easy driving information guide.
Helpful Driving Information
- Start Your Drivers Ed Course Today
- Signs, Signals, and Markings
- Being Fit to Drive
- Driving Techniques
- City, Rural, and Freeway Driving
- Sharing the Road with Others
- Car Information
- Auto Central
- Useful Driving Terms
Online Drivers Ed
Courses available for all skill levels. Select your state to get started.
Get ready for the permit test with DriversEd.com
Safe driving depends on your ability to notice many things at once. Our eyes provide two types of visions: central vision and peripheral or side vision. Central vision allows us to make very important judgments like estimating distance and understanding details in the path ahead, whereas peripheral vision helps us detect events to the side that are important to us, even when we're not looking directly at them. Most driving mistakes are caused by bad habits in the way drivers use their eyes.
IPDE (Identify, Predict, Decide, and Execute) is an important concept in defensive driving. To know more about its principles, read carefully the following section.
In order to avoid last minute moves and spot possible traffic hazards, you should always look down the road ahead of your vehicle. Start braking early if you see any hazards or traffic ahead of you slowing down. Also check the space between your car and any vehicles in the lane next to you. It is very important to check behind you before you change lanes, slow down quickly, back up, or drive down a long or steep hill. You should also glance at your instrument panel often to ensure there are no problems with the vehicle and to verify your speed.
- Using Your Eyes Effectively
- Visual Search Categories
- Scanning The Road
Hear about tips, offers, & more.
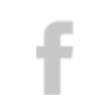
- General Questions
- Payment Info
- System Requirements
- Course Requirements
- Privacy Policy
- Terms and Conditions
- California Driving Lessons
- Georgia Driving Lessons
- Ohio Driving Lessons
- Texas Driving Lessons
- California Traffic School
- Michigan BDIC
- Texas Defensive Driving
- Texas DPS Driving Record
- More States
- Arizona Drivers Ed
- California Drivers Ed
- Colorado Drivers Ed
- Florida Drivers Ed
- Florida Permit Test
- Florida Drug & Alcohol Course
- Georgia Drivers Ed
- Idaho Drivers Ed
- Indiana Drivers Ed
- Minnesota Drivers Ed
- Nebraska Drivers Ed
- Ohio Drivers Ed
- Oklahoma Drivers Ed
- Pennsylvania Drivers Ed
- Texas Drivers Ed
- Texas Parent-Taught Drivers Ed
- Utah Drivers Ed
- Virginia Drivers Ed
- Wisconsin Drivers Ed
- Colorado 55+ Mature Drivers Ed
- Nationwide 18+ Adult Drivers Ed
- Illinois 18-21 Adult Drivers Course
- Texas 18+ Adult Drivers Course
- Become a Partner
- Facebook FanPage
- Twitter Feed
- Driving Information
- Drivers Ed App
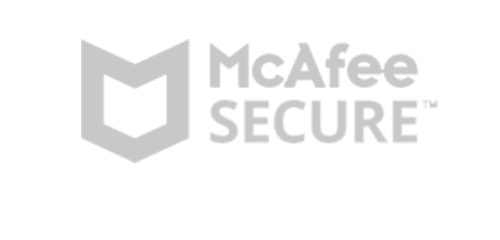
© 1997-2024 DriversEd.com. All rights reserved. Please see our privacy policy for more details.
Thank you for visiting nature.com. You are using a browser version with limited support for CSS. To obtain the best experience, we recommend you use a more up to date browser (or turn off compatibility mode in Internet Explorer). In the meantime, to ensure continued support, we are displaying the site without styles and JavaScript.
- View all journals
- Explore content
- About the journal
- Publish with us
- Sign up for alerts
- Review Article
- Published: 08 March 2017
Five factors that guide attention in visual search
- Jeremy M. Wolfe 1 &
- Todd S. Horowitz 2
Nature Human Behaviour volume 1 , Article number: 0058 ( 2017 ) Cite this article
11k Accesses
474 Citations
49 Altmetric
Metrics details
- Human behaviour
- Visual system
How do we find what we are looking for? Even when the desired target is in the current field of view, we need to search because fundamental limits on visual processing make it impossible to recognize everything at once. Searching involves directing attention to objects that might be the target. This deployment of attention is not random. It is guided to the most promising items and locations by five factors discussed here: bottom-up salience, top-down feature guidance, scene structure and meaning, the previous history of search over timescales ranging from milliseconds to years, and the relative value of the targets and distractors. Modern theories of visual search need to incorporate all five factors and specify how these factors combine to shape search behaviour. An understanding of the rules of guidance can be used to improve the accuracy and efficiency of socially important search tasks, from security screening to medical image perception.
This is a preview of subscription content, access via your institution
Access options
Access Nature and 54 other Nature Portfolio journals
Get Nature+, our best-value online-access subscription
$29.99 / 30 days
cancel any time
Subscribe to this journal
Receive 12 digital issues and online access to articles
$119.00 per year
only $9.92 per issue
Buy this article
- Purchase on Springer Link
- Instant access to full article PDF
Prices may be subject to local taxes which are calculated during checkout
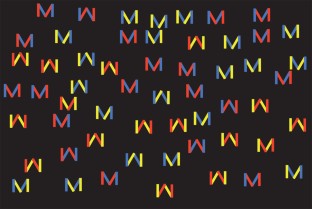
Similar content being viewed by others
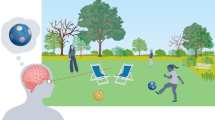
Incorporating the properties of peripheral vision into theories of visual search
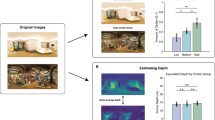
Active visual search in naturalistic environments reflects individual differences in classic visual search performance
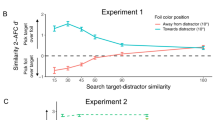
Feature-based attention warps the perception of visual features
Hyman, I. E., Boss, S. M., Wise, B. M., McKenzie, K. E. & Caggiano, J. M. Did you see the unicycling clown? Inattentional blindness while walking and talking on a cell phone. Appl. Cognitive Psych. 24 , 597–607 (2010).
Article Google Scholar
Keshvari, S. & Rosenholtz, R. Pooling of continuous features provides a unifying account of crowding. J. Vis. 16 , 39 (2016).
Article PubMed PubMed Central Google Scholar
Rosenholtz, R., Huang, J. & Ehinger, K. A. Rethinking the role of top-down attention in vision: effects attributable to a lossy representation in peripheral vision. Front. Psychol. http://dx.doi.org/10.3389/fpsyg.2012.00013 (2012).
Wolfe, J. M. What do 1,000,000 trials tell us about visual search? Psychol. Sci. 9 , 33–39 (1998).
Moran, R., Zehetleitner, M., Liesefeld, H., Müller, H. & Usher, M. Serial vs. parallel models of attention in visual search: accounting for benchmark RT-distributions. Psychon. B. Rev. 23 , 1300–1315 (2015).
Townsend, J. T. & Wenger, M. J. The serial-parallel dilemma: a case study in a linkage of theory and method. Psychon. B. Rev. 11 , 391–418 (2004).
Egeth, H. E., Virzi, R. A. & Garbart, H. Searching for conjunctively defined targets. J. Exp. Psychol. Human 10 , 32–39 (1984).
Article CAS Google Scholar
Kristjansson, A. Reconsidering visual search. i-Perception http://dx.doi.org/10.1177/2041669515614670 (2015).
Wolfe, J. M. Visual search revived: the slopes are not that slippery: a comment on Kristjansson (2015). i-Perception http://dx.doi.org/10.1177/2041669516643244 (2016).
Neider, M. B. & Zelinsky, G. J. Exploring set size effects in scenes: identifying the objects of search. Vis. Cogn. 16 , 1–10 (2008).
Wolfe, J. M., Alvarez, G. A., Rosenholtz, R., Kuzmova, Y. I. & Sherman, A. M. Visual search for arbitrary objects in real scenes. Atten. Percept. Psychophys. 73 , 1650–1671 (2011).
Kovacs, I. & Julesz, B. A closed curve is much more than an incomplete one: effect of closure in figure-ground segmentation. Proc. Natl Acad. Sci. USA 90 , 7495–7497 (1993).
Article CAS PubMed PubMed Central Google Scholar
Taylor, S. & Badcock, D. Processing feature density in preattentive perception. Percept. Psychophys. 44 , 551–562 (1988).
Article CAS PubMed Google Scholar
Wolfe, J. M. & DiMase, J. S. Do intersections serve as basic features in visual search? Perception 32 , 645–656 (2003).
Article PubMed Google Scholar
Buetti, S., Cronin, D. A., Madison, A. M., Wang, Z. & Lleras, A. Towards a better understanding of parallel visual processing in human vision: evidence for exhaustive analysis of visual information. J. Exp. Psychol. Gen. 145 , 672–707 (2016).
Duncan, J. & Humphreys, G. W. Visual search and stimulus similarity. Psychol. Rev. 96 , 433–458 (1989).
Koehler, K., Guo, F., Zhang, S. & Eckstein, M. P. What do saliency models predict? J. Vis. 14 , 14 (2014).
Koch, C. & Ullman, S. Shifts in selective visual attention: towards the underlying neural circuitry. Human Neurobiol. 4 , 219–227 (1985).
CAS Google Scholar
Itti, L., Koch, C. & Niebur, E. A model of saliency-based visual attention for rapid scene analysis. IEEE T. Pattern Anal. 20 , 1254–1259 (1998).
Itti, L. & Koch, C. A saliency-based search mechanism for overt and covert shifts of visual attention. Vision. Res 40 , 1489–1506 (2000).
Bruce, N. D. B., Wloka, C., Frosst, N., Rahman, S. & Tsotsos, J. K. On computational modeling of visual saliency: examining what's right, and what's left. Vision Res. 116 , 95–112 (2015).
Zhang, L., Tong, M. H., Marks, T. K., Shan, H. & Cottrell, G. W. SUN: A Bayesian framework for saliency using natural statistics. J. Vis. 8 , 1–20 (2008).
Henderson, J. M., Malcolm, G. L. & Schandl, C. Searching in the dark: cognitive relevance drives attention in real-world scenes. Psychon. Bull. Rev. 16 , 850–856 (2009).
Tatler, B. W., Hayhoe, M. M., Land, M. F. & Ballard, D. H. Eye guidance in natural vision: reinterpreting salience. J. Vis. 11 , 5 (2011).
Nuthmann, A. & Henderson, J. M. Object-based attentional selection in scene viewing. J. Vis. 10 , 20 (2010).
Einhäuser, W., Spain, M. & Perona, P. Objects predict fixations better than early saliency. J. Vis. 8 , 18 (2008).
Stoll, J., Thrun, M., Nuthmann, A. & Einhäuser, W. Overt attention in natural scenes: objects dominate features. Vision Res. 107 , 36–48 (2015).
Maunsell, J. H. & Treue, S. Feature-based attention in visual cortex. Trends Neurosci. 29 , 317–322 (2006).
Nordfang, M. & Wolfe, J. M. Guided search for triple conjunctions. Atten. Percept. Psychophys. 76 , 1535–1559 (2014).
Friedman-Hill, S. R. & Wolfe, J. M. Second-order parallel processing: visual search for the odd item in a subset. J. Exp. Psychol. Human 21 , 531–551 (1995).
Olshausen, B. A. & Field, D. J. Sparse coding of sensory inputs. Curr. Opin. Neurobiol. 14 , 481–487 (2004).
DiCarlo, J. J., Zoccolan, D. & Rust, N. C. How does the brain solve visual object recognition? Neuron 73 , 415–434 (2012).
Vickery, T. J., King, L.-W. & Jiang, Y. Setting up the target template in visual search. J. Vis. 5 , 8 (2005).
Neisser, U. Cognitive Psychology (Appleton-Century-Crofts, 1967).
Treisman, A. & Gelade, G. A feature-integration theory of attention. Cognitive Psychol. 12 , 97–136 (1980).
Wolfe, J. M., Cave, K. R. & Franzel, S. L. Guided search: an alternative to the feature integration model for visual search. J. Exp. Psychol. Human 15 , 419–433 (1989).
Wolfe, J. M. in Oxford Handbook of Attention (eds Nobre, A. C & Kastner, S. ) 11–55 (Oxford Univ. Press, 2014).
Google Scholar
Wolfe, J. M. & Horowitz, T. S. What attributes guide the deployment of visual attention and how do they do it? Nat. Rev. Neurosci. 5 , 495–501 (2004).
Alexander, R. G., Schmidt, J. & Zelinsky, G. J. Are summary statistics enough? Evidence for the importance of shape in guiding visual search. Vis. Cogn. 22 , 595–609 (2014).
Yamins, D. L. K. & DiCarlo, J. J. Using goal-driven deep learning models to understand sensory cortex. Nat. Neurosci. 19 , 356–365 (2016).
Reijnen, E., Wolfe, J. M. & Krummenacher, J. Coarse guidance by numerosity in visual search. Atten. Percept. Psychophys. 75 , 16–28 (2013).
Godwin, H. J., Hout, M. C. & Menneer, T. Visual similarity is stronger than semantic similarity in guiding visual search for numbers. Psychon. Bull. Rev. 21 , 689–695 (2014).
Gao, T., Newman, G. E. & Scholl, B. J. The psychophysics of chasing: a case study in the perception of animacy. Cogn. Psychol. 59 , 154–179 (2009).
Meyerhoff, H. S., Schwan, S. & Huff, M. Perceptual animacy: visual search for chasing objects among distractors. J. Exp Psychol. Human 40 , 702–717 (2014).
Notebaert, L., Crombez, G., Van Damme, S., De Houwer, J. & Theeuwes, J. Signals of threat do not capture, but prioritize, attention: a conditioning approach. Emotion 11 , 81–89 (2011).
Wolfe, J. M. & Franzel, S. L. Binocularity and visual search. Percept. Psychophys. 44 , 81–93 (1988).
Paffen, C., Hooge, I., Benjamins, J. & Hogendoorn, H. A search asymmetry for interocular conflict. Atten. Percept. Psychophys. 73 , 1042–1053 (2011).
Paffen, C. L., Hessels, R. S. & Van der Stigchel, S. Interocular conflict attracts attention. Atten. Percept. Psychophys. 74 , 251–256 (2012).
Zou, B., Utochkin, I. S., Liu, Y. & Wolfe, J. M. Binocularity and visual search—revisited. Atten. Percept. Psychophys. 79 , 473–483 (2016).
Hershler, O. & Hochstein, S . At first sight: a high-level pop out effect for faces. Vision Res. 45 , 1707–1724 (2005).
Golan, T., Bentin, S., DeGutis, J. M., Robertson, L. C. & Harel, A. Association and dissociation between detection and discrimination of objects of expertise: evidence from visual search. Atten. Percept. Psychophys. 76 , 391–406 (2014).
VanRullen, R. On second glance: still no high-level pop-out effect for faces. Vision Res. 46 , 3017–3027 (2006).
Hershler, O. & Hochstein, S. With a careful look: still no low-level confound to face pop-out. Vision Res. 46 , 3028–3035 (2006).
Frischen, A., Eastwood, J. D. & Smilek, D. Visual search for faces with emotional expressions. Psychol. Bull. 134 , 662–676 (2008).
Dugué, L., McLelland, D., Lajous, M. & VanRullen, R. Attention searches nonuniformly in space and in time. Proc. Natl Acad. Sci. USA 112 , 15214–15219 (2015).
Gerritsen, C., Frischen, A., Blake, A., Smilek, D. & Eastwood, J. D. Visual search is not blind to emotion. Percept. Psychophys. 70 , 1047–1059 (2008).
Aks, D. J. & Enns, J. T. Visual search for size is influenced by a background texture gradient. J. Exp. Psychol. Human 22 , 1467–1481 (1996).
Richards, W. & Kaufman, L. ‘Centre-of-gravity’ tendencies for fixations and flow patterns. Percept. Psychophys 5 , 81–84 (1969).
Kuhn, G. & Kingstone, A. Look away! Eyes and arrows engage oculomotor responses automatically. Atten. Percept. Psychophys. 71 , 314–327 (2009).
Rensink, R. A. in Human Attention in Digital Environments (ed. Roda, C. ) Ch 3, 63–92 (Cambridge Univ. Press, 2011).
Book Google Scholar
Enns, J. T. & Rensink, R. A. Influence of scene-based properties on visual search. Science 247 , 721–723 (1990).
Zhang, X., Huang, J., Yigit-Elliott, S. & Rosenholtz, R. Cube search, revisited. J. Vis. 15 , 9 (2015).
Wolfe, J. M. & Myers, L. Fur in the midst of the waters: visual search for material type is inefficient. J. Vis. 10 , 8 (2010).
Kunar, M. A. & Watson, D. G. Visual search in a multi-element asynchronous dynamic (MAD) world. J. Exp. Psychol. Human 37 , 1017–1031 (2011).
Ehinger, K. A. & Wolfe, J. M. How is visual search guided by shape? Using features from deep learning to understand preattentive “shape space”. In Vision Sciences Society 16th Annual Meeting (2016); http://go.nature.com/2l1azoy
Vickery, T. J., King, L. W. & Jiang, Y. Setting up the target template in visual search. J. Vis. 5 , 81–92 (2005).
Biederman, I., Mezzanotte, R. J. & Rabinowitz, J. C. Scene perception: detecting and judging objects undergoing relational violations. Cognitive Psychol. 14 , 143–177 (1982).
Henderson, J. M. Object identification in context: the visual processing of natural scenes. Can. J. Psychol. 46 , 319–341 (1992).
Henderson, J. M. & Hollingworth, A. High-level scene perception. Annu. Rev. Psychol. 50 , 243–271 (1999).
Vo, M. L. & Wolfe, J. M. Differential ERP signatures elicited by semantic and syntactic processing in scenes. Psychol. Sci. 24 , 1816–1823 (2013).
‘t Hart, B. M., Schmidt, H. C. E. F., Klein-Harmeyer, I. & Einhä user, W. Attention in natural scenes: contrast affects rapid visual processing and fixations alike. Philos. T. Roy. Soc. B 368 , http://dx.doi.org/10.1098/rstb.2013.0067 (2013).
Henderson, J. M., Brockmole, J. R., Castelhano, M. S. & Mack, M. L. in Eye Movement Research: Insights into Mind and Brain (eds van Gompel, R., Fischer, M., Murray, W., & Hill, R. ) 537–562 (Elsevier, 2007).
Rensink, R. A. Seeing, sensing, and scrutinizing. Vision Res. 40 , 1469–1487 (2000).
Castelhano, M. S. & Henderson, J. M. Initial scene representations facilitate eye movement guidance in visual search. J. Exp. Psychol. Human 33 , 753–763 (2007).
Vo, M. L.-H. & Henderson, J. M. The time course of initial scene processing for eye movement guidance in natural scene search. J. Vis. 10 , 14 (2010).
Hollingworth, A. Two forms of scene memory guide visual search: memory for scene context and memory for the binding of target object to scene location. Vis. Cogn. 17 , 273–291 (2009).
Oliva, A. in Neurobiology of Attention (eds Itti, L., Rees, G., & Tsotsos, J. ) 251–257 (Academic Press, 2005).
Greene, M. R. & Oliva, A. The briefest of glances: the time course of natural scene understanding. Psychol. Sci. 20 , 464–472 (2009).
Castelhano, M. & Heaven, C. Scene context influences without scene gist: eye movements guided by spatial associations in visual search. Psychon. B. Rev. 18 , 890–896 (2011).
Malcolm, G. L. & Henderson, J. M. Combining top-down processes to guide eye movements during real-world scene search. J. Vis. 10 , 1–11 (2010).
Torralba, A., Oliva, A., Castelhano, M. S. & Henderson, J. M. Contextual guidance of eye movements and attention in real-world scenes: the role of global features on object search. Psychol. Rev. 113 , 766–786 (2006).
Vo, M. L. & Wolfe, J. M. When does repeated search in scenes involve memory? Looking at versus looking for objects in scenes. J. Exp. Psychol. Human 38 , 23–41 (2012).
Vo, M. L.-H. & Wolfe, J. M. The role of memory for visual search in scenes. Ann. NY Acad. Sci. 1339 , 72–81 (2015).
Hillstrom, A. P., Scholey, H., Liversedge, S. P. & Benson, V. The effect of the first glimpse at a scene on eye movements during search. Psychon. B. Rev. 19 , 204–210 (2012).
Hwang, A. D., Wang, H.-C. & Pomplun, M. Semantic guidance of eye movements in real-world scenes. Vision Res. 51 , 1192–1205 (2011).
Watson, D. G. & Humphreys, G. W. Visual marking: prioritizing selection for new objects by top-down attentional inhibition of old objects. Psychol. Rev. 104 , 90–122 (1997).
Donk, M. & Theeuwes, J. Prioritizing selection of new elements: bottom-up versus top-down control. Percept. Psychophys. 65 , 1231–1242 (2003).
Maljkovic, V. & Nakayama, K. Priming of popout: I. Role of features. Mem. Cognition 22 , 657–672 (1994).
Lamy, D., Zivony, A. & Yashar, A. The role of search difficulty in intertrial feature priming. Vision Res. 51 , 2099–2109 (2011).
Wolfe, J., Horowitz, T., Kenner, N. M., Hyle, M. & Vasan, N. How fast can you change your mind? The speed of top-down guidance in visual search. Vision Res. 44 , 1411–1426 (2004).
Wolfe, J. M., Butcher, S. J., Lee, C. & Hyle, M. Changing your mind: on the contributions of top-down and bottom-up guidance in visual search for feature singletons. J. Exp. Psychol. Human 29 , 483–502 (2003).
Kristjansson, A. Simultaneous priming along multiple feature dimensions in a visual search task. Vision Res. 46 , 2554–2570 (2006).
Kristjansson, A. & Driver, J. Priming in visual search: separating the effects of target repetition, distractor repetition and role-reversal. Vision Res. 48 , 1217–1232 (2008).
Sigurdardottir, H. M., Kristjansson, A. & Driver, J. Repetition streaks increase perceptual sensitivity in visual search of brief displays. Vis. Cogn. 16 , 643–658 (2008).
Kruijne, W. & Meeter, M. Long-term priming of visual search prevails against the passage of time and counteracting instructions. J. Exp. Psychol. Learn. 42 , 1293–1303 (2016).
Chun, M. & Jiang, Y. Contextual cuing: implicit learning and memory of visual context guides spatial attention. Cogn. Psychol. 36 , 28–71 (1998).
Chun, M. M. & Jiang, Y. Top-down attentional guidance based on implicit learning of visual covariation. Psychol. Sci. 10 , 360–365 (1999).
Kunar, M. A., Flusberg, S. J., Horowitz, T. S. & Wolfe, J. M. Does contextual cueing guide the deployment of attention? J. Exp. Psychol. Human 33 , 816–828 (2007).
Geyer, T., Zehetleitner, M. & Muller, H. J. Contextual cueing of pop-out visual search: when context guides the deployment of attention. J. Vis. 10 , 20 (2010).
Schankin, A. & Schubo, A. Contextual cueing effects despite spatially cued target locations. Psychophysiology 47 , 717–727 (2010).
PubMed Google Scholar
Schankin, A., Hagemann, D. & Schubo, A. Is contextual cueing more than the guidance of visual-spatial attention? Biol. Psychol. 87 , 58–65 (2011).
Peterson, M. S. & Kramer, A. F. Attentional guidance of the eyes by contextual information and abrupt onsets. Percept. Psychophys. 63 , 1239–1249 (2001).
Tseng, Y. C. & Li, C. S. Oculomotor correlates of context-guided learning in visual search. Percept. Psychophys. 66 , 1363–1378 (2004).
Wolfe, J. M., Klempen, N. & Dahlen, K. Post-attentive vision. J. Exp. Psychol. Human 26 , 693–716 (2000).
Brockmole, J. R. & Henderson, J. M. Using real-world scenes as contextual cues for search. Vis. Cogn. 13 , 99–108 (2006).
Hollingworth, A. & Henderson, J. M. Accurate visual memory for previously attended objects in natural scenes. J. Exp. Psychol. Human 28 , 113–136 (2002).
Flowers, J. H. & Lohr, D. J. How does familiarity affect visual search for letter strings? Percept. Psychophys. 37 , 557–567 (1985).
Krueger, L. E. The category effect in visual search depends on physical rather than conceptual differences. Percept. Psychophys. 35 , 558–564 (1984).
Frith, U. A curious effect with reversed letters explained by a theory of schema. Percept. Psychophys. 16 , 113–116 (1974).
Wang, Q., Cavanagh, P. & Green, M. Familiarity and pop-out in visual search. Percept. Psychophy. 56 , 495–500 (1994).
Qin, X. A., Koutstaal, W. & Engel, S. The hard-won benefits of familiarity on visual search — familiarity training on brand logos has little effect on search speed and efficiency. Atten. Percept. Psychophys. 76 , 914–930 (2014).
Fan, J. E. & Turk-Browne, N. B. Incidental biasing of attention from visual long-term memory. J. Exp. Psychol. Learn. 42 , 970–977 (2015).
Huang, L. Familiarity does not aid access to features. Psychon. B. Rev. 18 , 278–286 (2011).
Wolfe, J. M., Boettcher, S. E. P., Josephs, E. L., Cunningham, C. A. & Drew, T. You look familiar, but I don't care: lure rejection in hybrid visual and memory search is not based on familiarity. J. Exp. Psychol. Human 41 , 1576–1587 (2015).
Anderson, B. A., Laurent, P. A. & Yantis, S. Value-driven attentional capture. Proc. Natl Acad. Sci. USA 108 , 10367–10371 (2011).
MacLean, M. & Giesbrecht, B. Irrelevant reward and selection histories have different influences on task-relevant attentional selection. Atten. Percept. Psychophys. 77 , 1515–1528 (2015).
Anderson, B. A. & Yantis, S. Persistence of value-driven attentional capture. J. Exp. Psychol. Human 39 , 6–9 (2013).
Moran, R., Zehetleitner, M. H., Mueller, H. J. & Usher, M. Competitive guided search: meeting the challenge of benchmark RT distributions. J. Vis. 13 , 24 (2013).
Wolfe, J. M. in Integrated Models of Cognitive Systems (ed. Gray, W. ) 99–119 (Oxford Univ. Press, 2007).
Proulx, M. J. & Green, M. Does apparent size capture attention in visual search? Evidence from the Müller–Lyer illusion. J. Vis. 11 , 21 (2011).
Kunar, M. A. & Watson, D. G. When are abrupt onsets found efficiently in complex visual search? Evidence from multielement asynchronous dynamic search. J. Exp. Psychol. Human 40 , 232–252 (2014).
Shirama, A. Stare in the crowd: frontal face guides overt attention independently of its gaze direction. Perception 41 , 447–459 (2012).
von Grunau, M. & Anston, C. The detection of gaze direction: a stare-in-the-crowd effect. Perception 24 , 1297–1313 (1995).
Enns, J. T. & MacDonald, S. C. The role of clarity and blur in guiding visual attention in photographs. J. Exp. Psychol. Human 39 , 568–578 (2013).
Li, H., Bao, Y., Poppel, E. & Su, Y. H. A unique visual rhythm does not pop out. Cogn. Process. 15 , 93–97 (2014).
Download references
Author information
Authors and affiliations.
Department of Surgery, Visual Attention Lab, Brigham and Women's Hospital, 64 Sidney Street, Suite 170, Cambridge, 02139-4170, Massachusetts, USA
Jeremy M. Wolfe
Division of Cancer Control and Population Sciences, Basic Biobehavioral and Psychological Sciences Branch, Behavioral Research Program, National Cancer Institute, 9609 Medical Center Drive, 3E-116, Rockville, 20850, Maryland, USA
Todd S. Horowitz
You can also search for this author in PubMed Google Scholar
Corresponding author
Correspondence to Jeremy M. Wolfe .
Ethics declarations
Competing interests.
J.M.W occasionally serves as an expert witness or consultant (paid or unpaid) on the applications of visual search to topics from legal disputes (for example, how could that truck have hit that clearly visible motorcycle?) to consumer behaviour (for example, how could we redesign this shelf to attract more attention to our product?).
Rights and permissions
Reprints and permissions
About this article
Cite this article.
Wolfe, J., Horowitz, T. Five factors that guide attention in visual search. Nat Hum Behav 1 , 0058 (2017). https://doi.org/10.1038/s41562-017-0058
Download citation
Received : 11 October 2016
Accepted : 27 January 2017
Published : 08 March 2017
DOI : https://doi.org/10.1038/s41562-017-0058
Share this article
Anyone you share the following link with will be able to read this content:
Sorry, a shareable link is not currently available for this article.
Provided by the Springer Nature SharedIt content-sharing initiative
This article is cited by
Eye and head movements in visual search in the extended field of view.
- Niklas Stein
- Tamara Watson
- Szonya Durant
Scientific Reports (2024)
Using a flashlight-contingent window paradigm to investigate visual search and object memory in virtual reality and on computer screens
- Julia Beitner
- Jason Helbing
- Melissa Lê-Hoa Võ
Distracted by Previous Experience: Integrating Selection History, Current Task Demands and Saliency in an Algorithmic Model
- Neda Meibodi
- Hossein Abbasi
- Dominik Endres
Computational Brain & Behavior (2024)
Framing the fallibility of Computer-Aided Detection aids cancer detection
- Melina A. Kunar
- Derrick G. Watson
Cognitive Research: Principles and Implications (2023)
- Thomas L. Botch
- Brenda D. Garcia
- Caroline E. Robertson
Scientific Reports (2023)
Quick links
- Explore articles by subject
- Guide to authors
- Editorial policies
Sign up for the Nature Briefing newsletter — what matters in science, free to your inbox daily.

BRIEF RESEARCH REPORT article
The visual search strategies underpinning effective observational analysis in the coaching of climbing movement.
- 1 Human Sciences Research Centre, College of Life and Natural Sciences, University of Derby, Derby, United Kingdom
- 2 Lattice Training Ltd., Chesterfield, United Kingdom
Despite the importance of effective observational analysis in coaching the technical aspects of climbing performance, limited research informs this aspect of climbing coach education. Thus, the purpose of the present research was to explore the feasibility and the utility of a novel methodology, combining eye tracking technology and cued retrospective think-aloud (RTA), to capture the cognitive–perceptual mechanisms that underpin the visual search behaviors of climbing coaches. An analysis of gaze data revealed that expert climbing coaches demonstrate fewer fixations of greater duration and fixate on distinctly different areas of the visual display than their novice counterparts. Cued RTA further demonstrated differences in the cognitive–perceptual mechanisms underpinning these visual search strategies, with expert coaches being more cognizant of their visual search strategy. To expand, the gaze behavior of expert climbing coaches was underpinned by hierarchical and complex knowledge structures relating to the principles of climbing movement. This enabled the expert coaches to actively focus on the most relevant aspects of a climber’s performance for analysis. The findings demonstrate the utility of combining eye tracking and cued RTA interviewing as a new, efficient methodology of capturing the cognitive–perceptual processes of climbing coaches to inform coaching education/strategies.
Introduction
Climbing’s acceptance as an Olympic event in Tokyo 2020 is recognition of the sports’ increasing popularity and professionalization ( Bautev and Robinson, 2019 ). As demand increases, so too will the need for effective coaching, thus requiring coach educators to consider how coaching expertise is developed ( Sport England, 2018 ). Climbing coaches employ a range of complex and inter-related strategies to facilitate physical, technical, mental, and tactical improvements ( Currell and Jeukendrup, 2008 ). However, to date, climbing research has predominantly focused on the physiological and the psychological aspects of performance, somewhat neglecting the importance of the technical components of climbing ( Taylor et al., 2020 ). Furthermore, the process by which climbing coaches facilitate technical improvements in their athletes is wholly under-researched.
The characteristics that define expertise in the coaching of climbing movement, and the process by which expertise is developed, have yet to be explored. Wider expertise research has sought to identify the key characteristics of expert performance; among others, one of the key hallmarks that define expert performance is superior visual search behavior ( Ericsson, 2017 ). Research in a variety of sporting contexts (i.e., athletes, officials, and coaches) has demonstrated that experts have a superior ability to pick up on salient postural cues and detect patterns of movement and can more accurately predict the probabilities of likely event occurrences ( Williams et al., 2018 , p. 663). The superior visual search behavior of expert coaches is thought to be due to more refined domain-specific knowledge and memory structures ( Williams and Ward, 2007 ). Declarative and procedural knowledge, acquired through extensive deliberate practice, enables expert coaches to extract the most salient information from the visual display to identify the key aspects of the athlete’s performance that can subsequently be targeted for improvement ( Hughes and Franks, 2004 ).
Yet without a systematic approach to observational analysis, coaches potentially threaten the validity of their analysis ( Knudson, 2013 ). To understand how coaches analyze and evaluate climbing performance, it is argued that a fundamental step in this process is characterizing the underlying cognitive–perceptual mechanisms that underpin expertise ( Spitz et al., 2016 ). To enable this, the study of expertise in sport has commonly adopted the “Expert Performance Approach” (EPA) ( Ericsson and Smith, 1991 ). In EPA, the superior performance of experts is captured, identifying the mediating mechanisms underlying their performance by recording process-tracing measures such as eye movements and/or verbalizations ( Ford et al., 2009 ). Such advances have begun to enable significant insight into the cognitive–perceptual mechanisms underlying expert performance ( Gegenfurtner et al., 2011 ). For example, lightweight mobile eye tracking devices provide a precise, non-intrusive, millisecond-to-millisecond measurement of where, for how long, and in what sequence coaches focus their visual attention when viewing athlete performance ( Duchowski, 2007 ).
Gegenfurtner et al. (2011) conducted a meta-analysis of 65 eye tracking studies to identify the common characteristics of expert performance. They concluded that the superior performance of experts, across a variety of different domains (sport, medicine, aviation, etc.), could be explained by a combination of three factors: First, experts develop specific long-term working memory skills because of accumulated deliberate practice. Second, expert coaches can optimize the amount of processed information by ignoring task-irrelevant information. This allows for a greater proportion of their attentional resources to be allocated to more task-relevant areas of the visual display ( Haider and Frensch, 1999 ). Finally, they suggest that expert–novice performance differences in visual search are explained by an enhanced ability among experts to utilize their peripheral vision.
To date, however, there has been no eye tracking studies conducted on the visual search strategies of climbing coaches. Yet in other sports, eye tracking technology has yielded insight into differences between expert and novice coaches, which can be used to inform coaching strategies. Here eye tracking research conducted with coaches in basketball ( Damas and Ferreira, 2013 ), tennis ( Moreno et al., 2006 ), gymnastics ( Moreno et al., 2002 ), and football ( Iwatsuki et al., 2013 ) has demonstrated that expert coaches focus on distinctly different locations. Experts fixate their attention on the most salient areas of the visual display as compared to novices ( Williams et al., 1999 ). Additionally, experts demonstrate fewer fixations of greater duration in relatively static tasks/sports ( Mann et al., 2007 ; Gegenfurtner et al., 2011 ).
Most eye tracking research has, nonetheless, been conducted in laboratory settings, leading some researchers to challenge the ecological validity of the approach ( Hüttermann et al., 2018 ). Adding to this, Mann et al.(2007 ; see also Gegenfurtner et al., 2011 ) argue that the more realistic the experimental design is to the realities of the sporting context, the more likely it is that experts will be able to demonstrate their enhanced cognitive–perceptual skills afforded by their increased context-specific knowledge ( Travassos et al., 2013 ). Thus, some researchers have cast doubt on whether the results of laboratory studies can be transferred beyond their immediate context into the complex realities of the coaching environment ( Renshaw et al., 2019 ). Moving forward, therefore, the use of mobile eye tracking technology potentially enables researchers to capture the expert performance of coaches in naturalistic coaching environments, thus enhancing ecological validity and ensuring transferability to coaching practice.
Although eye tracking enables researchers to investigate the processes of visual attention, the relevance of specific gaze location biases to the coaching process still requires elaboration, that is, eye tracking gaze data can tell us where someone is looking, but importantly not why. Over-reliance on averaged and uncontextualized gaze data potentially oversimplifies and limits our understanding of the coaching process ( Dicks et al., 2017 ). Indeed one of the main conceptual concerns with sports expertise research is the relative neglect of the cognitive processes underpinning expert performance ( Moran et al., 2018 ). As Abernethy (2013) identifies, there remains a lack of evidence on the defining characteristics of sports expertise and how such characteristics are developed. Hence, additional methodological approaches are needed to complement eye tracking if the mechanisms underpinning the superior cognitive–perceptual skills of expert coaches are to be captured.
Currently, two such methodologies are proposed. These are concurrent think-aloud (CTA)—and retrospective think-aloud (RTA). In CTA, the participants verbalize their thought process during the actual task (e.g., Ericsson et al., 1993 ), whereas in RTA, the participants verbalize their thought process immediately after the task (e.g., Afonso and Mesquita, 2013 ). In critique, as we can mentally process visual stimuli much faster than we can verbalize our observations, it is argued that, when using CTA, verbalizations are often incomplete ( Wilson, 1994 ). Furthermore, attempting to verbalize complex cognitively demanding tasks while simultaneously performing them affects the user’s task performance and associated gaze behavior ( Holmqvist et al., 2011 ). The alternative, to record participants thinking aloud after the task, circumvents this disruption to the participants’ performance in the primary task. However, due to the time-lag between the primary task and RTA, a “loss of detail from memory or fabulation” may occur ( Holmqvist et al., 2011 , p. 104). The limitations of RTA are, however, potentially negated when it is combined with eye tracking technology.
Cued RTA utilizes eye tracking gaze data, as an objective reference point to stimulate memory recall, and structure RTA, reducing loss of detail from memory and fabulation ( Hyrskykari et al., 2008 ). Furthermore, cued RTA provides explicit detail as to the declarative and procedural knowledge that underpin the coach’s visual search strategies, adding depth and meaning to otherwise uncontextualized gaze data ( Gegenfurtner and Seppänen, 2012 ). Cued RTA can therefore be adopted for both empirical and theoretical reasons. First, cued RTA is confirmatory in that RTA data enable the researcher to verify the gaze data for accuracy (e.g., fixation location and allocation of attention), and gaze data provide an objective location to reduce memory loss and fabulation when conducting RTA. Second, cued RTA enables the researcher to elicit a greater level of insight into the cognitive–perceptual mechanisms that underpin the visual search strategies of coaches. It is therefore proposed that cued RTA is potentially more effective than either eye tracking or RTA methodologies applied in isolation.
Thus, in the present study, we explored the feasibility and the utility of a novel methodology, combining eye tracking technology with cued RTA, to capture the cognitive–perceptual mechanisms underpinning the visual search behaviors of climbing coaches. As this was a first trial of the combined methodology, three expert and three novice coaches were asked to observe and analyze the live climbing performances of intermediate boulderers in a naturalistic and ecologically valid setting.
Materials and Methods
Participants.
A total of six UK climbing coaches were recruited for the present study based on their level of expertise (see Moreno et al., 2002 ). The “expert” group (successful elite, as defined by Swann et al., 2015 ) consisted of three national team coaches with a minimum of 5 years of professional coaching experience (three males; 8.3 ± 1.5 years). The “novice” group ( Nash and Sproule, 2011 ) consisted of three club-level coaches, with a minimum of 1 year of coaching experience (one female, two males; 3.6 ± 2.1 years). All the participants had normal or corrected-to-normal vision and voluntarily agreed to participate following the local University of Derby ethical approval.
Climber/Bouldering Problems
The coaches were asked to observe the same intermediate (V4/F6B) climber (male; 21 years) climb four different boulder problems (2 × vertical, 1 × slab, 1 × roof) at a grade of V4/F6B ( Draper et al., 2016 ) at a national center climbing wall. Each boulder problem was repeated three times, requiring the coach to view a total of 12 attempts lasting approximately 16 s each (15.87 ± 0.81 s). The boulder problems were of a maximum height of 4 m and ranged from six to eight moves for each problem. The problems were selected in consultation with an independent national-level coach to ensure that they were judged to be of an appropriate level for the grade and representative of a normal coaching setting.
Visual Gaze Behavior
Mobile eye tracking glasses (SMI ETG 2.0; SensoMotoric Instruments, Tetlow, Germany; binocular, 60 Hz) were used to record the coaches’ visual gaze behavior. The gaze data were collected via a lightweight smart recorder (Samsung Galaxy 4) using SMI IViewX software. This enabled the recording of visual gaze data in a real-world setting. Prior to capturing eye tracking data, a three-point calibration procedure was implemented by placing three targets in a triangular configuration at a distance of 5 m. The coaches were placed 5 m away from the base of each boulder problem; i.e., at the optimum viewing angle for each specific problem (as decided by an independent national-level coach), and instructed to remain stationary. However, they could move their heads to ensure that the climber remained in the eye-tracker’s recordable visual field. To validate the accuracy, a nine-point calibration grid was placed on each boulder problem, with the markers placed at the outermost areas of the visual field that the coach would be required to observe. This ensured that the gaze data were accurate across the entire visual field. The dependent variable data collected included fixation count, fixation duration, and fixation location.
Retrospective Think-Aloud Data Capture
Retrospective think-aloud was conducted using gaze data to cue responses from the coaches: i.e., the coaches were asked to explain individual fixation locations during their analysis of the climber’s performance, verbalizing their relevance to their coaching process. The gaze data were presented to the coach as video replay with the coach’s own visual gaze scan-path super-imposed (see Figure 1 ). This scan-path showed the most recent 2-s of gaze data appearing to the coaches as a connected string of fixations (circles) and saccades (connecting lines). Each attempt was replayed at 100% speed and then slowed down to 25%.
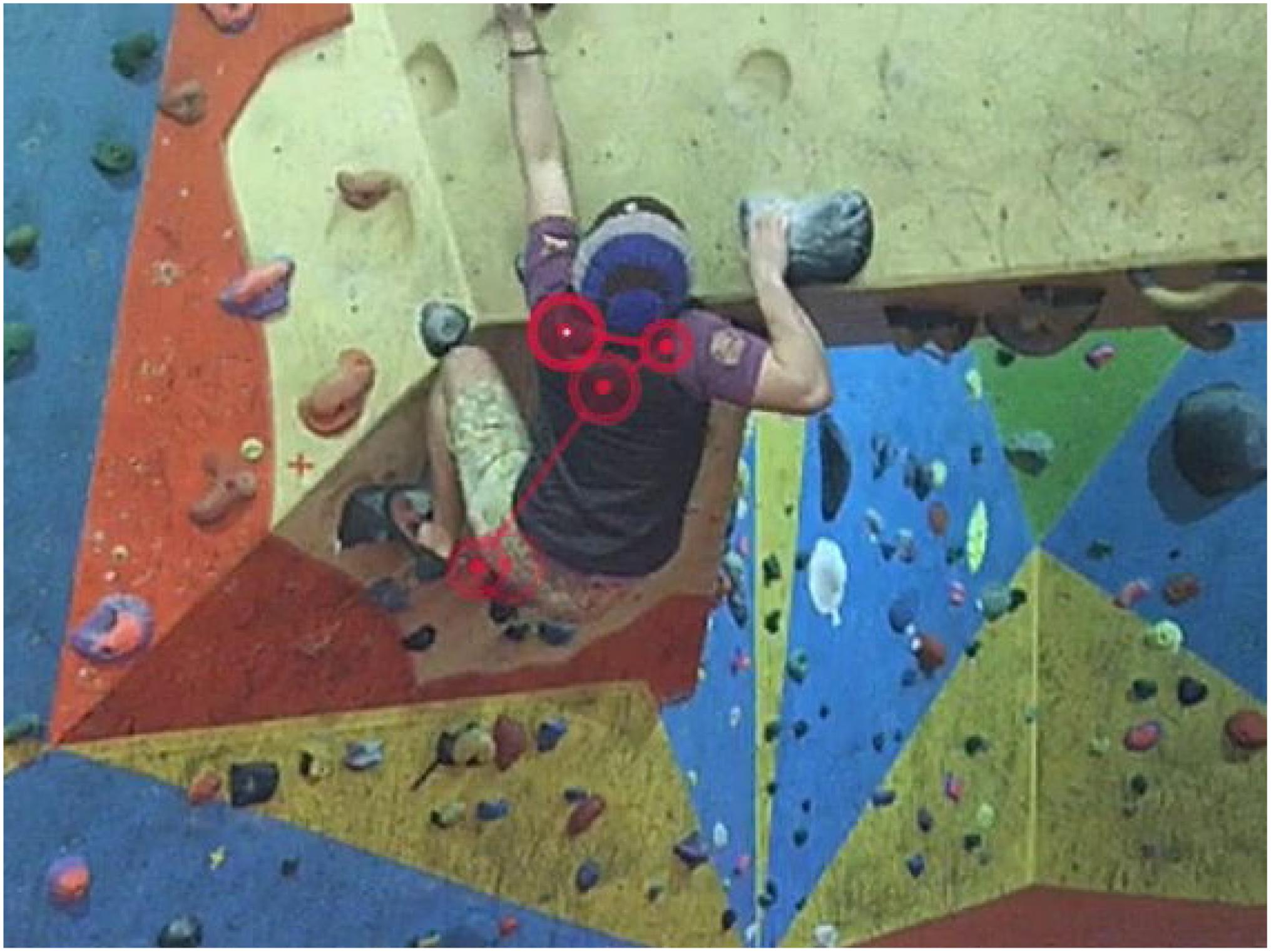
Figure 1. Example of how the gaze data were presented to coaches to cue retrospective think-aloud: visual gaze data super-imposed as 2-s scan path [a connected string of fixations (circles) and saccades (connecting lines)] to cue verbal responses (i.e., why coaches focus on specific fixation locations).
Other Materials
A demographic questionnaire captured the coaches’ prior experience: i.e., highest level of coaching experience, accumulated coaching experience, and current coaching role/responsibilities.
Once the participants had completed the demographic questionnaire, they were fitted with mobile eye tracking glasses and undertook the calibration process. The coaches were then instructed to observe the climber to assess their quality of movement and identify movement errors. It was further explained that they would be required to verbalize their analysis of the climber’s performance later in the experiment. Each coach observed the same climber climb four different boulder problems at a grade of V4, viewing three attempts for each problem. Once each coach had observed all 12 attempts, gaze data were downloaded for further review using SMI BeGaze (V3.2, SensoMotoric Instruments, Tetlow, Germany) analysis software. Using the BeGaze RTA function, the cued RTA interviews were conducted immediately after the collection of gaze data using video replay with the gaze data super-imposed to cue verbal responses. After viewing the gaze data in real time, the participants were asked to scroll through gaze data at 25% speed, explaining why they focused on specific fixation locations and their relevance to the analyses. The fixations discussed were self-selected by the participant in order to reduce researcher bias. The gaze data were replayed until each coach had exhausted all fixations they could recall.
The eye tracking metrics analyzed were: (a) “fixation rate” (i.e., average number of fixations per second), (b) “average fixation duration” (i.e., average fixation duration of all fixations throughout the entire viewing period), and (c) “total fixation duration” (i.e., total duration of a viewer’s fixations landing on a given visual element throughout the entire viewing period) within pre-defined areas of interest. Visual fixations were defined as periods where the eye remained stable in the same location (within 1° degree of tolerance) for a minimum of 120 ms ( Catteeuw et al., 2009 ). The visual gaze data were analyzed using the “semantic gaze mapping” function of SMI BeGaze to manually code fixations against three predefined areas of interest. These were the hands, the feet, and the core regions. Only the gaze data collected while the climber was attempting the problem were included in analysis. As the length of recordings differed for individual coach’s visual gaze behavior due to small variations (±5%) in the athlete’s performance, the data were normalized by cropping the recordings so that each trial was of equal duration to the shortest trial. This enabled the eye tracking metrics (e.g., “total fixation duration”) to be analyzed for comparison between coaches/groups. To enable comparison in visual search strategy, the aggregated gaze data as a function of expert or novice group were used to produce heat maps ( Holmqvist et al., 2011 ). Additional analysis was pursued using Microsoft Excel (Version 15.37, Santa Rosa, CA, United States). Due to the small sample size, the magnitude of differences was determined using Cohen’s d ( Cohen, 1988 ).
The cued RTA data were recorded concurrently, ensuring that the interview responses were not separated from the context of the coaches’ individual gaze data. The cued RTA data were transcribed verbatim, and inductive thematic analysis was conducted in accordance to the six-step process outlined by Braun and Clarke (2006) . Two members of the research team initially conducted thematic analyses independently before comparing and auditing the analysis process (i.e., first- and second-level codes and final themes). Issues of credibility and transferability were addressed by a process of member checking to ensure a good “fit” between the coaches’ views and the researchers’ final interpretation of themes, as well as ensuring that the themes transfer to the wider coaching context ( Tobin and Begley, 2004 ).
The eye tracking data quality was 98.6% (±0.9), i.e., 98.6% of the samples were captured. An analysis of the gaze data revealed distinct differences between expert and novice groups. The experts demonstrated slower fixation rates (experts 2.23 ± 0.20/s, novices 2.44s ± 0.37/s; d = 0.71) and greater average fixation durations (experts 315 ± 30 ms, novices 261 ± 59 ms; d = 1.07) than their novice counterparts. In other words, the experts demonstrated fewer fixations but of greater duration.
Furthermore, distinct differences were identified in the locations that the groups allocated attentional resources to. The experts allocated a greater proportion of their attention to the proximal (core) features of the climber’s body, demonstrating a greater number of fixations (experts 58.7 ± 24.5, novices 17.4 ± 1.4; d = 2.4) and longer total fixation durations to core body areas (experts 23.6 ± 14.5 s, novices 4.5 ± 1.2 s; d = 1.9). The experts additionally placed less attention on the climber’s hand placements than the novices did, with fewer total fixations (experts 41.0 ± 25.9, novices 69.5 ± 27.6; d = 1.1) and shorter total fixation durations (experts 16.6 ± 11.6 s, novices 25.8 ± 0.4 s; d = 1.1) toward hand placements. Finally, the experts spent more time fixating their attention on the climber’s foot placements than the novices did, with greater numbers of total fixations (experts 44.7 ± 14.6, novices 38.5 ± 14.9; d = 0.4) and longer total fixation durations (experts 20.2 ± 4.7 s, novices 11.1 ± 1.4 s; d = 2.6) toward foot placements. These differences between the expert and the novice coaches’ visual search strategy were evident from the aggregated heat maps ( Figure 2 ), which illustrate that the experts focused more attention on proximal features (e.g., hips, lumbar region, and center of back), whereas the novices almost solely focused on distal features (e.g., feet and hands).
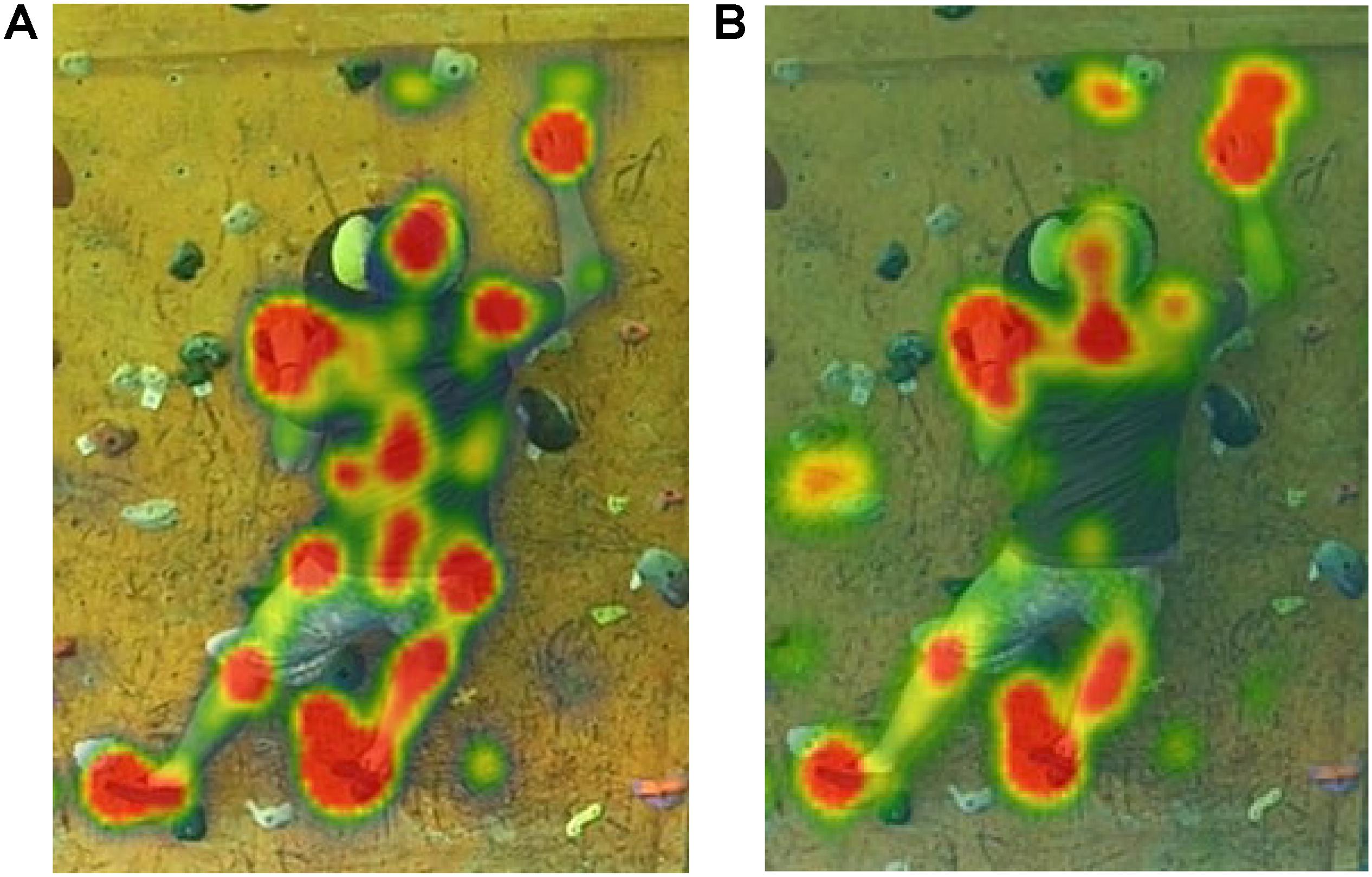
Figure 2. Aggregated heat maps of expert (A) and novice (B) coaches’ gaze behavior over 12 boulder problems illustrate notable differences in the allocation of visual attention to different regions of the climber’s body.
Retrospective Think-Aloud Data
The interview durations (min) differed noticeably between the expert and the novice coaches (experts 75.3 ± 12.3, novices 38.0 ± 11.5; d = 3.1), reflecting the level of detail that each group was able to provide while explaining their visual gaze data. The thematic analyses revealed three themes: “cognizance of visual search behavior,” “knowledge in the principles of movement and their application,” and “systematic visual search strategy.” Table 1 illustrates the first- and second-level codes that contribute to the three main themes.
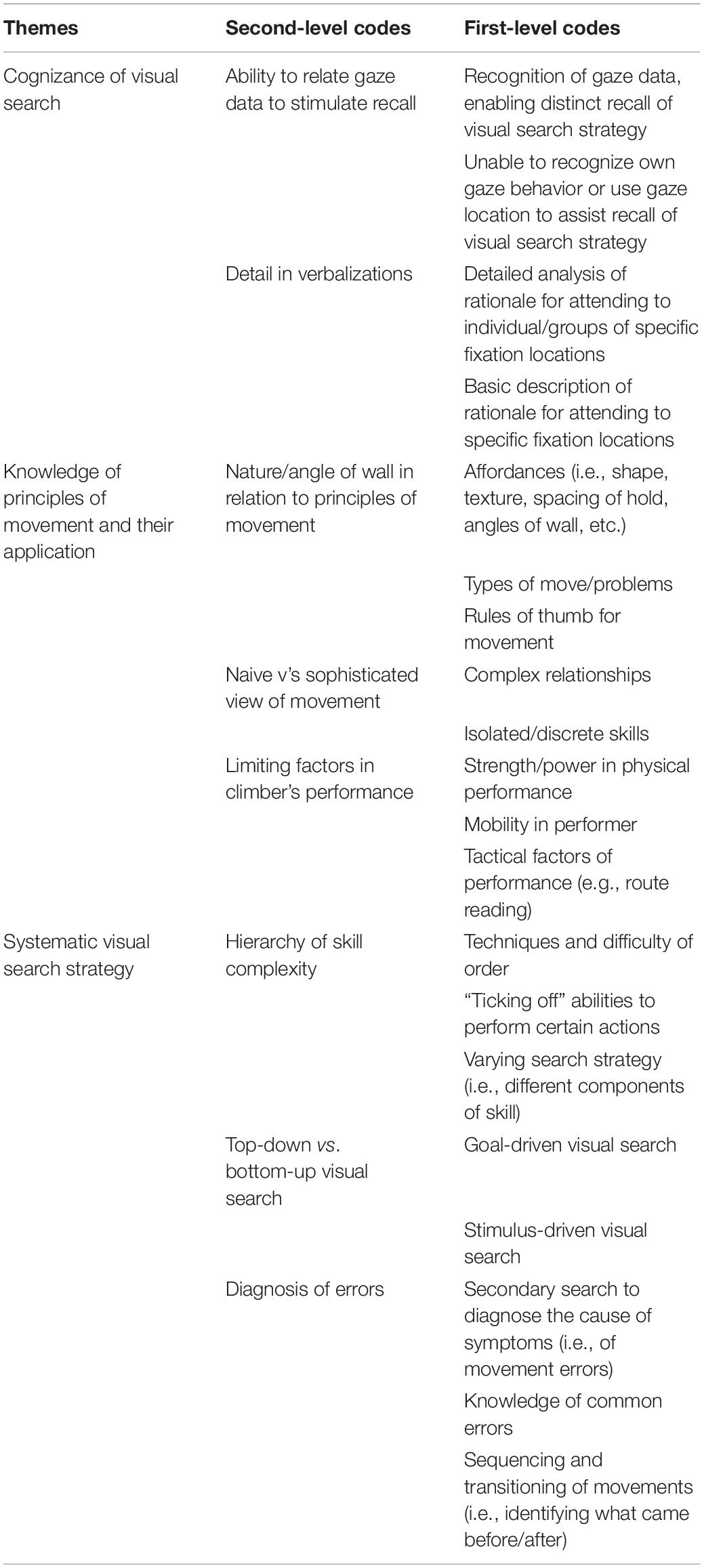
Table 1. Organization of data codes from the thematic analysis.
In respect to the first theme, the expert coaches were far more cognizant of their visual search behavior, being able to verbalize their thought process and provide rationale that explains how the gaze data relate to their coaching process. For example, one expert coach stated:
“I can tell immediately these are my eye movements… You can see I am going through my standard functional movement screening process here. This point here, I am looking at whether hip mobility limiting the climber’s ability to rock-over.” (participant E3)
The novice group, by comparison, was often unable to make any link between their gaze data and their coaching process, simply passing no comment or stating: “I’m not sure why I was looking there” (participant N2). One coach was particularly candid by stating:
“To be honest, I don’t really know what I’m looking for when I’m coaching. I know to look for messy footwork, so that’s what I look for. Beyond that, I don’t know what to look for.” (participant N3)
Considering the second theme, the expert coaches demonstrated a far greater understanding of the principles of movement and their application. Here they demonstrated more complex frameworks and principles of movement that applied to the nature and the angle of the problem. For example, one expert coach succinctly described their process as follows:
“Climbing is a really complex 3D interrelationship between the climber and infinitely varied points of contacts, at differing and changing angles. I try to think of how those points of contact can be used in conjunction, so that the climber can move their center of mass into the optimal position for that particular situation. When the climber is not achieving that position, I try to diagnose secondary factors that may be prohibiting them.” (participant E2)
By comparison, the novice coaches often discussed specific aspects of technique in isolation. For example, participant N1 stated: “So I’m looking for bad footwork here, then I’m looking for if they are holding the hold in the right way.” Comments relating to isolated aspects of technique were common among the novice group with little to no reference to the complex interrelationships between the components of the movement system and their interaction with the environment.
Finally, in reference to the third theme, the expert coaches eluded to a hierarchy of skills that guided their priorities for analysis. Participant E2 observed that:
“If you can see, I am looking at completely different areas during each attempt…looking at different aspects of their performance. I start by looking at the most basic aspects of technique, building up a picture of their ability, working through to more complex skills. When I start to see errors creeping in, I look to see if it is a consistent pattern or just a one-off. If there is a consistent pattern, that is usually the aspect of their climbing I look to address first.”
By contrast, the process of the novice coaches was continually described as a process of search for foot placement errors and search for hand placement errors, continually repeating this cycle. Thus, while both groups eluded to the skills that they prioritized, the above quote highlights how expert verbalizations were more comprehensive and demonstrated a logical/systematic progression in skill complexity. By comparison, novice verbalizations demonstrated a limited and rudimentary grasp of the critical factors that underpin the climbing movement.
Despite the importance of observational analysis in the coaching of climbing movement, the cognitive–perceptual mechanisms underpinning the visual search behavior of climbing coaches have not previously been explored. This study sets out to explore the feasibility and the utility of a previously underutilized methodology within sports expertise research, namely, if mobile eye tracking data, captured in a naturalistic and ecologically valid coaching environment, combined with cued RTA interviews can effectively capture the mechanisms that underpin the visual search behavior in expert and novice coaches. Here the results revealed that the gaze behavior of expert climbing coaches is characterized by fewer fixations, but fixations that were of longer duration than those of novice coaches. Additionally, that experts coaches tend to focus a greater proportion of their attention on proximal regions, whereas the novice coaches typically focused on distal regions. Finally, the RTA analysis revealed that the experts were more cognizant of their visual search strategy, detailing how their visual gaze behavior is guided by a systematic hierarchical process underpinned by complex knowledge structures relating to the principles of climbing movement.
A major finding of the current research was that visual attentional strategies differed between expert and novice climbing coaches. We observed that the expert coaches demonstrated fewer fixations—but these were of greater duration, suggesting that the accumulated context-specific experience of the expert coaches enables them to develop a more efficient visual search behavior. The expert coaches selectively attend to only the most task-relevant areas of the visual display, requiring them to make fewer fixations (of longer duration) to efficiently extract relevant information from specific gaze locations ( Ericsson and Kintsch, 1995 ; Haider and Frensch, 1999 ). These findings accord with previous studies investigating the visual search strategies of coaches in similar self-paced individual sports (e.g., coaching a tennis serve; Moreno et al., 2006 ).
The current research further highlighted the relevance of specific fixation locations to more efficient visual search. The proportion of attentional resources that coaches allocated to specific locations varied distinctly between experts and novices. The experts spent nearly five times as long focusing on the proximal regions of the climber’s body (or core) as compared to the novice coaches (refer again to Figure 2 ), supporting Lamb et al. (2010) notion that the observational strategies of coaches may be overly influenced by the motion of distal segments due to the greater range of motion and velocities than that of proximal segments. It is therefore proposed that the climber’s core represents one of the most salient areas upon which to analyze a climbing performance. Fluency of the center of mass, as defined by the geometric index of entropy, has been shown to be an important performance characteristic ( Cordier et al., 1994 ; Taylor et al., 2020 ). Identifying the most salient areas to analyze a climbing performance may provide a viable means to inform future coach training, helping novice coaches make their visual search behaviors more efficient ( Spitz et al., 2018 ). However, identifying gaze location alone is of limited practical value to developing coaches unless its relevance is made explicit ( Nash et al., 2011 ).
The addition of cued RTA to the eye tracking methodology revealed three themes that provide insight into the cognitions underpinning the visual attentional strategies of novice vs . expert coaches. First, the expert coaches were far more cognizant of their visual search behavior, providing a far more explicit rationale for how their gaze data related to their coaching process. The inability of novice coaches to recall and elaborate on their visual gaze data suggests a randomized and inefficient visual search strategy, that is, they were unclear as to why they fixated on specific locations or what information they hoped to acquire by doing so. Second, the experts were able to provide rich descriptions of the critical factors that underpin successful movement and relate such principles to their gaze data. Here they demonstrated more complex frameworks and principles of movement applied to the nature and the angle of the problem. Comparatively, the novice coaches provided very little detail on how principles of movement guide their visual search, suggesting that a lack of knowledge regarding the critical factors that underpin climbing movement may be a key factor that limits the effectiveness of their observational analysis. Finally, the experts were more proactive and systematic in their analysis, with their visual search strategy underpinned by a hierarchy of skills ( Gegenfurtner et al., 2011 ). It is likely that the lack of a systematic approach to observational analysis observed among novice coaches potentially limits the validity and the effectiveness of their analysis ( Knudson, 2013 ).
Based on the insights above, it is proposed that the use of cued RTA interviews potentially offers a deeper insight into the cognitive–perceptual process of coaches than the use of eye tracking or think-aloud methodologies employed in isolation. By capturing the declarative and the procedural knowledge that expert coaches utilize to guide their visual search strategy, valuable insight is acquired as to the systematic processes that expert coaches employ to analyze a climbing performance, that is, where the most salient areas of the visual display are and why they are important to the analysis of a climbing performance. Coach educators may be able to utilize such insights to provide developing coaches with a more explicit rationale to guide their visual search, enhancing the efficiency and the quality of their observational analysis.
In sum, the present results demonstrate the utility of combining eye tracking technology and cued RTA as a methodology for capturing the cognitive–perceptual processes of climbing coaches. In combining these methods, a range of different cognitions and perceptual behaviors were observed as a consequence of coaching expertise. Combining these technologies potentially offers a valid and a reliable method to capture the processes underpinning the observational analysis of a climbing movement. Indeed the same methodological approach could be applied in a variety of coaching contexts. This stated, a number of limitations and recommendations for future research are highlighted. Despite the ecological validity of the present research, the results must be interpreted tentatively given the small sample size. Furthermore, viewing the live performance of a single athlete presents challenges to study repeatability. Researchers will need to weigh the benefits of ecological validity against replicability. Future research would also benefit from exploring whether the visual search strategies of coaches remain consistent with a greater number of athletes of varying ability, anthropometrics, and style. This will help a comprehensive framework for the observational analysis of a climbing movement to be developed.
Data Availability Statement
The datasets generated for this study are available on request to the corresponding author.

Ethics Statement
The studies involving human participants were reviewed and approved by the Human Sciences Research Ethics Committee, University of Derby. The participants provided their written informed consent to participate in this study. Written informed consent was obtained from the individual(s) for the publication of any potentially identifiable images or data included in this manuscript.
Author Contributions
JM, DG, and NT contributed to the design of the study and in data collection. JM and NT performed data analysis and wrote the first draft of the manuscript. JM, FM, DS, DG, and NT revised the manuscript to produce the final draft, which was subsequently reviewed by all the authors.
Funding for open access fees is being provided internally through research budgets associated with the Human Sciences Research Centre, College of Life and Natural Sciences, University of Derby.
Conflict of Interest
DG was employed by the company Lattice Training Ltd.
The remaining authors declare that the research was conducted in the absence of any commercial or financial relationships that could be construed as a potential conflict of interest.
Abernethy, B. (2013). “Research: informed practice,” in Developing Sport Expertise: Researchers And Coaches Put Theory Into Practice , eds D. Farrow, J. Baker, and C. MacMahon (Abingdon: Routledge), 249–255.
Google Scholar
Afonso, J., and Mesquita, I. (2013). Skill-based differences in visual search behaviours and verbal reports in a representative film-based task in volleyball. Intern. J. Perform. Analy. Sport 13, 669–677. doi: 10.1080/24748668.2013.11868679
CrossRef Full Text | Google Scholar
Bautev, M., and Robinson, L. (2019). Organizational evolution and the olympic games: the case of sport climbing. Sport Soc. 22, 1674–1690. doi: 10.1080/17430437.2018.144099
Braun, V., and Clarke, V. (2006). Using thematic analysis in psychology. Q. Res. Psychol. 3, 77–101.
Catteeuw, P., Helsen, W., Gilis, B., Van Roie, E., and Wagemans, J. (2009). Visual scan patterns and decision-making skills of expert assistant referees in offside situations. J. Sport Exerc. Psychol. 31, 786–797. doi: 10.1123/jsep.31.6.786
Cohen, J. (1988). Statistical Power Analyses For The Behavioral Sciences , 2nd Edn, Hillsdale, NJ: Lawrence Erlbaum.
Cordier, P., France, M. M., Pailhous, J., and Bolon, P. (1994). Entropy as a global variable of the learning process. Hum. Mov. Sci. 13, 745–763. doi: 10.1016/0167-9457(94)90016-7
Currell, K., and Jeukendrup, A. E. (2008). Validity, reliability and sensitivity of measures of sporting performance. Sports Med. 38, 297–316. doi: 10.2165/00007256-200838040-00003
Damas, R. S., and Ferreira, A. (2013). Patterns of visual search in basketball coaches. An analysis on the level of performance. Rev. Psicol. Deport. 22, 199–204.
Dicks, M., Button, C., Davids, K., Chow, J. Y., and Van der Kamp, J. (2017). Keeping an eye on noisy movements: on different approaches to perceptual-motor skill research and training. Sports Med. 47, 575–581. doi: 10.1007/s40279-016-0600-3
Draper, N., Giles, D., Schöffl, V., Fuss, F., Watts, P., and Wolf, P. (2016). ‘Comparative grading scales, statistical analyses, climber descriptors and ability grouping: international rock climbing research association position statement’. Sports Technol. 8, 88–94. doi: 10.1080/19346182.2015.1107081
Duchowski, A. (2007). Eye Tracking Methodology: Theory and Practice. London: Springer.
Ericsson, K. A. (2017). Expertise and individual differences: the search for the structure and acquisition of experts’ superior performance. WIRES Cogn. Sci. 8:e1382. doi: 10.1002/wcs.1382
Ericsson, K. A., and Kintsch, W. (1995). Long-term working memory. Psychol. Rev. 102, 211–245. doi: 10.1037/0033-295X.102.2.211
Ericsson, K. A., Krampe, R. T., and Tesch-Römer, C. (1993). The role of deliberate practice in the acquisition of expert performance. Psychol. Rev. 100, 363–406. doi: 10.1037/0033-295x.100.3.363
Ericsson, K. A., and Smith, J. (1991). “Prospects and limits of the empirical study of expertise: an introduction,” in Towards a General Theory Of Expertise: Prospects And Limits , eds K. A. Ericsson and J. Smith (Cambridge: Cambridge University Press), 1–29.
Ford, P., Coughlan, E., and Williams, M. (2009). The expert-performance approach as a framework for understanding and enhancing coaching performance, expertise and learning. Intern. J. Sports Sci. Coach. 4, 451–463. doi: 10.1260/174795409789623919
Gegenfurtner, A., Lehtinen, E., and Saljo, R. (2011). Expertise differences in the comprehension of visualizations: a meta-analysis of eye-tracking research in professional domains. Educ. Psychol. Rev. 23, 523–552.
Gegenfurtner, A., and Seppänen, M. (2012). Transfer of expertise: an eye tracking and think aloud study using dynamic medical visualizations. Comput. Educ. 63, 393–403.
Haider, H., and Frensch, P. A. (1999). Eye movement during skill acquisition: more evidence for the information-reduction hypothesis. J. Exp. Psychol. 25, 172–190. doi: 10.1037/0278-7393.25.1.172
Holmqvist, K., Nystrom, M., Andersson, R., Dewhurst, R., Jarodzka, H., and Van der Weijer, J. (2011). Eye-Tracking: A Comprehensive Guide To Methods And Measures. New York, NY: Oxford University Press.
Hughes, N., and Franks, I. (2004). “The nature of feedback,” in Notational Analysis of Sport: Systems for Better Coaching and Performance in Sport , eds I. M. Franks and M. Hughes (London: Routledge), 17–39.
Hüttermann, S., Noël, B., and Memmert, D. (2018). Eye tracking in high-performance sports: evaluation of its application in expert athletes. Intern. J. Comput. Sci. Sport 17, 182–203. doi: 10.2478/IJCSS-2018-0011
Hyrskykari, A., Ovaska, S., Majaranta, P., Räihä, K.-J., and Lehtinen, M. (2008). Gaze path stimulation in retrospective think-aloud. J. Eye Mov. Res. 2, 1–18.
Iwatsuki, A., Hirayama, T., and Mase, K. (2013). Analysis of soccer coach’s eye gaze behavior. Proc. IAPR Asian Conf. Pattern Recogn. 2013, 793–797. doi: 10.1109/ACPR.2013.185
Knudson, D. V. (2013). Qualitative Analysis Of Human Movement. Leeds: Human Kinetics.
Lamb, P., Bartlett, R., and Robins, A. (2010). Self-organising maps: an objective method for clustering complex human movement. Intern. J. Comput. Sci. 9, 20–29.
Mann, D. T. Y., Williams, A. M., Ward, P., and Janelle, C. M. (2007). Perceptual-cognitive expertise in sport: a meta-analysis. J. Sport Exerc. Psychol. 29, 457–478. doi: 10.1123/jsep.29.4.457
Moran, A., Campbell, M., and Ranieri, D. (2018). Implications of eye tracking technology for applied sport psychology. J. Sport Psychol. Action 9, 249–259. doi: 10.1080/21520704.2018.1511660
Moreno, F. J., Reina, R., Luis, V., and Sabido, R. (2002). Visual search strategies in experienced and inexperienced gymnastic coaches. Percept. Mot. Skills 95, 901–902. doi: 10.2466/pms.2002.95.3.901
Moreno, F. J., Romero, F., Reina, R., and del Campo, V. L. (2006). Visual behaviour of tennis coaches in a court and video-based conditions (Análisis del comportamiento visual de entrenadores de tenis en situaciones de pista y videoproyección.) RICYDE. Rev. Int. Cienc. Deporte. 2, 29–41. doi: 10.5232/ricyde2006.00503
Nash, C., Sproule, J., and Horton, P. (2011). Excellence in coaching: The art and skill of elite practitioners. Res. Q. Exerc. Sport 82, 229–238. doi: 10.5641/027013611X13119541883744
Nash, C., and Sproule, J. (2011). Insights into experiences: reflections of an expert and novice coach. Intern. J. Sports Sci. Coach. 6, 149–161. doi: 10.1260/1747-9541.6.1.149
Renshaw, I., Davids, K., Araújo, D., Lucas, A., Roberts, W. M., Newcombe, D. J., et al. (2019). Evaluating weaknesses of “perceptual- cognitive training” and “brain training” methods in sport: an ecological dynamics critique. Front. Psychol. 9:2468. doi: 10.3389/fpsyg.2018.02468
Spitz, J., Put, K., Wagemans, J., Williams, A. M., and Helsen, W. F. (2016). Visual search behaviors of association football referees during assessment of foul play situations. Cogn. Res. Principl. Implicat. 1:12. doi: 10.1186/s41235-016-0013-8
Spitz, J., Put, K., Wagemans, J., Williams, A. M., and Helsen, W. F. (2018). The role of domain-generic and domain-specific perceptual-cognitive skills in association football referees. Psychol. Sport Exerc. 34:10. doi: 10.1016/j.psychsport.2017.09.010
Sport England (2018). Active Lives Adult Survey May 17/18 Report. Available at: https://www.sportengland.org/media/13768/active-lives-adult-may-17-18-report.pdf (accessed March 3, 2020).
Swann, C., Moran, A., and Piggott, D. (2015). Defining elite athletes: issues in the study of expert performance in sport psychology. Psychol. Sport Exerc. 16, 3–14. doi: 10.1016/j.psychsport.2014.07.004
Taylor, N., Giles, D., Panáčková, M., Panáčková, P., Mitchell, J., Chidley, J., et al. (2020). A novel tool for the assessment of sport climber’s movement performance. Intern. J. Sports Physiol. Perform. doi: 10.1123/ijspp.2019-0311 [Epub ahead of print].
Tobin, G. A., and Begley, C. M. (2004). Methodological rigour within a qualitative framework. J. Adv. Nurs. 48, 388–396. doi: 10.1111/j.1365-2648.2004.03207
Travassos, B., Araújo, D., Davids, K., O’Hara, K., Leitão, J., and Cortinhas, A. (2013). Expertise effects on decision-making in sport are constrained by requisite response behaviours-A meta-analysis. Psychol. Sport Exerc. 14, 211–219. doi: 10.1016/j.psychsport.2012.11.002
Williams, A. M., Davids, K., and Williams, J. G. (1999). Visual Perception And Action In Sport. London: E & FN Spon.
Williams, A. M., Ford, P., Hodges, J., and Ward, P. (2018). “Expertise in sport: Specificity, plasticity and adaptability,” in Handbook of Expertise And Expert Performance , 2nd Edn, eds K. A. Ericsson, N. Charness, R. Hoffman, and A. M. Williams (Cambridge: Cambridge University Press), 653–674.
Williams, A. M., and Ward, P. (2007). Perceptual-cognitive expertise in sport: exploring new horizons. Handb. Sport Psychol. 29, 203–223.
Wilson, T. D. (1994). Commentary to feature review THE PROPPER PROTOCOL: validity and completeness of verbal reports. Psychol. Sci. 8, 249–253.
Keywords : eye tracking, think-aloud, sport, education, expertise, gaze behavior, coaching
Citation: Mitchell J, Maratos FA, Giles D, Taylor N, Butterworth A and Sheffield D (2020) The Visual Search Strategies Underpinning Effective Observational Analysis in the Coaching of Climbing Movement. Front. Psychol. 11:1025. doi: 10.3389/fpsyg.2020.01025
Received: 02 December 2019; Accepted: 24 April 2020; Published: 28 May 2020.
Reviewed by:
Copyright © 2020 Mitchell, Maratos, Giles, Taylor, Butterworth and Sheffield. This is an open-access article distributed under the terms of the Creative Commons Attribution License (CC BY) . The use, distribution or reproduction in other forums is permitted, provided the original author(s) and the copyright owner(s) are credited and that the original publication in this journal is cited, in accordance with accepted academic practice. No use, distribution or reproduction is permitted which does not comply with these terms.
*Correspondence: James Mitchell, [email protected]
Disclaimer: All claims expressed in this article are solely those of the authors and do not necessarily represent those of their affiliated organizations, or those of the publisher, the editors and the reviewers. Any product that may be evaluated in this article or claim that may be made by its manufacturer is not guaranteed or endorsed by the publisher.
January 17, 2013
Searching Science: How the Brain Finds What You're Looking for
A target-locating test from Science Buddies
By Science Buddies
Key concepts Visual search Perception Distractions Reaction time
Introduction Have you ever wondered what makes you notice a certain person or object when you're rushing along in a crowd? Why do some things stand out whereas others melt into the background? In this activity you can explore the psychology of how things get noticed by studying how our brains help us perform a visual search. Specifically, you'll look at how changing the number and type of visual distractions affects a person's ability to find what they're looking for.
Background Have you ever looked for something you really need to find—quickly? The classic example is when someone loses a set of keys. This frustrating situation is the perfect example of performing what cognitive psychologists call a visual search. During a visual search, an observer (the person who is searching for the keys, for example) looks for a target (the keys) in the midst of distracters (all the other stuff in a home). By making the target easier to see, such as by putting the keys on a big, bright red key chain, the observer could improve on their visual search and improve its chances of success.
On supporting science journalism
If you're enjoying this article, consider supporting our award-winning journalism by subscribing . By purchasing a subscription you are helping to ensure the future of impactful stories about the discoveries and ideas shaping our world today.
What properties are important for performing a successful visual search? Consider the following exercise to help you think about the variables: If you had a printed page full of letter L’s in blue ink and just one letter T in red ink, it would be pretty easy to find the red T, right? However, what if half of the L’s were blue and half were red? In the latter situation, there are more complex distracters, making finding the target (the red letter T) more difficult.
Materials • Computer with Internet connection • Piece of paper and pen or pencil • At least three volunteers (including yourself)
Preparation • In your Web browser, go to the Cognitive Science Visual Search Web page developed by cognitive psychologist Tom Busey of Indiana University Bloomington. (Depending on what Internet browser you have and whether Java is enabled on your computer, you will either need to run the Java applet directly from the Web page or download the software to your computer. To use the Java applet, simply select the "Run Applet" button. To download the software, click on the movie link and follow the instructions to download the software to your desktop.) • In the Visual Search applet or downloaded program, select the "Targets" tab at the top. In the "Target 1" section click on the large drop-down menu and select the image of a hot dog (the images are arranged alphabetically). The hot dog image should appear in the box after selecting it. Make sure the box next to "Display Target 1" is checked. • Now click on the "Distracters" tab at the top. In the "Distracter 1" section, click on the large drop-down menu and select the image of a burger. The burger image should appear in the box. Make sure the box next to "Display Distracter 1" is checked. • Next click on the "Do Experiment" tab. Make sure the "Use Circular Display" box is unchecked ! Click on the "Start Experiment" button when you're ready and follow the instructions, pressing "f" if you see the hotdog or "j" if you do not see it. Tip: Make sure nothing distracting is going on when you do the experiment! • After you are done it will tell you to click on the button below to quit and view your results, which will show up on the "Do Experiment" screen. Do not worry about your results quite yet. Instead, do the experiment one or two more times, or until you feel comfortable with the system, and then move on to the procedure below.
Procedure • After you have tried doing the experiment with one distracter a few times and feel comfortable with it, do the experiment again. • This time when you get the results of the experiment, write down the numbers in the "Mean Reaction Times for Target Present Trials" and "Mean Reaction Times for Target Absent Trials" boxes under "All Trials." (Ignore the numbers in parentheses.) • Go back to the "Distracters" tab at the top. In the "Distracter 2" section, click on the large drop-down menu and select the image of a pizza slice. Check the box next to "Display Distracter 2." • Click on the "Do Experiment" tab and run it by clicking "Start Experiment" and following the instructions. • When you get the results of the experiment, again write down the numbers in boxes in the "All Trials" section. Did it take you more or less time to react with two distracters compared with one? • Go back to the "Distracters" tab and add a third distracter, this time a peach. Do the experiment and again write down the relevant reaction time numbers. Did it take you more or less time to react with three distracters compared with one or two? • Go back to the "Distracters" tab and add a fourth distracter, this time a carrot. Run the experiment again and write down the relevant reaction time numbers. Did it take you more or less time to react with four distracters compared with fewer ones? • Overall, how did the reaction time change as more distracters were added? Is this what you would have expected? Did it take longer for someone to react when there was a target present or absent? • Repeat this activity with at least two other volunteers so that you have tested it with a total of three different people. For each person, be sure to let him or her try the experiment with only one distracter a few times before you start collecting the numbers from their experiments. For each volunteer, did you see the same correlation between reaction time and number of distracters? • Extra: Repeat this activity but try changing some different variables and see how it affects reaction time and the percent of the answers that are correct. You could try changing the number of targets instead of distracters. Is it easier or harder to spot multiple targets? You could also measure percent correct instead of response time. Do people give fewer correct answers as more distracters are present? Try changing the number of images by changing the number of rows and columns. Is it harder to find the target when there are more images? You could also try changing the images, such as using symbols, letters or numbers instead of types of food, or change the colors of the target and distracter. Is it harder to find the target as its similarity to the distracters increases? • Extra: This cognitive test has real-world applications that you could investigate. Look into how logos and brand names are designed to be noticed, the way Web sites are designed to be easy to navigate, how points of interest on a map are marked or other data are displayed in a way that highlights what's important. How are visual search properties used in these different areas?
Observations and results Did the reaction time increase as more distracters were added? Did it take longer for volunteers to answer when the target was absent compared with when it was present?
You should have seen that, in general, the reaction time needed to do the visual search increased as more distracters were added. (There may have been some exceptions, such as a person taking only slightly longer to do a visual search with three distracters present in comparison with four, but it should have clearly taken a good deal more time to find the target when there were four distracters compared with when there was just one.) When more distracters are present, it makes finding the target more difficult. (Think of the example with the red letter T target and letter L distracters that became more distracting when they changed from all blue to half red.) This makes people take more time in their visual search, even if the target is not there. In fact, you should have seen that people actually take more time when the target is absent compared with when it is present, as they may spend more time checking and rechecking to make sure that the target is really not there.
More to explore Cognitive Science Software: Visual Search , from Tom Busey at Indiana University Bloomington Research Explains How the Brain Finds Waldo , from ScienceDaily The Truth Behind Where's Waldo? , from ScienceDaily The Brains Behind Where's Waldo?, from Science Buddies
This activity brought to you in partnership with Science Buddies
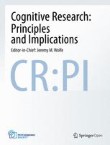
Visual Search in Real-World and Applied Contexts
Call for papers.
Co-organizers:
CRPI Editor in Chief: Jeremy M. Wolfe, Brigham & Women’s Hospital and Harvard Medical School
Guest Editor: Trafton Drew, University of Utah, [email protected]
Assistant Guest Editor : Lauren H. Williams, University of Utah, [email protected]
Visual search tasks are an everyday part of the human experience - ranging from hunting for a specific recipe ingredient in the pantry to monitoring for road hazards and informational signs while driving. Due to its ubiquity in everyday life, visual search has been extensively studied in the laboratory for decades even if laboratory tasks are unable to capture the full complexity of real-world visual search tasks. In recent years, there has been a growing body of research seeking to narrow the gaps in our understanding of visual search behavior between the laboratory and the real world. The purpose of this special issue in Cognitive Research: Principles and Implications (CRPI) is to bring together this important research.
We anticipate one set of submissions involving studies of visual search behavior in applied situations, such as driving, data visualization, website design, and expert visual search tasks (e.g., baggage screening, radiology). These papers should make an effort to connect specific applied settings to basic principles in the study of visual attention. A second set of papers will use more general laboratory search tasks to further our understanding of visual search behavior in real world situations. Both types of papers fit CRPI’s mission to publish “use-inspired basic research”; research that starts from a problem in the world and illuminates fundamental properties of perception and cognition.
Potential topics of interest include but are not limited to: individual differences in search performance, scene grammar, search in three-dimensional (3D) environments, visual & motor system interactions in visual search (e.g., rummaging or foraging tasks), search for imprecise or categorical search targets, quitting behavior, visual search in dynamic environments, real-world search errors and their laboratory analogues (the low prevalence effect, subsequent search misses, inattentional blindness), hybrid visual search, and interventions designed to improve visual search performance. We invite you to contribute.
CRPI is the open access journal of the Psychonomic Society. Its mission is to publish use-inspired basic research : fundamental cognitive research that grows from hypotheses about real-world problems. As with all Psychonomic Society journals, submissions to CRPI are subject to rigorous peer review.
For manuscripts accepted for the special issue, the publication fee may be fully or partially waived depending on the number of manuscripts accepted for the special issue. The authors should indicate when they submit a manuscript if they are requesting a waiver of the publication fee. Please email any of the editors with questions about submissions.
Deadline: manuscripts should be submitted before August 1, 2020
You can find manuscript submission details at http://cognitiveresearchjournal.springeropen.com/submission-guidelines/preparing-your-manuscript
- Editorial Board
- Sign up for article alerts and news from this journal
- 2023 Reviewer Acknowledgements
- 2023 Psychonomic Society Best Article Award
Affiliated with
Cognitive Research: Principles and Implications is affiliated with The Psychonomic Society
- ISSN: 2365-7464 (electronic)
Annual Journal Metrics
2022 Citation Impact 4.1 - 2-year Impact Factor 4.1 - 5-year Impact Factor 1.383 - SNIP (Source Normalized Impact per Paper) 1.004 - SJR (SCImago Journal Rank)
2023 Speed 10 days submission to first editorial decision for all manuscripts (Median) 206 days submission to accept (Median)
2023 Usage 1,160,777 downloads 9,403 Altmetric mentions
- More about our metrics

An official website of the United States government
The .gov means it’s official. Federal government websites often end in .gov or .mil. Before sharing sensitive information, make sure you’re on a federal government site.
The site is secure. The https:// ensures that you are connecting to the official website and that any information you provide is encrypted and transmitted securely.
- Publications
- Account settings
- My Bibliography
- Collections
- Citation manager
Save citation to file
Email citation, add to collections.
- Create a new collection
- Add to an existing collection
Add to My Bibliography
Your saved search, create a file for external citation management software, your rss feed.
- Search in PubMed
- Search in NLM Catalog
- Add to Search
Visual Search: How Do We Find What We Are Looking For?
Affiliations.
- 1 Department of Ophthalmology, Harvard Medical School, Boston, Massachusetts 02115, USA.
- 2 Department of Radiology, Harvard Medical School, Boston, Massachusetts 02115, USA.
- 3 Visual Attention Lab, Brigham & Women's Hospital, Cambridge, Massachusetts 02139, USA; email: [email protected].
- PMID: 32320631
- DOI: 10.1146/annurev-vision-091718-015048
In visual search tasks, observers look for targets among distractors. In the lab, this often takes the form of multiple searches for a simple shape that may or may not be present among other items scattered at random on a computer screen (e.g., Find a red T among other letters that are either black or red.). In the real world, observers may search for multiple classes of target in complex scenes that occur only once (e.g., As I emerge from the subway, can I find lunch, my friend, and a street sign in the scene before me?). This article reviews work on how search is guided intelligently. I ask how serial and parallel processes collaborate in visual search, describe the distinction between search templates in working memory and target templates in long-term memory, and consider how searches are terminated.
Keywords: foraging; parallel processing; serial processing; visual attention; visual search; working memory.
PubMed Disclaimer
Similar articles
- Guided Search 6.0: An updated model of visual search. Wolfe JM. Wolfe JM. Psychon Bull Rev. 2021 Aug;28(4):1060-1092. doi: 10.3758/s13423-020-01859-9. Epub 2021 Feb 5. Psychon Bull Rev. 2021. PMID: 33547630 Free PMC article. Review.
- Probabilistic rejection templates in visual working memory. Chetverikov A, Campana G, Kristjánsson Á. Chetverikov A, et al. Cognition. 2020 Mar;196:104075. doi: 10.1016/j.cognition.2019.104075. Epub 2019 Dec 14. Cognition. 2020. PMID: 31841813
- Hybrid foraging search: Searching for multiple instances of multiple types of target. Wolfe JM, Aizenman AM, Boettcher SE, Cain MS. Wolfe JM, et al. Vision Res. 2016 Feb;119:50-9. doi: 10.1016/j.visres.2015.12.006. Epub 2016 Jan 20. Vision Res. 2016. PMID: 26731644 Free PMC article.
- Guidance toward and away from distractors in repeated visual search. Höfler M, Gilchrist ID, Körner C. Höfler M, et al. J Vis. 2015;15(5):12. doi: 10.1167/15.5.12. J Vis. 2015. PMID: 26067530
- The role of memory for visual search in scenes. Le-Hoa Võ M, Wolfe JM. Le-Hoa Võ M, et al. Ann N Y Acad Sci. 2015 Mar;1339(1):72-81. doi: 10.1111/nyas.12667. Epub 2015 Feb 12. Ann N Y Acad Sci. 2015. PMID: 25684693 Free PMC article. Review.
- Multiple visual items can be simultaneously compared with target templates in memory. Zheng Y, Lou J, Lu Y, Li Z. Zheng Y, et al. Atten Percept Psychophys. 2024 Jun 5. doi: 10.3758/s13414-024-02906-6. Online ahead of print. Atten Percept Psychophys. 2024. PMID: 38839716
- Editorial: Rising stars in systems neuroscience: 2022. Fallah M, Haam J, Ledonne A. Fallah M, et al. Front Syst Neurosci. 2024 May 14;18:1414351. doi: 10.3389/fnsys.2024.1414351. eCollection 2024. Front Syst Neurosci. 2024. PMID: 38808259 Free PMC article. No abstract available.
- Hierarchical Constraints on the Distribution of Attention in Dynamic Displays. Xu H, Zhou J, Shen M. Xu H, et al. Behav Sci (Basel). 2024 May 11;14(5):401. doi: 10.3390/bs14050401. Behav Sci (Basel). 2024. PMID: 38785892 Free PMC article.
- Reliability and validity of a novel attention assessment scale (broken ring enVision search test) in the Chinese population. Shi Y, Zhang Y. Shi Y, et al. Front Psychol. 2024 May 9;15:1375326. doi: 10.3389/fpsyg.2024.1375326. eCollection 2024. Front Psychol. 2024. PMID: 38784625 Free PMC article.
- Eye and head movements in visual search in the extended field of view. Stein N, Watson T, Lappe M, Westendorf M, Durant S. Stein N, et al. Sci Rep. 2024 Apr 17;14(1):8907. doi: 10.1038/s41598-024-59657-5. Sci Rep. 2024. PMID: 38632334 Free PMC article.
Publication types
- Search in MeSH
LinkOut - more resources
Full text sources.
- Ingenta plc
Research Materials
- NCI CPTC Antibody Characterization Program
Miscellaneous
- NCI CPTAC Assay Portal
- Citation Manager
NCBI Literature Resources
MeSH PMC Bookshelf Disclaimer
The PubMed wordmark and PubMed logo are registered trademarks of the U.S. Department of Health and Human Services (HHS). Unauthorized use of these marks is strictly prohibited.
- A-Z Publications
Annual Review of Vision Science
Volume 6, 2020, review article, visual search: how do we find what we are looking for.
- Jeremy M. Wolfe 1,2,3
- View Affiliations Hide Affiliations Affiliations: 1 Department of Ophthalmology, Harvard Medical School, Boston, Massachusetts 02115, USA 2 Department of Radiology, Harvard Medical School, Boston, Massachusetts 02115, USA 3 Visual Attention Lab, Brigham & Women's Hospital, Cambridge, Massachusetts 02139, USA; email: [email protected]
- Vol. 6:539-562 (Volume publication date September 2020) https://doi.org/10.1146/annurev-vision-091718-015048
- First published as a Review in Advance on April 22, 2020
- Copyright © 2020 by Annual Reviews. All rights reserved
In visual search tasks, observers look for targets among distractors. In the lab, this often takes the form of multiple searches for a simple shape that may or may not be present among other items scattered at random on a computer screen (e.g., Find a red T among other letters that are either black or red.). In the real world, observers may search for multiple classes of target in complex scenes that occur only once (e.g., As I emerge from the subway, can I find lunch, my friend, and a street sign in the scene before me?). This article reviews work on how search is guided intelligently. I ask how serial and parallel processes collaborate in visual search, describe the distinction between search templates in working memory and target templates in long-term memory, and consider how searches are terminated.
Article metrics loading...
Full text loading...
Literature Cited
- Adams WJ. 2008 . Frames of reference for the light-from-above prior in visual search and shape judgements. Cognition 107 : 137– 50 [Google Scholar]
- Anderson BA. 2014 . On the precision of goal-directed attentional selection. J. Exp. Psychol. Hum. Percept. Perform. 40 : 1755– 62 [Google Scholar]
- Anderson BA , Laurent PA , Yantis S 2011 . Value-driven attentional capture. PNAS 108 : 10367– 71 [Google Scholar]
- Ásgeirsson ÁG , Kristjánsson Á 2019 . Attentional priming does not enable observers to ignore salient distractors. Vis. Cogn. 27 : 595– 608 [Google Scholar]
- Awh E , Belopolsky AV , Theeuwes J 2012 . Top-down versus bottom-up attentional control: a failed theoretical dichotomy. Trends Cogn. Sci. 16 : 437– 43 [Google Scholar]
- Bacon WF , Egeth HE. 1994 . Overriding stimulus-driven attentional capture. Percept. Psychophys. 55 : 485– 96 [Google Scholar]
- Baldassi S , Verghese P. 2002 . Comparing integration rules in visual search. J. Vis. 2 : 559– 70 [Google Scholar]
- Bauer B , Jolicœur P , Cowan WB 1996 . Visual search for colour targets that are or are not linearly-separable from distractors. Vis. Res. 36 : 1439– 66 [Google Scholar]
- Beck VM , Hollingworth A , Luck SJ 2011 . Simultaneous control of attention by multiple working memory representations. Psychol. Sci. 23 : 887– 98 [Google Scholar]
- Becker S , Atalla M , Folk CL 2020 . Conjunction search: Can we simultaneously bias attention to features and relations. Atten. Percept. Psychophys. 82 : 246– 68 [Google Scholar]
- Becker SI. 2010 . The role of target-distractor relationships in guiding attention and the eyes in visual search. J. Exp. Psychol. Gen. 139 : 247– 65 [Google Scholar]
- Belopolsky AV , Theeuwes J. 2012 . Updating the premotor theory: The allocation of attention is not always accompanied by saccade preparation. J. Exp. Psychol. Hum. Percept. Perform. 38 : 902– 14 [Google Scholar]
- Berbaum KS , Franken EA , Caldwell RT , Shartz K , Madsen M 2019 . Satisfaction of search in radiology. The Handbook of Medical Image Perception and Techniqu e s E Samei, EA Krupinski 121– 66 Cambridge, UK: Cambridge Univ. Press. , 2nd ed.. [Google Scholar]
- Berbaum KS , Franken EA Jr. , Dorfman DD , Rooholamini SA , Coffman CE et al. 1991 . Time course of satisfaction of search. Invest. Radiol. 26 : 640– 48 [Google Scholar]
- Biederman I , Glass AL , Stacy EW 1973 . Searching for objects in real-world scenes. J. Exp. Psychol. 97 : 22– 27 [Google Scholar]
- Boettcher SEP , Draschkow D , Dienhart E , Võ MLH 2018 . Anchoring visual search in scenes: assessing the role of anchor objects on eye movements during visual search. J. Vis. 18 : 11 [Google Scholar]
- Brams S , Ziv G , Levin O , Spitz J , Wagemans J et al. 2019 . The relationship between gaze behavior, expertise, and performance: a systematic review. Psychol. Bull. 145 : 10 980– 1027 [Google Scholar]
- Brascamp JW , Pels E , Kristjansson A 2011 . Priming of pop-out on multiple time scales during visual search. Vis. Res. 51 : 1972– 78 [Google Scholar]
- Bravo MJ , Farid H. 2014 . Informative cues can slow search: the cost of matching a specific template. Attention Percept. Psychophys. 76 : 32– 39 [Google Scholar]
- Bravo MJ , Farid H. 2016 . Observers change their target template based on expected context. Atten. Percept. Psychophys. 78 : 829– 37 [Google Scholar]
- Buetti S , Xu J , Lleras A 2019 . Predicting how color and shape combine in the human visual system to direct attention. Sci. Rep. 9 : 20258 [Google Scholar]
- Cain MS , Adamo SH , Mitroff SR 2013 . A taxonomy of errors in multiple-target visual search. Vis. Cogn. 21 : 899– 921 [Google Scholar]
- Castelhano M , Heaven C. 2011 . Scene context influences without scene gist: eye movements guided by spatial associations in visual search. Psychon. Bull. Rev. 18 : 890– 96 [Google Scholar]
- Castelhano MS , Henderson JM. 2007 . Initial scene representations facilitate eye movement guidance in visual search. J. Exp. Psychol. Hum. Percept. Perform. 33 : 753– 63 [Google Scholar]
- Cave KR , Bush WS , Taylor TGG 2010 . Split attention as part of a flexible attentional system for complex scenes: comment on Jans, Peters, and De Weerd 2010. Psychol. Rev. 117 : 685– 95 [Google Scholar]
- Chan LK , Hayward WG. 2014 . No attentional capture for simple visual search: evidence for a dual-route account. J. Exp. Psychol. Hum. Percept. Perform. 40 : 2154– 66 [Google Scholar]
- Chan LKH , Hayward WG. 2009 . Feature integration theory revisited: dissociating feature detection and attentional guidance in visual search. J. Exp. Psychol. Hum. Percept. Perform. 35 : 119– 32 [Google Scholar]
- Charnov EL. 1976 . Optimal foraging, the marginal value theorem. Theor. Popul. Biol. 9 : 129– 36 [Google Scholar]
- Chun M , Jiang Y. 1998 . Contextual cueing: Implicit learning and memory of visual context guides spatial attention. Cogn. Psychol. 36 : 28– 71 [Google Scholar]
- Chun MM , Wolfe JM. 1996 . Just say no: How are visual searches terminated when there is no target present. Cogn. Psychol. 30 : 39– 78 [Google Scholar]
- Cousineau D , Shiffrin RM. 2004 . Termination of a visual search with large display size effects. Spat. Vis. 17 : 327– 52 [Google Scholar]
- Cowan N. 1995 . Attention and Memory: An Integrated Framework Oxford, UK: Oxford Univ. Press [Google Scholar]
- Cowan N. 2001 . The magical number 4 in short-term memory: a reconsideration of mental storage capacity. Behav. Brain Sci. 24 : 87– 114 [Google Scholar]
- Cunningham CA , Egeth HE. 2016 . Taming the white bear: initial costs and eventual benefits of distractor inhibition. Psychol. Sci. 27 : 476– 85 [Google Scholar]
- Cunningham CA , Wolfe JM. 2014 . The role of object categories in hybrid visual and memory search. J. Exp. Psychol. Gen. 143 : 1585– 99 [Google Scholar]
- Desimone R , Duncan J. 1995 . Neural mechanisms of selective visual attention. Annu. Rev. Neurosci. 18 : 193– 222 [Google Scholar]
- Dickinson CA , Zelinsky GJ. 2005 . Marking rejected distractors: a gaze-contingent technique for measuring memory during search. Psychon. Bull. Rev. 12 : 1120– 26 [Google Scholar]
- Dowd EW , Mitroff SR. 2013 . Attentional guidance by working memory overrides saliency cues in visual search. J. Exp. Psychol. Hum. Percept. Perform. 39 : 1786– 96 [Google Scholar]
- Drew T , Boettcher SP , Wolfe JM 2015 . Searching while loaded: Visual working memory does not interfere with hybrid search efficiency but hybrid search uses working memory capacity. Psychon. Bull. Rev. 23 : 201– 12 [Google Scholar]
- Eckstein M , Koehler K , Welbourne LE , Akbas EP 2017 . Humans but not deep neural networks often miss giant targets in scenes. Curr. Biol. 27 : 2827– 32.e3 [Google Scholar]
- Egeth H. 1977 . Attention and preattention. The Psychology of Learning and Motivation GH Bower 277– 320 New York: Academic [Google Scholar]
- Egeth HE , Virzi RA , Garbart H 1984 . Searching for conjunctively defined targets. J. Exp. Psychol. Hum. Percept. Perform. 10 : 32– 39 [Google Scholar]
- Ehinger KA , Hidalgo-Sotelo B , Torralba A , Oliva A 2009 . Modeling search for people in 900 scenes: a combined source model of eye guidance. Vis. Cogn. 17 : 945– 78 [Google Scholar]
- Enns JT , Rensink RA. 1990 . Influence of scene-based properties on visual search. Science 247 : 721– 23 [Google Scholar]
- Evans KK , Birdwell RL , Wolfe JM 2013 . If you don't find it often, you often don't find it: why some cancers are missed in breast cancer screening. PLOS ONE 8 : 5 e64366 [Google Scholar]
- Failing M , Theeuwes J. 2017 . Selection history: how reward modulates selectivity of visual attention. Psychon. Bull. Rev. 25 : 514– 38 [Google Scholar]
- Fecteau JH , Munoz DP. 2006 . Salience, relevance, and firing: a priority map for target selection. Trends Cogn. Sci. 10 : 382– 90 [Google Scholar]
- Foster DH , Ward PA. 1991 . Horizontal-vertical filters in early vision predict anomalous line-orientation frequencies. Proc. R. Soc. Lond. B 243 : 83– 86 [Google Scholar]
- Frintrop S , Rome E , Christensen HI 2010 . Computational visual attention systems and their cognitive foundations: a survey. ACM Trans. Appl. Percept. 7 : 6 [Google Scholar]
- Gaspelin N , Vecera S. 2019 . An introduction to the special issue on “Dealing with Distractors in Visual Search. .” Vis. Cogn. 27 : 183– 84 [Google Scholar]
- Ghazizadeh A , Griggs W , Hikosaka O 2016 . Object-finding skill created by repeated reward experience. J. Vis. 16 : 17 [Google Scholar]
- Green BF , Anderson LK. 1956 . Color coding in a visual search task. J. Exp. Psychol. 51 : 19– 24 [Google Scholar]
- Greene MR , Oliva A. 2009 . The briefest of glances: the time course of natural scene understanding. Psychol. Sci. 20 : 464– 72 [Google Scholar]
- Grubert A , Eimer M. 2018 . The time course of target template activation processes during preparation for visual search. J. Neurosci. 38 : 9527– 38 [Google Scholar]
- Gunseli E , Meeter M , Olivers CNL 2014 . Is a search template an ordinary working memory? Comparing electrophysiological markers of working memory maintenance for visual search and recognition. Neuropsychologia 60 : 29– 38 [Google Scholar]
- Hamker FH. 2004 . A dynamic model of how feature cues guide spatial attention. Vis. Res. 44 : 501– 21 [Google Scholar]
- Helmholtz HV. 1924 . Treatise on Physiological Optics Rochester, NY: Opt. Soc. Am. [Google Scholar]
- Henderson JM , Ferreira F. 2004 . Scene perception for psycholinguists. The Interface of Language, Vision, and Action: Eye Movements and the Visual World JM Henderson, F Ferreira 1– 58 New York: Psychol. Press [Google Scholar]
- Henderson JM , Hayes TR. 2017 . Meaning guides attention in real-world scenes. Nat. Hum. Behav. 1 : 743– 47 [Google Scholar]
- Henderson JM , Hayes TR. 2018 . Meaning guides attention in real-world scene images: evidence from eye movements and meaning maps. J. Vis. 18 : 10 [Google Scholar]
- Hickey C , Chelazzi L , Theeuwes J 2010 . Reward changes salience in human vision via the anterior cingulate. J. Neurosci. 30 : 11096– 103 [Google Scholar]
- Hillstrom AP. 2000 . Repetition effects in visual search. Percept. Psychophys. 62 : 800– 17 [Google Scholar]
- Hoffman JE. 1979 . A two-stage model of visual search. Percept. Psychophys. 25 : 319– 27 [Google Scholar]
- Hoffman JE. 1996 . Visual attention and eye movements. Attention H Pashler 119– 54 London: Univ. Coll. Lond. Press [Google Scholar]
- Horowitz TS. 2017 . Prevalence in visual search: from the clinic to the lab and back again. Jpn. Psychol. Res. 59 : 65– 108 [Google Scholar]
- Horowitz TS , Wolfe JM. 1998 . Visual search has no memory. Nature 394 : 575– 77 [Google Scholar]
- Horowitz TS , Wolfe JM. 2001 . Search for multiple targets: Remember the targets, forget the search. Percept. Psychophys. 63 : 272– 85 [Google Scholar]
- Horowitz TS , Wolfe JM. 2003 . Memory for rejected distractors in visual search. Vis. Cogn. 10 : 257– 98 [Google Scholar]
- Huang L , Holcombe AO , Pashler H 2004 . Repetition priming in visual search: episodic retrieval, not feature priming. Mem. Cogn. 32 : 12– 20 [Google Scholar]
- Hubner R. 2001 . A formal version of the Guided Search (GS2) model. Percept. Psychophys. 63 : 945– 51 [Google Scholar]
- Hulleman J , Olivers CNL. 2017 . The impending demise of the item in visual search. Behav. Brain Sci. 40 : e132 [Google Scholar]
- Itti L , Koch C. 2000 . A saliency-based search mechanism for overt and covert shifts of visual attention. Vis. Res. 40 : 1489– 506 [Google Scholar]
- Itti L , Koch C. 2001 . Computational modelling of visual attention. Nat. Rev. Neurosci. 2 : 194– 203 [Google Scholar]
- Itti L , Koch C , Niebur E 1998 . A model of saliency-based visual attention for rapid scene analysis. IEEE Trans. Pattern Anal. Mach. Intell. 20 : 1254– 59 [Google Scholar]
- Jackson SL , Cook AJ , Miglioretti DL , Carney PA , Geller BM et al. 2012 . Are radiologists’ goals for mammography accuracy consistent with published recommendations. Acad. Radiol. 19 : 289– 95 [Google Scholar]
- Jans B , Peters JC , De Weerd P 2010 . Visual spatial attention to multiple locations at once: The jury is still out. Psychol. Rev. 117 : 637– 82 [Google Scholar]
- Jiang YV , Won B-Y , Swallow KM , Mussack DM 2014 . Spatial reference frame of attention in a large outdoor environment. J. Exp. Psychol. Hum. Percept. Perform. 40 : 1346– 57 [Google Scholar]
- Jonides J , Yantis S. 1988 . Uniqueness of abrupt visual onset in capturing attention. Percept. Psychophys. 43 : 346– 54 [Google Scholar]
- Jung K , Han SW , Min Y 2019 . Search efficiency is not sufficient: The nature of search modulates stimulus-driven attention. Atten. Percept. Psychophys. 81 : 61– 70 [Google Scholar]
- Kerzel D. 2019 . The precision of attentional selection is far worse than the precision of the underlying memory representation. Cognition 186 : 20– 31 [Google Scholar]
- Kim H , Anderson BA. 2018 . Dissociable components of experience-driven attention. Curr. Biol. 29 : 841– 45.e2 [Google Scholar]
- Kingsley HL. 1932 . An experimental study of ‘search. .’ Am. J. Psychol. 44 : 314– 18 [Google Scholar]
- Klein R. 1988 . Inhibitory tagging system facilitates visual search. Nature 334 : 430– 31 [Google Scholar]
- Klein R , Farrell M. 1989 . Search performance without eye movements. Percept. Psychophys. 46 : 476– 82 [Google Scholar]
- Klein RM , MacInnes WJ. 1999 . Inhibition of return is a foraging facilitator in visual search. Psychol. Sci. 10 : 346– 52 [Google Scholar]
- Koch C , Ullman S. 1985 . Shifts in selective visual attention: towards the underlying neural circuitry. Hum. Neurobiol. 4 : 219– 27 [Google Scholar]
- Kowler E , Anderson E , Dosher B , Blaser E 1995 . The role of attention in the programming of saccades. Vis. Res. 35 : 1897– 916 [Google Scholar]
- Kriegeskorte N. 2015 . Deep neural networks: a new framework for modeling biological vision and brain information processing. Annu. Rev. Vis. Sci. 1 : 417– 46 [Google Scholar]
- Kristjansson A. 2006 . Simultaneous priming along multiple feature dimensions in a visual search task. Vis. Res. 46 : 2554– 70 [Google Scholar]
- Kristjánsson Á , Egeth HE. 2020 . How feature integration theory integrated cognitive psychology, neurophysiology, and psychophysics. Atten. Percept. Psychophys. 82 : 7– 23 [Google Scholar]
- Kristjánsson T , Thornton IM , Kristjánsson Á 2018 . Time limits during visual foraging reveal flexible working memory templates. J. Exp. Psychol. Hum. Percept. Perform. 44 : 827– 35 [Google Scholar]
- Kruijne W , Meeter M. 2015 . The long and the short of priming in visual search. Atten. Percept. Psychophys. 77 : 1558– 73 [Google Scholar]
- Lamy D , Antebi C , Aviani N , Carmel T 2008 . Priming of pop-out provides reliable measures of target activation and distractor inhibition in selective attention. Vis. Res. 48 : 30– 41 [Google Scholar]
- Lamy D , Yashar A , Ruderman L 2013 . Orientation search is mediated by distractor suppression: evidence from priming of pop-out. Vis. Res. 81 : 29– 35 [Google Scholar]
- Li Z. 2002 . A salience map in primary visual cortex. Trends Cogn. Sci. 6 : 9– 16 [Google Scholar]
- Liesefeld HR , Müller HJ. 2019 . Distractor handling via dimension weighting. Curr. Opin. Psychol. 29 : 160– 67 [Google Scholar]
- Liesefeld H , Müller HJ. 2020 . A theoretical attempt to revive the serial/parallel-search dichotomy. Atten. Percept. Psychophys. 82 : 228– 245 [Google Scholar]
- Lleras A , Wang Z , Ng GJP , Ballew K , Xu J , Buetti S 2020 . A target contrast signal theory of parallel processing in goal-directed search. Atten. Percept. Psychophys. 82 : 394– 425 [Google Scholar]
- Maljkovic V , Nakayama K. 1994 . Priming of popout: I. Role of features. Mem. Cogn. 22 : 657– 72 [Google Scholar]
- McCarley JS , Wang RF , Kramer AF , Irwin DE , Peterson MS 2003 . How much memory does oculomotor search have. Psychol. Sci. 14 : 422– 26 [Google Scholar]
- Miconi T , Groomes L , Kreiman G 2016 . There's Waldo! A normalization model of visual search predicts single-trial human fixations in an object search task. Cereb. Cortex 26 : 3064– 82 [Google Scholar]
- Mitroff SR , Biggs AT , Adamo SH , Dowd EW , Winkle J , Clark K 2014 . What can 1 billion trials tell us about visual search. J. Exp. Psychol. Hum. Percept. Perform. 41 : 1– 5 [Google Scholar]
- Moran R , Zehetleitner M , Liesefeld H , Müller H , Usher M 2015 . Serial vs. parallel models of attention in visual search: accounting for benchmark RT-distributions. Psychon. Bull. Rev. 23 : 1300– 15 [Google Scholar]
- Moran R , Zehetleitner MH , Mueller HJ , Usher M 2013 . Competitive guided search: meeting the challenge of benchmark RT distributions. J. Vis. 13 : 24 [Google Scholar]
- Müller HJ , Heller D , Ziegler J 1995 . Visual search for singleton feature targets within and across feature dimensions. Percept. Psychophys. 57 : 1– 17 [Google Scholar]
- Najemnik J , Geisler WS. 2009 . Simple summation rule for optimal fixation selection in visual search. Vis. Res. 49 : 1286– 94 [Google Scholar]
- Navalpakkam V , Itti L. 2006 . Top-down attention selection is fine grained. J. Vis. 6 : 1180– 93 [Google Scholar]
- Neider MB , Zelinsky GJ. 2008 . Exploring set size effects in scenes: identifying the objects of search. Vis. Cogn. 16 : 1– 10 [Google Scholar]
- Neisser U. 1967 . Cognitive Psychology New York: Appleton, Century, Crofts [Google Scholar]
- Neumann E , DeSchepper BG. 1991 . Costs and benefits of target activation and distractor inhibition in selective attention. J. Exp. Psychol. Learn. Mem. Cogn. 17 : 1136– 45 [Google Scholar]
- Nobre AC , Kastner S. 2014 . Oxford Handbook of Attention Oxford, UK: Oxford Univ. Press [Google Scholar]
- Nodine CF , Krupinski EA , Kundel HL , Toto L , Herman GT 1992 . Satisfaction of search (SOS). Invest. Radiol. 27 : 571– 73 [Google Scholar]
- Nodine CF , Mello-Thoms C. 2019 . Acquiring expertise in radiologic image interpretation. The Handbook of Medical Image Perception and Techniques E Samei, EA Krupinski 139– 56 Cambridge, UK: Cambridge Univ. Press 2nd ed . [Google Scholar]
- Oliva A. 2005 . Gist of the scene. Neurobiology of Attention L Itti, G Rees, J Tsotsos 251– 57 New York: Academic [Google Scholar]
- Oliva A , Torralba A. 2001 . Modeling the shape of the scene: a holistic representation of the spatial envelope. Int. J. Comput. Vis. 42 : 145– 75 [Google Scholar]
- Olivers CN , Peters J , Houtkamp R , Roelfsema PR 2011 . Different states in visual working memory: when it guides attention and when it does not. Trends Cogn. Sci. 15 : 327– 34 [Google Scholar]
- Palmer EM , Van Wert MJ , Horowitz TS , Wolfe JM 2019 . Measuring the time course of selection during visual search. Atten. Percept. Psychophys. 81 : 47– 60 [Google Scholar]
- Palmer J. 1995 . Attention in visual search: distinguishing four causes of a set size effect. Curr. Dir. Psychol. Sci. 4 : 118– 23 [Google Scholar]
- Palmer J , McLean J. 1995 . Imperfect, unlimited-capacity, parallel search yields large set-size effects Paper presented at the Meeting of the Society for Mathematical Psychology Irvine, CA: [Google Scholar]
- Palmer J , Verghese P , Pavel M 2000 . The psychophysics of visual search. Vis. Res. 40 : 1227– 68 [Google Scholar]
- Pereira EJ , Castelhano MS. 2014 . Peripheral guidance in scenes: the interaction of scene context and object content. J. Exp. Psychol. Hum. Percept. Perform. 40 : 2056– 72 [Google Scholar]
- Pereira EJ , Castelhano MS. 2019 . Attentional capture is contingent on scene region: using surface guidance framework to explore attentional mechanisms during search. Psychon. Bull. Rev. 26 : 1273– 81 [Google Scholar]
- Posner MI. 1980 . Orienting of attention. Q. J. Exp. Psychol. 32 : 3– 25 [Google Scholar]
- Rajsic J , Ouslis NE , Wilson DE , Pratt J 2017 . Looking sharp: Becoming a search template boosts precision and stability in visual working memory. Atten. Percept. Psychophys. 79 : 1643– 51 [Google Scholar]
- Rangelov D , Muller HJ , Zehetleitner M 2011 . Dimension-specific intertrial priming effects are task-specific: evidence for multiple weighting systems. J. Exp. Psychol. Hum. Percept. Perform. 37 : 100– 14 [Google Scholar]
- Ratcliff R. 1978 . A theory of memory retrieval. Psychol. Rev. 85 : 59– 108 [Google Scholar]
- Ratcliff R , McKoon G. 2008 . The diffusion decision model: theory and data for two-choice decision tasks. Neural Comput 20 : 873– 922 [Google Scholar]
- Rensink RA. 2000 . Seeing, sensing, and scrutinizing. Vis. Res. 40 : 1469– 87 [Google Scholar]
- Rizzolatti G , Riggio L , Dascola I , Umilta C 1987 . Reorienting attention across the horizontal and vertical meridians: evidence in favor of a premotor theory of attention. Neuropsychologia 25 : 31– 40 [Google Scholar]
- Robbins CJ , Chapman P. 2018 . Drivers’ visual search behavior toward vulnerable road users at junctions as a function of cycling experience. Hum. Factors 60 : 889– 901 [Google Scholar]
- Rosenholtz R , Huang J , Ehinger KA 2012 . Rethinking the role of top-down attention in vision: effects attributable to a lossy representation in peripheral vision. Front. Psychol. 3 : 13 [Google Scholar]
- Rosenholtz RE. 2011 . What your visual system sees where you are not looking. Proc. SPIE: Human Vision and Electronic Imaging, XVI BE Rogowitz, TN Pappas, art. 786510 San Francisco, CA: SPIE [Google Scholar]
- Samei E , Krupinski EA 2018 . The Handbook of Medical Image Perception and Techniques Cambridge, UK: Cambridge Univ. Press. , 2nd ed.. [Google Scholar]
- Schill HM , Cain MS , Josephs EL , Wolfe JM 2020 . Axis of rotation as a basic feature in visual search. Atten. Percept. Psychophys. 82 : 31– 43 [Google Scholar]
- Schwarz W , Miller JO. 2016 . GSDT: an integrative model of visual search. J. Exp. Psychol. Hum. Percept. Perform. 42 : 1654– 75 [Google Scholar]
- Serences JT , Yantis S. 2006 . Selective visual attention and perceptual coherence. Trends Cogn. Sci. 10 : 38– 45 [Google Scholar]
- Sharan L , Rosenholtz R , Adelson EH 2014 . Accuracy and speed of material categorization in real-world images. J. Vis. 14 : 12 [Google Scholar]
- Shi Z , Allenmark F , Zhu X , Elliott MA , Müller HJ 2020 . To quit or not to quit in dynamic search. Atten. Percept. Psychophys. 82 : 799– 817 [Google Scholar]
- Soto D , Heinke D , Humphreys GW , Blanco MJ 2005 . Early, involuntary top-down guidance of attention from working memory. J. Exp. Psychol. Hum. Percept. Perform. 31 : 248– 61 [Google Scholar]
- Sternberg S. 1966 . High-speed scanning in human memory. Science 153 : 652– 54 [Google Scholar]
- Sully J. 1892 . The Human Mind: A Text-Book of Psychology New York: D. Appleton & Co. [Google Scholar]
- Theeuwes J. 2013 . Feature-based attention: It is all bottom-up priming. Philos. Trans. R. Soc. B 368 : 20130055 [Google Scholar]
- Theeuwes J. 2018 . Visual selection: usually fast and automatic; seldom slow and volitional. J. Cogn. 1 : 29 [Google Scholar]
- Thorpe S , Fize D , Marlot C 1996 . Speed of processing in the human visual system. Nature 381 : 520– 52 [Google Scholar]
- Treisman A. 1996 . The binding problem. Curr. Opin. Neurobiol. 6 : 171– 78 [Google Scholar]
- Treisman A , Gelade G. 1980 . A feature-integration theory of attention. Cogn. Psychol. 12 : 97– 136 [Google Scholar]
- Treue S. 2014 . Object- and feature-based attention: monkey physiology. Oxford Handbook of Attention AC Nobre, S Kastner 573– 600 Oxford, UK: Oxford Univ. Press [Google Scholar]
- Tsotsos J. 2011 . A Computational Perspective on Visual Attention Cambridge, MA: MIT Press [Google Scholar]
- Tsotsos JK , Culhane SN , Wai WYK , Lai Y , Davis N , Nuflo F 1995 . Modeling visual attention via selective tuning. Artif. Intell. 78 : 507– 45 [Google Scholar]
- Tsotsos JK , Eckstein MP , Landy MS 2015 . Computational models of visual attention. Vis. Res. 116 : Pt. B 93– 94 [Google Scholar]
- Tuddenham WJ. 1962 . Visual search, image organization, and reader error in roentgen diagnosis. Studies of the psycho-physiology of roentgen image perception. Radiology 78 : 694– 704 [Google Scholar]
- van Loon AM , Olmos-Solis K , Olivers CNL 2017 . Subtle eye movement metrics reveal task-relevant representations prior to visual search. J. Vis. 17 : 13 [Google Scholar]
- VanRullen R , Thorpe SJ. 2001 . Is it a bird? Is it a plane? Ultra-rapid visual categorisation of natural and artifactual objects. Perception 30 : 655– 68 [Google Scholar]
- Verghese P. 2001 . Visual search and attention: a signal detection approach. Neuron 31 : 523– 35 [Google Scholar]
- Vickery TJ , King L-W , Jiang Y 2005 . Setting up the target template in visual search. J. Vis. 5 : 81– 92 [Google Scholar]
- Vo ML , Wolfe JM. 2013 . Differential ERP signatures elicited by semantic and syntactic processing in scenes. Psychol. Sci. 24 : 1816– 23 [Google Scholar]
- Vo MLH , Henderson JM. 2009 . Does gravity matter? Effects of semantic and syntactic inconsistencies on the allocation of attention during scene perception. J. Vis. 9 : 24 [Google Scholar]
- von der Malsburg C. 1981 . The correlation theory of brain function. Models of Neural Networks , Vol. II : Temporal Aspects of Coding and Information Processing in Biological Systems E Domany, JL van Hemmen, K Schulten 95– 119 Berlin: Springer [Google Scholar]
- von Muhlenen A , Muller HJ , Muller D 2003 . Sit-and-wait strategies in dynamic visual search. Psychol. Sci. 14 : 309– 14 [Google Scholar]
- Vul E , Rieth C , Lew TF , Rich AN 2020 . The structure of illusory conjunctions reveals hierarchical binding of multi-part objects. Atten. Percept. Psychophys. 82 : 550– 63 [Google Scholar]
- Williams L , Drew T. 2019 . What do we know about volumetric medical image search? A review of the basic science and medical image perception literatures. Cogn. Res. Princ. Implic. 4 : 21 [Google Scholar]
- Wolfe JM. 1994 . Guided Search 2.0: a revised model of visual search. Psychon. Bull. Rev. 1 : 202– 38 [Google Scholar]
- Wolfe JM. 1998a . Visual search. Attention H Pashler 13– 74 Hove, UK: Psychol. Press [Google Scholar]
- Wolfe JM. 1998b . What do 1,000,000 trials tell us about visual search. Psychol. Sci. 9 : 33– 39 [Google Scholar]
- Wolfe JM. 2003 . Moving towards solutions to some enduring controversies in visual search. Trends Cogn. Sci. 7 : 70– 76 [Google Scholar]
- Wolfe JM. 2007 . Guided Search 4.0: current progress with a model of visual search. Integrated Models of Cognitive Systems W Gray 99– 119 Oxford, UK: Oxford Univ. Press [Google Scholar]
- Wolfe JM. 2012 . Saved by a log: How do humans perform hybrid visual and memory search. Psychol. Sci. 23 : 698– 703 [Google Scholar]
- Wolfe JM. 2013 . When is it time to move to the next raspberry bush? Foraging rules in human visual search. J. Vis. 13 : 10 [Google Scholar]
- Wolfe JM. 2014 . Approaches to visual search: feature integration theory and guided search. Oxford Handbook of Attention AC Nobre, S Kastner 11– 55 Oxford, UK: Oxford Univ. Press [Google Scholar]
- Wolfe JM. 2016 . Use-inspired basic research in medical image perception. Cogn. Res. Princ. Implic. 1 : 17 [Google Scholar]
- Wolfe JM. 2017 . Visual attention: size matters. Curr. Biol. 27 : R1002– 3 [Google Scholar]
- Wolfe JM. 2018 . Visual search. Stevens’ Handbook of Experimental Psychology and Cognitive Neuroscience J Wixted 569– 623 Hoboken, NJ: Wiley [Google Scholar]
- Wolfe JM , Alvarez GA , Rosenholtz R , Kuzmova YI , Sherman AM 2011a . Visual search for arbitrary objects in real scenes. Atten. Percept. Psychophys. 73 : 1650– 71 [Google Scholar]
- Wolfe JM , Brunelli DN , Rubinstein J , Horowitz TS 2013 . Prevalence effects in newly trained airport checkpoint screeners: Trained observers miss rare targets, too. J. Vis. 13 : 33 [Google Scholar]
- Wolfe JM , Butcher SJ , Lee C , Hyle M 2003 . Changing your mind: on the contributions of top-down and bottom-up guidance in visual search for feature singletons. J. Exp. Psychol. Hum. Percept. Perform. 29 : 483– 502 [Google Scholar]
- Wolfe JM , Cain MS , Ehinger KA , Drew T 2015 . Guided Search 5.0: meeting the challenge of hybrid search and multiple-target foraging Paper presented at the Annual Meeting of the Vision Science Society St. Petersburg, FL: May 15– 20 [Google Scholar]
- Wolfe JM , Cave KR , Franzel SL 1989 . Guided Search: an alternative to the Feature Integration model for visual search. J. Exp. Psychol. Hum. Percept. Perform. 15 : 419– 33 [Google Scholar]
- Wolfe JM , Evans KK , Drew T 2018 . The first moments of medical image perception. The Handbook of Medical Image Perception and Techniques E Samei, EA Krupinski 188– 96 Cambridge, UK: Cambridge Univ. Press [Google Scholar]
- Wolfe JM , Friedman-Hill SR , Bilsky AB 1994 . Parallel processing of part/whole information in visual search tasks. Percept. Psychophys. 55 : 537– 50 [Google Scholar]
- Wolfe JM , Friedman-Hill SR , Stewart MI , O'Connell KM 1992 . The role of categorization in visual search for orientation. J. Exp. Psychol. Hum. Percept. Perform. 18 : 34– 49 [Google Scholar]
- Wolfe JM , Gancarz G. 1996 . Guided Search 3.0: a model of visual search catches up with Jay Enoch 40 years later. Basic and Clinical Applications of Vision Science V Lakshminarayanan 189– 92 Dordrecht, Neth.: Kluwer Acad. [Google Scholar]
- Wolfe JM , Horowitz TS. 2004 . What attributes guide the deployment of visual attention and how do they do it. Nat. Rev. Neurosci. 5 : 495– 501 [Google Scholar]
- Wolfe JM , Horowitz TS. 2017 . Five factors that guide attention in visual search. Nat. Hum. Behav. 1 : 0058 [Google Scholar]
- Wolfe JM , Horowitz TS , Kenner NM 2005 . Rare targets are often missed in visual search. Nature 435 : 439– 40 [Google Scholar]
- Wolfe JM , Horowitz TS , Palmer EM , Michod KO , VanWert MJ 2010a . Getting into Guided Search. Tutorials in Visual Cognition V Coltheart 93– 120 Hove, UK: Psychol. Press [Google Scholar]
- Wolfe JM , Myers L. 2010 . Fur in the midst of the waters: visual search for material type is inefficient. J. Vis. 10 : 8 [Google Scholar]
- Wolfe JM , Palmer EM , Horowitz TS 2010b . Reaction time distributions constrain models of visual search. Vis. Res. 50 : 1304– 11 [Google Scholar]
- Wolfe JM , Van Wert MJ 2010 . Varying target prevalence reveals two dissociable decision criteria in visual search. Curr. Biol. 20 : 121– 24 [Google Scholar]
- Wolfe JM , Vo ML , Evans KK , Greene MR 2011b . Visual search in scenes involves selective and nonselective pathways. Trends Cogn. Sci. 15 : 77– 84 [Google Scholar]
- Wu C-C , Wolfe JM. 2019 . Eye movements in medical image perception: a selective review of past, present and future. Vision 3 : 32 [Google Scholar]
- Yantis S. 1993 . Stimulus-driven attentional capture. Curr. Dir. Psychol. Sci. 2 : 156– 61 [Google Scholar]
- Yu X , Geng JJ. 2019 . The attentional template is shifted and asymmetrically sharpened by distractor context. J. Exp. Psychol. Hum. Percept. Perform. 45 : 336– 53 [Google Scholar]
- Zelinsky G. 2008 . A theory of eye movements during target acquisition. Psychol. Rev. 115 : 787– 835 [Google Scholar]
- Zelinsky GJ , Sheinberg DL. 1997 . Eye movements during parallel/serial visual search. J. Exp. Psychol. Hum. Percept. Perform. 23 : 244– 62 [Google Scholar]
Data & Media loading...
- Article Type: Review Article
Most Read This Month
Most cited most cited rss feed, deep neural networks: a new framework for modeling biological vision and brain information processing, a revised neural framework for face processing, capabilities and limitations of peripheral vision, visual adaptation, microglia in the retina: roles in development, maturity, and disease, circuits for action and cognition: a view from the superior colliculus, neuronal mechanisms of visual attention, the functional neuroanatomy of human face perception, scene perception in the human brain, the organization and operation of inferior temporal cortex.
Foraging behavior in visual search: A review of theoretical and mathematical models in humans and animals
- Published: 21 March 2021
- Volume 86 , pages 331–349, ( 2022 )
Cite this article
- Marcos Bella-Fernández ORCID: orcid.org/0000-0001-6621-0199 1 , 2 ,
- Manuel Suero Suñé 1 &
- Beatriz Gil-Gómez de Liaño 3
2122 Accesses
13 Citations
5 Altmetric
Explore all metrics
Visual search (VS) is a fundamental task in daily life widely studied for over half a century. A variant of the classic paradigm—searching one target among distractors—requires the observer to look for several (undetermined) instances of a target (so-called foraging) or several targets that may appear an undefined number of times (recently named as hybrid foraging). In these searches, besides looking for targets, the observer must decide how much time is needed to exploit the area, and when to quit the search to eventually explore new search options. In fact, visual foraging is a very common search task in the real world, probably involving additional cognitive functions than typical VS. It has been widely studied in natural animal environments, for which several mathematical models have been proposed, and just recently applied to humans: Lévy processes, composite and area-restricted search models, marginal value theorem, and Bayesian learning (among others). We conducted a systematic search in the literature to understand those mathematical models and study its applicability in human visual foraging. The review suggests that these models might be the first step, but they seem to be limited to fully comprehend foraging in visual search. There are essential variables involving human visual foraging still to be established and understood. Indeed, a jointly theoretical interpretation based on the different models reviewed could better account for its understanding. In addition, some other relevant variables, such as certain individual differences or time perception might be crucial to understanding visual foraging in humans.
This is a preview of subscription content, log in via an institution to check access.
Access this article
Subscribe and save.
- Get 10 units per month
- Download Article/Chapter or Ebook
- 1 Unit = 1 Article or 1 Chapter
- Cancel anytime
Price excludes VAT (USA) Tax calculation will be finalised during checkout.
Instant access to the full article PDF.
Rent this article via DeepDyve
Institutional subscriptions
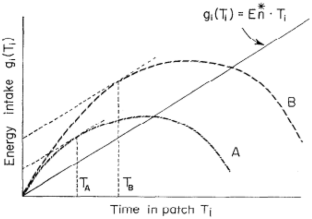
Reprinted from Charnov, E. L. (1976) Optimal Foraging, the Marginal Value Theorem. Theoretical Population Biology, 9(2), p. 132. Copyright by Elsevier. Reprinted with permission
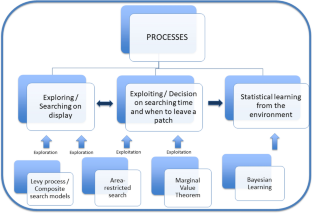
Similar content being viewed by others
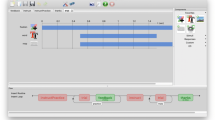
PsychoPy2: Experiments in behavior made easy
The interface theory of perception.
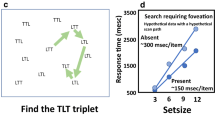
Guided Search 6.0: An updated model of visual search
Adachi, T., Costa, D. P., Robinson, P. W., Peterson, S. H., Yamamichi, M., Naito, Y., & Takahashi, A. (2017). Searching for prey in a three‐dimensional environment: Hierarchical movements enhance foraging success in northern elephant seals. Functional Ecology, 31 (2), 361–369.
Google Scholar
Adler, F. R., & Kotar, M. (1999). Departure time versus departure rate: How to forage optimally when you are stupid. Evolutionary Ecology Research, 1, 411–421.
Ahmed, L., & de Fockert, J. W. (2012). Focusing on attention: The effects of working memory capacity and load on selective attention. PLoS ONE, 7 (8), e43101.
PubMed PubMed Central Google Scholar
Alonso, J. C., Alonso, J. A., Bautista, L. M., & Muñoz-Pulido, R. (1995). Patch use in cranes: A field test of optimal foraging predictions. Animal Behavior, 49, 1367–1379.
Aplin, L. M., Farine, D. R., Mann, R. P., & Sheldon, B. C. (2014). Individual-level personality influences social foraging and collective behavior in wild birds. Proceedings of the Royal Society B, 281, 20141016.
Arkes, H. R., & Ayton, P. (1999). The sunk cost and Concorde effects: Are humans less rational than lower animals? Psychological Bulletin, 125 (5), 591–600.
Arkes, H. R., & Blumer, C. (1985). The psychology of sunk cost. Organizational Behavior and Human Decision Processes, 35 (1), 124–140.
Aswani, S. (1998). Patterns of marine harvest effort in southwestern New Georgia, Solomon Islands: Resource management or optimal foraging? Ocean & Coastal Management, 40 (2–3), 207–235.
Auger-Méthé, M., Derocher, A. E., DeMars, C. A., Plank, M. J., Codling, E. A., & Lewis, M. A. (2016). Evaluating random search strategies in three mammals from distinct feed guilds. Journal of Animal Ecology, 85 (5), 1411–1421.
Auger-Méthé, M., Derocher, A., Plank, M. J., Codling, E., & Lewis, M. A. (2015). Differentiating the Lévy walk from a composite correlated random walk. Methods in Ecology and Evolution, 6, 1179–1189.
Bailey, H., Lyubchich, V., Wingfield, J., Fandel, A., Garrod, A., & Rice, A. N. (2019). Empirical evidence that large marine predator foraging behavior is consistent with area-restricted search theory. Ecology, 100 (8), e02743.
PubMed Google Scholar
Baronchelli, A., & Radicchi, F. (2013). Lévy flights in human behavior and cognition. Chaos, Solitons & Fractals, 56, 101–105.
Bartumeus, F. (2007). Lévy processes in animal movement: An evolutionary hypothesis. Fractals, 15 (2), 151–162.
Bartumeus, F., Raposo, E., Viswanathan, G. M., & Da Luz, M. (2014). Stochastic optimal foraging: Tuning intensive and extensive dynamics in random searches. PLoS ONE, 9 (9), e106373.
Baumann, C., Singmann, H., Gershman, S. J., & Von Helversen, B. (2020). A linear threshold model for optimal behavior model. Proceedings of the National Academy of Sciences, 117 (23), 12750–12755.
Benedix, J. H. (1993). Area-restricted search by the plains pocket gopher ( Geomys bursarius ) in tallgrass prairie habitat. Behavioral Ecology, 4 (4), 318–324.
Benhamou, S. (2007). How many animals really do the Lévy walk? Ecology, 88, 1962–1969.
Benhamou, S., & Collet, J. (2015). Ultimate failure of the Lévy Foraging Hypothesis: Two-scale searching strategies outperform scale-free ones even when prey are scarce and cryptic. Journal of Theoretical Biology, 387, 221–227.
Bennison, A., Quinn, J. L., Debney, A., & Jessop, M. (2019). Tidal drift removes the need for area-restricted search in foraging Atlantic puffins. Biology Letters, 15 (7), 20190208.
Bertrand, S., Bertrand, A., Guevara-Carrasco, R., & Gerlotto, F. (2007). Scale-invariant movements of fishermen: The same foraging strategy as natural predators. Ecological Applications, 17 (2), 331–337.
Bettinger, R. L., & Grote, M. N. (2016). Marginal value theorem, patch choice, and human foraging response in varying environments. Journal of Anthropolical Archaeology, 42, 79–87.
Biernaskie, J. M., Walker, S. C., & Gegear, R. J. (2009). Bumblebees learn to forage like Bayesians. The American Naturalist, 174 (3), 413–423.
Biggs, A. T. (2017). Getting satisfied with “satisfaction of search”: How to measure errors during multiple-target search. Attention, Perception & Psychophysics, 79, 1353–1365.
Biggs, A. T., Clark, K., & Mitroff, S. R. (2017). Who should be searching? Differences in personality can affect visual search accuracy. Personality and Individual Differences, 116, 353–358.
Bixter, M. T., & Luhmann, C. C. (2013). Adaptive intertemporal preferences in foraging-style environments. Frontiers in Neuroscience, 7, 93.
Boccignone, G., & Ferraro, M. (2004). Modelling gaze shift as a constrained random walk. Physica A: Statistical Mechanics and its Applications, 331, 207–218.
Bowers, J. S., & Davis, C. J. (2012). Bayesian just-so stories in psychology and neuroscience. Psychological Bulletin, 138 (3), 389–414.
Brockmann, D., & Geisel, T. (2000). The ecology of gaze shifts. Neurocomputing, 32–33, 643–650.
Brown, C. T., Liebovitch, L. S., & Glendon, R. (2007). Lévy flights in dobe ju/’hoansi foraging patterns. Human Ecology, 35 (1), 129–138.
Cain, M. S., Vul, E., Clark, K., & Mitroff, S. R. (2012). A Bayesian optimal foraging model of human visual search. Psychological Science, 23 (9), 1047–1054.
Cassini, M. H., Kacelnik, A., & Segura, E. T. (1990). The tale of the screaming hairy armadillo, the guinea pig and the marginal value theorem. Animal Behavior, 39, 1030–1050.
Cassini, M. H., Lichtenstein, G., Ongay, J. P., & Kacelnik, A. (1993). Foraging behavior in guinea pigs: Further tests of the marginal value theorem. Behavioral Processes, 29, 99–112.
Charnov, E. L. (1976). Optimal foraging, the marginal value theorem. Theoretical Population Biology, 9 (2), 129–136.
Constantino, S. M., & Daw, N. D. (2015). Learning the opportunity cost time in a patch-foraging task. Cognitive, Affective and Behavioral Neuroscience, 15 (4), 837–853.
Cowie, R. J. (1977). Optimal foraging in great tits (Parus major). Nature, 268, 137–139.
Crook, K. A., & Davoren, G. K. (2014). Underwater behaviour of common murres foraging on capelin: Influences of prey density and antipredator behaviour. Marine Ecology Progress Series, 501, 279–290.
Cunha, M., & Caldieraro, F. (2009). Sunk-cost effects on purely behavioral investments. Cognitive Science, 33, 105–113.
Cuthill, I. C., Haccou, P., & Kacelnik, A. (1994). Starlings ( Sturnus vulgaris ) exploiting patches: Response to long-term changes in travel time. Behavioral Ecology, 5 (1), 81–90.
Da Silveira, N. S., Niebuhr, B. B. S., Muylaert, R. L., Ribeiro, M. C., & Pizo, M. A. (2016). Effects of land cover on the movement of frugivorous birds in a heterogeneous landscape. PLoS ONE, 11 (6), e0156688.
Dall, S. R. X., Giraldeau, L. A., Olsson, O., McNamara, J., & Stephens, D. W. (2005). Information and its use by animals in evolutionary ecology. Trends in Ecology and Evolution, 20 (4), 187–193.
Davidson, J. D., & El-Hadi, A. (2019). Foraging as an evidence accumulation process. PLoS Computational Biology, 15 (7), e1007060.
Dawkins, R., & Carlisle, T. R. (1976). Parental investment, mate desertion, and a fallacy. Nature, 262, 131–133.
De Knegt, H. J., Hengeveld, H. J., van Langevelde, F., de Boer, W. F., & Kirkman, K. P. (2007). Patch density determines movement patterns and foraging efficiency of large herbivores. Behavioral Ecology, 18 (6), 1065–1072.
Devries, D. R., Stein, R. A., & Chesson, P. L. (1989). Sunfish foraging among patches: The patch-departure decision. Animal Behavior, 37, 455–464.
Edwards, A. M. (2011). Overturning conclusions of Lévy flight movement patterns by fishing boats and foraging animals. Ecology, 92 (6), 1247–1257.
Edwards, A. M., Phillips, R. A., Watkins, N. W., Freeman, M. P., Murphy, E. J., Afanasyev, V., Buldyrev, S. V., da Luz, M. G. E., Raposo, E. P., Stanley, H. E., & Viswanathan, G. M. (2007). Revisiting Lévy flights search patterns of wandering albatrosses, bumblebees and deer. Nature, 449, 1044–1045.
Ehinger, K. A., & Wolfe, J. M. (2016). When is it time to move to the next map? Optimal foraging in guided visual search. Attention, Perception & Psychophysics, 78, 2135–2151.
Eliassen, S. (2006) Foraging ecology and learning. Adaptive behavioral strategies and the value of information (Doctoral Thesis, University of Bergen, Norway). Recovered from https://users.soe.ucsc.edu/~msmangel/Eliassen%20Thesis.pdf
Eliassen, S., Jorgensen, C., Mangel, M., & Giske, J. (2007). Exploration or exploitation: Life expectancy changes the value of learning in foraging strategies. Oikos, 116 (3), 513–523.
Eliassen, S., Jorgensen, C., Mangel, M., & Giske, J. (2009). Quantifying the adaptive value of learning in foraging behavior. The American Naturalist, 174 (4), 478–489.
Fauchald, P., & Tveraa, T. (2003). Using first-passage time in the analysis of area-restricted search and habitat selection. Ecology, 84 (2), 282–288.
Ferguson, T. S. (1989). Who solved the secretary problem? Statistical Science, 4 (3), 282–296.
Ferreira, A. S., Raposo, E. P., Viswanathan, G. M., & Da Luz, M. G. E. (2014). The influence of environment on Lévy ransom search efficiency: Fractality and memory effects. Physica A: Statistical Mechanics and its Applications, 391 (11), 3234–3246.
Fougnie, D., Cormiea, S. M., Zhang, J., Alvarez, G. A., & Wolfe, J. M. (2015). Winter is coming: How humans forage in a temporally structured environment. Journal of Vision, 15 (11), 1–11.
Franken, I. H. A., van Strien, J. W., Nijs, I., & Muris, P. (2008). Impulsivity is associated with behavioral decision-making processes. Psychiatry Research, 158 (2), 155–163.
Fronhofer, E. A., Hovestadt, T., & Poethke, H. J. (2013). From random walks to informed movement. Oikos, 122 (6), 857–866.
Fu, W. T. (2012). From Plato to the world wide web: Information foraging on the internet. In P. M. Todd, T. T. Hills, & T. W. Robbins (Eds.), Cognitive search: Evolution, algorithms, and the brain (pp. 283–299). MIT Press.
Gil-Gómez de Liaño, B., Quirós-Godoy, M., Pérez-Hernández, E., Cain, M., & Wolfe, J. M. (2018). Understanding visual search and foraging in cognitive development. Journal of Vision, 18 (10), 635.
Gil-Gómez de Liaño, B., Quirós-Godoy, M., Pérez-Hernández, E., & Wolfe, J. M. (2020). Efficiency and accuracy of visual search develop at different rates from early childhood through early adulthood. Psychonomic Bulletin & Review, 27 (3), 504–511.
Giraldeau, L. A., & Kramer, D. L. (1982). The marginal value theorem: A quantitative test using load size variation in a central place forager, the Eastern chipmunk, Tamias striatus. Animal Behavior, 30, 1036–1042.
Green, R. F. (1980). Bayesian birds: A simple example of Oaten’s stochastic model of optimal foraging. Theoretical Population Biology, 18, 244–256.
Green, R. F. (1984). Stopping rules for optimal foragers. The American Naturalist, 123, 30–40.
Grobelny, J., Michalski, R., & Weron, R. (2015) Is human visual activity in simple human-computer interaction search tasks a Lévy flight? In Proceedings of the 2nd international conference on physiological computing systems (pp. 67–71).
Grondin, S. (2010). Timing and time perception: A review of recent behavioral and neuroscience findings and theoretical directions. Attention, Perception & Psychophysics, 72 (3), 561–582.
Hamer, K. C., Humphreys, E. M., Magalhaes, M. C., Garthe, S., Hennicke, J., Peters, G., Gremillet, D., Skov, H., & Wanless, S. (2009). Fine-scale foraging behaviour of a medium-ranger marine predator. Journal of Animal Ecology, 78 (4), 880–889.
Haskell, D. G. (1997). Experiments and a model examining learning in the area-restricted search behavior of ferrets ( Mustela putorius furo). Behavioral Ecology, 8 (4), 448–455.
Hayward, M. W., Ortmann, S., & Kowalczyk, R. (2015). Risk perception by endangered European bison Bison bonasus is context (condition) dependent. Landscape Ecology, 30 (10), 2079–2093.
Hemingway, C. T., Ryan, M. J., & Page, R. A. (2018). Cognitive constraints on optimal foraging in frog-eating bats. Animal Behavior, 143, 43–50.
Higginson, A. D., Fawcett, T. W., Houston, A. I., & McNamara, J. M. (2018). Trust your gut: Using physiological states as a source of information is almost as effective as optimal Bayesian learning. Proceedings of the Royal Society B, 285, 20172411.
Hill, S., Burrows, M. T., & Hughes, R. N. (2002). Adaptive search in juvenile plaice foraging for aggregated and dispersed prey. Journal of Fish Biology, 61 (5), 1255–1267.
Hills, T. T. (2006). Animal foraging and the evolution of goal-directed cognition. Cognitive Science, 30, 3–41.
Hills, T. T., & Adler, F. R. (2002). Time’s crooked arrow: Optimal foraging and rate-biased time perception. Animal Behaviour, 64 (4), 589–597.
Hills, T. T., & Hertwig, R. (2010). Information search in decisions from experience: Do our patterns of sampling foreshadow our decisions? Psychological Science, 21 (12), 1787–1792.
Hills, T. T., Jones, M. N., & Todd, P. M. (2012). Optimal foraging in semantic memory. Psychological Review, 119 (2), 431–440.
Hills, T. T., Kallf, C., & Wiener, J. M. (2013). Adaptive Lévy processes and area-restricted search in human foraging. PLoS ONE, 8 (4), e60488.
Hills, T. T., Todd, P. M., Lazer, D., Redish, A. D., Couzin, I. D., & The Cognitive Research Group. (2015). Exploration versus exploitation in space, mind, and society. Trends in Cognitive Sciences, 19 (1), 46–54.
Humphries, N. E., Queiroz, N., Ryer, J. R. M., Pade, N. G., Musyl, M. K., Schaefer, K. M., Fuller, D. W., Brunnschweiler, J. M., Doyle, T. K., Houghtom, J. D. R., Hays, G. C., Jones, C. S., Noble, L. R., Wearmouth, V. J., Southall, E. J., & Sims, D. W. (2010). Environmental context explains Lévy and Brownian movement patterns of marine predators. Nature, 465, 1066–1069.
Humphries, N. E., Schaefer, K. M., Fuller, D. W., Phillips, G. E. M., Wilding, C., & Sims, D. W. (2016). Scale-dependent to scale-free: Daily behavioral switching and optimized searching in a marine predator. Animal Behavior, 113, 189–201.
Humphries, N. E., & Sims, D. W. (2014). Optimal foraging strategies: Lévy walks balance searching and patch exploitation under a very broad range of conditions. Journal of Theoretical Biology, 358, 179–193.
Humphries, N. E., Weimerskirch, H., Queiroz, N., Southall, E. J., & Sims, D. W. (2012). Foraging success of biological Lévy flights recorded in situ. Proceedings of the National Academy of Sciences of the United States of America, 109 (19), 7169–7174.
Hutchinson, J. M. C., Stephens, D. W., Bateson, M., Couzin, I., Dukas, R., Giraldeau, L. A., Hills, T. T., Méry, F., & Winterhalder, B. (2012). Searching for fundamentals and commonalities of search. In P. M. Todd, T. T. Hills, & T. W. Robbins (Eds.), Cognitive Search: Evolution, algorithms, and the brain (pp. 47–65). MIT Press.
Hutchinson, J. M. C., Wilke, A., & Todd, P. M. (2008). Patch leaving in humans: Can a generalist adapt its rules to dispersal across patches? Animal Behavior, 75, 1331–1349.
Jacobs, R. A., & Kruschke, J. K. (2011). Bayesian learning theory applied to human cognition. Cognitive Science, 2 (1), 8–21.
Johánnesson, Ó. I., Kristjánsson, Á., & Thornton, I. M. (2017). Are foraging patterns related to working memory and inhibitory control? Japanese Psychological Research, 59 (2), 152–166.
Jones, M., & Love, B. C. (2011). Bayesian fundamentalism or enlightenment? On the explanatory status and theoretical contributions of Bayesian models of cognition. Behavioral and Brain Sciences, 34 (4), 169–188.
Kacelnik, A., & Marsh, B. (2002). Cost can increase preference in starlings. Animal Behaviour, 63 (2), 245–250.
Kagan, E., & Ben-Gal, I. (2015). Search and foraging: Individual motion and swarm dynamics . CRC Press.
Kallf, C., Hills, T. T., & Wiener, J. M. (2010). Human foraging behavior: A virtual reality investigation on area restricted search in humans. Proceedings of the Annual Meeting of the Cognitive Sciences Society, 32 (32), 168–173.
Kareiva, P., & Odell, G. (1987). Swarms of predators exhibit “preytaxis” if individual predators use area-restricted search. The American Naturalist, 130 (2), 233–270.
Keasar, T., Shmida, A., & Motro, U. (1996). Innate movement rules in foraging bees: Flight distances are affected by recent rewards and are correlated with choice of flower type. Behavioral Ecology and Sociobiology, 39 (6), 381–388.
Killeen, P. R., Palombo, G. M., Gottlob, L. R., & Beam, J. (1996). Bayesian analysis of foraging by pigeons ( Columba livia ). Journal of Experimental Psychology: Animal Behavior Processes, 22 (4), 480–496.
Killeen, P. R., Smith, J. P., & Hanson, S. J. (1981). Central place foraging in Rattus norvegicus . Animal Behavior, 29, 64–70.
Knill, D. C., & Pouget, A. (2004). The Bayesian brain: the role of uncertainty in neural coding and computation. Trends in Neurosciences, 27 (2), 712–719.
Koelega, H. S. (1992). Extraversion and vigilance: 30 years of inconsistencies. Psychological Bulletin, 112 (2), 239–258.
Kölzsch, A., Alzate, A., Bartumeus, F., de Jager, M., Weerman, E. J., Hengeveld, G. M., Naguib, M., Nolet, B. A., & van de Koppel, J. (2015). Experimental evidence for inherent Lévy search behaviour in foraging animals. Proceedings of the Royal Society B, 282, 20150424.
Krebs, J. R., Ryan, J. C., & Charnov, E. L. (1974). Hunting by expectation or optimal foraging? A study of patch use by chickadees. Animal Behavior, 22, 953–964.
Kristjánsson, Á. (2000). In search of remembrance: Evidence for memory in visual search. Psychological Science, 11 (4), 328–332.
Kristjánsson, Á., Björnsson, A. S., & Kristjánsson, T. (2020). Foraging with Anne Treisman: Features versus conjunctions, patch leaving and memory for foraged locations. Attention, Perception, & Psychophysics, 82, 818–831.
Kristjánsson, Á., Johánnesson, Ó. I., & Thornton, I. M. (2014). Common attentional constraints in visual foraging. PLoS ONE, 9 (6), e100752.
Kristjánsson, Á., Ólafsdóttir, I. M., & Kristjánsson, T. (2019). Visual foraging tasks provide new insights into the orienting of visual attention: Methodological considerations. In S. Pollmann (Ed.), Spatial learning and attentional guidance (pp. 3–21). Humana.
Kristjánsson, T., & Kristjánsson, Á. (2018). Foraging through multiple targets reveals the flexibility of visual working memory. Acta Psychologica, 183, 108–115.
Kristjánsson, T., Thornton, I. M., Chetverikov, A., & Kristjánsson, Á. (2020). Dynamics of visual attention revealed in foraging tasks. Cognition, 194, 104032.
Kristjánsson, T., Thornton, I. M., & Kristjánsson, Á. (2018). Time limits during visual foraging reveal flexible working memory templates. Journal of Experimental Psychology: Human Perception and Performance, 44 (6), 827–835.
Lee, M. D., & Wagenmakers, E. J. (2013). Bayesian cognitive models . University Press.
Leising, A. W., & Franks, P. J. S. (2002). Does Acartia clausi (Copepoda Calanoida) use an area-restricted search foraging strategy to find food? Hydrobiologia, 480 (1–3), 193–207.
Lenow, J. K., Constantino, S. M., Daw, N. D., & Phelps, E. A. (2017). Chronic and acute stress promote overexploitation in serial decision making. The Journal of Neuroscience, 37 (23), 5681–5689.
Lihoreau, M., Ings, T. C., Chittka, L., & Reynolds, A. M. (2016). Signatures of a global optimal searching strategy in the three-dimensional foraging flights of bumblebees. Scientific Reports, 6, 30401.
Lode, T. (2000). Functional response and area-restricted search in a predator: Seasonal exploitation of anurans by the European polecat, Mustela putorius . Austral Ecology, 25 (3), 223–231.
Magalhaes, P., & White, K. G. (2014). The effect of a prior investment on choice: The sunk cost effect. Journal of Experimental Psychology: Animal Learning and Cognition, 40 (1), 22–37.
Marcus, G. F., & Davis, E. (2013). How robust are probabilistic models of higher-level cognition? Psychological Science, 24 (12), 2351–2360.
Marell, A., Ball, J. P., & Hofgaard, A. (2002). Foraging and movement paths of female reindeer: Insights from fractal analysis, correlated random walks, and Lévy flights. Canadian Journal of Zoology, 80 (5), 854–865.
Marshall, H. H., Carter, A. J., Ashford, A., Rowcliffe, J. M., & Cowlishaw, G. (2013). How do foragers decide when to leave a patch? A test of alternative models under natural and experimental conditions. Journal of Animal Ecology, 82, 894–902.
Mata, R., Wilke, A., & Czienskowski, U. (2009). Cognitive aging and adaptive foraging behavior. Journal of Gerontology: Psychological Sciences, 64B (4), 474–481.
Mata, R., Wilke, A., & Czienskowski, U. (2013). Foraging across the life span: Is there a reduction in exploration with aging? Frontiers in Neuroscience, 7, 53.
Mazur, J. E., & Vaughan, W. (1987). Molar optimization versus delayed reinforcement as explanations of choice between fixed-ratio and progressive-ratio schedules. Journal of the Experimental Analysis of Behavior, 48, 251–261.
McArthur, R. H., & Pianka, E. R. (1966). On optimal use of a patchy environment. The American Naturalist, 100, 603–609.
McEvoy, J. F., Hall, G. P., & McDonald, P. G. (2019). Movements of Australian Wood Ducks ( Chenonetta jubata ) in an agricultural landcape. Emu-Austral Ornithology, 119 (2), 147–156.
McNair, J. N. (1982). Optimal giving-up time rules and the marginal value theorem. The American Naturalist, 119 (4), 511–529.
McNamara, J. (1982). Optimal patch use in a stochastic environment. Theoretical Population Biology, 21, 269–288.
McNamara, J., Green, R., & Olsson, O. (2006). Bayes’ theorem and its application in animal behavior. Oikos, 112, 243–251.
McNamara, J., & Houston, A. I. (1980). The application of statistical decision theory to animal behavior. Journal of Theoretical Biology, 85, 673–690.
Mehlhorn, K., Newell, B. R., Todd, P. M., Lee, M. D., Morgan, K., Braithwaite, V. A., Hausmann, D., Fiedler, K., & Gonzalez, C. (2015). Unpacking the exploration-exploitation tradeoff: A synthesis of human an animal literatures. Decision, 2 (3), 191.
Mekern, V. N., Sjoerds, Z., & Hommel, B. (2019). How metacontrol biases and adaptivity impact performance in cognitive search tasks. Cognition, 182, 251–259.
Miramontes, O., De Souza, O., Hernández, D., & Ceccon, E. (2012). Non-Lévy mobility patterns of Mexican Me’Phaa peasants searching for fuel wood. Human Ecology, 40 (2), 167–174.
Newton, T., Slade, P., Butler, N., & Murphy, P. (1992). Personality and performance on a simple visual search task. Personality and Individual Differences, 13 (3), 381–382.
Nolet, B. A., & Mooij, W. M. (2002). Search paths of swans foraging on spatially autocorrelated tubers. Journal of Animal Ecology, 71 (3), 451–462.
Nolting, B. C. (2013) Random search models of foraging behavior: Theory, simulation, and observation. Doctoral Dissertation. University of Nebraska-Lincoln.
Nolting, B. C., Hinkelman, T. M., Brassil, C. E., & Tehumberg, B. (2015). Composite random search strategies based on non-directional sensory cues. Ecological Complexity, 22, 126–138.
Nonacs, P. (2001). State dependent behavior and the marginal value theorem. Behavioral Ecology, 12 (1), 71–83.
Nonacs, P., & Soriano, J. L. (1998). Patch sampling behaviour and future foraging expectations in Argentine Ants, linepithema humile. Animal Behavior, 55 (3), 519–527.
Oaten, A. (1977). Optimal foraging in patches: A case for stochasticity. Theoretical Population Biology, 12, 263–285.
Ólafsdóttir, I. M., Gestsdóttir, S., & Kristjánsson, A. (2019). Visual foraging and executive functions: A developmental perspective. Acta Psychologica, 193, 203–213. https://doi.org/10.1016/j.actpsy.2019.01.005
Article PubMed Google Scholar
Olivers, C. N. L., Peters, J., Houtkamp, R., & Roelfsema, P. R. (2011). Different states in visual working memory: When it guides attention and when it does not. Trends in Cognitive Science, 15 (7), 327–334.
Olsson, O., & Brown, J. S. (2006). The foraging benefits of information and the penalty of ignorance. Oikos, 112, 260–273.
Olsson, O., & Brown, J. S. (2010). Smart, smarter, smartest: foraging information states and coexistence. Oikos, 119, 292–303.
Olsson, O., & Holmgren, N. M. A. (1998). The survival-rate-maximizing policy for Bayesian foragers: Wait for good news. Behavioral Ecology, 9 (4), 345–353.
Osborne, J. L., Smith, A., Clark, S. J., Reynolds, D. R., Barron, M. C., Lim, K. S., & Reynolds, A. M. (2013). The ontogeny of bumblebee flight trajectories: From naïve explorers to expert foragers. PLoS ONE, 8 (11), e78681.
Pacheco-Cobos, L., Winterhalder, B., Cuatianquiz-Lima, C., Rosetti, M. F., Hudson, R., & Ross, C. (2019). Nahua mushroom gatherers use area-restricted search strategies that conform to marginal value theorem predictions. Proceedings of the National Academy of Sciences, 116 (21), 10339–10347.
Pachur, T., Raaijmakers, J. G. W., Davelaar, E. J., Daw, N. D., Dougherty, M. R., Hommel, B., Lee, M. D., Polyn, S. M., Ridderinkhoff, K. R., Todd, P. M., & Wolfe, J. M. (2012). Unpacking cognitive search: Mechanisms and processes. In P. M. Todd, T. T. Hills, & T. W. Robbins (Eds.), Cognitive search: Evolution, algorithms, and the brain (pp. 237–253). MIT Press.
Paiva, V. H., Geraldes, P., Ramirez, I., Garthe, S., & Ramos, J. A. (2010). How area-restricted search of a pelagic seabird changes while performing a dual foraging strategy. Oikos, 119 (9), 1423–1434.
Palyulin, V. V., Chechkin, A. V., & Metzner, R. (2014). Lévy flights do not always optimize random blind search for sparse targets. Proceedings of the National Academy of Sciences, 111 (8), 2931–2936.
Papastamatiou, Y. P., Desalles, P. A., & McCauley, D. J. (2012). Area-restricted searching by manta rays and their response to spatial scale in lagoon habitats. Marine Ecology Progress Series, 456, 233–244.
Pattison, K. F., Zentall, T. R., & Watanabe, S. (2014). Sunk cost: Pigeons (Columba livia), too, show bias to complete a task rather than shift to another. Journal of Comparative Psychology, 126 (1), 1–9.
Payne, J. W. (1976). Task complexity and contingent processing in decision making: An information search and protocol analysis. Organizational Behavior and Human Performance, 16 (2), 366–387.
Peltier, C., & Becker, M. W. (2017). Individual differences predict low prevalence visual search performance. Cognitive Research: Principles and Implications, 2 (5), 1–11.
Peterson, M. S., Kramer, A. F., Wang, R. F., Irwin, D. E., & McCarley, J. S. (2001). Visual search has memory. Psychological Science, 12 (4), 287–292.
Pierce, G. J., & Ollason, J. G. (1987). Eight reasons why optimal foraging theory is a complete waste of time. Oikos, 49, 111–117.
Pinaud, C., & Weimerskirch, H. (2007). At-sea distribution and scale-dependent foraging behaviour of petrels and albatrosses: A comparative study. Journal of Animal Ecology, 76 (1), 9–19.
Plank, M. J., & James, A. (2008). Optimal foraging: Lévy pattern or process? Journal of the Royal Society Interface, 5, 26.
Pyke, G. H. (1978). Optimal Foraging in hummingbirds: Testing the Marginal Value Theorem. The American Zoologist, 18, 739–752.
Pyke, G. H. (2015). Understanding movements of organisms: It’s time to abandon the Lévy-foraging hypothesis. Methods in Ecology and Evolution, 6 (1), 1–16.
Pyke, G. H., Pulliam, H. R., & Charnov, E. L. (1977). Optimal foraging: A selective review of theory and tests. The Quarterly Review of Biology, 52 (2), 137–154.
Raichlen, D. A., Wood, B. M., Gordon, A. D., Mabulla, A. Z. P., Marloew, F. W., & Pontzer, H. (2014). Evidence of Lévy walk foraging patterns in human hunter-gatherers. Proceedings of the National Academy of Sciences of the United States of America, 111 (2), 728–733.
Ramos-Fernández, G., Mateos, J. L., Miramontes, O., Cocho, G., Larralde, H., & Ayala-Orozco, B. (2004). Lévy walk patterns in the foraging movement of spider monkeys ( Ateles geoffroyi ). Behavioral Ecology and Sociobiology, 55 (3), 223–230.
Ratcliff, R. (1978). A theory of memory retrieval. Psychological Review, 85, 59–108.
Reynolds, A. (2012). Distinguishing between Lévy walks and strong alternative models. Ecology, 93 (5), 1228–1233.
Reynolds, A. (2018) Current status and future directions of Lévy walk research. Biology Open, 7 (1), bio030106.
Reynolds, A. M., Paiva, V. H., Cecere, J. G., & Focardi, S. (2016). Lévy patterns in seabirds are multifaceted describing both spatial and temporal patterning. Frontiers in Zoology, 13 (29), 1–12.
Reynolds, A. M., Swain, J. L., Smith, A. D., Martin, A. P., & Osborne, J. L. (2009). Honeybees use a Lévy flight search strategy and odour-mediated anemotaxis to relocate food sources. Behavioral Ecology and Sociobiology, 64 (1), 115–123.
Ross, C., Pacheco-Cobos, L., & Winterhalder, B. (2018). A general model of forager search: Adaptive encounter-conditional heuristics outperform Lévy flights in the search for patchily distributed prey. Journal of Theoretical Biology, 455, 357–369.
Ross, C., & Winterhalder, B. (2018). Evidence for encounter-conditional, area-restricted search in a preliminary study of Colombian blowgun hunters. PLoS ONE, 13 (12), e0207633.
Samu, F. (1993). Wolf spider feeding strategies: Optimality of prey consumption in Pardosa Hortensis. Oecologia, 94 (1), 139–145.
Sang, K. (2017) Modeling exploration/exploitation behavior and the effect of individual differences . Doctoral Dissertation. Indiana University.
Schreirer, A. L., & Grove, M. (2014). Recurrent patterning in the daily foraging routes of Hamadryas baboons ( Papyo hamadryas ): Spatial memory in large-scale versus small-scale space. American Journal of Primatology, 76 (5), 421–435.
Shlesinger, M. F. (2009). Random searching. Journal of Physics A: Mathematical and Theoretical, 42 (43), 434001.
Shlesinger, M. F., & Klafter, J. (1986). Lévy walks versus Lévy flights. In H. E. Stanley & N. Ostrowsky (Eds.), On growth and form. Fractal and non-fractal patterns in physics . Martinus Nijhoff Publishers.
Shore, D. I., & Klein, R. M. (2000). On the manifestations of memory in visual search. Spatial Vision, 14 (1), 59–75.
Sims, D. W., Southall, E. J., Humphries, N. E., Hays, G. C., Bradshaw, C. J. A., Pitchford, J. W., James, A., Ahmed, M. Z., Brierley, A. S., Hindell, M. A., Morrit, D., Musyl, M. K., Righton, D., Shepard, E. L. C., Wearmouth, V. J., Wilson, R. P., Witt, M. J., & Metcalfe, J. D. (2008). Scaling laws of marine predator search behavior. Nature, 451, 1098.
Soman, D. (2001). The mental accounting of sunk time costs: Why time is not like money. Journal of Behavioral Decision Making, 14 (3), 169–185.
Stephens, D. W. (2008). Decision ecology: Foraging and the ecology of animal decision making. Cognitive, Affective & Behavioral Neuroscience, 8 (4), 475–484.
Stephens, D. W., & Charnov, E. (1982). Optimal foraging: Some simple stochastic models. Behavioral Ecology and Sociobiology, 10, 215–263.
Stephens, D. W., Couzin, I., & Giraldeau, L. A. (2012). Ecological and behavioral approaches to search behavior. In P. M. Todd, T. T. Hills, & T. W. Robbins (Eds.), Cognitive search: evolution, algorithms, and the brain (pp. 25–45). MIT Press.
Stephens, D. W., & Krebs, J. R. (1986). Foraging theory . Princeton University Press.
Tentelier, C., Lacroix, M. N., & Fauvergue, X. (2009). Inflexible wasps: The aphid parasitoid Lysiphlebus testaceipes does not track multiple changes in habitat profitability. Animal Behaviour, 77 (1), 95–100.
Thiel, A., & Hoffmeister, T. S. (2004). Knowing your habitat: Linking patch-encounter rate and patch exploitation rate in parasitoids. Behavioral Ecology, 15 (3), 419–425.
Thums, M., Bradshaw, C. J. A., & Hindell, M. A. (2011). In situ measures of foraging success and prey encounter reveal marine habitat-dependent search strategies. Ecology, 92 (6), 1258–1270.
Thums, M., Bradshaw, C. J. A., Sumner, M. D., Horsburgh, J. M., & Hindell, M. A. (2012). Depletion of deep marine food patches forces divers to give up early. Journal of Animal Ecology, 82, 72–83.
Toscano, B. J., Gownaris, N. J., Heerhartz, S. M., & Monaco, C. J. (2016). Personality, foraging behavior and specialization: Integrating behavioral and food web ecology at the individual level. Oecologia, 182 (1), 55–69.
Turrin, C., Fagan, N. A., Dal Monte, O., & Chang, S. W. C. (2017). Social resources foraging is guided by the principles of marginal value theorem. Scientific Reports, 7, 1–13.
Valone, T. J. (2006). Are animals capable of Bayesian updating? An empirical review. Oikos, 112, 252–259.
Van Gils, J. A. (2010). State-dependent Bayesian foraging on spatially autocorrelated food distributions. Oikos, 119, 237–244.
Viswanathan, G. M., Afanasiev, V., Buldyrev, S. V., Murphy, E. J., Prince, P. A., & Stanley, H. E. (1996). Lévy flight search patterns of wandering albatrosses. Nature, 381, 413–415.
Viswanathan, G. M., Buldyrev, S. V., Havlin, S., da Luz, M. G. E., Raposo, E. P., & Stanley, H. E. (1999). Optimizing the success of random searches. Nature, 401, 911–914.
Viswanathan, G. M., da Luz, M. G. E., Raposo, E. P., & Stanley, H. E. (2011). The physics of foraging. An introduction to random searches and biological encounters . University Press.
Viswanathan, G. M., Raposo, E. P., & da Luz, M. G. E. (2008). Lévy flights and superdiffusion in the context of biological encounters and random searches. Physics of Life Reviews, 5, 133–150.
Volchenkov, D., Helbach, J., Tscherepanow, M., & Kühnel, S. (2013). Exploration-exploitation trade-off features a saltatory search behavior. Journal of the Royal Society Interface, 10, 20130352.
PubMed Central Google Scholar
Von Helversen, B., Mata, R., Samanez-Larkin, G. R., & Wilke, A. (2018). Foraging, exploration or search? On the (lack of) convergent validity between three behavioral paradigms. Evolutionary Behavioral Sciences, 12 (3), 152–162.
Wajnberg, E. (2012). Multi-objective behavioural mechanisms are adopted by foraging animals to achieve several optimality goals simultaneously. Journal of Animal Ecology, 81, 503–511.
Weimerskirch, H., Pinaud, D., Pawlowski, F., & Bost, C. A. (2007). Does prey capture induce area-restricted search? A fine-scale study using GPS in a marine predator, the wandering albatross. The American Naturalist, 170 (5), 734–743.
Weissburg, M. (1993). Sex and the single forager: Gender-specific energy maximization strategies in fiddler crabs. Ecology, 74 (2), 279–291.
Wiegand, I., Seidel, C., & Wolfe, J. M. (2019). Hybrid foraging search in younger and older age. Psychology and aging, 34 (6), 805–820.
Wilke, A., Hutchinson, J. M. C., Todd, P. M., & Czienskowski, U. (2009). Fishing for the right words: Decision rules for human foraging behavior in internal search tasks. Cognitive Science, 33, 497–529.
Wittman, M., & Paulus, M. P. (2008). Decision making, impulsivity, and time perception. Trends in Cognitive Sciences, 12 (1), 7–12.
Wolfe, J. M. (2007). Guided search 4.0. Current progress with a model of visual search. In W. D. Gray (Ed.), Integrated models of cognitive systems (pp. 99–119). University Press.
Wolfe, J. M. (2012). Saved by a log: How do humans perform hybrid visual and memory search? Psychological Science, 23 (7), 698–703.
Wolfe, J. M. (2013). When is it time to move to the next raspberry bush? Foraging rules in human visual search. Journal of Vision, 13 (3), 1–17.
Wolfe, J. M. (2020). Guided Search 6.0: An upgrade with five forms of guidance, three types of functional visual fields, and two, distinct search templates. Journal of Vision, 20 (11), 303.
Wolfe, J. M., Aizenman, A. M., Boettcher, S. E. P., & Cain, M. S. (2016). Hybrid foraging search: Searching for multiple instances of multiple types of targets. Vision Research, 119, 50–59.
Wolfe, J. M., Cain, M. S., & Aizenman, A. M. (2019). Guidance and selection history in hybrid visual foraging search. Attention, Perception, & Psychophysics, 81 (3), 637–653.
Wolfe, J. M., Cain, M. S., & Alaoui-Soce, A. (2018). Hybrid value foraging: How the value of targets shapes human foraging behavior. Attention, Perception, & Psychophysics, 80, 609–621.
Wolfe, J. M., & Horowitz, T. S. (2017). Five factors that guide attention in visual search. Nature Human Behaviour, 1 (3), 1–8.
Woodman, G. F., & Chun, M. M. (2006). The role of working memory and long-term memory in visual search. Visual Cognition, 14 (4–8), 808–830.
Wosniak, M. E., Raposo, E. P., Viswanathan, G. M., & Da Luz, M. G. E. (2015). Efficient search of multiple types of targets. Physical Review E, 92, 062135.
Wu, C. C., & Wolfe, J. M. (2019). Eye movements in medical image perception: A selective review of past, present and future. Vision, 3, 32.
Zaburdaev, V., Denisov, S., & Klafter, J. (2015). Lévy walks. Reviews of Modern Physics, 87 (2), 483–530.
Zermatten, A., van der Linden, M., d’Acremont, M., Jermann, F., & Bechara, A. (2005). Impulsivity and decision making. Journal of Nervous and Mental Disease, 193 (10), 647–650.
Zhang, J., Gong, X., Fougnie, D., & Wolfe, J. M. (2015). Using the past to anticipate the future in human foraging behavior. Vision Research, 111, 66–74.
Zhao, K., Jurdak, R., Liu, J., Westcott, D., Kusy, B., Parry, H., Sommer, P., & McKeown, A. (2015). Optimal Lévy-flight foraging in a finite landscape. Journal of the Royal Society: Interface, 12, 1–12.
Zimmer, I., Wilson, R. P., Gilbert, C., Beaulieu, M., Ancel, A., & Ploetz, J. (2008). Foraging movements of emperor penguins at pointe geologie Antarctica. Polar Biology, 31 (2), 229–243.
Download references
The present work has been supported by the financed research project of the “Ministerio de Economía y Competitividad de España, Dirección General de Investigación Científica y Técnica”. Ref. PSI2015-69358-R. Granted to IP Beatriz Gil-Gómez de Liaño.
Author information
Authors and affiliations.
Universidad Autónoma de Madrid, Madrid, Spain
Marcos Bella-Fernández & Manuel Suero Suñé
Universidad Pontificia de Comillas, Madrid, Spain
Marcos Bella-Fernández
Universidad Complutense de Madrid. Centro de Tecnología Biomédica-Universidad Politécnica de Madrid, Madrid, Spain
Beatriz Gil-Gómez de Liaño
You can also search for this author in PubMed Google Scholar
Corresponding author
Correspondence to Marcos Bella-Fernández .
Ethics declarations
Conflict of interest.
Marcos Bella-Fernández declares that he has no conflict of interest. Manuel Suero Suñé declares that he has no conflict of interest. Beatriz Gil-Gómez de Liaño declares that she has no conflict of interest.
Ethical approval
This article does not contain any studies with human participants or animals performed by the authors.
Additional information
Publisher's note.
Springer Nature remains neutral with regard to jurisdictional claims in published maps and institutional affiliations.
Rights and permissions
Reprints and permissions
About this article
Bella-Fernández, M., Suero Suñé, M. & Gil-Gómez de Liaño, B. Foraging behavior in visual search: A review of theoretical and mathematical models in humans and animals. Psychological Research 86 , 331–349 (2022). https://doi.org/10.1007/s00426-021-01499-1
Download citation
Received : 25 April 2020
Accepted : 02 March 2021
Published : 21 March 2021
Issue Date : March 2022
DOI : https://doi.org/10.1007/s00426-021-01499-1
Share this article
Anyone you share the following link with will be able to read this content:
Sorry, a shareable link is not currently available for this article.
Provided by the Springer Nature SharedIt content-sharing initiative
- Find a journal
- Publish with us
- Track your research

An official website of the United States government
The .gov means it’s official. Federal government websites often end in .gov or .mil. Before sharing sensitive information, make sure you’re on a federal government site.
The site is secure. The https:// ensures that you are connecting to the official website and that any information you provide is encrypted and transmitted securely.
- Publications
- Account settings
Preview improvements coming to the PMC website in October 2024. Learn More or Try it out now .
- Advanced Search
- Journal List
- HHS Author Manuscripts

Five Factors that Guide Attention in Visual Search
Jeremy m wolfe.
Brigham and Women’s Hospital / Harvard Med
Todd S Horowitz
NIH, National Cancer Inst.
How do we find what we are looking for? Fundamental limits on visual processing mean that even when the desired target is in our field of view, we often need to search, because it is impossible to recognize everything at once. Searching involves directing attention to objects that might be the target. This deployment of attention is not random. It is guided to the most promising items and locations by five factors discussed here: Bottom-up salience, top-down feature guidance, scene structure and meaning, the previous history of search over time scales from msec to years, and the relative value of the targets and distractors. Modern theories of search need to specify how all five factors combine to shape search behavior. An understanding of the rules of guidance can be used to improve the accuracy and efficiency of socially-important search tasks, from security screening to medical image perception.
How can a texting pedestrian walk right into a pole, even though it is clearly visible 1 ? At any given moment, our attention and eyes are focused on some aspects of the scene in front of us, while other portions of the visible world go relatively unattended. We deploy this selective visual attention because we are unable to fully process everything in the scene at the same time. We have the impression of seeing everything in front of our eyes, but over most of the visual field we are probably seeing something like visual textures, rather than objects 2 , 3 Identifying specific objects and apprehending their relationships to each other typically requires attention, as our unfortunate texting pedestrian can attest.
Figure 1 illustrates this point. It is obvious that this image is filled with Ms and Ws in various combinations of red, blue, and yellow, but it takes attentional scrutiny to determine whether or not there is a red and yellow M.

On first glimpse, you know something about the distribution of colors and shapes but not how those colors and shapes are bound to each other. Find ‘M’s that are red and yellow.
The need to attend to objects in order to recognize them raises a problem. At any given moment, the visual field contains a very large, possibly uncountable number of objects. We can count the Ms and Ws of Figure 1 , but imagine looking at your reflection in the mirror. Are you an object? What about your eyes or nose or that small spot on your chin? If object recognition requires attention, and if the number of objects is uncountable, how do we manage to get our attention to a target object in a reasonable amount of time? Attention can process items at a rate of, perhaps, 20-50 items per second. If you were looking for a street sign in an urban setting containing a mere 1000 possible objects (every window, tire, door handle, piece of trash, etc.), it would take 20-50 seconds just to find that sign. It is introspectively obvious that you routinely find what you are looking for in the real world in a fraction of that time. To be sure, there are searches of the needle-in-a-haystack, Where’s Waldo? variety that take significant time, but routine searches for the saltshaker, the light switch, your pen, and so forth, obviously proceed much more quickly. Search is not overwhelmed by the welter of objects in the world because search is guided to a (often very small) subset of all possible objects by several sources of information. The purpose of this article is to briefly review the growing body of knowledge about the nature of that guidance.
We will discuss five forms of guidance:
- Bottom-up, stimulus-driven guidance in which the visual properties of some aspects of the scene attract more attention than others.
- Top-down, user-driven guidance in which attention is directed to objects with known features of desired targets.
- Scene guidance in which attributes of the scene guide attention to areas likely to contain targets.
- Guidance based on the perceived value of some items or features.
- Guidance based on the history of prior search.
Measuring Guidance
We can operationalize the degree of guidance in a search for a target by asking what fraction of all items can be eliminated from consideration. One of the more straight-forward methods to do this is to present observers with visual search displays like those in Figure 2 and measure the reaction time (RT) required for them to report whether or not there is a target (here a “T), as a function of the number of items ( set size ). The slope of the RT x set size function is a measure of the efficiency of search. For a search for a T among Ls ( Fig 2A ), the slope would be in the vicinity of 20-50 msec/item 4 . We believe that this reflects serial deployment of attention from item to item 5 though this need not be the case 6 .

The basic visual search paradigm. A target (here a ‘T‘) is presented amidst a variable number of distractors. Search ‘efficiency’ can be indexed by the slope of the function relating reaction time (RT) to the visual set size. If the target in 2B is a red T, the slope for 2B will be half of that for 2A because attention can be limited to just half of the items in 2B.
In Fig. 2B , the target is a red T. This search would be faster and more efficient 7 because attention can be guided to the red items. If half the items are red (and if guidance is perfect), the slope will be reduced by about half, suggesting that, at least in this straightforward case, slopes index the amount of guidance.
The relationship of slopes to guidance is not entirely simple, even for arrays of items like those in Fig 2 8 but see 9 . Matters become far more complex with real world scenes where the visual set size is not easily defined 10 , 11 . However, if the slope is cut in half when half the items acquire some property, like the color red in 2B, it is reasonable to assert that search has been guided by that property 9 .
The problem of distractor rejection
As shown in Figure 2 , a stimulus attribute can make search slopes shallower by limiting the number of items in a display that need to be examined. However, guidance of attention is not the only factor that can modulate search slopes. If observers are attending to each item in the display (in series or in parallel), the slope of the RT x set size function can also be altered by changing how long it takes to reject each distractor. Thus, if we markedly reduced the contrast of Figure 2A , the RT x set size function would become steeper, not because of a change in guidance but because it would now take longer to decide if any given item was a T or an L.
Bottom-up guidance by stimulus salience
Attention is attracted to items that differ from their surroundings, if those differences are large enough and if those differences occur in one of a limited set of attributes that guide attention. The basic principles are illustrated in Figure 3 .

Which items ‘pop-out’ of this display, and why?
Three items ‘pop-out’ of this display. The purple item on the left differs from its neighbors in color. It is identical to the purple item just inside the upper right corner of the image. That second purple item is not particularly salient even though it is the only other item in that shade of purple; its neighbors are close enough in color that the differences in color do not attract attention. The bluish item to its left is salient by virtue of an orientation difference. The square item a bit further to the left is salient because of the presence of a ‘closure’ feature 12 or the absence of a collection of line terminations 13 . We call properties like color, orientation, or closure basic (or guiding ) features, because they can guide the deployment of attention. Other properties may be striking when one is directly attending to an item, and may be important for object recognition, but they do not guide attention. For example, the one ‘plus’ in the display is not salient, even though it possesses the only X-intersection in the display, because intersection type is not a basic feature 14 . The ‘pop-out’ we see in Figure 3 is not just subjective phenomenonology. Pop-out refers to extremely effective guidance, and is diagnosed by a near-zero slope of the RT x set size function; though there may be systematic variability even in these ‘flat’ slopes 15 .
There are two fundamental rules of bottom-up salience 16 . Salience of a target increases with difference from the distractors ( target-distractor – TD- heterogeneity ) and with the homogeneity of the distractors ( distractor-distractor –DD- homogeneity ) along basic feature dimensions. Bottom-up salience is the most extensively modeled aspect of visual guidance nicely reviewed in 17 . The seminal modern work on bottom-up salience is Koch and Ullman’s 18 description of a winner-take-all network for deploying attention. Subsequent decades have seen the development of several influential bottom-up models e.g. 19 , 20 – 22 . However, bottom-up salience is just one of the factors guiding attention. By itself, it does only modestly well in predicting the deployment of attention (usually indexed by eye fixations). Models do quite well predicting search for salience, but not as well predicting search for other sorts of targets 17 . This is quite reasonable. If you are looking for your cat in the bedroom, it would be counterproductive to have your attention visit all the shiny, colorful objects first. Thus, a bottom-up saliency model will not do well if the observer has a clear top-down goal 23 . One might think that bottom-up salience would dominate if observers free-viewed a scene in the absence of such a goal, but bottom-up models can be poor at predicting fixations even when observers “free view” scenes without specific instructions 24 . It seems that observers generate their own, idiosyncratic tasks, allowing other guiding forces to come into play. It is worth noting that salience models work better if they are not based purely on local features but acknowledge the structure of objects in the field of view 25 . For instance, while the most salient spot in an image might be the edge between the cat’s tail and the white sheet on the bed, fixations are more likely to be directed to middle of the cat 26 , 27 .
Top-down Feature Guidance
Returning to Figure 1 , if you search for Ws with yellow elements, you can guide your attention to yellow items and subsequently determine if they are Ws or Ms 7 . This is feature guidance, sometimes referred to as feature-based attention 28 . Importantly, it is possible to guide attention to more than one feature at a time. Thus, search for a big, red, vertical item can benefit from our knowledge of its color, size, and orientation 29 . Following the TD heterogeneity rule, search efficiency is dependent on the number of features shared by targets and distractors 29 , and observers appear to be able to guide to multiple target features simultaneously 30 . This finding raises the attractive possibility that search for an arbitrary object among other arbitrary objects would be quite efficient because objects would be represented sparsely in a high-dimensional space. Such sparse coding has been invoked to explain object recognition 31 , 32 . However, search for arbitrary objects turns out not to be particularly efficient 11 , 33 . By itself, guidance to multiple features does not appear to be an adequate account of how we search for objects in the real world (see the section on scene guidance, below).
What are the guiding attributes?
Feature guidance bears some metaphorical similarity to your favorite computer search engine. You enter some terms into the search box and an ordered list of places to attend is returned. A major difference between internet search engines and the human visual search engine is that human search uses only a very small vocabulary of search terms (i.e., features). The idea that there might be a limited set of features that could be appreciated “preattentively” 34 was at the heart of Treisman’s “Feature Integration Theory” 35 . She predicted that targets defined by unique features would pop-out of displays. Subsequent theorists modified this proposal to suggest that features could guide the deployment of attention 7 36 .
There are probably only a couple dozen attributes that can guide attention. The visual system can detect and identify a vast number of stimuli, but it cannot use arbitrary properties to guide attention the way that Google or Bing can use arbitrary search terms. A list of guiding attributes is found in Table 1 . This article does not list all of the citations that support each entry. Many of these can be found in older versions of the list 37 , 38 . Recent changes to the list are marked in color in Table 1 and citations are given for those.
The guiding attributes for feature search
Color | Motion | Orientation | Size (incl. length, spatial freq., and apparent size ) |
Luminance onset (flicker) but see | Luminance polarity | Vernier offset | Stereoscopic depth & tilt |
Pictorial depth cues But see . | Shape | Line termination | Closure |
Curvature | Topological status | ||
Lighting direction (shading) | Expansion / Looming | Number | Glossiness (luster) |
Aspect ratio | eye of origin / binocular rivalry | ||
Novelty | Letter Identity Alphanumeric Category | Familiarity – over-learned sets, in general | |
Intersection | Optic flow | Color change | 3-D volumes (eg. geons) |
Luminosity | Material type | Scene Category | Duration |
Stare-in-crowd , | Biological motion | Your name | Threat |
Semantic Category (Animal, artifact, etc) | Blur | Visual rhythm | Animacy/Chasing |
Threat | |||
Faces among other objects | Familiar Faces | Emotional faces | Schematic Faces |
Cast shadows | Amodal Completion | Apparent Depth |
Attributes like color are deemed to be “undoubted” because multiple experiments from multiple labs attest to their ability to guide attention. “Probable” feature dimensions may be merely probable because we are not sure how to define the feature. Shape is the most notable entry here. It seems quite clear that something about shape guides attention 49 . It is less clear exactly what that might be, though the success of deep learning algorithms in enabling computers to classify objects may open up new vistas for understanding human search for shape 50 .
The attributes described as “possible” await more research. Often these attributes only have a single paper supporting their entry on the list, as in the case of numerosity: Can you direct attention to the pile with “more” elements in it, once you eliminate size, density, and other confounding visual factors? Perhaps 51 , but it would be good to have converging evidence. Search for the magnitude of a digit (e.g. “find the highest number”) is not guided by the semantic meaning of the digits but by their visual properties 52
The list of attributes that do not guide attention is, of course, potentially infinite. Table 1 lists a few plausible candidates that have been tested and found wanting. For example, there has been considerable interest recently in what could be called “evolutionarily motivated” candidates for guidance. What would enhance our survival if we could find it efficiently? Looking at a set of moving dots on a computer screen, we can perceive that one is “chasing” another 53 . However, this aspect of animacy does not appear to be a guiding attribute 47 . Nor does “threat” (defined by association with electric shock) seem to guide search 48 .
Some caution is needed here because a failure to guide is a negative finding and it is always possible that, were the experiment done correctly, the attribute might guide after all. Thus, early research 54 found that binocular rivalry and eye-of-origin information did not guide attention, but more recent work 55 , 56 suggests that it may be possible to guide attention to interocular conflict, and our own newer data 57 indicates that rivalry may guide attention if care is taken to suppress other signals that interfere with that guidance. Thus, binocular rivalry was listed under “doubtful cases & probable non-features” in 37 , but is now listed under “possible guiding attributes” in Table 1 .
Faces remain a problematic candidate for feature status, with a substantial literature yielding conflicting results and conclusions. Faces are quite easy to find among other objects 58 , 59 but there is dispute about whether the guiding feature is “face-ness” or some simpler stimulus attribute 60 , 61 . A useful review by Frischen et al. 62 argues that “preattentive search processes are sensitive to and influenced by facial expressions of emotion”, but this is one of the cases where it is hard to reject the hypothesis that the proposed feature is modulating the processing of attended items, rather than guiding the selection of which items to attend. Suppose that, once attended, it takes 10 msec longer to disengage attention from an angry face than from a neutral face. The result would be that search would go faster (10 msec/item faster) when the distractors were neutral than when they were angry. Consequently, an angry target among neutral distractors would be found more efficiently than a neutral face among angry. Evidence for guidance by emotion would be stronger if the more efficient emotion searches were closer to pop-out than to classic inefficient, unguided searches, e.g., T among Ls, 63 . Typically, this is not the case. For example, Gerritsen et al 64 report that “Visual search is not blind to emotion” but, in a representative finding, search for hostile faces produced a slope of 64 msec/item, which is quite inefficient, even if somewhat more efficient than the 82 msec/item for peaceful target faces (p1054).
There are stimulus properties that, while they may not be guiding attributes in their own right, do modulate the effectiveness of other attributes. For example, apparent depth modulates apparent size, and search is guided by that apparent size 65 . Finally, there are properties of the display that influence the deployment of attention. These could be considered aspects of “scene guidance” (see the next major section, below). For example, attention tends to be attracted to the center of gravity in a display 66 . Elements like arrows direct attention even if they, themselves do not pop-out 67 . As discussed by Rensink 68 , these and related factors can inform graphic design and other situations where the creator of an images wants to control how the observer consumes that image.
There have been some general challenges to the enterprise of defining specific features, notably the hypothesis that many of the effects attributed to the presence or absence of basic features are actually produced by crowding in the periphery 3 . For example, is efficient search for cubes lit from one side among cubes lit from another side evidence for preattentive processing of 3D shape and lighting 69 , or merely a by-product of the way these stimuli are represented in peripheral vision 41 ? Resolution of this issue requires a set of visual search experiments with stimuli that are “uncrowded”. This probably means using low set sizes; for example, see the evidence that material type is not a guiding attribute 70 .
A different challenge to the preattentive feature enterprise is the possibility that too many discrete features are proposed. Perhaps many specific features form a continuum of guidance by a single, more broadly defined attribute. For instance, the cues to the 3D layout of the scene include stereopsis, shading, linear perspective and more. These might be part of a single attribute describing the 3D disposition of an object. Motion, onsets, and flicker might be part of a general dynamic change property 71 . Most significantly, we might combine the spatial features of line termination, closure, topological status, orientation, and so forth into a single shape attribute with properties defined by the appropriate layer of the right convolutional neural net (CNN). Such nets have shown themselves capable of categorizing objects, so one could imagine a preattentive CNN guiding attention to objects as well 72 . At this writing, such an idea remains a promissory note. Regardless of how powerful CNNs may become, humans cannot guide attention to entirely arbitrary/specific properties in order to find particular types of object 73 and it is unknown if some intermediate representation in a CNN could capture the properties of the human search engine. If it did, we might well find that such a layer represented a space with dimensions corresponding to attributes like size, orientation, line termination, vernier offset, and so forth, but this remains to be seen.
Guidance by scene properties
While the field of visual search has largely been built on search for targets in arbitrary 2D arrays of items, most real world search takes place in structured scenes, and this structure provides a source of guidance. To illustrate, try search for any humans in Figure 4 . Depending on the resolution of the image as you are viewing it, you may or may not be able to see legs poking out from behind the roses by the gate. Regardless, what should be clear is that the places you looked were strongly constrained. Biederman, Mezzanotte, and Rabinowitz 74 suggested a distinction between semantic and syntactic guidance.

Scene Guidance: Where is attention guided if you are looking for humans? What if the target was a bird?
Syntactic guidance has to do with physical constraints. You don’t look for people on the front surface of the wall or in the sky because people typically need to be supported against gravity. Semantic guidance refers to the meaning of the scene. You don’t look for people on the top of the wall, not because they could not be there but because they are unlikely to be there given your understanding of the scene, whereas you might scrutinize the bench. Scene guidance would be quite different (and less constrained) if the target were a bird. The use of the terms “semantic” and “syntactic” should not be seen as tying scene processing too closely to linguistic processing, nor should the two categories be seen as neatly non-overlapping 75 76 . Nevertheless, the distinction between syntactic and semantic factors, as roughly defined here, can be observed in electrophysiological recordings: scenes showing semantic violations (e.g., a bar of soap sitting next to the computer on the desk) produce different neural signatures than scenes showing syntactic violations (e.g., a computer mouse on top of the laptop screen) 77 . While salience may have some influence in this task 78 , it does not appear to be the major force guiding attention here 24 , 79 . But note that feature guidance and scene guidance work together. People certainly could be on the lawn, but you do not scrutinize the empty lawn in Figure 4 because it lacks the correct target features.
Extending the study of guidance from controlled arrays of distinct items to structured scenes poses some methodological challenges. For example, how do we define the set size of a scene? Is “rose bush” an item in Figure 4 , or does each bloom count as an item? In bridging between the world of artificial arrays of items and scenes, perhaps the best we can do is to talk about the “effective set size” 80 10 , the number of items/locations that are treated as candidate targets in a scene give a specific task. If you are looking for the biggest flower, each rose bloom is part of the effective set. If you are looking for a human, those blooms are not part of the set. While any estimate of effective set size is imperfect, it is a very useful idea and it is clear that, for most tasks, the effective set size will be much smaller than the set of all possible items 11 .
Preview methods have been very useful in examining the mechanisms of scene search 81 . A scene is flashed for a fraction of a second and then the observer searches for a target. The primary data are often eye tracking records. Often, these experiments involve searching while the observer’s view of the scene is restricted to a small region around the point of fixation (“gaze-contingent” displays). Very brief exposures (50-75 msec) can guide deployment of the eyes once search begins 82 . A preview of the specific scene is much more useful than a preview of another scene of the same category, though the preview scene does not need to be the same size as the search stimulus 81 . Importantly, the preview need not contain the target in order to be effective 83 . Search appears to be more strongly guided by a relatively specific scene ‘gist’ 80 84 , an initial understanding of the scene that does not rely on recognizing specific objects 85 . The gist includes both syntactic (e.g., spatial layout) and semantic information, and this combination can provide powerful search guidance. Knowledge about the target provides an independent source of guidance 86 , 87 . These sources of information provide useful ‘priors’ on where targets might be (“If there is a vase present, it’s more likely to be on a table than in the sink”), that are more powerful than memory for where a target might have been seen 88 89 , 90 in terms of guiding search.
Preview effects may be fairly limited in search of real scenes. If the observer searches a fully visible scene rather than being limited to a gaze-contingent window, guidance by the preview is limited to the first couple of fixations 91 . Once search begins, guidance is presumably updated based on the real scene, rendering the preview obsolete. In gaze-contingent search, the effects last longer because this updating cannot occur. This updating can be seen in the work of Hwang et al. 76 , where, in the course of normal search, the semantic content of the current fixation in a scene influences the target of the next fixation.
Modulation of search by prior history
In this section, we summarize evidence showing that the prior history of the observer, especially the prior history of search, modulates the guidance of attention. We can organize these effects by their time scale, from within a trial (on the order of 100s of ms) to lifetime learning (on the order of years).
A number of studies have demonstrated the preview benefit : when half of the search array is presented a few hundred msec before the rest of the array, the effective set size is reduced, either because attention is guided away from the old “marked” items (visual marking 92 ) and/or toward the new items (onset prioritization 93 ).
On a slightly longer timescale, priming phenomena are observed from trial to trial within an experiment, and can be observed over seconds to weeks. The basic example is “priming of pop-out” 94 , in which an observer might be asked to report the shape of the one item of unique color in a display. If that item is the one red shape among green on one trial, responses will be faster if the next trial repeats red among green as compared to a switch to green among red; though the search in both cases will be a highly efficient, color pop-out search. More priming of pop-out is found if the task is harder 95 . Note that it is neither the response nor the reporting feature which is repeated in priming of pop-out, but the target-defining or selection feature.
More generally, seeing the features of the target makes search faster than reading a word cue describing the target, even for overlearned targets. This priming by target features takes about 200 msec to develop 96 . Priming by the features of a prior stimulus can be entirely incidental; simply repeating the target from trial to trial is sufficient 97 . More than one feature can be primed at the same time 97 , 98 and both target and distractor features can be primed 97 , 99 . Moreover, it is not just that observers are more ready to report targets with the primed feature; priming actually boosts sensitivity (i.e., d ’) 100 . Such priming can last for at least a week 101 .
Observers can also incidentally learn information over the course of an experiment that can guide search. In contextual cueing 102 , a subset of the displays are repeated across several blocks of trials. While observers do not notice this repetition, RTs are faster for repeated displays than for novel, unrepeated displays 103 . The contextual cueing effect is typically interpreted as an abstract form of scene guidance: just as you learn that, in your friend’s kitchen, the toaster is on the counter next to the coffeemaker, you learn that, in this configuration of rotated Ls, the T is in the bottom left corner. However, evidence for this interpretation is mixed. RT x set size slopes are reduced for repeated displays 102 in some experiments, but not in others 104 . Contextual cueing effects can also be observed in cases such as pop-out search and 105 , attentionally-cued search, 106 , where guidance is already nearly perfect. Kunar et al. 104 suggested that contextual cueing reflects response facilitation, rather than guidance. Again, the evidence is mixed. There is a shift towards a more liberal response criterion for repeated displays 107 , but this is not correlated with the size of the contextual cueing RT effect. In pop-out search, sensitivity to the target improves for repeated displays without an effect on decision criterion 105 . It seems likely that observed contextual cueing effects reflect a combination of guidance effects and response facilitation, the mix depending on the specifics of the task. Oculomotor studies show that the context is often not retrieved and available to guide attention until a search has been underway for several fixations 108 , 109 . Thus, the more efficient the search, the greater the likelihood that the target will be found before the context can be retrieved. Indeed, in simple letter displays, search does not become more efficient even when the same display is repeated several hundred times 110 , presumably because searching de novo is always faster than waiting for context to become available. Once the task becomes more complex (e.g., searching for that toaster) 111 , it becomes worthwhile to let memory guide search 112 113 .
Over years and decades, we become intimately familiar with, for example, the characters of our own written language. There is a long-running debate about whether familiarity (or, conversely, novelty) might be a basic guiding attribute. Much of this work has been conducted with overlearned categories like letters. While the topic is not settled, semantic categories like “letter” probably do not guide attention 114 , 115 , though mirror-reversed letters may stand out against standard letters 116 117 . Instead, items made familiar in LTM can modulate search 42 , 118 though there are limits on the effects of familiarity in search 119 120 .
Modulation of search by the value of items
In the past few years, there has been increasing interest in the effects of reward or value on search. Value proves to be a strong modulator of guidance. For instance, if observers are rewarded more highly for red items than for green, they will subsequently guide attention toward red, even if this is irrelevant to the task 121 . Note, color is the guiding feature; value modulates its effectiveness. The learned associations of value do not need to be task relevant or salient in order to have their effects 122 and learning can be very persistent with value-driven effects being seen half a year after acquisition 123 . Indeed, the effects of value may be driving some of the long-term familiarity effects described in the previous paragraph 42 .
Visual search is mostly effortless. Unless we are scrutinizing aerial photographs for hints to North Korea’s missile program, or hunting for signs of cancer in a chest radiograph, we typically find what we are looking for in seconds or less. This remarkable ability is the result of attentional guidance mechanisms. While thirty-five years or so of research has given us a good grasp of the mechanisms of bottom-up salience, top-down feature-driven guidance and how those factors combine to guide attention 124 , 125 , we are just beginning to understand how attention is guided by the structure of scenes and the sum of our past experiences. Future challenges for the field will include understanding how discrete features might fit together in a continuum of guidance and extending our theoretical frameworks from two-dimensional scenes to immersive, dynamic, three-dimensional environments.
Competing Interests:
JMW occasionally serves as an expert witness or consultant for which this article might be relevant. TSH has no competing interests to declare.
Contributor Information
Jeremy M Wolfe, Brigham and Women’s Hospital / Harvard Med.
Todd S Horowitz, NIH, National Cancer Inst.
BIBLIOGRAPHY

IMAGES
VIDEO
COMMENTS
Proactive scanning. Never allow yourself to stay focused on one point on the roadway while driving. Sure, you need to monitor the situation on the road directly in front of your vehicle, but you also need to monitor the gauges inside your car and the situation on the roadway some distance ahead. Organizing a visual search of the roadway is ...
Also check the space between your car and any vehicles in the lane next to you. It is very important to check behind you before you change lanes, slow down quickly, back up, or drive down a long or steep hill. You should also glance at your instrument panel often to ensure there are no problems with the vehicle and to verify your speed.
In Fig. 2b, the target is a red T.This search would be faster and more efficient 7 because attention can be guided to the red items. If half the items are red (and if guidance is perfect), the ...
Systematic visual search strategy: Hierarchy of skill complexity: Techniques and difficulty of order "Ticking off" abilities to perform certain actions: Varying search strategy (i.e., different components of skill) Top-down vs. bottom-up visual search: Goal-driven visual search: Stimulus-driven visual search: Diagnosis of errors
Here, the development of viable systematic search strategies for journal articles, books, book chapters and other sources, selection of appropriate databases, search tools and selection methods are described and illustrated with examples from rheumatology. The up-keep of skills over time, and the acquisition of localised information sources ...
In the experimental condition, N = 107 received training in the use of systematic visual search. Control group participants were only able to identify a circa mean 33% of observable hazards in the kitchens. In contrast experimental group participants, using systematic visual search, observed a circa mean 50% of observable hazards present. ...
Searching for a systematic review. The most advanced type of literature search is the search necessary to be able to write a systematic review. A systematic review has to qualify for being 'systematic' and not being an editorial or similar, which means that it has to cover 'all' relevant published studies in the area.
This paper describes Guided Search 6.0 (GS6), a revised model of visual search. When we encounter a scene, we can see something everywhere. However, we cannot recognize more than a few items at a time. Attention is used to select items so that their features can be "bound" into recognizable objects. Attention is "guided" so that items can be processed in an intelligent order. In GS6 ...
Search. 5. Select suitable references from those that have been retrieved. 6. Assess whether the search was satisfactory. 7. Redesign the search strategy and/or choose other databases/search tools, where needed. 8. Repeat steps 2-6, if necessary.
During a search operation, global image features and local salience are computed in parallel. These two sources of guidance are combined within a priority map that governs the order of scene regions fixated. The inclusion of contextual guidance allows the model outperform a model based solely on visual salience.
This systematic review identified all studies which investigated whether driving experience related to drivers' visual search by comparing the visual search of novice and experienced drivers. Since this is a between subject comparison of novice and experienced driver groups from the population, the studies feature no formal randomisation as ...
Finally, the experts were more proactive and systematic in their analysis, with their visual search strategy underpinned by a hierarchy of skills (Gegenfurtner et al., 2011). It is likely that the lack of a systematic approach to observational analysis observed among novice coaches potentially limits the validity and the effectiveness of their ...
During a visual search, an observer (the person who is searching for the keys, for example) looks for a target (the keys) in the midst of distracters (all the other stuff in a home).
Specific task-related search patterns, like drilling through CT scans and systematic search in chest X-rays, were found to be related to high expert levels. One study investigated teaching of visual search strategies, and did not find a significant effect on perceptual performance. ... It is important to identify visual search patterns that do ...
Visual search tasks are an everyday part of the human experience - ranging from hunting for a specific recipe ingredient in the pantry to monitoring for road hazards and informational signs while driving. Due to its ubiquity in everyday life, visual search has been extensively studied in the laboratory for decades even if laboratory tasks are ...
Given visual search success vs. failure can have life-or-death implications, effective training is critical. ... This paper shows that, while experts have a more systematic search pattern and perform better than students, there were no performance improvements found in students that underwent a systematic search training. This highlights the ...
In visual search tasks, observers look for targets among distractors. In the lab, this often takes the form of multiple searches for a simple shape that may or may not be present among other items scattered at random on a computer screen (e.g., Find a red T among other letters that are either black or red.). In the real world, observers may ...
In visual search tasks, observers look for targets among distractors. In the lab, this often takes the form of multiple searches for a simple shape that may or may not be present among other items scattered at random on a computer screen (e.g., Find a red T among other letters that are either black or red.). In the real world, observers may search for multiple classes of target in complex ...
Visual search (VS) is a fundamental task in daily life widely studied for over half a century. A variant of the classic paradigm—searching one target among distractors—requires the observer to look for several (undetermined) instances of a target (so-called foraging) or several targets that may appear an undefined number of times (recently named as hybrid foraging). In these searches ...
Visual search is an everyday task that requires the coordination of vision, attention, and memory. The challenge in studying a complex task like visual search is trying to determine the relative contributions of each of these components. Eye movements provide incredibly useful data for parsing complex tasks that rely on visual processing.
The purpose of this article is to briefly review the growing body of knowledge about the nature of that guidance. We will discuss five forms of guidance: Bottom-up, stimulus-driven guidance in which the visual properties of some aspects of the scene attract more attention than others. Top-down, user-driven guidance in which attention is ...
Visual search is a common task that people perform throughout their daily life, and the number of applications inspired by the human visual search mechanism is potentially large. ... the FIT provided for the first time a systematic framework for combining the two. The serial/parallel dichotomy entails the definition of basic features that can ...