- Syllabus (Spring & Summer)
- Syllabus (Fall)
- Orientation
- Meet the Instructors
- Google Earth Download and Tutorials
- Capstone Project: Directions and Requirements
- Library Resources
- Getting Help
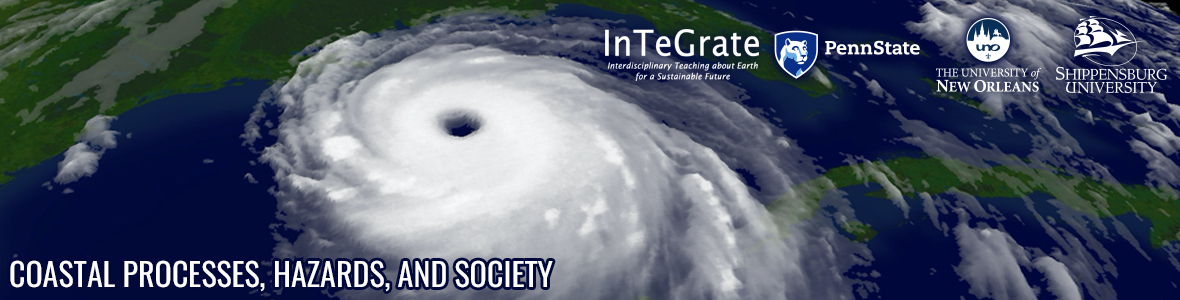

Case Study: Puerto Rico and Hurricane Maria

Differential response and recovery times across U.S. communities hit by 2017 storms
The 2017 Atlantic hurricane season went down in history as having the most named storms since 2005. In 2005 there were 28 named storms and 15 of them becoming hurricanes, while in 2017 there were 17 named storms and 10 of these became hurricanes. The three most notable out of these storms – Harvey (landfall on August 25 in Texas), Irma (Landfall September 10 in Florida) and Maria, (Landfall on September 20 in Puerto Rico) followed in close succession and broke records for their intensity in the places they impacted.
Maria achieved its peak intensity over the eastern Caribbean and made landfall in Yabucao, southeastern Puerto Rico as a strong category 4 storm, where winds of 175 mph (280 km/h) were recorded. It was the first Category 4 hurricane to directly impact the island in 85 years, and it caused widespread damage that was felt for months, if not years.
Prior to Maria’s landfall, hundreds of shelters were set up, and evacuation orders were given. An uptick in travel from the island just before the storm was indicative of the numbers of people fleeing to the U.S. mainland to stay with family.
Compounding the impacts of Maria on the island was the damage from Category 5 Hurricane Irma just 2 weeks prior, especially to the electrical grid, which was crippled by Maria, leaving all 3.4 million residents without electricity for an extended period. Six months after the storm, many were still without power and had not been able to repair their houses. In fact, the combined impacts of hurricanes Irma and Maria resulted in Puerto Rico Electrical Power Authority (PREPA) filing for bankruptcy. Imagine all the functions that are impossible without power, besides the misery of living in a tropical climate without any means to refrigerate food or enjoy air-conditioning. All means of modern communication are impossible because cell phone towers are one of the first things to be destroyed by the high winds of a major storm.
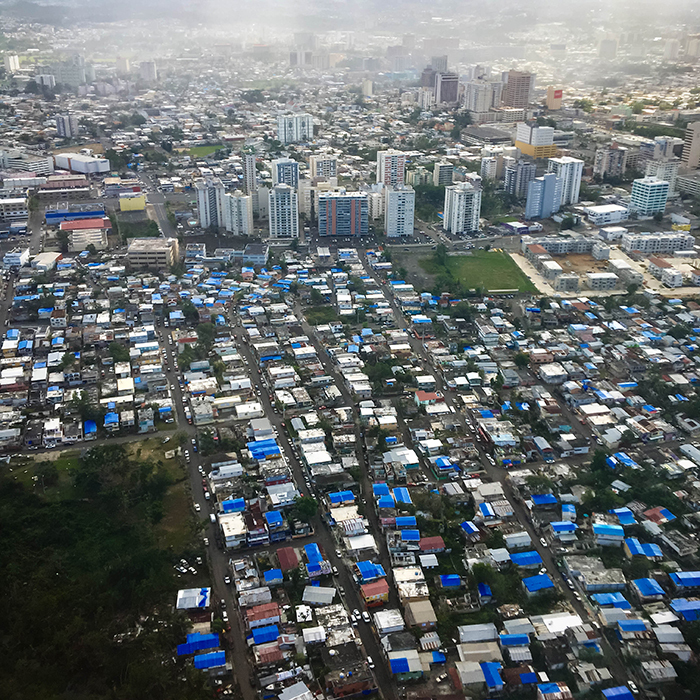
The number of people killed by Hurricane Maria proved challenging to count. An initial number of 65 deaths directly attributable to the storm was officially recorded by the federal government. This number did not account for those whose deaths were from indirect causes linked to the hurricane, due to deprivation caused by things like lack of power and medical services and a multitude of other causes during the response and recovery phases. Six months after Maria’s landfall, an estimate of 2,975 indirect deaths was made by examining the mortality rate for that time period compared to the same period during previous years. The sensitive populations – the elderly, the very young, and the sick - suffer the most in the immediate aftermath of a disaster like a hurricane, and it is among these groups that the most deaths occurred in Maria.
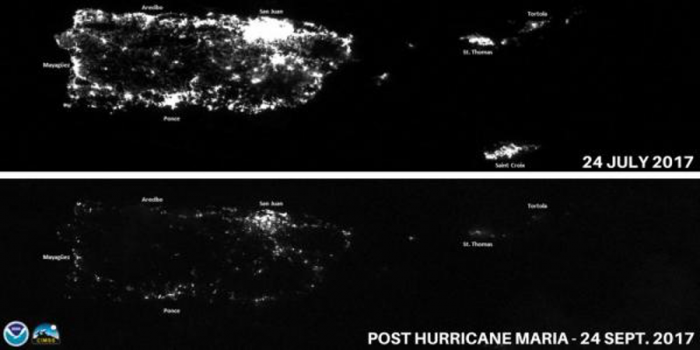
Residents of the U.S. territory were able to apply for aid through FEMA, but reports indicated that supplies moved slowly to destinations, hampering recovery. In November 2017, two months after the storm, 60% were still without power and perhaps 20% without water, so that many had to collect water from streams and rainwater. Many stories in the media at the six-month mark highlighted the misery of a slow recovery. There was a lack of adequate infrastructure repair, medical services, and the simple availability of supplies throughout this time, although many charity organizations supported the effort.
An objective study by BMJ Global Health: Quantifying inequities in US federal response to hurricane disaster in Texas and Florida compared with Puerto Rico , investigated the question of whether the federal response to Hurricane Maria in Puerto Rico was significantly smaller and slower than the responses to Hurricane Irma in Florida and Hurricane Harvey in Texas. Their conclusion was that the response to Maria was indeed lacking and did not align with the storm severity or the needs of the affected communities. They note that “Increases in mortality and adverse health outcomes due to differentiated disaster response and recovery efforts may promote inequity among populations that receive less aid.” In other words, Puerto Rico did not receive the same level of aid as Texas and Florida after Harvey and Irma respectively, and the people of Puerto Rico suffered as a result. They go on to suggest reasons for this in this way: “There may be many different reasons why the federal response varied in each case. Ability to access the affected area based on geography and distance, existing infrastructure aiding or acting as barriers to response efforts, disaster fatigue, as well as issues of racial bias and perceptions of differential citizenship all may have affected the appropriation and delivery of resources and funding to affected areas in each hurricane. Different mechanisms for the varied responses may be more or less justified. However, what cannot be contested is that the responses were in fact different across critical time points, and these differences have serious consequences for acute and long-term health outcomes and recovery efforts.”
The data in the report clearly show a disparity in the amount of aid received and the time taken for aid to be received. This article highlights the critical nature of getting aid to its target in a timely manner. Slow responses lead to serious negative outcomes, as Puerto Rican residents can attest. Be sure to read Echoes of Katrina: Post-Maria Public Health Threats and Trauma .
- Help & FAQ
Classroom Case Study: Modeling the Impacts of Hurricane María in Puerto Rico
- Administration, Leadership & Technology
- Urban Initiative
- Teaching and Learning
Research output : Other contribution
- Translanguaging
- Computer Science Education
- Bilingual Education
- Computational Literacies
- teacher professional development
Access to Document
- Fall 2020 - PiLa-CS - Hurricane Maria Case Study License: CC BY-NC-SA
Other files and links
- Resource home on the PiLa-CS website
Fingerprint
- Puerto Rico Social Sciences 100%
- classroom Social Sciences 44%
- grant Social Sciences 23%
- student Social Sciences 6%
T1 - Classroom Case Study: Modeling the Impacts of Hurricane María in Puerto Rico
AU - James, Sarane
AU - Silfa, Karen
AU - Vogel, Sara
AU - Ascenzi-Moreno, Laura
AU - Hoadley, Christopher
AU - Ma, Jasmine
PY - 2020/10
Y1 - 2020/10
N2 - In this middle school bilingual science unit, students used computational modeling in Scratch to aid their discussions about a topic that hit close to home for students: Hurricane María.Sponsored by the National Science Foundation under NSF grants CNS-1738645 and DRL-1837446. Any opinions, findings, and conclusions or recommendations expressed in this material are those of the author(s) and do not necessarily reflect the views of the National Science Foundation.
AB - In this middle school bilingual science unit, students used computational modeling in Scratch to aid their discussions about a topic that hit close to home for students: Hurricane María.Sponsored by the National Science Foundation under NSF grants CNS-1738645 and DRL-1837446. Any opinions, findings, and conclusions or recommendations expressed in this material are those of the author(s) and do not necessarily reflect the views of the National Science Foundation.
KW - Translanguaging
KW - Computer Science Education
KW - Bilingual Education
KW - Computational Literacies
KW - teacher professional development
UR - https://pila-cs.org/educator-resources
M3 - Other contribution
PB - Participating in Literacies and Computer Science (PiLaCS)
CY - New York

An official website of the United States government
The .gov means it’s official. Federal government websites often end in .gov or .mil. Before sharing sensitive information, make sure you’re on a federal government site.
The site is secure. The https:// ensures that you are connecting to the official website and that any information you provide is encrypted and transmitted securely.
- Publications
- Account settings
Preview improvements coming to the PMC website in October 2024. Learn More or Try it out now .
- Advanced Search
- Journal List
- Proc Natl Acad Sci U S A
- v.117(51); 2020 Dec 22

Quantifying the dynamics of migration after Hurricane Maria in Puerto Rico
Rolando j. acosta.
a Department of Biostatistics, Harvard T. H. Chan School of Public Health, Boston, MA, 02115;
Nishant Kishore
b Center for Communicable Disease Dynamics, Department of Epidemiology, Harvard T. H. Chan School of Public Health, Boston, MA, 02115;
Rafael A. Irizarry
c Department of Data Science, Dana-Farber Cancer Institute, Boston, MA, 02215
Caroline O. Buckee
Author contributions: R.J.A., N.K., R.A.I., and C.O.B. designed research; R.J.A. and N.K. performed research; R.J.A., N.K., and R.A.I. analyzed data; and R.J.A., N.K., and C.O.B. wrote the paper.
Associated Data
Facebook data are available to researchers and nonprofits pending a signed data use agreement at Facebook Data for Good ( https://dataforgood.fb.com/tools/disease-prevention-maps/ ). Teralytics data are available to researchers at nonprofits pending a data use agreement at Teralytics ( https://www.teralytics.net ). Airline data is freely available online at Indicadores.PR ( https://indicadores.pr/dataset/vuelos-pasajeros-aereos-y-carga-puerto-rico/resource/fc6d7591-7ccd-4332-9d44-991559912f70 ). ACS data is freely available online at US Census ( https://www.census.gov/quickfacts/PR ). Data to reproduce all figures can be found at GitHub ( https://github.com/RJNunez/pr-migration-paper ) ( 30 ).
Significance
Understanding the population composition and distribution of a region affected by a major natural disaster is vital for the allocation of resources to communities in need and critical to inform mortality estimates. Currently, the US Census Bureau is the only institution that publishes reliable population estimates for the United States and its territories. Since these are published once per year, it is impossible to use census-based population estimates to assess short-term postdisaster out-of-jurisdiction migration and within-jurisdiction migration. The utilization of social media traces, coupled with mobile phone data, could provide live estimates of postdisaster population changes in disaster-affected areas.
Population displacement may occur after natural disasters, permanently altering the demographic composition of the affected regions. Measuring this displacement is vital for both optimal postdisaster resource allocation and calculation of measures of public health interest such as mortality estimates. Here, we analyzed data generated by mobile phones and social media to estimate the weekly island-wide population at risk and within-island geographic heterogeneity of migration in Puerto Rico after Hurricane Maria. We compared these two data sources with population estimates derived from air travel records and census data. We observed a loss of population across all data sources throughout the study period; however, the magnitude and dynamics differ by the data source. Census data predict a population loss of just over 129,000 from July 2017 to July 2018, a 4% decrease; air travel data predict a population loss of 168,295 for the same period, a 5% decrease; mobile phone-based estimates predict a loss of 235,375 from July 2017 to May 2018, an 8% decrease; and social media-based estimates predict a loss of 476,779 from August 2017 to August 2018, a 17% decrease. On average, municipalities with a smaller population size lost a bigger proportion of their population. Moreover, we infer that these municipalities experienced greater infrastructure damage as measured by the proportion of unknown locations stemming from these regions. Finally, our analysis measures a general shift of population from rural to urban centers within the island. Passively collected data provide a promising supplement to current at-risk population estimation procedures; however, each data source has its own biases and limitations.
In the aftermath of natural disasters, both short-term population displacement and longer-term migration may occur, leaving some affected regions permanently altered ( 1 – 3 ). Measuring population displacement is a priority in the immediate days and weeks after an event like a hurricane or flood for the provision of aid and other supplies to communities in need. It is also critical to inform mortality estimates and other measures of public health interest that require an up-to-date denominator since census estimates may be rendered inaccurate ( 4 , 5 ). Measuring shifts in demographic composition and the geographic distribution of populations on longer timescales is also critical to rebuilding efforts and to the development of frameworks for building resilience to future disasters. Currently, however, few data sources can be used to rapidly assess and monitor population displacement in the short- and medium-term timescales after disasters happen ( 6 ).
In the absence of reliable migration data in the wake of natural disasters, the population size estimate used by government agencies and researchers generally relies on census estimates and assumes a linear change in population size between intervals or a constant population size since the most recent estimate ( 7 , 8 ). New approaches to estimating fluctuating denominators in near real time would greatly improve disaster response and the assessment of local needs in the short- and long-term aftermath. Rapid censuses conducted in short intervals before and after a disaster are both logistically and financially impractical. In an increasingly digitally connected world, however, passively collected digital records are often maintained by technological services providers for billing or marketing purposes. These data, such as flight information, mobile phone data, or social media traces, can provide insight into the fluctuation of their respective populations at a high temporal and geographic resolution, both before and after a disaster. Assuming that appropriate steps are taken to anonymize and aggregate these data streams in secure ways, novel data streams offer ways to assess the needs of populations more accurately ( 9 , 10 ).
Hurricane Maria made landfall in Puerto Rico as a category 4 storm on 20 September 2017, becoming the third costliest hurricane in US history ( 11 , 12 ). In the ensuing weeks, the damage to infrastructure caused by the storm resulted in a widespread lack of access to electricity, communication, and health services ( 13 , 14 ). Population displacement off the island and within Puerto Rico was widespread, although this was difficult to monitor directly. Direct and indirect mortality caused by the storm also increased in the months after the hurricane ( 8 , 13 – 17 ), but estimating mortality was made more complicated by the migration of populations because the population at risk in different parts of the island was shifting over time. Due to Hurricane Maria, the US Census Bureau ceased operations of the Puerto Rico Community Survey (PRCS; a monthly survey of 36,000 housing units across the island) from October to December 2017. Operations resumed in January 2018, with early results showing an island-wide increase in out migration in 2017 compared with 2016 ( 18 ). The most recent updates from the PRCS show a continued increase in out migration with a general population that decreased by 4% from 2017 to 2018 ( 19 ).
In this study, we evaluate two passively collected data sources and compare them with data on air travel and census data to evaluate the effects of Hurricane Maria on large-scale population fluctuation in Puerto Rico. We investigate the ability of these data sources to estimate the island-wide population at risk postdisaster and identify within-island geographic heterogeneity in population migration after the storm. We observe a nonlinear change in population at risk following Maria and show that this difference is affected by rurality. These passively collected data sources also provide insight into regions that are more heavily affected by disasters and can augment the resources available to first responders. Each data source has its own limitations and biases and could be used in conjunction with traditional census-based population estimates to improve the response to natural disasters, and to understand how to build more resilient systems in anticipation of future events.
Data Sources.
We compared four independent datasets to estimate population changes over the course of a year after the hurricane. First, we obtained the intercensal yearly population estimates provided by the American Community Survey (ACS) for 2010 to 2018, a yearly survey conducted by the US Census Bureau. The ACS estimates are for 1 July of each year. We considered this estimate to be our gold standard.
Second, we extracted data from Disaster Maps provided by Facebook’s Data for Good team. Specifically, for a group of people determined to be living in Puerto Rico the week before Hurricane Maria, weekly estimates from 21 August 2017 to 30 July 2018 on the number of these users residing in 77 of the 78 municipalities on the island were available. Note that these data are a closed cohort of known individuals, so no new users appear in the data, and there is a constant rate of attrition of users expected. However, this is also the only dataset on a municipality, rather than an island-wide, level.
Third, Teralytics provided island-wide daily population proportion estimates from 31 May 2017 to 30 April 2018 based on cell phone usage patterns, analyzed in partnership with an unnamed mobile operator on the island. Since we do not know which operator the data are from, it was impossible to assess the geographic bias in ownership in this group. The proportions were calculated relative to a baseline population determined by Teralytics using a method unavailable to the authors. Due to the unreliability of data for the 4 wk after Hurricane Maria, presumably due primarily to low connectivity, proportion estimates were not provided for this time period.
Finally, we obtained Airline Passenger Traffic (APT) data from the US Bureau of Transportation Statistics (BTS) through the Puerto Rico Institute of Statistics. The data are composed of monthly counts of passengers who arrived and left the island per month from January 2010 to February 2018. These data are unbiased, but they have a coarse temporal and geographic scale and cannot account for the same individuals on repeated trips or distinguish Puerto Rican residents from short-term aid workers and other visitors.
Details on how each of these datasets was constructed are included in Methods , and a side-by-side comparison is found in SI Appendix , Table S1 .
Population Decreased after Hurricane Maria.
We found agreement across all data sources of a consistent loss of population from 1 July 2017 to 1 July 2018; however, the dynamics and magnitude of the loss differ between data types ( Fig. 1 ). The ACS predicts a population loss of 129,848, a 4% decrease; the APT data predict a population loss of 168,295, a 5% decrease; the Teralytics data predict a population loss of 235,375, an 8% decrease; and the Facebook data predict a 17% decrease, which equates to a total estimated population loss of 475,779 on the island. In all cases, we observed a sharp drop after the hurricane. Both the APT data and Teralytics data show a rebound in population and stabilization after 31 December 2017. The Facebook data do not show stabilization until April 2018. We note that Facebook data are based on a closed cohort that is not able to measure population increases due to immigration to Puerto Rico. However, it does represent the cohort that likely experienced Hurricane Maria since the selection criteria used by Facebook’s algorithm ensure that transient populations, such as tourists, are not included in the closed cohort.

Population estimates for each data source.
Small Municipalities Lost a Larger Portion of Their Population Compared with Large Ones.
Facebook provided municipality-level data that allowed us to assess within-island migration. For each municipality, the data included weekly counts of individuals still in the municipality and new to the municipality. For privacy protection, the new to municipality data were not included if the number of users was below a prespecified threshold ( Methods has details on the data imputation techniques used, as well as a sensitivity analysis comparing different imputation approaches). We found that the share of users belonging to each municipality was highly correlated with the baseline population size of each municipality ( SI Appendix , Fig. S1 ). On average, municipalities with a smaller population size lost a bigger proportion of their population during the study period ( Fig. 2 ). The municipality of Toa Baja was a large outlier ( SI Appendix , Fig. S2 ) due to a reported surge of new individuals moving there at the end of the study period. Possible explanations for Toa Baja being an outlier are provided in Discussion . Apart from this outlier, San Juan, which is the capital of Puerto Rico, is the only municipality with more individuals at the end of the study period relative to baseline.

Small municipalities lost a larger portion of their population compared with large ones. ( A ) Relative change in the population of Facebook cohort members per municipality. Each curve corresponds to a different municipality. Population size in July 2017 is denoted by color. ( B ) Average percentage change in population at the end of the study period, relative to baseline, compared with the ACS population size of the municipality at baseline. The geographical regions are presented in SI Appendix , Fig. S5 . We fitted a linear model to each population curve and computed the average percentage change using the first and last fitted values. The vertical line corresponds to the day when Hurricane Maria made landfall in Puerto Rico.
Within-Island Migration Shifted Populations from Rural to Urban Regions.
All municipalities experienced loss of their baseline resident populations. Much of this loss is explained by off-island immigration ( Fig. 3 ). However, the locality gaining the most new residents was San Juan ( Fig. 3 ). In fact, for urban areas, the baseline resident population loss was compensated by in migration from other municipalities. For example, San Juan experienced a 19% loss of its baseline resident population but ended the study period with a 7% increase in total population, suggesting an in migration of 26% by the end of the study period. Another appealing aspect of these data is that they provide information on the destination of those displaced ( Fig. 3 B ). Note that the top two destinations for Facebook users in our cohort were Miami, FL, and Jacksonville, FL, respectively. These results taken together suggest a migration pipeline from rural to urban municipalities and likely off island.

Immigration of Puerto Rican Facebook (FB) cohort members within and outside of Puerto Rico. ( A ) Top six municipalities in Puerto Rico in terms of influx of FB cohort members who were located elsewhere before Hurricane Maria. ( B ) Top five destinations of FB cohort members after Hurricane Maria. The “Others” curve corresponds to the influx of all other destinations together. The vertical lines correspond to the day when Hurricane Maria made landfall in Puerto Rico.
Infrastructure Damage Was Greater in Rural Areas.
Disaster Maps provided information about the proportion of people whose location is unknown for a particular week. Unknown locations may be the result of 1) people stopping use of Facebook, 2) people having changed their Facebook behavior, or 3) loss of electricity and communication infrastructure. In the first full week of data collection immediately after the hurricane, approximately half of the cohort members did not register a location ( Fig. 4 A ), likely due to the widespread loss of infrastructure. During this week, the proportion of users with reported unknown locations was substantially higher in the rural areas compared with in urban areas ( Fig. 4 B and SI Appendix , Fig. S3 ). We focused our analysis to only include the week immediately following the impact of Hurricane Maria to ensure that any loss of individuals in a region was not likely to be due to population migration. This is especially important as there was widespread damage to roads and loss of transportation infrastructure during this time ( 12 ).

Facebook users with unknown locations. ( A ) Percentage of individuals whose location was unknown by municipality. The vertical line denotes the day Hurricane Maria made landfall. Baseline population size is denoted in color. ( B ) Percentage of individuals with unknown location relative to San Juan for the week of 2 October 2017, the week after Hurricane Maria. The municipality in white represents Las Marias, the one municipality for which we had no data.
In SI Appendix , Table S2 , we show the top 15 municipalities that had a greater proportion of cohort members with their location unknown compared with the island-wide average. While rurality is directly linked with availability of infrastructure, the Facebook data highlight the heterogeneity in loss of access to resources in the immediate aftermath of a disaster. Some municipalities continued to have missing data relative to the island-wide average for many months.
We show that passively collected data sources for estimating population displacement may provide insights into the dynamics of migration following a natural disaster that cannot be obtained using traditional methods. Our results point to a consistent and long-term loss of population in Puerto Rico after Hurricane Maria. In the Teralytics data and the flight data, the decrease in population levels off in December 2017, and in January 2018, we see a rebound in Puerto Rico’s population. In the Facebook data, the population loss continues until April 2018, resulting in the lowest overall population estimate. Both datasets provided island-wide population estimates that were lower than the ACS vintage 2018 point estimate. The discrepancies in population estimates are explained, in part, by the different sources. For example, the Facebook data constitute a closed cohort, whereas the Teralytics and flight data are two open cohorts with different inclusion and exclusion dynamics, and ACS data are based on surveys carried out on the island ( SI Appendix , Table S1 ). Nevertheless, taken together, these data sources highlight the dynamic and consistent population displacement in the wake of the hurricane.
The decline in the population before Hurricane Maria may seem surprising but a closer inspection of the context yields two explanations for this phenomenon. 1) The dwindle in population coincides with the end of summer; hence, we expect tourists and visitors to leave the island around this time ( 20 ). Note that this is captured by both the Teralytics and APT data, which is expected. 2) The sharp decrease beginning a few weeks before the storm may be explained by the close encounter with Hurricane Irma, which scraped the northeastern part of the archipelago ( 21 ). Population displacement estimates based on the demographic balancing equation yield similar results ( 22 ). Although each has its own biases, these passively collected data estimates follow similar trends and show dynamic population fluctuations. In all cases, this decrease in population is markedly different from the trend since 2010 ( SI Appendix , Fig. S4 ).
Our findings further suggest a rural–urban shift in population. Using Facebook data to analyze within-island movements, we observed persistent migration from rural to urban areas of Puerto Rico. This may be explained by individuals migrating from rural to urban areas in search of basic needs in the short term and staying due to increased access to resources in the long term. Out migration from rural areas continued in the Facebook cohort throughout the available data, becoming more concentrated in urban areas. Previous household surveys suggest greater out migration among younger individuals, potentially changing the demographic distribution of rural vs. urban regions in important ways ( 14 ).
In the immediate aftermath of a disaster, electricity, communication, and transportation are often affected, leading to sparse information on the areas most heavily affected. Assuming that individuals will continue to use services like mobile phones and Facebook if they had access, the lack of interaction with these services could serve as a proxy for damage from the hurricane. Spatial and temporal granularity in these immediately available datasets could augment satellite imagery and primary data sources to more readily target priority areas for response. In the Facebook data, we can see that in the weeks immediately following Hurricane Maria, we identify areas that had larger proportions of cohort members whose locations were unknown compared with San Juan. Travel in most of these areas was impossible due to debris that was blocking streets and highways. Hence, this finding is not confounded with the rural–urban shift in the population described above. These areas coincide with rural municipalities found to be some of the first declared as disaster zones posthurricane ( 23 ).
Passively collected data provide a promising supplement to current at-risk population estimation procedures; however, each data source has its own biases and limitations. The population estimates from Facebook vs. Teralytics diverged significantly both before (by >9,500 people on 20 September 2017) and after (by >210,000 people on 20 April 2018) the hurricane. These data are not necessarily comparable since neither the market share of mobile phone providers nor Facebook users are likely to be perfectly representative of the overall population. In general, we expect those not included in the Facebook or Teralytics sample to be those without phones or internet access, which would likely include children, the elderly, and the very poor ( 24 ).
For privacy reasons, we were unable to analyze the demographic composition of the Facebook cohort, but these data represent a meaningful proportion of the Facebook population ( 25 ). Further, we showed that the percentage of users from each municipality is highly correlated with the baseline population size. The Facebook data also represent a closed cohort, unlike the other sources. Membership into this group is defined during the 5-wk precrisis period, and no individuals can enter afterward. This means that this cohort will inherently only decrease in size. This rate of decrease is driven by 1) the baseline mortality rate, 2) the excess mortality due to the crisis, and 3) the baseline rate of discontinuation of Facebook use. One important advantage of Facebook data is the ability to analyze within-island movement patterns, which is not possible using flight or Teralytics estimates. Teralytics analyzed mobile phone data from a particular mobile operator of unknown market share and uses a proprietary algorithm to generate their population estimates, precluding any evaluation of primary sources or inherent biases. In both cases, it is impossible to accurately quantify the direction or magnitude of the biases. We have specified the benefits and drawbacks of each data source in SI Appendix , Table S1 .
Despite the limitations of each dataset, passively collected data sources still provide a useful estimation of population displacement. The imputation procedure and the normalization of each dataset using baseline population values aim to ameliorate some of these limitations ( Methods ). The limitations of Facebook’s data suggest that we are underestimating the true population size. However, the imputation procedure introduced here aims to mitigate that. Further, Facebook has expanded and introduced new datasets that do not suffer from the same limitations as the one used here ( 26 ). Research is needed to assess the capability of those datasets to estimate population size, with particular focus on expanding the study window to include data from previous or subsequent years and perhaps incorporate other similar regions during the same period for comparison. Given the paucity of data available immediately after a disaster, these data streams provide a clear benefit over the gold standard estimate for humanitarian purposes.
Our data show that emigration after disasters has a nonlinear effect on the count of population at risk and that this emigration is heterogeneous by rurality, affecting the denominators of many key population statistics. As interest in passively collected data grows and these tools are further refined to overcome current limitations, they can provide a more temporally and spatially nuanced picture of population movements after disasters.
We obtained yearly population estimates from 2010 to 2018 from the intercensal yearly population estimates provided by the ACS, a yearly survey conducted by the US Census Bureau. Specifically, we have the vintage 2018 estimates that were published last year.
Facebook is a social media company with over 2 billion monthly active users. In 2017, the Data for Good team within Facebook launched Disaster Maps with the goal of aiding response organizations with information vital to optimal resource allocation in postdisaster settings. These maps are built using privacy-preserving techniques including aggregation and deidentification that protect individual privacy. The Harvard team accessed these data free of charge through Data for Good’s standard license agreement, which allows partners working in humanitarian operations and research to improve their work through the use of Disaster Maps.
For this study, we used the original version of Facebook’s Displacement Maps, a specific product within Disaster Maps ( 27 ), which has since been updated to improve its data sources and methodology. However, work is needed to assess the functionality of these improvements in the context described here. Displacement Maps are generated by first defining a geographic bounding box along with an index date defining the disaster event of interest. In this case, the geographic bounding box consisted of the entire island of Puerto Rico, and the index date was 20 September 2017, the day that Hurricane Maria made landfall on the island. In these data, Puerto Rico is divided into 188 nonoverlapping geographical tags (geotags), and users are assigned a home location defined as the geotags where they had the most interactions with the Facebook platform through a browser during the 5 wk prior to the index date. In the original Displacement Maps methodology, the location of these interactions was determined from associated internet protocol addresses; the new methodology instead utilizes location-based data from cell phones. Displacement Maps generate a closed population consisting of people using Facebook satisfying the following two conditions: 1) they registered an interaction with Facebook services from in the geographic bounding box during the 5 wk preceding the index date, and 2) they were present in their home location during the week before the disaster ( 28 ).
They then followed this cohort through July 2018 and calculated the most commonly occurring geotag each week, aggregating total numbers of cohort members per geotag. Geotags with fewer than 100 people at baseline were excluded to protect privacy. Then, for each of the 49 wk after the index date, the dataset includes the number of crisis-affected people in their home location, the number of new users, and the number of unknown users for each geotag. Cohort members were defined as having an unknown location if they did not register an interaction during the week for which data were aggregated. For our results, we assumed these people with unknown locations were in their home location. We then combined geotag counts into Puerto Rico’s 78 municipalities. Due to low counts, the municipality of Las María had no data. Below, we describe how we imputed other missing data.
Teralytics.
Teralytics is a tech company that works with governments and private clients to assess human movement by partnering with mobile network operators. Specifically, the partnership allows the company to access and analyze the data that cell towers receive from mobile devices. From them, we obtained island-wide daily population proportion estimates relative to an undisclosed baseline from 31 May 2017 to 30 April 2018, based on all subscribers of a major undisclosed telecom company that created events in Puerto Rico. Events were defined as signal exchanges between a cell phone and the nearest cell phone tower. These signal exchanges occurred, for example, when a phone call was made or a text message was sent. The data were filtered by the provider, and only subscribers with activity all over the Teralytics analysis period were considered (31 May 31 2017 to 30 April 2018). Activity was defined as an event on at least 10 d/mo for all 12 mo. Due to the unreliability of data, proportion estimates for 4 wk after Hurricane Maria were not provided. For every day, a distinct number of subscribers in Puerto Rico is computed by considering events generated from different mobile devices. It is important to note that Teralytics is a commercial company that operates by competitively sourcing, cleaning, and preprocessing these data. Therefore, much of this analytic pipeline is proprietary and a black box to researchers. We have taken measures in our analysis to more readily index and compare this source with others; however, as noted in SI Appendix , Table S1 , all of these sources have their benefits and drawbacks.
Aviation Records.
Finally, through the Puerto Rico Institute of Statistics we obtained APT data from the US BTS. The data are composed of monthly counts of passengers who arrived and left the island per month from January 2010 to February 2018. The per-month difference between these two numbers will be referred to as net migration. We added the monthly net migrations to the vintage 2017 population estimates corresponding to the same date and interpolated using a linear model between these data points. This resulted in daily population estimates from July 2010 to July 2018 that account for flight passenger movement ( 29 ).
SI Appendix , Table S1 has a side-by-side comparison of all of the data sources. This research proposal was reviewed by the Harvard T. H. Chan School of Public Health Institutional Review Board and was deemed exempt as nonhuman subjects research.
Estimating Population Size.
We estimated island-wide population sizes using each of the four data sources. For the ACS data, we simply interpolated the points for each year ( SI Appendix , Fig. S3 ). For the other three sources, we defined population size estimate N t for time t using the following:
where N 0 = 3,325,001 , the ACS population estimate for 1 July 2017; m t is a source-specific measurement for time t ; and m 0 is a source-specific baseline. For the APT data, baseline was defined as 1 July 2017, m t corresponds to the sum of N 0 and the cumulative net passenger movement for month t , and m 0 is the sum of N 0 and the cumulative net passenger movement at baseline. The formula above, therefore, is simply the cumulative sum, starting on 1 July 2017, of passengers arriving and passengers leaving:
where i represents the i th month after baseline and p a s s e n g e r o u t i and p a s s e n g e r i n i are the passengers leaving and entering Puerto Rico in month i , respectively. For the cell phone data, the baseline was also defined as 1 July 2017; m t and m 0 represent the proportions provided by Teralytics for time t and the proportion corresponding to baseline, respectively. Finally, for the Facebook data baseline was defined as 21 August 2017, which corresponds to the first observation in the dataset. Here, m t corresponds to the Facebook population at time t , and m 0 represents the Facebook population at baseline.
For the municipality-level data, we aggregated the city-specific data from Displacement Maps by municipality in Puerto Rico while maintaining the temporal resolution at weeks. For all analyses, we assumed that cohort members who were defined as unknown for the week were present in their home town locations and were either unable to or chose not to interact with Facebook services using a browser in that period.
To evaluate the utility of Displacement Maps, a tool to target municipalities for resource allocation, we evaluated the proportion of the population with unknown locations every week compared with baseline. Our primary assumption here is that in the immediate aftermath of a disaster, any discontinuation of interaction with Facebook services defined at baseline would primarily be caused by loss of access to infrastructure, death, or other factors related to the event. Therefore, a higher proportion of municipality-specific cohort members whose location was unknown would be a proxy for impacts of the disaster in that region.
Imputation.
In Facebook’s dataset, Puerto Rico is divided into 188 nonoverlapping geotags. For each geotag, the dataset contains the number of crisis-affected people in their home location at time t , denoted here as home; the number of new users at time t , denoted here as new; and the number of users whose location is unknown at time t , denoted here as unknown. For privacy-preserving reasons, geotags were excluded from the data if they had fewer than 100 Facebook cohort members at time t . We, therefore, applied an imputation approach along with a sensitivity analysis to provide intervals of possible values. First, we computed the distribution of the maximum number of consecutive new missing values for each geotag. Thus, the j th geotag had a corresponding value, c j ( n e w ) , that represents the maximum number of consecutive weeks when the new variable was missing. Second, we denote a threshold τ that corresponds to the maximum number of successive weeks we are willing to accept and a default imputation value δ to be used later. Then, we split the data into the geotags that comply with the threshold and those that did not [i.e., if c j ( n e w ) ≤ τ, then geotag j complies with the threshold]. For the geotags that complied with the threshold, we imputed the missing values using a linear interpolation of the observed values. For the geotags that did not comply with the threshold, we imputed the missing values with δ . Finally, we computed the distribution of the maximum number of consecutive home missing values for each tag. Hence, similar to above, the j th geotag has a corresponding value, c j ( h o m e ) , that corresponds to the maximum number of consecutive weeks when the home variable was missing. Then, we excluded all geotags where the newly computed statistic was greater than τ ( SI Appendix , Fig. S6 ). For our results, we used τ = 8 and δ = 99 ( SI Appendix , Fig. S7 ). Note that δ = 99 is the maximum number that the missing values can take. We conducted a sensitivity analysis where δ = 0, the minimum number that the missing values can take ( SI Appendix , Fig. S8 ). Our sensitivity analysis shows that the results do not change much.
Supplementary Material
Supplementary file, acknowledgments.
We thank Teralytics for providing the mobile phone data used in this project; specifically, thanks to Canay Deniz, Lara Montini, and Andrea Samdahl for the discussions. We also thank Facebook for providing the displacement data used in the project; specifically, we thank Shankar Iyer, Laura McGorman, Paige Maas, and Facebook’s Data for Good team for lengthy discussions of their algorithm and possible biases. Finally, we thank Deepak Lamba-Nieves for suggesting readings regarding migration in Puerto Rico.
The authors declare no competing interest.
This article is a PNAS Direct Submission.
This article contains supporting information online at https://www.pnas.org/lookup/suppl/doi:10.1073/pnas.2001671117/-/DCSupplemental .
Data Availability.

- 22.8 inches in Dominica
- 38 inches in Puerto Rico
- 10-13 inches in Guadeloupe, Dominican Republic
- Three small unconfirmed tornadoes observed by a storm chaser in Puerto Rico.
- N/A in Dominica
- 6-9 ft. in Puerto Rico
- 1-3 ft. in North Carolina
- Dominica: At least $1.31 billion.
- Guadeloupe: $120 million.
- Puerto Rico: $90 billion (90% confidence interval of $65-$115 billion). Costliest hurricane in modern Puerto Rican history.
Thank you for visiting nature.com. You are using a browser version with limited support for CSS. To obtain the best experience, we recommend you use a more up to date browser (or turn off compatibility mode in Internet Explorer). In the meantime, to ensure continued support, we are displaying the site without styles and JavaScript.
- View all journals
- My Account Login
- Explore content
- About the journal
- Publish with us
- Sign up for alerts
- Open access
- Published: 25 March 2019
Hurricane María tripled stem breaks and doubled tree mortality relative to other major storms
- María Uriarte ORCID: orcid.org/0000-0002-0484-0758 1 ,
- Jill Thompson 2 &
- Jess K. Zimmerman 3
Nature Communications volume 10 , Article number: 1362 ( 2019 ) Cite this article
6119 Accesses
83 Citations
97 Altmetric
Metrics details
- Climate-change ecology
- Community ecology
- Forest ecology
An Author Correction to this article was published on 01 May 2019
This article has been updated
Tropical cyclones are expected to intensify under a warming climate, with uncertain effects on tropical forests. One key challenge to predicting how more intense storms will influence these ecosystems is to attribute impacts specifically to storm meteorology rather than differences in forest characteristics. Here we compare tree damage data collected in the same forest in Puerto Rico after Hurricanes Hugo (1989, category 3), Georges (1998, category 3), and María (2017, category 4). María killed twice as many trees as Hugo, and for all but two species, broke 2- to 12-fold more stems than the other two storms. Species with high density wood were resistant to uprooting, hurricane-induced mortality, and were protected from breakage during Hugo but not María. Tree inventories and a wind exposure model allow us to attribute these differences in impacts to storm meteorology. A better understanding of risk factors associated with tree species susceptibility to severe storms is key to predicting the future of forest ecosystems under climate warming.
Similar content being viewed by others
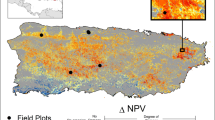
Hurricane-Induced Rainfall is a Stronger Predictor of Tropical Forest Damage in Puerto Rico Than Maximum Wind Speeds
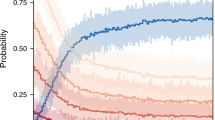
Excess forest mortality is consistently linked to drought across Europe
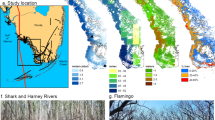
Storm surge and ponding explain mangrove dieback in southwest Florida following Hurricane Irma
Introduction.
Cyclonic storms (hurricanes, cyclones, and typhoons) represent the dominant natural disturbance in coastal tropical forests across the Caribbean, the Indian subcontinent, Southeast Asia, Indo-Malaysia, and northern Australia 1 . Since these storms derive their energy from ocean heat, and sea surface temperatures have increased in most regions of tropical-cyclone formation during the past decades, maximum wind speeds are projected to rise and storms to intensify 2 , with some of the most significant increases in the North Atlantic 3 , 4 , 5 . Climate warming has also led to higher atmosphere moisture content, which is expected to increase tropical-cyclone rainfall rates 6 . Models predict that by 2100 in the North Atlantic basin, maximum sustained hurricane wind speeds will increase by 6–15%, coupled with increases of 20% in precipitation within 100 km of the storm center 7 . While it is difficult to attribute any specific storm to the effects of a warming climate 4 , 8 , the extremely active 2017 hurricane season in the North Atlantic, with Harvey in Texas and Irma and María in the Caribbean and Florida, portends some of the projected shifts in hurricane regimes under a warming climate.
The expected changes in hurricane winds and rainfall may have profound consequences for the long-term resilience of tropical forests in the North Atlantic basin. The challenge to predicting how particularly severe storms, such as Hurricane María, will influence tropical forest ecosystems is to determine whether any change in observed tree damage and mortality could be attributed to differences in storm characteristics (i.e., wind speed and rainfall) rather than to differences in topographic exposure to wind or the structure and composition of forests at the time the storm struck. Here we employ tree damage and mortality data collected after three storms in a secondary tropical forest in Puerto Rico that developed after human disturbance during the first half of the 20th century 9 . We use these data to evaluate the effects of differences in wind speeds and tropical-cyclone rainfall rates on the forest and to identify the risk factors that moderated species vulnerabilities to storms of varying severities. Data derive from the 16-ha Luquillo Forest Dynamics Plot (LFDP) after three hurricanes: Hugo in 1989, Georges in 1998, and María in 2017 (Fig. 1a ). At the time of landfall on the island, Hurricane Hugo was a category 3 storm with wind speeds of 166 Km/hr and total rainfall of ca. 200 mm 10 . Hurricane Georges was also a category 3 storm with wind speeds (144 Km/hr) and total rainfall (ca. 200 mm) 11 similar to those observed for Hugo. In contrast, Hurricane María struck the island as a category 4 storm with sustained winds up to 250 Km/hr and 500 mm of precipitation fell over 24 h 12 . María transformed tropical forests across the island into leafless tangles of damaged and downed trees (Fig. 1b ). María was the strongest hurricane to make direct landfall in Puerto Rico since Hurricane San Felipe in 1928 13 and presages what climate warming will mean for hurricanes in the North Atlantic.
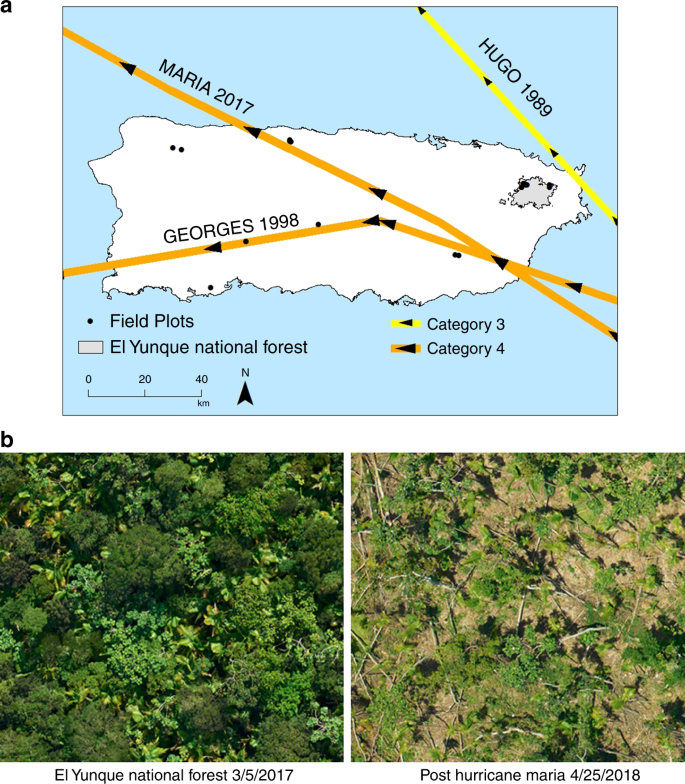
Tracks of Hurricanes Hugo, Georges, and María ( a ); and aerial photographs of the study area in El Yunque National Forest before and after Hurricane María ( b ). Images courtesy of D. Morton, NASA
Our results show that María killed twice as many trees as Hugo, and for all but two species, broke 2- to 12-fold more stems than the other two storms. Extensive tree inventories and a wind exposure model allow us to ascribe these differences in impacts to variation in storm meteorology (i.e., wind speed and rainfall). Tree species with high density wood were particularly resistant to uprooting and hurricane-induced mortality in all storms, but were protected from breakage during Hugo but not María. Large trees were also more likely to break in María but not Hugo. Taken together, these results suggest that more severe storms expected under a changing climate can alter species composition and size composition of these forests.
Stem damage and mortality
Hurricane María killed twice as many stems as Hugo in the LFDP (Fig. 2 , Supplementary Table 1 – 3 ). The proportion of uprooted stems, however, was similar for Hugo and María but lower for Georges. The most striking difference between María and the other two hurricanes was a 2- to 12-fold increase (average = 3.27-fold) in species-specific stem break rates for all but two species, the palm Prestoea acuminata var montana , and the pioneer species Cecropia schreberiana (Supplementary Table 3 ). Despite differences in history, structure and composition of the forest was remarkably similar ahead of each of these hurricanes. The large disparity in the impacts of María compared to the other two storms cannot be explained by differences in tree diameter sizes in the forest at the time of impact (Supplementary Figures 1 and 2 , Supplementary Table 4 ). Exposure of each tree in the LFDP to wind was calculated using the EXPOS model (see Methods) for the three hurricanes and exposure of the forest to storm winds was far greater during Hurricane Hugo than during Georges or María (Supplementary Figure 3 ) because of the track of the storms across the island and the position of the study site (Fig. 1a ). Consequently, plot position relative to the storm track does not account for the observed differences in damage among storms.
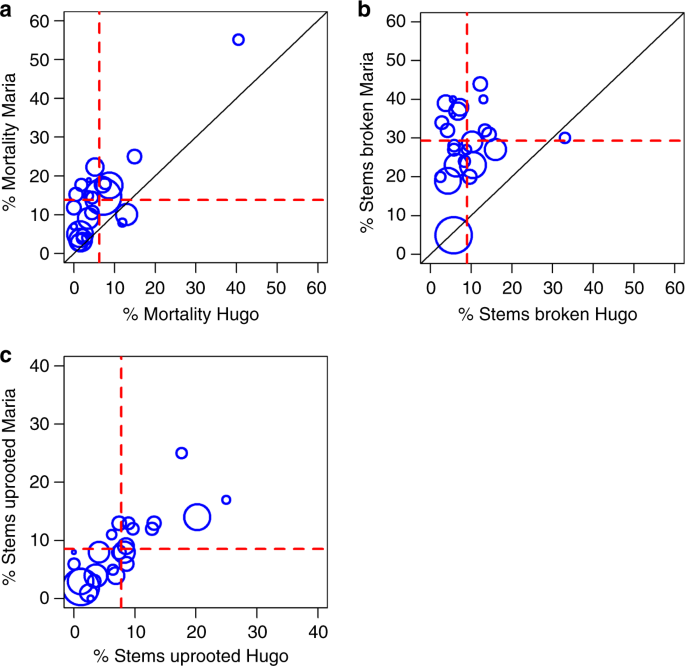
Rates of immediate mortality ( a ), stem break ( b ), and uprooting ( c ) as a result of Hurricanes Hugo (1989) and María (2017) for 24 tree species. Size of circles is proportional to the number of stems. Dashed red lines indicate community-average rates
Models of the probability of individual stem breakage or mortality that incorporated diameter size and wind exposure further reinforce the notion that differences in the effects of the three storms did not reflect differences in forest structure at the time of impact nor in topographic exposure to hurricane-force winds (Supplementary Table 5 ). Rather, differences in rates of stem break reflect to a large degree higher vulnerability of large diameter trees during H. María, suggesting that differences in the meteorological characteristics of the hurricanes were responsible for the striking differences in rates of stem break among storms (Fig. 3 , Supplementary Table 6 ).
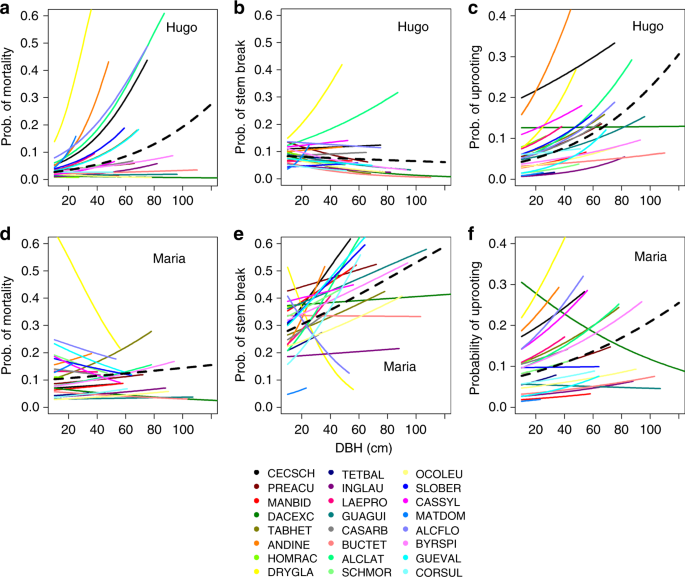
Relationship between species diameter and rates of stem break ( a , d ), uprooting ( b , e ), and mortality ( c , f ) in H. Hugo and María. Black dashed lines depict community-wide rates. Individual species estimates are restricted to the maximum observed dbh in the data
Selective pressure of hurricanes on tree species
Hurricanes exert selective pressure on forests by damaging some species more than others 14 . Insight into the drivers of the observed disparities in damage between the three storms can be derived from examining variation in species responses to the storms. At the study site, the only two species to exhibit similar responses to the three hurricanes were the palm P. acuminata and the pioneer species C. schreberiana; the palm had the lowest rates of stem break in both storms and C. schreberiana had the highest (Supplementary Table 3 ). Rates of damage for most species were generally lower for H. Georges (Supplementary Table 3 ) but this hurricane damaged a smaller area of the forest than Hugo and María and we had a less damage data for Georges. The abundance of P. acuminata doubled, and C. schreberiana quadrupled between 1995 and 2016 (Supplementary Table 1 ), suggesting that Hurricanes Hugo and Georges increased the number of individuals of these species in the forest.
We examined the association between species-specific characteristics, namely maximum tree height, specific leaf area, and wood density, and the rates of mortality and modes of damage in Hurricanes Hugo, Georges, and María. Species with high wood density suffered lower immediate mortality during both Hugo and María and were less likely to uproot during María, or break during Hugo (Fig. 4 , Supplementary Table 7 ). During H. María, however, dense wood did not afford species any protection from stem break as there was no differences between rates of stem break between species with high and low-density wood. Rates of stem break, uprooting, and mortality over periods free of storms were also unrelated to wood density (Supp. Tables 3 and 7 ).
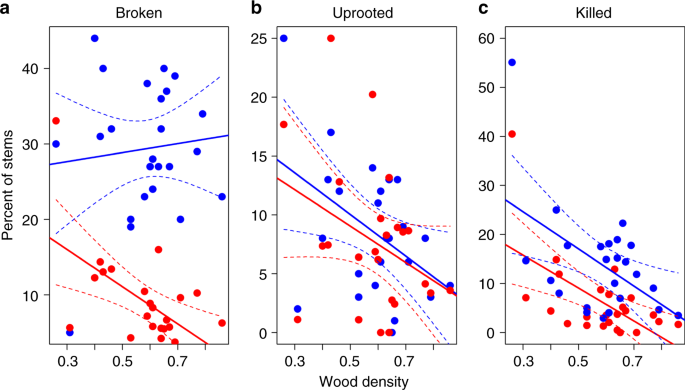
Relationship between wood density and rates of stem break ( a ), uprooting ( b ), and immediate mortality ( c ) for 24 tree species during Hurricanes Hugo (red dots) and María (blue dots). Dashed lines indicate 95% confidence intervals
Lagged tree mortality from hurricane damage
Windstorms are prominent causes of tree mortality 15 . Background mortality rates for trees > 10 cm diameter at breast height in wet tropical forests range between 1 and 2% per year and rarely exceed 3% 15 , 16 , 17 . Because coastal and island tropical forests often experience large catastrophic windstorms, species are adapted to these disturbances and tree mortality rates even after a severe windstorm tend to be low, ranging between 7 and 12%, only 2–3 times background mortality rates 15 , 18 . Wind induced mortality, however, is often delayed and results from branch and canopy damage during storms 19 , 20 , 21 . To estimate potential rates of delayed mortality resulting from stem breakage during María, we used census data collected in 1995 after the passage of Hurricane Hugo. In the 5 years following Hurricane Hugo, 30% of broken stems and 55% of those uprooted during the storm died (Fig. 5 and Supplementary Table 8 ). Although these numbers to some degree reflect stand dynamics following the storm 20 , they provide a reasonable estimation of the likely effects of damage from María on the fate of severely damaged trees.
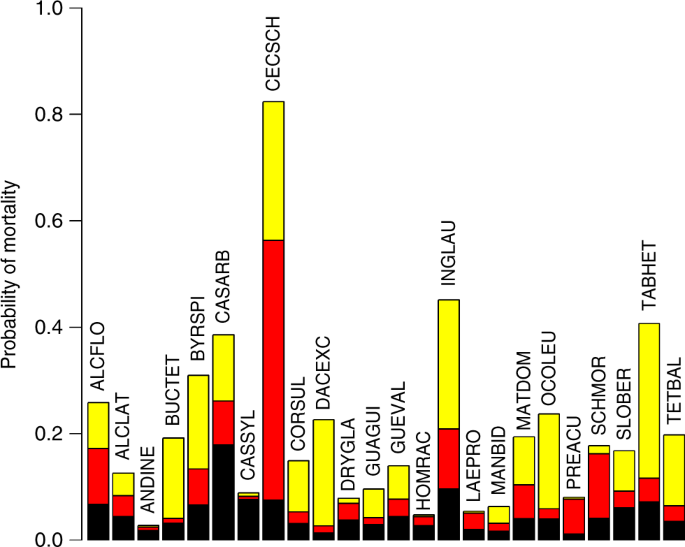
Background (black) and hurricane-induced mortality, immediate (red) and delayed (yellow), from Hugo. Delayed mortality rates are estimated for trees ≥ 10 dbh that were present at the time of the storm and damaged and died between the completion of the damage assessment in 1991 and the 1995 census. Background mortality represent tree mortality over the same period for undamaged trees
The large disparity in the impacts of María compared to the other two storms cannot be explained by differences in tree diameter sizes in the forest at the time of impact or differences in wind exposure. Hurricane María combined extreme precipitation and strong winds that may have reduced soil stability and cohesion and thus root anchorage, while simultaneously exerting a strong dynamical force on the stem and crown. Dynamic wind loadings typically drive roots to bend and twist as a result of the rotational pivoting of the trunk, and if the wind is strong enough, it will lead to uprooting 22 . Pre-hurricane soil moisture in particular is a major controlling factor in the nature of damage (uprooting vs. stem breakage) 23 . In dry soils, stem breakage is the dominant type of damage while in wet soils uprooting is more common 23 . Given the fact that H. Hugo and Georges were considerable drier than María, it is surprising that uprooting rates were not higher during María than the other two storms. This observation coupled the sharp increase in stem breaks during H. María, particularly for larger trees, may have been driven by the passage of Hurricane Irma, a category 4 storm that skirted the north of the island on 7 September 2017, 2 weeks before 20 September, when María struck the island. Although Irma did not make landfall on Puerto Rico (Fig. 1 ), it removed a substantial amount of tree foliage (Zimmerman, pers. obs), possibly reducing wind drag forces over the canopy and how such forces are transmitted to the base of the tree, favoring stem break over uprooting 22 .
The impact of each of the severe hurricanes that have affected the Luquillo forest between 1989 and 2017 may not be independent. The amount of damage depends on the state of the forest after the previous hurricane and the degree of recovery from prior storms. In particular, trees present when Hugo struck the forest had developed over 57 years without hurricane damage and likely had larger crowns with greater wind resistance that results in greater damage (e.g., uprooting). Although diameter distributions for the majority of species did not differ significantly between H. Hugo and Georges, it is likely that some of the old and large trees damaged during H. Hugo had smaller crowns and were then less affected by H. Georges, possibly accounting for lower rates of damage during this storm. Twenty years had passed between H. Georges and María, allowing the canopy to recover. Nevertheless, loss of foliage to H. Irma may have shaped the type of damage (i.e., a greater number of stem breaks) María inflicted on the forest. It is also plausible that other factors (e.g., biomechanical properties of tree species) underlie the different impacts observed across the three storms.
Species vulnerability to hurricanes depends on the strength of wood, stem diameter, shape and size of the crown, leaf features, and extent and depth of root system 24 , 25 , 26 . Species with weaker wood 27 , higher canopy-diameter ratios, and shallower root systems 28 generally suffer greater damage and mortality. Previous work after Hurricane Hugo and Georges at the site examined the relationship between damage and successional specialization and our results corroborate those findings: pioneer species with low-density wood are generally more vulnerable to hurricanes than old-growth high wood density specialists 24 , 25 . Our results however, also demonstrate that species with high density wood and large trees, although largely resistant to breakage in less severe storms, may be vulnerable to stem break during hurricanes of María’s severity. Given high rates of mortality of broken and uprooted stems, it is likely that trajectories of forest recovery from H. María will substantially diverge from recovery from Hugo, with consequences for both size structure and species composition of the forest.
Predicting the effects of climate warming on wind disturbance regimes and their effect on forest faces a number of challenges 29 . The susceptibility of forest ecosystems to wind damage is determined by tree and stand characteristics as well as site factors 23 . A number of studies have identified factors for tree species 24 , 25 , 26 but our understanding of the biomechanical characteristics of trees and how they relate to other aspects of tree life histories and physiology, is extremely limited. Successional specialization is of particular interest because second-growth forests account for over 70% of forest cover in tropical regions 30 and generally have a greater proportion of low wood density pioneer type species that may be more prone to storm damage. Predicting how these ecosystems will fare under a warming climate will require a more nuanced understanding of the relationship between successional specialization and the biomechanical characteristics that moderate species and forest vulnerability to hurricanes. A second, and perhaps more urgent issue that we highlight in this paper is that risk factors associated with resistance to an ‘average’ storm (i.e., high density wood) may be quite different than those mediating impacts of the more severe storms forecasted under warming. Cyclonic storms select for windstorm resistance [e.g. 14 ,] and the rapidity of change in storm disturbance regimes may exceed the capacity for adaptation of the forest communities 31 , yielding a depauperate forest dominated by a few pioneers (e.g., C. schreberiana ) and wind resistant species (e.g., palms) that can withstand high severity storms. Our results also demonstrate that large trees are particularly vulnerable to storms of María’s severity, presaging potential shifts in the size structure and carbon storage potential of these forests.
The Luquillo Forest Dynamics Plot (LFDP) is a 16-ha permanent, mapped forest plot located in the Luquillo Mountains of northeastern Puerto Rico (SW corner 18° 20’ N, 65° 49’ W). The forest is classified as subtropical wet in the Holdridge life zone system 32 . Vegetation and topography of this research area are typical of the tabonuco ( Dacryodes excelsa ) forest zone. Rainfall averages 3500 mm per year. Elevation ranges from 333 to 428 m a.s.l. All of the soils are formed from volcaniclastic rock. The forest has experienced substantial natural and human disturbances during the past century. Prior to 1934, parts of the LFDP were subjected to light logging and agriculture, but the forest structure and canopy cover had substantially recovered when in 1989, after a period of 57 years with no major storm, Hurricane Hugo struck the forest 9 . Basal area was estimated to average 36.7 m 2 ha −1 at the time of hurricane Hugo, 30.85 m 2 ha −1 at the time Georges struck, and 38.37 m 2 ha −1 in 2016, the year before María struck the forest.
Tree damage data in the LFDP was collected over a maximum 15 months following each of the three hurricanes. The surveys recorded several qualitative and quantitative observations on tree damage resulting from the hurricane, such as uprooting or stem break, and any type of damage to stems, tree crowns and branches (see ref. 24 for details). To assess damage and immediate mortality from H. Hugo, a survey of all trees ≥ 10 cm diameter at breast height (dbh = diameter at 1.3 m from the ground) was conducted between 1990 and 1991. All trees in the plot were surveyed again in 1995–1996 and we used these data to assess the effects of damage during Hugo on delayed mortality. Following Hurricane Georges in 1998 damage to woody stems ≥ 10 cm dbh was assessed in 40 subplots (20 × 20 m in size) in a grid pattern (60 m spacing) distributed regularly across the LFDP rather than for the entire plot. In 2018, we surveyed damage and immediate mortality from Hurricane María for all trees ≥ 10 cm dbh in the LFDP.
Wood density (g −1 cm −3 ) and specific leaf area (SLA, cm −2 g −1 ) measurements were collected for at least 10 individuals per species using standard protocols 33 . Briefly, leaf area was measured on sun-lit foliage of mature individuals and leaves were dried for 48 h and weighed to calculate specific leaf area (SLA). For wood density calculations tree cores were extracted, measured for volume, and oven-dried before weighing. For all analyses, we used the mean value for each trait for each species.
Analyses of hurricane tree damage and immediate mortality
To compare the effects of the three hurricanes on tree species damage and mortality, we selected species with at least 40 stems in the damage assessment. This criterion yielded 24 tree species for Hugo (88% of stems assessed), María (81%) and 12 for Georges (89%) (Supp. Table 1 ). We then compared the percentage of stems for each species that were uprooted, broken or, for Hugo and María, likely killed by the hurricanes. To eliminate the possibility that differences in damage in the three hurricanes were driven by variation in stem diameter size distributions or wind exposure, we conducted two sets of analyses. We first compared size diameter distributions and topographic wind exposure for each species for the three hurricanes using a χ 2 test. Exposure to hurricane winds for each tree during each storm was estimated using a topographic model (EXPOS), which determines the degree of exposure to winds given hurricane track and wind speed data, using a 5 m resolution, LiDAR-derived digital elevation map (DEM) 34 . The model assumes that hurricane movement over land decreases sustained wind speeds and increases inflow angles, and then calculates spatial variation in exposure at the spatial resolution of the DEM. This model has been shown to accurately reconstruct exposure to hurricane winds in Puerto Rico at the landscape scale, when compared to historical records 13 , 34 . Track and wind speed data for the three hurricanes was obtained from NOAA and the LiDAR-derived 5 m Digital Elevation Model was obtained from the USGS 3DEP elevation program ( https://nationalmap.gov/3DEP/ ). In a second analysis, we fitted mixed models of the probability of severe damage (stem break and uprooting) and immediate mortality for individual stems that incorporated tree diameter and wind exposure information with species as random effects. Random effects for this model estimate species variation in average rates of hurricane mortality or damage for each storm after accounting for the effects of variation in stem diameter size or exposure. As a result, these random effects allow us to estimate variation in species damage or hurricane mortality that can be attributed to differences in the meteorological characteristics of the storms. To prevent confounding of species-specific damage and mortality with inter-specific variation in tree diameter size, we standardized diameter size within species for each storm by subtracting species-specific means from individual stem values. Models were fitted using the “lme4” package in R Statistical software 35 .
Analyses of lagged, hurricane-induced tree mortality
Beyond its effects on immediate mortality, biomass loss and damage during a hurricane also leads to delayed mortality 19 . These effects typically play out over 3–5 years 1 , 19 . To evaluate the effects of the hurricanes on delayed tree mortality, we used data collected in the 1995–1996 census and mixed models to estimate the increase in probability of delayed mortality that could be attributed to stem breakage or uprooting damage, which was above background mortality rates. Random effects for species were included as intercepts (background rates) and as slopes for the damage effect on mortality. Background rates estimate probability of mortality between 1990 and 1995 that could not be attributed to damage suffered in H. Hugo. Species-specific random slopes for effects of severe damage (stem break or uprooting) on delayed mortality recorded in 1995 estimate increases in probability of mortality that can be attributed to severe damage (i.e., stem break or uprooting) during H. Hugo. Severe damage was coded as a binary variable (0, 1). Note that background rates over this period are likely overestimates relative to storm-free periods since even if not broken or uprooted, stems suffered crown damage that could affect subsequent survival 20 .
Reporting summary
Further information on experimental design is available in the Nature Research Reporting Summary linked to this article.
Code availability
Code used for analyses is available upon request.
Data availability
All damage and census data collected between 1990 and 2016 is available in the Luquillo LTER data catalog http://luq.lter.network/datacatalog . Damage data collected after Hurricane María is available upon request with some restrictions.
Change history
01 may 2019.
The original version of this Article omitted the following from the Acknowledgements: Gabriel Arellano was instrumental in collecting post-María Hurricane data, as part of the Next Generation Ecosystem Experiments-Tropics, funded by the U.S. Department of Energy, Office of Science, Office of Biological and Environmental Research. This has now been corrected in both the PDF and HTML versions of the Article.
Lugo, A. E. Visible and invisible effects of hurricanes on forest ecosystems: an international review. Austral Ecol. 33 , 368–398 (2008).
Article Google Scholar
Balaguru, K., Foltz, G. R. & Leung, L. R. Increasing magnitude of hurricane rapid intensification in the central and eastern tropical Atlantic. Geophys. Res. Lett. 45 , 4238–4247 (2018).
Article ADS Google Scholar
Emanuel, K. The dependence of hurricane intensity on climate. Nature 326 , 483–485 (1987).
Emanuel, K. Increasing destructiveness of tropical cyclones over the past 30 years. Nature 436 , 686–688 (2005).
Article ADS CAS Google Scholar
Webster, P. J., Holland, G. J., Curry, J. A. & Chang, H. R. Changes in tropical cyclone number, duration, and intensity in a warming environment. Science 309 , 1844–1846 (2005).
Hartmann, D. L. e. a. Climate Change 2013: The Physical Science Basis. Contribution of Working Group I to the Fifth Assessment Report of the Intergovernmental Panel on Climate Change (ed T. F. et al. Stocker) 159–254 (Cambridge University Press, Cambridge, 2013).
Knutson, T. R. et al. Tropical cyclones and climate change. Nat. Geosci. 3 , 157–163 (2010).
Elsner, J. B., Kossin, J. P. & Jagger, T. H. The increasing intensity of the strongest tropical cyclones. Nature 455 , 92–95 (2008).
Thompson, J. et al. Land use history, environment, and tree composition in a tropical forest. Ecol. Appl. 12 , 1344–1363 (2002).
Scatena, F. N. & Larsen, M. C. Physical aspects of Hurricane Hugo in Puerto Rico. Biotropica 23 , 317–323 (1991).
USGS. Vol. Fact Sheet 040–099, https://pubs.usgs.gov/fs/FS-040-99/pdf/fs-040-99.pdf (United States Geological Survey, Virginia, 1999).
Pasch, R. J., Penny, A. B., and Berg, R. Hurricane María (AL152017). (National Hurricane Center, USA, 2018).
Boose, E. R., Serrano, M. I. & Foster, D. R. Landscape and regional impacts of hurricanes in Puerto Rico. Ecol. Monogr. 74 , 335–352 (2004).
Griffith, M. P., Noblick, L. R., Dowe, J. L., Husby, C. E. & Calonje, M. A. Cyclone tolerance in New World Arecaceae: biogeographic variation and abiotic natural selection. Ann. Bot. (Lond.) 102 , 591–598 (2008).
Lugo, A. E. & Scatena, F. Background and catastrophic tree mortality in moist, wet, and rain forests. Biotropica 28 , 585–599 (1996).
Phillips, O. L. & Gentry, A. H. Increasing turnover through time in tropical forests. Science 263 , 954–958 (1994).
Condit, R., Hubbell, S. P. & Foster, R. B. Mortality rates of 205 neotropical tree and shrub species and the impact of a severe drought. Ecol. Monogr. 65 , 419–439 (1995).
Walker, L. R., Voltzow, J., Ackerman, J. D., Fernandez, D. S. & Fetcher, N. Immediate impact of Hurricane Hugo on a Puerto Rican forest. Ecology 73 , 691–694 (1992).
Walker, L. R. Timing of post-hurricane tree mortality in Puerto Rico. J. Trop. Ecol. 11 , 315–320 (1995).
Uriarte, M., Canham, C. D., Thompson, J. & Zimmerman, J. K. A Neighborhood analysis of tree growth and survival in a hurricane-driven tropical forest. Ecol. Monogr. 74 , 591–614 (2004).
Tanner, E. V. J., Rodriguez-Sanchez, F., Healey, J. R., Holdaway, R. J. & Bellingham, P. J. Long-term hurricane damage effects on tropical forest tree growth and mortality. Ecology 95 , 2974–2983 (2014).
Niklas, K. J. Tree biomechanics with special reference to tropical trees in Tropical Tree Physiology (eds. Goldstein, G. and Santiago, L.S.) 6 , 413–435 (2016).
Xi, W., Peet, R. K., Decoster, J. K. & Urban, D. L. Tree damage risk factors associated with large, infrequent wind disturbances of Carolina forests. Forestry 81 , 317–334 (2008).
Zimmerman, J. K. et al. Responses of tree species to hurricane winds in subtropical wet forest in Puerto Rico: implications for tropical tree life histories. J. Ecol. 82 , 911–922 (1994).
Canham, C. D., Thompson, J., Zimmerman, J. K. & Uriarte, M. Variation in susceptibility to hurricane damage as a function of storm intensity in Puerto Rican tree species. Biotropica 42 , 87–94 (2010).
Asner, G. P. & Goldstein, G. Correlating stem biomechanical properties of Hawaiian canopy trees with hurricane wind damage. Biotropica 29 , 145–150 (1997).
Webb, S. L. Contrasting windstorm consequences in 2 forests, Itasca State Park, Minnesota. Ecology 70 , 1167–1180 (1989).
Putz, F. E. & Sharitz, R. R. Hurricane damage to old-growth forest in Congaree swamp National Monument, South Carolina, USA. Can. J. For. Res. 21 , 1765–1770 (1991).
Seidl, R. et al. Modelling natural disturbances in forest ecosystems: a review. Ecol. Modell. 222 , 903–924 (2011).
FAO. Global Forest Resources Assessment. (Food and Agriculture Organization of the United Nations, Rome, Italy, 2010).
Johnstone, J. F. A. C. D. et al. Changing disturbance regimes, ecological memory, and forest resilience. Front. Ecol. Environ. 14 , 369–378 (2016).
Ewel, J. J. & Whitmore, J. L. The ecological life zones of Puerto Rico and the U.S. Virgin Islands. (International Institute of Tropical Forestry, San Juan, 1973).
Cornelissen, J. H. C. et al. A handbook of protocols for standardised and easy measurement of plant functional traits worldwide. Aust. J. Bot. 51 , 335–380 (2003).
Boose, E. R., Foster, D. R. & Fluet, M. Hurricane impacts to tropical and temperate forest landscapes. Ecol. Monogr. 64 , 369–400 (1994).
Bates, D., Machler, M., Bolker, B. M. & Walker, S. C. Fitting linear mixed-effects models using lme4. J. Stat. Softw. 67 , 1–48 (2015).
Download references
Acknowledgements
We thank the field crews who collected tree census and damage data. Research was supported by NSF award DEB-1801315 to M.U. and J.K.Z. and awards DEB-9705814, DEB-0080538, DEB-0218039, BSR-8811902, DEB-9411973, DEB-0620910, DEB-1239764, and DEB-1546686 to the Luquillo LTER. Gabriel Arellano was instrumental in collecting post-María Hurricane data, as part of the Next Generation Ecosystem Experiments-Tropics, funded by the U.S. Department of Energy, Office of Science, Office of Biological and Environmental Research.

Author information
Authors and affiliations.
Department of Ecology Evolution and Environmental Biology, Columbia University, 1200 Amsterdam Avenue, New York, NY, 10027, USA
María Uriarte
Centre for Ecology & Hydrology Bush Estate, Penicuik, Midlothian, EH26 0QB, UK
Jill Thompson
Department of Environmental Sciences, University of Puerto Rico, San Juan, Puerto Rico, 00925, USA
Jess K. Zimmerman
You can also search for this author in PubMed Google Scholar
Contributions
M.U. conceived the paper, analyzed the data, and wrote the first draft. J.K.Z., J.T., and M.U. collected census and damage data and contributed to writing.
Corresponding author
Correspondence to María Uriarte .
Ethics declarations
Competing interests.
The authors declare no competing interests.
Additional information
Journal peer review information : Nature Communications thanks Kristina Anderson-Teixeira and the other anonymous reviewers for their contribution to the peer review of this work. Peer reviewer reports are available.
Publisher’s note: Springer Nature remains neutral with regard to jurisdictional claims in published maps and institutional affiliations.
Supplementary information
Peer review file, reporting summary, rights and permissions.
Open Access This article is licensed under a Creative Commons Attribution 4.0 International License, which permits use, sharing, adaptation, distribution and reproduction in any medium or format, as long as you give appropriate credit to the original author(s) and the source, provide a link to the Creative Commons license, and indicate if changes were made. The images or other third party material in this article are included in the article’s Creative Commons license, unless indicated otherwise in a credit line to the material. If material is not included in the article’s Creative Commons license and your intended use is not permitted by statutory regulation or exceeds the permitted use, you will need to obtain permission directly from the copyright holder. To view a copy of this license, visit http://creativecommons.org/licenses/by/4.0/ .
Reprints and permissions
About this article
Cite this article.
Uriarte, M., Thompson, J. & Zimmerman, J.K. Hurricane María tripled stem breaks and doubled tree mortality relative to other major storms. Nat Commun 10 , 1362 (2019). https://doi.org/10.1038/s41467-019-09319-2
Download citation
Received : 18 September 2018
Accepted : 05 March 2019
Published : 25 March 2019
DOI : https://doi.org/10.1038/s41467-019-09319-2
Share this article
Anyone you share the following link with will be able to read this content:
Sorry, a shareable link is not currently available for this article.
Provided by the Springer Nature SharedIt content-sharing initiative
This article is cited by
Predictors of tree damage and survival in agroforests after major cyclone disturbance in fiji.
- Ashley McGuigan
- Mesulame Tora
- Tamara Ticktin
Agroforestry Systems (2024)
Disturbance Level Mediates the Differential Resistance of Tropical Dry Forest Soil and Vegetation Attributes to High-Intensity Hurricanes
- Cristina Montiel-González
- Ángel E. Bravo-Monzón
- Luis Daniel Avila-Cabadilla
Ecosystems (2024)
Impact of Hurricane Maria on mold levels in the homes of Piñones, Puerto Rico
- B. Bolaños-Rosero
- X. Hernández-González
Air Quality, Atmosphere & Health (2023)
An evaluation of vegetation loss due to the super typhoon Sarika in Hainan Island of China
- Xiao Fengjin
- Liu Qiufeng
Natural Hazards (2023)
Hurricane driven changes in vegetation structure and ecosystem services in tropical urban yards: a study case in San Juan, Puerto Rico
- Sofía Olivero-Lora
- Julissa Rojas-Sandoval
- Juan L. Orengo-Rolón
Urban Ecosystems (2022)
By submitting a comment you agree to abide by our Terms and Community Guidelines . If you find something abusive or that does not comply with our terms or guidelines please flag it as inappropriate.
Quick links
- Explore articles by subject
- Guide to authors
- Editorial policies
Sign up for the Nature Briefing newsletter — what matters in science, free to your inbox daily.

Uncovering Hurricane Maria’s true toll
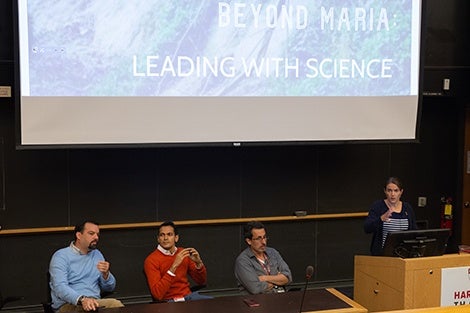
September 28, 2018 – After Hurricane Maria slammed into Puerto Rico on September 20, 2017, statistician and genomics expert Rafael Irizarry of Harvard T.H. Chan School of Public Health, a native of Puerto Rico, was hearing dire reports of death and destruction from friends and family on the island. So when the governor of Puerto Rico announced in early December 2017 that there had been only 16 storm-related deaths, Irizarry thought the number sounded “impossible.”
Concerned that the Puerto Rican government was unaware of the storm’s true death toll, Harvard Chan School’s Caroline Buckee and Satchit Balsari initiated a study of storm-related mortality, working with co-authors including Irizarry and other experts from Harvard Chan School and Puerto Rico. Published last May, the study estimated that there had been somewhere between 800 and 8,500 excess deaths in Puerto Rico related to the hurricane through the end of December 2017.
A year after the storm, on September 21, 2018, Buckee, Balsari, Irizarry, and Domingo Marqués-Reyes, associate professor at Albizu University in Puerto Rico, discussed their study, other estimates of hurricane-related deaths, the difficulty of obtaining official mortality data, and the hardships Puerto Ricans faced in the weeks and months after Maria. Buckee moderated the panel.
Watch a webcast of the panel discussion
Estimating mortality
Buckee, associate professor of epidemiology, presented a timeline that showed the various points when hurricane-related death estimates were made over the past year. Penn State researchers, using partially released registry data from the Puerto Rican government, estimated that the storm had led to 1,064 excess deaths through November 21. On December 9, the Puerto Rican government placed the death toll at 64. That same day, the New York Times estimated a toll of 1,052. On December 18, the Puerto Rican government ordered a recount, and on February 22, 2018 it announced that George Washington University would conduct it. On May 29, Harvard Chan School released its study, drawing considerable media attention. Five days later, under intense scrutiny from the media and others, the government of Puerto Rico finally released all of its registry data, which showed that there had been nearly 1,400 excess deaths between September and December 2017. George Washington University’s study came out on August 27. It estimated that, through February 2018, there had been 2,975 excess deaths due to the hurricane—a number that was accepted by Puerto Rican authorities.
Irizarry, professor of biostatistics, spoke about the difficulty of trying to obtain official Puerto Rican death data, both from government officials and from other researchers working on their own estimates. When the Harvard Chan study came out, the researchers made all their data and code public so that others could reanalyze it. Their goal was to ensure the availability of as much hurricane-related death information as possible prior to the next hurricane season.
Balsari, research fellow at the FXB Center for Health and Human Rights , discussed the analytic method, known as cluster sampling, used in the Harvard Chan study. The researchers picked randomly chosen households in different parts of Puerto Rico and knocked on doors to ask if anyone in the house had died after the storm and how they had died. The researchers sought information not only on “direct” deaths—those that occurred during the storm, from either drowning or other weather-related accidents—but also “indirect deaths” that occurred in the storm’s aftermath, including those of people unable to receive needed medical treatment.
The Harvard Chan study revealed that illness and death continued for months after Hurricane Maria, driven by a loss of infrastructure—lack of electricity, lack of clean water, and lack of cell phone service, Balsari said. “This is something that we as public health practitioners have to be extremely attuned to, because we’re going to see this over and over and over again as our populations age and become more dependent on infrastructure for their medical care,” he said. “You need a refrigerator to refrigerate your insulin. You’re a dialysis patient, you need to be able to go to the dialysis center. If you have a nebulizer at home, you need to be able to plug the nebulizer into your power port.”
Emotional effects
Marqués-Reyes—who himself went about 90 days without power, 50 without running water, and 20 without cell service after the storm—discussed the hurricane’s emotional toll. He said many Puerto Ricans experienced survivors’ guilt and post-traumatic stress disorder. There were increases in domestic violence and alcohol use. Suicides and suicide-related hotline calls spiked.
Even months after the storm, there hadn’t been official acknowledgement of the magnitude of Puerto Rico’s losses, Marqués-Reyes said—“not even a minute of silence for the people that died.” But after the Harvard Chan study came out, people began a mourning process that was long overdue, including a “shoe memorial” in front of the capitol building in San Juan, in which each pair of shoes represented a life lost.
Marqués-Reyes said that people, organizations, and private companies in Puerto Rico are working hard to be prepared for the next big storm. People are now aware of the vulnerabilities of those who need dialysis and those who have respiratory or heart problems. “Now everyone knows that something as simple as high blood pressure can become deadly when you have no running water, nothing to drink and you’re eating Spam from a can,” he said.
– Karen Feldscher
photo: Nilagia McCoy
- Reference Manager
- Simple TEXT file
People also looked at
Original research article, case studies of multihazard damage: investigation of the interaction of hurricane maria and the january 2020 earthquake sequence in puerto rico.
- 1 Department of Civil and Environmental Engineering, University of Connecticut, Storrs, CT, United States
- 2 Department of Civil and Environmental Engineering, Rice University, Houston, TX, United States
This paper is motivated by the unique findings and observations from reconnaissance visits after the earthquake series in Puerto Rico in January 2020. It aims to discuss the potential interactions of Hurricane Maria and 2020 earthquake series and the considerations they underscore for future field reconnaissance missions. Traditionally, post-disaster damage assessment activities focus on one hazard and overlook the potentially cascading effects of multiple hazards on structures and infrastructure. This paper provides case studies showing the possible interaction of multiple hazards and their cascading effects observed in Puerto Rico. Infrastructure surveyed includes port facilities, buildings (particularly historical structures), and bridge structures. The data collected during the reconnaissance missions reveal how the impacts of Hurricane Maria, along with infrastructure aging and delayed repair and recovery activities, may have influenced the damage level and failure modes observed during the earthquake sequence a few years after. These case studies illustrate the nature of multihazard interactions and how these effects should be documented during post-disaster assessments. Beyond the insights gained from the case studies illustrated in this paper, the field survey instrument is provided as a basis for future reconnaissance studies, and the full set of reconnaissance data collected are published on the NSF funded NHERI DesignSafe cyberinfrastructure. As a result, this work not only provides data from Puerto Rico that can inform future damage and recovery modeling efforts, but also offers survey instruments and a field data collection process that is particularly tailored to cases where multihazard effects are at play.
1 Introduction
Regions across the United States and worldwide are generally at risk from more than one natural hazard which vary in spatial and temporal scale, occurrence potential, and intensity. These hazards may include earthquakes, hurricanes, floods, tsunamis, landslides, tornadoes, snowstorms, and fires, among others. Design engineers, infrastructure owners, risk managers, and other stakeholders are challenged to address the potential impacts of one or more natural hazards. Current design philosophies fail to consider the complex and intertwined effects of multiple hazards ( Roy and Matsagar, 2021 ). The development and implementation of effective design and risk mitigation strategies requires an understanding of the performance of structures and infrastructure in a multihazard environment, considering cases of concurrent, non-concurrent, or cascading hazards. Unique engineering challenges are posed by such multihazard problems ( Li et al., 2012 ). Ongoing work in the community ranges from characterizing the multihazard potential ( Gill and Malamud, 2014 ; Hayes et al., 2014 ), simulating behavior under cascading hazards ( Echevarria et al., 2015b ; Echevarria et al., 2015 ; Imani et al., 2015 ; Keller and Pessiki, 2015 ), testing structures under concurrent hazard loading ( Baheru et al., 2014 ), to modeling risk and resilience in multihazard settings ( Barbato et al., 2013 ; Kameshwar and Padgett, 2014 ; Echevarria et al., 2015a ; Jaimes et al., 2015 ; van de Lindt et al., 2015 ). Unfortunately, the lack of field data to support a variety of multihazard analyses to adequately characterize system failure modes or validate the existing models has slowed the progress in this domain ( Bruneau et al., 2017 ).
In this regard, the cascading effects of two inherently different events that impacted Puerto Rico presented a unique opportunity for researchers to collect invaluable data and provide empirical evidence for potential multihazard interactions. A team of researchers with diverse backgrounds in structural engineering, risk assessment, and risk mitigation for complex and interconnected infrastructure systems conducted reconnaissance missions to document and analyze the damages during the January 2020 earthquake series ( Miranda et al., 2020 ) with linkage to Hurricane Maria, the Category four storm that struck the island in September 2017 ( FEMA, 2018 ). The nomenclature proposed by Zaghi et al. (2016 ) to discuss multihazard design will be used in the framing of this paper. The case of two subsequent punctuated events, i.e., earthquake series and hurricane, as occurred in Puerto Rico is a classic case of non-concurrent multihazard scenarios. In such a case, degradation of structural capacity or shifts in boundary conditions from the primary event, along with the incremental or incomplete restoration processes prior to subsequent events, can have a significant impact on performance. This premise is illustrated below in Figure 1 . Here, it is shown how either a delayed repair or no repair negatively impacts system performance during a second event. For example, for a port structure, gradual decay makes the structure more vulnerable. After an event, the damaged structure could go through a faster decay than before as shown with the larger negative slope. However, this interaction between chronic stressors and multiple hazard events is not well understood, and data is scarce to fill this knowledge gap ( Zaghi et al., 2016 ).
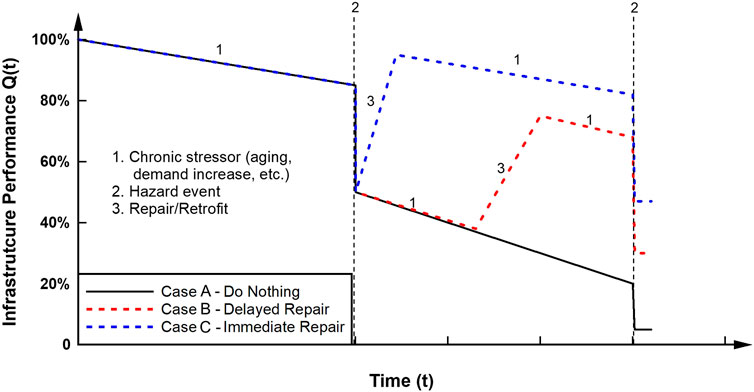
FIGURE 1 . Schematic showing change in system performance in subsequent hazards based on repair timeline.
While it is well known that earthquakes can have a different impact on structures that suffer from corrosion damage or scour ( Shiraki et al., 2007 ; Wang et al., 2014 ; Burke and Bruneau, 2016 ; Argyroudis et al., 2020 ), analyses are commonly performed on models of intact structures. This can be detrimental given that structures are rarely hit by an event in their intact form, i.e., new construction. Researchers have studied the combined effects of chronic and extreme stressors on the reliability and resilience of infrastructure systems throughout their lifetime ( Ouyang et al., 2012 ; Yang and Frangopol, 2019 ; Liu et al., 2020 ), underscoring the significance of considering the interactions of multiple hazards at different stages in the system’s service life, rather than modeling them independently. For the specific case of bridges ( Kumar and Gardoni, 2014 ; Padgett and Kameshwar, 2016 ; Vishnu and Padgett, 2020 ), several studies examined their vulnerability to impacts such as hurricanes and earthquakes, along with gradual stressors such as aging and sea-level rise, while emphasizing the pressing need for multihazard empirical data to support model development and validation. While several researchers have discussed procedures for multihazard risk assessment predicting cascading failures ( Eisenberg et al., 2020 ; Wei et al., 2022 ), the community lacks empirical data showcasing the impacts of such hazards.
During the investigation in Puerto Rico, it was found that some of the damage scenarios may be explained in a multihazard context that accounts for an interaction of impacts from the recent earthquakes and Hurricane Maria. The earthquakes exposed several unexpected and undocumented vulnerabilities of the infrastructure. As there is limited knowledge on the possible interactions of hurricanes and seismic events, the team investigated conditions that could lead to multihazard damages including: 1) structures and sites that experienced soil erosion during Hurricane Maria, 2) landslide-driven hydrologic effects of Maria that may be worsened during the earthquakes, 3) structural repairs that changed boundary conditions in subsequent events, 4) structures that showed signs of damage after the occurrence of the aftershocks, and 5) structural design concepts, such as elevated construction to avoid flooding, that makes structures more vulnerable to earthquakes.
This paper intends to present a thought-provoking series of case studies which encourage future reconnaissance missions through the lens of multihazard impacts. The main contributions of the work are: 1) presenting actual field observations and insights on multi-hazard interactions, 2) providing access to the comprehensive set of data collected, curated, and published for reuse on DesignSafe CI, and 3) sharing a field survey instrument and systematic field survey approach for future use in reconnaissance cases with potential multihazard effects. It is important to note that this paper relies heavily on observations, limited publicly available data, and the firsthand accounts of infrastructure owners or operators collected during the field visits. For that reason, significant interpretation was needed by the authors. The authors acknowledge that some of the findings presented may be viewed differently by other experts, but the aim is to share the data and information gleaned from conversations during our multiple visits and post-mission reflections. An overview of the region and susceptibility to hazards is presented first, followed by the details of four case studies of multihazard interactions. This work not only provides data from Puerto Rico that can inform future damage and recovery modeling efforts, but also presents survey instruments and a field data collection process that is particularly tailored to cases where multihazard effects are at play.
2 Overview of location and events
2.1 location of interest.
Ponce, Puerto Rico’s second largest city, is located on the island’s southern coast. During the 1800s, Ponce was an important port for shipping sugar, rum, coffee, and tobacco ( Tillman, 2009 ). As the sugar industry evaporated in the 20th century, the economy experienced a downturn that resulted in an increased reliance on tourism. In addition, the island has grappled with high unemployment and poverty rates, government disinvestment in public services, and crime. Natural hazards in this area expose vulnerabilities from colonial subjugation, economic hardship, environmental injustice, infrastructural neglect, and corruption from the island’s political class ( Lloréns, 2018 ). In the following section, we will provide an overview of the threat from natural disasters and detail the two phenomena that are the focus of this paper: Hurricane Maria and the 2020 earthquake series.
2.2 Overview of hazards
2.2.1 hurricanes.
Puerto Rico is subject to frequent and severe impacts from hurricanes, particularly between June and November ( Havidan, 1997 ; Emery et al., 2004 ; López-Marrero et al., 2019 ). Puerto Rico experiences some of the highest hurricane frequency in the North Atlantic basin. Storms typically approach from the east and south-east, and the most intense storms often form on the western coast of Africa and reach Puerto Rico near maximum intensity ( Emery et al., 2004 ). Based on data from 1900 to 2017, a total of 33 hurricanes passed over Puerto Rico or within 86 miles from the coast. Furthermore, 14 of the 33 reached wind velocities of Category three or higher ( López-Marrero et al., 2019 ).
The 2017 Atlantic hurricane season was one of the costliest and most destructive on record. For the first time, three Category four hurricanes made landfall in the US ( Blake, 2018 ). One such event was Hurricane Maria, which devastated Puerto Rico and many islands in the Caribbean. On 20 September 2017, Hurricane Maria’s center crossed the southeast coast of Puerto Rico near Yabucoa around 10:15 UTC. At that time, the maximum wind speed was 155 mph, which is just below Category five intensity. The hurricane’s center crossed the island from southeast to northwest and emerged into the Atlantic around 18:00 UTC ( Pasch et al., 2017 ). The estimated storm surge levels at the coast are shown in Figure 2 . The scale of the devastation, costliness of the damage, and delay in releasing federal aid left critical infrastructure unrepaired prior to the earthquake series.
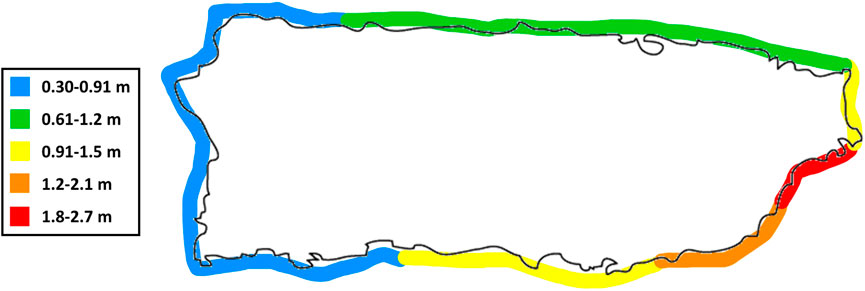
FIGURE 2 . Estimated storm surge inundation along the coasts of Puerto Rico adapted from Pasch et al. (2017) .
2.2.2 Earthquakes
The island of Puerto Rico is located between the North American and the Caribbean tectonic plates. The boundary is characterized primarily by left-lateral motion along east-west striking faults ( Jansma et al., 2000 ). The Puerto Rico-Virgin Islands microplate is one of three microplates located in this complex plate boundary zone ( Raussen and Lykke-Andersen, 2009 ), and is part of the Caribbean plate. On the northern side of the island, the North American plate is subducting obliquely beneath the Caribbean plate ( Vičič et al., 2022 ). This is bounded by the east-west striking Puerto Rico trench ( Jansma et al., 2000 ). On the southern side of the island, the Muertos trough, an east-west striking fault defines the southern limit of the Puerto Rico-Virgin Islands microplate ( Jansma et al., 2000 ). Seismicity in and around Puerto Rico averages hundreds of earthquakes per year ( Jansma et al., 2000 ).
A series of significant seismic events occurred in the southwestern region of Puerto Rico in early January 2020. The first major event (M w 5.8) occurred on January 6th at 10:32 a.m. UTC. The second significant event (M w 6.4) occurred on January 7th at 8:24 a.m. UTC. The third significant event (M w 5.6) occurred on January 7th at 11:18 a.m. UTC. These earthquakes caused significant damage to many structures near Guayanilla and Ponce, Puerto Rico. The start of the seismic events occurred on 28 December 2019, and the region continued to experience aftershocks as of March 2020 ( Miranda et al., 2020 ). A summary of all magnitude five and larger earthquakes between 29 December 2019 and 17 January 2020 are listed in Table 1 ( Van Der Elst et al., 2020 ). The PGA contours from the M w 6.4 event are shown in Figure 3 .
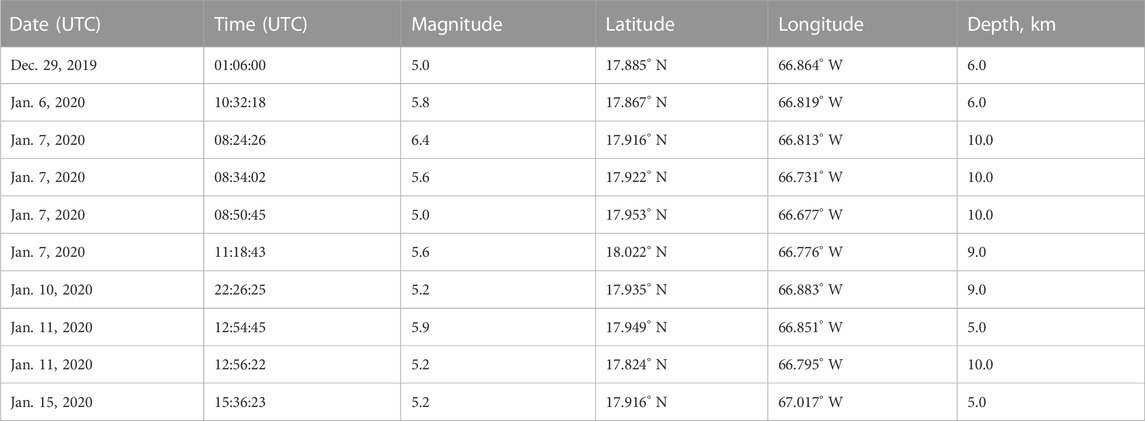
TABLE 1 . Summary of magnitude five and larger earthquakes between December 29th, 2019–17 January 2020 ( Van Der Elst et al., 2020 ).
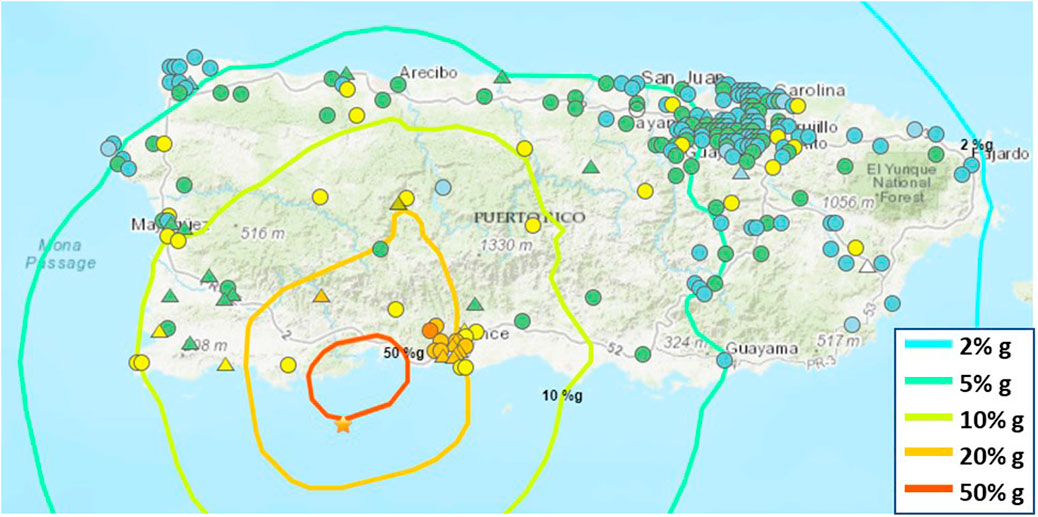
FIGURE 3 . PGA contours estimated from ShakeMap for 7 January 2020, M w 6.4 earthquake ( USGS, 2020 ).
3 Reconnaissance methods
The reconnaissance missions were funded through an NSF RAPID Project. The first visits were conducted January 23rd-24th and February 24th-26th, 2020. The final visit was set to occur in March 2020 but was delayed due to COVID-19 travel restrictions. When travel bans were lifted, the final visit was conducted on April 21st-23rd, 2021. The inspections focused on structures in Southern Puerto Rico that sustained damages during the earthquake series. The damages were compared to previous seismic events to determine if the level of damage was consistent with the magnitude of the earthquake or if additional factors likely contributed to the damage. Traditional post-disaster damage assessment activities focus on one hazard and overlook the potentially destructive cascading effects of multiple hazards on structures and infrastructure. Therefore, the focus of this study was to identify the compounding impacts of Hurricane Maria and the 2020 earthquake series. Through this inspection, a comprehensive data set was collected to understand if the impacts of Hurricane Maria resulted in an increased level of damage or contributed to unexpected modes of failure during the recent earthquake series.
Multiple methods were used to ensure a comprehensive set of data was collected. The data collection methods included a damage assessment survey, multiple image types, images and videos taken with an unmanned aerial system (UAS) operated by a team member, and Lidar scan data. All data from the missions can be found on DesignSafe-CI ( Zaghi et al., 2021 ; Hain et al., 2022 ). The team made use of the RAPID Facility Field Data Collection App (Rapp) survey application to ensure a systematic approach toward data collection ( Berman et al., 2020 ). The survey included guiding questions to be answered at each site location at the start of data collection. The survey questions are included below in Table 2 . The survey is meant to be used in the numerical order shown. The damage assessment survey was completed by 2-3 of the team members to ensure it the findings were consistent.
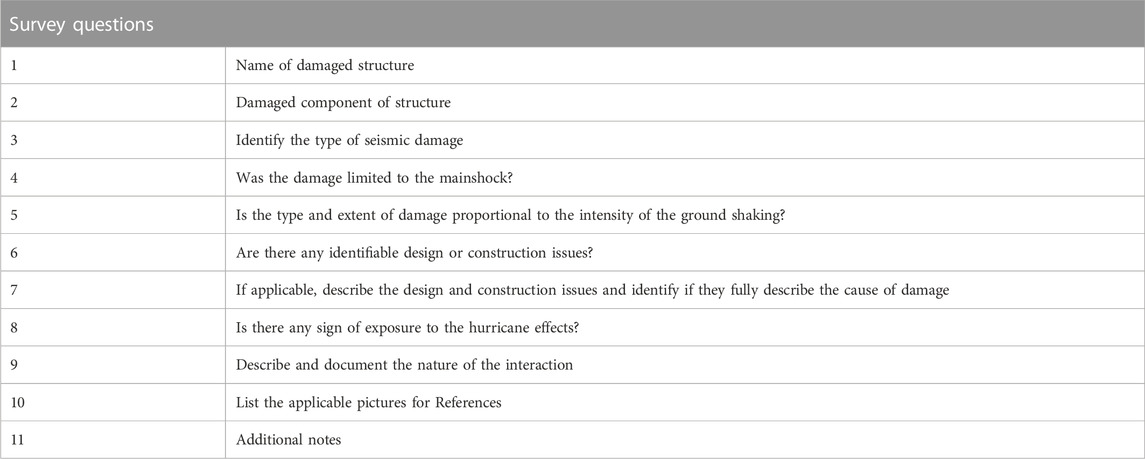
TABLE 2 . Damage assessment survey.
4 Results from multihazard case studies
4.1 case 1: historic buildings in downtown ponce, pr shock-aftershock.
One of the most prevalent examples of multihazard events is the successive nature of the initial earthquake and corresponding aftershocks. While most earthquakes are accompanied by aftershocks, typical designs only consider the maximum magnitude event. As the research team visited Puerto Rico on three occasions after the initial earthquake series, they were able to evaluate the progression of structural damages in the aftershock events.
As the economy of the Ponce region is largely focused on tourism, the team focused on historical structures. These buildings were vulnerable to seismic loads for several reasons, including their age and the brittle nature of the construction materials and structural systems. A sample of the damage progression over time is shown in Figure 4 . During the initial events, this building sustained severe damage including shear cracking at the awning and at multiple locations along the roof as shown in Figures 4A, B . At this point, the building was roped off and entry was halted for safety concerns. As the aftershocks continued, the damaged worsened, as shown in Figure 4C . One of the shear cracks along the side of the building expanded and the brick wall collapsed which exposed the structural support system of the roof. It is also important to note that the front of the structure was supported by temporary bracing that was installed after the main shock, which prevented the collapse of this portion. This shows that minimal external bracing/shoring or strengthening is an effective strategy in preventing unrecoverable damage or total collapse during aftershocks. This is particularly important for brittle structures that lack an engineered system to provide ductility and energy dissipation, and those with historic significance. To minimize damage in subsequent events it is critical to first identify the repair priorities and then allocate the resources needed to execute the repairs. When identifying repair priorities, safety, economic impact, and historical significance should be considered.
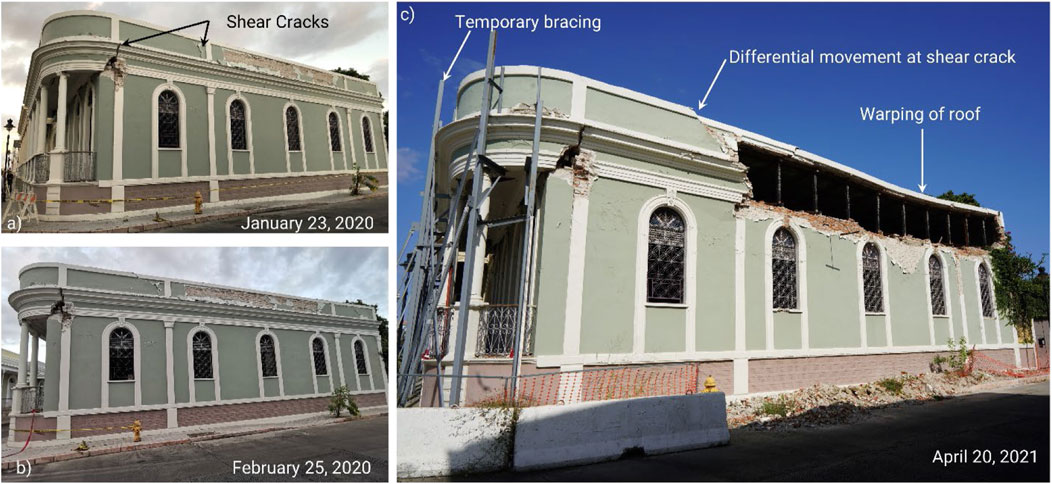
FIGURE 4 . Damage progression of sample historical building in downtown Ponce under aftershock events. Images were taken during consecutive visits on (A) 23 January 2020, (B) 25 February 2020, and (C) 20 April 2021.
A second example of a historical building that suffered damage during the initial earthquake series is shown below in Figure 5 . In Figure 5A , shear cracks on the unreinforced masonry wall around the window opening are visible. This hotel is located in downtown Ponce. It experienced temporary closures after the damage was discovered from the mainshock. The damage was repaired quickly, and the window was removed to form a more robust lateral load resisting system as shown in Figure 5B . This illustrates how the timely implementation of repairs can protect vulnerable, but significant, structures from increased damage.
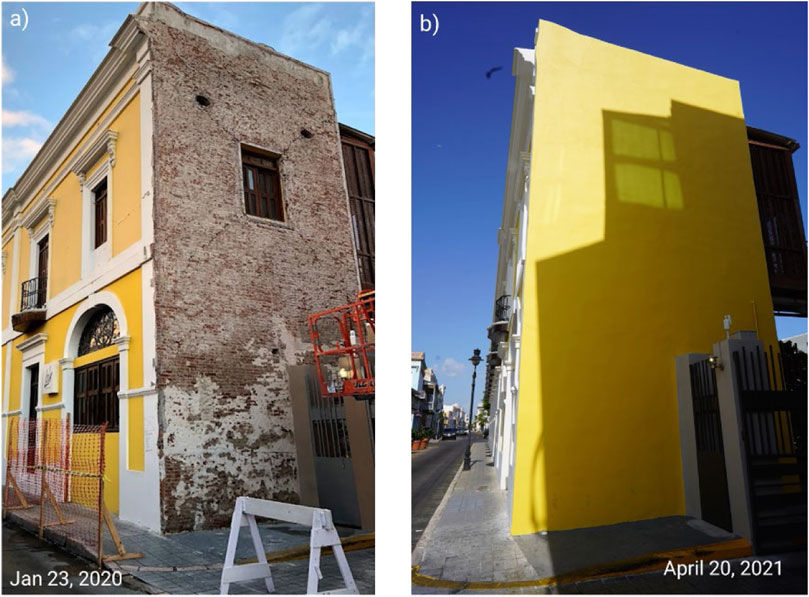
FIGURE 5 . Historical hotel in Downtown Ponce showing (A) damage after initial earthquake series and (B) view of repaired wall segment.
In the context of societal and economic impacts, damages after the hurricane and the slow recovery led to mass migration from the affected areas. In the aftermath of the earthquake series, the number of individuals leaving Puerto Rico increased ( Sanchez, 2020 ; Aranda et al., 2022 ). This included a large number of small business owners supporting the tourism industry. The research team identified that a lack of, or delayed, access to federal funding through FEMA significantly slowed the economic recovery of the region, which adds to the vulnerability. For example, the team noticed that several historic buildings in Ponce that needed restoration after the earthquakes were abandoned by the owners. At the time of the visits, the city officials were in conversation with the historic preservation community to evaluate if some of these buildings have to be demolished because of public safety concerns.
4.2 Case 2: Rafael Cordero Santiago Port of the Americas, Hurricane Maria–earthquake series
This case study details the multihazard impacts from punctuated stressors, i.e., Hurricane Maria and the earthquake series. The extent of damage on ports facilities due to hurricanes is often directly correlated to the proximity of the port to the hurricane landfall. Damages can be attributed to several characteristics of the hurricane, including storm surge, wave heights, wind speed, debris impact, and the duration of exposure. Hurricane damages can include disruptions to key features, such as the navigation channel, or structures, such as piers and wharfs, as well as destruction of ancillary structures, equipment, and cargo throughout the port ( Wakeman, 2013 ). While the port experienced notable damage from Hurricane Maria alone, this section will focus on the physical impacts from the storm that influenced its performance during the earthquake series.
This case highlights one pier that sustained severe damage during the earthquakes, shown below in Figure 6 . The level of damage sustained was greater than what is expected from earthquakes of this magnitude, suggesting additional factors contributed to the damage. Major damage types included seaward displacement of panels ( Figures 6A, C ), bowing of the berth ( Figure 7 ), and collapse of panels ( Figures 6A, B ). Upon inspection, the team determined the slab failure of the pier that occurred during the earthquake series was caused by compromised support conditions. Key personnel from the site noted washout from the pier was evident after Hurricane Maria. In addition, evidence of coastal erosion in the region was noted in Geotechnical Extreme Events Reconnaissance report following the hurricane ( Silva-Tulla et al., 2018 ). The erosion of the supporting soil fill, as shown in Figure 6B , made the unreinforced slab-on-grade vulnerable to the seismic shaking.
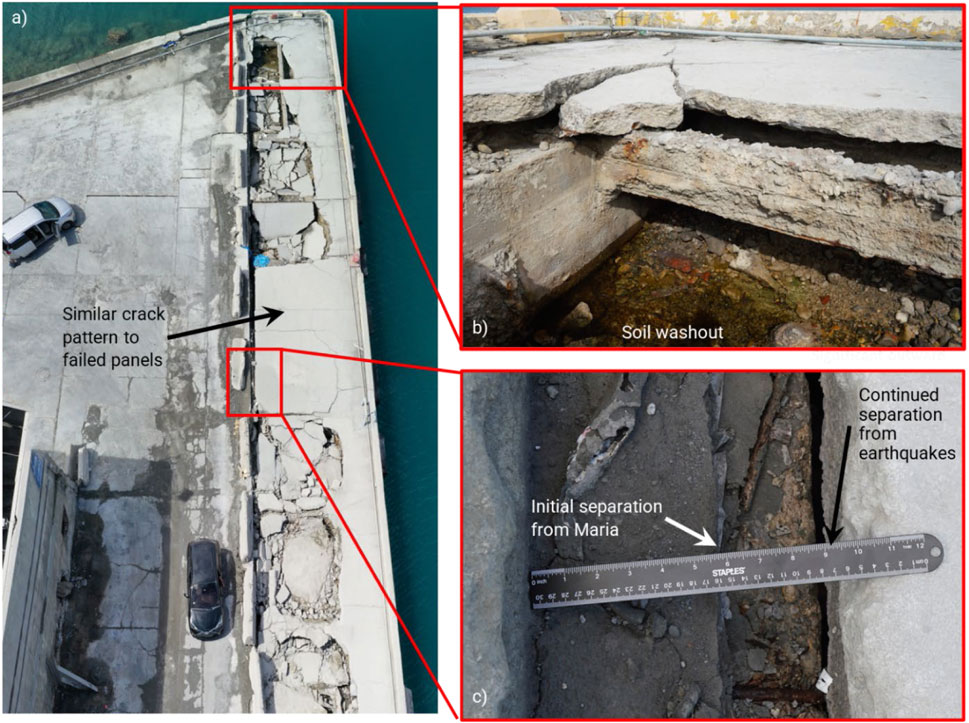
FIGURE 6 . Images of damages to Pier following January 2020 earthquake series including (A) an aerial view of the overall damage, (B) washout of soil underneath collapsed panel, and (C) representative image showing separation of main slab and pier.
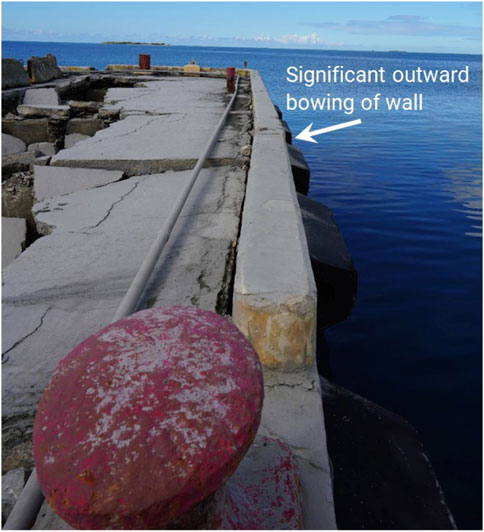
FIGURE 7 . View of bowing of berth at Pier 2.
As shown in Figure 7 , there was severe bowing of the berth at the upper region of the pier. This bowing shows an area on the edge of the pier that is experiencing larger outward displacements compared to other areas along the length, which suggests more significant pile damage at this location. It is unlikely the bowing is caused by large soil or water pressure at this location, as washout of the soil fill within the damaged regions was observed.
The port was closed for a short period of time due to damage incurred from the hurricane, yet the port was partially restored due to its critical role. While the port was allocated funds to address damages from Hurricane Maria, at the time of inspection the federal funds still had not been released. Due to this delay, the condition of many structures continued to degrade, worsening the performance in the earthquake series. It is not in the scope of this paper to discuss the reasons for delaying funds, rather we aim to highlight the ramification of such actions in terms of safety and higher overall repair costs following subsequent events. This should be a key consideration for policymakers when responding to and planning for future natural disasters.
The damages caused significant delays and postponement of the expansion plans, which were to transform the port into an integrated transport center and logistics platform for international trade in the Caribbean region. The goal of this development strategy was to convert the facility into a third-generation, multi-cargo port, in order to meet the growing needs of the container ship sector and the demands of globalization. This delay has exacerbated the economic impact of the disaster to the affected region, as the lack of capacity to manage the increased cargo volume has led to further economic losses.
4.3 Case 3: Rafael Cordero Santiago Port of the Americas, chronic stressors–earthquake series
Ports are located in highly-corrosive coastal environments. The two most commonly used structural materials, steel and reinforced concrete, are both vulnerable to corrosion deterioration ( Maniglio et al., 2021 ). As such, ports are at a higher risk of accelerated damage due to corrosion compared to other structures. Design considerations, such as increasing the thickness of the cover concrete, using corrosion resistant reinforcements such as epoxy coated or stainless-steel rebar, or including sacrificial thickness or a concrete coating for steel piles, can mitigate the adverse impacts of corrosion damage. However, implementing such strategies often results in increased costs and not all options were available during the construction of older port structures. For reinforced concrete elements, such as beams, slabs, and piles, corrosion of reinforcement is likely to occur if widespread cracking or insufficient cover is present. Corrosion of the reinforcement leads to the spalling of cover concrete, which then further exposes reinforcement. This corrosion weakens the structural elements and may lead to widespread damage under extreme loadings, such as hurricanes and earthquakes.
The majority of the earthquake damage at the ports inspected was exacerbated by underlying corrosion of the structure. This was likely worsened or initiated in Hurricane Maria due to acceleration of corrosion damage in structural components due to increased exposure to sea water. The high level of corrosion present contributed to the spalling and failure of elements in the seismic events. Piers had substantial corrosion in the superstructure. This included the beams ( Figure 8A ), support structures for slabs ( Figure 8B ) and the concrete barrier surrounding the edge of the piers ( Figure 8C ). In many cases, the corrosion damage was not observable prior to the earthquakes when the concrete spalled, but the level of corrosion in the exposed elements indicates that corrosion has been occurring for some time. This makes it challenging to identify corrosion in inspections and, therefore, unlikely to be repaired until it reaches a critical level. Understanding the progression of corrosion and how this deterioration impacts structural behavior under extreme events is critical for predicting performance of aging structures and should be considered in design.
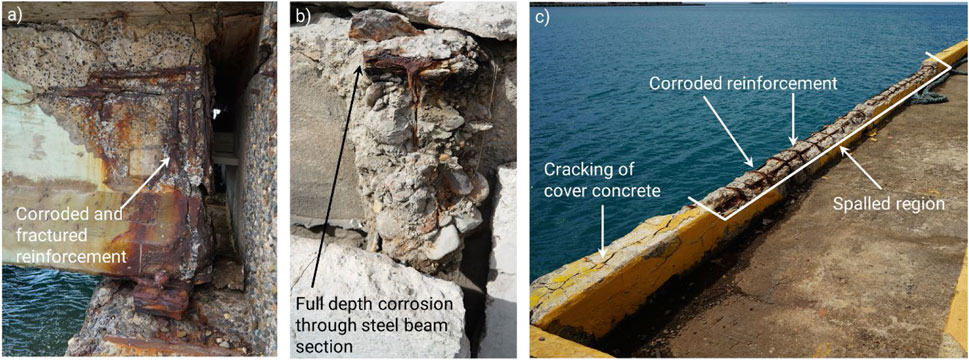
FIGURE 8 . Elements at the ports inspected had severe corrosion damage that was observable after the earthquake series. Sample views of different elements with visible deterioration from corrosion include (A) beams, (B) support structures for slabs, and (C) concrete barrier surrounding the edge of the piers.
While the economic impact of this multihazard scenario is not immediately evident, it can be viewed from the perspective of the expansion plans discussed earlier. Any expansion requires repair and replacement of the existing corrosion damaged elements. This could potentially lead to delays and increased costs due to the need to repair or remove the existing structures.
4.4 Case 4: Bridge in Guayanilla, Hurricane Maria—earthquake series
During Hurricane Maria, the Guayanilla River flooded, which resulted in washout and debris impacts. This was observed on the bridge carrying PR-127, which spans the river. The washout was most severe at the abutment, where exposed piles can be seen on the left of Figure 9A . The bridge was closed and required repair, including backfilling behind the abutment and fill work in front of the abutment ( Maria, 2017 ). This bridge was not visited in the missions in 2020, but after seeing the drone footage from after Hurricane Maria, was added to the inspection list for the 2021 mission. Following the earthquake series and as of April 2021, the bridge remained open to traffic. A panoramic view of the bridge taken with a drone is shown in Figure 9B . Upon further inspection, the research team found several examples of seismic damage, including cracking at the abutments from pounding, bent anchor bolts at the bearings, and spalling at the top of the columns, as shown in Figures 9D, E . There was also evidence of geotechnical damage, as shown in Figure 9C , but it was not clear if this was left over from hurricane Maria, or a result of the earthquakes.
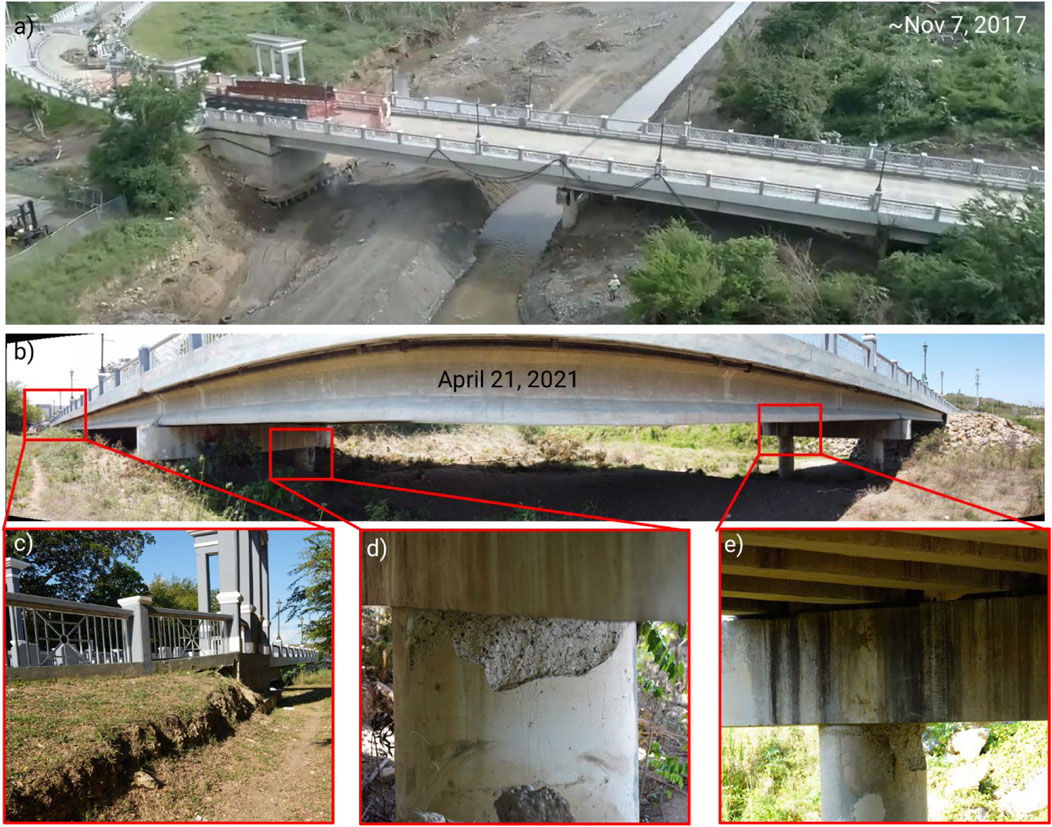
FIGURE 9 . Bridge in Guayanilla, PR over the Guayanilla River. These photos include (A) a screenshot from a drone flyover taken 50 days after hurricane Maria ( Maria, 2017 ), (B) an overall view of the bridge taken on the final reconnaissance mission, (C) the geotechnical impacts observed at the abutment, (D) cracking on support column on Pier 1, and (E) cracking of support column on Pier 2.
This case study was included to show an example of where the multihazard effect was not clear. While there was no direct evidence, the soil washout that occurred at the abutment and piers may have contributed to more extensive damage to the bridge columns than was observed at other bridges in the area. In addition, if this spalling is not repaired in a timely fashion, the column reinforcement at the critical plastic hinge zone will be subjected to premature corrosion damage, which will further weaken the structure.
The restoration of transportation infrastructure was prioritized in the aftermath of the hurricane, and the bridge structures visited were found to be in a relatively good condition. This was an important factor in avoiding any disruptive failure of the bridges after the earthquakes, thus ensuring continued access to the impacted areas.
5 Discussion
The cases presented illustrate multihazard impacts observed on different structure types and under different hazards. Multihazard reconnaissance is significantly more challenging because the state of the structure is not always documented after the first event, or through time. In addition, a consistent data collection approach is needed to ensure that the data collected at different times are comparable in terms of location and focus. The founding of the Structural Extreme Events Reconnaissance (StEER) Network in 2018 was a notable first step in this direction. However, we need to promote the use of a multihazard approach in reconnaissance. The traditional approach has been focused on documenting damage from a single event and ensuring meaningful information can be extracted from the observation data. It is important to evaluate the damages in the context of the condition of the structure and the potential interaction of multiple hazards, otherwise the findings may be misleading. Findings from reconnaissance are used to inform changes to design codes, thus it is critical to make sure the correct conclusions are drawn. For example, if we see failure of concrete elements without paying attention to level of corrosion or a change in the support conditions, we may misattribute the cause. While the data in this paper was not directly used to assess safety levels of the structures noted, it is our hope that the data presented will encourage the multihazard perspective in future reconnaissance missions. The safety assessment process requires well-calibrated and generalizable analytical models of structural types and systems, which is a major undertaking that was outside of the scope of this effort. However, as an intermediate approach, additional questions can be added to surveys in current field safety evaluation protocols and manuals to note potential evidence of prior damages due to other hazards or aging as well as the interaction of effects from multiple hazards.
Another consideration for the future of multihazard design in regions such as Puerto Rico is addressing conflicting design objectives. The design of buildings in Puerto Rico is largely dependent on the location of the structure. Most buildings in coastal areas of the island are concrete structures. These buildings resist high winds, but their large mass makes them vulnerable to the earthquakes. In addition, elevated construction is used for the buildings to prevent water damage due to storm surge. However, this system is vulnerable to earthquakes because of the soft story behavior as shown in Figure 10A . As additional regions become exposed to new and more extreme natural hazards due to climate change, the research on multihazard damages and design will need to be implemented in design codes.
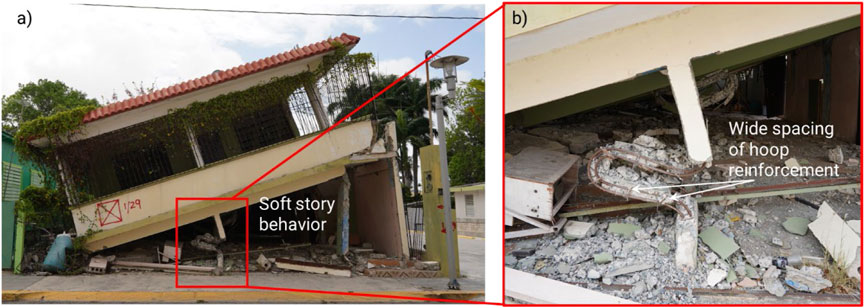
FIGURE 10 . Damage of building in Guánica, PR following earthquake series showing (A) overall damage and soft-story failure mode and (B) close up of support column collapse.
We would be remiss not to note the importance of contractors and inspectors adhering to designs. During the reconnaissance mission, the team overserved many buildings that appeared to have construction flaws such as poor-quality concrete or insufficient steel reinforcement ( Figure 10B ). Due to the limited information available to the team, it is unclear if the issues were caused in the design or construction phase. While these findings do not fall under the purview of multihazard design or inspection per se , they are critical components of forensic engineering to ascertain failure patterns.
6 Conclusion
This paper presents a series of case studies from multiple reconnaissance missions in the region surrounding Ponce, PR, analyzing the damages from the January 2020 earthquake series with linkage to Hurricane Maria, the Category four storm that struck the island in September 2017. In cases of subsequent hazards, degradation of structural capacity or shifts in boundary conditions from the primary event, along with the incremental or incomplete restoration processes prior to subsequent events, can have a significant impact on performance. The cascading effects of the two inherently different events that impacted Puerto Rico presented a unique opportunity for researchers to collect invaluable data and provide empirical evidence to characterize multihazard interactions.
Beyond the key insights gained from investigation of the case studies described in this paper, this study introduced a new field survey instrument. This instrument emphasized unique considerations for future field reconnaissance missions to systematically consider the potential for multiple event effects over short and long time periods. Moreover, the full dataset from multiple waves of reconnaissance was published on NHERI’s DesignSafe cyberinfrastructure platform to allow sharing and data reuse. Such data could provide a future opportunity to verify and validate structural system level damage and restoration models, particularly for the understudied area of life-cycle resilience in which incomplete recovery or damage accumulation affects subsequent event performance.
Data availability statement
The datasets presented in this study can be found in online repositories. The names of the repository/repositories and accession number(s) can be found in the article/supplementary material.
Author contributions
AH, AZ, and JP contributed to conception and design of the study, AT organized the database, AH, AZ, AT, and JP interpreted the results. AH wrote the first draft of the manuscript. AZ, AT, and JP wrote sections of the manuscript. All authors contributed to manuscript revision, read, and approved the submitted version.
This paper presents an overview of the reconnaissance missions in Ponce, PR and the surrounding region to document multihazard impacts from Hurricane Maria and the January 2020 earthquake series. The missions, data processing and curation were conducted under NSF Awards #2022390 and #2022427.
Acknowledgments
The authors would like to acknowledge the support and guidance of the RAPID Facility at the University of Washington, particularity Dr. Michael Grilliot who assisted with data collection and processing from the first field visit. In addition, the project would not have been possible without Angela Lanning, who supported the field missions and lead the archiving of the data.
Conflict of interest
The authors declare that the research was conducted in the absence of any commercial or financial relationships that could be construed as a potential conflict of interest.
Publisher’s note
All claims expressed in this article are solely those of the authors and do not necessarily represent those of their affiliated organizations, or those of the publisher, the editors and the reviewers. Any product that may be evaluated in this article, or claim that may be made by its manufacturer, is not guaranteed or endorsed by the publisher.
Aranda, E., Blackwell, R., Escue, M., and Rosa, A. (2022). Cascading disasters: The impact of hurricane Maria and Covid-19 on post-disaster Puerto Rican migrants’ adaptation and integration in Florida. Lat. Stud. 2022, 1–24. doi:10.1057/s41276-022-00390-3
PubMed Abstract | CrossRef Full Text | Google Scholar
Argyroudis, S. A., Mitoulis, S. A., Hofer, L., Zanini, M. A., Tubaldi, E., and Frangopol, D. M. (2020). Resilience assessment framework for critical infrastructure in a multi-hazard environment: Case study on transport assets. Sci. Total Environ. 714, 136854. doi:10.1016/j.scitotenv.2020.136854
Baheru, T., Chowdhury, A. G., Bitsuamlak, G., Masters, F. J., and Tokay, A. (2014). Simulation of wind-driven rain associated with tropical storms and hurricanes using the 12-fan Wall of Wind. Build. Environ. 76, 18–29. doi:10.1016/j.buildenv.2014.03.002
CrossRef Full Text | Google Scholar
Barbato, M., Petrini, F., Unnikrishnan, V. U., and Ciampoli, M. (2013). Performance-based hurricane engineering (PBHE) framework. Struct. Saf. 45, 24–35. doi:10.1016/j.strusafe.2013.07.002
Berman, J. W., Wartman, J., Olsen, M., Irish, J. L., Miles, S. B., Tanner, T., et al. (2020). Natural hazards reconnaissance with the NHERI RAPID facility. Front. Built Environ. 6, 573067. doi:10.3389/fbuil.2020.573067
Blake, E. S. (2018). The 2017 atlantic hurricane season: Catastrophic losses and costs. Weatherwise 71 (3), 28–37. doi:10.1080/00431672.2018.1448147
Bruneau, M., Barbato, M., Padgett, J. E., Zaghi, A. E., Mitrani-Reiser, J., and Li, Y. (2017). State of the art of multihazard design . Virginia, United States: American Society of Civil Engineers .
Google Scholar
Burke, S., and Bruneau, M. (2016). Effect of surface roughness on cyclic ductility of corroded steel. J. Struct. Eng. 142 (6), 04016014. doi:10.1061/(asce)st.1943-541x.0001425
Echevarria, A., Zaghi, A. E., Chiarito, V., Christenson, R., and Woodson, S. (2015). Experimental comparison of the performance and residual capacity of CFFT and RC bridge columns subjected to blasts. J. Bridge Eng. 21 (1), 04015026. doi:10.1061/(asce)be.1943-5592.0000762
Echevarria, A., Zaghi, A. E., Christenson, R., and Accorsi, M. (2015a). CFFT bridge columns for multihazard resilience. J. Struct. Eng. 2015, C4015002.
Echevarria, A., Zaghi, A. E., Christenson, R., and Plank, R. (2015b). Residual axial capacity comparison of CFFT and RC bridge columns after fire. Polymers 7 (5), 876–895. doi:10.3390/polym7050876
Eisenberg, D. A., Park, J., and Seager, T. P. (2020). Linking cascading failure models and organizational networks to manage large-scale blackouts in South Korea. J. Manag. Eng. 36 (5), 04020067. doi:10.1061/(asce)me.1943-5479.0000820
Emery, R., Serrano, M. I., and Foster, D. R. (2004). Landscape and regional impacts of hurricanes in Puerto Rico. Ecol. Monogr. 74 (2), 335–352. doi:10.1890/02-4057
FEMA, (2018). 2017 hurricane season FEMA after-action report . Washington, DC: Federal Emergency Management Agency .
Gill, J. C., and Malamud, B. D. (2014). Reviewing and visualizing the interactions of natural hazards. Rev. Geophys. 52 (4), 680–722. doi:10.1002/2013rg000445
Hain, A., Zaghi, A., and Padgett, J. (2022). “Data collection of damage to Puerto Rico's infrastructure due to Hurricane Maria and the 2019-2020 Puerto Rico earthquakes - mission 2,” in RAPID: Multi-hazard damage to Puerto Rico’s infrastructure - investigation of the interaction of hurricane and earthquakes . version 1 (Austin, TX: Natural Hazards Engineering Research Infrastructure: Cyberinfrastructure (DesignSafe) 2020–2025 ). Available at: https://www.nsf.gov/awardsearch/showAward?AWD_ID=2022469 . doi:10.17603/ds2-1mny-a490
Havidan, R. (1997). A socioeconomic analysis of hurricanes in Puerto Rico: An overview of disaster mitigation and preparedness. Hurricanes 1997, 121–143. doi:10.1007/978-3-642-60672-4_7
Hayes, G. P., McNamara, D. E., Seidman, L., and Roger, J. (2014). Quantifying potential earthquake and tsunami hazard in the Lesser Antilles subduction zone of the Caribbean region. Geophys. J. Int. 196 (1), 510–521. doi:10.1093/gji/ggt385
Imani, R., Mosqueda, G., and Bruneau, M. (2015). Finite element simulation of concrete-filled double-skin tube columns subjected to postearthquake fires. J. Struct. Eng. 141 (12), 04015055. doi:10.1061/(asce)st.1943-541x.0001301
Jaimes, M. A., Reinoso, E., and Esteva, L. (2015). Risk analysis for structures exposed to several multi-hazard sources. J. Earthq. Eng. 19 (2), 297–312. doi:10.1080/13632469.2014.962673
Jansma, P. E., Mattioli, G. S., Lopez, A., DeMets, C., Dixon, T. H., Mann, P., et al. (2000). Neotectonics of Puerto Rico and the Virgin Islands, northeastern Caribbean, from GPS geodesy. Tectonics 19 (6), 1021–1037. doi:10.1029/1999tc001170
Kameshwar, S., and Padgett, J. E. (2014). Multi-hazard risk assessment of highway bridges subjected to earthquake and hurricane hazards. Eng. Struct. 78, 154–166. doi:10.1016/j.engstruct.2014.05.016
Keller, W. J., and Pessiki, S. (2015). Effect of earthquake-induced damage on the sidesway response of steel moment-frame buildings during fire exposure. Earthq. Spectra 31 (1), 273–292. doi:10.1193/123112eqs362m
Kumar, R., and Gardoni, P. (2014). Effect of seismic degradation on the fragility of reinforced concrete bridges. Eng. Struct. 79, 267–275. doi:10.1016/j.engstruct.2014.08.019
Li, Y., Ahuja, A., and Padgett, J. E. (2012). Review of methods to assess, design for, and mitigate multiple hazards. J. Perform. Constr. Facil. 26 (1), 104–117. doi:10.1061/(asce)cf.1943-5509.0000279
Liu, W., Song, Z., and Ouyang, M. (2020). Lifecycle operational resilience assessment of urban water distribution networks. Reliab. Eng. Syst. Saf. 198, 106859. doi:10.1016/j.ress.2020.106859Get
Lloréns, H. (2018). Ruin Nation: In Puerto Rico, Hurricane Maria laid bare the results of a long-term crisis created by dispossession, migration, and economic predation. NACLA Rep. Am. 50 (2), 154–159. doi:10.1080/10714839.2018.1479468
López-Marrero, T., Heartsill-Scalley, T., Carlos, F-L-G-R., Escalera-Garcia, I. A., and Echevarria-Ramos, M. (2019). Broadening our understanding of hurricanes and forests on the caribbean island of Puerto Rico: Where and what should we study now? Forests 10 (9), 710. doi:10.3390/f10090710
Maniglio, M., Balomenos, G. P., Padgett, J. E., and Cimellaro, G. P. (2021). Parameterized coastal fragilities and their application to aging port structures subjected to surge and wave. Eng. Struct. 237, 112235. doi:10.1016/j.engstruct.2021.112235
Maria, H. (2017). PhotoSkyView. Hurricane Maria - the main bridge of Guayanilla after 50 Days (huracán Maria) - phantom 4Pro. https://www.youtube.com/watch?v=XHTrmqMBkDk&list=PLLvcsDpvAIDjPaWbKuWRKRFtYGtPqlILy&index=7 .
Miranda, E., Acosta Vera, A., Aponte, L., Archbold, J., Cortes, M., Du, A., et al. StEER - 7 jan. 2020 Puerto Rico Mw 6.4 earthquake: Preliminary virtual reconnaissance report (PVRR). 2020.
Ouyang, M., Dueñas-Osorio, L., and Min, X. (2012). A three-stage resilience analysis framework for urban infrastructure systems. Struct. Saf. 36, 23–31. doi:10.1016/j.strusafe.2011.12.004
Padgett, J. E., and Kameshwar, S. (2016). “Supporting life cycle management of bridges through multi-hazard reliability and risk assessment,” in Multi-hazard approaches to civil infrastructure engineering (Berlin, Germany: Springer ), 41–58.
Pasch, R. J., Penny, A. B., and Berg, R. (2017). Hurricane Maria (AL152017) . United States: National Hurricane Center .
Rathje, E., Dawson, C., Padgett, J. E., Pinelli, J-P., Stanzione, D., Adair, A., et al. (2017). DesignSafe: A new cyberinfrastructure for natural hazards engineering . Reston, VA: American Society of Civil Engineers .
Raussen, S., and Lykke-Andersen, H. (2009). “Micro plate tectonics in the Virgin Islands Basin, north eastern Caribbean,” in AGU Fall Meeting Abstracts , Chicago, IL , 12-16, December, 2022 .
Roy, T., and Matsagar, V. (2021). Multi-hazard analysis and design of structures: Status and research trends. Struct. Infrastructure Eng. 2021, 1–30. doi:10.1080/15732479.2021.1987481
Sanchez, R. (2020). For Puerto Rico’s earthquake families, trauma continues thousands of miles from home . Georgia, United States: CNN .
Shiraki, N., Shinozuka, M., Moore, J. E., Chang, S. E., Kameda, H., and Tanaka, S. (2007). System risk curves: Probabilistic performance scenarios for highway networks subject to earthquake damage. J. Infrastructure Syst. 13 (1), 43–54. doi:10.1061/(asce)1076-0342(2007)13:1(43)
Silva-Tulla, F., Pando, M., Soto, A., Morales, A., Pradel, D., Inci, G., et al. (2018). Geotechnical impacts of hurricane Maria in Puerto Rico . Virginia, United States: ASCE . Report No GEER-057.
Tillman, B. F. (2009). Measuring globalization's influence on the cultural landscape: Spatial succession in the plaza of Ponce, Puerto Rico. Southeast. Geogr. 49 (4), 340–353. doi:10.1353/sgo.0.0056
USGS, M. (2020). 6.4 - 8km S of indios, Puerto Rico. Available at: https://earthquake.usgs.gov/earthquakes/eventpage/us70006vll/map?shakemap-code=us70006vll&shakemap-source=us&shakemap-pga=true&shakemap-stations=true&shakemap-mmi-contours=false .
van de Lindt, J. W., Ellingwood, B. R., McAllister, T., Gardoni, P., Cox, D. T., Cutler, H., et al. (2015). “Computational environment for modeling and enhancing community resilience: Introducing the center for risk-based community resilience planning,” in Second International Conference on Performance-based and Lifecycle Structural Engineering (PLSE 2015) , Brisbane, AU , December 9-11, 2015 .
Van Der Elst, N., Hardebeck, J. L., and Michael, A. J. (2020). Potential duration of aftershocks of the 2020 southwestern Puerto Rico earthquake . US Geological Survey: US Geological Survey , 2331–1258.
Vičič, B., Momeni, S., Borghi, A., Lomax, A., and Aoudia, A. (2022). The 2019–2020 Southwest Puerto Rico earthquake sequence: Seismicity and faulting. Seismol. Soc. Am. 93 (2A), 533–543. doi:10.1785/0220210113
Vishnu, N., and Padgett, J. E. (2020). Interaction of life-cycle phases in a probabilistic life-cycle framework for civil infrastructure system sustainability. Sustain. Resilient Infrastructure 5 (5), 289–310. doi:10.1080/23789689.2019.1574514
Wakeman, T. H. (2013). University Transportation Research Center—Region 2 . Washington, DC: University Transportation Research Center, US Department of Transportation . 49997-56-24. Available at: https://rosap.ntl.bts.gov/view/dot/27004 .
Wang, Z., Dueñas-Osorio, L., and Padgett, J. E. (2014). Influence of scour effects on the seismic response of reinforced concrete bridges. Eng. Struct. 76, 202–214. doi:10.1016/j.engstruct.2014.06.026
Wei, L., Hu, K., Hu, X., Wu, C., and Zhang, X. (2022). Quantitative multi-hazard risk assessment to buildings in the Jiuzhaigou valley, a world natural heritage site in Western China. Geomatics, Nat. Hazards Risk 13 (1), 193–221. doi:10.1080/19475705.2021.2004244
Yang, D. Y., and Frangopol, D. M. (2019). Life-cycle management of deteriorating civil infrastructure considering resilience to lifetime hazards: A general approach based on renewal-reward processes. Reliab. Eng. Syst. Saf. 183, 197–212. doi:10.1016/j.ress.2018.11.016
Zaghi, A. E., Padgett, J. E., Bruneau, M., Barbato, M., Li, Y., Mitrani-Reiser, J., et al. (2016). Establishing common nomenclature, characterizing the problem, and identifying future opportunities in multihazard design. J. Struct. Eng. 142 (12), H2516001. doi:10.1061/(asce)st.1943-541x.0001586
Zaghi, A. P., Hain, J., Tafur, A., Lanning, A., and Grilliot, A., (2021). “Data collection of damage to Puerto Rico's infrastructure due to Hurricane Maria and the 2019-2020 Puerto Rico earthquakes,” in RAPID: Multi-hazard damage to Puerto Rico’s infrastructure - investigation of the interaction of hurricane and earthquakes . (Austin, TX: Natural Hazards Engineering Research Infrastructure: Cyberinfrastructure (DesignSafe) 2020–2025 ). Available at: https://www.nsf.gov/awardsearch/showAward?AWD_ID=2022469 . version 1. doi:10.17603/ds2-jj5g-yg64
Keywords: multihazard, reconnaissance, earthquakes, Hurricane Maria, cascading effects
Citation: Hain A, Zaghi AE, Padgett JE and Tafur A (2023) Case studies of multihazard damage: Investigation of the interaction of Hurricane Maria and the January 2020 earthquake sequence in Puerto Rico. Front. Built Environ. 9:1128573. doi: 10.3389/fbuil.2023.1128573
Received: 20 December 2022; Accepted: 27 January 2023; Published: 13 February 2023.
Reviewed by:
Copyright © 2023 Hain, Zaghi, Padgett and Tafur. This is an open-access article distributed under the terms of the Creative Commons Attribution License (CC BY). The use, distribution or reproduction in other forums is permitted, provided the original author(s) and the copyright owner(s) are credited and that the original publication in this journal is cited, in accordance with accepted academic practice. No use, distribution or reproduction is permitted which does not comply with these terms.
*Correspondence: Alexandra Hain, [email protected]
This article is part of the Research Topic
Technology Transfer from the Natural Hazards Engineering Research Infrastructure (NHERI)

An official website of the United States government
Here’s how you know
Official websites use .gov A .gov website belongs to an official government organization in the United States.
Secure .gov websites use HTTPS A lock ( Lock A locked padlock ) or https:// means you’ve safely connected to the .gov website. Share sensitive information only on official, secure websites.
https://www.nist.gov/disaster-failure-studies/hurricane-maria-program
Disaster & failure studies
Hurricane maria, nist research and investigation.
Español sitio web
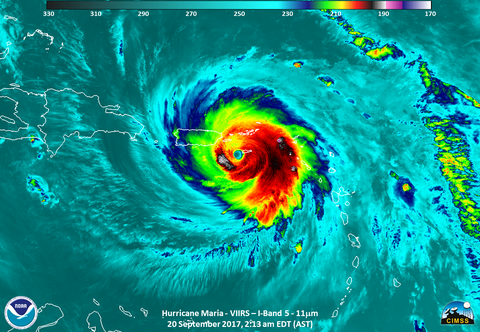
On September 20, 2017, Hurricane Maria devastated much of Puerto Rico, damaging buildings that its communities relied on for medical care, safety, communications and more. To better understand how the buildings and infrastructure failed, and how we can prevent such failures in the future, in 2018 NIST launched a multi-year effort to study how critical buildings performed during the storm, as well as how emergency communications systems worked.
The goal of this effort is to make recommendations to improve building codes, standards and practices to make communities across the U.S. more resilient to hurricanes and other disasters. NIST has a long history of studying disasters so that we can learn from them and improve our buildings or procedures. For example, after the World Trade Center disaster, NIST’s recommendations led to changes in how we build buildings and respond to emergencies. Additionally, thanks to NIST’s work studying the effects of tornadoes, building codes and communications practices have been changed to keep people safer.
In Puerto Rico, NIST seeks to understand Hurricane Maria’s wind environment and the conditions that led to injuries and deaths; how critical buildings and designated safe areas within them performed—including their dependence on electricity, water, transportation and other infrastructure; how emergency communications systems performed and the public’s response to such communications; and the impacts to, and recovery of, selected businesses, hospitals and schools, as well as the critical social functions they provide.
The NIST team is involving local and regional emergency management officials; building departments, transportation and other public utilities; education and healthcare officials and staff; and local, regional, and Commonwealth elected officials and civil servants as well as individuals. NIST also is coordinating its work with other federal agencies, private sector organizations, and academic institutions. Several contractors are assisting the NIST team’s engineers, sociologists, economists, meteorologists, and other researchers. Because NIST is not a regulatory agency, it does not issue new codes or standards. However, NIST will work with all relevant public and private citizens to encourage voluntary implementation of the recommendations in its final report.
Information Collection in Progress NIST contractors are now collecting information for multiple Hurricane Maria related projects. If you or your organization (e.g., business, school, hospital) have been contacted, please consider participating. Your response will contribute to recommended improvements in building codes, standards, and practices to make communities in Puerto Rico and across the United States more resilient to hurricanes and other disasters. See the Hurricane Maria Projects page for project-specific information. If you have questions about this collection of information, please contact Dr. Maria Dillard, NIST: Maria.Dillard [at] NIST.gov (Maria[dot]Dillard[at]NIST[dot]gov) . or via telephone 202-281-0908.
Media Contact
- Jennifer Huergo [email protected] (301) 975-6343
- ALL OTHER INQUIRIES [email protected]

Research examines tweets during Hurricane María to analyze social media use during disasters
Social media can be a useful tool for communicating during extreme weather events. Omar Pérez Figueroa, an urban and regional planning professor at the University of Illinois Urbana-Champaign, examined how Twitter (now X) was used when Hurricane María hit Puerto Rico in 2017.
His findings are published in the Journal of Environmental Studies and Sciences .
Pérez Figueroa studies how impoverished and marginalized communities address environmental inequalities, water governance and disaster resilience.
For his research on social media and risk communication, he collected data from Twitter before the platform changed its name, so he continued to refer to it as Twitter for his study. He analyzed a sample of more than 2,000 tweets from before Hurricane María made landfall to six months after the storm to determine "what the event tells us about the reactions and behavior of people, and what is useful to understand to be better prepared."
Pérez Figueroa analyzed the timing of the tweets and their geographic distribution. Most of them were made prior to landfall, with smaller peaks of activity at later times -- for example, when the Federal Emergency Management Agency deleted Puerto Rican disaster statistics from its website. In the U.S., those tweeting about the hurricane were highly concentrated in Florida, California, New York and Texas, all areas with large Puerto Rican populations.
The sample included tweets from Puerto Rico, despite the collapse of the island's power grid. Pérez Figueroa said Twitter doesn't require a strong signal, making sending messages under disaster conditions more accessible.
He identified three main categories of messages: information about what was happening on the ground in Puerto Rico; understanding the social and political conditions that turned the hurricane into a disaster; and emotional messages seeking help or expressing support. Those in Puerto Rico used social media to inform others about their condition and location, ask for and receive help, and document the disaster.
Pérez Figueroa wrote that many tweets about the sociopolitical causes of the disaster blamed local and federal governments for their slow and inefficient response. He said it underscored the use of social media in solidarity and grassroots recovery efforts; as a tool to pressure for prompt government response and the development of policies that address vulnerabilities; and to communicate needs and raise awareness among people not directly affected by the local event about where to donate.
The tweets included information about the Guajataca Dam failure as it occurred.
"Being able to access critical data during and after a disaster can provide the difference between life and death, especially for those on the ground. Recognizing the increasing use of social media for disaster response and aid can increase communities' disaster resilience, especially when the communities hardest hit have limited communication and are remote, like the case of Puerto Rico," Pérez Figueroa wrote.
In addition to aiding in preparedness and recovery for future disasters, the research served as a case study to test and refine a framework for examining disaster social media communication, Pérez Figueroa said.
"Understanding how individuals use Twitter during disasters and in the immediate aftermath will be important as hurricanes occur more frequently, and as the public continues to turn to social media as a mainstream news source. Public health and disaster response organizations will benefit from a more detailed understanding as to who and how communication on social media can play a role in mitigating the public health burdens that result from disaster events," he wrote.
Pérez Figueroa said areas for future research include how traditional media can influence discussions on social media, how narratives on the two differ, whether social media influences emergency responders and policymakers to improve disaster response, and how groups from different socioeconomic levels use social media during disasters.
- Natural Disasters
- Environmental Awareness
- Severe Weather
- Disaster Plan
- Social Issues
- Media and Entertainment
- Popular Culture
- Disaster recovery plan
- List of disasters
- Adventure travel
- Weather forecasting
- Timeline of environmental events
- Social science
Story Source:
Materials provided by University of Illinois at Urbana-Champaign, News Bureau . Original written by Jodi Heckel. Note: Content may be edited for style and length.
Journal Reference :
- Omar Pérez-Figueroa, Nícola Ulibarrí, Suellen Hopfer. A content analysis of social media discourse during Hurricane María: filling a void when traditional media are silent . Journal of Environmental Studies and Sciences , 2024; DOI: 10.1007/s13412-024-00909-1
Cite This Page :
Explore More
- Otters Use Tools to Survive a Changing World
- Monogamy in Mice: Newly Evolved Type of Cell
- Sustainable Electronics, Doped With Air
- Male Vs Female Brain Structure
- Breeding 'Carbon Gobbling' Plants
- Why Do We Overindulge?
- Jelly Sea Creatures and Underwater Robotics
- Teeth of Sabre-Toothed Tigers
- Robotic 'SuperLimbs' Could Help Moonwalkers
- Solving Quantum Many-Body Problems
Trending Topics
Strange & offbeat.

IMAGES
COMMENTS
Hurricane Maria. Project Author: Emily Dougherty-Samowitz. In this case study, students follow the work of Rafael Irizarry and collaborators, as they use tools covered in AP Stats (random sampling methods & inference) to correct the narrative on Hurricane Maria's death toll in Puerto Rico. This flexible project can be used during the school ...
Case Study: Puerto Rico and Hurricane Maria Differential response and recovery times across U.S. communities hit by 2017 storms. ... The data in the report clearly show a disparity in the amount of aid received and the time taken for aid to be received. This article highlights the critical nature of getting aid to its target in a timely manner.
"Our study presents a new methodology to assess the social vulnerability of extreme weather events, using Hurricane Maria as a case study." Reconstructing Hurricane Maria. The study reconstructs the impacts of Hurricane Maria on Puerto Rico through a combination of geophysical models, engineering models of both the power and water grid ...
Hurricane Maria devastated Puerto Rico in September 2017. During the storm, sustained winds of 155 mph caused 1.5 million grid-connected customers across the island to suffer from long duration power failures. Historically, data provided by utilities have been the sole source of outage information for response operations.
We describe the supervised treatment-effect approach in detail and illustrate its applicability using panel data encompassing the 2017 Hurricane Maria event across various municipalities in Puerto Rico. ... A Human Impacts-Driven Framework for Quantifying Disaster-Dependent Social Vulnerability: A Case Study of Hurricane Maria in Puerto Rico ...
That study estimated that there had been 151,000 excess deaths from violence in Iraq between March 2003 and June 2006 — about one-quarter of what Lafta and his colleagues had found. A farmer in ...
The NIST study has four main points of focus. First, it seeks to understand the hurricane's wind environment and the conditions that led to injuries and deaths. Second, it evaluates the performance of critical buildings and designated safe areas within them, including their dependence on electricity, water, transportation and other ...
periods over 2012-2016 (pre-Hurricane Maria), compared to the data from the RAMP deployed at UPR-Rio Piedras (Nov 22-Dec 21, 2017, post-Hurricane Maria). Also shown are the distributions for past data (Nov-Dec 2012-2016) for maximum daily 1-hour CO at the two "high concentration" EQB sites, including a near-road site at Guaynabo.
On September 20, 2017, only two weeks after Irma devastated the island of Puerto Rico, Hurricane Maria made landfall as a Category 4 hurricane. Maria caused catastrophic damage, and left the citizens of Puerto Rico without power and other critical products and services for the longest period of time in Puerto Rican history [4].
Figure 12 shows the results obtained from Hurricane Irma data, and Figure 13 shows the topics obtained from Hurricane Maria data. Different than traditional clustering techniques in which one data point (e.g. tweet text in our case) can only belong to one cluster/topic, in topic modelling, it can belong to different topics.
On September 20, 2017, only two weeks after Irma devastated the island of Puerto Rico, Hurricane Maria made landfall as a Category 4 hurricane. Maria caused catastrophic damage, and left the citizens of Puerto Rico without power and other critical products and services for the longest period of time in Puerto Rican history [4].
Abstract. In this middle school bilingual science unit, students used computational modeling in Scratch to aid their discussions about a topic that hit close to home for students: Hurricane María. Sponsored by the National Science Foundation under NSF grants CNS-1738645 and DRL-1837446. Any opinions, findings, and conclusions or ...
In a new study, led by Jorge González-Cruz of the University at Albany's Atmospheric Sciences Research Center (ASRC), researchers are now using data from Hurricane Maria to assess the critical ...
Social capital and individual well-being in the post-disaster period: The case of Hurricane Maria in Puerto Rico, International Journal of Disaster Risk Reduction, 103, (104308), (2024). https ...
Activity was defined as an event on at least 10 d/mo for all 12 mo. Due to the unreliability of data, proportion estimates for 4 wk after Hurricane Maria were not provided. For every day, a distinct number of subscribers in Puerto Rico is computed by considering events generated from different mobile devices.
We select Puerto Rico as the case study region and Hurricanes Irma and Maria as the disaster events because of their substantial and longitudinal disaster impacts on the island, causing power outages and water shortages for the entire island for several months (Román et al., 2019). As of 2020, Puerto Rico's population stands at 2.87 million ...
A great deal of flooding occurred due to the more than 30 inches of rain that fell. It was the third costliest hurricane in U.S. history, behind Katrina (2005) and Harvey (2017). While the official death count from Maria was 64, a Harvard study published on May 29, 2018 estimated the number of deaths to be around 4,645 (95% confidence interval ...
Stem damage and mortality. Hurricane María killed twice as many stems as Hugo in the LFDP (Fig. 2, Supplementary Table 1-3).The proportion of uprooted stems, however, was similar for Hugo and ...
September 28, 2018 - After Hurricane Maria slammed into Puerto Rico on September 20, 2017, statistician and genomics expert Rafael Irizarry of Harvard T.H. Chan School of Public Health, a native of Puerto Rico, was hearing dire reports of death and destruction from friends and family on the island. So when the governor of Puerto Rico announced in early December 2017 that there had been only ...
This paper is motivated by the unique findings and observations from reconnaissance visits after the earthquake series in Puerto Rico in January 2020. It aims to discuss the potential interactions of Hurricane Maria and 2020 earthquake series and the considerations they underscore for future field reconnaissance missions. Traditionally, post-disaster damage assessment activities focus on one ...
See the Hurricane Maria Projects page for project-specific information. If you have questions about this collection of information, please contact Dr. Maria Dillard, NIST: [email protected]. or via telephone 202-281-0908. Resilience and Disaster and failure studies.
Research examines tweets during Hurricane María to analyze social media use during disasters Date: April 9, 2024 Source: University of Illinois at Urbana-Champaign, News Bureau