- Analytic Perspective
- Open access
- Published: 04 December 2007

Case-cohort design in practice – experiences from the MORGAM Project
- Sangita Kulathinal 1 ,
- Juha Karvanen 2 ,
- Olli Saarela 2 ,
- Kari Kuulasmaa 2 &
the MORGAM Project
Epidemiologic Perspectives & Innovations volume 4 , Article number: 15 ( 2007 ) Cite this article
28k Accesses
91 Citations
1 Altmetric
Metrics details
When carefully planned and analysed, the case-cohort design is a powerful choice for follow-up studies with multiple event types of interest. While the literature is rich with analysis methods for case-cohort data, little is written about the designing of a case-cohort study. Our experiences in designing, coordinating and analysing the MORGAM case-cohort study are potentially useful for other studies with similar characteristics. The motivation for using the case-cohort design in the MORGAM genetic study is discussed and issues relevant to its planning and analysis are studied. We propose solutions for appending the earlier case-cohort selection after an extension of the follow-up period and for achieving maximum overlap between earlier designs and the case-cohort design. Approaches for statistical analysis are studied in a simulation example based on the MORGAM data.
1 Introduction
The MORGAM (MONICA, Risk, Genetics, Archiving, and Monograph) Project is an ongoing multinational collaborative study with the overall aim of studying a limited number of well-defined phenotypes and several hundred genetic factors by pooling data from cohorts defined in MONICA (Multinational MONItoring of trends and determinants in CArdiovascular disease) and other similar cross-sectional risk factor surveys [ 1 , 2 ]. In brief, MORGAM cohorts are the respondents of random survey samples from geographically defined populations for whom several baseline measurements were made. The MORGAM cohorts are followed up prospectively for all-cause mortality and non-fatal coronary heart disease (CHD) and stroke events.
The study aims at exploring the relationships between the development of cardiovascular diseases and their classic and genetic risk factors. MORGAM opted for a case-cohort design for its genetic study because genotyping of the entire cohorts is not viable due to the cost consideration and because there is interest in several definitions of a case. Cohort sampling designs are used in follow-up studies when large cohorts are needed to observe enough cases but it is not feasible to collect data on all covariates for the whole cohort. Commonly used designs such as the case-control or the nested case-control design require genotyping of all the cases and matched controls for each case. The case-cohort design requires genotyping of: (1) a random subsample of the original cohort (subcohort), selected independently of the definition of cases; and, (2) all cases outside the subcohort, i.e. all members of the cohort developing any or all events of interest during the follow-up. The union of (1) and (2) is referred to as the case-cohort set. A conceptual illustration of case-cohort design is presented in Figure 1 . Note that the cases are overrepresented in the case-cohort set compared to the original cohort.
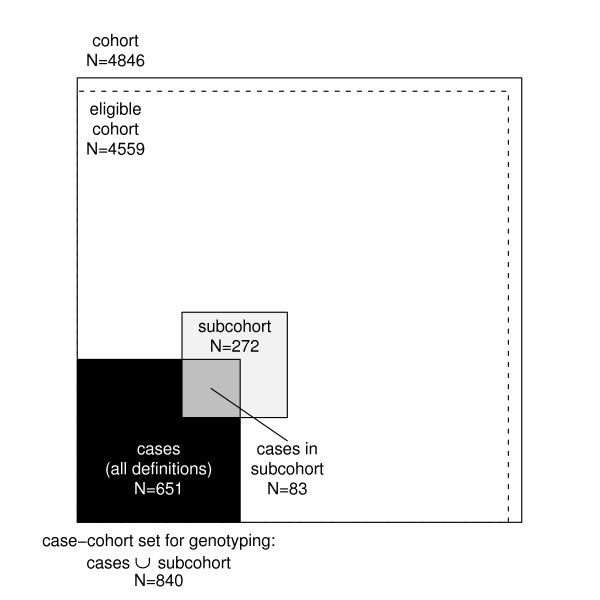
Conceptual illustration of the case-cohort design in the example cohort. Areas are proportional to numbers of observations.
Compared to the designs where case-matched controls are selected, a distinct advantage of the case-cohort design is that the selected subcohort can be used for analysing several endpoints of interest. Furthermore, as the subcohort forms a random sample of the original cohort, it can be used to assess the genetic distribution of the population. If the subcohort is selected efficiently, the statistical power of gene-disease association is not substantially reduced compared to the alternative where the full cohort is genotyped. The theoretical foundation for the design was formulated in 1986 by Prentice [ 3 ] although an epidemiological study design similar to the case-cohort design was suggested already in 1975 by Kupper et al. [ 4 ] and in 1982 by Miettinen [ 5 ]. During the past twenty years, several authors have considered the case-cohort design from various viewpoints including sampling of the subcohort, weighting methods for the analysis, variance estimation, and comparison with the case-control and the nested case-control design.
Nowadays the case-cohort design is one of the standard designs under prospective follow-up studies and the analysis methods can be implemented in commonly used statistical software packages such as R [ 6 ] and SAS [ 7 ].
The sampling of the subcohort itself has gained relatively little attention in literature. It is important to note that the follow-up and covariate data collected for the complete cohort can be utilised in choosing the subcohort to improve the efficiency of the design. The sampling probabilities may be defined within strata formed using matching variables or at the individual level. The stratified case-cohort design is studied by Borgan et al. [ 8 ], Kulich and Lin [ 9 ] and Samuelsen et al. [ 10 ]. Kim and De Gruttola [ 11 ] compare various strategies for cohort sampling and propose an efficient subcohort sampling procedure where the sampling probabilities are proportional to predictive probabilities calculated from a logistic regression model that explains the probability of being a case by matching variables. Using this approach the distribution of important background variables will be similar for cases and the subcohort. A modification of this approach is applied in the MORGAM Project. Cai and Zeng [ 12 ] and Kim et al. [ 13 ] consider the calculation of sample size and power in case-cohort studies.
Much of the literature on time-to-event analysis of case-cohort data has concentrated on the relative risk model and modifications to Cox's partial likelihood [ 14 ]. Adjustments to the partial likelihood are required because the cases are overrepresented in the case-cohort set and therefore unadjusted risk sets in the partial likelihood would not represent the original study cohort. The original pseudolikelihood estimator proposed by Prentice [ 3 ] uses a weighting where risk sets at event times consist of subcohort members at risk while the cases outside the subcohort enter the risk sets only at their event time. A slight modification by Self and Prentice [ 15 ] did not include the non-subcohort cases in the risk sets at all. Kalbfleisch and Lawless [ 16 ] suggested including all cases in the risk sets with weight one and weighting the remaining subcohort members with inverse subcohort sampling probability. Barlow [ 17 ] proposed a time-dependent weighting where the weights for the subcohort members are defined as the ratio of the number of cohort members at risk to the number of subcohort members at risk. Barlow [ 18 ] approximated this quantity by the inverse of the subcohort sampling fraction. Kulich and Lin [ 9 ] propose a class of weighted estimators with general time-varying weights. Samuelsen et al. [ 10 ] presents an analysis approach for general cohort sampling designs, including the case-cohort design, where the weighting is based on post-stratification on case status and other factors.
The different weighting schemes are compared by Barlow et al. [ 18 ], Petersen et al. [ 19 ] and most recently by Onland-Moret et al. [ 20 ]. The results suggest that when the size of the subcohort is sufficiently large (for instance, over 15% of the full cohort according to [ 20 ]) all weighting schemes give similar estimates that differ only slightly from the full cohort estimates. When the size of the subcohort is small compared to the original cohort, the authors report that the Prentice estimator may have better small sample properties than the other approaches.
Variance estimation under the case-cohort design is an important topic because, for example, the standard variance estimators for relative hazard parameters in the Cox regression model are not valid for the case-cohort situation. The lack of variance estimators suitable for the Cox regression analysis of case-cohort data in standard statistical software may have initially limited the application of the design [ 18 ]. Self and Prentice [ 15 ] give conditions for the consistency and asymptotic normality of the pseudolikelihood estimator. Wacholder [ 21 ], Lin and Ying [ 22 ] and Barlow [ 17 ] have proposed variance estimators for Cox regression analysis under the case-cohort design. Barlow [ 17 ] showed that the robust variance estimator of Lin and Wei [ 23 ] is equivalent to a jackknife variance estimator, which can be directly applied to a case-cohort situation. Program codes for the computation of estimators are provided by Barlow et al. [ 18 ], Therneau and Li [ 24 ] and Langholz and Jiao [ 25 ]. Robust variance estimation is implemented in the recent versions of R and SAS software and can be applied in analysis of case-cohort data when appropriate weighting is used.
In addition to the pseudolikelihood based time-to-event analysis, some authors have recently considered a full likelihood approach where the cohort sampling design is handled as a missing data problem. In this approach the likelihood expression is constructed for the complete cohort instead of the case-cohort set. Parameter estimation can then be carried out using the expectation maximisation (EM) algorithm [ 26 ] or Bayesian data augmentation [ 27 ]. The full likelihood estimation is computationally more demanding due to the large amount of missing covariate data generated by the design but has systematically better performance, although the gain in efficiency is minor in case of a rare disease [ 26 ]. Further gain in efficiency can be achieved through modeling of possible dependencies between the covariate collected under the case-cohort design and the covariates collected for the complete cohort [ 27 ]. An alternative likelihood based approach which uses only the case-cohort set but maximises a likelihood that is conditioned on the inclusion in the case-cohort set was recently proposed by Saarela and Kulathinal [ 28 ]. The likelihood based approaches potentially allow use of more general survival models.
The case-cohort design and the nested case-control designs have been compared in various settings. Wacholder [ 21 ] compared the practical aspects of the designs. Langholz and Thomas [ 29 , 30 ] reported results from a simulation studies where under some settings the case-cohort design was found to be inferior to the nested case-control design. Zhao and Lipsitz [ 31 ] discuss twelve two-stage designs including the case-control and the case-cohort designs as special cases. Chen and Lo [ 32 ] establish a link between the case-cohort and the case-control sampling in terms of the estimation of regression parameters in Cox's model. Chen [ 33 ] studied the case-cohort, the nested case-control and the case-control designs through a unified approach.
In the above-mentioned references the use of the case-cohort design has been demonstrated, e.g., with data evaluating the efficacy of mammography screening in reducing breast cancer mortality [ 17 ], data from occupational exposure study of nickel refinery workers [ 18 ], data from an AIDS clinical trial [ 11 ], data on premature death of adult adoptees [ 19 ] and data on body mass index and cardiovascular disease [ 20 ]. In recent epidemiological studies, the case-cohort design has been applied, for instance, in a study of the risk of myocardial infarction following radiation therapy for breast cancer [ 34 ], a study of alcohol intake and cardiovascular disease [ 35 ], a study of the relation between cancer and medication exposures [ 36 ], and a study of occupational exposures and breast cancer among women textile workers [ 37 ].
The review of the literature on the case-cohort design reveals that the practical aspects of the study design have gained relatively little attention. For instance, in a recent methodological comparison [ 20 ], the authors explicitly state that they do not provide suggestions as to how to design a case-cohort study. In epidemiological study reports, the study design is usually briefly described. This paper describes the case-cohort design of the MORGAM Project in detail and discusses analysis approaches for case-cohort data with the intention of providing proper guidelines which would be helpful in designing studies with similar characteristics.
In the present article, we describe the procedure used in selecting the subcohort in the MORGAM case-cohort design and approaches to statistical analysis of the case-cohort data. In section 2, the MORGAM case-cohort selection is described in detail. Section 3 deals with completing the case-cohort set after extending of the follow-up period. In section 4, the MORGAM case-cohort design is compared with a local study design and a selection procedure for the MORGAM subcohort is proposed to ensure maximal overlap between the two designs. Section 5 deals with the assessment of the selection and data management issues. Statistical analysis approaches are described in section 6. Subcohort selection procedures and some analysis methods are compared with a simulation study in section 7. We conclude with a Discussion. Various aspects of the case-cohort design are illustrated using a single MORGAM cohort.
2 Selection of cases and subcohort in MORGAM
As mentioned in the Introduction, the subcohort selection procedures are not described in detail in the literature. In this section, we give a detailed account of subcohort selection procedure developed in MORGAM. The selection of cases and subcohort for each cohort is done centrally at the MORGAM Data Centre, Helsinki, after the baseline and follow-up data have been received from the participating centre and their quality have been approved. These data are used in identifying the cases and for selection of the subcohort using the common criteria and selection procedures for each cohort.
2.1 Eligible cohort for genetic sub-study
Availability of DNA and consent for the use of DNA are basic requirements for a genetic study. In MORGAM, availability of baseline data on the most important classic risk factors of cardiovascular diseases, namely smoking status, blood pressure and cholesterol is an additional requirement. An individual is considered eligible for the genetic sub-study if there is consent for the use of DNA to study both CHD and stroke and the information on smoking, blood pressure, cholesterol and DNA are available [ 38 ]. A cohort consisting of the eligible individuals is referred to as an eligible cohort for the genetic study. For some cohorts, it is feasible to assess the availability of DNA for the selected case-cohort set only. In such a case, availability of DNA is not a requirement for the eligibility, but the reasons for missing DNA for some individuals are assessed carefully in order to ensure that their absence will not unduly bias the results of the study.
2.2 Definitions of cases
As mentioned in the Introduction, several definitions of a case are of interest. They are defined using events such as different types of CHD, stroke, venous thromboembolic disease and death during the follow-up, as well as history of cardiovascular disease or stroke observed at the baseline examination. Based on the data from the baseline examination and follow-up of CHD, stroke, venous thromboembolic events and all-cause mortality, an individual experiencing any of these is a defined as a case and is selected for genotyping. For details, we refer the reader to the MORGAM Manual [ 38 ].
2.3 Subcohort sampling
Stratification.
The smallest geographic unit that can be identified in the MORGAM data is called a reporting unit (RU). It is often reasonable to combine RUs for data analysis, in particular if they represent small adjacent populations where the baseline surveys were carried out at the same time. Such combinations of RUs are called Reporting Unit Aggregates (RUA), and the individuals examined in the same survey (specified by calender period) in a RUA constitute a MORGAM cohort. The number of cohorts and their baseline years vary RUA by RUA. Within each RUA, the MORGAM case-cohort design is stratified according to cohort and sex. The procedure of selection of a sample from each stratum is described below.
Size of the subcohort
The size of subcohort can be defined as a fraction of the whole cohort or proportionally to number of cases. Because the proportion of cases vary cohort by cohort in the MORGAM Project, the size of subcohort was made proportional to the number of cases. It is known from the theory of the case-control design that the asymptotic relative efficiency for a study involving k controls per case is k /( k + 1), which takes values of 0.5, 0.67, 0.75, 0.8 and 0.83 for k from 1 to 5 [ 39 ]. Because of this and the limitation of the genotyping budget, the subcohort size within each stratum is defined using the main study endpoints as twice the maximum of the number of first acute CHD (fatal or non-fatal) and first stroke (fatal or non-fatal) events during the follow-up. Relatively strict definition of disease endpoints are used in defining the subcohort size compared to the wider definition used in the definition of cases (see section 2.2). This gives more freedom for defining the endpoint of interest at the analysis stage while not needlessly expanding the subcohort size. The subcohort thus selected should be large enough for studying also other endpoints because the number of cases furnished from other endpoints are generally smaller than the number of CHD and stroke cases. However, if the total subcohort size for a RUA (that is all cohorts within the RUA) happens to be less than 100 then the subcohort size in each stratum is adjusted so that the total size for the RUA is 100. This will allow the possibility of estimation of the genotypic distribution for each RUA.
Sampling within the stratum
The number of major endpoint events during the follow-up increases strongly with the age of the individual at baseline. Therefore, if all members of the cohort had an equal probability of being selected in the subcohort, the power of the design would suffer from the fact that the average age at baseline of the cases would be much higher than the average age of the subcohort. The power can be increased to a level comparable to the power of an age-matched case-control design by selecting the individuals of the subsample using age-distribution similar to the distribution of the baseline age of the cases [ 11 ].
For the cohort sampling in MORGAM, such a function of age is the mortality rate estimated using a logistic regression model for each RUA, by combining data from all its cohorts. The mortality rate is used because it is easy to define and it is a reasonably common endpoint in all cohorts, hence providing stable estimates. The increase of the mortality rate with age is reasonably similar to the increase of the rate of coronary and stroke events which are the main endpoints. An individual with age b i at baseline is selected for the sample with probability proportional to
Each individual is selected to the subcohort by the pre-assigned probability and such n individuals are drawn from the stratum without replacement using the Hanurav-Vijayan algorithm [ 40 , 41 ]. The selection procedure is implemented in SAS using the procedure proc surveyselect with method=pps [ 7 ]. If a fixed sample size is not a strict requirement, a more straightforward sampling procedure would be to select an individual i independently of the other individuals to the subcohort with probability p ( S i = 1). In this case the sample size is random with expectation same as n above.
For the purpose of illustration, let us consider a MORGAM cohort which included 2419 men and 2427 women so that their age-distribution was uniform over the age-group of 25–64 years. The baseline examination of this cohort took place in the year 1997 and the first follow-up ended in 2003. After the assessment of baseline and follow-up data for their quality, 2282 men and 2277 women were identified as eligible for the genetic study. In Table 1 under the column heading 2003, the number of CHD and stroke cases and the resulting subcohort sizes are given. Figure 1 shows the total number of individuals selected for genotyping. The age distributions of the cohort, the subcohort, and the CHD cases for men and women in this cohort are presented in the Figure 2 . The uniform age distribution of the cohort is seen as the nearly straight line passing through the origin while the age distribution of the subcohort is clearly different but similar to the age distribution of the CHD cases.
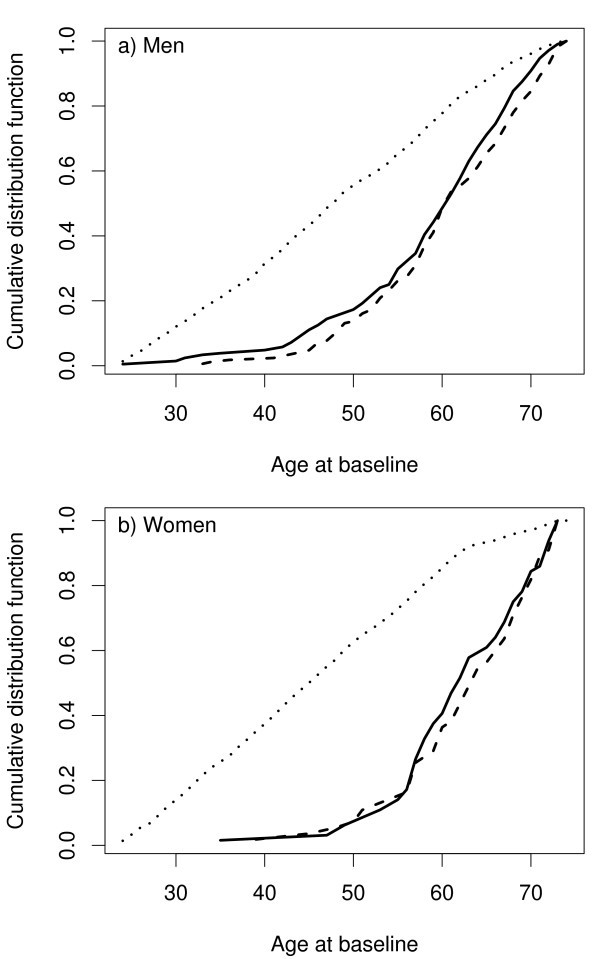
Age distribution of the cohort (dotted line), the subcohort (solid line) and the CHD cases (dashed line) in the example cohort.
3 Sampling after extension of the follow-up period
Because MORGAM is an on-going project, the MORGAM participating centres are encouraged to extend the follow-up period continually. Extension in the follow-up period results in an increase in the number of observed CHD and stroke cases and hence an increase in the desired size of the subcohort. In this section, we describe the procedure used to augment the already selected subcohort to the desired size.
As mentioned in section 2, for the subcohort sampling in MORGAM, an individual with age b i at baseline is selected for the sample with probability proportional to f ( b i ) where the function of age is obtained from the total death rate of the cohort using a logistic model. With increase in the number of deaths, there is a change in the function though the change is usually very small.
Let n 0 be the subcohort size and f 0 be the function used for sampling probabilities using the initial follow-up period. Let S 0 i be a binary random variable taking value 1 with the sampling probability and 0 otherwise. An individual i is selected in the sample with probability
Let n (> n 0 ) be the new subcohort size and f be the function of age used for sampling probabilities using the initial as well as the extended follow-up period. The selection indicator for the combined selection is denoted as S i and the target selection probability is
The question is how to augment the earlier subcohort of size n 0 with a sample of size n - n 0 so that the selection probability for an individual i is ultimately p ( S i = 1). Using simple arguments of probabilities and noting that p ( S i = 1 | S 0 i = 1) = 1, it can be seen that
which gives the closed form expression for the selection probability given that the individual was not selected in the first stage as
Note that the above probability is always less than or equal to 1 since p ( S i = 1) is always less than or equal to 1.
Let us assume that a sample of size n 0 has been selected with the ultimate selection probability for individual i as p ( S 0 i = 1). The following algorithm can be given for the enlargement of the sample
1. Obtain p ( S i = 1), i = 1, 2, ..., N using the extended follow-up data. Determine the new subcohort size n .
2. Calculate p ( S i = 1 | S 0 i = 0) using (1).
3. Select n - n 0 individuals out of N - n 0 individuals who were not selected in the first phase with probability proportional to p ( S i = 1 | S 0 i = 0). The sampling is made using the Hanurav-Vijayan algorithm that is implemented in SAS as procedure proc surveyselect with method=pps.
Because the sample size is fixed, only the proportions of the sampling probabilities can be fixed. Therefore, the sampling probabilities p ( S i = 1 | S 0 i = 0) are only approximations of the actual sampling probabilities obtained from proc surveyselect. However, our experience is that the differences between the actual sampling probabilities and the desired sampling probabilities are negligible and p ( S i = 1) can be used as the ultimate sampling probability.
The follow-up data from the cohort described in section 3 were updated by extending the follow-up to the year 2004. There was an increase in the number of CHD and stroke cases as can be seen under the column headed 2004 in Table 1 and hence, there was an increase in the subcohort size. The procedure described earlier in this section was used to select 28 individuals so as to augment the earlier subochort of size 244 and to arrive at the total size of 272 for the subcohort after the extension. Figure 3 shows the selection probabilities for the first and the second phase.
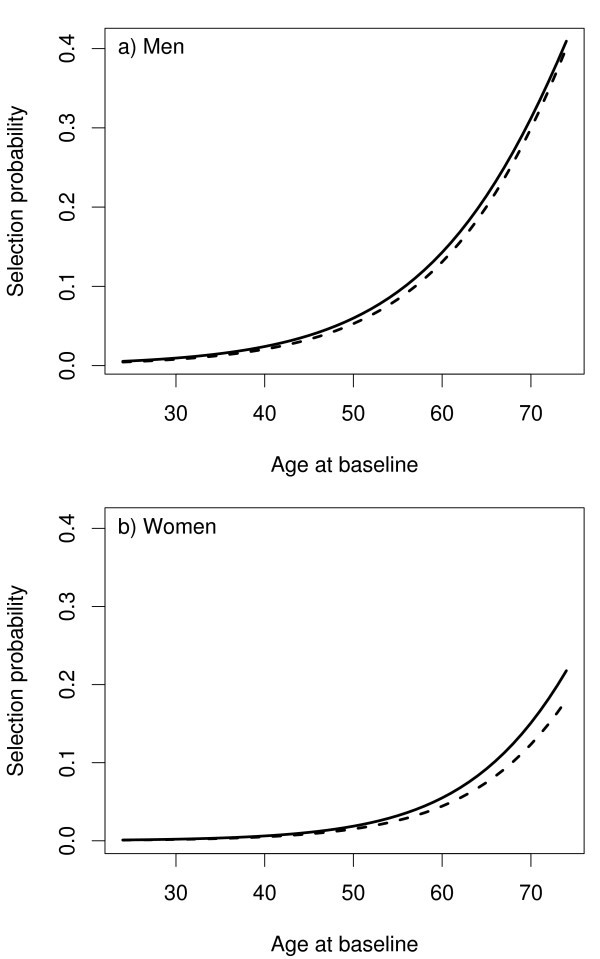
The selection probabilities p ( S i = 1) as a function of age at baseline in the first selection (dashed line) and in the second selection (solid line) of the example cohort.
4 MORGAM case-cohort design and locally designed studies
Some MORGAM centres have designed case-control or case-cohort studies for local use [ 42 ] and it may be beneficial for these centres that the MORGAM case-cohort set is selected in such a way that there is maximum overlap with the local case-control or case-cohort set. On the other hand, the case-cohort selection strategy should be similar in all MORGAM cohorts because it is important to treat the participating centres equally. In practice, the MORGAM subcohort is defined as a random sample of the cohort where the selection probabilities are defined as in sections 2.3 and 3. Thus, the goal is to select the MORGAM case-cohort set such a way that these competing objectives can be fulfilled.
Let the subcohort selected locally be described here using a binary variable S 0 i with value 1 with the local sampling probabilities p ( S 0 i = 1). For example, in a MORGAM cohort where a local study was conducted, individuals younger than 35 years at baseline were assigned sampling probability p ( S 0 i = 1) = 0, otherwise p ( S 0 i = 1) varied from 0.060 to 0.200 depending on the sex and cohort but not on the age.
Let p ( S i = 1), i = 1, 2, ..., N be the MORGAM selection probabilities. To ensure the maximum overlap, the subcohort is selected conditional on the local selection status S 0 i :
If individual i was selected to the local subcohort, select i to the MORGAM subcohort with probability
If individual i was not selected to the local subcohort, select i to the MORGAM subcohort with probability
In order to verify that sampling procedure described by equations ( 2 ) and ( 3 ) gives the required sampling probabilities p ( S i = 1) the following argumentation may be presented. Let us first suppose that p ( S i = 1) ≤ p ( S 0 i = 1). Now
which is the required selection probability. Similarly when p ( S i = 1) > p ( S 0 i = 1),
All the subjects with the conditional probabilities equal to one are selected and let n 1 be the size of such selected individuals. Following the algorithm described in section 3 and using equations ( 2 ) and ( 3 ) in Step 2 of the algorithm, n - n 1 individuals are selected.
5 Selection diagnostics and data management
MORGAM being a multi-centre study, ensuring uniformity with respect to the selection procedures and data management is a challenge. Hence, we describe briefly the assessment of the selection and data management procedures used for the case-cohort data.
The case-cohort study is designed on a cohort-to-cohort basis when the data for the cohort are complete and assessed for quality. The subcohort selection procedure described above is followed for each cohort. While computing the selection probabilities using the logistic regression model, the convergence and the values of the parameter estimators are checked and compared with the other cohorts. Typically, in the logistic regression model, the estimated coefficient for the age at baseline varies from 0.07 to 0.10 and the intercept term varies from -7.0 to -10.0 in the population cohorts. Major deviations from these values lead to further investigation. As mentioned earlier, a subcohort with a larger size needs to be selected if the follow-up data are updated with a longer follow-up. The data are again checked for their quality and it may be possible that some of the data items are altered compared to the earlier data.
The data through the process of selection get generated at different times and a well-defined protocol for data management listing the data items to be stored for future use, is required. Information on the selection is saved for each cohort including individuals not in the case-cohort set. The data on the date and the phase of the selection, eligibility for the genetic case-cohort study, selection probability, subcohort selection status and case status (one variable for each case type) are stored. We refer the reader to MORGAM manual [ 38 ] for the definitions and structure of these data items for the transfer into the database. Note that these data are essential for all the subsequent analyses. The selection is summarised in a table comprising the size of eligible cohort, the size of subcohort, the number of different cases, the number of cases in subcohort and the total number of subjects selected for genotyping.
6 Statistical analysis of case-cohort data
At the design stage of the case-cohort study, several endpoints are generally used for defining cases for which the covariate data are collected, and hence for defining the case-cohort set. This is illustrated in Figure 1 . For the purpose of statistical analysis, a case-cohort set specific to a single endpoint of interest is specified. In the following we introduce some notation to describe the analysis case-cohort set. Let S i , E i and O i be binary variables taking value one if the individual i is selected into the subcohort, is a case according to the definition used in the analysis and is a member of the case-cohort set for the present definition of a case, respectively. Let C = {1, 2, ..., N }, S = { i ∈ C : S i = 1}, E = { i ∈ C : E i = 1} and O = { i ∈ C : O i = 1} = S ∪ E denote the complete cohort, subcohort, cases and case-cohort set, respectively. Special analysis methods are needed for sets S and O because of the unequal sampling probabilities p ( S i = 1) and because the cases E are overrepresented in the case-cohort set O .
6.1 Estimation of summary statistics
When C can be considered as a representative sample of a background population, the subcohort can be used to estimate the population characteristics using the Horvitz-Thompson weighting approach [ 43 ], where the sampled subjects are weighted with the inverses of their inclusion probabilities in the sample. For example, let us consider estimation of genotypic or allelic frequencies for a biallelic SNP with alleles A and a and genotypes AA , Aa and aa . The Horvitz-Thompson estimator for population frequencies p AA , p Aa and p aa is
where π i = p ( S i = 1) and g ∈ { AA , Aa , aa }. It might also be of interest to compare the mean levels of baseline characteristics like cholesterol and blood pressure in the genotype classes. These are age-dependent characteristics and therefore weighting and possibly age-standardisation are required. Let x i be the baseline measurement of interest for individual i . An appropriate weighted estimator for a mean in the above genotypic classes is (see, for example, Särndal et al. [ 44 ], p. 185–186)
If age-standardisation is also used, π i should be interpreted accordingly. Weighted analyses should always be checked for influential observations. Because the subcohort is sampled with probabilities that increase with age, it can include young individuals with small selection probabilities and large weights. The influence of individual j in the above estimator of mean is
where set S - j is the subcohort without individual j . The influences can be plotted to detect the influential observations. They can also be used to estimate the standard error of an estimator. A jackknife variance estimator for the above estimator of the mean is given by
6.2 Analysis of time-to-event data
Survival analysis under a case-cohort design using the Cox's relative risk model can be carried out with an adjustment to the standard partial likelihood [ 3 ]. The standard expression for partial likelihood contribution for case i ∈ E in the full cohort situation would be
where Y j ( T i ) is the at risk indicator and λ j ( T i ) is the hazard rate for individual j at event time T i . This can be interpreted as the probability of event happening to individual i given an event and the risk set at time T i . Replacing here the set C with the case-cohort set O would be incorrect as the case-cohort set is enriched by cases and using set O without an adjustment would not result in correct estimates for the regression coefficients. Several weighting schemes have been proposed in the literature to adjust the partial likelihood for case-cohort situation and some of these have been summarised in Table 2 . As in Prentice [ 3 ], Kalbfleisch and Lawless [ 16 ] and Barlow [ 17 ], we refer the resulting weighted expressions as pseudolikelihood expressions. In general form, the weighted pseudolikelihood contribution for the case-cohort situation can be expressed as
where w j ( T i ) is a possibly time-dependent weight for individual j . The original weighting proposed by Prentice [ 3 ] uses unit weights for the subcohort members, while cases outside the subcohort contribute to the risk sets only at their event times, giving expression (4) the form
The weighting proposed by Barlow [ 17 ] aims to retain the original interpretation of a partial likelihood as a conditional probability. Here the subcohort members are weighted by the inverse of the sampling fraction at the event time and the sum of the weights in (4) then estimates the size of the risk set in the full cohort. Therefore this weighting scheme can also be used for estimation of absolute risks. Because of difficulty in implementing time dependent weighting, Barlow [ 18 ] used the overall sampling fraction to estimate the weights.
In the MORGAM Project the subcohort sampling probabilities are defined at individual level and are also a part of the analysis data available for investigators. Because of this it would seem natural to utilise these in the analysis. One alternative would be to weight the subcohort members with the inverses of their individual sampling probabilities, with cases outside the subcohort contributing to the risk sets only at their event times. Denoting the covariate collected for the case-cohort set as g i and other additional covariates as x i , expression (4) can now be written as
Kalbfleisch and Lawless [ 16 ] proposed a slightly different approach, where also the cases outside the subcohort contribute to the risk set with weight one and the remaining subcohort members are weighted with the inverse of subcohort sampling probability. Using the individual selection probabilities, the resulting pseudolikelihood contribution can then be written as
Both (5) and (6) approximately retain the probabilistic interpretation of Cox's partial likelihood, that is, given event and risk set at time T i they approximate the probability of event occurring to individual i .
These weighting approaches also resemble the Horvitz-Thompson method described in the previous section. The analysis approach and notation above have referred to a single endpoint of interest; for pseudolikelihood based analysis of multiple endpoints under the competing risks setting we refer to Sørensen and Andersen [ 45 ].
Usual asymptotic standard error estimates for Cox regression analysis are not valid in the case-cohort situation. A robust variance estimator for regression estimates under the case-cohort setting is proposed by Barlow [ 17 ]. This is a jackknife estimator
Pseudolikelihood based parameter estimation can be carried out using SAS procedure PHREG or R function coxph. Examples of SAS and R code for weighting alternative (5) are presented in the Appendix 1. In both codes, a weighted data set is formed first. Cases outside the subcohort are included in the risk set only from a very short time before the event and with weight one. Non-cases in the subcohort are weighted with the inverse of the subcohort sampling probability. Cases in the subcohort require two records: one censored observation for the time before event with inverse sampling probability weight and one uncensored observation from a very short time before the event with weight one. In SAS, robust standard errors can be computed by defining an ID variable identifying the subjects in the data set and specifying COVSANDWICH(AGGREGATE) in the PHREG procedure. In R, robust variance estimates are obtained by defining CLUSTER(ID) in the model equation of the coxph function. Here again ID is a variable identifying the subjects. It should be noted that in SAS versions 9.0 onwards it is possible to specify a weight variable directly while in SAS versions before this the logarithm of the weight variable has to be defined as an offset term.
7 Simulation study
Figure 2 demonstrated that the subcohort selection procedure described in section 2.3 results in similar age distributions for the subcohort and CHD cases in our example cohort. The purpose of the simulation study here is to compare this selection procedure to one where the subcohort would be selected as a simple random sample from the study cohort without adjusting for age. Also, we can compare the efficiency of the case-cohort design to a situation where all data would be collected for the complete cohort. To create a realistic simulation example, we used the endpoint and covariate data for men of our example cohort and simulated only the partially observed genetic covariate and subcohort selection. This way the results tell directly how the alternative methods would have compared to the selected method in the real situation. Following the notations introduced in the previous section, we define E i = 1 to mean CHD event during the follow-up for individual i , while E i = 0 means right censoring. T i denotes the age at the event time or right censoring. In covariates x i we included daily smoking, mean blood pressure, non-HDL cholesterol and body mass index, all of which are observed for the full cohort. Given this data, we simulated a binary covariate g i for our example cohort with fixed effect γ and population frequency π and applied different subcohort selection and estimation procedures for the resulting simulated datasets. Details of the simulation model are described in the Appendix 2. Same covariates x i as in the simulation model were also used in the case-cohort analysis.
The subcohort size was set to 208 as in the real selection (see Table 1 ). Compared to Table 1 , a wider definition of CHD endpoint was used for the analysis resulting in 107 incident CHD cases after exclusion of individuals who had cardiovascular disease already at cohort baseline from the analysis. In total the example cohort included 2074 men free of disease at the cohort baseline. The subcohort selection probabilities were defined using logistic model for total mortality in the example cohort as described in section 2.3. For comparison, subcohorts were also selected using simple random sampling without any age adjustment. Relative hazard parameters, γ for the simulated covariate and β for the fixed covariates, were estimated for each of the simulated datasets. The results for the regression coefficient γ are summarised in Table 3 . Estimates for β parameters behaved very similarly to γ and thus are not reported here. Table 3 shows, as expected, that the age adjusted subcohort selection procedure gives lower variance and higher test power compared to simple random sampling.
The simulation results suggest that the estimators of the regression coefficients have some bias away from zero that seems to increase with the true value of the parameter. We also repeated the simulations with negative values of γ (results not shown) and the bias was always away from zero. The robust variance estimator used seem to have slight negative bias which appears to be more severe in the simple random sampling situation. It is to be expected that these biases are small sample properties of the estimators and will disappear with larger sample size [ 20 ]. In MORGAM the aim is to pool case-cohort sets from several cohorts for analysis and therefore in practice the sample sizes will be larger than in the current example. All the estimation methods considered gave reasonable results. The estimators based on (5) and (6) had a near identical performance, while the Prentice estimator seemed to work better than these in terms of the observed bias in the point estimates. This observation matches the results reported recently by Onland-Moret et al. [ 20 ]. Also, the Prentice estimator gave slightly lower variance than the estimators which utilised the subcohort sampling probabilities but on the other hand the negative bias in the robust variance estimates seemed to be slightly larger in the Prentice weighting used with the MORGAM subcohort selection.
8 Discussion
The cost-effectiveness and the availability of software for the analysis make the case-cohort design appealing among epidemiologists. The literature on the case-cohort design is rich with the articles on the analysis of case-cohort data but little is written about the designing of the case-cohort studies. In this paper we have considered implementation of case-cohort design in the MORGAM Project and proposed procedures to accommodate an earlier case-cohort selection. There are several advantages of the case-cohort design in the MORGAM Project. Firstly, the case-cohort design allows the study of multiple endpoints using the same subcohort and gives more freedom for defining the endpoint of interest at the analysis stage. Secondly, because the subcohort is a random sample of the original cohort and selected without reference to any specific case definition, the subcohort can be used to estimate population parameters. Our subcohort selection procedure is general and can be applied in other situations.
In epidemiological studies age-matched controls are commonly used to improve the efficiency of the design. In MORGAM this is achieved by matching the age distribution rather than matching the individuals, as is demonstrated in Figure 2 . Another feature of the MORGAM case-cohort design is a natural extension to incorporate updated follow-up data after extending the follow-up period. Similar extension can be employed to achieve maximum overlap between the locally planned designs and the MORGAM case-cohort design. The sampling of the subcohort in MORGAM cohorts depends on the observed number of events and the mortality rate estimated from the data. Alternatively, this information could be acquired from external sources, for example, population mortality data and population event registers.
The genetic substudy of MORGAM is analysed as a prospective study even though the selection of the case-cohort set and genotyping of individuals are done retrospectively. Because the genotype information can be assumed static over time, this study avoids many potential complications that would arise if the collected covariate information depended on the time of taking the specimen. For example, no further matching is needed to ensure that the measured covariate values are compatible and the application of case-cohort design is straightforward.
When carefully planned and analysed, case-cohort designs are powerful choices for follow-up studies with multiple event types. Our experiences in designing, coordinating and analysing the MORGAM case-cohort study are potentially useful for other studies with similar characteristics. To summarise, for efficient selection of the subcohort, we recommend use of the follow-up and covariate data collected for the entire cohort. The proposed subcohort selection procedure has a natural extension to augmenting the subcohort after identification of new cases, for example due to extension of follow-up. For obtaining summary statistics based on the subcohort, it is obvious that the Horvitz-Thompson style of weighting has to be used as described in section 6.1 but otherwise the design gives the freedom to choose the suitable analysis method at the data analysis stage and we recommend that different methods should be tried and the results be compared. The likelihood based approaches will open up new avenues to the analysis of case-cohort data. The case-cohort designs and the analysis of case-cohort data continue to remain interesting research problems.
Appendix 1: program codes for case-cohort analysis of proportional hazards regression model
These codes implement pseudolikelihood analysis with inverse subcohort sampling probability weighting as defined in (5). The Prentice weighting can be applied by giving a vector of unit probabilities or not defining the weight or offset variable in the model definition.
ccregression <- function(dataset, covariates, selected, idvar,
censvar, agestart, agestop, prob, subcoh) {
# Arguments for the function are:
# dataset: R data frame; if this is created by reading a CSV file,
# missing data in that file has to coded with empty fields and
# indicator variables have to be coded as 1s and 0s.
# covariates: A vector of covariate names.
# selected: Logical expression indicating the observations to be selected into analysis.
# Following variables are entered as R expressions:
# idvar: Identification variable for the individuals.
# censvar: Case status (1=case, 0=not case); can be a logical expression.
# agestart: Variable for age at the start of the follow-up.
# agestop: Variable for age at the end of the follow-up.
# prob: Variable for subcohort selection probability.
# subcoh: Variable for inclusion in the subcohort.
attach(dataset)
dataset <- dataset[eval(selected),]
n <- nrow(dataset)
epsilon <- 0.00001
idvar <- eval(idvar)
censvar <- as.numeric(eval(censvar))
agestart <- as.numeric(eval(agestart))
agestop <- as.numeric(eval(agestop))
prob <- as.numeric(eval(prob))
subcoh <- as.numeric(eval(subcoh))
z <- matrix(NA, n, length(covariates))
for (i in 1:length(covariates))
z[,i] <- as.numeric(dataset[,names(dataset) == covariates[i]])
colnames(z) <- covariates
detach(dataset)
start <- NULL
stop <- NULL
cens <- NULL
weight <- NULL
keys <- NULL
for (i in 1:n) {
# Case outside subcohort
if ((censvar[i]) & (!subcoh[i])) {
start <- c(start, agestop[i]-epsilon)
stop <- c(stop, agestop[i])
cens <- c(cens, 1)
weight <- c(weight, 1)
keys <- c(keys, idvar[i])
}
# Non-case in subcohort
else if ((!censvar[i]) & (subcoh[i])) {
start <- c(start, agestart[i])
cens <- c(cens, 0)
weight <- c(weight, 1/prob[i])
# Case in subcohort
else if ((censvar[i]) & (subcoh[i])) {
stop <- c(stop, agestop[i]-epsilon)
}
y <- Surv(start, stop, cens)
z_ <- z[match(keys, idvar),]
return(coxph(y ~ z_ + cluster(as.factor(keys)), weights=weight))
%MACRO ccregression(dataset, covariates, selected, idvar,
case, agestart, agestop, prob, subcoh);
/*
Arguments for the macro are:
dataset: a SAS data file.
covariates: List of covariate names.
selected: Logical expression indicating the observations to be selected into analysis.
idvar: Identification variable for the individuals.
censvar: Case status (1=case, 0=not case); can be a logical expression.
agestart: Variable for age at the start of the follow-up.
agestop: Variable for age at the end of the follow-up.
prob: Variable for subcohort selection probability.
subcoh: Variable for inclusion in the subcohort.
*/
DATA casecoh; SET &dataset.(WHERE=(&selected.));
RUN;
%LET epsilon=0.00001;
DATA weighted; SET casecoh;
IF &case. THEN DO;
/* cases within the subcohort */
IF (&subcoh. NE 0) THEN DO;
start = &agestart.;
survtime= &agestop. - &epsilon.;
cens = 0;
w = 1/&prob.;
wt = log(w);
OUTPUT;
END;
/* all cases */
survtime = &agestop.;
start = survtime - &epsilon.;
cens = 1;
w = 1;
wt = log(w);
OUTPUT;
END;
/* non-cases within the subcohort */
ELSE IF (&subcoh. NE 0) AND NOT(&case.) THEN DO;
start = &agestart.;
w = 1/&prob.;
cens = 0;
PROC SORT DATA=weighted;
BY &idvar. start survtime;
/* SAS 8.1 -> */
PROC PHREG DATA=weighted COVSANDWICH(AGGREGATE);
MODEL (start,survtime)*cens(0) = &covariates. / RL OFFSET=wt;
ID &idvar.;
/* SAS 9.0 -> */
MODEL (start,survtime)*cens(0) = &covariates. / RL;
WEIGHT w;
Appendix 2: simulation details
For simulating a binary covariate with given effect γ and population frequency μ , given observed event time and covariate data, we defined a probability model for all data as
where the condition T i ≥ b i means that the analysis is restricted to subjects who are healthy at the age b i at the start of the follow-up. The survival model used is the proportional hazards Weibull regression
λ i ( t ) = ακ ( αt ) κ -1 exp( β'x i + γg i ).
The covariate distribution is defined as
Given the observed data on ( T i , E i , x i ) and parameters ( κ , α , β , γ , μ ), the binary covariate for individual i ∈ C can be sampled from conditional distribution
Fixing the regression coefficient γ and allele frequency μ , parameters β , κ and λ and covariates g i were simulated using Markov chain Monte Carlo sampling. 5000 datasets of covariates g i were produced with six different combinations of parameter values γ and μ as shown in Table 3 , and subcohort selection and parameter estimation were carried out for each of these.
Tunstall-Pedoe H, (Ed): MONICA Monograph and Multimedia Sourcebook Geneva: WHO 2003.
Google Scholar
Evans A, Salomaa V, Kulathinal S, Asplund K, Cambien F, Ferrario M, Perola M, Peltonen L, Shields D, Tunstall-Pedoe H, K Kuulasmaa for The MORGAM Project: MORGAM (an international pooling of cardiovascular cohorts). International Journal of Epidemiology 2005, 34: 21–27.
Article PubMed Google Scholar
Prentice RL: A case-cohort design for epidemiologic cohort studies and disease prevention trials. Biometrika 1986, 73: 1–11.
Article Google Scholar
Kupper LL, McMichael AJ, Spirtas R: A Hybrid Epidemiologic Study Design Useful in Estimating Relative Risk. Journal of the American Statistical Association 1975, 70: 524–528.
Miettinen O: Estimability and estimation in case-referent studies. American Journal of Epidemiology 1976, 103: 226–235.
CAS PubMed Google Scholar
Team RDC: [ http://www.R-project.org ] R: A language and environment for statistical computing Vienna, Austria: R Foundation for Statistical Computing 2006.
Inc SI: SAS/STAT User's Guide, Version 8. 2000.
Borgan Ø, Langholz B, Samuelsen SO, Goldstein L, Pogoda J: Exposure stratified case-cohort designs. Lifetime Data Analysis 2000, 6: 39–58.
Article CAS PubMed Google Scholar
Kulich M, Lin DY: Improving the Efficiency of Relative-Risk Estimation in Case-Cohort Studies. Journal of the American Statistical Association 2004, 99 (467) : 832–844.
Samuelsen SO, Nestad H, Skrondahl A: Stratified case-cohort analysis of general cohort sampling designs. Scandinavian Journal of Statistics 2007, 34: 103–119.
Kim S, Gruttola VD: Strategies for Cohort Sampling Under the Cox Proportional Hazards Model, Application to an AIDS Clinical Trial. Lifetime Data Analysis 1999, 5: 149–172.
Cai J, Zeng D: Sample size/power calculation for case-cohort studies. Biometrics 2004, 60: 1015–1024.
Kim MY, Xue X, Du Y: Approaches for calculating power for case-cohort studies. Biometrics 2006, 62: 929–933.
Cox DR: Regression models and life tables (with discussion). Journal of the Royal Statistical Society, Series B 1972, 74: 187–200.
Self SG, Prentice RL: Asymptotic Distribution theory and efficiency results for case-cohort studies. The Annals of Statistics 1988, 15: 54–81.
Kalbfleisch JD, Lawless JF: Likelihood analysis of multistate models for disease incidence and mortality. Statistics in Medicine 1988, 7: 149–160.
Barlow WE: Robust variance estimation for the case-cohort design. Biometrics 1994, 50: 1064–1072.
Barlow WE, Ichikawa L, Rosner D, Izumi S: Analysis of case-cohort designs. Journal of Clinical Epidemiology 1999, 12: 1165–1172.
Petersen L, Sørensen TIA, Andersen PK: Comparison of case-cohort estimators based on data on premature death of adult adoptees. Statistics in Medicine 2003, 22: 3795–3803.
Onland-Moret NC, van der A DL, van der Schouw YT, Buschers W, Elias SG, van Gils CH, Koerselman J, Roest M, Grobbee DE, Peeters PHM: Analysis of case-cohort data: a comparison of different methods. Journal of Clinical Epidemiology 2007, 60: 350–355.
Wacholder S: Practical considerations in choosing between the case-cohort and nested case-control designs. Epidemiology 1991, 2: 155–158.
Lin DY, Ying Z: Cox regression with incomplete covariate measurements. Journal of the American Statistical Association 1993, 88: 1341–1349.
Lin DY, Wei LJ: The robust inference for the Cox proportional hazards model. Journal of the American Statistical Association 1989, 84: 1074–1078.
Therneau TM, Li H: Computing the Cox model for case cohort designs. Lifetime Data Analysis 1999, 5: 99–112.
Langholz B, Jiao J: Computational methods for case-cohort studies. Computational Statistics & Data Analysis 2007, 51: 3737–3748.
Scheike TH, Martinussen T: Maximum likelihood estimation for Cox's regression model under case-cohort sampling. Scandinavian Journal of Statistics 2004, 31: 283–293.
Kulathinal S, Arjas E: Bayesian inference from case-cohort data with multiple end-points. Scandinavian Journal of Statistics 2006, 33: 25–36.
Saarela O, Kulathinal S: Conditional likelihood inference in a case-cohort design: An application to haplotype analysis. [ http://www.bepress.com/ijb/vol3/iss1/1 ] The International Journal of Biostatistics 2007., 3:
Langholz B, Thomas DC: Nested case-control and case-cohort methods of sampling from a cohort: a critical comparison. American Journal of Epidemiology 1990, 131: 169–176.
Langholz B, Duncan DC: Efficiency of cohort sampling designs: some suprising results. Biometrics 1991, 47: 1563–1571.
Zhao LP, Lipsitz S: Designs and analysis of two-stage studies. Statistics in Medicine 1992, 11: 769–782.
Chen K, Lo S: Case-cohort and case-control analysis with Cox's model. Biometrika 1999, 85: 755–754.
Chen K: Generalised case-cohort sampling. Journal of the Royal Statistical Society, Series B 2001, 63: 823–838.
Paszata LF, Vallisb KA, Benka VMA, Groomec PA, Mackillopc WJ, Wielgosz A: A population-based case-cohort study of the risk of myocardial infarction following radiation therapy for breast cancer. Radiotherapy and Oncology 2007, 82 (3) : 294–300.
Friesema IHM, Zwietering PJ, Veenstra MY, Knottnerus JA, Garretsen HFL, Lemmens PHHM: Alcohol intake and cardiovascular disease and mortality: the role of pre-existing disease. Journal of Epidemiology and Community Health 2007, 61: 441–446.
Bernatsky S, Boivin JF, Joseph L, Gordon C, Urowitz M, Gladman D, Ginzler E, Fortin P, Bae SC, Barr S, Isenberg D, Rahman A, Petri M, Alarcón G, Aranow C, Dooley MA, Rajan R, Sénécal JL, Zummer M, Manzi S, Edworthy S, Ramsey-Goldman R, Clarke A: The relationship between cancer and medication exposures in systemic lupus erythematosus: a case-cohort study. Annals of the rheumatic diseases 2007. doi:101136/ard.2006.069039
Ray RM, Gao DL, Li W, Wernli KJ, Astrakianakis G, Seixas NS, Camp JE, Fitzgibbons ED, Feng Z, Thomas DB, Checkoway H: Occupational exposures and breast cancer among women textile workers in Shanghai. Epidemiology 2007, 8 (3) : 383–392.
Project M: MORGAM Manual. [ http://www.ktl.fi/publications/morgam/manual/contents.htm ]
Ury HK: Efficiency of case-control studies with multiple controls per case: continuos and dichotomous data. Biometrics 1975, 31: 643–649.
Hanurav TV: Optimum utilization of auxiliary information: π ps sampling of two units from a stratum. Journal of the Royal Statistical Society, Series B 1967, 29: 374–391.
Vijayan K: An exact π ps sampling scheme: generalization of a method of Hanurav. Journal of the Royal Statistical Society, Series B 1968, 30: 556–566.
Kulathinal S, Niemelä M, Kuulasmaa K: contributors from Participating Centres for the MORGAM Project Description of MORGAM cohorts. [ http://www.ktl.fi/publications/morgam/cohorts/index.html ]
Horvitz DG, Thompson DJ: A generalization of sampling without replacement from a finite universe. Journal of the American Statistical Association 1952, 47: 663–685.
Särndal CE, Swensson B, Wretman J: Model assisted survey sampling New York: Springer-Verlag 1992.
Sørensen P, Anderson PK: Competing risks analysis of the case-cohort design. Biometrika 2000, 87: 49–59.
Download references
Acknowledgements
The research was supported by the European Commission by the MORGAM Project grant under the Biomed 2 programme and by the GenomEUtwin Project grant under the programme 'Quality of Life and Management of the Living Resources' of 5th Framework Programme and by the Academy of Finland via its grant number 53646. The first author was a visiting researcher at the Department of Mathematics and Statistics, University of Helsinki and her research was supported by the Academy of Finland via its grant number 114786.
The participants of the MORGAM Project contributed to and made decision on the study design. MORGAM Participating Centres and Principal Investigators: Australia, Newcastle, (Patrick McElduff, Faculty of Health, University of Newcastle; Former Principal Investigator: Annette Dobson, University of Queensland, Brisbane); Denmark, Glostrup (Torben Jørgensen, Københavns AMT, Centre of Preventive Medicine, Glostrup); Finland, FINRISK (Veikko Salomaa, National Public Health Institute, Helsinki); Finland, ATBC (Jarmo Virtamo, National Public Health Institute, Helsinki); France, PRIME/Strasbourg (Dominique Arveiler, Department of Epidemiology and Public Health, Faculty of Medicine, Strasbourg); France, PRIME/Toulouse (Jean Ferrières, Department of Epidemiology, Faculty of Medicine, Toulouse-Purpan, Toulouse); France, PRIME/Lille, (Philippe Amouyel, Department of Epidemiology and Public Health, Pasteur Institute of Lille); Germany, Augsburg (Angela Döring, GSF-National Research Centre for Environment and Health, Institute of Epidemiology, Neuherberg); Italy, Brianza, (Giancarlo Cesana and Marco Ferrario, University of Milan – Bicocca, Monza); Italy, Friuli (Diego Vanuzzo and Lorenza Pilotto, Centre for Cardiovascular Prevention, ASS4 "Medio Friuli", Udine); Italy, Rome, (Simona Giampaoli and Luigi Palmieri, National Institute of Health, Rome); Lithuania, Kaunas, (Abdonas Tamosiunas, Former Principal Investigator: Stanislava Domarkiene, Kaunas University of Medicine, Institute of Cardiology, Kaunas); Poland, Krakow (Andrzej Pajak, Unit of Clinical Epidemiology and Population Studies, School of Public Health, Jagiellonian University, Krakow); Poland, Warsaw, (Grazyna Broda, Department of Cardiovascular Epidemiology and Prevention, National Institute of Cardiology, Warsaw); Russian Federation, Novosibirsk, (Yuri Nikitin, Institute of Internal Medicine, Novosibirsk); Sweden, Northern Sweden, (Birgitta Stegmayr, Umeå University Hospital, Department of Medicine, Umeå); United Kingdom, PRIME/Belfast, (Alun Evans, The Queen's University of Belfast, Belfast, Northern Ireland); United Kingdom, Scotland (Hugh Tunstall-Pedoe, University of Dundee, Dundee, Scotland); United Kingdom, Caerphilly, (John Yarnell, The Queen's University of Belfast, Belfast, Northern Ireland). National Coordinators: France, PRIME/France: Pierre Ducimetière, National Institute of Health and Medical Research (U258), Paris; Italy: Marco Ferrario, University of Insubria, Varese, Italy. Coordinating Centre: Alun Evans, Department of Epidemiology and Public Health, The Queen's University of Belfast, Belfast, Northern Ireland. MORGAM Data Centre: Kari Kuulasmaa, Sangita Kulathinal, Juha Karvanen, Olli Saarela, Department of Health Promotion and Chronic Disease Prevention, National Public Health Institute (KTL), Helsinki, Finland. Participating Laboratories: Markus Perola, Department of Molecular Medicine, National Public Health Institute (KTL), Helsinki, Finland; François Cambien, Laurence Tiret, INSERM U525, Paris, France; Denis Shields, Royal College of Surgeons in Ireland, Dublin, Ireland. MORGAM Management Group: Alun Evans, Department of Epidemiology and Public Health, The Queen's University of Belfast, Northern Ireland; Stefan Blankenberg, Department of Medicine II, Johannes Gutenberg-University, Mainz, Germany; François Cambien, INSERM U525, Paris, France; Marco Ferrario, University of Insubria, Varese, Italy; Kari Kuulasmaa, MORGAM Data Centre, National Public Health Institute, Finland; Leena Peltonen, Department of Molecular Medicine, National Public Health Institute, Finland; Markus Perola, Department of Molecular Medicine, National Public Health Institute, Finland; Veikko Salomaa, Department of Health Promotion and Chronic Disease Prevention, National Public Health Institute, Finland; Denis Shields, Clinical Pharmacology, Royal College of Surgeons in Ireland, Dublin, Ireland; Birgitta Stegmayr, University of Umeå, Sweden; Hugh Tunstall-Pedoe, Cardiovascular Epidemiology Unit, University of Dundee, Scotland; Kjell Asplund, The National Board of Health and Welfare, Stockholm, Sweden.
Author information
Authors and affiliations.
Indic Society for Education and Development, , 1, Swami Enterprises Complex. Tigrania road, Tapovan Bridge, Nashik, 422 009, India
Sangita Kulathinal
Department of Health Promotion and Chronic Disease Prevention, National Public Health Institute, Mannerheimintie 166, 00300, Helsinki, Finland
Juha Karvanen, Olli Saarela & Kari Kuulasmaa
You can also search for this author in PubMed Google Scholar
Corresponding author
Correspondence to Juha Karvanen .
Additional information
Competing interests.
The author(s) declare that they have no competing interests.
Rights and permissions
Open Access This article is published under license to BioMed Central Ltd. This is an Open Access article is distributed under the terms of the Creative Commons Attribution License ( https://creativecommons.org/licenses/by/2.0 ), which permits unrestricted use, distribution, and reproduction in any medium, provided the original work is properly cited.
Reprints and permissions
About this article
Cite this article.
Kulathinal, S., Karvanen, J., Saarela, O. et al. Case-cohort design in practice – experiences from the MORGAM Project. Epidemiol Perspect Innov 4 , 15 (2007). https://doi.org/10.1186/1742-5573-4-15
Download citation
Received : 13 November 2006
Accepted : 04 December 2007
Published : 04 December 2007
DOI : https://doi.org/10.1186/1742-5573-4-15
Share this article
Anyone you share the following link with will be able to read this content:
Sorry, a shareable link is not currently available for this article.
Provided by the Springer Nature SharedIt content-sharing initiative
Epidemiologic Perspectives & Innovations
ISSN: 1742-5573
- Search Menu
- Advance articles
- Editor's Choice
- 100 years of the AJE
- Collections
- Author Guidelines
- Submission Site
- Open Access Options
- About American Journal of Epidemiology
- About the Johns Hopkins Bloomberg School of Public Health
- Journals Career Network
- Editorial Board
- Advertising and Corporate Services
- Self-Archiving Policy
- Dispatch Dates
- Journals on Oxford Academic
- Books on Oxford Academic
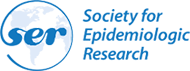
Article Contents
Background and motivation, two-phase studies and weighted analyses, discussion and conclusions.
- < Previous
Using the Whole Cohort in the Analysis of Case-Cohort Data
- Article contents
- Figures & tables
- Supplementary Data
Norman E. Breslow, Thomas Lumley, Christie M. Ballantyne, Lloyd E. Chambless, Michal Kulich, Using the Whole Cohort in the Analysis of Case-Cohort Data, American Journal of Epidemiology , Volume 169, Issue 11, 1 June 2009, Pages 1398–1405, https://doi.org/10.1093/aje/kwp055
- Permissions Icon Permissions
Case-cohort data analyses often ignore valuable information on cohort members not sampled as cases or controls. The Atherosclerosis Risk in Communities (ARIC) study investigators, for example, typically report data for just the 10%–15% of subjects sampled for substudies of their cohort of 15,972 participants. Remaining subjects contribute to stratified sampling weights only. Analysis methods implemented in the freely available R statistical system ( http://cran.r-project.org/ ) make better use of the data through adjustment of the sampling weights via calibration or estimation. By reanalyzing data from an ARIC study of coronary heart disease and simulations based on data from the National Wilms Tumor Study, the authors demonstrate that such adjustment can dramatically improve the precision of hazard ratios estimated for baseline covariates known for all subjects. Adjustment can also improve precision for partially missing covariates, those known for substudy participants only, when their values may be imputed with reasonable accuracy for the remaining cohort members. Links are provided to software, data sets, and tutorials showing in detail the steps needed to carry out the adjusted analyses. Epidemiologists are encouraged to consider use of these methods to enhance the accuracy of results reported from case-cohort analyses.
One of the principal justifications for large cohort studies is the ability to conduct substudies on selected participants so that expensive covariates need not be ascertained for everyone. The nested case-control study ( 1 ), in which individually matched controls are sampled from case risk sets, is the oldest and most widely used design for collection of additional covariates to estimate hazard rates and ratios in the context of Cox regression ( 2 ). The case-cohort design ( 3–5 ), in which controls are sampled without regard to failure times as part of a “subcohort” (cohort random sample), has become more popular as its advantages have become better known. For example, the single subcohort may be used to estimate population frequencies of covariates (e.g., genotypes), to select controls for multiple failure time outcomes (e.g., diagnoses of diabetes and heart disease), and to conduct analyses by using multiple time scales (e.g., time-on-study and attained age). Sometimes, the nested case-control design is infeasible because the vital status of cohort members needed for risk set construction is unknown prior to their selection into a potential subcohort ( 6 ).
Published analyses of case-cohort studies routinely fail to utilize all available data. The original analysis method ( 5 ) does not accommodate case sampling or stratified sampling of controls and makes inefficient use of cases not in the subcohort. Hence, most analyses today utilize the “robust” approach of Barlow et al. ( 7 , 8 ). This approach involves Cox regression, with case and control observations weighted by their inverse sampling probabilities ( 9 ). A major drawback to both approaches is that they ignore information on cohort members not sampled as cases or controls. Survey statisticians ( 10 ) and biostatisticians ( 11 ) have each proposed methods for recovery of this information by adjusting the sampling weights. These methods are now implemented in the freely available R statistical system ( http://cran.r-project.org/ ) in the NestedCohort package of Mark and Katki ( 12 ) and the survey package of Lumley ( 13 ). Both packages accommodate stratified random sampling of cases and controls on the basis, for example, of rare covariate patterns ( 14 ).
In this paper, our goal is to demonstrate important strengths as well as limitations of these newly available tools. We compare results obtained by using adjusted weights with those obtained with standard weights in a reanalysis of data from a published case-cohort study and in analyses of simulated case-cohort samples.
The Atherosclerosis Risk in Communities (ARIC) study ( 15 ) often uses case-cohort methodology. The cohort consists of 15,972 participants under active follow-up since 1987–1989 for atherosclerosis and its clinical sequelae. Using samples of stored biologic tissue, ARIC investigators studied candidate genotypes ( 16–18 ) and biomarkers of inflammation ( 19 , 20 ) as possible risk factors for coronary heart disease and related endpoints. Ballantyne et al. ( 20 ) identified 12,819 ARIC participants who were free from coronary heart disease and had plasma samples taken at their second follow-up visit (1990–1992). Stored plasma for participants who developed incident coronary heart disease prior to 1999, or who were selected in a cohort random sample, was assayed for levels of lipoprotein-associated phospholipase A 2 (Lp-PLA 2 ) and C-reactive protein. Cohort sampling was stratified into 8 strata based on age, sex, and ethnicity. After exclusions because of missing data, 608 cases and 740 noncases remained for estimation of hazard ratios for coronary heart disease in tertiles of Lp-PLA 2 and C-reactive protein using a weighted Cox regression analysis appropriate for stratified case-cohort studies ( 7 , 14 ).
As do many epidemiologists, ARIC investigators ( 16–20 ) ignored most of their data. Apart from known sampling fractions, their analyses involved only those cases or controls sampled as part of the substudy. Since cases were deliberately overrepresented, the substudy included many of the most informative subjects. Nonetheless, important variables in the regression models were ignored for nearly 90% of the cohort. The Ballantyne et al. study ( 20 ) ignored data on smoking history, low density lipoprotein and high density lipoprotein cholesterol, and diabetes, all of which were used for secondary adjustment of the hazard ratios for Lp-PLA 2 and C-reactive protein. Other data items were ignored that, although not used in the regression, were correlated with biomarkers measured for sampled participants and hence provided potentially valuable information about them. Through reanalysis of the Ballantyne et al. data, we demonstrate in the sequel how main cohort data may be incorporated into the analysis to improve precision of regression coefficients.
Survey statisticians recognize the case-cohort study as a 2-phase, stratified sampling design. The first-phase sample is the cohort itself, considered a sample from some target population. The second-phase sample, stratified by using information from phase 1, consists of cases and controls in the subcohort. We first describe 2-phase designs and summarize some statistical properties of weighted estimates. Next, we report reanalyses of the Ballantyne et al. ( 20 ) case-cohort data. Finally, we report results of analyses of simulated case-cohort data from the National Wilms Tumor Study (NWTS) ( 21 , 22 ). The NWTS data, R code for the survey package, and related tutorials are available online ( http://faculty.washington.edu/norm/IEA08.html ).
Two-phase stratified sampling
Suppose the N subjects in the cohort (phase 1 sample) are classified into K strata on the basis of information known for everyone and that the numbers N k of subjects in each stratum are determined ( N = N 1 + N 2 + ··· + N K ). For the substudy (phase 2 sample), n k ≤ N k subjects are sampled at random without replacement (no subject is sampled more than once) from the k th stratum, with the sampling from each stratum conducted independently. The total number of subjects sampled at phase 2, for whom biologic material is analyzed or additional information otherwise obtained, is n = n 1 + n 2 + · · · + n K . Associated with each subject is a sampling weight N k / n k depending on only the subject's stratum. In a weighted analysis, the contribution from a sampled subject is up-weighted so the total contribution from each stratum is representative of the total contribution assuming all cohort members from that stratum had been analyzed.
Table 1 illustrates the design using the ARIC data. The slight differences in totals from those reported previously ( 20 ) arise because some participants, including 9 in the original substudy, had not given proper consent. A few more, including 3 in the original substudy, lacked information on body mass index. This factor was the most important predictor of C-reactive protein and hence a key auxiliary variable. After exclusions for missing values of baseline variables for main cohort subjects, and for missing biomarker variables at phase 2, N = 12,345 remained in the main cohort and n = 1,336 remained at phase 2, including 604 coronary heart disease cases. Sampling of the original subcohort had been stratified on sex, race, and age. The cases were treated as an additional, ninth stratum ( K = 9) in our analyses. Table 1 shows the distribution of cohort and sampled subjects over the strata, with the standard sampling weights in the last row. Since they are based on observed sampling fractions, the weights are slightly different and more accurate than those used previously ( 20 ). The weight of 1.2 for cases illustrates the importance of being able to handle sampling of both cases and controls in case-cohort analyses ( 23 ).
Stratified Sampling Design for the Atherosclerosis Risk in Communities Study
Abbreviation: CHD, coronary heart disease.
Weighted estimates and their sampling properties
Improving precision.
Survey statisticians adjust the weights to reduce the phase 2 variance when auxiliary variables V , correlated with variables in the regression model, are available for all subjects. The simplest method, poststratification, replaces the K sampling strata with a finer stratification incorporating the auxiliary information. In an ARIC case-cohort study of glutathione- S -transferase genotypes as a susceptibility factor in smoking-related coronary heart disease, smoking data were ignored for all but 10% of subjects even though smoking was a risk factor of primary interest ( 16 ). Poststratification on smoking history would have improved the analysis. Poststratified analyses of simulated case-control data have been reported for the NWTS cohort ( 24 ).
Calibration ( 25 , 26 ) adjusts the weights to be as close as possible to the sampling weights subject to the constraint that the cohort total of V is equal to its weighted sum among sampled subjects. Estimation ( 11 ) uses as weights the reciprocals of inclusion probabilities estimated from a logistic regression model that predicts which cohort subjects are sampled at phase 2. Here, the requirement is that the observed total of V in the sample equals the predicted total: the sum over the cohort of V multiplied by the estimated sampling probability. It is important to include the sampling strata as a factor (“dummy” variables) in the logistic model to account for the bias in the phase 2 sample. If dummy variables corresponding to the original or finer (poststratified) strata are the only auxiliary variables, calibrated and estimated weights are identical, being equal to inverse sampling fractions for each stratum. Adjusted weights increase precision through their dependence on the auxiliary information available for all cohort subjects.
As described in a companion paper for statisticians ( 27 ), Cox regression coefficients obtained by using calibrated and estimated weights have very similar theoretical properties. Both are consistent and asymptotically normal. Depending on the choice of auxiliary variables, both can attain minimum variance in the class of “augmented” inverse probability weighted estimates ( 11 , 12 ). To approximate the optimum choice of auxiliary variables, we adopted the “plug-in” approach of Kulich and Lin ( 28 ). It requires separate models for prediction of the values of each partially missing variable (ascertained for phase 2 subjects only) and is likely of greatest use when there are only 1 or 2 such variables. The method has 4 steps:
Develop weighted regression models from the phase 2 data for prediction of the partially missing variables from information available for all subjects. (For the Ballantyne et al. study ( 20 ), this means prediction of Lp-PLA 2 and C-reactive protein.)
Use the prediction equations to impute values of the partially missing variables for all cohort subjects.
Using imputed values for the partially missing variables and known values for other variables, fit the Cox model to the whole cohort and determine the imputed delta-beta (estimated influence function contribution obtained as a residual in the R coxph program) for each cohort subject.
Use the imputed delta-betas as auxiliary variables in calibration or estimation of the weights, and estimate β by weighted Cox regression analysis of the phase 2 data.
As demonstrated below, adjustment by calibration or estimation has the potential to reduce the phase 2 variances for some regression coefficients to negligible levels. The variances for others are left virtually unchanged.
Reanalysis of ARIC data
Similar procedures were followed and similar results obtained for the separate analyses of C-reactive protein and Lp-PLA 2 . Following the 4 steps just described, we first predicted Lp-PLA 2 by using linear regression on white race, male sex, low density lipoprotein cholesterol, high density lipoprotein cholesterol, systolic and diastolic blood pressures, and the sex × race interaction (coefficients not shown). The prediction was not very successful, with R 2 = 0.28 ( Figure 1 ). Nonetheless, it was used to impute Lp-PLA 2 (step 2) and thus to calculate auxiliary variables (step 3) used for adjustment of weights.

Scatter plot and nonparametric regression curve showing predicted values of lipoprotein-phospholipase A 2 (μg/L) plotted against measured values. Predicted values are based on weighted linear regression from phase 2 data (the Atherosclerosis Risk in Communities case-cohort study).
Results are shown in Table 2 . Variances for each regression coefficient are obtained by summing the squares of the phase 1 and phase 2 standard errors. Hazard ratios and 95% confidence intervals for the middle and upper tertiles of Lp-PLA 2 relative to the lowest were 1.05 (95% confidence interval: 0.76, 1.46) and 1.18 (95% confidence interval: 0.85, 1.64), respectively, when estimated by using standard weights. The corresponding estimates reported by ARIC—in model 2, Table 4 of Ballantyne et al. ( 20 )—were 1.02 (95% confidence interval: 0.73, 1.43) and 1.16 (95% confidence interval: 0.85, 1.65). In spite of differences in data sets and the fact that ARIC used slightly different sampling weights and a slightly different method of variance estimation ( 7 ), the results of the reanalysis were close to the original, particularly with regard to precision as measured by widths of the confidence intervals.
Results of Reanalysis of Data From a Case-Cohort Study of Lp-PLA 2 : the Atherosclerosis Risk in Communities Study a
Abbreviations: Coef, regression coefficient; HDL-C, high density lipoprotein cholesterol (mg/L); LDL-C, low density lipoprotein cholesterol (mg/L); Lp-PLA 2 0.310– and 0.422–, approximate middle and upper tertiles, respectively, of lipoprotein-associated phospholipase A 2 (μg/L); SBP, systolic blood pressure (mm Hg); SE 1 , phase 1 standard error; SE 2 , phase 2 standard error.
N = 12,345; n = 1,336 including 604 coronary heart disease cases.
When standard weights were used, the contribution of phase 2 sampling to the overall variance exceeded the phase 1 contribution for all but 1 coefficient. For the adjustment covariates known for all, both calibration and estimation reduced the estimated phase 2 standard error dramatically, calibration consistently more so. The overall standard errors were very similar to the estimates (phase 1 standard error) if complete data had been available for all subjects. For the tertiles of Lp-PLA 2 , however, there was virtually no change; in fact, both adjustment methods resulted in very slight increases in the phase 2 standard error. The phase 1 standard errors were nearly identical for the 3 weighting schemes, reflecting the fact that they all represent variability in the unobserved β ~ N .
Results for C-reactive protein (not shown) were similar, with R 2 = 0.21. The increase in precision by adjustment of the weights was again confined to coefficients of baseline covariates.
To investigate possible improvement in precision when studying the interaction between a partially missing covariate and 1 available for everyone, we searched for baseline covariates that exhibited an interaction with Lp-PLA 2 . Table 3 reports findings for a model having a grouped linear × linear interaction with systolic blood pressure. When standard weights were used, the hazard ratios estimated separately for the middle and upper tertiles of Lp-PLA 2 relative to the lowest, for subjects with average systolic blood pressure, were exp(0.137) = 1.15 (95% confidence interval: 0.81, 1.62) and exp(0.303) = 1.35 (95% confidence interval: 0.95, 1.92), respectively. This finding was consistent with a grouped linear model having a hazard ratio of approximately 1.156 per tertile. The interaction coefficient suggested that the per-tertile hazard ratio decreased by a factor of exp(−0.0672) = 0.935 for each 10-mm Hg increase in systolic blood pressure. Although of clinically important magnitude, this decrease was not statistically significant ( Z = −1.89, P = 0.062).
Results of Reanalysis of Data From a Case-Cohort Study of Lp-PLA 2 : Interaction With SBP
Abbreviations: Coef, regression coefficient; Lp-PLA 2 0.310– and 0.422–, approximate middle and upper tertiles, respectively, of lipoprotein-associated phospholipase A 2 (μg/L); SBP, systolic blood pressure (mm Hg); SE 1 , phase 1 standard error; SE 2 , phase 2 standard error.
The covariates age in years/10, male sex, white race, former smoker, never smoked, SBP/100, low density lipoprotein cholesterol/100, high density lipoprotein cholesterol/100, and diabetes were also included in the model, but results for only Lp-PLA 2 and its interaction with SBP are shown. The interaction term used “grouped linear” values of 1, 2, 3 for the 3 tertiles of Lp-PLA 2 and centered SBP (in units of 100 mm Hg) at its mean value.
Calibration and estimation of the weights reduced the phase 2 standard errors of the adjustment covariates (not shown) and left effectively unchanged those for the main effects of Lp-PLA 2 ( Table 3 ), just as observed for the no-interaction model. There was, however, a reduction of about 10% in the phase 2 standard error of the interaction coefficient. This reduction led to changes in the associated test statistics, Z = −2.10, P = 0.036 for calibration and Z = −2.02, P = 0.043 for estimation, both now significant. Because systolic blood pressure was selected from among several covariates examined for interaction effects, it would be imprudent to draw substantive conclusions from this reanalysis. It serves primarily to illustrate the potential for improvement in precision of interaction coefficients, even when there is none for the corresponding main effects.
Simulated case-cohort data
The NWTS cohort consisted of 3,915 patients with Wilms tumor diagnosed during 1980–1994 and followed until the earliest of disease progression or death for “event-free survival.” Baseline covariates available for all patients from the registering institutions included “favorable” vs. “unfavorable” histology, stage of disease (I–IV), age at diagnosis, and tumor diameter. Histology evaluated by the central reference laboratory was also available for everyone, which allowed repeated drawing of stratified phase 2 samples in which central histology was treated as known for sampled subjects only. Since the normally unobservable β ~ N was available, the phase 2 variance could be determined empirically. Institutional histology was strongly related to central histology: of 439 unfavorable history tumors (central laboratory), 324 were classified unfavorable history by the patient's institution, for a sensitivity of 74%; 3,418 of 3,476 favorable histology tumors were correctly classified, for a specificity of 98%.
Sixteen strata were formed on the basis of event-free survival, stage, institutional histology, and age (2 groups each). All subjects were sampled from the 13 smallest strata: all cases, all institutional unfavorable history, and all patients less than 1 year of age with stage III–IV disease ( Table 4 ). Since the 13 strata all had a sampling weight of 1, they could be collapsed into a single analysis stratum with no effect on the results. Random samples of sizes 120, 160, and 120 were selected from the 3 largest strata to yield a phase 2 sample consisting of all 669 cases and 660 sampled controls. Kulich and Lin ( 28 ) used nearly the same sampling scheme with the NWTS data to evaluate their “combined, doubly weighted” estimate for the same problem. Their sample sizes varied, with expectations of 120, 160, and 120 for the 3 sampled strata, which would be expected to decrease precision very slightly in comparison with fixed sample sizes.
Stratified Sampling Design for the National Wilms Tumor Study
Age in years at diagnosis of Wilms tumor.
Ten thousand stratified phase 2 samples were drawn in this fashion. For each, we estimated Cox regression coefficients by using standard weights, calibrated weights, and estimated weights following the 4-step procedure. The Cox and imputation models, which used different variable codings to achieve the best fit for their distinct purposes, were again those of Kulich and Lin ( 28 ). The Cox model included central histology, age as a piecewise linear variable with change point at 1 year, stage (III–IV vs. I–II), diameter, and the interactions histology × age and stage × diameter. Central histology (unfavorable history) was imputed by using institutional histology, stage (IV vs. I–III), age (>10 years vs. ≤10 years), tumor diameter (linear), and the interaction histology × stage in the logistic regression equation. The R 2 value between true and predicted unfavorable history was 0.59. Imputed delta-betas, augmented by addition of 1 for numerical reasons, served as auxiliary variables for calibration. For estimation, delta-betas multiplied by sampling weights served as auxiliaries.
Results From 10,000 Simulated Phase 2 Samples From the National Wilms Tumor Study
Abbreviations: Age 0 and Age 1 , piecewise linear terms for age at diagnosis (years) before and after 1 year, respectively; ASE, average (total) standard error; diameter, diameter (cm) of the excised tumor; SE 1 , robust phase 1 standard error; SMSE, square root of mean squared phase 2 error; stage: binary indicator of stage III–IV disease.
Results agreed well with the sampling properties outlined above. Consider, for example, the standard errors for unfavorable history shown in the first row of Table 5 . The total variance estimated by using standard weights, 0.537 2 = 0.288, was approximately equal to the sum of the phase 1 and phase 2 components, 0.503 2 + 0.192 2 = 0.290. Since this relation holds only in expectation and in large samples, of course, not all table entries exhibit it so closely.
Calibration and estimation both improved precision. Gains were greatest for covariates known for all: age, stage, and tumor diameter. Ratios of standard to adjusted square root of the mean squared error for the 5 model terms involving these covariates alone ranged from 3.0 to 4.4 (median = 3.5) for calibration and from 1.7 to 2.7 (median = 2.3) for estimation. In several instances, the phase 2 variance was negligible in comparison with phase 1. Substantial gains were also achieved for the unfavorable history main effect, whose phase 2 standard error was reduced by 29%, and more modest gains for the interaction effect of unfavorable history with the initial slope of age. The phase 2 standard error for the interaction of unfavorable history with age beyond 1 year was effectively unchanged, but the lack of change matters little in view of the small, statistically insignificant coefficient. Overall performance using calibrated and estimated weights was quite comparable to that of the “combined, doubly weighted” estimate shown in Table 3 of Kulich and Lin ( 28 ).
Substantial gains were observed from calibration and estimation of the sampling weights in the simulated NWTS case-cohort studies. While most pronounced for baseline covariates known for all, important gains were also observed for the main effect and an interaction involving unfavorable history. These gains were possible because there was a strong surrogate, institutional histology, for the partially missing variable. By reducing the number of slides sent to the central reference laboratory from 3,915 to 1,329, and thereby lowering costs, the investigators could in principle have estimated the hazard ratios of interest with little loss of precision. (Central histology was essential, of course, for many other purposes.)
Comparable gains were observed for only baseline covariates upon reanalysis of the ARIC case-cohort data, most likely because of lack of good predictors for Lp-PLA 2 and C-reactive protein. Our limited experience in other contexts indicates that R 2 for prediction of the partially missing variable should be at least 0.5 to substantially improve precision of the corresponding regression coefficient. Modest, but important gains were evident, however, for the linear interaction of Lp-PLA 2 with systolic blood pressure. This finding suggests that the methodology might usefully be applied to the ARIC case-cohort study of glutathione- S -transferase and smoking ( 16 ) and to other studies of genotype-environment interaction in which the environmental factor is known for everyone. Even if there is no obvious improvement in precision of estimation of principal risk factors, the knowledge that they have made more complete use of the available information should give epidemiologists greater confidence in their results.
Our simulations demonstrated that efficiency gains from weighted Cox regression with calibrated or estimated weights were similar to those found with the more complicated estimate of Kulich and Lin ( 28 ). It too was designed to achieve near optimality in the class of augmented inverse probability weighted estimates. Theoretically, the best choice for auxiliary variables would be conditional expectations, given the phase 1 data, of influence function contributions for the Cox model ( 11 ). We approximated these unknown quantities by using imputation, as described in the 4-step procedure. Further numerical work involving alternative choices for auxiliary variables, and further practical comparisons of calibration and estimation, are warranted.
The goal of our case-cohort analyses was to approximate as closely as possible results that we would have obtained had we been able to fit the standard (unweighted) Cox model to complete data for the entire cohort. Such results are usually expressed as point and interval estimates of model parameters under the assumption that the cohort is a simple random sample from a target population described by the model. In fact, the ARIC cohort was constructed by survey sampling of approximately 4,000 adults 45–64 years of age from each of 4 US communities. The target population is best viewed as a hypothetical population comprising a large mix of subjects “like those” in the 4 communities ( 31 ). If results differed systematically between communities, the appeal of generalizing to this target would be lessened.
We considered Cox regression modeling of stratified case-cohort data. The principle of increasing precision through adjustment of sampling weights applies much more generally. The R survey package accommodates a variety of analyses of data from 2-phase stratified samples including estimation and log-linear modeling of population frequencies in contingency tables and estimation of regression coefficients in generalized linear models. Adjustment of sampling weights using auxiliary variables enhances precision in these analyses. The NestedCohort package is restricted to Cox regression and adjustment by estimation. However, it provides estimates of the baseline (cumulative) hazard function and thus of failure probabilities, which are important in many applications ( 12 ).
Stratified case-cohort studies involve data missing by design. Sometimes, as for biomarkers in the ARIC study, phase 2 data are also missing by chance ( 12 ). The methods proposed here assume that, within each stratum, the phase 2 subjects with complete data still constitute a random sample from the cohort. This assumption may be relaxed by adding variables to the logistic model used to predict which subjects are sampled for phase 2 and have complete data. Of course, one can never be certain that the probability of having complete data does not further depend on the missing values themselves, so the possibility of bias remains when data are missing by chance.
Stratified case-cohort studies based on large cohorts are increasingly common designs in epidemiology. Analyses to date have largely ignored relevant information available for the parent cohort. Improvements in statistical methodology described here, and their implementation in the freely available R software system, can help prevent this waste of valuable information. We have demonstrated that adjustment of sampling weights via calibration or estimation, using information available for the entire cohort, can sometimes dramatically improve the precision of estimated hazard ratios. We have also provided links to related R code, data sets, and tutorials and we encourage readers to utilize these tools.
Abbreviations
Atherosclerosis Risk in Communities
lipoprotein-associated phospholipase A 2
National Wilms Tumor Study
Author affiliations: Department of Biostatistics, University of Washington, Seattle, Washington (Norman E. Breslow, Thomas Lumley); Department of Medicine, Baylor College of Medicine, Houston, Texas (Christie M. Ballantyne); Department of Biostatistics, University of North Carolina, Chapel Hill, North Carolina (Lloyd E. Chambless); and Department of Probability and Mathematical Statistics, Charles University, Prague, Czech Republic (Michal Kulich).
The Atherosclerosis Risk in Communities study is carried out as a collaborative study supported by National Heart, Lung, and Blood Institute contracts N01-HC-55-15, N01-HC-55016, N01-HC-55018, N01-HC-555019, N01-HC-55020, N01-HC-55021, and N01-HC-55022. The National Wilms Tumor Study and the methodological studies are supported by grants R01-CA-054498 and R01-CA-40644 and earlier grants from the National Cancer Institute.
The authors thank the staff of the ARIC and NWTS studies for their important contributions.
Conflict of interest: none declared.
Google Scholar
Google Preview
- calibration
- nephroblastoma
- coronary heart disease
Email alerts
Citing articles via, looking for your next opportunity.
- Recommend to your Library
Affiliations
- Online ISSN 1476-6256
- Print ISSN 0002-9262
- Copyright © 2024 Johns Hopkins Bloomberg School of Public Health
- About Oxford Academic
- Publish journals with us
- University press partners
- What we publish
- New features
- Open access
- Institutional account management
- Rights and permissions
- Get help with access
- Accessibility
- Advertising
- Media enquiries
- Oxford University Press
- Oxford Languages
- University of Oxford
Oxford University Press is a department of the University of Oxford. It furthers the University's objective of excellence in research, scholarship, and education by publishing worldwide
- Copyright © 2024 Oxford University Press
- Cookie settings
- Cookie policy
- Privacy policy
- Legal notice
This Feature Is Available To Subscribers Only
Sign In or Create an Account
This PDF is available to Subscribers Only
For full access to this pdf, sign in to an existing account, or purchase an annual subscription.
Thank you for visiting nature.com. You are using a browser version with limited support for CSS. To obtain the best experience, we recommend you use a more up to date browser (or turn off compatibility mode in Internet Explorer). In the meantime, to ensure continued support, we are displaying the site without styles and JavaScript.
- View all journals
- Explore content
- About the journal
- Publish with us
- Sign up for alerts
- Published: 16 August 2021
Case-cohort design in hematopoietic cell transplant studies
- Jianwen Cai ORCID: orcid.org/0000-0002-4945-6733 1 &
- Soyoung Kim ORCID: orcid.org/0000-0003-1404-0575 2
Bone Marrow Transplantation volume 57 , pages 1–5 ( 2022 ) Cite this article
3594 Accesses
1 Citations
2 Altmetric
Metrics details
- Biostatistics
- Cancer stem cells
- Epidemiology
A Correction to this article was published on 02 December 2021
This article has been updated
Series Editors– Note
Imagine you and your colleagues have done 1000 transplants in persons with acute myeloid leukaemia (AML) in 1st remission. 5 percent of the 20 percent of recipients relapsing posttransplant have an isolated central nervous system relapse. You are curious and want to know whether there is anything special about this 5 percent, specifically whether this risk corelates with any pretransplant clinical and laboratory co-variates. You have extensive clinical data and some typical laboratory data on all 1000 but you suspect the culprit is mutation topography. What to do? Fortunately you have bio-banked DNA from the 1000. If resources and monies are not limiting you can do targeted or next generation sequencing on all 1000 DNA samples and off you go. However, most of us lack unlimited resources and monies. How can you sensibly and efficiently tackle this research problem? The answer is a case-cohort design study. In the typescript which follows Profs. Cai and Kim explain how to accomplish this. If you follow their advice you may need only to analyze samples from < 300 recipients rather than 1000 to test your hypothesis. They explain how to design such a study and provide references to estimate sample size.
Sadly, their typescript will not tell you how to get funding for the study, whish poor devil who will have to write the protocol, worse, who will shepherd it though endless committees for approval and the like. Help on these issues is outside the scope of our statistics series. In this context we suggest advice from Woody Allen’s article in the New Yorker: The Kugelmass Episode (April 24, 1977). When Prof. Kugelmass (English, City College) tells his analyst Dr. Mandel he has fallen in love with Emma Bovary who died of arsenic poisoning near Rouen, France 120 years earlier the analyst says: After all, I’m an analyst, not a magician . Kugelmass’ reply: Then perhaps what I need is a magician and is off to Coney Island to find one. Good luck, the magician may still be there! (Note: This typescript is R-rated. It contains an equation.)
Robert Peter Gale, Imperial College London, and Mei-Jie Zhang, Medical College of Wisconsin and CIBMTR.
Introduction
Case-cohort study-design, 1st proposed by Prentice in 1986 is a commonly-used cost-effective outcome-dependent study-design embedded in large cohort studies [ 1 , 2 , 3 ]. This design is used to reduce costs or conserve resources when the rate of the outcome event of interest is low and/or resources to ascertain exposure data are limited. The case-cohort sample consists of a (stratified) random sample of the full cohort supplemented by cases who are not in the random sample. There are several advantages to the case-cohort design: (1) it reduces the cost/effort for collecting redundant data on non-cases; (2) the random sample can be used for monitoring study progress; (3) data collected through a case-cohort study-design can be used to study the prospective relationship between the exposure and the outcome; and (4) because the random sample is selected independent of the outcome of interest collected exposure data can be used to study other outcomes of future studies. The nested case-control study is an alternative study design to the case-cohort design. In a nested case-control study, controls are selected at each failure time, consequently there is no representative random sample from the full cohort and the data collected from one nested case-control study cannot be easily used to study other outcomes.
The case-cohort study design can be used in transplant research. For example, the Center for International Blood & Marrow Transplant Research (CIBMTR) has two levels of data collection: (1) Transplant Essential Data (TED); and (2) Comprehensive Report Form (CRF) data. Collecting CRF data takes more resources than TED data. Transplant centers designated as CRF centers collect CRF data on some but not all recipients at their center. CRF data include detailed information such as co-variates as pretransplant conditioning, acute graft- versus -host disease (G v HD) etc .
Consider a study correlating to identify pretransplant co-variates with risk of developing a central nervous system (CNS) cancer posttransplant [ 4 ]. Posttransplant CNS cancers are rare occurring in <1% of recipients. A case-cohort study can be an efficient way to interrogate this question. At a CRF center one could select a random recipient sample and select all recipients developing a CNS cancer. CRF data can then be collected on the selected random sample and on the few subjects with CNS cancer. CRF data could include co-variates such as age at radiotherapy, prior CNS radiation exposure to anti-cancer drugs crossing the blood brain barrier, G v HD, corticosteroid exposure and others.
Competing risks are common in transplant recipients studies including death from leukemia recurrence before developing a CNS cancer. It is important to analyze competing risks data from case-cohort studies properly. In this tutorial we briefly describe case-cohort study-design and data available from a case-cohort design. We also introduce the commonly used analytic method, the cause-specific hazards model, and software for analyzing data from case-cohort studies with competing risks.
Case-cohort study design and the data structure
Let T i and C i be the potential failure and censoring times and µ i (=1, 2, …, or K) denote the cause of failure for subject i (= 1,…, n). Without losing generality we denote the event of interest as ‘cause 1′ (µ i = 1) and refer to it as the ‘cause of interest’ or ‘event of interest’. If there is only one cause of failure (i.e., K = 1) this reduces to the situation with a uni-variable survival outcome. Let X i (i.e., minimum of T i and C i ) and ∆ I (i.e., = 1 if T i is observed before C i and otherwise 0) denote the observed time and failure indicator. Let Z i (t) denote co-variates. For a case-cohort study, we sample a random sub-cohort of all subjects and all subjects with the event of interest regardless of whether they are in the selected subcohort. Figure 1 provides an illustration on the case-cohort sample. Co-variate information Z i (t) can be decomposed into two parts as Z i (t) = (Z iC (t), Z iE (t)), where Z iC (t) are available on the entire cohort and Z iE (t) are co-variates only available for subjects in the case-cohort sample. For example, Z iE (t) can include the CRF data such as pretransplant radiation dose and Z iC (t) can include TED level data such as age at transplant and sex. Let ξ i be an indicator for subject i being selected into the sub-cohort. The observable data is {X i , ∆ i , ∆ i µ i , ξ i , Z iC (t), Z iE (t)} if subject i is in the case-cohort sample, and {X i , ∆ i, ∆ i µ i , ξ i , Z iC (t)} otherwise.
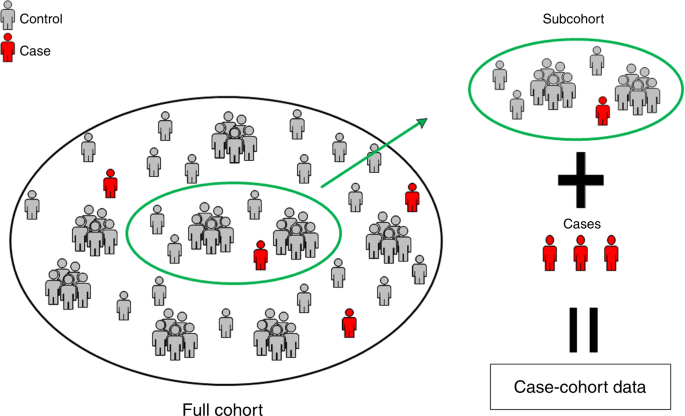
illustration for subjects selection in the case-cohort design.
For example, suppose we are interested in assessing the impacts of mutations ASXL1, EZH2, SRSF2, IDH1, IDH2 , and TP53 on death [ 5 ]. Collecting these data from stored DNA samples is expensive. To reduce cost and preserve samples we can design a case-cohort study. Assume there are 1000 subjects in the full cohort, 20% die and we set the selection probability of the sub-cohort at 25%. The size of the case-cohort dataset is 400 subjects, 250 in the sub-cohort and 150 outside the sub-cohort. Overall, 200 subjects died and 200 are alive. In this scenario mutations data are collected on only these subjects whereas survival data and other co-variates such as age and sex are collected from all 1000 subjects in this study.
Models and weights for case-cohort studies
For competing risks data there are in general two commonly used models: (1) the cause-specific proportional hazards; and (2) sub-distribution hazards. The cause-specific hazards model is useful when one’s interest is in studying disease etiology whereas the sub-distribution hazards model is of greater interest when the emphasis is on estimating actual risk and prognosis. Here we focus on cause-specific hazard model for case-cohort studies because of the availability of statistical software packages.
The hazard function in the cause-specific hazard model for cause k is given by:
where \(\lambda _{0k}\left( t \right)\) is an unspecified baseline hazard function and β k is an unknown parameter of interest. The effects of risk factor for cause k outcome can be measured by the hazard ratio exp ( β k ). In the cause-specific hazard model one treats subjects who experienced competing risks as censored. When there is only one cause (i.e., K = 1) the cause-specific hazard model is reduced to the Cox proportional hazards model.
Because we lack extensive co-variate data outside the case-cohort sample the estimation method for the Cox proportional hazards model needs to be modified. The so-called weighed partial likelihood is widely-used for case-cohort design. The key to the weighted partial likelihood is to understand the weighting of subjects with the event of interest and sub-cohort subjects without the event of interest. Several weighting functions for case-cohort design are proposed [ 6 , 7 , 8 ]. In this tutorial, we focus on a time-independent weight function which uses the sub-cohort sampling probability, denoted by α. Specifically, weights for subjects with the event of interest is 1 because all subjects in the full cohort with the event of interest are included in the case-cohort sample i.e., cases in the case-cohort sample are all cases in the full cohort. In contrast, some subjects without the event of interest are not in the case-cohort sample. Consequently, sub-cohort subjects without the event of interest are weighted by 1/α. For example, suppose α is 25%. Then the weight for subjects in the sub-cohort who do not experience the event of interest is 1/0.25 = 4 indicating one subject in the sub-cohort without the event represents four subjects without the event in the full cohort. In practice sampling probability α is unknown and needs to be estimated.
To analyze case-cohort data using SAS (PHREG procedure), two steps are required. Step (1) create weights for each subject. Step (2) calculate the robust variance to account for case-cohort data structure. The example SAS code is provided in the Supplementary material . In PHREG procedure, “COVS(AGGREGATE)” and “ID” statement options allow to calculate robust sandwich type of variance. The R statistical package provides similar capabilities. An example of R code is in the Supplementary material . We now show how to fit these cause-specific models using CIBMTR data.
Consider the transplant dataset reported by Ustun et al. (2018) of 7128 subjects receiving a 1st allograft for acute myeloid leukemia, acute lymphoblastic leukemia, or myelodysplastic syndrome from January, 2008 to December, 2012 [ 9 ]. The primary outcome of interest is a fungal infection in this data. 589 (8%) had a fungal infection by day 100 and 1059 (15%) died without a fungal infection before day 100. In a case-cohort study we create a case-cohort sample by randomly selecting 20% of subjects from the 7128 to 1434 subjects to form a sub-cohort. Next, we add everyone not in the sub-cohort who had a fungal infection before day 100 (Fig. 2 ). 115 of the 1434 randomly-selected subjects had a fungal infection before day 100, 163 died before day 100 without a fungal infection and 1156 had neither a fungal infection nor died before day 100. Next, we add 474 subjects (589–115) with a fungal infection before day 100 not in the randomly-selected sub-cohort bringing numbers of subjects in the case-cohort sample to 1908 (1434 + 474). In this case-cohort sample, 1319 (1434 − 115) did not have a fungal infection before day 100 and were weighted by 1/0.2 = 5 whereas 589 had a fungal infection before day 100 and were weighted by 1.
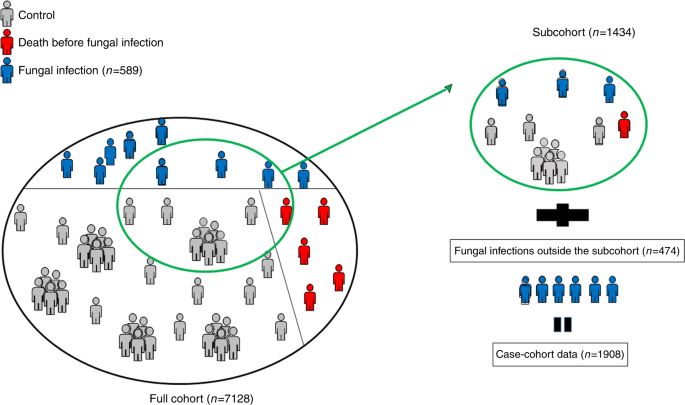
the case-cohort sample for the fungal infection.
Co-variates of interest in this study were age at transplant, graft-type, G v HD prophylaxis, and year of transplant. We checked the proportional hazards assumption by testing whether the coefficient of log transformed time × each co-variate is equal to zero and all p values were >0.05.
Data of co-variate frequencies in the full and sub-cohorts displayed in Table 1 indicate reasonable comparability. Next, we fit the cause-specific hazard model using the case-cohort sample and fit the same model using the full cohort to compare results. Note the full cohort analysis is only possible because we generated the case-cohort sample from the full cohort. This full cohort analysis would not be possible in real case-cohort studies. Table 2 shows hazard ratios, 95% confidence intervals and p values. Hazard ratios based on the case-cohort sample are very close to those based on the full cohort. The data indicate age at transplant, graft-type, G v HD prophylaxis, and year of transplant are significantly correlated with risk of a fungal infection before day 100 in the full and the case-cohort sample. As expected, the 95% confidence intervals for the case-cohort ( N = 1908) are wider than those for the full cohort ( N = 7128).
Conclusion/discussion
Case-cohort design is an efficient, cost effective statistical method when an event(s) of interest is rare and/or when obtaining co-variate data is difficult and/or expensive and has great potential in hematopoietic cell transplant research. We provide a brief review of the case-cohort design and show how to properly analyze case-cohort data when there are competing risks using statistical software packages. In this tutorial we considered only cause-specific hazards models for competing risks but one can easily apply these weighting scheme to sub-distribution hazards model such as the Fine-Gray model [ 10 , 11 ].
In our example we selected the sub-cohort by simple random sampling but stratified sampling can also be used to ensure balance for important co-variates. Also, in the tutorial we only considered time-independent weights. Several methods have been proposed to improve efficiency for case-cohort studies using time-dependent weights and extra information such as auxiliary co-variate data whereby time-dependent weights are calculated among subjects at-risk at each time point [ 12 , 13 ]. The case-cohort design can also be used to analyze multiple outcomes [ 14 , 15 , 16 , 17 , 18 ]. Lastly, there are sample size and power calculations. Sample size estimation is an important first step for designing a study and formulae for these are available [ 19 , 20 ].
We hope readers will find this discussion useful and share it with their center statisticians. We expect increased use of the case-cohort method to tackle important questions in hematopoietic cell transplantation in the near future.
Change history
02 december 2021.
A Correction to this paper has been published: https://doi.org/10.1038/s41409-021-01522-4
Prentice RL. A case-cohort design for epidemiologic cohort studies and disease prevention trials. Biometrika. 1986;73:1–1.
Article Google Scholar
Knuiman MW, Divitini ML, Olynyk JK, Cullen DJ, Bartholomew HC. Serum ferritin and cardiovascular disease: a 17-year follow-up study in Busselton, Western Australia. Am J Epidemiol. 2003;158:144–9.
Article CAS Google Scholar
Ballantyne CM, Hoogeveen RC, Bang H, Coresh J, Folsom AR, Heiss G, et al. Lipoprotein-associated phospholipase A2, high-sensitivity C-reactive protein, and risk for incident coronary heart disease in middle-aged men and women in the Atherosclerosis Risk in Communities (ARIC) study. Circulation. 2004;109:837–42.
Gabriel M, Shaw BE, Brazauskas R, Chen M, Margolis DA, Sengelov H, et al. Risk factors for subsequent central nervous system tumors in pediatric allogeneic hematopoietic cell transplant: a study from the Center for International Blood and Marrow Transplant Research (CIBMTR). Biol Blood Marrow Transpl. 2017;23:1320–6.
Gupta V, Kennedy JA, Capo-Chichi JM, Kim S, Hu ZH, Alyea EP, et al. Genetic factors rather than blast reduction determine outcomes of allogeneic HCT in BCR-ABL–negative MPN in blast phase. Blood Adv. 2020;4:5562–73.
Self SG, Prentice RL. Asymptotic distribution theory and efficiency results for case-cohort studies. Ann Statistics.1988;16:64–81.
Barlow WE. Robust variance estimation for the case-cohort design. Biometrics. 1994;50:1064–72.
Borgan O, Langholz B, Samuelsen SO, Goldstein L, Pogoda J. Exposure stratified case-cohort designs. Lifetime Data Anal. 2000;6:39–58.
Ustun C, Young JA, Papanicolaou GA, Kim S, Ahn KW, Chen M, et al. Bacterial blood stream infections (BSIs), particularly post-engraftment BSIs, are associated with increased mortality after allogeneic hematopoietic cell transplantation. Bone Marrow Transpl. 2019;54:1254–65.
Fine JP, Gray RJ. A proportional hazards model for the subdistribution of a competing risk. J Am Stat Assoc. 1999;94:496–509.
Kim S, Xu Y, Zhang MJ, Ahn KW. Stratified proportional subdistribution hazards model with covariate‐adjusted censoring weight for case‐cohort studies. Scand J Stat. 2020;47:1222–42.
Kulich M, Lin DY. Improving the efficiency of relative-risk estimation in case-cohort studies. J Am Stat Assoc. 2004;99:832–44.
Samuelsen SO, Ånestad H, Skrondal A. Stratified case‐cohort analysis of general cohort sampling designs. Scand J Stat. 2007;34:103–19.
Kang S, Cai J. Marginal hazards model for case-cohort studies with multiple disease outcomes. Biometrika. 2009;96:887–901.
Kim S, Cai J, Lu W. More efficient estimators for case-cohort studies. Biometrika. 2013;100:695–708.
Kim S, Cai J, Couper D. Improving the efficiency of estimation in the additive hazards model for stratified case–cohort design with multiple diseases. Stat Med. 2016;35:282–93.
Kim S, Zeng D, Cai J. Analysis of multiple survival events in generalized case‐cohort designs. Biometrics. 2018;74:1250–60.
Langholz B, Thomas DC. Nested case-control and case-cohort methods of sampling from a cohort: a critical comparison. Am J Epidemiol. 1990;131:169–76.
Cai J, Zeng D. Sample size/power calculation for case–cohort studies. Biometrics. 2004;60:1015–24.
Cai J, Zeng D. Power calculation for case-cohort studies with non-rare events. Biometrics. 2007;63:1288–95.
Download references
Acknowledgements
The authors would like to thank Drs Robert Peter Gale and Mei-Jie Zhang for inviting us to contribute this paper. This work was partially supported by grants from the National Cancer Institute (U24CA076518, and P01CA142538) and National Institute of Environmental Health Science (P30ES010126).
Author information
Authors and affiliations.
Department of Biostatistics, University of North Carolina at Chapel Hill, Chapel Hill, NC, USA
Jianwen Cai
Division of Biostatistics, Medical College of Wisconsin, Wauwatosa, WI, USA
Soyoung Kim
You can also search for this author in PubMed Google Scholar
Contributions
Both JC and SK wrote the paper and SK analyzed the data example.
Corresponding author
Correspondence to Soyoung Kim .
Ethics declarations
Competing interests.
The authors declare no competing interests.
Additional information
Publisher’s note Springer Nature remains neutral with regard to jurisdictional claims in published maps and institutional affiliations.
The original online version of this article was revised: The grant support number U24CA233032 was given incorrectly should not have been included in the publication and has been removed entirely.
In addition the Series Editors’ Note was missing from this article and should have read:
Supplementary information
Supplementary material, rights and permissions.
Reprints and permissions
About this article
Cite this article.
Cai, J., Kim, S. Case-cohort design in hematopoietic cell transplant studies. Bone Marrow Transplant 57 , 1–5 (2022). https://doi.org/10.1038/s41409-021-01433-4
Download citation
Received : 12 July 2021
Revised : 14 July 2021
Accepted : 03 August 2021
Published : 16 August 2021
Issue Date : January 2022
DOI : https://doi.org/10.1038/s41409-021-01433-4
Share this article
Anyone you share the following link with will be able to read this content:
Sorry, a shareable link is not currently available for this article.
Provided by the Springer Nature SharedIt content-sharing initiative
Quick links
- Explore articles by subject
- Guide to authors
- Editorial policies


An official website of the United States government
The .gov means it’s official. Federal government websites often end in .gov or .mil. Before sharing sensitive information, make sure you’re on a federal government site.
The site is secure. The https:// ensures that you are connecting to the official website and that any information you provide is encrypted and transmitted securely.
- Publications
- Account settings
Preview improvements coming to the PMC website in October 2024. Learn More or Try it out now .
- Advanced Search
- Journal List
- HHS Author Manuscripts

SAMPLE SIZE/POWER CALCULATION FOR STRATIFIED CASE-COHORT DESIGN
Applied Statistics, Department of Mathematical Sciences, The University of Memphis; Department of Biostatistics, CSL Behring
Jianwen Cai
Department of Biostatistics, School of Public Health, University of North Carolina Chapel Hill
Donglin Zeng
Associated data.
The Case-cohort (CC) study design usually has been used for risk factor assessment in epidemiologic studies or disease prevention trials for rare diseases. The sample size/power calculation for the CC design is given in Cai and Zeng [ 1 ]. However, the sample size/power calculation for a stratified case-cohort (SCC) design has not been addressed before. This article extends the results of Cai and Zeng [ 1 ] to the SCC design. Simulation studies show that the proposed test for the SCC design utilizing small sub-cohort sampling fractions is valid and efficient for situations where the disease rate is low. Furthermore, optimization of sampling in the SCC design is discussed and compared with proportional and balanced sampling techniques. An epidemiological study is provided to illustrate the sample size calculation under the SCC design.
1. Introduction
Time-to-event is a commonly used endpoint for the risk factor assessment in epidemiologic studies or disease prevention trials [ 2 – 7 ]. The case-cohort (CC) design, originally proposed by Prentice [ 8 ], has often been used in studying the time to event when the disease is rare and the cost of collecting the risk factor information is high. A CC sample consists of a sub-cohort, which is a random sample of the full cohort, and all the subjects with the event (cases). Statistical analysis methods for analyzing data from the CC study design have been described in many publications [ 8 – 20 ]. For rare diseases, Cai and Zeng [ 1 ] proposed a log-rank type of test statistic, which is equivalent to the score test based on a pseudo-partial likelihood function, similar to that was described in Self and Prentice [ 9 ]. Furthermore, Cai and Zeng [ 1 ] provided an explicit procedure for calculating the sample size and power based on their proposed test.
In studies where the study populations are not homogenous or the original cohort is assembled through a stratified design, a stratified case-cohort (SCC) design may be more appropriate [ 21 – 22 ]. The SCC sample consists of the stratified sub-cohorts selected by a stratified random sampling from the full cohort, and all the cases. For example, the MONICA, Risk, Genetics, Archiving, and Monograph (MORGAM) study [ 23 ] is a multinational collaborative cohort study that prospectively followed the development of coronary heart disease (CHD) and stroke events. One goal of this study was to identify risk genotypes for predicting a CHD event. Since the CHD incidence rate differs by gender, and genotyping is expensive, a possible cost-effective design can be a stratified case-cohort design, where the gender is considered as a stratification factor so that the different proportion of sub-cohort samples is selected for each gender group.
Although stratified methods for analyzing data from the SCC design have been studied extensively [ 18 , 24 ], the sample size and power calculations of the SCC design have not been previously addressed. This paper aims to fill this gap. Specifically, we propose a stratified log-rank statistic and derive expressions for sample size and power calculations. In addition, we compare different sampling strategies including proportional sampling, balanced sampling, and optimal sampling designs. Several simulation studies are presented to evaluate the proposed method using the MORGAM study. We further compare the stratified design/test with the unstratified design/test in the conclusion and discussion section.
2. Stratified case-cohort design and stratified log-rank test
2.1 notation.
Assume that there are n subjects and L strata in a stratified full cohort, and n l subjects in stratum l ( l = 1, …, L ). Assume two groups indicating the expensive and dichotomous exposure status (for example, the standard versus the wild type single nucleotide polymorphism) and assume n lj subjects in exposure group j ( j = 1, 2) of stratum l . Assuming that T lij represents the event time and C lij the censoring time for subject i in exposure group j and stratum l ( i = 1, …, n lj ), it is reasonable to assume the T lij s are independent of each other. Let X lij = T lij ∧ C lij be the observed time, where a ∧ b denotes the minimum of a and b , and Δ lij = I ( T lij ≤ C lij ) the failure indicator, in which Δ lij = 1 denotes observed failure and Δ lij = 0 denotes censoring.
In the SCC design, the exposure status is obtained for all the cases and a stratified sub-cohort sample. Specifically, we assume that ñ l subjects are randomly sampled into a sub-cohort from n l subjects in stratum l , and the sub-cohort size is n ∼ = ∑ l = 1 L n ∼ l . Let ξ lij = 1 denote that subject i in group j and stratum l is selected into the sub-cohort and ξ lij = 0 otherwise. Let γ l be the proportion of subjects in group 1 and (1− γ l ) the proportion of subjects in group 2 in stratum l . All subjects in the sub-cohort and all events in the L strata make up the stratified case-cohort sample.
2.2 Test statistic
A log-rank type of test is used to compare the hazard rates between the two groups in SCC. The null hypothesis is H 0 : Λ l1 ( t ) = Λ l2 ( t ), l = 1, …, L , t ∈ [0, Γ], where Γ is the length of study period and Λ lj ( t ) the cumulative hazard function of the event time t in group j in stratum l . To construct a log-rank type test for the stratified case-cohort sample, we first notice that a weighted stratified log-rank test statistic for the full cohort [ 9 ] may be expressed as W n ∗ = ∑ l = 1 L ∫ 0 Γ ω ( t ) Y ¯ l 1 ( t ) Y ¯ l 2 ( t ) Y ¯ l 1 ( t ) + Y ¯ l 2 ( t ) { d N ¯ l 1 ( t ) Y ¯ l 1 ( t ) - d N ¯ l 2 ( t ) Y ¯ l 2 ( t ) } , where Ȳ lj ( t ) is the number of subjects at risk and N ̄ lj ( t ) is a counting process representing the number of events at time t in group j and stratum l, and ω ( t ) is a weight function. The formula above can also be expressed as
For the full cohort, the log-rank test statistic is known to be the same as the score function of the Cox partial likelihood function [ 1 , 9 ].
The test statistic W n ∗ requires the covariate information of the full cohort; in a SCC sample the covariate information is only available for the subjects in the sub-cohort and the cases. We propose to use the sub-cohort data to approximate Ȳ lj ( t ) by Ỹ lj ( t )/ p l , where Ỹ lj ( t ) is the number of subjects at risk for group j and stratum l in the sub-cohort, and p l is the sampling fraction of the sub-cohort in stratum l . Hence, we obtain the following stratified case-cohort test statistic:
where Y ∼ l j ( t ) = ∑ i = 1 n ∼ l j I ( X lij ≥ t ) , and ñ lj is the number of subjects in group j and stratum l in the sub-cohort. Since all the quantities in the summation contribute to W n only if Δ li1 = 1 or Δ li2 = 1, W n can be obtained based on the observed data. It is also easy to verify that this test statistic is the score function of the stratified version of the pseudo partial likelihood function, and, following the results in [ 9 ], W n has an asymptotic normal distribution.
2.3 Asymptotic variance
The asymptotic variance of W n is the summation of the asymptotic variance of W nl from all the strata. The traditional case-cohort design is considered as a special case of SCC with the number of strata L = 1 [ 9 , 18 ]. Assume the proportion of subjects in group 1 is γ l = n l 1 / n l , γ l ∈ (0, 1), and ñ l / n l converges to p l in stratum l as n goes to ∞ (i.e., p l = lim ñ l / n l ). According to Self and Prentice [ 9 ], under H 0 , n −1/2 W n has an asymptotic normal distribution: n −1/2 W n → D N (0, σ 2 + ψ ), where σ 2 = ∑ l = 1 L v l σ l 2 and ψ = ∑ l = 1 L v l ψ l with v l = n l / n , where σ l 2 and ψ l correspond to the asymptotic variance of the log-rank test based on stratum l in the full cohort, and the variation resulting from sampling from stratum l for the sub-cohort, respectively. Under the null hypothesis H 0 : Λ l1 ( t ) = Λ l2 ( t ) = Λ l ( t ), t ∈ [0, Γ], let S l ( t ) = S lj ( t ) = P ( T lj ≥ t ), and π lj ( t ) = P ( C lj ≥ t ), then the results in Self and Prentice [ 9 ] give
with event time w ∈ [0, Γ] and a ∨ b denoting the maximum of a and b .
The estimator for the asymptotic variance for W n , σ ^ W n 2 , can be derived based on the arguments similar to those in Cai and Zeng [ 1 ]. Specifically, σ ^ W n 2 is given by σ ^ W n 2 = σ ^ 2 + ψ ^ , where
with p ̂ l = ñ l /n l being the estimate of p l , and σ ̂ 2 being the estimate of σ 2 given by
Since all the quantities expressed above contribute to σ ̂ 2 and ψ ̂ only when Δ li 1 = 1 or Δ li 2 = 1, σ ^ W n 2 can be obtained from the observed data. The derivations are given in the Web Appendix .
Therefore, to test the equality of the cumulative hazard function of the event time between the two groups in SCC, i.e., to test the null hypothesis H 0 : Λ l1 ( t ) = Λ l2 ( t ) , l = 1, …, L , t ∈ [0, Γ] vs. the alternative hypothesis H A : Λ l1 ( t ) ≠ Λ l2 ( t ) (two-sided) at the significance level α , we reject H 0 if | n - 1 / 2 W n / σ ^ W n 2 | > Z 1 - α / 2 , where Z α is the (100 α ) th percentile of the standard normal distribution.
3. Sample size and power calculation
The sample size and power estimation formula is derived and simplified based on the alternative hypothesis H A : Λ l1 ( t ) = e θ Λ l2 ( t ), t ∈ [0, Γ] where θ = O ( 1 / n ) , where the log-hazards ratios between the two exposure groups are assumed to be constant across the strata. We further assume the following conditions: (i) the censoring distributions are the same in the two groups; (ii) the number of failures is very small (i.e., failure proportion 0 italic> p D ≪ 1) in the full cohort; and (iii) there are no ties of failures. For the sample size and power calculation, we consider the test statistic with ω ( t ) = 1.
Under the alternative hypothesis H A , the asymptotic expectation of n −1/2 W n is the same as the asymptotic expectation of the usual log-rank test statistic for the full cohort under H A and can be approximated by n - 1 / 2 ∑ l = 1 L ∫ 0 Γ Y ¯ l 1 ( t ) Y ¯ l 2 ( t ) Y ¯ l 1 ( t ) + Y ¯ l 2 ( t ) [ d Λ l 1 ( t ) - d Λ l 2 ( t ) ] ≈ n - 1 / 2 ∑ l = 1 L θ ( 1 - γ l ) D l 1 , where D lj is the total number of failures in group j ( j = 1,2) in stratum l. Additionally, σ ̂ 2 can be approximated by 1 / n ∑ l = 1 L ( ( 1 - γ l ) 2 D l 1 + γ l 2 D l 2 ) following the exact approximation and algebra as Cai and Zeng [ 1 ] for each stratum. To simplify ψ ̂ , since the failures are much fewer than the stratum sizes, we approximate ∑ j = 1 2 Y ¯ l j ( t ) by ( n l − D l /2), where D l = D l 1 + D l 2 . Since the size of the risk set in stratum l of the sub-cohort is about p l times the size of the risk set in stratum l of the full cohort, ψ ̂ can be approximated by 1 n ∑ l = 1 L ( 1 - p l ) ( n l - D l / 2 ) p l γ l ( 1 - γ l ) ( D l 1 + D l 2 ) 2 . Hence, the non-centrality parameter for n - 1 / 2 W n / σ ^ W n 2 under the alternative is approximately n - 1 / 2 ∑ l = 1 L θ ( 1 - γ l ) D l 1 1 / n ∑ l = 1 L ( ( 1 - γ l ) 2 D l 1 + γ l 2 D l 2 ) + 1 / n ∑ l = 1 L ( 1 - p l ) ( n l - D l / 2 ) p l γ l ( 1 - γ l ) ( D l 1 + D l 2 ) 2 , which can be simplified as n 1 / 2 θ ∑ l = 1 L ( γ l ( 1 - γ l ) p D l v l ) ∑ l = 1 L { γ l ( 1 - γ l ) p D l v l ( 1 + ( 1 - p l ) ( 1 - p D l / 2 ) p l p D l ) } , where p Dl is the failure proportion in stratum l and v l is the proportion of stratum l in the full cohort ( v l = n l / n ). Consequently, the power function is
where n is the total number of subjects in the full cohort, θ is the log hazard ratio, α is the significance level, p Dl is the failure proportion in stratum l , v l is the proportion of stratum l , γ l is the proportion of subjects in group 1 and (1− γ l ) is the proportion of subjects in group 2 in stratum l , and p l is the sub-cohort sampling fraction in stratum l. For rare diseases, p Dl is very small. By dropping p Dl /2, the formula ( 4 ) can be further simplified as Φ ( Z α / 2 + n 1 / 2 ∣ θ ∣ ∑ l = 1 L ( γ l ( 1 - γ l ) p D l v l ) ∑ l = 1 L { ( γ l ( 1 - γ l ) p D l v l ) ( 1 + ( 1 / p l - 1 ) p D l ) } ) .
When L = 1, the above function can be further simplified as Φ ( Z α / 2 + n ∼ 1 / 2 ∣ θ ∣ γ ( 1 - γ ) p D p + ( 1 - p ) P D ) , in which p D is the failure proportion and ñ = np . This is the same power function of the CC design as reported in Cai and Zeng [ 1 ]. When p l =1, we obtain the power function of the stratified log-rank test for the full cohort, which is given by:
4. Proportional, balanced, and optimal designs
This section describes the power issues for two commonly used stratified sampling methods, namely the proportional and balanced designs. Also described is an allocation strategy that maximizes the power.
4.1 Proportional design
The proportional design is commonly used in stratified studies. Under the proportional design, the number of subjects in the sub-cohort at each stratum is proportional to the size of the stratum in the population. For example, consider the full cohort size n = 2,000, and there are 4 strata with the strata proportion of 0.1, 0.2, 0.3 and 0.4; i.e., there are 200, 400, 600, and 800 subjects in the 4 strata, respectively. The sub-cohort consists of 200 subjects. With the proportional design, the numbers of samples in each stratum are 20, 40, 60, and 80, respectively. Under such a design, the sub-cohort sampling proportions are the same for all strata, i.e., p l = p for l.
To detect a log hazard ratio of θ with power β and significance level α , the required total sub-cohort size is at least:
where [x] denotes the smallest integer that is bigger than x, and B 2 = n 1 / 2 θ ∑ l = 1 L ( γ l ( 1 - γ l ) p D l v l ) Z 1 - α / 2 + Z β . The sampling proportion p = ñ / n , and the required number of subjects in stratum l is ñ l = ñv l , l = 1, 2, …, L .
4.2 Balanced design
Another popular stratified sampling approach is the balanced design. Under such a design, the number of subjects in a sub-cohort is the same across the strata. For example, consider the full cohort size n = 2,000 with 4 strata, and a total of 200 subjects is required for the sub-cohort, each stratum would contain 50 sampled subjects. To detect a log hazard ratio θ with a power β and a significance level α , the required total sub-cohort size ñ is at least
The sub-cohort size in stratum l is ñ l = ñ / L and the sub-cohort sampling proportion p l = ñ l / n l = ñ /( Lnv l ).
4.3 Optimal design
In many studies, the number of subjects that can be included in sub-studies is limited because of financial and resource constraints. In these studies, we are given the total number of subjects in the sub-cohort. The distribution of the number of subjects to each of the stratum in the sub-cohort needs to be determined. We consider an optimal design strategy which provides the highest power under such situation. Specifically, we propose an optimal design with a set of p l which provides the highest power for a given ñ . This optimization problem is solved by using the Lagrange multipliers method following the steps below.
Maximizing the power function for a given ñ is equivalent to minimizing the denominator ∑ l = 1 L ( γ l ( 1 - γ l ) p D l v l ) ( 1 + 1 - p l ( 1 - p D l / 2 ) p l p D l ) in the formula ( 4 ), a function of p l , subject to ∑ l = 1 L p l v l = n ∼ / n , a constraint function of p l . We obtain the Lagrange function Ξ ( p l , λ ) = ∑ l = 1 L ( ( γ l ( 1 - γ l ) p D l v l ) ( 1 + 1 - p l ( 1 - p D l / 2 ) p l p D l ) ) + λ ∗ ( ∑ l = 1 L ( p l v l ) - n ∼ n ) . Furthermore, we have
After solving these two sets of equations, we obtain the optimal sub-cohort sampling proportion
Hence, the optimal power for a given ñ is calculated as
To achieve a power β with a significance level α based on the optimal design, the required total sub-cohort size is given by n ∼ = [ n ( ∑ l = 1 L γ l ( 1 - γ l ) / ( 1 - p D l / 2 ) p D l v l ) 2 B 2 2 - ∑ l = 1 L ( γ l ( 1 - γ l ) p D l v l ( 1 - p D l / ( 1 - p D l / 2 ) ) ) ] , where B 2 = n 1 / 2 θ ∑ l = 1 L ( γ l ( 1 - γ l ) p D l v l ) Z 1 - α / 2 + Z β . Therefore,
From the formula ( 8 ), we observe that under the situation when γ l is similar across the strata and p Dl is very small (disease is rare), the optimal n ∼ l = p D l p D n ∼ or n ∼ l = D l D n ∼ . Furthermore, under the homogeneous situation where p Dl is similar across the strata, the optimal p l is close to ñ / n the estimate p from the proportional design. It means that the proportional design is nearly optimal when the event rate is homogeneous across the strata.
We obtain the number of subjects in stratum l using ñ l = p l nv l , and the SCC total sample size n scc = n ∑ l = 1 L ( p l v l + ( 1 - p l ) p D l v l ) , in which p l is obtained from the formulae in the sections for the proportional, balanced, or optimal design above, depending on the desired design.
4.4 Practical note: minimal detectable log-hazard ratio
The denominator of the total sub-cohort size ñ formula in the previous section needs to be positive. This condition is written as
Since the failure rate p Dl is usually fairly small for the case-cohort studies, p D l - p D l 2 / ( 1 - p D l 2 ) ≈ p D l . Hence, θ 0 ≈ ( Z 1 - α / 2 + Z β ) n 1 / 2 ∑ l = 1 L ( γ l ( 1 - γ l ) p D l v l ) , which is the log-hazard ratio that can be detected with the entire cohort. This condition implies that the stratified case-cohort design will not be able to detect a hazard ratio smaller than the one that can be detected by using the entire cohort, which is a reasonable restriction.
5. Numeric results
5.1 theoretical power.
Table 1 shows the theoretical power of the SCC design, as well as the power of the full cohort and the sub-cohort. The power function ( 4 ) is used to calculate P SCC , the power of the SCC design, while formula ( 5 ) is used to calculate P Full , the power of the full cohort. The sub-cohort power P Sub is obtained by substituting n with ñ in the full cohort power function, where ñ is the sub-cohort size n ∼ = n ∑ l = 1 L v l p l . The power P Full , P SCC , and P Sub are calculated for the different combinations of the full cohort size n , the event proportion p Dl , the group 1 proportion γ l , the log-hazard ratio θ , and the sub-cohort sampling fraction p l in stratum l. The significant level is set at α = 0.05 and the number of strata is L = 4. The event proportion p D in the table is a mean value over all strata. For instance, at the mean value of 10%, p Dl are set to 9%, 8%, 11%, and 10% for each of the 4 strata, respectively. Similarly, at the mean value of 5% (1%), p Dl are set to 4%, 5%, 4.5%, and 6% (0.8%, 1%, 1.2%, and 0.9%) for each of the 4 strata, respectively. In the example where the full cohort size n = 2,000, the event proportion p D = 10%, the group 1 proportion γ l = 0.3, and the log-hazard ratio θ = 0.5, the SCC sample with the 10% sub-cohort sampling proportion yields a power of 0.634, while the power for the full cohort and for the stratified random sample are 0.894 and 0.172, respectively. In another example where the full cohort size n = 10,000, the event proportion p D = 1%, the group 1 proportion γ l = 0.3, and the log-hazard ratio θ = 1.0, the SCC sample with the 1% sub-cohort sampling yields a power of 0.898 while the powers for the full cohort and for the stratified random sample are 0.996 and 0.067, respectively. The results in Table 1 suggest that the SCC design is an efficient and attractive solution in situations with low event proportions and small sub-cohort sampling fractions.
Theoretical Power of Stratified Case-Cohort Design
n =full cohort size, p D =mean event proportion, γ l =group 1 proportion, θ =log-hazard ratio, p l =sub-cohort sampling fraction in stratum l. P SCC =theoretical power of SCC, P Full =theoretical power of full cohort, and P Sub =theoretical power of sub-cohort. Significant level α = 0.05.
5.2 Type I error and power for the stratified log-rank test
Simulation studies are conducted to evaluate the empirical type I error and the empirical power for the stratified log-rank test using the SCC, the full cohort, and the sub-cohort data. The simulation procedures and their results are presented in the Web Appendix (Tables A and B) .
Appendix Table A shows the empirical type I error for the stratified log-rank test using the SCC ( SCC ), the full cohort ( Full ), and the sub-cohort ( Sub ) samples. The significance level α is set at 0.05 and the number of strata L = 4. Various values are considered for the full cohort size n , the stratum proportion v l , the event proportion p Dl , the group 1 proportion γ l , and the sub-cohort sampling fraction p l in stratum l. Overall, the empirical type I error rates in the SCC samples are fairly close to the nominal 0.05 level.
Appendix Table B presents the empirical power for the log-rank tests in the SCC the full cohort and the sub-cohort samples. In addition, the theoretical power is compared with the empirical power. It is observed that the test based on the SCC design is more powerful than using the sub-cohort, and the power based on the full cohort provides the upper bound. Note that in real studies, it is usually impossible to collect all the full cohort information required to conduct the log-rank test. As illustrated in Appendix Table B , using only a small fraction of the subjects, the power of the SCC design is over 50% of the power with the full cohort. As expected, when the sampling rate increases, the power of the SCC increases. Overall, the empirical power is very close to the theoretical powers. In the additional simulations, we consider the different group 1 proportions across strata and the results are similar.
5.3 Proportional, balanced, and optimal designs comparison
Power comparison under homogeneous and heterogeneous event rates.
We compare the proportional, balanced, and optimal sampling methods in order to investigate which one is more efficient in the SCC design. Two situations where the event rates are relatively homogeneous or heterogeneous over the strata are considered for comparison. In the situation where the event rates are homogeneous, the event proportion p Dl at each stratum is relatively similar to each other. In the situation where the event rates are heterogeneous, the event proportions p Dl over the strata have a wide range. The corresponding analysis results in both homogeneous and heterogeneous situations are presented in Table 2 .
Theoretical Power of Proportional, Balanced, and Optimal Sampling in SCC
p D =mean event proportion, γ l =group 1 proportion, θ =log-hazard ratio, ñ =sub-cohort size, n SCC =SCC sample size, p l =sub-cohort sampling fraction in stratum l. P prop =proportional power, P Bal =balanced power, and P Opt =optimal power. Set1 (Set2): event proportion=9%, 30%, 5%, and 20% (4%, 25%, 10%, and 6%) for strata 1–4.
Results for the SCC with homogeneous event rates are presented for a theoretical power based on proportional, balanced, and optimal sampling for SCC with various combinations of the full cohort size n , the event proportion p Dl , the group 1 proportion γ l , the log hazard ratio θ , and the sub-cohort size ñ . The number of strata is L = 4 with the stratum proportions ( v l ) of 0.1, 0.2, 0.3, and 0.4, respectively. The event proportion p D in the table is a mean value over all strata. Specifically, at the level of 10%, p Dl s are set to 9%, 8%, 11%, and 10% for each stratum. Similarly, at p D = 5%, 4 strata have 4%, 5%, 4.5%, and 6%, respectively. The sub-cohort sampling fractions p l in stratum l for the proportional, balanced, and optimal designs are calculated by ñ / n , ñ / Lnv l , and the formula ( 8 ), respectively. The total SCC sizes n scc (prop), n scc (bal), and n scc (opt) are then calculated using the formula n ∑ l = 1 L ( p l v l + ( 1 - p l ) p D l v l ) . The theoretical powers P prop , P Bal , and P opt are calculated using the power formula ( 4 ). The power ratio ( P Bal vs. P prop ) is presented in percent (%).
Table 2 indicates that the total SCC sample sizes from the three methods are generally similar under homogeneous circumstances. For instance, where the full cohort size n = 2,000, the event proportion p D = 10%, the group 1 proportion γ l = 0.3, the log hazard ratio θ = 0.5, and the stratified sub-cohort size = 200, the total SCC sample sizes are 376, 377, and 376 for proportional, balanced, and optimal samplings, respectively. The results show that the power from proportional method P prop is at least equal to or larger than P Bal in all the situations and the power ratio ( P Bal vs. P prop ) has a range from 83% to 100%. These results suggest that, when the event rates are homogeneous over the strata, the proportional sampling is more efficient than the balanced sampling. Furthermore, we observe that the powers from the proportional method and the optimal design remain close, which indicates that, when the event rates are homogeneous and the exposure group 1 proportion γ l is the same over strata, the proportional method is close to the optimal sampling strategy.
Table 2 also provides results for situations with heterogeneous event rates over strata. The set-up is similar to the homogeneous situation, except that the event rates are set to a wide range over strata. Two sets of combination of p Dl ( l = 1, 2, 3, 4) are examined. Set1 gives the values of p Dl to 9%, 30%, 5%, and 20% for the 4 strata and Set2 gives the values of p Dl to 4%, 25%, 10%, and 6% for the 4 strata, respectively. Results in Table 2 indicate that for the given set up and given ñ , in a heterogeneous situation, the total SCC sample sizes from the proportional and balanced methods are similar. The power for these two methods is also similar with slightly more power for the proportional method in most cases in Set1 and Set2. As expected, among all three methods, the optimal design yields the highest theoretical power ( P opt ) with the smallest total SCC sample size. For instance, where the full cohort size n = 2,000, the event proportion p Dl is as in Set1, the group 1 proportion γ l = 0.3, the log hazard ratio θ = 0.5, and the stratified sub-cohort size = 200, the powers ( n scc ) are 0.637 (495) for the proportional, 0.590 (496) for the balanced, and 0.731 (485) for the optimal design. Thus, under the heterogeneous event rate situation, the optimal design indeed provides more powerful test over the other two designs.
Additional simulation studies are conducted to examine whether the sample size formulae for each design produce sufficient power, specifically, for a full cohort size n = 2,000 with 4 strata and overall disease rate p D of 5% (4%, 5%, 4.5%, or 6% over the strata) or 10% (9%, 8%, 11%, or 10% over the strata). The group 1 proportion γ l is set to 0.3 for all strata and the log hazard ratio is set to 0.55 or 0.693.
To target a power of 80% at the significance level of 0.05, we first calculate the sub-cohort size at each stratum ñ l , the stratum sampling proportion p l , the total sub-cohort size ñ , and the total number of subjects in SCC n scc by using the formulae given in Section 4 for each of the proportional, balanced and optimal sampling designs. We then carry out simulations using the derived sample sizes to examine whether the empirical powers achieve the target 80%. The simulation procedure is similar to that for Appendix Table B . The results are summarized in Table 3 . From Table 3 , we observe that the sample sizes calculated from the formulae do provide close to sufficient power empirically in each design. The results in Table 3 also indicate that to achieve the same power, the optimal design gives the smallest sample size among the three designs, the proportional is the second smallest, and the balanced has the largest for all 3 samples. The average sub-cohort size saving of the optimal vs. the balanced approach is approximately 20%.
Sample Size Comparison in Optimal, Proportional, and Balanced Designs
All samples have a full cohort size =2000, group 1 proportion =0.3, significant level α =0.05, and power =80%. n l =size of stratum l in full cohort, v l =proportion of stratum l, p Dl =event proportion in stratum l, θ =log-hazard ratio, p l =sub-cohort sampling fraction in stratum l, ñ (ñ l )=sub-cohort size (at stratum l), n SCC =SCC sample size, NE:E= Non-event : Event in SCC. T =empirical power. The sample size is rounded up to the nearest integer as appropriate.
6. The MORGAM Study
This section presents the MORGAM study [ 23 ] as an example to illustrate the efficiency of a SCC design. The MORGAM study is a multinational collaborative cohort study prospectively followed the development of CHD and stroke events. A total of 4,559 subjects including 2,282 males and 2,277 females were assessed at the baseline visit in 1997; by 2003, ninety-six CHD events were observed in males (CHD incidence p Dl = 0.042) and 24 in females (CHD incidence p Dl = 0.011). The CHD incidence rates differ by gender, and the testing for genotyping is expensive, so a cost-effective SCC design may be needed. The SCC design examines the relationship between the genetic risk factor and the CHD incidence where gender is considered as a stratification factor. The study is designed with 80% power and a 0.05 significance level, and assumes the genetic risk factor frequency is about 0.4 for both the male and the female strata. The full cohort and strata information for this design are displayed in Table 4 .
MORGAM Study Sample Size Calculation
n l =size of stratum l in full cohort, v l =proportion of stratum l, p Dl =event proportion in stratum l, γ l =group 1 proportion, θ =log-hazard ratio, p l =sub-cohort sampling fraction in stratum l, n SCC =SCC sample size, Sub-cohort=sub-cohort size in stratum l, Non-event=number of subjects with non-event in stratum l in SCC, Event=number of subjects with event in stratum l in SCC, NE:E=Ratio of the number of non-events to the number of events. Significant level α = 0.05. Power =80%. The sample size is rounded up to the nearest integer as appropriate.
Assume that a hazard ratio of 2 is to be detected. Note that the minimal detectable hazard ratio based on the entire MORGAM study is 1.9. Table 4 presents the sample size calculation using the proportional, balanced, and optimal sampling methods. Under the optimal (proportional) design, a total of 154 (210) subjects is required for the sub-cohort, 123 (105) of which are from the male stratum and 31 (105) from the female stratum; the total SCC sample size is 269 (325). The balanced design requires a sample size similar to that of the proportional method because of the similar strata proportion v l for the male and the female (i.e., 2,282 subjects are in the male stratum and 2,277 in the female stratum). However, both the proportional and balanced methods require approximate 20% more sub-cohort subjects than the optimal design.
Interestingly, under the optimal design, the sub-cohort size at stratum l is proportional to the ratio of the number of the events at stratum l vs. all events, that is, n ∼ l = D l D n ∼ . For instance, D ninety-six events were observed in the male stratum, which is 80% of the total number of events observed in the full cohort (120). The required sub-cohort size at the male stratum is 123, 80% of the overall sub-cohort size (154).
The non-event vs. event ratio has been examined for all three sampling methods. All methods yield a ratio greater than 1 to ensure the good precision of testing. The optimal method has the smallest overall non-event vs. event ratio of 1.2 among all methods, supporting the conclusion that the optimal method is the most efficient among others.
7. Conclusion and discussion
We have proposed a stratified log-rank type test statistic for the SCC design and provided the power calculation formula. We have investigated the proportional, balanced, and optimal sampling methods, and derived the corresponding sample size calculation formulae. The simulation studies show that the proposed stratified log-rank type test statistic is valid for the finite SCC samples. The simulations also indicate that the power of the SCC design can be fairly high compared with the full cohort when the event rate is low. The empirical power is similar to the theoretical power.
Additional simulation studies have also been conducted to compare the proportional, balanced, and optimal samplings methods. The results show that when the event rates are relatively homogeneous across the strata, the proportional method is superior to the balanced method and is close to the optimal method. However, when the event rates are heterogeneous over the strata, the power for the proportional method is slightly higher than that for the balanced in most of the finite samples. Overall, the optimal method yields the highest power along with the smallest required sample size among all three methods.
Stratified sampling is commonly used in the survey sampling to improve the estimation precision for the population quantity of interest. In some situations, the stratified sampling may be unnecessary but it often leads to the more efficient estimators as compared with the unstratified design, e.g., a more precise estimation of the exposure risk effects, especially when subjects from the same stratum are homogeneous (due to the strong association between the exposure group and the stratum). Furthermore, the stratified design ensures the representation of the small subgroups in the population. When the sampling is stratified, it is natural to consider a stratified test, although an unstratified test statistic can be used when the association between the stratum and the outcome is proportional. Our proposed stratified and nonparametric test statistic naturally accounts for the non-proportionality if it exists.
Our paper only considers the combination of stratified sampling and stratified test when strata in both the design stage and the test stage are the same. In practice, the stratified sampling and the stratified test may be used very differently: when there is a strong association between stratified variable and exposure, the stratified sampling may be used to improve the design efficiency; however, if one believes a strong non-proportional association between failure time and exposure variable across strata, the stratified test needs to be adopted to ensure the test validity. In the Web Appendix III , we use the power formula ( 4 ) to compare the stratified design with the unstratified design analytically. The results show that in general, the stratified design tends to have a higher power than the unstratified design with stratified or unstratified test. Therefore, when both associations are present, it is necessary to take the current approach with both stratified sampling and stratified test. In the situation when the disease proportions or the strata distribution are not available, we suggest to conduct a pilot study to obtain this information before planning a stratified case-cohort study.
The situation becomes more complex when the stratified variable in the design stage is not the same as the stratified variable in the test stage. Generalizing our sample size/power calculation to address this complex situation will be an interesting future study.
Supplementary Material
Supp appendix 01, acknowledgments.
The authors thank Dr. E. Olusegun George for his comments which have led to an improved presentation. This work was partially supported by National Institute of Health grant P01 CA142538 and National Center for Research Resources grant UL1 RR025747.
Contributor Information
Wenrong Hu, Applied Statistics, Department of Mathematical Sciences, The University of Memphis; Department of Biostatistics, CSL Behring.
Jianwen Cai, Department of Biostatistics, School of Public Health, University of North Carolina Chapel Hill.
Donglin Zeng, Department of Biostatistics, School of Public Health, University of North Carolina Chapel Hill.
Click through the PLOS taxonomy to find articles in your field.
For more information about PLOS Subject Areas, click here .
Loading metrics
Open Access
Peer-reviewed
Research Article
A Review of Published Analyses of Case-Cohort Studies and Recommendations for Future Reporting
* E-mail: [email protected]
Affiliation Medical Research Council Epidemiology Unit, University of Cambridge School of Clinical Medicine, Cambridge, United Kingdom
Affiliation École Nationale de la Statistique et de l’Administration Économique Paris Tech, Paris, France
Affiliation Department of Public Health and Primary Care, University of Cambridge School of Clinical Medicine, Cambridge, United Kingdom
Affiliation Medical Research Council Biostatistics Unit, Cambridge Institute of Public Health, Cambridge, United Kingdom
- Stephen J. Sharp,
- Manon Poulaliou,
- Simon G. Thompson,
- Ian R. White,
- Angela M. Wood
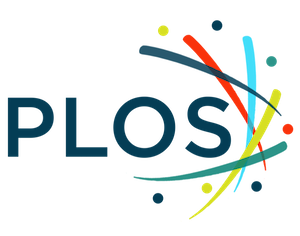
- Published: June 27, 2014
- https://doi.org/10.1371/journal.pone.0101176
- Reader Comments
The case-cohort study design combines the advantages of a cohort study with the efficiency of a nested case-control study. However, unlike more standard observational study designs, there are currently no guidelines for reporting results from case-cohort studies. Our aim was to review recent practice in reporting these studies, and develop recommendations for the future. By searching papers published in 24 major medical and epidemiological journals between January 2010 and March 2013 using PubMed, Scopus and Web of Knowledge, we identified 32 papers reporting case-cohort studies. The median subcohort sampling fraction was 4.1% (interquartile range 3.7% to 9.1%). The papers varied in their approaches to describing the numbers of individuals in the original cohort and the subcohort, presenting descriptive data, and in the level of detail provided about the statistical methods used, so it was not always possible to be sure that appropriate analyses had been conducted. Based on the findings of our review, we make recommendations about reporting of the study design, subcohort definition, numbers of participants, descriptive information and statistical methods, which could be used alongside existing STROBE guidelines for reporting observational studies.
Citation: Sharp SJ, Poulaliou M, Thompson SG, White IR, Wood AM (2014) A Review of Published Analyses of Case-Cohort Studies and Recommendations for Future Reporting. PLoS ONE 9(6): e101176. https://doi.org/10.1371/journal.pone.0101176
Editor: Joel Joseph Gagnier, University of Michigan, United States of America
Received: April 2, 2014; Accepted: June 3, 2014; Published: June 27, 2014
Copyright: © 2014 Sharp, et al. This is an open-access article distributed under the terms of the Creative Commons Attribution License , which permits unrestricted use, distribution, and reproduction in any medium, provided the original author and source are credited.
Data Availability: The authors confirm that all data underlying the findings are fully available without restriction. All relevant data are within the paper and its Supporting Information files.
Funding: SJS was supported by the Medical Research Council www.mrc.ac.uk [Unit Programme number MC_UU_12015/1]. IRW was supported by the Medical Research Council www.mrc.ac.uk [Unit Programme number U105260558]. MP, SGT and AMW were supported by the British Heart Foundation www.bhf.org.uk [grant number CH/12/2/29428]. The funders had no role in study design, data collection and analysis, decision to publish, or preparation of the manuscript.
Competing interests: The authors have declared that no competing interests exist.
Introduction
The case-cohort study design was originally proposed by Prentice [1] . Nested within a larger cohort, the study comprises a random “subcohort” of individuals from the original cohort (sampled irrespective of disease status), together with all cases [ Figure 1 ]. The main advantage of the case-cohort study design over a cohort study is that full covariate data are only needed on the cases and subcohort individuals, not all the original cohort, potentially saving time and money if measures such as biomarkers or genotypes are required. An advantage of a case-cohort study over a nested case-control study is that the same random subcohort can be used as the comparison group for studying different diseases, rather than identifying a new set of controls for each disease. Also, the process of obtaining measurements on baseline samples from individuals in the random subcohort can be initiated at any time after the original cohort has been set up, whereas in a nested case-control study the cases need to be identified before the controls can be defined and the measurement process begin.
- PPT PowerPoint slide
- PNG larger image
- TIFF original image
Included in the study are a subcohort of individuals randomly sampled from the original cohort, together with all incident cases of the disease of interest. Because the subcohort is a random sample from the whole original cohort, it includes some incident cases. The subcohort sampling fraction is the proportion of individuals in the original cohort who are included in the random subcohort, and is defined at the start of the study.
https://doi.org/10.1371/journal.pone.0101176.g001
To make inferences from a case-cohort study, it is necessary to account for the over-representation of cases in the sample. Cox proportional hazards (PH) regression models need to be weighted, with cases outside the subcohort only included in the risk set at the time of their event [1] . Different weighting methods have been described in detail [2] and compared by simulation [3] . The usual standard error estimates from the Cox PH model are not valid in the weighted versions, and should be replaced by alternatives such as a robust jack-knife estimator [4] . Weighted Cox regression models can be fit using standard statistical software packages, including Stata [5] and R [6] .
The STROBE (Strengthening the Reporting of Observational Studies in Epidemiology) Statement is a checklist of 22 items [7] which provides guidance to authors on the reporting of three types of observational study design: cohort, case-control and cross-sectional studies. However, there is currently no published guidance for case-cohort studies. The aim of this work is to review recent practice in reporting of case-cohort studies, and make recommendations to improve the consistency and quality of reporting these studies in the future.
Materials and Methods
Search strategy.
We used the electronic search engines PubMed, Scopus and Web of Knowledge to identify papers reporting analyses of case-cohort studies published between January 2010 and March 2013. We restricted the search to 24 major general medical and epidemiological journals/databases [ Appendix S1 ]. We searched paper titles and abstracts for the keywords “case-cohort” and “case cohort”.
For each paper, we identified the original cohort from which the case-cohort study was constructed, and recorded the number of individuals in the following groups: original cohort, subcohort, total cases, subcohort cases and subcohort non-cases. Where the information was available, we recorded these numbers both before and after exclusion of individuals due to application of specific eligibility criteria for the analysis (e.g. exclusion of individuals with missing values of particular covariates). We recorded whether or not the subcohort was selected by stratified sampling and the stratification factors if it was. For papers where the information was available, we calculated the subcohort sampling fraction as the ratio of the reported size of the subcohort to that of the original cohort, using values before any exclusion criteria were applied. We noted which of the groups of individuals (as defined above) were described using summarized baseline characteristics. We recorded the statistical methods used (and choice of weights, if applicable), whether statistical modelling assumptions were tested, and how missing data were handled in the analysis.
Initial assessment of all papers was carried out by one assessor (MP) and a random selection of 20% of the papers was appraised independently by a second assessor (AW). All discrepancies were resolved by discussion between the two assessors.
Papers included in review
We identified 47 published papers using our search strategy. Fifteen papers were excluded from the review for the following reasons: used the term “case-cohort” incorrectly to describe the study they were reporting (N = 9 papers), reported a meta-analysis of published case-cohort and case-control studies (N = 1), used case-cohort analysis methods even though the data included were not from a case-cohort study (N = 1), discussed specific methods for the design and analysis of case-cohort studies (N = 3), described the protocol for a planned case-cohort study (N = 1). The remaining 32 papers (list of references in Appendix S2 ) were published in eight of the 24 journals/databases considered, with 15 papers published in PLOS ONE. Within the journals covered by this review, the number of published papers reporting case-cohort studies increased between 2010 and 2012 (2010∶5, 2011∶9, 2012∶13), with five papers already published in the first three months of 2013.
Initial cohorts on which case-cohort studies were based
ARIC, EPIC (8 countries), EPIC-Potsdam (one of the centres within EPIC), MONICA/KORA and the Netherlands cohort study were each the original cohort for more than one paper [ Figure 2 ]. Three individual EPIC-Europe centres and two other groupings of EPIC centres were each the original cohort for exactly one paper. Treating each EPIC centre or grouping of centres as a separate cohort, the 32 papers were based on 17 original cohorts. The sizes of each original cohort before and after exclusions (where reported) are shown in Figure 2 . The median size of original cohort before exclusions was 48532 (interquartile range 14610 to 124426). In six papers the size of the original cohort after exclusions was not reported.
Total length of bar represents number before exclusions, length of black bar represent number after exclusions. Where the bar is all black, the size of the original cohort after exclusions was not reported. Where the bar is all grey, there were no exclusions from the original cohort. Bars are labelled according to the number of the paper in the reference list in Appendix S2 .
https://doi.org/10.1371/journal.pone.0101176.g002
Subcohort sampling
The median subcohort sampling fraction was 4.1% (interquartile range 3.7% to 9.1%) [ Figure 3 ]. The subcohort sampling fraction was similar, but not always identical for case-cohort studies based on the same original cohort [ Figure 3 ], which suggests that in some of the papers, exclusion criteria had already been applied to either the original cohort or the subcohort, without these being mentioned in the paper.
Bars are labelled according to the number of the paper in the reference list in Appendix S2 .
https://doi.org/10.1371/journal.pone.0101176.g003
All papers provided a reference to another publication describing the original cohort, and stated that the subcohort was a random sample from the cohort. Nine of the 17 original cohorts used stratified sampling to select the subcohort. The stratifying variables were age, gender, race, centre or a combination of these.
Summarizing baseline characteristics
The papers varied in the groups within which baseline characteristics were summarized, and also whether results of statistical comparisons of characteristics between groups were presented [ Table 1 ]. There was a similar number of examples of summaries within cases/subcohort (11) and cases/non-cases (9); none of 11 papers presented any statistical comparisons between cases and subcohort, while seven of nine papers did present statistical comparisons between cases and non-cases. Where characteristics were summarized within exposure groups, this was most commonly done within the subcohort (nine papers); there were four examples of statistical comparisons being performed between exposure groups. Five of 32 papers provided some quantitative summaries of the characteristics of the original cohort from which the subcohort had been sampled.
https://doi.org/10.1371/journal.pone.0101176.t001
Estimating association between exposure and outcome
All except one paper used some form of Cox regression model to estimate the association between the exposure and disease [ Table 2 ]; the other paper used logistic regression. Of the 31 papers using Cox regression, nine used age as the underlying timescale rather than time in study. Twenty papers specified that a weighted Cox model was used, with 10 using Prentice weights [1] and seven using Barlow weights [2] ; in the other three papers it was unclear which weights had been used. One paper applied an extrapolation approach to recreate the original cohort from the case-cohort sample. Seventeen papers specified that robust standard errors were calculated and 12 reported that the PH assumption was tested. Kaplan-Meier plots of cumulative survival or cumulative incidence functions were presented in five papers, although in two of these papers it was unclear whether estimation of these functions had taken the case-cohort design into account.
https://doi.org/10.1371/journal.pone.0101176.t002
Further aspects of analyses
The four papers based on the EPIC (8 countries) cohort and two papers based on other groupings of EPIC centres, where the subcohort sampling was stratified by centre, described a two-stage approach to reflect the stratified design: first centre-specific models were fit to the data, and second meta-analysis methods were applied to combine the estimates of association across centres. For this second step, five papers used random effects and one used fixed effects meta-analysis.
All the case-cohort studies had individuals with missing values of baseline covariates which were relevant to the analysis being performed; in 27 papers these individuals were excluded from the analysis, while in five papers there was an attempt to include them. In one paper, individuals with missing baseline covariates had their baseline redefined as the first visit with complete data. Four papers described imputation approaches either for the primary or sensitivity analysis.
In this paper we have identified important variability and areas for improvement in the reporting of case-cohort studies in major general medical and epidemiological journals, all of which would be expected to have rigorous statistical review policies. It seems likely that there could be a greater degree of variability and lower quality of reporting in journals with less intensive statistical scrutiny. As with all reviews of this type, deficiencies in reporting do not necessarily imply that the analysis approaches used were inappropriate; however, the findings suggest that some guidance on minimum requirements for reporting these studies could be helpful to authors, journal reviewers and editors. Below, we highlight key aspects of the design and analysis of these studies which should be reported to enable readers to assess the appropriateness of the analyses; these recommendations could be used alongside existing STROBE guidance for reporting observational studies [7] .
Recommendations
Study design..
Having indicated that a paper is reporting results from a case-cohort study, the original cohort study on which the case-cohort study is based should be described and/or referenced. The case definition, methods of case ascertainment, and dates of start and end of follow-up should be provided. The method for selecting the random subcohort and any exclusion criteria that were applied to the analysis, should be stated. If the sampling was stratified, the stratification factor(s) and rationale for using a stratified design should be provided.
Participants.
The numbers of ascertained cases and individuals in the random subcohort should be stated both before and after any exclusion criteria have been applied. The number of individuals in the original cohort should also be provided, ideally both before and after application of the same exclusion criteria. If the size of the original cohort after applying exclusion criteria is unknown because the criteria include, for example, excluding individuals with missing data on a variable that is only measured in the case-cohort sample, then this should be stated explicitly. The subcohort sampling fraction should be presented. If the design was stratified, all the above information should be provided within each stratum. The rationale for choosing a particular sampling fraction should be explained, and reasons given if it differs between strata.
Descriptive information.
Characteristics of study participants, including information on exposures and potential confounders, should be summarized in the usual way using either means and standard deviations, medians and interquartile ranges, or numbers and proportions depending on the type and distribution of the variable. There are various possible groupings of individuals in a case-cohort study for which characteristics could be summarized. If the purpose is to identify variables that are associated with the outcome (i.e. being a case), then characteristics should be summarized in (1) all cases and (2) either all subcohort individuals or all non-cases (i.e. the subcohort excluding cases). If the purpose is to identify variables that are associated with the exposure of interest, then characteristics should be summarized in the subcohort within groups defined by the exposure (groups based on either standard pre-defined cut-offs or quantiles of the exposure distribution in the subcohort). Descriptive information should be presented for the sample included in the analysis after application of exclusion criteria. Consistent with existing STROBE guidance for observational studies [7] , significance tests should be avoided in descriptive tables.
It can be helpful to present some descriptive information (where available) for participants in the original cohort from which the random subcohort was sampled, to enable readers to judge the generalizability of the findings and also to assess the extent to which the subcohort used in the analysis is truly representative of the original cohort.
Statistical methods.
The statistical methods used to estimate the association between exposure and outcome should be stated; for a case-cohort study, methods should appropriately account for the oversampling of cases in the study design. If weights have been used (e.g. for weighted Cox regression), then the weighting method and rationale for its choice should be given. In particular, if Barlow weights have been used, the subcohort sampling fraction should be stated explicitly, since the inverse of the sampling fraction is used to weight subcohort non-cases and cases in the subcohort before they become a case [2] . If the sampling fraction has been calculated as the subcohort size after exclusions as a proportion of the original cohort before exclusions, then the potential impact of using this value in the Barlow-weighted analysis should be explored in sensitivity analyses, which should be described and discussed.
If some form of Cox regression model has been used, the proportional hazards assumption should be assessed for each covariate in the analysis. Appropriate methods for assessing this assumption include fitting and testing interactions between covariates and the underlying analysis timescale, or using a correlation test based on Schoenfeld residuals [8] ; an extended version of the Schoenfeld residuals test has been proposed for weighted Cox models [9] .
If a stratified sampling design has been used, then a description of how the stratifying factor(s) was accounted for in the analysis should be given. Potential approaches include stratifying the baseline hazard function by the relevant factor(s), fitting separate analysis models within each stratum and combining stratum-specific estimates of association using meta-analysis [10] , or using the methods for analysing stratified case-cohort designs described by Borgan et al [11] .
Existing STROBE guidance [7] recommends the use of Kaplan-Meier plots for a cohort study; these can also be helpful for presenting results of a case-cohort study, although Kaplan-Meier estimates need to take into account the oversampling of cases in this design [9] .
Further considerations
Most of the papers identified in our review excluded individuals with missing covariate data. This approach results in a loss of efficiency and only gives unbiased estimates if missingness can be assumed to be independent of outcome, conditional on observed covariates [12] . Some papers attempted imputation approaches, but further research is needed into how the case-cohort design should be accounted for in the imputation model, before specific recommendations can be made.
The main focus of our review was on the use of case-cohort studies to estimate associations between an exposure and an outcome, rather than to develop and evaluate risk prediction models (although such papers were not excluded from the scope). Following recent publication of two papers describing adaptations to the case-cohort setting of standard measures of risk prediction [13] , [14] , it seems likely that more papers on risk prediction will appear in future. Our recommendations for reporting (above) would still apply; a clear description of the subcohort sampling fraction and how it was calculated would be particularly important given its pivotal role in these methods.
Despite the fact that the case-cohort design was first proposed nearly 30 years ago, it is still relatively uncommon compared with other observational study designs, and specific issues related to design and analysis are likely to be less well known to the majority of researchers. Our review suggests that in recent years the use of case-cohort studies appears to be increasing, and therefore we hope our recommendations, which are summarized in Table 3 , will help authors, reviewers and editors to achieve greater consistency and quality in how they are reported in the future.
https://doi.org/10.1371/journal.pone.0101176.t003
Supporting Information
Appendix s1..
List of journals/databases included in the literature search.
https://doi.org/10.1371/journal.pone.0101176.s001
Appendix S2.
References of 32 papers included in review.
https://doi.org/10.1371/journal.pone.0101176.s002
Author Contributions
Conceived and designed the experiments: AMW SJS SGT IRW. Performed the experiments: AMW MP. Analyzed the data: MP AMW SJS. Contributed to the writing of the manuscript: SJS AMW SGT IRW.
- View Article
- Google Scholar
- 6. Ploner M, Heinze G (2013) coxphw: Weighted Cox regression. R package version 2.13. http://CRAN.R-project.org/package=coxphw .
- 8. Collett D (2003) Modelling survival data in medical research. Chapman & Hall/CRC Texts in Statistical Science Second Edition.
Sample size/power calculation for stratified case-cohort design
Affiliation.
- 1 Applied Statistics, Department of Mathematical Sciences, The University of Memphis, Memphis, TN, U.S.A.; Department of Biostatistics, CSL Behring, King of Prussia, PA, U.S.A.
- PMID: 24889145
- PMCID: PMC4159408
- DOI: 10.1002/sim.6215
The case-cohort (CC) study design usually has been used for risk factor assessment in epidemiologic studies or disease prevention trials for rare diseases. The sample size/power calculation for a stratified CC (SCC) design has not been addressed before. This article derives such result based on a stratified test statistic. Simulation studies show that the proposed test for the SCC design utilizing small sub-cohort sampling fractions is valid and efficient for situations where the disease rate is low. Furthermore, optimization of sampling in the SCC design is discussed and compared with proportional and balanced sampling techniques. An epidemiological study is provided to illustrate the sample size calculation under the SCC design.
Keywords: case-cohort design; power calculation; sample size; sampling technique; stratified case-cohort design.
Copyright © 2014 John Wiley & Sons, Ltd.
Publication types
- Research Support, N.I.H., Extramural
- Cohort Studies
- Computer Simulation
- Coronary Disease / epidemiology*
- Epidemiologic Research Design*
- Likelihood Functions
- Rare Diseases / economics
- Rare Diseases / epidemiology*
- Risk Assessment / methods*
- Sample Size
- Stroke / epidemiology*
Grants and funding
- R01 CA082659/CA/NCI NIH HHS/United States
- UL1 TR001111/TR/NCATS NIH HHS/United States
- R37 GM047845/GM/NIGMS NIH HHS/United States
- UL1 RR025747/RR/NCRR NIH HHS/United States
- P01 CA142538/CA/NCI NIH HHS/United States
- Journal home
- Advance online publication
- About the journal
- J-STAGE home
- Annals of Clinical Epidemiolog ...
- Volume 4 (2022) Issue 2
- Article overview
Department of Health Services Research, Faculty of Medicine, University of Tsukuba Faculty of Epidemiology and Population Health, London School of Hygiene and Tropical Medicine
Tokyo University of Science, Department of Information and Computer Technology
Corresponding author

2022 Volume 4 Issue 2 Pages 33-40
- Published: 2022 Received: - Available on J-STAGE: April 04, 2022 Accepted: - Advance online publication: - Revised: -
(compatible with EndNote, Reference Manager, ProCite, RefWorks)
(compatible with BibDesk, LaTeX)
Matching is a technique through which patients with and without an outcome of interest (in case-control studies) or patients with and without an exposure of interest (in cohort studies) are sampled from an underlying cohort to have the same or similar distributions of some characteristics. This technique is used to increase the statistical efficiency and cost efficiency of studies. In case-control studies, besides time in risk set sampling, controls are often matched for each case with respect to important confounding factors, such as age and sex, and covariates with a large number of values or levels, such as area of residence (e.g., post code) and clinics/hospitals. In the statistical analysis of matched case-control studies, fixed-effect models such as the Mantel-Haenszel odds ratio estimator and conditional logistic regression model are needed to stratify matched case-control sets and remove selection bias artificially introduced by sampling controls. In cohort studies, exact matching is used to increase study efficiency and remove or reduce confounding effects of matching factors. Propensity score matching is another matching method whereby patients with and without exposure are matched based on estimated propensity scores to receive exposure. If appropriately used, matching can improve study efficiency without introducing bias and could also present results that are more intuitive for clinicians.
Matching is mainly used in observational studies, including case-control and cohort studies. Matching is a technique by which patients with and without an outcome of interest (in case-control studies) or patients with and without an exposure of interest (in cohort studies) are sampled from an underlying cohort to have the same or similar distributions of characteristics such as age and sex.
The main purpose of matching is to increase study efficiency for data collection and subsequent statistical analysis. Matching helps researchers reduce the volume of data for collection without much loss of information (i.e., improving cost efficiency) and obtain more precise estimates than simple random sampling of the same number of patients (i.e., improving statistical efficiency). In addition, in cohort studies, matching can remove or reduce confounding effects of matching factors.
This paper aims to introduce basic principles of matching in case-control and cohort studies, with some recent examples.
Fig. 1 Graphical representation of cumulative incidence sampling (A), case-control sampling (B), and risk set sampling (C) for 10 example patients in a cohort. ● indicates an outcome onset and time at selection as a case. ○ indicates time at selection as a control.
1. Wacholder S. The case-control study as data missing by design: estimating risk differences. Epidemiology 1996;7:144–150.
2. Noma H, Tanaka S. Analysis of case-cohort designs with binary outcomes: improving efficiency using whole-cohort auxiliary information. Stat Methods Med Res 2017;26:691–706.

Fig. 2 Graphical representation of a risk set sampling for 10 example patients in a population-based cohort. ● indicates an outcome onset and time at selection as a case. ○ indicates time at selection as a control.

In a study requiring primary data collection, case-control study designs are efficient because only information on cases and selected controls, instead of all people in the underlying cohort, is collected and used for statistical analysis. Especially for rare outcomes, a cohort study recruiting many people to observe a sufficient number of outcomes is not feasible. However, a case-control design would still be feasible, with reduced costs and efforts.
3. Schuemie MJ, Ryan PB, Man KKC, Wong ICK, Suchard MA, Hripcsak G. A plea to stop using the case-control design in retrospective database studies. Stat Med 2019;38:4199–4208.
4. Schneeweiss S, Suissa S.Discussion of Schuemie et al. “A plea to stop using the case-control design in retrospective database studies”. Stat Med 2019;38:4209–4212.
Similar to cohort studies, case-control studies typically require confounder adjustment using stratified analysis or regression modeling. To further improve statistical efficiency in adjusted analyses, case-control studies may match controls on confounders to be adjusted for, i.e., sampling a control(s) with an identical (or nearly identical) value of confounders for each case. When the total number of cases and controls to be sampled is fixed, the adjusted odds ratio estimates are likely to be less variable (i.e., more statistically efficient) in case-control data matched on strong confounders than in unmatched data.
Besides common confounding factors such as age and sex, area of residence (e.g., post code) or clinics/hospitals (which patients are registered to or visit) are sometimes matched between cases and controls. If variables with a large number of values or levels (e.g., over 1,000 post codes or clinics/hospitals) are adjusted for as “surrogate” confounders in the statistical analysis, at least one case and one control in each area (or clinic/hospital) are needed; otherwise, the data are discarded in the fixed-effect models (stratification). Although a case and control may rarely come from the same area (or clinic/hospital) in unmatched case-control sampling, matching can ensure that the pairs (or sets) of cases and controls are derived from the same area (or clinics/hospitals). Consequently, the odds ratio adjusted for these variables can be efficiently estimated.
5. Rothman KJ, Lash TL. 6 Epidemiologic study design with validity and efficiency considerations. Modern epidemiology 4th edition. Lippincott Williams & Wilkins, 2021:105–140.
A structured process for the validation of a decision-analytic model: application to a cost-effectiveness model for risk-stratified national breast screening
- Original Research Article
- Open access
- Published: 16 May 2024

Cite this article
You have full access to this open access article
- Stuart J. Wright ORCID: orcid.org/0000-0002-4064-7998 1 ,
- Ewan Gray ORCID: orcid.org/0000-0003-3840-5268 2 ,
- Gabriel Rogers ORCID: orcid.org/0000-0001-9339-7374 1 ,
- Anna Donten ORCID: orcid.org/0000-0002-4896-6002 1 &
- Katherine Payne ORCID: orcid.org/0000-0002-3938-4350 1
204 Accesses
Explore all metrics
Decision-makers require knowledge of the strengths and weaknesses of decision-analytic models used to evaluate healthcare interventions to be able to confidently use the results of such models to inform policy. A number of aspects of model validity have previously been described, but no systematic approach to assessing the validity of a model has been proposed. This study aimed to consolidate the different aspects of model validity into a step-by-step approach to assessing the strengths and weaknesses of a decision-analytic model.
A pre-defined set of steps were used to conduct the validation process of an exemplar early decision-analytic-model-based cost-effectiveness analysis of a risk-stratified national breast cancer screening programme [UK healthcare perspective; lifetime horizon; costs (£; 2021)]. Internal validation was assessed in terms of descriptive validity, technical validity and face validity. External validation was assessed in terms of operational validation, convergent validity (or corroboration) and predictive validity.
The results outline the findings of each step of internal and external validation of the early decision-analytic-model and present the validated model (called ‘MANC-RISK-SCREEN’). The positive aspects in terms of meeting internal validation requirements are shown together with the remaining limitations of MANC-RISK-SCREEN.
Following a transparent and structured validation process, MANC-RISK-SCREEN has been shown to have satisfactory internal and external validity for use in informing resource allocation decision-making. We suggest that MANC-RISK-SCREEN can be used to assess the cost-effectiveness of exemplars of risk-stratified national breast cancer screening programmes (NBSP) from the UK perspective.
Implications
A step-by-step process for conducting the validation of a decision-analytic model was developed for future use by health economists. Using this approach may help researchers to fully demonstrate the strengths and limitations of their model to decision-makers.
Similar content being viewed by others
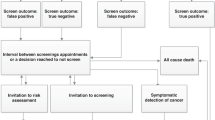
The cost-effectiveness of risk-stratified breast cancer screening in the UK
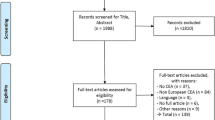
Cost effectiveness of breast cancer screening and prevention: a systematic review with a focus on risk-adapted strategies
Cost-effectiveness models in breast cancer screening in the general population: a systematic review.
Avoid common mistakes on your manuscript.
1 Introduction
A suite of recommendations have been developed in the healthcare context which are designed to enable decision analysts to have a structured approach to developing, building and appraising the quality of decision-analytic models [ 1 , 2 , 3 , 4 , 5 , 6 ]. A crucial element in these recommendations is the need for validation [ 7 ]. Enabling decision-makers’ trust and confidence, by conducting a systematic and transparent process of validation, is a vital component that decision analysts should take seriously so the decision-analytic model has sufficient credibility [ 6 ]. A fundamental component supporting the process of validation is the need for transparency in the decision-analytic model structure and use of data. Simplistically, transparency can be achieved by using open-source programming languages, such as R, and making the code public [ 8 , 9 , 10 ]. This level of transparency is necessary, but not sufficient, to enable the informed use of decision-analytic models to guide resource allocation decisions. The process of validation for a published decision-analytic model should also be transparent.
There are numerous recommendations and guidelines suggesting the need for, and approaches to, decision-analytic model validation. Such recommendations and guidelines, for example, the Technical Verification (TECH-VER) and Assessment of the Validation Status of Health-Economic Decision Models (AdViSHe) checklists, have been produced by small groups of individual researchers (of note, for example, McCabe and Dixon) or groups of researchers, reaching consensus or making task forces as part of international societies such as the International Society for Pharmacoeconomics and Outcomes Research [ 6 , 11 , 12 , 13 ]. There are, however, correspondingly few publications that explicitly report the steps to completing model validation [ 14 ].
A particular clinical area where model validation may be valuable is in the evaluation of cancer screening models. Such models can be very complex, incorporating natural history models, which explain how cancers grow and spread over time. Changes to cancer screening programmes can have implications for large numbers of individuals, so ensuring that the assumptions and predictions of cancer screening models are correct is particularly important for decision-makers. In the UK, in 2023, the current national breast cancer screening programme (NBSP) invites women, via a letter sent to their home address, to have a mammogram that is then repeated every 3 years. The current eligible age-range for the UK-NBSP starts within 3 years of a woman reaching their 50th birthday up to the age of 70 years (inclusive) [ 16 ]. This means that in the UK over 2 million women attend breast cancer screening annually.
The aim of breasts cancer screening is to identify cancers at an earlier stage, making them more treatable [ 15 ]. However, there are harms to screening, including the risk of false-positive results and overdiagnosis of cancers which would never have grown to a size which would have caused harm to the woman. Risk-stratified national breast screening programmes (NBSP) are being suggested as a potential adaptation to existing programmes that offer a mammogram (X-ray of the breast) to all women in a selected age group. The approach to a NBSP can vary in terms of the age at which screening is first offered to women in the population (NBSP starting age), interval between screenings (NBSP screening interval), age at which screening is stopped (NBSP stopping age), number of X-rays used (one- or two-view mammography), supplementary screening technologies used (ultrasound and/or magnetic resonance imaging) and interpretation of the X-ray (manual or digital).
Factors known to influence a women’s 10-year risk of developing breast cancer have been used to develop risk prediction models developed in various formats and with criteria to categorise woman into specified risk groups [ 17 , 18 , 19 , 20 , 21 , 22 , 23 , 24 , 25 ]. For example, the Tyrer–Cuzick risk calculator asks women to record their age, weight and height (to calculate body mass index), age at menarche, obstetric history, age at menopause (if applicable), history of a benign breast condition that increases breast cancer risk, history of ovarian cancer, use of hormone replacement therapy and family history (including breast and ovarian cancer, Ashkenazi inheritance, BRCA 1 and 2 genetic testing) [ 26 , 27 ]. The ‘score’ from this risk-calculator may then be used to categorise a women into pre-defined risk categories, such as population-average-level risk (10-year risk of 2 to < 3%); low (below average) risk (10-year risk of < 2%); above-average risk (10-year risk of 3 to < 5%); moderate risk (10-year risk of 5 to <8%); high risk (10-year risk of ≥ 8%) [ 28 ]. When a woman’s risk of breast cancer has been estimated, the intensity of screening can be altered for women in different risk categories. The frequency of screening can be increased for those at higher risk to find more cancers at an earlier stage, improving treatment outcomes. For women at low risk of cancer, the frequency of screening can potentially be reduced to decrease the degree of overdiagnosis and false-positive results in this group while also saving healthcare resources. A risk-stratified breast cancer screening programme may therefore be able to improve the balance of the benefits and harms of screening while not requiring a significant increase in the number of screens. To date, there are no examples of a risk-stratified NBSP used in practice, but there is consensus about the need for different types of evidence to support their introduction [ 29 ].
Generating trial-based clinical evidence of the effectiveness of a risk-stratified NBSP compared with existing approaches to NBSP is neither feasible nor perhaps desirable due to the inherent limitations of the necessary follow-up and the challenges of including women from different risk groups. Within this context, and in keeping with the predominant view, economic evidence needed to understand the potential value of risk-stratified NBSP should come from decision-analytic-model-based analyses using appropriate methods that answer the specified decision problem [ 30 , 31 , 32 , 33 ]. Tappenden and Chillcott suggest the need to include a process involving cycles of model checking and validation [ 34 ] mirroring the recommendations for an iterative approach towards a definitive evidence base made by Sculpher and colleagues [ 35 ].
This study aimed to design a structured process to update and then assess the internal and external validity of a decision-analytic model. This structured approach to model validation was then applied to update and validate a case study model structured to estimate the incremental healthcare costs and health consequences of exemplar risk-stratified national breast screening programmes (NBSP) in the UK [ 36 ]. The resulting outputs of the validated model (called MANC-RISK-SCREEN) reporting the healthcare costs and health consequences of a risk-stratified NBSP compared with universal triyearly screening, universal biyearly screening or no screening in the UK setting will be published separately in a follow-up paper.
A pre-defined set of steps were used to conduct the process of validation of a published decision-analytic-model-based cost-effectiveness analysis [ 36 ]. This early economic evaluation sought to estimate the cost-effectiveness of different breast cancer screening strategies in the UK, including risk-based approaches. This study was reported in detail in the original paper, but the model code was not made publicly available, and the predictions of the model were not explored. There is growing interest in risk-based breast cancer screening in the UK, and as such, a full update and validation of this model was conducted to provide decision-makers with transparent information about the strengths and weaknesses of the model.
There are numerous, and inconsistent, definitions of the process of decision-analytic model validation [ 13 ]. This study therefore took a pragmatic approach to describe the required steps of validation that are needed (in a normative sense) to enable a transparent description of the process. The contribution of individual recommendations, identified in the extant literature, used to inform the discrete steps of the process of decision-analytic model validation are cited where relevant in the sections that follow.
2.1 Description of the original decision-analytic model
The original decision-analytic model reported in Gray et al. (2015) [ 36 ], the focus for this validation process, was developed to address the decision problem: “What are the key drivers of the incremental costs and benefits of example stratified breast screening programs compared with the current National Breast Cancer Screening Program?” Gray and colleagues conducted an early economic analysis. The key characteristics of the ‘Gray’ decision-analytic model, a discrete event simulation, are outlined in Table 1 . Further details regarding the Gray model can be found in Appendix 1 .
2.2 The components of the model validation process
The validation process aimed to explore the degree of internal and external validity. Internal validation has been described in terms of three criteria [ 6 , 37 ]: descriptive validity, to assess whether the degree of simplification used in the decision-analytic model structure still adequately represents the natural history of the specified disease and/or pathways of care; technical validity, to assess whether the decision-analytic model was appropriately programmed to produce the intended outputs from the specified inputs; and face validity, to assess whether the decision-analytic model produces outputs consistent with theoretical basis of disease and the intervention [ 12 ]. External validation can be described in terms of three criteria: operational validation, to assess whether the outputs produced by the decision-analytic model are sufficiently accurate; convergent validity (or corroboration), to compare the decision-analytic model with other published approaches addressing a similar decision problem; and predictive validity, to assess whether the outputs produced by the model sufficiently represent outputs from alternative sources. For the purpose of validation, the following six steps, broken down by criterion, were carried out.
2.2.1 Face Validity
Face validity refers to whether the decision-analytic model is measuring what is intended—in this case whether the model structure and parameter values produce outputs that are clinically credible. Face validity is a type of internal validity that captures first-order validation as defined by Haji Ali Afzali and colleagues [ 38 ]. The process of assessing face validity is often intuitive and subjective in that it requires value judgements to be made by the decision analyst. These value judgements require the decision analyst to be explicit about the criteria used when assessing face validity. There are no available published criteria to assess the face validity of a decision-analytic model. Assessing face validity was, therefore, reliant on the team of decision analysts, with input from relevant clinical expertise, producing an adequate explicit description of whether, and how, the outputs are consistent pre-defined elements (description of the intervention and comparators, assignment to risk categories, natural history of cancer, treatment of cancer by disease stage) for a decision-maker to assess the credibility of the decision-analytic model in this regard. We assessed whether sufficient face validity had been achieved by presenting the results to a group of experts in breast screening [ 39 ]. The threshold for face validity of the model was the agreement by a consensus group of stakeholders involved in the implementation of breast cancer screening that the model represented a close approximation of reality.
2.2.2 Descriptive Validity
Descriptive validity has been viewed as being synonymous with the model conceptualisation process [ 2 ] and ensuring the model structure and pathways being represented are adequate while recognising that all models are a simplification of reality. The process of understanding the degree of descriptive validity has also been referred to as conceptual validation as part of published criteria ‘Assessment of the Validation Status of Health-Economic decision models (AdViSHE)’ for assessing model validation to assess: ‘whether the theories and assumptions underlying the conceptual model … are correct and the models representation of the problem entity and the models’ structure, logic and mathematical and causal relationships are ‘reasonable’ for the intended purpose of the model’ [ 11 ]. Assessing descriptive validity was a subjective process and required ‘expert’ input from people with relevant knowledge of the disease and intervention being represented by the decision-analytic model and supported by people with relevant technical expertise in decision-analytic modelling. Similar to the application of survey-based consensus methods, such as Delphi [ 40 ], it is also necessary to have a clear threshold of what is a ‘sufficient’ level of ‘descriptive validity’, which required taking account of the purpose of the decision-analytic model (the decision problem). We assessed whether sufficient descriptive validity had been achieved when all experts in risk-stratified breast screening involved in providing input agreed the model structure was appropriate.
2.2.3 Technical Verification
Technical verification is a type of internal validity that captures second-order validation [ 38 ] and involves a debugging process and assessment of the accuracy of the decision-analytic model in terms of inputs creating ‘valid’ outputs. Technical verification essentially answers the question: does the decision-analytic model do the calculations correctly? The process of completing technical verification was supported by following a published verification checklist designed to ‘reduce errors in models and improve their credibility’ called Technical Verification (TECH-VER) [ 12 ]. The TECH-VER checklist is a highly detailed list of steps to be used by decision analysts to reduce the chance of errors in coding the model structure and calculating data inputs from external data sources (e.g. generating measures of overall survival). The TECH-VER checklist does not generate an overall score of technical validity but relies on a decision analyst describing which criteria are relevant and have been met with a description of how. A decision analyst external to the core research team was employed to complete technical verification and produce a TECH-VER report. We assessed whether sufficient technical verification had been achieved using the TECH-VER report from this independent expert. The model was deemed to meet the threshold for technical validity if, following technical verification, there were no remaining issues which would affect the potential ordering of strategies in terms of their cost-effectiveness.
2.2.4 Operational Validation
The process of assessing operational validation is, perhaps, the one most readily interpreted, using lay terms, as assessing ‘external’ validity. Haji Ali Afzali and colleagues [ 38 ] call this third-order validation. Operational validation involves comparing decision-analytic model outputs using different sources inputs that may come from (i) data that were used in the original model (dependent operational validation) or (ii) data identified from alternative sources (independent operational validation) [ 11 ]. The operational validation of MANC-RISK-SCREEN involved determining whether the clinical outputs of the model aligned with an external data source (independent operational validation). Intuitively, independent operational validation is more robust, in terms of assessing operational validation, than dependent operational validation. However, both independent and dependent validation have key roles when assessing external validity. The threshold for external validity would be deemed to have been met if it was not possible to change the input parameters to improve the fit of given outputs (for example, cancer incidence by age or distribution of cancer stages) to external data without worsening the fit of other output categories.
2.2.5 Predictive Validity
In the context of decision-analytic models, predictive validation is about understanding how well the analysis has predicted future events [ 38 ]. We employed the interpretation of predictive validity offered by Gray and colleagues as a process to test the impact on outputs when more data have become available. In this way it was possible to see whether the decision-analytic model had predicted future events [ 41 ]. The degree of congruence between predicted and ‘actual’ (future) events was assessed qualitatively.
2.2.6 Cross-Validation
Cross-validation (our preferred term) is also referred to as assessing convergent validity. Cross-validation is used to assess whether two different decision-analytic models designed to address the same decision problem produce similar results. This process requires an alternative decision-analytic model that addresses a similar decision problem to be available. It is most commonly applied for decision-analytic models that have multiplicative purposes rather than in instances when a bespoke structure has been created for a single decision problem. A well-established process of assessing convergent validity has been set up by the Mount Hood challenge for decision-analytic models in the area of diabetes [ 7 ]. Convergent validity is also a descriptive process in which the decision analyst should outline the ways in which different decision-analytic models are the same. We conducted a rapid review of the PubMed database up to the year 2022 to identify relevant alternative models looking at risk-based breast screening in the UK. Models were selected based on the similarity of their participants, interventions, comparators, and outcomes (PICO). Differences between different decision-analytic models in terms of the outputs produced were identified. Where the degree of convergent validity could not be directly compared due to variations in the PICO, the results were assessed qualitatively.
2.3 Completing the Model Validation Process
A team of six health economists, supported by an external expert in building decision-analytic models for national decision-making bodies, conducted the model validation process. The first meeting between the six health economists set the thresholds for when the model validation process was sufficient. There were discrete thresholds for each component of model validation, which are described in each relevant section. This team of health economists worked closely with a national group of experts in breast screening as part of the process assessing, in particular, face and descriptive validity [ 39 ]. The process of model validation involved going through each component part of validation in a stepwise manner. The external expert completed the TECH-VER process. At the end of the model validation process, two published checklists were completed: TECH-VER and AdViSHE.
This section describes the results from the validation of the Gray model. Following model updates and validation, the Gray model was named ‘MANC-RISK-SCREEN’. The TECH-VER
checklists and AdViSHE checklists are reported in Supplementary Appendices 2 and 3, respectively. All code and documentation relating to MANC-RISK-SCREEN are located on GitHub (see https://github.com/stuwrighthealthecon/MANC-RISK-SCREEN ). GitHub is an online site designed to share software and model code [ 42 ].
3.1 Validation of MANC-RISK-SCREEN
The development and validation, together with the independent assessment process, of the original model by Gray started in February 2021. The version of the decision-analytic model called MANC-RISK-SCREEN was produced in June 2022. The process of updating and validating the decision-analytic model took place in discrete steps:
Independently reproducing the original Gray decision-analytic model to check for errors and identify areas for improvement;
Updating the decision-analytic model to finalise the structure of the decision-analytic model;
Updating input parameters from the Gray decision-analytic model to produce MANC-RISK-SCREEN;
Checking the face validity of MANC-RISK-SCREEN with experts in breast screening;
Checking the descriptive validity MANC-RISK-SCREEN with experts in breast screening;
Conducting independent technical verification of MANC-RISK-SCREEN;
Operational validation of MANC-RISK-SCREEN;
Assessing the predictive validity of MANC-RISK-SCREEN for specified targets;
Cross-validation of MANC-RISK-SCREEN.
These steps were performed in sequence. The steps addressed each of the components of the model validation process.
3.2 Reproducing the Original Model
The decision-analytic model was re-built by a health economist (Stuart Wright) not involved in the design and conduct of the original decision-analytic model built by Gray and colleagues. The health economist (SW) first read the original R code, including accompanying functions, and wrote a text-based algorithm (see the documentation folder of the GitHub repository) in Microsoft Word explaining the steps taken in each stage of the model to conduct the analysis. This text-based algorithm was then checked by the lead modeller in the early economic evaluation (EG), who clarified any areas of confusion.
The health economist (SW) then used the text-based algorithm to reconstruct the model in a new R script, and this script was then compared with the original to detect potential errors in both model versions. Only two significant errors (that could influence the estimated cost-effectiveness) were identified in the original Gray model during this process. To determine whether a cancer was screen detected, a random number was drawn and compared with the value of a variable representing the proportion of cancers that are screen detected in the health system. In the original code, the cancer was assigned to be a screen-detected cancer if the random number was greater than the value of the variable. However, this should only have occurred if the random number was lower than the value of the variable and was changed in the updated model. As the value of this variable was set to 0.5 and not varied in the probabilistic sensitivity analysis, this error had not had an impact on the published early economic analysis results [ 36 ]. In addition, the original Gray model did not include a cost of follow-up testing for false-positive screening results, thereby potentially overestimating the cost-effectiveness of strategies with more frequent screening. Therefore, the cost of follow-up testing for false-positive screening was added to the R code for MANC-RISK-SCREEN.
During the recoding process, other changes to the original Gray model were made for improvements in the speed of execution, for example, defining variables before loops rather than in them, or, cosmetically, in making the code more readable. An example of the latter was the inclusion of four required R functions in a single accompanying script rather than four individual ones.
3.3 Structural Update
When MANC-RISK-SCREEN was built in R, structural changes and additional features were included. A key change was that the categorisation of breast tumours was changed from Nottingham Prognostic Indicator (NPI)-based classification to a stage-based classification, as this significantly increased the availability of relevant data for key input parameters. The start age of the screening was changed to a fixed age of 50 years rather than uniformly varying between 47 and 51 years. This change was made because the varying start age had only been applied to some of the strategies in the early model and was deemed to potentially bias the results.
The original Gray model was populated with individual-level data for a population of women aged between 50 and 70 years ( n = 53,596) recruited to a cohort study in England called Predicting Risk of Cancer at Screening (PROCAS) 1 to provide estimates of the distribution of estimated 10-year risk of breast cancer [ 24 ]. The MANC-RISK-SCREEN model was populated with updated data from a second cohort study in England called PROCAS 2 that recruited 15,613 women aged between 50 and 70 years [ 25 ]. These estimates were calculated in the original cohort studies using an adaptation of the Tyrer–Cuzick risk assessment tool. The Tyrer–Cuzick risk calculator was modified into a two-page survey to collect the information required to calculate individual 10-year breast cancer risk: family history information (including number and ages of sisters, current age or age at death of mother and details of any relatives affected by breast or ovarian cancer), hormonal risk factors (age at menarche, menopausal status and hormone replacement therapy use and parity) and lifestyle information [current body mass index (BMI), BMI at age of 20 years, clothing size, alcohol consumption and exercise habits) [ 24 ]. The calculated risk scores in the cohort study sample were, for the purpose of the intervention arm in the decision-analytic model, divided into three risk categories: 10-year risk < 3.5%, directed to triyearly screening; 10-year risk between ≥ 3.5 and < 8%, directed to biyearly screening; and 10-year risk ≥ 8%, directed to annual screening. Changes in the risk groups used in recently published clinical research meant that in the updated model the risk thresholds used to define the different risk groups have changed [ 25 ]. In the MANC-RISK-SCREEN model, moderate risk is defined as 5–8%, rather than 3.5–8% as in the PROCAS-based strategies. This also means that normal risk is defined as less than 5% in the first PROCAS strategy. In the second risk-based strategy with less frequent screening for women at lower risk, normal risk is now defined as a 10-year risk between 1.5 and 5%.
Parameters relating to three types of imperfect uptake for risk-stratified screening were added: uptake for risk prediction (do the clinicians use the risk-prediction tool?), uptake for receipt of risk prediction (do women get their individual risk level?) and uptake for changed screening intervals (do women decide to change their screening interval?). A number of additional screening strategies were added to the model, including reduced (every five or six years) screening for women at low (below-average) 10-year risk of breast cancer, and a fully stratified screening programme with more frequent screening for those at higher risk and less frequent screening for those at lower risk.
3.4 Update Input Parameters
The original early analysis was published by Gray in 2017, and the decision-analytic model validation process was started in early 2021. This time period meant it was likely that values of the parameters used in the Gray decision-analytic model were likely to be out of date. A comprehensive update of decision-analytic model inputs for MANC-RISK-SCREEN was conducted. The process of updating the input parameters is provided in detail in the documentation folder in the model GitHub repository. Systematic reviews were conducted (by AD and RH) to identify more recent health utility and cost estimates by breast cancer stage. The cost of stratification was updated to incorporate estimates from a published micro-costing study [ 43 ]. New values for screening-related parameters were identified from published audits and reports on the status of the National Health Service (NHS) breast cancer screening programme [ 15 ]. Studies citing the sources of clinical parameters, including the tumour growth model, were searched to determine whether any newer appropriate values were available.
Following the search for new parameter values, the following parameters were updated in the final MANC-RISK-SCREEN model: the proportion of cancers detected by screening, all-cause mortality, cancer-stage-specific mortality, breast cancer incidence by age, breast cancer risk, the proportion of cancers that are ductal carcinoma in situ, the proportion of cancers diagnosed at different stages based on their size, mammographic sensitivity by Volpara breast density group, screening recall rate, all costs in the model and utility values for stage I–III and IV breast cancer.
3.5 Face Validity
Following the reconstruction and parameter update, preliminary results from MANC-RISK-SCREEN were presented at a close-out meeting of the research programme (called PROCAS 2) funding the validation process [ 25 , 39 ]. This meeting was attended by 58 individuals (38 face-to-face and 20 virtual) with relevant expertise in breast screening from academic, clinical and/or policy-making perspectives.
Two suggestions from this meeting were to include uptake for risk stratification and screening attendance. These two parameters were subsequently added to the original Gray decision-analytic model. Data on screening uptake, reflecting the correlation between an individual’s previous and future attendance, were sourced from the annual UK breast cancer screening report [ 15 ]. Parameters relating to an individual’s uptake of risk prediction, the feedback of their risk information and the changing of their screening intervals were added to the MANC-RISK-SCREEN model. In the current model iteration, it is assumed that uptake for risk prediction is perfect, but the impact of imperfect uptake for risk prediction on the cost-effectiveness of a risk-stratified NBSP will be explored in future work. Further suggestions to extend the decision-analytic model to estimate the cost-effectiveness of adding single-nucleotide polymorphisms (SNPs) to the risk stratification strategy and of adding in the impact of starting women at high risk of breast cancer on preventive medicines are topics for further development of MANC-RISK-SCREEN.
3.6 Descriptive Validity
The descriptive validity of the model was assessed on a continual basis by monthly meetings between the six health economists involved in the validation of MANC-RISK-SCREEN. Two of these health economists (SW and KP) directly interacted with two clinical experts in risk-based breast screening, a statistician involved in generating the risk-prediction model underpinning the Tyrer–Cuzick algorithm and a health psychologist involved in assessing uptake as part of the PROCAS 2 programme.
These supporting researchers were consulted on key changes to the assumptions of the model. These were the change of the treatment aspect of the model from Nottingham Prognostic Indicator to stage-based treatment and the inclusion of uptake which depended on participants’ previous attendance at screening. The switch to stage-based treatment was deemed to be acceptable, although it was identified that there are more granular stages of breast cancer than simply stages 1,II,III and IV. As data for treatment costs and utilities were not available at this level of detail, it was assumed that cancer only fell into these stages. In future versions of the model, data will be sought for more granular staging of breast cancer.
The researchers approved of the move to using different uptake parameters to reflect women’s history of participation in screening. It was deemed that this more closely represented the reality of non-attendance and attendance being correlated.
3.7 Technical Verification
To complete technical verification of MANC-RISK-SCREEN involving an assessment of an error check, an independent experienced R user with expertise in producing decision-analytic models for national decision-making body was employed to follow the TECH-VER checklist. This analyst also made suggestions about improving analysis time and documentation for the model. A number of errors were identified in MANC-RISK-SCREEN in this process. The duration of quality-adjusted life-years (QALYs) experienced was forced to be an integer year, and so sometimes patients had higher QALYs than life-years. This problem was solved by allowing a fraction of a year to be lived in the last year of the vector collecting quality of life values for each year. A problem in one of the functions meant that patients diagnosed with cancer sometimes lived longer than they would have done without the cancer. This was addressed by setting the age of death to the minimum of the age of cancer death or the age of all-cause mortality. A problem with two of the screening strategies was identified whereby a variable was being called by an out-of-date name, meaning the model would not run. In the updated MANC-RISK-SCREEN model, all references to the out-of-date parameter name were updated to the current name. In addition, an error was identified with the use of supplemental screening, whereby in some iterations of an ‘if’ statement, no value was assigned to a variable, causing problems further on in the model. This problem was solved by setting a baseline value for the parameter to take in the absence of supplemental screening being used. Following these updates, technical verification was performed again by a member of the research team, and no further problems were found.
3.8 Operational Validation
Results used in the operational validation were generated from the model output using the scenario of the current (3-year interval) screening programme targeted at women aged between 50 and 70 years. The independent operational validation of MANC-RISK-SCREEN involved determining whether the clinical outputs of the model aligned with epidemiological data on breast cancer observed in the UK [ 15 , 44 , 45 , 46 ].
Operational validation targets were selected based on our belief that close correspondence of these model outputs to targets may increase confidence in model primary cost-effectiveness results (see https://cisnet.cancer.gov/ ). Target selection was also limited by the availability of target data or summary statistics. Selected targets were related to incidence and detection rates. Survival by cancer stage was also considered as a target, but the authors are not aware of any sources of these data for the UK other than those used to generate the input parameters for the model. During operational validation it was observed that estimated age-specific cancer incidence under the current screening scenario was close to that reported in national cancer incidence statistics [source: Office for National Statistics (ONS) cancer incidence UK 2017 [ 44 ]].
The estimated proportion of breast cancers detected by screening matched the proportion reported in national breast cancer screening audits [source: NHS Digital Official Statistics [ 45 ]].
The estimated stage/size distribution of cancers detected at screening and through all diagnostic routes matched that reported in available registry data [Source: Cancer Research UK (CRUK) compiled from registries in each nation [ 46 ] and NHS Digital Official Statistics [ 45 ]].
3.9 Predictive Validity
The observed and predicted age-specific breast cancer incidence rates are reported in Table 2 and displayed in Fig. 1 . The cancer rates were visually similar for women before screening age. After the age of 50 years, MANC-RISK-SCREEN appears to underestimate cancer rates compared with the UK registry data from the years 2016–2018. There was a larger underestimation for the ≥ 80-year-old age groups. A potential explanation for this divergence is the use of all-cause mortality data from the years 2018–2020, which may incorporate higher mortality as age increased due to the beginning of the coronavirus pandemic. The ONS mortality data used to derive life expectancy due to all-cause mortality were subsequently changed to the data from the years 2016–2018. However, when the root mean squared error was calculated to compare the cancer incidence predictions of the model with those observed in the health system, using the earlier all-cause mortality data marginally reduced the fit of the model (90.103 versus 86.212 deviation in incidence per 100,000 per year).
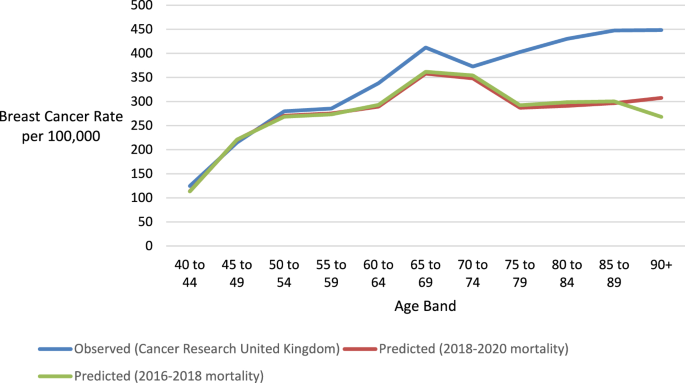
Predicted and observed age-specific incidence rate. Data source: [ 46 ]
An alternative explanation for the difference in cancer incidence observed is that there is a difference in the probability a woman will be diagnosed with breast cancer in reality (1 in 7 or 14.3%) compared with the value used in the model (11.8%). The latter lower figure is driven by the average lifetime breast cancer risk for the women who participated in the study used to populate the model (PROCAS 2), which is lower than the population average. To determine whether the difference in lifetime breast cancer risk was likely to be the cause of differences in incidence by age, the predicted incidence rates by MANC-RISK-SCREEN were inflated by the proportional difference in lifetime risk (Fig. 2 ). In this case the model-predicted rate appears to track the actual rate more closely, if at a little higher rate. The predicted and observed rates diverge at the age of 70 years, although to a lesser degree than with the unadjusted rates. When comparing the root mean squared error, using an inflated rate of lifetime cancer risk improves the fit of the model (54.882 versus 86.212 deviation in incidence per 100,000 per year). This suggests that the differences in the lifetime cancer risk between the sample from the BC-PREDICT sample and the general population explain a large part, but not all, of the deviation in the cancer incidence by age.
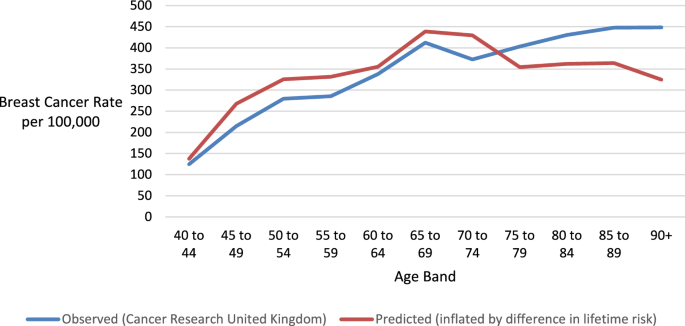
Inflation of predicted incidence rates estimated by MANC-RISK-SCREEN by the proportional difference in lifetime risk. Data source: [ 46 ]
It was not possible to assess whether the risk-prediction tool used to assign a risk score in PROCAS 2 had sufficient predictive value in the general population. MANC-RISK-SCREEN was populated using an observed distribution of 10-year risk in the women recruited to PROCAS 2. There is evidence that the data sources used to develop these risk-prediction models mean they may perform poorly in ethnically diverse populations [ 47 ]. An alternative data source would be needed to assess whether the predicted assignment to risk categories would be observed in a UK population. It was, therefore, not possible to assess the predictive ability of the Tyrer–Cuzick risk-prediction tool.
The original Gray model overestimated the proportion of cancer identified by screening, producing a value of 50.2% compared with 43% published by NHS England [ 45 ]. A potential explanation for the higher proportion of cancers identified by screening in the Gray model was due to the approach taken to code imperfect screening uptake. In the Gray model, individuals were assigned a probability of 60.5% of attending their first screen. Individuals that had attended at least one screen were assigned an 85.2% probability of attending further screens. In the UK-NBSP it has been observed that women who do not attend their first screen have a reduced likelihood of attending subsequent screens [ 45 ]. As such, MANC-RISK-SCREEN was recoded such that women had a 60.5% of attending their first screen and, if they did not attend this screen, only a 19.1% chance of attending subsequent screens. When a woman had attended at least one screen, the probability that she would attend subsequently was increased to 85.2% in MANC-RISK-SCREEN. Following this change, MANC-RISK-SCREEN predicted that 43% of cancers in the age group eligible for screening would be detected by screening, and this estimate exactly matched the proportion observed in the UK-NBSP.
Table 3 shows the proportions of cancers observed and predicted to be of different stages at diagnosis for cancers diagnosed clinically or by screening. The observed rates are taken form women diagnosed with breast cancer in England [ 46 ]. Cancers of unknown size have been excluded from MANC-RISK-SCREEN. The proportion of cancers have been adjusted to incorporate ductal carcinoma in situ that are reported separately. Across all cancers, the Gray model generated too many cancers at stage III (18.9% versus 8% in UK-NBSP) and too few at stage I (28.6% versus 39.4% in UK-NBSP). In addition, the Gray model predicted too few ductal carcinoma in situs (DCIS; 5.5% versus 12.9% in UK-NBSP) [ 48 ].
In the Gray model, it was assumed the DCIS were only diagnosed as part of a UK-NBSP and assigned an occurrence in 21% of the available cancers regardless of the tumour size. This assumption is likely to be why the proportion of tumours diagnosed as DCIS were considerably lower in the Gray model when compared with the observed data, as in reality DCIS can also be diagnosed clinically. In addition, the approach of allocating any-sized cancer as DCIS regardless of size in MANC-RISK-SCREEN may have affected the stage distribution; DCIS are likely to be smaller than cancers of other stages. In the Gray model the matrix used to determine the probability that a cancer of a given size was of stage I, II or III, an assumption had been made using data from source studies [ 49 , 50 ]. One of the source studies (Kollias et al., 1999 [ 49 ]) included estimates in which there was lymph node involvement in a cancer, and this was equally likely to involve one or more than one node [ 49 ]. Cancers with more than one lymph node involved are disproportionately likely to be at a higher stage compared with one or fewer lymph nodes, and this may have biased cancers estimated in the Gray model towards a higher stage of diagnosis. To address these issues, MANC-RISK-SCREEN was recoded such that cancers were allocated to a stage or as DCIS based on their size. Data from a study of DCIS were incorporated into the input matrix of the probability of a cancer of a given size being diagnosed at different stages [ 51 ]. In addition, the proportion of cancers with lymph node involvement in the study where these data were available (Wen et al., 2015 [ 50 ]) was used to adjust the distribution of cancer from the study where lymph node involvement was not available (Kollias et al, 1999) [ 49 ].
The predicted proportion of cancers of different stages generated by MANC-RISK-SCREEN is shown in Table 4 . The estimated proportion of cancers diagnosed as DCIS are similar to the values observed in data from Cancer Research UK, with a maximum deviation of two percentage points [ 52 ]. The observed values were derived by using the size of cancers diagnosed through the UK-NBSP applying the cancer stage by size matrix (see the parameter update document in the GitHub repository). In some cases, the band of cancer size reported in the UK-NBSP data spanned two bands of cancer size used in the matrix. In these situations, it was assumed that cancer size was evenly distributed across the two bands. Tumours greater than 5cm were assumed to be stage IV at diagnosis. The proportion of DCIS were added from separate data available from Cancer Research UK [ 52 ]. To compare the closeness of the predicted distribution of cancer stages to those observed in England, the root mean squared error of the predictions were calculated. These values represent the average percentage point deviation of the model predictions from those observed in the health service.
The distribution of stages for screen-detected cancers estimated in MANC-RISK-SCREEN had low deviation (2.706 percentage points) from the values observed in the UK-NBSP. However, adjustments subsequently made to the cancer stage by size matrix to improve the fit for the distribution of stages of cancer for all diagnosis routes (6.903 versus 8.616 percentage point deviation) resulted in greater deviations from the observed data for screen-detected cancer (5.023 versus 2.706 percentage point deviation). Therefore, the stage by size matrix in the final MANC-RISK-SCREEN model uses a combination of the Wen et al. data, which has details of lymph node involvement, and the Kollias et al. data with the likelihood of lymph node involvement for different sizes of cancer taken from the Wen et al. data [ 49 , 50 ]. This choice of data sacrifices improved fit in the distribution of screen-detected cancers at the expense of a smaller loss of fit in the distribution of the stages of all diagnosed cancers.
3.10 Cross-Validation
There is one alternative decision-analytic-model-based economic evaluation of a risk-stratified NBSP published relevant to the UK setting [ 53 ]. Pashayan et al. investigated the cost-effectiveness of alternative risk-stratified NBSP conceptualised as the addition of a risk threshold to the existing age threshold used to determine who is offered screening. On face value, the Pashayan model appears to be directly comparable to the Gray model and MANC-RISK-SCREEN. However, it was not possible to conduct a formal cross-validation of the Pashayan model and MANC-RISK-SCREEN in terms of model outputs because the stated decision problems, intervention under evaluation, decision-analytic model types and structures were not comparable (Table 5 ). Although the original model was shared with two further academic groups, the results of the additional models created as part of this work were not available for comparison at the time of the validation exercise.
4 Discussion
This study reports the development and use of a validation process which was then applied to a case study early decision-analytic-model-based cost-effectiveness analysis (CEA) of a risk-stratified NBSP [ 36 ]. Existing validation concepts were consolidated into a single step-by-step process resulting in the transparent presentation of the assumptions, strengths and weaknesses of a decision-analytic model.
The application of this validation process aimed to assess an existing decision-analytic model structure and understand whether it adequately captures the relevant pathways representing the risk stratification process, using a version of the Tyrer–Cuzick risk assessment tool with defined risk categories and assigned screening intervals, and subsequent interventions, the current breast screening programme, the natural history of breast cancer and treatment of breast cancer. Input parameters were updated as part of this validation process, but this is likely to be an ongoing process, as recommended by Sculpher and colleagues [ 30 ], as and when new data become available. This study has illustrated how the development and use of the validation process is a resource-intensive exercise involving the combined skills of health economists and experts relevant to the specific decision problem (evaluation of risk-stratified breast screening programmes).
Through the process of model validation, the strengths and weaknesses of the MANC-RISK-SCREEN have been discussed transparently, allowing decision-makers to gauge the quality of the model when using it to inform decisions as to the potential introduction of risk-stratified breast cancer screening. MANC-RISK-SCREEN is now available as an open-source model published on GitHub. A structured and transparent validation process was followed to produce MANC-RISK-SCREEN, which is now proposed to be a decision-analytic model with the potential to inform whether, and how, healthcare resources should be diverted towards risk-stratified NBSP implemented using different components. The component parts of a risk-stratified NBSP can be varied in terms of the age at which screening is first offered to women in the population (NBSP starting age), interval between screenings (NBSP screening interval), age at which screening is stopped (NBSP stopping age), number of X-rays used (one- or two-view mammography), supplementary screening technologies used (ultrasound and/or magnetic resonance imaging), interpretation of the X-ray (manual or digital), approach used to calculate a women’s risk of breast cancer, whether supplementary breast density measurements are taken, classification of the risk categories, approach taken to feedback risk to the women and strategies recommended as a result of identifying a women to be at high risk.
The operational validation, together with the assessment of predictive validity, were the main components assessing the external validity of MANC-RISK-SCREEN. It was planned to supplement external validation with cross-validation with a published model. The cross-validation was not successful, because the only decision-analytic model available for comparison did not match in terms of the interventions used for comparison or model structure. The external validity of MANC-RISK-SCREEN is not perfect. We suggest that decision analysts or decision-makers wanting to use MANC-RISK-SCREEN are aware that it overpredicts clinically diagnosed stage III cancers and underpredicts clinically diagnosed stage I. This overprediction is likely to affect the results of comparisons of screening programmes compared with no screening.
4.1 Limitations
The main limitation as part of this validation process was the need to rely on estimating intermediate outcomes generated by the decision-analytic model against data available from a limited range of sources reporting outcomes. These data sources only report outcomes from a single scenario: the current screening programme. There is, therefore, limited ability to discriminate between a decision-analytic model that performs well or poorly at the task of predicting comparative cost-effectiveness of alternative screening programmes. The specific targets that were selected for assessing predictive ability were based on the available data sources, rather than choosing targets that would be most informative for decision-making.
When comparing the predicted distribution of cancer stages detected at screening, cancers of unknown size were omitted from the observed data, as the MANC-RISK-SCREEN model does not currently produce such cancers. Such cancers may be those that occur in individuals who die between the detection of the cancer and receiving a full diagnosis. This may predominantly include those of lower socio-economic status or those who face barriers to accessing health system services, such as those from ethnic minorities. Omitting such cancers may therefore mean that the results of the model are biased. Including unstaged cancers in a future version of the model is therefore a priority alongside updates identified by the expert group, such as imperfect uptake for risk prediction and the addition of preventative medicine for those at higher risk of cancer.
Due to the large number of parameters in the decision-analytic model and paucity of data with which to fit the model to, calibration of most of the model was not conducted in the Gray model or MANC-RISK-SCREEN. The tumour growth model was calibrated. For the remaining parameters in the model, a key focus was to avoid overfitting the model to the UK national screening context that is the source of the available data for use as input parameters.
A related issue to validation of MANC-RISK-SCREEN that needs consideration is the limitations of the risk-prediction models used to allocate a women’s individual risk. The data sources used to develop these risk-prediction models mean they perform poorly in ethnically diverse populations [ 47 ]. The impact of this limitation will be most apparent if risk-based NBSP are rolled out into practice. In the absence of datasets to assess the predictive value of the risk-prediction models, it is impossible to know the extent of the impact of poorly performing risk prediction on the cost-effectiveness of risk-stratified NBSP.
5 Conclusion
This study has reported a structured and transparent validation process of an early decision-analytic model built to assess the potential cost-effectiveness of exemplar risk-stratified NBSP compared with current NBSP or no screening. The validation has suggested MANC-RISK-SCREEN has sufficient internal validity. There are some concerns regarding external validity, but these can only be rectified as and when new data sources become available to populate MANC-RISK-SCREEN.
Caro JJ, Briggs AH, Siebert U, Kuntz KM. Modeling good research practices—overview: a report of the ISPOR-SMDM Modeling Good Research Practices Task Force-1 on behalf of the ISPOR-SMDM Modeling Good Research Practices Task Force. Value Health. 2012;15.
Roberts M, Russell LB, Paltiel AD, Chambers M, McEwan P, Krahn M. Conceptualizing a model: a report of the ISPOR-SMDM Modeling Good Research Practices Task Force—2. Value Health [Internet]. 2012 [cited 2019 Sep 17];32. https://doi.org/10.1177/0272989X12454941
Siebert U, Alagoz O, Bayoumi AM, Jahn B, Owens DK, Cohen DJ, et al. State-transition modeling: a report of the ISPOR-SMDM Modeling Good Research Practices Task Force-3. Value Health. 2012;15:812–20.
Article PubMed Google Scholar
Karnon J, Stahl J, Brennan A, Caro JJ, Mar J, Möller J. Modeling using discrete event simulation: a report of the ISPOR-SMDM Modeling Good Research Practices Task Force-4. Value Health J Int Soc Pharmacoecon Outcomes Res. 2012;15:821–7.
Article Google Scholar
Briggs AH, Weinstein MC, Fenwick EAL, Karnon J, Sculpher MJ, Paltiel AD. Model parameter estimation and uncertainty analysis: a report of the ISPOR-SMDM Modeling Good Research Practices Task Force Working Group-6. Med Decis Mak. 2012;32:722–32.
Eddy DM, Hollingworth W, Caro JJ, Tsevat J, McDonald KM, Wong JB. Model transparency and validation: a report of the ISPOR-SMDM Modeling Good Research Practices Task Force-7. Med Decis Mak. 2012;32:733–43.
Kent S, Becker F, Feenstra T, Tran-Duy A, Schlackow I, Tew M, et al. The challenge of transparency and validation in health economic decision modelling: a view from Mount Hood. Pharmacoeconomics. 2019;37:1305–12.
Article PubMed PubMed Central Google Scholar
Emerson J, Bacon R, Kent A, Neumann PJ, Cohen JT. Publication of decision model source code: attitudes of health economics authors. Pharmacoeconomics. 2019;37:1409.
Sampson CJ, Arnold R, Bryan S, Clarke P, Ekins S, Hatswell A, et al. Transparency in decision modelling: what, why, who and how? Pharmacoeconomics. 2019;37:1355–69.
Alarid-Escudero F, Krijkamp EM, Pechlivanoglou P, Jalal H, Kao SYZ, Yang A, et al. A need for change! A coding framework for improving transparency in decision modeling. Pharmacoeconomics. 2019;37:1329–39.
Vemer P, Corro Ramos I, van Voorn GAK, Al MJ, Feenstra TL. AdViSHE: a validation-assessment tool of health-economic models for decision makers and model users. Pharmacoeconomics. 2016;34:349–61.
Article CAS PubMed Google Scholar
Büyükkaramikli NC, Rutten-van Mölken MPMH, Severens JL, Al M. TECH-VER: a verification checklist to reduce errors in models and improve their credibility. Pharmacoeconomics. 2019;37:1391–408.
McCabe C, Dixon S. Testing the validity of cost-effectiveness models. Pharmacoeconomics. 2000;17:501–13.
Nair V, Auger S, Kochanny S, Howard FM, Ginat D, Pasternak-Wise O, et al. Development and validation of a decision analytical model for posttreatment surveillance for patients with oropharyngeal carcinoma. JAMA Netw Open. 2022;5:e227240–e227240.
NHS Digital. Breast Screening Programme, England 2019–20 [Internet]. NHS Digit. 2021 [cited 2023 Aug 14]. https://digital.nhs.uk/data-and-information/publications/statistical/breast-screening-programme/england---2019-20 .
Godley KC, Gladwell C, Murray PJ, Denton E. The UK breast screening program – what you need to know. Climacteric. 2017;20:313–20.
Tyrer J, Duuy SW, Cuzick J. A breast cancer prediction model incorporating familial and personal risk factors. Stat Med. 2004;23:1111–30.
Lee A, Mavaddat N, Wilcox AN, Cunningham AP, Carver T, Hartley S, et al. BOADICEA: a comprehensive breast cancer risk prediction model incorporating genetic and nongenetic risk factors. Genet Med. 2019;21:1708–18.
Evans DGR, Howell A. Breast cancer risk-assessment models. Breast Cancer Res. 2007;9:1–8.
Pashayan N, Antoniou AC, Ivanus U, Esserman LJ, Easton DF, French D, et al. Personalized early detection and prevention of breast cancer: ENVISION consensus statement. Nat Rev Clin Oncol. 2020;17:687–705.
Evans DGR, Warwick J, Astley SM, Stavrinos P, Sahin S, Ingham S, et al. Assessing individual breast cancer risk within the U.K. National Health Service Breast Screening Program: a new paradigm for cancer prevention. Cancer Prev Res (Phila Pa). 2012;5:943–51.
Esserman LJ. The WISDOM Study: breaking the deadlock in the breast cancer screening debate. NPJ Breast Cancer. 2017;3:1–7.
Roux A, Cholerton R, Sicsic J, Moumjid N, French DP, Giorgi Rossi P, et al. Study protocol comparing the ethical, psychological and socio-economic impact of personalised breast cancer screening to that of standard screening in the “My Personal Breast Screening” (MyPeBS) randomised clinical trial. BMC Cancer. 2022. p. 22.
Evans DG, Astley S, Stavrinos P, Harkness E, Donnelly LS, Dawe S, et al. Improvement in risk prediction, early detection and prevention of breast cancer in the NHS Breast Screening Programme and family history clinics: a dual cohort study. Programme Grants Appl Res. 2016;4:1–210.
French DP, Astley S, Astley S, Brentnall AR, Cuzick J, Dobrashian R, et al. What are the benefits and harms of risk stratified screening as part of the NHS breast screening programme? Study protocol for a multi-site non-randomised comparison of BC-predict versus usual screening (NCT04359420). BMC Cancer. 2020;20:1–14.
Amir E, Evans DG, Shenton A, Lalloo F, Moran A, Boggis C, et al. Evaluation of breast cancer risk assessment packages in the family history evaluation and screening programme. J Med Genet. 2003;40:807–14.
Article CAS PubMed PubMed Central Google Scholar
Tyrer-Cuzick Risk Calculator for Breast Cancer Risk Assessment | MagView [Internet]. [cited 2023 Oct 16]. https://ibis-risk-calculator.magview.com/
Brentnall AR, Harkness EF, Astley SM, Donnelly LS, Stavrinos P, Sampson S, et al. Mammographic density adds accuracy to both the Tyrer-Cuzick and Gail breast cancer risk models in a prospective UK screening cohort. Breast Cancer Res BCR [Internet]. 2015 [cited 2022 Feb 24];17. https://pubmed.ncbi.nlm.nih.gov/26627479/
Clift AK, Dodwell D, Lord S, Petrou S, Brady SM, Collins GS, et al. The current status of risk-stratified breast screening. Br J Cancer. 2021;126:533–50.
Sculpher MJ, Claxton K, Drummond M, McCabe C. Whither trial-based economic evaluation for health care decision making? Health Econ. 2006;15:677–87.
Akehurst R, Anderson P, Brazier J, Brennan A, Briggs A, Buxton M, et al. Decision analytic modelling in the economic evaluation of health technologies. Pharmacoeconomics. 2000;17:443–4.
McGuire A, Morris S. What is it to be a model? Trials and tribulations in economic evaluation. Health Econ Prev Care. 2000;1:33–6.
Buxton MJ, Drummond MF, Van Hout BA, Prince RL, Sheldon TA, Szucs T, et al. Modelling in economic evaluation: an unavoidable fact of life. Health Econ. 1997;6:217–27.
Tappenden P, Chilcott JB. Avoiding and identifying errors and other threats to the credibility of health economic models. Pharmacoeconomics. 2014;32:967–79.
Sculpher M, Drummond M, Buxton M. The iterative use of economic evaluation as part of the process of health technology assessment. J Health Serv Res Policy. 1997;2:26–30.
Gray E, Donten A, Karssemeijer N, van Gils C, Evans DG, Astley S, et al. Evaluation of a stratified national breast screening program in the United Kingdom: an early model-based cost-effectiveness analysis. Value Health. 2017;20:1100–9.
Hammerschmidt T, Goertz A, Wagenpfeil S, Neiss A, Wutzler P, Banz K. Validation of health economic models: the example of EVITA. Value Health. 2003;6:551–9.
Haji Ali Afzali H, Gray J, Karnon J. Model performance evaluation (validation and calibration) in model-based studies of therapeutic interventions for cardiovascular diseases. Appl Health Econ Health Policy. 2013;11:85–93.
Mcwilliams L, Gareth Evans D, Payne K, Harrison F, Howell A, Howell SJ, et al. Implementing risk-stratified breast screening in England: an agenda setting meeting. Cancers. 2022;14:4636.
Jones J, Hunter D. Consensus methods for medical and health services research. Br Med J. 1995;311:376–80.
Article CAS Google Scholar
Gray AM, Clarke PM, Wolstenholme JL, Wordsworth S. Applied Methods of Cost-effectiveness Analysis in Healthcare [Internet]. Oxford University Press; 2010 [cited 2022 Aug 19]. https://books.google.com/books/about/Applied_Methods_of_Cost_effectiveness_An.html?id=wUJd0qYTIb8C .
Build software better, together [Internet]. GitHub. [cited 2023 Oct 16]. https://github.com .
Wright SJ, Eden M, Ruane H, Byers H, Evans DG, Harvie M, et al. Estimating the cost of 3 risk prediction strategies for potential use in the United Kingdom National Breast Screening Program. Med Decis Mak Policy Pract. 2023;8:238146832311713.
Google Scholar
Office for National Statistics. Cancer registration statistics, England [Internet]. 2019 [cited 2022 Aug 19]. https://www.ons.gov.uk/peoplepopulationandcommunity/healthandsocialcare/conditionsanddiseases/bulletins/cancerregistrationstatisticsengland/2017 .
NHS Digital Screening and Immunisations Team. Breast Screening Programme. NHS Digital; 2021.
Cancer Research UK. Breast cancer incidence (invasive) statistics [Internet]. 2022 [cited 2022 Apr 14]. https://www.cancerresearchuk.org/health-professional/cancer-statistics/statistics-by-cancer-type/breast-cancer/incidence-invasive#heading-One .
Evans DG, van Veen EM, Byers H, Roberts E, Howell A, Howell SJ, et al. The importance of ethnicity: are breast cancer polygenic risk scores ready for women who are not of white European origin? Int J Cancer. 2022;150:73–9.
Cancer Research UK. Early Diagnosis Data Hub [Internet]. 2022 [cited 2022 Apr 14]. https://crukcancerintelligence.shinyapps.io/EarlyDiagnosis/ .
Kollias J, Murphy CA, Elston CW, Ellis IO, Robertson JFR, Blarney RW. The prognosis of small primary breast cancers. Eur J Cancer. 1999;35:908–12.
Wen J, Ye F, Li S, Huang X, Yang L, Xiao X, et al. The practicability of a novel prognostic index (PI) model and comparison with Nottingham Prognostic Index (NPI) in stage I-III breast cancer patients undergoing surgical treatment. PLoS ONE. 2015;10: e0143537.
Cheng L, Al-Kaisi NK, Gordon NH, Liu AY, Gebrail F, Shenk RR. Relationship between the size and margin status of ductal carcinoma in situ of the breast and residual disease. JNCI J Natl Cancer Inst. 1997;89:1356–60.
Cancer Research UK. In situ breast carcinoma incidence statistics [Internet]. 2022 [cited 2022 Nov 3]. https://www.cancerresearchuk.org/health-professional/cancer-statistics/statistics-by-cancer-type/breast-cancer/incidence-in-situ .
Pashayan N, Morris S, Gilbert FJ, Pharoah PDP. Cost-effectiveness and benefit-to-harm ratio of risk-stratified screening for breast cancer: a life-table model. JAMA Oncol. 2018;4:1504–10.
Download references
Acknowledgements
We acknowledge the input of D Gareth Evans, Sue Astley (The University of Manchester), Nico Karssemeijer (Radboud University), Carla van Gils [University Medical Center (UMC) Utrecht, div. Julius Centrum] into the conceptualisation and structure of the decision-analytic-model-based cost-effectiveness analysis that informed the design of this study. We would also like to thank Tom Jones for input into subsequent technical verification of the model and Martin Herrerias Azcue for producing the R-Shiny interface for the model. We would also like to thank Rob Hainsworth for his contribution to the updating of the model parameters.
Author information
Authors and affiliations.
Division of Population Health, Health Services Research and Primary Care, Manchester Centre for Health Economics, The University of Manchester, Oxford Road, Manchester, M139PL, UK
Stuart J. Wright, Gabriel Rogers, Anna Donten & Katherine Payne
GRAIL, New Penderel House 4th Floor, 283-288 High Holborn, London, WC1V 7HP, UK
You can also search for this author in PubMed Google Scholar
Corresponding author
Correspondence to Stuart J. Wright .
Ethics declarations
Financial support for this study was provided in part by a grant from the National Institute for Health Research Predicting Risk of Cancer at Screening (PROCAS) 2 Programme Grant (Ref: RP-PG-1214-20016) and by the International Alliance for Cancer Early Detection, an alliance between Cancer Research UK, the Canary Center at Stanford University, the University of Cambridge, Oregon Health & Science University (OHSU) Knight Cancer Institute, University College London and The University of Manchester. The funding agreements ensured the authors’ independence in designing the study, interpreting the data, writing and publishing the report.
Author contributions
All authors meet International Committee of Medical Journal Editors (ICMJE) criteria for authorship. SW formulated the research question, updated the model parameters, ran analyses and led the writing of the manuscript. EG formulated the research question and contributed to writing the manuscript. GR completed the checklists and contributed to writing the manuscript. AD contributed to updating the model parameters and writing the manuscript. KP formulated the research question, provided advice on the design for the overall study, and produced a first draft of the manuscript. KP acts as guarantor for this work. This manuscript has been read and approved by all the authors.
Conflict of interest
Ewan Gray is an employee of Grail LLC and has received consultancy fees from Dxcover Limited and Wobble Genomics Limited. All remaining authors have no conflicts of interest to declare.
Ethics approval and consent to participate
Ethical approval was not required for this study, which used existing published data and information.
Data and code availability
The R code for the decision-analytic model structure is publicly available in a GitHub repository: https://github.com/stuwrighthealthecon/MANC-RISK-SCREEN . The repository is also archived using Zenodo and can be accessed using the doi: https://doi.org/10.5281/zenodo.7105246 .
Supplementary Information
Below is the link to the electronic supplementary material.
Supplementary file1 (DOCX 56 KB)
Rights and permissions.
Open Access This article is licensed under a Creative Commons Attribution-NonCommercial 4.0 International License, which permits any non-commercial use, sharing, adaptation, distribution and reproduction in any medium or format, as long as you give appropriate credit to the original author(s) and the source, provide a link to the Creative Commons licence, and indicate if changes were made. The images or other third party material in this article are included in the article's Creative Commons licence, unless indicated otherwise in a credit line to the material. If material is not included in the article's Creative Commons licence and your intended use is not permitted by statutory regulation or exceeds the permitted use, you will need to obtain permission directly from the copyright holder. To view a copy of this licence, visit http://creativecommons.org/licenses/by-nc/4.0/ .
Reprints and permissions
About this article
Wright, S.J., Gray, E., Rogers, G. et al. A structured process for the validation of a decision-analytic model: application to a cost-effectiveness model for risk-stratified national breast screening. Appl Health Econ Health Policy (2024). https://doi.org/10.1007/s40258-024-00887-z
Download citation
Accepted : 30 April 2024
Published : 16 May 2024
DOI : https://doi.org/10.1007/s40258-024-00887-z
Share this article
Anyone you share the following link with will be able to read this content:
Sorry, a shareable link is not currently available for this article.
Provided by the Springer Nature SharedIt content-sharing initiative
- Find a journal
- Publish with us
- Track your research
- Introduction
- Methodology
- Conclusions
- Article Information
Linear regression models were fitted and adjusted for maternal education level, maternal body mass index (BMI), total minutes of physical activity per week, breastfeeding, center size, and NOVA classification system groups 2 and 3. HDL indicates high-density lipoprotein; HOMA-IR, homeostasis model assessment for insulin resistance; LDL, low-density lipoprotein.
a For HDL cholesterol, a positive β coefficient signifies low risk.
eAppendix. Additional Study Assessments
eTable 1. Distribution of FFQ Items Into 4 Groups According to the Degree of Their Processing Established by NOVA Classification System
eFigure 1. Flow Chart of the Children Included in the Analysis
eTable 2. Association Between Energy-Adjusted Ultraprocessed Food Consumption in Tertiles (in g/day) and CVD Risk Factors at Baseline
eFigure 2. Linear Regression Models Replacing 100 g of UPF With 100 g of Unprocessed/Minimally Processed Foods
eTable 3. Maternal Socioprofessional Stratified Regression Association Between 1-SD Increment of Energy-Adjusted Ultraprocessed Food Consumption (in g/day) and CVD Risk Factors at Baseline
eTable 4. Maternal Education Level Stratified Regression Association Between 1-SD Increment of Energy-Adjusted Ultraprocessed Food Consumption (in g/day) and CVD Risk Factors at Baseline
Data Sharing Statement
See More About
Sign up for emails based on your interests, select your interests.
Customize your JAMA Network experience by selecting one or more topics from the list below.
- Academic Medicine
- Acid Base, Electrolytes, Fluids
- Allergy and Clinical Immunology
- American Indian or Alaska Natives
- Anesthesiology
- Anticoagulation
- Art and Images in Psychiatry
- Artificial Intelligence
- Assisted Reproduction
- Bleeding and Transfusion
- Caring for the Critically Ill Patient
- Challenges in Clinical Electrocardiography
- Climate and Health
- Climate Change
- Clinical Challenge
- Clinical Decision Support
- Clinical Implications of Basic Neuroscience
- Clinical Pharmacy and Pharmacology
- Complementary and Alternative Medicine
- Consensus Statements
- Coronavirus (COVID-19)
- Critical Care Medicine
- Cultural Competency
- Dental Medicine
- Dermatology
- Diabetes and Endocrinology
- Diagnostic Test Interpretation
- Drug Development
- Electronic Health Records
- Emergency Medicine
- End of Life, Hospice, Palliative Care
- Environmental Health
- Equity, Diversity, and Inclusion
- Facial Plastic Surgery
- Gastroenterology and Hepatology
- Genetics and Genomics
- Genomics and Precision Health
- Global Health
- Guide to Statistics and Methods
- Hair Disorders
- Health Care Delivery Models
- Health Care Economics, Insurance, Payment
- Health Care Quality
- Health Care Reform
- Health Care Safety
- Health Care Workforce
- Health Disparities
- Health Inequities
- Health Policy
- Health Systems Science
- History of Medicine
- Hypertension
- Images in Neurology
- Implementation Science
- Infectious Diseases
- Innovations in Health Care Delivery
- JAMA Infographic
- Law and Medicine
- Leading Change
- Less is More
- LGBTQIA Medicine
- Lifestyle Behaviors
- Medical Coding
- Medical Devices and Equipment
- Medical Education
- Medical Education and Training
- Medical Journals and Publishing
- Mobile Health and Telemedicine
- Narrative Medicine
- Neuroscience and Psychiatry
- Notable Notes
- Nutrition, Obesity, Exercise
- Obstetrics and Gynecology
- Occupational Health
- Ophthalmology
- Orthopedics
- Otolaryngology
- Pain Medicine
- Palliative Care
- Pathology and Laboratory Medicine
- Patient Care
- Patient Information
- Performance Improvement
- Performance Measures
- Perioperative Care and Consultation
- Pharmacoeconomics
- Pharmacoepidemiology
- Pharmacogenetics
- Pharmacy and Clinical Pharmacology
- Physical Medicine and Rehabilitation
- Physical Therapy
- Physician Leadership
- Population Health
- Primary Care
- Professional Well-being
- Professionalism
- Psychiatry and Behavioral Health
- Public Health
- Pulmonary Medicine
- Regulatory Agencies
- Reproductive Health
- Research, Methods, Statistics
- Resuscitation
- Rheumatology
- Risk Management
- Scientific Discovery and the Future of Medicine
- Shared Decision Making and Communication
- Sleep Medicine
- Sports Medicine
- Stem Cell Transplantation
- Substance Use and Addiction Medicine
- Surgical Innovation
- Surgical Pearls
- Teachable Moment
- Technology and Finance
- The Art of JAMA
- The Arts and Medicine
- The Rational Clinical Examination
- Tobacco and e-Cigarettes
- Translational Medicine
- Trauma and Injury
- Treatment Adherence
- Ultrasonography
- Users' Guide to the Medical Literature
- Vaccination
- Venous Thromboembolism
- Veterans Health
- Women's Health
- Workflow and Process
- Wound Care, Infection, Healing
Get the latest research based on your areas of interest.
Others also liked.
- Download PDF
- X Facebook More LinkedIn
Khoury N , Martínez MÁ , Garcidueñas-Fimbres TE, et al. Ultraprocessed Food Consumption and Cardiometabolic Risk Factors in Children. JAMA Netw Open. 2024;7(5):e2411852. doi:10.1001/jamanetworkopen.2024.11852
Manage citations:
© 2024
- Permissions
Ultraprocessed Food Consumption and Cardiometabolic Risk Factors in Children
- 1 Universitat Rovira i Virgili Departament de Bioquímica i Biotecnologia, Unitat de Nutrició Humana, ANUT-DSM group, Spain
- 2 Institut d’Investigació Sanitària Pere Virgili, Reus, Spain
- 3 Consorcio Centro de Investigación Biomédica en Red, M. P. Fisiopatología de la Obesidad y Nutrición, Instituto de Salud Carlos III, Madrid, Spain
- 4 Metabolism and Investigation Unit, Maimónides Institute of Biomedicine Research of Córdoba, Reina Sofia University Hospital, University of Córdoba, Córdoba, Spain
- 5 Primary Care Interventions to Prevent Maternal and Child Chronic Diseases of Perinatal and Developmental Origin, Instituto de Salud Carlos III, Madrid, Spain
- 6 Unit of Pediatric Gastroenterology, Hepatology and Nutrition, Pediatric Service, Hospital Clínico Universitario de Santiago, Santiago de Compostela, Spain
- 7 Pediatric Nutrition Research Group, Health Research Institute of Santiago de Compostela, Unit of Investigation in Nutrition, Growth and Human Development of Galicia-Universidad de Santiago de Compostela, Santiago de Compostela, Spain
- 8 Growth, Exercise, Nutrition and Development Research Group, University of Zaragoza, Spain
- 9 Instituto Agroalimentario de Aragón, University of Zaragoza, Spain
- 10 Instituto de Investigación Sanitaria de Aragón, Zaragoza, Spain
- 11 Center for Nutrition Research, Faculty of Pharmacy and Nutrition, Department of Nutrition, Food Science and Physiology, University of Navarra, Pamplona, Spain
- 12 Navarra Medical Research Institute, Pamplona, Spain
- 13 Department of Preventive Medicine and Public Health, University of Valencia, Spain
- 14 Hospital del Mar Medical Research Institute, Barcelona, Spain
- 15 Centre d’Atenció Primària, Institut Català de la Salut, Reus, Spain
- 16 Pediatrics, Nutrition, and Development Research Unit, Universitat Rovira I Virgili, Reus, Spain
Question What is the association of consuming ultraprocessed foods (UPFs) with cardiometabolic risk factors in children?
Findings In this cross-sectional study of 1426 children, higher consumption of UPFs was positively associated with body mass index, waist circumference, fat mass index, and fasting plasma glucose and negatively associated with high-density lipoprotein cholesterol concentrations.
Meaning These findings highlight the need for public health initiatives to promote the replacement of UPFs with unprocessed or minimally processed foods.
Importance High intake of ultraprocessed foods (UPFs) has been associated with higher cardiometabolic risk in adults; however, the evidence in children is limited.
Objective To investigate the association between UPF consumption and cardiometabolic risk factors in the Childhood Obesity Risk Assessment Longitudinal Study (CORALS).
Design, Setting, and Participants This baseline cross-sectional analysis was conducted using the data of CORALS participants recruited between March 22, 2019, and June 30, 2022. Preschool children (aged 3-6 years) were recruited from schools and centers in 7 cities in Spain. Inclusion criteria included informed consent signed by parents or caregivers and having a completed a set of questionnaires about the child’s prenatal history at home. Exclusion criteria included low command of Spanish or unstable residence.
Exposure Energy-adjusted UPF consumption (in grams per day) from food frequency questionnaires and based on the NOVA food classification system.
Main Outcomes and Measures Age- and sex-specific z scores of adiposity parameters (body mass index [BMI], fat mass index, waist-to-height ratio, and waist circumference) and cardiometabolic parameters (diastolic and systolic blood pressure, fasting plasma glucose, homeostasis model assessment for insulin resistance, high-density and low-density lipoprotein cholesterol, and triglycerides) were estimated using linear regression models.
Results Of 1509 enrolled CORALS participants, 1426 (mean [SD] age, 5.8 [1.1] years; 698 boys [49.0%]) were included in this study. Mothers of children with high UPF consumption were younger, had a higher BMI, were more likely to have overweight or obesity, and had lower education levels and employment rates. Compared with participants in the lowest tertile of energy-adjusted UPF consumption, those in the highest tertile showed higher z scores of BMI (β coefficient, 0.20; 95% CI, 0.05-0.35), waist circumference (β coefficient, 0.20; 95% CI, 0.05-0.35), fat mass index (β coefficient, 0.17; 95% CI, 0.00-0.32), and fasting plasma glucose (β coefficient, 0.22; 95% CI, 0.06-0.37) and lower z scores for HDL cholesterol (β coefficient, −0.19; 95% CI, −0.36 to −0.02). One-SD increments in energy-adjusted UPF consumption were associated with higher z scores for BMI (β coefficient, 0.11; 95% CI, 0.05-0.17), waist circumference (β coefficient, 0.09; 95% CI, 0.02-0.15), fat mass index (β coefficient, 0.11; 95% CI, 0.04-1.18), and fasting plasma glucose (β coefficient, 0.10; 95% CI, 0.03-0.17) and lower HDL cholesterol (β coefficient, −0.07; 95% CI, −0.15 to −0.00). Substituting 100 g of UPFs with 100 g of unprocessed or minimally processed foods was associated with lower z scores of BMI (β coefficient, −0.03; 95% CI, −0.06 to −0.01), fat mass index (β coefficient, −0.03; 95% CI, −0.06 to 0.00), and fasting plasma glucose (β coefficient, −0.04; 95% CI, −0.07 to −0.01).
Conclusions and Relevance These findings suggest that high UPF consumption in young children is associated with adiposity and other cardiometabolic risk factors, highlighting the need for public health initiatives to promote the replacement of UPFs with unprocessed or minimally processed foods.
The presence of abnormal cardiometabolic risk factors often begins in childhood, highlighting the importance of identifying and controlling them early to delay or prevent cardiovascular disease (CVD) in the future. 1 Modifiable risk factors (eg, diet and physical activity) may contribute to the development of recognized cardiometabolic risk factors. 2 - 4 Emerging studies have shed light on the potential role of ultraprocessed foods (UPFs) in determining the risk of chronic diseases, 5 - 7 independent of their nutritional profiles. 8
Commonly, UPFs represent a category of food products that undergo extensive industrial processing, often containing multiple ingredients, additives, and preservatives to make them not only convenient (ready to eat) but also palatable and appealing. This approach has been used to create the most widely used UPF classification, the NOVA Food Classification system. 9 , 10 Ultraprocessed foods are typically rich in saturated fats, sugars, sodium, and other substances (eg, additives) and lower in essential nutrients, all of which are associated with cardiometabolic health. 11 , 12 Due to their high availability and affordability and wide marketing to children, UPFs have become increasingly frequent in modern diets, particularly among children, adolescents, 13 - 15 and their families, and especially among individuals and families with low socioeconomic status and educational levels in which obesity is more prevalent. 16 Additionally, the habits established during early childhood often track to later ages 17 and carry into adulthood, compounding the risk of CVD and other noncommunicable diseases. 18 - 20
Previous observational studies in adults have reported positive associations between UPF consumption and obesity, 21 type 2 diabetes, 22 CVD, 23 and all-cause mortality 24 ; however, the epidemiologic evidence in children remains limited and controversial. 25 While the majority of studies have reported unfavorable associations with body mass index (BMI), others did not find this association, and few have focused on cardiometabolic risk factors. 25
Given the public health burden of CVDs and the increasing availability of UPFs, having a better understanding of potential associations between UPF consumption and cardiometabolic risk factors in children is essential. Therefore, the aim of this study was to examine the associations between UPF consumption and cardiometabolic risk factors in a population of Spanish preschool children (aged 3-6 years).
This cross-sectional study was conducted using data from the Childhood Obesity Risk Assessment Longitudinal Study (CORALS), which followed the Strengthening the Reporting of Observational Studies in Epidemiology ( STROBE ) reporting guideline. The ethics committee of each of recruitment center approved the study protocol, which was conducted following the standards of the Declaration of Helsinki. 26 Parents or caregivers provided written informed consent.
CORALS is an ongoing prospective multicenter study conducted in 7 Spanish centers aiming to identify potential risk factors for childhood obesity over a 10-year follow-up period. A detailed description of the CORALS is published elsewhere. 27 Between March 22, 2019, and June 30, 2022, eligible participants aged 3 to 6 years at enrollment were recruited from schools across 7 cities in Spain. To be enrolled in the study, parents or caregivers had to sign a consent form, attend the inclusion face-to-face visit and complete several questionnaires at home for data collection on leisure time physical activity, 3-day food consumption, and sociodemographics. The exclusion criteria included belonging to a family with difficulty collaborating due to low command of Spanish or unstable residence.
To estimate the dietary intake of UPFs, trained dietitians (B.P.-V., S.d.L.H.D., M.L.M.-B., K.A.P.-V., and R.V.-C.) used the validated, semiquantitative, 125-item food and beverage frequency COME-Kids questionnaire. 28 Participants with energy intake below the first percentile or above the 99th percentile were excluded to minimize misreporting (details are provided in the eAppendix in Supplement 1 ). The NOVA Food Classification system was used to determine the consumption of food depending on its degree of processing 27 (details are provided in the eAppendix and eTable 1 in Supplement 1 ).
Adiposity measurements and cardiometabolic risk factor assessments were conducted in health care centers. Weight and body fat mass were measured using a precision scale and an octopolar multifrequency bioelectrical impedance device (MC780MAS; Tanita). Height was measured using a portable seca 213 stadiometer according to standard procedures. Body mass index was calculated and categorized as underweight or normal weight or as overweight or obesity based on pediatric cutoffs. 29 Waist circumference was determined using a flexible, nonextensible measuring tape. The fat mass index was estimated by dividing body fat mass in kilograms by height in meters squared. 30 Waist-to-height ratio was estimated by dividing waist circumference in centimeters by height in centimeters. 31
Blood pressure was measured in the nondominant arm 3 times, with a 5-minute gap between each measurement, using an automatic oscillometer (M3 Intelligence HEM-75051-EV; OMRON Healthcare) equipped with a child-sized cuff. Eight-hour fasting blood samples were collected from participants, and serum total cholesterol, high-density lipoprotein (HDL) cholesterol, low-density lipoprotein (LDL) cholesterol, triglycerides, plasma glucose, and insulin concentrations were measured using standard procedures. Homeostasis model assessment for insulin resistance (HOMA-IR) was calculated as fasting insulin (μIU/mL) × fasting glucose (mmol/L) / 22.5.
Parents or caregivers were provided with a set of questionnaires to complete at home, gathering information on early life factors, maternal characteristics, and lifestyle patterns. To assess physical activity, the total time (in hours) of engagement in sports and physical activities per week was estimated using a previously validated questionnaire. 32 An 18-item questionnaire for children was used to assess adherence to the Mediterranean diet, an indicator of diet quality. 27
The current analysis was conducted using the CORALS database updated through January 20, 2023. Analysis of descriptive baseline characteristics are reported as mean (SD) or median (IQR) for continuous variables and as numbers with percentages for categorical variables using one-way analysis of variance and χ 2 test, respectively.
Consumption of UPFs (in grams per day) was adjusted for total energy intake using the residual regression method. 33 Intake of UPFs in grams per day was calculated instead of energy percentage to account for foods with no energy content (eg, artificially sweetened beverages) and for nonnutritional concerns associated with food processing (eg, food additives). Participants were categorized by tertiles of energy-adjusted UPF consumption, ranging from tertile 1 for the lowest intake to tertile 3 for the highest intake.
Age- and sex-specific z scores of each outcome were estimated from standardized residuals conducted using linear regression models. Missing data of less than 5% for covariates were imputed to the mean and to the highest frequency category for quantitative and qualitative confounders, respectively. 34 Multivariable linear regression models were fitted to assess the associations (β coefficient and 95% CI) between tertiles of energy-adjusted UPFs and z scores of cardiometabolic risk factors. The first tertile (lowest intake) was considered the reference. Models were adjusted for maternal education level (primary or lower, secondary or university), maternal BMI (underweight, normal overweight, obesity), physical activity (minutes per week), exclusive breastfeeding (yes or no), recruitment center size (<200, 200-400, >400 participants), and NOVA group 1, 2, or 3 (as detailed in eTable 1 in Supplement 1 ). To assess the linear trend, the median value of each tertile of UPF consumption was modeled as a continuous variable. The analysis was also conducted in a continuous form, with a 1-SD increment and using the same confounders.
Additionally, a simulation model was fitted to substitute 100 g of consumed UPFs with 100 g of unprocessed or minimally processed food to examine the association of healthier food consumption with the outcomes. The theoretical impact of substituting 1 food group for another was assessed by introducing both variables simultaneously as continuous variables into the model. Differences in the β coefficients, variances, and covariance were used to estimate the β coefficients and 95% CIs for the substitution association. Sensitivity analyses were conducted to assess associations according diet quality, maternal education, and socioprofessional level (details provided in the eAppendix in Supplement 1 ).
Data were analyzed using Stata, version 14 software (StataCorp LLC). All statistical tests were 2-sided, and P < .05 was deemed statistically significant.
A total of 1426 participants (mean [SD] age, of 5.8 [1.1] years; 698 boys [49.0%] and 728 girls [51.0%]) were included in this study after excluding 54 participants lacking the food and beverage frequency questionnaire and 29 with missing data or implausible reported energy intake (eFigure 1 in Supplement 1 ). The characteristics of the study population across tertiles of energy-adjusted UPF consumption are shown in Table 1 . Children in the third tertile (highest UPF consumption) had a higher BMI z score, waist-to-height ratio, fat mass index, systolic blood pressure, and overweight or obesity prevalence and lower HDL and LDL cholesterol. Mothers whose children were categorized in the highest tertile of energy-adjusted UPF consumption were younger, had a higher BMI, were more prone to be living with overweight or obesity, were less likely to have exclusively breastfed their children, and had lower educational achievement and employment rates.
General dietary characteristics of participants are shown in Table 2 . Children in the top tertile were more likely to consume higher amounts of total energy, carbohydrates, yogurt, other dairy products, sugar and candy, and sugary beverages and lower amounts of protein, fat, monounsaturated and polyunsaturated fatty acids, fiber, milk, cheese, white meat, unprocessed red meat, eggs, fish, seafood, vegetables, fruits, nuts, legumes, whole and refined cereals, and oils and fat.
Cross-sectional associations between energy-adjusted UPF consumption across tertiles and by 1-SD increment (in grams per day) and cardiometabolic risk factors are shown in Table 3 . Compared with participants in the lowest tertile, those in the top tertile had higher z scores of waist circumference (β coefficient, 0.20; 95% CI, 0.05-0.35), BMI (β coefficient, 0.20; 95% CI, 0.05-0.35), fat mass index (β coefficient, 0.17; 95% CI, 0.00-0.32), and fasting plasma glucose (β coefficient, 0.22; 95% CI, 0.06-0.37). Additionally, participants in the highest tertile had a lower z score of HDL cholesterol (β coefficient, −0.19; 95% CI, −0.36 to −0.02). After adjusting for the Mediterranean diet score (12 points), the associations were maintained for the z scores of fasting plasma glucose (β coefficient, 0.17; 95% CI, 0.03-0.31) and HDL cholesterol (β coefficient, −0.20; 95% CI, −0.36 to −0.05) (eTable 2 in Supplement 1 ). Positive associations were also observed between 1-SD increments of UPF consumption and z scores of waist circumference (β coefficient, 0.09; 95% CI, 0.02-0.15), fat mass index (β coefficient, 0.11; 95% CI, 0.04-1.18), BMI (β coefficient, 0.11; 95% CI, 0.05-0.17), and fasting plasma glucose (β coefficient, 0.10; 95% CI, 0.03-0.17) and negatively associated with HDL cholesterol (β coefficient, −0.07; 95% CI, −0.15 to 0.00) ( Table 3 ). Likewise, after further adjusting for the Mediterranean diet score, the associations between 1-SD increments remained significant for the z scores of fasting plasma glucose (β coefficient, 0.08; 95% CI, 0.02-0.13) and HDL cholesterol (β coefficient, −0.07; 95% CI, −0.13 to −0.01) (eTable 2 in Supplement 1 ).
Similar positive associations among fat mass index, BMI, and plasma glucose were observed, irrespective of the animal or vegetable origin of the UPFs consumed. Substitution of 100 g of UPFs with 100 g of unprocessed or minimally processed foods was associated with a decrease in z scores of fat mass index (β coefficient, −0.03; 95% CI, −0.06 to 0.00) and BMI (β coefficient, −0.03; 95% CI, −0.06 to −0.01), and fasting plasma glucose (β coefficient, −0.04; 95% CI, −0.07 to −0.01) ( Figure ). The same models adjusted for the Mediterranean diet score showed positive associations for z scores of fasting plasma glucose (β coefficient, −0.04; 95% CI, −0.06 to −0.01) and inverse association for z score of HDL cholesterol (β coefficient, 0.03; 95% CI, 0.00-0.07) (eFigure 2 in Supplement 1 ). No associations were shown for the other outcomes.
In children whose mothers were unemployed, a positive association was found between energy-adjusted UPF consumption and z scores of waist circumference (β coefficient, 0.26; 95% CI, 0.14-0.39), fat mass index (β coefficient, 0.20; 95% CI, 0.07-0.34), waist-to-height ratio (β coefficient, 0.21; 95% CI, 0.09-0.34), BMI (β coefficient, 0.18; 95% CI, 0.04-0.31), fasting plasma glucose (β coefficient, 0.14; 95% CI, 0.03-0.25), and diastolic blood pressure (β coefficient, 0.14; 95% CI, 0.0-0.27). In children with employed mothers, a positive association was observed between 1-SD increments in energy-adjusted UPF consumption and z scores of fasting plasma glucose (β coefficient, 0.09; 95% CI, 0.01-0.17) and a negative association in case of the HDL cholesterol (β coefficient, −0.09; 95% CI, −0.18 to −0.01) (eTable 3 in Supplement 1 ).
Children whose mothers had a low education level had higher z scores of waist circumference (β coefficient, 0.14; 95% CI, 0.05-0.23), fat mass index (β coefficient, 0.15; 95% CI, 0.06-0.25), BMI (β coefficient, 0.15; 95% CI, 0.06-0.24), and fasting plasma glucose (β coefficient, 0.11; 95% CI, 0.02-0.19). Children whose mothers had a high education level had a lower HDL cholesterol z score (β coefficient, −0.15; 95% CI, −0.26 to −0.03) (eTable 4 in Supplement 1 ).
To our knowledge, this study is the first to assess the associations between UPF consumption and various cardiometabolic risk factors in young children. In this large cross-sectional study, UPF consumption was positively associated with z scores of BMI, waist circumference, fat mass index, and fasting plasma glucose concentration and inversely associated with HDL cholesterol concentration.
Our findings are in line with those of previous studies. 35 - 37 Consumption of UPFs at age 4 years was associated with increased BMI z scores at age 10 years in the Generation XXI cohort, while no association was found at age 7 years. 35 Another study showed that high UPF consumption in children aged 7 to 13 years was associated with increased BMI growth trajectories. 36 Similarly, lower UPF intake in Spanish children aged 4 to 7 years was associated with lower BMI z scores at age 7 years, though this association became nonsignificant after adjusting for maternal factors. 37
A global study by Neri et al 38 revealed that increased UPF consumption was associated with higher dietary energy density and intake of free sugars, alongside decreased total fiber intake, potentially contributing to childhood obesity. Additionally, findings from the Avon Longitudinal Study of Parents and Children showed that high UPF consumption was associated with unfavorable fat mass index trajectories from age 7 to 24 years. 36 Similarly, in a Brazilian cohort, UPF consumption during preschool years was associated with increases in waist circumference from preschool to school age. 39 Other studies found no significant association between UPF consumption and HDL cholesterol and fasting plasma glucose concentrations. 37 , 40 Therefore, to our knowledge, our study is the first in children to find significant associations with the aforementioned risk factors and is in line with other studies assessing adult populations. 41
Our results provide new insight into the association between UPF consumption and health and the importance of recognizing that early dietary habits in childhood might have future implications on cardiometabolic health. While the magnitude of the associations reported in our study may be considered of limited clinical relevance, it is important to note that our study consisted of young children. Therefore, if such minimal differences can reveal a significant association, they may serve as an early warning of future cardiometabolic conditions.
Our results are in line with previous studies showing that the main UPF products consumed are pastries, sweet beverages, cookies, and candies. 42 - 44 In addition, our results support the findings of other European studies that have shown that children of mothers with lower education or with lower socioeconomic status are more likely to consume UPFs. These findings suggest that educational and socioeconomic factors may contribute to the purchase of low-cost and unhealthy foods, such as UPFs, increasing the risk of health disorders. 37 , 45 , 46
Several possible mechanisms could explain our results. First, UPFs contain higher amounts of sodium, energy, fat, and sugar and lower amounts of fiber, which are well recognized as contributors to cardiometabolic risk factors. 47 In addition, some UPFs may be linked to a higher glycemic response, and it has been shown that high consumption of sugar-sweetened beverages may delay the internal satiety signal, leading to excessive calorie intake and higher glycemic load. 48 - 50 Moreover, excessive consumption of energy, saturated fat, and sugar contributes to weight gain and a higher risk of obesity, which is an important risk factor in CVD. 51 Furthermore, our study showed that children who consumed high amounts of UPFs tended to have lower intakes of fruits and vegetables, which, along with a healthy dietary pattern, are known to be beneficial for cardiometabolic health. 52
Most of the associations were maintained in our study after further adjusting the models to Mediterranean diet adherence, suggesting that other intrinsic UPF factors may play an important role in determining these associations (eg, additives). Animal and cellular studies revealed potential cardiovascular risks from authorized additives such as sulfites, monosodium glutamate, and emulsifiers. 53 - 58 Food processing generates contaminants such as acrylamide and acrolein, which have been linked, respectively, to increased odds and risk of cardiovascular disease. 59 , 60 Ultraprocessed foods may contain chemicals such as bisphenols and perfluoroalkyl substances that have been associated with a higher risk of cardiometabolic outcomes in children. 61 , 62
The NOVA Food Classification system has sparked debate among researchers due to disagreements over UPF definitions, bias concerns, and the system’s contribution to dietary guidelines. 63 , 64 The NOVA system itself has some limitations, as it does not consider that certain minimal processing could improve the final product (eg, fermentation in milk) and adopts a vague definition of what is considered a cosmetic additive, which has led to considering carotenoids as an additive that increases the potential harmfulness of a product. 65 Despite these limitations, NOVA categories have consistently shown associations with cardiometabolic health in adults.
This study has several strengths. Most importantly, the study was conducted in a large sample size from 7 different geographic areas of Spain. The study also assessed cardiometabolic risk factors not considered in other similar studies. 21 - 24
Our study also has several limitations. First, because the study is observational, we cannot draw conclusions on cause and effect. Second, our study involved preschool children from Spain, which means that the generalization of our findings to different populations is not appropriate. Third, some grade of misclassification could be present in our study since UPF consumption was estimated from a food and beverage frequency questionnaire that was not specifically developed to assess this type of food, which could result in either an overestimation or underestimation of consumption within various NOVA categories. Additionally, imprecise estimations could also arise from the use of a food and beverage frequency questionnaire, which may be influenced by social desirability bias. Finally, we cannot dismiss that associations may be due to residual confounding or that undetected cardiometabolic disorders in our study population may exist due to age.
In this large cross-sectional study, UPFs consumption was positively associated with fasting plasma glucose levels, BMI, waist circumference, and fat mass index and inversely associated with HDL cholesterol concentration. These findings highlight the importance of promoting unprocessed or minimally processed foods and reducing UPF consumption, particularly starting from early ages. However, further prospective studies are warranted to validate our findings.
Accepted for Publication: March 16, 2024.
Published: May 17, 2024. doi:10.1001/jamanetworkopen.2024.11852
Open Access: This is an open access article distributed under the terms of the CC-BY License . © 2024 Khoury N et al. JAMA Network Open .
Corresponding Author: Nancy Babio, PhD, Unitat de Nutrició Humana, Departament de Bioquímica i Biotecnología, Universitat Rovira i Virgili, c/Sant Llorenç 21, 43201 Reus, Spain ( [email protected] ).
Author Contributions: Ms Khoury and Dr Babio had full access to all of the data in the study and take responsibility for the integrity of the data and the accuracy of the data analysis. Ms Khoury and Dr M. Á. Martínez contributed equally to this work as co–first authors. Prof Salas-Salvadó and Dr Babio contributed equally to this work as co–senior authors.
Concept and design: Khoury, M. Á. Martínez, Miguel-Berges, Navas-Carretero, Portoles, Leis, J. F. Martínez, Flores-Rojas, Moreno Aznar, Gil-Campos, Salas-Salvadó, Babio.
Acquisition, analysis, or interpretation of data: Khoury, M. Á. Martínez, Garcidueñas-Fimbres, Pastor-Villaescusa, Leis, Pérez-Vega, Jurado-Castro, Vázquez-Cobela, Andía Horno, J. A. Martínez, Picáns-Leis, Luque, Moreno Aznar, Castro-Collado, Gil-Campos, Salas-Salvadó, Babio.
Drafting of the manuscript: Khoury, Leis, de las Heras Delgado, Vázquez-Cobela, Andía Horno, J. A. Martínez, Flores-Rojas, Salas-Salvadó, Babio.
Critical review of the manuscript for important intellectual content: Khoury, M. Á. Martínez, Garcidueñas-Fimbres, Pastor-Villaescusa, Leis, Miguel-Berges, Navas-Carretero, Portoles, Pérez-Vega, Jurado-Castro, Vázquez-Cobela, J. A. Martínez, Picáns-Leis, Luque, Moreno Aznar, Castro-Collado, Gil-Campos, Salas-Salvadó, Babio.
Statistical analysis: Khoury, Garcidueñas-Fimbres, de las Heras Delgado, Vázquez-Cobela.
Obtained funding: Khoury, Navas-Carretero, J. A. Martínez, Flores-Rojas, Moreno Aznar, Gil-Campos, Salas-Salvadó, Babio.
Administrative, technical, or material support: Leis, Pérez-Vega, Vázquez-Cobela, Andía Horno, J. A. Martínez, Picáns-Leis, Luque, Gil-Campos, Salas-Salvadó, Babio.
Supervision: M. Á. Martínez, Leis, Portoles, Vázquez-Cobela, J. A. Martínez, Picáns-Leis, Luque, Moreno Aznar, Gil-Campos, Babio.
Conflict of Interest Disclosures: Prof Salas-Salvadó reported being a nonpaid member of the scientific boards of the International Nut and Dried Fruit Foundation, Danone Institute International, and Fundación Eroski; receiving institutional grants from the International Nut and Dried Fruit Foundation; and receiving personal fees from Danone Institute Spain. No other disclosures were reported.
Funding/Support: The establishment of the Childhood Obesity Risk Assessment Longitudinal Study (CORALS) cohort in the first year of the study (2019) was supported by an agreement between Consorcio Centro de Investigación Biomédica en Red, M. P. Fisiopatología de la Obesidad y Nutrición and Danone Institute Spain. This study was supported by grants 2021FI B 00145 from the Agència de Gestió d’Ajuts Universitaris de Recerca (Ms Khoury) and CD21/00045 from Sara Borrell (Dr M. Á. Martínez).
Role of the Funder/Sponsor: The funders had no role in the design and conduct of the study; collection, management, analysis, and interpretation of the data; preparation, review, or approval of the manuscript; and decision to submit the manuscript for publication.
Data Sharing Statement: See Supplement 2 .
Additional Contributions: The authors thank all the CORALS participants and their parents or caregivers as well as the health centers and primary schools for their collaboration, the CORALS personnel for outstanding support, and the staff of all associated primary care centers for exceptional work. The authors also acknowledge the Institut d’Investigació Sanitària Pere Virgili Biobank (PT20/00197), which is integrated in the Instituto de Salud Carlos III Platform for Biobanks and Biomodels.
- Register for email alerts with links to free full-text articles
- Access PDFs of free articles
- Manage your interests
- Save searches and receive search alerts
- Open access
- Published: 10 May 2024
Association of neutrophil-lymphocyte ratio with all-cause and cardiovascular mortality in US adults with diabetes and prediabetes: a prospective cohort study
- Guangshu Chen 1 na1 ,
- Li Che 2 , 3 na1 ,
- Meizheng Lai 1 ,
- Ting Wei 4 ,
- Chuping Chen 1 ,
- Ping Zhu 1 &
- Jianmin Ran 1
BMC Endocrine Disorders volume 24 , Article number: 64 ( 2024 ) Cite this article
245 Accesses
Metrics details
The neutrophil-lymphocyte ratio (NLR) is a novel hematological parameter to assess systemic inflammation. Prior investigations have indicated that an increased NLR may serve as a potential marker for pathological states such as cancer and atherosclerosis. However, there exists a dearth of research investigating the correlation between NLR levels and mortality in individuals with diabetes and prediabetes. Consequently, this study aims to examine the connection between NLR and all-cause as well as cardiovascular mortality in the population of the United States (US) with hyperglycemia status.
Data were collected from a total of 20,270 eligible individuals enrolled for analysis, spanning ten cycles of the National Health and Nutrition Examination Survey (NHANES) from 1999 to 2018. The subjects were categorized into three groups based on tertiles of NLR levels. The association of NLR with both all-cause and cardiovascular mortality was evaluated using Kaplan-Meier curves and Cox proportional hazards regression models. Restricted cubic splines were used to visualize the nonlinear relationship between NLR levels and all-cause and cardiovascular mortality in subjects with diabetes after accounting for all relevant factors.
Over a median follow-up period of 8.6 years, a total of 1909 subjects with diabetes died, with 671 deaths attributed to cardiovascular disease (CVD). And over a period of 8.46 years, 1974 subjects with prediabetes died, with 616 cases due to CVD. The multivariable-adjusted hazard ratios (HRs) comparing high to low tertile of NLR in diabetes subjects were found to be 1.37 (95% CI, 1.19–1.58) for all-cause mortality and 1.63 (95% CI, 1.29–2.05) for CVD mortality. And the correlation between high to low NLR tertile and heightened susceptibility to mortality from any cause (HR, 1.21; 95% CI, 1.03–1.43) and CVD mortality (HR, 1.49; 95% CI, 1.08–2.04) remained statistically significant (both p -values for trend < 0.05) in prediabetes subjects. The 10-year cumulative survival probability was determined to be 70.34%, 84.65% for all-cause events, and 86.21%, 94.54% for cardiovascular events in top NLR tertile of diabetes and prediabetes individuals, respectively. Furthermore, each incremental unit in the absolute value of NLR was associated with a 16%, 12% increase in all-cause mortality and a 25%, 24% increase in cardiovascular mortality among diabetes and prediabetes individuals, respectively.
Conclusions
The findings of this prospective cohort study conducted in the US indicate a positive association of elevated NLR levels with heightened risks of overall and cardiovascular mortality among adults with diabetes and prediabetes. However, potential confounding factors for NLR and the challenge of monitoring NLR’s fluctuations over time should be further focused.
Peer Review reports
The increasing rates of diabetes worldwide and the high number of diabetes-related deaths, especially from cardiovascular issues, have led to a focus on identifying factors that can predict mortality in individuals with diabetes [ 1 , 2 , 3 ]. Extensive research has consistently revealed a strong association between cardiovascular disease and inflammatory biomarkers [ 4 , 5 ]. The body’s innate (neutrophils) and adaptive (lymphocytes) immune responses are balanced by the neutrophil-to-lymphocyte ratio [ 6 ]. It has recently gained recognition as a valuable indicator of systemic inflammation, encompassing both infectious and non-infectious conditions, such as cardiovascular disease [ 7 , 8 ], tumors [ 9 , 10 , 11 , 12 ], septicemia [ 13 , 14 ], and mental disorders [ 15 , 16 ]. The development of diabetes may be caused by chronic inflammation, according to research [ 17 , 18 ].
However, because of the related costs and measurement challenges, the use of several inflammatory markers in ordinary clinical practice has been restricted. Neutrophils’ negative effects on blood vessel linings are measured with NLR, an easy-to-use, affordable test based on well-studied white blood cell traits. Few cohort studies have examined the relationship between NLR levels and long-term health effects [ 19 , 20 , 21 , 22 ]. Regrettably, most of these studies have mainly concentrated on investigating the correlation between NLR and diabetes-related complications. To date, there has been limited scholarly investigation into the correlation between NLR and mortality among individuals with diabetes and prediabetes. Thus, the goal of this study is to look at the relationship between NLR and cardiovascular and overall mortality in US adults with hyperglycemia.
The design and population of the study
A population-based cross-sectional survey, the NHANES was created expressly to collect detailed information on the health and nutritional status of US households. The NHANES interview component encompasses inquiries on demographics, socioeconomic factors, dietary habits, and health-related matters and is accessible to external researchers. The NHANES study methodology has been extensively described by the US Centers for Disease Control and Prevention [ 23 ]. The National Center for Health Statistics granted approval for NHANES, and each participant gave written consent. Data from 10 cycles of NHANES conducted between 1999 and 2018 were utilized. Initially, a total of 52,398 individuals aged 20 years and above were included. Subsequently, 1159 cases of pregnant women were excluded, screening out 9433 diabetes cases and 17,200 prediabetes cases according to diagnosis standards. Then we exclude cases without complete data on NLR and within 1% extreme of NLR. To consider the possible influence of glucocorticoid-steroid usage on neutrophil and lymphocyte levels, extra individuals who had consumed oral or inhaled cortisol in the previous month were not included. Furthermore, cases were excluded due to factors such as inadequate follow-up time, and mortality within two years. In the analysis, a grand total of 7246 cases of eligible diabetes and 13,024 cases of eligible prediabetes were ultimately considered (refer to Fig. 1 ).
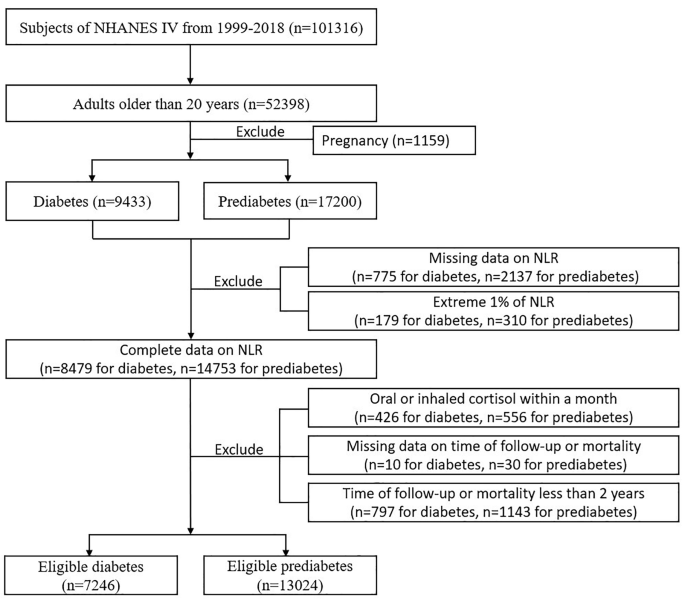
Flowchart about the inclusion and exclusion of eligible subjects
Based on NLR tertiles, baseline characteristics of participants with diabetes and prediabetes were acquired. The study employed weighted Kaplan-Meier (KM) survival curves to investigate differences in overall and CVD mortality among different NLR levels. Cumulative survival rates were presented in a risk table as weighted percentages. Among hyperglycemia subjects, dose-response relationships between NLR and mortality were demonstrated using restricted cubic splines (RCS) curves. The RCS curves depicted hazard ratios and 95% confidence intervals (CIs) through a solid line and gray shading. Any variants that influence neutrophil and lymphocyte counts were considered in our models to adjust the association between NLR and mortality. The models were modified to account for factors such as age, gender, ethnicity, level of education, ratio of family income to poverty, drinking habits, smoking habits, BMI, eGFR, HbA1c levels, duration of diabetes, medication for lowering glucose, CVD, hypertension, hyperlipidemia, cancer, chronic obstructive pulmonary disease (COPD), depression, and anemia. We further stratified different confounders to see the interaction effect on the association of NLR with overall and CVD mortality.
Assessment of hyperglycemia and NLR
Diabetes was characterized by fulfilling any of the subsequent conditions: surpassing 7.0 mmol/L in fasting plasma glucose levels, having random plasma glucose levels or 2 h-glucose of 75-g oral glucose tolerance above 11.0 mmol/L, exhibiting HbA1c levels of 6.5% or greater (with serum hemoglobin level higher than 100 g/dL), utilizing insulin or self-reporting a medical professional’s diagnosis. Prediabetes was diagnosed according to one of the following conditions: fasting plasma glucose levels being 5.6-7.0mmol/L, random plasma glucose levels or 2 h-glucose of 75-g oral glucose tolerance being 7.8–11.0 mmol/L, HbA1c levels being 5.7–6.4% (with serum hemoglobin level higher than 100 g/dL), or self-reported history.
Automated hematology analyzing devices were used to obtain the counts of lymphocytes and neutrophils, with the unit expressed as ×1,000 cells/mm3. To calculate the neutrophil-to-lymphocyte ratio, divide the count of neutrophils by the count of lymphocytes. We categorized NLR into tertiles to further explore the relationship between different levels of NLR and mortality.
All-cause and CVD mortality ascertainment
Mortality data, including all-cause and cardiovascular disease outcomes, were obtained from the National Death Index linked to the NHANES database until the end of December 2019.
The follow-up period persisted until the time of death or the conclusion of the period, starting from the date of blood analysis. The International Classification of Diseases, Tenth Revision (ICD-10) codes I00–09, I11, I13, I20–51, and I60–69 were used to identify mortality related to cardiovascular disease.
Covariates assessment
Baseline data on eligible respondents were gathered using the Computer-Assisted Personal Interviewing (CAPI) system and the Family and Sample Person Demographics questionnaires. This data included information on age, gender, ethnicity, education level, family income-poverty ratio, smoking and drinking habits, usage of glucose-lowering medication, healthy eating index (HEI) scores, and past medical history such as cardiovascular disease (coronary heart disease, congestive heart failure, angina, heart attack or stroke), hypertension, cancer, and COPD. The NHANES protocol was used to assign weights to all baseline data. Using the physical examination data from NHANES, the body mass index (BMI) was calculated by dividing the body weight (measured in kilograms) by the square of the height (measured in meters). The study collected neutrophil, lymphocyte, and hemoglobin counts from a peripheral whole-blood test. Furthermore, serum creatinine, HbA1c, TC, LDL-C, HDL-C, TG, and fasting glucose were obtained through laboratory tests. To guarantee precise and uniform blood test procedures, the NHANES followed the Laboratory Procedure Manual. Interviewers recorded the duration of diabetes, considering newly diagnosed cases as having a duration of 0 year. The CKD-EPI equation was utilized to determine the estimated glomerular filtration rate (eGFR). Hyperlipidemia was characterized by fulfilling any of the subsequent conditions: total cholesterol (TC) levels equal to or exceeding 200 mg/dL, triglyceride (TG) levels equal to or surpassing 150 mg/dL, high-density lipoprotein cholesterol (HDL-C) levels less than or equal to 40 mg/dL in males and 50 mg/dL in females, low-density lipoprotein cholesterol (LDL-C) levels equal to or exceeding 130 mg/dL, or self-reported utilization of medications for reducing cholesterol. The depression group was identified using the PHQ-9 [ 24 ]. Depression status was defined as having a depression score greater than four. Anemia was diagnosed by establishing the serum hemoglobin (Hb) threshold (g/dL) for different demographic groups (non-pregnant women over 15 years old with levels below 120 g/dL, and men over 15 years old with levels below 130 g/dL). The HEI-2015 scores were employed as an indicator of dietary quality, with a higher score indicating a more nutritionally balanced diet [ 25 ]. MET scores were utilized to evaluate physical activity levels following the national physical activity guidelines (low physical activity being defined as less than 500 MET/wk and high physical activity being defined as 500 MET/wk or more) [ 26 ].
Statistical analysis
Cox proportional hazards regression after survey-weight was used to explore the association of NLR level with overall and CVD-specific mortality based on different models in diabetes and prediabetes groups. All data were adjusted for survey weights in accordance with the analytic guidelines provided by NHANES due to the complex design. Whereas continuous data were shown as mean (standard error), categorical variables were shown as numbers (percentages). To investigate the disparities among groups in terms of baseline characteristics, we employed Weighted Chi-Square tests and Kruskal-Wallis tests. Using several models in the diabetic and prediabetes groups, Cox proportional hazards regression was used to assess the internal connection between NLR levels and overall and cardiovascular-specific death. The hazard ratios and 95% CIs were derived through survey-weighted calculations. We conducted three models to explore the relationship. Model 1 took into account factors such as age (below 65 or over 65), sex (male or female), race/ethnicity (Hispanic Mexican, non-Hispanic Black, non-Hispanic White, or others), marital status (married/cohabiting, single), family income-to-poverty ratio (< 1.0, 1.0–3.0, or ≥ 3.0), and education level (less than high school, high school or equivalent, or college or above). Model 2 included BMI (< 30 or ≥ 30.0 kg/m2), smoking status (never, former, or current), drinking status (non-drinker or ever drinker), physical activity (low or high), HEI scores, and cancer (no or yes) as additional adjustments. Model 3 further adjusted for eGFR (< 30, 30–60, >=60 ml/min/1.73 m²), anemia (no or yes), hypertension (no or yes), hyperlipidemia (no or yes), depression (no or yes), COPD (no or yes), use of hypotensive drug (no or yes), and use of lipid-lowering drug (no or yes) based on model 2. Diabetes duration, HbA1c levels, and use of antidiabetic drugs were adjusted additionally for diabetes subjects on model 3. The study employed statistical analysis to establish the first tertile of NLR as the reference group for evaluating the correlation between moderate-high NLR levels and mortality across various models. To handle missing values in the covariates of the study, we employed the technique of multiple imputation [ 27 ].
Weighted KM curves were employed to depict cumulative overall and CVD survival probability, stratified by tertiles of NLR levels. The risk table presented precise information regarding deaths and survival probability at different follow-up intervals. After fully adjusting for the mentioned covariates, RCS curves were utilized to visually depict the nonlinear correlation between NLR levels and both overall and CVD mortality in individuals with diabetes and prediabetes.
Subgroup analyses were performed to look at the relationship between NLR levels and death in people with diabetes and prediabetes. The subgroups were categorized using a variety of clinical and demographic characteristics, including age, gender, ethnicity, education, BMI, drinking and smoking habits, physical activity levels, and the occurrence of cancer, hypertension, and CVD. To ensure the strength of our findings, we performed sensitivity analyses. A particular analysis was carried out on individuals who had a prior record of cardiovascular disease or not. Following that, individuals who reported having no CVD and cancer at the baseline condition were also analyzed. The data underwent analysis using R software version 4.2.2 (R Foundation for Statistical Computing, Vienna, Austria), and a two-sided P -value < 0.05 was utilized to ascertain statistical significance.
Baseline characteristics analyses
Our study included a total of 7246 and 13,024 adults aged 20 years or older who had been diagnosed with diabetes and prediabetes, respectively. In the diabetes group, the participants’ average age was 58.6 years, with males accounting for 51.50% and whites representing 35.59%. In the prediabetes group, the participants’ average age was 52.0 years, with males accounting for 52.62% and whites representing 41.75%. For all-cause mortality, approximately one-third of the subjects died from cardiovascular disease both in the diabetes and prediabetes group. Therefore, we conducted an analysis of hazard ratios for both all-cause and CVD mortality. The subjects were categorized into three groups based on tertiles of NLR levels: tertile 1 (0.68–1.71), tertile 2 (0.71–2.48), and tertile 3 (2.48–7.58) for diabetes subjects and tertile 1 (0.62–1.60), tertile 2 (1.60–2.29), tertile 3 (2.29–6.23) for prediabetes subjects. For diabetes subjects, in comparison to the lower tertile of NLR, individuals in the upper tertile of NLR exhibited characteristics such as advanced age, male gender, non-Hispanic white ethnicity, higher educational attainment, and lower HbA1c levels. Additionally, they demonstrated a greater prevalence of alcohol consumption, smoking, hypertension, CVD, cancer, COPD, and anemia. Furthermore, this group displayed moderate family income, BMI, and eGFR. Such a trend was seen in prediabetes subjects, as indicated in Table 1 .
All-cause and CVD mortality with tertiles of NLR levels and survival analyses
Over a period of 8.0 years, 1909 people with diabetes died, with 671 cases attributed to cardiovascular causes, 305 cases to cancer, and 933 cases to other causes. And over a period of 8.46 years, 1974 people with prediabetes died, with 616 cases attributed to cardiovascular causes, 479 cases to cancer, and 879 cases to other causes. The hazard ratios for all-cause and CVD mortality among individuals with diabetes and prediabetes, based on tertiles of NLR levels, are presented in Table 2 a and Table 2 b, respectively.
After adjusting for potential confounders, every unit increment in the absolute value of NLR, equivalent to 50SD in diabetes subjects or 100SD in prediabetes subjects, resulted in a 16% higher risk of mortality from any cause (HR, 1.16; 95% CI, 1.10–1.23) and a 25% higher risk of mortality from cardiovascular disease (HR 1.25; 95% CI 1.14–1.37) in diabetes subjects, and a 12% higher risk of mortality from overall cause (HR, 1.12; 95% CI, 1.05–1.19) and a 24% higher risk of mortality from cardiovascular disease (HR 1.24; 95% CI 1.11–1.37) in prediabetes subjects. The hazard ratios for all-cause mortality and CVD mortality after multiple adjustments in diabetes subjects, comparing high to low NLR tertile, were found to be 1.37 (95% CI, 1.19–1.58) and 1.63 (95% CI, 1.29–2.05), respectively. And the correlation between high to low NLR tertile and heightened susceptibility to mortality from any cause (HR, 1.21; 95% CI, 1.03–1.43) and CVD mortality (HR, 1.49; 95% CI, 1.08–2.04) remained statistically significant (both p -values for trend < 0.05) in prediabetes subjects.
The Kaplan-Meier survival curves, adjusted for weights, were analyzed based on tertiles of NLR. The findings indicated that individuals in the highest tertile of NLR exhibited the lowest cumulative probability of survival for both all-cause and cardiovascular events, as depicted in Fig. 2 . Specifically, when NLR levels exceeded 2.48 in diabetes participants, the 10-year cumulative survival probability was determined to be 70.34% for all-cause events and 86.21% for cardiovascular events. And when NLR levels exceeded 2.29 in prediabetes participants, the 10-year cumulative survival probability was found to be 84.65% for all-cause events and 94.54% for cardiovascular events.
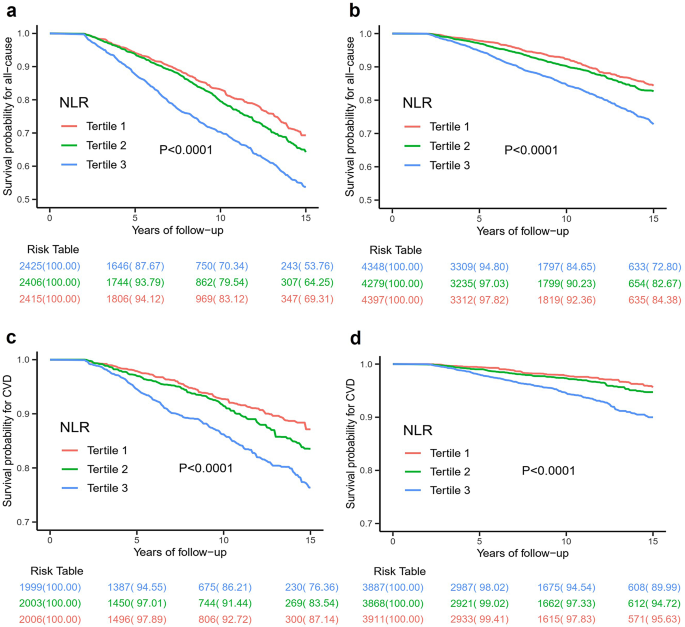
Weighted Kaplan–Meier survival curves for all-cause mortality for diabetes ( a ) and prediabetes ( b ) subjects according to tertiles of NLR. Tertiles of NLR in diabetes subjects: Tertile 1: 0.68–1.71; Tertile 2: 1.71–2.48; Tertile 3: 2.48–7.58; Tertiles of NLR in prediabetes subjects: Tertile 1: 0.62–1.60; Tertile 2: 1.60–2.29; Tertile 3: 2.29–6.23. Weighted Kaplan–Meier survival curves for CVD mortality for diabetes ( c ) and prediabetes ( d ) subjects according to tertiles of NLR. Tertiles of NLR in diabetes subjects: Tertile 1: 0.68–1.68; Tertlie 2: 1.68–2.42; Tertile 3: 2.42–7.58; Tertiles of NLR in prediabetes subjects: Tertile 1: 0.62–1.58; Tertile 2: 1.58–2.25; Tertile 3: 2.25–6.23
The RCS curves effectively depicted the non-linear correlation between NLR levels and both overall and cardiovascular mortality among adults with diabetes and prediabetes, following comprehensive adjustments as illustrated in Fig. 3 . Significantly, there was a clear correlation between NLR levels and the mentioned mortality outcomes, demonstrating a dose-response relationship ( P -value = 0). Furthermore, our analysis revealed a positive linear trend in the correlation between NLR levels and both overall and cardiovascular death, as evidenced by non-significant P -values for nonlinearity ( P -value = 0.706 and 0.997, respectively) in diabetes group and nonlinearity ( P -value = 0.229 and 0.279, respectively) in prediabetes group.
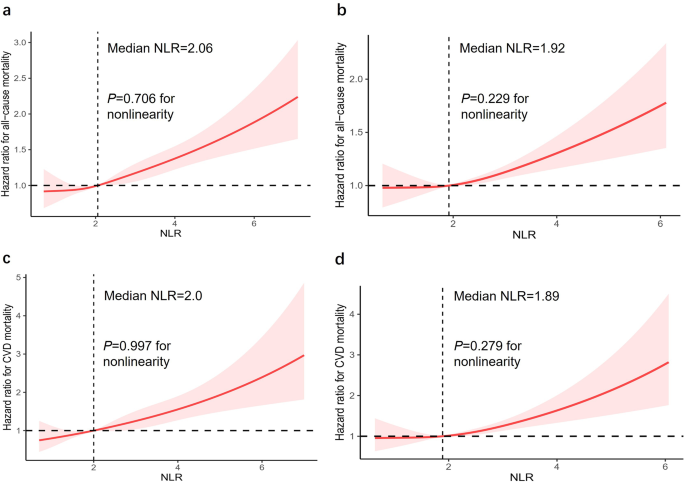
Dose-response associations between NLR and all-cause mortality in diabetes ( a ) and prediabetes ( b ) subjects. Both p -values for overall was 0. p -value for nonlinearity in diabetes and prediabetes subjects was 0.706 and 0.229, respectively. Dose-response associations between NLR and CVD mortality in diabetes ( c ) and prediabetes ( d ) subjects. Both p-values for overall was 0. p -value for nonlinearity in diabetes and prediabetes subjects was 0.997 and 0.279, respectively. The solid line and gray shading showed hazard ratios and 95% CIs, respectively. Models were adjusted for age, sex, race/ethnicity, education level, family income-poverty ratio, drinking status, smoking status, BMI, eGFR, CVD, hypertension, hyperlipidemia, cancer, COPD, depression status, anemia, physical activity, HEI scores, use of hypotensive drug, use of lipid-lowering drug. Diabetes duration, HbA1c, and use of antidiabetic drug were adjusted additionally for diabetes subjects
Subgroup and sensitivity analyses
A noteworthy correlation was observed between NLR levels and baseline history of CVD in the prediabetes group for CVD mortality ( P = 0.02 for interaction) (Fig. 4 c, d). In the subset of prediabetes individuals without a prior history of CVD, the modified hazard ratio (95% CI) for CVD mortality was 1.15 (1.08, 1.24). On the other hand, in the subset of prediabetes individuals who have a previous CVD, the CVD mortality had an adjusted hazard ratio (95% CI) of 1.05 (0.95, 1.17). The association between NLR levels and overall mortality in hyperglycemia subjects remained stable irrespective of different stratifying factors (Fig. 4 a, b), including age, gender, ethnicity, educational attainment, family income-poverty ratio, alcohol consumption, tobacco use, BMI, eGFR, CVD, hypertension, hyperlipidemia, cancer, COPD, depression, anemia, use of hypotensive drug, and use of lipid-lowering drug. Diabetes duration, HbA1c level, and use of antidiabetic drug were adjusted additionally for diabetes subjects.
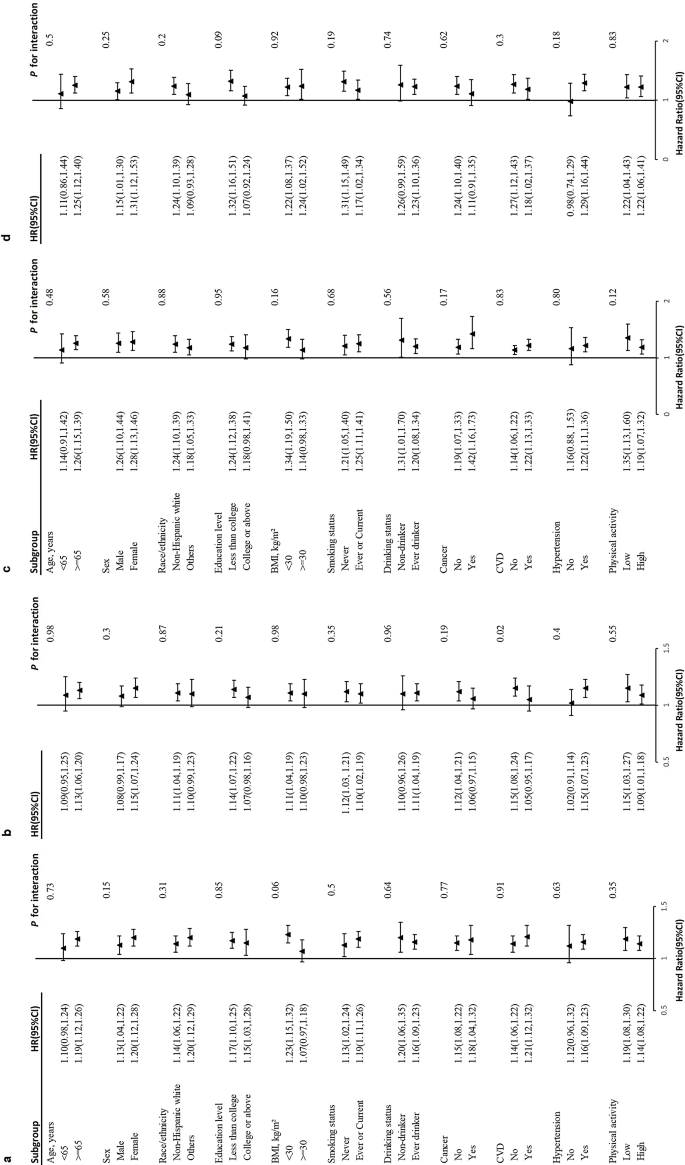
Stratified analyses of the association between NLR and all-cause mortality in diabetes ( a ) and prediabetes ( b ) subjects. Stratified analyses of the association between NLR and CVD mortality in diabetes ( c ) and prediabetes ( d ) subjects. Models were adjusted for age, sex, race/ethnicity, education level, family income-poverty ratio, drinking status, smoking status, BMI, eGFR, CVD, hypertension, hyperlipidemia, cancer, COPD, depression, anemia, physical activity, HEI scores, use of hypotensive drug, use of lipid-lowering drug except for the corresponding subgroup variables. Diabetes duration, HbA1c and use of antidiabetic drug were adjusted additionally for diabetes subjects
In the subgroup without prior history of CVD, the multiple-adjusted hazard ratios for all-cause mortality and CVD mortality in diabetes subjects, comparing high to low NLR tertile, were found to be 1.33(95% CI, 1.09–1.61) and 1.93 (95% CI, 1.34–2.78), respectively. And the correlation between high to low NLR tertile and mortality from any cause (HR, 1.26; 95% CI, 1.04–1.54) remained statistically significant in prediabetes subjects (Table S1 a and S1 b). In the subgroup without a baseline history of CVD and cancer, the hazard ratios for overall and cardiovascular mortality exhibit similar significant differences (Table S3 a and S3 b). In the subgroup with a history of CVD, the hazard ratios for all-cause mortality after full adjustments in diabetes subjects, comparing high to low NLR tertile, were found to be 1.57 (95% CI, 1.28–1.593). And the correlation between high to low NLR tertile and mortality from CVD events (HR, 1.62; 95% CI, 1.05–2.50) remained statistically significant in prediabetes subjects (Table S2 a and S2 b).
Our study included 20,270 hyperglycemia subjects with a longer than 8 years of follow-up period. By the conclusion of the study, a total of 1909 deaths were recorded in the diabetes group, with 671 attributed to cardiovascular causes and 1238 attributed to other diseases. And a total of 1974 deaths were recorded in the prediabetes group, with 616 attributed to cardiovascular causes and 1358 attributed to other causes. The findings indicated that participants with elevated levels of NLR exhibited lower levels of HbA1c but a higher prevalence of cardiovascular disease and cancer at the beginning of the study. Additionally, these individuals demonstrated a higher incidence of both overall and cardiovascular mortality. Consequently, it can be inferred that heightened NLR levels may serve as an independent prognostic factor for mortality from any cause and cardiovascular disease in hyperglycemia people.
Many investigations have explored the correlation between NLR and the long-term complications and prognosis of diabetes [ 21 , 22 , 28 ]. For Scottish diabetic populations, higher NLR levels were found to increase the prevalence of retinopathy [ 21 ], particularly among individuals below the age of 65 and those with well-managed glycemic control. A study from Rio de Janeiro [ 29 ] noted that elevated NLR increased all-cause mortality by up to 19% in patients with type 2 diabetes mellitus (at 10.5 years of follow-up), but the sample size of the study was only 689 people. Through the analysis of a substantial sample size of 32,328 subjects from the NHANES database, Chen discovered a significant association between elevated NLR and increased risk of overall and cardiovascular death in the general population [ 30 ]. When the NLR value was greater than 3, the general population had a 43% increased risk of all-cause mortality and a 44% increased risk of cardiovascular mortality. In contrast, the NLR levels in our study were divided by tertiles, and when the NLR value was greater than 2.48, the diabetic population had a similar risk of all-cause mortality as the general population, but a higher risk of cardiovascular mortality. Such a trend was also significant in subjects with prediabetes. By analyzing seven cycles of 3251 diabetic patients in the NHANSE database, Dong et al. found a strong link between high NLR levels and increased risks of death and heart-related death in people with diabetes [ 31 ]. When the NLR was greater than 3.48, diabetic patients had a doubled risk of all-cause mortality and a 1.8-fold increase in cardiovascular mortality. However, this study failed to adequately incorporate covariates that affect the outcome, such as underlying cardiovascular disease, history of cancer, lifestyle, and medication use, and did not take into account subjects with prediabetes. While most studies have focused on the association of NLR with complications and poor prognosis of diabetes, few studies have been conducted on the association of NLR with poor prognosis of prediabetes. To our current understanding, our study represents the initial comprehensive examination of the relationship between NLR levels and mortality in both diabetes and prediabetes populations after full consideration of multivariable adjustments.
Our research found that diabetes individuals with NLR levels above 2.48 had a significantly higher risk of mortality from any cause (37%) and cardiovascular disease (63%) compared to those with lower NLR levels. Additionally, our dose-response analysis demonstrated a positive correlation between NLR levels and both all-cause and CVD mortality. After accounting for confounding factors in diabetes subjects, there was a 16% increased risk of all-cause mortality and a 25% increased risk of CVD mortality for every unit increase in the absolute value of NLR. The Kaplan-Meier survival plots indicated a notable correlation between heightened NLR levels and heightened susceptibility to mortality from any cause and cardiovascular disease. These findings provide compelling evidence for the close correlation between elevated NLR levels and unfavorable outcomes in terms of overall and cardiovascular mortality. In our study, prediabetes has a higher risk of death due to its progression to diabetes, highlighting the importance of inflammatory markers in predicting poor outcomes in those with high blood sugar. Therefore, it is plausible that NLR, serving as an inflammation marker, may possess intrinsic abilities to predict the probability of all-cause and cardiovascular mortality in individuals with diabetes and prediabetes. The evaluation of the NLR is subject to various influencing factors, including age, race, corticosteroid usage, and the presence of chronic diseases such as cancer, diabetes, obesity, depression, neoplasms, heart disease, and anemia. These factors impact the function, activity, behavior, and dynamic changes of neutrophil and lymphocyte counts [ 32 , 33 , 34 ]. Utilizing the extensive and reliable NHANES database, this study comprehensively accounted for confounding variables related to NLR. It ensured the credibility of NLR’s predictive value for mortality risk in hyperglycemia subjects. There is no normal reference range for NLR in healthy adults, and the average level of NLR is related to race [ 35 , 36 , 37 ]. Forget et al. [ 36 ] found that typical NLR values in a group of healthy, non-elderly people ranged from 0.78 to 3.53 in a sizable retrospective case-control study. In our research, we found a significant increase in deaths from any cause and cardiovascular events among diabetic adults with NLR levels above 2.48 and prediabetic adults with NLR levels above 2.29.
Our primary findings were robust and withstood rigorous sensitivity analyses. Without the influence of a baseline history of CVD and cancer, the relationship between different levels of NLR and mortality was still notable. In subgroup analyses, a noteworthy interaction is observed solely between NLR levels and baseline CVD history among prediabetes subjects. Within this subgroup, participants lacking a history of CVD exhibit a higher risk of overall mortality unexpectedly. This trend persists across three distinct models in sensitivity analyses, particularly among individuals with higher tertile NLR levels. The improvement can likely be credited to the common use of certain diabetes drugs, like GLP-1R agonists and SGLT-2 inhibitors, which have been shown to greatly enhance heart health and reduce death rates [ 38 , 39 , 40 ]. These drugs are often prescribed to diabetics with heart conditions, but people without known heart issues might not get them as promptly or at all. In diabetic participants with concurrent cardiovascular disease, the predictive value of NLR in determining cardiovascular mortality outcomes may be limited. In contrast, NLR may be more valuable in identifying adverse outcomes in participants who have not yet developed cardiovascular disease.
This prospective cohort study possesses several strengths. Notably, the routine administration of a peripheral whole blood test ensured the inclusion of a broader population, owing to its affordability and clinical relevance. As a result, the findings obtained in relation to the correlation between the NLR and the risk of mortality in individuals with diabetes and prediabetes are considered to be more reliable. Ultimately, 7246 eligible diabetes subjects and 13,024 eligible prediabetes subjects were successfully enrolled in the study. Afterward, considering that NLR is susceptible to a variety of possible factors and that there is a correlation between overall and cardiovascular mortality and hyperglycemia, we performed a thorough adjustment for known confounding variables. We developed several clinical models to fully assess the prognostic significance of NLR in the mortality of individuals with diabetes and prediabetes. Nonetheless, it is important to acknowledge that this study has some limitations, as certain unidentified confounding variables may not have been accounted for during the adjustment process. NHANES did not provide information on acute illnesses during blood collection or the reliability of neutrophil and lymphocyte counts. Participants with extreme NLR values, 1%, were removed from the analysis to reduce disease-related distortion and improve the accuracy of the initial NLR assessment. It is essential to highlight that NLR indicates the relative equilibrium between the body’s bone marrow and lymphocyte profiles, and monitoring the dynamic changes in NLR is crucial for predicting mortality outcomes in hyperglycemia adults. The NHANES database did not include any follow-up data on neutrophils and lymphocytes, thus precluding the ability to elucidate the influence of changes in NLR on mortality outcomes. Nevertheless, our study unequivocally demonstrates a positive association between elevated baseline NLR levels and increased risks of all-cause and cardiovascular mortality in participants with diabetes and prediabetes. Therefore, we expect that forthcoming prospective studies will be carried out to determine the exact significance of NLR in forecasting mortality associated with overall and cardiovascular mortality in adults with hyperglycemia.
The measurement of NLR is readily accessible and economically viable in clinical practice. Our study has demonstrated the potential of NLR as a predictive factor for mortality in subjects with both diabetes and prediabetes. In addition to conventional risk factors, it is crucial to acknowledge the impact of low-grade inflammation on the adverse outcomes of all-cause and cardiovascular disease mortality in the hyperglycemia population. It’s crucial to explore other factors that could affect NLR and tackle the difficulties in tracking its changes over time.
Data availability
All data used and analyzed in this study were available on the NHANES website.
Abbreviations
Body mass index
Computer-Assisted Personal Interviewing
Confidence intervals
Chronic obstructive pulmonary disease
Cardiovascular disease
Estimated glomerular filtration rate
Glycated hemoglobin A1c
High-density lipoprotein cholesterol
Healthy eating index
Hazard ratios
Low-density lipoprotein cholesterol
National Health and Nutrition Examination Survey
- Neutrophil-lymphocyte ratio
Total cholesterol
Triglyceride
United States
Kähm K, Laxy M, Schneider U, Rogowski WH, Lhachimi SK, Holle R. Health care costs associated with incident complications in patients with type 2 diabetes in Germany. Diabetes Care. 2018;41(5):971–8.
Article PubMed Google Scholar
Sun H, Saeedi P, Karuranga S, Pinkepank M, Ogurtsova K, Duncan BB, Stein C, Basit A, Chan JCN, Mbanya JC, et al. IDF diabetes atlas: global, regional and country-level diabetes prevalence estimates for 2021 and projections for 2045. Diabetes Res Clin Pract. 2022;183:109119.
Zheng Y, Ley SH, Hu FB. Global aetiology and epidemiology of type 2 diabetes mellitus and its complications. Nat Rev Endocrinol. 2018;14(2):88–98.
Liberale L, Badimon L, Montecucco F, Lüscher TF, Libby P, Camici GG. Inflammation, aging, and cardiovascular disease: JACC review topic of the week. J Am Coll Cardiol. 2022;79(8):837–47.
Article CAS PubMed PubMed Central Google Scholar
Akhmerov A, Parimon T. Extracellular vesicles, inflammation, and cardiovascular disease. Cells. 2022;11(14).
Buonacera A, Stancanelli B, Colaci M, Malatino L. Neutrophil to lymphocyte ratio: an emerging marker of the relationships between the Immune System and diseases. Int J Mol Sci 2022, 23(7).
Wang X, Chen X, Wang Y, Peng S, Pi J, Yue J, Meng Q, Liu J, Zheng L, Chan P, et al. The association of lipoprotein(a) and neutrophil-to-lymphocyte ratio combination with atherosclerotic cardiovascular disease in Chinese patients. Int J Gen Med. 2023;16:2805–17.
Khan UH, Pala MR, Hafeez I, Shabir A, Dhar A, Rather HA. Prognostic value of hematological parameters in older adult patients with acute coronary syndrome undergoing coronary intervention: a single centre prospective study. J Geriatr Cardiol. 2023;20(8):596–601.
Article PubMed PubMed Central Google Scholar
Savioli F, Morrow ES, Dolan RD, Romics L, Lannigan A, Edwards J, McMillan DC. Prognostic role of preoperative circulating systemic inflammatory response markers in primary breast cancer: meta-analysis. Br J Surg. 2022;109(12):1206–15.
Article Google Scholar
Russo E, Guizzardi M, Canali L, Gaino F, Costantino A, Mazziotti G, Lania A, Uccella S, Di Tommaso L, Ferreli F, et al. Preoperative systemic inflammatory markers as prognostic factors in differentiated thyroid cancer: a systematic review and meta-analysis. Rev Endocr Metab Disord. 2023;24(6):1205–16.
Article CAS PubMed Google Scholar
Yamamoto T, Kawada K, Obama K. Inflammation-related biomarkers for the prediction of prognosis in colorectal cancer patients. Int J Mol Sci. 2021;22(15).
Fan Z, Shou L. Prognostic and clinicopathological impacts of systemic immune-inflammation index on patients with diffuse large B-cell lymphoma: a meta-analysis. Ther Adv Hematol. 2023;14:20406207231208973.
Martins PM, Gomes TLN, Franco EP, Vieira LL, Pimentel GD. High neutrophil-to-lymphocyte ratio at intensive care unit admission is associated with nutrition risk in patients with COVID-19. JPEN J Parenter Enter Nutr. 2022;46(6):1441–8.
Article CAS Google Scholar
Huang Z, Fu Z, Huang W, Huang K. Prognostic value of neutrophil-to-lymphocyte ratio in sepsis: a meta-analysis. Am J Emerg Med. 2020;38(3):641–7.
Frota IJ, de Oliveira ALB, De Lima DN Jr., Costa Filho CWL, Menezes CES, Soares MVR, Chaves Filho AJM, Lós DB, Moreira RTA, Viana GA, et al. Decrease in cognitive performance and increase of the neutrophil-to-lymphocyte and platelet-to-lymphocyte ratios with higher doses of antipsychotics in women with schizophrenia: a cross-sectional study. BMC Psychiatry. 2023;23(1):558.
Karageorgiou V, Milas GP, Michopoulos I. Neutrophil-to-lymphocyte ratio in schizophrenia: a systematic review and meta-analysis. Schizophr Res. 2019;206:4–12.
Berbudi A, Rahmadika N, Tjahjadi AI, Ruslami R. Type 2 diabetes and its impact on the immune system. Curr Diabetes Rev. 2020;16(5):442–9.
PubMed PubMed Central Google Scholar
Rohm TV, Meier DT, Olefsky JM, Donath MY. Inflammation in obesity, diabetes, and related disorders. Immunity. 2022;55(1):31–55.
Guo X, Zhang S, Zhang Q, Liu L, Wu H, Du H, Shi H, Wang C, Xia Y, Liu X, et al. Neutrophil:lymphocyte ratio is positively related to type 2 diabetes in a large-scale adult population: a Tianjin chronic low-grade systemic inflammation and health cohort study. Eur J Endocrinol. 2015;173(2):217–25.
Liu X, Zhang Q, Wu H, Du H, Liu L, Shi H, Wang C, Xia Y, Guo X, Li C, et al. Blood neutrophil to lymphocyte ratio as a predictor of hypertension. Am J Hypertens. 2015;28(11):1339–46.
Rajendrakumar AL, Hapca SM, Nair ATN, Huang Y, Chourasia MK, Kwan RS, Nangia C, Siddiqui MK, Vijayaraghavan P, Matthew SZ, et al. Competing risks analysis for neutrophil to lymphocyte ratio as a predictor of diabetic retinopathy incidence in the Scottish population. BMC Med. 2023;21(1):304.
Wan H, Wang Y, Fang S, Chen Y, Zhang W, Xia F, Wang N, Lu Y. Associations between the neutrophil-to-lymphocyte ratio and diabetic complications in adults with diabetes: a cross-sectional study. J Diabetes Res. 2020;2020:6219545.
US Centers for Disease Control and Prevention. National health and nutrition examination survey. https://www.cdc.gov/nchs/nhanes/about_nhanes.htm (accessed Nov 19, 2020).
Manea L, Boehnke JR, Gilbody S, Moriarty AS, McMillan D. Are there researcher allegiance effects in diagnostic validation studies of the PHQ-9? A systematic review and meta-analysis. BMJ Open. 2017;7(9):e015247.
Qiu Z, Chen X, Geng T, Wan Z, Lu Q, Li L, Zhu K, Zhang X, Liu Y, Lin X, et al. Associations of serum carotenoids with risk of cardiovascular mortality among individuals with type 2 diabetes: results from NHANES. Diabetes Care. 2022;45(6):1453–61.
MacGregor KA, Gallagher IJ, Moran CN. Relationship between insulin sensitivity and menstrual cycle is modified by BMI, fitness, and physical activity in NHANES. J Clin Endocrinol Metab. 2021;106(10):2979–90.
Zhu P, Lao G, Chen C, Luo L, Gu J, Ran J. TSH levels within the normal range and risk of cardiovascular and all-cause mortality among individuals with diabetes. Cardiovasc Diabetol. 2022;21(1):254.
Mureșan AV, Tomac A, Opriș DR, Bandici BC, Coșarcă CM, Covalcic DC, Hălmaciu I, Akácsos-Szász OZ, Rădulescu F, Lázár K et al. Inflammatory markers used as predictors of subclinical atherosclerosis in patients with diabetic polyneuropathy. Life (Basel). 2023;13(9).
Cardoso CRL, Leite NC, Salles GF. Importance of hematological parameters for micro- and macrovascular outcomes in patients with type 2 diabetes: the Rio De Janeiro type 2 diabetes cohort study. Cardiovasc Diabetol. 2021;20(1):133.
Chen Y, Wang W, Zeng L, Mi K, Li N, Shi J, Yang S. Association between neutrophil-lymphocyte ratio and all-cause mortality and cause-specific mortality in US adults, 1999–2014. Int J Gen Med. 2021;14:10203–11.
Dong G, Gan M, Xu S, Xie Y, Zhou M, Wu L. The neutrophil-lymphocyte ratio as a risk factor for all-cause and cardiovascular mortality among individuals with diabetes: evidence from the NHANES 2003–2016. Cardiovasc Diabetol. 2023;22(1):267.
Papachristodoulou E, Kakoullis L, Christophi C, Psarelis S, Hajiroussos V, Parperis K. The relationship of neutrophil-to-lymphocyte ratio with health-related quality of life, depression, and disease activity in SLE: a cross-sectional study. Rheumatol Int. 2023;43(10):1841–8.
Golsorkhtabaramiri M, McKenzie J, Potter J. Predictability of neutrophil to lymphocyte ratio in preoperative elderly hip fracture patients for post-operative short-term complications: a retrospective study. BMC Musculoskelet Disord. 2023;24(1):227.
Duncan BB, Schmidt MI, Pankow JS, Ballantyne CM, Couper D, Vigo A, Hoogeveen R, Folsom AR, Heiss G. Low-grade systemic inflammation and the development of type 2 diabetes: the atherosclerosis risk in communities study. Diabetes. 2003;52(7):1799–805.
Howard R, Scheiner A, Kanetsky PA, Egan KM. Sociodemographic and lifestyle factors associated with the neutrophil-to-lymphocyte ratio. Ann Epidemiol. 2019;38:11–e2116.
Forget P, Khalifa C, Defour JP, Latinne D, Van Pel MC, De Kock M. What is the normal value of the neutrophil-to-lymphocyte ratio? BMC Res Notes. 2017;10(1):12.
Azab B, Camacho-Rivera M, Taioli E. Average values and racial differences of neutrophil lymphocyte ratio among a nationally representative sample of United States subjects. PLoS ONE. 2014;9(11):e112361.
Packer M, Anker SD, Butler J, Filippatos G, Pocock SJ, Carson P, Januzzi J, Verma S, Tsutsui H, Brueckmann M, et al. Cardiovascular and renal outcomes with empagliflozin in heart failure. N Engl J Med. 2020;383(15):1413–24.
Nauck MA, Quast DR, Wefers J, Meier JJ. GLP-1 receptor agonists in the treatment of type 2 diabetes - state-of-the-art. Mol Metab. 2021;46:101102.
Palmer SC, Tendal B, Mustafa RA, Vandvik PO, Li S, Hao Q, Tunnicliffe D, Ruospo M, Natale P, Saglimbene V, et al. Sodium-glucose cotransporter protein-2 (SGLT-2) inhibitors and glucagon-like peptide-1 (GLP-1) receptor agonists for type 2 diabetes: systematic review and network meta-analysis of randomised controlled trials. BMJ. 2021;372:m4573.
Download references
Acknowledgements
We express our gratitude to Jing Zhang from the Second Department of Infectious Disease at Shanghai Fifth People’s Hospital, Fudan University, for his valuable contributions to the NHANES database. His exceptional efforts in developing the nhanesR package and webpage have greatly facilitated the exploration of the NHANES database.
This work is supported by the Fundamental and Applied Research Project from Joint Funding between Municipal Government and University/College (202201020073).
Author information
Guangshu Chen and Li Che contributed equally to this work.
Authors and Affiliations
Department of Endocrinology and Metabolism, Guangzhou Red Cross Hospital, Jinan University, Guangzhou, 510220, Guangdong, China
Guangshu Chen, Meizheng Lai, Chuping Chen, Ping Zhu & Jianmin Ran
State Key Laboratory of Respiratory Disease, National Clinical Research Center for Respiratory Disease, Guangzhou Institute of Respiratory Health, The First Affiliated Hospital of Guangzhou Medical University, Guangzhou, 510120, China
Department of Pulmonary and Critical Care Medicine, The First Affiliated Hospital of Jinan University, Guangzhou, 510630, China
Department of Hematology, Guangzhou Red Cross Hospital, Jinan University, Guangzhou, 510220, China
You can also search for this author in PubMed Google Scholar
Contributions
JR and PZ took charge of conception and design. GC and LC wrote the main manuscript text. CC prepared Figs. 1 , 2 , 3 and 4 , ML and TW prepared Table 1 , and 2 , S1 - S3 . All authors finally approved the manuscript.
Corresponding authors
Correspondence to Ping Zhu or Jianmin Ran .
Ethics declarations
Ethics approval and consent to participate.
Approval for NHANES was acquired from the National Center for Health Statistics, and every participant provided written consent.
Consent for publication
Not Applicable.
Competing interests
The authors declare no competing interests.
Additional information
Publisher’s note.
Springer Nature remains neutral with regard to jurisdictional claims in published maps and institutional affiliations.
Electronic supplementary material
Below is the link to the electronic supplementary material.
Supplementary Material 1: Table S1a
Hazard ratios of all-cause and CVD mortality by tertiles of NLR levels in diabetic subjects without baseline history of CVD. Table S1b Hazard ratios of all-cause and CVD mortality by tertiles of NLR levels in prediabetic subjects without baseline history of CVD. Table S2a Hazard ratios of all-cause and CVD mortality by tertiles of NLR levels in diabetic subjects with baseline history of CVD. Table S2b Hazard ratios of all-cause and CVD mortality by tertiles of NLR levels in diabetic subjects with baseline history of CVD. Table S3a Hazard ratios of all-cause and CVD mortality by tertiles of NLR levels in diabetic subjects without baseline history of CVD and cancer. Table S3b Hazard ratios of all-cause and CVD mortality by tertiles of NLR levels in prediabetic subjects without baseline history of CVD and cancer
Rights and permissions
Open Access This article is licensed under a Creative Commons Attribution 4.0 International License, which permits use, sharing, adaptation, distribution and reproduction in any medium or format, as long as you give appropriate credit to the original author(s) and the source, provide a link to the Creative Commons licence, and indicate if changes were made. The images or other third party material in this article are included in the article’s Creative Commons licence, unless indicated otherwise in a credit line to the material. If material is not included in the article’s Creative Commons licence and your intended use is not permitted by statutory regulation or exceeds the permitted use, you will need to obtain permission directly from the copyright holder. To view a copy of this licence, visit http://creativecommons.org/licenses/by/4.0/ . The Creative Commons Public Domain Dedication waiver ( http://creativecommons.org/publicdomain/zero/1.0/ ) applies to the data made available in this article, unless otherwise stated in a credit line to the data.
Reprints and permissions
About this article
Cite this article.
Chen, G., Che, L., Lai, M. et al. Association of neutrophil-lymphocyte ratio with all-cause and cardiovascular mortality in US adults with diabetes and prediabetes: a prospective cohort study. BMC Endocr Disord 24 , 64 (2024). https://doi.org/10.1186/s12902-024-01592-7
Download citation
Received : 26 February 2024
Accepted : 24 April 2024
Published : 10 May 2024
DOI : https://doi.org/10.1186/s12902-024-01592-7
Share this article
Anyone you share the following link with will be able to read this content:
Sorry, a shareable link is not currently available for this article.
Provided by the Springer Nature SharedIt content-sharing initiative
- Prediabetes
- All-cause mortality
- Cardiovascular mortality
- Cohort study
BMC Endocrine Disorders
ISSN: 1472-6823
- General enquiries: [email protected]
- Open access
- Published: 17 May 2024
Association between metabolic syndrome and kidney cancer risk: a prospective cohort study
- Lin Wang 1 ,
- Chao Sheng 1 ,
- Hongji Dai 1 &
- Kexin Chen 1
Lipids in Health and Disease volume 23 , Article number: 142 ( 2024 ) Cite this article
121 Accesses
Metrics details
Kidney cancer has become known as a metabolic disease. However, there is limited evidence linking metabolic syndrome (MetS) with kidney cancer risk. This study aimed to investigate the association between MetS and its components and the risk of kidney cancer.
UK Biobank data was used in this study. MetS was defined as having three or more metabolic abnormalities, while pre-MetS was defined as the presence of one or two metabolic abnormalities. Hazard ratios (HRs) and 95% confidence intervals (CIs) for kidney cancer risk by MetS category were calculated using multivariable Cox proportional hazards models. Subgroup analyses were conducted for age, sex, BMI, smoking status and drinking status. The joint effects of MetS and genetic factors on kidney cancer risk were also analyzed.
This study included 355,678 participants without cancer at recruitment. During a median follow-up of 11 years, 1203 participants developed kidney cancer. Compared to the metabolically healthy group, participants with pre-MetS (HR= 1.36, 95% CI: 1.06-1.74) or MetS (HR= 1. 70, 95% CI: 1.30-2.23) had a significantly greater risk of kidney cancer. This risk increased with the increasing number of MetS components ( P for trend < 0.001). The combination of hypertension, dyslipidemia and central obesity contributed to the highest risk of kidney cancer (HR= 3.03, 95% CI: 1.91-4.80). Compared with participants with non-MetS and low genetic risk, those with MetS and high genetic risk had the highest risk of kidney cancer (HR= 1. 74, 95% CI: 1.41-2.14).
Conclusions
Both pre-MetS and MetS status were positively associated with kidney cancer risk. The risk associated with kidney cancer varied by combinations of MetS components. These findings may offer novel perspectives on the aetiology of kidney cancer and assist in designing primary prevention strategies.
Kidney cancer, a malignancy involving the urinary system, is the 14th most common cancer worldwide according to GLOBOCAN 2020 [ 1 , 2 ]. Kidney cancer has posed a great burden on patients’ health and economic costs due to severe symptoms and difficulty in early detection and treatment [ 3 ]. Although tobacco, obesity, diabetes and hypertension are recognized as contributors in kidney cancer development [ 4 ], the cause of kidney cancer remains elusive. It is, therefore, important to identify additional indicators associated with kidney cancer for targeted preventive strategies in high-risk populations [ 5 ].
Metabolic syndrome (MetS) is a complex constellation of symptoms involving hypertension, central obesity, dyslipidemia, hypertriglyceridemia and hyperglycemia [ 6 ]. The increasing prevalence of MetS poses great challenges to public health [ 7 ]. Growing evidence suggests that MetS could be associated with overall cancer or site-specific cancers, such as liver cancer and colorectal cancer [ 8 ]. MetS is characterized by insulin resistance and chronic inflammatory state, possibly involved in cancer development and progression [ 9 ]. Emerging evidence links metabolic alterations and kidney cancer development from both animal experiments and population studies [ 10 ]. Under the condition of abnormal metabolism, tumor cells may gain more energy by increasing glycolysis and fatty acid oxidation, thereby promoting the development of kidney cancer [ 11 ]. To gather additional epidemiological evidence on the association linking MetS with kidney cancer, several population-based studies were carried out. However, most of the existing studies are retrospective, with limited sample sizes, and have shown inconsistent results [ 12 , 13 , 14 , 15 , 16 , 17 , 18 , 19 , 20 , 21 , 22 , 23 ]. Therefore, it is necessary to conduct further investigation into the relationship of MetS with kidney cancer risk.
Furthermore, environmental exposures and genetic factors may jointly affect the development of kidney cancer [ 24 ]. Until now, genome-wide association studies (GWAS) have uncovered genetic risk loci associated with kidney cancer [ 25 ]. The polygenic risk score (PRS), which is calculated by multiple genetic variants, serves as a comprehensive assessment of genetic susceptibility to diseases in individuals [ 26 ]. However, previous research has mainly assessed the single impact of PRS on kidney cancer [ 27 ]. The joint impact of MetS and PRS on kidney cancer remains unclear.
Based on a cohort of the European population, this study aimed to comprehensively examine the relationship of MetS and its components with kidney cancer risk and further explore the possible joint effect of MetS and PRS on kidney cancer. The findings of this study may offer novel perspectives on the etiology of kidney cancer and assist in designing primary prevention strategies.
Study design and participants
The study utilized data acquired from the UK Biobank, a prospective cohort, recruited over 500,000 individuals from 22 local assessment centers across the UK in 2006 - 2010 [ 28 ]. The cohort consisted of participants aged between 37 and 73 years. During the baseline assessment, individuals completed questionnaires, had physical measurements and had blood, urine and saliva samples gathered. This study excluded (a) participants with cancer at baseline (apart from non-melanoma skin cancer, ICD-10 (International Classification of Diseases, 10th revision) code (C44), (b) participants with missing MetS components, and (c) participants with missing covariates. The final analysis included 355,678 participants totally. The detailed flowchart of participant inclusion and exclusion was shown in Additional file: Figure S1 .
Assessment of MetS status
MetS was diagnosed based on the National Cholesterol Education Program Adult Treatment Panel III (NCEP-ATP III) criteria [ 7 ], with the presence of 3-5 of the subsequent metabolic abnormalities: (a) central obesity, defined as waist circumference [WC] ≥ 102 cm for males or WC ≥ 88 cm for females); (b) hypertension, a measured systolic blood pressure [SBP] ≥ 130 mmHg or diastolic blood pressure [DBP] ≥ 85 mmHg, and or taking antihypertensive drugs); (c) dyslipidemia, defined as high-density lipoprotein cholesterol [HDL-C] less than 1 mmol/L for males or less than 1.3 mmol/L for females, and or taking elevating HDL-C drugs; (d) hyperglycemia, defined as glycated haemoglobin [HbA1c] ≥ 42 mmol/mol or taking drugs for diabetes); (e) hypertriglyceridemia, defined as triglyceride [TG] ≥ 1.7 mmol/L or taking lowering TG drugs). Participants with one or two metabolic abnormalities were categorized into the pre metabolic syndrome (pre-MetS) group, while participants with none of the above-mentioned abnormalities were categorized into the metabolically healthy group.
WC was measured using a 200-cm tape measure (SECA). A HEM-907XL (Omron) was used to measure blood pressure twice after an interval of 5 minutes or more, and the mean of the two measured values was calculated [ 29 ]. The study chose a more stable marker, HbA1c, as a substitute to define hyperglycemia due to the low proportion (< 6%) of participants who had fasting glucose measurements. Medications for hypertension, low HDL-C, hypertriglyceridemia and hyperglycemia were defined as previously described [ 30 ].
Ascertainment of outcomes
The study used an ICD-10 code of C64 to define kidney cancer. Cancer status was ascertained through hospital in-patient admission records, death or cancer registries data. This study followed the individuals from baseline enrollment until the earliest occurrence of the following events: first registration with kidney cancer, death, lost-of-follow-up, or the endpoint date (2021/01/31 in Scotland and 2020/02/29 in England and Wales).
This study adjusted for known kidney cancer risk factors and potential confounders, including sociodemographic information, lifestyle factors, dietary intake, and physical measurements. Covariate information was obtained through touch screen questionnaire and physical measurements. This study considered sociodemographic characteristics such as age, sex (male/female), race (white/others), education level (college or university degree/others) and the townsend deprivation index (TDI). Lifestyle factors consisted of smoking status (former or current/never) and drinking status (former or current/never). Diet intake was assessed using the food frequency questionnaire including vegetable intake (< 3 tablespoons a day/≥ 3 tablespoons a day), fruit intake (< 3 pieces a day/≥ 3 pieces a day), fish intake (< 2 times a week/≥ 2 times a week) and red meat intake (< 2 times a week/≥ 2 times a week). Physical measurement index included body mass index (BMI), which was calculated by weight (kg)/height (m) 2 .
- Polygenic risk score
The single nucleotide polymorphisms (SNPs) related to kidney cancer risk and their respective weights were obtained from previous GWAS studies [ 25 , 31 ]. Standard PRS weights were used, and they corresponded to the log odds ratio (β) for each risk allele, with detailed information provided in Additional file: Table S1 . A set of quality control procedures has been conducted on genomic data from the UK Biobank before the release of the processed data [ 32 ]. As GWAS studies on kidney cancer risk were conducted in European ancestry populations, self-reported white ancestry may not accurately represent European ancestry. Therefore, this study constructed the PRS based on the genotypes of individuals with Caucasian ancestry. Specifically, individuals were further excluded when they had missing genotypes, non-Caucasian ancestry, gender inconsistency, kinship relationships, or poor quality samples. A total of 107,797 participants were excluded, the subsequent analysis involved 247,881 remaining participants. In the PRS calculation, this study summed the weight of individual SNPs after each was multiplied by the allelic dosage, and then divided accumulated value by the number of SNPs.
where M j is the number of SNPs observed for individual j , S i is the weight of SNP i and G ij is the allelic dosage of each SNP i in the genotype of individual j .
The PRS was classified into low (0-50th percentiles) and high (50-100th percentiles) genetic risk.
Statistical analysis
Continuous variables were presented as mean (standard deviation), while categorical variables were shown as number (percentage). The study performed one-way analysis of variance (ANOVA) or Chi-squared test to compare baseline information among the metabolically healthy, pre-MetS and MetS groups.
The study constructed Cox regression models to evaluate the relationship between MetS status, MetS components and kidney cancer risk. Model 1 included age and sex, while Model 2 further included race, education level, TDI and dietary intake; Model 3, the full model, additionally incorporated BMI based on Model 2. The associations of exposures were presented by hazard ratios (HRs) and 95% confidence intervals (95% CIs). The study further investigated the associations between the combinations of main MetS component and kidney cancer risk. The Schoenfeld test showed no violation of the proportional hazards assumption. Prespecified subgroup analyses were conducted according to sex (male/female), age (≥ 60 years old/< 60 years old), BMI (≥ 30 kg/m 2 /25 – 29.9 kg/m 2 /< 25 kg/m 2 ), smoking status (current/former/never), and drinking status (current/ former/never). The study used the Wald test to assess the significance of an interaction term. To evaluate the nonlinear relationship of each MetS component with kidney cancer risk, restricted cubic splines (RCS) with four knots (5%, 35%, 65%, and 95%) was applied. Additionally, in the Caucasian population, this study used multivariable Cox regression to evaluate the relationship of MetS, PRS with kidney cancer risk.
Sensitivity analyses were conducted to confirm the results stability. First, to eliminate the effect of reverse causality, individuals who was follow-up less than 2 years were excluded; Second, the study excluded individuals who had outliers for MetS components. Values below 1% or above 99% quantile were regarded as outliers. Third, the study excluded individuals with diabetes at recruitment. Fourth, individuals with kidney cancer ascertained through the death registries data were excluded.
A 2-sided P value < 0.05 was determined as statistically significant and all statistical analyses were used with R software (4.2.1).
Baseline characteristics
In total, 355,678 participants were involved. The mean (SD) age was 56.3 (8.1) years and the proportion of males was 46.8%. Participants were stratified into three categories based on the MetS status: the metabolically healthy group (16.1%), the pre-MetS group (56.3%) and the MetS group (27.6%) (Table 1 ). Compared with the metabolically healthy group, participants with pre-MetS or MetS tended to be older, male and former or never drinkers, with a lower level of education and a history of former or current smoking. Individuals with MetS tended to have a greater proportion of non-white ancestry, a BMI≥ 30 kg/m 2 and a greater TDI than those from the pre-MetS and metabolically healthy groups. As expected, the pre-MetS and MetS groups had elevated WC, DBP, SBP, TG and HbA1c levels, along with reduced HDL-C levels. Additionally, the proportion of taking statins, antidiabetic medications and antihypertensive medications was 35.8%, 7.1% and 40.8% respectively in the MetS group. For each MetS component, hypertension showed the highest prevalence rate (69%), with hyperglycemia having the lowest prevalence rate (8%) (Additional file: Figure S2). Furthermore, this study compared the baseline information between participants with all MetS components and those with missing components, and found there were no difference between the two groups (Additional file: Table S2).
MetS and kidney cancer
Compared with non-MetS (metabolically healthy + pre-MetS) group, MetS status showed a positive association with kidney cancer risk (HR= 1.28, 95% CI: 1.11-1.46) (Table 2 ). Additionally, there was a higher HR for MetS and pre-MetS (pre-MetS: HR= 1.36, 95% CI: 1.06-1.74; MetS: HR= 1.70, 95% CI: 1.30-2.23) compared to metabolically healthy group to develop kidney cancer. Compared to individuals with no MetS components, those with one to five components had HRs of 1.26 (0.97-1.64), 1.52 (1.17-1.98), 1.64 (1.24-2.16), 2.02 (1.49-2.75), and 2.65 (1.82-3.86), successively ( P for trend < 0.001). The results remained consistent across all sensitivity analyses (Additional file: Table S3-S6). Subgroup analyses results demonstrated that the associations of MetS with kidney cancer risk according to sex, age, BMI and smoking status were consistent. MetS exhibited a greater association with kidney cancer risk among current drinkers than former/never drinkers. However, no evidence of interaction was observed within these five groups (all P for interaction > 0.05, Fig. 1 ).
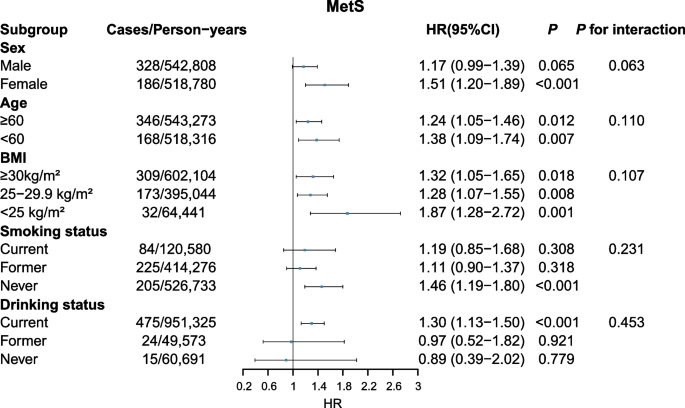
Association of MetS with risk of kidney cancer stratified by different subgroups
MetS components and kidney cancer
All five MetS components (binary classification) were associated with kidney cancer risk across Model 1 and Model 2 (all P < 0.05). However, after additionally adjusting for BMI, only hypertension (HR= 1. 29, 95% CI: 1.10-1.51), central obesity (HR= 1. 22, 95% CI: 1.04-1.42) and dyslipidemia (HR= 1. 63, 95% CI: 1.43-1.86) remained significantly associated with kidney cancer (Table 2 ). This study further explored the relationships of main MetS component combinations with kidney cancer risk (Table 3 ). In Model 3, the highest HRs for kidney cancer risk based on component combinations were BP + HDL: 2.34 (1.44-3.81) for pre-MetS and BP + HDL + WC: 3.03 (1.91-4.80) for MetS, respectively. The results of sensitivity analyses were consistent (Additional file: Table S7 and S8). In the subgroup analyses (Additional file: Figure S3-S7), hypertriglyceridemia was predominantly associated with a greater risk of kidney cancer in women (HR= 1.37, 95%CI: 1.12-1.68) than in men (HR= 0.90, 95%CI: 0.77-1.05, P for interaction = 0.002, Additional file: Figure S3). The associations of central obesity, hyperglycemia, dyslipidemia and hypertension with kidney cancer risk were not substantially different based on these subgroups (all P for interaction > 0.05, Additional file: Figure S4-7).
When examining the non-linear relationship of MetS components (continuous) with kidney cancer risk, only HDL-C and WC demonstrated significant associations with kidney cancer risk (HDL-C: P for overall < 0.001; WC: P for overall = 0.002). The study revealed an L-shaped relationship between HDL-C and kidney cancer risk ( P for nonlinearity = 0.002). Higher WC exhibited a positive association with kidney cancer risk, without evidence of nonlinearity ( P for nonlinearity = 0.780). Modelling the MetS component with the RCS suggested no association between DBP, SBP, TG or HbA1c and kidney cancer risk (all P for overall > 0.05, all P for nonlinearity > 0.05, Fig. 2 ).
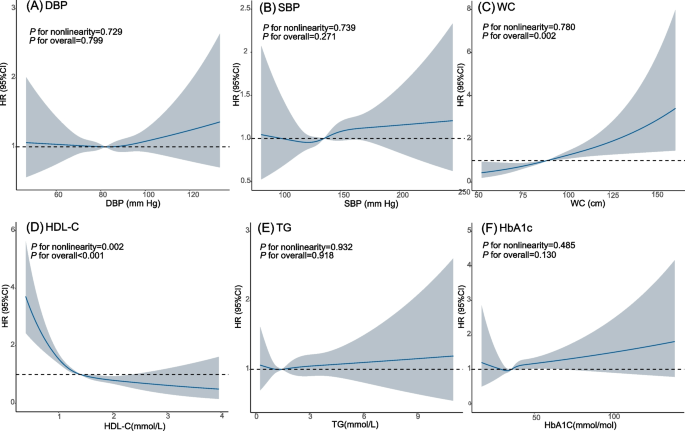
Restricted cubic spline analysis for the associations between MetS components and kidney cancer risk
MetS, PRS and kidney cancer
The study further explored the relationship of PRS with kidney cancer risk, along with evaluating the combined effect of MetS and PRS across multivariable Cox models. The results demonstrated that individuals at high genetic risk had a greater kidney cancer risk (HR = 1.36, 95% CI: 1.19-1.56) when in comparison to those at low genetic risk. In Model 3 , each 1-SD increase in PRS level corresponded to a 16% rise in kidney cancer risk (HR = 1.16, 95% CI: 1.09-1.24). Compared with participants with non-MetS and low PRS, those with MetS and high PRS had a significantly greater kidney cancer risk (HR= 1.74, 95% CI: 1.41-2.14) (Table 4 ).
The study found a positive relationship between both pre-MetS and MetS and kidney cancer risk, with the risk increased corresponding to the MetS components number. The risk associated with kidney cancer varied by MetS components combinations. Additionally, there was an obviously higher kidney cancer risk in individuals with high PRS and MetS, suggesting that PRS and MetS could exert a joint effect on kidney cancer risk.
Limited prospective studies have examined the relationship between MetS and kidney cancer risk, showing inconsistent conclusions. Kailuan [ 16 ] and Me-Can [ 17 ] cohort studies results demonstrated a positive association between MetS and kidney cancer risk, which consisted with results in this study. Another SMART cohort study conducted among individuals with cardiovascular diseases (CVD) observed no relationship between MetS and kidney cancer, possibly due to metabolic alterations caused by CVD [ 18 ]. Several retrospective cohort studies [ 12 , 14 , 19 , 20 ], case–control studies [ 13 , 21 , 22 ], cross-sectional study [ 23 ], and meta-analysis [ 15 ] found that MetS could increase kidney cancer risk. Nevertheless, most studies have retrospective designs, limited sample sizes, and low statistical efficiency. Several studies suggested positive associations between pre-MetS and heart disease [ 33 , 34 ], however, few research studied on the relationship of pre-MetS with kidney cancer risk. The study revealed an increase in kidney cancer risk among individuals suffering from pre-MetS, indicating the necessity of implementing intervention measures to prevent kidney cancer among this population.
Among the five MetS components, the study found that hypertension, central obesity and dyslipidemia were linked to kidney cancer at a higher risk. Hypertension is a common contributing factor to kidney cancer [ 35 ]. Unlike general obesity, central obesity exhibits excessive accumulation of abdominal fat. One cohort study exhibited a 1.32-fold increase in kidney cancer risk in participants suffering from central obesity, with the risk increasing by increasing WC [ 36 ], which consisted with findings in the study. For dyslipidemia, previous reports showed a positive association for low HDL-C with kidney cancer risk [ 37 ], similar to the findings of present study. Furthermore, this study found an L-shaped nonlinear relationship between HDL-C level and kidney cancer risk. Similar nonlinear relationships between HDL-C level and all-cause mortality were identified [ 38 ]. There were no statistically significant associations between hypertriglyceridemia or hyperglycemia and kidney cancer risk in this study, consistent with findings from a case-control study using the Kailuan database [ 13 ]. However, some studies have reported that high TG levels increased kidney cancer risk [ 12 , 39 ]. The heterogeneity in the results could be attributed to different studied populations and adjusted factors. A case-control study from Taiwan also reported no associations between hyperglycemia and kidney cancer [ 40 ]. In this study, various effects of MetS component combinations on kidney cancer risk were identified. Notably, the risks of participants in some pre-MetS groups (e.g., high BP + low HDL, HR=2.34) were higher than those in some MetS groups (e.g., BP + WC + TG, HR=1.35). This finding suggests that individuals with high BP and low HDL should be targeted for early prevention and management, even if they do not satisfy the criteria for diagnosing MetS. Kidney cancer risk was highest among individuals with a combination of high BP, low HDL and increased WC in the MetS group. The observed association is plausible because only these three factors showed significant associations with kidney cancer risk in former MetS component analyses. Moreover, hypertension, dyslipidemia or central obesity might interact through common pathophysiological pathways (such as insulin resistance) [ 41 ] in tumorigenesis. Further investigations are required to clarify the potential mechanisms linking MetS component combinations to kidney cancer development. The genetic variants identified through GWAS can be utilized for constructing PRS to identify high-risk populations for preventing diseases [ 42 ]. In this study, kidney cancer risk substantially increased in participants with MetS and high PRS. Considering the relative immutability of genetic risk, intervention measures aimed at populations with MetS and high PRS could be efficient to lower kidney cancer incidence.
Although the pathogenesis of MetS and kidney cancer remains unclear, there are some potential mechanisms for MetS to increase kidney cancer risk. Insulin resistance promotes reactive oxygen species production, leading to DNA damage and facilitating malignant transformation [ 43 ]. Hyperinsulinemia elevates type 1 insulin-like growth factor (IGF-1), activating downstream signaling pathways such as PI3K/Akt/mTOR, which promotes cell proliferation, inhibits apoptosis, and induces carcinogenesis [ 44 ]. Obesity changes the levels adipocyte-secreted hormone, among which adiponectin inhibits cell proliferation, leptin stimulates cell proliferation and facilitates invasion and migration [ 45 ]. Additionally, obesity can lead to an increase of pro-inflammatory factors, which may inhibit the immune system function and promote tumor growth [ 46 ]. Furthermore, hypertension may affect the development of kidney cancer through chronic renal hypoxia, lipid peroxidation and angiotensin system disorders [ 47 , 48 , 49 ]. In the presence of multiple coexisting components, various mechanisms may act synergistically to influence kidney cancer risk. Hypertension and dyslipidemia, mechanistically linked, could exacerbate atherosclerosis and impact vascular endothelial growth factor, thereby influencing tumor growth [ 41 ]. Hyperglycemia and hypertension share common mechanisms, such as oxidative stress, which may interact with other pathways to accelerate the development of kidney cancer [ 50 ]. However, biological mechanisms underlying the effects of MetS component combinations on kidney cancer are not clear, and further research is needed.
Study strengths and limitations
A significant strength of this study is its comprehensive and detailed measurements of metabolic factors within a large prospective cohort design. The study followed up with > 1000 cases of kidney cancer, which provided high statistical power and allowed for detailed examinations of subgroups. Additionally, the risk associated with kidney cancer varied by combinations of MetS component in this study. High-risk populations were characterized by the coexistence of multiple components, such as the combination of ‘BP+HDL+ WC’. Furthermore, this study found the combined effect of PRS and MetS on kidney cancer initially.
Several limitations existed in this study. First, MetS components were measured only once at baseline. Therefore, the dynamic trends of metabolic risks cannot be evaluated; Second, the impact of confounding factors cannot be completely eliminated, though the models were adjusted for a variety of factors; Third, kidney cancer cases ( N =19) identified by death registries data may overestimate follow-up period, potentially influencing the findings. Finally, the extrapolation of the results is limited since study population were mostly of European ancestry.
In this study, both pre-MetS and MetS were associated with higher risk of kidney cancer. Combinations of MetS components had various effects on kidney cancer. BP, HDL and WC were among the strongest metabolic risk factors for kidney cancer. Consequently, inclusion of the population’s metabolic status becomes imperative in designing primary prevention strategies for kidney cancer. Additionally, the combination of MetS and PRS may better predict the kidney cancer risk in population, facilitating early prevention efforts.
Availability of data and materials
UK Biobank data is available at https://www.ukbiobank.ac.uk/ . This research was conducted under approved project #76092.
Abbreviations
- Metabolic syndrome
Pre metabolic syndrome
Body mass index
Systolic blood pressure
Diastolic blood pressure
Waist circumference
Glycated haemoglobin
High-density lipoprotein cholesterol
Triglyceride
Hazard ratio
Confidence interval
International Classification of Diseases
Standard deviation
Siegel RL, Miller KD, Fuchs HE, Jemal A. Cancer statistics, 2021. CA Cancer J Clin. 2021;71:7–33.
Article PubMed Google Scholar
Sung H, Ferlay J, Siegel RL, Laversanne M, Soerjomataram I, Jemal A, et al. Global cancer statistics 2020: GLOBOCAN estimates of incidence and mortality worldwide for 36 cancers in 185 countries. CA Cancer J Clin. 2021;71:209–49.
Safiri S, Kolahi A-A, Mansournia MA, Almasi-Hashiani A, Ashrafi-Asgarabad A, Sullman MJM, et al. The burden of kidney cancer and its attributable risk factors in 195 countries and territories, 1990–2017. Sci Rep. 2020;10:13862.
Article CAS PubMed PubMed Central Google Scholar
Scelo G, Larose TL. Epidemiology and risk factors for kidney cancer. J Clin Oncol. 2018;36:3574–81.
Article CAS PubMed Central Google Scholar
Rossi SH, Klatte T, Usher-Smith J, Stewart GD. Epidemiology and screening for renal cancer. World J Urol. 2018;36:1341–53.
Article PubMed PubMed Central Google Scholar
Eckel RH, Grundy SM, Zimmet PZ. The metabolic syndrome. Lancet. 2005;365:1415–28.
Article CAS PubMed Google Scholar
Alberti KGMM, Eckel RH, Grundy SM, Zimmet PZ, Cleeman JI, Donato KA, et al. Harmonizing the metabolic syndrome: a joint interim statement of the international diabetes federation task force on epidemiology and prevention; national heart, lung, and blood institute; american heart association; world heart federation; international atherosclerosis society; and international association for the study of obesity. Circulation. 2009;120:1640–5.
Esposito K, Chiodini P, Colao A, Lenzi A, Giugliano D. Metabolic syndrome and risk of cancer: a systematic review and meta-analysis. Diabetes Care. 2012;35:2402–11.
Fahed G, Aoun L, Bou Zerdan M, Allam S, Bou Zerdan M, Bouferraa Y, et al. Metabolic syndrome: updates on pathophysiology and management in 2021. Int J Mol Sci. 2022;23:786.
Massari F, Ciccarese C, Santoni M, Brunelli M, Piva F, Modena A, et al. Metabolic alterations in renal cell carcinoma. Cancer Treat Rev. 2015;41:767–76.
Chakraborty S, Balan M, Sabarwal A, Choueiri TK, Pal S. Metabolic reprogramming in renal cancer: events of a metabolic disease biochimica et biophysica acta (BBA). Rev Cancer. 2021;1876:188559.
CAS Google Scholar
Lee HY, Han K-D, Woo IS, Kwon H-S. Association of metabolic syndrome components and nutritional status with kidney cancer in young adult population: a nationwide population-based cohort study in korea. Biomedicines. 2023;11:1425.
Jiang R, Li Z, Wang X, Cai H, Wu S, Chen S, et al. Association of metabolic syndrome and its components with the risk of kidney cancer: A cohort-based case-control study. THC. 2023;31:1235–44.
Article Google Scholar
Oh TR, Han K-D, Choi HS, Kim CS, Bae EH, Ma SK, et al. Metabolic syndrome resolved within two years is still a risk factor for kidney cancer. J Clin Med. 2019;8:1329.
Du W, Guo K, Jin H, Sun L, Ruan S, Song Q. Association between metabolic syndrome and risk of renal cell cancer: a meta-analysis. Front Oncol. 2022;12:928619.
Jiang R, Wang X, Li Z, Cai H, Sun Z, Wu S, et al. Association of metabolic syndrome and its components with the risk of urologic cancers: a prospective cohort study. BMC Urol. 2023;23:150.
Häggström C, Rapp K, Stocks T, Manjer J, Bjørge T, Ulmer H, et al. Metabolic factors associated with risk of renal cell carcinoma. PLoS One. 2013;8:e57475.
van Kruijsdijk RCM, van der Graaf Y, Peeters PHM, Visseren FLJ. Second manifestations of ARTerial disease (SMART) study group Cancer risk in patients with manifest vascular disease: effects of smoking, obesity, and metabolic syndrome. Cancer Epidemiol Biomarkers Prev. 2013;22:1267–77.
Choe JW, Hyun JJ, Kim B, Han K-D. Influence of metabolic syndrome on cancer risk in hbv carriers: a nationwide population based study using the national health insurance service database. J Clin Med. 2021;10:2401.
Ko S, Yoon S-J, Kim D, Kim A-R, Kim E-J, Seo H-Y. Metabolic risk profile and cancer in korean men and women. J Prev Med Public Health. 2016;49:143–52.
López-Jiménez T, Duarte-Salles T, Plana-Ripoll O, Recalde M, Xavier-Cos F, Puente D. Association between metabolic syndrome and 13 types of cancer in Catalonia: A matched case-control study. PLoS One. 2022;17:e0264634.
Bulut S, Aktas BK, Erkmen AE, Ozden C, Gokkaya CS, Baykam MM, et al. Metabolic syndrome prevalence in renal cell cancer patients. Asian Pac J Cancer Prev. 2014;15:7925–8.
Suarez Arbelaez MC, Nackeeran S, Shah K, Blachman-Braun R, Bronson I, Towe M, et al. Association between body mass index, metabolic syndrome and common urologic conditions: a cross-sectional study using a large multi-institutional database from the United States. Ann Med. 2023;55:2197293.
Hsieh JJ, Purdue MP, Signoretti S, Swanton C, Albiges L, Schmidinger M, et al. Renal cell carcinoma. Nat Rev Dis Primers. 2017;3:17009.
Scelo G, Purdue MP, Brown KM, Johansson M, Wang Z, Eckel-Passow JE, et al. Genome-wide association study identifies multiple risk loci for renal cell carcinoma. Nat Commun. 2017;8:15724.
Lewis CM, Vassos E. Polygenic risk scores: from research tools to clinical instruments. Genome Med. 2020;12:44.
Harrison H, Li N, Saunders CL, Rossi SH, Dennis J, Griffin SJ, et al. The current state of genetic risk models for the development of kidney cancer: a review and validation. BJU Int. 2022;130:550–61.
Matthews PM, Sudlow C. The UK Biobank. Brain. 2015;138:3463–5.
Dregan A, Rayner L, Davis KAS, Bakolis I, de la Torre JA, Das-Munshi J, et al. Associations between depression, arterial stiffness, and metabolic syndrome among adults in the UK biobank population study: a mediation analysis. JAMA Psychiatry. 2020;77:598–606.
Liang YY, Chen J, Peng M, Zhou J, Chen X, Tan X, et al. Association between sleep duration and metabolic syndrome: linear and nonlinear Mendelian randomization analyses. J Transl Med. 2023;21:90.
Henrion MYR, Purdue MP, Scelo G, Broderick P, Frampton M, Ritchie A, et al. Common variation at 1q24.1 (ALDH9A1) is a potential risk factor for renal cancer. PLoS One. 2015;10:e0122589.
Bycroft C, Freeman C, Petkova D, Band G, Elliott LT, Sharp K, et al. The UK biobank resource with deep phenotyping and genomic data. Nature. 2018;562:203–9.
Kim T-E, Kim H, Sung J, Kim D-K, Lee M-S, Han SW, et al. The association between metabolic syndrome and heart failure in middle-aged male and female: Korean population-based study of 2 million individuals. Epidemiol Health. 2022;44:e2022078.
Kwon CH, Kim H, Kim SH, Kim BS, Kim H-J, Sung JD, et al. The impact of metabolic syndrome on the incidence of atrial fibrillation: a nationwide longitudinal cohort study in South Korea. J Clin Med. 2019;8:1095.
Campi R, Rebez G, Klatte T, Roussel E, Ouizad I, Ingels A, et al. Effect of smoking, hypertension and lifestyle factors on kidney cancer — perspectives for prevention and screening programmes. Nat Rev Urol. 2023;20:669–81.
Nam GE, Cho KH, Han K, Kim CM, Han B, Cho SJ, et al. Obesity, abdominal obesity and subsequent risk of kidney cancer: a cohort study of 23.3 million East Asians. Br J Cancer. 2019;121:271–7.
Zhang C, Yu L, Xu T, Hao Y, Zhang X, Liu Z, et al. Association of dyslipidemia with renal cell carcinoma: a 1 ∶ 2 matched case-control study. PLoS One. 2013;8:e59796.
Mørland JG, Magnus P, Vollset SE, Leon DA, Selmer R, Tverdal A. Associations between serum high-density lipoprotein cholesterol levels and cause-specific mortality in a general population of 345 000 men and women aged 20–79 years. Int J Epidemiol. 2023;52(4):1257–67. https://doi.org/10.1093/ije/dyad011 .
Van Hemelrijck M, Garmo H, Hammar N, Jungner I, Walldius G, Lambe M, et al. The interplay between lipid profiles, glucose, BMI and risk of kidney cancer in the Swedish AMORIS study. Int J Cancer. 2012;130:2118–28.
Lai SW, Liao KF, Lai HC, Tsai PY, Sung FC, Chen PC. Kidney cancer and diabetes mellitus: a population-based case-control study in Taiwan. Ann Acad Med Singap. 2013;42:120–4.
Chapman MJ, Sposito AC. Hypertension and dyslipidaemia in obesity and insulin resistance: pathophysiology, impact on atherosclerotic disease and pharmacotherapy. pharmacology & Therapeutics. 2008;117:354–73.
Article CAS Google Scholar
Archambault AN, Su Y-R, Jeon J, Thomas M, Lin Y, Conti DV, et al. Cumulative burden of colorectal cancer-associated genetic variants is more strongly associated with early-onset vs late-onset cancer. Gastroenterology. 2020;158:1274–1286.e12.
Godsland IF. Insulin resistance and hyperinsulinaemia in the development and progression of cancer. Clin Sci (Lond). 2009;118:315–32.
Chiefari E, Mirabelli M, La Vignera S, Tanyolaç S, Foti DP, Aversa A, et al. Insulin resistance and cancer in search for a causal link. Int J Mol Sci. 2021;22:11137.
Gati A, Kouidhi S, Marrakchi R, El Gaaied A, Kourda N, Derouiche A, et al. Obesity and renal cancer: role of adipokines in the tumor-immune system conflict. Oncoimmunology. 2014;3:e27810.
Mendonça FM, De Sousa FR, Barbosa AL, Martins SC, Araújo RL, Soares R, et al. Metabolic syndrome and risk of cancer: which link? Metabolism. 2015;64:182–9.
Sharifi N, Farrar WL. Perturbations in hypoxia detection: a shared link between hereditary and sporadic tumor formation? Med Hypotheses. 2006;66:732–5.
Gago-Dominguez M, Castelao JE, Yuan J-M, Ross RK, Yu MC. Lipid peroxidation: a novel and unifying concept of the etiology of renal cell carcinoma (United States). Cancer Causes Control. 2002;13:287–93.
Sobczuk P, Szczylik C, Porta C, Czarnecka AM. Renin angiotensin system deregulation as renal cancer risk factor. Oncol Lett. 2017;14:5059–68.
PubMed PubMed Central Google Scholar
Cheung BMY, Li C. Diabetes and hypertension: is there a common metabolic pathway? Curr Atheroscler Rep. 2012;14:160–6.
Download references
Acknowledgements
We gratefully acknowledge the participants in the UK Biobank. The authors also thank the staff of the UK Biobank study for their important contributions.
This project was supported by National Key Research and Development Program of China (2021YFC2500400), Tianjin Municipal Science and Technology Bureau (22JCYBJC01040), the 14th five-year Special Project of Cancer Prevention and Treatment for the Youth Talents of Tianjin Cancer Institute and Hospital (YQ-08) and Tianjin Key Medical Discipline (Specialty) Construction Project (TJYXZDXK-009A).
Author information
Authors and affiliations.
Department of Epidemiology and Biostatistics, Key Laboratory of Molecular Cancer Epidemiology, Key Laboratory of Prevention and Control of Human Major Diseases, Ministry of Education, National Clinical Research Center for Cancer, Tianjin Medical University Cancer Institute and Hospital, Tianjin Medical University, Tianjin, 300060, China
Lin Wang, Han Du, Chao Sheng, Hongji Dai & Kexin Chen
You can also search for this author in PubMed Google Scholar
Contributions
L.W.: Conceptualization, methodology, acquisition, data curation, formal analysis, software, visualization, writing-original draft. H. Du: Formal analysis, validation, methodology. C.S.: Data curation, methodology. H. Dai.: Conceptualization, funding, design, methodology, writing-review & editing. K.C.: Conceptualization, funding, project administration. All authors read and approved the final manuscript.
Corresponding authors
Correspondence to Hongji Dai or Kexin Chen .
Ethics declarations
Ethics approval and consent to participate.
Ethics approval for the UK Biobank was obtained from the North West Multi‑centre Research Ethics Committee (Ref: 11/NW/0382). All participants provided written informed consent.
Consent for publication
Not applicable.
Competing interests
The authors declare no competing interests.
Additional information
Publisher’s note.
Springer Nature remains neutral with regard to jurisdictional claims in published maps and institutional affiliations.
Supplementary Information
Supplementary material 1., rights and permissions.
Open Access This article is licensed under a Creative Commons Attribution 4.0 International License, which permits use, sharing, adaptation, distribution and reproduction in any medium or format, as long as you give appropriate credit to the original author(s) and the source, provide a link to the Creative Commons licence, and indicate if changes were made. The images or other third party material in this article are included in the article's Creative Commons licence, unless indicated otherwise in a credit line to the material. If material is not included in the article's Creative Commons licence and your intended use is not permitted by statutory regulation or exceeds the permitted use, you will need to obtain permission directly from the copyright holder. To view a copy of this licence, visit http://creativecommons.org/licenses/by/4.0/ . The Creative Commons Public Domain Dedication waiver ( http://creativecommons.org/publicdomain/zero/1.0/ ) applies to the data made available in this article, unless otherwise stated in a credit line to the data.
Reprints and permissions
About this article
Cite this article.
Wang, L., Du, H., Sheng, C. et al. Association between metabolic syndrome and kidney cancer risk: a prospective cohort study. Lipids Health Dis 23 , 142 (2024). https://doi.org/10.1186/s12944-024-02138-5
Download citation
Received : 15 February 2024
Accepted : 08 May 2024
Published : 17 May 2024
DOI : https://doi.org/10.1186/s12944-024-02138-5
Share this article
Anyone you share the following link with will be able to read this content:
Sorry, a shareable link is not currently available for this article.
Provided by the Springer Nature SharedIt content-sharing initiative
- Renal cancer
- Hypertension
- Central obesity
- Dyslipidemia
Lipids in Health and Disease
ISSN: 1476-511X
- General enquiries: [email protected]

IMAGES
VIDEO
COMMENTS
A covariate-stratified case-cohort design could be used to maximize precision for groups of particular interest ... Because case-cohort studies include a sample of the cohort, this design potentially opens up questions and methods that specifically leverage time-varying covariates, ...
Introduction. The case-cohort study design was originally proposed by Prentice .Nested within a larger cohort, the study comprises a random "subcohort" of individuals from the original cohort (sampled irrespective of disease status), together with all cases [Figure 1].The main advantage of the case-cohort study design over a cohort study is that full covariate data are only needed on the ...
This scoping review collects and presents an analysis of the methods and tools currently used to design, build and analyze stratification and validation cohorts. It explores the current use of these tools and methods, the criteria to consider when applying them and the existing regulation that affects them.
For paired cohort studies or case-cohort designs, the formulae can be found in Kasiulevicius et al17 and Cai and Zeng.9 When the outcome of interest in a cohort study is continuous (although it is less common), we would like to compare the means of two cohorts. The formula based on the minimum detectable difference can be found in Woodward.18
When carefully planned and analysed, the case-cohort design is a powerful choice for follow-up studies with multiple event types of interest. While the literature is rich with analysis methods for case-cohort data, little is written about the designing of a case-cohort study. Our experiences in designing, coordinating and analysing the MORGAM case-cohort study are potentially useful for other ...
A study combining two study designs, the case-cohort design, is a combination of a case-control and cohort design that can be either prospective or retrospective. ... To control for smoking, the study population could be stratified according to smoking status. The association between exposure to asbestos and cancer can then be assessed ...
Stratified case-cohort studies involve data missing by design. Sometimes, as for biomarkers in the ARIC study, phase 2 data are also missing by chance . The methods proposed here assume that, within each stratum, the phase 2 subjects with complete data still constitute a random sample from the cohort. This assumption may be relaxed by adding ...
This article compares five ways of analyzing stratified case-cohort studies, using data from the EPIC-InterAct study and artificial data sets. A two-stage Cox model with random-effect meta-analysis gave the most reliable results in the widest variety of scenarios and is a flexible model that makes fewer assumptions than the other models ...
A case-cohort study has all the previously mentioned advantages that are present in a nested case-control study. In addition, a major advantage of the case-cohort design is the ability to study several disease outcomes using the same subcohort. ... Their case-crossover design with a time-stratified approach was adopted from Maclure. 10. Maclure ...
It has been demonstrated that the case-cohort study design, for a single disease outcome, is more efficient than a nested case-control study design; however, the difference is very small [1]. Compared to the nested case-control studies, a major advantage of the case-cohort design is the ability to study several disease outcomes using the same
Case-cohort study-design, 1st proposed by Prentice in 1986 is a commonly-used cost-effective outcome-dependent study-design embedded in large cohort studies [1,2,3]. This design is used to reduce ...
Generalized case-cohort design has been proposed to assess the effects of exposures on survival outcomes when measuring exposures is expensive and events are not rare in the cohort. In such design, expensive exposure information is collected from both a (stratified) randomly selected subcohort and a subset of individuals with events.
A variant of the case-cohort design is proposed for the situation in which a correlate of the exposure (or prognostic factor) of interest is available for all cohort members, and exposure information is to be collected for a case-cohort sample. The cohort is stratified according to the correlate, and the subcohort is selected by stratified ...
• Case-cohort design is another option - With appropriate sampling and analysis, the HR estimates the HR in the full cohort - In a case-cohort study you can also estimate e.g. rates, rate differences, risks - That is an advantage of the case-cohort design over the NCC, where you typically
Abstract. A variant of the case-cohort design is proposed for the situation in which a correlate of the exposure (or prognostic factor) of interest is available for all cohort members, and exposure information is to be collected for a case-cohort sample. The cohort is stratified according to the correlate, and the subcohort is selected by ...
In studies where the study populations are not homogenous or the original cohort is assembled through a stratified design, a stratified case-cohort (SCC) design may be more appropriate [21-22]. The SCC sample consists of the stratified sub-cohorts selected by a stratified random sampling from the full cohort, and all the cases.
A cohort study is a type of observational study that follows a group of participants over a period of time, examining how certain factors (like exposure to a given risk factor) affect their health outcomes. The individuals in the cohort have a characteristic or lived experience in common, such as birth year or geographic area.
The case-cohort study design combines the advantages of a cohort study with the efficiency of a nested case-control study. However, unlike more standard observational study designs, there are currently no guidelines for reporting results from case-cohort studies. Our aim was to review recent practice in reporting these studies, and develop recommendations for the future. By searching papers ...
A variant of the case-cohort design is proposed for the situation in which a correlate of the exposure (or prognostic factor) of interest is available for all cohort members, and exposure information is to be collected for a case-cohort sample. The cohort is stratified according to the correlate, and the subcohort is selected by stratified random sampling. A number of possible methods for the ...
The case-cohort (CC) study design usually has been used for risk factor assessment in epidemiologic studies or disease prevention trials for rare diseases. The sample size/power calculation for a stratified CC (SCC) design has not been addressed before. This article derives such result based on a stratified test statistic.
Matching is mainly used in observational studies, including case-control and cohort studies. Matching is a technique by which patients with and without an outcome of interest (in case-control studies) or patients with and without an exposure of interest (in cohort studies) are sampled from an underlying cohort to have the same or similar distributions of characteristics such as age and sex.
In this case, stratified sampling allows for more precise measures of the variables you wish to study, with lower variance within each subgroup and therefore for the population as a whole. Allowing for a variety of data collection methods; Sometimes you may need to use different methods to collect data from different subgroups.
This study reports the development and use of a validation process which was then applied to a case study early decision-analytic-model-based cost-effectiveness analysis (CEA) of a risk-stratified NBSP . Existing validation concepts were consolidated into a single step-by-step process resulting in the transparent presentation of the assumptions ...
A case-cohort study is a design in which cases and controls are drawn from within a prospective study. All cases who developed the outcome of interest during the follow-up are selected and compared with a random sample of the cohort. This randomly selected control sample could, by chance, include some cases. Exposure is defined prior to ...
A recent population-based cohort study found a positive association between ultra-processed foods (UPFs) consumption and all-cause mortality in adults. Specifically, meat/poultry/seafood based ready-to-eat products, sugar-sweetened beverages, and dairy-based desserts showed strong associations with higher mortality rates.
Background The neutrophil-lymphocyte ratio (NLR) is a novel hematological parameter to assess systemic inflammation. Prior investigations have indicated that an increased NLR may serve as a potential marker for pathological states such as cancer and atherosclerosis. However, there exists a dearth of research investigating the correlation between NLR levels and mortality in individuals with ...
Our study has some limitations. As a retrospective cohort study, it is at risk of including confounding variables. The time period examined here also included a global pandemic, which had an impact on rates of referral, diagnosis and in-patient admission.
Background Kidney cancer has become known as a metabolic disease. However, there is limited evidence linking metabolic syndrome (MetS) with kidney cancer risk. This study aimed to investigate the association between MetS and its components and the risk of kidney cancer. Methods UK Biobank data was used in this study. MetS was defined as having three or more metabolic abnormalities, while pre ...
Background: The effect of flavonoid consumption on all-cause and special-cause mortality remains unclear among populations with hypertension. Methods: A total of 6110 people with hypertension from three NHANES survey cycles (2007-2008, 2009-2010, and 2017-2018) were enrolled in this study. Cox proportional hazard models were conducted to estimate the association between the intake of ...