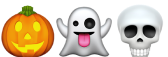
- Onsite training
3,000,000+ delegates
15,000+ clients
1,000+ locations
- KnowledgePass
- Log a ticket
01344203999 Available 24/7

Six Sigma Case Study: Everything You Need to Know
Explore the field of Six Sigma Case Studies in our comprehensive blog. From defining the methodology to real-world applications, our 'Six Sigma Case Study: Everything You Need to Know' blog sheds light on this powerful problem-solving tool. Uncover success stories and learn how Six Sigma can drive efficiency and quality improvements in various industries.

Exclusive 40% OFF
Training Outcomes Within Your Budget!
We ensure quality, budget-alignment, and timely delivery by our expert instructors.
Share this Resource
- Six Sigma Black Belt Upgrade
- Lean Six Sigma Green Belt
- Six Sigma Yellow Belt
- Six Sigma Black Belt
- Lean Six Sigma Black Belt
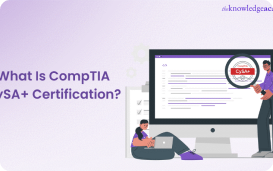
By analysing such case studies, one can gain insights into the successful application of Six Sigma in various industries and understand its impact on process improvement. Read this blog on Six Sigma Case Study to learn how real-world businesses have achieved remarkable process improvement and cost savings.
Table of Contents
1) Understanding Six Sigma Methodology
2) Six Sigma Case Study
a) Improving customer service
b) Improving delivery efficiency
3) Conclusion
Understanding Six Sigma Methodology
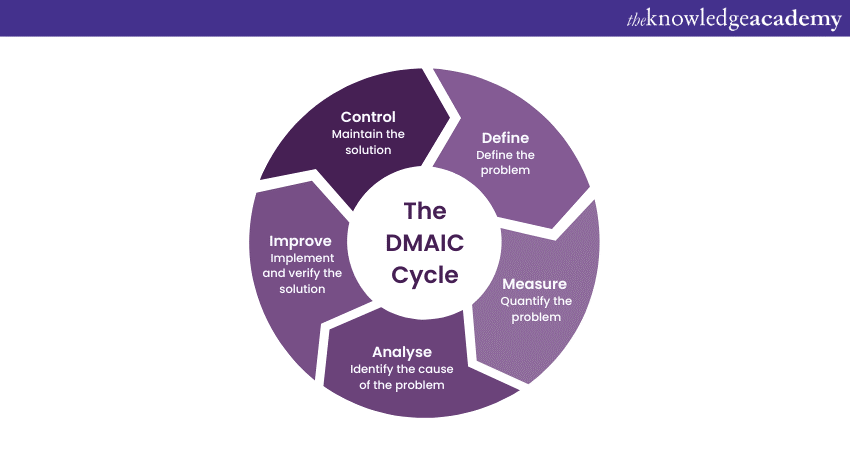
By applying statistical analysis and data-driven decision-making, Six Sigma helps organisations identify the root cause of problems and implement effective solutions. It emphasises the importance of process standardisation, continuous improvement, and customer satisfaction. With its focus on rigorous measurement and analysis, Six Sigma enables organisations to drive efficiency, reduce waste, and deliver exceptional products and services. The methodology follows a step-by-step process called Define, Measure, Analyse, Improve, and Control (DMAIC). These five phases are briefly explained below:
a) Define: The project goals and customer requirements are clearly defined in this phase.
b) Measure: In this phase, data is collected to understand the process's current state and identify improvement areas.
c) Analyse: This phase focuses on analysing data to determine the root cause of defects or variations.
d) Improve: This phase involves implementing solutions and making necessary changes to eliminate the identified issues.
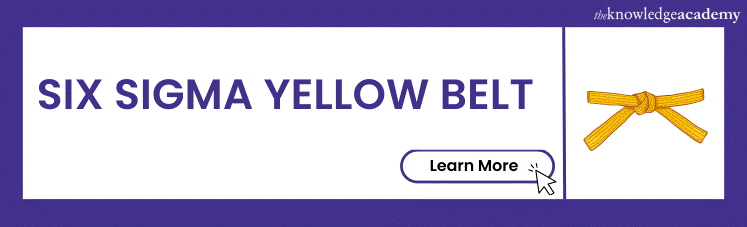
Six Sigma Case Study
In this section we discuss two Six Sigma Case Study that will help you understand and use it better.
Case Study 1: Improving customer service
This Six Sigma Case Study will focus on a telecommunications company facing significant customer service challenges. The issues included long wait times, frequent call transfers, unresolved issues, and many more. The company decided to apply Six Sigma methodologies to enhance customer satisfaction.
a) Define phase: Using the DMAIC approach, the team began by defining the problem: long wait times and inefficient call handling. They set a goal to reduce average wait time and increase first-call resolution rates.
b) Measure phase: In this phase, data was collected to analyse call volume, wait times, and reasons for call transfers. This helped identify bottlenecks and areas for improvement.
c) Analyse phase: During this phase, the team discovered that inadequate training and complex call routing were key contributors to the problems. They also found that certain product issues required better resolution protocols.
d) Improve phase: In this phase, targeted solutions were introduced and implemented to address these issues. The team revamped the training program, ensuring agents were well-trained and equipped to handle customer inquiries. They simplified call routing and introduced automated prompts for quicker issue resolution.
e) Control phase: Finally, monitoring systems were established in the control phase to track key metrics and ensure sustained improvements. Regular feedback loops were implemented to identify emerging challenges and make necessary adjustments.
The results were exceptional. Average wait times were reduced by 40%, and first-call resolution rates increased by 25%. Customer satisfaction scores improved significantly, leading to increased loyalty and positive word-of-mouth.
This Six Sigma Case Study highlights how Six Sigma methodologies can drive transformative improvements in customer service. By focusing on data analysis, process optimisation, and continuous monitoring, organisations can achieve outstanding outcomes and deliver exceptional customer experiences.
Understand the in-depth process of Six Sigma with our Six Sigma Yellow Belt Course . Join now!
Case Study 2: Improving delivery efficiency
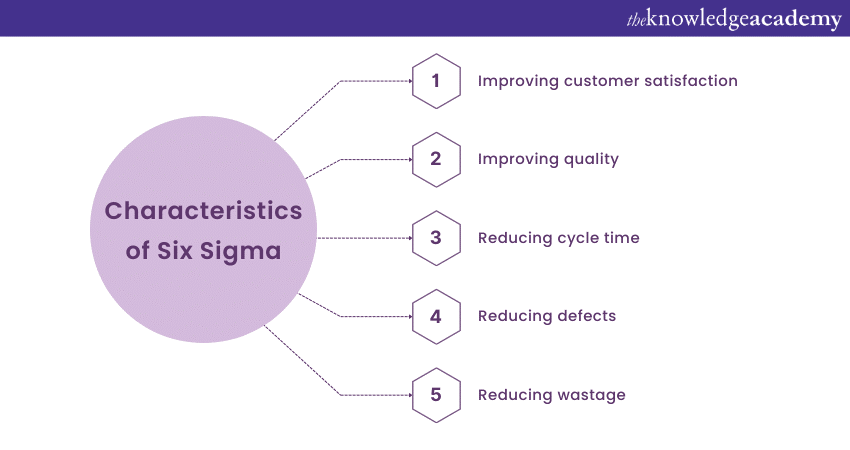
a) Define phase: The business used the Voice of the Customer (VoC) tool to understand customer needs and expectations. They identified prompt delivery, correct product selection, and a knowledgeable distribution team as crucial customer requirements.
b) Measure phase: The team collected data to evaluate the problem of slow delivery. They discovered that their Order Fulfillment Cycle Time (OFCT) was 46% longer than competitors, leading to customer dissatisfaction.
c) Analyse phase: The team brainstormed potential causes of slow delivery, including accuracy of sales plans, buffer stock issues, vendor delivery performance, and manufacturing schedule delays. They conducted a regression analysis, revealing that inadequate buffer stock for high-demand products was the main issue affecting delivery efficiency.
d) Improve phase: The distributor implemented a monthly demand review to ensure that in-demand products are readily available. They emphasised ordering and providing customers with the specific products they desired.
e) Control phase: The team developed plans to monitor sales of the top 20% of bestselling products, avoiding over or under-supply situations. They conducted annual reviews to identify any changes in demand and proactively adjust product offerings.
By applying Six Sigma Principles , the plumbing product distributor significantly improved its delivery efficiency, addressing the root cause of customer dissatisfaction. Prompt action, data-driven decision-making, and ongoing monitoring allowed them to meet customer expectations, enhance its reputation, and maintain a competitive edge in the industry. This case demonstrates the power of Lean Six Sigma in driving operational excellence and customer-centric improvements.
Take the next step in your professional development and boost your career by joining our Six Sigma Green Belt course .
Conclusion
We hope this blog gives you enough insights into the Six Sigma Case Study. This blog showcased the effectiveness of its methodology in driving transformative improvements. By applying DMAIC and using customer insights and data analysis, organisations have successfully resolved delivery inefficiencies, improving customer satisfaction and operational performance. The blog highlights how Six Sigma can be a powerful framework for organisations seeking excellence and exceptional value.
Learn the six-sigma methodology to achieve business objectives with our Six Sigma Certification Training today!
Frequently Asked Questions
Upcoming business improvement resources batches & dates.
Mon 10th Jun 2024
Mon 17th Jun 2024
Sat 22nd Jun 2024, Sun 23rd Jun 2024
Mon 24th Jun 2024
Mon 1st Jul 2024
Mon 8th Jul 2024
Mon 15th Jul 2024
Sat 20th Jul 2024, Sun 21st Jul 2024
Mon 22nd Jul 2024
Mon 29th Jul 2024
Mon 5th Aug 2024
Mon 12th Aug 2024
Sat 17th Aug 2024, Sun 18th Aug 2024
Mon 19th Aug 2024
Tue 27th Aug 2024
Mon 2nd Sep 2024
Mon 9th Sep 2024
Sat 14th Sep 2024, Sun 15th Sep 2024
Mon 16th Sep 2024
Mon 23rd Sep 2024
Mon 30th Sep 2024
Mon 7th Oct 2024
Sat 12th Oct 2024, Sun 13th Oct 2024
Mon 14th Oct 2024
Mon 21st Oct 2024
Mon 28th Oct 2024
Mon 4th Nov 2024
Sat 9th Nov 2024, Sun 10th Nov 2024
Mon 11th Nov 2024
Mon 18th Nov 2024
Mon 25th Nov 2024
Mon 2nd Dec 2024
Sat 7th Dec 2024, Sun 8th Dec 2024
Mon 9th Dec 2024
Mon 16th Dec 2024
Mon 6th Jan 2025
Mon 13th Jan 2025
Mon 20th Jan 2025
Mon 27th Jan 2025
Mon 3rd Feb 2025
Mon 10th Feb 2025
Mon 17th Feb 2025
Mon 24th Feb 2025
Mon 3rd Mar 2025
Mon 10th Mar 2025
Mon 17th Mar 2025
Mon 24th Mar 2025
Mon 31st Mar 2025
Mon 7th Apr 2025
Mon 14th Apr 2025
Tue 22nd Apr 2025
Mon 28th Apr 2025
Tue 6th May 2025
Mon 12th May 2025
Mon 19th May 2025
Tue 27th May 2025
Mon 2nd Jun 2025
Mon 9th Jun 2025
Mon 16th Jun 2025
Mon 23rd Jun 2025
Mon 30th Jun 2025
Mon 7th Jul 2025
Mon 14th Jul 2025
Mon 21st Jul 2025
Mon 28th Jul 2025
Mon 4th Aug 2025
Mon 11th Aug 2025
Mon 18th Aug 2025
Tue 26th Aug 2025
Mon 1st Sep 2025
Mon 8th Sep 2025
Mon 15th Sep 2025
Mon 22nd Sep 2025
Mon 29th Sep 2025
Mon 6th Oct 2025
Mon 13th Oct 2025
Mon 20th Oct 2025
Mon 27th Oct 2025
Mon 3rd Nov 2025
Mon 10th Nov 2025
Mon 17th Nov 2025
Mon 24th Nov 2025
Mon 1st Dec 2025
Mon 8th Dec 2025
Mon 15th Dec 2025
Get A Quote
WHO WILL BE FUNDING THE COURSE?
My employer
By submitting your details you agree to be contacted in order to respond to your enquiry
- Business Analysis
- Lean Six Sigma Certification
Share this course
Our biggest spring sale.

We cannot process your enquiry without contacting you, please tick to confirm your consent to us for contacting you about your enquiry.
By submitting your details you agree to be contacted in order to respond to your enquiry.
We may not have the course you’re looking for. If you enquire or give us a call on 01344203999 and speak to our training experts, we may still be able to help with your training requirements.
Or select from our popular topics
- ITIL® Certification
- Scrum Certification
- Change Management Certification
- Business Analysis Courses
- Microsoft Azure Certification
- Microsoft Excel Courses
- Microsoft Project
- Explore more courses
Press esc to close
Fill out your contact details below and our training experts will be in touch.
Fill out your contact details below
Thank you for your enquiry!
One of our training experts will be in touch shortly to go over your training requirements.
Back to Course Information
Fill out your contact details below so we can get in touch with you regarding your training requirements.
* WHO WILL BE FUNDING THE COURSE?
Preferred Contact Method
No preference
Back to course information
Fill out your training details below
Fill out your training details below so we have a better idea of what your training requirements are.
HOW MANY DELEGATES NEED TRAINING?
HOW DO YOU WANT THE COURSE DELIVERED?
Online Instructor-led
Online Self-paced
WHEN WOULD YOU LIKE TO TAKE THIS COURSE?
Next 2 - 4 months
WHAT IS YOUR REASON FOR ENQUIRING?
Looking for some information
Looking for a discount
I want to book but have questions
One of our training experts will be in touch shortly to go overy your training requirements.
Your privacy & cookies!
Like many websites we use cookies. We care about your data and experience, so to give you the best possible experience using our site, we store a very limited amount of your data. Continuing to use this site or clicking “Accept & close” means that you agree to our use of cookies. Learn more about our privacy policy and cookie policy cookie policy .
We use cookies that are essential for our site to work. Please visit our cookie policy for more information. To accept all cookies click 'Accept & close'.
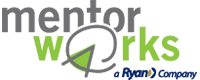
Lean Six Sigma within the Manufacturing Industry: Case Studies
Canadian enterprises are consistently integrating Lean Six Sigma initiatives with notable achievements. When executed strategically and with foresight, Lean Six Sigma activities typically result in reducing inefficiencies, eliminating waste, and enhancing profitability. This versatile methodology can be tailored to address various business obstacles, offering a systematic approach to organizational enhancement.
This blog outlines case studies exemplifying the use of Lean Six Sigma strategies to eliminate outdated business procedures that failed to meet contemporary market norms. These entities revamped their operations, resulting in immediate improvements to both revenue generation and profitability. If you are in the manufacturing industry, this blog can be a helpful resource for gaining inspiration to build out your own Lean Six Sigma process.
This is the third blog of a three part series: Part 1: Canadian Guide to Lean Manufacturing Part 2: How to Use the DMAIC Framework for Lean Manufacturing Part 3: Lean Six Sigma within the Manufacturing Industry: Case Studies
Case 1: Increasing Capacity and Production Output
An Ontario manufacturer embarked on its journey with automated technologies over 15 years ago by adopting a Flexible Manufacturing System (FMS) machining cell equipped with one machine and a 16-pallet system. The immediate surge in productivity from this single equipment propelled the company into an expansion phase, necessitating a move to a larger facility.
The new facility offered ample space for innovative technologies, leading to the installation of additional computer numerical control (CNC) machines to enhance unattended operations. This resulted in a threefold increase in automated production capacity prompting the company’s operating costs to decrease by 20%, while output surged by an impressive 375%. Further efficiencies were achieved with the acquisition of a machine-tending robot, which streamlined the loading and unloading of parts onto the CNC machine, boosting output by an additional 67% and facilitating round-the-clock production.
Subsequent equipment enhancements included the deployment of a robotic deburring machine, addressing the strenuous deburring process previously handled by human labor. Automating this process not only reduced post-processing costs by 30% but also contributed to increased productivity and employee morale.
To optimize information flow across departments, the manufacturer implemented an enterprise resource planning (ERP) system, enabling seamless unattended operations and facilitating “lights-out” production, resulting in the creation of hundreds of units without human intervention.
Case 2: Re-Imagining Organizational Structure
For over four decades, a Canadian welder and fabricator specializing in custom-designed industrial equipment witnessed remarkable growth and success. Originating as a small-scale job shop catering to the oil and gas sector, the company evolved from crafting individual pieces to producing hundreds of bespoke products annually.
Despite this trajectory, the journey was not without its challenges. Early expansions exposed inefficiencies in processes, leading to diminished operational effectiveness and customer satisfaction. Following a transition to a larger facility, inadequate floor space and suboptimal processes precipitated a decline in performance, exacerbated by the economic downturn of 2009, which served as a catalyst for significant organizational restructuring.
The Lean Six Sigma transformation commenced with a systematic evaluation of existing processes, identifying inefficiencies, and laying the groundwork for streamlining and standardization. Implementation of 5S principles instilled order and cleanliness in the work environment, fostering a culture of continuous improvement.
Beyond process enhancements, the management team undertook a comprehensive reorganization, realigning roles and responsibilities to support the newly optimized workflows. The shop floor underwent a complete redesign to facilitate function-based process flows, promoting collaboration among work teams and enhancing productivity while minimizing safety risks.
Notably, the ethos of operational excellence permeated the organization, with enthusiastic employee engagement driving the adoption of 5S methodologies and fostering a culture of innovation, where novel improvement ideas originated from the shop floor.
Case 3: Energy Efficiency & Sustainable Process
An Ontario-based cement producer found escalating energy costs were eroding their profits, particularly during the early to mid-2000s. With nearly half of their production expenses allocated to energy, they recognized the need to mitigate these costs to remain competitive regionally and globally, and embarked on a proactive strategy.
In 2009, the company engaged a local energy consultant to develop a comprehensive energy management program. Swift implementation of this initiative yielded tangible improvements in the production process. Notably, a significant transformation occurred with the shift of production to off-peak hours, capitalizing on lower energy costs during these periods. This strategic adjustment resulted in a notable reduction in energy expenses as a proportion of overall production costs, thereby enhancing competitiveness and preserving a larger share of revenues.
Moreover, conducting production cycles during nighttime facilitated more frequent cleaning and machine maintenance during daytime hours, contributing to additional cost savings and minimizing machine downtime.
The adoption of Lean Six Sigma principles in this endeavor underscores the efficacy of structured problem-solving methodologies in addressing complex operational challenges. The results speak volumes: approximately $10 million in energy-related production costs were saved, and approximately 32,000 tonnes of greenhouse gas emissions were eliminated over an eight-year period.
This case exemplifies the symbiotic relationship between Lean Six Sigma methodologies and government funding initiatives in driving sustainable business practices and fostering economic growth. Leveraging government grants and loans to support continuous improvement projects not only mitigates financial constraints but also aligns with national objectives of enhancing competitiveness, revenue generation, and environmental sustainability.
Funding Opportunities for Lean Six Sigma Projects
There are several manufacturing grants available to support your manufacturing processes. Learn more below about which types of funding are available to subsidize your next project.
Business Expansion Funding
Lean Six Sigma initiatives play a pivotal role in identifying opportunities for business expansion. Through meticulous performance analysis and demand forecasting, lean organizations streamline operations to effectively manage growth. Common strategies involve expanding or relocating production facilities, particularly for organizations that have bolstered profitability through operational improvements. These endeavors are supported by a range of funding programs across Canada, offering grants and loans to enhance internal processes, increase output, and facilitate market expansion, including financial assistance for trade show participation and export activities.
Visit our Business Expansion Funding directory to find the appropriate business expansion funding program for your project.
Funding for Technology Adoption
While Lean Six Sigma emphasizes continuous improvement, integrating innovative technologies can expedite growth. New technological advancements complement lean methodologies by enhancing efficiencies beyond traditional improvement strategies. Investments in robotics and advanced manufacturing equipment are instrumental in optimizing operations and minimizing waste. Technology grants and loans are available at both provincial and federal levels, tailored to key industries to foster technological innovation and competitiveness.
Explore our Capital & Tech Adoption Funding Directory to learn more about available grant programs to support your manufacturing business.
Funding for Hiring and Training
A skilled and motivated workforce is essential for implementing a lean culture within an organization. Hiring and training initiatives are integral components of Lean Six Sigma projects, ensuring consistent delivery of quality products and services. Proactive recruitment and training efforts mitigate talent gaps and establish succession plans. Government grants offset wage costs for new hires, particularly youth, and subsidize training programs to cultivate a skilled workforce. These grants cover internal and third-party training initiatives, supporting ongoing skill development and organizational growth.
Onboard the right members and train them to become highly skilled with Hiring & Training funding programs. You can upskill your labor without incurring new costs.
Funding for Research and Development
Research and development underpin the foundation of Lean Six Sigma projects, driving sustainable performance improvements. Formalizing the research and development process enables businesses to leverage insights for growth and innovation. Grants for research and development projects are available nationwide, fostering collaboration between businesses and academic institutions to spur innovation. These programs accelerate operational strengths through innovative research, with government funding catalyzing the R&D process and fostering long-term organizational resilience.
The Canadian government highly prioritizes Research and Development projects. There are many funding options available to upgrade your processes – explore our directory today.
Develop a Proactive Funding Plan
Lean Six Sigma project plans provide a great starting point to launch a sustainable business development strategy, but these plans will not happen if the right actions are not taken. Developing a Proactive Funding Plan for continuous improvement helps align government funding so that lean projects can be completed more frequently.
Download our free guide on how to Build A Government Funding Plan . With this slide deck, business executives will be able to identify projects and priorities well-suited for funding; apply for funding programs at the best time possible; and understand government impacts required for funding approval.
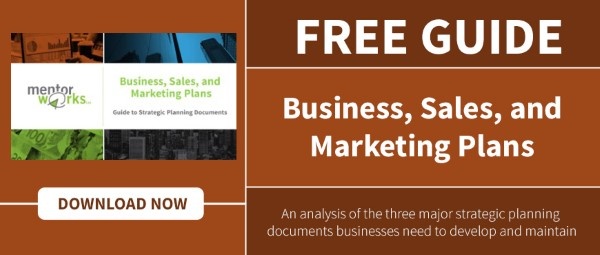
Leave a Reply Cancel reply
Your email address will not be published. Required fields are marked *
Save my name, email, and website in this browser for the next time I comment.
- Our Courses
- About DCM Learning
- Training Resources
- Inhouse Training

Lean Six Sigma Project Examples | 17 Full Case Studies
Ready to begin your first Lean Six Sigma project? Looking for examples for inspiration or reference to get you started? Here are some project storyboards from different industries and from home. Remember, Lean Six Sigma can help you with more than just work!
- Reducing Underwriting Resubmits by Over 20%
Governments
- A Call to Change: Pioneering Lean Six Sigma at Los Angeles County
- Can Lean Six Sigma Be Applied in County Government?
- How the City of San Antonio Increased Payments for Street Maintenance Using Lean Six Sigma
- Reducing Bid Tab Creation Cycle Time by 22%
- Reducing Cycle Time for Natural Disaster Response by 50%
Manufacturing
- Increasing First Run Parts From 60% to 90% With Lean Six Sigma
- Reducing Bent/Scratched/Damaged (BSD) Scrap for Building Envelopes
- Reducing Lead Time in Customer Replacement Part Orders by 41%
- Reducing Learning Curve Ramp for Temp Employees by 2 Weeks
- Reducing Purchase Order Lead Time by 33% Using Lean Six Sigma
- Herding Cats Using Lean Six Sigma: How to Plan for and Manage the Chaos of Parallel Processes
- Lean Six Sigma Increases Daily Meat Production by 25%
- Lean Six Sigma Helps Feed People In Need 45% Faster
- Accelerating Lean Productivity With Immersive Collaboration
- Reducing Incorrect Router Installations by 60% for Call One
- Reducing Software Bug Fix Lead Time From 25 to 15 Days
Lean Reviews: Stories from Our Customers
Over the past 2 years, over 2,000 learners (2,197 to be exact) have come to DCM to learn more about lean and get certified. Read (and watch) their reviews to see how our courses have helped them achieve their career goals.
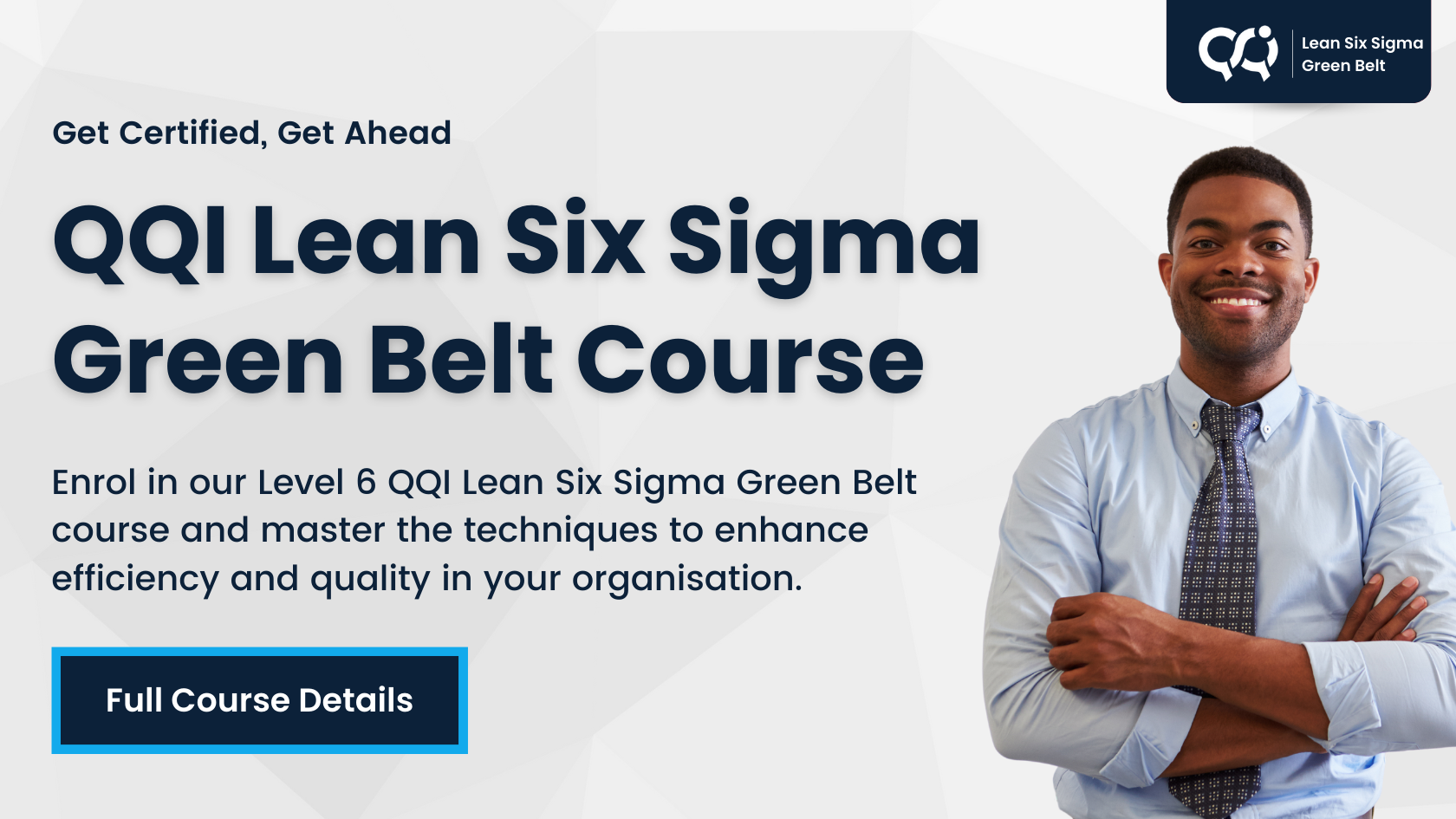
Inhouse Tailored Training for Your Team
We provide training programs that are developed by industry, for industry. Our range of programs can be delivered in a way that suits the needs of your business to offer your employees learning that is accessible and flexible.
We add value to your business by providing specialised, flexible and scalable training that meets your training needs. As your workforce grows and evolves, our globally certified and industry-validated learning solutions can assess, train and qualify your employees. For more information on how we can help please visit the in-house training page .
Membership, Stay Connected. Stay Relevant.
Completing a program is a point-in-time exercise that delivers huge value, but there is a next step to maintaining the currency of your skills in the ever-evolving professional world.
Membership is the next step .
A unique platform, membership is designed to ensure that you are in tune and up-to-date with the latest tools, trends and developments. Being a member provides just-in-time training and continuous professional development, and an exclusive and evolving content library informed by subject matter experts and industry leaders.
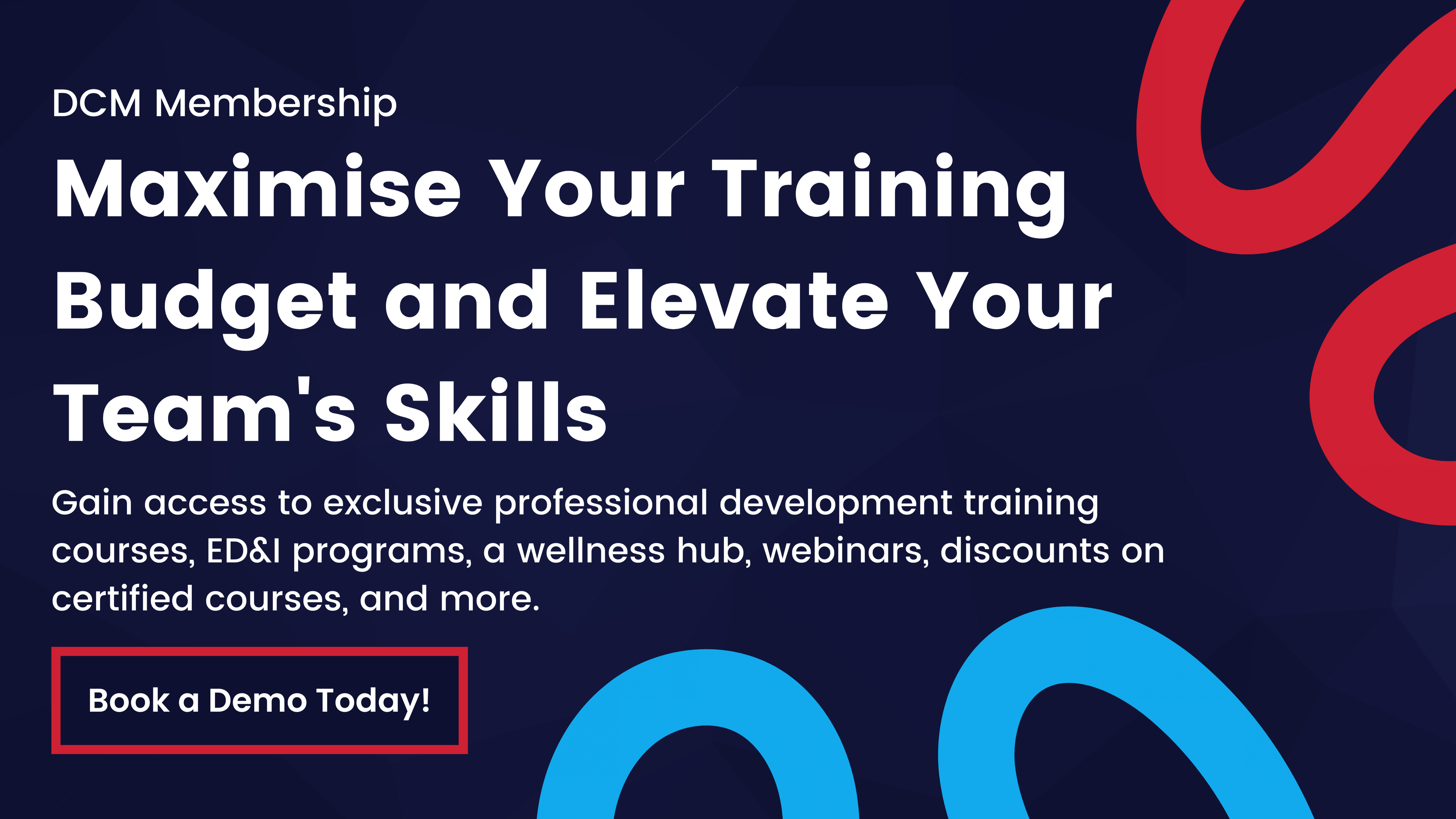
Unlock your full learning potential with our free DCM membership! Join our community and access exclusive resources to boost your educational journey.
Thanks for signing up. Here are some training courses for you to explore.
By clicking “Start Learning”, you agree to automatically become a DCM member at no additional cost, unless already a member. You have the option to opt out at any time.
Free Bitesize Courses
We have over 100 free courses available to explore, all created by expert trainers, packed full of practical exercises and real-world examples.
Make sure you Safeguard Your Training Investment . DCM offer courses accredited by:
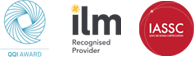
Course Categories
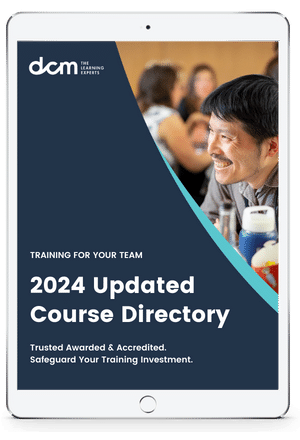
*Add your email and we will send you the full course directory to review at a time that suits you.
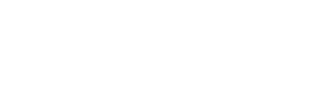
Open Your Brochure
Just to let you know, we are offering €200 credit for the course when you become a member with DCM.
We are very proud of our advisory team in DCM Learning. If you have any questions at all please feel free to ask our team. They will be more than happy to help.
We value your privacy and we will only get in touch about upcoming courses or events that are available to members.
It's so simple... We'll send you the €200 credit and access to the exclusive members area with a full list of the free courses.
By clicking “Get Your Voucher”, you agree to our terms and privacy policy .
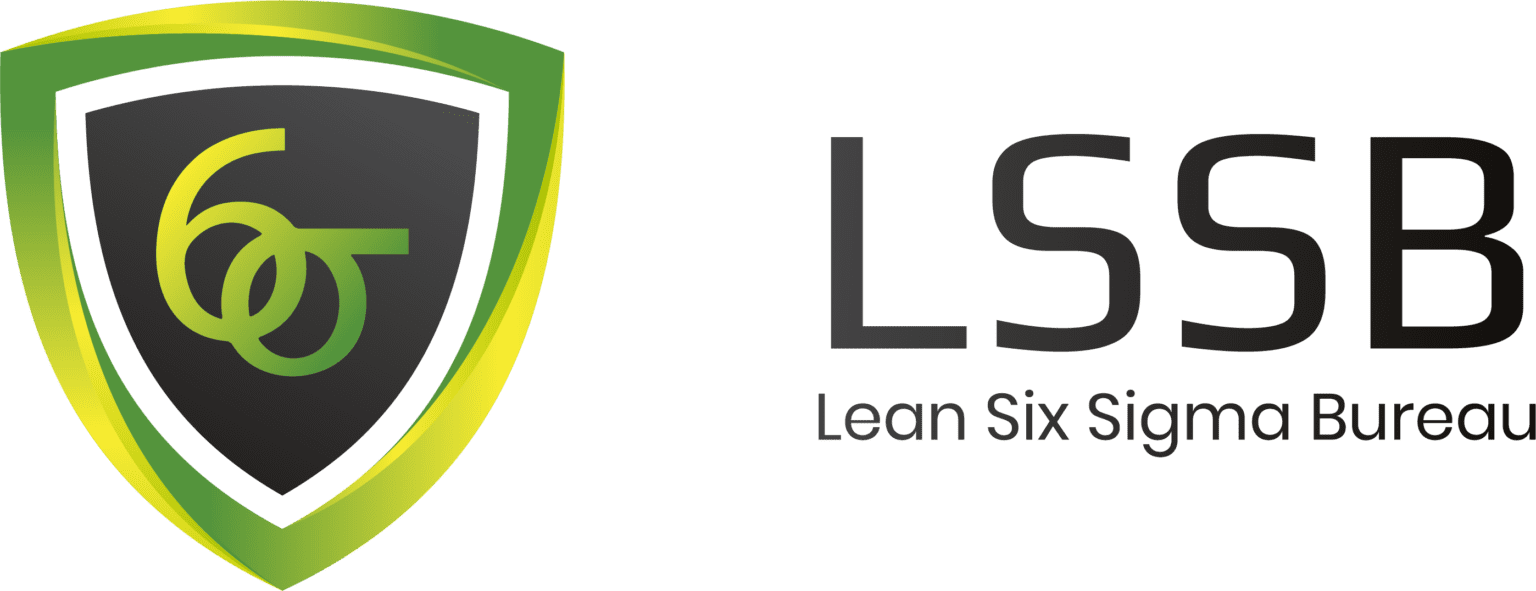
Shopping Cart
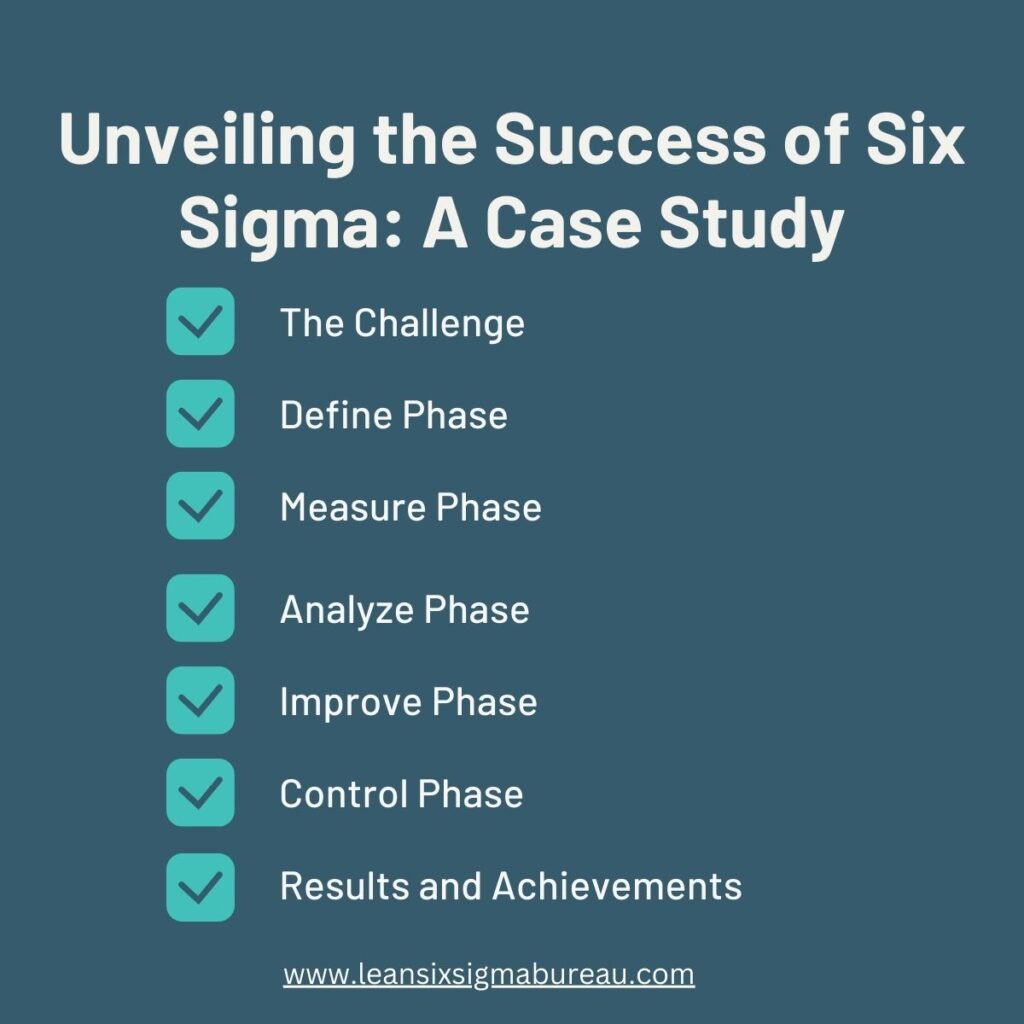
Learning path
- Lean Six Sigma White Belt (EN)
- Lean Six Sigma Yellow Belt (EN)
- Lean Six Sigma Green Belt (EN)
- Lean Six Sigma Black Belt (EN)
Quick links
- Get Certified
Get In Touch
- [email protected]
- Book a call
Follow Social Media
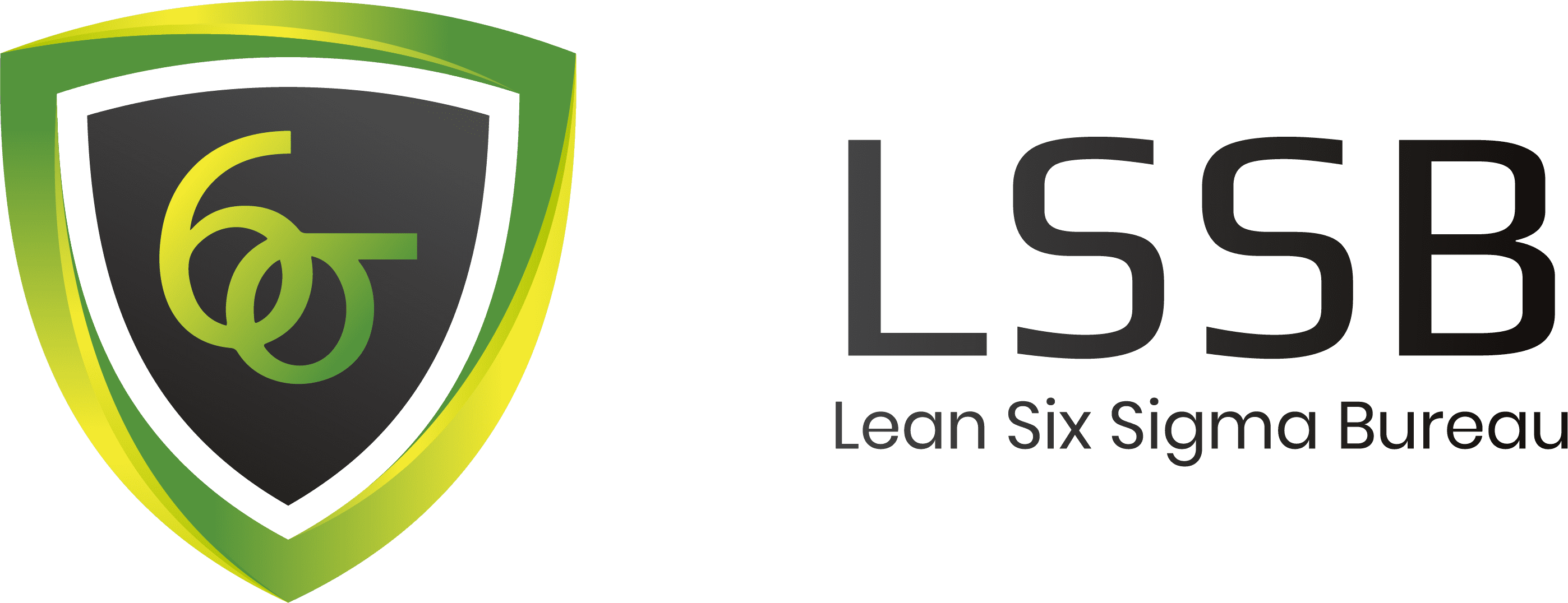
There was a problem reporting this post.
Block Member?
Please confirm you want to block this member.
You will no longer be able to:
- See blocked member's posts
- Mention this member in posts
- Invite this member to groups
- Message this member
- Add this member as a connection
Please note: This action will also remove this member from your connections and send a report to the site admin. Please allow a few minutes for this process to complete.
Lean Six Sigma for the improvement of company processes: the Schnell S.p.A. case study
The TQM Journal
ISSN : 1754-2731
Article publication date: 15 October 2021
Issue publication date: 17 December 2021
The aim of this study is to develop an in-depth case study on the implementation on Lean six sigma (LSS) in Schnell S.p.A., Italian company leader of an important multinational industrial group, highlighting the benefits that can be achieved from a careful application of this method, the main challenges and organizational learning from its implementation.
Design/methodology/approach
The study has been developed with a qualitative approach, creating a single in-depth case study, with the participant observation of researchers in the project which lasted 4 months. Periodic weekly meetings were done with the working group to exchange feedback on the development of the project to share opinions and data.
A project has been developed to stabilize the procurement process of a pull-type production cell, which experienced delays in supply lead times. The causes of the problems in their process of managing the supply of the production cell were found and some inefficiencies in the internal process of fulfillment of supply orders have been intercepted, the optimization of which has allowed the generation of an automatic system for sending supply orders, coming directly from the production line.
Originality/value
This study described the path and dynamics of the transformation process that business organizations undertake for optimizing their profitability and competitive advantage, placing emphasis on an innovative methodology for conducting business process improvement projects, which constitutes its operating philosophy on the effective and efficient use of company resources and skills, to guarantee to the company the achievement of a lasting and defensible competitive advantage over time.
- Lean thinking
- Lean production
- Quality management
- Continuous improvement
Murmura, F. , Bravi, L. , Musso, F. and Mosciszko, A. (2021), "Lean Six Sigma for the improvement of company processes: the Schnell S.p.A. case study", The TQM Journal , Vol. 33 No. 7, pp. 351-376. https://doi.org/10.1108/TQM-06-2021-0196
Emerald Publishing Limited
Copyright © 2021, Federica Murmura, Laura Bravi, Fabio Musso and Aleksandra Mosciszko
Published by Emerald Publishing Limited. This article is published under the Creative Commons Attribution (CC BY 4.0) licence. Anyone may reproduce, distribute, translate and create derivative works of this article (for both commercial and non-commercial purposes), subject to full attribution to the original publication and authors. The full terms of this licence may be seen at http://creativecommons.org/licences/by/4.0/legalcode
1. Introduction
The development of an effective quality improvement or continuous improvement strategy is a key factor for long-term success of modern organizations. Over the last decade, Lean Six Sigma (LSS) has become one of the most popular and proven business process improvement methodologies organizations have ever witnessed in the past ( Antony et al. , 2017 ), and it has been accepted globally as a management strategy for achieving Process Excellence ( Gijo et al. , 2019 ).
Lean Six Sigma is a management strategy for improving corporate productivity and profitability, that aim to maximize the Customer satisfaction by reducing constraints which the company organization is subject in terms of activities that do not create value for the Customer. In practice, LSS is an improvement strategy that analyze quantitative data on business performance to identify, eliminate and control problems and inefficiencies related to manufacturing cost, service cost, quality, productivity and customer satisfaction ( Singh and Rathi, 2019 ; Snee, 2010 ) throughout the business processes.
The objectives of quality and efficiency, supported by Lean Six Sigma, are made by DMAIC: a structured method for improving the performance of existing processes ( Sordan et al. , 2020 ), based on the application of the concepts Define, Measure, Analyze, Improve and Control. It provides a standardized guideline for the elaboration of improvement projects and provides different statistical tools and techniques appropriate to each phase of the DMAIC cycle ( Sordan et al. , 2020 ) able to lead to the root causes of business problems and to eliminate the wastes and reduce the variation, thus, ensuring substantial improvement in business processes ( Bhat et al. , 2020 ).
The term LSS was first introduced into literature around 2000 LSS, while LSS teaching was established in 2003 as part of the evolution of Six Sigma ( Timans et al. , 2012 ). Since that time, there has been a noticeable increase in LSS popularity and deployment in the industrial world ( Shah et al. , 2008 ) and researchers had the interest to publish more papers on LSS to try to come up with a comprehensive approach to achieve continuous improvement. However, as suggested by Albliwi et al. (2015) , there are still many gaps that need to be addressed in LSS literature such as benefits, motivation factors, challenges and limitations ( Pepper and Spedding, 2010 ; Laureani and Antony, 2012 ), and there is also a lack of research in the relation between LSS and organizational learning and in recent years a lot of systematic literature reviews on the topic have been published on the topic but only few case studies have been analyzed in the research field to cover this gap.
Therefore, the aim of this study is to cover this gap, by developing an in-depth case study on the implementation on LSS in an Italian company leader of an important multinational industrial group, that is Schnell S.p.A., that constitutes its main research and production center and provides technological, organizational and commercial support for the entire group. Schnell operates in over 150 countries around the world through its 11 subsidiaries, over 50 agents and resellers, and a dense network of service centers.
This research work has the objective of highlighting the benefits that can be achieved from a careful application of LSS method in the company, the main challenges and also organizational learning from LSS implementation, showing its application in details in an important reality like that of Schnell S.p.A.
The paper is structured as follows: Section 2 depicts the theoretical background, describing the merging of Lean Production and Six Sigma and defining the critical success factors of lean six sigma implementation; Section 3 defines the methodology used, Section 4 presents and discusses the results of the case study while the last section draws the main conclusions.
2. Literature review
2.1 the merging of two quality philosophies: lean production and six sigma.
The LSS notion was announced to the world in 2002, when Michael George used it for the first time in the book “Lean Six Sigma: Combining Six Sigma with Lean Speed” ( Sordan et al. , 2020 ; Sreedharan and Raju, 2016 ). He is the founder and Chief Executive Officer of the George Group, one of the largest LSS project consulting firms in the United States.
Although its appearance is quite recent, LSS arise from two complementary but different approaches ( Sordan et al. , 2020 ): Toyota Production System (TPS), a famous organizational orientation developed in Japan, from the 1960s and 1980s, spread with the concept of “Lean Thinking”; and Six Sigma, a technical quality management program, introduced by Motorola Corporation in manufacturing arena in 1987 ( Singh and Rathi, 2019 ).
The synergy between Lean and Six Sigma created a data-driven ( Sreedharan and Raju, 2016 ) and top-down business strategy to improve the quality and productivity of organizations ( Singh and Rathi, 2019 ; Sordan et al. , 2020 ).
When we talk about Lean Thinking, we are talking about a business culture, based on respect, trust and cooperation between employees and oriented by a constant search for perfection that allows to reach the highest quality of products and services offered by the company and consequently to maximize customer satisfaction.
To achieve this goal of perfection and to optimize profits, corporate actions must be aimed at a constant effort to reduce costs and wastes of tangible and intangible resources, by distinguishing valued-added activities from non-value-added activities and eliminating wastes that increases cost without adding value in the eyes of the customer ( Antony et al. , 2017 ; Cudney et al. , 2014 ): activities that are unnecessary and not required for the operations of the business ( Jayaram, 2016 ).
Lean Thinking emphasizes on productivity improvement along with speed to respond to customer needs and create a streamlined, high-quality system that produces finished products at the pace of customer demand with little or no waste ( Lande et al. , 2016 ).
Wastes are called Muda, and they can be defined as real sins that hinder the ideals of perfection. The eight types of waste are defined as transport, inventory, motion, waiting, overproduction, overprocessing, defects and non-utilized skills ( Gijo et al. , 2019 ). To identify and eliminate Muda, Lean strategy brings a set of proven tools and techniques that allow to reduce lead times, inventories, set up times, equipment downtime, scrap, rework and other wastes of the hidden factory ( Lande et al. , 2016 ). Corbett (2011) affirms that while lean focuses on the elimination of waste and improving flow, it has some secondary effects: quality is improved; the product spends less time in the process, thereby reducing the chances of damage and obsolescence.
But we have to remember that the commitment to Lean Thinking must start at the top management level and should be cascaded down to various levels across the organization to improve flow and efficiency of processes ( Antony et al. , 2017 ).
Six Sigma (SS) is a business process improvement and problem-solving approach ( Lande et al. , 2016 ) that seeks to find and eliminate causes of variability, as well as defects or mistakes in business processes, by focusing on process outputs which are critical in the eyes of customers ( Antony et al. , 2017 ). The main objective of Six Sigma is to obtain “zero defect” or, in statistical terms, to reduce defects up to 3.4 parts per million opportunities ( Singh et al. , 2019 ).
To study variability, Six Sigma utilizes a problem-solving methodology to define, measure, analyze, improve and control processes and implement cost-effective solutions leading to significant financial savings ( Singh et al. , 2019 ) not only for manufacture sectors but also remove the defects throughout the corporations ( Singh and Rathi, 2019 ). This methodology is called DMAIC and it emphasizes on variation reduction, defect reduction and process evaluation (the effectiveness issue).
The complementarity between both approaches can be justified when the deficiencies inherent in each of them are observed, acting in isolation ( Sordan et al. , 2020 ). Both had produced tremendous results but had limitations: Lean is not well suited to resolving complex problems that require intensive data analysis, and advanced statistical methods, and, Six Sigma implementation showed how not every problem can be resolved with only a big data collection ( Antony et al. , 2017 ).
Lean does not address variation within a process; rather it addresses variation between end-to-end processes which appears in the form of waste. One of the major limitations of Lean is that it cannot be used to tackle problems related to process stability and capability ( Gijo et al. , 2019 ) and it tends to work best with “solution known” problems, where we realize that we are not operating to best practices, Lean implements them and make rapid improvements with minimal data collection ( Hoerl and Gardner, 2010 ). Six Sigma is most effective when used for improvement projects intended to drive processes towards process entitlement, in situations where the solution to the problem is unknown ( Snee and Hoerl, 2007 ).
As stated by Pepper and Spedding (2010) if lean is implemented without Six Sigma, there is a lack of tools to fully exploit the improvement of its potential. Conversely, if Six Sigma is adopted without lean thinking, there would be a cache of tools for the improvement team to use, but no strategy or framework to bring one's application to a system.
Combining Lean manufacturing principles and Six Sigma tools and techniques enables organizations to form a powerful improvement combination ( Hoerl and Gardner, 2010 ; Lande et al. , 2016 ) that has allowed many organizations to solve more problems quicker ( Antony et al. , 2017 ). It is a successful integration because Lean focuses on improving the flow of information and materials between the steps in the process and Six Sigma works to improve the value-adding transformations which occur with in the process steps ( Antony et al. , 2017 ).
LSS defines an approach, but of course does not dictate the specific progression of the project or dictate the unique mix of tools to be used, which of course needs to be problem specific ( Hoerl and Gardner, 2010 ). The appropriate blend of Lean and Six Sigma tools useful on any one problem therefore depends on the nature of the specific problem being solved ( Antony et al. , 2017 ).
The marriage between these two methodologies provides a more integrated, coherent and holistic approach to continuous improvement ( Pepper and Spedding, 2010 ) and has led to the creation of a breakthrough managerial concept ( Sordan et al. , 2020 ; Chiarini, 2012 ) with the aim to create a new business culture that breaks the link with the traditional way of working in all productive functions. LSS adds a new task to daily working duties: the recovery of operational efficiency through training growth of people, extensive use of data culture and problem-solving methodologies; all activities that simultaneously allow the improvement of quality, the costs and business complexities reduction, the increasing revenue ( Galdino de Freitas and Gomes Costa, 2017 ; Jayaram, 2016 ) and, finally, greater reliability of the services provided to the end customer. The application of LSS methodology results in reduced waste, defects and improve process, which in turn provide high-quality products at minimum cost, and this leads to customer delight, which ultimately raises the societal living standard ( Singh et al. , 2019 ; Jayaram, 2016 ), the well-being of employees and the quality of the work environment (Galdino de Freitas and Gomes Costa, 2017 ).
LSS aims not only to improve financial results through the improvement of company production processes, but it targets to help organizations build an adequate relationship with society, employees and the environment ( Galdino de Freitas and Gomes Costa, 2017 ).
Both Lean and SS require a company to focus on its products and customers and LSS as a part of management strategy to increase the market share and maximize profit ( Lande et al. , 2016 ). It produces benefits in terms of better operational efficiency, cost-effectiveness and higher process quality, because it promotes total employee participation from both top-down and bottom-up as a win-win practice to both management and staff members ( Gijo et al. , 2019 ).
2.2 Critical success factors of lean six sigma implementation
Lean Six Sigma strategy is versatile in nature and has a lot of applications in a variety of industries.
It can be applied in manufacturing as well as non-manufacturing environment ( Singh and Rathi, 2019 ). It has broad applicability in service, healthcare, government, non-profits, education ( Antony et al. , 2017 ) automotive, textile, steel and aerospace industries ( Sordan et al. , 2020 ). Although LSS has its roots in manufacturing, it is proven to be a well-established process excellence methodology in almost every sector despite its size and nature ( Gijo et al. , 2019 ). It is useful in small-and medium-size organizations as well as large organizations ( Antony et al. , 2017 ).
LSS is also suitable for less experienced organizations: Bhat et al. (2020) write about the successful deployment of LSS strategy in an Indian industry with orthodox industrial practices, limited manpower, constrained capital and confined knowledge on scientific improvement practices, and the research proves that even a novice user can effectively participate and implement LSS with proper mentoring to enhance the system.
Regardless of the sector in which the LSS is applied, this shows the spread of LSS in various organizations as one of the best strategies for organizational excellence ( Sreedharan and Raju, 2016 ). But it is important to remember that achieving maximum strategic and management efficiency cannot be based on the replication of principles and models of Lean approach.
Each organization is immersed in different social, cultural and economic conditions. For this reason, lean tools must be sized and customized on business contexts and simultaneously the entire business organization must be adapted to the changes that Lean Six Sigma generates and that it needs to be applied effectively ( Lande et al. , 2016 ; Raval et al. , 2018 ; Singh et al. , 2019 ; Gijo et al. , 2019 ).
These requirements for cultural change are the main critical success factors for LSS ( Sreedharan and Raju, 2016 ).
Critical success factors are the actions and processes that must be controlled by the management ( Lande et al. , 2016 ) during the implementation of a LSS project.
Top management involvement and commitment ( Lande et al. , 2016 ; Gijo et al. , 2019 ). The top management involvement and commitment are essential for successful implementation ( Pepper and Spedding, 2010 ) of any LSS initiative. It must personally support all improvement initiatives and integrate the LSS culture into entire organizations. Its active participation can multiply the positive project effects and make a significant impact at all levels ( Gijo et al. , 2019 ). If the top management will not take initiatives and not show their full involvement it could cause the failure of LSS implementation ( Singh et al. , 2019 ).
Employee involvement, empowerment and training ( Lande et al. , 2016 ; Gijo et al. , 2019 ; Bhat et al. , 2020 ). The cultural growth of internal staff is the heart of LSS programs because it offers necessary tools to create a clear vision of the project, to focus on teamwork and, above all, to fight the resistance to cultural and operational changes ( Singh et al. , 2019 ; Sunder and Antony, 2018 ). Employee training also contributes to gain a high level of internal communication which facilitates the implementation of LSS ( Lande et al. , 2016 ; Singh et al. , 2019 ; Gijo et al. , 2019 ; Bhat et al. , 2020 ). Training is necessary to create a supporting infrastructure (the belt system) and a holistic approach to improvement including area of application and methodology used ( Antony et al. , 2017 ). The belt system includes Master Black Belt, Black Belt, Green Belt, Yellow Belt and depending on the complexity of the problem considered and skills required to solve it, the appropriate Belts are selected ( Gijo et al. , 2019 ) to play the role of leadership and guidance of the project team.
Linking LSS to business strategy and customer satisfaction ( Lande et al. , 2016 ). Improvement projects must be closely linked with maximizing customer satisfaction. Top management defines business objectives and identifies improvement projects capable of guaranteeing greater remuneration in terms of optimizing company productivity and profitability, as well as projects that can be reached using available resources, which do not require high investments and which allow to obtain undisputed results with limited deadlines in a limited period of time. Improper linkage between organizational objective and customer's requirement leads to failure of LSS implementation ( Singh et al. , 2019 ; Singh et al. , 2019 ; Gijo et al. , 2019 ).
3. Methodology
The study is a conceptual development and it has been developed with a qualitative approach, creating a single in-depth case study of Schnell S.p.A. that derives from a Group Purchasing Excellence Project. The case study allowed for examining in depth the implementation of a Lean Six Sigma improvement project for the transformation and simplification of the production process of the Schnell “Alfa” and “Beta” machines with the aim to reduce the delivery times of its products ( Yin, 1994 ). The case study was developed with the participant observation of researchers in the project which lasted 4 months, starting from November 4, 2019 to March 4, 2020. As for the participant observation, the researcher was directly involved in the LSS implementation activities, collaborating with the working group in the figure of the project manager, and facing directly obstacles and problems that emerged during these stages of the same (par. 4.2.1.1 will define the detailed description of the project). Periodic weekly meetings were done with the entire working group to exchange feedback on the development of the project, to share opinions and data. Participant observation activity was triangulated with secondary data, such as company reports and the website, collected during the period of support in the company. Secondary data have been used mostly to describe Schnell history, structure and the services it offers to customers.
Minitab 19 statistical analysis software was used to describe and summarize the data collected during the project and shown in the result section.
4. Results and discussion
4.1 company profile: schnell s.p.a.
Schnell S.p.A. is an Italian company that has been operating for almost 60 years in the manufacturing sector of automatic machines and plants for processing iron for reinforced concrete. It was born in 1962 thanks to the devotion of a group of entrepreneurs, driven by the dream of transforming the tiring and dirty world of iron working, into a modern industry, dedicated to conquering the global market. The company embarks on its own path by offering a first innovative solution that allowed faster binding of the reinforcing bars, flanked by the production of construction site machinery for cutting and bending the bars. The rise in the automatic machinery sector has started with the development of mechanisms for the production of cylindrical cages; however, the real change of course compared to its competitors will take place with the addition of electric servomotors, used, before now, only in fields such as robotics and military industry. Thanks to this type of instrumentation, Schnell machines are characterized by high power, speed, reliability and precision. They guarantee to the customer the achievement of economies of scale and better production techniques due to the high productivity offered, reduced set up times and low maintenance costs. Schnell S.p.A. offers the market a high range of machines and systems that allow a variety of processing of iron for reinforced concrete, including straightening, stirrup bending and shaping machines for bending, shaping and cutting iron in rolls or bars; cage making machines for the formation of cylindrical poles and cages for construction; machines and plants for the production of electrowelded mesh; machines for wire straightening and cold rolling lines; rotor straightening machines for processing steel wires for the industrial sector; machines for the production of prefabricated insulating panels for building construction; software for the management of iron processing centers using Schnell automatic machines. As a result of the high quality of these products, Schnell S.p.A. has managed to win the trust of its customers all over the world, reaching a turnover of over 100 million euros.
The Schnell Group is characterized by a staff of over 700 employees worldwide, and is made up of 5 production plants; 7 centers for installation, sales, spare parts and after-sales services; Schnell Software (Spain), which is a center for the creation and development of software systems for the management and organization of production carried out using Schnell machines and Schnell Home S.r.l., production center of machines for the construction of innovative elements for building construction, called “Concrewall”. Achieving a highly competitive advantage over its competitors in the same sector was possible due to constant investments in research, development and technological innovation of products and processes. Product innovation, since the company is always ready to respond to market needs through the development of a customer-oriented approach, which allows to offer integrated and customized production solutions. Process innovation, since, as stated in the “Integrated Quality Policy” and “Purchasing Excellence Group Program” of Schnell S.p.A., the efforts of the whole company are oriented to create effective methods of managing internal operational processes, with a view to maximizing end customer satisfaction.
As a result of the constant commitment in this direction, at the end of 2007, Schnell S.p.A. managed to obtain the quality system certification according to the ISO 9001 standard, delivered by the prestigious certification body TUV Italy, and renewed in 2019 in compliance with the updates undergone by the standard in September 2015.
The important results obtained in terms of product and process quality was also possible due to the dissemination and application of Lean Manufacturing principles and methodologies.
4.2 The development of the lean six sigma project in Schnell S.p.A
The layout of the cell, the equipment and the production tools have been designed and arranged horizontally following the phases of the process;
The production plans were planned on order, therefore, on the basis of the orders received from its customers, following the production theories with the pull logic;
The manufacturing of the machines was organized in small batches conducted with the one-piece flow system;
The management of the entire procurement process of raw materials and production components has been entrusted to the Kanban system;
The line operators have been trained to complete all manufacturing operations in complete autonomy.
The products supplied with their own identification codes;
Periodicity of reordering;
Minimum order quantity;
Delivery Lead Time (in working days);
Safety Stock Level: quantity of products to be held in the warehouse as a mandatory stock;
Technical specifications of production;
Specifications for packaging and delivery.
For further stabilization of the production process, aimed at increasing product quality, the characterizing element of the In-Lining Line was to reach a Free-Pass quality level. This qualitative incoming methodology has allowed a high reduction in the variability of the external production process, of the components characterized therein, while requiring significant direct and indirect investments by sourcing.
The entire In-Lining apparatus is governed by a vital element for the correct planning of the production phases: the supply Lead Time.
This index represents the time elapsing from the time of issue of the purchase order to the time of actual receipt of the goods. It allows to efficiently plan the supply of production components, and therefore, to define the periods for sending purchase orders.
Lead time of supply;
On-time Delivery (the ratio between the number of orders processed on time and the number of total orders processed, in the period considered).
With a view to Project Management, a work team was set up with the task of studying and analyzing the procurement process of the In-Lining line, and the phases of the Plan-Do-Check-Act (PDCA) and DMAIC approach were followed for the implementation of the project.
4.2.1 “Define” phase
The objective of the first phase of the project was to identify all the aspects necessary to define the process to be improved, therefore, to develop a planning prospectus called Project Charter containing: the representation of the problem detected, the objectives to be achieved, the requirements required from the customer, the inputs and outputs of the process and the metrics necessary to measure it, the enhancement of the current process and possible savings achievable by improving the process, the team members, and finally, the deadlines of the project phases.
4.2.1.1 Project description
Analyzing the lead times of supply of the supplying process of the In-Lining Line, conducted with the Kanban system, it was reported that the most important supplier in terms of quantity, tends not to respect the agreed delivery terms.
Upper specification limit (USL) = LEAD TIME 5 days (working);
Lower specification limit (LSL) = LEAD TIME 2 days (working).
Analyze the deliveries to the line of the last available calendar period, from 01/11/2018 to 31/10/2019;
Perform stratification of the detected deliveries, until the root causes are reached;
Define the initiatives and control charts to ensure the stability of the procurement process over time.
Lead Time of supply;
Defects per Unit – DPU;
Defects Per Million of Opportunity – DPMO;
Sigma Level.
The project team was made up of the members defined in Table 1 .
The implementation of the DMAIC phases was organized through the Gantt Chart ( Figure 1 ), with the aim of a precise subdivision over time of the individual activities to be carried out, while all the information that defines the project was collected in the Project Charter document of Figure 2 .
4.2.1.2 Project risk analysis
During the planning of the project, different potential risks were identified that could affect the smooth running of the project. These were found in relation to different sources from which they could derive (see Table 2 ).
Severity (P): expresses the potential damage that the occurrence of the risk could cause in the implementation of the project;
Occurrence (G): expresses the probability that the risk may occur;
Detection (R): expresses the probability of risk detection once it has occurred.
Each variable was assigned a score from 1 to 5, in which 1 represents an insignificant risk condition and 5 that of extreme risk (only for the Detection variable, the lower the score assigned, the greater the probability of risk detection).
The most critical risks have been identified through the Risk Priority Index – Risk Priority Number (RPN) obtained from formula f.1. f .1 ) RPN = S × O × D
The highest priority was checked for the risks “Inability to use software” and “Insufficient knowledge and skills of members” (see Table 3 ).
4.2.1.3 Process representation
To obtain a macro view of the process, the Supplier Input Process Output Customer (SIPOC) diagram has been developed ( Figure 3 ) which highlights the main elements that make up the activities examined.
4.2.2 “Measure” phase
The second phase was aimed at defining and measuring the progress of the process at the current stage. For a better representation, the flow of activities necessary to replenish the In-Lining line has been outlined through the Flow Chart ( Figure 4 ) which identifies on the left side the operations that add value within the process (AV), while, on the right side, those with non-added value (NAV), therefore considered as waste.
The process was further represented through the Value Stream Mapping technique ( Figure 5 ) which allowed to estimate a total Process Time (P/T) of 11.6202 h (11 h 37 min and 12 s), divided into 11.40417h (11 h 24 min and 15 s) for value-added activities and 2.216 h (12 min and 57 s) for non-value-added activities. Together with downtime and shipping times, the entire process is performed with a maximum total Lead Time (L/T) of 8 days, 8 h, 5 min and 28 s.
4.2.2.1 Data collection
PRODUCT A.1;
PRODUCT A.2;
PRODUCT B.1;
PRODUCT B.2;
PRODUCT C.1;
PRODUCT C.2;
These products are characterized by belonging to similar categories, therefore, with the aim of greater interpretation and a better comparison of data, the population has been grouped into stratified categories with reference to the product group to which they belong, type of production component and final product.
4.2.2.2 Interpretation of data with statistical tools
In the first phase, the graphical summary analysis was performed ( Figure 6 ) showing the results of the Anderson-Darling Normality Test, the descriptive statistics and the confidence intervals for the mean, median and standard deviation of the data population in exam. The graphs show that deliveries are characterized by an average delivery lead time of 9.4324 working days which falls within a range of 70 working days. The recorded variation therefore determines a standard deviation of 14.4877.
Second, from the Anderson-Darling normality test, a p -value <0.005 is obtained: this value demonstrates that the analyzed data derive from a distribution that cannot be approximated to a Gaussian model.
The current result is a consequence of the fact that in the population, in correspondence with the value in the 3rd Quartile of 7 days and Maximum of 74 working days, irregular values can be highlighted, called outliers, which arise from particular causes of a special type, and which therefore prevent a regular data analysis and interpretation, negatively affecting all study results.
It was highlighted that these were four deliveries relating to the same order, made on August 31, 2018, of two components of CATEGORY C, in particular of PRODUCT C.2.
Through a more in-depth investigation, it was possible to observe that the supply agreement was drawn up and confirmed prior to the first delivery of the product in the sample phase. Consequently, the high delivery lead time was justified by the fact that the supplier had to provide totally new products, the production of which had to be studied and adapted to their production processes.
Given the particular situation, to carry out a more meaningful analysis, it was decided not to consider the indicated outliers values, and to run the graphical summary analysis again, this time on a population made up of N = 70 units ( Figure 7 ).
In this case, the standard deviation assumes the value 4.1852, the average delivery Lead Time tends to reduce to the value of 6.1429 working days; however, again it is possible to deduce a p -value < 0.005; therefore, the data derives from a distribution that cannot be approximated to a Gaussian model. It is possible to conclude that the entire process is not under statistical control: the distribution consists of values that cannot be approximated to a Gaussian model, characterized by a supply trend that cannot be predicted over time.
On the basis of these results, it was possible to state that the supplier encountered numerous difficulties in fulfilling supply orders from the In-Lining Line, since the delivery process of the components was characterized by Lead Times that deviate significantly compared to the average lead time recorded (see Figure 8 ).
To express the supplier's performance in terms of Process Sigma, the values of Table 4 were taken into consideration, which summarizes the variables necessary for the calculation of the Defects Per Units (DPU), the Defects Per Opportunity (DPO) and the Defects per Million of opportunity (DPMO) index: (1) DPU = Numerosità difetti rilevata ( D ) Numerosità campione ( U ) (2) DPO = DPU Opportunità di errore ( O ) (3) DPMO = DPO × 1.000.000
The supply of the In-Lining line is characterized by a Sigma Level equal to 1.85, therefore, the current process is carried out with a yield of 63.51%.
4.2.3 “Analyze” phase
Based on the considerations obtained from the measurements made in the Measure phase, in this third stage of the project the team's goal was to intercept the categories of components that found the greatest difficulties in the procurement process.
Considering the high variability of the delivery process, in order to identify priority areas of intervention, the analysis was further processed through the Pareto diagram and, for easier interpretation, it was carried out by stratifying the data on the basis of the single category of belonging (see Figures 9 and 10 ).
It was observed that 39% of deliveries ( Table 5 ), carried out in the period under consideration, were carried out outside the established lead time specifications of 5 working days. The supplier presents the greatest number of critical issues with the fulfillment of orders relating to the GROUP A category, in particular with the fulfillment of PRODUCT A.1 and PRODUCT A.2, and to a lesser extent, with PRODUCT B.1 and PRODUCT B.2.
For the GROUP B category, difficulties were found in the delivery of the PRODUCT C.2 and PRODUCT D components; however, for the latter, the non-conformities found cannot be analyzed, as they are insignificant.
4.2.4 “Improve” phase
In the Improve phase, the purpose of the study activity was to identify the root causes of the problems that the Business Partner identified in the process of fulfilling the supply orders of the In-Lining line, and secondly to identify the paths for improvement to correct the criticalities detected.
4.2.4.1 Root cause analysis
The study was developed by analyzing the temporal trend of orders in the period considered for each PRODUCT category indicated at the end of the Analyze phase. For deliveries with greater difficulty, inquiries were carried out on the dates of issue and actual delivery of supply orders. In this phase, the help offered by the Production Planner of the Production Department who deals with the management of the production planning of the In-Lining cell was of great support. First of all, it was possible to deepen that in the delivery process of PRODUCT A.1 and PRODUCT C.2, in relation to the deliveries of the orders of the week 3/2019 and 2/2019, issued respectively with Lead Time of 23 and 22 working days, the supplier communicated the breakdown of a machinery necessary for the production of the components; therefore, it was not able to respect the contractual specifications. The Lead Time values detected here can be considered as outliers, determined by causes of a special type.
By analyzing PRODUCT A.2, it was possible to ascertain that some phases of the production process of the supplier in question were carried out in outsourcing to external suppliers not regulated by subcontracting contracts and, therefore, without evaluations in terms of lead time. As a result of this type of production management, instabilities in the internal delivery process have been generated.
For some deliveries, the supply lead time has been calculated incorrectly.
The supplier tends not to comply with lead time specifications, especially after prolonged company closure periods and in correspondence with orders processed in short periods.
To identify the root cause of the difficulties highlighted, the Five Why (5Why) method was used, which allowed to identify the cause-and-effect relationships of the problems to be analyzed ( Table 6 ). With the help of this problem finding tool, it was possible to ascertain that for some deliveries examined, the delivery lead time was calculated incorrectly as for orders corresponding to the deliveries themselves, the generation date did not correspond to the date of sending the order to the supplier. The system for sending supply orders for the In-Lining line provides that the verification and approval phase, carried out after the automatic proposal generation phase, takes place manually through the action of the Back Office – Purchase Department operator. In situations of absence of the operator, or late approval of the order, the supplier receives the document on a different date from that of issue.
With reference to the second problem identified, it was analyzed that the Business Partner highlights critical issues in terms of supply lead time, in relation to the fulfillment of orders received following prolonged company closure periods and for those received in short periods.
In the first case, these are deliveries made in the time interval corresponding to the periods of early January, late April and early September: time intervals that follow the periods of company holidays for national holidays.
It was assumed that prior to these company holiday periods, the warehouse safety stock was entirely consumed and not restored with further production of components. Therefore, it was considered that the supplier finds it difficult to ensure the restart of the post–holiday production activity through the forecast of its monthly requirements; therefore, it is unable to prevent the stock breaking of its warehouse.
For the second case, however, the supplier presented difficulties in fulfilling the orders placed in correspondence of short periods. More precisely, an out of specification Lead Time was highlighted in correspondence with the second/third order received in a monthly time interval. Also, for this criticality it has been hypothesized that there may be difficulties in ensuring an efficient planning of production activities and a correct forecast of one's monthly requirements, without incurring stock-outs in one's warehouse.
Activate an automatic system for generating, approving and sending orders to the supplier;
Arrange a meeting with the business partner in order to discuss the critical issues detected in the period studied.
With the aim of preventing further errors in the measurement system of the supply lead time indicator, and therefore overcoming the time gaps recorded between the generation phase and the order sending phase, the information technology (IT) department was entrusted with the task to generate an IT system that can automatically complete the entire process of fulfilling the supply orders coming from the In-Lining line. Considering the utmost importance of this improvement activity, the automatism created was implemented in the process starting from the first week of February 2020.
Check the efficiency of internal production planning;
Verify whether the process of managing the economic lot and purchasing the components creates an imbalance in the company loan;
Check if all the clauses contained in the stipulated subcontracting contract have been effectively understood;
Check if in the production planning phase, the periodicity of reordering of components is taken into consideration.
Lastly, having ascertained the delivery problems encountered when supplying the PRODUCT A.2 component, the Management of the production process of the In-Lining line carried out a strategic Make or Buy analysis. As a result of the evaluation carried out, on 14/11/2019, the subcontracting contract was canceled and the procurement of the components was entrusted to an alternative Business Partner.
4.2.5 “Control” phase
In the last phase of the DMAIC project, some activities were identified and implemented in order to keep under control the improvement activities introduced in the Improve phase.
To verify the operation and validity of the automated system for generating, approving and sending the supply orders of the In-Lining line, the IT department has launched a checkup mechanism with the aim of transmitting to the Purchase Department a daily report on the effective sending of orders created automatically.
Considering, however, the need to investigate the possible difficulties encountered, the meeting with the Business Partner was scheduled for the second week of March.
4.3 Benefits deriving from the implementation of the project
After an accurate analysis of the problem related to the reduction of lead time and its causes, it has emerged that the main concern is that in most cases the supply lead time has been calculated incorrectly, while in others supplier tends not to comply with lead time specifications, mostly after company closure periods and when orders are processed in short periods.
First, the implementation of the project has made the company become fully aware of the inefficiencies present in the delivery process of some of its components, allowing a high reduction in the variability of the external production process of these components. Reducing delivery times has also allowed to better plan the supply of production components, defining the periods for sending purchase orders. An automatic system for managing supplier orders has been activated, and it has permitted to reduce errors during the order creation and management process, having a positive effect on the consolidation of the process under consideration. Moreover, a meeting with suppliers was carried out and it has permitted to discuss and confirm together with the business partners the clauses contained in the subcontracting contract, to better plan the periodicity of reordering of components, but also internally improve the efficiency of production planning. From a quantitative point of view, the benefits will be assessed over the long term, with a careful analysis.
5. Conclusion, implications and future research directions
This study was carried out with the main objective of describing the path and dynamics of the transformation process that business organizations undertake with the aim of optimizing their profitability and competitive advantage following the profound environmental changes to which they are subject to, placing emphasis on an innovative methodology for conducting business process improvement projects, known as Lean Six Sigma, which constitutes its operating philosophy on the effective and efficient use of company resources and skills, to guarantee to the company the achievement of a lasting and defensible competitive advantage over time. Lean Six Sigma has been presented in this research as a methodology for improving business productivity, which operates through the reduction of the constraints and inefficiencies of each production and transactional process, aspiring to the maximum satisfaction of the internal and external customer and is configured as a real strategy, which offers to the human resources an innovative way of thinking and working based on training growth, data culture and the use of problem-solving methodologies that allow the improvement of quality, the reduction of costs and company complexities. In this detailed case study, the DMAIC technique was applied in a project to stabilize the procurement process of a pull-type production cell, which experienced some problems in terms of delays in supply lead times.
Thanks to the analyses carried out and the results obtained with the processing of the DMAIC phases, it was possible to highlight the potential causes of the problems that the business partner could have presented in their process of managing the supply of the production cell. Furthermore, some inefficiencies in the internal process of fulfillment of supply orders have been intercepted, the optimization of which has allowed the generation of an automatic system for sending supply orders, coming directly from the production line; a small tweak that will undoubtedly have a positive effect on the consolidation of the process under consideration, as the purchase department will be able to both keep order fulfillment under control and develop a more efficient measurement of business partner performance indicators.
With the development of the project, it was possible to structure the initial guidelines for the subsequent in-depth analysis of the critical issues identified. In particular, for the stabilization of the entire process, Schnell S.p.A. will have to develop an intense relationship of collaboration and mutual growth with his supplier to identify and implement the best solutions to the variability of the supply order fulfillment process.
The practical implementation of the Lean Six Sigma project confirmed the validity and power of the principles professed by this improvement methodology: the importance of customer orientation and the elimination of waste of resources; the value of a work team and the continuous search for qualitative and quantitative data that support and facilitate the decisions of each member of the group.
It was particularly fruitful to discover how collaboration and involvement within an LSS working group amplifies the skills and knowledge of each participant and generates a widespread climate of enthusiasm and strong determination for continuous improvement in every area, both at work and personal level.
Another practical implication that emerged from the study was the high importance to be attributed to the process of measuring company performance. From a consistent database and their level of reliability, it is possible to identify important opportunities for improvement and savings in terms of company resources; the data make it possible to highlight significant problems and inefficiencies, otherwise not recognizable, which are the result of high company costs that impact on company profitability.
The research shows how Lean Six Sigma can offer companies high advantages in achieving the highest quality in the value creation process, however, to ensure the successful success of projects, the desire for change must arise from the depths of top management; it will have to assume the role of promoter of the LSS culture and philosophy, so that the tools of the methodology are effective in managing and guiding the improvement and transformation actions, one step at a time, with rigor and discipline, but with the involvement of all own resources, with the greatest possible efficiency and effectiveness.
The main limitation of the study derives from the qualitative methodology adopted, that while it permits to analyze in depth and broadly all the phases of implementation of the LSS in the company, highlighting the difficulties encountered during the activities and the benefits obtained, these results should be integrated with an analysis on a large sample of companies that have developed similar projects to be more generalizable. Future research should be oriented on developing a quantitative analysis on LSS implementation. In any case, a qualitative study of this depth can give ideas for improvement and development for companies similar in structure and dimension to Schnell S.p.A.
Gantt Chart of the project
Project charter
SIPOC diagram – supplier, input, process, output, customer
Flow Chart: Diagram of the procurement process through the Kanban system
Value stream mapping of the procurement process of the In-Lining line with Kanban system
Population stratification
Graphical summary statistical analysis of Lead Times recorded in the period 01/01/2018–31/10/2019. Population with numbers N = 74
Graphical summary statistical analysis of Lead Times recorded in the period 01/01/2018–31/10/2019. Population with numbers N = 70
Frequency of deliveries with centered and delayed lead time (a) and boxplot lead time (b) for sub-category
Frequency of deliveries with centered and delayed lead time (a) and boxplot lead time (b) for sub-category type
Composition of the project team
Project risk analysis
Project risk and calculation of the Risk Priority Index
Process sigma calculation
Report of the performances analyzed in the period November 2018–October 2019
Five why matrix
Albliwi , S.A. , Antony , J. and halim Lim , S.A. ( 2015 ), “ A systematic review of Lean Six Sigma for the manufacturing industry ”, Business Process Management Journal , Vol. 21 No. 3 , pp. 665 - 691 , doi: 10.1108/BPMJ-03-2014-0019 .
Antony , J. , Snee , R. and Hoerl , R. ( 2017 ), “ Lean Six Sigma: yesterday, today and tomorrow ”, International Journal of Quality and Reliability Management , Vol. 34 No. 7 , pp. 1073 - 1093 .
Bhat , S. , Gijo , E.V. , Rego , A.M. and Bhat , V.S. ( 2020 ), “ Lean Six Sigma competitiveness for micro, small and medium enterprises (MSME): an action research in the Indian context ”, The TQM Journal , Vol. 33 No. 2 , pp. 379 - 406 , doi: 10.1108/TQM-04-2020-0079 .
Chiarini , A. ( 2012 ), Lean Organization: From the Tools of the Toyota Production System to Lean Office , Vol. 3 Springer Science & Business Media .
Corbett , L.M. ( 2011 ), “ Lean Six Sigma: the contribution to business excellence ”, International Journal of Lean Six Sigma , Vol. 2 No. 2 , pp. 118 - 131 , doi: 10.1108/20401461111135019 .
Cudney , E. , Elrod , C. and Stanley , S. ( 2014 ), “ A systematic literature review of Six Sigma practices in education ”, International Journal of Six Sigma and Competitive Advantage , Vol. 8 Nos 3/4 , pp. 163 - 175 .
Galdino de Freitas , J. and Gomes Costa , H. ( 2017 ), “ Impacts of Lean Six Sigma over organizational sustainability. A systematic literature review on Scopus base ”, International Journal of Lean Six Sigma , Vol. 8 No. 1 , pp. 89 - 108 .
Gijo , E.V. , Jiju , A. and Vijaya , S.M. ( 2019 ), “ Application of Lean Six Sigma in IT support services – a case study ”, The TQM Journal , Vol. 31 No. 3 , pp. 417 - 435 .
Hoerl , R.W. and Gardner , M.M. ( 2010 ), “ Lean six sigma, creativity, and innovation ”, International Journal of Lean Six, Sigma , Vol. 1 No. 1 , pp. 30 - 38 .
Jayaram , A. ( 2016 ), “ Lean six sigma approach for global supply chain management using industry 4.0 and IIoT ”, Conference: 2016 2nd International Conference on Contemporary Computing and Informatics (IC3I) , pp. 89 - 94 .
Lande , M. , Shrivasatava , R.L. and Seth , D. ( 2016 ), “ Critical success factors for Lean Six Sigma in SMEs (small and medium enterprises) ”, The TQM Journal , Vol. 28 No. 4 , pp. 613 - 635 .
Laureani , A. and Antony , J. ( 2012 ), “ Standards for lean six sigma certification ”, International Journal of Productivity and Performance Management , Vol. 61 No. 1 , pp. 110 - 120 .
Pepper , M.P.J. and Spedding , T.A. ( 2010 ), “ The evolution of lean Six Sigma ”, International Journal of Quality and Reliability Management , Vol. 27 No. 2 , pp. 138 - 155 .
Raval , S.J. , Kant , R. and Shankar , R. ( 2018 ), “ Lean Six Sigma implementation: modelling the interaction among the enablers ”, Production Planning and Control , Vol. 29 No. 12 , pp. 1010 - 1029 .
Shah , R. , Chandrasekaran , A. and Linderman , K. ( 2008 ), “ In pursuit of implementation patterns: the context of Lean and Six Sigma ”, International Journal of Production Research , Vol. 46 No. 23 , pp. 6679 - 6699 .
Singh , M. and Rathi , R. ( 2019 ), “ A structured review of Lean Six Sigma in various industrial sectors ”, International Journal of Lean Six Sigma , Vol. 10 No. 2 , pp. 622 - 664 .
Singh , M. , Kumar , P. and Rathi , R. ( 2019 ), “ Modelling the barriers of Lean Six Sigma for Indian micro-small medium enterprises. An ISM and MICMAC approach ”, The TQM Journal , Vol. 31 No. 5 , pp. 673 - 695 .
Snee , R.D. ( 2010 ), “ Lean Six Sigma–getting better all the time ”, International Journal of Lean Six Sigma , Vol. 1 No. 1 , pp. 9 - 29 .
Snee , R.D. and Hoerl , R.D. ( 2007 ), “ Integrating lean and Six Sigma: a holistic approach ”, Six Sigma Forum Magazine , Vol. 6 No. 3 , pp. 15 - 21 .
Sordan , J.E. , Optime , P.C. , Pimenta , M.L. , Chiabert , P. and Lombardi , F. ( 2020 ), “ Lean Six Sigma in manufacturing process: a bibliometric study and research agenda ”, The TQM Journal , Vol. 32 No. 3 , pp. 381 - 399 .
Sreedharan , V.R. and Raju , R. ( 2016 ), “ A systematic literature review of Lean Six Sigma in different industries ”, International Journal of Lean Six Sigma , Vol. 7 No. 4 , pp. 430 - 466 , doi: 10.1108/IJLSS-12-2015-0050 .
Sunder , M.V. and Antony , J. ( 2018 ), “ A conceptual Lean Six Sigma framework for quality excellence in higher education institutions ”, International Journal of Quality and Reliability Management , Vol. 35 No. 4 , pp. 857 - 874 .
Timans , W. , Antony , J. , Ahaus , K. and Solingen , R. ( 2012 ), “ Implementation of Lean Six Sigma in small and medium-sized manufacturing enterprises in The Netherlands ”, Journal of Operational Research Society , Vol. 63 No. 3 , pp. 339 - 353 .
Yin , R.K. ( 1994 ), Case Study Research: design and Methods , 2nd ed. , Sage Publishing , Thousand Oaks, CA .
Acknowledgements
The authors acknowledge Schnell S.p.A. for supporting the research providing the data that allowed the realization of the case study.
Corresponding author
Related articles, we’re listening — tell us what you think, something didn’t work….
Report bugs here
All feedback is valuable
Please share your general feedback
Join us on our journey
Platform update page.
Visit emeraldpublishing.com/platformupdate to discover the latest news and updates
Questions & More Information
Answers to the most commonly asked questions here
Open Access is an initiative that aims to make scientific research freely available to all. To date our community has made over 100 million downloads. It’s based on principles of collaboration, unobstructed discovery, and, most importantly, scientific progression. As PhD students, we found it difficult to access the research we needed, so we decided to create a new Open Access publisher that levels the playing field for scientists across the world. How? By making research easy to access, and puts the academic needs of the researchers before the business interests of publishers.
We are a community of more than 103,000 authors and editors from 3,291 institutions spanning 160 countries, including Nobel Prize winners and some of the world’s most-cited researchers. Publishing on IntechOpen allows authors to earn citations and find new collaborators, meaning more people see your work not only from your own field of study, but from other related fields too.
Brief introduction to this section that descibes Open Access especially from an IntechOpen perspective
Want to get in touch? Contact our London head office or media team here
Our team is growing all the time, so we’re always on the lookout for smart people who want to help us reshape the world of scientific publishing.
Home > Books > Applications of Design for Manufacturing and Assembly
Application of Six Sigma in Semiconductor Manufacturing: A Case Study in Yield Improvement
Submitted: 02 March 2018 Reviewed: 21 August 2018 Published: 03 January 2019
DOI: 10.5772/intechopen.81058
Cite this chapter
There are two ways to cite this chapter:
From the Edited Volume
Applications of Design for Manufacturing and Assembly
Edited by Ancuţa Păcurar
To purchase hard copies of this book, please contact the representative in India: CBS Publishers & Distributors Pvt. Ltd. www.cbspd.com | [email protected]
Chapter metrics overview
2,396 Chapter Downloads
Impact of this chapter
Total Chapter Downloads on intechopen.com

Total Chapter Views on intechopen.com
The purpose of this chapter is to outline systematic implementation of the Six Sigma DMAIC methodology as a case study in solving the problem of poor wafer yields in semiconductor manufacturing. The chapter also describes well-known industry standard business processes to be implemented and benchmarked in a semiconductor wafer fabrication facility to manage defect and yield issues while executing a Six Sigma project. The execution of Six Sigma enabled identification of the key process factors, root cause analysis, desired performance levels, and Cpk improvement opportunities. Implementing multilevel factorial design of experiments (DOE) study revealed critical input parameters on process tools contributing to defect formation. Improvement performed on these process tools resulted in in-line defect reduction and ultimately improving final yields.
- semiconductor manufacturing
- design of experiments (DOE)
Author Information
Prashant reddy gangidi *.
- Independent Scientist, California, USA
*Address all correspondence to: [email protected]
1. Introduction
Six Sigma framework is a continuous improvement strategy that minimizes defects and process variation toward an achievement of 3.4 defects per million opportunities in design, manufacturing, and service-oriented industries [ 1 , 2 , 3 ]. Six Sigma practitioners often lead cross-functional teams in an organization to find and eliminate the causes of the errors, defects, lead, and cycle time delays in business processes. With rapid advancements in computers, artificial intelligence, and automotive vehicles, biomedical imaging semiconductor manufacturers find themselves constantly battling the demanding needs of the industry to sustain Moore’s law and manufacture smaller chips to support next-generation software and hardware products. In order to manufacture nanometer range scale chips, there is a tremendous impetus toward developing advanced process control and measurement system capabilities. Defects become a big challenge in the efforts to reduce feature size in most semiconductor fabs as they negatively impact product yields [ 4 ]. Six Sigma methodology is often neglected in most fabs, and this chapter gives an overview of its importance and how it can be implemented to reduce defects with the help of a general case study. This chapter presents the step-by-step application of the Six Sigma define, measure, analyze, improve, control (DMAIC) approach to eliminate defects in a lithography process of a semiconductor manufacturing organization. This has helped to reduce defects in the process and thereby improve the final probe yields on a critical technology node. During the measure and analyze phases of the project, data were collected from the processes to understand the baseline performance and for validation of causes. These data were studied through various graphical and statistical analyses. Chi-square test, ANOVA, design of experiments (DOE), control charts, fishbone analysis, FMEA, etc. were used to make meaningful and scientifically proven conclusions about the process and the related causes [ 5 ].
2. Six Sigma literature review
The primary methodology of Six Sigma is the application of DMAIC problem-solving steps. A brief explanation for each of the steps involved is as follows:
Define (D): The first stage focuses on analysis of customer identification, feedback, and requirements along with forming the project stakeholder team [ 6 ]. The project team looks at critical to quality (CTQ) and cost of quality (COQ) improvement projects that need to be addressed keeping the end goal of customer satisfaction in mind by defining project scope/problem statement and budget scheduling. It is very critical to define an accurate problem statement along with the scope to ensure the Six Sigma project team will invest all the time, skills, and resources in the right direction.
Measure (M ): This is the data collection phase where types of data, measurement scales, and sampling and collection methods are evaluated. All the initial metrics of the business case are established to measure the problem scale [ 2 , 7 ].
Analyze (A) : Measure and model relationships between variables, hypothesis testing, root cause analysis using cause and effect analysis tools such as fishbone/Ishikawa diagrams, 8D methodology, and 5 Whys analysis [ 2 , 7 ].
Improve (I) : Post root cause analysis, this phase tries to understand optimum levels of factors responsible for causing the problem via design of experiments (DOE), giving insights to determine corrective and preventative actions. This stage involves lean strategies such as the Kaizen Blitz, poka-yoke (mistake-proofing), cycle time reduction, etc. [ 7 , 8 , 9 ].
Control (C) : Primary objective in this last phase is to maintain and sustain control over the process and suggest improvement activities to minimize variation and defects. Statistical process control (SPC), total productive maintenance (TPM), and control plan development are some key tools used in the control phase [ 10 ].
3. Six Sigma application to defect reduction in semiconductor fabrication sites (FABS)
In this section, some business process areas have been identified to focus on benchmarking before considering Six Sigma project execution. Once these business process areas are well established, then only deploying Six Sigma teams would be beneficial to see tangible results. Motorola was one of the pioneers of Six Sigma methodology along with General Electric. Apart from Motorola, no other semiconductor manufacturing company has openly advocated the use of Six Sigma but has definitely inherited a lot of concepts and molded them into different terminologies. A big challenge to Six Sigma implementation is management support, and based on historical success rates of such projects initiated at their respective firms, the management decides to stick to their existing problem-solving methodologies or use some concepts from Six Sigma and other techniques used in industries such as aviation and automotive.
The most important goal for any semiconductor fab is to improve the final product yields [ 4 ]. Yield is directly correlated to contamination, design margin, process, and equipment errors along with fab operators [ 11 ]. Figure 1 referenced from Integrated Circuit Engineering Corp. shows the ranking of top yield loss causing problems across various fabs [ 4 ]. Six Sigma DMAIC methodology can be used as an effective quality and reliability management tool to solve most of these issues, and several literature papers in the form of case studies have been published regarding the same.

Figure 1.
Ranking of yield loss causing problems in fabs.
Sources of random defects could be the equipment, fab personnel, process margins, process chemicals and gases, or cleanroom itself. Data collected from Integrated Circuit Engineering Corp. (ICE) over the last three decades have been shown in Figure 2 .

Figure 2.
Bar chart showing wafer contamination source.
Human and cleanroom sources of contamination have been steadily declining due to advanced training in this field being developed over the years at various universities and corporations along with rapid strides in automation and artificial intelligence that have modernized clean rooms and minimized human contact in handling wafers. Engineers and upper-level management of these fabs must adopt a systematic methodology in resolving yield losses that occur due to process and equipment variations, and in this chapter, some basic business processes have been described that must exist in a fab for it to achieve maximum operational efficiency and produce high-quality chips. Six Sigma DMAIC methodology could then be used as and when required to improve these business processes.
3.1. Contamination and control protocols
Semiconductor processing involves several process fluids and gases, especially in lithography, film/metal deposition, etching, and cleaning steps. These fluids and gases contain impurity elements that can be dangerous to silicon devices [ 4 ]. These elements could be classified as the heavy metals, alkali metals, and light elements. Heavy metals such as Fe, Cu, Ni, Zn, Cr, Au, Hg, and Ag could result in wafer scraps and back end yield fallout due to corrosion in electroplating and metal deposition processing steps. Alkali metals such as Na, K, and Li and light elements like Al, Mg, Ca, C, S, Cl, and F could pose processing problems resulting in defects, which could be yield killing [ 4 ]. These elements also sometimes accumulate along the chambers, handlers, chucks, etc. of various equipment used in fabs resulting in tool downtime which greatly affects production schedules. Moreover, these elements could also pose safety problems to fab personnel. To understand potential risks and sources of these impure elements, a highly cross-functional FMEA team can be deployed to map out all the processes where source chemicals and gases are used along with identifying potential fail modes, severity, occurrence, and detection capabilities. The RPN exercise can be continued to drive improvements at each processing step where such impure elements are likely to occur.
3.2. Defect process mapping and yield management system
Defect density is defined as the total number of defects calculated per unit area on the wafer die [ 4 ]. In order to reduce defect density between processes, engineers need to identify the specific process steps, equipment, input materials, etc. that are the major contributors to the defect density. This involves the construction of a detailed process flow diagram for isolated segments of the process and the use of various problem-solving tools such as using the Six Sigma concepts, cause and effect diagrams, design of experiments, Pareto principles, etc. to tie the total defects measured at the end of the process sequence to the likely sources in the process flow. Most cutting-edge fabs have automated scripts using machine learning principles to have correlation between in-line defects and final yield loss. Advanced data mining software can quickly scan through very large data sets involving integrated circuit parameters, processing parameters, equipment parameters, probe bins, defect metrics, etc. to come up with various models which the Six Sigma team can use to infer meaningful results during the analyze phase of DMAIC. Thus, having a good defect management and yield monitoring system while benchmarking to industry leaders will enable semiconductor fabs to execute Six Sigma projects efficiently while maintaining a competitive edge in the market.
3.3. Role of SPC in defect monitoring
Due to the high number of processing steps and the possibility of defects forming from any source, fabs must have particle monitoring (PMON) charts which are effectively attribute charts that track defects per million opportunities (DPMO) on bare silicon wafers to plot particles coming from the equipment. This helps to isolate defect sources solely coming from process equipment and can be tied with regular total productive maintenance (TPM) cycles in the fab. Process engineering/metrology experts must be able to decide the sampling frequency in this case.
In-line defect metrology teams must be able to skillfully partition the line in placing SPC charts to control defect metrics based on historical learnings. Most fabs that do not have Six Sigma experts on their team usually have a hard time in determining the number of control charts and end up oversampling or undersampling. Attribute charts for key defect metrics that have downstream product yield impact or customer reliability issues should be given the highest priority in establishing control charts.
4. Case study
This section describes a case study wherein Six Sigma DMAIC methodology was used to tackle a probe yield issue due to an in-line defect contamination occurring in a lithography process step.
4.1. Phase 1: Define
This phase of the DMAIC methodology aims to define the scope and goals of the improved project in terms of customer requirements and to develop a process that delivers these requirements. The first step toward solving any problem in the Six Sigma methodology is by formulating a team of people associated with the process [ 12 ]. For the case study in discussion, suspect of in-line process step was not known, and only the critical business impacting factor of yield loss data was known. The initial team comprised of a Certified Six Sigma Black Belt (CSSBB) who were the site quality engineering manager, yield engineering managers, failure analysis engineers, and technicians along with defect metrology engineers. Roles of the team members are shown in the project charter ( Table 1 ). Next, the problem statement addressing CTQ and magnitude of the problem was identified. For a span of 13 weeks, one of the factory’s key products had been failing for bin fallout along the edges of the wafer resulting in a yield loss of 7% for dies. Failure analysis team was contacted to perform extensive cross-sectional analysis of the defect location. Based on the information available to the Six Sigma team at this stage, problem statement was defined as follows:
Table 1.
Project charter.
Problem Statement: Defects occurring on about 25% of wafers around the edge result in bin failure and die loss on specific technology node.
Here, the CTQ metric of die yield loss could directly be correlated to the number of such defects forming during in-line manufacturing that cause poor yields which results in delayed shipments and dissatisfied customers. The Six Sigma team’s next tasks will be outlined in the subsequent sections showing measurement, data analysis, root cause drill down, and corrective action implementation.
4.2. Phase 2: Measure
In this phase, data correlation was conducted to see the correlation between in-line defect counts per wafer to the number of failing dies per wafer at the final probe and percentage of edge die yield loss. Results are shown in Figure 4 .
It is evident from Figure 3 , that the correlation between CTQ metric edge die yield losses to the number of in-line defect counts occurring per wafer is linear with the high R 2 -adjusted value approaching close to 1. Six Sigma project team must reduce in-line defect count to less than 50 defects per wafer to have no die yield fallout at the final probe test.

Figure 3.
Regression plot of (a) number of failing die per wafer at final probe and (b) percentage edge die yield loss per wafer to number of defects per wafer in-line.
4.3. Phase 3: Analyze
The analysis phase consisted of searching through brainstorming rounds, the possible factors that may be affecting the electrical performance of the product. This stage of the Six Sigma process improvement methodology is often termed as Thought Process Mapping [ 13 ] wherein process experts and Six Sigma champions assimilate existing facts and data collected so far and look for initial trends and themes to find clues to go after. The factors that were considered most important were raised as hypotheses and tested by several statistical tests.
Wafer fabrication line is partitioned into three modules—front end of line (FEOL), middle of line (MOL), and back end of line (BEOL)—where each module involves complex steps such as lithography, thin-film depositions, etching, planarization, and diffusion. Inspection sampling plans are strategically placed across several processing steps within these three modules considering cost, cycle time, and wafer throughput times. In this case, the project team was interested to see if there was any in-line defect inspection step that could replicate the defect pattern shown on probe bin wafer map. The project team decided to inspect additional sample wafers through this step and perform failure analysis on the defect locations. More in-line inspection recipes were set up strategically right after metal patterning lithography processes to study defect formation and evolution as the wafers progressed through manufacturing steps.
Through in-line inspections set up across these modules, it was observed that the defect under study was first detected after the metal patterning process. Optical image of the defect was taken along with SEM analysis post BEOL to see defect evolution. This gave an initial indication to the Six Sigma team that metal patterning process and perhaps tool variation in lithography must be analyzed further. There was a need for lithography experts to now help the Six Sigma team in root cause analysis, so lithography process engineering manager, two process engineers, and two process technicians were added to the project team. The project charter was revised to include the new members into the stakeholder team.
Since there is a strong clue of the issue coming from the lithography process area, Six Sigma project team decided to add lithography process experts into the stakeholder team. The project team’s next objective was to brainstorm different failure modes that could be occurring with scanner tools in the lithography step and understand root causes from a scientific perspective. For this, the fishbone analysis was used to list several possible root causes across the six Ms—measurement, materials, method, mother nature/environment, manpower, and machine.
Different tools were used to validate the potential causes listed in the fishbone diagram, and a summary of the tools is listed in Table 2 .
Table 2.
Cause validation plan.
Gemba revealed that there was only one resist supplier to the patterning process, so supplier variation is not a root cause. SEM imaging did not reveal any polymer shearing defects. DOE carried out on resist coating process included a multilevel factorial design where coating speeds were varied from low to high to see if defects could be produced, but it was not the case. Fab contamination studies also showed that particles were well within control and environmental impacts to the formation of in-line defects were negligible. Careful review of all SOPs, manufacturing protocols, preventative maintenance log books, shift pass-downs, etc. did not confirm the validation of any cause. Majority of the effort was then spent in analyzing tool-to-tool variation, which was performed by ANOVA.
For confidentiality purposes, the exact supplier/tool names used in the factory are not mentioned in this chapter. There were three major tools in the factory running this product line, which will be addressed as Tools 1, 2, and 3 for analysis. One-way analysis of variation (ANOVA) of defect metric count with tool set was plotted (see Figure 4 ).

Figure 4.
One-way ANOVA for defect metric by tool set.
Tool 1 mean value was not only above the target defect count value of 50 but was also significantly above Tools 2 and 3, clearly indicating a problem with this tool.
4.3.1. Tool toggle statistical significance
Since there are multiple tool sets and data is non-normal, the Wilcoxon method is used for multiple tool set comparisons [ 14 ]. (α = 0.05). H o : Tool 1 toggle is statistically insignificant; H a : Tool 1 toggle is statistically significant. It is observed that p-value is less than 0.05 when Tool 1 is compared with other two tools as per Figure 5 .

Figure 5.
Nonparametric test data comparison.
Based on Wilcoxon test, reject H o and accept H a . Therefore, Tool 1 toggle is statistically significant and the toggle to defect metric is real. Next, the project team was tasked to look at SPC charts of all critical parameters of all three tools. It was found that the parameter scanner speed and exposure dosage of the immersion hood for Tool 1 were out of control (OOC) and mean value higher than Tools 2 and 3 as per the individuals and moving range (IMR) charts (see Figure 6 ).

Figure 6.
IMR chart comparison for scanner speed and exposure dosage by tool set.
Normal quantile plots for all three tools along with process capability index (Cpk) were calculated as shown in Figure 7 .

Figure 7.
(a) Normal quantile plots for scanner speed and (b) for exposure dosage across three tool sets.
SPC charts and normal quantile plots reveal a significant drift in parameter settings for Tool 1 compared to Tools 2 and 3. SPC charts show very tight distribution of points for Tools 2 and 3, but Tool 1 is not only out of spec but also has high amount of wafer-to-wafer variation for scanner speed and exposure dosage.
Out of all the possible failure modes evaluated and tested, it was confirmed that the source of defect could possibly be coming from Tool 1 metal pattern processing step in lithography area due to significant variation in scanner speed and exposure dosage. The theory behind defect formation due to inaccurate scanner speed and exposure dosage is described below which is a commonly observed phenomenon in the industry [ 14 ].
4.3.2. Defect formation theory
This defect is caused by a bubble forming in the scanner just prior to exposure. Light passing through the air bubble instead of the immersion water causes light refraction which creates a dipole. Attenuation of light can be seen outside of the ring as shown in Figure 8 [ 14 ].

Figure 8.
Light diffraction from bubble surface.
The system setup usually comprises a wafer placed on the scanner’s robotic arm which moves at speeds varying from 1100 to 1400 mm/s per the manufacturer as shown in Figure 9 . The space between wafer and immersion hoods (where the light source is located) is filled with water as an immersion fluid to increase image resolution by a fraction equal to the refractive index of the fluid [ 14 , 15 , 16 ].


Figure 9.
Schematic cross section of an immersion lithographic scanning device [ 17 ].
The scanner speed of the stage should be optimized to increase productivity without creating defects on the substrate by losing droplets [ 17 ]. Immersion liquid level could be a source of a bubble inclusion in the immersion space. Fluid behavior in the region of the recess may cause bubbles to form. This bubble may apply a heat load onto a surface onto which it lands, for example, the wafer surface resulting in poor lithographic imaging performance. If the exposure dosage inside the immersion hood is inadequate, bubbles could be entrapped inside the immersion fluid which is water in this case [ 14 , 15 , 16 , 17 ]. If the scanner moves too fast, the bubble extraction seal (BES) will not have adequate time to suck the bubble out resulting in the bubble being left on the wafer edge. BES extracts the bubbles between the scanner and wafer table.
The Six Sigma project team focused on fixing two main issues here contributing to defect formation—(1) inadequate exposure dosage resulting in air bubble entrapment inside the immersion hood and (2) inadequate scanner speed not giving enough time for BES to suck the bubbles out. Tool 1 settings have drifted significantly from other two tools and has a strong correlation to the high defect counts resulting in yield loss. The next step for the Six Sigma team is to identify optimal tool settings to minimize defect count and have all the three tools operating at these settings. This is discussed in the next phase of Improve.
4.4. Phase 4: Improve
In this phase, optimal tool settings for scanner speed and exposure dosage will be derived using the design of experiments (DOE) full factorial design. Corrective actions addressing the root cause will also be discussed in this section.
Output response is the minimum defect count, while the input factors are scanner speed and tool exposure dosage. Scanner speed values can range from 1000 mm/s to 1400 mm/s per supplier, while the exposure dosage range is from 90 to 100 nC/cm. 16 run DOE table in JMP was created and resulted in prediction profiler obtained after the defect count values for all 16 runs were recorded as shown in Figure 10 . Using the maximum desirability function in JMP, optimum settings were found to be the scanner speed, 1178 mm/s, and exposure dosage, 94.2 nC/cm.

Figure 10.
Prediction profiler indicating optimum tool settings for maximum desirability.
Based on the above findings for scanner speed and exposure dosage, appropriate interim and long-term corrective actions were proposed and implemented.
4.5. Phase 5: Control
This is the final phase of the Six Sigma DMAIC methodology. Some of the questions that arise after the four phases of problem-solving methodology are as follows: How can one control and monitor the tool parameter settings in line? How can one monitor the in-line defect rate at multiple steps? The simple answer is via statistical process control (SPC) charts. Actual SPC charts on proprietary software have not been shown, but data was exported to JMP has been shown in Figure 11(a) and (b) post optimal setting discovery and corrective action implementation across all three tools.

Figure 11.
(a) Individual chart for scanner speed and (b) individual chart for exposure dosage.
It is observed that all three tools have mean values for both the critical parameters very close to the desired settings (1178 mm/s for scanner speed and 94.2 nC/cm for dosage) derived using DOE and have much tighter process control with occasional OOC points which will be addressed real time via the corrective actions implemented. To check process capability post improvements, capability analysis was plotted in JMP ( Figure 12(a) and (b) ).

Figure 12.
(a) Process capability analysis for scanner speed and (b) process capability analysis for exposure dosage for Tool 1.
Binomial chart plots and Cpk values being >1.33 (1.88 for scanner speed and 2.7 for exposure dosage) clearly indicate high process capability and stability for the lithography metal patterning process post-tool setting optimization.
4.5.1. Process FMEA and control plan development
Six Sigma project team decided to implement FMEA process and has appropriate corrective actions in place as part of control plan. Design-related failure modes and improvements were escalated to the tool manufacturer, while the fab focused on process FMEA for the metal patterning process. Figure 13 shows the FMEA template for one of the processing steps in the patterning stage (wafer cleaning) which can be used across FEOL, MOL, and BEOL modules. Most of the corrective actions were implemented over 8 weeks, while some of them were part of continuous improvement activities. This FMEA table should be carried out for other subprocess steps involved in the metal patterning process such as resist application, BARC, etc.

Figure 13.
PFMEA snapshot for wafer cleaning in patterning process.
Clearly, the new tool settings have greatly reduced the number of in-line defects occurring post-pattern processing steps. The line was then released to run production lots after 8 weeks of improve and control phase actions. This concludes the final step of the DMAIC methodology. The final conclusions and tangible improvement results are discussed in the next section.
5. Conclusions
Post DMAIC, as the mean defect count was continuously controlled and monitored below 50, the final probe yield test data also showed a significant reduction in die yield loss to almost 0% (100% yield) post-DMAIC implementation ( Figure 14 ).

Figure 14.
Edge die yield loss graph over 26-week time frame.
It can thus be concluded that DMAIC methodology properly executed under an experienced Six Sigma project team with the support of management is a powerful tool that can reduce process variations and improve product yields, eliminating waste and improving customer satisfaction which ultimately has a significant financial impact on the organization. Six Sigma can be used widely in semiconductor manufacturing environments where there is a tremendous need for defect reduction and tighter process control as the industry advances to smaller technology nodes.
Special note
The author would like to inform the readers that data represented in this paper are not the actual values and closely approximated values have been reported to give an understanding of data analysis, interpretation and application of six sigma methodology.
- 1. Cavanagh RR, Neuman RP, Pande PS. The Six Sigma Way. New York: McGraw-Hill; 2014
- 2. Quality Council of Indiana. CSSBB Primer. West Terre Haute, Ind. Quality Council of Indiana; Vol. 42010. 2014. pp. 125-134
- 3. Gutiérrez H, y De la Vara R. Control Estadístico de Calidad y Seis Sigma. México, D.F: Mc Graw Hill Interamericana Editores, S.A. de C.V; 2004
- 4. Yield and Yield Management. Integrated Circuit Engineering Corp (ICE). Retrieved from http://smithsonianchips.si.edu/ice/cd/CEICM/SECTION3.pdf
- 5. Gijo EV, Scariab J, Antony J. Application of Six Sigma methodology to reduce defects of a grinding process. Quality and Reliability Engineering International. 2011; 27 :1235-1237
- 6. ISO/TS 16949:2009. Quality Management Systems. Geneva: ISO; 2008
- 7. George ML. Lean Six Sigma. New York: McGraw-Hill; 2002
- 8. Breyfogle III. Implementing Six Sigma. 2nd ed. New York: John Wiley & Sons; 2003
- 9. Nakajima S. Introduction to TPM: Total Productive Maintenance. Cambridge, MA: Productivity Press; 1998
- 10. Wise S, Fair D. The Control Chart Dilemma. ASQ, Knoxville, Tennessee, USA: Quality Progress; 1998
- 11. Stapper CH, Rosner RJ. Integrated circuit yield management and yield analysis: Development and implementation. IEEE TSM. 1995; 8 :95-112
- 12. Banuelas R, Antony J, Brace M. An application of Six Sigma to reduce waste. Quality and Reliability Engineering International. 2005; 21 :553
- 13. DeLayne Stroud J. iSixSigma. 2018. Retrieved from https://www.isixsigma.com/implementation/project-selection-tracking/use-thought-map-increase-efficiency/
- 14. Western Electric. Statistical Quality Control Handbook. Indianapolis, IN: AT&T; 1996
- 15. Wei Y. Bubble and Antibubble Defects in 193i Lithography. Bellingham, Washington, USA: SPIE; 2008
- 16. ASML. US Patent (US7199858). Lithographic Apparatus and Device Manufacturing Method. 2007
- 17. Riepen M. Contact Line Dynamics in Immersion Lithography. Netherlands: ASML Research
© 2019 The Author(s). Licensee IntechOpen. This chapter is distributed under the terms of the Creative Commons Attribution 3.0 License , which permits unrestricted use, distribution, and reproduction in any medium, provided the original work is properly cited.
Continue reading from the same book
Edited by Ancuta Carmen Păcurar
Published: 03 January 2019
By Martha Roselia Contreras Valenzuela, Alejandro Dav...
1125 downloads
By Jonathan Becedas and Andrés Caparrós
1529 downloads
By Ruta Miniotaite
1184 downloads

Top Six Sigma Case Study 2024
Home Blog Quality Top Six Sigma Case Study 2024

Six Sigma is an array of methods and resources for enhancing corporate operations. When Bill Smith was an engineer at Motorola, he introduced it in 1986 to find and eliminate mistakes and defects, reduce variance, and improve quality and efficiency. Six Sigma was first used in manufacturing as a quality control tool. When long-term defect levels are less than 3.4 defects per million opportunities ( DPMO ), Six Sigma quality is reached.
Six Sigma case study offers a glimpse into how various companies have harnessed the five distinct phases: defining, measuring, analyzing, improving, and controlling, principles of Six Sigma to overcome challenges, streamline processes, and improve across diverse industries.

What Are Six Sigma Case Studies, and Why Are They Important?
Six Sigma case studies examples show how Six Sigma techniques have been used in businesses to solve issues or enhance operations. For practitioners and companies pondering enforcing Six Sigma concepts, these case studies are an invaluable resource to learn the advantages and efficacy of Six Sigma adoption.
Here are the reasons why six sigma case study is important:
Success Illustration: Case studies demonstrate how Six Sigma projects generate tangible advantages like better productivity, fewer defects, and more customer satisfaction while providing unambiguous evidence of their efficacy.
Learning Opportunities: They deliver vital insights to use Six Sigma tools and processes realistically and allow others to learn from successful approaches and avoid common errors.
ROI Demonstration: Case studies provide quantitative data to show the return on investment from Six Sigma projects, which helps justify resources and get support for future initiatives.
Promoting Adoption: They cultivate a continuous improvement culture and show how Six Sigma concepts can be used in different situations and sectors, which encourages other businesses to embrace the methodology.
Become a Six Sigma Certified Professional and lead process improvement teams to success. Learn how to streamline processes and drive organizational growth in any industry. Join our Lean 6 Sigma training courses and transform your career trajectory with valuable skills and industry recognition.
Six Sigma Case Studies
Let us discuss some real-world case study on six sigma examples of successful Six Sigma undertakings through case studies:
1. Six Sigma Success: Catalent Pharma Solutions
Do you know how Six Sigma techniques turned things around for Catalent Pharma Solutions?
Six Sigma methodologies, initially presented by Motorola in 1986 and prominently used by General Electric during CEO Jack Welch's leadership, are essential for enhancing customer contentment via defect minimization. Catalent Pharma Solutions, a top pharmaceutical development business, employed Six Sigma to address high mistake rates in its Zydis product line. By applying statistical analysis and automation, training employees to various belt levels, and implementing Six Sigma procedures, Catalent was able to maintain product batches and boost production. This case study illustrates how Six Sigma approaches are beneficial for businesses across all industries as they can improve processes, prevent losses, and aid in cost reduction.
2. TDLR's Record Management: A Six Sigma Success Story
The Texas Department of Licensing and Regulation (TDLR) faced escalating costs due to the storage of records, prompting a Six Sigma initiative led by Alaric Robertson. By implementing Six Sigma methodologies, process mapping, and systematic review, TDLR successfully reduced storage costs and streamlined record management processes. With a team effort and strategic changes, TDLR has achieved significant cost savings and improved efficiency. The project also led to the establishment of a robust records management department within TDLR.
3. Six Sigma Environmental Success: Baxter Manufacturing
Baxter Manufacturing utilized Six Sigma principles to enhance its environmental performance and aim for greater efficiency. Through the implementation of Lean manufacturing and accurate data collection, Baxter reduced waste generation while doubling revenue and maintaining waste levels. With a cross-functional team trained in Six Sigma, the company achieved significant water and cost savings without major investments in technology. It led to promotions for team leaders and showcased the effectiveness of Six Sigma in improving environmental sustainability.
4. Aerospace Manufacturer Boosts Efficiency With Six Sigma
Have you heard about how Six Sigma principles transformed an aerospace parts manufacturer? Here is the 6 Sigma case study for aerospace parts manufacturer
A small aerospace parts manufacturer used Six Sigma to cut machining cycle time, reducing costs. Key engineers obtained Six Sigma certification and led the project, involving management and operators. Using DMAIC, they analyzed data, identified root causes, and implemented lean solutions. The process yielded a 46% reduction in cycle time and an 80% decrease in variation, enhanced productivity and profitability. The case highlights how Six Sigma principles can benefit businesses of all sizes and emphasizes the importance of training for successful implementation.
Enroll in the Lean Six Sigma Green Belt certification online training to advance your career! Gain expertise in process improvement and organizational transformation with expert-led training and real-world case studies. Start now to become a certified professional in quality management.
5. Ford Motors: Driving Success
This is a case study on Six Sigma i ncorporated by Ford Motors to streamline processes, improve quality, significantly reduce costs, and reduce environmental impact. Initially met with skepticism, Ford's implementation overcame challenges, achieving remarkable results: $2.19 billion in waste reduction, $1 billion in savings, and a five-point increase in customer satisfaction. Ford's Consumer-driven Six Sigma initiative set a benchmark in the automotive industry and proved the efficacy of data-driven problem-solving. Despite obstacles, Ford's Six Sigma exemplifies transformative success in process improvement and customer satisfaction enhancement.
6. 3M's Pollution Prevention Six Sigma Success
Have you checked out how 3M tackled pollution with Six Sigma? It's pretty remarkable. 3M leveraged Six Sigma to pioneer pollution prevention, saving $1 billion and averting 2.6 million pounds of pollutants over 31 years. With 55,000 employees trained and 45,000 Lean Six Sigma projects completed, they focused on waste reduction and energy efficiency. Results included a 61% decrease in volatile air emissions and a 64% reduction in EPA Toxic Release Inventory. Surpassing goals, they doubled Pollution Prevention Pays projects and showcased Six Sigma's prowess in cost-saving measures.
7. Microsoft Sigma Story Lean Six Sigma
By using Lean Six Sigma case studies, Microsoft increased customer interactions and profitability through waste removal and process optimization. They concentrated on improving the quality of the current process and reducing problems by utilizing the DMAIC technique. Eight areas were the focus of waste elimination: motion, inventory, non-value-added procedures, waiting periods, overproduction, defects, and underutilized staff talent. Microsoft streamlined processes and encouraged innovation, which allowed them to maintain productivity and client satisfaction even as technology changed.
8. Xerox's Lean Six Sigma Success Story Six Sigma
It is another important case study of the Six Sigma project. When Xerox implemented Lean Six Sigma in 2003, the organization underwent a significant transformation. They reduced variance and eliminated waste as they painstakingly optimized internal operations. It improved their operational effectiveness and raised the caliber of their goods and services. Through extensive training programs for staff members, Xerox enabled its employees to spearhead projects aimed at improving different departments and functions. The organization saw significant improvements in customer satisfaction and service performance.
9. A Green Belt Project Six Sigma Case Study
It is one of the best examples of a Six Sigma case study. Anne Cesarone's Green Belt project successfully reduced router configuration time by 16 minutes, a remarkable 55% improvement. Anne maintained router inventory, made improvements to documentation and configuration files, and started router requests sooner by resolving last-minute requests and setup mistakes. The initiative resulted in less router programming time from 29 to 13 minutes, an increase in router order lead time of 11 days, and a 60% drop in incorrect configurations. These raised customer happiness and increased operational effectiveness while proving the benefits of process improvement initiatives.
10. Improving Street Maintenance Payments with Lean Six Sigma
Jessica Shirley-Saenz, a Black Belt at the City of San Antonio, used Lean Six Sigma to address delays in street maintenance payments Lean Six Sigma case study examples. Contractors were experiencing extended payment times, risking project delays and city infrastructure integrity. Root causes included payment rejections and delayed invoicing. By implementing quantity tolerance thresholds, centralizing documentation processes, and updating payment workflows, monthly payment requests increased from 97 to 116. Rejected payments decreased from 17 to 12, reducing the rejection percentage from 58% to 42%, saving $6.6 million.
Six Sigma's effectiveness spans industries, from healthcare to technology. Case studies demonstrate its ability to optimize processes and improve outcomes. From healthcare facilities streamlining patient care to tech companies enhancing software development, Six Sigma offers adaptable solutions for diverse challenges. These real-world examples illustrate how its methodologies drive efficiency, quality, and customer satisfaction. Professionals can learn valuable lessons from using Six Sigma in healthcare studies, identify strategies to overcome obstacles and facilitate continuous improvement. Organizations can emulate best practices and implement similar initiatives to achieve measurable results by studying successful implementations.
Ready to enhance your skills and advance your career with Six Sigma certification? Join our comprehensive KnowledgeHut's best lean Six Sigma courses to master Six Sigma principles and methodologies. Become a sought-after professional in IT, Manufacturing, Healthcare, Finance, and more industries. Enroll now to accelerate your career growth!
Frequently Asked Questions (FAQs)
Six Sigma case studies are available in various formats and places, such as books, academic journals, professional publications, and Internet sites. Many companies that have effectively adopted Six Sigma publish their case studies on their websites or at industry exhibitions and conferences.
Six Sigma case studies provide insightful information on how businesses have addressed certain issues, enhanced procedures, and produced noticeable outcomes. Professionals gain knowledge about best practices, prevalent errors to avoid, and creative problem-solving methods in several industries and circumstances.
Professionals can share their Six Sigma case studies through industry forums, professional networking platforms, blogs, and social media. They can submit their case studies to publications or at conferences and workshops to reach a wider audience within the Six Sigma community.

Shivender Sharma
Shivendra Sharma, an accomplished author of the international bestseller 'Being Yogi,' is a multifaceted professional. With an MBA in HR and a Lean Six Sigma Master Black Belt, he boasts 15 years of experience in business and digital transformation, strategy consulting, and process improvement. As a member of the Technical Committee of the International Association of Six Sigma Certification (IASSC), he has led multi-million dollar savings through organization-wide transformation projects. Shivendra's expertise lies in deploying Lean and Six Sigma tools across global stakeholders in EMEA, North America, and APAC, achieving remarkable business results.
Avail your free 1:1 mentorship session.
Something went wrong
Upcoming Quality Management Batches & Dates

Take advantage of your time, International Six Sigma Inc. offers both Instructor-led Live Virtual classes as well as Online Self-Paced training . Enroll Today!
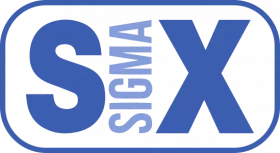
Case Studies
Get a complete insight to our projects. Learn on what problems we faced, what were the parameters, and how we weaved the way to business success. Have a look at our case studies.
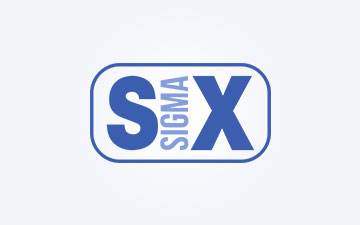
Demonstration of Six Sigma Principles through Catalent Pharma Solutions
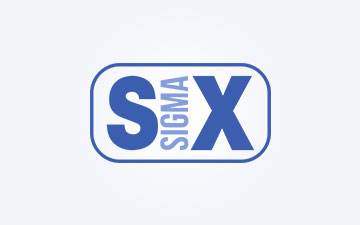
Case Study: Lean Six Sigma Improves Environment – Baxter Manufacturing
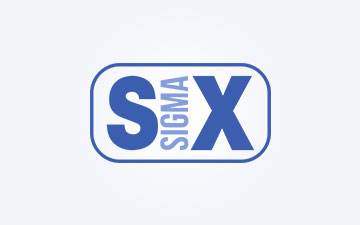
Case Study: Lean 6 Sigma in Aerospace Manufacturer Streamlines Processes & Improves Cycle Time
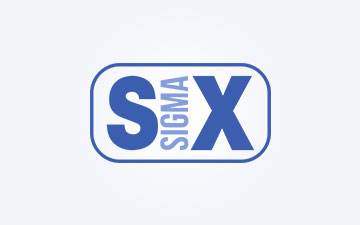
3M Six Sigma Case Study – Pollution Prevention – 6sigma
At home or workplace, we have you covered
Training Options
More than 500 locations
Classroom Training
Learn anytime, anywhere
Online Training
Live instructor-led training
Webinar Training
Customized training programs
On-site Training
Accelerated training programs
Blended Training
For streamlined business plans
Operational Excellence
Exclusive consulting services
Consulting Services
Fully customized programs
Group/Corporate Training
International six sigma inc. accreditation & affiliations.
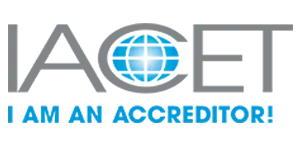
Email: [email protected] Toll Free in the US: (866) 409-1363
Courses / Training
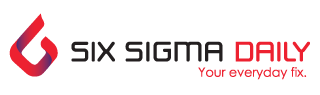
Case Study: Portuguese Tire Manufacturer Saves Thousands Using Six Sigma’s DMAIC Methodology
A tire manufacturing company in Portugal has provided an excellent study in implementing Six Sigma and how it can impact business performance.
Six Sigma already has proven its value in the automobile industry . Companies including Ford and Toyota have made the methodology a key component of their success.
A recent study of implementation of Six Sigma at Continental Mabor, a tire manufacturing company located in Famalicao, Portugal, provides a step-by-step look at putting Six Sigma’s DMAIC methodology into place.
The study, published at the 2017 Manufacturing Engineering Society International Conference, was written by F.J.G. Silva, a professor in the school of engineering at Polytechnic of Porto, Portugal. It reported that the use of Six Sigma focused on improving the rubber extrusion process of two tire products: the tread and the sidewall. The primary goal was reduction of wasted material in the process.
Continental Mabor instituted Six Sigma because tire manufacturing is an intensely competitive business around the globe and “continuous flexibility and adaptation” is necessary, Silva wrote, adding that to achieve success, “it is crucial to seek operational excellence.”
Here is an overview of how Continental Mabor approached implementing the Six Sigma methodology of DMAIC , which stands for define, measure, analyze, improve and control.
Doing The Research
Continental Mabor started its Six Sigma journey by researching books and published scientific articles on Six Sigma methodology,
The company focused on improvements in the rubber extrusion process, particularly the mixing, preparation and construction departments. The mixing department receives raw materials that are transformed into compound sheets that are used in the preparation department on seven extrusion lines which focus on tread and sidewall extrusion. The ultimate “customer” for the extrusion process is the construction department.
The amount of material generated in the process – which is later reused for other purposes – is one of the indicators for the company on how efficient the operation is running. The focus is to limit the amount of extra material generated during the tread and sidewall extrusion process, called “work off.”
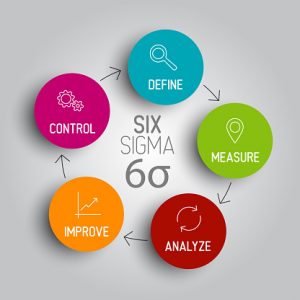
To accurately define the problem areas in the process, the company drew up a project charter that identifies problems, establishes objectives and defines the scope of the project (including the employee teams involved). A project charter also:
- Establishes the business case for how the project will impact overall organizational strategy
- Clearly measures the impact on the business of the current problem and measures the gap between where things are and the desired state
- Creates a clear scope for the project with identification of the areas where teams will focus to prevent “scope creep” – moving into areas outside the defined perimeter of the project
To create the charter, the company used a Gantt chart, a horizontal chart that maps out a product schedule. They also used a SIPOC diagram to plot the extrusion process in greater detail. SIPOC stands for supplier, inputs, process, outputs and customer. A SIPOC is a way to see an entire process in one graph and see the relationship between inputs and suppliers and the output for customers.
To get a handle on the current state of the extrusion process, Continental Mabor leaders then created a data collection plan . This included measuring the amount of rejected material during the extrusion process. Data was collected for 30 weeks, with 10 three-hour trials conducted each week. After this period of measurement, the company could determine the percentage of unused work off material generated in the tread and sidewall extrusion processes.
With the amount of data collected, the focus then turned to finding the root causes of the defects in the process that caused variation in the amount of materials wasted. The company used a Ishikawa diagram to find the cause and effect relationship between various activities and inputs into the process and the problem of generating unused material. They then used a Pareto chart to prioritize which potential causes seemed to have the most unfavorable impact.
They discovered that one machine in the sidewall extrusion process was not performing as well as others, leading to a significant increase in extra material. In the tread extrusion process, they discovered that the method for feeding the machines was creating problems with machine stoppage and jamming.
In this phase, a list was made of all the problems and root causes, then the subsequent action taken to improve these issues. These include changes to the machinery itself and changes in the methods used by employees to feed material into the machine.
With the improvements in place, data was then collected on the changes in the process. In this case, they were very significant. The company reduced the amount of work off material by five tons per day. After factoring in the cost of improvements to the machinery, the positive impact to the company’s bottom line was $165,000 euros per year, which translates to a little more than $200,000 U.S. dollars.
In his conclusion on the process improvement at Continental Mabor, Silva wrote that “the use of Six Sigma methodology played a decisive role in the achievement of the proposed goal, ensuring that there was a systematic and disciplined approach to the issues at hand through the DMAIC cycle.”
It also provides an excellent step-by-step education in how to implement Six Sigma successfully.
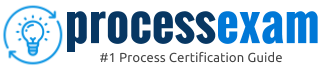
- Foundation Level (CTFL)
- Foundation Level - Agile Tester (CTFL-AT)
- Advanced Level - Agile Technical Tester (CTAL-ATT)
- Advanced Level - Technical Test Analyst (CTAL-TTA)
- Advanced Level - Test Analyst (CTAL-TA)
- Advanced Level - Test Manager (CTAL-TM)
- Certified Tester - Mobile Application Testing (CT-MAT)
- Certified Tester - AI Testing (CT-AI)
- Certified Tester - Model-Based Tester (CT-MBT)
- Expert Level - Test Management (CTEL-TM)
- Certified Tester Gambling Industry Tester (CT-GT)
- ISTQB Certified Tester Acceptance Testing (CT-AcT)
- ISTQB Certified Tester Agile Test Leadership at Scale (CT-ATLaS)
- ISTQB Certified Tester Automotive Software Tester (CT-AuT)
- ISTQB Certified Tester Game Testing (CT-GaMe)
- ISTQB Certified Tester Performance Testing (CT-PT)
- ISTQB Certified Tester Security Tester (CT-SEC)
- ISTQB Certified Tester Test Automation Engineer (CT-TAE)
- ISTQB Certified Tester Usability Testing (CT-UT)
- Certified Six Sigma Black Belt (CSSBB)
- Certified Six Sigma Green Belt (CSSGB)
- Certified Six Sigma Yellow Belt (CSSYB)
- Certified Quality Improvement Associate (CQIA)
- Certified Quality Auditor (CQA)
- Certified Quality Engineer (CQE)
- ASQ Certified Manager of Quality/Organizational Excellence (CMQ/OE)
- Certified Software Quality Engineer (CSQE)
- Certified Reliability Engineer (CRE)
- Certified Quality Inspector (CQI)
- Certified Quality Technician (CQT)
- ASQ Certified Master Black Belt (CMBB)
- ASQ Certified Quality Process Analyst (CQPA)
- ASQ Certified Supplier Quality Professional (CSQP)
- Certified Calibration Technician (CCT)
- Certified Food Safety and Quality Auditor (CFSQA)
- Certified Medical Device Auditor (CMDA)
- Certified Pharmaceutical GMP Professional (CPGP)
- ASTQB Certified Mobile Tester (ASTQB-MT)
- SHRM Certified Professional (SHRM-CP)
- SHRM Senior Certified Professional (SHRM-SCP)
- Certified System Administrator (CSA)
- Certified Application Specialist - Performance Analytics (CAS-PA)
- Certified Implementation Specialist - Software Asset Management (CIS-SAM)
- Certified Implementation Specialist - Event Management (CIS-EM)
- Certified Implementation Specialist - IT Service Management (CIS-ITSM)
- Certified Implementation Specialist - Discovery (CIS-Discovery)
- Certified Implementation Specialist - Human Resources (CIS-HR)
- Certified Implementation Specialist - Vulnerability Response (CIS-VR)
- Certified Implementation Specialist - Project Portfolio Management (CIS-PPM)
- Certified Implementation Specialist - Customer Service Management (CIS-CSM)
- Certified Implementation Specialist - Service Mapping (CIS-SM)
- Certified Implementation Specialist - Security Incident Response (CIS-SIR)
- Certified Implementation Specialist - Risk and Compliance (CIS-RC)
- Certified Implementation Specialist - Hardware Asset Management (CIS-HAM)
- ServiceNow Certified Implementation Specialist - Cloud Provisioning and Governance (CIS-CPG)
- ServiceNow Certified Implementation Specialist - Field Service Management (CIS-FSM)
- ServiceNow Certified Implementation Specialist - Service Provider (CIS-SP)
- ServiceNow Certified Implementation Specialist - Third-party Risk Management (CIS-TPRM)
- ServiceNow Certified Implementation Specialist - Vendor Risk Management (CIS-VRM)
- OMG Certified UML Professional 2 (OCUP 2) - Foundation Level
- OMG Certified Expert in BPM 2 (OCEB 2) - Fundamental Level
- OMG Certified UML Professional 2 (OCUP 2) - Intermediate
- OMG Certified UML Professional 2 (OCUP 2) - Advanced Level
- OMG-Certified Systems Modeling Professional - Model User
- PRINCE2 2017 Foundation
- ITIL Foundation
- PeopleCert Certified Software Tester (CSTE)
- PeopleCert Certified Software Business Analyst (CSBA)
- Professional Scrum Master I (PSM I)
- Professional Scrum Master II (PSM II)
- Professional Scrum Product Owner I (PSPO I)
- Professional Scrum Product Owner II (PSPO II)
- Professional Scrum Developer (PSD I)
- Professional Agile Leadership (PAL I)
- Professional Agile Leadership - Evidence Based Management (PAL-EBM)
- Scaled Professional Scrum (SPS)
- Professional Scrum with Kanban level I (PSK I)
- Professional Scrum with User Experience (PSU I)
- Professional Scrum Master III (PSM III)
- Professional Scrum Product Owner III (PSPO III)
- ITIL 4 Foundation
- ITIL Practitioner
- PRINCE2 Foundation
- PRINCE2 Practitioner
- PRINCE2 Agile Foundation
- APICS Certified Supply Chain Professional (CSCP)
- APICS Certified in Logistics, Transportation and Distribution (CLTD)
- APICS Certified in Transformation for Supply Chain (CTSC)
- Certified in Planning and Inventory Management (CPIM P1)
- Certified in Planning and Inventory Management (CPIM P2)
- LSSA Certified Lean Six Sigma Black Belt (LSSA-BB)
- LSSA Certified Lean Six Sigma Green Belt (LSSA-GB)
- LSSA Certified Lean Six Sigma Yellow Belt (LSSA-YB)
- Certified Business Analysis Professional (CBAP)
- Certification of Capability in Business Analysis (CCBA)
- Entry Certificate in Business Analysis (ECBA)
- IIBA Agile Analysis Certification (AAC)
- IIBA Business Data Analytics (CBDA)
- IIBA Certificate in Cybersecurity Analysis (CCA)
- IIBA Certificate in Product Ownership Analysis (CPOA)
- Lean Six Sigma Black Belt - Foundation
- Lean Six Sigma Green Belt - Foundation
- Lean Six Sigma Yellow Belt - Foundation
- International Change Management - Foundation
- Agile Project Management - Foundation
- SAFe Advanced Scrum Master (SASM)
- SAFe Agile Product Management (APM)
- SAFe Agile Software Engineer (ASE)
- SAFe Agilist (SA)
- SAFe DevOps Practitioner (SDP)
- SAFe Government Practitioner (SGP)
- SAFe Lean Portfolio Manager (LPM)
- SAFe Practitioner (SP)
- SAFe Product Owner/Product Manager (POPM)
- SAFe Program Consultant (SPC)
- SAFe Release Train Engineer (RTE)
- SAFe Scrum Master (SSM)
- SAFe for Architects (ARCH)
- APMP Foundation-Level Certification (Foundation)
- DevOps Foundation (DOFD)
- USGBC LEED Accredited Professional Building Design and Construction (AP BD+C)
- USGBC LEED Accredited Professional Homes (AP Homes)
- USGBC LEED Accredited Professional Neighborhood Development (AP ND)
- USGBC LEED Green Associate
- IASSC Certified Lean Six Sigma Black Belt (ICBB)
- IASSC Certified Lean Six Sigma Green Belt (ICGB)
- IASSC Certified Lean Six Sigma Yellow Belt (ICYB)
- Certified Associate in Project Management (CAPM)
- Project Management Professional (PMP)
- PMI Professional in Business Analysis (PMI-PBA)
- Program Management Professional (PgMP)
- Portfolio Management Professional (PfMP)
- PMI Risk Management Professional (PMI-RMP)
- PMI Scheduling Professional (PMI-SP)
- PMI Agile Certified Practitioner (PMI-ACP)
- PMI Construction Professional (PMI-CP)
- PMI Disciplined Agile Coach (DAC)
- PMI Disciplined Agile Scrum Master (DASM)
- PMI Disciplined Agile Value Stream Consultant (DAVSC)
- Associate Professional in Human Resources (aPHR)
- Professional in Human Resources (PHR)
- Senior Professional in Human Resources (SPHR)
- Global Professional in Human Resources (GPHR)
- Associate Professional in Human Resources - International (aPHRi)
- Professional in Human Resources - California (PHRca)
- Professional in Human Resources - International (PHRi)
- Senior Professional in Human Resources - International (SPHRi)
- Certified Pega System Architect (CPSA)
- Certified Pega Senior System Architect (CPSSA)
- Certified Pega Robotics System Architect (CPRSA)
- Certified Pega Business Architect (CPBA)
- Certified Pega Decisioning Consultant (CPDC)
- Certified Pega Data Scientist (CPDS)
- Certified Pega Lead System Architect (CPLSA)
- TOGAF 9 Foundation - Level 1 (OG0-091)
- TOGAF 9 Certified - Level 2 (OG0-092)
- TOGAF 9 Combined (OG0-093)
- ArchiMate 3 Foundation (OGA-031)
- TOGAF Business Architecture Part 1 (OGB-001)
- TOGAF Enterprise Architecture Part 1 (OGEA-101)
- TOGAF Enterprise Architecture Part 2 (OGEA-102)
Six Sigma - Manufacturing Case Study
How to reduce labor expenses using six sigma in manufacturing industry.
In any industry, when production increases above planned for levels whether due to sudden increased demand or unanticipated constraints in the system contract labor may be brought in as a quick fix. While this makes available some flexibility in operations, increased labor costs is a problem.
Six Sigma techniques can be used to reduce these enhanced labor costs and the attending productivity concerns. Implementation of cost accounting to these productivity enhancements.
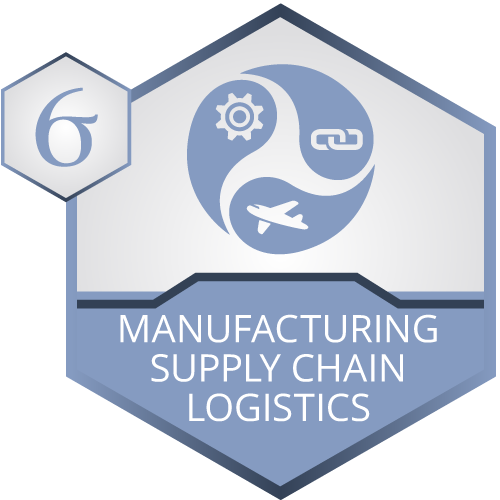
Case Background
Four years back a Flavors and Food Additives Company in Asia, Westgate, had a swell in production in one of its product lines (liquids) due to the transfer of production from an associate and, at the same time, an increase in sales. Because of the strict labor laws and strong union power in the Asian country, the company was unenthusiastic to hire additional permanent personnel as that could lead to over-employment within its workforce. Requesting employees to work overtime also held little appeal, due to several penalties related to overtime imposed by the government.
Westgate chose to go with a third option – hiring casual (i.e., temporary) labor to handle additional production loads when needed. The casual labor market in this location is provided by one third-party service provider that has long had a good relationship with Westgate. This practice was supposed to help maintain the cost-effective use of casual labor by Westgate. In its place, Westgate experienced an unreasonably high increase in casual labor cost.
Manufacturing Leverage Index
This index is calculated by subtracting the labor cost percent change from the production change percent change. For example, if production increases 20 percent and casual labor costs increase 40 percent during the same period, the index is -20 percent. Ideally, the percent production change should be higher than the labor percent change; the goal is for the index to be a positive number and reflect “positive leverage.”
In March 2012, the finance and operation teams of Westgate were asked to control their casual labor costs. As part of this struggle, the company initiated using a manufacturing leverage index as a key performance indicator (KPI); this index compared the casual labor costs to the growth in production volume. After four months, the KPI indicated there was no important progress in the relationship between casual labor and production. In a June meeting with internal and external shareholders to address that disconnect, Westgate chose to use the continuous improvement methodology of Six Sigma to reduce the costs of casual labor.
A process map of the current lay- out (Figure 1) shown that the manpower requirement was based on the product order receipt; any gap was handled by added casual labor. In theory, any rise in casual labor should match a corresponding increase in production volume. High casual labor costs is not a matter of question in and of itself. When the casual labor cost increase is higher than the production volume increase, however, it becomes a problematic condition, as signified by a negative manufacturing leverage index.
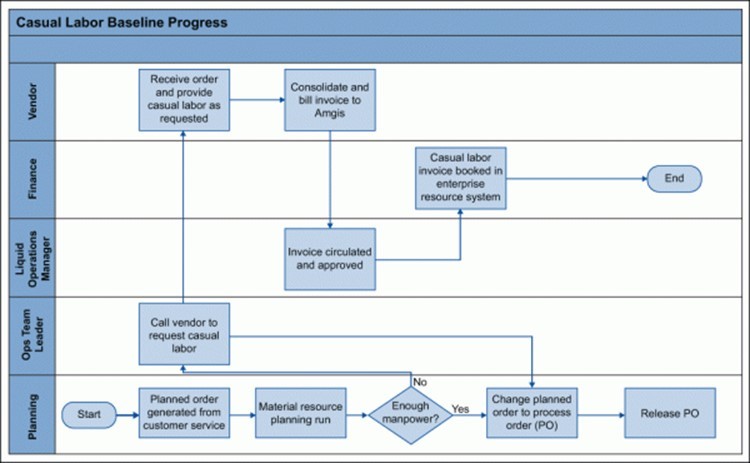
For the duration of the first six months of 2012, contract hours (and costs) improved while volume remained constant or decreased compared to 2011, as shown in Figure 2. A cause-and-effect analysis was concluded in July to understand better the various factors involved in this labor and production relationship.
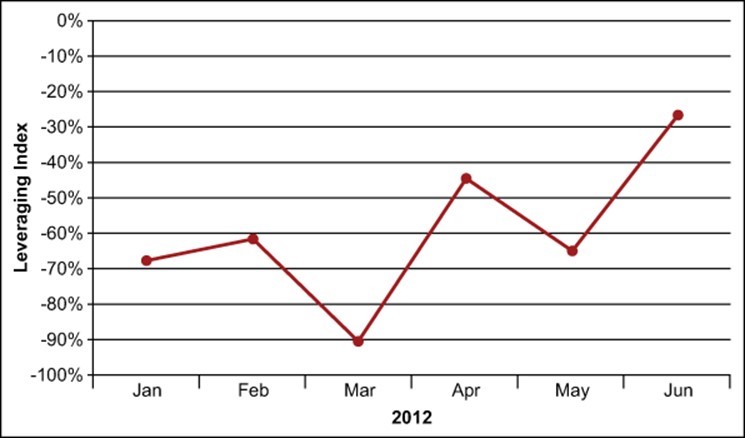
Well, ahead consultations with members of the operations team, the Accounting department, and the planning department, the various causes for the contract labor hiring were detailed and measured done with a rating system.
Not suddenly, there were gaps in the planning process. When the organization received a production order, it was processed without checking whether it could be processed and completed within the limited period?.That resulted in needless manpower spent time on cleaning the machines and equipment after producing each order; preparing, measuring and weighing the raw material necessary to produce an order; and paperwork preparation.
The casual labor salesperson was also a participant in improving the manufacturing leverage index. Upon Westgate’ request, it provided all labor request records for 2012 notifying who at the company had demanded the labor, for which work area, for how long, etc. The records showed that the majority of demands were made by team leaders, but there were also unjustified demands made by nonauthorized personnel.
Invoices not being authorized and processed on time. This reduced distinguishability of the problem, allowing more unnecessary casual labor hiring.
Small batch orders that could have been joined with other orders for optimized production. As noted previously, this led to more work than necessary by employees to prepare the production line, and thus, created a simulated need for more casual labor to fill in.
Based on the cause analysis, the most important causes were addressed by corrective and preventive actions.
All orders must be checked by the product planning department to confirm the minimum production quantity. If an order does not meet the minimum production quantity, it will be held for consolidation with other orders to reach optimum production levels.
No casual labor request can be made until there is confirmation that there is no idle or surplus manpower available from other internal production lines.
Casual labor authorization was removed from team leaders and given only to production managers. These authorization points were communicated to the salesperson; they are required to check that the person requesting additional labor is authorized to make that request by Westgate. All requests from nonauthorized persons will be immediately rejected.
A formal, documented policy was formed for casual labor engagement.
Also, Westgate asked the service provider to stop sending invoices by mail; all invoices must be sent electronically. This not only helped improve the speed of invoice processing but also helped get payment authorizations completed properly by using the existing workflow system of Westgate.
This progress reduced the labor hours by up to 30 percent after July 2012. The manufacturing leverage index correspondingly improved, reaching as high as 60 percent from an earlier low of -90 percent, as shown in Figure. A revised process map that shows developments in the salesperson invoices, manpower allocations and planning is given in Figure . The developments were measured by using hypothesis testing – a t-test for the mean labor hours before and after the progress . The p-value is less than 0.05 so the null hypothesis is rejected, confirming that there is a significant difference in the mean value, and the performance of the process has improved (Figure 6).
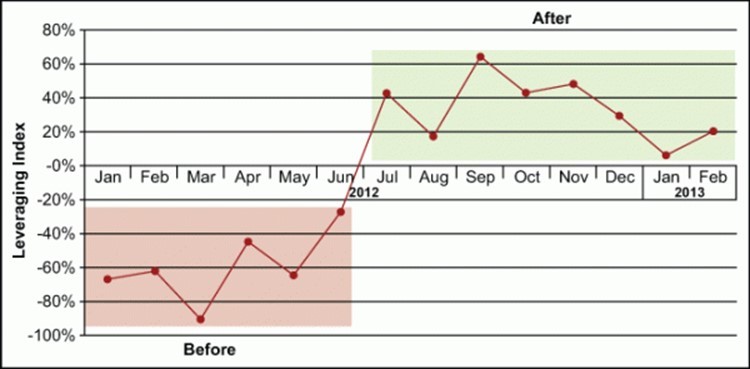
The progress was reviewed and confirmed as justifiable. The objective of Westgate remains to realize control based on a clearly defined system rather than leaving decisions up to individuals who define their priorities and processes.
To continue to enhance production quantity, the organization established a minimum process quantity in the workflow system. As a failsafe, a warning message will pop up if the minimum quantity is not reached with one order; the product planning team will not let unfinished orders proceed to production. All invoices follow the workflow system so that only the authorized personnel can view and accept the invoices. A follow-up email will be sent routinely to remind those authorized staffers if there is an invoice pending their approval – a further automated tool to help sustain improvements. Finally, the policies for requesting casual labor have been clearly communicated internally across the production team and with the service provider.
This case study exhibits the deployment of Six Sigma tools in finance for improvements in labor costs. The analysis involved four internal shareholders (local operations, planning, finance and the regional management team) as well as one external stakeholder. All data was collected either from the company’s existing workflow system or provided by the casual labor salesperson. Six people actively worked on this project while more than 12 contributed their time during a period of one month. No additional costs were spent on this improvement project.
- Six sigma Techniques |
- Six Sigma Tools and Techniques |
- Six Sigma Case Study
Live Testimonials
- Expert Tips for APM Certification: Certified Agile Product Management Exam
- Certified SAFe LPM: Uncover Practice Tests and Exam Strategies
- IIBA CBDA Exam Mastery: Proven Study Tips and Practice Tests
- ServiceNow CIS-SAM Certification: Earnings Guide
- CT-TAE Certification: Leading the Automation Revolution
Lean Six Sigma Training Certification

- Facebook Instagram Twitter LinkedIn YouTube
- (877) 497-4462
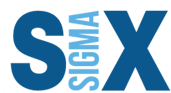
Full Factorial Design: Comprehensive Guide for Optimal Experimentation
June 4th, 2024
The full factorial design distinguishes itself as a robust, enlightening experimentation approach across industries.
At its core, this systematic method examines multiple metrics’ collective effects on an outcome simultaneously.
Considering all factor level combinations furnishes holistic comprehension beyond individual impacts—illuminating intricate relationships shaping complex systems’ behaviors.
The factorial design’s strength lies in realistically emulating dynamics’ nuances where variables interact intricately nonlinearly. Accounting for interplays guards against oversimplification. This casts light on underlying realities profoundly, priming informed resolutions and refinement pursuits.
From manufacturing to research frontiers, ponder occasions where exhaustively investigating factor relations meaningfully addresses specific problems or opportunities.
Key Highlights
- A comprehensive exploration of factor effects and interactions
- Robust methodology for process understanding and optimization
- Applications across diverse industries, including manufacturing, pharmaceuticals, and marketing
- Rigorous statistical analysis techniques, such as ANOVA and regression modeling
- Insights into factor-level optimization for enhanced process performance
- Evaluation of alternative designs, including fractional factorial and response surface methodologies
- Best practices for experimental design, data collection, and analysis
Introduction to Full Factorial Design
The full factorial design stands out as a comprehensive and robust approach, enabling researchers and practitioners to unlock valuable insights and drive meaningful change across diverse industries.
The full factorial design is a systematic way to investigate the effects of multiple factors on a response variable simultaneously.
By considering all possible combinations of factor levels, this experimental strategy provides a holistic understanding of not only the individual factor effects but also the intricate interactions that can shape outcomes in complex systems.
A full factorial design is an experimental design that considers the effects of multiple factors simultaneously on a response variable.
It involves manipulating all possible combinations of the levels of each factor, enabling researchers to determine the main effects of individual factors as well as their interactions statistically.
This comprehensive approach ensures that no potential interaction is overlooked, providing a complete picture of the system under investigation.
The key benefits of employing a full factorial design include:
- Main effects : Researchers can identify which factors have the most significant impact on the response variable, allowing them to focus their efforts on the most influential factors.
- Interaction effects : By accounting for interactions between factors, full factorial designs reveal how the effect of one factor depends on the level of another factor, providing insights into the complex relationships within the system.
- Optimization: With a comprehensive understanding of the main effects and interactions, researchers can estimate the optimal settings for the independent variables, leading to the best possible outcome for the response variable.
The versatility of the full factorial design makes it a valuable tool across various industries, including:
- Manufacturing : Optimizing processes, improving product quality, and reducing defects by identifying key variables and their interactions.
- Pharmaceuticals : Formulating and developing drugs by assessing factors such as excipient concentrations, drug particle size, and processing conditions on bioavailability, stability, and release profiles.
- Marketing: Optimizing promotional strategies by evaluating the effects of factors like ad content, media channels, target audience segments, and pricing on consumer response.
Fundamentals of Design of Experiments (DOE)
Before delving into the intricacies of full factorial design, it is essential to understand the fundamental principles of Design of Experiments (DOE) , a systematic approach to investigating the relationships between input variables (factors) and output variables (responses) .
DOE provides a structured framework for planning, executing, and analyzing experiments, ensuring reliable and insightful results.
Understanding Factors and Levels
In a DOE study, the variables that are manipulated or controlled by the experimenter are known as independent variables or factors.
These can be further classified into:
- Numerical factors : Variables that can take on a range of numerical values, such as temperature, pressure, or time.
- Categorical factors : Variables that have distinct, non-numerical levels, such as material type or production method.
The outcome or characteristic of interest that is measured and analyzed in an experiment is referred to as the response variable or dependent variable.
Common examples include product yield, strength, purity, or customer satisfaction.
Each independent variable in a DOE study can be set at different levels or values.
The choice of factor levels is crucial, as it determines the range of conditions under which the experiment is conducted.
Carefully selecting factor levels ensures that the study captures the relevant region of interest and provides meaningful insights into the system’s behavior.
Principles of DOE
Replication refers to the practice of repeating the same experimental run multiple times under identical conditions.
This allows researchers to estimate the inherent variability in the experimental process and ensures the reliability of the results by providing a measure of experimental error.
Randomization is the process of randomly assigning experimental runs to different factor level combinations.
This helps to mitigate the potential impact of nuisance variables and ensures that any observed effects can be attributed to the factors under investigation, rather than uncontrolled sources of variation .
Blocking is a technique used to account for known sources of variability in an experiment, such as differences in equipment, operators, or environmental conditions.
By grouping experimental runs into homogeneous blocks, researchers can isolate and quantify the effects of these nuisance variables, ensuring more precise estimates of the factor effects.
Types of Full Factorial Designs
Full factorial designs can be classified into different types based on the number of levels for each factor and the nature of the factors themselves.
Understanding the various types of full factorial designs is crucial for selecting the appropriate experimental strategy to address specific research questions or process optimization objectives.
2-Level Full Factorial Design
The 2-level full factorial design, where each factor has two levels (typically labeled as “low” and “high”), is commonly employed in screening experiments.
These experiments aim to identify the most significant factors influencing the response variable, allowing researchers to focus their efforts on the most promising factors in subsequent, more in-depth investigations.
By evaluating the main effects and interactions in a 2-level full factorial design, researchers can determine which factors have a statistically significant impact on the response variable.
This information is invaluable in prioritizing factors for further optimization or confirming their negligible influence, thereby streamlining the overall experimental process.
3-Level Full Factorial Design
Unlike the 2-level design, which assumes a linear relationship between factors and the response variable, the 3-level full factorial design allows for the investigation of quadratic effects.
These nonlinear effects can be important in scenarios where the response variable exhibits curvature or a peak/valley behavior within the explored factor ranges.
By incorporating three levels for each factor, researchers can model the curvature in the response surface more accurately.
This enhanced understanding of the system’s behavior enables more precise optimization and provides insights into potential optimal operating regions or factor level combinations.
Mixed-Level Full Factorial Design
In many real-world applications, experiments may involve a combination of categorical factors (e.g., material type, production method) and continuous factors (e.g., temperature, pressure).
The mixed-level full factorial design accommodates this scenario by allowing researchers to investigate the effects of both types of factors simultaneously, providing a comprehensive understanding of the system.
Analyzing Full Factorial Design Experiments
Once the experimental data has been collected, the next step is to analyze the results to gain insights into the main effects, interactions, and optimal factor level combinations.
Several statistical techniques are employed in the analysis of full factorial experiments, each serving a specific purpose and providing valuable information for process understanding and optimization.
Analysis of Variance (ANOVA)
Analysis of Variance (ANOVA) is a powerful statistical tool used to determine the significance of main effects (individual factor effects) and interaction effects (combined effects of multiple factors) on the response variable.
By partitioning the total variability in the data into components attributable to each factor and their interactions, ANOVA enables researchers to identify the most influential factors and their relationships.
ANOVA also provides a framework for hypothesis testing, allowing researchers to assess whether the observed effects are statistically significant or simply due to random variability.
This rigorous approach ensures that conclusions drawn from the experimental data are statistically valid and reliable.
Regression Analysis
Regression analysis is another essential tool in the analysis of full factorial experiments.
It involves fitting a mathematical model to the experimental data , relating the response variable to the independent variables (factors) and their interactions.
This model can be used to predict the response variable for any combination of factor levels within the experimental region.
Once a suitable regression model has been obtained, researchers can employ optimization techniques to identify the factor level combinations that maximize or minimize the response variable.
These techniques often involve solving the regression equation subject to relevant constraints, enabling the determination of optimal operating conditions for the process under investigation.
Graphical Analysis & Full Factorial Design
Graphical analysis is a powerful tool for visualizing and interpreting the results of full factorial experiments.
Interaction plots are particularly useful for examining the presence and nature of interactions between factors.
These plots display the response variable as a function of one factor at different levels of another factor, allowing researchers to identify and understand complex relationships within the system.
Main effects plots , on the other hand, illustrate the individual impact of each factor on the response variable, providing a visual representation of the main effects.
These plots can aid in quickly identifying the most influential factors and assessing the relative importance of each factor in the experimental domain.
Advantages and Limitations of Full Factorial Design
While the full factorial design offers numerous advantages in terms of comprehensiveness and insight, it is important to recognize its limitations and potential drawbacks.
Understanding both the strengths and limitations of this experimental approach is crucial for making informed decisions and optimizing the trade-offs between resource allocation and the desired level of process understanding.
Advantages of Full Factorial Design
One of the primary advantages of the full factorial design is its ability to provide comprehensive insights into the system under investigation.
By considering all possible factor combinations, researchers can obtain a complete picture of the main effects, interactions, and potential curvature in the response surface, leading to a thorough understanding of the process dynamics.
Unlike some experimental designs that may overlook or confound interactions, the full factorial design explicitly accounts for interactions between factors.
This capability is particularly valuable in complex systems where the effect of one factor may depend on the level of another factor, allowing researchers to unravel these intricate relationships and optimize processes accordingly.
With a comprehensive understanding of the main effects and interactions, full factorial experiments enable researchers to estimate the optimal settings for the independent variables, leading to the best possible outcome for the response variable.
This optimization potential is invaluable in various industries, where process efficiency , product quality, and cost-effectiveness are paramount.
Limitations of Full Factorial Design
One of the primary limitations of the full factorial design is its resource-intensive nature.
As the number of factors and levels increases, the number of experimental runs required grows exponentially, leading to higher costs, longer experimental durations, and greater logistical challenges.
Related to the resource-intensive aspect, full factorial designs often require large sample sizes to ensure statistical validity and reliable estimates of main effects and interactions.
This can be particularly challenging in situations where resources are limited or experimental conditions are difficult to replicate.
The comprehensiveness of the full factorial design can also lead to an overwhelming amount of data, especially when dealing with numerous factors and levels.
Analyzing and interpreting such large datasets can be a daunting task, requiring advanced statistical techniques and computational resources.
Alternative Designs and Extensions
While the full factorial design is a powerful and comprehensive experimental strategy, some alternative designs and extensions can be employed depending on the specific requirements and constraints of the research or industrial application.
These alternative approaches can offer trade-offs between experimental complexity, resource requirements, and the level of information obtained.
Fractional Factorial Designs
Fractional factorial designs are a class of experimental designs that involve studying only a carefully chosen fraction of the full factorial design.
By sacrificing the ability to estimate certain higher-order interactions, fractional factorial designs can significantly reduce the number of experimental runs required, making them more resource-efficient.
Fractional factorial designs are particularly useful in screening experiments, where the primary goal is to identify the most influential factors before conducting more detailed investigations.
These designs can help researchers prioritize their efforts and allocate resources more effectively.
Response Surface Methodology
Response Surface Methodology (RSM) is a collection of statistical techniques used to model and optimize processes with multiple input variables.
The Central Composite Design (CCD) is a widely used RSM design that combines a factorial design with additional axial and center points, allowing for the estimation of quadratic effects and potential curvature in the response surface.
Another popular RSM design is the Box-Behnken Design, which is a spherical, rotatable, or nearly rotatable design.
This design is particularly efficient for exploring quadratic response surfaces and optimizing processes with three or more factors.
Unlike the Central Composite Design, the Box-Behnken Design does not include any points at the vertices of the cubic region defined by the upper and lower limits of the factors.
Taguchi Methods
Developed by Genichi Taguchi, the Taguchi methods are a set of techniques for robust parameter design and quality improvement.
One of the key elements of the Taguchi approach is the use of orthogonal arrays, which are a special class of fractional factorial designs.
Orthogonal arrays allow for the simultaneous investigation of multiple factors with a minimal number of experimental runs, making them an attractive option when resources are limited.
The Taguchi methods emphasize the concept of robust parameter design, which aims to identify factor-level combinations that minimize the variability in the response variable while achieving the desired target value.
This approach is particularly valuable in manufacturing and product development, where robustness to environmental and operational variations is critical for maintaining consistent performance and quality.
The full factorial design stands as a potent experimental strategy.
Considering every factor combination furnishes holistic comprehension of effects, interplays, and nonlinearities.
This lights pathways empowering better comprehension and optimization across varied sectors.
Advancing capabilities and statistical techniques envision expanding factorial applications.
Machine learning may analyze data efficiently while adaptive designs responsively calibrate based on real-time insights.
Additionally, sustainability priorities could drive factor prioritizations maximizing resource optimization and lessening environmental impacts.
Full factorials offer rigorous yet flexible methods unlocking enriched wisdom from intricate systems.
While exhaustive, generated learnings inspire noteworthy refinements throughout performance, quality, and workflows.
By following best practices, judiciously leveraging other designs, and tracking innovations, researchers and specialists harness factorials’ full gifts in driving innovations remarkably within respective realms.
Their gifts in illuminating intricate relations deserve recognition and prudent application wherever circumstances permit comprehensive experimentation.
SixSigma.us offers both Live Virtual classes as well as Online Self-Paced training. Most option includes access to the same great Master Black Belt instructors that teach our World Class in-person sessions. Sign-up today!
Virtual Classroom Training Programs Self-Paced Online Training Programs
SixSigma.us Accreditation & Affiliations
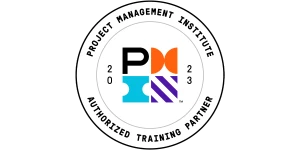
Monthly Management Tips
- Be the first one to receive the latest updates and information from 6Sigma
- Get curated resources from industry-experts
- Gain an edge with complete guides and other exclusive materials
- Become a part of one of the largest Six Sigma community
- Unlock your path to become a Six Sigma professional
" * " indicates required fields
Interpretive structural modeling of lean six sigma critical success factors in perspective of industry 4.0 for Indian manufacturing industries
- ORIGINAL ARTICLE
- Published: 30 May 2024
Cite this article
- Pramod Kumar 1 ,
- Jaiprakash Bhamu 2 ,
- Sunkulp Goel 3 &
- Dharmendra Singh ORCID: orcid.org/0000-0002-6726-310X 2
15 Accesses
Explore all metrics
This paper aims to identify and analyze critical success factors (CSFs) of Lean Six Sigma (LSS) implementation in context to Industry 4.0 (I4.0) in Indian manufacturing industries. Twenty CSFs are identified from literature and expert’s opinion. A survey was conducted through administration of designed questionnaire in Indian manufacturing industries and reliability of the factors was tested calculating Cronbach’s alfa (α) value for all responses. Thereafter, out of twenty CSFs, sixteen were found reliable. Further, these sixteen factors were analyzed employing Interpretive Structural Modeling (ISM) technique and leveled as per developed model. The MICMAC analysis is employed for determining driving and dependence power of CSFs. The developed model provides a platform for the practitioners/researchers to design a framework for successful implementation of LSS in view of current manufacturing paradigm of I4.0. On analyzing the data using ISM technique, the ‘ Organizational culture and belief ’, ‘ Effective top management commitment and attitude ’ and ‘ Motivated and skilled manpower ’ are observed to be the most significant CSFs which drive the path for proper implementation of LSS in Indian manufacturing industries. The developed model will enable the practitioners to draw the effective strategy for proper implementation of LSS in view of Industry 4.0. The results will give an edge to the management to think strategically for improvements in this competitive environment.
This is a preview of subscription content, log in via an institution to check access.
Access this article
Price includes VAT (Russian Federation)
Instant access to the full article PDF.
Rent this article via DeepDyve
Institutional subscriptions
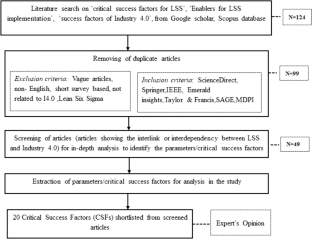
Similar content being viewed by others
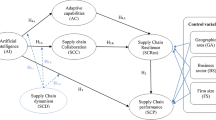
Artificial intelligence-driven innovation for enhancing supply chain resilience and performance under the effect of supply chain dynamism: an empirical investigation
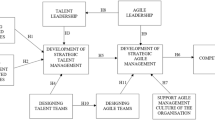
Competitiveness Through Development of Strategic Talent Management and Agile Management Ecosystems
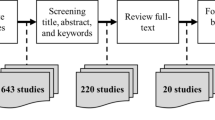
Key performance indicators for business models: a systematic review and catalog
Abu Bakar FA, Subari K, Mohd Daril MA (2015) Critical success factors of Lean Six Sigma deployment: a current review. Int J Lean Six Sigma 6(4):339–348
Article Google Scholar
Andersson R, Hilletofth P, Manfredsson P, Hilmola OP (2014) Lean Six Sigma strategy in telecom manufacturing. Ind Manag Data Syst 114(6):904–921
Antony J, Setijono D, Dahlgaard JJ (2014) lean six sigma and innovation—an exploratory study among UK organizations. Total Qual Manag Bus Excell 27:124–140
Assarlind M, Aaboen L (2013) Forces affecting one lean six sigma adoption process. Int J Lean Six Sigma 5(3):324–340
Bag S, Telukdarie A, Pretorius JHC, Gupta S (2018) Industry 4.0 and supply chain sustainability: framework and future research directions. Benchmarking Int J. https://doi.org/10.1108/BIJ-03-2018-056/full/html
Bertola P, Teunissen J (2018) Fashion 4.0. Innovating fashion industry through digital transformation. Res J Text Appar 22(4):352–369
Bhamu J, Sangwan KS (2014) Lean manufacturing: literature review and research issues. Int J Oper Prod Manag 34(7):876–940
Brun A (2011) Critical success factors of six sigma implementations in Italian companies. Int J Prod Econ 131(1):158–164
Chiarini A (2013) Differences between six sigma applications in manufacturing and the service industry. Int J Prod Qual Manag 12(3):345–360
Google Scholar
Chiarini A, Kumar M (2020) Lean six sigma and industry 4.0 integration for operational excellence: evidence from Italian manufacturing companies. Prod Plan Control 32(13):1–18
Churchill G, Iacobucci D (2002) Marketing Research, Methodological Foundations, 8th edn. Harcourt Publishing, London
Devi KS, Paranitharan KP, Agniveesh AI (2021) Interpretive framework by analysing the enablers for implementation of Industry 4.0: an ISM approach. Total Qual Manag Bus Excell 32(13–14):1494–514
Dewangan DK, Agrawal R, Sharma V (2015) Enablers for competitiveness of indian manufacturing sector: an ISM-Fuzzy MICMAC analysis. Proc Soc Behav Sci 189:416–32
Drohomeretski E, Gouvea da Costa SE, Pinheiro de Lima E, da Garbuio PA (2014) Lean, six sigma and lean six sigma: an analysis based on operations strategy. Int J Prod Res 52(3):804–824
Franchetti M, Barnala P (2013) Lean six sigma at a material recovery facility: a case study. Int J Lean Six Sigma 4(3):251–264
Gijo EV, Antony J (2014) Reducing patient waiting time in outpatient department using lean six sigma methodology. Qual Reliab Eng Int 30(8):1481–1491
Gunasekharan S, Elangovan D, Parthiban P (2014) A Comprehensive study to evaluate the critical success factors affecting lean concept in Indian manufacturing industries. Appl Mech Mater 592:2569–2576
Jayaraman K, Kee TL, Soh KL (2012) The perceptions and perspectives of Lean Six Sigma (LSS) practitioners. TQM J 24(5):433–446
Kashyap A, Yadav AK, Vatsa ON, Chandaka TN, Shukla OJ (2023) Investigation of the critical success factors in the implementation of the lean industry 4.0 in manufacturing supply chain: an ISM approach. Manag Environ Qual 34(4):981–996. https://doi.org/10.1108/MEQ-04-2022-0109
Lande M, Shrivastava RL, Seth D (2016) Critical success factors for lean six sigma in SMEs (small and medium enterprises). TQM J 28(4):613–635
Lin D, Lee CKM, Lau H, Yang Y (2018) Strategic response to Industry 4.0: an empirical investigation on the Chinese automotive industry. Ind Manag Data Syst 118(3):589–605
Luthra S, Yadav G, Kumar A, Anosike A, Mangla SK, Garg D (2020) Study of key enablers of industry 4.0 practices implementation using ISM-Fuzzy MICMAC Approach, In: Proceedings of the International Conference on Industrial Engineering and Operations Management Dubai, UAE, March 10–12, 2020., IEOM Society International, pp. 241–251.
Machado CG, Winroth MP, Carlsson D, Almstrom P, Centerholt V, Hallin M (2019) Industry 4.0 readiness in manufacturing companies: challenges and enablers towards increased digitalization. Proc CIRP 81:1113–1118
Manville G, Greatbanks R, Krishnasamy R, Parker DW (2012) Critical success factors for Lean Six Sigma programmes: a view from middle management. Int J Qual Reliab Manag 29(1):7–20
Mittal S, Khan MA, Romero D, Wuest T (2019) Smart manufacturing : characteristics, technologies and enabling factors. J Eng Manuf Smart Manuf Digit Fact 233(5):1342–1361
Moeuf A, Lamouri S, Pellerin R, Tamayo-Giraldo S, Tobon-Valencia E, Eburdy R (2019) Identification of critical success factors, risks and opportunities of Industry 4.0 in SMEs. Int J Prod Res 58(5):1–17
Mohammed IR, Shankar R, Banwet DK (2008) Creating flex-lean-agile value chain by outsourcing: an ISM-based interventional roadmap. Bus Process Manag J 14(3):338–389
Naslund D (2013) Lean and six sigma—critical success factors revisited. Int J Qual Serv Sci 5(1):86–100
Nicoletti B, Vergata T (2013) Lean Six Sigma and digitize procurement. Int J Lean Six Sigma 4(2):184–203
Panigrahi RR, Jena D, Mishra PC (2022) Inventory automation practices and productivity: a study on steel manufacturing firms. Int J Appl Syst Stud 9(3):195–213
Panigrahi RR, Jena D, Meher JR, Shrivastava AK (2023) Assessing the impact of supply chain agility on operational performances-a PLS-SEM approach. Meas Bus Excell 27(1):1–24
Panizzolo R, Garengo P, Sharma ML, Gore A (2012) Lean manufacturing in developing countries: evidence from Indian SMEs. Prod Plan Control Manag Oper 23(10/11):769–788
Pongboonchai-Empl T, Antony J, Garza-Reyes JA, Komkowski T, Tortorella GL (2023) Integration of Industry 4.0 technologies into Lean Six Sigma DMAIC: a systematic review. Prod Plan Control. https://doi.org/10.1080/09537287.2023.2188496
Psomas E (2016) The underlying factorial structure and significance of the Six Sigma difficulties and critical success factors. TQM J 28(4):530–546
Rathi R, Kaswan MS, Antony J, Cross J, Garza-Reyes JA, Furterer SL (2023) Success factors for the adoption of green lean six sigma in healthcare facility: an ISM-MICMAC study. Int J Lean Six Sigma 14(4):864–897
Samanta M, Virmani N, Singh RK, Haque SN, Jamshed M (2023) Analysis of critical success factors for successful integration of lean six sigma and Industry 4.0 for organizational excellence. TQM J. https://doi.org/10.1108/TQM-07-2022-0215
Shamsi MA, Alam A (2018) Exploring lean six sigma implementation barriers in information technology industry. Int J Lean Six Sigma 9(4):523–542
Singh N, Panigrahi R, Panigrahi RR, Shrivastava AK (2024) An integrated total interpretive structural modeling and MICMAC model for uncovering enterprise agility barriers in the insurance industry. Decis Anal J 10(100421):1–11
Skalli D, Charkaouli A, Cherrafi A (2022) Assessing interactions between lean six-sigma, circular economy and industry 4.0: toward an integrated perspective. IFAC PapersOnLine 55(10):3112–3117
Snee RD (2010) Lean Six Sigma-getting better all the time. Int J Lean Six Sigma 1(1):9–29
Article MathSciNet Google Scholar
Soti A, Shankar R, Kaushal OP (2010) Modeling the enablers of Six Sigma using interpreting structural modeling. J Model Manag 5(2):124–141
Swarnakar V, Tiwari AK, Singh AR (2020) Evaluating critical failure factors for implementing sustainable lean six sigma framework in manufacturing organization: a case experience. Int J Lean Six Sigma 11(6):1069–1104
Thomas AJ, Francis M, Fisher R, Byard P (2016) Implementing Lean Six Sigma to overcome the production challenges in an aerospace company. Prod Plan Control 27(7/8):591–603
Titmarsh R, Assad F, Harrison R (2020) Contributions of lean six sigma to sustainable manufacturing requirements: an Industry 4.0 perspective. Proc CIRP 90:589–593
Tripathy S, Sahu S, Ray PK (2013) Interpretive structural modelling for critical success factors of R&D performance in Indian manufacturing firms. J Model Manag 8(2):212–240
Vaidya S, Ambad P, Bhosle S (2018) Industry 4.0—a glimpse proceedings of second international conference on materials manufacturing and design engineering. Proc Manuf 20:233–238
Warfield JW (1974) Developing interconnected matrices in structural modeling institute of electrical and electronics engineering transactions on systems. Man Cybern 4(1):51–81
MathSciNet Google Scholar
Yadav G, Desai TN (2016) Lean Six Sigma: a categorized review of the literature. Int J Lean Six Sigma 7(1):2–24
Yadav G, Desai TN (2017) Analyzing lean six sigma enablers: a hybrid ISM- Fuzzy MICMAC approach. TQM J 29(3):488–511
Yadav G, Seth D, Desai TN (2018) Application of hybrid framework to facilitate lean six sigma implementation: a manufacturing company case experience. Prod Plan Control 29(3):185–201
Yadav N, Shankar R, Singh SP (2020) Impact of Industry 4.0/ICTs, Lean Six Sigma and quality management systems on organizational performance. TQM J 32(4):815–835
Zhang A, Venkatesh VG, Wang JX, Venkatesh M, Wan M, Qu T (2019) Drivers of Industry 4.0-enabled smart waste management in supply chain operations: a circular economy perspective in China. Prod Plan Control 34(10):1–51
Zwetsloot IM, Kuiper A, Akkerhuis TS, de Koning H (2018) Lean Six Sigma meets data science: integrating two approaches based on three case- studies. Qual Eng 30(3):419–431
Kamble S, Gunasekaran A, Dhone NC (2020) Industry 4 0 and lean manufacturing practices for sustainable organizational performance in Indian manufacturing companies. Int J of Prod Res 58(5):1319–1337
Nafchi MZ, Mohelska H (2020) Organizational culture as an indication of readiness to implement industry4.0. Information 11(174):1–11
Muller JM, Kiel D, Voigt KI (2018) What drives the implementation of industry 4.0? The role of opportunities and challenges in the context of sustainability. Sustainability 10(247):1–24
Rossin F, Forget P, Lamouri S, Pellerin R (2019) Impacts of industry 4.0 technologies on Lean principles. Int J Prod Res. 50(6):1644–1661
Bhat A, Patil S, Belur J (2020) ISM fuzzy technique for management decision-making to reduce employee turnover: a study of Indian manufacturing industry. J Commerce Management 9(2):16–39
Arcidiacono G, Pieroni A (2018) The revolution Lean six sigma 4.0. Int J Adv Sci Eng Inform Tech. 8(1):141–149
Kamble SS, Gunasekaran A, Sharma R (2018) Analysis of the driving and dependence power of barriers to adopt industry 4.0 in Indian manufacturing industry. Comput Ind 101:107–119
Black SA, Porter LJ (1996) Identification of the critical factors of TQM. Decis Sci 27(1):1–21
Download references
The authors did not receive any funding from any organization for the submitted work.
Author information
Authors and affiliations.
Department of Mechanical Engineering, Vivekananda Global University, Jaipur, Rajasthan, 303012, India
Pramod Kumar
Department of Mechanical Engineering, Government Engineering College, Bikaner, Rajasthan, 334001, India
Jaiprakash Bhamu & Dharmendra Singh
Department of Mechanical Engineering, Teeranthakar Mahaveer University, Moradabad, UP, 244001, India
Sunkulp Goel
You can also search for this author in PubMed Google Scholar
Corresponding author
Correspondence to Dharmendra Singh .
Ethics declarations
Conflict of interest.
The authors have no potential conflicts of interest to declare that are relevant to the content of this article.
Human and animal rights
Authors do not involve any human/animal participants in research.
Informed consent
Research is conducted on data collected.
Additional information
Publisher's note.
Springer Nature remains neutral with regard to jurisdictional claims in published maps and institutional affiliations.
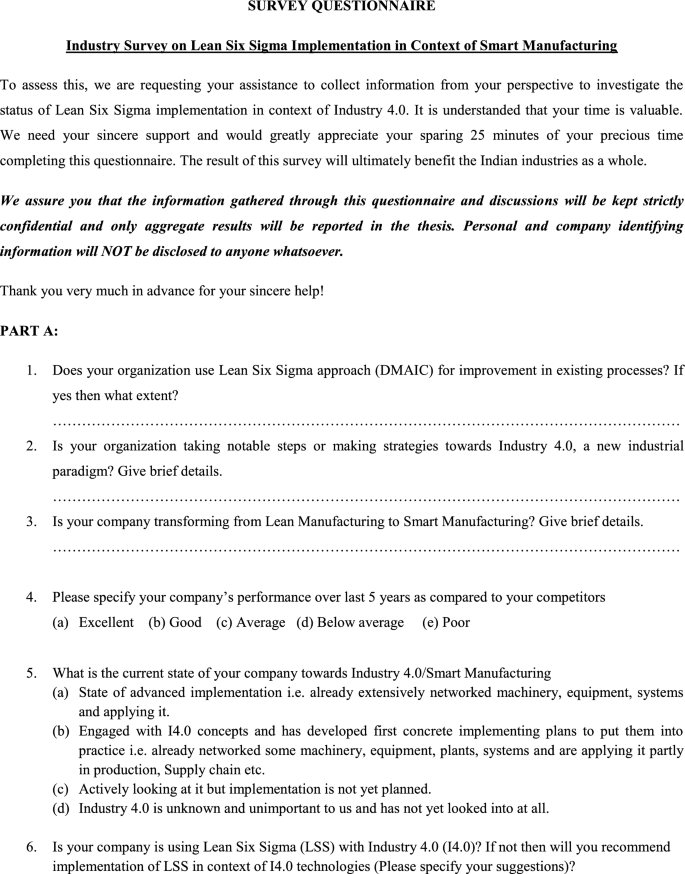
Rights and permissions
Springer Nature or its licensor (e.g. a society or other partner) holds exclusive rights to this article under a publishing agreement with the author(s) or other rightsholder(s); author self-archiving of the accepted manuscript version of this article is solely governed by the terms of such publishing agreement and applicable law.
Reprints and permissions
About this article
Kumar, P., Bhamu, J., Goel, S. et al. Interpretive structural modeling of lean six sigma critical success factors in perspective of industry 4.0 for Indian manufacturing industries. Int J Syst Assur Eng Manag (2024). https://doi.org/10.1007/s13198-024-02375-y
Download citation
Received : 15 May 2023
Revised : 04 April 2024
Accepted : 14 May 2024
Published : 30 May 2024
DOI : https://doi.org/10.1007/s13198-024-02375-y
Share this article
Anyone you share the following link with will be able to read this content:
Sorry, a shareable link is not currently available for this article.
Provided by the Springer Nature SharedIt content-sharing initiative
- Lean six sigma
- Industry 4.0
- Critical success factors
- Interpretive structural modeling
- Indian manufacturing industries
- Find a journal
- Publish with us
- Track your research

COMMENTS
Six Sigma Case Study is a data-driven methodology widely used in organisations to improve process efficiency and quality. It helps reduce process defects and variations, enhancing customer satisfaction and increasing profitability. It provides a real-world example of how its methodology was applied to identify and solve a problem, showcasing ...
This blog outlines case studies exemplifying the use of Lean Six Sigma strategies to eliminate outdated business procedures that failed to meet contemporary market norms. These entities revamped their operations, resulting in immediate improvements to both revenue generation and profitability. If you are in the manufacturing industry, this blog ...
Manufacturing. Increasing First Run Parts From 60% to 90% With Lean Six Sigma. Reducing Bent/Scratched/Damaged (BSD) Scrap for Building Envelopes. Reducing Lead Time in Customer Replacement Part Orders by 41%. Reducing Learning Curve Rampu0003 for Temp Employees by 2 Weeks. Reducing Purchase Order Lead Time by 33% Using Lean Six Sigma.
The main purpose of this chapter is to explore the Lean Six Sigma (LSS) in the manufacturing sector. This chapter focuses on the different critical aspects of LSS. ... Muda MS. Lean six sigma implementation: Multiple case studies in a developing country. International Journal of Lean Six Sigma. 2019; 10 (1):523-539. DOI: 10.1108/IJLSS-08-2017-0096
This research examines a case study on the implementation of an effective approach to advanced Lean Six Sigma problem-solving within a pharmaceutical manufacturing site which manufactures acetaminophen (paracetamol containing pain relief) tablets. Though this study was completed in a single manufacturing company, the implementation of this study delivers important application and results that ...
Radha Krishna GA, Dangayach GS (2007) Six Sigma implementation at an auto component manufacturing plant: a case study. Int J Six Sigma Compet Adv 3(3):282-302. Article Google Scholar Rathore P, Kota S, Chakrabarti A (2011) Sustainability through remanufacturing in India: a case study on mobile handsets.
Continuous improvement is the prime requirement for all industries to sustain and grow in the competitive global market. This paper is a case study of a manufacturing industry in Taiwan, facing the problem of rejection in the brushless motor product. The DMAIC (Define, Measure, Analyze, Improve, and Control) cycle was used to improve processes to reduce the rejection rate. In the Define phase ...
In the world of process improvement methodologies, Six Sigma stands tall as a beacon of excellence. This data-driven approach has consistently proven its efficacy in eliminating defects, reducing variations, and enhancing operational efficiencies across diverse industries.Today, let's delve into a compelling case study that illustrates the transformative power of Six Sigma implementation ...
In their study, Tanık and S en conducted an analysis of three phases of the six sigma program, which consisted of 20 separate six sigma projects, at a large-scale automotive supplier company that ...
Lean Six Sigma Case Studies. Welcome to the Lean Six Sigma Academy's Case Studies section! Here, you will find a collection of real-world examples of how companies have successfully implemented the Lean Six Sigma methodology to improve their business operations. Each case study includes an overview of the business challenge that was faced ...
Lean Six Sigma combines the waste-reduction principles of Lean manufacturing with the process improvement tools of Six Sigma. The aim is to create a more efficient and error-free production process. Lean Six Sigma is data-driven and focuses on customer satisfaction as its ultimate goal. It seeks to eliminate defects, overproduction, waiting ...
This article discusses the successful implementation of Six Sigma methodology in a high precision and critical process in the manufacture of automotive products. The Six Sigma define-measure-analyse-improve-control approach resulted in a reduction of tolerance-related problems and improved the first pass yield from 85% to 99.4%.
Case Study: Lean Six Sigma Improves Environment - Baxter Manufacturing. Six Sigma best practices are instrumental in changing the way businesses operate. Motorola recognized this in the 1980s when the company employed Six Sigma principles in manufacturing processes. What began as a methodology to reduce defects in a production line has ...
The case study allowed for examining in depth the implementation of a Lean Six Sigma improvement project for the transformation and simplification of the production process of the Schnell "Alfa" and "Beta" machines with the aim to reduce the delivery times of its products . The case study was developed with the participant observation ...
Singh and Lal [31] demonstrated a study of Six Sigma for an automobile manufacturing industry producing engine mufflers. The rejection rate of muffler production decreased from 8.21 to 4.81% as a result of Six Sigma deployment, the industry's Sigma level increased from 2.89 to 3.16, and process capability increased from 91.73% to 95.19%.
The purpose of this chapter is to outline systematic implementation of the Six Sigma DMAIC methodology as a case study in solving the problem of poor wafer yields in semiconductor manufacturing. The chapter also describes well-known industry standard business processes to be implemented and benchmarked in a semiconductor wafer fabrication facility to manage defect and yield issues while ...
This research examines a case study on the implementation of an effective approach to advanced Lean Six Sigma problem-solving within a pharmaceutical manufacturing site which manufactures ...
Abstract and Figures. This paper presents a Six Sigma project conducted at a semiconductor company dedicated to the manufacture of circuit cartridges for inkjet printers. They are tested ...
Start now to become a certified professional in quality management. 5. Ford Motors: Driving Success. This is a case study on Six Sigma i ncorporated by Ford Motors to streamline processes, improve quality, significantly reduce costs, and reduce environmental impact. Initially met with skepticism, Ford's implementation overcame challenges ...
Six Sigma Improves the Environment: A Look at a Baxter Manufacturing. August 16th, 2013. Six Sigma best practices are instrumental in changing the way businesses operate. Motorola recognized this in the 1980s when the company employed Six Sigma principles in manufacturing processes. What began as a methodology to reduce defects in a production ...
Case Study: Lean Six Sigma Improves Environment - Baxter Manufacturing. Case Study: Lean 6 Sigma in Aerospace Manufacturer Streamlines Processes & Improves Cycle Time ... Manufacturing (139) Master Black Belt Curriculum (1) Master Black Belt training (1) Measure (3) Media (2) Military (3) Negotiation (1) Non-Profit Accrediting Bodies (1 ...
Case Study: Portuguese Tire Manufacturer Saves Thousands Using Six Sigma's DMAIC Methodology. SHARE ON: admin — February 19, 2018. A tire manufacturing company in Portugal has provided an excellent study in implementing Six Sigma and how it can impact business performance. Six Sigma already has proven its value in the automobile industry.
This progress reduced the labor hours by up to 30 percent after July 2012. The manufacturing leverage index correspondingly improved, reaching as high as 60 percent from an earlier low of -90 percent, as shown in Figure. A revised process map that shows developments in the salesperson invoices, manpower allocations and planning is given in ...
This paper presents the application of Lean Six Sigma methodology to improve the time and quality of attention to a service company's non-face-to-face channel "telephone line" in a low- and medium-income country. The study problem focuses on long waiting times and the quality of care received. To address this problem, implementing the ...
The full factorial design stands out as a comprehensive and robust approach, enabling researchers and practitioners to unlock valuable insights and drive meaningful change across diverse industries. The full factorial design is a systematic way to investigate the effects of multiple factors on a response variable simultaneously.
Business, Engineering. Purpose This paper aims to identify leadership behaviours in the manufacturing sector in Jordan that enable successful Lean Six Sigma implementation. Design/methodology/approach Qualitative data were collected through 27 interviews to identify the leadership behaviours that facilitate Lean Six Sigma and a focus group to ...
This paper aims to identify and analyze critical success factors (CSFs) of Lean Six Sigma (LSS) implementation in context to Industry 4.0 (I4.0) in Indian manufacturing industries. Twenty CSFs are identified from literature and expert's opinion. A survey was conducted through administration of designed questionnaire in Indian manufacturing industries and reliability of the factors was tested ...
Question: Case Study: Six SigmaThe following fictional case in the food processing industry, illustrates how Six Sigma can be leveraged to make improvements to quality. As you read through the case, think about the process of implementing a Six Sigma project using the DMAIC method.Acme FoodIn late 2012, with the recent outbreaks of bacteria ...
Complete understanding and experience implementing Lean Manufacturing Concepts: 5S, JIT, SMED, KanBan, VSM, One Piece Flow, etc. Working knowledge of Six-Sigma or other data-driven decision making experience highly desirable. Lean Six-Sigma Green Belt, equivalent certification, or proven experience in a Lean Six-Sigma environment highly desirable.