- Research article
- Open access
- Published: 14 March 2019

Prognostic models for breast cancer: a systematic review
- Minh Tung Phung ORCID: orcid.org/0000-0002-3573-4501 1 ,
- Sandar Tin Tin 1 &
- J. Mark Elwood 1
BMC Cancer volume 19 , Article number: 230 ( 2019 ) Cite this article
15k Accesses
94 Citations
3 Altmetric
Metrics details
Breast cancer is the most common cancer in women worldwide, with a great diversity in outcomes among individual patients. The ability to accurately predict a breast cancer outcome is important to patients, physicians, researchers, and policy makers. Many models have been developed and tested in different settings. We systematically reviewed the prognostic models developed and/or validated for patients with breast cancer.
We conducted a systematic search in four electronic databases and some oncology websites, and a manual search in the bibliographies of the included studies. We identified original studies that were published prior to 1st January 2017, and presented the development and/or validation of models based mainly on clinico-pathological factors to predict mortality and/or recurrence in female breast cancer patients.
From the 96 articles selected from 4095 citations found, we identified 58 models, which predicted mortality ( n = 28), recurrence ( n = 23), or both ( n = 7). The most frequently used predictors were nodal status ( n = 49), tumour size ( n = 42), tumour grade ( n = 29), age at diagnosis ( n = 24), and oestrogen receptor status ( n = 21). Models were developed in Europe ( n = 25), Asia ( n = 13), North America ( n = 12), and Australia ( n = 1) between 1982 and 2016. Models were validated in the development cohorts ( n = 43) and/or independent populations ( n = 17), by comparing the predicted outcomes with the observed outcomes ( n = 55) and/or with the outcomes estimated by other models ( n = 32), or the outcomes estimated by individual prognostic factors ( n = 8). The most commonly used methods were: Cox proportional hazards regression for model development ( n = 32); the absolute differences between the predicted and observed outcomes ( n = 30) for calibration; and C-index/AUC ( n = 44) for discrimination.
Overall, the models performed well in the development cohorts but less accurately in some independent populations, particularly in patients with high risk and young and elderly patients. An exception is the Nottingham Prognostic Index, which retains its predicting ability in most independent populations.
Conclusions
Many prognostic models have been developed for breast cancer, but only a few have been validated widely in different settings. Importantly, their performance was suboptimal in independent populations, particularly in patients with high risk and in young and elderly patients.
Peer Review reports
Breast cancer is the most common cancer in women worldwide [ 1 ]. The disease is highly heterogeneous with wide variations in prognosis [ 2 ]. Prognosis means the probability or risk that an outcome (such as deaths, complications, quality of life, pain, or disease regression) develops over a specific time, based on both clinical and non-clinical profiles [ 3 ]. In breast cancer patients, 5-year relapse-free survival (RFS) ranges from 65 to 80% [ 4 ], and 10-year overall survival (OS) ranges from 55 to 96% [ 5 ].
Prognosis for breast cancer is important in several ways. Firstly, it informs patients about the future course of their illness [ 3 ]. Two Australian surveys found that survival time information was desired by 87 and 85% of early and metastatic breast cancer patients, respectively [ 6 , 7 ]. Secondly, prognosis is essential for breast cancer treatment: the more precise is the outcome predicted, the better a patient is allocated the right treatment [ 3 , 8 , 9 , 10 ]. For example, patients whose prognosis is very poor may be considered for aggressive treatments, while those with a good prognosis may be saved from overtreatment and its related side-effects and financial costs [ 11 , 12 ]. Thirdly, prognosis can be used for the inclusion and stratification of patients in experimental studies [ 8 , 9 ]. Finally, prognosis helps policy makers compare mortality rates among hospitals and institutions [ 3 , 13 ].
Many models have been developed to predict breast cancer prognosis. The number of models has increased rapidly, accompanying with the great variance in terms of patients included, methods of development, predictors, outcomes, presentations, and performance in different settings [ 11 , 14 ]. Nevertheless, to our knowledge, only two reviews of prognostic models for breast cancer have been conducted, but with limitations. An earlier review reported 54 models that were developed between 1982 and 2001, with a focus on model development methods rather than model performance in different populations [ 11 ]. A more recent review included only 26 models published up to July 2012 [ 14 ]. This systematic review was undertaken to identify all prognostic models that have been published up to 2017, and to assess how the models performed in different settings.
Study search
A systematic search was conducted in EMBASE, PUBMED, Web of Science, COCHRANE, and in specific breast cancer and oncology websites, including: American Society of Clinical Oncology (ASCO) https://www.asco.org/ , Journal of the National Comprehensive Cancer Network (JNCCN) http://www.jnccn.org/ , Memorial Sloan Kettering Cancer Centre (MSKCC) https://www.mskcc.org/ , MD Anderson Cancer Centre https://www.mdanderson.org/ , Mayo Clinic http://www.mayoclinic.org/ , and European Society for Medical Oncology (ESMO) http://www.esmo.org/ . A manual search in the bibliographies of selected articles was also conducted. The search terms used were “prognostic model”, “breast cancer”, and their synonyms (see details in Additional file 1 ).
Eligibility criteria
This review included all research articles that presented the development and/or validation of prognostic models for female breast cancer, were published in English prior to 1st January 2017 and were available in full text. The review was restricted to the models that were developed based on at least two different clinico-pathological factors and/or commonly used biomolecular factors, such as hormonal receptor status or human epidermal growth factor receptor 2 (HER2) status, and predicted mortality and/or recurrence of women who were diagnosed with primary breast cancer. Articles that reported the development of a model for specific patient groups (those with invasive ductal carcinoma or invasive lobular carcinoma, those who have undergone surgery) were included. Articles that presented the development of a model for rare histological subtypes of breast cancer or special types of patients (such as those with metastases, those with hormonal receptor negative or positive, those with node negative or positive, those with neoadjuvant or adjuvant therapy) were excluded due to their limited generalisability.
Study selection and data extraction
Publications were screened in three levels - titles, abstracts, and full texts. From each selected article, relevant information was extracted into a data extraction sheet using the TRIPOD [ 15 ] and CHAMRS checklist [ 16 ], and included: authors, year of publication, objectives, name of models, study design, source of data, targeted populations, methods of development and/or validation, risk groups, outcomes, predictors, results of the development and/or validation, limitations and strengths.
The selected articles were categorised into three groups: those that presented model development, those that presented internal validation, and those that presented external validation. For the articles that presented the development of more than one model, we reviewed the best model only if the study indicated the best model, or we reviewed all the models presented if the study did not select the best model. Internal validation is defined here as the validation of a model in participants selected from the model development cohorts, or in patients recruited from the same source as in the development cohorts but at different times. External validation is defined as the validation of a model in patients from sources independent from the development cohorts [ 8 ].
Assessment of risk of bias in individual studies
The risk of bias within individual studies was assessed by using a modified version of the QUIPS (QUality In Prognosis Studies) tool, which was originally designed to assess bias in studies of prognostic factors [ 17 , 18 ]. The tool originally comprises six domains – Study Participation, Prognostic Factor Measurement, Outcome Measurement, Statistical Analysis and Reporting, Study Confounding, and Study Attrition, each of which is guided by three to seven prompting items. The last two domains were omitted as these are not relevant to the studies included in this review. The overall rating for each of the remaining four domains was assigned as low, moderate, or high risk of bias [ 17 ].
The risk of bias was assessed separately for development (and internal validation) studies and external validation studies. For articles that presented both model development and external validation, the risk of bias was assessed separately for each part. For articles that presented internal validation without model development, the risk of bias was assessed similarly to the external validation studies.
The systematic search in the four databases generated 4084 records, supplemented by 11 publications found in other sources (Fig. 1 ) . We excluded 2466 duplicates. We screened the titles and then the abstracts of the remaining records and excluded 1355 records. We reviewed the full text of the remaining 274 articles and identified 96 eligible articles, of which 54 presented model development, 42 presented internal validation and 49 presented external validation. Twenty four studies that met the eligibility criteria but were not available in full text are presented in Additional file 2 (model development) and Additional file 3 (model validation).
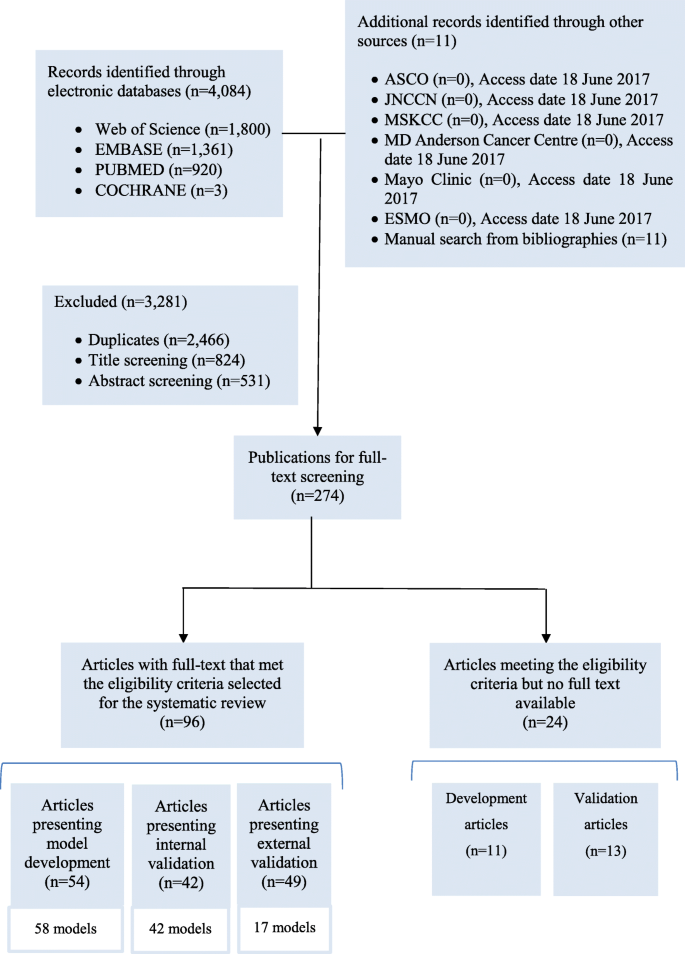
Flow diagram of the literature search process
Study characteristics
The studies were published between 1982 and 2016, mostly retrospective and hospital-based. Participants were mostly from Europe, Asia, and North America (Table 1 ) .
Of the 54 model development studies identified, 42 developed only one model, nine developed more than one model and selected the best performing model(s) [ 19 , 20 , 21 , 22 , 23 , 24 , 25 , 26 , 27 ], whereas three studies developed more than one model but did not select the best model(s) [ 28 , 29 , 30 ]. In total, we reviewed 58 models. More detailed information about each development study is presented in Additional file 4 .
Among the 42 internal validation studies, 38 developed models and validated them, while four only validated the existing models: three studies validated the Nottingham Prognostic Index (NPI) [ 31 , 32 , 33 ], and one validated the Morphometric Prognostic Index (MPI) [ 34 ] (see details in Additional file 5 ).
Of the 49 external validation studies, 38 validated the existing models only, 10 developed new models and then validated them [ 19 , 35 , 36 , 37 , 38 , 39 , 40 , 41 , 42 , 43 ], and one externally validated an existing model (Adjuvant!) and then developed a new model [ 44 ]. More detailed information about the external validation studies is presented in Additional file 6 .
Risk of bias in individual studies
The risk of bias was assessed for 54 studies in the development part (Table 2 ), and 53 studies in the validation part (Table 3 ). In all the four domains of the QUIPS tool, most studies had low or moderate risk of bias while only a small number were at high risk of bias.
Model development
Of the 58 models identified, 49 were developed independently, while nine were derived from the existing models, of which five were derived from the NPI, one from Adjuvant!, one from IBTR! (the model predicts the risk of ipsilateral breast tumour recurrence), and two from PREDICT v1.1. The version PREDICT v1.2, also called PREDICT+, added HER2 status as a predictor into the first version PREDICT v1.1 [ 35 ]. The version PREDICT v1.3 added Ki67, a nuclear protein used as a marker of cell proliferation, into PREDICT v1.2 [ 36 ].
The models predicted mortality ( n = 28), recurrence ( n = 23), or both ( n = 7), mostly based on participants in Europe ( n = 25), followed by Asia ( n = 13), North America ( n = 12), and Australia ( n = 1). Cox proportional hazards (PH) regression ( n = 32) was the most commonly used method for model development, followed by artificial neural networks ( n = 6), decision trees ( n = 4), logistic regression ( n = 3), and Bayesian methods ( n = 3). The most commonly used predictors include nodal status ( n = 49), tumour size ( n = 42), tumour grade ( n = 29), age at diagnosis ( n = 24), and oestrogen receptor (ER) status ( n = 21). The models were presented as regression formula ( n = 13), followed by online tools ( n = 8), decision trees ( n = 5), nomograms ( n = 4) and score chart ( n = 1) (Table 4 ).
Seventeen models have been externally validated by independent researchers ( n = 8) or by the model developers ( n = 15). These models were developed to support clinical decision making ( n = 14) or evaluating the prognostic value of specified clinical factors ( n = 3) (Additional file 7 ). Additional file 8 presents the characteristics of these models.
The models that were most frequently validated include Adjuvant! ( n = 17), the NPI ( n = 15), and PREDICT v1.3 ( n = 5). Among the 17 studies that externally validated Adjuvant!, three had high risk of bias in Prognostic Factor Measurements [ 35 , 45 , 46 ], one was at low risk of bias across the QUIPS domains [ 47 ], while the remaining studies had low or moderate risk of bias. Among the 15 studies that externally validated the NPI, three were at high risk of bias in Prognostic Factor Measurement [ 37 , 48 , 49 ], one was at high risk of bias in Statistical Analysis and Presentation [ 50 ], three were at low risk across the domains [ 47 , 51 , 52 ], and the rest had low or moderate risk of bias. All the five studies that externally validated PREDICT v1.3 had low or moderate risk of bias (Table 5 ).
While the web-based programmes Adjuvant! and PREDICT v1.3 estimate the possible survival time for breast cancer patients, the NPI assigns a prognostic index (PI) score to each individual patient based on the calculation (0.2x tumour size in cm) + lymph node stage + tumour grade . Originally, the NPI was developed based on the lymph node stage, but later the authors suggested that the number of involved nodes can replace the lymph node stage [ 32 ]. At the outset, a patient will be classified into one of three prognostic groups based on their NPI score: good prognostic group (PI< 3.4), moderate prognostic group (3.4 ≤ PI≤5.4), and poor prognostic group (PI> 5.4) [ 53 ]. Some validation studies of the NPI further divided the samples into six smaller prognostic groups [ 47 , 54 ].
Model validation
Internal validation.
Forty two models were internally validated by comparing the predicted outcomes to (a) the observed outcomes ( n = 20); (b) the outcomes predicted by the NPI or Adjuvant! ( n = 7); (c) the outcomes predicted by prognostic factors ( n = 4); or (d) the outcomes predicted by other newly developed models ( n = 15). The sampling methods for internal validation were cross-validation ( n = 13), random-splitting ( n = 11), or bootstrap ( n = 5); some internal validation cohorts were exactly the same to the development cohorts ( n = 13), or they were the development cohorts with longer follow-up ( n = 1), or they were specific subgroups of the development cohorts ( n = 1), or they were the combination of the development cohorts and the newly recruited patients in the same centres ( n = 1), or they were different patients from the development cohorts but in the same hospitals ( n = 1). The models were assessed for overall performance ( n = 3), calibration (the level of agreement between the predicted and observed outcomes) ( n = 12), discrimination (the extent to which a model can discriminate patients with the outcomes and those without the outcomes) ( n = 28), and clinical usefulness ( n = 13). Brier scores ( n = 2), calibration plots ( n = 7), Kaplan-Meier curves ( n = 23), and accuracy rates ( n = 11) were most commonly used to assess the models’ overall performance, calibration, discrimination, and clinical usefulness, respectively (Table 6 ) .
Overall, most models performed well in the internal validation cohorts, some even showed better performance than the existing models [ 19 , 22 , 37 , 44 , 55 , 56 ] or prognostic factors [ 43 , 53 , 57 ].
External validation
Only 17 models have been externally validated by comparing the predicted outcomes with the observed outcomes ( n = 35), with the outcomes predicted by other models ( n = 10), or with the outcomes predicted by single prognostic factors ( n = 4). Participants were recruited in countries different from the development cohorts ( n = 39) or in the same countries but different centres/sources ( n = 9). The models were assessed for overall performance ( n = 2) (using explained variation R 2 ( n = 1) and Brier score ( n = 1)); calibration ( n = 32) (mainly using calibration plots ( n = 20) and/or the comparison of the predicted (E) to the observed outcomes (O) ( n = 30)); discrimination ( n = 37) (mainly using Harrell’s C-index/AUC (Area under the Receiver operating characteristic (ROC) curve) ( n = 22), Kaplan-Meier curve ( n = 20), and/or log-rank test ( n = 18)); and clinical usefulness ( n = 2) (using accuracy rate ( n = 2) and sensitivity/specificity ( n = 1)). Some studies that compared two or more models tested the agreement between the models ( n = 4), using Kappa coefficient (κ) ( n = 1) and correlation coefficients (Pearson or Spearman) ( n = 3). Univariate ( n = 10) and multivariate analysis ( n = 6) were used to test if prognostic factors and prognostic scores were significant to outcomes (Table 6 ). A summary of the external validation studies is presented in Additional file 9 .
In general, the models performed less accurately in some independent populations, particularly in patients with high risk, in young and elderly patients. For example, Adjuvant! predicted prognosis accurately in patients from France [ 58 ], Canada [ 45 , 46 ], and those with low grade tumours, but less accurate in patients from UK [ 59 ], Ireland [ 60 ], Malaysia [ 61 ], South Korea [ 44 ], Taiwan [ 62 ], those with lympho-vascular invasion [ 45 , 61 ], BRCA1-mutation carriers [ 63 ], and those with high grade tumours [ 44 , 58 , 59 , 61 , 62 ]. Studies showed inconsistent results of Adjuvant! in patients aged 40 years or less [ 35 , 44 , 45 , 46 , 47 , 54 , 58 , 59 , 61 , 62 , 64 ] and elderly patients [ 45 , 46 , 54 , 59 , 61 , 65 , 66 ]. Similarly, PREDICT v1.3 performed well in Malaysian patients [ 67 ], but less accurately in patients with BRCA1 mutations [ 63 ], patients aged 40 years or less [ 67 ], and those with ER positive and HER2 negative tumours [ 68 ], and inconsistently in elderly patients [ 67 , 69 ]. An exception is the NPI, which performed well in most populations, including patients from Italy [ 51 , 70 , 71 ], Sweden [ 72 ], Denmark [ 48 ], Belgium [ 73 ], Norway [ 37 ], Japan [ 52 ], India [ 50 ], New Zealand [ 37 ], patients aged 40 years or less [ 47 ], metastatic patients [ 74 ], those with triple negative breast cancer [ 75 ], and those treated with neoadjuvant chemotherapy [ 49 ].
Studies that compared different models in independent datasets
In the three studies that compared the NPI and Adjuvant! conducted by independent researchers, no model was shown to be better than the other. One study showed that both models performed accurately in the overall cohort of Iranian patients, but less accurately in some subgroups [ 54 ]. Another study found that Adjuvant! showed better discrimination ability than the NPI in Irish breast cancer patients, although Adjuvant! underestimated the 10-year OS [ 60 ]. However, the third study showed that, in British breast cancer patients aged 40 years or less, the NPI’s prediction was nearly similar to the observed outcomes, while Adjuvant! seemed to overestimate the 10-year OS, although the study power was not sufficient to generate a statistically significant difference [ 47 ] (see details in Additional file 10 ).
None of the three models compared by independent researchers– PREDICT v1.3, Adjuvant!, and CancerMath– was found to be superior. In the studies that compared PREDICT v1.3 and Adjuvant!, both did not predict the 10-year OS well in BRCA1-mutation carriers [ 63 ] and in patients aged 65 years or more [ 66 , 69 ], with statistically significant differences between the predicted and observed outcomes ( P < 0.05). PREDICT v1.3 accurately predicted the 5-year OS in elderly patients, though not in all subgroups, but the authors could not compare that model with Adjuvant! because the latter did not predict the 5-year OS [ 69 ]. When PREDICT v1.3, Adjuvant!, and CancerMath were compared in patients with ER positive and HER2 negative tumours, all the three models inaccurately predicted the 10-year OS, with statistically significant differences between the predicted and observed outcomes ( P < 0.05) [ 68 ] (see details in Additional file 10 ) .
There are four studies that developed new models, and then compared them to existing models in independent datasets (see details in Additional file 11 ). In its development study, PREDICT v1.1 showed better performance than Adjuvant! in predicting 10-year breast cancer specific survival (BCSS), but poorer performance in 10-year OS in the overall cohort [ 46 ]. PREDICT v1.1 was better in some sub-groups (10-year OS in patients with grade 3 tumours, lymphovascular positive tumours, and node negative tumours; 10-year BCSS in patients with node positive tumours, tumour size > 21 mm, and ER positive tumours), whereas Adjuvant! was better in others (10-year OS in patients with tumour size > 21 mm, grade 2 tumours, and ER positive tumours; 10-year BCSS in patients with grade 3 tumours, ER negative tumours, and node negative tumours) [ 46 ]. In its development study, PREDICT v1.2 showed significantly better performance than PREDICT v1.1 and Adjuvant! in the HER2 positive subgroup, possibly because it was developed by adding HER2 status as a prognostic factor into PREDICT v1.1 [ 35 ]. However, in the overall cohort, Adjuvant! was better in predicting OS while both versions of PREDICT were better in predicting BCSS [ 35 ]. The development study of the iNPI showed that this version discriminated slightly better than the original version NPI, but the difference was not significant [ 37 ]. The development study of PREDICT v1.3 showed that this new version improved both calibration and discrimination compared to the previous version PREDICT v1.2 in patients with ER positive tumours [ 36 ].
This study reviewed 96 articles that presented the development and/or validation of prognostic models for breast cancer. To our knowledge, this is the most comprehensive review of prognostic models for breast cancer. A previous review reported only six models based on clinico-pathological factors [ 14 ]. However, our findings may be affected by publication bias [ 8 , 76 ] as well as the diversity of terms used in prognostic research [ 14 , 77 ]. The review may have missed some relevant studies that were published after December 2016, for example, PREDICT v2.0, which added age at diagnosis as a predictor into PREDICT v1.3 [ 78 ].
Due to the heterogeneity of study designs, inclusion criteria, measurement techniques, methods of analysis, and methods of handling of continuous variables, meta-analysis was not undertaken as recommended previously [ 76 , 79 ]. Instead, we assessed the risk of bias for each individual study using the modified QUIPS tool.
The original QUIPS tool was developed to assess bias in studies establishing the relationship between a prognostic factor and an outcome [ 17 ], in which confounders may play an important role. In contrast, we are interested in outcome prediction studies where causality and confounding are not a concern [ 9 ]. Therefore, we did not assess the confounding issue of the selected articles. We also omitted the domain of Study Attrition because, although most of the selected studies described attempts to track loss to follow-up to some extent, none of them reported specific information required by the QUIPS tool (including: the proportion of study sample dropping out of the study, attempts to collect their information, reasons for loss to follow-up, their key characteristics, and if these characteristics are different from those who completed the study [ 17 ]).
We found that most studies were at moderate or low risk of bias, which contrasts with the findings in other systematic reviews that most studies were at poor quality [ 11 , 77 ]. However, the previous reviews did not report the detailed quality assessment of each study.
Most studies included in this review used a retrospective design, and therefore had issues related to missing data and a lack of consistency in predictor and outcome measurement [ 9 , 11 , 77 ]. Prospective cohort studies have been suggested as the best design for predictive modelling because they enable not only clear and consistent definitions but also prospective measurement of predictors and outcomes [ 3 , 9 ].
Similar to the previous systematic reviews [ 8 , 77 , 80 , 81 ], we found that most studies (59%) did not report, or did not satisfy the suggested minimum requirements for the numbers of events, i.e., 10 events per candidate variable for model development studies, and 100 events for model validation studies [ 11 , 82 , 83 , 84 , 85 , 86 , 87 ]. A small number of events could mislead the results of validation measures, for example, misleadingly high value of the C-index [ 85 ].
We found that the most commonly used prognostic factors in the models were nodal status, tumour size, and tumour grade, followed by age at diagnosis and ER status, as reported in other reviews [ 11 , 88 ]. The NPI was one of the simplest and oldest models, and included only nodal status, tumour size, and tumour grade. There are several attempts to improve the prognostic values of the NPI by adding other novel predictors, such as age at diagnosis [ 89 ], hormonal receptor status [ 37 , 89 , 90 ], and HER2 status [ 37 , 55 , 90 , 91 ]. However, such modification has not been proven to be better than the NPI in independent populations. Future research may evaluate the added prognostic value of other important variables to the NPI and other models.
The use of gene expression or novel biomolecular factors is increasing due to their potential to provide molecular phenotyping that recognises distinct tumour categorisations not evident by traditional factors [ 92 , 93 ]. However, we excluded models based on genetic profiles or novel biomolecular factors because these factors are not yet widely adopted in clinical practice. Additionally, since models that include both genetic and traditional factors are suggested to be superior to those based on either set of features alone [ 94 , 95 ], studies of the prognostic value of any new marker should look at the extra benefit of including it when traditional clinico-pathological variables are also included.
The most commonly used method for model development was Cox PH regressions as reported in other reviews [ 11 , 96 ]. Cox PH regressions are simple but have been criticised because the PH assumption may not always hold, since the strengths of prognostic factors change over time in the “real world” [ 19 , 29 , 97 ]. To address this, alternative methods such as artificial neural networks, support vector machines, or multistate models have been applied. These models may perform better than Cox PH models but have not been validated in independent populations, limiting generalisability [ 22 , 23 , 24 , 26 ]. Furthermore, clinical validity is more important than statistical validity [ 11 ]. As the models developed based on Cox PH regressions, such as the NPI or PREDICT, showed good performance in many populations, Cox PH regressions will still dominate the literature on model development methods.
Differences in the methodological issues pointed out in our review may be explained by differences in the purpose of developing the model (e.g., to support clinical decision making, to evaluate the prognostic value of a specific factor, or to compare statistical methods used to develop the model). However, not many developers explicitly stated the purposes of their models. Nevertheless, the models that have gone to further external validation were developed mainly to support clinical decision making. These models were considered useful in clinical practice.
Only one of 49 external validation studies in our review tested “clinical usefulness”, which was defined by the authors as the ability for a model to classify patients into low risk and high risk groups better than without that model, and the measure used was accuracy rate [ 98 ]. However, a model’s ability to classify patients into two risk groups may not reflect its usefulness in clinical settings. A prognostic model can be useful if it classifies patients into more than two risk groups to influence therapy or to save patients from unnecessary treatments or to estimate survival time for patients [ 8 ]. Future research may consider more relevant measures to assess clinical usefulness such as the improvement of clinical decision making when applying a model, patients’ insights about model reports, or how doctors communicate with patients about model results.
Previous reviews reported that Hosmer-Lemeshow goodness-of-fit test was used most frequently to test the deviations in calibration plots [ 77 , 81 ] but we found that the difference between the predicted and observed outcomes was more commonly used (Table 6 ). Steyerberg and Vergouwe (2014) did not recommend the Hosmer-Lemeshow goodness-of-fit test because it only provides a p -value instead of providing the direction and magnitude of miscalibration [ 99 ]. This test has also been criticised for being arbitrary and imprecise as the p-value is dependent on miscalibration and sample size [ 99 ]. Instead, Steyerberg and Vergouwe (2014) advocated the use of the intercept of the calibration plot, also called calibration-in-the-large [ 99 ], which is closely related to the difference between the predicted and observed outcomes, either absolute or relative difference [ 100 ].
We found that C-index/AUC was the most commonly used method to assess discrimination, followed by Kaplan-Meier curves and log-rank tests, as reported in previous systematic reviews in several clinical fields [ 9 , 77 , 96 ]. Log-rank tests were not recommended because they do not give an estimate of the magnitude of the separation of the risk groups [ 96 ]. In contrast, C-index, or AUC for a binary endpoint, was advocated by several authors [ 99 ].
This review focused on models that have been externally validated in several settings by independent researchers for many reasons. Firstly, external validation is preferable to internal validation to test a model’s transportability as the case-mix (or the distribution of predictors) in an independent population is unlikely to be identical with that in the model development population [ 85 ]. Secondly, to enhance the generalisability of a model, it should ideally be validated in different settings with diversity of case-mixes [ 85 ]. A model with good performance in diverse settings is more likely to be generalisable to a plausibly related, but untested population [ 13 , 85 , 86 ]. Finally, a reliable model should be tested by independent researchers in different settings [ 8 , 101 ]. If model development and external validation are undertaken by the same researchers, there may be a temptation to revise the model to fit the external validation data [ 8 ]. A clear distinction between the external validation studies conducted by independent researchers and by model developers should be made to reduce inflated findings and “spin” [ 102 , 103 , 104 ].
The studies that compared Adjuvant!, CancerMath, PREDICT v1.3, and the NPI in independent datasets by independent researchers did not find the superiority of one model over the others. When they were validated individually, only the NPI performed well in most independent populations, whereas the other models were accurate in just some populations. The NPI has been advocated by several authors and is one of the few models that are used in clinical practice [ 11 ]. The advantage of the NPI is its simplicity, which is an important criterion in developing a useful model [ 105 ]. Additionally, the model shows good reducibility and transportability because it performed well in diverse settings when validated by independent researchers. The model has good discrimination in most populations, and is therefore clinically useful because it classifies patients into risk groups to influence therapy or save patients from unnecessary treatments [ 8 , 11 ]. However, most studies that validated the NPI only assessed its discrimination but not calibration, because the model cannot estimate prognosis of individual patients. Some studies assigned OS for all patients in the same NPI group based on previous reports [ 47 , 54 , 73 ]. This practice is criticised as inappropriate, since estimates based on data at a period in the past are probably not well calibrated for patients today. Advanced treatments, such as hormonal therapies or targeted therapies, in addition to improvement in detection and diagnosis, may improve the survival within the NPI groups [ 106 ]. Regular updates would be required for better prediction of prognosis for each group.
The performance of a particular model may vary across different populations. For example, the NPI, a UK-based model, performed well in most countries in Europe (Italy, Sweden, Denmark, Belgium, Norway), and even in Asia (Japan, India), but was less accurate in Irish patients. The US-based model Adjuvant! showed good performance in a large Dutch population, but poor performance in patients from the UK or Asia (Malaysia, South Korea, Taiwan). Therefore, a reliable validation study should be conducted before a model is applied in other populations.
Most studies in our review showed that models were less accurate in patients aged under 40 years or over 65 years, although some studies showed opposite results. Likewise, a previous review concluded that Adjuvant! was less accurate in young and elderly patients in most studies [ 14 ]. However, most validation studies lack generalisability because they were based on small numbers of events or did not report the numbers of events. Only a few studies with appropriate numbers of events were designed to assess models’ performance in young and elderly patients only. These studies found that PREDICT v1.3 was less accurate in predicting 10-year OS [ 69 ], whereas Adjuvant! overpredicted 10-year OS and event-free survival (EFS) in Dutch elderly patients [ 66 ]. Nonetheless, it is difficult to know if the poor performance of models in young and elderly patients was attributable to age only, or to other effect modifiers such as ethnicity.
We reviewed the development and/or validation of 58 models predicting mortality and/or recurrence for female breast cancer. These models varied in terms of methods of development and/or validation, predictors, outcomes, and patients included. Most models have been developed in Europe, Asia, and North America. We found that models performed well in internal validation cohorts, but the results were unpredictable in external validation cohorts, especially in young and elderly patients, and in high risk patients. NPI is an exception, which performed well in most independent populations. Therefore, models should be validated before being applied in another population.
Abbreviations
- Adjuvant!Online
Akaike information criterion
Area under the curve
Breast cancer-specific survival
Bayesian information criterion
Cox proportional hazards regression
Concordance probability estimate
Disease-free survival
Event-free survival
Oestrogen receptor
Human epidermal growth factor receptor 2
Ipsilateral breast tumour recurrence
Local-regional recurrence
Mitotic Activity Index
Morphometric Prognostic Index
Nottingham Prognostic Index
Negative predictive value
Overall survival
Positive predictive value
Progesterone receptor
QUality In Prognosis Studies
Randomised controlled trial
Relapse-free survival
Receiver operating characteristic
Standard deviation
Standard error
Standardised mortality ratio
The United Kingdom
The United States of America
Tumour, Node, and Metastasis
Coleman MP, Quaresma M, Berrino F, Lutz J, de Angelis R, Capocaccia R, Baili P, Rachet B, Gatta G, Hakulinen T. Cancer survival in five continents: a worldwide population-based study (CONCORD). Lancet Oncol. 2008;9(8):730–56.
Article PubMed Google Scholar
Polyak K. Heterogeneity in breast cancer. J Clin Invest. 2011;121(10):3786–8.
Article CAS PubMed PubMed Central Google Scholar
Moons KG, Royston P, Vergouwe Y, Grobbee DE, Altman DG. Prognosis and prognostic research: what, why, and how? BMJ. 2009;338:b375.
Buchholz TA, Strom EA, McNeese MD. In: Cox JD, Ang KK, editors. The breast. In Radiation oncology: Rationale, technique, results. St. Louis, Missouri: Mosby; 2003. p. 333–86.
Google Scholar
Blamey R, Ellis I, Pinder S, Lee A, Macmillan R, Morgan D, Robertson J, Mitchell M, Ball G, Haybittle J. Survival of invasive breast cancer according to the Nottingham prognostic index in cases diagnosed in 1990–1999. Eur J Cancer. 2007;43(10):1548–55.
Article CAS PubMed Google Scholar
Hagerty RG, Butow PN, Ellis PA, Lobb EA, Pendlebury S, Leighl N, Goldstein D, Lo SK, Tattersall MH. Cancer patient preferences for communication of prognosis in the metastatic setting. J Clin Oncol. 2004;22(9):1721–30.
Lobb EA, Kenny DT, Butow PN, Tattersall MH. Women’s preferences for discussion of prognosis in early breast cancer. Health Expect. 2001;4(1):48–57.
Altman DG, Royston P. What do we mean by validating a prognostic model? Stat Med. 2000;19(4):453–73.
3.0.CO;2-5" data-track-action="article reference" href="https://doi.org/10.1002%2F%28SICI%291097-0258%2820000229%2919%3A4%3C453%3A%3AAID-SIM350%3E3.0.CO%3B2-5" aria-label="Article reference 8" data-doi="10.1002/(SICI)1097-0258(20000229)19:4 3.0.CO;2-5">Article CAS PubMed Google Scholar
Steyerberg EW. Clinical prediction models: a practical approach to development, validation, and updating. New York: Springer Science & Business Media; 2008.
Eskelinen M, Lipponen P, Papinaho S, Aaltomaa S, Kosma VM, Klemi P, Syrjanen K. DNA flow-cytometry, nuclear morphometry, mitotic indexes and steroid-receptors as independent prognostic factors in female breast cancer. Int J Cancer. 1992;51(4):555–61.
Altman DG. Prognostic models: a methodological framework and review of models for breast cancer. Cancer Investig. 2009;27(3):235–43.
Article Google Scholar
Clark GM. Do we really need prognostic factors for breast cancer? Breast Cancer Res Treat. 1994;30(2):117–26.
Justice AC, Covinsky KE, Berlin JA. Assessing the generalisability of prognostic information. Ann Intern Med. 1999;130(6):515–24.
Engelhardt EG, Garvelink MM, de Haes JH, Van der Hoeven JJ, Smets EM, Pieterse AH, Stiggelbout AM. Predicting and communicating the risk of recurrence and death in women with early-stage breast cancer: a systematic review of risk prediction models. J Clin Oncol. 2013;32(3):238–50.
Collins GS, Reitsma JB, Altman DG, Moons KGM. Transparent reporting of a multivariable prediction model for individual prognosis or diagnosis (TRIPOD): the TRIPOD statement. BMC Med. 2015;13(1):1.
Article PubMed PubMed Central Google Scholar
Moons KG, de Groot JAH, Bouwmeester W, Vergouwe Y, Mallett S, Altman DG, Reitsma JB, Collins GS. Critical appraisal and data extraction for systematic reviews of prediction modelling studies: the CHARMS checklist. PLoS Med. 2014;11(10):e1001744.
Hayden JA, Van der Windt DA, Cartwright JL, Côté P, Bombardier C. Assessing bias in studies of prognostic factors. Ann Intern Med. 2013;158(4):280–6.
Hayden JA, Côté P, Bombardier C. Evaluation of the quality of prognosis studies in systematic reviews. Ann Intern Med. 2006;144(6):427–37.
Campbell HE, Gray AM, Harris AL, Briggs AH, Taylor MA. Estimation and external validation of a new prognostic model for predicting recurrence-free survival for early breast cancer patients in the UK. Br J Cancer. 2010;103(6):776–86.
Chao CM, Yu YW, Cheng BW, Kuo YL. Construction the model on the breast cancer survival analysis use support vector machine, logistic regression and decision tree. J Med Syst. 2014;38:106.
Fleming ST, Rastogi A, Dmitrienko A, Johnson KD. A comprehensive prognostic index to predict survival based on multiple comorbidities: a focus on breast cancer. Med Care. 1999;37(6):601–14.
Kim W, Kim KS, Lee JE, Noh DY, Kim SW, Jung YS, Park MY, Park RW. Development of novel breast cancer recurrence prediction model using support vector machine. J Breast Cancer. 2012;15(2):230–8.
Lisboa PJG, Wong H, Harris P, Swindell R. A Bayesian neural network approach for modelling censored data with an application to prognosis after surgery for breast cancer. Artif Intell Med. 2003;28(1):1–25.
Ture M, Tokatli F, Omurlu IK. The comparisons of prognostic indexes using data mining techniques and cox regression analysis in the breast cancer data. Expert Syst Appl. 2009;36(4):8247–54.
Choi JP, Han TH, Park RW. A hybrid Bayesian network model for predicting breast cancer prognosis. J Kor Soc Med Informatics. 2009;15(1):49–57.
Jerez JM, Franco L, Alba E, Llombart-Cussac A, Lluch A, Ribelles N, Munarriz B, Martin M. Improvement of breast cancer relapse prediction in high risk intervals using artificial neural networks. Breast Cancer Res Treat. 2005;94(3):265–72.
Delen D, Walker G, Kadam A. Predicting breast cancer survivability: a comparison of three data mining methods. Artif Intell Med. 2005;34(2):113–27.
Tokatli ZF, Ture M, Kurt Omurlu I, Csar Alas R, Uzal MC. Developing and comparing two different prognostic indexes for predicting disease-free survival of nonmetastatic breast cancer patients. Turkish J Med Sci. 2011;41(5):769–80.
Ripley RM, Harris AL, Tarassenko L. Neural network models for breast cancer prognosis. Neural Comput Applic. 1998;7(4):367–75.
Cheng SH, Horng CF, Clarke JL, Tsou MH, Tsai SY, Chen CM, Jian JJ, Liu MC, West M, Huang AT, Prosnitz LR. Prognostic index score and clinical prediction model of local regional recurrence after mastectomy in breast cancer patients. Int J Radiat Oncol Biol Phys. 2006;64(5):1401–9.
Kollias J, Murphy CA, Elston CW, Ellis IO, Robertson JFR, Blamey RW. The prognosis of small primary breast cancers. Eur J Cancer. 1999;35(6):908–12.
Galea MH, Blamey RW, Elston CE, Ellis IO. The Nottingham prognostic index in primary breast cancer. Breast Cancer Res Treat. 1992;22(3):207–19.
Todd JH, Dowle C, Williams MR, Elston CW, Ellis IO, Hinton CP, Blamey RW, Haybittle JL. Confirmation of a prognostic index in primary breast cancer. Br J Cancer. 1987;56(4):489–92.
van Diest PJ, Baak JP. The morphometric prognostic index is the strongest prognosticator in premenopausal lymph node-negative and lymph node-positive breast cancer patients. Hum Pathol. 1991;22(4):326–30.
Wishart GC, Bajdik CD, Dicks E, Provenzano E, Schmidt MK, Sherman M, Greenberg DC, Green AR, Gelmon KA, Kosma VM, Olson JE, Beckmann MW, Winqvist R, Cross SS, Severi G, Huntsman D, Pylkas K, Ellis I, Nielsen TO, Giles G, Blomqvist C, Fasching PA, Couch FJ, Rakha E, Foulkes WD, Blows FM, Begin LR, van’t Veer LJ, Southey M, Nevanlinna H, Mannermaa A, Cox A, Cheang M, Baglietto L, Caldas C, Garcia-Closas M, PDP P. PREDICT Plus: Development and validation of a prognostic model for early breast cancer that includes HER2. Br J Cancer. 2012;107(5):800–7.
Wishart GC, Rakha E, Green A, Ellis I, Ali HR, Provenzano E, Blows FM, Caldas C, Pharoah PDP. Inclusion of Ki67 significantly improves performance of the PREDICT prognostication and prediction model for early breast cancer. Eur J Surg Oncol. 2014;40(5):607.
van Belle V, van Calster B, Brouckaert O, Bempt IV, Pintens S, Harvey V, Murray P, Naume B, Wiedswang G, Paridaens R, Moerman P, Amant F, Leunen K, Smeets A, Drijkoningen M, Wildiers H, Christiaens M, Vergote I, Van Huffel S, Neven P. Qualitative assessment of the progesterone receptor and HER2 improves the Nottingham prognostic index up to 5 years after breast Cancer diagnosis. J Clin Oncol. 2010;28(27):4129–34.
Sanghani M, Truong PT, Raad RA, Niemierko A, Lesperance M, Olivotto IA, Wazer DE, Taghian AG. Validation of a web-based predictive nomogram for ipsilateral breast tumour recurrence after breast conserving therapy. J Clin Oncol. 2010;28(5):718–22.
Witteveen A, Vliegen IMH, Sonke GS, Klaase JM, Jzerman MJ, Siesling S. Personalisation of breast cancer follow-up: a time-dependent prognostic nomogram for the estimation of annual risk of locoregional recurrence in early breast cancer patients. Breast Cancer Res Treat. 2015;152(3):627–36.
Wishart GC, Azzato EM, Greenberg DC, Rashbass J, Kearins O, Lawrence G, Caldas C, Pharoah PDP. PREDICT: a new UK prognostic model that predicts survival following surgery for invasive breast cancer. Breast Cancer Res. 2010;12:R1.
Chen YC, Lai HW, Wang WC, Kuo YL. Validation of breast Cancer survival prediction model with SEER database. J Integr Oncol. 2016;5(3):174.
Michaelson JS, Chen LL, Bush D, Fong A, Smith B, Younger J. Improved web-based calculators for predicting breast carcinoma outcomes. Breast Cancer Res Treat. 2011;128(3):827–35.
de Laurentiis M, de Placido S, Bianco AR, Clark GM, Ravdin PM. A prognostic model that makes quantitative estimates of probability of relapse for breast cancer patients. Clin Cancer Res. 1999;5(12):4133–9.
PubMed Google Scholar
Jung M, Choi EH, Nam CM, Rha SY, Jeung HC, Lee SH, Yang WI, Roh JK, Chung HC. Application of the adjuvant!Online model to Korean breast cancer patients: an assessment of prognostic accuracy and development of an alternative prognostic tool. Ann Surg Oncol. 2013;20(8):2615–24.
Olivotto IA, Bajdik CD, Ravdin PM, Speers CH, Coldman AJ, Norris BD, Davis GJ, Chia SK, Gelmon KA. Population-based validation of the prognostic model adjuvant! For early breast cancer. J Clin Oncol. 2005;23(12):2716–25.
Wishart GC, Bajdik CD, Azzato EM, Dicks E, Greenberg DC, Rashbass J, Caldas C, Pharoah PDP. A population-based validation of the prognostic model PREDICT for early breast cancer. Eur J Surg Oncol. 2011;37(5):411–7.
Hearne BJ, Teare MD, Butt M, Donaldson L. Comparison of Nottingham prognostic index and adjuvant!Online prognostic tools in young women with breast cancer: review of a single-institution experience. BMJ Open. 2015;5(1):e005576.
Balslev I, Axelsson CK, Zedeler K, Rasmussen BB, Carstensen B, Mouridsen HT. The Nottingham prognostic index applied to 9,149 patients from the studies of the Danish breast Cancer cooperative group (DBCG). Breast Cancer Res Treat. 1994;32(3):281–90.
Chollet P, Amat S, Belembaogo E, Cure H, de Latour M, Dauplat J, Le Bouedec G, Mouret-Reynier MA, Ferriere JP, Penault-Llorca F. Is Nottingham prognostic index useful after induction chemotherapy in operable breast cancer? Br J Cancer. 2003;89(7):1185–91.
Yadav R, Sen R, Chauhan P. Calculation of NPI score: prognosis of breast cancer. Indian J Public Health Res Dev. 2015;6(2):199–202.
D'Eredita G, Giardina C, Martellotta M, Natale T, Ferrarese F. Prognostic factors in breast cancer: the predictive value of the Nottingham prognostic index in patients with a long-term follow-up that were treated in a single institution. Eur J Cancer. 2001;37(5):591–6.
Okugawa H, Yamamoto D, Uemura Y, Sakaida N, Yamada M, Tanaka K, Kamiyama Y. Prognostic factors in breast cancer: the value of the Nottingham prognostic index for patients treated in a single institution. Surg Today. 2009;8(39):738.
Haybittle JL, Blamey RW, Elston CW, Johnson J, Doyle PJ, Campbell FC, Nicholson RI, Griffiths K. A prognostic index in primary breast cancer. Br J Cancer. 1982;45(3):361–6.
Rejali M, Tazhibi M, Mokarian F, Gharanjik N, Mokarian R. The performance of the Nottingham prognosis index and the adjuvant!Online decision making tool for prognosis in early-stage breast Cancer patients. Int J Prev Med. 2015;6:93.
Masarwah A, Auvinen P, Sudah M, Dabravolskaite V, Arponen O, Sutela A, Oikari S, Kosma VM, Vanninen R. Prognostic contribution of mammographic breast density and HER2 overexpression to the Nottingham prognostic index in patients with invasive breast cancer. BMC Cancer. 2016;16:833.
Article PubMed PubMed Central CAS Google Scholar
Fan YP, Liu CL, Chiang IJ, Lin CY. Development of a prognostic nomogram for identifying those factors which influence the 2- and 5-year survival chances of Taiwanese women diagnosed with breast cancer. Eur J Cancer Care. 2011;20(5):620–6.
Wen J, Ye F, He X, Li S, Huang X, Xiao X, Xie X. Development and validation of a prognostic nomogram based on the log odds of positive lymph nodes (LODDS) for breast cancer. Oncotarget. 2016;7(15):21046–53.
Hajage D, de Rycke Y, Bollet M, Savignoni A, Caly M, Pierga J, Horlings HM, Van de Vijver MJ, Vincent-Salomon A, Sigal-Zafrani B. External validation of Adjuvant!Online breast cancer prognosis tool. Prioritising recommendations for improvement. PLoS One. 2011;6(11):e27446.
Campbell HE, Taylor MA, Harris AL, Gray AM. An investigation into the performance of the adjuvant!Online prognostic programme in early breast cancer for a cohort of patients in the United Kingdom. Br J Cancer. 2009;101(7):1074–84.
Quintyne KI, Woulfe B, Coffey JC, Gupta RK. Correlation between Nottingham prognostic index and adjuvant!Online prognostic tools in patients with early-stage breast cancer in mid-Western Ireland. Clin Breast Cancer. 2013;13(4):233–8.
Bhoo-Pathy N, Yip C, Hartman M, Saxena N, Taib NA, Ho G, Looi L, Bulgiba AM, van der Graaf Y, Verkooijen HM. Adjuvant!Online is overoptimistic in predicting survival of Asian breast cancer patients. Eur J Cancer. 2012;48(7):982–9.
Kuo Y, Chen D, Change T. Accuracy validation of adjuvant!Online in Taiwanese breast cancer patients: a 10-year analysis. BMC Med Inf Decis Mak. 2012;12(1):108.
Plakhins G, Irmejs A, Gardovskis A, Subatniece S, Liepniece-Karele I, Purkalne G, Teibe U, Trofimovics G, Miklasevics E, Gardovskis J. Underestimated survival predictions of the prognostic tools adjuvant!Online and PREDICT in BRCA1-associated breast cancer patients. Familial Cancer. 2013;12(4):683.
Mook S, Schmidt MK, Rutgers EJ, van de Velde AO, Visser O, Rutgers SM, Armstrong N, van’t Veer LJ, Ravdin PM. Calibration and discriminatory accuracy of prognosis calculation for breast cancer with the online adjuvant! Program: a hospital-based retrospective cohort study. Lancet Oncol. 2009;10(11):1070–6.
Mojir Sheibani K, Novin K, Tabatabaeefar M, Azadeh P, Mafi AR, Moghadam S, Kamal FH, Motlagh AG. The validation study of adjuvant!Online using Iranian breast cancer data. Rep Radiother Oncol. 2013;1(3):97–102.
de Glas NA, van de Water W, Engelhardt EG, Bastiaannet E, de Craen AJM, Kroep JR, Putter H, Stiggelbout AM, Weijl NI, van de Velde CJH. Validity of adjuvant!Online program in older patients with breast cancer: a population-based study. Lancet Oncol. 2014;15(7):722–9.
Wong HS, Subramaniam S, Alias Z, Taib NA, Ho GF, Ng CH, Yip CH, Verkooijen HM, Hartman M, Bhoo-Pathy N. The predictive accuracy of PREDICT: a personalized decision-making tool for southeast Asian women with breast cancer. Medicine (Baltimore). 2015;94(8):e593.
Laas E, Mallon P, Delomenie M, Gardeux V, Pierga JY, Cottu P, Lerebours F, Stevens D, Rouzier R, Reyal F. Are we able to predict survival in ER-positive HER2-negative breast cancer? A comparison of web-based models. Br J Cancer. 2015;112(5):912–7.
de Glas NA, Bastiaannet E, Engels CC, de Craen AJM, Putter H, van de Velde CJH, Hurria A, Liefers GJ, JEA P. Validity of the online PREDICT tool in older patients with breast cancer: a population-based study. Br J Cancer. 2016;114(4):395–400.
Megha T, Neri A, Malagnino V, Caruso S, Onorati M, Roviello F, Tosi P. Traditional and new prognosticators in breast cancer: Nottingham index, Mib-1 and oestrogen receptor signalling remain the best predictors of relapse and survival in a series of 289 cases. Cancer Biol Ther. 2010;9(4):266–73.
Sidoni A, Bellezza G, Cavaliere A, Del Sordo R, Scheibel M, Bucciarelli E. Prognostic indexes in breast cancer: comparison of the Nottingham and Adelaide indexes. Breast. 2004;13(1):23–7.
Sundquist M, Thorstenson S, Brudin L, Nordenskjöld B. Applying the Nottingham prognostic index to a Swedish breast cancer population. Breast Cancer Res Treat. 1999;53(1):1–8.
van Belle V, Decock J, Hendrickx W, Brouckaert O, Pintens S, Moerman P, Wildiers H, Paridaens R, Christiaens MR, Van Huffel S, Neven P. Short-term prognostic index for breast Cancer: NPI or Lpi. Pathol Res Int. 2010;2011:918408.
Liu M, Huang W, Wang A, Huang C, Huang C, Chang T, Pi C, Yang H. Prediction of outcome of patients with metastatic breast cancer: evaluation with prognostic factors and Nottingham prognostic index. Support Care Cancer. 2010;18(12):1553–64.
Albergaria A, Ricardo S, Milanezi F, Carneiro V, Amendoeira I, Vieira D, Cameselle-Teijeiro J, Schmitt F. Nottingham prognostic index in triple-negative breast cancer: a reliable prognostic tool? BMC Cancer. 2011;11(1):299.
Thompson SG. Why and how sources of heterogeneity should be investigated. In: Egger M, Smith GD, Altman DG, editors. Systematic reviews in health care: meta-analysis in context. 2nd ed. London: BMJ; 2001. p. 157–75.
Chapter Google Scholar
Collins GS, de Groot JA, Dutton S, Omar O, Shanyinde M, Tajar A, Voysey M, Wharton R, Yu L, Moons KG. External validation of multivariable prediction models: a systematic review of methodological conduct and reporting. BMC Med Res Methodol. 2014;14(1):40.
dos Reis FJ C, Wishart GC, Dicks EM, Greenberg D, Rashbass J, Schmidt MK, van den Broek AJ, Ellis IO, Green A, Rakha E, Maishman T, Eccles DM, PDP P. An updated PREDICT breast cancer prognostication and treatment benefit prediction model with independent validation. Breast Cancer ReS. 2017;19(1):58.
Blettner M, Sauerbrei W, Schlehofer B, Scheuchenpflug T, Friedenreich C. Traditional reviews, meta-analyses and pooled analyses in epidemiology. Int J Epidemiol. 1999;28(1):1–9.
Mallett S, Royston P, Dutton S, Waters R, Altman DG. Reporting methods in studies developing prognostic models in cancer: a review. BMC Med. 2010;8(1):20.
Bouwmeester W, Zuithoff NPA, Mallett S, Geerlings MI, Vergouwe Y, Steyerberg EW, Altman DG, Moons KGM. Reporting and methods in clinical prediction research: a systematic review. PLoS Med. 2012;9(5):e1001221.
Article PubMed Central Google Scholar
Schumacher M, Hollaander N, Schwarzer G, Sauerbrei W. Prognostic factor studies. In: Crowley J, editor. Handbook of Statistics in Clinical Oncology. New York: Marcel Dekker; 2001. p. 321–78.
Harrell FE Jr, Lee KL, Matchar DB, Reichert TA. Regression models for prognostic prediction: advantages, problems, and suggested solutions. Cancer Treat Rep. 1985;69(10):1071–7.
Feinstein AR. Multivariable analysis: an introduction. New Haven: Yale University press; 1996.
Book Google Scholar
Collins GS, Ogundimu EO, Altman DG. Sample size considerations for the external validation of a multivariable prognostic model: a resampling study. Stat Med. 2016;35(2):214–26.
Vergouwe Y, Moons KGM, Steyerberg EW. External validity of risk models: use of benchmark values to disentangle a case-mix effect from incorrect coefficients. Am J Epidemiol. 2010;172(8):971–80.
Peek N, Arts DGT, Bosman RJ, van der Voort PHJ, de Keizer NF. External validation of prognostic models for critically ill patients required substantial sample sizes. J Clin Epidemiol. 2007;60(5):491–501.
Cianfrocca M, Goldstein LJ. Prognostic and predictive factors in early-stage breast cancer. Oncologist. 2004;9(6):606–16.
Hawkins RA, Tesdale AL, Prescott RJ, Forster T, McIntyre MA, Baker P, Jack WJL, Chetty U, Dixon JM, Killen ME, Hulme MJ, Miller WR. Outcome after extended follow-up in a prospective study of operable breast cancer: key factors and a prognostic index. Br J Cancer. 2002;87(1):8–14.
Rakha EA, Soria D, Green AR, Lemetre C, Powe DG, Nolan CC, Garibaldi JM, Ball G, Ellis IO. Nottingham prognostic index plus (NPI+): a modern clinical decision making tool in breast cancer. Br J Cancer. 2014;110(7):1688–97.
Suen D, Chow LWC. Prognostic contribution of the HER-2 oncogene overexpression to the Nottingham prognostic index in breast cancer. Biomed Pharmacother. 2006;60(6):293–7.
West M, Blanchette C, Dressman H, Huang E, Ishida S, Spang R, Zuzan H, Olson JA Jr, Marks JR, Nevins JR. Predicting the clinical status of human breast cancer by using gene expression profiles. Proc Natl Acad Sci U S A. 2001;98(20):11462–7.
Huang E, Cheng SH, Dressman H, Pittman J, Tsou MH, Horng CF, Bild A, Iversen ES, Liao M, Chen CM. Gene expression predictors of breast cancer outcomes. Lancet. 2003;361(9369):1590–6.
Parisi F, González AM, Nadler Y, Camp RL, Rimm DL, Kluger HM, Kluger Y. Benefits of biomarker selection and clinico-pathological covariate inclusion in breast cancer prognostic models. Breast Cancer Res. 2010;12(5):R66.
Tang G, Cuzick J, Costantino JP, Dowsett M, Forbes JF, Crager M, Mamounas EP, Shak S, Wolmark N. Risk of recurrence and chemotherapy benefit for patients with node-negative, oestrogen receptor–positive breast cancer: recurrence score alone and integrated with pathologic and clinical factors. J Clin Oncol. 2011;29(33):4365–72.
Mallett S, Royston P, Waters R, Dutton S, Altman DG. Reporting performance of prognostic models in cancer: a review. BMC Med. 2010;8(1):21.
Gomez-Ruiz JA, Jerez-Aragones JM, Munoz-Perez J, Alba-Conejo E. A neural network based model for prognosis of early breast cancer. Appl Intell. 2004;20(3):231–8.
Collan Y, Kumpusalo L, Pesonen E, Eskelinen M, Pajarinen P, Kettunen K. Prediction of survival in breast cancer: evaluation of different multivariate models. Anticancer Res. 1998;18(1B):647–50.
CAS PubMed Google Scholar
Steyerberg EW, Vergouwe Y. Towards better clinical prediction models: seven steps for development and an ABCD for validation. Eur Heart J. 2014;35(29):1925–31.
Riley RD, Ensor J, Snell KI, Debray TP, Altman DG, Moons KG, Collins GS. External validation of clinical prediction models using big datasets from e-health records or IPD meta-analysis: opportunities and challenges. BMJ. 2016;353:i3140.
Laupacis A, Sekar N. Clinical prediction rules: a review and suggested modifications of methodological standards. JAMA. 1997;277(6):488–94.
Boutron I, Dutton S, Ravaud P, Altman DG. Reporting and interpretation of randomised controlled trials with statistically nonsignificant results for primary outcomes. JAMA. 2010;303(20):2058–64.
Kuehn BM. Striving for a more perfect peer review: editors confront strengths, flaws of biomedical literature. JAMA. 2013;310(17):1781–3.
Ochodo EA, de Haan MC, Reitsma JB, Hooft L, Bossuyt PM, Leeflang MM. Overinterpretation and misreporting of diagnostic accuracy studies: evidence of “spin”. Radiology. 2013;267(2):581–8.
Altman DG, Vergouwe Y, Royston P, Moons KG. Prognosis and prognostic research: validating a prognostic model. BMJ. 2009;338:b605.
Lundin J. The Nottingham prognostic index- from relative to absolute risk prediction. Eur J Cancer. 2007;43(10):1498–500.
Asare EA, Liu L, Hess KR, Gordon EJ, Paruch JL, Palis B, Dahlke AR, McCabe R, Cohen ME, Winchester DP, Bilimoria KY. Development of a model to predict breast cancer survival using data from the National Cancer Data Base. Surgery. 2016;159(2):495–502.
Baak J, van Dop H, Kurver PHJ, Hermans JO. The value of morphometry to classic prognosticators in breast cancer. Cancer. 1985;56(2):374–82.
3.0.CO;2-9" data-track-action="article reference" href="https://doi.org/10.1002%2F1097-0142%2819850715%2956%3A2%3C374%3A%3AAID-CNCR2820560229%3E3.0.CO%3B2-9" aria-label="Article reference 108" data-doi="10.1002/1097-0142(19850715)56:2 3.0.CO;2-9">Article CAS PubMed Google Scholar
Broet P, de la Rochefordiere A, Scholl SM, Fourquet A, de Rycke Y, Pouillart P, Mosseri V, Asselain B. Analysing prognostic factors in breast cancer using a multistate model. Breast Cancer Res Treat. 1999;54(1):83–9.
Brown JM, Benson EA, Jones M. Confirmation of a long-term prognostic index in breast cancer. Breast. 1993;2(3):144–7.
Bryan RM, Mercer RJ, Bennett RC, Rennie GC. Prognostic factors in breast cancer and the development of a prognostic index. Br J Surg. 1986;73(4):267–71.
Bucinski A, Baczek T, Kaliszan R, Nasal A, Krysinski J, Zaluski J. Artificial neural network analysis of patient and treatment variables as a prognostic tool in breast cancer after mastectomy. Adv Clin Exp Med. 2005;14(5):973–9.
Collan Y, Eskelinen MJ, Nordling SA, Lipponen P, Pesonen E, Kumpusalo LM, Pajarinen P, Kettunen KO. Prognostic studies in breast cancer: multivariate combination of nodal status, proliferation index, tumour size, and DNA ploidy. Acta Oncol. 1994;33(8):873–8.
Fuster E, Garcia-Vilanova A, Narbona B, Romero R, Llombart-Bosch A. A statistical approach to an individualized prognostic index (IPI) for breast cancer survivability. Cancer. 1983;52(4):728–36.
3.0.CO;2-9" data-track-action="article reference" href="https://doi.org/10.1002%2F1097-0142%2819830815%2952%3A4%3C728%3A%3AAID-CNCR2820520427%3E3.0.CO%3B2-9" aria-label="Article reference 114" data-doi="10.1002/1097-0142(19830815)52:4 3.0.CO;2-9">Article CAS PubMed Google Scholar
Jerez Aragonés JM, Peláez Sánchez JI, Dona JM, Alba E. A neuro-fuzzy decision model for prognosis of breast cancer relapse. In: Conejo R, Urretavizcaya M, Perez-de-la-Cruz J, editors. Lecture Notes in Computer Science. Volume 3040. Berlin: Springer; 2004. p. 638–45.
Jhajharia S, Varshney HK, Verma S, Kuma J. A neural network based breast cancer prognosis model with PCA processed features. Jaipur, India: Proceedings of the 2016 International Conference on Advances in Computing, Communications and Informatics (ICACCI); 2016.
Kim W, Kim KS, Park RW. Nomogram of naive Bayesian model for recurrence prediction of breast cancer. Healthc Inform Res. 2016;22(2):89–94.
Liu YQ, Cheng W, Lu Z. Decision tree based predictive models for breast cancer survivability on imbalanced data. Proceedings of the 2009 3rd international conference on bioinformatics and biomedical engineering: 2009; Beijing , China. 2009.
Lovekin C, Ellis IO, Locker A, Robertson JFR, Bell J, Nicholson R, Gullick WJ, Elston CW, Blamey RW. C-erbB-2 oncoprotein expression in primary and advanced breast cancer. Br J Cancer. 1991;63(3):439–43.
Mazouni C, Bonnier P, Romain S, Martin PM. A nomogram predicting the probability of primary breast cancer survival at 2- and 5-years using pathological and biological tumor parameters. J Surg Oncol. 2011;103(8):746–50.
Musial M, Grodecka-Gazdecka S, Kycler W. Prognostic index in lobular breast cancer. Rep Pract Oncol Radiother. 2005;10(4):165–72.
Ni YB, Tsang JYS, Chan SK, Tse GM. A novel morphologic-molecular recurrence predictive model refines traditional prognostic tools for invasive breast carcinoma. Ann Surg Oncol. 2014;21(9):2928–33.
Paik S, Hazan R, Fisher ER, Sass RE, Fisher B, Redmond C, Schlessinger J, Lippman ME, King CR. Pathologic findings from the National Surgical Adjuvant Breast and bowel project: prognostic significance of erbB-2 protein overexpression in primary breast cancer. J Clin Oncol. 1990;8(1):103–12.
Putter H, van der Hage J, de Bock GH, Elgalta R, van de Velde CJ. Estimation and prediction in a multi-state model for breast cancer. Biom J. 2006;48(3):366–80.
Ravdin PM, Siminoff LA, Davis GJ, Mercer MB, Hewlett J, Gerson N, Parker HL. Computer program to assist in making decisions about adjuvant therapy for women with early breast cancer. J Clin Oncol. 2001;19(4):980–91.
Sanghani M, Balk E, Cady B, Wazer D. Predicting the risk of local recurrence in patients with breast cancer: an approach to a new computer-based predictive tool. Am J Clin Oncol. 2007;30(5):473–80.
Shek LLM, Godolphin W. Model for breast-Cancer survival - relative prognostic roles of axillary nodal status, TNM stage, oestrogen-receptor concentration, and tumour necrosis. Cancer Res. 1988;48(19):5565–9.
van Nes JGH, Putter H, van Hezewijk M, Hille ETM, Bartelink H, Collette L, van de Velde CJH. EORTC breast Canc grp: tailored follow-up for early breast cancer patients: a prognostic index that predicts locoregional recurrence. Ejso. 2010;36(7):617–24.
Wen J, Ye F, Li S, Huang X, Yang L, Xiao X, Xie X. The practicability of a novel prognostic index (PI) model and comparison with Nottingham prognostic index (NPI) in stage I-III breast cancer patients undergoing surgical treatment. PLoS One. 2015;10(11):e0143537.
Aaltomaa S, Lipponen P, Eskelinen M, Kosma VM, Marin S, Alhava E, Syrjänen K. Predictive value of a morphometric prognostic index in female breast cancer. Oncology. 1993;50(1):57–62.
Alexander AI, Mercer RJ, Muir IM, Bennett RC, Rennie GC. Validation of a prognostic index in breast cancer. ANZ J Surg. 1987;57(6):399–403.
Article CAS Google Scholar
Carbone A, Serra FG, Rinelli A, Terribile D, Valentini M, Bellantone R, Rossi S, Ausili-Cefaro G, Nardone L, Piantelli M, Capelli A, Ranelletti FO. Morphometric prognostic index in breast cancer. Anal Quant Cytol Histol. 1999;21(3):250–4.
Green AR, Soria D, Stephen J, Powe DG, Nolan CC, Kunkler I, Thomas J, Kerr GR, Jack W, Cameron D. Nottingham prognostic index plus: validation of a clinical decision making tool in breast cancer in an independent series. J Pathol. 2016;2(1):32–40.
CAS Google Scholar
Jung SP, Hur SM, Lee SK, Kim S, Choi M, Bae SY, Kim J, Kim MK, Kil WH, Choe J. Validation of a web-based tool to predict the ipsilateral breast tumour recurrence (IBTR! 2.0) after breast-conserving therapy for Korean patients. J Breast Cancer. 2013;16(1):97–103.
Kindts I, Laenen A, Peeters S, Janssen H, Depuydt T, Nevelsteen I, Van Limbergen E, Weltens C. Validation of the Web-Based IBTR! 2.0 Nomogram to Predict for Ipsilateral Breast Tumor Recurrence After Breast-Conserving Therapy. Int J Radiat Oncol Biol Phys. 2016;95(5):1477–84.
Lende TH, Janssen EAM, Gudlaugsson E, Voorhorst F, Smaaland R, van Diest P, Søiland H, Baak JPA. In patients younger than age 55 years with lymph node–negative breast cancer, proliferation by mitotic activity index is prognostically superior to adjuvant! J Clin Oncol. 2010;29(7):852–8.
Maishman T, Copson E, Stanton L, Gerty S, Dicks E, Durcan L, Wishart GC, Pharoah P, Eccles D. An evaluation of the prognostic model PREDICT using the POSH cohort of women aged ⩽ 40 years at breast cancer diagnosis. Br J Cancer. 2015;112(6):983–91.
Miao H, Hartman M, Verkooijen HM, Taib NA, Wong H, Subramaniam S, Yip C, Tan E, Chan P, Lee S. Validation of the CancerMath prognostic tool for breast cancer in Southeast Asia. BMC Cancer. 2016;16(1):820.
Ribelles N, Barnadas A, Lopez D, Vaquero M, Rosell R, Sanchez J. Validation of breast cancer prognostic models. Oncol Rep. 1997;4(2):419–23.
Altman DG. Analysis of Survival times. In: Altman DG, editor. Practical statistics for Medical research. 1st ed. London: Chapman and Hall; 1992. p. 365–93.
Bland JM, Altman DG. The log-rank test. BMJ. 2004;328(7447):1073.
Heller G, Mo Q. Estimating the concordance probability in a survival analysis with a discrete number of risk groups. Lifetime Data Anal. 2016;22(2):263–79.
Verweij PJM, van Houwelingen HC. Cross-validation in survival analysis. Stat Med. 1993;12(24):2305–14.
Download references
Acknowledgements
We thank the New Zealand Aid Programme for funding MP to pursue his Master of Public Health at the University of Auckland. This study is a part of his Master’s thesis.
The research received no specific grant from any funding agency in the public, commercial, or non-for-profit sectors.
Availability of data and materials
The datasets supporting the conclusions of this article are included within the article and its additional files.
Author information
Authors and affiliations.
Epidemiology and Biostatistics, School of Population Health, The University of Auckland, Private Bag 92019, Auckland, 1142, New Zealand
Minh Tung Phung, Sandar Tin Tin & J. Mark Elwood
You can also search for this author in PubMed Google Scholar
Contributions
ME and ST developed the concept. MP, ST, and ME designed the study, searched the publications and reviewed the articles. All authors contributed to the interpretation of the data and to drafts of the report and reviewed and approved the submission.
Corresponding author
Correspondence to Minh Tung Phung .
Ethics declarations
Ethics approval and consent to participate.
Not applicable
Consent for publication
Competing interests.
The authors declare that they have no competing interests.
Publisher’s Note
Springer Nature remains neutral with regard to jurisdictional claims in published maps and institutional affiliations.
Additional files
Additional file 1:.
Search terms. (XLSX 10 kb)
Additional file 2:
Model development studies that were excluded because of no full text. (XLSX 13 kb)
Additional file 3:
Model validation studies that were excluded because of no full text. (XLSX 12 kb)
Additional file 4:
Articles that presented model development and/or internal validation. (XLSX 47 kb)
Additional file 5:
Articles that presented only the internal validation. (XLSX 12 kb)
Additional file 6:
Articles that presented model external validation. (XLSX 39 kb)
Additional file 7:
Number of external validation studies. (XLSX 10 kb)
Additional file 8:
Characteristics of the models validated in external populations. (XLSX 11 kb)
Additional file 9:
Overview of external validation studies. (XLSX 16 kb)
Additional file 10:
Studies that compared models by independent researchers. (XLSX 10 kb)
Additional file 11:
Studies that compared models by models’ developers. (XLSX 9 kb)
Rights and permissions
Open Access This article is distributed under the terms of the Creative Commons Attribution 4.0 International License ( http://creativecommons.org/licenses/by/4.0/ ), which permits unrestricted use, distribution, and reproduction in any medium, provided you give appropriate credit to the original author(s) and the source, provide a link to the Creative Commons license, and indicate if changes were made. The Creative Commons Public Domain Dedication waiver ( http://creativecommons.org/publicdomain/zero/1.0/ ) applies to the data made available in this article, unless otherwise stated.
Reprints and permissions
About this article
Cite this article.
Phung, M.T., Tin Tin, S. & Elwood, J.M. Prognostic models for breast cancer: a systematic review. BMC Cancer 19 , 230 (2019). https://doi.org/10.1186/s12885-019-5442-6
Download citation
Received : 28 August 2018
Accepted : 06 March 2019
Published : 14 March 2019
DOI : https://doi.org/10.1186/s12885-019-5442-6
Share this article
Anyone you share the following link with will be able to read this content:
Sorry, a shareable link is not currently available for this article.
Provided by the Springer Nature SharedIt content-sharing initiative
- Breast cancer
- Prognostic model
- Predictive model
- Nottingham prognostic index
ISSN: 1471-2407
- Submission enquiries: [email protected]
- General enquiries: [email protected]
- Introduction
- Conclusions
- Article Information
Proportion of studies being answered Y/PY (yes/probably yes), N/PN (no/probably no), and NI (no information) for each PROBAST item. Development studies only.
Numbers of development studies that reported each TRIPOD item. D, development study only; V, validation study only.
eTable 1. PRISMA Statement, Information Flow Through the Systematic Search Process
eTable 2. PRISMA Statement for Abstract Checklist
eAppendix 1. Search Strategy Conducted on September 22, 2021
eAppendix 2. Data Collection Questionnaire
eAppendix 3. PROBAST Questionnaire
eAppendix 4. TRIPOD Checklist
eTable 3. Papers Excluded on Full-text
eTable 4. Overview of Calculation per Included Model
eTable 5. Regression Models
eTable 6. PROBAST Quality Analysis Results
eFigure 1. PROBAST Quality Analysis Results of 07 Studies (06 Model Development Studies and 01 Model Validation Studies)
eFigure 2. TRIPOD Checklist
Data Sharing Statement
- Predicting Risk of Cardiotoxic Effects in Breast Cancer: Are We There Yet? JAMA Network Open Invited Commentary February 23, 2023 Tauben Averbuch, MD; Mamas A. Mamas, MD, PhD; Harriette G. C. Van Spall, MD, MPH
See More About
Sign up for emails based on your interests, select your interests.
Customize your JAMA Network experience by selecting one or more topics from the list below.
- Academic Medicine
- Acid Base, Electrolytes, Fluids
- Allergy and Clinical Immunology
- American Indian or Alaska Natives
- Anesthesiology
- Anticoagulation
- Art and Images in Psychiatry
- Artificial Intelligence
- Assisted Reproduction
- Bleeding and Transfusion
- Caring for the Critically Ill Patient
- Challenges in Clinical Electrocardiography
- Climate and Health
- Climate Change
- Clinical Challenge
- Clinical Decision Support
- Clinical Implications of Basic Neuroscience
- Clinical Pharmacy and Pharmacology
- Complementary and Alternative Medicine
- Consensus Statements
- Coronavirus (COVID-19)
- Critical Care Medicine
- Cultural Competency
- Dental Medicine
- Dermatology
- Diabetes and Endocrinology
- Diagnostic Test Interpretation
- Drug Development
- Electronic Health Records
- Emergency Medicine
- End of Life, Hospice, Palliative Care
- Environmental Health
- Equity, Diversity, and Inclusion
- Facial Plastic Surgery
- Gastroenterology and Hepatology
- Genetics and Genomics
- Genomics and Precision Health
- Global Health
- Guide to Statistics and Methods
- Hair Disorders
- Health Care Delivery Models
- Health Care Economics, Insurance, Payment
- Health Care Quality
- Health Care Reform
- Health Care Safety
- Health Care Workforce
- Health Disparities
- Health Inequities
- Health Policy
- Health Systems Science
- History of Medicine
- Hypertension
- Images in Neurology
- Implementation Science
- Infectious Diseases
- Innovations in Health Care Delivery
- JAMA Infographic
- Law and Medicine
- Leading Change
- Less is More
- LGBTQIA Medicine
- Lifestyle Behaviors
- Medical Coding
- Medical Devices and Equipment
- Medical Education
- Medical Education and Training
- Medical Journals and Publishing
- Mobile Health and Telemedicine
- Narrative Medicine
- Neuroscience and Psychiatry
- Notable Notes
- Nutrition, Obesity, Exercise
- Obstetrics and Gynecology
- Occupational Health
- Ophthalmology
- Orthopedics
- Otolaryngology
- Pain Medicine
- Palliative Care
- Pathology and Laboratory Medicine
- Patient Care
- Patient Information
- Performance Improvement
- Performance Measures
- Perioperative Care and Consultation
- Pharmacoeconomics
- Pharmacoepidemiology
- Pharmacogenetics
- Pharmacy and Clinical Pharmacology
- Physical Medicine and Rehabilitation
- Physical Therapy
- Physician Leadership
- Population Health
- Primary Care
- Professional Well-being
- Professionalism
- Psychiatry and Behavioral Health
- Public Health
- Pulmonary Medicine
- Regulatory Agencies
- Reproductive Health
- Research, Methods, Statistics
- Resuscitation
- Rheumatology
- Risk Management
- Scientific Discovery and the Future of Medicine
- Shared Decision Making and Communication
- Sleep Medicine
- Sports Medicine
- Stem Cell Transplantation
- Substance Use and Addiction Medicine
- Surgical Innovation
- Surgical Pearls
- Teachable Moment
- Technology and Finance
- The Art of JAMA
- The Arts and Medicine
- The Rational Clinical Examination
- Tobacco and e-Cigarettes
- Translational Medicine
- Trauma and Injury
- Treatment Adherence
- Ultrasonography
- Users' Guide to the Medical Literature
- Vaccination
- Venous Thromboembolism
- Veterans Health
- Women's Health
- Workflow and Process
- Wound Care, Infection, Healing
Get the latest research based on your areas of interest.
Others also liked.
- Download PDF
- X Facebook More LinkedIn
Kaboré EG , Macdonald C , Kaboré A, et al. Risk Prediction Models for Cardiotoxicity of Chemotherapy Among Patients With Breast Cancer : A Systematic Review . JAMA Netw Open. 2023;6(2):e230569. doi:10.1001/jamanetworkopen.2023.0569
Manage citations:
© 2024
- Permissions
Risk Prediction Models for Cardiotoxicity of Chemotherapy Among Patients With Breast Cancer : A Systematic Review
- 1 Health across Generations Team, Inserm U1018, Centre for Research in Epidemiology and Population Health, Villejuif, France
- 2 Université Joseph Ki-Zerbo, Ouagadougou, Burkina Faso
- 3 Department of Cardiology, CHU Dijon-Bourgogne, Dijon, France
- 4 Center for Primary Care and Public Health, Unisanté, University of Lausanne, Lausanne, Switzerland
- Invited Commentary Predicting Risk of Cardiotoxic Effects in Breast Cancer: Are We There Yet? Tauben Averbuch, MD; Mamas A. Mamas, MD, PhD; Harriette G. C. Van Spall, MD, MPH JAMA Network Open
Question What is the evidence for use of clinical prediction models to predict cardiotoxicity related to chemotherapy treatment in women with breast cancer?
Findings This systematic review identifies 7 studies and 6 outcome prediction models for cardiotoxicity in different populations of patients with breast cancer. Most prognostic models report good to excellent discrimination but fall short in addressing bias due to methodological weaknesses, particularly for sample size, lack of overfitting consideration, handling of missing data, and insufficient model performance assessment.
Meaning These results suggest that there is insufficient evidence to provide personalized risk prediction of cardiotoxicity related to breast cancer treatment in clinical practice; thus, researchers should adhere to best practices in the process of developing and validating predictive models.
Importance Cardiotoxicity is a serious adverse effect that can occur in women undergoing treatment for breast cancer. Identifying patients who will develop cardiotoxicity remains challenging.
Objective To identify, describe, and evaluate all prognostic models developed to predict cardiotoxicity following treatment in women with breast cancer.
Evidence Review This systematic review searched the Medline, Embase, and Cochrane databases up to September 22, 2021, to include studies developing or validating a prediction model for cardiotoxicity in women with breast cancer. The Prediction Model Risk of Bias Assessment Tool (PROBAST) was used to assess both the risk of bias and the applicability of the prediction modeling studies. Transparency reporting was assessed with the Transparent Reporting of a Multivariable Prediction Model for Individual Prognosis or Diagnosis (TRIPOD) tool.
Findings After screening 590 publications, we identified 7 prognostic model studies for this review. Six were model development studies and 1 was an external validation study. Outcomes included occurrence of cardiac dysfunction (echocardiographic parameters), heart failure, and composite clinical outcomes. Model discrimination, measured by the area under receiver operating curves or C statistic, ranged from 0.70 (95% IC, 0.62-0.77) to 0.87 (95% IC, 0.77-0.96). The most common predictors identified in final prediction models included age, baseline left ventricular ejection fraction, hypertension, and diabetes. Four of the developed models were deemed to be at high risk of bias due to analysis concerns, particularly for sample size, handling of missing data, and not presenting appropriate performance statistics. None of the included studies examined the clinical utility of the developed model. All studies met more than 80% of the items in TRIPOD checklist.
Conclusions and Relevance In this systematic review of the 6 predictive models identified, only 1 had undergone external validation. Most of the studies were assessed as being at high overall risk of bias. Application of the reporting guidelines may help future research and improve the reproducibility and applicability of prediction models for cardiotoxicity following breast cancer treatment.
Breast cancer is now the world’s most commonly diagnosed cancer, and it is responsible for 1 in 6 cancer deaths among women. 1 Research has yielded several developments in breast cancer diagnosis and treatment that improve morbidity and mortality outcomes. Breast cancer survival 5 years after diagnosis now exceeds 80% in most high-income countries. 2 Currently, there are more than 7.7 million breast cancer survivors around the world; this reflects improvements in diagnostics and treatment strategies including radiation therapy, chemotherapy, and biologic agents. 3 , 4
Unfortunately, these therapeutics can cause potentially life-threatening adverse effects. Myocardial dysfunction and heart failure (HF), frequently described as cardiotoxicity, are serious adverse effects that women undergoing breast cancer treatment can experience. 5 In the literature, the incidence of cardiotoxicity was reported to be as high as 34% when anthracycline and targeted agents are combined. 6 Moreover, breast cancer survivors have a significantly increased risk of death due to cardiovascular disease (CVD). 7
Given that cardiotoxicity is a significant factor in the prognosis for patients with breast cancer, many studies have aimed to identify predictors of cardiotoxicity. Age, obesity, hypertension, diabetes, and previous anthracycline treatment have been identified as major predictors of cardiac toxicity. 8 - 11 Several scores that can assist health care professionals and patients with breast cancer in assessing prognostic indicators and other decisions made in primary care have been developed in this setting. With the increasing number of breast cancer survivors and adverse cardiovascular events, it appears important to systematically assess the available prediction models and their ability to predict cardiotoxicity. The purpose of this study was to identify, describe, and appraise all prognostic models developed to predict cardiotoxicity related to chemotherapy treatment in women with breast cancer.
A systematic review was performed according to the Preferred Reporting Items for Systematic Reviews and Meta-Analysis ( PRISMA ) reporting guideline. The study protocol was registered with the Prospero International Prospective Register of Systematic Reviews ( CRD42021290441 ). An ethics statement was not required because this study was based exclusively on published research.
The PICOTS (population, intervention model, comparator, outcome, timing and settings) strategy was adopted for the search. 12 We included all developed prognostic models and their corresponding external validation studies (intervention model), involving women diagnosed with breast cancer at any stage (population) who subsequently had cardiotoxicity, defined as changes in LVEF or all symptomatic cardiac toxic effects after breast cancer therapy (outcome), at any time during follow-up (timing), with no predefined comparator (comparator) or setting (setting). We excluded review articles, conference abstract and studies with only patients with metastatic breast cancer. Two reviewers (E.G.K. and A.K.) independently performed the first screening by title and abstract. The 2 investigators independently undertook all study processes including online database search, study selection, data extraction, and critical appraisal. Any disagreements were discussed to reach a consensus. When a consensus was not obtained, third-party experts were invited to research, discuss, and finally reach a decision.
On September 22, 2021, we searched the electronic bibliographic databases Embase, Medline (PubMed), and Cochrane with no restriction on publication date. We developed a search strategy from a combination of free text and Medical Subject Heading terms for BC, cardiotoxicity, and HF (eAppendix 1 in Supplement 1 ).
We adapted the standardized data extraction form containing items based on the general guidelines of the Critical Appraisal and Data Extraction for Systematic Reviews of Prediction Modelling Studies (CHARMS) checklist. 13 Data were extracted for general information, source of data, participants, predictors, outcome, and analysis (eAppendix 2 in Supplement 1 ).
We used PROBAST (Prediction model Risk Of Bias Assessment Tool) to assess the risk of bias of the individual prognostic models investigated. 14 Risk of bias (ROB) was assessed according to the 4 PROBAST domains (participants, predictors, outcome, and analysis) and applicability according to 3 domains (participants, predictors, and outcome). The ROB was assessed to be either high, low, or unclear based on signaling questions (eAppendix 3 in Supplement 1 ).
We used the Transparent Reporting of a Multivariable Prediction Model for Individual Prognosis or Diagnosis ( TRIPOD ) reporting guideline to assess good reporting of studies developing or validating multivariable prediction models (eAppendix 4 in Supplement 1 ). 15 In the absence of sufficient data for a meta-analysis, we have used a narrative synthesis instead.
The search identified 590 potentially relevant citations related to prognostic models for cardiotoxicity in breast cancer. After removal of 156 duplicates, the titles and abstracts of the remaining 434 references were screened. We identified 16 studies for full-text assessment that would possibly fulfill our predefined inclusion criteria. Of these, we excluded 10 references (eTable 3 in Supplement 1 ). One study was included following a manual search. 16 The PRISMA flowchart of the search strategy and the selection process is illustrated in Figure 1 . Of the 7 studies included in this review, 6 focused on model development 16 - 21 and 1 22 focused on external validation ( Tables 1 and 2 ).
Of the 6 model development studies, 3 produced scoring systems, 17 , 18 , 20 while the other 3 16 , 19 , 21 were logistic regression prediction models (eAppendix 4 and 5 in Supplement 1 ). The prognostic models were mostly developed after 2019.
Of 6 studies, 3 used retrospective data 17 , 18 , 20 and 3 used prospective data. 16 , 19 , 21 Three studies included patients with localized breast cancer, 16 , 17 , 21 1 study included patients with localized and metastatic breast cancer, 20 and 2 studies did not report the cancer stage. 18 , 19 The numbers of participants in the 6 studies ranged from 217 to 8068 patients, and the mean age ranged between 49 and 73 years. Anthracyclines, alone or combined with trastuzumab, were used in all women in 4 studies. 16 , 18 , 19 , 21
Follow-up ranged from 1 to 7 years. Two studies assessed the 1-year risk of cardiotoxicity. 19 , 21 The other studies reported had a longer follow-up period: 3-year risk of HF or cardiomyopathy 17 ; 3-to-10-year risk of HF 18 ; risk of major adverse cardiovascular events (MACE) at 1, 3, and 7 years 20 ; and 5-year risk of cardiac death or congestive HF. 16
The definition of cardiac events was not uniform. Three of the included studies used clinical criteria 17 , 18 , 20 ; 2 of them used administrative data with International Classification of Diseases, Ninth Revision (ICD-9) and International Statistical Classification of Diseases and Related Health Problems, Tenth Revision (ICD-10) codes to define HF, 17 , 18 and 1 of them used MACE as the outcome, which was a composite of HF, myocardial infarction, stroke, cardiovascular deaths. 20 In 3 studies, clinical criteria were used in conjunction with left ventricular parameters. 16 , 19 , 21
The included models used between 2 and 20 predictors ( Table 1 ). Most often used predictors were age 16 - 18 , 20 , 21 and comorbidities. Comorbidities included: diabetes 17 , 18 , 20 ; hypertension 17 , 18 , 20 , 21 ; body mass index 20 , 21 ; atrial fibrillation 17 ; prior coronary artery disease 17 , 18 , 20 ; kidney failure 17 , 20 ; and prior stroke. 18 , 20 Some studies explored other predictors such as: disease characteristics (regional or distant invasion) 18 ; therapeutic methods used such as dose of chemotherapy agents (specifically anthracycline doses) 18 , 20 or dose of irradiation to left breast 20 ; biochemical predictors such as lipid profile 20 ; and echocardiographic parameters such as baseline LVEF 16 , 19 , 21 and LVEF change. 19 The models and their calculations are described in eTables 4 and 5 in Supplement 1 .
Calibration was reported in 4 studies. 16 - 18 , 20 One study did not report discrimination. 17 Of the 5 prognostic models for which the C index was reported, 2 models 19 , 20 had excellent discrimination (0.80-0.89) and 3 16 , 18 , 21 had acceptable discrimination (0.70-0.79), irrespective of cardiac event definition ( Table 1 ).
In the PROBAST quality analysis, 1 study was rated as low ROB, 18 1 other as unclear ROB, 21 and the remaining model development studies had high ROB due to concerns in the analysis domain ( Figure 2 ; eFigure 1 in Supplement 1 ). Lack of overfitting consideration (item 4.8: internal validation techniques consists of random split-sample of participants 17 , 18 , 20 or no internal validation has been performed 19 ), reasonable number of participants with the outcome (item 4.1: fewer than 10 events per variable 19 , 20 ), and participants lost to follow-up or with missing data handled inappropriately (item 4.3 and 4.4: participants with missing data 16 , 19 or loss to follow up 19 were excluded from analysis) were identified as bias related to analysis. We rated as low ROB the analysis domain for the prediction model of Fogarassy et al 18 because the development study was based on a large data set and a high number of events per variable (EPV) stratum. In Upshaw and al, 21 the development study was rated as unclear ROB due to insufficient model performance assessment (item 4.7: calibration not reported). We rated concern for applicability of all the development studies as low across all domains (eTable 6 in Supplement 1 ).
TRIPOD scores varied from 25 to 28 (of 31) in the 6 development studies ( Figure 3 ; eFigure 2 in Supplement 1 ). All studies met more than 80% of the items in the TRIPOD checklist. Two studies 16 , 17 were published before the TRIPOD’s publication in 2015. The items that were most often reported included the abstract (item 2), introduction (item 3), and source of data (item 4) for all studies. Lack of reporting on participant selection (item 13a) or models’ predictive performance (item 10d) were noted in 4 studies. 17 , 18 , 20 , 21 Only 1 study 19 reported unadjusted association between each candidate predictor and outcome (item 14b). No study reported masked assessment of outcome (item 6b) and predictors (item 7b). Missing data handling (item 9) was not reported in 1 study. 18
We identified 1 study (Milks et al 22 ) externally validating the prediction model by Ezaz et al. 17 It was derived from data from a retrospective, monocentric cohort study. The recruitment period for the study was from 2010 to 2014, and follow-up was not reported. Patients with all stage breast cancer treated by doxorubicin alone or in association with trastuzumab were included. A total of 183 patients were included with 33 events. The reported discrimination was excellent (AUC = 0.86). Calibration was not reported (item 4.7 rated “no information”). We rated the ROB as high due to analysis concerns, including item 4.1 (the number of participants with the outcome was under 100) and item 4.2 (categorical variables like age were categorized using cut-points that were different from those of the development study), and a lack of specified exclusion criteria ( Figure 2 ). The TRIPOD score was 21 (total 31). Insufficient reporting of the title (item 1), source of data (item 4a), participants (item 5c, and 13), missing data handling (item 9), and model performance were identified.
In their study, Milks et al 22 also suggest that superior prediction assessment for cancer-therapy–related cardiac dysfunction can be achieved by combining left ventricular global longitudinal strain (LV-GLS) and the clinical risk score from Ezaz et al. 17 The combined model had superior receiver-operating characteristics ( C = 0.9629).
Fogarassy et al 18 was the only included study that had a low overall risk of bias. However, neither study was externally validated. None of the included studies explored net benefit analysis with respect to the developed models.
We conducted a systematic literature search that identified 7 studies and six outcome prediction models for cardiotoxicity in different breast cancer populations, including patients with breast cancer at any stage, ERBB2 (formerly HER2 )-negative patients, ERBB2 -positive patients and patients eligible for trastuzumab. One of the models 17 underwent external validation in a separate external validation study. 22 The performance of these models and their applicability were assessed. Although most prognostic models reported good to excellent discrimination, of the 6 models, only 1 had low ROB and 1 other unclear ROB; the other 4 models had high ROB due to statistical analysis concerns, particularly for sample size, handling of missing data and not presenting appropriate performance statistics.
Two studies included in this review used registry data to develop their model. Registry data are commonly used in clinical prediction models because they provide large sample sizes and good representativeness while at the same time being relatively inexpensive and easy to obtain. 23 Ezaz et al 17 used the SEER-Medicare registry to identify 1664 American women diagnosed with early stage breast cancer, and Fogarassy et al 18 used the Hungarian National Cancer Registry to include 8068 patients. Nevertheless, we should be cautious because the amount of detail in data collection and whether outcome assessment was conducted by protocol may be a limitation for prognostic analyses in patient registries. 24
Two models included patients with advanced cancer stage (ie, metastasis), 18 , 20 whereas other models included early breast cancer stage. As expected, in the study by Fogarassy et al, 18 advanced cancer with distant metastases was confirmed as a significant predictor associated with heart failure. This likely reflects the choice of chemotherapy in addition to its potential impact on cardiotoxicity progression. Pinder et al 25 published a large analysis of the Medicare database for patients with breast cancer older than 65 years, and identified advanced cancer stage as significant predictor for heart failure. In addition, Cho et al 26 found that metastasis increased doxorubicin-induced cardiotoxicity by 2.66-fold and concomitant trastuzumab increased it by 4.08-fold. In the metastatic setting, ERBB2 -targeted therapies are used until disease progression or toxicity. 27 These findings suggest that cancer stage may be relatively important predictor in cardiotoxicity prediction models.
There was significant heterogeneity in the definition of cardiac events and follow-up. One study used a MACE outcome to develop a model to estimate the risk of MACE in women with breast cancer. Kim et al 18 utilized a 4-point MACE outcome. Although MACE is an increasingly common and standardized primary outcome of interest in randomized controlled trials, there are potential limitations in studies using administrative databases. A systematic review reported substantial heterogeneity for the MACE composite endpoints used in studies based on administrative databases. 28 “Acute myocardial infarction and stroke” and “acute myocardial infarction, stroke, and all-cause death” were the 2 most common composite MACE definitions. This diversity made it challenging to compare findings across studies or to aggregate multiple study results for meta-analyses or systematic reviews when considering different treatment or research questions. 28
The diagnosis of cardiotoxicity is currently defined as a greater than 10% reduction in the ejection fraction (EF) and an absolute value of less than 53% according to the American Society of Echocardiography (ASE) and the European Society of Cardiovascular Imaging (EACVI), 29 while the cutoff is 50% according to the European Society of Cardiology. 5 In the past, the definition of cardiotoxicity related to chemotherapy was restricted to ventricular dysfunction. However, the introduction of new treatments in the last few years has widened the concept of cardiotoxicity to a broader definition of cardiovascular toxicity, which incorporates arterial hypertension, ischemia, cardiomyopathy, myocarditis, arrhythmic complications, long QT, and arterial and venous thrombosis. 30 Future studies should therefore include a uniform and established definition of cardiotoxicity and a longer follow-up period to determine the effects of cancer treatments on long-term cardiotoxicity risk.
Most predictors included in the 6 prognostic models of this review were clinical variables. Incorporating a greater number of variables such as biomarkers (cardiac troponin and natriuretic peptides) or genetic information (microRNAs, proteomics and metabolomics) is another potential way to improve the prediction tool. 31 Moreover, because in different patients, the same biomarker level could have a different meaning depending on whether it is stable or rising, repeated measurements of multiple biomarkers reflecting different pathophysiological pathways may convey incremental prognostic value to single or even repeated biomarker measurements of established biomarkers. 32 The cumulative dose of anthracyclines has been proven to be an important factor associated with cardiotoxicity in patients receiving anthracycline-containing therapy. 33 , 34 In our study, models that included both the cumulative dose of anthracyclines and several comorbidities showed a better predictive performance than models that did not include these variables, except for the study by Goel et al, 19 that had excellent discrimination using only baseline and change in LVEF. This suggests that the cumulative dose of anthracyclines could play a large role in the risk of cardiotoxicity in patients with breast cancer.
Model overfitting and the resulting optimism are important concerns in prediction models. We can define overfitting specific to prediction models as fitting a statistical model with too many degrees of freedom in the modeling process. 24 Although 5 studies conducted internal validations, only 2 studies 16 , 21 applied internal validation properly by using bootstrapping techniques to overcome overfitting. In addition, although the demonstration of the performance of a model in an independent population is a necessary step before recommending its widespread use, only one of these models was externally validated. 35 Inappropriate EPV for sample size can cause high risks of overfitting and biased predictions. 36 EPV above 20 is recommended as the minimum sample size for model development 37 and validation samples should contain at least 100 individuals who develop the outcome of interest. 38 In this review, the sample size of 3 development models (EPV below 20) and the external model validation (less than 100 events) was not appropriate.
While the key messages were consistent, 4 included studies were at high overall ROB. One predictive model was only validated once, and we did not consider there to be enough information to assess its suitability in different situations. This review reveals that there is insufficient evidence to provide personalized risk prediction of cardiotoxicity related to breast cancer treatment in clinical practice.
To the best of our knowledge, this is the first systematic review to focus on prediction models of cardiotoxicity in patients with breast cancer, including a thorough evaluation of the ROB and reporting quality. A main strength of this study is the adoption of the PROBAST and TRIPOD guidelines as benchmarks.
However, we acknowledge that there were several limitations to this systematic review. We only included studies published in English and did not search gray literature. However, it is unlikely that any missed studies would have significantly changed our main findings. Another weakness was that we were unable to perform our planned meta-analysis due to the lack of eligible studies.
This review identified 6 prognostic models developed to predict risk of cardiotoxicity following treatment in women with breast cancer. The prediction models were designed in diverse clinical settings and populations, and with a range of included predictors. We are not yet able to accurately predict cardiotoxicity outcomes for a given patient with breast cancer based on their clinical parameters. Most of the models identified in this review fell short in addressing bias due to methodological weaknesses. To improve the overall quality in future predictive models, researchers should adhere to best practices in the development process. Investigators should also be aware of the potential benefits and weaknesses of various methods for model creation and internal validation. More external validation studies are needed to assess the performance and clinical applicability of existing models before they can successfully be used in clinical practice.
Accepted for Publication: December 20, 2022.
Published: February 23, 2023. doi:10.1001/jamanetworkopen.2023.0569
Open Access: This is an open access article distributed under the terms of the CC-BY License . © 2023 Kaboré EG et al. JAMA Network Open .
Corresponding Author: Elisé G. Kaboré, MD, Health across Generations Team, Inserm U1018, Centre for Research in Epidemiology and Population Health (CESP), 114 rue Edouard Vaillant, Villejuif 94805, France ( [email protected] ; [email protected] ).
Author Contributions: Drs E. Kaboré and Guenancia had full access to all of the data in the study and takes responsibility for the integrity of the data and the accuracy of the data analysis.
Concept and design: E. Kaboré, Macdonald, A. Kaboré, Arveux, Meda, Guenancia.
Acquisition, analysis, or interpretation of data: E. Kabore, A. Kaboré, Didier, Boutron-Ruault.
Drafting of the manuscript: E. Kaboré, A. Kaboré.
Critical revision of the manuscript for important intellectual content: Macdonald, A. Kaboré, Didier, Arveux, Meda, Boutron-Ruault, Guenancia.
Statistical analysis: Kabore.
Administrative, technical, or material support: E. Kaboré, A. Kaboré, Meda.
Supervision: Macdonald, Arveux, Meda, Boutron-Ruault, Guenancia.
Conflict of Interest Disclosures: Dr Boutron-Ruault reported receiving personal fees from Mayoli-Spindler, Gilead, and ViiV for presentations outside the submitted work. No other disclosures were reported.
Data Sharing Statement : See Supplement 2 .
- Register for email alerts with links to free full-text articles
- Access PDFs of free articles
- Manage your interests
- Save searches and receive search alerts
- Open access
- Published: 16 October 2021
Light at night and risk of breast cancer: a systematic review and dose–response meta-analysis
- Teresa Urbano ORCID: orcid.org/0000-0003-1490-5508 1 ,
- Marco Vinceti ORCID: orcid.org/0000-0002-0551-2473 1 , 2 ,
- Lauren A. Wise ORCID: orcid.org/0000-0003-2138-3752 2 &
- Tommaso Filippini ORCID: orcid.org/0000-0003-2100-0344 1
International Journal of Health Geographics volume 20 , Article number: 44 ( 2021 ) Cite this article
7987 Accesses
31 Citations
40 Altmetric
Metrics details
Breast cancer is the most common malignancy in women and the second leading cause of cancer death overall. Besides genetic, reproductive, and hormonal factors involved in disease onset and progression, greater attention has focused recently on the etiologic role of environmental factors, including exposure to artificial lighting such as light-at-night (LAN). We investigated the extent to which LAN, including outdoor and indoor exposure, affects breast cancer risk. We performed a systematic review of epidemiological evidence on the association between LAN exposure and breast cancer risk, using a dose–response meta-analysis to examine the shape of the relation. We retrieved 17 eligible studies through September 13, 2021, including ten cohort and seven case–control studies. In the analysis comparing highest versus lowest LAN exposure, we found a positive association between exposure and disease risk (risk ratio [RR] 1.11, 95% confidence interval-CI 1.07–1.15), with comparable associations in case–control studies (RR 1.14, 95% CI 0.98–1.34) and cohort studies (RR 1.10, 95% CI 1.06–1.15). In stratified analyses, risk was similar for outdoor and indoor LAN exposure, while slightly stronger risks were observed for premenopausal women (premenopausal: RR 1.16, 95% CI 1.04–1.28; postmenopausal: 1.07, 95% CI 1.02–1.13) and for women with estrogen receptor (ER) positive breast cancer (ER + : RR 1.09, 95% CI 1.02–1.17; ER–: RR 1.07, 95% CI 0.92–1.23). The dose–response meta-analysis, performed only in studies investigating outdoor LAN using comparable exposure assessment, showed a linear relation up to 40 nW/cm 2 /sr after which the curve flattened, especially among premenopausal women. This first assessment of the dose–response relation between LAN and breast cancer supports a positive association in selected subgroups, particularly in premenopausal women.
Introduction
Breast cancer is the most common cancer in women in both developed and undeveloped countries [ 1 ]. In Italy, there were an estimated 55,000 new cases in 2020 [ 2 ], and while incidence is increasing, mortality rates have significantly decreased across the years. Several factors appear to be involved in both etiology and prognosis of this malignancy, including selected genes, ageing, family history, reproductive factors, long-term use of postmenopausal female hormones, lifestyle [ 3 , 4 ], and environmental factors such as exposure to chemical endocrine disruptors [ 5 , 6 , 7 , 8 , 9 ].
In 2007, the International Agency for Research on Cancer (IARC) defined night-shift work as ‘probably carcinogenic to humans’ i.e. belonging to category 2A [ 10 ], due to a possible link with breast cancer [ 11 , 12 ] and with prostate and colorectal cancer [ 13 ]. The definition of night-shift work, also identified as graveyard shift, refers to a work schedule involving the sleeping hours of the general population. Among the exposure linked to graveyard shift work there is light-at-night (LAN), which induces disruption of the circadian rhythm and oxidative stress [ 14 ]. In particular, LAN could be involved in breast cancer etiology through different mechanistic pathways including DNA damage, impairment of melatonin and estrogen secretion, inflammation, and disruption of metabolic function [ 15 ]. Exposure to LAN can cause circadian and sleep disruptions, which may adversely affect different inflammatory and immunological pathways, thereby decreasing production of circulating natural killer cells or enhancing pro-inflammatory effects [ 16 , 17 ]. Since sleep has antioxidant effects, its disruption may also cause increased production of reactive oxygen species [ 18 , 19 ]. When evaluating exposure to LAN, both outdoor (e.g., streetlamps, illuminated buildings, lights from vehicles) and indoor artificial sources (e.g., domestic lights, electric devices-derived illumination) are relevant in increasing circadian disruption and therefore the risk of developing cancer. Outdoor LAN is generally assessed using satellite-derived data, while indoor is often evaluated through surveys on night habits and bedroom light characteristics [ 20 ].
Two recent meta-analyses summarized data on the association between LAN and breast cancer risk, investigating the effects of the highest vs. the lowest LAN exposure categories [ 21 , 22 ]. Since the publication of these meta-analyses, three large studies of the LAN-breast cancer association have been published [ 23 , 24 , 25 ]. In the present report, we update the meta-analysis with these new studies, perform subgroup analyses by breast cancer subtype and other factors, and more comprehensively assess the epidemiological evidence about LAN and breast cancer risk. In addition, we apply dose–response meta-analyses to assess, for the first time, the shape of the association between LAN and breast cancer.
We followed the Preferred Reporting Items for Systematic Reviews and Meta-Analysis (PRISMA) 2020 statement [ 26 ] to perform this review.
Study identification and selection
The research question was configured according to PECOS statement (Population, Exposure, Comparator(s), Outcomes, and Study design): “Is exposure to light-at-night, as assessed through indoor and outdoor exposure to lighting sources, positively associated with risk of breast cancer in non-experimental studies?” and “Is there a dose–response association between LAN and breast cancer incidence?” [ 27 ]. Accordingly, we carried out a systematic literature search for publications available as of September 13, 2021 in the PubMed/MEDLINE, Embase and Web of Science (WoS) databases. We used search terms linked to “breast cancer” and “lighting” in PubMed, WoS, and Embase databases with no language restrictions (Additional file 1 : Table S1). We also performed citation chasing by scanning the reference list of included studies and of previous reviews, as well as backward/forward citation retrieval to identify additional relevant papers [ 28 ]. Inclusion criteria were as follows: titles including LAN and breast cancer; mentioning case–control/case-cohort/cohort studies; monitoring LAN from space according to the US Defense Meteorological Satellite Program (DMSP) Operational Linescan System or from the Visible Infrared Imaging Radiometer Suite Day-Night Band (DNB); evaluating indoor LAN based on self-reported questionnaires and mentioning LAN levels as low, medium or high, and darkness and nightlight levels, and habits of sleeping with lights on; reporting of risk estimates for breast cancer, along with their 95% confidence intervals, or availability of enough data to calculate them.
Two authors reviewed all titles and abstracts independently, and any conflicts were resolved with the help of third author. For each included study, we extracted information about design, population size and characteristics, country, study period and years of follow-up, risk estimates (either odds ratio, risk ratio, or hazard ratio) along with their 95% confidence interval (CI) of breast cancer, adjustment factors, type of exposure assessment, and dose of exposure.
Quality assessment
We assessed the quality in the included studies by using the Risk Of Bias In Non-randomized Studies of Exposures (ROBINS-E) tool [ 29 ]. We classified studies as having low, moderate, or high risk of bias according to seven domains: bias due to confounding; bias in selecting participants in the study; bias in exposure classification; bias due to departures from intended exposures; bias due to missing data; bias in outcome measurement; and bias in the selection of reported results. In Additional file 1 : Table S2, we report criteria for risk of bias evaluation, performed by two authors. In case of disagreement, a third author helped in the final decision. A study’s overall risk of bias was considered high or moderate if at least one domain was judged at high or moderate risk, otherwise it was classified as having a low risk of bias.
Statistical analysis
We performed a meta-analysis comparing breast cancer incidence in the highest versus lowest levels of LAN exposure using a restricted maximum likelihood random effect model, which bases estimates on a likelihood function calculated from a transformed set of data [ 30 ]. Additionally, whenever possible, we carried out a dose–response meta-analysis of breast cancer risk according to increasing LAN exposure through a random-effects model, using a one-stage approach as previously implemented in other fields [ 31 , 32 , 33 ]. Specifically, for each LAN category, we used the mean or the median value, or the midpoint for the intermediate categories, whichever was available. For the highest and lowest exposure categories, if the average values were not reported and were ‘open’, we used as boundary a value 20% higher or lower than the closest cut-point. We used a restricted cubic spline model with three knots at fixed percentiles (10th, 50th, and 90th) and we considered the correlation within each set of published effect estimates using generalized least-squares regression through a multivariate random-effect meta-analysis, incorporating the restricted maximum likelihood method [ 30 , 34 ].
Besides the overall group, we also performed stratified analyses according to menopausal status (pre and postmenopausal), body mass index-BMI (< 25 and ≥ 25), estrogen receptor-ER status (ER + and ER–) of cases, and type of LAN exposure (outdoor and indoor). Furthermore, we explored the role of possible effect modifiers, by dividing the studies according to the country-specific estimated annual sunshine hours [ 35 ] into the three subgroups (< 2000, 2000–3000, and > 3000 annual mean sunshine hours), and country solar ultraviolet B (UV-B) radiation [ 36 ].
We assessed the potential for small-study bias using funnel plots for studies reporting highest versus lowest exposure, and by performing Egger’s test [ 37 , 38 ] and trim-and-fill analysis [ 39 ]. We also evaluated the effect of variation across studies through the graphical overlay of study-specific predicted curves by using fixed and random effects [ 34 ]. Finally, we assessed heterogeneity by reporting I 2 statistics, and by carrying out stratified analyses whenever possible such as for LAN exposure assessment method, menopausal status, participants’ weight (normal vs overweight/obese), and ER status. We used Stata software (v 16.1, 2021—Stata Corp., College Station, TX), namely its ‘meta’ and ‘drmeta’ routines, for data analysis.
Overall, of the 494 individual studies identified after removal of duplicates, we excluded 465 studies due to title and abstract screening, and 13 additional studies after full-text evaluation, leaving 17 studies eventually fulfilling inclusion criteria (Fig. 1 ). Main reasons of exclusion were the following: insufficient data, commentaries, reviews or meta-analyses, editorials, ecological studies, or lack of LAN exposure assessment (reasons reported in detail in Additional file 1 : Table S3).
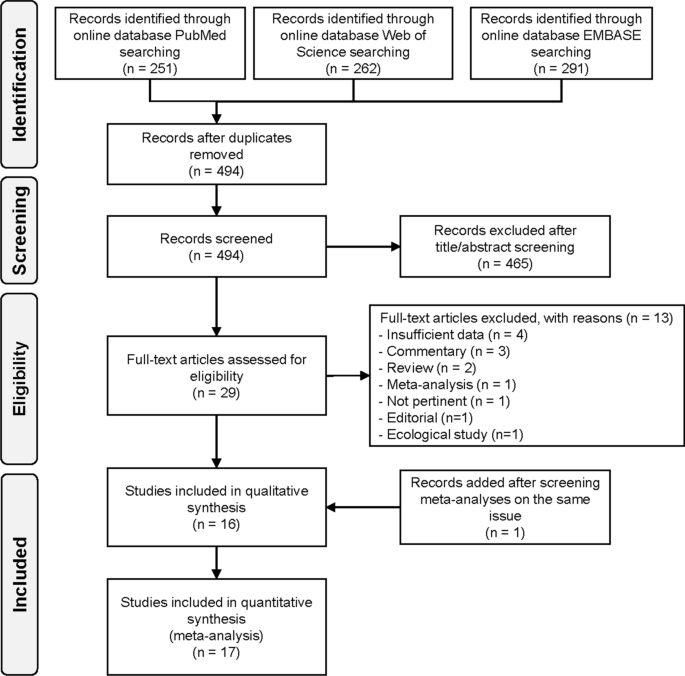
Flowchart summarizing the literature search and identification
Table 1 presents characteristics of the 17 included studies, three with case-cohort design [ 40 , 41 , 42 ], six cohort [ 23 , 24 , 25 , 43 , 44 , 45 ], and eight case–control [ 46 , 47 , 48 , 49 , 50 , 51 , 52 , 53 ]. The studies were published during 2001–2021, mainly in North America (N = 10), followed by Europe (N = 3), Israel (N = 2), Australia (N = 1), and China (N = 1). Follow-up duration was reported in only two studies (16 and 6.1 years) [ 23 , 42 ]. LAN was assessed according to two main methods: from outdoor (N = 7) [ 23 , 24 , 40 , 41 , 43 , 44 , 52 ] and indoor (N = 11) sources [ 41 , 42 , 43 , 45 , 46 , 47 , 48 , 49 , 50 , 51 , 53 ]. In all cohort and case-cohort studies assessing outdoor LAN, the unit of measurement for LAN was nano-Watt/square centimeters/steradian (nW/cm 2 /sr) [ 23 , 24 , 40 , 43 , 44 , 52 ]. The only exception was a Spanish study that assessed outdoor LAN using an index of outdoor blue light spectrum to calculate melatonin suppression index (MSI). MSI was estimated at each pixel of images of Madrid and Barcelona detected from the International Space Station (ISS) [ 41 ]. Indoor LAN data were evaluated based on self-reported questionnaires, for example, referring to use of lamps during the night or other sources of artificial light in the bedroom while sleeping. Additional characteristics of studies included in the systematic review are shown in the Additional file 1 : Table S4.
Risk of bias assessment (Additional file 1 : Table S5) showed that most studie s were at low risk of bias due to confounding, while four w ere at moderate risk of bias because they did not control for some breast cancer risk factors, typically family history of breast cancer, postmenopausal hormone use, or sm oking [ 24 , 40 , 47 , 48 ]. Concerning exposure assessment, studies assessing outdoor LAN exposure were at low risk of bias [ 23 , 24 , 25 , 40 , 44 , 52 ], while those assessing both outdoor and indoor LAN or only indoor LAN were generally at moderate risk due to possible misclassification bias [ 41 , 42 , 43 , 45 , 46 , 47 , 49 , 50 , 51 , 53 ]. One study was considered at high risk of bias because exposure assessment was based on a non-validated self-administered questionnaire [ 48 ]. All other domains were considered at low risk of bias in all studies, although four studies were judged at moderate risk of bias because some information (i.e., smoking or menopausal status) had been collected but not reported, no differences were found, or no data were presented [ 44 , 46 , 51 ].
Comparing the highest versus the lowest LAN exposure category, we consistently found positive associations with breast cancer risk (summary RR = 1.11, 95% CI 1.07–1.15). In subgroup analyses (Table 2 ), we found positive associations for outdoor (RR = 1.11, 95% CI 1.07–1.16) and indoor (RR = 1.08, 95% CI 1.00–1.17) LAN exposure, as well as both for case–control (RR = 1.11, 95% CI 0.97–1.28) and cohort studies (RR = 1.11, 95% CI 1.07–1.15) as shown in Fig. 2 and Additional file 1 : Figure S1.
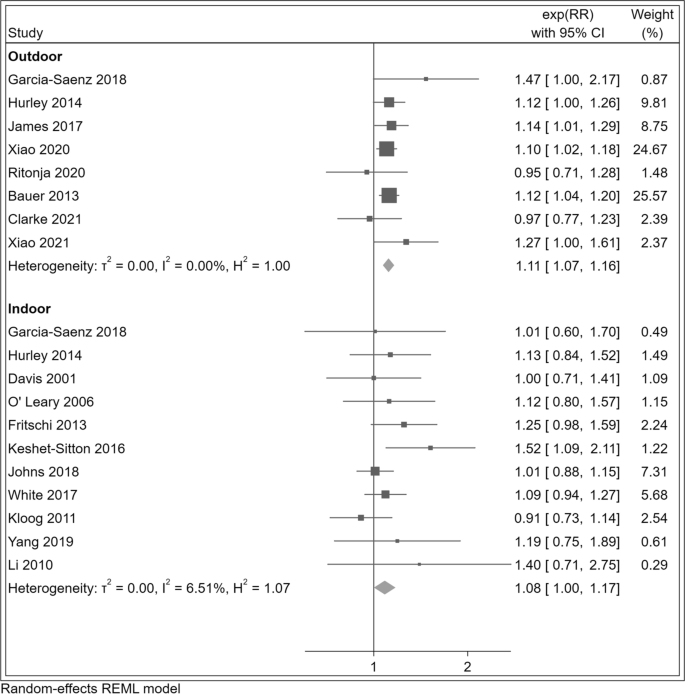
Risk ratio (RR) with 95% confidence interval (CI) for the association between light at night exposure and r isk o f breast cancer (N = 17 studies) com paring the highest versus the lowest exposure category in studies assessing outdoor and indoor exposure. The squares represent point estimates of RR and horizontal lines represent their 95% confidence intervals (CIs). The area of each square is proportional to the inverse of the variance of the estimated log RR. The diamonds represent the combined RR for each subgroup and the overall RR for all studies. The solid line represents RR = 1
Eight studies assessed breast cancer risk among both pre and postmenopausal women at the moment of diagnosis, while one was restricted to postmenopausal women only. The summary RR was slightly stronger among premenopausal women (RR = 1.16, 95% CI 1.04–1.28) than postmenopausal women (RR = 1.07, 95% CI 1.02–1.13) (Fig. 3 ). A slight positive association among premenopausal women also emerged in the cohort/case-cohort study subgroup and for outdoor LAN exposure. Conversely, for the case–control category and indoor LAN exposure, the RR was slightly higher among postmenopausal women (Table 2 and Additional file 1 : Figure S2).
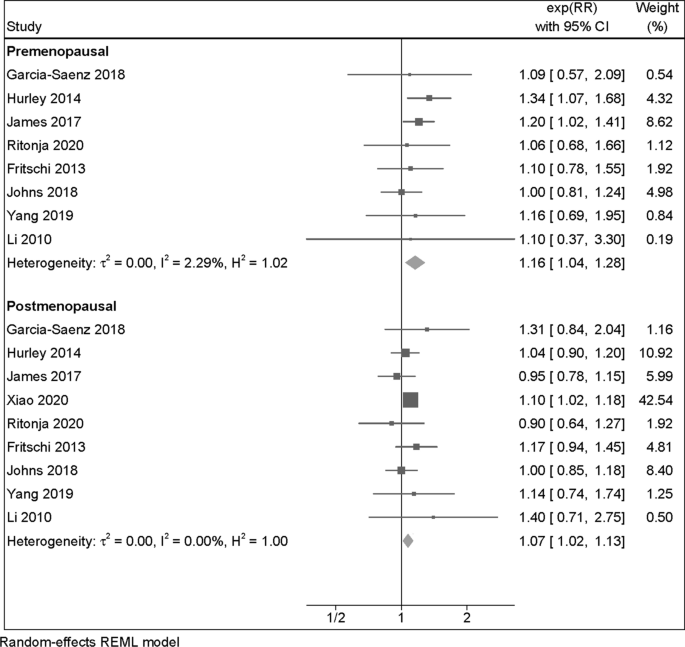
Risk ratio (RR) with 95% confidence interval (CI) for the association between light at night exposure and risk of breast cancer (N = 9 studies) among premenopausal and postmenopausal women, comparing the highest versus the lowest exposure category. The area of each grey square is proportional to the inverse of the variance of the estimated log RR. Black diamonds represent point estimates of RR and horizontal lines represent their 95% confidence intervals (CIs). The open diamonds represent the combined RR for each subgroup and the overall RR for all studies. The solid line represents RR = 1
In the dose–response meta-analysis, we found a positive linear relation up to 40 nW/cm 2 /sr of outdoor LAN exposure, after which a plateau was reached (Fig. 4 ). A substantially comparable pattern was noted for all women and in analyses stratified according to menopausal status.
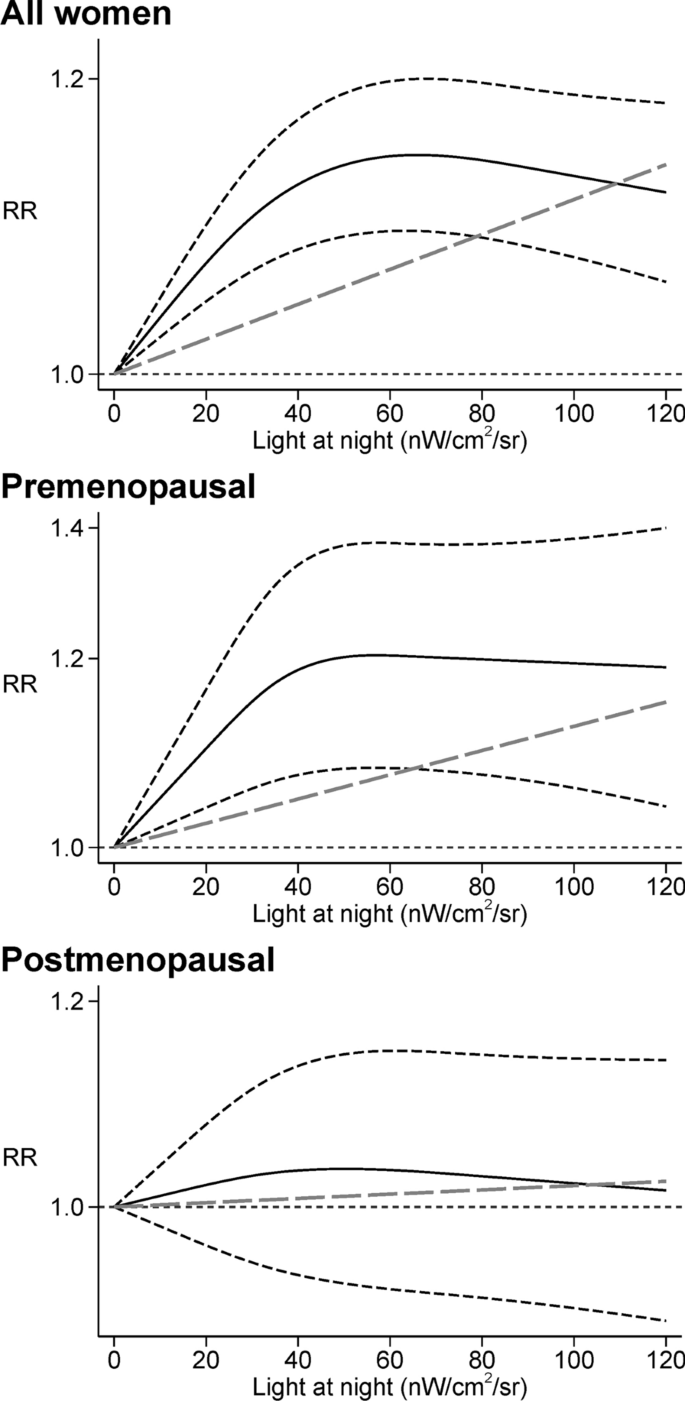
Dose–response meta-analysis between light at night and risk of breast cancer among all women [ 23 , 24 , 25 , 40 , 43 , 44 , 52 ] and between light at night and risk of breast cancer in premenopausal [ 43 , 44 , 52 ] and postmenopausal women [ 23 , 43 , 44 , 52 ]. Spline curve (black solid line) with 95% confidence limits (black dashed lines), and linear trend estimation (long-dashed gray line). RR risk ratio
Concerning possible effect-modification by ER status, few differences emerged between women with ER + and E R–b r east cancer , for whom the summary RRs were 1.09 (95% CI 1.02–1.17) and 1.07 (95% CI 0.92–1.23), respectively (Table 2 and Additional file 1 : Figure S3). In addit ion, in the dose–response meta-analysis we found that the risk was slightly higher in the ER − subgroup compared to the ER + one. A difference also emerged above 30 nW/cm 2 /sr of outdoor LAN exposure, when the curve flattened in the ER + subgroup while continued to increase in the ER − one (Additional file 1 : Figure S4).
With regards to BMI status, the two studies of breast cancer risk among women with BMI < 25 or BMI ≥ 25 yielded similar positive summary RRs in both categories, though RRs were stronger in the normal-weight (BMI < 25) group (RR = 1.17, 95% CI 1.00–1.36 and RR = 1.07, 95% CI 0.87–1.32, respectively (Table 2 and Additional file 1 : Figure S5). A monotonic positive association emerged in the dose–response meta-analysis for the two BMI subgroups, despite the very low number of studies (Additional file 1 : Figure S6).
We also performed stratified analyses according to the annual sunshine hours’ map of the world (Table 2 and Additional file 1 : Figure S7), dividing the 17 studies by country latitude. We divided the countries in three different groups of annual sunshine hours (< 2000 h: Canada, UK, Denmark; 2000–3000 h: US, Western Australia, China; > 3000 h: Spain, Israel). Countries with annual sunshine hours < 2000 exhibited null association (RR = 0.97, 95% CI 0.87–1.08). Conversely, we found a stronger association between LAN in the subgroup of countries with 2000 to 3000 annual sunshine hours (RR = 1.12, 95% CI 1.08–1.17) as well as in countries with more than 3000 annual sunshine hours, i.e., Spain and Israel (RR = 1.30, 95% CI 1.11–1.52) (Additional file 1 : Figure S8). These findings were also observed in analyses stratified by postmenopausal status (Additional file 1 : Figure S9) and indoor/outdoor exposure, although for indoor exposure, the risk ratio was slightly higher in countries with 2000 to 3000 annual sunshine hours than those with more than 3000 (Table 2 and Additional file 1 : Figure S10). Finally, when we accounted for estimated equinoctial UV-B irradiance map (Additional file 1 : Figure S11) we found a null association between LAN and breast cancer risk in studies from countries with less UV-B irradiance (RR = 0.97, 95% CI 0.87–1.08), while an inverse association was observed in the outdoor exposure subgroup (RR = 0.91, 95% CI 0.76–1.09). Conversely, there was a positive association in studies from countries with higher (> 0.58 W/m 2 ) UV-B irradiance levels (Additional file 1 : Figure S12), as also confirmed when considering either outdoor or indoor exposure (Table 2 ).
Exclusion of the one study [ 48 ] considered at high risk of bias did not substantially alter the results (Additional file 1 : Table S6). Findings were also similar when we additionally excluded the two studies considered at moderate risk of bias in selection of reported results [ 46 , 51 ] (Additional file 1 : Table S7). To further limit the effect of potential biases, we then performed the analyses excluding three additional studies [ 24 40 , 47 ] considered at moderate risk of bias due to confounding. Still, there were little changes in the results, and the estimates were substantially confirmed (Additional file 1 : Table S8). Similarly, analysis of conditional study-specific lines arising from the estimated random-effects model yielded homogeneous results overall and among premenopausal women, while among postmenopausal women, slightly higher variation was noted (Additional file 1 : Figure S13). Finally, evaluation of small-study bias suggested no occurrence of bias due to symmetric distribution and no studies were added when running trim-an-fill analysis both in overall studies (Additional file 1 : Figure S14) and in analyses stratified by menopausal status (Additional file 1 : Figure S15) and exposure assessment (Additional file 1 : Figure S16).
Higher urbanization has prompted substantial changes in peoples’ lifestyles as compared with our ancestors. Nowadays, over 80% of the World’s population and close to 100% of the people in the United States and Europe live under skies polluted by light [ 54 ], one of the key environmental factors characterizing the Western world environment . Besides residence-related artificial light (i.e., urban light pollution), other sources of non-natural LAN are electronic devices (TVs, smartphones, tablets, computers, etc.) or lights turned on during night at home or at the workplace. The possible carcinogenic effects of LAN has been recently assessed also by the U.S. National Toxicology Program (NTP) cancer hazard assessment [ 55 ]. NTP concluded that there was moderate evidence for a causal relation between LAN exposure and human cancer, since LAN may act through different mechanisms of circadian disruption and its biological effects are the same of well-known recognized carcinogens [ 55 ].
Over the last twenty years, the association between LAN exposure and breast cancer risk has been assessed primarily in occupational settings, specifically among night-shift workers [ 56 , 57 , 58 , 59 , 60 ]. These studies generally found a slight to substantial excess for breast cancer in women working graveyard shifts. Most recently, epidemiological studies investigating LAN exposure, in most cases independently from nightshift work, and its association with risk of breast cancer in the general population have greatly increased. In longitudinal studies, metrics of outdoor LAN have been collected through sophisticated methods such as the US DMSP Operational Line-Scan System or the Visible Infrared Imaging Radiometer Suite DNB, and expressed as nW/cm 2 /sr, except for one study [ 41 ], which was based on a visual artificial light-at-night (ALAN) assessment to estimate ground-based spectrum of the light emission, and melatonin suppression index for outdoor blue light spectrum. A Canadian study used both DNB and DMSP data. Even if DNB has a higher resolution and a calibrated radiometer, DMSP was used in our analysis for comparison with other studies [ 52 ].
Most case–control studies, in turn, have assessed LAN exposure using self-administered questionnaires regarding sleep and/or night habits. Exposure assessment in these studies has included frequency of waking up and turning on lights during night, sleeping with the TV on or off, darkness level in the room, residency near strong artificial LAN sources, wearing a mask while sleeping, keeping lights on or off while sleeping.
There is some biological plausibility for a LAN breast cancer association, given the observation that repeated exposure to artificial light during night hours might induce DNA damage and oxidative stress, alter melatonin and estrogen synthesis and metabolism, inflammation and immune function, and disrupt metabolic function [ 18 ]. More specifically, three mechanisms have been proposed to explain the link between LAN and some types of cancers. LAN could inhibit melatonin secretion directly [ 61 ], through sleep deprivation (also affecting cell proliferation and cytokines production [ 62 ]), and through chronodisruption [ 63 , 64 ]. Along these lines, previous studies referred more generally to night shift work than to LAN exposure, but night shift work is a far more complex exposure, including among other changes in sleep habits, sleep deprivation, eating during the night [ 61 ]. For this reason, we focused our attention more specifically on exposure to LAN as a factor associated with higher incidence of breast cancer, rather than night shift work, though the latter may confound to some extent the association between LAN and breast cancer risk.
We found a consistent positive association between LAN and breast cancer risk overall and among several subgroups, including premenopausal women, those with BMI < 25, and those living in countries experiencing more than 3000 sunshine hours a year. The risk of developing breast cancer was almost monotonically associated with outdoor LAN up to the value of 40 nW/cm 2 /sr, above which the threshold of the curve flattened. The association was stronger among premenopausal women, suggesting that younger women or women with higher endogenous levels of estrogens may have greater susceptibility to the effects of LAN. Effect measure modification by menopausal status could be due to different underlying biological mechanisms. Previous studies have reported that the suppressive effect of LAN on melatonin secretion may be stronger among younger people, tending to decrease with age [ 62 , 65 ]. In addition, LAN may impact on the length of the menstrual cycle through endocrine-disrupting properties, thus leading to higher breast cancer risk in the premenopausal period [ 66 ].
With regard to confounding factors, solar UV-B radiation is thought to be protective for breast cancer development. The inverse association between cancer risk and UV-B radiation was hypothesized for the first time by the Garland brothers in 1980 [ 67 ] who theorized sunlight-induced increases in vitamin D 3 may confer protection. The final product of the vitamin D 3 metabolism is the calcitriol, which has many anti-carcinogenic properties including inhibiting cellular proliferation [ 68 ]. Across the years, many studies have investigated the potential protective role of the UV-B radiation against different types of cancer [ 69 , 70 , 71 , 72 ]. A remaining question is the relationship between LAN and ultraviolet radiation. We found a positive association between LAN and breast cancer risk in countries exposed to higher levels of UV-B radiation (> 0.58 W/m 2 ). Conversely, there was no association between LAN and breast cancer risk in countries with low UV-B irradiance (< 0.58 W/m 2 ).
We considered another confounding factor that also correlates with UV-B radiation and could influence the outcome risk: the influence of annual sunshine hours [ 73 , 74 ]. We found an increased breast cancer risk associated with LAN in countries where annual sunshine exposure exceeded 3000 h. A positive association was also found in countries where sunshine ranged 2000–3000 h/years, while no appreciable association was observed in countries where there were fewer sunshine hours. This could be explained by different habits of people living in different countries, which may reflect epigenetics adaptation [ 75 ]. As indicated by a 2014 Italian study, humans’ biological clocks may have adapted to different environmental conditions during migrations, consistent with studies on insects [ 76 ], birds [ 77 ], and fish [ 78 ] living at different latitudes. These studies analyzed, in particular, the evolution of circadian genes which may be related to selective pressure exerted from latitude, temperature, ultraviolet radiation flux [ 79 ]. Consequently, people living in countries exposed to less than 2000 annual sunshine hours are less susceptible to higher frequency of light during night compared to people living in countries exposed to more annual sunshine hours. An explanation may be the presence of different alleles of their circadian genes, which acted to adapt the organism to different living conditions such as different latitudes. [ 80 ]. Finally, we may hypothesize that if people residing in “darkest countries” have artificial light kept on also during the daytime, they may not be as strongly influenced by higher levels of LAN because of different environment-adaptive alleles which acted to adapt the organism to light regimes diverse from the natural ones, as has been shown in animals [ 81 ]. In the meantime, another hypothesis that may explain the stronger association in relation to the annual sunshine hours is represented by the cumulative effect of longer daily sunshine hours with LAN exposure. In fact, those living in “brightest countries” are exposed to higher levels of light, which may lead to greater melatonin suppression levels and chronodisruption. Hence, the combination of daily sunshine hours and LAN may increase breast cancer risk.
Our results are relatively consistent with two previous meta-analyses [ 21 , 22 ], with the exception of the subgroup analysis according to menopausal status, where our results were similar to those of Lai et al. [ 21 ] but conflicted with those of Wu et al. [ 22 ]. However, to our knowledge this meta-analysis is the first to have assessed the dose–response between LAN and breast cancer risk, particularly among premenopausal women. In addition, owing to three new, recently-published studies we could include in the present review, we could re-assess the LAN-breast cancer relation according to ER cancer type, also performing a dose–response meta-analysis in these subgroups. Though our findings support a harmful effect of LAN in both the ER + and ER − breast cancer subtypes, at high exposure levels i.e., above 30 nW/cm 2 /sr the curve flattened in women with ER + disease but still increased in the ER − subgroup.
Our review has some strengths and limitations. Firstly, we used a newly developed meta-analytic tool for exploring the full shape of the dose–response, enabling us to assess the shape of the relation between LAN exposure and breast cancer risk over a wide range of exposure and across population subgroups. Our approach also yielded some indications of the threshold exposure levels that can increase breast cancer risk. Moreover, we systematically used the most adjusted model from each included study, thereby accounting for major confounders of the association.
Nonetheless, we acknowledge that some summary estimates are still statistically unstable due to the low number of studies still characterizing some subgroups. In addition, we could not rule out that unmeasured confounding was still likely in the investigated studies, and therefore influenced the findings. An example of such potential identified confounder could be the possibility that air pollution is heavier in urban areas, where the highest levels of LAN exposure are also detectable. In particular, LAN exposure may correlate with higher levels of traffic-related pollutants, including noise, as indicated by its inverse correlation with greenness and green space diversity [ 82 , 83 ]. Unfortunately, only one study included traffic noise in the multivariable model, thus hampering the evaluation of any independent effects of this factor and its potential for confounding in LAN-related studies. Additionally, other confounders may be those related to the occupational night environment, especially for studies assessing LAN exposure among nightshift workers [ 55 ]. Another limitation could be the limited capacity of outdoor LAN to adequately reflect personal light exposure due to differences in indoor lightning, use of electronic devices, nighttime activities, or window treatments, being these only some of the potential other sources of exposure [ 83 , 84 ]. Therefore, future studies should ideally use validated questionnaires combined with satellite data to more accurately measure individual LAN exposure. Finally, funnel plots and trim-and-fill analysis suggest a negligible probability of small-study effects in overall and stratified analyses.
Conclusions
Our review suggests a positive association between LAN exposure and risk of breast cancer, particularly in some subgroups, especially in premenopausal women, while few differences substantially emerged according to ER status, thus ongoing efforts to minimize LAN exposure might contribute to decrease human burden of diseases [ 85 , 86 , 87 ].
Availability of data and materials
All data generated or analyzed during this study are included in this published article and its additional information files.
Abbreviations
Artificial Light At Night
Body Mass Index
Confidence Interval
Estrogen Receptor
US Defense Meteorological Satellite Program Operational Linescan System
Visible Infrared Imaging Radiometer Suite Day-Night Band
Heterogeneity
Light At Night
Ultraviolet B
Sung H, Ferlay J, Siegel RL, Laversanne M, Soerjomataram I, Jemal A, Bray F. Global cancer statistics 2020: GLOBOCAN estimates of incidence and mortality worldwide for 36 cancers in 185 countries. CA Cancer J Clin. 2021;71(3):209–49.
Article PubMed Google Scholar
AIRTUM: The numbers of cancer in Italy-2020. In . https://www.registri-tumori.it/cms/sites/default/files/pubblicazioni/new_NDC2020-operatori-web.pdf ; 2020.
Sun YS, Zhao Z, Yang ZN, Xu F, Lu HJ, Zhu ZY, Shi W, Jiang J, Yao PP, Zhu HP. Risk factors and preventions of breast cancer. Int J Biol Sci. 2017;13(11):1387–97.
Article CAS PubMed PubMed Central Google Scholar
Lei S, Fan P, Wang M, Zhang C, Jiang Y, Huang S, Fang M, He Z, Wu A. Elevated estrogen receptor beta expression in triple negative breast cancer cells is associated with sensitivity to doxorubicin by inhibiting the PI3K/AKT/mTOR signaling pathway. Exp Ther Med. 2020;20(2):1630–6.
White AJ, Keller JP, Zhao S, Carroll R, Kaufman JD, Sandler DP. Air pollution, clustering of particulate matter components, and breast cancer in the Sister Study: a U.S. wide cohort. Environ Health Perspect. 2019;127(10):107002.
Article PubMed PubMed Central Google Scholar
Goldberg MS, Villeneuve PJ, Crouse D, To T, Weichenthal SA, Wall C, Miller AB. Associations between incident breast cancer and ambient concentrations of nitrogen dioxide from a national land use regression model in the Canadian National Breast Screening Study. Environ Int. 2019;133(Pt B):105182.
Article CAS PubMed Google Scholar
Filippini T, Torres D, Lopes C, Carvalho C, Moreira P, Naska A, Kasdagli MI, Malavolti M, Orsini N, Vinceti M. Cadmium exposure and risk of breast cancer: a dose-response meta-analysis of cohort studies. Environ Int. 2020;142:105879.
Gaudet HM, Christensen E, Conn B, Morrow S, Cressey L, Benoit J. Methylmercury promotes breast cancer cell proliferation. Toxicol Rep. 2018;5:579–84.
Adani G, Filippini T, Wise LA, Halldorsson TI, Blaha L, Vinceti M. Dietary intake of acrylamide and risk of breast, endometrial, and ovarian cancers: a systematic review and dose-response meta-analysis. Cancer Epidemiol Biomarkers Prev. 2020;29(6):1095–106.
IARC working group on the evaluation of carcinogenic risks to humans. Painting, firefighting, and shiftwork. IARC Monogr Eval Carcinog Risks Hum. 2010;98:1–818.
Straif K, Baan R, Grosse Y, Secretan B, El Ghissassi F, Bouvard V, Altieri A, Benbrahim-Tallaa L, Cogliano V, WHO International Agency For Research on Cancer Monograph Working Group. Carcinogenicity of shift-work, painting, and fire-fighting. Lancet Oncol. 2007;8(12):1065–6.
Papantoniou K, Castano-Vinyals G, Espinosa A, Aragones N, Perez-Gomez B, Ardanaz E, Altzibar JM, Sanchez VM, Gomez-Acebo I, Llorca J, et al. Breast cancer risk and night shift work in a case-control study in a Spanish population. Eur J Epidemiol. 2016;31(9):867–78.
IARC working group on the evaluation of carcinogenic hazards to humans. Night shift work. IARC Monogr Eval Carcinog Hazards Hum. 2020;124:1–381.
Rumanova VS, Okuliarova M, Zeman M. Differential effects of constant light and dim light at night on the circadian control of metabolism and behavior. Int J Mol Sci. 2020;21(15):5478.
Article CAS PubMed Central Google Scholar
Russart KLG, Nelson RJ. Light at night as an environmental endocrine disruptor. Physiol Behav. 2018;190:82–9.
Irwin MR, Olmstead R, Carroll JE. Sleep disturbance, sleep duration, and inflammation: a systematic review and meta-analysis of cohort studies and experimental sleep deprivation. Biol Psychiatry. 2016;80(1):40–52.
Castanon-Cervantes O, Wu M, Ehlen JC, Paul K, Gamble KL, Johnson RL, Besing RC, Menaker M, Gewirtz AT, Davidson AJ. Dysregulation of inflammatory responses by chronic circadian disruption. J Immunol. 2010;185(10):5796–805.
Samuelsson LB, Bovbjerg DH, Roecklein KA, Hall MH. Sleep and circadian disruption and incident breast cancer risk: an evidence-based and theoretical review. Neurosci Biobehav Rev. 2018;84:35–48.
McEwen BS, Karatsoreos IN. Sleep deprivation and circadian disruption: stress, allostasis, and allostatic load. Sleep Med Clin. 2015;10(1):1–10.
Cho Y, Ryu SH, Lee BR, Kim KH, Lee E, Choi J. Effects of artificial light at night on human health: a literature review of observational and experimental studies applied to exposure assessment. Chronobiol Int. 2015;32(9):1294–310.
Lai KY, Sarkar C, Ni MY, Cheung LWT, Gallacher J, Webster C. Exposure to light at night (LAN) and risk of breast cancer: a systematic review and meta-analysis. Sci Total Environ. 2021;762:143159.
Wu Y, Gui SY, Fang Y, Zhang M, Hu CY. Exposure to outdoor light at night and risk of breast cancer: a systematic review and meta-analysis of observational studies. Environ Pollut. 2021;269:116114.
Xiao Q, James P, Breheny P, Jia P, Park Y, Zhang D, Fisher JA, Ward MH, Jones RR. Outdoor light at night and postmenopausal breast cancer risk in the NIH-AARP diet and health study. Int J Cancer. 2020;147(9):2363–72.
Clarke RB, Amini H, James P, von Euler-Chelpin M, Jorgensen JT, Mehta A, Cole-Hunter T, Westendorp R, Mortensen LH, Loft S, et al. Outdoor light at night and breast cancer incidence in the Danish Nurse Cohort. Environ Res. 2021;194:110631.
Xiao Q, Gierach GL, Bauer C, Blot WJ, James P, Jones RR. The association between outdoor artificial light at night and breast cancer risk in black and white women in the Southern Community Cohort Study. Environ Health Perspect. 2021;129(8):87701.
Page MJ, McKenzie JE, Bossuyt PM, Boutron I, Hoffmann TC, Mulrow CD, Shamseer L, Tetzlaff JM, Akl EA, Brennan SE, et al. The PRISMA 2020 statement: an updated guideline for reporting systematic reviews. PLoS Med. 2021;18(3):e1003583.
Morgan RL, Whaley P, Thayer KA, Schunemann HJ. Identifying the PECO: A framework for formulating good questions to explore the association of environmental and other exposures with health outcomes. Environ Int. 2018;121(Pt 1):1027–31.
European network for Health Technology Assessment (EUnetHTA): Process of information retrieval for systematic reviews and health technology assessments on clinical effectiveness. Version 2.0, December 2019.
Morgan RL, Thayer KA, Santesso N, Holloway AC, Blain R, Eftim SE, Goldstone AE, Ross P, Ansari M, Akl EA, et al. A risk of bias instrument for non-randomized studies of exposures: a users’ guide to its application in the context of GRADE. Environ Int. 2019;122:168–84.
Orsini N, Spiegelman D: Meta-analysis of dose-response relationships. In: Handbook of Meta-Analysis. Edited by Schmid CH, Stijnen T, White I, 1st Edition. New York: Chapman and Hall/CRC; 2021.
Filippini T, Hatch EE, Rothman KJ, Heck JE, Park AS, Crippa A, Orsini N, Vinceti M. Association between outdoor air pollution and childhood leukemia: a systematic review and dose-response meta-analysis. Environ Health Perspect. 2019;127(4):46002.
Filippini T, Malavolti M, Whelton PK, Naska A, Orsini N, Vinceti M. Blood pressure effects of sodium reduction: dose-response meta-analysis of experimental studies. Circulation. 2021;143(16):1542–67.
Vinceti M, Filippini T, Wise LA, Rothman KJ. A systematic review and dose-response meta-analysis of exposure to environmental selenium and the risk of type 2 diabetes in nonexperimental studies. Environ Res. 2021;197:111210.
Crippa A, Discacciati A, Bottai M, Spiegelman D, Orsini N. One-stage dose-response meta-analysis for aggregated data. Stat Methods Med Res. 2019;28(5):1579–96.
De Jong S, Auping WL, Oosterveld WT, Usanov A, Abdalla M, Van de Bovenkamp A, Frattina C: The geopolitical impact of climate mitigation policies: How hydrocarbon exporting rentier states and developing nations can prepare for a more sustainable future. The Hague Centre for Strategic Studies 2017.
Mohr S, Garland C, Gorham E, Grant W, Highfill R, Garland F: Mapping Vitamin D deficiency, breast cancer, and colorectal cancer. 2005.
Egger M, Smith GD, Phillips AN. Meta-analysis: principles and procedures. BMJ. 1997;315(7121):1533–7.
Lin L, Shi L, Chu H, Murad MH. The magnitude of small-study effects in the Cochrane Database of Systematic Reviews: an empirical study of nearly 30 000 meta-analyses. BMJ Evid Based Med. 2020;25(1):27–32.
Duval S, Tweedie RL. A non-parametric “trim and fill” method of accounting for publication bias in meta-analysis. J Am Stat Assoc. 2000;95(449):89–98.
Google Scholar
Bauer SE, Wagner SE, Burch J, Bayakly R, Vena JE. A case-referent study: light at night and breast cancer risk in Georgia. Int J Health Geogr. 2013;12:23.
Garcia-Saenz A, Sanchez de Miguel A, Espinosa A, Valentin A, Aragones N, Llorca J, Amiano P, Martin-Sanchez V, Guevara M, Capelo R et al : Evaluating the association between artificial light-at-night exposure and breast and prostate cancer risk in Spain (MCC-Spain Study). Environ Health Perspect 2018, 126(4):047011.
Johns LE, Jones ME, Schoemaker MJ, McFadden E, Ashworth A, Swerdlow AJ. Domestic light at night and breast cancer risk: a prospective analysis of 105 000 UK women in the Generations Study. Br J Cancer. 2018;118(4):600–6.
Hurley S, Goldberg D, Nelson D, Hertz A, Horn-Ross PL, Bernstein L, Reynolds P. Light at night and breast cancer risk among California teachers. Epidemiology. 2014;25(5):697–706.
James P, Bertrand KA, Hart JE, Schernhammer ES, Tamimi RM, Laden F. Outdoor light at night and breast cancer incidence in the Nurses’ Health Study II. Environ Health Perspect. 2017;125(8):087010.
White AJ, Weinberg CR, Park YM, D’Aloisio AA, Vogtmann E, Nichols HB, Sandler DP. Sleep characteristics, light at night and breast cancer risk in a prospective cohort. Int J Cancer. 2017;141(11):2204–14.
Davis S, Mirick DK, Stevens RG. Night shift work, light at night, and risk of breast cancer. J Natl Cancer Inst. 2001;93(20):1557–62.
Fritschi L, Erren TC, Glass DC, Girschik J, Thomson AK, Saunders C, Boyle T, El-Zaemey S, Rogers P, Peters S, et al. The association between different night shiftwork factors and breast cancer: a case-control study. Br J Cancer. 2013;109(9):2472–80.
Keshet-Sitton A, Or-Chen K, Yitzhak S, Tzabary I, Haim A. Can avoiding light at night reduce the risk of breast cancer? Integr Cancer Ther. 2016;15(2):145–52.
Kloog I, Portnov BA, Rennert HS, Haim A. Does the modern urbanized sleeping habitat pose a breast cancer risk? Chronobiol Int. 2011;28(1):76–80.
Li Q, Zheng T, Holford TR, Boyle P, Zhang Y, Dai M. Light at night and breast cancer risk: results from a population-based case-control study in Connecticut, USA. Cancer Causes Control. 2010;21(12):2281–5.
O’Leary ES, Schoenfeld ER, Stevens RG, Kabat GC, Henderson K, Grimson R, Gammon MD, Leske MC, Electromagnetic Fields and Breast Cancer on Long Island Study Group. Shift work, light at night, and breast cancer on Long Island, New York. Am J Epidemiol. 2006;164(4):358–66.
Ritonja J, McIsaac MA, Sanders E, Kyba CCM, Grundy A, Cordina-Duverger E, Spinelli JJ, Aronson KJ. Outdoor light at night at residences and breast cancer risk in Canada. Eur J Epidemiol. 2020;35(6):579–89.
Yang W, Shi Y, Ke X, Sun H, Guo J, Wang X. Long-term sleep habits and the risk of breast cancer among Chinese women: a case-control study. Eur J Cancer Prev. 2019;28(4):323–9.
Falchi F, Cinzano P, Duriscoe D, Kyba CC, Elvidge CD, Baugh K, Portnov BA, Rybnikova NA, Furgoni R. The new world atlas of artificial night sky brightness. Sci Adv. 2016;2(6):e1600377.
Article PubMed PubMed Central CAS Google Scholar
US National Toxicology Program: NTP cancer hazard assessment report on night shift work and light at night. In: NTP Cancer Hazard Assessment Report on Night Shift Work and Light at Night. Research Triangle Park (NC); 2021.
Hansen J, Lassen CF. Nested case-control study of night shift work and breast cancer risk among women in the Danish military. Occup Environ Med. 2012;69(8):551–6.
Hansen J, Stevens RG. Case-control study of shift-work and breast cancer risk in Danish nurses: impact of shift systems. Eur J Cancer. 2012;48(11):1722–9.
Lie JA, Kjuus H, Zienolddiny S, Haugen A, Stevens RG, Kjaerheim K. Night work and breast cancer risk among Norwegian nurses: assessment by different exposure metrics. Am J Epidemiol. 2011;173(11):1272–9.
Megdal SP, Kroenke CH, Laden F, Pukkala E, Schernhammer ES. Night work and breast cancer risk: a systematic review and meta-analysis. Eur J Cancer. 2005;41(13):2023–32.
Pukkala E, Helminen M, Haldorsen T, Hammar N, Kojo K, Linnersjo A, Rafnsson V, Tulinius H, Tveten U, Auvinen A. Cancer incidence among Nordic airline cabin crew. Int J Cancer. 2012;131(12):2886–97.
Costa G, Haus E, Stevens R. Shift work and cancer - considerations on rationale, mechanisms, and epidemiology. Scand J Work Environ Health. 2010;36(2):163–79.
Haus EL, Smolensky MH. Shift work and cancer risk: potential mechanistic roles of circadian disruption, light at night, and sleep deprivation. Sleep Med Rev. 2013;17(4):273–84.
Touitou Y, Reinberg A, Touitou D. Association between light at night, melatonin secretion, sleep deprivation, and the internal clock: health impacts and mechanisms of circadian disruption. Life Sci. 2017;173:94–106.
Erren TC, Reiter RJ, Piekarski C. Light, timing of biological rhythms, and chronodisruption in man. Naturwissenschaften. 2003;90(11):485–94.
Herljevic M, Middleton B, Thapan K, Skene DJ. Light-induced melatonin suppression: age-related reduction in response to short wavelength light. Exp Gerontol. 2005;40(3):237–42.
Stevens RG, Rea MS. Light in the built environment: potential role of circadian disruption in endocrine disruption and breast cancer. Cancer Causes Control. 2001;12(3):279–87.
Garland CF, Garland FC. Do sunlight and vitamin D reduce the likelihood of colon cancer? Int J Epidemiol. 1980;9(3):227–31.
Moukayed M, Grant WB. The roles of UVB and vitamin D in reducing risk of cancer incidence and mortality: a review of the epidemiology, clinical trials, and mechanisms. Rev Endocr Metab Disord. 2017;18(2):167–82.
Altieri B, Grant WB, Della Casa S, Orio F, Pontecorvi A, Colao A, Sarno G, Muscogiuri G. Vitamin D and pancreas: the role of sunshine vitamin in the pathogenesis of diabetes mellitus and pancreatic cancer. Crit Rev Food Sci Nutr. 2017;57(16):3472–88.
Chen W, Clements M, Rahman B, Zhang S, Qiao Y, Armstrong BK. Relationship between cancer mortality/incidence and ambient ultraviolet B irradiance in China. Cancer Causes Control. 2010;21(10):1701–9.
Ong JS, Cuellar-Partida G, Lu Y, Australian Ovarian Cancer S, Fasching PA, Hein A, Burghaus S, Beckmann MW, Lambrechts D, van Nieuwenhuysen E et al : Association of vitamin D levels and risk of ovarian cancer: a Mendelian randomization study. Int J Epidemiol 2016, 45(5):1619–1630.
Grant WB. Low ultraviolet-B exposure may explain some of the link between night shift work and increased risk of prostate cancer. Int J Cancer. 2015;137(4):999.
IARC working group on the evaluation of carcinogenic risks to humans. Solar and ultraviolet radiation. IARC Monogr Eval Carcinog Risks Hum. 2012;100D:35–101.
Holick MF. Biological effects of sunlight, ultraviolet radiation, visible light, infrared radiation and vitamin D for health. Anticancer Res. 2016;36(3):1345–56.
CAS PubMed Google Scholar
Hancock AM, Witonsky DB, Alkorta-Aranburu G, Beall CM, Gebremedhin A, Sukernik R, Utermann G, Pritchard JK, Coop G, Di Rienzo A. Adaptations to climate-mediated selective pressures in humans. PLoS Genet. 2011;7(4):e1001375.
Kyriacou CP, Peixoto AA, Sandrelli F, Costa R, Tauber E. Clines in clock genes: fine-tuning circadian rhythms to the environment. Trends Genet. 2008;24(3):124–32.
Johnsen A, Fidler AE, Kuhn S, Carter KL, Hoffmann A, Barr IR, Biard C, Charmantier A, Eens M, Korsten P, et al. Avian Clock gene polymorphism: evidence for a latitudinal cline in allele frequencies. Mol Ecol. 2007;16(22):4867–80.
O’Malley KG, Banks MA. A latitudinal cline in the Chinook salmon ( Oncorhynchus tshawytscha ) Clock gene: evidence for selection on PolyQ length variants. Proc Biol Sci. 2008;275(1653):2813–21.
CAS PubMed PubMed Central Google Scholar
Forni D, Pozzoli U, Cagliani R, Tresoldi C, Menozzi G, Riva S, Guerini FR, Comi GP, Bolognesi E, Bresolin N, et al. Genetic adaptation of the human circadian clock to day-length latitudinal variations and relevance for affective disorders. Genome Biol. 2014;15(10):499.
Putilov AA, Dorokhov VB, Poluektov MG. How have our clocks evolved? Adaptive and demographic history of the out-of-African dispersal told by polymorphic loci in circadian genes. Chronobiol Int. 2018;35(4):511–32.
Hancock AM, Alkorta-Aranburu G, Witonsky DB, Di Rienzo A. Adaptations to new environments in humans: the role of subtle allele frequency shifts. Philos Trans R Soc Lond B Biol Sci. 2010;365(1552):2459–68.
Stanhope J, Liddicoat C, Weinstein P. Outdoor artificial light at night: a forgotten factor in green space and health research. Environ Res. 2021;1:111012.
Article CAS Google Scholar
Huss A, van Wel L, Bogaards L, Vrijkotte T, Wolf L, Hoek G, Vermeulen R. Shedding some light in the dark-a comparison of personal measurements with satellite-based estimates of exposure to light at night among children in the Netherlands. Environ Health Perspect. 2019;127(6):67001.
Jones RR. Exposure to artificial light at night and risk of cancer: where do we go from here? Br J Cancer. 2021;124(9):1467–8.
Helbich M, Browning M, Huss A. Outdoor light at night, air pollution and depressive symptoms: a cross-sectional study in the Netherlands. Sci Total Environ. 2020;744:140914.
Munzel T, Hahad O, Daiber A. The dark side of nocturnal light pollution. Outdoor light at night increases risk of coronary heart disease. Eur Heart J. 2021;42(8):831–4.
Walker WH 2nd, Bumgarner JR, Walton JC, Liu JA, Melendez-Fernandez OH, Nelson RJ, DeVries AC. Light pollution and cancer. Int J Mol Sci. 2020;21(24):9360
Download references
Acknowledgements
Not applicable.
This work was supported by a grant “Dipartimenti di Eccellenza 2018 − 2022, MIUR, Italy” to the Department of Biomedical, Metabolic and Neural Sciences (Drs. Filippini, Urbano and Vinceti) and by a grant FAR IMPULSO2020 no. 494/2020 (Dr. Filippini).
Author information
Authors and affiliations.
CREAGEN - Environmental, Genetic and Nutritional Epidemiology Research Center, Department of Biomedical, Metabolic and Neural Sciences, University of Modena and Reggio Emilia, Modena, Italy
Teresa Urbano, Marco Vinceti & Tommaso Filippini
Department of Epidemiology, Boston University School of Public Health, Boston, MA, USA
Marco Vinceti & Lauren A. Wise
You can also search for this author in PubMed Google Scholar
Contributions
TU and TF designed the original study, and with MV and LAW analysed and interpreted the data and drafted the article. All authors read and approved the final manuscript.
Corresponding author
Correspondence to Marco Vinceti .
Ethics declarations
Ethics approval and consent to participate, consent for publication, competing interests.
The authors declare that they have no competing interest.
Additional information
Publisher's note.
Springer Nature remains neutral with regard to jurisdictional claims in published maps and institutional affiliations.
Supple mentary Information
Additional file 1..
Additional figures and tables.
Rights and permissions
Open Access This article is licensed under a Creative Commons Attribution 4.0 International License, which permits use, sharing, adaptation, distribution and reproduction in any medium or format, as long as you give appropriate credit to the original author(s) and the source, provide a link to the Creative Commons licence, and indicate if changes were made. The images or other third party material in this article are included in the article's Creative Commons licence, unless indicated otherwise in a credit line to the material. If material is not included in the article's Creative Commons licence and your intended use is not permitted by statutory regulation or exceeds the permitted use, you will need to obtain permission directly from the copyright holder. To view a copy of this licence, visit http://creativecommons.org/licenses/by/4.0/ . The Creative Commons Public Domain Dedication waiver ( http://creativecommons.org/publicdomain/zero/1.0/ ) applies to the data made available in this article, unless otherwise stated in a credit line to the data.
Reprints and permissions
About this article
Cite this article.
Urbano, T., Vinceti, M., Wise, L.A. et al. Light at night and risk of breast cancer: a systematic review and dose–response meta-analysis. Int J Health Geogr 20 , 44 (2021). https://doi.org/10.1186/s12942-021-00297-7
Download citation
Received : 18 June 2021
Accepted : 01 October 2021
Published : 16 October 2021
DOI : https://doi.org/10.1186/s12942-021-00297-7
Share this article
Anyone you share the following link with will be able to read this content:
Sorry, a shareable link is not currently available for this article.
Provided by the Springer Nature SharedIt content-sharing initiative
- Breast cancer
- Light-at-night
- Menopausal status
- Dose–response meta-analysis
International Journal of Health Geographics
ISSN: 1476-072X
- Submission enquiries: [email protected]
Thank you for visiting nature.com. You are using a browser version with limited support for CSS. To obtain the best experience, we recommend you use a more up to date browser (or turn off compatibility mode in Internet Explorer). In the meantime, to ensure continued support, we are displaying the site without styles and JavaScript.
- View all journals
- My Account Login
- Explore content
- About the journal
- Publish with us
- Sign up for alerts
- Review Article
- Open access
- Published: 04 May 2024
Clinical Studies
Impact of the COVID-19 pandemic on breast cancer patient pathways and outcomes in the United Kingdom and the Republic of Ireland – a scoping review
- Lynne Lohfeld ORCID: orcid.org/0000-0003-4711-7305 1 na1 ,
- Meenakshi Sharma 1 na1 ,
- Damien Bennett 2 ,
- Anna Gavin 1 , 2 ,
- Sinéad T. Hawkins ORCID: orcid.org/0000-0002-3340-2917 1 , 2 ,
- Gareth Irwin 3 ,
- Helen Mitchell 2 ,
- Siobhan O’Neill 3 &
- Charlene M. McShane 1
British Journal of Cancer ( 2024 ) Cite this article
283 Accesses
3 Altmetric
Metrics details
- Breast cancer
- Health services
The COVID-19 pandemic brought unplanned service disruption for breast cancer diagnostic, treatment and support services. This scoping review describes these changes and their impact in the UK and the Republic of Ireland based on studies published between January 2020 and August 2023. Thirty-four of 569 papers were included. Data were extracted and results thematically organized. Findings include fewer new cases; stage shift (fewer early- and more late-stage disease); and changes to healthcare organization, breast screening and treatment. Examples are accepting fewer referrals, applying stricter referral criteria and relying more on virtual consultations and multi-disciplinary meetings. Screening service programs paused during the pandemic before enacting risk-based phased restarts with longer appointment times to accommodate reduced staffing numbers and enhanced infection-control regimes. Treatments shifted from predominantly conventional to hypofractionated radiotherapy, fewer surgical procedures and increased use of bridging endocrine therapy. The long-term impact of such changes are unknown so definitive guidelines for future emergencies are not yet available. Cancer registries, with their large sample sizes and population coverage, are well placed to monitor changes to stage and survival despite difficulties obtaining definitive staging during diagnosis because surgery and pathological assessments are delayed. Multisite longitudinal studies can also provide guidance for future disaster preparedness.
Similar content being viewed by others
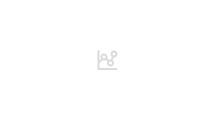

The COVID-19 pandemic and impact on breast cancer diagnoses: what happened in England in the first half of 2020
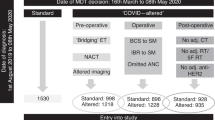
Breast cancer management pathways during the COVID-19 pandemic: outcomes from the UK ‘Alert Level 4’ phase of the B-MaP-C study
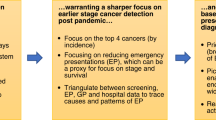
Transforming post pandemic cancer services
Introduction.
Approximately 60,000 people are diagnosed with breast cancer annually in the United Kingdom (UK) and the Republic of Ireland (RoI) [ 1 , 2 ]. Services for screening, diagnosing, treating and follow up of patients provided through national health care services varied by country. During both the initial phase of the COVID-19 pandemic in 2020 and throughout subsequent peaks in transmission, various restrictions were implemented that limited and/or changed how breast cancer was diagnosed, treated and managed in much of the world [ 3 ], including the UK and RoI. Given the importance of early detection and treatment of cancer, there is concern over how COVID- related service delays may affect cancer patients now and in the future regarding stage at diagnosis, prognosis and mortality [ 4 ]. Because potentially life-changing decisions about cancer patients’ care have been made rapidly without the benefit of prior experience, there has been a sudden increase in studies examining possible pandemic impacts on breast cancer services and patients. To better understand the full impact of the COVID-19 pandemic on breast cancer diagnosis, treatment and patient outcomes in the UK and RoI, we conducted a scoping review that would examine findings from several studies conducted in these countries.
Scoping reviews aim to rapidly map key concepts in a research area that have not been studied comprehensively and identify research gaps in the existing literature [ 5 ].
The present scoping review used Arksey and O’Malley’s [ 6 ] framework, minus the last step of expert validation of findings due to resource constraints. Generally, this type of review does not include a critical appraisal of the constituent material. The Preferred Reporting Items for Systematic reviews and Meta-Analyses extension for Scoping Reviews (PRISMA-ScR) checklist was used to report the review findings [ 7 ].
A systematic search was conducted on five electronic databases -- PubMed, Medline, Web of Science, Embase and PyschInfo -- using key words and MeSH headings for breast cancer services and outcomes in the countries of interest (Fig. 1 ). Inclusion criteria were publication in English in a peer-reviewed journal between 1 January 2020 and 31 August 2023, and reporting on primary data collected in the UK or RoI. Papers excluded from this report either did not meet the inclusion criteria or: described an intervention other than healthcare system changes or patient outcomes directly related to breast cancer; provided data from multiple locations without separately identifying results from the UK and/or the RoI; or were systematic reviews, conference abstracts, or proceedings, or unpublished (grey) literature. A hand search of the reference lists of each included paper was done.
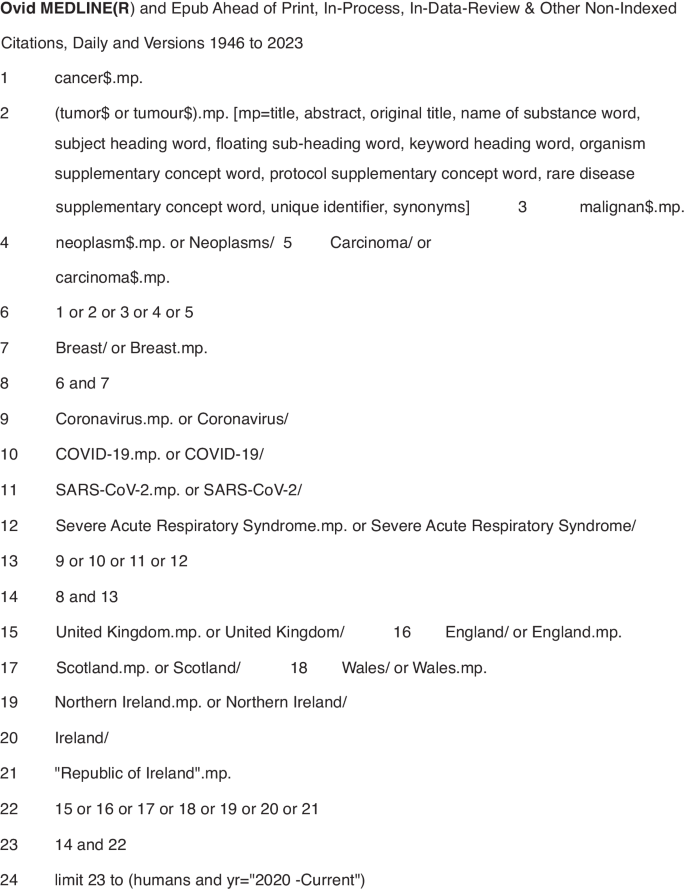
Symbols: $ is a wildcard to expand the search term and find both British and American spellings of the same word. .mp. means multi-purpose for an Advanced search without specifying a particular field. / means the term preceding it is from the MeSH headings in MEDLINE.
Results from each electronic database were imported into the Covidence systematic review software [ 8 ], an online tool to support doing systematic reviews that automatically removes duplicate entries. Title and abstract screening was done independently by three reviewers (CM, LL, MS) who discussed differences of opinion about papers’ eligibility until reaching consensus. After removing ineligible studies, the remaining papers were downloaded and independently screened by the reviewers against the inclusion and exclusion criteria. Any differences of opinion were resolved through discussion. The reviewers included a cancer epidemiologist, a public health professional and a medical anthropologist.
Data were extracted from the selected papers and entered into an Excel spreadsheet containing information on the bibliography (authors, title, journal, publication date), study aims and design, geographic location, and key findings (Table 1 , Supplementary Material). Results were then organised thematically to describe the impact of the COVID-19 pandemic on the organisation of breast cancer services, referrals/diagnosis and number of cases, and treatment.
A study protocol was not written and registered. The scoping review is part of a larger study on the impact of COVID-19 on breast cancer services in Northern Ireland.
The electronic database search returned 569 studies. Following duplicate removal ( n = 228), over half (176/341, 51.6%) of the screened studies were deemed irrelevant, leaving 165 studies for full-text review. Of these studies, 129 were excluded, primarily because they were published as a conference abstract. The remaining 34 papers used in the review included 16 studies conducted in England [ 9 , 10 , 11 , 12 , 13 , 14 , 15 , 16 , 17 , 18 , 19 , 20 , 21 , 22 , 23 , 24 ], four in Scotland [ 25 , 26 , 27 , 28 ], three in [ 29 , 30 , 31 ] Wales, one in Northern Ireland [ 32 ], three in the UK [ 33 , 34 , 35 ], one in Ireland [ 36 ] and six that used data from multiple countries which included at least one site in the UK and/or [ 37 , 38 , 39 , 40 , 41 , 42 ] RoI. No additional studies of interest were identified in the hand search of reference lists (Fig. 2 ).
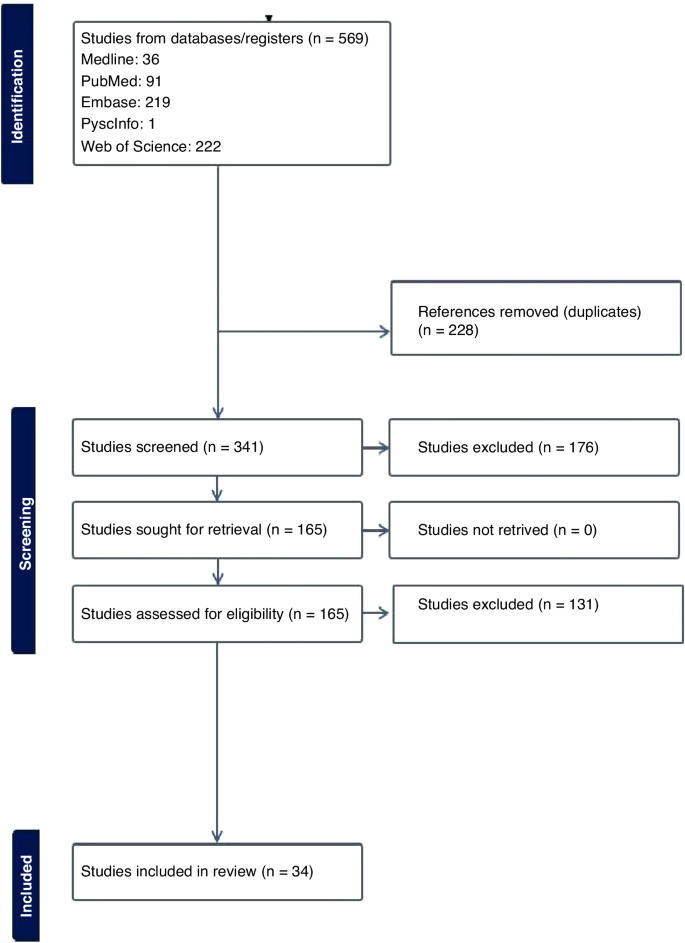
Prisma flowchart.
Impact of the COVID-19 pandemic on the organisation of breast cancer services
During the first wave of the COVID-19 pandemic (March–April 2020), population-based breast cancer screening programs were paused in many jurisdictions, including the UK and RoI. There were also major changes in how members of multidisciplinary teams (MDTs) met to develop treatment plans for breast cancer patients [ 11 , 37 ]. One study in an English hospital tested the acceptability of video-conferencing MDT meetings with participants attending in person or from a remote location. After overcoming minor technical difficulties (e.g. uninterrupted access to online meetings, ensuring participants had the necessary equipment to attend meetings remotely) all the participants indicated that online meetings were acceptable or their preferred mode of communication [ 11 ]. Another study surveyed breast pathologists in the UK and RoI who reported their MDTs often met in small virtual meetings [ 37 ]. Although nearly three-quarters of them indicated their workload and productivity decreased during the pandemic, 36% reported improved efficiency [ 37 ]. No study reported on the optimal balance between virtual and in-person meetings.
Three studies examined changes made to referral pathways to breast clinics or units in response to the COVID-19 pandemic [ 14 , 19 , 23 ]. One study, using data from England’s National Health Service, reported a 28% decline in referrals for suspected breast cancer during the first six months of 2020 compared to the same period in 2019 [ 14 ]. Another research group reported an even greater decline (−35%) in the number of women attending a one-stop rapid breast clinic in England during the initial lockdown (March-April 2020) compared to June-July that year [ 23 ].
A study reported on rapid adaptations made by a London-based breast cancer service in line with The Royal College of Surgeons guidelines to reduce the risk of COVID-19 [ 19 ]. Examples include providing space to maintain the recommended two metre distance between people; fewer appointments plus longer time between them to allow for thorough cleaning of surfaces; following stricter criteria for urgent referrals; and conducting routine follow-up appointments over the phone. In addition, although diagnostic imaging with ultrasound and mammogram continued to be available, all routine surveillance imaging was deferred for three months. Operations were conducted by small teams of specialists who travelled to a “cold” (free of COVID-19 cases) private hospital [ 19 ]. Virtual appointments quickly became the norm for many patients. However, as noted by one research team [ 14 ] this increased the potential for greater inequality of access to care by the elderly or people of lower socioeconomic status.
Several studies observed smaller-than-expected numbers of attendees at breast cancer screening and treatment centres [ 9 , 23 , 26 , 41 ]. This was noteworthy given the association between early detection through screening and the potential to reduce treatment needed potential to reduce treatment needed with better patient outcomes. Reasons for the downtrend in attendance ranged from centres issuing fewer invitations to ensure adequate time between appointments for cleaning equipment [ 26 ], to women declining invitations to be screened due to fears of being exposed to SARS-CoV-2 when in a healthcare facility [ 9 ].
Other investigators focused on how to effectively restart breast screening programs [ 18 , 26 ]. A Scottish study described the benefits of using a phased approach for this, giving priority to high-risk women, followed by recalling program participants, issuing new invitations to women of screening (age 50–70 years or older) or those who had missed or cancelled earlier appointments [ 26 ]. In another study [ 18 ], researchers in London investigated whether switching from sending women invitations to attend a specific appointment (“timed appointments”) to having them book their sessions (“open appointments”) would reduce the backlog of unscreened eligible women. Both invitation types were used between September 2020 and March 2021, allowing researchers to conduct a natural experiment to examine which approach had the greatest response [ 18 ]. The authors found significantly fewer women responded to the open than to the timed invitation (−7.5%) and estimated that if timed invitations were exclusively used approximately 12,000 more women would have attended screening and about 100 more women with breast cancer would have been detected [ 18 ].
The Impact of COVID-19 on referrals, diagnoses and numbers of patients with breast cancer
A major concern regarding COVID-19 is the possible effect that delaying or modifying diagnosis and treatment would have on patients, including those with symptomatic disease, and the potential for excess breast cancer deaths. An English study used national data to estimate the impact of curtailing screening during the first lockdown on predicted breast cancer deaths from 2020 to 2029. The authors estimated up to 687 additional deaths in that 10-year period [ 13 ]. Routinely collected NHS England data were used to compare referral patterns and time to first treatment for breast cancer during the pandemic (first half of 2020) compared to the same period in 2019 [ 14 ]. Results showed a 28% decrease in diagnostic services and 16% of patients receiving their first treatment. They also noted that hormonal therapy, administered in tablet form, had become a frequent alternative to surgery – the mainstay treatment for breast cancer before the pandemic [ 14 ].
Five studies reported on the number of new breast cancer cases during the pandemic in Wales and England [ 10 , 20 , 22 , 29 , 30 ], with results varying widely by location and time period. For example, a Welsh study [ 29 ] found a 2% reduction of cases in April 2020 compared to the same period in 2019, whereas an English study reported a 17.9% reduction in March-April 2020 versus 2019 [ 20 ]. Three other English studies [ 10 , 22 , 30 ] reported reductions in the number of new diagnoses ranging from 19.1% to 29.5%.
Four studies [ 10 , 22 , 28 , 30 ] reported on changes in disease severity or stage of cancer at diagnosis, finding clear evidence of stage migration to more advanced cases attributed to delayed diagnosis of new cases.
Most breast cancer diagnoses are confirmed through pathology. A study [ 32 ] from Northern Ireland compared the number of pathologically-diagnosed (PD) breast cancer cases before the pandemic (2017–2019) with numbers during the early pandemic. The researchers found 105 fewer breast cancer cases in 2020, with the greatest reductions in the early months (−40% in April, −52% in May) [ 32 ]. A UK-based study [ 39 ] compared population-based cancer registry data from Northern Ireland, Scotland and Wales, with sharp declines in the number of patients with breast cancers in each country (−53.5% in Northern Ireland, −45.3% in Scotland, −43.5% in Wales). The finding of fewer PD-confirmed cases of breast cancer was also reported in a study [ 36 ] conducted in the histopathology departments of two university hospitals in Northwest RoI. The larger hospital reported a decline of 21.5% and 14.4% in the first six months of 2020 compared to 2019 for samples from small biopsy diagnostic procedures and cancer resection cases, respectively [ 36 ].
The Impact of COVID-19 on Treatment: As noted in several studies [ 17 , 21 , 24 , 25 , 31 , 34 , 35 , 40 , 42 ], efforts to reduce the risk of exposure to COVID-19 SARS-CoV-2 for patients and healthcare providers resulted in fewer surgical, radiotherapy or systemic treatments of breast cancer patients. There were also changes to facility procedures used to reduce the amount of time patients were potentially exposed in medical facilities.
Four studies [ 17 , 21 , 40 , 42 ] addressed changes to surgical treatment during the pandemic. One of them reported on an international web-based poll with over 100 oncological surgeons that included practitioners from the UK. In both Scotland and England, surgical priority was given to patients with ER-negative disease first followed by those with HER2-positive disease, and that neoadjuvant chemotherapy was to be given following standard criteria. In England, there was also a recommendation to focus on providing minimal treatment via day surgery, with neoadjuvant chemotherapy to be reserved for patients whose disease was deemed to be inoperable [ 42 ].
Another study found a 34% decline in “radical surgery with curative intent” for breast cancer done in a large London cancer centre from March to September 2020 compared to 2019 [ 40 ]. Surgical practices were also altered, such as having procedures done by only consultant surgeons because junior doctors were redeployed to COVID-19-related duties during the first two months of the pandemic [ 40 ]. Another study [ 21 ], conducted at the Oxford University Hospitals in England, reported the unit followed recommendations from the Association of Breast Surgery and did not perform immediate or delayed breast reconstruction between the start of lockdown (23 March 2020) and the end of May despite the known psychological and physical benefits of immediate reconstruction for many women. In two English hospitals surgical procedures continued during the pandemic but at greatly reduced numbers compared to 2019, with declines in both immediate and delayed reconstructive surgeries. Patients also had significantly shorter hospital stays post-surgery [ 17 ].
Widespread changes to radiotherapy regimens also occurred during the pandemic. Earlier, conventional treatment entailed giving 40–42.5 Gray (Gy) units of radiation divided into 15 treatments or ‘fractions’ (F) over a 3-week period. During the pandemic, this protocol was replaced in many centres with a hypofractionated radiation regimen consisting of a smaller amount of radiation divided into five treatments given over a week (26GyF5). The impetus for this was the publication of guidelines by The Royal College of Radiologists [ 43 ] recommending this shift based on findings from the FAST-Forward non-inferiority trial [ 44 ] and the B-MaP-C study [ 45 ].
Radiation oncology teams quickly complied, reporting increases during the pandemic (up from 13 to 48% in Wales, [ 31 ] and 0.2% to 60.6% in England [ 24 ] and 2.7% to 46.1% in Scotland [ 27 ]), as well as during the pandemic. (up from <1% in February to 70% in April 2020 in a study from England and Wales [ 38 ]).
Another four studies [ 12 , 25 , 34 , 35 ] examined changes in systemic anticancer treatment (SACT), noting this was used as a “bridging” or pre-operative treatment while waiting for breast cancer surgery during the pandemic. One study from England [ 42 ] found a 33% decrease in the number of patients registered for SACT immediately after the initial lockdown (April–June 2020) compared to numbers from September 2019 to February 2020.
Modifying or halting cancer treatments was also identified in the B-Map-C study [ 45 ] -- a multicentre national project involving 64 breast units in the UK – which reported that 59% of all breast cancer patients received a “COVID-altered” management plan (e.g. interrupted neoadjuvant chemotherapy or bridging endocrine therapy instead of surgery) during the initial pandemic period from March 16 to May 8, 2020 [ 34 ]. In contrast, a study conducted in a hospital in England found that 56% of women being treated for breast cancer chose to continue SACT despite clear recommendations from the National Institute for Health and Care Excellence (NICE guidelines) [ 46 ] that such treatment should stop during the pandemic to reduce the risk of exposure to SARS-CoV-2 in a hospital setting. Some authors suggest this indicates that many patients feared the effects of not treating their cancer more than they feared COVID-19 [ 35 ].
The studies included in this scoping review identified unprecedented changes to breast cancer services over a short period of time. During the COVID-19 pandemic people with non-urgent stage disease typically diagnosed via screening (e.g. breast, colorectal or cervical cancer) saw a decrease in the number of new cases due to temporary closures or reduced healthcare facility capacity [ 47 ]. This pattern is borne out by population-based data from national cancer registries reporting 11–21% fewer cases diagnosed during the pandemic in ROI [ 1 ] and the UK [ 47 , 48 , 49 , 50 , 51 ] despite a year-on-year increase in cases.
Evidence exists for both overdiagnosis and benefits from diagnosing breast cancer through screening. [ 52 ] It is inevitable that pauses in population-based screening programs during the pandemic resulted in fewer early-stage cancers being diagnosed. However, the long-term deleterious effects of halting screening programs during health emergencies has yet to be determined. None of the included papers in the review were able to provide evidence of direct harm to patients due to reduced detection rates, despite evidence of more advanced disease on detection. In fact, one study clearly indicated that such delays may have less of an impact than commonly believed for surgeries conducted <12 weeks after diagnosis [ 53 ]. The full extent of harm caused to people with breast cancer can only be answered once enough data comparing outcomes related to delayed services before, during and after the pandemic have been analysed.
The studies examined in this scoping review point to efforts made to continue to offer timely services, including early detection and treatment, with a focus on identifying high-priority patients based on tumour- and patient-related characteristics [ 52 ] taking into account availability of healthcare personnel and services during the pandemic [ 54 , 55 ]. Recovery plans for future emergencies [ 56 ] must help implementers decide whether to prioritise rapid resumption of breast screening programs or preserve symptomatic diagnostic services [ 4 ] while taking measures to minimise the risk of communicable disease transmission for patients and staff in breast clinics [ 33 ].
There are also lessons to be learned about the benefits of rapidly incorporating evidence from high-quality studies, such as the FAST-FORWARD clinical trial demonstrating the effectiveness of hypofractionated radiotherapy for eligible patients, into clinical practice during the pandemic [ 44 ]. Another modification was to preferentially offer neoadjuvant therapy over surgery for triple negative or HER2+ patients during the pandemic. This likely was to reduce through flow in chemotherapy departments, thereby reducing the risk of exposing immunocompromised patients to SARS-CoV-2 [ 28 ], although future studies will be needed to determine the effectiveness and long-term impact of this change.
It is also important to adapt international guidelines to fit local conditions [ 57 ]. Factors to consider would be how to continue providing services while safeguarding patients and staff given local resources, what criteria to use when identifying high-priority patients during times of reduced service availability, ensuring that resources are available for increased use of remote/virtual consultations and MDT meetings, as well as developing locally acceptable approaches to phasing in full services post-emergency [ 58 ].
Other recommendations for breast cancer programs focus on ways to avoid undertreatment with neoadjuvant therapy and, in some cases, providing breast-conserving operations [ 54 ] in “clean” surgical sites even during a health emergency. Benefits from continuing to operate include ensuring that surgical trainees continue developing their skills, and so there will be more clinicians available to help reduce the backlog of patients once operations resume [ 54 ]. Second, it should reduce the number of women experiencing unnecessary anxiety and depression, which have been found in patients waiting considerable time for their breast surgery [ 59 , 60 ]. Third, as recommended by the British Association of Plastic, Reconstructive and Aesthetic Surgeons in the UK [ 61 ], resuming breast reconstruction quickly can help prevent unnecessarily long or repeat procedures due to tissue change that occurs over time after a mastectomy, which increase hospital stay and potentially the risk of exposure to SARS-CoV-2. However, the link between length of stay and infection rates has yet to be proven. It is also important to consider the surgical environment, as noted by The Royal College of Surgeons in May 2020 [ 62 ]. This included guidelines for the “four Ps”: the Place for surgeries should be reconfigured to provide a safe setting for patients and clinicians; People should return to their pre-COVID work in order to reduce the backlog of elective cases; PPE should be made available for all staff; and no major surgery for Positive Tests (i.e. if patients test positive for COVID-19) except for life-, (limb- or sight-saving procedures) [ 21 ]. Future research will determine if these actions are effective in reducing the risk of infection with SARS-CoV-2.
Public awareness campaigns should also be delivered that includes the clear communication [ 55 ] for people with relevant symptoms to seek medical care promptly [ 57 ], even at the height of a pandemic or other emergency.
Looking to the future, it will be important to fund research on the long-term impact of delayed or interrupted breast cancer services on patient outcomes such as cancer incidence, stage, tumour size and ultimately survival [ 15 , 16 , 63 ]. For instance, previous studies have found survival differences for women with breast cancer only if the delay in services was longer than 12 weeks [ 53 , 62 ]. Several of the papers in this review reported results from single-site retrospective studies [ 62 ], which is problematic because it is not possible to generalise their findings to other settings or populations. This problem can be alleviated by using data from multicentre investigations and national cancer registries. However, there are issues with obtaining timely information from registries. First, many registries do not have data on cancer recurrences, which makes it difficult to accurately assess the impact of health emergencies. Efforts to address this gap are being led by the European Network of Cancer Registries [ 58 ]. Second, cancer registries use patient-level data retrospectively after they are received and cleaned. Further delays in producing reports were identified during the COVID-19 pandemic, when monitoring was curtailed due to registry staff working off-site or allocated to pandemic-related duties. This delayed data analysis and report preparation. Several registries have reported they can address such problems in the future by adopting novel methods for more quickly assessing the impact of modified and interrupted services during health emergencies [ 64 ].
Although studies have documented changes in the breast cancer service profile and outcomes during the COVID-19 pandemic, there is no evidence available on whether these measures helped minimise the spread of the SARS-CoV-2 infection. Further research is also needed on the long-term effects of changes to breast cancer services for patients who had advanced disease on initial presentation or whose treatment was delayed [ 65 ]. Findings from such studies can be used to update models that predict the number of excess deaths from breast cancer due to interrupting care [ 66 ].
Studies are needed to provide insights into the following: how health emergencies affect the cost and availability of services while considering how closely they follow disaster preparedness guidelines; more accurate estimates of cancer risks and consequences for designing optimal recovery strategies [ 59 , 60 , 61 ]; and recommendations on how to address the backlog of breast cancer cases requiring surgery or other treatment in a timely and safe manner [ 67 , 68 ].
Perhaps the most important gap in current literature on the impact of COVID-19 on breast cancer services and patients is research to document the patient voice and experience, as well as research to evaluate improvements in service timeliness and efficiency during the pandemic which has not compromised patient satisfaction and safety.
Health emergencies like the COVID-19 pandemic are the norm rather than the exception. There are valuable lessons to be learned from existing studies conducted in the short time since the end of the pandemic. There is also a need to pool data and design future studies to provide more evidence to guide future plans on how to best meet the needs of women (and men) with breast cancer during future emergencies. It is impossible to completely prepare for future health emergencies, especially those involving novel pathogens. Evidence extrapolated from other infectious diseases, and recommendations by experts (e.g. oncologists, pathologists and patients) on how to better manage cancer treatments in future emergencies should be considered [ 69 ].
Strengths and limitations
To our knowledge, this is the first scoping review to examine the published literature on the impact of the COVID-19 pandemic on breast cancer services and patient outcomes in the UK and RoI. The review was conducted following a strict protocol carried out by three reviewers with conflicts resolved by consensus.
Because of the short time since the end of the pandemic, findings from more definitive, longitudinal, population-based studies were not available to include in this review. The authors also chosen not to review the grey literature because there is no established guidelines for producing a rigorous review of material that does not meet the level of evidence expected by healthcare providers, commissioners and policymakers.
Another limitation is the wide variation in study design and context, such as the stage of the pandemic when data were being collected, among the studies included in the review. Of particular concern was the large number of retrospective, single-centre studies with data from a relatively homogeneous population, making it difficult to generalise findings beyond a particular study setting.
This scoping review presents a coherent picture of current published knowledge on the impact of the COVID-19 pandemic on breast cancer services and patient outcomes in the UK and RoI. It also recommends ways to fill current knowledge gaps on this topic, summarising findings from studies documenting changes made to breast cancer services provided during the COVID-19 pandemic in the UK and RoI.
The long-term impact of these changes are still unknown. Lessons for future disaster preparedness will come from large-scale, multisite studies and cancer registries using data collected before, during and after the pandemic. Results will be useful for developing guidelines to help reduce the impact of future medical emergencies on people with breast cancer and on healthcare systems and providers.
Data availability
The dataset generated and/or analysed during the current study is available from the corresponding author on reasonable request.
Ireland NCR. Data & statistics 2024[Available from: https://www.ncri.ie/data ].
UK CR. Breast cancer statistics [Available from: https://www.cancerresearchuk.org/healthprofessional/cancer-statistics/statistics-by-cancer-type/breast-cancer ].
Spicer J, Chamberlain C, Papa S. Provision of cancer care during the COVID-19 pandemic. Nat Rev Clin Oncol. 2020;17:329–31.
Article CAS PubMed PubMed Central Google Scholar
Figueroa JD, Gray E, Pashayan N, Deandrea S, Karch A, Vale DB, et al. The impact of the Covid-19 pandemic on breast cancer early detection and screening. Prev Med. 2021;151:106585.
Article PubMed PubMed Central Google Scholar
Mays N, Pope C, Popay J. Systematically reviewing qualitative and quantitative evidence to inform management and policy-making in the health field. J Health Serv Res Policy. 2005;10:6–20.
Article PubMed Google Scholar
Arksey H, O’Malley L. Scoping studies: towards a methodological framework. Int J Soc Res Methodol. 2005;8:19–32.
Article Google Scholar
Tricco AC, Lillie E, Zarin W, O’Brien KK, Colquhoun H, Levac D, et al. PRISMA extension for scoping reviews (PRISMA-ScR): checklist and explanation. Ann Intern Med. 2018;169:467–73.
Covidence systematic review software. Veritas Health Innovation, Melbourne, Australia.
Armitage RC, Morling JR. The impact of COVID-19 on national screening programmes in England. Public Health. 2021;198:174–6.
Article CAS PubMed Google Scholar
Borsky K, Shah K, Cunnick G, Tsang-Wright F. Pattern of breast cancer presentation during the COVID-19 pandemic: results from a cohort study in the UK. Future Oncol. 2022;18:437–43.
Cathcart P, Smith S, Clayton G. Strengths and limitations of video-conference multidisciplinary management of breast disease during the COVID-19 pandemic. Br J Surg. 2021;108:e20–e1.
Clark JJ, Dwyer D, Pinwill N, Clark P, Johnson P, Hackshaw A. The effect of clinical decision making for initiation of systemic anticancer treatments in response to the COVID-19 pandemic in England: a retrospective analysis. Lancet Oncol. 2021;22:66–73.
Duffy SW, Seedat F, Kearins O, Press M, Walton J, Myles J, et al. The projected impact of the COVID-19 lockdown on breast cancer deaths in England due to the cessation of population screening: a national estimation. Br J Cancer. 2022;126:1355–61.
Gathani T, Clayton G, MacInnes E, Horgan K. The COVID-19 pandemic and impact on breast cancer diagnoses: what happened in England in the first half of 2020. Br J Cancer. 2021;124:710–2.
Gathani T, Reeves G, Dodwell D, Horgan K, Kearins O, Kan SW, et al. Impact of the COVID-19 pandemic on breast cancer referrals and diagnoses in 2020 and 2021: a population-based study in England. Br J Surg. 2022;109:29–30.
Gathani T, Dodwell D, Horgan K. The impact of the first 2 years of the COVID-19 pandemic on breast cancer diagnoses: a population-based study in England. Br J Cancer. 2023;128:481–3.
Ho W, Köhler G, Haywood RM, Rosich-Medina A, Masud D. Microsurgical autologous breast reconstruction in the midst of a pandemic: A single-unit COVID-19 experience. J Plast Reconstr Aesthet Surg. 2022;75:112–7.
Hudson SM, Binysh K, Duffy SW. Did the use of open invitations in place of timed appointment invitations reduce the uptake of breast screening in the London region during the COVID-19 recovery? J Med Screen. 2023;30:87–91.
Joseph AO, Joseph JP, Pereira B, Gahir J. Coronavirus outbreak: reorganising the breast unit during a pandemic. Eur J Surg Oncol. 2020;46:1176–7.
MacInnes EG, Piper J, Tait C, Waterworth A, Achuthan R, Hogan B, et al. Breast cancer surgery during the COVID-19 pandemic peak in the UK: operative outcomes. Cureus 2020;12:e9280.
PubMed PubMed Central Google Scholar
Markeson D, Freeman Romilly N, Potter M, Tucker S, Kalu P. Restarting plastic surgery: drawing on the experience of the initial COVID-19 pandemic to inform the safe resumption of services. J Plast Reconstr Aesthet Surg. 2020;73:2121–6.
Purushotham A, Roberts G, Haire K, Dodkins J, Harvey-Jones E, Han L, et al. The impact of national non-pharmaceutical interventions (‘lockdowns’) on the presentation of cancer patients. Ecancermedicalscience 2021;15:1180.
Shetty G, Datta U, Rea I, Rai S, Hwang MJ, Hoar F, et al. Rapid implementation of triaging system for assessment of breast referrals from primary care centres during the COVID-19 pandemic. Ann R Coll Surg Engl. 2021;103:576–82.
Spencer K, Jones CM, Girdler R, Roe C, Sharpe M, Lawton S, et al. The impact of the COVID-19 pandemic on radiotherapy services in England, UK: a population-based study. Lancet Oncol. 2021;22:309–20.
Baxter MA, Murphy J, Cameron D, Jordan J, Crearie C, Lilley C, et al. The impact of COVID-19 on systemic anticancer treatment delivery in Scotland. Br J Cancer. 2021;124:1353–6.
Campbell C, Sommerfield T, Clark GRC, Porteous L, Milne AM, Millar R, et al. COVID-19 and cancer screening in Scotland: a national and coordinated approach to minimising harm. Prev Med. 2021;151:106606.
Grocutt L, Rutherford A, Caldwell D, Wilkinson C, Chalmers AJ, Dempsey L, et al. The impact of COVID-19 on radiotherapy services in Scotland, UK: a population-based study. Clin Oncol. 2023;35:e227–e34.
Article CAS Google Scholar
Romics L, Doughty J, Stallard S, Mansell J, Blackhall V, Lannigan A, et al. A prospective cohort study of the safety of breast cancer surgery during COVID-19 pandemic in the West of Scotland. Breast 2021;55:1–6.
Bansal GJ, Saleem Z. The symptomatic breast services in a university hospital: pandemic peak compared to the pre-pandemic year and future implications. Ir J Med Sci. 2022;191:2475–9.
Greene G, Griffiths R, Han J, Akbari A, Jones M, Lyons J, et al. Impact of the SARS-CoV-2 pandemic on female breast, colorectal and non-small cell lung cancer incidence, stage and healthcare pathway to diagnosis during 2020 in Wales, UK, using a national cancer clinical record system. Br J Cancer. 2022;127:558–68.
Higgins E, Walters S, Powell E, Staffurth J. The impact of the acute phase of COVID-19 on radiotherapy demand in South East Wales. Clin Oncol (R Coll Radio). 2020;32:e217.
Mitchell H, McLean J, Gavin AT, Visser O, Millar E, Luff T, et al. Impact of COVID-19 control on lung, breast, and colorectal pathological cancer diagnoses. A comparison between the Netherlands, Aotearoa New Zealand, and Northern Ireland. BMC Cancer. 2023;23:700.
Bansal GJ, Chopra S. Symptomatic breast services in the post-COVID-19 era. Br J Hosp Med. 2021;82:1–3.
Dave RV, Kim B, Courtney A, O’Connell R, Rattay T, Taxiarchi VP, et al. Publisher Correction: breast cancer management pathways during the COVID-19 pandemic: outcomes from the UK ’Alert Level 4’ phase of the B-MaP-C study. Br J Cancer. 2021;125:905.
Gatfield ER, Mukesh MB, Loo SW. Adjuvant systemic anti-cancer therapy in early breast cancer during the COVID-19 pandemic: differences between clinicians and patients in perception of treatment risks and benefits. Clin Oncol. 2020;32:e218.
O’Connor E, O’Dowd G, Phelan S. Impact of COVID-19 on small biopsy diagnostic procedures and cancer resection surgeries in the North-West of Ireland. J Clin Pathol. 2022;75:270–3.
Elghobashy M, Wahab L, Gunavardhan A, O’Sullivan E, Provenzano E, Deb R, et al. Impact of COVID-19 on the practice of breast pathologists: a survey of breast pathologists in the UK and Ireland. J Clin Pathol. 2023;76:234–8.
Gannon MR, Dodwell D, Miller K, Horgan K, Clements K, Medina J, et al. Change in the use of fractionation in radiotherapy used for early breast cancer at the start of the COVID-19 pandemic: a population-based cohort study of older women in England and Wales. Clin Oncol. 2022;34:e400–e9.
Greene GJ, Thomson CS, Donnelly D, Chung D, Bhatti L, Gavin AT, et al. Whole-population trends in pathology-confirmed cancer incidence in Northern Ireland, Scotland and Wales during the SARS-CoV-2 pandemic: a retrospective observational study. Cancer Epidemiol. 2023;84:102367.
Monroy-Iglesias MJ, Tagliabue M, Dickinson H, Roberts G, De Berardinis R, Russell B, et al. Continuity of cancer care: the surgical experience of two large cancer hubs in London and Milan. Cancers. 2021;13:1597.
Puricelli Perin DM, Elfström KM, Bulliard JL, Burón A, Campbell C, Flugelman AA, et al. Early assessment of the first wave of the COVID-19 pandemic on cancer screening services: The International Cancer Screening Network COVID-19 survey. Prev Med. 2021;151:106642.
Rocco N, Montagna G, Di Micco R, Benson J, Criscitiello C, Chen L, et al. The impact of the COVID-19 pandemic on surgical management of breast cancer: global trends and future perspectives. Oncologist 2021;26:e66–e77.
All our publications | The Royal College of Radiologists 2024 [Available from: https://www.rcr.ac.uk/our-services/all-our-publications/ .
Murray Brunt A, Haviland JS, Wheatley DA, Sydenham MA, Alhasso A, Bloomfield DJ, et al. Hypofractionated breast radiotherapy for 1 week versus 3 weeks (FAST-Forward): 5-year efficacy and late normal tissue effects results from a multicentre, non-inferiority, randomised, phase 3 trial. Lancet 2020;395:1613–26.
Courtney A, O’Connell R, Rattay T, Kim B, Cutress RI, Kirwan CC, et al. The B-MaP-C study: breast cancer management pathways during the COVID-19 pandemic. Study protocol. Int J Surg Protoc. 2020;24:1–5.
National Institute for Health and Care Excellence (NICE). 2022. COVID-19 rapid guideline: delivery of systemic anticancer treatments. [Available from: https://www.nice.org.uik/guidance/NG161 ].
Northern Ireland Cancer Registry (NICR). 2024. Official Statistics: Cancer incidence and survival statistics for Northern Ireland: 1993-2021. [Available from: https://www.qub.ac.uk/research-centres/nicr/CancerInformation/official-statistics/ ].
NHS England. 2022. Cancer registration statistics, England 2020. [Available from: https://digitanl.nhs.uk/data-and-information/publications/statistical/cancer-registration-statistics/england-2020 ].
Breast Cancer Now. 2024. How the pandemic impacted breast cancer diagnosis in Wales [Available from: https://breastcancernow.org/about-us/campaign-news/how-pandemic-impacted-breast-cancer-diagnosis-in-wales/ ].
Marmot M, Allen J, Goldblatt P, Herd E, Morrison J Build Back Fairer: The COVID-19 Marmot Review. [Available from: https://www.health.org.uk/publications/build-back-fairer-the-covid-19-marmot-review ].
Public Health Scotland. 2022. Scottish Public Health Observatory quarterly update. [Available from: https://publichealthscotland.scot/publications/scottish-public-health-observatory-quarterly-update/scottish-publich-health0s/scottish-public-health-observatory-quarterly-update-march-2022 ].
Gasparri ML, Gentilini OD, Lueftner D, Kuehn T, Kaidar-Person O, Poortmans P. Changes in breast cancer management during the Corona Virus Disease 19 pandemic: an international survey of the European Breast Cancer Research Association of Surgical Trialists (EUBREAST). Breast 2020;52:110–5.
Bleicher RJ. Timing and delays in breast cancer evaluation and treatment. Ann Surg Oncol. 2018;25:2829–38.
Petropoulou Z, Arkadopoulos N, Michalopoulos NV breast cancer and COVID-19: challenges in surgical management. Cancers. 2022;14:5360.
Feletto E, Grogan P, Nickson C, Smith M, Canfell K, How has COVID-19 impacted cancer screening? Adaptation of services and the future outlook in Australia. Public Health Res Pract. 2020;30:e042026.
Hamilton W. Cancer diagnostic delay in the COVID-19 era: what happens next? Lancet Oncol. 2020;21:1000–2.
Linck PA, Garnier C, Depetiteville MP, MacGrogan G, Mathoulin-Pélissier S, Quénel-Tueux N, et al. Impact of the COVID-19 lockdown in France on the diagnosis and staging of breast cancers in a tertiary cancer centre. Eur Radio. 2022;32:1644–51.
European Network of Cancer Registries. 2024. Working groups. [Available from: https://encr.eu/Activities/Working-groups .]
Maringe C, Spicer J, Morris M, Purushotham A, Nolte E, Sullivan R, et al. The impact of the COVID-19 pandemic on cancer deaths due to delays in diagnosis in England, UK: a national, population-based, modelling study. Lancet Oncol. 2020;21:1023–34.
Degeling K, Baxter NN, Emery J, Jenkins MA, Franchini F, Gibbs P, et al. An inverse stage-shift model to estimate the excess mortality and health economic impact of delayed access to cancer services due to the COVID-19 pandemic. Asia Pac J Clin Oncol. 2021;17:359–67.
Yong JH, Mainprize JG, Yaffe MJ, Ruan Y, Poirier AE, Coldman A, et al. The impact of episodic screening interruption: COVID-19 and population-based cancer screening in Canada. J Med Screen. 2021;28:100–7.
Kothari A, Fentiman IS. 22. Diagnostic delays in breast cancer and impact on survival. Int J Clin Pr. 2003;57:200–3.
Toss A, Callegari V, Cortesi G, Civallero M, Armocida C, Piacentini F. COVID-related disruption in mammographic screening: a year later. ESMO Open. 2022;7:100539.
National Disease Registration Service. 2021. COVID-19 rapid cancer registration and treatment data. [Available from: https://www.cancerdata.nhs.uk/covid-19/rcrd .]
Trojanowski M, Radomyski P, Matuszewski K, Litwiniuk M, Wierzchosławska E, Kycler W, Impact of the COVID-19 pandemic on breast cancer stage at diagnosis in a regional cancer center in Poland between 2019 and 2021. J Pers Med. 2022;12:1486.
Walker MJ, Meggetto O, Gao J, Espino-Hernández G, Jembere N, Bravo CA, et al. Measuring the impact of the COVID-19 pandemic on organized cancer screening and diagnostic follow-up care in Ontario, Canada: a provincial, population-based study. Prev Med. 2021;151:106586.
Miller MM, Meneveau MO, Rochman CM, Schroen AT, Lattimore CM, Gaspard PA, et al. Impact of the COVID-19 pandemic on breast cancer screening volumes and patient screening behaviors. Breast Cancer Res Treat. 2021;189:237–46.
DeBoer RJ, Fadelu TA, Shulman LN, Van Loon K. Applying Lessons learned from low-resource settings to prioritize cancer care in a pandemic. JAMA Oncol. 2020;6:1429–33.
Curigliano G, Banerjee S, Cervantes A, Garassino MC, Garrido P, Girard N, et al. Managing cancer patients during the COVID-19 pandemic: an ESMO multidisciplinary expert consensus. Ann Oncol. 2020;31:1320–35.
Download references
Acknowledgements
Our thanks go to Breast Cancer Now, the research and support charity that provided funding for the “Impact of the COVID-19 Pandemic on the Diagnosis and Treatment of Breast Cancer” project, of which this scoping review is a part. We also thank Ms Paula Darragh and Dr Jamie Roebuck (Cancer Intelligence Officers, Northern Ireland Cancer Registry) for their work on the project.
This review was funded by Breast Cancer Now as part of a larger “Understanding the Impact of COVID-19 on Breast Cancer Services in Northern Ireland” study. The funder played no role in the decisions made during this review.
Author information
These authors contributed equally: Lynne Lohfeld, Meenakshi Sharma.
Authors and Affiliations
Queen’s University Belfast, Centre for Public Health, School of Medicine, Dentistry & Biomedical Sciences, Royal Victoria Hospital, 247 Grosvenor Road, Belfast, BT12 6BA, Northern Ireland, UK
Lynne Lohfeld, Meenakshi Sharma, Anna Gavin, Sinéad T. Hawkins & Charlene M. McShane
Northern Ireland Cancer Registry, Centre for Public Health, School of Medicine, Dentistry & Biomedical Sciences, Queen’s University Belfast, Mulhouse Building, Grosvenor Road, Belfast, BT12 6DP, Northern Ireland, UK
Damien Bennett, Anna Gavin, Sinéad T. Hawkins & Helen Mitchell
Belfast Health and Social Care Trust, 51 Lisburn Road, Belfast, BT9 7AB, Northern Ireland, UK
Gareth Irwin & Siobhan O’Neill
You can also search for this author in PubMed Google Scholar
Contributions
LL conceived and designed the work, acquired the data, played an important role in interpreting the results, drafted and revised the manuscript, approved the final version, and agreed to be accountable for all aspects of the work in ensuring that questions related to the accuracy or integrity of any part of the work are appropriately investigated and resolved. MS and CMMcS conceived and designed the work, acquired the data, played an important role in interpreting the results, revised the manuscript, approved the final version, and agreed to be accountable for all aspects of the work. DB, AG, STH, GI, HM, and SON conceived the work, played an important role in interpreting the results, revised the manuscript, approved the final version and agreed to be accountable for all aspects of the work.
Corresponding author
Correspondence to Lynne Lohfeld .
Ethics declarations
Competing interests.
The authors declare no competing interests.
Ethics approval and consent to participate
Competing interestsNo ethics approval or consent to participate was needed for this scoping review of published literature.
Consent for publication
No consent for publication was needed for this scoping review.
Additional information
Publisher’s note Springer Nature remains neutral with regard to jurisdictional claims in published maps and institutional affiliations.
Supplementary information
Table 1: characteristics of 34 included studies, preferred reporting items for systematic reviews and meta-analyses extension for scoping reviews (prisma-scr) checklist, rights and permissions.
Open Access This article is licensed under a Creative Commons Attribution 4.0 International License, which permits use, sharing, adaptation, distribution and reproduction in any medium or format, as long as you give appropriate credit to the original author(s) and the source, provide a link to the Creative Commons licence, and indicate if changes were made. The images or other third party material in this article are included in the article’s Creative Commons licence, unless indicated otherwise in a credit line to the material. If material is not included in the article’s Creative Commons licence and your intended use is not permitted by statutory regulation or exceeds the permitted use, you will need to obtain permission directly from the copyright holder. To view a copy of this licence, visit http://creativecommons.org/licenses/by/4.0/ .
Reprints and permissions
About this article
Cite this article.
Lohfeld, L., Sharma, M., Bennett, D. et al. Impact of the COVID-19 pandemic on breast cancer patient pathways and outcomes in the United Kingdom and the Republic of Ireland – a scoping review. Br J Cancer (2024). https://doi.org/10.1038/s41416-024-02703-w
Download citation
Received : 27 November 2023
Revised : 12 April 2024
Accepted : 22 April 2024
Published : 04 May 2024
DOI : https://doi.org/10.1038/s41416-024-02703-w
Share this article
Anyone you share the following link with will be able to read this content:
Sorry, a shareable link is not currently available for this article.
Provided by the Springer Nature SharedIt content-sharing initiative
Quick links
- Explore articles by subject
- Guide to authors
- Editorial policies

The Risk of Breast Implant-Associated Anaplastic Large Cell Lymphoma; A Systematic Review and Meta-Analysis
- Breast Surgery
- Open access
- Published: 09 May 2024
Cite this article
You have full access to this open access article
- Ali Mohamed Elameen 1 ,
- Mohamed Awad AlMarakby 2 ,
- Tarek Ibrahim Atta 3 &
- Asmaa Ali Dahy 2
75 Accesses
Explore all metrics
Breast implant-associated anaplastic large cell lymphoma (BIA-ALCL) is an emerging disorder that has gained global attention throughout the past era. The present meta-analysis was performed to retrieve the risk of BIA-ALCL from population-based epidemiological studies. Factors associated with BIA-ALCL were evaluated to identify patients at higher risk of BIA-ALCL.
A systematic literature search was executed throughout 12 databases. All epidemiological studies encompassing patients with breast implants either for aesthetic or reconstructive purposes and reported the risk of BIA-ALCL were included. Studies reported the risk factors of BIA-ALCL were included.
The present meta-analysis included 17 articles, encompassing 525,475 patients with breast implants. There were 254 patients with BIA-ALCL with a mean duration to the diagnosis of BIA-ALCL of 13.16 years (95% CI 11.7–14.6, P < 0.001). There were 44 patients with textured breast implants and two with smooth implants. Patients with breast implants were 28.86 times more at high risk of BI-ALCL (95% CI 3.123–266.681). The risk ranged from 0 to 1 per 1000 cases with breast implants, with a similar risk among patients seeking aesthetic and reconstructive surgeries. The risk was 0 to 1 case per 1000 cases among patients with textured breast implants. There was a significant association between the history of breast cancer and BIA-ALCL ( P = 0.0016).
This meta-analysis confirmed the association between breast implants and ALCL. There was a similar risk of BIA-ALCL among patients with aesthetic or reconstructive surgeries. Patients with a history of breast cancer were at higher risk of BIA-ALCL.
Level of Evidence III
This journal requires that authors assign a level of evidence to each article. For a full description of these Evidence-Based Medicine ratings, please refer to the Table of Contents or the online Instructions to Authors www.springer.com/00266 .
Similar content being viewed by others

Current Considerations of Breast Implant–Associated Anaplastic Large Cell Lymphoma in Breast Surgery: a Systematic Review
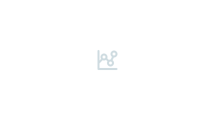
Is Breast Implant Associated—Anaplastic Large Cell Lymphoma linked to textured implants?
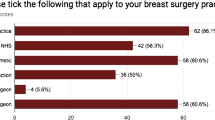
The current UK perspective of breast surgeons on breast implant-associated anaplastic large cell lymphoma (BIA-ALCL)
Avoid common mistakes on your manuscript.
Introduction
Aesthetic and reconstructive breast surgeries are among the most performed surgical procedures worldwide. In the USA, more than 500,000 patients are undergoing aesthetic breast surgeries annually, in which augmentation mammoplasty is the most common procedure [ 1 , 2 ]. The more patients are diagnosed with breast cancer, the more women seek breast reconstruction surgeries. Implant-based breast reconstruction (IBBR) is the most carried out reconstructive surgery after mastectomy. IBBR accounts for more than 80% of all women subjecting to breast reconstruction [ 3 ]. Worldwide, more than 35 million women are subjected to implant-based breast surgeries, with nearly 450 thousand breast implants used yearly in the USA [ 4 , 5 ]. The increasing demand for implant-based breast surgeries reflects the ultimate need for women to recreate aesthetically pleasant breasts with acceptable functional and physical outcomes [ 6 ].
The advancement in implant manufacturing techniques considerably improved the outcomes of breast surgeries. Implants maintain the anatomical boundaries of the breasts, allowing for more aesthetically appealing natural breast [ 7 ]. Breast implants are categorized based on surface roughness, filling materials, and shape. Based on the surface of the implants, these devices are assorted into textured or smooth implants. Recently, there has been an increased usage rate of smooth breast implants at the expense of textured breast implants [ 8 ]. This might be attributed to the considerable degree of biofilm formation and seroma associated with textured breast implants [ 9 ]. The biofilm surrounding the textured implants may stimulate lymphocyte production, which triggers a cycle of inflammation that ultimately results in breast implant-associated anaplastic large cell lymphoma (BIA-ALCL) [ 10 ].
BIA-ALCL has raised concerns about the safety and future of breast implants. It is a rare type of T-Cell lymphoma that arises around the breast implants. It is the disease of the breast implant capsule and not of the breast tissue itself [ 11 ] . However, lack of knowledge is a significant barrier to managing patients with BIA-ALCL appropriately, with little being known regarding the relationship between breast implants and ALCL. This results in considerable debate about the future safety of breast implants in aesthetic and reconstructive settings [ 12 ].
The growing interest in BIA‐ALCL reflects its implications for implant-based breast surgeries. A possible correlation between breast implants and ALCL could change the reconstructive options, choice of breast implants, and the future of other implanted devices [ 13 ]. The exact number of patients with BIA-ALCL is challenging to determine due to the lack of global disease awareness [ 14 ]. Despite the progress in calculating the risk of BIA-ALCL, the results of previously published systematic reviews are inconsistent [ 15 , 16 ]. There is a considerable variation in the estimated current risk of BIA-ALCL. This variation may be due to underreporting, duplicated cases, misdiagnosis, difficulty obtaining a valid clinical history from the affected cases, differences in the diagnostic approaches, and inadequate data related to the total number of patients with breast implants [ 17 ].
A comprehensive understanding of breast implant safety remains a common goal of plastic and reconstructive surgeons [ 18 ]. Herein, the current meta-analysis was performed to retrieve the risk of BIA-ALCL from population-based epidemiological studies. This risk was categorized based on the type of breast implant and breast surgery. Furthermore, factors associated with BIA-ALCL were evaluated to identify patients at higher risk of BIA-ALCL. Recognizing such evidence is crucial to ensuring the safety of implant-based breast surgeries and optimizing preventive measures for patients at higher risk of BIA-ALCL.
Materials and Methods
The Preferred Reporting Items for Systematic Reviews and Meta-Analysis (PRISMA) guidelines [ 19 ] and the recommendations of the Cochrane Collaboration [ 20 ] were followed while conducting the present study. This systematic review was reported based on the guidelines of Meta-analysis of observational studies in epidemiology [ 21 ] (Supplementary Table 1 ).
Study Selection
All epidemiological studies encompassed patients with breast implants either for aesthetic or reconstructive purposes and reported the number of patients who developed BIA-ALCL apart from the total cohort were included. Studies reported the risk factors of BIA-ALCL in patients with breast implants were included. There was no restriction on the patient’s age, sex, race, or place. On the contrary, non-epidemiology-based studies or those that did not report the associations between breast implants and anaplastic large cell lymphoma (ALCL) were ousted. Studies with unextractable data, guidelines, review articles, case reports, non-human studies, case series, comments, editorials, posters, letters, and books were excluded. The screening processes were performed blindly to retrieve the possibly relevant articles. The discrepancy between the reviewers was solved by discussion. The screening and eligibility processes were reported using PRISMA flow chart.
Data Source
A comprehensive systematic literature review was carried out throughout twelve databases from inception until 16 April 2023. The following databases were searched: Google Scholar, PubMed, Web of Science (ISI), SIGLE, Scopus, Virtual Health Library (VHL), Clinical trials, Controlled Trials (RCT), NYAM, Cochrane Collaboration, EMBASE, and WHO International Clinical Trials Registry Platform (ICTRP). No restrictions were employed on patients’ age, ethnicity, race, language, or place. The search strategy applied individualized controlled vocabulary terms based on each searched database’s guidelines. A combination of medical subject headings and text words was used to retrieve a wide range of potentially eligible articles. Citation tracking, cross-referencing, and screening of all references of previously published systematic reviews were performed. The following keywords were used in every possible combination; ‘Breast’, ‘Mammary’, ‘Implant’, ‘Implants’, ‘Prosthesis’, ‘Expander’, ‘BIA-ALCL’, ‘Anaplastic’,’ Lymphoma,’ ‘Lymphomas’, ‘lymphoproliferative’.
Data Extraction
The demographic characteristics of the included studies were retrieved. This included study’s title, the first author’s second name, study design, year of publication, study period, and study region. Furthermore, patients’ demographic characteristics were extracted, including the total number of patients with breast implants, the number of patients who developed BIA-ALCL, patients’ age at the diagnosis of BIA-ALCL, diagnostic workup, and history of breast cancer. The data related to breast implants were extracted, including the reasons for breast implants, time from implants to the diagnosis of BIA-ALCL, times of breast implants, types of breast implants, side of the implants, and the number of implants. The data related to BIA-ALCL were retrieved, including the lymphoma localization, type of ALCL, stage of BIA-ALCL, and treatment-related data. The data were extracted independently in a well-structured Microsoft Excel spreadsheet.
Quality Assessment of the Included Studies
The quality of the observational studies was calculated using the National Institute of Health (NIH) quality assessment tool [ 22 ]. The quality of the included studies was assorted into good, fair, and bad when the score was > 65%, 30–65%, and < 30%, respectively.
Statistical Analysis
The pooled summary of odds ratio (OR) was computed by pooling the effect sizes from all the included articles to confirm the association between breast implants and ALCL. The cumulative incidence of BIA-ALCL was estimated by pooling the incidence value and their 95% CI (confidence intervals) to calculate the pooled cumulative incidence. The prevalence of BIA-ALCL was calculated by calculating the event rate and 95% CIs for each study and followed by pooling the effect sizes of all studies to evaluate the summary risk with 95% CI. The fixed-effect model was used when a fixed population effect size was assumed. Conversely, the random-effects model was implemented when the statistical heterogeneity was established. Statistical heterogeneity was calculated using Higgins I 2 statistic, and the Cochrane Q ( χ 2 test), at the value of > 50% and P < 0.10, respectively [ 23 ]. Publication bias was revealed with the asymmetrical distribution of the included studies within the funnel plot along the middle line and based on Egger’s regression test ( P -value < 0.10). The trim and fill method of Duvall and Tweedie was executed to investigate the impact of publication bias on the overall significance level [ 24 ].
Random-effects meta-regression was conducted to explore the potential associations with BIA-ALCL. Subgroup analysis was performed based on the type of breast surgery, the types of breast implants, and based on study regions. The data were analysed using Comprehensive Meta-Analysis v3 software. The significance was revealed at the value of P < 0.05 [ 25 ].
A systematic literature search of 12 databases revealed 4029 articles. Of them, 995 reports were excluded as duplicates, resulting in 3034 articles eligible for screening. The title and abstract screening process excluded 2984 studies, yielding 50 articles suitable for full-text screening. Consequently, 36 studies were excluded, resulting in 14 articles included for data extraction, in which one study was excluded. Four studies were identified through manual searching, resulting in 17 articles that were finally included for systematic review and meta-analysis. The literature search process, identification of studies, and eligibility are shown in Fig. 1
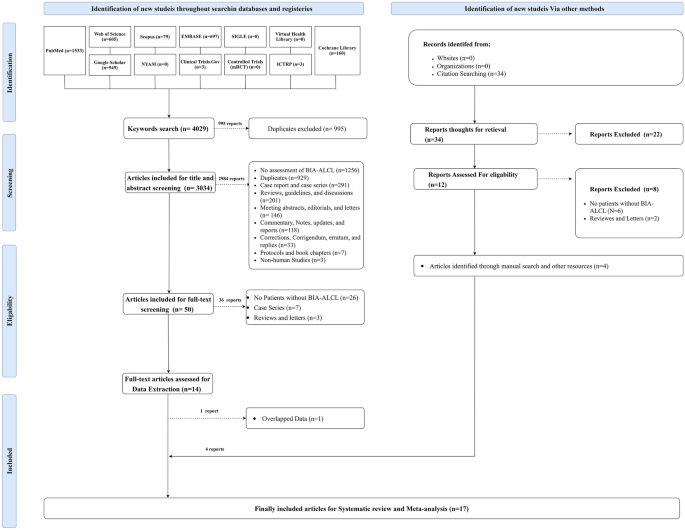
PRISMA 2020 flow diagram for updated systematic reviews which included searches of databases, registers, other sources, and screening
Demographic Characteristics and Quality of the Included Studies
The present systematic review and meta-analysis included 17 articles, encompassing 525475 patients with breast implants [ 26 , 27 , 28 , 29 , 30 , 31 , 32 , 33 , 34 , 35 , 36 , 37 , 38 , 39 , 40 , 41 , 42 ]. There were ten articles of prospective study design and seven reports of retrospective design. Ten articles included data from the USA. There were 254 patients with BIA-ALCL throughout the follow-up periods, which ranged from five to 30.9 years. The average age at diagnosis of BIA-ALCL ranged from 24 to 77 years, with an average time from implants to diagnosis ranging from one to 39 years with a mean duration of 13.16 years (95% CI 11.7–14.6, P < 0.001). There were 42 patients with a history of breast cancer (Table 1 ).
There were 44 patients with textured breast implants, and two with smooth implants had BIA-ALCL. Furthermore, there were 28 patients with stage I BIA-ALCL and six with stage II BIA-ALCL. Thirty-three patients received surgical therapy; 15 patients were treated with chemotherapy in addition to the surgical treatment. The included observational studies revealed a fair quality of four studies [ 26 , 28 , 36 , 40 ]. The quality of the remaining 13 articles was good (Table 2 ).
The Risk of BIA-ALCL
Total risk of bia-alcl.
Fourteen studies included 525475 patients with breast implants and reported the number of cases of BIA-ALCL [ 26 , 27 , 28 , 29 , 30 , 31 , 35 , 36 , 37 , 38 , 39 , 40 , 41 , 42 ] . In the random-effects model ( I 2 = 96.08%, P < 0.001), the risk of BIA-ALCL ranged from 0 to 1 per 1000 cases of breast implants (event rate 0, 95% CI 0–0.1; P < 0.001). Evidence of publication bias was detected by the asymmetrical distribution of studies along the middle line of the funnel plot and based on Egger’s regression test (Intercept = − 5.43, P = 0.00124). The trim and fill method was applied to assess the impact of publication bias on the overall estimate after imputing possible missing studies. This revealed no significant impact of publication bias on the significant level of the overall estimate ( P < 0.001) (Fig. 2 A and B ).
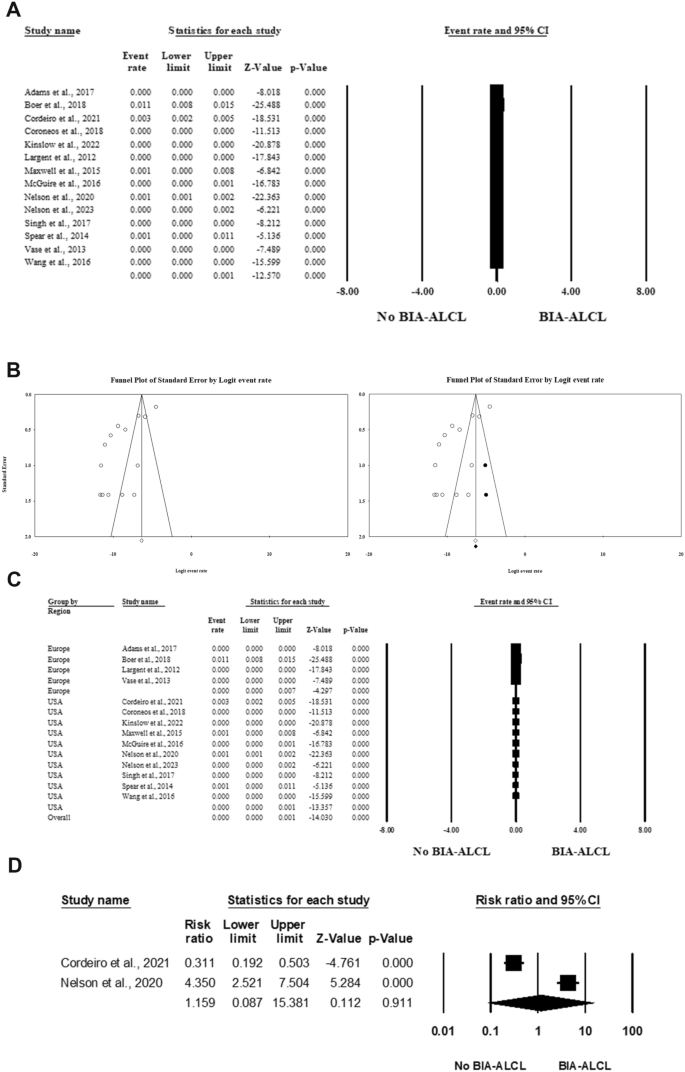
Forest plot of summary analysis of the ( A ) Event rate and 95% CI of the prevalence of BIA-ALCL among patients with breast implants. ( B ) Funnel plots showing evidence of publication bias on the left side and after imputing the studies on the right side. ( C ) Subgroup analysis of the event rate and 95% CI of the prevalence of BIA-ALCL among patients with breast implants based on the regions of the included studies. ( D ) Cumulative incidence of BIA-ALCL per 1000 patients per year among patients with breast implants. The size of the black squares is proportional to the statistical weight of each trial. The black diamond represents the pooled point estimate. The positioning of both diamonds and squares (along with 95% CIs) beyond the vertical line (unit value) suggests a significant outcome
Subgroup analysis based on the study regions revealed a relatively high prevalence of BIA-ALCL in the European region. The prevalence of BIA-ALCL ranged from 0 to 7 cases per 1000 patients with breast implants in Europe (event rate 0, 95% CI 0–0.7; P < 0.001), in contrast to 0 to 1 case per 1000 patients in the USA (event rate 0, 95% CI 0–0.1; P < 0.001) (Fig. 2 C).
Cumulative Risk of BIA-ALCL
Two studies included 12919 cases with breast implants that reported the cumulative incidence of BIA-ALCL per 1000 patients per year [ 27 , 37 ]. In the random-effects model ( I 2 = 98.023%, P < 0.001), the cumulative risk of BIA-ALCL per 1000 persons per year was 1.159 (0.087 to 15.381, P = 0.911) (Fig. 2 D).
The Risk of BIA-ALCL Based on the Type of Breast Surgery
The risk of BIA-ALCL among patients seeking breast implants for aesthetic purposes was evaluated among twelve articles, including 301905 patients [ 26 , 27 , 29 , 30 , 31 , 35 , 36 , 37 , 38 , 39 , 40 , 41 ]. The prevalence of BIA-ALCL ranged from 0 to 1 case per 1000 patients with breast implants (Event rate= 0, 95% CI 0–0.1, P < 0.001) in the random-effects model ( I 2 = 91.99%, P < 0.001). Twelve articles included 301905 patients with breast implants for reconstructive purposes [ 26 , 27 , 29 , 30 , 31 , 35 , 36 , 37 , 38 , 39 , 40 , 41 ] . In the random-effects model ( I 2 = 91.3%, P < 0.001), the prevalence of BIA-ALCL among patients with breast implants for reconstructive surgeries ranged from 0 to 1 case per 1000 patients with breast implants (Event rate= 0, 95% CI 0–0.1, P < 0.001) (Fig. 3 A and B ).

Forest plot of summary analysis of the ( A ) Event rate and 95% CI of the prevalence of BIA-ALCL among patients seeking breast implants for aesthetic purposes. ( B ) Event rate and 95% CI of the prevalence of BIA-ALCL among patients seeking breast implants for reconstructive purposes. ( C ) Event rate and 95% CI of the prevalence of BIA-ALCL among patients with textured breast implants. ( D ) Event rate and 95% CI of the prevalence of BIAALCL among patients with smooth breast implants. The size of the black squares is proportional to the statistical weight of each trial. The black diamond represents the pooled point estimate. The positioning of both diamonds and squares (along with 95% CIs) beyond the vertical line (unit value) suggests a significant outcome
The Risk of BIA-ALCL Based on the Types of Breast Implants
Nine articles included 226525 patients with breast implants that reported the prevalence of BIA-ALCL among patients with textured implants [ 26 , 27 , 29 , 31 , 37 , 38 , 39 , 40 , 41 ]. The risk of BIA-ALCL was 0 (95% CI 0–0.001) with a risk ranging from 0 to one per 1000 cases with textured breast implants ( P <0.001) in the random-effects model ( I 2 = 93.48%, P < 0.001). (Fig. 3 C)
Five articles included 177634 patients with breast implants and reported the risk of BIA-ALCL among patients with smooth implants [ 29 , 31 , 37 , 39 , 40 ]. The risk of BIA-ALCL was 0 among patients with smooth breast implants ( P < 0.001) in the random-effects model (I 2 = 52.64%, P = 0.076) (Fig. 3 D).
The risk of BIA-ALCL among patients with silicone breast implants was evaluated within nine articles encompassing 234807 patients with breast implants [ 26 , 27 , 29 , 31 , 36 , 38 , 39 , 40 , 41 ]. In the random-effects model (I 2 = 94.97%, P < 0.001), the risk of BIA-ALCL ranged from 0 to one per 1000 cases (event rate 0; 95% CI 0–0.001; P < 0.001) (Fig. 4 A).
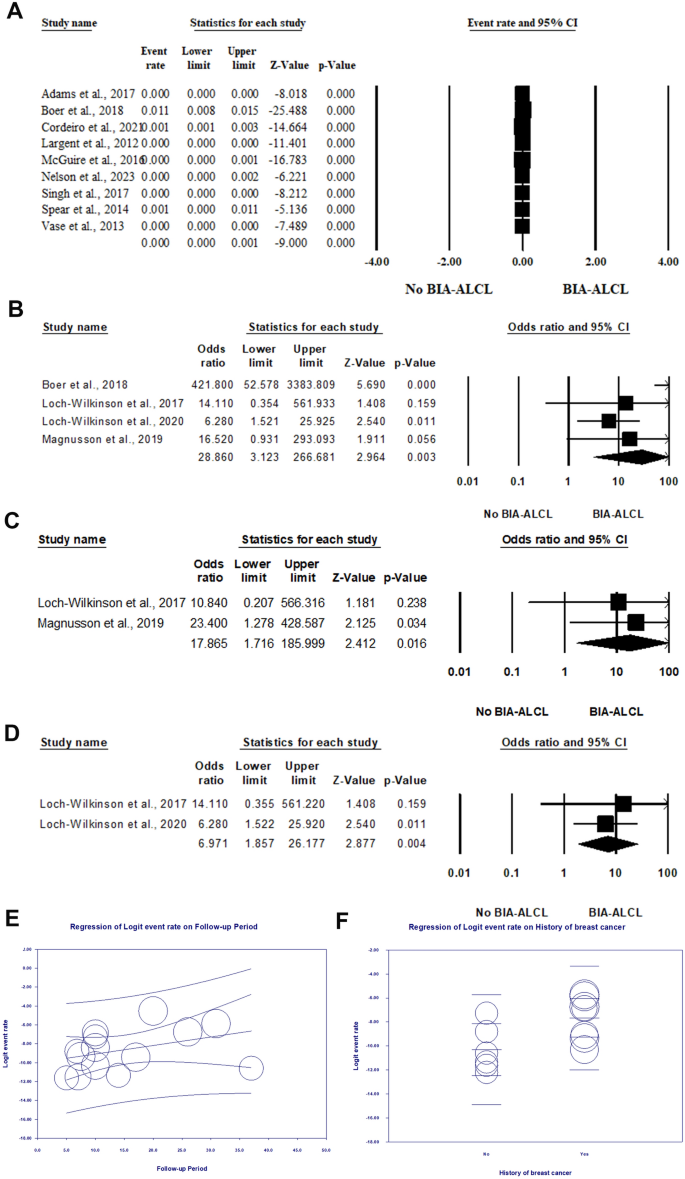
Forest plot of summary analysis of the ( A ) Event rate and 95% CI of the prevalence of BIA-ALCL among patients with silicone breast implants. ( B ) Odds ratio (OR) and 95% CI of the association between breast implants and ALCL. ( C ) Odds ratio (OR) and 95% CI of the association between polyurethane breast implants and ALCL. ( D ) Odds ratio (OR) and 95% CI of the association between biocell textured breast implants and ALCL. ( E ) Weighted random-effects meta regression analysis regressing risk of BIA-ALCL against the follow-up period. ( F ) Weighted random-effects meta regression analysis regressing risk of BIA-ALCL against the history of breast cancer. The size of the black squares is proportional to the statistical weight of each trial. The black diamond represents the pooled point estimate. The positioning of both diamonds and squares (along with 95% CIs) beyond the vertical line (unit value) suggests a significant outcome
Factors Associated with BIA-ALCL
Association between types of breast implants and alcl.
Four articles reported the association between breast implants and ALCL [ 29 , 32 , 33 , 34 ]. In the random-effects model (I 2 = 72.28%, P = 0.013), patients with breast implants were 28.86 times more vulnerable to developing BIA-ALCL (OR 28.86, 95% CI 3.123–266.681, P = 0.003) (Fig. 4 B).
Two articles [ 32 , 34 ] reported the association between polyurethane breast implants and BIA-ALCL. In the random-effects model (I 2 = 0%, P = 0.75), patients with polyurethane breast implants were at 17.86 times higher risk of developing BIA-ALCL (OR 17.86, 95%CI; 1.71 to 185.99, P = 0.016). In this respect, two studies [ 32 , 33 ] evaluated the association between biocell textured breast implants and BIA-ALCL. The risk of BIA-ALCL was 6.971 times among patients with biocell textured implants (OR 6.971, 95% CI 1.857–26.177, P = 0.004) in the random-effects model (I 2 = 0%, P = 0.688) (Fig. 4 C and D ).
Association between BIA-ALCL and Follow-up periods
Fourteen articles comprised 525290 patients with breast implants included for meta-regression analysis [ 26 , 27 , 28 , 29 , 30 , 31 , 35 , 36 , 37 , 38 , 39 , 40 , 41 , 42 ]. The analysis revealed no statistically significant impact of follow-up periods on the risk of BIA-ALACL (Coefficient = 0.0902, 95% CI − 0.041, 0.22; P = 0.18) with proportional of the total between study variance explained by the model (R 2 ) of 0.18. (Fig. 4 E)
Association between BIA-ALCL and history of breast cancer
The association between the history of breast cancer and the risk of BIA-ALCL was evaluated within 13 articles, including 401898 patients with breast implants [ 26 , 27 , 28 , 29 , 30 , 31 , 35 , 36 , 37 , 38 , 39 , 40 , 41 ]. The random meta-regression model revealed a statistically significant positive association between the history of breast cancer and BIA-ALCL ( P = 0.0016) with a coefficient of 2.63 (95% CI 0.47, 4.97). The model explained 22% of the total between study variance with R 2 of 0.22 (Fig. 4 F).
BIA-ALCL is an emerging disorder that has gained global attention throughout the past era [ 43 ]. The knowledge regarding the prevalence, risk factors, and management of BIA-ALCL needs to be more extensive. The current literature is insufficient to draw conclusive evidence for current clinical practice. This is because most previously published systematic reviews are based on case reports without quantitative data synthesis [ 44 ]. Therefore, the present meta-analysis was conducted to retrieve the available evidence from well-structured epidemiological studies on the prevalence and risk factors of BIA-ALCL.
The present study confirmed the association between breast implants and ALCL. The risk of BIA-ALCL ranged from 0 to 1 per 1000 cases, with a similar risk of BIA-ALCL among patients who received breast implants for reconstruction and aesthetic purposes. The risk of BIA-ALCL was relatively low among patients with textured or smooth breast implants. Breast implants increase the risk of BIA-ALCL 28.86 times more than the risk of ALCL among the general population, with a higher risk among patients with polyurethane breast implants. Patients with a history of breast cancer were at higher risk of BIA-ALCL. These findings highlight the ultimate role of comprehensive counselling for patients seeking implant-based breast surgeries regardless of the type of surgery and type of breast implants. This counselling should include the risk and symptoms of BIA-ALCL with close follow-up for the most susceptible patients [ 45 ].
The current meta-analysis showed a relatively similar risk of BIA-ALCL between textured and smooth implants. Consistent with this finding, Nava et al., highlighted the non-exclusive association between textured implants and BIA-ALCL [ 46 ]. These findings highlighted a potential risk of publication bias in this issue as many previously published studies revealed only risk of BIA-ALCL with textured implants. Lynch et al. reported a risk of BIA-ALCL associated with textured implants ranging from 1:355 to 1:5100. However, this systematic review reported no BIA-ALCL associated with smooth breast implants [ 15 ]. Furthermore, Gidengil et al. reported most cases of BIA-ALCL were associated with textured implants. They highlighted the limited interpretation of these findings without knowledge of the total number of patients with textured implants and other types of implants in the general population [ 47 ]. The lack of accurate data about the denominator in the previously published studies conveys a high risk of bias regarding the precise risk of BIA-ALCL. The present meta-analysis included only epidemiology-based studies that identified the denominator to tackle the limitations of the previously published reviews.
The current meta-analysis revealed a relatively higher risk of BIA-ALCL in Europe than in the USA. In the USA, the use of textured implants peaked in 2016 with a utilization rate of nearly 18% and 41% for aesthetic and reconstructive breast surgeries. Based on the Dutch Breast Implant Registry, textured implants are used in 88% of cases, while smooth implants are used in 7 to 11% of aesthetic breast surgeries in Europe. The substantial variation in the risk of BIA-ALCL between the USA and Europe may reveal the role of ethnicity and genetic predisposition in developing BIA-ALCL. Furthermore, the large proportion of patients with textured implants outside the USA may be associated with the high rate of reporting cases with BIA-ALCL associated with textured implants [ 48 , 49 ]. Noteworthy, most of the data from the USA is related to the FDA alert of implant safety and the significant number of implant-based breast surgeries. BIA-ALCL is extremely rare in Africa, Asia, and Latin America, with no epidemiology-based studies including patients from these regions. This reflects the risk of underreporting bias and highlights the need to establish validated registries in these regions to estimate the risk of BIA-ALCL accurately.
The present meta-analysis revealed a higher risk of BIA-ALCL associated with breast implants. This risk was approximately threefold higher among patients with polyurethane breast implants than those with textured biocell breast implants. This finding may reveal a proportionate relationship between the risk of BIA-ALCL and surface texturization. Parallel with these findings, Girardi et al., reported an association between ALCL and breast implants, with comprehensive knowledge about the disease that should be given to all patients before breast implant surgeries [ 50 ]. These findings infer a more significant association between breast implants and ALCL but not an exclusive causal relationship. There is an urgent need for more data about the etiopathogenesis of BIA-ALCL since the number of events is small owing to the rarity of the disease. The association between implant types and the risk of BIA-ALCL necessitates further evaluation and should be studied in the context of other confounders. This is because of the limited clinical data in the literature regarding the implant types, texturization, manufacturing, and the risk of BIA-ALCL [ 4 , 51 ].
There was a considerable risk of BIA-ALCL among patients with a history of breast cancer. This finding demonstrates some patients’ immunological and genetic predisposition to develop BIA-ALCL. Parallel with these findings, Jabagi et al., reported a higher risk of haematological malignancies after post-operative breast cancer treatment, particularly among young patients who received radiotherapy or chemotherapy [ 52 ]. Wang et al., reported an increased risk of non-Hodgkin lymphoma after radiation therapy among patients with breast ductal carcinoma [ 53 ]. Exposure to high dosages of ionizing radiation results in chromosomal alterations and somatic mutations. This increases the risk of myeloid malignancies, leukaemias, and lymphomas, particularly in the irradiated organs [ 54 , 55 ]. Alkylating chemotherapeutic agents induce mutations of the genes responsible for cell proliferation and tumour suppressors, activating the oncogenic cascade. This results in abnormal cell differentiation and proliferation, resulting in haematological malignancies [ 56 , 57 ]. These findings highlighted the importance of close follow-up for patients with a history of breast cancer and subjected to implant-based breast surgeries either for reconstructive or aesthetic purposes.
The present systematic review and meta-analysis gathered the dispersed evidence related to the risk of BIA-ALCL in the literature. The study included only epidemiological studies, allowing for estimating the current risk of BIA-ALCL among the largest cohort in the literature. However, some limitations were to be considered while interpreting the retrieved evidence for the current clinical practice. Many of the included articles were retrospective designs with a considerable risk of information bias. Furthermore, there was significant statistical heterogeneity between most of the included studies. This variation was attributable to the difference in the demographic characteristics, surgical data, implant types, study designs, diagnostic workups, and follow-up periods. The random-effects model, subgroup analysis, and meta-regression analysis were performed to mitigate the potential impacts of this heterogeneity on the overall effect estimates. Subsequently, the published prospective and retrospective studies comprised incomplete data on history, presentation, histology, and management. The rarity of BIA-ALCL is challenging for healthcare providers with inadequate experience regarding diagnosis, reporting, and management of the disease.
Conclusions
The current meta-analysis confirmed the association between breast implants and ALCL. The estimated risk of the disease ranged from 0 to 1 case per 1000 patients with breast implants. There was a relatively similar risk of BIA-ALCL among patients with aesthetic or reconstructive surgeries and patients with smooth or textured breast implants. Patients with a history of breast cancer were at higher risk of BIA-ALCL. This knowledge could help clinicians identify the current risk and the predictors of BIA-ALCL among breast implant patients. Identifying such evidence is critical for preventing the potential repercussions of BIA-ALCL on the future of implant-based breast surgeries.
(2018) Cosmetic Surgery National Data Bank Statistics. Aesthetic Surgery Journal 38(Suppl. 3):1–24
Gupta V, Yeslev M, Winocour J, Bamba R, Rodriguez-Feo C, Grotting JC, Higdon KK (2017) Aesthetic breast surgery and concomitant procedures: incidence and risk factors for major complications in 73,608 cases. Aesthetic Surg J 37(5):515–527
Article Google Scholar
American Society of Plastic Surgeons (2020) Plastic surgery statistics report
Collett DJ, Rakhorst H, Lennox P, Magnusson M, Cooter R, Deva AK (2019) Current risk estimate of breast implant–associated anaplastic large cell lymphoma in textured breast implants. Plast Reconstruct Surg 143(3S):30S-40S
Article CAS Google Scholar
Marra A, Viale G, Pileri SA, Pravettoni G, Viale G, De Lorenzi F, Nolè F, Veronesi P, Curigliano G (2020) Breast implant-associated anaplastic large cell lymphoma: a comprehensive review. Cancer Treat Rev 1(84):101963
Jalalabadi F, Doval AF, Neese V, Andrews E (2021) Spiegel AJ (2021) Breast implant utilization trends in USA versus Europe and the impact of BIA-ALCL publications. Plast Reconstruct Surg Global Open 9(3):e3449
Williams DC, Seifman MA, Hunter-Smith DJ (2019) Patient related outcome measures for breast augmentation mammoplasty: a systematic review. Gland Surg 8(4):425
Article PubMed PubMed Central Google Scholar
Vishwanath S, Pellegrini B, Parker E, Earnest A, Kalbasi S, Gartoulla P, Elder E, Farrell G, Moore C, Cooter RD, Ahern S (2021) Breast device surgery in Australia early results from the Australian Breast Device Registry. J Plast Reconstruct Aesthet Surg 74(10):2719–2730
Tandon VJ, DeLong MR, Ballard TN, Clemens MW, Brandt KE, Kenkel JM, Cederna PS (2018) Evolving trends in textured implant use for cosmetic augmentation in the United States. Plast Reconstruct Surg 142(6):1456–1461
Mehdi AS, Bitar G, Sharma RK, Iyengar S, El-Sharkawi D, Tasoulis MK, Attygalle AD, Cunningham D, Sharma B, RMH BIA-ALCL Working Group (2022) Breast implant-associated anaplastic large cell lymphoma (BIA-ALCL): A good practice guide, pictorial review, and new perspectives. Clin Radiol 77(2):79–87
Sharma K, Gilmour A, Jones G, O’Donoghue JM, Clemens MW (2022) A Systematic review of outcomes following breast implant associated anaplastic large cell lymphoma (BIA-ALCL). JPRAS Open. https://doi.org/10.1016/j.jpra.2022.08.008
Mehta-Shah N, Ghione P (2020) An updated approach and understanding of breast implant-associated anaplastic large cell lymphoma. J Natl Compr Cancer Netw 20(3):309–315
McKernan CD, Vorstenbosch J, Chu JJ, Nelson JA (2022) Breast implant safety: an overview of current regulations and screening guidelines. J Gen Int Med 37(1):212–216
Cunha A, Horta R, Barreiro D (2021) Epidemiological profile in Portugal of breast implant-associated anaplastic large cell lymphoma: a cross-sectional study. Acta Med Port 34(9):572–579
Article PubMed Google Scholar
Lynch EB, DeCoster RC, Vyas KS, Rinker BD, Yang M, Vasconez HC, Clemens MW (2021) Current risk of breast implant-associated anaplastic large cell lymphoma: a systematic review of epidemiological studies. Ann Breast Surg 5:30. https://doi.org/10.21037/abs-20-96
Leberfinger AN, Behar BJ, Williams NC, Rakszawski KL, Potochny JD, Mackay DR, Ravnic DJ (2017) Breast implant–associated anaplastic large cell lymphoma: a systematic review. JAMA Surg 152(12):1161–1168
Real DSS, Resendes BSA (2023) Breast implant-associated anaplastic large-cell lymphoma: a systematic literature review. Revis Brasileira de Cirurgia Plást 34:531–538
Google Scholar
Rohrich RJ, Kaplan J (2020) Are breast implants safe? Plast Reconstruct Surg 145(2):587–589
Page MJ, McKenzie JE, Bossuyt PM, Boutron I, Hoffmann TC, Mulrow CD, Shamseer L, Tetzlaff JM, Akl EA, Brennan SE, Chou R (2021) The PRISMA 2020 statement: an updated guideline for reporting systematic reviews. Int J Surg 1(88):105906
Olsen O (2019) The guidelines on non-randomised studies. Cochrane Reviews
Stroup DF, Berlin JA, Morton SC, Olkin I, Williamson GD, Rennie D, Moher D, Becker BJ, Sipe TA, Thacker SB (2000) Meta-analysis of observational studies in epidemiology: a proposal for reporting. JAMA 283(15):2008–2012
Article CAS PubMed Google Scholar
National Heart, Lung and Blood Institute (2014) National Institute of Health quality assessment tool for observational cohort and cross-sectional studies. In: Bethesda: National Heart. Lung, and Blood Institute
Higgins JP, Thompson SG, Deeks JJ, Altman DG (2003) Measuring inconsistency in meta-analyses. BMJ 327(7414):557
Duval S, Tweedie R (2000) Trim and fill: a simple funnel-plot–based method of testing and adjusting for publication bias in meta-analysis. Biometrics 56(2):455–463
Borenstein M (2022) Comprehensive meta-analysis software. Syst Rev Health Res Meta Anal Context 22:535–548
Adams WP Jr, Culbertson EJ, Deva AK, Magnusson MR, Layt C, Jewell ML, Mallucci P, Hedén P (2017) Macrotextured breast implants with defined steps to minimize bacterial contamination around the device: experience in 42,000 implants. Plast Reconstruct Surg 140(3):427–431
Cordeiro PG, Ghione P, Ni A, Hu Q, Ganesan N, Galasso N, Dogan A, Horwitz SM (2020) Risk of breast implant associated anaplastic large cell lymphoma (BIA-ALCL) in a cohort of 3546 women prospectively followed long term after reconstruction with textured breast implants. J Plast Reconstruct Aesthet Surg 73(5):841–846
Coroneos CJ, Selber JC, Offodile AC, Butler CE, Clemens MW (2019) US FDA breast implant postapproval studies: long-term outcomes in 99,993 patients. Ann Surg 269(1):30–36
de Boer M, van Leeuwen FE, Hauptmann M, Overbeek LI, de Boer JP, Hijmering NJ, Sernee A, Klazen CA, Lobbes MB, van der Hulst RR, Rakhorst HA (2018) Breast implants and the risk of anaplastic large-cell lymphoma in the breast. JAMA Oncol 4(3):335–341
Kinslow CJ, DeStephano DM, Rohde CH, Kachnic LA, Cheng SK, Neugut AI, Horowitz DP (2022) Risk of anaplastic large cell lymphoma following postmastectomy implant reconstruction in women with breast cancer and ductal carcinoma in situ. JAMA Netw Open 5(11):e2243396
Largent J, Oefelein M, Kaplan H, Okerson T, Boyle P (2011) Risk of lymphoma in women with breast implants: Analysis of clinical studies. J Clin Oncol 29((15_suppl)):1588
Loch-Wilkinson A, Beath KJ, Knight RJ, Wessels WL, Magnusson M, Papadopoulos T, Connell T, Lofts J, Locke M, Hopper I, Cooter R (2017) Breast implant–associated anaplastic large cell lymphoma in Australia and New Zealand: high-surface-area textured implants are associated with increased risk. Plast Reconstruct Surg 140(4):645–654
Loch-Wilkinson A, Beath KJ, Magnusson MR, Cooter R, Shaw K, French J, Vickery K, Prince HM, Deva AK (2020) Breast implant-associated anaplastic large cell lymphoma in Australia: a longitudinal study of implant and other related risk factors. Aesthet Surg J 40(8):838–846
Magnusson M, Beath K, Cooter R, Locke M, Prince HM, Elder E, Deva AK (2019) The epidemiology of breast implant–associated anaplastic large cell lymphoma in Australia and New Zealand confirms the highest risk for grade 4 surface breast implants. Plast Reconstruct Surg 143(5):1285–1292
Maxwell GP, Van Natta BW, Bengtson BP, Murphy DK (2015) Ten-year results from the Natrelle 410 anatomical form-stable silicone breast implant core study. Aesthet Surg J 35(2):145–155
McGuire P, Reisman NR, Murphy DK (2017) Risk factor analysis for capsular contracture, malposition, and late seroma in subjects receiving Natrelle 410 form-stable silicone breast implants. Plast Reconstruct Surg 139(1):1
Nelson JA, Dabic S, Mehrara BJ, Cordeiro PG, Disa JJ, Pusic AL, Matros E, Dayan JH, Allen RJ Jr, Coriddi M, Polanco TO (2021) Breast implant-associated anaplastic large cell lymphoma incidence: determining an accurate risk. Ann Surg 272(3):403
Nelson JA, McKernan CD, Rubenstein RN, Shamsunder MG, Poulton R, Dabic S, Mehrara BJ, Disa JJ, Cordeiro PG, McCarthy CM (2023) Risk of breast implant-associated anaplastic large cell lymphoma (BIA-ALCL) in patients with temporary exposure to textured tissue expanders: an analysis of 3310 patients. Plast Reconstruct Surg 13:10–97
Singh N, Picha GJ, Hardas B, Schumacher A, Murphy DK (2017) Five-year safety data for more than 55,000 subjects following breast implantation: comparison of rare adverse event rates with silicone implants versus national norms and saline implants. Plast Reconstruct Surg 140(4):666–679
Spear SL, Murphy DK (2014) Natrelle round silicone breast implants: core study results at 10 years. Plast Reconstruct Surg 133(6):1354
Vase MØ, Friis S, Bautz A, Bendix K, Sørensen HT, d’Amore F (2013) Breast implants and anaplastic large-cell lymphoma: a Danish population-based cohort studybreast implants and anaplastic large-cell lymphoma. Cancer Epidemiol Biomark Prev 22(11):2126–2129
Wang SS, Deapen D, Voutsinas J, Lacey JV Jr, Lu Y, Ma H, Clarke CA, Weisenburger D, Forman S, Bernstein L (2016) Breast implants and anaplastic large cell lymphomas among females in the California Teachers Study cohort. Br J Haematol 74(3):480
Clemens MW, Jacobsen ED, Horwitz SM (2019) 2019 NCCN consensus guidelines on the diagnosis and treatment of breast implant-associated anaplastic large cell lymphoma (BIA-ALCL). Aesthet Surg J 39(Supplement_1):S3–S13
Rupani A, Frame JD, Kamel D (2015) Lymphomas associated with breast implants: a review of the literature. Aesthet Surg J 35(5):533–544
U.S. Food and Drug Administration (2020) The FDA requests Allergan voluntarily recall Natrelle BIOCELL textured breast implants and tissue expanders from the market to protect patients. FDA Safety Communication, 2019
Nava MB, Chiodini P, Catanuto G, Rocco N (2021) Is Breast Implant Associated—Anaplastic Large Cell Lymphoma linked to textured implants? Aesthet Plast Surg 45:1–9
Gidengil CA, Predmore Z, Mattke S, van Busum K, Kim B (2015) Breast implant–associated anaplastic large cell lymphoma: a systematic review. Plast Reconstruct Surg 135(3):713–720
Matros E, Shamsunder MG, Rubenstein RN, Polanco TO, McCarthy CM, Nelson JA, Gutowski K, Steering Committee TOPS (2021) Textured and smooth implant use reported in the tracking operations and outcomes for plastic surgeons database: epidemiologic implications for BIA-ALCL. Plast Reconstruct Surg Global Open 9(3):e3499
Becherer BE, de Boer M, Spronk PE, Bruggink AH, de Boer JP, van Leeuwen FE, Mureau MA, van der Hulst RR, de Jong D, Rakhorst HA (2019) The Dutch Breast Implant Registry: registration of breast implant–associated anaplastic large cell lymphoma—a proof of concept. Plast Reconstruct Surg 143(5):1298–1306
Girardi JDM, Brito GV (2019) Existe associação do uso de implantes mamários texturizados com linfoma anaplásico de células grandes? Vigilância Sanitária Em Debate 7(4):85-95
Hwang K, Kim H, Kim HM, Kim JH (2021) Risk of anaplastic large-cell lymphoma (ALCL) in cases of late seroma formation after breast implant insertion. Eplast 21:e4
Jabagi MJ, Goncalves A, Vey N, Le Tri T, Zureik M, Dray-Spira R (2019) Risk of hematologic malignant neoplasms after postoperative treatment of breast cancer. Cancers 11(10):1463
Article CAS PubMed PubMed Central Google Scholar
Wang K, Li Z, Chen X, Zhang J, Xiong Y, Zhong G, Shi Y, Li Q, Zhang X, Li H, Xiang T (2021) Risk of hematologic malignancies after breast ductal carcinoma in situ treatment with ionizing radiation. NPJ Breast Cancer 7(1):21
Schubauer-Berigan MK, Daniels RD, Fleming DA, Markey AM, Couch JR, Ahrenholz SH, Burphy JS, Anderson JL, Tseng CY (2007) Risk of chronic myeloid and acute leukemia mortality after exposure to ionizing radiation among workers at four US nuclear weapons facilities and a nuclear naval shipyard. Radiat Res 167(2):222–232
Gillis NK, Ball M, Zhang Q, Ma Z, Zhao Y, Yoder SJ, Balasis ME, Mesa TE, Sallman DA, Lancet JE, Komrokji RS (2017) Clonal haemopoiesis and therapy-related myeloid malignancies in elderly patients: a proof-of-concept, case-control study. Lancet Oncol 18(1):112–121
Zhang B, Zhang X, Li M, Kong L, Deng X, Yu J (2016) How breast cancer chemotherapy increases the risk of leukemia: thoughts about a case of diffuse large B-cell lymphoma and leukemia after breast cancer chemotherapy. Cancer Biol Ther 17(2):125–128
Krishnan B, Morgan GJ (2017) Non-Hodgkin lymphoma secondary to cancer chemotherapy. Cancer Epidemiol Biomark Prev 16(3):377–380
Download references
Open access funding provided by The Science, Technology & Innovation Funding Authority (STDF) in cooperation with The Egyptian Knowledge Bank (EKB).
Author information
Authors and affiliations.
Department of Plastic and Reconstructive Surgery, El-Sahel Teaching Hospital, Cairo, Egypt
Ali Mohamed Elameen
Department of Plastic and Reconstructive Surgery, Faculty of Medicine For Girls, Al-Azhar University, Gameat Al Azhar, Nasr City, Cairo, Egypt
Mohamed Awad AlMarakby & Asmaa Ali Dahy
Department of Plastic and Reconstructive Surgery, EL Helmeya Military Hospital, Military Medical Academy, Cairo, Egypt
Tarek Ibrahim Atta
You can also search for this author in PubMed Google Scholar
Corresponding author
Correspondence to Mohamed Awad AlMarakby .
Ethics declarations
Conflict of interest.
The authors declare that they have no conflicts of interest to disclose.
Informed Consent
For this type of study, informed consent is not required.
Human and Animal Rights
This article does not contain any studies with human participants or animals performed by any of the authors.
Additional information
Publisher's note.
Springer Nature remains neutral with regard to jurisdictional claims in published maps and institutional affiliations.
Supplementary Information
Below is the link to the electronic supplementary material.
Supplementary file1 (DOCX 28 KB)
Rights and permissions.
Open Access This article is licensed under a Creative Commons Attribution 4.0 International License, which permits use, sharing, adaptation, distribution and reproduction in any medium or format, as long as you give appropriate credit to the original author(s) and the source, provide a link to the Creative Commons licence, and indicate if changes were made. The images or other third party material in this article are included in the article's Creative Commons licence, unless indicated otherwise in a credit line to the material. If material is not included in the article's Creative Commons licence and your intended use is not permitted by statutory regulation or exceeds the permitted use, you will need to obtain permission directly from the copyright holder. To view a copy of this licence, visit http://creativecommons.org/licenses/by/4.0/ .
Reprints and permissions
About this article
Elameen, A.M., AlMarakby, M.A., Atta, T.I. et al. The Risk of Breast Implant-Associated Anaplastic Large Cell Lymphoma; A Systematic Review and Meta-Analysis. Aesth Plast Surg (2024). https://doi.org/10.1007/s00266-024-03956-9
Download citation
Received : 05 December 2023
Accepted : 16 February 2024
Published : 09 May 2024
DOI : https://doi.org/10.1007/s00266-024-03956-9
Share this article
Anyone you share the following link with will be able to read this content:
Sorry, a shareable link is not currently available for this article.
Provided by the Springer Nature SharedIt content-sharing initiative
- Anaplastic large cell lymphoma
- Breast implants
- Find a journal
- Publish with us
- Track your research
What's your breast cancer risk? Here's how to use assessment quizzes, check for symptoms and more.
S earches for breast cancer symptoms soared by as much as 300% in the day after actress Olivia Munn announced her breast cancer diagnosis . Searches for breast cancer risk assessment tools like the one Munn used spiked as well. In a post she shared on Instagram, Munn urged all women to ask their health care providers to calculate their risks using these tools, which are also available to the public online. But breast cancer risk assessment quizzes aren't the only way to catch tumors early. Here’s what experts have to say about breast cancer risk assessment tools and screenings, as well as symptoms to look out for.
What are breast cancer risk assessment tools, and how do they work?
These tools are basically calculators, but instead of numbers, they use information about your medical history, your family health history, your age, race, reproductive history and in some cases your height and weight to estimate your breast cancer risk.
A number of different tools exist, but the two most commonly used ones are the Breast Cancer Risk Assessment Tool (BCRAT, formerly called the “Gail Model”) and the International Breast Cancer Intervention Study (IBIS) model, previously called the Tyrer-Cuzick Risk Assessment Calculator. Both are available online and are free to use.
The BCRAT “tends to underestimate the risk a little because it is very simple and doesn’t have a lot of different things you have to answer,” Dr. Jason Mouabbi , a breast oncologist with MD Anderson Cancer Center, tells Yahoo Life. On the other hand, the IBIS tool tends to overestimate risks, he says. That’s because it requires more information. Mouabbi uses both tools to get the most accurate picture of someone’s risks.
Using the IBIS tool will tell you your risk of developing breast cancer in the next 10 years and over your lifetime, while the BCRAT tool provides your five-year and lifetime risks (up to age 90). Both tests should be used only by adults.
A lifetime risk score of 20% or above (generally calculated by the IBIS model) is considered high risk. Using the BCRAT test, a five-year risk of more than 1.66% is considered high. But there are some important caveats and considerations for what those scores mean, as well as other important factors and symptoms to pay attention to.
Important things to know before using breast cancer risk assessment tools
First of all, it’s best to have your health care provider do your assessment for you. “You really don’t want to do it wrong, because it can lead to unnecessary anxiety,” Mouabbi says. These risk assessment quizzes are well known to oncologists, but any health care provider can help you take one.
Second, the tools may be less accurate for younger people. The BCRAT calculator says it’s only supposed to be used to calculate the risks for women between the ages of 35 and 85. The IBIS model doesn’t note any age limitations, but Mouabbi says he only uses either calculator to calculate risks for those ages 30 and older.
Most importantly, these assessment tools should only be used by people who have an “average” risk of breast cancer. In the U.S., there is a 1 in 8 chance that a woman will develop breast cancer, according to the American Cancer Society (ACS). But when it comes to using a risk assessment tool, the difference between an average and a high risk of breast cancer really refers to whether or not someone has tested positive for mutations to the BRCA genes that are linked to higher risks of breast cancer.
Find out your family history and genetic risk
One of the most important steps you can take to understand your breast cancer risks — with or without using an assessment tool — is to find out your family health history, which is a major factor in the risk calculation.
If you decide to use a risk assessment tool on your own, “you should try to talk to a health care provider to see if you’ve used the right risk assessment test based on your family history,” Susan Brown , a registered nurse and senior director of health information and publications at the Susan G. Komen Foundation, tells Yahoo Life.
Most people who develop breast cancer do not have a family history of the disease. But having a first-degree relative such as a sister, mother or daughter who has had breast cancer puts a person at about double the risk of having it themselves, according to Breastcancer.org.
There are a handful of genes and genetic mutations that are linked to greater risks of breast cancer, including BRCA1 and BRCA2 mutations. Because breast cancer appears to run in families beyond these genes, scientists assume that there are other genetic factors that haven't yet been identified, meaning family history is an important risk factor even if you know you don't carry these mutations.
People who know they have these mutations — based on genetic testing — are already at high risk and should be taking precautions, rather than relying on risk assessment quizzes, Mouabbi says. “Those mutations do not skip generations,” he says. “Someone in the family will already have had it.” A family history of breast cancer is a good reason to get BRCA testing, which can cost anywhere from $250 to $5,000, but may be covered by insurance.
About 1 in every 500 women in the U.S. has a mutation in either the BRCA1 or BRCA2 gene, according to the Centers for Disease Control and Prevention (CDC). These mutations are estimated to increase the risk of breast cancer four- to seven-fold and put carriers at a 10% to 46% higher risk of ovarian cancer.
But, says Brown, “all people are at risk for breast cancer — even men get breast cancer.” Even if risk assessment tools and genetic screening results don't “raise any red flags, it doesn’t mean they shouldn’t talk to their health care provider” about screening and prevention, she says.
What to do once you know your risk
If your breast cancer risk is below 20%, your health care provider is more likely to advise you to follow standard screening recommendations and get mammograms annually starting as early as age 40. If you’ve had a mammogram, radiologists will be able to tell you if you have dense breasts and should get additional screening. Whatever your score is, remember that it changes over the course of your life, so if you score under 20% one year, you shouldn’t assume you’ll remain low-risk the next, Mouabbi cautions.
Those who score 20% or above should start to get more frequent and additional screenings, such as MRIs and ultrasounds, which can help detect breast cancer cases sometimes missed by mammography. The good news, Mouabbi says, is that having a high risk score should automatically mean your insurance has to cover additional screening, which can otherwise get expensive.
It also makes you eligible for preventative treatment, he says. Some women with a high risk of breast cancer can be prescribed tamoxifen; the estrogen-blocking drug is used to treat breast cancer at high doses, but it can also be used at lower doses to reduce risks by 43%, according to one University of Toronto study .
Know the common symptoms of breast cancer — and your body
Knowing your risk based on quiz scores can help you and your health care provider come up with the right screening and prevention strategies. But Brown notes that there’s no substitute for simply knowing and paying attention to your own body.
Experts, including both the U.S. Preventive Services Task Force (USPSTF) and the ACS, no longer recommend routine breast self-exams because habitually checking for lumps has not been shown to improve survival odds for women with breast cancer. However, there's still value in people “knowing what's normal for them,” says Brown, and taking note when something changes. That means keeping an eye out for:
Changes in the size or shape of one or both of your breasts
Skin dimpling (which can make your breast look as if it has a texture like an orange peel)
Pain in your breast or nipple
A nipple turning inward
Red, flaky, dry or thick nipple or breast skin
Nipple discharge
Swollen lymph nodes (usually under the arm or around the collarbone)
“If you notice a change in your breast or underarm, do not sit at home and wonder about it, do not consult Dr. Google; go see your health care provider,” says Brown. Half of women ages 50 and over and 70% of women under 50 who are diagnosed with breast cancer get that diagnosis by detecting a change themselves, according to the American College of Obstetricians and Gynecologists (ACOG).
“Most people who find a change in their breast don’t turn out to have breast cancer,” Brown says. “But those changes are found when women are just going about their daily lives — showering, dressing or a partner finding a change — so it’s important for women to know those warning signs and be conscious of changes in their own bodies.”
Estimation of age of onset and progression of breast cancer by absolute risk dependent on polygenic risk score and other risk factors
Affiliations.
- 1 Department of Applied Health Research, University College London, London, UK.
- 2 Department of Statistical Science, University College London, London, UK.
- 3 Department of Public Health and Primary Care, University of Cambridge, Cambridge, UK.
- 4 Department of Oncology, University of Cambridge, Cambridge, UK.
- 5 School of Medicine, Cardiff University, Cardiff, UK.
- 6 Department of Computational Biomedicine, Cedars Sinai Medical Center, Los Angeles, California, USA.
- PMID: 38174903
- PMCID: PMC7615824
- DOI: 10.1002/cncr.35183
Background: Genetic, lifestyle, reproductive, and anthropometric factors are associated with the risk of developing breast cancer. However, it is not yet known whether polygenic risk score (PRS) and absolute risk based on a combination of risk factors are associated with the risk of progression of breast cancer. This study aims to estimate the distribution of sojourn time (pre-clinical screen-detectable period) and mammographic sensitivity by absolute breast cancer risk derived from polygenic profile and the other risk factors.
Methods: The authors used data from a population-based case-control study. Six categories of 10-year absolute risk based on different combinations of risk factors were derived using the Breast and Ovarian Analysis of Disease Incidence and Carrier Estimation Algorithm. Women were classified into low, medium, and high-risk groups. The authors constructed a continuous-time multistate model. To calculate the sojourn time, they simulated the trajectories of subjects through the disease states.
Results: There was little difference in sojourn time with a large overlap in the 95% confidence interval (CI) between the risk groups across the six risk categories and PRS studied. However, the age of entry into the screen-detectable state varied by risk category, with the mean age of entry of 53.4 years (95% CI, 52.2-54.1) and 57.0 years (95% CI, 55.1-57.7) in the high-risk and low-risk women, respectively.
Conclusion: In risk-stratified breast screening, the age at the start of screening, but not necessarily the frequency of screening, should be tailored to a woman's risk level. The optimal risk-stratified screening strategy that would improve the benefit-to-harm balance and the cost-effectiveness of the screening programs needs to be studied.
Keywords: absolute risk; breast cancer; multistate model; natural history; polygenic risk score; sensitivity; sojourn time.
© 2024 The Authors. Cancer published by Wiley Periodicals LLC on behalf of American Cancer Society.
- Age of Onset
- Breast Neoplasms* / diagnosis
- Breast Neoplasms* / epidemiology
- Breast Neoplasms* / genetics
- Case-Control Studies
- Genetic Predisposition to Disease
- Genetic Risk Score
- Middle Aged
- Risk Assessment
- Risk Factors
Grants and funding
- 2017NOVPR1024/BCN_/Breast Cancer Now/United Kingdom
- v203477/Z/16/Z/WT_/Wellcome Trust/United Kingdom
- U19CA148065-01/NH/NIH HHS/United States
- C490/A16561/CRUK_/Cancer Research UK/United Kingdom
- C490/A10124/CRUK_/Cancer Research UK/United Kingdom
- PPRPGM-Nov20\100002/CRUK_/Cancer Research UK/United Kingdom
- U19 CA148065/CA/NCI NIH HHS/United States
- WT_/Wellcome Trust/United Kingdom
- C1287/A10710/CRUK_/Cancer Research UK/United Kingdom
- \#2017NovPR1024/BBC_/Breast Cancer Now/United Kingdom

IMAGES
COMMENTS
Breast Cancer Risk Assessment and Screening in Average-Risk Women: 3: 2003 2011 2017: ... Song F., Chen K. Assessment of performance of the Gail model for predicting breast cancer risk: a systematic review and meta-analysis with trial sequential analysis. Breast Cancer Res. 2018; 20 (1):18. doi: 10.1186/s13058-018-0947-5. [PMC free article] ...
This systematic review identified 40 studies with 47 risk prediction models developed and/or validated for breast cancer among different ethnic groups. Most of the studies used logistic regression to develop breast cancer risk prediction models for Caucasian women by case-control data. The most widely used risk factor was reproductive factors.
A systematic review of qualitative studies where an increased breast cancer risk result has been provided should provide an insight into how those in receipt of a clinically derived risk estimate ...
The increased breast cancer risk (OR 3.89) found among general population women with BI-RADS density D compared to women with BI-RADS density A is lower than what has been reported by previous reviews as by Boyd et al. [24] and McCormack et al. [17]. In these studies higher risk estimates ranging from a four-to five-fold increased risk in women ...
Individualised breast cancer risk prediction models may be key for planning risk-based screening approaches. Our aim was to conduct a systematic review and quality assessment of these models ...
Breast cancer is the most common cancer in women worldwide [].The disease is highly heterogeneous with wide variations in prognosis [].Prognosis means the probability or risk that an outcome (such as deaths, complications, quality of life, pain, or disease regression) develops over a specific time, based on both clinical and non-clinical profiles [].
This systematic review reports that among US women at average risk for breast cancer, mammographic screening is associated with reduction in breast cancer mortality, but the magnitude of the benefits and harms of different screening strategies remains uncertain.
Single nucleotide polymorphisms (SNPs) in the form of a polygenic risk score (PRS) have emerged as a promising factor that could improve the predictive performance of breast cancer (BC) risk prediction tools. This study aims to appraise and critically assess the current evidence on these tools. Studies were identified using Medline, EMBASE and the Cochrane Library up to November 2022 and were ...
Previous systematic reviews have consistently identified primary care providers' lack of knowledge about breast cancer risk assessment and management as a significant barrier to engagement [19,24,25,62]. In line with this, the present review found that insufficient education/training was a prevalent barrier reported for both risk assessment ...
In this systematic review, we thoroughly investigated the existing literature on the application of DL to digital mammography, radiomics, genomics, and clinical information for breast cancer risk assessment. We critically analyzed these studies and discussed their findings, highlighting the promising prospects of DL techniques for breast cancer ...
Background: The benefits and harms of breast screening may be better balanced through a risk-stratified approach. We conducted a systematic review assessing the accuracy of questionnaire-based risk assessment tools for this purpose. Methods: Population: asymptomatic women aged ≥40 years; Intervention: questionnaire-based risk assessment tool (incorporating breast density and polygenic risk ...
Evidence Review This systematic review searched the Medline, Embase, and Cochrane databases up to September 22, 2021, to include studies developing or validating a prediction model for cardiotoxicity in women with breast cancer. The Prediction Model Risk of Bias Assessment Tool (PROBAST) was used to assess both the risk of bias and the ...
Background: Individualised breast cancer risk prediction models may be key for planning risk-based screening approaches. Our aim was to conduct a systematic review and quality assessment of these models addressed to women in the general population. Methods: We followed the Cochrane Collaboration methods searching in Medline, EMBASE and The ...
Breast cancer is the most common malignancy in women and the second leading cause of cancer death overall. Besides genetic, reproductive, and hormonal factors involved in disease onset and progression, greater attention has focused recently on the etiologic role of environmental factors, including exposure to artificial lighting such as light-at-night (LAN). We investigated the extent to which ...
In 2015, the (ECIBC) was launched to develop the European Guidelines on Breast Cancer Screening and Diagnosis. This systematic review informed the recommendations about mammography screening for early detection of BC in asymptomatic women at average risk.
This systematic review identified 40 studies with 47 risk prediction models developed and/or validated for breast cancer among different ethnic groups. Most of the studies used logistic regression to develop breast cancer risk prediction models for Caucasian women by case-control data. The most widely used risk factor was reproductive factors.
Background: The benefits and harms of breast screening may be better balanced through a risk-stratified approach. We conducted a systematic review assessing the accuracy of questionnaire-based risk assessment tools for this purpose. Methods: Population: asymptomatic women aged ≥40 years; Intervention: questionnaire-based risk assessment tool (incorporating breast density and polygenic risk ...
This study is a systematic review of breast cancer risk prediction models by using meta-analysis and the Prediction model study Risk Of Bias Assessment Tool (PROBAST). 13 14 The aim of our study is to systemati-cally review published studies of risk prediction models for breast cancer in the general population, find more
Greater breast cancer risk with regards to BMI also correlates with the concomitant family history of breast cancer . ... Protani M., Coory M., Martin J. Effect of obesity on survival of women with breast cancer: Systematic review and meta-analysis. Breast Cancer Res. Treat.
This systematic review and meta-analysis aimed to assess the safety of systemic HRT on risk of disease recurrence in BC survivors. Methods: A systematic search of PubMed up to April 20, 2021 was conducted to identify randomized controlled trials (RCTs) that investigated the risk of disease recurrence with the use of HRT in BC survivors. A ...
The COVID-19 pandemic brought unplanned service disruption for breast cancer diagnostic, treatment and support services. This scoping review describes these changes and their impact in the UK and ...
Advancements in breast cancer survival rates make the issues of quality of life and psycho-physical wellbeing in survivors central goals of comprehensive care. Anticancer treatments, along with outstanding results on prognosis [ 1 ], also carry burdensome toxicities for every aspect (physical, emotional, social, or financial) of a woman's ...
The present systematic review and meta-analysis included 17 articles, encompassing 525475 patients with breast implants ... The association between the history of breast cancer and the risk of BIA-ALCL was evaluated within 13 articles, including 401898 patients with breast implants [26,27,28,29,30,31, ...
Similarly, a systematic review summarized the evidence regarding the relationship between Fe and breast cancer risk and concluded that this trace element can apply a stimulatory function in breast cancer . Accordingly, it was established that higher iron concentrations can enhance risk of breast cancer in women (41-44). However, iron abundantly ...
Abstract. Background: Evidences which prove relation between breastfeeding women and risk of breast cancer have been limited. Objective: A meta-analysis was carried out on the basis of published literature from clinical trials and studies among different parts of the world. Methods: Studies were analyzed and extracted using PRISMA flowchart.
Semantic Scholar extracted view of "Comment on "The risk of breast cancer and gynecologic malignancies after ovarian stimulation: Meta-analysis of cohort study"." by Zhi-ting Zhang et al. ... Ovulation induction drug and ovarian cancer: an updated systematic review and meta-analysis. Liang-hong Yu Jiafan Sun +5 authors Xiuli Wang. Medicine.
The risk of breast cancer in women is hypothesized to be reduced by breastfeeding which involves two primary mechanisms: (1) differentiation of breast cells where they are modified to generate milk postpregnancy, ... (MOOSE) and preferred items for systematic reviews and meta-analysis (PRISMA). The stages of identifying the related and ...
Centers for Disease Control and Prevention. (CDC). These mutations are estimated to increase the risk of breast cancer four- to seven-fold and put carriers at a 10% to 46% higher risk of ovarian ...
The results of dose-response analysis also showed that an increment of 1 mg/day was inversely related to the risk of breast cancer (RR = 0.94; 95% CI = 0.90-0.99). Conclusions: Results from our meta-analysis indicated that dietary vitamin B2 intake is weakly related to the reduced risk of breast cancer. Additional research is also necessary to ...
However, it is not yet known whether polygenic risk score (PRS) and absolute risk based on a combination of risk factors are associated with the risk of progression of breast cancer. This study aims to estimate the distribution of sojourn time (pre-clinical screen-detectable period) and mammographic sensitivity by absolute breast cancer risk ...