Advertisement

AI in Human Resource Management: Literature Review and Research Implications
- Published: 24 January 2024
Cite this article
- Yuming Zhai 1 ,
- Lixin Zhang 1 &
- Mingchuan Yu ORCID: orcid.org/0000-0001-7576-509X 2
1048 Accesses
Explore all metrics
This study sorted out the literature on the application of AI in HRM from 2012 to 2021 using CiteSpace to derive the history of research in this field. Further, the research emphasis has shifted from the AI algorithm level to the application level. We proposed a conceptual paradox model to explain the positive and negative effects of AI in workplaces. We also discussed theoretically the practical implications of this study. Finally, this study offers relevant information that can help support and expand future research.
This is a preview of subscription content, log in via an institution to check access.
Access this article
Price includes VAT (Russian Federation)
Instant access to the full article PDF.
Rent this article via DeepDyve
Institutional subscriptions
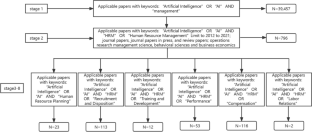
Similar content being viewed by others
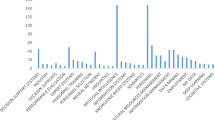
Artificial intelligence and HRM: identifying future research Agenda using systematic literature review and bibliometric analysis
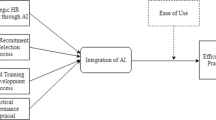
Impact of Artificial Intelligence on HR practices in the UAE
A Bibliometric Analysis of Artificial Intelligence and Human Resource Management
Availability of data and materials.
The datasets generated for this study are available on request to the corresponding author.
Aboytes-Ojeda, M., Castillo-Villar, K. K., & Eksioglu, S. D. (2019). Modeling and optimization of biomass quality variability for decision support systems in biomass supply chains. Annals of Operations ResEarch, 314 (2), 319–346.
Article Google Scholar
Aghion, P., Jones, B. F., & Jones, C. I. (2017). Artificial intelligence and economic growth . NBER Working Papers.
Book Google Scholar
Alavi, M., Marakas, G. M., & Yoo, Y. (2002). A comparative study of distributed learning environments on learning outcomes. Information Systems Research, 13 , 404–415.
Ang, S., & Cummings, L. L. (1994). Panel analysis of feedback-seeking patterns in face-to-face, computer-mediated, and computer-generated communication environments. Perceptual and Motor Skills, 79 , 67–73.
Angrave, D., Charlwood, A., Kirkpatrick, I., Lawrence, M., & Stuart, M. (2016). HR andanalytics: Why HR is set to fail the big data challenge. Human Resource Management Journal, 26 (1), 1–11.
Atsalakis, G. (2014). New technology product demand forecasting using a fuzzy inference system. Operational Research, 14 (2), 225–236.
Bader, V., & Kaiser, S. (2019). Algorithmic decision-making? The user interface and its role for human involvement in decisions supported by artificial intelligence. Organization, 26 (5), 655–672.
Bag, S., Gupta, S., Kumar, A., & Sivarajah, U. (2021). An integrated artificial intelligence framework for knowledge creation and B2B marketing rational decision making for improving firm performance. Industrial Marketing Management, 92 , 178–189.
Ballouki, I., Douimi, M., & Ouzizi, L. (2017). Decision support tool for supply chain configuration considering new product re-design: An agent-based approach. Journal of Advanced Manufacturing Systems, 16 (4), 291–315.
Bankins, S., & Formosa, P. (2020). When AI meets PC: Exploring the implications of workplace social robots and a human-robot psychological contract. European Journal of Work and Organizational Psychology, 29 (2), 215–229.
Beer, D. (2017). The social power of algorithms. Information, Communication & Society, 20 , 1–13.
Beer, M., Spector, B., & Lawrence, P. R. (1984). Managing human assets: The groundbreaking . Harvard Business Program.
Google Scholar
Bell, M. P., Marquardt, D., & Berry, D. P. (2014). Diversity, immigration, and the new American multi-racial hierarchy. Journal of Managerial Psychology, 29 (3), 285–303.
Benbya, H., Davenport, T. H., & Pachidi, S. (2021). Artificial intelligence in organizations: Current state and future opportunities . Social Science Electronic Publishing.
Bera, K., Schalper, K. A., Rimm, D. L., Velcheti, V., & Madabhushi, A. (2019). Artificial intelligence in digital pathology-new tools for diagnosis and precision oncology. Nature Reviews Clinical Oncology, 16 (11), 703–715.
Borges, A. F., Laurindo, F. J., Spínola, M. M., Gonçalves, R. F., & Mattos, C. A. (2021). The strategic use of artificial intelligence in the digital era: Systematic literature review and future research directions. International Journal of Information Management, 57 , 102225.
Braganza, A., Chen, W., Canhoto, A., & Sap, S. (2021). Productive employment and decent work: The impact of AI adoption on psychological contracts, job engagement and employee trust. Journal of Business Research, 131 , 485–494.
Brown, K. G. (2001). Using computers to deliver training: Which employees learn and why. Personnel Psychology, 54 , 271–296.
Brynjolfsson, E., & McAfee, A. (2017). The business of artificial intelligence . Harvard Business Review. https://starlab-alliance.com/wp-content/uploads/2017/09/AI-Article.pdf
Brynjolfsson, E., Rock, D., & Syverson, C. (2017). Artificial intelligence and the modern productivity paradox: A clash of expectations and statistics . National Bureau of Economic Research.
Cançado, L. V., Vendramine, F. M., Corrêa, A. D., Oliveira, J. E., Castro, P. S., Chapman, D. S., Uggerslev, K. L., & Webster, J. (2003). Applicant reactions to face-to-face and technology-mediated interviews: A field investigation. Journal of Applied Psychology, 88 , 944–953.
Cappelli, P., Tambe, P., & Yakubovich, V. (2019). Artificial intelligence in human resources management: challenges and a path forward . Social Science Electronic Publishing.
Cardy, R. L. and Miller, J. S. (2005). eHR and performance management: A consideration of positive potential and the dark side. In H. G. Gueutal, & D. L. Stone (Eds.) , 138–165.
Chapman, D. S., Uggerslev, K. L., & Webster, J. (2003). Applicant reactions to face-to-face and technology-mediated interviews: A field investigation. Journal of Applied Psychology, 88 , 944–953.
Charlwood, A., & Guenole, N. (2022). Can HR adapt to the paradoxes of artificial intelligence. Human Resource Management Journal, 20 , 545–566.
Chen, C. (2017). Science mapping: A systematic review of the literature. Journal of Data and Information Science, 2 (2), 1–40.
Cheng, M. (2017). Causal modeling in HR analytics: A practical guide to models, pitfalls, and suggestions . Academy of Management Proceedings.
Cheng, M. M., & Hackett, R. D. (2019). A critical review of algorithms in HRM: Definition, theory, and practice . Academy of Management Annual Meeting Proceedings.
Chowdhury, R., Rakova, B., Cramer, H., & Yang, J. (2020). Putting responsible AI into practice . MIT Sloan Management Review.
Cleveland Jeanette, N., Byrne Zinta, S., & Cavanagh, T. M. (2015). The future of HR is RH: Respect for humanity at work. Human Resource Management Review, 25 (2), 146–161.
Collings, D. G., Nyberg, A. J., Wright, P. M., & McMackin, J. (2021). Leading through paradox in a COVID-19 world: Human resources comes of age. Human Resource Management Journal, 31 (4), 819–833.
Davenport, T., Guha, A., Grewal, D., & Bressgott, T. (2020). How artificial intelligence will change the future of marketing. Journal of the Academy of Marketing Science, 48 (1), 24–42.
der Aalst, Van, et al. (2018). Robotic Process Automation. Department of Informatics, Decision Sciences and Systems, Technical University of Munich (TUM),. Boltzmannstr, 3 , 85748. Munich, Germany.
Dineen, B. R., & Allen, D. G. (2013). Internet recruiting 2.0: Shifting paradigms. In K. Y. T. Yu & D. M. Cable (Eds.), The Oxford Handbook of Recruitment (pp. 382–401). Oxford University Publishers.
Dineen, B. R., & Soltis, S. M. (2011). Recruitment: A review of research and emerging directions. In S. Zedeck (Ed.), APA handbook of industrial and organizational psychology , 43–66.
Duggan, J., Sherman, U., Carbery, R., & McDonnell, A. (2020). Algorithmic management and app-work in the gig economy: A research agenda for employment relations and HRM. Human Resource Management Journal, 30 (1), 114–132.
Earley, P. C. (1988). Computer-generated performance feedback in the magazine-subscription industry. Organizational Behavior and Human Decision Processes, 41 , 50–64.
European Commission. (2019). A definition of artificial intelligence: Main capabilities and disciplines . EC Report. https://ec.europa.eu/futurium/en/system/files/ged/ai_hleg_definition_of_ai_18_december_1.pdf
Fay, C. H., & Nardoni, R. E. (2009). In M. J. Kavanagh & M. Thite (Eds.), Performance management, compensation, benefits, payroll, and the human resource information system (pp. 338–360)
Ferreira, K. J., Lee, B. H. A., & Simchi-Levi, D. (2016). Analytics for an online retailer: Demand forecasting and price optimization. Manufacturing and Service Operations Management, 18 (1), 69–88.
Flechsig, C., Lohmer, J., & Lasch, R. (2019). Realizing the full potential of robotic process automation through a combination with BPM. Logistics management: Strategies and instruments for digitalizing and decarbonizing supply chains-Proceedings of the German Academic Association for Business Research, Halle, 2019 (pp. 104–119). Springer International Publishing.
Chapter Google Scholar
Ford, M. (2015). The rise of the robots: Technology and the treat of a jobless future . Basic Books.
Gigerenzer, G., & Todd, P. M. (1999). Fast and frugal heuristics: The adaptive toolbox (pp. 3–34). Oxford University Press.
Gill, M. (2000). E-learning technology and strategy for organizations. In K. Fry (Ed.), The business of e-learning: Bringing your organization in the knowledge economy. Sydney, Austrailia: University of Technology.
Gillhan, J. (2018). The macroeconomic impact of artificial intelligence . PWC & Company Report.
Guerrero, L., & Posthuma, R. A. (2014). Perceptions and behaviors of Hispanic workers: A review. Journal of Managerial Psychology, 29 (6), 3–30.
Gupta, S., Modgil, S., & Bhattacharyya, S. (2022). Artificial intelligence for decision support systems in the field of operations research: Review and future scope of research. Annals of Operations Research, 308 , 215–274.
Guner, H. U., Chinnam, R. B., & Murat, A. (2016). Simulation platform for anticipative plant-level maintenance decision support system. International Journal of Production Research, 54 (6), 1785–1803.
Haenlein, M., & Kaplan, A. (2019). A brief history of artificial intelligence: On the past, present, and future of artificial intelligence. California Management Review, 61 (4), 5–14.
Herrmann, H. (2022). The arcanum of artificial intelligence in enterprise applications: Toward a unified framework. Journal of Engineering and Technology Management, 66 , 101716.
Hirschman, A. O. (1970). Exit, voice, and loyalty. Responses to decline in firms, organizations, and states . Cambridge, MA: Harvard University Press.
Hsiao, J. P., Jaw, C., Huan, T.-C., & Woodside, A. G. (2015). Applying complexity theory to solve hospitality contrarian case conundrums. International Journal of Contemporary Hospitality Management, 27 (4), 608–647.
Jarrahi, M. H. (2018). Artificial intelligence and the future of work: Human-AI symbiosis in organizational decision making. Business Horizons, 61 (4), 577–586.
Jatobá, M., Santos, J., Gutierriz, I., Moscon, D., Fernandes, P. O., & Teixeira, J. P. (2016). Evolution of artificial intelligence research in human resources. Procedia Computer Science, 164 , 137–142.
Jia, Q., Guo, Y., Li, Y., & Chen, Y. (2018). A conceptual artificial intelligence application framework in human resource management (pp. 106–114). Proceedings of the 18th International Conference on Electronic Business.
Jeske, D., & Santuzzi, A. M. (2015). Monitoring what and how: Psychological implications of electronic performance monitoring. New Technology, Work and Employment, 30 (1), 62–78.
Jin, X.-H., & Zhang, G. (2011). Modelling optimal risk allocation in PPP projects using artificial neural networks. International Journal of Project Management, 29 (5), 591–603.
Johnson, R. D., & Gueutal, H. G. (2011). Transforming HR through technology: The use of her and human resource information systems in organizations. SHRM effective practices guidelines series. Alexandria, Virginia: SHRM.
Johnson, R. D., Hornik, S., & Salas, E. (2008). An empirical examination of factors contributing to the creation of successful e-learning environments. International Journal of Human-Computer Studies, 66 , 356–369.
Kaplan, J. (2016). Humans Need not apply: A guide to wealth and work in the age of artificial intelligence . Yale University Press.
Kaplan, A., & Haenlein, M. (2019). Siri, Siri, in my hand: Who’s the fairest in the land? On the interpretations, illustrations, and implications of artificial intelligence. Business Horizons, 62 (1), 15–25.
Kaplan, A., & Haenlein, M. (2020). Rulers of the world, unite! The challenges and opportunities of artificial intelligence. Business Horizons, 63 (1), 37–50.
Larson, L., & DeChurch, L. A. (2020). Leading teams in the digital age: Four perspectives on technology and what they mean for leading teams. The Leadship Quarterly, 31 (1), 101–377.
Lee, M. K. (2018). Understanding perception of algorithmic decisions: Fairness, trust, and emotion in response to algorithmic management. Big Data & Society, 5 (1), 1–15.
Lee, K. C., Lee, N., & Lee, H. (2012). Multi-agent knowledge integration mechanism using particle swarm optimization. Technological Forecasting and Social Change, 79 (3), 469–484.
Lee, M. K., Jain, A., Cha, H. J., Ojha, S., & Kusbit, D. (2019). Procedural justice in algorithmic fairness: Leveraging transparency and outcome control for fair algorithmic mediation. Proceedings of the ACM on Human-Computer Interaction, 3 (CSCW), 1–26.
Leonardi, P., & Contractor, N. (2018). Better people analytics measure who they know, not just who they are . Harvard Business Review.
Li, M. (2019). Are your algorithms upholding your standards of fairness? Harvard Business Review. https://hbr.org/2019/11/are-your-algorithms-upholding-your-standards-of-fairness
Li. (2021). The application of artificial intelligence in enterprise financial risk prevention and control—Based on big data environment. Finance and Accounting Communications, 20 , 62.
Lichtenthaler, U. (2019). Extremes of acceptance: Employee attitudes toward artificial intelligence. Journal of Business Strategy, 41 (5), 39–45.
Lin, Y., Lei, H., Addo, P. C., et al. (2016). Machine learned resume-job matching solution . arXiv preprint arXiv:1607.07657
Loebbecke, C., & Picot, A. (2015). Reflections on societal and business model transformation arising from digitization and big data analytics: A research agenda. The Journal of Strategic Information Systems, 24 (3), 149–157.
Mariana, B., & Drăguţ, L. D. (2016). Random forest in remote sensing: A review of applications and future directions. Isprs Journal of Photogrammetry and Remote Sensing, 114 , 24–31.
McCarthy, J., Minsky, M. L., Rochester, N., & Shannon, C. E. (2006). A proposal for the Dartmouth summer research project on artificial intelligence. AI Magazine, 27 (4), 12–14.
McCorduck, P. (1979). Machines who think: A personal inquiry into the history and prospects of artificial intelligence . San Francisco, CA: W.H. Freeman and Company.
Mesquita, L. D. (2018). The “artificial” consumer: Approaches between artificial intelligence and marketing (pp. 3–6). Enanpad, Curitiba: ANPAD Meetings.
Mikalef, P., & Gupta, M. (2021). Artificial intelligence capability: Conceptualization, measurement calibration, and empirical study on its impact on organizational creativity and firm performance. Information & Management, 58 (3), 103–434.
Moher, D., Liberati, A., Tetzlaff, J., & Altman, D. G. (2009). Preferred reporting items for systematic reviews and meta-analyses: The PRISMA statement. Annals of Internal Medicine, 151 , 264–269.
Nascimento, M. A., & Anna, Q. M. (2010). The quest for artificial intelligence: A history of ideas and achievements (pp. 1–4). Enanpad, São Paulo: ANPAD Meetings.
Nilsson, N. (1971). Problem-solving methods in artificial intelligence . New York: McGraw-Hill.
Nilsson, N. J. (2010). The Quest for Artificial Intelligence: A history of Idea sand Achievements. Cambridge University Press, Kybernetes, 40 (9/10), 1553–1553.
O’Connell, M. S., Doverspike, D., Gillikin, S., & Meloun, J. M. (2001). Computer anxiety: Effects on computerized testing and implications for e-recruiting. Journal of e-Commerce and Psychology, 1 , 25–39.
OECD (2019). Artificial intelligence in society. OECD Publishing. Organisation for Economic Co-operation and Development. https://www.oecd.org/publications/artificial-intelligence-in-society-eedfee77-en.htm
Oracle and Future Workplace. (2019). Global research highlights how AI is changing the relationship between people and technology at work . Oracle.
Orvis, K. A., Brusso, R. C., Wasserman, M. E., & Fisher, S. L. (2011). Enabled for e-learning? The moderating role of personality in determining the optimal degree of learner control in an e-learning environment. Human Performance, 24 , 60–78.
Pessach, D., Singer, G., Avrahami, D., Ben-Gal, H. C., Shmueli, E., & Ben-Gal, I. (2020). Employees recruitment: A prescriptive analytics approach via machine learning and mathematical programming. Decision Support Systems, 134 , 113–290.
Petriglieri, G., Ashford, S. J., & Wrzesniewski, A. (2019). Agony and ecstasy in the gig economy: Cultivating holding environments for precarious and personalized work identities. Administrative Science Quarterly, 64 , 124–170.
Phillips-Wren, G., Mora, M., Forgionne, G. A., & Gupta, J. N. (2009). An integrative evaluation framework for intelligent decision support systems. European Journal of Operational Research, 195 (3), 642–652.
Piccoli, G., Ahmad, R., & Ives, B. (2001). Web-based virtual learning environments: A research framework and a preliminary assessment of effectiveness in basic IT skills training. MIS Quarterly, 25 , 401–426.
Raisch, S., & Krakowski, S. (2021). Artificial intelligence and management: The automation–augmentation paradox. Academy of Management Review, 46 (1), 192–210.
Rampersad, G. (2020). Robot will take your job: Innovation for an era of artificial intelligence. Journal of Business Research, 116 , 68–74.
Ransbotham, S., Candelon, F., Kiron, D., LaFountain, B., & Khodabandeh, S. (2021). The cultural benefits of artificial intelligence in the Enterprise . Cambridge, MA, USA: MIT Sloan Management Review and Boston Consulting Group.
Reiter-Palmon, R., Brown, M., Sandall, D. L., Buboltz, C., & Nimps, T. (2006). Development of an O*NET web-based job analysis and its implementation in the U.S. Navy: Lessons learned. Human Resource Management Review, 16 , 294–309.
Ritzer, G. (2011). The Mcdonaldization of Society . Sage.
Ruël, H., Bondarouk, T., & Looise, J. K. (2004). E-HRM: Innovation or irritation. An explorative empirical study in five large companies on web-based HRM. Management Revue, 15 , 364–380.
Salas, E., DeRouin, R., & Littrell, L. (2005). Research based guidelines for distance learning: What we know so far. In H. G. Gueutal & D. L. Stone (Eds.), The brave new world of eHR: Human resources management in the digital age (pp. 104–137). San Francisco: Jossey Bass.
Silverman, R. E., & Gellman, L. (2015). Women in the workplace (A special report) – Apps to battle job bias: Software takes on hiring and workplace practices. The Wall Street Journal Eastern edition.
Stein, V., & Scholz, T. M. (2020). Manufacturing revolution boosts people issues: The evolutionary need for “human automation resource management” in smart factories. European Management Review, 17 (2), 391–406.
Stoltzfus, J. C. (2011). Logistic regrssion: A brief primer. Academic Emergency Medicine, 10 , 1099–1104.
Stone, D. L., Deadrick, D. L., Lukaszewski, K. M., & Johnson, R. (2015). The influence of technology on the future of human resource management. Human Resource Management Review, 25 (2), 216–231.
Stone, D. L., Lukaszewski, K., & Isenhour, L. C. (2005). E-Recruiting: Online strategies for attracting talent. In H. G. Gueutal & D. L. Stone (Eds.), The Brave New World of eHR: Human Resources Management in the Digital Age (pp. 22–53). Jossey Bass.
Sullivan, J. (2014). A walk through the HR department of 2020 (pp. 7–9). Workforce Solutions Review.
Tambe, P., Hitt, L. M., Rock, D., & Brynjolfsson, E. (2019). IT, AI and the growth of intangible capital . Available at SSRN 3416289.
Toniolo, K., Masiero, E., Massaro, M., & Bagnoli, C. (2020). Sustainable business models and artificial intelligence: Opportunities and challenges. Knowledge, people, and digital transformation (pp. 103–117). Springer.
Ulrich, D., Younger, J., Brockbank, W., & Ulrich, M. (2012). HR from the outside in: Six competencies for the future of human resources . McGraw Hill.
van den Broek, E., Sergeeva, A., Huysman, M., & Huysman Vrije, M. (2021). When the machine meets the expert: An ethnography of developing AI for hiring. Management Information Systems Quarterly, 45 (3), 1557–1580.
Vrontis, D., Christofi, M., Pereira, V. E., Tarba, S. Y., Makrides, A., & Trichina, E. (2021). Artificial intelligence, robotics, advanced technologies and human resource management: A systematic review. The International Journal of Human Resource Management, 33 , 1237–1266.
Wallace, P., & Clariana, R. B. (2005). Test mode familiarity and performance-Gender and race comparisons of test scores among computer literate students in advanced information systems. Journal of Information Systems Education, 16 , 177–183.
Wamba-Taguimdje, S.-L., Wamba, S. F., Kamdjoug, J. R. K., & Wanko, C. E. T. (2020). Influence of artificial intelligence (AI) on firm performance: The business value of AI-based transformation projects. Business Process Management Journal, 26 (7), 1893–1924.
Wang, X., Wang, L., Zhang, L., Xu, X., Zhang, W., & Xu, Y. (2017). Developing an employee turnover risk evaluation model using case-based reasoning. Information Systems Frontiers, 19 (3), 569–576.
Welsh, E. T., Wanberg, C. R., Brown, E. G., & Simmering, M. J. (2003). E-learning: Emerging uses, empircial results and future direction. International Journal of Training and Development, 7 , 245–258.
Wei, J. (2019). Applying machine learning to human resource management systems . Huazhong University of Science and Technology. Dissertation.
Winston, P. H. (1984). Artificial intelligence (2nd ed., pp. 75–80). Addison-Wesley Longman Publishing Co. Inc.
Winston, P. E. (2015). Model-based story summary . Atlanta: 6th International Workshop on Computational Models of Narrative.
Xu, Q., Liu, X., Jiang, C., & Yu, K. (2016). Nonparametric conditional autoregressive expectile model via neural network with applications to estimating financial risk. Applied Stochastic Models in Business and Industry, 32 (6), 882–908.
Zhou, Z. (2016). Machine learning . Tsinghua University Press.
Zhou, S., Yu, B., Sun, A., Long, C., Li, J., Yu, H., & Li, Y. (2022). A survey on neural open information extraction: Current status and future directions . arXiv preprint arXiv:2205.11725
Download references
This study was supported by the National Natural Science Foundation of China (Grant Nos. 71802134 and 72372079).
Author information
Authors and affiliations.
School of Economics & Management, Shanghai Institute of Technology, Shanghai, 201418, China
Yuming Zhai & Lixin Zhang
College of Business Administration, Ningbo University of Finance and Economics, Ningbo, 315175, China
Mingchuan Yu
You can also search for this author in PubMed Google Scholar
Corresponding author
Correspondence to Mingchuan Yu .
Ethics declarations
Conflict of interest.
The authors declared no competing interests.
Additional information
Publisher's note.
Springer Nature remains neutral with regard to jurisdictional claims in published maps and institutional affiliations.
Rights and permissions
Springer Nature or its licensor (e.g. a society or other partner) holds exclusive rights to this article under a publishing agreement with the author(s) or other rightsholder(s); author self-archiving of the accepted manuscript version of this article is solely governed by the terms of such publishing agreement and applicable law.
Reprints and permissions
About this article
Zhai, Y., Zhang, L. & Yu, M. AI in Human Resource Management: Literature Review and Research Implications. J Knowl Econ (2024). https://doi.org/10.1007/s13132-023-01631-z
Download citation
Received : 03 May 2023
Accepted : 13 November 2023
Published : 24 January 2024
DOI : https://doi.org/10.1007/s13132-023-01631-z
Share this article
Anyone you share the following link with will be able to read this content:
Sorry, a shareable link is not currently available for this article.
Provided by the Springer Nature SharedIt content-sharing initiative
- Artificial intelligence
- Human resource management
- Bibliometric review
- Paradox lens
- Find a journal
- Publish with us
- Track your research

Human Resource Management
- Find Articles Help
- Human Resources Dictionary & Glossary of Terms
- Find Course Journal TItles - Possibly Delete
- Search Tips and Tutorials
- Finding Dissertations
- APA Citation Help
What is a Literature Review?
- RefWorks Tutorials
- HRM 310 Weekly Resources (2020)
- HRM 311 Weekly Resources (2020)
- Jobs, Careers, Recruiting
- Professional Associations/Organizations
- Training and Development
- Find Books and eBooks
- Get Items from Other Libraries/ILLiad (Interlibrary Loan)
The Purpose of a Literature Review is to gain an understanding of the existing research relevant to a particular topic or area of study and to present that knowledge in the form of a written report. Learning important concepts, and research methods will bring insights into the topic chosen. Ultimately, achieving a better understanding of a particular discipline and/or topic based upon the research findings.
Writing the Literature Review: Step-by-Step Tutorial for Graduate Students - David Taylor, University of Maryland, Collage Park
The Literature Review Part Two - David Taylor, University of Maryland, College Park
- << Previous: APA Citation Help
- Next: RefWorks >>
- Last Updated: May 16, 2024 2:18 PM
- Guide URL: https://libguides.wilmu.edu/HRM/MHR

- Open access
- Published: 14 May 2024
Protocol for a scoping review study on learning plan use in undergraduate medical education
- Anna Romanova ORCID: orcid.org/0000-0003-1118-1604 1 ,
- Claire Touchie 1 ,
- Sydney Ruller 2 ,
- Victoria Cole 3 &
- Susan Humphrey-Murto 4
Systematic Reviews volume 13 , Article number: 131 ( 2024 ) Cite this article
79 Accesses
Metrics details
The current paradigm of competency-based medical education and learner-centredness requires learners to take an active role in their training. However, deliberate and planned continual assessment and performance improvement is hindered by the fragmented nature of many medical training programs. Attempts to bridge this continuity gap between supervision and feedback through learner handover have been controversial. Learning plans are an alternate educational tool that helps trainees identify their learning needs and facilitate longitudinal assessment by providing supervisors with a roadmap of their goals. Informed by self-regulated learning theory, learning plans may be the answer to track trainees’ progress along their learning trajectory. The purpose of this study is to summarise the literature regarding learning plan use specifically in undergraduate medical education and explore the student’s role in all stages of learning plan development and implementation.
Following Arksey and O’Malley’s framework, a scoping review will be conducted to explore the use of learning plans in undergraduate medical education. Literature searches will be conducted using multiple databases by a librarian with expertise in scoping reviews. Through an iterative process, inclusion and exclusion criteria will be developed and a data extraction form refined. Data will be analysed using quantitative and qualitative content analyses.
By summarising the literature on learning plan use in undergraduate medical education, this study aims to better understand how to support self-regulated learning in undergraduate medical education. The results from this project will inform future scholarly work in competency-based medical education at the undergraduate level and have implications for improving feedback and supporting learners at all levels of competence.
Scoping review registration:
Open Science Framework osf.io/wvzbx.
Peer Review reports
Competency-based medical education (CBME) has transformed the approach to medical education to focus on demonstration of acquired competencies rather than time-based completion of rotations [ 1 ]. As a result, undergraduate and graduate medical training programs worldwide have adopted outcomes-based assessments in the form of entrustable professional activities (EPAs) comprised of competencies to be met [ 2 ]. These assessments are completed longitudinally by multiple different evaluators to generate an overall impression of a learner’s competency.
In CBME, trainees will progress along their learning trajectory at individual speeds and some may excel while others struggle to achieve the required knowledge, skills or attitudes. Therefore, deliberate and planned continual assessment and performance improvement is required. However, due to the fragmented nature of many medical training programs where learners rotate through different rotations and work with many supervisors, longitudinal observation is similarly fragmented. This makes it difficult to determine where trainees are on their learning trajectories and can affect the quality of feedback provided to them, which is a known major influencer of academic achievement [ 3 ]. As a result, struggling learners may not be identified until late in their training and the growth of high-performing learners may be stifled [ 4 , 5 , 6 ].
Bridging this continuity gap between supervision and feedback through some form of learner handover or forward feeding has been debated since the 1970s and continues to this day [ 5 , 7 , 8 , 9 , 10 , 11 ]. The goal of learner handover is to improve trainee assessment and feedback by sharing their performance and learning needs between supervisors or across rotations. However, several concerns have been raised about this approach including that it could inappropriately bias subsequent assessments of the learner’s abilities [ 9 , 11 , 12 ]. A different approach to keeping track of trainees’ learning goals and progress along their learning trajectories is required. Learning plans (LPs) informed by self-regulated learning (SRL) theory may be the answer.
SRL has been defined as a cyclical process where learners actively control their thoughts, actions and motivation to achieve their goals [ 13 ]. Several models of SRL exist but all entail that the trainee is responsible for setting, planning, executing, monitoring and reflecting on their learning goals [ 13 ]. According to Zimmerman’s SRL model, this process occurs in three stages: forethought phase before an activity, performance phase during an activity and self-reflection phase after an activity [ 13 ]. Since each trainee leads their own learning process and has an individual trajectory towards competence, this theory relates well to the CBME paradigm which is grounded in learner-centredness [ 1 ]. However, we know that medical students and residents have difficulty identifying their own learning goals and therefore need guidance to effectively partake in SRL [ 14 , 15 , 16 , 17 ]. Motivation has also emerged as a key component of SRL, and numerous studies have explored factors that influence student engagement in learning [ 18 , 19 ]. In addition to meeting their basic psychological needs of autonomy, relatedness and competence, perceived learning relevance through meaningful learning activities has been shown to increase trainee engagement in their learning [ 19 ].
LPs are a well-known tool across many educational fields including CBME that can provide trainees with meaningful learning activities since they help them direct their own learning goals in a guided fashion [ 20 ]. Also known as personal learning plans, learning contracts, personal action plans, personal development plans, and learning goals, LPs are documents that outline the learner’s roadmap to achieve their learning goals. They require the learner to self-identify what they need to learn and why, how they are going to do it, how they will know when they are finished, define the timeframe for goal achievement and assess the impact of their learning [ 20 ]. In so doing, LPs give more autonomy to the learner and facilitate objective and targeted feedback from supervisors. This approach has been described as “most congruent with the assumptions we make about adults as learners” [ 21 ].
LP use has been explored across various clinical settings and at all levels of medical education; however, most of the experience lies in postgraduate medical education [ 22 ]. Medical students are a unique learner population with learning needs that appear to be very well suited for using LPs for two main reasons. First, their education is often divided between classroom and clinical settings. During clinical training, students need to be more independent in setting learning goals to meet desired competencies as their education is no longer outlined for them in a detailed fashion by the medical school curriculum [ 23 ]. SRL in the workplace is also different than in the classroom due to additional complexities of clinical care that can impact students’ ability to self-regulate their learning [ 24 ]. Second, although most medical trainees have difficulty with goal setting, medical students in particular need more guidance compared to residents due to their relative lack of experience upon which they can build within the SRL framework [ 25 ]. LPs can therefore provide much-needed structure to their learning but should be guided by an experienced tutor to be effective [ 15 , 24 ].
LPs fit well within the learner-centred educational framework of CBME by helping trainees identify their learning needs and facilitating longitudinal assessment by providing supervisors with a roadmap of their goals. In so doing, they can address current issues with learner handover and identification as well as remediation of struggling learners. Moreover, they have the potential to help trainees develop lifelong skills with respect to continuing professional development after graduation which is required by many medical licensing bodies.
An initial search of the JBI Database, Cochrane Database, MEDLINE (PubMed) and Google Scholar conducted in July–August 2022 revealed a paucity of research on LP use in undergraduate medical education (UGME). A related systematic review by van Houten–Schat et al. [ 24 ] on SRL in the clinical setting identified three interventions used by medical students and residents in SRL—coaching, LPs and supportive tools. However, only a couple of the included studies looked specifically at medical students’ use of LPs, so this remains an area in need of more exploration. A scoping review would provide an excellent starting point to map the body of literature on this topic.
The objective of this scoping review will therefore be to explore LP use in UGME. In doing so, it will address a gap in knowledge and help determine additional areas for research.
This study will follow Arksey and O’Malley’s [ 26 ] five-step framework for scoping review methodology. It will not include the optional sixth step which entails stakeholder consultation as relevant stakeholders will be intentionally included in the research team (a member of UGME leadership, a medical student and a first-year resident).

Step 1—Identifying the research question
The overarching purpose of this study is to “explore the use of LPs in UGME”. More specifically we seek to achieve the following:
Summarise the literature regarding the use of LPs in UGME (including context, students targeted, frameworks used)
Explore the role of the student in all stages of the LP development and implementation
Determine existing research gaps
Step 2—Identifying relevant studies
An experienced health sciences librarian (VC) will conduct all searches and develop the initial search strategy. The preliminary search strategy is shown in Appendix A (see Additional file 2). Articles will be included if they meet the following criteria [ 27 ]:
Participants
Medical students enrolled at a medical school at the undergraduate level.
Any use of LPs by medical students. LPs are defined as a document, usually presented in a table format, that outlines the learner’s roadmap to achieve their learning goals [ 20 ].
Any stage of UGME in any geographic setting.
Types of evidence sources
We will search existing published and unpublished (grey) literature. This may include research studies, reviews, or expert opinion pieces.
Search strategy
With the assistance of an experienced librarian (VC), a pilot search will be conducted to inform the final search strategy. A search will be conducted in the following electronic databases: MEDLINE, Embase, Education Source, APA PsycInfo and Web of Science. The search terms will be developed in consultation with the research team and librarian. The search strategy will proceed according to the JBI Manual for Evidence Synthesis three-step search strategy for reviews [ 27 ]. First, we will conduct a limited search in two appropriate online databases and analyse text words from the title, abstracts and index terms of relevant papers. Next, we will conduct a second search using all identified key words in all databases. Third, we will review reference lists of all included studies to identify further relevant studies to include in the review. We will also contact the authors of relevant papers for further information if required. This will be an iterative process as the research team becomes more familiar with the literature and will be guided by the librarian. Any modifications to the search strategy as it evolves will be described in the scoping review report. As a measure of rigour, the search strategy will be peer-reviewed by another librarian using the PRESS checklist [ 28 ]. No language or date limits will be applied.
Step 3—Study selection
The screening process will consist of a two-step approach: screening titles/abstracts and, if they meet inclusion criteria, this will be followed by a full-text review. All screening will be done by two members of the research team and any disagreements will be resolved by an independent third member of the team. Based on preliminary inclusion criteria, the whole research team will first pilot the screening process by reviewing a random sample of 25 titles/abstracts. The search strategy, eligibility criteria and study objectives will be refined in an iterative process. We anticipate several meetings as the topic is not well described in the literature. A flowchart of the review process will be generated. Any modifications to the study selection process will be described in the scoping review report. The papers will be excluded if a full text is not available. The search results will be managed using Covidence software.
Step 4—Charting the data
A preliminary data extraction tool is shown in Appendix B (see Additional file 3 ). Data will be extracted into Excel and will include demographic information and specific details about the population, concept, context, study methods and outcomes as they relate to the scoping review objectives. The whole research team will pilot the data extraction tool on ten articles selected for full-text review. Through an iterative process, the final data extraction form will be refined. Subsequently, two members of the team will independently extract data from all articles included for full-text review using this tool. Charting disagreements will be resolved by the principal and senior investigators. Google Translate will be used for any included articles that are not in the English language.
Step 5—Collating, summarising and reporting the results
Quantitative and qualitative analyses will be used to summarise the results. Quantitative analysis will capture descriptive statistics with details about the population, concept, context, study methods and outcomes being examined in this scoping review. Qualitative content analysis will enable interpretation of text data through the systematic classification process of coding and identifying themes and patterns [ 29 ]. Several team meetings will be held to review potential themes to ensure an accurate representation of the data. The PRISMA Extension for Scoping Reviews (PRISMA-ScR) will be used to guide the reporting of review findings [ 30 ]. Data will be presented in tables and/or diagrams as applicable. A descriptive summary will explain the presented results and how they relate to the scoping review objectives.
By summarising the literature on LP use in UGME, this study will contribute to a better understanding of how to support SRL amongst medical students. The results from this project will also inform future scholarly work in CBME at the undergraduate level and have implications for improving feedback as well as supporting learners at all levels of competence. In doing so, this study may have practical applications by informing learning plan incorporation into CBME-based curricula.
We do not anticipate any practical or operational issues at this time. We assembled a team with the necessary expertise and tools to complete this project.
Availability of data and materials
All data generated or analysed during this study will be included in the published scoping review article.
Abbreviations
- Competency-based medical education
Entrustable professional activity
- Learning plan
- Self-regulated learning
- Undergraduate medical education
Frank JR, Snell LS, Cate OT, et al. Competency-based medical education: theory to practice. Med Teach. 2010;32(8):638–45.
Article PubMed Google Scholar
Shorey S, Lau TC, Lau ST, Ang E. Entrustable professional activities in health care education: a scoping review. Med Educ. 2019;53(8):766–77.
Hattie J, Timperley H. The power of feedback. Rev Educ Res. 2007;77(1):81–112.
Article Google Scholar
Dudek NL, Marks MB, Regehr G. Failure to fail: the perspectives of clinical supervisors. Acad Med. 2005;80(10 Suppl):S84–7.
Warm EJ, Englander R, Pereira A, Barach P. Improving learner handovers in medical education. Acad Med. 2017;92(7):927–31.
Spooner M, Duane C, Uygur J, et al. Self-regulatory learning theory as a lens on how undergraduate and postgraduate learners respond to feedback: a BEME scoping review : BEME Guide No. 66. Med Teach. 2022;44(1):3–18.
Frellsen SL, Baker EA, Papp KK, Durning SJ. Medical school policies regarding struggling medical students during the internal medicine clerkships: results of a National Survey. Acad Med. 2008;83(9):876–81.
Humphrey-Murto S, LeBlanc A, Touchie C, et al. The influence of prior performance information on ratings of current performance and implications for learner handover: a scoping review. Acad Med. 2019;94(7):1050–7.
Morgan HK, Mejicano GC, Skochelak S, et al. A responsible educational handover: improving communication to improve learning. Acad Med. 2020;95(2):194–9.
Dory V, Danoff D, Plotnick LH, et al. Does educational handover influence subsequent assessment? Acad Med. 2021;96(1):118–25.
Humphrey-Murto S, Lingard L, Varpio L, et al. Learner handover: who is it really for? Acad Med. 2021;96(4):592–8.
Shaw T, Wood TJ, Touchie T, Pugh D, Humphrey-Murto S. How biased are you? The effect of prior performance information on attending physician ratings and implications for learner handover. Adv Health Sci Educ Theory Pract. 2021;26(1):199–214.
Artino AR, Brydges R, Gruppen LD. Chapter 14: Self-regulated learning in health professional education: theoretical perspectives and research methods. In: Cleland J, Duning SJ, editors. Researching Medical Education. 1st ed. John Wiley & Sons; 2015. p. 155–66.
Chapter Google Scholar
Cleland J, Arnold R, Chesser A. Failing finals is often a surprise for the student but not the teacher: identifying difficulties and supporting students with academic difficulties. Med Teach. 2005;27(6):504–8.
Reed S, Lockspeiser TM, Burke A, et al. Practical suggestions for the creation and use of meaningful learning goals in graduate medical education. Acad Pediatr. 2016;16(1):20–4.
Wolff M, Stojan J, Cranford J, et al. The impact of informed self-assessment on the development of medical students’ learning goals. Med Teach. 2018;40(3):296–301.
Sawatsky AP, Halvorsen AJ, Daniels PR, et al. Characteristics and quality of rotation-specific resident learning goals: a prospective study. Med Educ Online. 2020;25(1):1714198.
Article PubMed PubMed Central Google Scholar
Pintrich PR. Chapter 14: The role of goal orientation in self-regulated learning. In: Boekaerts M, Pintrich PR, Zeidner M, editors. Handbook of self-regulation. 1st ed. Academic Press; 2000. p. 451–502.
Kassab SE, El-Sayed W, Hamdy H. Student engagement in undergraduate medical education: a scoping review. Med Educ. 2022;56(7):703–15.
Challis M. AMEE medical education guide No. 19: Personal learning plans. Med Teach. 2000;22(3):225–36.
Knowles MS. Using learning contracts. 1 st ed. San Francisco: Jossey Bass; 1986.
Parsell G, Bligh J. Contract learning, clinical learning and clinicians. Postgrad Med J. 1996;72(847):284–9.
Article CAS PubMed PubMed Central Google Scholar
Teunissen PW, Scheele F, Scherpbier AJJA, et al. How residents learn: qualitative evidence for the pivotal role of clinical activities. Med Educ. 2007;41(8):763–70.
Article CAS PubMed Google Scholar
van Houten-Schat MA, Berkhout JJ, van Dijk N, Endedijk MD, Jaarsma ADC, Diemers AD. Self-regulated learning in the clinical context: a systematic review. Med Educ. 2018;52(10):1008–15.
Taylor DCM, Hamdy H. Adult learning theories: Implications for learning and teaching in medical education: AMEE Guide No. 83. Med Teach. 2013;35(11):e1561–72.
Arksey H, O’Malley L. Scoping studies: towards a methodological framework. Int J Soc Res Methodol. 2005;8(1):19–32.
Peters MDJ, Godfrey C, McInerney P, Munn Z, Tricco AC, Khalol H. Chapter 11: Scoping reviews. In: Aromataris E, Munn Z, eds. JBI Manual for Evidence Synthesis. JBI; 2020. https://synthesismanual.jbi.global. . Accessed 30 Aug 2022.
McGowan J, Sampson M, Salzwedel DM, Cogo E, Foerster V, Lefebvre C. PRESS Peer Review of Electronic Search Strategies: 2015 Guideline Statement. J Clin Epidemiol. 2016;75:40–6.
Hsieh HF, Shannon SE. Three approaches to qualitative content analysis. Qual Health Res. 2005;15(9):1277–88.
Tricco AC, Lillie E, Zarin W, et al. PRISMA extension for scoping reviews (PRISMA-ScR): checklist and explanation. Ann Intern Med. 2018;169(7):467–73.
Venables M, Larocque A, Sikora L, Archibald D, Grudniewicz A. Understanding indigenous health education and exploring indigenous anti-racism approaches in undergraduate medical education: a scoping review protocol. OSF; 2022. https://osf.io/umwgr/ . Accessed 26 Oct 2022.
Download references
Acknowledgements
Not applicable.
This study will be supported through grants from the Department of Medicine at the Ottawa Hospital and the University of Ottawa. The funding bodies had no role in the study design and will not have any role in the collection, analysis and interpretation of data or writing of the manuscript.
Author information
Authors and affiliations.
The Ottawa Hospital – General Campus, 501 Smyth Rd, PO Box 209, Ottawa, ON, K1H 8L6, Canada
Anna Romanova & Claire Touchie
The Ottawa Hospital Research Institute, Ottawa, Canada
Sydney Ruller
The University of Ottawa, Ottawa, Canada
Victoria Cole
The Ottawa Hospital – Riverside Campus, Ottawa, Canada
Susan Humphrey-Murto
You can also search for this author in PubMed Google Scholar
Contributions
AR designed and drafted the protocol. CT and SH contributed to the refinement of the research question, study methods and editing of the manuscript. VC designed the initial search strategy. All authors reviewed the manuscript for final approval. The review guarantors are CT and SH. The corresponding author is AR.
Authors’ information
AR is a clinician teacher and Assistant Professor with the Division of General Internal Medicine at the University of Ottawa. She is also the Associate Director for the internal medicine clerkship rotation at the General campus of the Ottawa Hospital.
CT is a Professor of Medicine with the Divisions of General Internal Medicine and Infectious Diseases at the University of Ottawa. She is also a member of the UGME Competence Committee at the University of Ottawa and an advisor for the development of a new school of medicine at Toronto Metropolitan University.
SH is an Associate Professor with the Department of Medicine at the University of Ottawa and holds a Tier 2 Research Chair in Medical Education. She is also the Interim Director for the Research Support Unit within the Department of Innovation in Medical Education at the University of Ottawa.
CT and SH have extensive experience with medical education research and have numerous publications in this field.
SR is a Research Assistant with the Division of General Internal Medicine at the Ottawa Hospital Research Institute.
VC is a Health Sciences Research Librarian at the University of Ottawa.
SR and VC have extensive experience in systematic and scoping reviews.
Corresponding author
Correspondence to Anna Romanova .
Ethics declarations
Ethics approval and consent to participate, consent for publication, competing interests.
The authors declare that they have no competing interests.
Additional information
Publisher's note.
Springer Nature remains neutral with regard to jurisdictional claims in published maps and institutional affiliations.
Supplementary Information
Additional file 1. prisma-p 2015 checklist., 13643_2024_2553_moesm2_esm.docx.
Additional file 2: Appendix A. Preliminary search strategy [ 31 ].
Additional file 3: Appendix B. Preliminary data extraction tool.
Rights and permissions.
Open Access This article is licensed under a Creative Commons Attribution 4.0 International License, which permits use, sharing, adaptation, distribution and reproduction in any medium or format, as long as you give appropriate credit to the original author(s) and the source, provide a link to the Creative Commons licence, and indicate if changes were made. The images or other third party material in this article are included in the article's Creative Commons licence, unless indicated otherwise in a credit line to the material. If material is not included in the article's Creative Commons licence and your intended use is not permitted by statutory regulation or exceeds the permitted use, you will need to obtain permission directly from the copyright holder. To view a copy of this licence, visit http://creativecommons.org/licenses/by/4.0/ . The Creative Commons Public Domain Dedication waiver ( http://creativecommons.org/publicdomain/zero/1.0/ ) applies to the data made available in this article, unless otherwise stated in a credit line to the data.
Reprints and permissions
About this article
Cite this article.
Romanova, A., Touchie, C., Ruller, S. et al. Protocol for a scoping review study on learning plan use in undergraduate medical education. Syst Rev 13 , 131 (2024). https://doi.org/10.1186/s13643-024-02553-w
Download citation
Received : 29 November 2022
Accepted : 03 May 2024
Published : 14 May 2024
DOI : https://doi.org/10.1186/s13643-024-02553-w
Share this article
Anyone you share the following link with will be able to read this content:
Sorry, a shareable link is not currently available for this article.
Provided by the Springer Nature SharedIt content-sharing initiative
Systematic Reviews
ISSN: 2046-4053
- Submission enquiries: Access here and click Contact Us
- General enquiries: [email protected]

IMAGES
VIDEO
COMMENTS
Therefore, in order to understand how HR has evolved, one must also understand how the concept of the organization has and continues to evolve in the 21. st. century. Albeit the terms and modern understandings of HR are relatively recent additions to the literature, the foundations of HR (at least in the U.S.) have four distinct historical eras.
This study reviews Human Resource Management (HRM) literature by adopting a hybrid research approach - bibliometric. analaysis and content analysis - on 1802 documents from the Scopus database ...
Strategic human resource management (SHRM) research increasingly focuses on the performance effects of human resource (HR) systems rather than individual HR practices (Combs, Liu, Hall, & Ketchen, 2006).Researchers tend to agree that the focus should be on systems because employees are simultaneously exposed to an interrelated set of HR practices rather than single practices one at a time, and ...
To achieve this objective, this study performs a systematic literature review and content analysis of 93 papers from 75 journals. The main results of the research show that digital trends resulting from Industry 4.0 affect the field of HRM in 13 different themes, promoting trends and challenges for HRM, the workforce, and organizations.
This study aimed to critically analyze studies that examine HRM's role in innovative companies through a systematic literature review (SLR), bibliographic coupling and cluster analysis techniques. The SLR process starts with the definition, objectives and conceptual limitations ( Kraus et al., 2020 ).
Human Resources in Project Management-a Critical Analysis of existing literature and evolving factors ... -A Literature Review,Mansoor Hussain and Mushtaq Ahmad International Journal of Economics ...
In this systematic review, we will examine the existing literature on the relationship between HR system and work ethics, with a particular focus on the role of ethical leadership in promoting work ethics in the workplace. In the last decade, several studies have been conducted on HR systems and work ethics.
Given the significance of human resources and people management in the current dynamic environment, ... The systematic literature review method proposed by Denyer and Tranfield (2009) has been adopted for this study. The 227 studies have been categorized according to themes and analysed to understand research trends, consequences, limitations ...
1. Employee Perceptions of HRM as an Antecedent, Mediator, or Outcome. Nishii and Wright (Citation 2008) developed the SHRM process framework to unravel the link between HRM and performance to shed light on the processes through which HR practices impact organizational performance (Jiang et al., Citation 2013).The starting point of the SHRM process model is the concept of variation.
Using a systematic literature review process, we deconstruct the concept of human resources analytics as presented in a vast although fragmented literature, and we identify 106 key research topics associated to three major areas, i.e. enablers of HR analytics (technological and organizational), applications (descriptive and diagnostic ...
Here, we review the literature on the application of AI to HRM in enterprise management and its related effects. "Data Source" section introduces the process of conducting the literature review, and "Methods" section describes the bibliometric analysis.Data Sources. Data were retrieved on April 14, 2022, from the Web of Science (WOS) (with SCI-E) database created by Clarivate Analytics (United ...
The objective of this review is to explore the theoretical and empirical findings of research work, which link human resource management (HRM) practices with organisational innovation. Innovation ...
Purpose: This study aims to review the existing literature on human resource management (HRM) as a major theme and sub-theme of human resource (HR) analytics. This chapter has the objective of analysing the trends in HR analytics. Design/Methodology/Approach: It covered the publications between 2010 and 2021.
The Purpose of a Literature Review is to gain an understanding of the existing research relevant to a particular topic or area of study and to present that knowledge in the form of a written report. Learning important concepts, and research methods will bring insights into the topic chosen. Ultimately, achieving a better understanding of a particular discipline and/or topic based upon the ...
This study aims to determine the dimensions of HR Practices and Policies contained in various previous literatures. Journal analysis was carried out using a systematic literature review (SLR) method obtained from Scopus in 2016-2021 following inclusion and exclusion criteria with the keywords HR Policies and Practices in
When examining the research methods of the publications, we found that the majority, namely 20 studies (55.6%), were quantitative by nature, followed by 11 (30.6%) qualitative studies. Among them, four (11%) were conceptual, and one (2.8%) was a mixed-method study that applied qualitative and quantitative methods.
In line with Danese et al. (2018), Nolan and Garavan (2016), and Wang and Chugh (2014), we adopted a structured and systematic literature review process as per the sequence of the stages described in Fig. 1.In line with the established practice of an SLR, multiple databases (eight) were searched for relevant articles. These include Scopus, Web of Science, Science Direct, Pro Quest, Google ...
Examples of literature reviews. Step 1 - Search for relevant literature. Step 2 - Evaluate and select sources. Step 3 - Identify themes, debates, and gaps. Step 4 - Outline your literature review's structure. Step 5 - Write your literature review.
Literature Review Conceptualizing HR Systems SHRM can be defined as "the pattern of planned HR deployments and activities intended to enable an organization to achieve its goals" (Wright & McMahan, 1992: 298). Increasingly, the field has emphasized the importance of focusing on whether and how "systems" or "bun-
2.0 LITERATURE REVIEW 2.1 LITERATURE REVIEW OF HUMAN RESOURCE MANAGEMENT Leavitt (1965) Proposes that an organization can change four things its task or purpose, its technology, its structure and its employees. Hostage (1975) believes that a service firm‟s contact personnel comprise the major determinants of service quality.
Journal analysis was carried out using a systematic literature review (SLR) method obtained from Scopus in 2016-2021 following inclusion and exclusion criteria with the keywords HR Policies and Practices in order to obtain 15 journals. ... S. P. (2021). Systematic literature review of project manager ' s leadership competencies. 28(1), 1-30 ...
HR questions that can be answered by HR analytics, 4) a new model for HR analytics, and 5) challenges that exist for HR analytics. A comprehensive literature review an alysis was done to achieve ...
The current paradigm of competency-based medical education and learner-centredness requires learners to take an active role in their training. However, deliberate and planned continual assessment and performance improvement is hindered by the fragmented nature of many medical training programs. Attempts to bridge this continuity gap between supervision and feedback through learner handover ...
The way scholarly knowledge and in particular literature reviews are communicated today rather resembles static, unstructured, pseudo-digitized articles, which are hardly processable by machines and AI. This demo showcases a novel way to create and publish scholarly literature reviews, also called semantic reviews.
This paper reviews the literature on the human resource challenges faced by MSMEs in India, especially in Pune city. It examines the factors affecting employee retention, performance, satisfaction ...