
An official website of the United States government
The .gov means it’s official. Federal government websites often end in .gov or .mil. Before sharing sensitive information, make sure you’re on a federal government site.
The site is secure. The https:// ensures that you are connecting to the official website and that any information you provide is encrypted and transmitted securely.
- Publications
- Account settings
Preview improvements coming to the PMC website in October 2024. Learn More or Try it out now .
- Advanced Search
- Journal List
- CBE Life Sci Educ
- v.21(3); Fall 2022

Literature Reviews, Theoretical Frameworks, and Conceptual Frameworks: An Introduction for New Biology Education Researchers
Julie a. luft.
† Department of Mathematics, Social Studies, and Science Education, Mary Frances Early College of Education, University of Georgia, Athens, GA 30602-7124
Sophia Jeong
‡ Department of Teaching & Learning, College of Education & Human Ecology, Ohio State University, Columbus, OH 43210
Robert Idsardi
§ Department of Biology, Eastern Washington University, Cheney, WA 99004
Grant Gardner
∥ Department of Biology, Middle Tennessee State University, Murfreesboro, TN 37132
Associated Data
To frame their work, biology education researchers need to consider the role of literature reviews, theoretical frameworks, and conceptual frameworks as critical elements of the research and writing process. However, these elements can be confusing for scholars new to education research. This Research Methods article is designed to provide an overview of each of these elements and delineate the purpose of each in the educational research process. We describe what biology education researchers should consider as they conduct literature reviews, identify theoretical frameworks, and construct conceptual frameworks. Clarifying these different components of educational research studies can be helpful to new biology education researchers and the biology education research community at large in situating their work in the broader scholarly literature.
INTRODUCTION
Discipline-based education research (DBER) involves the purposeful and situated study of teaching and learning in specific disciplinary areas ( Singer et al. , 2012 ). Studies in DBER are guided by research questions that reflect disciplines’ priorities and worldviews. Researchers can use quantitative data, qualitative data, or both to answer these research questions through a variety of methodological traditions. Across all methodologies, there are different methods associated with planning and conducting educational research studies that include the use of surveys, interviews, observations, artifacts, or instruments. Ensuring the coherence of these elements to the discipline’s perspective also involves situating the work in the broader scholarly literature. The tools for doing this include literature reviews, theoretical frameworks, and conceptual frameworks. However, the purpose and function of each of these elements is often confusing to new education researchers. The goal of this article is to introduce new biology education researchers to these three important elements important in DBER scholarship and the broader educational literature.
The first element we discuss is a review of research (literature reviews), which highlights the need for a specific research question, study problem, or topic of investigation. Literature reviews situate the relevance of the study within a topic and a field. The process may seem familiar to science researchers entering DBER fields, but new researchers may still struggle in conducting the review. Booth et al. (2016b) highlight some of the challenges novice education researchers face when conducting a review of literature. They point out that novice researchers struggle in deciding how to focus the review, determining the scope of articles needed in the review, and knowing how to be critical of the articles in the review. Overcoming these challenges (and others) can help novice researchers construct a sound literature review that can inform the design of the study and help ensure the work makes a contribution to the field.
The second and third highlighted elements are theoretical and conceptual frameworks. These guide biology education research (BER) studies, and may be less familiar to science researchers. These elements are important in shaping the construction of new knowledge. Theoretical frameworks offer a way to explain and interpret the studied phenomenon, while conceptual frameworks clarify assumptions about the studied phenomenon. Despite the importance of these constructs in educational research, biology educational researchers have noted the limited use of theoretical or conceptual frameworks in published work ( DeHaan, 2011 ; Dirks, 2011 ; Lo et al. , 2019 ). In reviewing articles published in CBE—Life Sciences Education ( LSE ) between 2015 and 2019, we found that fewer than 25% of the research articles had a theoretical or conceptual framework (see the Supplemental Information), and at times there was an inconsistent use of theoretical and conceptual frameworks. Clearly, these frameworks are challenging for published biology education researchers, which suggests the importance of providing some initial guidance to new biology education researchers.
Fortunately, educational researchers have increased their explicit use of these frameworks over time, and this is influencing educational research in science, technology, engineering, and mathematics (STEM) fields. For instance, a quick search for theoretical or conceptual frameworks in the abstracts of articles in Educational Research Complete (a common database for educational research) in STEM fields demonstrates a dramatic change over the last 20 years: from only 778 articles published between 2000 and 2010 to 5703 articles published between 2010 and 2020, a more than sevenfold increase. Greater recognition of the importance of these frameworks is contributing to DBER authors being more explicit about such frameworks in their studies.
Collectively, literature reviews, theoretical frameworks, and conceptual frameworks work to guide methodological decisions and the elucidation of important findings. Each offers a different perspective on the problem of study and is an essential element in all forms of educational research. As new researchers seek to learn about these elements, they will find different resources, a variety of perspectives, and many suggestions about the construction and use of these elements. The wide range of available information can overwhelm the new researcher who just wants to learn the distinction between these elements or how to craft them adequately.
Our goal in writing this paper is not to offer specific advice about how to write these sections in scholarly work. Instead, we wanted to introduce these elements to those who are new to BER and who are interested in better distinguishing one from the other. In this paper, we share the purpose of each element in BER scholarship, along with important points on its construction. We also provide references for additional resources that may be beneficial to better understanding each element. Table 1 summarizes the key distinctions among these elements.
Comparison of literature reviews, theoretical frameworks, and conceptual reviews
Literature reviews | Theoretical frameworks | Conceptual frameworks | |
---|---|---|---|
Purpose | To point out the need for the study in BER and connection to the field. | To state the assumptions and orientations of the researcher regarding the topic of study | To describe the researcher’s understanding of the main concepts under investigation |
Aims | A literature review examines current and relevant research associated with the study question. It is comprehensive, critical, and purposeful. | A theoretical framework illuminates the phenomenon of study and the corresponding assumptions adopted by the researcher. Frameworks can take on different orientations. | The conceptual framework is created by the researcher(s), includes the presumed relationships among concepts, and addresses needed areas of study discovered in literature reviews. |
Connection to the manuscript | A literature review should connect to the study question, guide the study methodology, and be central in the discussion by indicating how the analyzed data advances what is known in the field. | A theoretical framework drives the question, guides the types of methods for data collection and analysis, informs the discussion of the findings, and reveals the subjectivities of the researcher. | The conceptual framework is informed by literature reviews, experiences, or experiments. It may include emergent ideas that are not yet grounded in the literature. It should be coherent with the paper’s theoretical framing. |
Additional points | A literature review may reach beyond BER and include other education research fields. | A theoretical framework does not rationalize the need for the study, and a theoretical framework can come from different fields. | A conceptual framework articulates the phenomenon under study through written descriptions and/or visual representations. |
This article is written for the new biology education researcher who is just learning about these different elements or for scientists looking to become more involved in BER. It is a result of our own work as science education and biology education researchers, whether as graduate students and postdoctoral scholars or newly hired and established faculty members. This is the article we wish had been available as we started to learn about these elements or discussed them with new educational researchers in biology.
LITERATURE REVIEWS
Purpose of a literature review.
A literature review is foundational to any research study in education or science. In education, a well-conceptualized and well-executed review provides a summary of the research that has already been done on a specific topic and identifies questions that remain to be answered, thus illustrating the current research project’s potential contribution to the field and the reasoning behind the methodological approach selected for the study ( Maxwell, 2012 ). BER is an evolving disciplinary area that is redefining areas of conceptual emphasis as well as orientations toward teaching and learning (e.g., Labov et al. , 2010 ; American Association for the Advancement of Science, 2011 ; Nehm, 2019 ). As a result, building comprehensive, critical, purposeful, and concise literature reviews can be a challenge for new biology education researchers.
Building Literature Reviews
There are different ways to approach and construct a literature review. Booth et al. (2016a) provide an overview that includes, for example, scoping reviews, which are focused only on notable studies and use a basic method of analysis, and integrative reviews, which are the result of exhaustive literature searches across different genres. Underlying each of these different review processes are attention to the s earch process, a ppraisa l of articles, s ynthesis of the literature, and a nalysis: SALSA ( Booth et al. , 2016a ). This useful acronym can help the researcher focus on the process while building a specific type of review.
However, new educational researchers often have questions about literature reviews that are foundational to SALSA or other approaches. Common questions concern determining which literature pertains to the topic of study or the role of the literature review in the design of the study. This section addresses such questions broadly while providing general guidance for writing a narrative literature review that evaluates the most pertinent studies.
The literature review process should begin before the research is conducted. As Boote and Beile (2005 , p. 3) suggested, researchers should be “scholars before researchers.” They point out that having a good working knowledge of the proposed topic helps illuminate avenues of study. Some subject areas have a deep body of work to read and reflect upon, providing a strong foundation for developing the research question(s). For instance, the teaching and learning of evolution is an area of long-standing interest in the BER community, generating many studies (e.g., Perry et al. , 2008 ; Barnes and Brownell, 2016 ) and reviews of research (e.g., Sickel and Friedrichsen, 2013 ; Ziadie and Andrews, 2018 ). Emerging areas of BER include the affective domain, issues of transfer, and metacognition ( Singer et al. , 2012 ). Many studies in these areas are transdisciplinary and not always specific to biology education (e.g., Rodrigo-Peiris et al. , 2018 ; Kolpikova et al. , 2019 ). These newer areas may require reading outside BER; fortunately, summaries of some of these topics can be found in the Current Insights section of the LSE website.
In focusing on a specific problem within a broader research strand, a new researcher will likely need to examine research outside BER. Depending upon the area of study, the expanded reading list might involve a mix of BER, DBER, and educational research studies. Determining the scope of the reading is not always straightforward. A simple way to focus one’s reading is to create a “summary phrase” or “research nugget,” which is a very brief descriptive statement about the study. It should focus on the essence of the study, for example, “first-year nonmajor students’ understanding of evolution,” “metacognitive prompts to enhance learning during biochemistry,” or “instructors’ inquiry-based instructional practices after professional development programming.” This type of phrase should help a new researcher identify two or more areas to review that pertain to the study. Focusing on recent research in the last 5 years is a good first step. Additional studies can be identified by reading relevant works referenced in those articles. It is also important to read seminal studies that are more than 5 years old. Reading a range of studies should give the researcher the necessary command of the subject in order to suggest a research question.
Given that the research question(s) arise from the literature review, the review should also substantiate the selected methodological approach. The review and research question(s) guide the researcher in determining how to collect and analyze data. Often the methodological approach used in a study is selected to contribute knowledge that expands upon what has been published previously about the topic (see Institute of Education Sciences and National Science Foundation, 2013 ). An emerging topic of study may need an exploratory approach that allows for a description of the phenomenon and development of a potential theory. This could, but not necessarily, require a methodological approach that uses interviews, observations, surveys, or other instruments. An extensively studied topic may call for the additional understanding of specific factors or variables; this type of study would be well suited to a verification or a causal research design. These could entail a methodological approach that uses valid and reliable instruments, observations, or interviews to determine an effect in the studied event. In either of these examples, the researcher(s) may use a qualitative, quantitative, or mixed methods methodological approach.
Even with a good research question, there is still more reading to be done. The complexity and focus of the research question dictates the depth and breadth of the literature to be examined. Questions that connect multiple topics can require broad literature reviews. For instance, a study that explores the impact of a biology faculty learning community on the inquiry instruction of faculty could have the following review areas: learning communities among biology faculty, inquiry instruction among biology faculty, and inquiry instruction among biology faculty as a result of professional learning. Biology education researchers need to consider whether their literature review requires studies from different disciplines within or outside DBER. For the example given, it would be fruitful to look at research focused on learning communities with faculty in STEM fields or in general education fields that result in instructional change. It is important not to be too narrow or too broad when reading. When the conclusions of articles start to sound similar or no new insights are gained, the researcher likely has a good foundation for a literature review. This level of reading should allow the researcher to demonstrate a mastery in understanding the researched topic, explain the suitability of the proposed research approach, and point to the need for the refined research question(s).
The literature review should include the researcher’s evaluation and critique of the selected studies. A researcher may have a large collection of studies, but not all of the studies will follow standards important in the reporting of empirical work in the social sciences. The American Educational Research Association ( Duran et al. , 2006 ), for example, offers a general discussion about standards for such work: an adequate review of research informing the study, the existence of sound and appropriate data collection and analysis methods, and appropriate conclusions that do not overstep or underexplore the analyzed data. The Institute of Education Sciences and National Science Foundation (2013) also offer Common Guidelines for Education Research and Development that can be used to evaluate collected studies.
Because not all journals adhere to such standards, it is important that a researcher review each study to determine the quality of published research, per the guidelines suggested earlier. In some instances, the research may be fatally flawed. Examples of such flaws include data that do not pertain to the question, a lack of discussion about the data collection, poorly constructed instruments, or an inadequate analysis. These types of errors result in studies that are incomplete, error-laden, or inaccurate and should be excluded from the review. Most studies have limitations, and the author(s) often make them explicit. For instance, there may be an instructor effect, recognized bias in the analysis, or issues with the sample population. Limitations are usually addressed by the research team in some way to ensure a sound and acceptable research process. Occasionally, the limitations associated with the study can be significant and not addressed adequately, which leaves a consequential decision in the hands of the researcher. Providing critiques of studies in the literature review process gives the reader confidence that the researcher has carefully examined relevant work in preparation for the study and, ultimately, the manuscript.
A solid literature review clearly anchors the proposed study in the field and connects the research question(s), the methodological approach, and the discussion. Reviewing extant research leads to research questions that will contribute to what is known in the field. By summarizing what is known, the literature review points to what needs to be known, which in turn guides decisions about methodology. Finally, notable findings of the new study are discussed in reference to those described in the literature review.
Within published BER studies, literature reviews can be placed in different locations in an article. When included in the introductory section of the study, the first few paragraphs of the manuscript set the stage, with the literature review following the opening paragraphs. Cooper et al. (2019) illustrate this approach in their study of course-based undergraduate research experiences (CUREs). An introduction discussing the potential of CURES is followed by an analysis of the existing literature relevant to the design of CUREs that allows for novel student discoveries. Within this review, the authors point out contradictory findings among research on novel student discoveries. This clarifies the need for their study, which is described and highlighted through specific research aims.
A literature reviews can also make up a separate section in a paper. For example, the introduction to Todd et al. (2019) illustrates the need for their research topic by highlighting the potential of learning progressions (LPs) and suggesting that LPs may help mitigate learning loss in genetics. At the end of the introduction, the authors state their specific research questions. The review of literature following this opening section comprises two subsections. One focuses on learning loss in general and examines a variety of studies and meta-analyses from the disciplines of medical education, mathematics, and reading. The second section focuses specifically on LPs in genetics and highlights student learning in the midst of LPs. These separate reviews provide insights into the stated research question.
Suggestions and Advice
A well-conceptualized, comprehensive, and critical literature review reveals the understanding of the topic that the researcher brings to the study. Literature reviews should not be so big that there is no clear area of focus; nor should they be so narrow that no real research question arises. The task for a researcher is to craft an efficient literature review that offers a critical analysis of published work, articulates the need for the study, guides the methodological approach to the topic of study, and provides an adequate foundation for the discussion of the findings.
In our own writing of literature reviews, there are often many drafts. An early draft may seem well suited to the study because the need for and approach to the study are well described. However, as the results of the study are analyzed and findings begin to emerge, the existing literature review may be inadequate and need revision. The need for an expanded discussion about the research area can result in the inclusion of new studies that support the explanation of a potential finding. The literature review may also prove to be too broad. Refocusing on a specific area allows for more contemplation of a finding.
It should be noted that there are different types of literature reviews, and many books and articles have been written about the different ways to embark on these types of reviews. Among these different resources, the following may be helpful in considering how to refine the review process for scholarly journals:
- Booth, A., Sutton, A., & Papaioannou, D. (2016a). Systemic approaches to a successful literature review (2nd ed.). Los Angeles, CA: Sage. This book addresses different types of literature reviews and offers important suggestions pertaining to defining the scope of the literature review and assessing extant studies.
- Booth, W. C., Colomb, G. G., Williams, J. M., Bizup, J., & Fitzgerald, W. T. (2016b). The craft of research (4th ed.). Chicago: University of Chicago Press. This book can help the novice consider how to make the case for an area of study. While this book is not specifically about literature reviews, it offers suggestions about making the case for your study.
- Galvan, J. L., & Galvan, M. C. (2017). Writing literature reviews: A guide for students of the social and behavioral sciences (7th ed.). Routledge. This book offers guidance on writing different types of literature reviews. For the novice researcher, there are useful suggestions for creating coherent literature reviews.
THEORETICAL FRAMEWORKS
Purpose of theoretical frameworks.
As new education researchers may be less familiar with theoretical frameworks than with literature reviews, this discussion begins with an analogy. Envision a biologist, chemist, and physicist examining together the dramatic effect of a fog tsunami over the ocean. A biologist gazing at this phenomenon may be concerned with the effect of fog on various species. A chemist may be interested in the chemical composition of the fog as water vapor condenses around bits of salt. A physicist may be focused on the refraction of light to make fog appear to be “sitting” above the ocean. While observing the same “objective event,” the scientists are operating under different theoretical frameworks that provide a particular perspective or “lens” for the interpretation of the phenomenon. Each of these scientists brings specialized knowledge, experiences, and values to this phenomenon, and these influence the interpretation of the phenomenon. The scientists’ theoretical frameworks influence how they design and carry out their studies and interpret their data.
Within an educational study, a theoretical framework helps to explain a phenomenon through a particular lens and challenges and extends existing knowledge within the limitations of that lens. Theoretical frameworks are explicitly stated by an educational researcher in the paper’s framework, theory, or relevant literature section. The framework shapes the types of questions asked, guides the method by which data are collected and analyzed, and informs the discussion of the results of the study. It also reveals the researcher’s subjectivities, for example, values, social experience, and viewpoint ( Allen, 2017 ). It is essential that a novice researcher learn to explicitly state a theoretical framework, because all research questions are being asked from the researcher’s implicit or explicit assumptions of a phenomenon of interest ( Schwandt, 2000 ).
Selecting Theoretical Frameworks
Theoretical frameworks are one of the most contemplated elements in our work in educational research. In this section, we share three important considerations for new scholars selecting a theoretical framework.
The first step in identifying a theoretical framework involves reflecting on the phenomenon within the study and the assumptions aligned with the phenomenon. The phenomenon involves the studied event. There are many possibilities, for example, student learning, instructional approach, or group organization. A researcher holds assumptions about how the phenomenon will be effected, influenced, changed, or portrayed. It is ultimately the researcher’s assumption(s) about the phenomenon that aligns with a theoretical framework. An example can help illustrate how a researcher’s reflection on the phenomenon and acknowledgment of assumptions can result in the identification of a theoretical framework.
In our example, a biology education researcher may be interested in exploring how students’ learning of difficult biological concepts can be supported by the interactions of group members. The phenomenon of interest is the interactions among the peers, and the researcher assumes that more knowledgeable students are important in supporting the learning of the group. As a result, the researcher may draw on Vygotsky’s (1978) sociocultural theory of learning and development that is focused on the phenomenon of student learning in a social setting. This theory posits the critical nature of interactions among students and between students and teachers in the process of building knowledge. A researcher drawing upon this framework holds the assumption that learning is a dynamic social process involving questions and explanations among students in the classroom and that more knowledgeable peers play an important part in the process of building conceptual knowledge.
It is important to state at this point that there are many different theoretical frameworks. Some frameworks focus on learning and knowing, while other theoretical frameworks focus on equity, empowerment, or discourse. Some frameworks are well articulated, and others are still being refined. For a new researcher, it can be challenging to find a theoretical framework. Two of the best ways to look for theoretical frameworks is through published works that highlight different frameworks.
When a theoretical framework is selected, it should clearly connect to all parts of the study. The framework should augment the study by adding a perspective that provides greater insights into the phenomenon. It should clearly align with the studies described in the literature review. For instance, a framework focused on learning would correspond to research that reported different learning outcomes for similar studies. The methods for data collection and analysis should also correspond to the framework. For instance, a study about instructional interventions could use a theoretical framework concerned with learning and could collect data about the effect of the intervention on what is learned. When the data are analyzed, the theoretical framework should provide added meaning to the findings, and the findings should align with the theoretical framework.
A study by Jensen and Lawson (2011) provides an example of how a theoretical framework connects different parts of the study. They compared undergraduate biology students in heterogeneous and homogeneous groups over the course of a semester. Jensen and Lawson (2011) assumed that learning involved collaboration and more knowledgeable peers, which made Vygotsky’s (1978) theory a good fit for their study. They predicted that students in heterogeneous groups would experience greater improvement in their reasoning abilities and science achievements with much of the learning guided by the more knowledgeable peers.
In the enactment of the study, they collected data about the instruction in traditional and inquiry-oriented classes, while the students worked in homogeneous or heterogeneous groups. To determine the effect of working in groups, the authors also measured students’ reasoning abilities and achievement. Each data-collection and analysis decision connected to understanding the influence of collaborative work.
Their findings highlighted aspects of Vygotsky’s (1978) theory of learning. One finding, for instance, posited that inquiry instruction, as a whole, resulted in reasoning and achievement gains. This links to Vygotsky (1978) , because inquiry instruction involves interactions among group members. A more nuanced finding was that group composition had a conditional effect. Heterogeneous groups performed better with more traditional and didactic instruction, regardless of the reasoning ability of the group members. Homogeneous groups worked better during interaction-rich activities for students with low reasoning ability. The authors attributed the variation to the different types of helping behaviors of students. High-performing students provided the answers, while students with low reasoning ability had to work collectively through the material. In terms of Vygotsky (1978) , this finding provided new insights into the learning context in which productive interactions can occur for students.
Another consideration in the selection and use of a theoretical framework pertains to its orientation to the study. This can result in the theoretical framework prioritizing individuals, institutions, and/or policies ( Anfara and Mertz, 2014 ). Frameworks that connect to individuals, for instance, could contribute to understanding their actions, learning, or knowledge. Institutional frameworks, on the other hand, offer insights into how institutions, organizations, or groups can influence individuals or materials. Policy theories provide ways to understand how national or local policies can dictate an emphasis on outcomes or instructional design. These different types of frameworks highlight different aspects in an educational setting, which influences the design of the study and the collection of data. In addition, these different frameworks offer a way to make sense of the data. Aligning the data collection and analysis with the framework ensures that a study is coherent and can contribute to the field.
New understandings emerge when different theoretical frameworks are used. For instance, Ebert-May et al. (2015) prioritized the individual level within conceptual change theory (see Posner et al. , 1982 ). In this theory, an individual’s knowledge changes when it no longer fits the phenomenon. Ebert-May et al. (2015) designed a professional development program challenging biology postdoctoral scholars’ existing conceptions of teaching. The authors reported that the biology postdoctoral scholars’ teaching practices became more student-centered as they were challenged to explain their instructional decision making. According to the theory, the biology postdoctoral scholars’ dissatisfaction in their descriptions of teaching and learning initiated change in their knowledge and instruction. These results reveal how conceptual change theory can explain the learning of participants and guide the design of professional development programming.
The communities of practice (CoP) theoretical framework ( Lave, 1988 ; Wenger, 1998 ) prioritizes the institutional level , suggesting that learning occurs when individuals learn from and contribute to the communities in which they reside. Grounded in the assumption of community learning, the literature on CoP suggests that, as individuals interact regularly with the other members of their group, they learn about the rules, roles, and goals of the community ( Allee, 2000 ). A study conducted by Gehrke and Kezar (2017) used the CoP framework to understand organizational change by examining the involvement of individual faculty engaged in a cross-institutional CoP focused on changing the instructional practice of faculty at each institution. In the CoP, faculty members were involved in enhancing instructional materials within their department, which aligned with an overarching goal of instituting instruction that embraced active learning. Not surprisingly, Gehrke and Kezar (2017) revealed that faculty who perceived the community culture as important in their work cultivated institutional change. Furthermore, they found that institutional change was sustained when key leaders served as mentors and provided support for faculty, and as faculty themselves developed into leaders. This study reveals the complexity of individual roles in a COP in order to support institutional instructional change.
It is important to explicitly state the theoretical framework used in a study, but elucidating a theoretical framework can be challenging for a new educational researcher. The literature review can help to identify an applicable theoretical framework. Focal areas of the review or central terms often connect to assumptions and assertions associated with the framework that pertain to the phenomenon of interest. Another way to identify a theoretical framework is self-reflection by the researcher on personal beliefs and understandings about the nature of knowledge the researcher brings to the study ( Lysaght, 2011 ). In stating one’s beliefs and understandings related to the study (e.g., students construct their knowledge, instructional materials support learning), an orientation becomes evident that will suggest a particular theoretical framework. Theoretical frameworks are not arbitrary , but purposefully selected.
With experience, a researcher may find expanded roles for theoretical frameworks. Researchers may revise an existing framework that has limited explanatory power, or they may decide there is a need to develop a new theoretical framework. These frameworks can emerge from a current study or the need to explain a phenomenon in a new way. Researchers may also find that multiple theoretical frameworks are necessary to frame and explore a problem, as different frameworks can provide different insights into a problem.
Finally, it is important to recognize that choosing “x” theoretical framework does not necessarily mean a researcher chooses “y” methodology and so on, nor is there a clear-cut, linear process in selecting a theoretical framework for one’s study. In part, the nonlinear process of identifying a theoretical framework is what makes understanding and using theoretical frameworks challenging. For the novice scholar, contemplating and understanding theoretical frameworks is essential. Fortunately, there are articles and books that can help:
- Creswell, J. W. (2018). Research design: Qualitative, quantitative, and mixed methods approaches (5th ed.). Los Angeles, CA: Sage. This book provides an overview of theoretical frameworks in general educational research.
- Ding, L. (2019). Theoretical perspectives of quantitative physics education research. Physical Review Physics Education Research , 15 (2), 020101-1–020101-13. This paper illustrates how a DBER field can use theoretical frameworks.
- Nehm, R. (2019). Biology education research: Building integrative frameworks for teaching and learning about living systems. Disciplinary and Interdisciplinary Science Education Research , 1 , ar15. https://doi.org/10.1186/s43031-019-0017-6 . This paper articulates the need for studies in BER to explicitly state theoretical frameworks and provides examples of potential studies.
- Patton, M. Q. (2015). Qualitative research & evaluation methods: Integrating theory and practice . Sage. This book also provides an overview of theoretical frameworks, but for both research and evaluation.
CONCEPTUAL FRAMEWORKS
Purpose of a conceptual framework.
A conceptual framework is a description of the way a researcher understands the factors and/or variables that are involved in the study and their relationships to one another. The purpose of a conceptual framework is to articulate the concepts under study using relevant literature ( Rocco and Plakhotnik, 2009 ) and to clarify the presumed relationships among those concepts ( Rocco and Plakhotnik, 2009 ; Anfara and Mertz, 2014 ). Conceptual frameworks are different from theoretical frameworks in both their breadth and grounding in established findings. Whereas a theoretical framework articulates the lens through which a researcher views the work, the conceptual framework is often more mechanistic and malleable.
Conceptual frameworks are broader, encompassing both established theories (i.e., theoretical frameworks) and the researchers’ own emergent ideas. Emergent ideas, for example, may be rooted in informal and/or unpublished observations from experience. These emergent ideas would not be considered a “theory” if they are not yet tested, supported by systematically collected evidence, and peer reviewed. However, they do still play an important role in the way researchers approach their studies. The conceptual framework allows authors to clearly describe their emergent ideas so that connections among ideas in the study and the significance of the study are apparent to readers.
Constructing Conceptual Frameworks
Including a conceptual framework in a research study is important, but researchers often opt to include either a conceptual or a theoretical framework. Either may be adequate, but both provide greater insight into the research approach. For instance, a research team plans to test a novel component of an existing theory. In their study, they describe the existing theoretical framework that informs their work and then present their own conceptual framework. Within this conceptual framework, specific topics portray emergent ideas that are related to the theory. Describing both frameworks allows readers to better understand the researchers’ assumptions, orientations, and understanding of concepts being investigated. For example, Connolly et al. (2018) included a conceptual framework that described how they applied a theoretical framework of social cognitive career theory (SCCT) to their study on teaching programs for doctoral students. In their conceptual framework, the authors described SCCT, explained how it applied to the investigation, and drew upon results from previous studies to justify the proposed connections between the theory and their emergent ideas.
In some cases, authors may be able to sufficiently describe their conceptualization of the phenomenon under study in an introduction alone, without a separate conceptual framework section. However, incomplete descriptions of how the researchers conceptualize the components of the study may limit the significance of the study by making the research less intelligible to readers. This is especially problematic when studying topics in which researchers use the same terms for different constructs or different terms for similar and overlapping constructs (e.g., inquiry, teacher beliefs, pedagogical content knowledge, or active learning). Authors must describe their conceptualization of a construct if the research is to be understandable and useful.
There are some key areas to consider regarding the inclusion of a conceptual framework in a study. To begin with, it is important to recognize that conceptual frameworks are constructed by the researchers conducting the study ( Rocco and Plakhotnik, 2009 ; Maxwell, 2012 ). This is different from theoretical frameworks that are often taken from established literature. Researchers should bring together ideas from the literature, but they may be influenced by their own experiences as a student and/or instructor, the shared experiences of others, or thought experiments as they construct a description, model, or representation of their understanding of the phenomenon under study. This is an exercise in intellectual organization and clarity that often considers what is learned, known, and experienced. The conceptual framework makes these constructs explicitly visible to readers, who may have different understandings of the phenomenon based on their prior knowledge and experience. There is no single method to go about this intellectual work.
Reeves et al. (2016) is an example of an article that proposed a conceptual framework about graduate teaching assistant professional development evaluation and research. The authors used existing literature to create a novel framework that filled a gap in current research and practice related to the training of graduate teaching assistants. This conceptual framework can guide the systematic collection of data by other researchers because the framework describes the relationships among various factors that influence teaching and learning. The Reeves et al. (2016) conceptual framework may be modified as additional data are collected and analyzed by other researchers. This is not uncommon, as conceptual frameworks can serve as catalysts for concerted research efforts that systematically explore a phenomenon (e.g., Reynolds et al. , 2012 ; Brownell and Kloser, 2015 ).
Sabel et al. (2017) used a conceptual framework in their exploration of how scaffolds, an external factor, interact with internal factors to support student learning. Their conceptual framework integrated principles from two theoretical frameworks, self-regulated learning and metacognition, to illustrate how the research team conceptualized students’ use of scaffolds in their learning ( Figure 1 ). Sabel et al. (2017) created this model using their interpretations of these two frameworks in the context of their teaching.

Conceptual framework from Sabel et al. (2017) .
A conceptual framework should describe the relationship among components of the investigation ( Anfara and Mertz, 2014 ). These relationships should guide the researcher’s methods of approaching the study ( Miles et al. , 2014 ) and inform both the data to be collected and how those data should be analyzed. Explicitly describing the connections among the ideas allows the researcher to justify the importance of the study and the rigor of the research design. Just as importantly, these frameworks help readers understand why certain components of a system were not explored in the study. This is a challenge in education research, which is rooted in complex environments with many variables that are difficult to control.
For example, Sabel et al. (2017) stated: “Scaffolds, such as enhanced answer keys and reflection questions, can help students and instructors bridge the external and internal factors and support learning” (p. 3). They connected the scaffolds in the study to the three dimensions of metacognition and the eventual transformation of existing ideas into new or revised ideas. Their framework provides a rationale for focusing on how students use two different scaffolds, and not on other factors that may influence a student’s success (self-efficacy, use of active learning, exam format, etc.).
In constructing conceptual frameworks, researchers should address needed areas of study and/or contradictions discovered in literature reviews. By attending to these areas, researchers can strengthen their arguments for the importance of a study. For instance, conceptual frameworks can address how the current study will fill gaps in the research, resolve contradictions in existing literature, or suggest a new area of study. While a literature review describes what is known and not known about the phenomenon, the conceptual framework leverages these gaps in describing the current study ( Maxwell, 2012 ). In the example of Sabel et al. (2017) , the authors indicated there was a gap in the literature regarding how scaffolds engage students in metacognition to promote learning in large classes. Their study helps fill that gap by describing how scaffolds can support students in the three dimensions of metacognition: intelligibility, plausibility, and wide applicability. In another example, Lane (2016) integrated research from science identity, the ethic of care, the sense of belonging, and an expertise model of student success to form a conceptual framework that addressed the critiques of other frameworks. In a more recent example, Sbeglia et al. (2021) illustrated how a conceptual framework influences the methodological choices and inferences in studies by educational researchers.
Sometimes researchers draw upon the conceptual frameworks of other researchers. When a researcher’s conceptual framework closely aligns with an existing framework, the discussion may be brief. For example, Ghee et al. (2016) referred to portions of SCCT as their conceptual framework to explain the significance of their work on students’ self-efficacy and career interests. Because the authors’ conceptualization of this phenomenon aligned with a previously described framework, they briefly mentioned the conceptual framework and provided additional citations that provided more detail for the readers.
Within both the BER and the broader DBER communities, conceptual frameworks have been used to describe different constructs. For example, some researchers have used the term “conceptual framework” to describe students’ conceptual understandings of a biological phenomenon. This is distinct from a researcher’s conceptual framework of the educational phenomenon under investigation, which may also need to be explicitly described in the article. Other studies have presented a research logic model or flowchart of the research design as a conceptual framework. These constructions can be quite valuable in helping readers understand the data-collection and analysis process. However, a model depicting the study design does not serve the same role as a conceptual framework. Researchers need to avoid conflating these constructs by differentiating the researchers’ conceptual framework that guides the study from the research design, when applicable.
Explicitly describing conceptual frameworks is essential in depicting the focus of the study. We have found that being explicit in a conceptual framework means using accepted terminology, referencing prior work, and clearly noting connections between terms. This description can also highlight gaps in the literature or suggest potential contributions to the field of study. A well-elucidated conceptual framework can suggest additional studies that may be warranted. This can also spur other researchers to consider how they would approach the examination of a phenomenon and could result in a revised conceptual framework.
It can be challenging to create conceptual frameworks, but they are important. Below are two resources that could be helpful in constructing and presenting conceptual frameworks in educational research:
- Maxwell, J. A. (2012). Qualitative research design: An interactive approach (3rd ed.). Los Angeles, CA: Sage. Chapter 3 in this book describes how to construct conceptual frameworks.
- Ravitch, S. M., & Riggan, M. (2016). Reason & rigor: How conceptual frameworks guide research . Los Angeles, CA: Sage. This book explains how conceptual frameworks guide the research questions, data collection, data analyses, and interpretation of results.
CONCLUDING THOUGHTS
Literature reviews, theoretical frameworks, and conceptual frameworks are all important in DBER and BER. Robust literature reviews reinforce the importance of a study. Theoretical frameworks connect the study to the base of knowledge in educational theory and specify the researcher’s assumptions. Conceptual frameworks allow researchers to explicitly describe their conceptualization of the relationships among the components of the phenomenon under study. Table 1 provides a general overview of these components in order to assist biology education researchers in thinking about these elements.
It is important to emphasize that these different elements are intertwined. When these elements are aligned and complement one another, the study is coherent, and the study findings contribute to knowledge in the field. When literature reviews, theoretical frameworks, and conceptual frameworks are disconnected from one another, the study suffers. The point of the study is lost, suggested findings are unsupported, or important conclusions are invisible to the researcher. In addition, this misalignment may be costly in terms of time and money.
Conducting a literature review, selecting a theoretical framework, and building a conceptual framework are some of the most difficult elements of a research study. It takes time to understand the relevant research, identify a theoretical framework that provides important insights into the study, and formulate a conceptual framework that organizes the finding. In the research process, there is often a constant back and forth among these elements as the study evolves. With an ongoing refinement of the review of literature, clarification of the theoretical framework, and articulation of a conceptual framework, a sound study can emerge that makes a contribution to the field. This is the goal of BER and education research.
Supplementary Material
- Allee, V. (2000). Knowledge networks and communities of learning . OD Practitioner , 32 ( 4 ), 4–13. [ Google Scholar ]
- Allen, M. (2017). The Sage encyclopedia of communication research methods (Vols. 1–4 ). Los Angeles, CA: Sage. 10.4135/9781483381411 [ CrossRef ] [ Google Scholar ]
- American Association for the Advancement of Science. (2011). Vision and change in undergraduate biology education: A call to action . Washington, DC. [ Google Scholar ]
- Anfara, V. A., Mertz, N. T. (2014). Setting the stage . In Anfara, V. A., Mertz, N. T. (eds.), Theoretical frameworks in qualitative research (pp. 1–22). Sage. [ Google Scholar ]
- Barnes, M. E., Brownell, S. E. (2016). Practices and perspectives of college instructors on addressing religious beliefs when teaching evolution . CBE—Life Sciences Education , 15 ( 2 ), ar18. https://doi.org/10.1187/cbe.15-11-0243 [ PMC free article ] [ PubMed ] [ Google Scholar ]
- Boote, D. N., Beile, P. (2005). Scholars before researchers: On the centrality of the dissertation literature review in research preparation . Educational Researcher , 34 ( 6 ), 3–15. 10.3102/0013189x034006003 [ CrossRef ] [ Google Scholar ]
- Booth, A., Sutton, A., Papaioannou, D. (2016a). Systemic approaches to a successful literature review (2nd ed.). Los Angeles, CA: Sage. [ Google Scholar ]
- Booth, W. C., Colomb, G. G., Williams, J. M., Bizup, J., Fitzgerald, W. T. (2016b). The craft of research (4th ed.). Chicago, IL: University of Chicago Press. [ Google Scholar ]
- Brownell, S. E., Kloser, M. J. (2015). Toward a conceptual framework for measuring the effectiveness of course-based undergraduate research experiences in undergraduate biology . Studies in Higher Education , 40 ( 3 ), 525–544. https://doi.org/10.1080/03075079.2015.1004234 [ Google Scholar ]
- Connolly, M. R., Lee, Y. G., Savoy, J. N. (2018). The effects of doctoral teaching development on early-career STEM scholars’ college teaching self-efficacy . CBE—Life Sciences Education , 17 ( 1 ), ar14. https://doi.org/10.1187/cbe.17-02-0039 [ PMC free article ] [ PubMed ] [ Google Scholar ]
- Cooper, K. M., Blattman, J. N., Hendrix, T., Brownell, S. E. (2019). The impact of broadly relevant novel discoveries on student project ownership in a traditional lab course turned CURE . CBE—Life Sciences Education , 18 ( 4 ), ar57. https://doi.org/10.1187/cbe.19-06-0113 [ PMC free article ] [ PubMed ] [ Google Scholar ]
- Creswell, J. W. (2018). Research design: Qualitative, quantitative, and mixed methods approaches (5th ed.). Los Angeles, CA: Sage. [ Google Scholar ]
- DeHaan, R. L. (2011). Education research in the biological sciences: A nine decade review (Paper commissioned by the NAS/NRC Committee on the Status, Contributions, and Future Directions of Discipline Based Education Research) . Washington, DC: National Academies Press. Retrieved May 20, 2022, from www7.nationalacademies.org/bose/DBER_Mee ting2_commissioned_papers_page.html [ Google Scholar ]
- Ding, L. (2019). Theoretical perspectives of quantitative physics education research . Physical Review Physics Education Research , 15 ( 2 ), 020101. [ Google Scholar ]
- Dirks, C. (2011). The current status and future direction of biology education research . Paper presented at: Second Committee Meeting on the Status, Contributions, and Future Directions of Discipline-Based Education Research, 18–19 October (Washington, DC). Retrieved May 20, 2022, from http://sites.nationalacademies.org/DBASSE/BOSE/DBASSE_071087 [ Google Scholar ]
- Duran, R. P., Eisenhart, M. A., Erickson, F. D., Grant, C. A., Green, J. L., Hedges, L. V., Schneider, B. L. (2006). Standards for reporting on empirical social science research in AERA publications: American Educational Research Association . Educational Researcher , 35 ( 6 ), 33–40. [ Google Scholar ]
- Ebert-May, D., Derting, T. L., Henkel, T. P., Middlemis Maher, J., Momsen, J. L., Arnold, B., Passmore, H. A. (2015). Breaking the cycle: Future faculty begin teaching with learner-centered strategies after professional development . CBE—Life Sciences Education , 14 ( 2 ), ar22. https://doi.org/10.1187/cbe.14-12-0222 [ PMC free article ] [ PubMed ] [ Google Scholar ]
- Galvan, J. L., Galvan, M. C. (2017). Writing literature reviews: A guide for students of the social and behavioral sciences (7th ed.). New York, NY: Routledge. https://doi.org/10.4324/9781315229386 [ Google Scholar ]
- Gehrke, S., Kezar, A. (2017). The roles of STEM faculty communities of practice in institutional and departmental reform in higher education . American Educational Research Journal , 54 ( 5 ), 803–833. https://doi.org/10.3102/0002831217706736 [ Google Scholar ]
- Ghee, M., Keels, M., Collins, D., Neal-Spence, C., Baker, E. (2016). Fine-tuning summer research programs to promote underrepresented students’ persistence in the STEM pathway . CBE—Life Sciences Education , 15 ( 3 ), ar28. https://doi.org/10.1187/cbe.16-01-0046 [ PMC free article ] [ PubMed ] [ Google Scholar ]
- Institute of Education Sciences & National Science Foundation. (2013). Common guidelines for education research and development . Retrieved May 20, 2022, from www.nsf.gov/pubs/2013/nsf13126/nsf13126.pdf
- Jensen, J. L., Lawson, A. (2011). Effects of collaborative group composition and inquiry instruction on reasoning gains and achievement in undergraduate biology . CBE—Life Sciences Education , 10 ( 1 ), 64–73. https://doi.org/10.1187/cbe.19-05-0098 [ PMC free article ] [ PubMed ] [ Google Scholar ]
- Kolpikova, E. P., Chen, D. C., Doherty, J. H. (2019). Does the format of preclass reading quizzes matter? An evaluation of traditional and gamified, adaptive preclass reading quizzes . CBE—Life Sciences Education , 18 ( 4 ), ar52. https://doi.org/10.1187/cbe.19-05-0098 [ PMC free article ] [ PubMed ] [ Google Scholar ]
- Labov, J. B., Reid, A. H., Yamamoto, K. R. (2010). Integrated biology and undergraduate science education: A new biology education for the twenty-first century? CBE—Life Sciences Education , 9 ( 1 ), 10–16. https://doi.org/10.1187/cbe.09-12-0092 [ PMC free article ] [ PubMed ] [ Google Scholar ]
- Lane, T. B. (2016). Beyond academic and social integration: Understanding the impact of a STEM enrichment program on the retention and degree attainment of underrepresented students . CBE—Life Sciences Education , 15 ( 3 ), ar39. https://doi.org/10.1187/cbe.16-01-0070 [ PMC free article ] [ PubMed ] [ Google Scholar ]
- Lave, J. (1988). Cognition in practice: Mind, mathematics and culture in everyday life . New York, NY: Cambridge University Press. [ Google Scholar ]
- Lo, S. M., Gardner, G. E., Reid, J., Napoleon-Fanis, V., Carroll, P., Smith, E., Sato, B. K. (2019). Prevailing questions and methodologies in biology education research: A longitudinal analysis of research in CBE — Life Sciences Education and at the Society for the Advancement of Biology Education Research . CBE—Life Sciences Education , 18 ( 1 ), ar9. https://doi.org/10.1187/cbe.18-08-0164 [ PMC free article ] [ PubMed ] [ Google Scholar ]
- Lysaght, Z. (2011). Epistemological and paradigmatic ecumenism in “Pasteur’s quadrant:” Tales from doctoral research . In Official Conference Proceedings of the Third Asian Conference on Education in Osaka, Japan . Retrieved May 20, 2022, from http://iafor.org/ace2011_offprint/ACE2011_offprint_0254.pdf
- Maxwell, J. A. (2012). Qualitative research design: An interactive approach (3rd ed.). Los Angeles, CA: Sage. [ Google Scholar ]
- Miles, M. B., Huberman, A. M., Saldaña, J. (2014). Qualitative data analysis (3rd ed.). Los Angeles, CA: Sage. [ Google Scholar ]
- Nehm, R. (2019). Biology education research: Building integrative frameworks for teaching and learning about living systems . Disciplinary and Interdisciplinary Science Education Research , 1 , ar15. https://doi.org/10.1186/s43031-019-0017-6 [ Google Scholar ]
- Patton, M. Q. (2015). Qualitative research & evaluation methods: Integrating theory and practice . Los Angeles, CA: Sage. [ Google Scholar ]
- Perry, J., Meir, E., Herron, J. C., Maruca, S., Stal, D. (2008). Evaluating two approaches to helping college students understand evolutionary trees through diagramming tasks . CBE—Life Sciences Education , 7 ( 2 ), 193–201. https://doi.org/10.1187/cbe.07-01-0007 [ PMC free article ] [ PubMed ] [ Google Scholar ]
- Posner, G. J., Strike, K. A., Hewson, P. W., Gertzog, W. A. (1982). Accommodation of a scientific conception: Toward a theory of conceptual change . Science Education , 66 ( 2 ), 211–227. [ Google Scholar ]
- Ravitch, S. M., Riggan, M. (2016). Reason & rigor: How conceptual frameworks guide research . Los Angeles, CA: Sage. [ Google Scholar ]
- Reeves, T. D., Marbach-Ad, G., Miller, K. R., Ridgway, J., Gardner, G. E., Schussler, E. E., Wischusen, E. W. (2016). A conceptual framework for graduate teaching assistant professional development evaluation and research . CBE—Life Sciences Education , 15 ( 2 ), es2. https://doi.org/10.1187/cbe.15-10-0225 [ PMC free article ] [ PubMed ] [ Google Scholar ]
- Reynolds, J. A., Thaiss, C., Katkin, W., Thompson, R. J. Jr. (2012). Writing-to-learn in undergraduate science education: A community-based, conceptually driven approach . CBE—Life Sciences Education , 11 ( 1 ), 17–25. https://doi.org/10.1187/cbe.11-08-0064 [ PMC free article ] [ PubMed ] [ Google Scholar ]
- Rocco, T. S., Plakhotnik, M. S. (2009). Literature reviews, conceptual frameworks, and theoretical frameworks: Terms, functions, and distinctions . Human Resource Development Review , 8 ( 1 ), 120–130. https://doi.org/10.1177/1534484309332617 [ Google Scholar ]
- Rodrigo-Peiris, T., Xiang, L., Cassone, V. M. (2018). A low-intensity, hybrid design between a “traditional” and a “course-based” research experience yields positive outcomes for science undergraduate freshmen and shows potential for large-scale application . CBE—Life Sciences Education , 17 ( 4 ), ar53. https://doi.org/10.1187/cbe.17-11-0248 [ PMC free article ] [ PubMed ] [ Google Scholar ]
- Sabel, J. L., Dauer, J. T., Forbes, C. T. (2017). Introductory biology students’ use of enhanced answer keys and reflection questions to engage in metacognition and enhance understanding . CBE—Life Sciences Education , 16 ( 3 ), ar40. https://doi.org/10.1187/cbe.16-10-0298 [ PMC free article ] [ PubMed ] [ Google Scholar ]
- Sbeglia, G. C., Goodridge, J. A., Gordon, L. H., Nehm, R. H. (2021). Are faculty changing? How reform frameworks, sampling intensities, and instrument measures impact inferences about student-centered teaching practices . CBE—Life Sciences Education , 20 ( 3 ), ar39. https://doi.org/10.1187/cbe.20-11-0259 [ PMC free article ] [ PubMed ] [ Google Scholar ]
- Schwandt, T. A. (2000). Three epistemological stances for qualitative inquiry: Interpretivism, hermeneutics, and social constructionism . In Denzin, N. K., Lincoln, Y. S. (Eds.), Handbook of qualitative research (2nd ed., pp. 189–213). Los Angeles, CA: Sage. [ Google Scholar ]
- Sickel, A. J., Friedrichsen, P. (2013). Examining the evolution education literature with a focus on teachers: Major findings, goals for teacher preparation, and directions for future research . Evolution: Education and Outreach , 6 ( 1 ), 23. https://doi.org/10.1186/1936-6434-6-23 [ Google Scholar ]
- Singer, S. R., Nielsen, N. R., Schweingruber, H. A. (2012). Discipline-based education research: Understanding and improving learning in undergraduate science and engineering . Washington, DC: National Academies Press. [ Google Scholar ]
- Todd, A., Romine, W. L., Correa-Menendez, J. (2019). Modeling the transition from a phenotypic to genotypic conceptualization of genetics in a university-level introductory biology context . Research in Science Education , 49 ( 2 ), 569–589. https://doi.org/10.1007/s11165-017-9626-2 [ Google Scholar ]
- Vygotsky, L. S. (1978). Mind in society: The development of higher psychological processes . Cambridge, MA: Harvard University Press. [ Google Scholar ]
- Wenger, E. (1998). Communities of practice: Learning as a social system . Systems Thinker , 9 ( 5 ), 2–3. [ Google Scholar ]
- Ziadie, M. A., Andrews, T. C. (2018). Moving evolution education forward: A systematic analysis of literature to identify gaps in collective knowledge for teaching . CBE—Life Sciences Education , 17 ( 1 ), ar11. https://doi.org/10.1187/cbe.17-08-0190 [ PMC free article ] [ PubMed ] [ Google Scholar ]
- Resources Home 🏠
- Try SciSpace Copilot
- Search research papers
- Add Copilot Extension
- Try AI Detector
- Try Paraphraser
- Try Citation Generator
- April Papers
- June Papers
- July Papers

Literature Review and Theoretical Framework: Understanding the Differences
Table of Contents
A literature review and a theoretical framework are both important components of academic research. However, they serve different purposes and have distinct characteristics. In this article, we will examine the concepts of literature review and theoretical framework, explore their significance, and highlight the key differences between the two.
Defining the Concepts: Literature Review and Theoretical Framework
Before we dive into the details, let's clarify what a literature review and a theoretical framework actually mean.
What is a Literature Review?
A literature review is a critical analysis and synthesis of existing research and scholarly articles on a specific topic. It involves reviewing and summarizing the current knowledge and understanding of the subject matter. By examining previous studies, the scholar can identify knowledge gaps, assess the strengths and weaknesses of existing research, and present a comprehensive overview of the topic.
When conducting a literature review, the scholar delves into a vast array of sources, including academic journals, books, conference proceedings, and reputable online databases. This extensive exploration allows them to gather relevant information, theories, and methodologies related to their research topic.
Furthermore, a literature review provides a solid foundation for the research by establishing the context and significance of the study. It helps researchers identify the key concepts, theories, and variables that are relevant to their research objectives. By critically analyzing the existing literature, scholars can identify research gaps and propose new avenues for scientific investigation.
Moreover, a literature review is not merely a summary of previous studies. It requires a critical evaluation of the methodologies used, the quality of the data collected, and the validity of the conclusions drawn.
Researchers must assess the credibility and reliability of the sources they include in their review to ensure the accuracy and robustness of their analysis.
What is a Theoretical Framework?
A theoretical framework provides a conceptual explanation for the research problem or question being investigated. It serves as a foundation that guides the formulation of hypotheses and research objectives. A theoretical framework helps researchers to analyze and interpret their findings by establishing a set of assumptions, concepts, and relationships that underpin their study. It provides a structured framework for organizing and presenting research outcomes.
When developing a theoretical framework, researchers draw upon existing theories and concepts from relevant disciplines to create a conceptual framework that aligns with their research objectives. This framework helps researchers to define the variables they will study, establish the relationships between these variables, and propose hypotheses that can be tested through empirical research.
Furthermore, a theoretical framework provides a roadmap for researchers to navigate through the complexities of their study. It helps them to identify the key constructs and variables that need to be measured and analyzed. By providing a clear structure, the theoretical framework ensures that researchers stay focused on their research objectives and avoid getting lost in a sea of information.
Moreover, a theoretical framework allows researchers to make connections between their study and existing theories or models. By building upon established knowledge, researchers can contribute to the advancement of their field and provide new insights and perspectives. The theoretical framework also helps researchers interpret their findings in a meaningful way and draw conclusions that have theoretical and practical implications.
In summary, both a literature review and a theoretical framework play crucial roles in the research process. While a literature review provides a comprehensive overview of existing knowledge and identifies research gaps, a theoretical framework establishes the conceptual foundation for the study and guides the formulation of research objectives and hypotheses. Together, these two elements contribute to the development of a robust and well-grounded research study.
The Purpose and Importance of Literature Reviews
Now that we have a clear understanding of what a literature review is, let's explore its purpose and significance.
A literature review plays a crucial role in academic research. It serves several purposes, including:
- Providing a comprehensive understanding of the existing literature in a particular field.
- Identifying the gaps, controversies, or inconsistencies in the current knowledge.
- Helping researchers to refine their research questions and objectives.
- Ensuring that the research being conducted is novel and contributes to the existing body of knowledge.
The Benefits of Conducting a Literature Review
There are numerous benefits to conducting a literature review, such as:
- Enhancing the researcher's knowledge and understanding of the subject area.
- Providing a framework for developing research hypotheses and objectives.
- Identifying potential research methodologies and approaches.
- Informing the selection of appropriate data collection and analysis methods.
- Guiding the interpretation and discussion of research findings.
The Purpose and Importance of Theoretical Frameworks
Moving on to theoretical frameworks, let us discuss their purpose and importance.
When conducting research, theoretical frameworks play a crucial role in providing a solid foundation for the study. They serve as a guiding tool for researchers, helping them navigate through the complexities of their research and providing a framework for understanding and interpreting their findings.
The Function of Theoretical Frameworks in Research
Theoretical frameworks serve multiple functions in research:
- Providing a conceptual framework enables researchers to clearly define the scope and direction of their study.
- Acting as a roadmap, guiding researchers in formulating their research objectives and hypotheses. It helps them identify the key variables and relationships they want to explore, providing a solid foundation for their research.
- Helping researchers identify and select appropriate research methods and techniques. When it comes to selecting research methods and techniques, theoretical frameworks are invaluable. They provide researchers with a lens through which they can evaluate different methods and techniques, ensuring that they choose the most appropriate ones for their study. By aligning their methods with the theoretical framework, researchers can enhance the validity and reliability of their research.
- Supporting the interpretation and explanation of research findings. Once the data has been collected, theoretical frameworks help researchers make sense of their findings. They provide a framework for interpreting and explaining the results, allowing researchers to draw meaningful conclusions. By grounding their analysis in a theoretical framework, researchers can provide a solid foundation for their findings and contribute to the existing body of knowledge.
- Facilitating the integration of new knowledge with existing theories and concepts. Theoretical frameworks also play a crucial role in the advancement of knowledge. By integrating new findings with existing theories and concepts, researchers can contribute to the development of their field.
The Advantages of Developing a Theoretical Framework
Developing a theoretical framework offers several advantages:
- Enhancing the researcher's understanding of the research problem. By developing a theoretical framework, researchers gain a deeper understanding of the research problem they are investigating. This enhanced understanding allows researchers to approach their study with clarity and purpose.
- Facilitating the selection of an appropriate research design. Choosing the right research design is crucial for the success of a study. A well-developed theoretical framework helps researchers select the most appropriate research design by providing a clear direction and focus. It ensures that the research design aligns with the research objectives and hypotheses, maximizing the chances of obtaining valid and reliable results.
- Helping researchers organize their thoughts and ideas systematically. This organization helps researchers stay focused and ensures that all aspects of the research problem are considered. By structuring their thoughts, researchers can effectively communicate their ideas and findings to others.
- Guiding the analysis and interpretation of research findings. When it comes to analyzing and interpreting research findings, a theoretical framework provides researchers with a framework to guide their process. It helps researchers identify patterns, relationships, and themes within the data, allowing for a more comprehensive analysis.
Developing a theoretical framework is essential for ensuring the validity and reliability of a study. By aligning the research with established theories and concepts, researchers can enhance the credibility of their study. A well-developed theoretical framework provides a solid foundation for the research, increasing the chances of obtaining accurate and meaningful results.
Differences Between Literature Reviews and Theoretical Frameworks
Now, let's explore the key differences between literature reviews and theoretical frameworks.
Key Differences:
- Focus: A literature review focuses on summarizing existing research, while a theoretical framework focuses on providing a conceptual foundation for the study.
- Scope: A literature review covers a broad range of related research, while a theoretical framework is more specific to the research problem at hand.
- Timing: A literature review is typically conducted early in the research process, while a theoretical framework is often developed alongside the research design.
- Purpose: A literature review aims to inform the research and establish its context, while a theoretical framework aims to guide the interpretation and analysis of findings.
In conclusion
Understanding the distinction between a literature review and a theoretical framework is crucial for conducting effective and meaningful academic research. While a literature review provides an overview of existing research, a theoretical framework guides the formulation, analysis, and interpretation of research. Both components are essential for building a strong foundation of knowledge in any field. By comprehending their purpose, significance, and key differences, researchers can enhance the quality and rigor of their research endeavors.
Love using SciSpace tools? Enjoy discounts! Use SR40 (40% off yearly) and SR20 (20% off monthly). Claim yours here 👉 SciSpace Premium
Learn more about Literature Review
5 literature review tools to ace your reseach (+2 bonus tools)
Role of AI in Systematic Literature Review
Evaluating literature review: systematic vs. scoping reviews
A complete guide on how to write a literature review
How to Use AI Tools for Conducting a Literature Review
You might also like

Boosting Citations: A Comparative Analysis of Graphical Abstract vs. Video Abstract
The Impact of Visual Abstracts on Boosting Citations

Introducing SciSpace’s Citation Booster To Increase Research Visibility
Have a language expert improve your writing
Run a free plagiarism check in 10 minutes, automatically generate references for free.
- Knowledge Base
- Dissertation
- What is a Literature Review? | Guide, Template, & Examples
What is a Literature Review? | Guide, Template, & Examples
Published on 22 February 2022 by Shona McCombes . Revised on 7 June 2022.
What is a literature review? A literature review is a survey of scholarly sources on a specific topic. It provides an overview of current knowledge, allowing you to identify relevant theories, methods, and gaps in the existing research.
There are five key steps to writing a literature review:
- Search for relevant literature
- Evaluate sources
- Identify themes, debates and gaps
- Outline the structure
- Write your literature review
A good literature review doesn’t just summarise sources – it analyses, synthesises, and critically evaluates to give a clear picture of the state of knowledge on the subject.
Instantly correct all language mistakes in your text
Be assured that you'll submit flawless writing. Upload your document to correct all your mistakes.
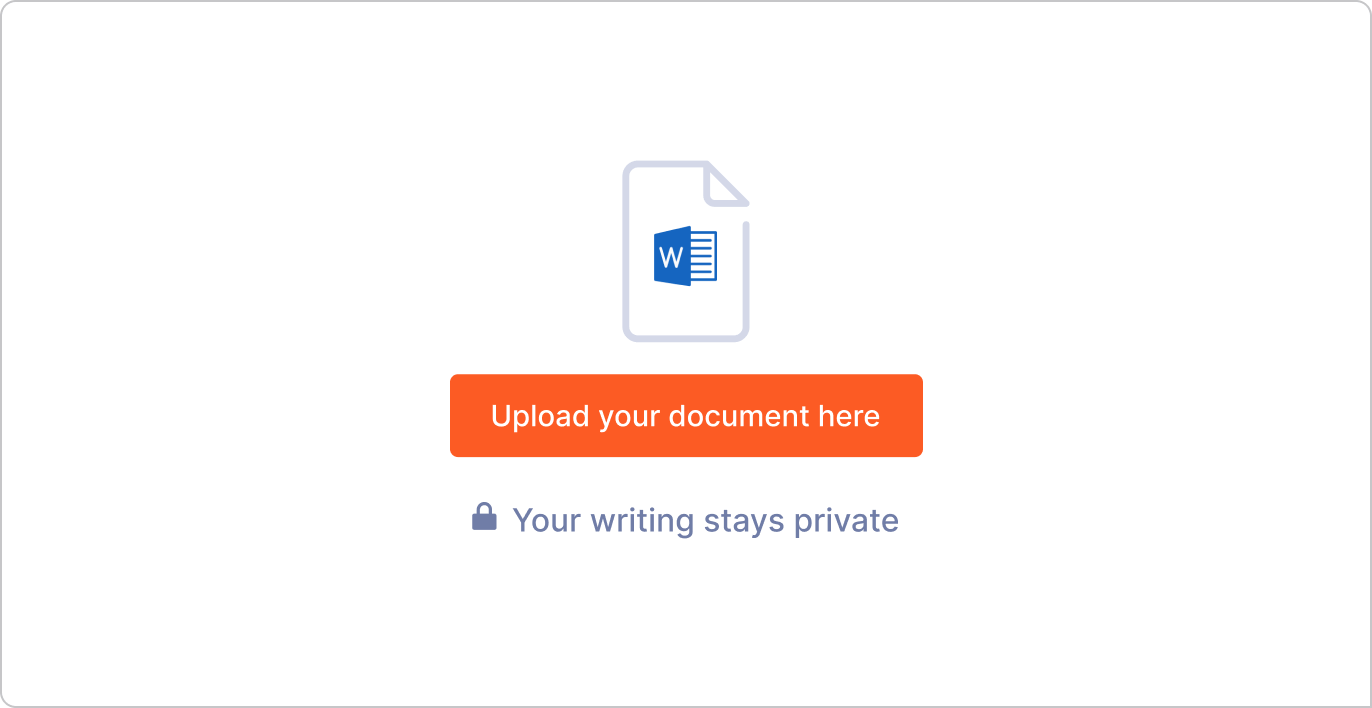
Table of contents
Why write a literature review, examples of literature reviews, step 1: search for relevant literature, step 2: evaluate and select sources, step 3: identify themes, debates and gaps, step 4: outline your literature review’s structure, step 5: write your literature review, frequently asked questions about literature reviews, introduction.
- Quick Run-through
- Step 1 & 2
When you write a dissertation or thesis, you will have to conduct a literature review to situate your research within existing knowledge. The literature review gives you a chance to:
- Demonstrate your familiarity with the topic and scholarly context
- Develop a theoretical framework and methodology for your research
- Position yourself in relation to other researchers and theorists
- Show how your dissertation addresses a gap or contributes to a debate
You might also have to write a literature review as a stand-alone assignment. In this case, the purpose is to evaluate the current state of research and demonstrate your knowledge of scholarly debates around a topic.
The content will look slightly different in each case, but the process of conducting a literature review follows the same steps. We’ve written a step-by-step guide that you can follow below.
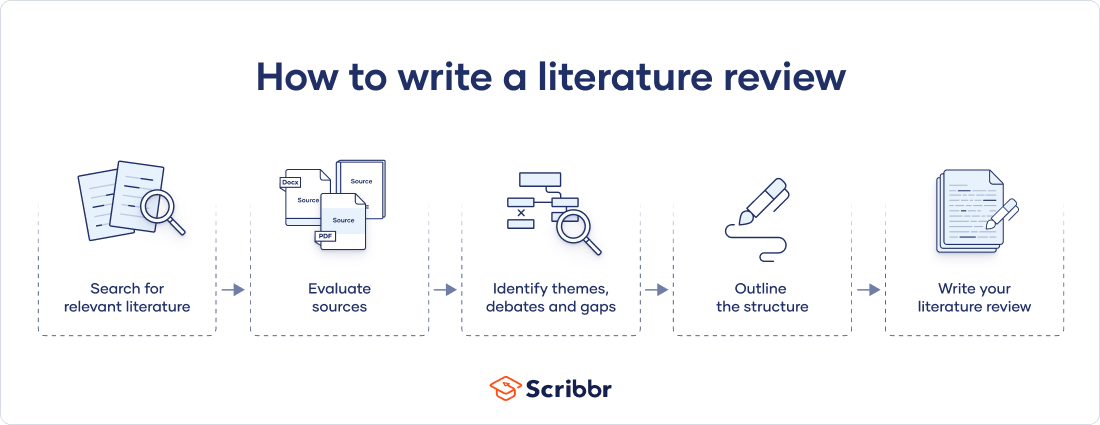
Prevent plagiarism, run a free check.
Writing literature reviews can be quite challenging! A good starting point could be to look at some examples, depending on what kind of literature review you’d like to write.
- Example literature review #1: “Why Do People Migrate? A Review of the Theoretical Literature” ( Theoretical literature review about the development of economic migration theory from the 1950s to today.)
- Example literature review #2: “Literature review as a research methodology: An overview and guidelines” ( Methodological literature review about interdisciplinary knowledge acquisition and production.)
- Example literature review #3: “The Use of Technology in English Language Learning: A Literature Review” ( Thematic literature review about the effects of technology on language acquisition.)
- Example literature review #4: “Learners’ Listening Comprehension Difficulties in English Language Learning: A Literature Review” ( Chronological literature review about how the concept of listening skills has changed over time.)
You can also check out our templates with literature review examples and sample outlines at the links below.
Download Word doc Download Google doc
Before you begin searching for literature, you need a clearly defined topic .
If you are writing the literature review section of a dissertation or research paper, you will search for literature related to your research objectives and questions .
If you are writing a literature review as a stand-alone assignment, you will have to choose a focus and develop a central question to direct your search. Unlike a dissertation research question, this question has to be answerable without collecting original data. You should be able to answer it based only on a review of existing publications.
Make a list of keywords
Start by creating a list of keywords related to your research topic. Include each of the key concepts or variables you’re interested in, and list any synonyms and related terms. You can add to this list if you discover new keywords in the process of your literature search.
- Social media, Facebook, Instagram, Twitter, Snapchat, TikTok
- Body image, self-perception, self-esteem, mental health
- Generation Z, teenagers, adolescents, youth
Search for relevant sources
Use your keywords to begin searching for sources. Some databases to search for journals and articles include:
- Your university’s library catalogue
- Google Scholar
- Project Muse (humanities and social sciences)
- Medline (life sciences and biomedicine)
- EconLit (economics)
- Inspec (physics, engineering and computer science)
You can use boolean operators to help narrow down your search:
Read the abstract to find out whether an article is relevant to your question. When you find a useful book or article, you can check the bibliography to find other relevant sources.
To identify the most important publications on your topic, take note of recurring citations. If the same authors, books or articles keep appearing in your reading, make sure to seek them out.
You probably won’t be able to read absolutely everything that has been written on the topic – you’ll have to evaluate which sources are most relevant to your questions.
For each publication, ask yourself:
- What question or problem is the author addressing?
- What are the key concepts and how are they defined?
- What are the key theories, models and methods? Does the research use established frameworks or take an innovative approach?
- What are the results and conclusions of the study?
- How does the publication relate to other literature in the field? Does it confirm, add to, or challenge established knowledge?
- How does the publication contribute to your understanding of the topic? What are its key insights and arguments?
- What are the strengths and weaknesses of the research?
Make sure the sources you use are credible, and make sure you read any landmark studies and major theories in your field of research.
You can find out how many times an article has been cited on Google Scholar – a high citation count means the article has been influential in the field, and should certainly be included in your literature review.
The scope of your review will depend on your topic and discipline: in the sciences you usually only review recent literature, but in the humanities you might take a long historical perspective (for example, to trace how a concept has changed in meaning over time).
Remember that you can use our template to summarise and evaluate sources you’re thinking about using!
Take notes and cite your sources
As you read, you should also begin the writing process. Take notes that you can later incorporate into the text of your literature review.
It’s important to keep track of your sources with references to avoid plagiarism . It can be helpful to make an annotated bibliography, where you compile full reference information and write a paragraph of summary and analysis for each source. This helps you remember what you read and saves time later in the process.
You can use our free APA Reference Generator for quick, correct, consistent citations.
The only proofreading tool specialized in correcting academic writing
The academic proofreading tool has been trained on 1000s of academic texts and by native English editors. Making it the most accurate and reliable proofreading tool for students.
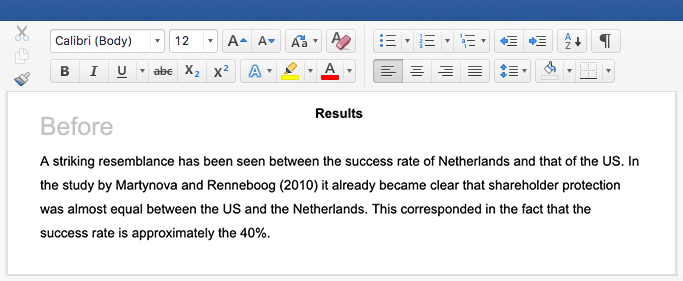
Correct my document today
To begin organising your literature review’s argument and structure, you need to understand the connections and relationships between the sources you’ve read. Based on your reading and notes, you can look for:
- Trends and patterns (in theory, method or results): do certain approaches become more or less popular over time?
- Themes: what questions or concepts recur across the literature?
- Debates, conflicts and contradictions: where do sources disagree?
- Pivotal publications: are there any influential theories or studies that changed the direction of the field?
- Gaps: what is missing from the literature? Are there weaknesses that need to be addressed?
This step will help you work out the structure of your literature review and (if applicable) show how your own research will contribute to existing knowledge.
- Most research has focused on young women.
- There is an increasing interest in the visual aspects of social media.
- But there is still a lack of robust research on highly-visual platforms like Instagram and Snapchat – this is a gap that you could address in your own research.
There are various approaches to organising the body of a literature review. You should have a rough idea of your strategy before you start writing.
Depending on the length of your literature review, you can combine several of these strategies (for example, your overall structure might be thematic, but each theme is discussed chronologically).
Chronological
The simplest approach is to trace the development of the topic over time. However, if you choose this strategy, be careful to avoid simply listing and summarising sources in order.
Try to analyse patterns, turning points and key debates that have shaped the direction of the field. Give your interpretation of how and why certain developments occurred.
If you have found some recurring central themes, you can organise your literature review into subsections that address different aspects of the topic.
For example, if you are reviewing literature about inequalities in migrant health outcomes, key themes might include healthcare policy, language barriers, cultural attitudes, legal status, and economic access.
Methodological
If you draw your sources from different disciplines or fields that use a variety of research methods , you might want to compare the results and conclusions that emerge from different approaches. For example:
- Look at what results have emerged in qualitative versus quantitative research
- Discuss how the topic has been approached by empirical versus theoretical scholarship
- Divide the literature into sociological, historical, and cultural sources
Theoretical
A literature review is often the foundation for a theoretical framework . You can use it to discuss various theories, models, and definitions of key concepts.
You might argue for the relevance of a specific theoretical approach, or combine various theoretical concepts to create a framework for your research.
Like any other academic text, your literature review should have an introduction , a main body, and a conclusion . What you include in each depends on the objective of your literature review.
The introduction should clearly establish the focus and purpose of the literature review.
If you are writing the literature review as part of your dissertation or thesis, reiterate your central problem or research question and give a brief summary of the scholarly context. You can emphasise the timeliness of the topic (“many recent studies have focused on the problem of x”) or highlight a gap in the literature (“while there has been much research on x, few researchers have taken y into consideration”).
Depending on the length of your literature review, you might want to divide the body into subsections. You can use a subheading for each theme, time period, or methodological approach.
As you write, make sure to follow these tips:
- Summarise and synthesise: give an overview of the main points of each source and combine them into a coherent whole.
- Analyse and interpret: don’t just paraphrase other researchers – add your own interpretations, discussing the significance of findings in relation to the literature as a whole.
- Critically evaluate: mention the strengths and weaknesses of your sources.
- Write in well-structured paragraphs: use transitions and topic sentences to draw connections, comparisons and contrasts.
In the conclusion, you should summarise the key findings you have taken from the literature and emphasise their significance.
If the literature review is part of your dissertation or thesis, reiterate how your research addresses gaps and contributes new knowledge, or discuss how you have drawn on existing theories and methods to build a framework for your research. This can lead directly into your methodology section.
A literature review is a survey of scholarly sources (such as books, journal articles, and theses) related to a specific topic or research question .
It is often written as part of a dissertation , thesis, research paper , or proposal .
There are several reasons to conduct a literature review at the beginning of a research project:
- To familiarise yourself with the current state of knowledge on your topic
- To ensure that you’re not just repeating what others have already done
- To identify gaps in knowledge and unresolved problems that your research can address
- To develop your theoretical framework and methodology
- To provide an overview of the key findings and debates on the topic
Writing the literature review shows your reader how your work relates to existing research and what new insights it will contribute.
The literature review usually comes near the beginning of your dissertation . After the introduction , it grounds your research in a scholarly field and leads directly to your theoretical framework or methodology .
Cite this Scribbr article
If you want to cite this source, you can copy and paste the citation or click the ‘Cite this Scribbr article’ button to automatically add the citation to our free Reference Generator.
McCombes, S. (2022, June 07). What is a Literature Review? | Guide, Template, & Examples. Scribbr. Retrieved 7 June 2024, from https://www.scribbr.co.uk/thesis-dissertation/literature-review/
Is this article helpful?
Shona McCombes
Other students also liked, how to write a dissertation proposal | a step-by-step guide, what is a theoretical framework | a step-by-step guide, what is a research methodology | steps & tips.
- USC Libraries
- Research Guides
Organizing Your Social Sciences Research Paper
- 5. The Literature Review
- Purpose of Guide
- Design Flaws to Avoid
- Independent and Dependent Variables
- Glossary of Research Terms
- Reading Research Effectively
- Narrowing a Topic Idea
- Broadening a Topic Idea
- Extending the Timeliness of a Topic Idea
- Academic Writing Style
- Applying Critical Thinking
- Choosing a Title
- Making an Outline
- Paragraph Development
- Research Process Video Series
- Executive Summary
- The C.A.R.S. Model
- Background Information
- The Research Problem/Question
- Theoretical Framework
- Citation Tracking
- Content Alert Services
- Evaluating Sources
- Primary Sources
- Secondary Sources
- Tiertiary Sources
- Scholarly vs. Popular Publications
- Qualitative Methods
- Quantitative Methods
- Insiderness
- Using Non-Textual Elements
- Limitations of the Study
- Common Grammar Mistakes
- Writing Concisely
- Avoiding Plagiarism
- Footnotes or Endnotes?
- Further Readings
- Generative AI and Writing
- USC Libraries Tutorials and Other Guides
- Bibliography
A literature review surveys prior research published in books, scholarly articles, and any other sources relevant to a particular issue, area of research, or theory, and by so doing, provides a description, summary, and critical evaluation of these works in relation to the research problem being investigated. Literature reviews are designed to provide an overview of sources you have used in researching a particular topic and to demonstrate to your readers how your research fits within existing scholarship about the topic.
Fink, Arlene. Conducting Research Literature Reviews: From the Internet to Paper . Fourth edition. Thousand Oaks, CA: SAGE, 2014.
Importance of a Good Literature Review
A literature review may consist of simply a summary of key sources, but in the social sciences, a literature review usually has an organizational pattern and combines both summary and synthesis, often within specific conceptual categories . A summary is a recap of the important information of the source, but a synthesis is a re-organization, or a reshuffling, of that information in a way that informs how you are planning to investigate a research problem. The analytical features of a literature review might:
- Give a new interpretation of old material or combine new with old interpretations,
- Trace the intellectual progression of the field, including major debates,
- Depending on the situation, evaluate the sources and advise the reader on the most pertinent or relevant research, or
- Usually in the conclusion of a literature review, identify where gaps exist in how a problem has been researched to date.
Given this, the purpose of a literature review is to:
- Place each work in the context of its contribution to understanding the research problem being studied.
- Describe the relationship of each work to the others under consideration.
- Identify new ways to interpret prior research.
- Reveal any gaps that exist in the literature.
- Resolve conflicts amongst seemingly contradictory previous studies.
- Identify areas of prior scholarship to prevent duplication of effort.
- Point the way in fulfilling a need for additional research.
- Locate your own research within the context of existing literature [very important].
Fink, Arlene. Conducting Research Literature Reviews: From the Internet to Paper. 2nd ed. Thousand Oaks, CA: Sage, 2005; Hart, Chris. Doing a Literature Review: Releasing the Social Science Research Imagination . Thousand Oaks, CA: Sage Publications, 1998; Jesson, Jill. Doing Your Literature Review: Traditional and Systematic Techniques . Los Angeles, CA: SAGE, 2011; Knopf, Jeffrey W. "Doing a Literature Review." PS: Political Science and Politics 39 (January 2006): 127-132; Ridley, Diana. The Literature Review: A Step-by-Step Guide for Students . 2nd ed. Los Angeles, CA: SAGE, 2012.
Types of Literature Reviews
It is important to think of knowledge in a given field as consisting of three layers. First, there are the primary studies that researchers conduct and publish. Second are the reviews of those studies that summarize and offer new interpretations built from and often extending beyond the primary studies. Third, there are the perceptions, conclusions, opinion, and interpretations that are shared informally among scholars that become part of the body of epistemological traditions within the field.
In composing a literature review, it is important to note that it is often this third layer of knowledge that is cited as "true" even though it often has only a loose relationship to the primary studies and secondary literature reviews. Given this, while literature reviews are designed to provide an overview and synthesis of pertinent sources you have explored, there are a number of approaches you could adopt depending upon the type of analysis underpinning your study.
Argumentative Review This form examines literature selectively in order to support or refute an argument, deeply embedded assumption, or philosophical problem already established in the literature. The purpose is to develop a body of literature that establishes a contrarian viewpoint. Given the value-laden nature of some social science research [e.g., educational reform; immigration control], argumentative approaches to analyzing the literature can be a legitimate and important form of discourse. However, note that they can also introduce problems of bias when they are used to make summary claims of the sort found in systematic reviews [see below].
Integrative Review Considered a form of research that reviews, critiques, and synthesizes representative literature on a topic in an integrated way such that new frameworks and perspectives on the topic are generated. The body of literature includes all studies that address related or identical hypotheses or research problems. A well-done integrative review meets the same standards as primary research in regard to clarity, rigor, and replication. This is the most common form of review in the social sciences.
Historical Review Few things rest in isolation from historical precedent. Historical literature reviews focus on examining research throughout a period of time, often starting with the first time an issue, concept, theory, phenomena emerged in the literature, then tracing its evolution within the scholarship of a discipline. The purpose is to place research in a historical context to show familiarity with state-of-the-art developments and to identify the likely directions for future research.
Methodological Review A review does not always focus on what someone said [findings], but how they came about saying what they say [method of analysis]. Reviewing methods of analysis provides a framework of understanding at different levels [i.e. those of theory, substantive fields, research approaches, and data collection and analysis techniques], how researchers draw upon a wide variety of knowledge ranging from the conceptual level to practical documents for use in fieldwork in the areas of ontological and epistemological consideration, quantitative and qualitative integration, sampling, interviewing, data collection, and data analysis. This approach helps highlight ethical issues which you should be aware of and consider as you go through your own study.
Systematic Review This form consists of an overview of existing evidence pertinent to a clearly formulated research question, which uses pre-specified and standardized methods to identify and critically appraise relevant research, and to collect, report, and analyze data from the studies that are included in the review. The goal is to deliberately document, critically evaluate, and summarize scientifically all of the research about a clearly defined research problem . Typically it focuses on a very specific empirical question, often posed in a cause-and-effect form, such as "To what extent does A contribute to B?" This type of literature review is primarily applied to examining prior research studies in clinical medicine and allied health fields, but it is increasingly being used in the social sciences.
Theoretical Review The purpose of this form is to examine the corpus of theory that has accumulated in regard to an issue, concept, theory, phenomena. The theoretical literature review helps to establish what theories already exist, the relationships between them, to what degree the existing theories have been investigated, and to develop new hypotheses to be tested. Often this form is used to help establish a lack of appropriate theories or reveal that current theories are inadequate for explaining new or emerging research problems. The unit of analysis can focus on a theoretical concept or a whole theory or framework.
NOTE: Most often the literature review will incorporate some combination of types. For example, a review that examines literature supporting or refuting an argument, assumption, or philosophical problem related to the research problem will also need to include writing supported by sources that establish the history of these arguments in the literature.
Baumeister, Roy F. and Mark R. Leary. "Writing Narrative Literature Reviews." Review of General Psychology 1 (September 1997): 311-320; Mark R. Fink, Arlene. Conducting Research Literature Reviews: From the Internet to Paper . 2nd ed. Thousand Oaks, CA: Sage, 2005; Hart, Chris. Doing a Literature Review: Releasing the Social Science Research Imagination . Thousand Oaks, CA: Sage Publications, 1998; Kennedy, Mary M. "Defining a Literature." Educational Researcher 36 (April 2007): 139-147; Petticrew, Mark and Helen Roberts. Systematic Reviews in the Social Sciences: A Practical Guide . Malden, MA: Blackwell Publishers, 2006; Torracro, Richard. "Writing Integrative Literature Reviews: Guidelines and Examples." Human Resource Development Review 4 (September 2005): 356-367; Rocco, Tonette S. and Maria S. Plakhotnik. "Literature Reviews, Conceptual Frameworks, and Theoretical Frameworks: Terms, Functions, and Distinctions." Human Ressource Development Review 8 (March 2008): 120-130; Sutton, Anthea. Systematic Approaches to a Successful Literature Review . Los Angeles, CA: Sage Publications, 2016.
Structure and Writing Style
I. Thinking About Your Literature Review
The structure of a literature review should include the following in support of understanding the research problem :
- An overview of the subject, issue, or theory under consideration, along with the objectives of the literature review,
- Division of works under review into themes or categories [e.g. works that support a particular position, those against, and those offering alternative approaches entirely],
- An explanation of how each work is similar to and how it varies from the others,
- Conclusions as to which pieces are best considered in their argument, are most convincing of their opinions, and make the greatest contribution to the understanding and development of their area of research.
The critical evaluation of each work should consider :
- Provenance -- what are the author's credentials? Are the author's arguments supported by evidence [e.g. primary historical material, case studies, narratives, statistics, recent scientific findings]?
- Methodology -- were the techniques used to identify, gather, and analyze the data appropriate to addressing the research problem? Was the sample size appropriate? Were the results effectively interpreted and reported?
- Objectivity -- is the author's perspective even-handed or prejudicial? Is contrary data considered or is certain pertinent information ignored to prove the author's point?
- Persuasiveness -- which of the author's theses are most convincing or least convincing?
- Validity -- are the author's arguments and conclusions convincing? Does the work ultimately contribute in any significant way to an understanding of the subject?
II. Development of the Literature Review
Four Basic Stages of Writing 1. Problem formulation -- which topic or field is being examined and what are its component issues? 2. Literature search -- finding materials relevant to the subject being explored. 3. Data evaluation -- determining which literature makes a significant contribution to the understanding of the topic. 4. Analysis and interpretation -- discussing the findings and conclusions of pertinent literature.
Consider the following issues before writing the literature review: Clarify If your assignment is not specific about what form your literature review should take, seek clarification from your professor by asking these questions: 1. Roughly how many sources would be appropriate to include? 2. What types of sources should I review (books, journal articles, websites; scholarly versus popular sources)? 3. Should I summarize, synthesize, or critique sources by discussing a common theme or issue? 4. Should I evaluate the sources in any way beyond evaluating how they relate to understanding the research problem? 5. Should I provide subheadings and other background information, such as definitions and/or a history? Find Models Use the exercise of reviewing the literature to examine how authors in your discipline or area of interest have composed their literature review sections. Read them to get a sense of the types of themes you might want to look for in your own research or to identify ways to organize your final review. The bibliography or reference section of sources you've already read, such as required readings in the course syllabus, are also excellent entry points into your own research. Narrow the Topic The narrower your topic, the easier it will be to limit the number of sources you need to read in order to obtain a good survey of relevant resources. Your professor will probably not expect you to read everything that's available about the topic, but you'll make the act of reviewing easier if you first limit scope of the research problem. A good strategy is to begin by searching the USC Libraries Catalog for recent books about the topic and review the table of contents for chapters that focuses on specific issues. You can also review the indexes of books to find references to specific issues that can serve as the focus of your research. For example, a book surveying the history of the Israeli-Palestinian conflict may include a chapter on the role Egypt has played in mediating the conflict, or look in the index for the pages where Egypt is mentioned in the text. Consider Whether Your Sources are Current Some disciplines require that you use information that is as current as possible. This is particularly true in disciplines in medicine and the sciences where research conducted becomes obsolete very quickly as new discoveries are made. However, when writing a review in the social sciences, a survey of the history of the literature may be required. In other words, a complete understanding the research problem requires you to deliberately examine how knowledge and perspectives have changed over time. Sort through other current bibliographies or literature reviews in the field to get a sense of what your discipline expects. You can also use this method to explore what is considered by scholars to be a "hot topic" and what is not.
III. Ways to Organize Your Literature Review
Chronology of Events If your review follows the chronological method, you could write about the materials according to when they were published. This approach should only be followed if a clear path of research building on previous research can be identified and that these trends follow a clear chronological order of development. For example, a literature review that focuses on continuing research about the emergence of German economic power after the fall of the Soviet Union. By Publication Order your sources by publication chronology, then, only if the order demonstrates a more important trend. For instance, you could order a review of literature on environmental studies of brown fields if the progression revealed, for example, a change in the soil collection practices of the researchers who wrote and/or conducted the studies. Thematic [“conceptual categories”] A thematic literature review is the most common approach to summarizing prior research in the social and behavioral sciences. Thematic reviews are organized around a topic or issue, rather than the progression of time, although the progression of time may still be incorporated into a thematic review. For example, a review of the Internet’s impact on American presidential politics could focus on the development of online political satire. While the study focuses on one topic, the Internet’s impact on American presidential politics, it would still be organized chronologically reflecting technological developments in media. The difference in this example between a "chronological" and a "thematic" approach is what is emphasized the most: themes related to the role of the Internet in presidential politics. Note that more authentic thematic reviews tend to break away from chronological order. A review organized in this manner would shift between time periods within each section according to the point being made. Methodological A methodological approach focuses on the methods utilized by the researcher. For the Internet in American presidential politics project, one methodological approach would be to look at cultural differences between the portrayal of American presidents on American, British, and French websites. Or the review might focus on the fundraising impact of the Internet on a particular political party. A methodological scope will influence either the types of documents in the review or the way in which these documents are discussed.
Other Sections of Your Literature Review Once you've decided on the organizational method for your literature review, the sections you need to include in the paper should be easy to figure out because they arise from your organizational strategy. In other words, a chronological review would have subsections for each vital time period; a thematic review would have subtopics based upon factors that relate to the theme or issue. However, sometimes you may need to add additional sections that are necessary for your study, but do not fit in the organizational strategy of the body. What other sections you include in the body is up to you. However, only include what is necessary for the reader to locate your study within the larger scholarship about the research problem.
Here are examples of other sections, usually in the form of a single paragraph, you may need to include depending on the type of review you write:
- Current Situation : Information necessary to understand the current topic or focus of the literature review.
- Sources Used : Describes the methods and resources [e.g., databases] you used to identify the literature you reviewed.
- History : The chronological progression of the field, the research literature, or an idea that is necessary to understand the literature review, if the body of the literature review is not already a chronology.
- Selection Methods : Criteria you used to select (and perhaps exclude) sources in your literature review. For instance, you might explain that your review includes only peer-reviewed [i.e., scholarly] sources.
- Standards : Description of the way in which you present your information.
- Questions for Further Research : What questions about the field has the review sparked? How will you further your research as a result of the review?
IV. Writing Your Literature Review
Once you've settled on how to organize your literature review, you're ready to write each section. When writing your review, keep in mind these issues.
Use Evidence A literature review section is, in this sense, just like any other academic research paper. Your interpretation of the available sources must be backed up with evidence [citations] that demonstrates that what you are saying is valid. Be Selective Select only the most important points in each source to highlight in the review. The type of information you choose to mention should relate directly to the research problem, whether it is thematic, methodological, or chronological. Related items that provide additional information, but that are not key to understanding the research problem, can be included in a list of further readings . Use Quotes Sparingly Some short quotes are appropriate if you want to emphasize a point, or if what an author stated cannot be easily paraphrased. Sometimes you may need to quote certain terminology that was coined by the author, is not common knowledge, or taken directly from the study. Do not use extensive quotes as a substitute for using your own words in reviewing the literature. Summarize and Synthesize Remember to summarize and synthesize your sources within each thematic paragraph as well as throughout the review. Recapitulate important features of a research study, but then synthesize it by rephrasing the study's significance and relating it to your own work and the work of others. Keep Your Own Voice While the literature review presents others' ideas, your voice [the writer's] should remain front and center. For example, weave references to other sources into what you are writing but maintain your own voice by starting and ending the paragraph with your own ideas and wording. Use Caution When Paraphrasing When paraphrasing a source that is not your own, be sure to represent the author's information or opinions accurately and in your own words. Even when paraphrasing an author’s work, you still must provide a citation to that work.
V. Common Mistakes to Avoid
These are the most common mistakes made in reviewing social science research literature.
- Sources in your literature review do not clearly relate to the research problem;
- You do not take sufficient time to define and identify the most relevant sources to use in the literature review related to the research problem;
- Relies exclusively on secondary analytical sources rather than including relevant primary research studies or data;
- Uncritically accepts another researcher's findings and interpretations as valid, rather than examining critically all aspects of the research design and analysis;
- Does not describe the search procedures that were used in identifying the literature to review;
- Reports isolated statistical results rather than synthesizing them in chi-squared or meta-analytic methods; and,
- Only includes research that validates assumptions and does not consider contrary findings and alternative interpretations found in the literature.
Cook, Kathleen E. and Elise Murowchick. “Do Literature Review Skills Transfer from One Course to Another?” Psychology Learning and Teaching 13 (March 2014): 3-11; Fink, Arlene. Conducting Research Literature Reviews: From the Internet to Paper . 2nd ed. Thousand Oaks, CA: Sage, 2005; Hart, Chris. Doing a Literature Review: Releasing the Social Science Research Imagination . Thousand Oaks, CA: Sage Publications, 1998; Jesson, Jill. Doing Your Literature Review: Traditional and Systematic Techniques . London: SAGE, 2011; Literature Review Handout. Online Writing Center. Liberty University; Literature Reviews. The Writing Center. University of North Carolina; Onwuegbuzie, Anthony J. and Rebecca Frels. Seven Steps to a Comprehensive Literature Review: A Multimodal and Cultural Approach . Los Angeles, CA: SAGE, 2016; Ridley, Diana. The Literature Review: A Step-by-Step Guide for Students . 2nd ed. Los Angeles, CA: SAGE, 2012; Randolph, Justus J. “A Guide to Writing the Dissertation Literature Review." Practical Assessment, Research, and Evaluation. vol. 14, June 2009; Sutton, Anthea. Systematic Approaches to a Successful Literature Review . Los Angeles, CA: Sage Publications, 2016; Taylor, Dena. The Literature Review: A Few Tips On Conducting It. University College Writing Centre. University of Toronto; Writing a Literature Review. Academic Skills Centre. University of Canberra.
Writing Tip
Break Out of Your Disciplinary Box!
Thinking interdisciplinarily about a research problem can be a rewarding exercise in applying new ideas, theories, or concepts to an old problem. For example, what might cultural anthropologists say about the continuing conflict in the Middle East? In what ways might geographers view the need for better distribution of social service agencies in large cities than how social workers might study the issue? You don’t want to substitute a thorough review of core research literature in your discipline for studies conducted in other fields of study. However, particularly in the social sciences, thinking about research problems from multiple vectors is a key strategy for finding new solutions to a problem or gaining a new perspective. Consult with a librarian about identifying research databases in other disciplines; almost every field of study has at least one comprehensive database devoted to indexing its research literature.
Frodeman, Robert. The Oxford Handbook of Interdisciplinarity . New York: Oxford University Press, 2010.
Another Writing Tip
Don't Just Review for Content!
While conducting a review of the literature, maximize the time you devote to writing this part of your paper by thinking broadly about what you should be looking for and evaluating. Review not just what scholars are saying, but how are they saying it. Some questions to ask:
- How are they organizing their ideas?
- What methods have they used to study the problem?
- What theories have been used to explain, predict, or understand their research problem?
- What sources have they cited to support their conclusions?
- How have they used non-textual elements [e.g., charts, graphs, figures, etc.] to illustrate key points?
When you begin to write your literature review section, you'll be glad you dug deeper into how the research was designed and constructed because it establishes a means for developing more substantial analysis and interpretation of the research problem.
Hart, Chris. Doing a Literature Review: Releasing the Social Science Research Imagination . Thousand Oaks, CA: Sage Publications, 1 998.
Yet Another Writing Tip
When Do I Know I Can Stop Looking and Move On?
Here are several strategies you can utilize to assess whether you've thoroughly reviewed the literature:
- Look for repeating patterns in the research findings . If the same thing is being said, just by different people, then this likely demonstrates that the research problem has hit a conceptual dead end. At this point consider: Does your study extend current research? Does it forge a new path? Or, does is merely add more of the same thing being said?
- Look at sources the authors cite to in their work . If you begin to see the same researchers cited again and again, then this is often an indication that no new ideas have been generated to address the research problem.
- Search Google Scholar to identify who has subsequently cited leading scholars already identified in your literature review [see next sub-tab]. This is called citation tracking and there are a number of sources that can help you identify who has cited whom, particularly scholars from outside of your discipline. Here again, if the same authors are being cited again and again, this may indicate no new literature has been written on the topic.
Onwuegbuzie, Anthony J. and Rebecca Frels. Seven Steps to a Comprehensive Literature Review: A Multimodal and Cultural Approach . Los Angeles, CA: Sage, 2016; Sutton, Anthea. Systematic Approaches to a Successful Literature Review . Los Angeles, CA: Sage Publications, 2016.
- << Previous: Theoretical Framework
- Next: Citation Tracking >>
- Last Updated: May 30, 2024 9:38 AM
- URL: https://libguides.usc.edu/writingguide

Home » Education » What is the Difference Between Literature Review and Theoretical Framework
What is the Difference Between Literature Review and Theoretical Framework
The main difference between literature review and theoretical framework is their function. The literature review explores what has already been written about the topic under study in order to highlight a gap, whereas the theoretical framework is the conceptual and analytical approach the researcher is going to take to fill that gap.
Literature review and theoretical framework are two indispensable components of research . Both are equally important for the foundation of a research study.
Key Areas Covered
1. What is Literature Review – Definition, Features 2. What is Theoretical Framework – Definition, Features 3. Difference Between Literature Review and Theoretical Framework – Comparison of Key Differences

What is a Literature Review
A literature review is a vital component of a research study. A literature review is a discussion on the already existing material in the subject area. Thus, this will require a collection of published (in print or online) work concerning the selected research area. In other words, a literature review is a review of the literature in the related subject area. A literature review makes a case for the research study. It analyzes the existing literature in order to identify and highlight a gap in the literature.

Moreover, a good literature review is a critical discussion, displaying the writer’s knowledge of relevant theories and approaches and awareness of contrasting arguments. A literature review should have the following features (Caulley, 1992)
- Compare and contrast different researchers’ views
- Identify areas in which researchers are in disagreement
- Group researchers who have similar conclusions
- Criticize the methodology
- Highlight exemplary studies
- Highlight gaps in research
- Indicate the connection between your study and previous studies
- Indicate how your study will contribute to the literature in general
- Conclude by summarizing what the literature indicates
Furthermore, the structure of a literature review is similar to that of an article or essay . Overall, literature reviews help researchers to evaluate the existing literature, identify a gap in the research area, place their study in the existing research and identify future research.
What is a Theoretical Framework
The theoretical framework is the research component that introduces and describes the theory that explains why the research problem under study exists. It is also the conceptual and analytical approach the researcher is going to take to fill the research gap identified by the literature review. Moreover, it is the structure that holds the structure of the research theory.
The researcher may not easily find the theoretical framework within the literature. Therefore, he or she may have to go through many research studies and course readings for theories and models relevant to the research problem under investigation. In addition, the theory must be selected based on its relevance, ease of application, and explanatory power.
Difference Between Literature Review and Theoretical Framework
A literature review is a critical evaluation of the existing published work in a selected research area, while a theoretical framework is a component in research that introduces and describes the theory behind the research problem.
Moreover, the literature review explores what has already been written about the topic under investigation in order to highlight a gap, whereas the theoretical framework is the conceptual and analytical approach the researcher is going to take to fill that gap. Therefore, a literature review is backwards-looking while theory framework is forward-looking.
In conclusion, the main difference between literature review and theoretical framework is their function. The literature review explores what has already been written about the topic under study in order to highlight a gap, whereas the theoretical framework is the conceptual and analytical approach the researcher is going to take to fill that gap.
1. Caulley, D. N. “Writing a critical review of the literature.” La Trobe University: Bundoora (1992). 2. “ Organizing Your Social Sciences Research Paper: Theoretical Framework .” Research Guide.
Image Courtesy:
About the Author: Hasa
Hasanthi is a seasoned content writer and editor with over 8 years of experience. Armed with a BA degree in English and a knack for digital marketing, she explores her passions for literature, history, culture, and food through her engaging and informative writing.
You May Also Like These
Leave a reply cancel reply.

Literature Review: Types of Literature Reviews
- Literature Review
- Purpose of a Literature Review
- Work in Progress
- Compiling & Writing
- Books, Articles, & Web Pages
Types of Literature Reviews
- Departmental Differences
- Citation Styles & Plagiarism
- Know the Difference! Systematic Review vs. Literature Review
It is important to think of knowledge in a given field as consisting of three layers.
- First, there are the primary studies that researchers conduct and publish.
- Second, are the reviews of those studies that summarize and offer new interpretations built from and often extending beyond the original studies.
- Third, there are the perceptions, conclusions, opinions, and interpretations that are shared informally that become part of the lore of the field.
In composing a literature review, it is important to note that it is often this third layer of knowledge that is cited as "true" even though it often has only a loose relationship to the primary studies and secondary literature reviews.
Given this, while literature reviews are designed to provide an overview and synthesis of pertinent sources you have explored, there are several approaches to how they can be done, depending upon the type of analysis underpinning your study. Listed below are definitions of types of literature reviews:
Argumentative Review This form examines literature selectively in order to support or refute an argument, deeply embedded assumption, or philosophical problem already established in the literature. The purpose is to develop a body of literature that establishes a contrarian viewpoint. Given the value-laden nature of some social science research [e.g., educational reform; immigration control], argumentative approaches to analyzing the literature can be a legitimate and important form of discourse. However, note that they can also introduce problems of bias when they are used to make summary claims of the sort found in systematic reviews.
Integrative Review Considered a form of research that reviews, critiques, and synthesizes representative literature on a topic in an integrated way such that new frameworks and perspectives on the topic are generated. The body of literature includes all studies that address related or identical hypotheses. A well-done integrative review meets the same standards as primary research in regard to clarity, rigor, and replication.
Historical Review Few things rest in isolation from historical precedent. Historical reviews are focused on examining research throughout a period of time, often starting with the first time an issue, concept, theory, phenomenon emerged in the literature, then tracing its evolution within the scholarship of a discipline. The purpose is to place research in a historical context to show familiarity with state-of-the-art developments and to identify the likely directions for future research.
Methodological Review A review does not always focus on what someone said [content], but how they said it [method of analysis]. This approach provides a framework of understanding at different levels (i.e. those of theory, substantive fields, research approaches, and data collection and analysis techniques), enables researchers to draw on a wide variety of knowledge ranging from the conceptual level to practical documents for use in fieldwork in the areas of ontological and epistemological consideration, quantitative and qualitative integration, sampling, interviewing, data collection and data analysis, and helps highlight many ethical issues which we should be aware of and consider as we go through our study.
Systematic Review This form consists of an overview of existing evidence pertinent to a clearly formulated research question, which uses pre-specified and standardized methods to identify and critically appraise relevant research, and to collect, report, and analyze data from the studies that are included in the review. Typically it focuses on a very specific empirical question, often posed in a cause-and-effect form, such as "To what extent does A contribute to B?"
Theoretical Review The purpose of this form is to concretely examine the corpus of theory that has accumulated in regard to an issue, concept, theory, phenomenon. The theoretical literature review help establish what theories already exist, the relationships between them, to what degree the existing theories have been investigated, and to develop new hypotheses to be tested. Often this form is used to help establish a lack of appropriate theories or reveal that current theories are inadequate for explaining new or emerging research problems. The unit of analysis can focus on a theoretical concept or a whole theory or framework.
* Kennedy, Mary M. "Defining a Literature." Educational Researcher 36 (April 2007): 139-147.
All content is from The Literature Review created by Dr. Robert Larabee USC
- << Previous: Books, Articles, & Web Pages
- Next: Departmental Differences >>
- Last Updated: Oct 19, 2023 12:07 PM
- URL: https://uscupstate.libguides.com/Literature_Review

- University of Texas Libraries
Literature Reviews
- What is a literature review?
- Steps in the Literature Review Process
- Define your research question
- Determine inclusion and exclusion criteria
- Choose databases and search
- Review Results
- Synthesize Results
- Analyze Results
- Librarian Support
What is a Literature Review?
A literature or narrative review is a comprehensive review and analysis of the published literature on a specific topic or research question. The literature that is reviewed contains: books, articles, academic articles, conference proceedings, association papers, and dissertations. It contains the most pertinent studies and points to important past and current research and practices. It provides background and context, and shows how your research will contribute to the field.
A literature review should:
- Provide a comprehensive and updated review of the literature;
- Explain why this review has taken place;
- Articulate a position or hypothesis;
- Acknowledge and account for conflicting and corroborating points of view
From S age Research Methods
Purpose of a Literature Review
A literature review can be written as an introduction to a study to:
- Demonstrate how a study fills a gap in research
- Compare a study with other research that's been done
Or it can be a separate work (a research article on its own) which:
- Organizes or describes a topic
- Describes variables within a particular issue/problem
Limitations of a Literature Review
Some of the limitations of a literature review are:
- It's a snapshot in time. Unlike other reviews, this one has beginning, a middle and an end. There may be future developments that could make your work less relevant.
- It may be too focused. Some niche studies may miss the bigger picture.
- It can be difficult to be comprehensive. There is no way to make sure all the literature on a topic was considered.
- It is easy to be biased if you stick to top tier journals. There may be other places where people are publishing exemplary research. Look to open access publications and conferences to reflect a more inclusive collection. Also, make sure to include opposing views (and not just supporting evidence).
Source: Grant, Maria J., and Andrew Booth. “A Typology of Reviews: An Analysis of 14 Review Types and Associated Methodologies.” Health Information & Libraries Journal, vol. 26, no. 2, June 2009, pp. 91–108. Wiley Online Library, doi:10.1111/j.1471-1842.2009.00848.x.
Meryl Brodsky : Communication and Information Studies
Hannah Chapman Tripp : Biology, Neuroscience
Carolyn Cunningham : Human Development & Family Sciences, Psychology, Sociology
Larayne Dallas : Engineering
Janelle Hedstrom : Special Education, Curriculum & Instruction, Ed Leadership & Policy
Susan Macicak : Linguistics
Imelda Vetter : Dell Medical School
For help in other subject areas, please see the guide to library specialists by subject .
Periodically, UT Libraries runs a workshop covering the basics and library support for literature reviews. While we try to offer these once per academic year, we find providing the recording to be helpful to community members who have missed the session. Following is the most recent recording of the workshop, Conducting a Literature Review. To view the recording, a UT login is required.
- October 26, 2022 recording
- Last Updated: Oct 26, 2022 2:49 PM
- URL: https://guides.lib.utexas.edu/literaturereviews
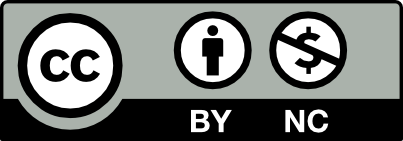
Have a language expert improve your writing
Run a free plagiarism check in 10 minutes, generate accurate citations for free.
- Knowledge Base
- Dissertation
Theoretical Framework Example for a Thesis or Dissertation
Published on October 14, 2015 by Sarah Vinz . Revised on July 18, 2023 by Tegan George.
Your theoretical framework defines the key concepts in your research, suggests relationships between them, and discusses relevant theories based on your literature review .
A strong theoretical framework gives your research direction. It allows you to convincingly interpret, explain, and generalize from your findings and show the relevance of your thesis or dissertation topic in your field.
Instantly correct all language mistakes in your text
Upload your document to correct all your mistakes in minutes
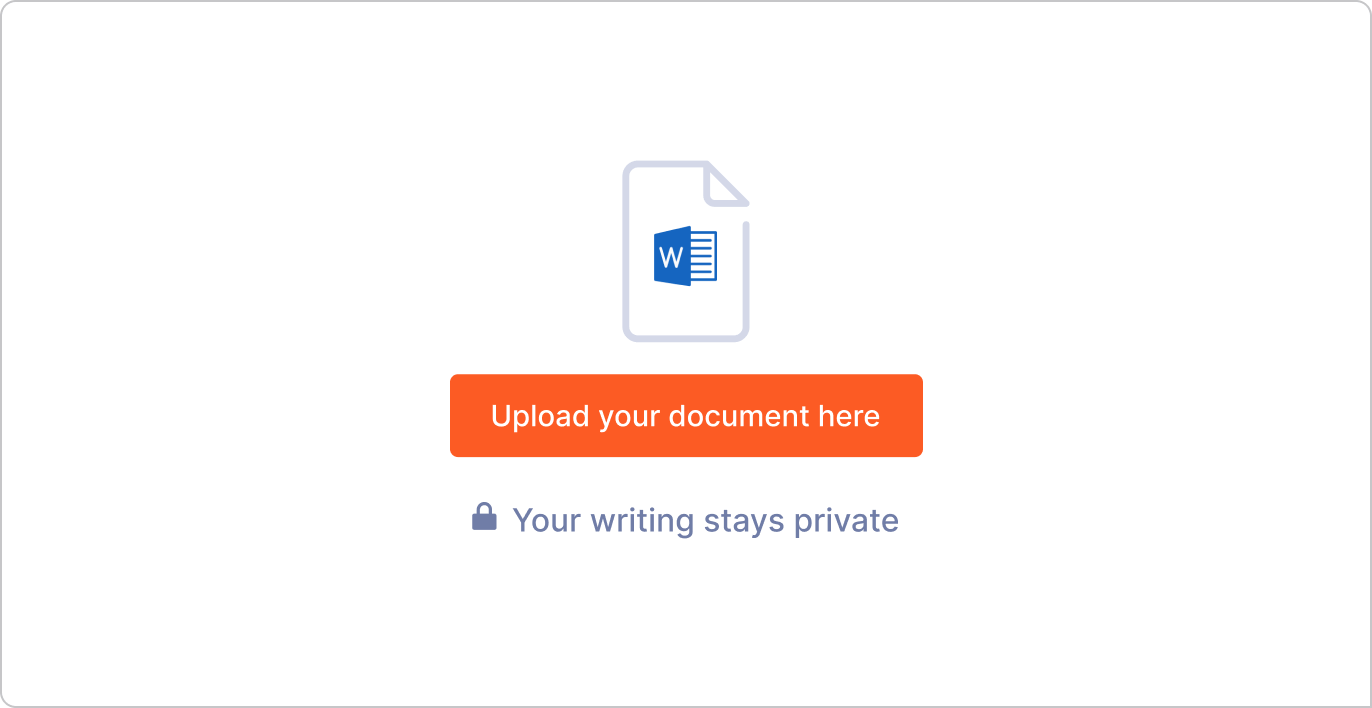
Table of contents
Sample problem statement and research questions, sample theoretical framework, your theoretical framework, other interesting articles.
Your theoretical framework is based on:
- Your problem statement
- Your research questions
- Your literature review
A new boutique downtown is struggling with the fact that many of their online customers do not return to make subsequent purchases. This is a big issue for the otherwise fast-growing store.Management wants to increase customer loyalty. They believe that improved customer satisfaction will play a major role in achieving their goal of increased return customers.
To investigate this problem, you have zeroed in on the following problem statement, objective, and research questions:
- Problem : Many online customers do not return to make subsequent purchases.
- Objective : To increase the quantity of return customers.
- Research question : How can the satisfaction of the boutique’s online customers be improved in order to increase the quantity of return customers?
The concepts of “customer loyalty” and “customer satisfaction” are clearly central to this study, along with their relationship to the likelihood that a customer will return. Your theoretical framework should define these concepts and discuss theories about the relationship between these variables.
Some sub-questions could include:
- What is the relationship between customer loyalty and customer satisfaction?
- How satisfied and loyal are the boutique’s online customers currently?
- What factors affect the satisfaction and loyalty of the boutique’s online customers?
As the concepts of “loyalty” and “customer satisfaction” play a major role in the investigation and will later be measured, they are essential concepts to define within your theoretical framework .
Don't submit your assignments before you do this
The academic proofreading tool has been trained on 1000s of academic texts. Making it the most accurate and reliable proofreading tool for students. Free citation check included.
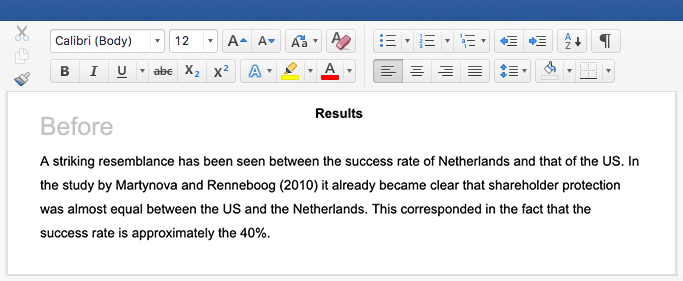
Try for free
Below is a simplified example showing how you can describe and compare theories in your thesis or dissertation . In this example, we focus on the concept of customer satisfaction introduced above.
Customer satisfaction
Thomassen (2003, p. 69) defines customer satisfaction as “the perception of the customer as a result of consciously or unconsciously comparing their experiences with their expectations.” Kotler & Keller (2008, p. 80) build on this definition, stating that customer satisfaction is determined by “the degree to which someone is happy or disappointed with the observed performance of a product in relation to his or her expectations.”
Performance that is below expectations leads to a dissatisfied customer, while performance that satisfies expectations produces satisfied customers (Kotler & Keller, 2003, p. 80).
The definition of Zeithaml and Bitner (2003, p. 86) is slightly different from that of Thomassen. They posit that “satisfaction is the consumer fulfillment response. It is a judgement that a product or service feature, or the product of service itself, provides a pleasurable level of consumption-related fulfillment.” Zeithaml and Bitner’s emphasis is thus on obtaining a certain satisfaction in relation to purchasing.
Thomassen’s definition is the most relevant to the aims of this study, given the emphasis it places on unconscious perception. Although Zeithaml and Bitner, like Thomassen, say that customer satisfaction is a reaction to the experience gained, there is no distinction between conscious and unconscious comparisons in their definition.
The boutique claims in its mission statement that it wants to sell not only a product, but also a feeling. As a result, unconscious comparison will play an important role in the satisfaction of its customers. Thomassen’s definition is therefore more relevant.
Thomassen’s Customer Satisfaction Model
According to Thomassen, both the so-called “value proposition” and other influences have an impact on final customer satisfaction. In his satisfaction model (Fig. 1), Thomassen shows that word-of-mouth, personal needs, past experiences, and marketing and public relations determine customers’ needs and expectations.
These factors are compared to their experiences, with the interplay between expectations and experiences determining a customer’s satisfaction level. Thomassen’s model is important for this study as it allows us to determine both the extent to which the boutique’s customers are satisfied, as well as where improvements can be made.
Figure 1 Customer satisfaction creation
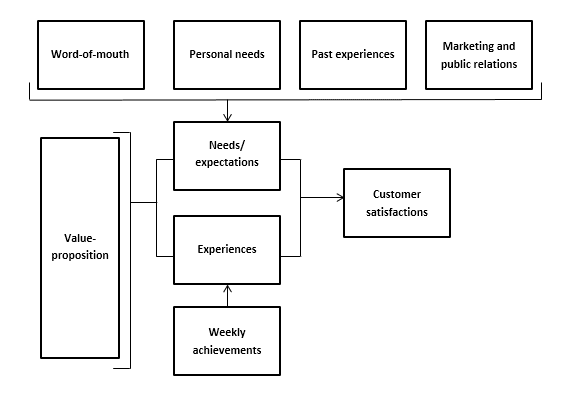
Of course, you could analyze the concepts more thoroughly and compare additional definitions to each other. You could also discuss the theories and ideas of key authors in greater detail and provide several models to illustrate different concepts.
If you want to know more about AI for academic writing, AI tools, or research bias, make sure to check out some of our other articles with explanations and examples or go directly to our tools!
Research bias
- Anchoring bias
- Halo effect
- The Baader–Meinhof phenomenon
- The placebo effect
- Nonresponse bias
- Deep learning
- Generative AI
- Machine learning
- Reinforcement learning
- Supervised vs. unsupervised learning
(AI) Tools
- Grammar Checker
- Paraphrasing Tool
- Text Summarizer
- AI Detector
- Plagiarism Checker
- Citation Generator
Cite this Scribbr article
If you want to cite this source, you can copy and paste the citation or click the “Cite this Scribbr article” button to automatically add the citation to our free Citation Generator.
Vinz, S. (2023, July 18). Theoretical Framework Example for a Thesis or Dissertation. Scribbr. Retrieved June 9, 2024, from https://www.scribbr.com/dissertation/theoretical-framework-example/
Is this article helpful?
Sarah's academic background includes a Master of Arts in English, a Master of International Affairs degree, and a Bachelor of Arts in Political Science. She loves the challenge of finding the perfect formulation or wording and derives much satisfaction from helping students take their academic writing up a notch.
Other students also liked
What is a theoretical framework | guide to organizing, how to write a literature review | guide, examples, & templates, what is a research methodology | steps & tips, what is your plagiarism score.
Thank you for visiting nature.com. You are using a browser version with limited support for CSS. To obtain the best experience, we recommend you use a more up to date browser (or turn off compatibility mode in Internet Explorer). In the meantime, to ensure continued support, we are displaying the site without styles and JavaScript.
- View all journals
- Explore content
- About the journal
- Publish with us
- Sign up for alerts
- Open access
- Published: 26 May 2024
A double machine learning model for measuring the impact of the Made in China 2025 strategy on green economic growth
- Jie Yuan 1 &
- Shucheng Liu 2
Scientific Reports volume 14 , Article number: 12026 ( 2024 ) Cite this article
454 Accesses
1 Altmetric
Metrics details
- Environmental economics
- Environmental impact
- Sustainability
The transformation and upgrading of China’s manufacturing industry is supported by smart and green manufacturing, which have great potential to empower the nation’s green development. This study examines the impact of the Made in China 2025 industrial policy on urban green economic growth. This study applies the super-slacks-based measure model to measure cities’ green economic growth, using the double machine learning model, which overcomes the limitations of the linear setting of traditional causal inference models and maintains estimation accuracy under high-dimensional control variables, to conduct an empirical analysis based on panel data of 281 Chinese cities from 2006 to 2021. The results reveal that the Made in China 2025 strategy significantly drives urban green economic growth, and this finding holds after a series of robustness tests. A mechanism analysis indicates that the Made in China 2025 strategy promotes green economic growth through green technology progress, optimizing energy consumption structure, upgrading industrial structure, and strengthening environmental supervision. In addition, the policy has a stronger driving effect for cities with high manufacturing concentration, industrial intelligence, and digital finance development. This study provides valuable theoretical insights and policy implications for government planning to promote high-quality development through industrial policy.
Similar content being viewed by others
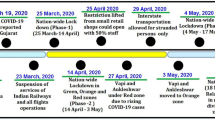
Positive effects of COVID-19 lockdown on air quality of industrial cities (Ankleshwar and Vapi) of Western India
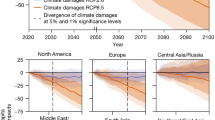
The economic commitment of climate change
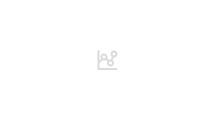
The impact of artificial intelligence on employment: the role of virtual agglomeration
Introduction.
Since China’s reform and opening up, the nation’s economy has experienced rapid growth for more than 40 years. According to the National Bureau of Statistics, China’s per capita GDP has grown from 385 yuan in 1978 to 85,698 yuan in 2022, with an average annual growth rate of 13.2%. However, obtaining this growth miracle has come at considerable social and environmental costs 1 . Current pollution prevention and control systems have not yet fundamentally alleviated the structural and root causes, impairing China’s economic progress toward high-quality development 2 . The report of the 20th National Congress of the Communist Party of China proposed that the future will be focused on promoting the formation of green modes of production and lifestyles and advancing the harmonious coexistence of human beings and nature. This indicates that transforming the mode of economic development is now the focus of the government’s attention, calling for advancing the practices of green growth aimed at energy conservation, emissions reduction, and sustainability while continuously increasing economic output 3 . As a result, identifying approaches to balance economic growth and green environmental protection in the development process and realize green economic growth has become an arduous challenge and a crucially significant concern for China’s high-quality economic development.
An intrinsic driver of urban economic growth, manufacturing is also the most energy-intensive and pollution-emitting industry, and greatly constrains urban green development 4 . China’s manufacturing industry urgently needs to advance the formation of a resource-saving and environmentally friendly industrial structure and manufacturing system through transformation and upgrading to support for green economic growth 5 . As an incentive-based industrial policy that emphasizes an innovation-driven and eco-civilized development path through the development and implementation of an intelligent and green manufacturing system, Made in China 2025 is a significant initiative for promoting the manufacturing industry’s transformation and upgrading, providing solid economic support for green economic growth 6 . To promote the effective implementation of this industrial policy, fully mobilize localities to explore new modes and paths of manufacturing development, and strengthen the urban manufacturing industry’s influential demonstration role in advancing the green transition, the Ministry of Industry and Information Technology of China successively launched 30 Made in China 2025 pilot cities (city clusters) in 2016 and 2017. The Pilot Demonstration Work Program for “Made in China 2025” Cities specified that significant results should be achieved within three to 5 years. After several years of implementation, has the Made in China 2025 pilot policy promoted green economic growth? What are the policy’s mechanisms of action? Are there differences in green economic growth effects in pilot cities based on various urban development characteristics? This study’s theoretical interpretation and empirical examination of the above questions can add to the growing body of related research and provide valuable insights for cities to comprehensively promote the transformation and upgrading of manufacturing industry to advance China’s high-quality development.
This study constructs an analytical framework at the theoretical level to analyze the impact of the Made in China 2025 strategy on urban green economic growth, and uses the double machine learning (ML) model to test its green economic growth effect. The contributions of this study are as follows. First, focusing on the field of urban green development, the study incorporates variables representing the potential economic and environmental effects of the Made in China 2025 policy into a unified framework to systematically examine the impact of the Made in China 2025 pilot policy on the urban green economic growth, providing a novel perspective for assessing the effects of industrial policies. Second, we investigate potential transmission mechanisms of the Made in China 2025 strategy affecting green economic growth from the perspectives of green technology advancement, energy consumption structure optimization, industrial structure upgrading, and environmental supervision strengthening, establishing a useful supplement for related research. Third, leveraging the advantage of ML algorithms in high-dimensional and nonparametric prediction, we apply a double ML model assess the policy effects of the Made in China 2025 strategy to avoid the “curse of dimensionality” and the inherent biases of traditional econometric models, and improve the credibility of our research conclusions.
The remainder of this paper is structured as follows. Section “ Literature review ” presents a literature review. Section “ Policy background and theoretical analysis ” details our theoretical analysis and research hypotheses. Section “ Empirical strategy ” introduces the model setting and variables selection for the study. Section “ Empirical result ” describes the findings of empirical testing and analyzes the results. Section “ Conclusion and policy recommendation ” summarizes our conclusions and associated policy implications.
Literature review
Measurement and influencing factors of green economic growth.
The Green Economy Report, which was published by the United Nations Environment Program in 2011, defined green economy development as facilitating more efficient use of natural resources and sustainable growth than traditional economic models, with a more active role in promoting combined economic development and environmental protection. The Organization for Economic Co-operation and Development defined green economic growth as promoting economic growth while ensuring that natural assets continue to provide environmental resources and services; a concept that is shared by a large number of institutions and scholars 7 , 8 , 9 . A considerable amount of research has assessed green economic growth, primarily using three approaches. First, single-factor indicators, such as sulfur dioxide emissions, carbon dioxide emissions intensity, and other quantified forms; however, this approach neglects the substitution of input factors such as capital and labor for the energy factor, which has certain limitations 5 , 10 . Second, studies have been based on neoclassical economic growth theory, incorporating factors of capital, technology, energy, and the environment, and constructing a green Solow model to measure green total factor productivity (GTFP) 11 , 12 . Third, based on neoclassical economic growth theory, some studies have simultaneously considered desirable and undesirable output, applying Shepard’s distance function, the directional distance function, and data envelopment analysis to measure GTFP 13 , 14 , 15 .
Economic growth is an extremely complex process, and green economic growth is also subject to a combination of multiple complex factors. Scholars have explored the influence mechanisms of green economic growth from perspectives of resource endowment 16 , technological innovation 17 , industrial structure 18 , human capital 19 , financial support 20 , government regulation 21 , and globalization 22 . In the field of policy effect assessment, previous studies have confirmed the green development effects of pilot policies such as innovative cities 23 , Broadband China 24 , smart cities 25 , and low-carbon cities 26 . However, few studies have focused on the impact of Made in China 2025 strategy on urban green economic growth and identified its underlying mechanisms.
The impact of Made in China 2025 strategy
Since the industrial policy of Made in China 2025 was proposed, scholars have predominantly focused on exploring its economic effects on technological innovation 27 , digital transformation 28 , and total factor productivity (TFP) 29 , while the potential environmental effects have been neglected. Chen et al. (2024) 30 found that Made in China 2025 promotes firm innovation through tax incentives, public subsidies, convenient financing, academic collaboration and talent incentives. Xu (2022) 31 point out that Made in China 2025 policy has the potential to substantially improve the green innovation of manufacturing enterprises, which can boost the green transformation and upgrading of China’s manufacturing industry. Li et al. (2024) 32 empirically investigates the positive effect of Made in China 2025 strategy on digital transformation and exploratory innovation in advanced manufacturing firms. Moreover, Liu and Liu (2023) 33 take “Made in China 2025” as an exogenous shock and find that the pilot policy has a positive impact on the high-quality development of enterprises and capital markets. Unfortunately, scholars have only discussed the impact of Made in China 2025 strategy on green development and environmental protection from a theoretical perspective and lack empirical analysis. Li (2018) 27 has compared Germany’s “Industry 4.0” and China’s “Made in China 2025”, and point out that “Made in China 2025” has clear goals, measures and sector focus. Its guiding principles are to enhance industrial capability through innovation-driven manufacturing, optimize the structure of Chinese industry, emphasize quality over quantity, train and attract talent, and achieve green manufacturing and environment. Therefore, it is necessary to systematically explore the impact and mechanism of Made in China 2025 strategy on urban green economic growth from both theoretical and empirical perspectives.
Causal inference based on double ML
The majority of previous studies have used traditional causal inference models to assess policy effects; however, some limitations are inherent to the application of these models. For example, the parallel trend test of the difference-in-differences model has stringent requirements on appropriate sample data; the synthetic control method can construct a virtual control group that conforms to the parallel trend, but it requires that the treatment group does not have the extreme value characteristics, and it is only applicable to “one-to-many” circumstances; and the propensity score matching (PSM) method involves a considerable amount of subjectivity in selecting matching variables. To compensate for the shortcomings of traditional models, scholars have started to explore the application of ML in the field of causal inference 34 , 35 , 36 , and double ML is a typical representative.
Double ML was formalized in 2018 34 , and the relevant research falls into two main categories. The first strand of literature applies double ML to assess causality concerning economic phenomena. Yang et al. (2020) 37 applied double ML using a gradient boosting algorithm to explore the average treatment effect of top-ranked audit firms, verifying its robustness compared with the PSM method. Zhang et al. (2022) 38 used double ML to quantify the impact of nighttime subway services on the nighttime economy, house prices, traffic accidents, and crime following the introduction of nighttime subway services in London in 2016. Farbmacher et al. (2022) 39 combined double ML with mediating effects analysis to assess the causal relationship between health insurance coverage and youth wellness and examine the indirect mechanisms of regular medical checkups, based on a national longitudinal health survey of youth conducted by the US Bureau of Labor Statistics. The second strand of literature has innovated methodological theory based on double ML. Chiang et al. (2022) 40 proposed an improved multidirectional cross-fitting double ML method, obtaining regression results for high-dimensional parameters while estimating robust standard errors for dual clustering, which can effectively adapt to multidirectional clustered sampled data and improve the validity of estimation results. Bodory et al. (2022) 41 combined dynamic analysis with double ML to measure the causal effects of multiple treatment variables over time, using weighted estimation to assess the dynamic treatment effects of specific subsamples, which enriched the dynamic quantitative extension of double ML.
In summary, previous research has conducted some useful investigations regarding the impact of socioeconomic policies on green development, but limited studies have explored the relationship between the Made in China 2025 strategy and green economic growth. This study takes 281 Chinese cities as the research object, and applies the super-slacks-based measure (SBM) model to quantify Chinese cities’ green economic growth from 2006 to 2021. Based on a quasi-natural experiment of Made in China 2025 pilot policy implementation, we use the double ML model to test the impact and transmission mechanisms of the policy on urban green economic growth. We also conduct a heterogeneity analysis of cities based on different levels of manufacturing agglomeration, industrial intelligence, and digital finance. This study applies a novel approach and provides practical insights for research in the field of industrial policy assessment.
Policy background and theoretical analysis
Policy background.
The Made in China 2025 strategy aims to encourage and support local exploration of new paths and models for the transformation and upgrading of the manufacturing industry, and to drive the improvement of manufacturing quality and efficiency in other regions through demonstration effects. According to the Notice of Creating “Made in China 2025” National Demonstration Zones issued by the State Council, municipalities directly under the central government, sub-provincial cities, and prefecture-level cities can apply for the creation of demonstration zones. Cities with proximity and high industrial correlation can jointly apply for urban agglomeration demonstration zones. The Notice clarifies the goals and requirements for creating demonstration zones in areas such as green manufacturing, clean production, and environmental protection. In 2016, Ningbo became the first Made in China 2025 pilot city, and a total of 12 cities and 4 city clusters were included in the list of Made in China 2025 national demonstration zones. In 2018, the State Council issued the Evaluation Guidelines for “Made in China 2025” National Demonstration Zone, which further clarified the evaluation process and indicator system of the demonstration zone. Seven primary indicators and 29 secondary indicators were formulated, including innovation driven, quality first, green development, structural optimization, talent oriented, organizational implementation, and coordinated development of urban agglomerations. This indicator system can evaluate the creation process and overall effectiveness of pilot cities (city clusters), which is beneficial for the promotion of successful experiences and models in demonstration areas.
Advancing green urban development is a complex systematic project that requires structural adjustment and technological and institutional changes in the socioeconomic system 42 . The Made in China 2025 strategy emphasizes the development and application of smart and green manufacturing systems, which can unblock technological bottlenecks in the manufacturing sector in terms of industrial production, energy consumption, and waste emissions, and empower cities to operate in a green manner. In addition, the Made in China 2025 policy established requirements for promoting technological innovation to advance energy saving and environmental protection, improving the rate of green energy use, transforming traditional industries, and strengthening environmental supervision. For pilot cities, green economy development requires the support of a full range of positive factors. Therefore, this study analyzes the mechanisms by which the Made in China 2025 strategy affects urban green economic growth from the four paths of green technology advancement, energy consumption structure optimization, industrial structure upgrading, and environmental supervision strengthening.
Theoretical analysis and research hypotheses
As noted, the Made in China 2025 strategy emphasizes strengthening the development and application of energy-saving and environmental protection technologies to advance cleaner production. Pilot cities are expected to prioritize the driving role of green innovation, promote clustering carriers and innovation platforms for high-tech enterprises, and guide the progress of enterprises’ implementation of green technology. Specifically, pilot cities are encouraged to optimize the innovation environment by increasing scientific and technological investment and financial subsidies in key areas such as smart manufacturing and high-end equipment and strengthening intellectual property protection to incentivize enterprises to conduct green research and development (R&D) activities. These activities subsequently promote the development of green innovation technologies and industrial transformation 43 . Furthermore, since quality human resources are a core aspect of science and technology innovation 44 , pilot cities prioritize the cultivation and attraction of talent to establish a stable human capital guarantee for enterprises’ ongoing green technology innovation, transform and upgrade the manufacturing industry, and advance green urban development. Green technology advances also contribute to urban green economic growth. First, green technology facilitates enterprises’ adoption of improved production equipment and innovation in green production technology, accelerating the change of production mode and driving the transformation from traditional crude production to a green and intensive approach 45 , promoting green urban development. Second, green technology advancement accelerates green innovations such as clean processes, pollution control technologies, and green equipment, and facilitates the effective supply of green products, taking full advantage of the benefits of green innovations 46 and forming a green economic development model to achieve urban green economic growth.
The Made in China 2025 pilot policy endeavors to continuously increase the rate of green and low-carbon energy use and reduce energy consumption. Under target constraints of energy saving and carbon control, pilot cities will accelerate the cultivation of high-tech industries in green environmental protection and high-end equipment manufacturing with advantages of sustainability and low resource inputs 47 to improve the energy consumption structure. Pilot cities also advance new energy sector development by promoting clean energy projects, subsidizing new energy consumption, and supporting green infrastructure construction and other policy measures 48 to optimize the energy consumption structure. Energy consumption structure optimization can have a profound impact on green economy development. Optimization means that available energy tends to be cleaner, which can reduce the manufacturing industry’s dependence on traditional fossil energy and raise the proportion of clean energy 49 , ultimately promoting green urban development. Pilot cities also provide financial subsidies for new energy technology R&D, which promotes the innovation and application of new technologies, energy-saving equipment, efficient resource use, and energy-saving diagnostics, which allow enterprises to save energy and reduce consumption and improve energy use efficiency and TFP 50 , advancing the growth of urban green economy.
At its core, the Made in China 2025 strategy promotes the transformation and upgrading of the manufacturing sector. Pilot cities guide and develop technology-intensive high-tech industries, adjust the proportion of traditional heavy industry, and improve the urban industrial structure. Pilot cities also implement the closure, merger, and transformation of pollution-intensive industries; guide the fission of professional advantages of manufacturing enterprises 51 ; and expand the establishment and development of service-oriented manufacturing and productive service industries to promote the evolution of the industrial structure toward rationalization and high-quality development 52 . Upgrading the industrial structure can also contribute to urban green economic growth. First, industrial structure upgrading promotes the transition from labor- and capital-intensive industries to knowledge- and technology-intensive industries, which optimizes the industrial distribution patterns of energy consumption and pollutant emissions and promotes the transformation of economic growth dynamics and pollutant emissions control, providing a new impetus for cities’ sustainable development 53 . Second, changes in industrial structure and scale can have a profound impact on the type and quantity of pollutant emissions. By introducing high-tech industries, service-oriented manufacturing, and production-oriented service industries, pilot cities can promote the transformation of pollution-intensive industries, promoting the adjustment and optimization of industrial structure and scale 54 to achieve the purpose of driving green urban development.
The Made in China 2025 strategy proposes strengthening green supervision and conducting green evaluations, establishing green development goals for the manufacturing sector in terms of emissions and consumption reduction and water conservation. This requires pilot cities to implement stringent environmental regulatory policies, such as higher energy efficiency and emissions reduction targets and sewage taxes and charges, strict penalties for excess emissions, and project review criteria 55 , which consolidates the effectiveness of green development. Under the framework of environmental authoritarianism, strengthening environmental supervision is a key measure for achieving pollution control and improving environmental quality 56 . Therefore, environmental regulatory enhancement can help cities achieve green development goals. First, according to the Porter hypothesis 57 , strong environmental regulatory policies encourage firms to internalize the external costs of environmental supervision, stimulate technological innovation, and accelerate R&D and application of green technologies. This response helps enterprises improve input–output efficiency, achieve synergy between increasing production and emissions reduction, partially or completely offset the “environmental compliance cost” from environmental supervision, and realize the innovation compensation effect 58 . Second, strict environmental regulations can effectively mitigate the complicity of local governments and enterprises in focusing on economic growth while neglecting environmental protection 59 , urging local governments to constrain enterprises’ emissions, which compels enterprises to conduct technological innovation and pursue low-carbon transformation, promoting urban green economic growth.
Based on the above analysis, we propose the mechanisms that promote green economic growth through Made in China 2025 strategy, as shown in Fig. 1 . The proposed research hypotheses are as follows:
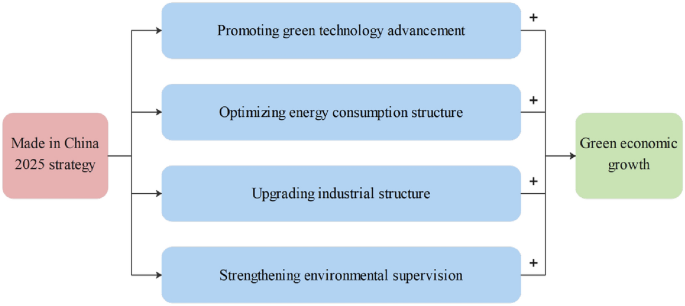
Mechanism analysis of Made in China 2025 strategy and green economic growth.
Hypothesis 1
The Made in China 2025 strategy promotes urban green economic growth.
Hypothesis 2
The Made in China 2025 strategy drives urban green economic growth through four channels: promoting green technology advancement, optimizing energy consumption structure, upgrading industrial structure, and strengthening environmental supervision.
Empirical strategy
Double ml model.
Compared with traditional causal inference models, double ML has unique advantages in variable selection and model estimation, and is also more applicable to the research problem of this study. Green economic growth is a comprehensive indicator of transformative urban growth that is influenced by many socioeconomic factors. To ensure the accuracy of our policy effects estimation, the interference of other factors on urban green economic growth must be controlled as much as possible; however, when introducing high-dimensional control variables, traditional regression models may face the “curse of dimensionality” and multicollinearity, rendering the accuracy of the estimates questionable. Double ML uses ML and regularization algorithms to automatically filter the preselected set of high-dimensional control variables to obtain an effective set of control variables with higher prediction accuracy. This approach avoids the “curse of dimensionality” caused by redundant control variables and mitigates the estimation bias caused by the limited number of primary control variables 39 . Furthermore, nonlinear relationships between variables are the norm in the evolution of economic transition, and ordinary linear regression may suffer from model-setting bias producing estimates that lack robustness. Double ML effectively overcomes the problem of model misspecification by virtue of the advantages of ML algorithms in handling nonlinear data 37 . In addition, based on the idea of instrumental variable functions, two-stage predictive residual regression, and sample split fitting, double ML mitigates the “regularity bias” in ML estimation and ensures unbiased estimates of the treatment coefficients in small samples 60 .
Based on the analysis above, this study uses the double ML model to assess the policy effects of the Made in China 2025 strategy. The partial linear double ML model is constructed as follows:
where i denotes the city, t denotes the year, and Y it represents green economic growth. Policy it represents the policy variable of Made in China 2025, which is set as 1 if the pilot is implemented and 0 otherwise. θ 0 is the treatment coefficient that is the focus of this study. X it denotes the set of high-dimensional control variables, and the ML algorithm is used to estimate the specific functional form \(\hat{g}(X_{it} )\) . U it denotes the error term with a conditional mean of zero.
Direct estimation of Eqs. ( 1 ) and ( 2 ) yields the following estimate of the treatment coefficient:
where n denotes the sample size.
Notably, the double ML model uses a regularization algorithm to estimate the specific functional form \(\hat{g}(X_{it} )\) , which prevents the variance of the estimate from being too large, but inevitably introduces a “regularity bias,” resulting in a biased estimate. To speed up the convergence of the \(\hat{g}(X_{it} )\) directions so that the estimates of the treatment coefficients satisfy unbiasedness with small samples, the following auxiliary regression is constructed:
where \(m(X_{it} )\) is the regression function of the treatment variable on the high-dimensional control variable, using ML algorithms to estimate the specific functional form \(\hat{m}(X_{it} )\) . V it is the error term with a conditional mean of zero.
The specific operation process follows three stages. First, we use the ML algorithm to estimate the auxiliary regression \(\hat{m}(X_{it} )\) and take its residuals \(\hat{V}_{it} = Policy_{it} - \hat{m}(X_{it} )\) . Second, we use the ML algorithm to estimate \(\hat{g}(X_{it} )\) and change the form of the main regression \(Y_{it} - \hat{g}(X_{it} ) = \theta_{0} Policy_{it} + U_{it}\) . Finally, we regress \(\hat{V}_{it}\) as an instrumental variable for Policy it , obtaining unbiased estimates of the treatment coefficients as follows:
Variable selection
- Green economic growth
We apply the super-SBM model to measure urban green economic growth. The super-SBM model is compatible with radial and nonradial characteristics, which avoids inflated results due to ignoring slack variables and deflated results due to ignoring the linear relationships between elements, and can truly reflect relative efficiency 61 . The SBM model reflects the nature of green economic growth more accurately compared with other models, and has been widely adopted by scholars 62 . The expression of the super-SBM model considering undesirable output is as follows:
where x is the input variable; y and z are the desirable and undesirable output variables, respectively; m denotes the number of input indicators; s 1 and s 2 represent the respective number of indicators for desirable and undesirable outputs; k denotes the period of production; i , r , and t are the decision units for the inputs, desirable outputs, and undesirable outputs, respectively; \(s^{ - }\) , \(s^{ + }\) , and \(s^{z - }\) are the respective slack variables for the inputs, desirable outputs, and undesirable outputs; and γ is a vector of weights. A larger \(\rho_{SE}\) value indicates greater efficiency. If \(\rho_{SE}\) = 1, the decision unit is effective; if \(\rho_{SE}\) < 1, the decision unit is relatively ineffective, indicating a loss of efficiency.
Referencing Sarkodie et al. (2023) 63 , the evaluation index system of green economic growth is constructed as shown in Table 1 .
Made in China 2025 pilot policy
The list of Made in China 2025 pilot cities (city clusters) published by the Ministry of Industry and Information Technology of China in 2016 and 2017 is matched with the city-level data to obtain 30 treatment group cities and 251 control group cities. The policy dummy variable of Made in China 2025 is constructed by combining the implementation time of the pilot policies.
Mediating variables
This study also examines the transmission mechanism of the Made in China 2025 strategy affecting urban green economic growth from four perspectives, including green technology advancement, energy consumption structure optimization, industrial structure upgrading, and strengthening of environmental supervision. (1) The number of green patent applications is adopted to reflect green technology advancement. (2) Energy consumption structure is quantified using the share of urban domestic electricity consumption in total energy consumption. (3) The industrial structure upgrading index is calculated using the formula \(\sum\nolimits_{i = 1}^{3} {i \times (GDP_{i} /GDP)}\) , where GDP i denotes the added value of primary, secondary, or tertiary industries. (4) The frequency of words related to the environment in government work reports is the proxy for measuring the intensity of environmental supervision 64 .
Control variables
Double ML can effectively accommodate the case of high-dimensional control variables using regularization algorithms. To control for the effect of other urban characteristics on green economic growth, this study introduces the following 10 control variables. We measure education investment by the ratio of education expenditure to GDP. Technology investment is the ratio of technology expenditure to GDP. The study measures urbanization using the share of urban built-up land in the urban area. Internet penetration is the number of internet users as a share of the total population at the end of the year. We measure resident consumption by the total retail sales of consumer goods per capita. The unemployment rate is the ratio of the number of registered unemployed in urban areas at the end of the year to the total population at the end of the year. Financial scale is the ratio of the balance of deposits and loans of financial institutions at the end of the year to the GDP. Human capital is the natural logarithm of the number of students enrolled in elementary school, general secondary schools, and general tertiary institutions per 10,000 persons. Transportation infrastructure is the natural logarithm of road and rail freight traffic. Finally, openness to the outside world is reflected by the ratio of actual foreign investment to GDP. Quadratic terms for the control variables are also included in the regression analysis to improve the accuracy of the model’s fit. We introduce city and time fixed effects as individual and year dummy variables to avoid missing information on city and time dimensions.
Data sources
This study uses 281 Chinese cities spanning from 2006 to 2021 as the research sample. Data sources include the China City Statistical Yearbook, the China Economic and Social Development Statistics Database, and the EPS Global Statistics Database. We used the average annual growth rate method to fill the gaps for the minimal missing data. To remove the effects of price changes, all data measured in monetary units are deflated using the consumer price index for each province for the 2005 base period. The descriptive statistics of the data are presented in Table 2 .
Empirical result
Baseline results.
The sample split ratio of the double ML model is set to 1:4, and we use the Lasso algorithm to predict and solve the main and auxiliary regressions, presenting the results in Table 3 . Column (1) does not control for fixed effects or control variables, column (2) introduces city and time fixed effects, and columns (3) and (4) add control variables to columns (1) and (2), respectively. The regressions in columns (1) and (2) are highly significant, regardless of whether city and time fixed effects are controlled. Column (4) controls for city fixed effects, time fixed effects, and the primary term of the control variable over the full sample interval, revealing that the regression coefficient of the Made in China 2025 pilot policy on green economic growth is positive and significant at the 1% level, confirming that the Made in China 2025 strategy significantly promotes urban green economic growth. Column (5) further incorporates the quadratic terms of the control variables and the regression coefficients remain significantly positive with little change in values. Therefore, Hypothesis 1 is verified.
Parallel trend test
The prerequisite for the establishment of policy evaluation is that the development status of cities before the pilot policy is introduced is similar. Referring to Liu et al. (2022) 29 , we adopt a parallel trend test to verify the effectiveness of Made in China 2025 pilot policy. Figure 2 shows the result of parallel trend test. None of the coefficient estimates before the Made in China 2025 pilot policy are significant, indicating no significant difference between the level of green economic growth in pilot and nonpilot cities before implementing the policy, which passes the parallel trend test. The coefficient estimates for all periods after the policy implementation are significantly positive, indicating that the Made in China 2025 pilot policy can promote urban green economic growth.
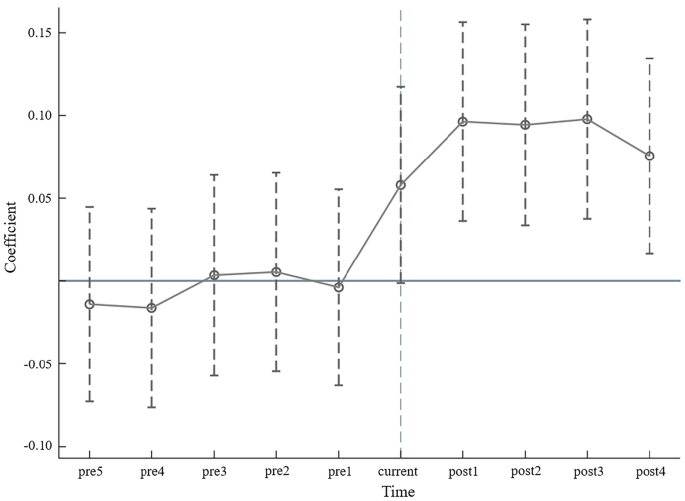
Parallel trend test.
Robustness tests
Replace explained variable.
Referencing Oh and Heshmati (2010) 65 and Tone and Tsutsui (2010) 66 , we use the Malmquist–Luenberger index under global production technology conditions (GML) and an epsilon-based measure (EBM) model to recalculate urban green economic growth. The estimation results in columns (1) and (2) of Table 4 show that the estimated coefficients of the Made in China 2025 pilot policy remain significantly positive after replacing the explanatory variables, validating the robustness of the baseline findings.
Adjusting the research sample
Considering the large gaps in the manufacturing development base between different regions in China, using all cities in the regression analysis may lead to biased estimation 67 . Therefore, we exclude cities in seven provinces with a poor manufacturing development base (Gansu, Qinghai, Ningxia, Xinjiang, Tibet, Yunnan, and Guizhou) and four municipalities with a better development base (Beijing, Tianjin, Shanghai, and Chongqing). The other city samples are retained to rerun the regression analysis, and the results are presented in column (3) of Table 4 . The first batch of pilot cities of the Made in China 2025 strategy was released in 2016, and the second batch of pilot cities was released in 2017. To exclude the effect of point-in-time samples that are far from the time of policy promulgation, the regression is also rerun by restricting the study interval to the three years before and after the promulgation of the policy (2013–2020), and the results are presented in column (4) of Table 4 . The coefficients of the Made in China 2025 pilot policy effect on urban green economic growth decrease after adjusting for the city sample and the time interval, but remain significantly positive at the 1% level. This, once again, verifies the robustness of the benchmark regression results.
Eliminating the impact of potential policies
During the same period of the Made in China 2025 strategy implementation, urban green economy growth may be affected by other relevant policies. To ensure the accuracy of the policy effect estimates, four representative policy categories overlapping with the sample period, including smart cities, low-carbon cities, Broadband China, and innovative cities, were collected and organized. Referencing Zhang and Fan (2023) 25 , dummy variables for these policies are included in the benchmark regression model and the results are presented in Table 5 . The estimated coefficient of the Made in China 2025 pilot policy decreases after controlling for the effects of related policies, but remains significantly positive at the 1% level. This suggests that the positive impact of the Made in China 2025 strategy on urban green economic growth, although overestimated, does not affect the validity of the study’s findings.

Reset double ML model
To avoid the impact of the double ML model imparting bias on the conclusions, we conduct robustness tests by varying the sample splitting ratio, the ML algorithm, and the model estimation form. First, we change the sample split ratio of the double ML model from 1:4 to 3:7 and 1:3. Second, we replace the Lasso ML algorithm with random forest (RF), gradient boosting (GBT), and BP neural network (BNN). Third, we replace the partial linear model based on the dual ML with a more generalized interactive model, using the following main and auxiliary regressions for the analysis:
among them, the meanings of each variable are the same as Eqs. ( 1 ) and ( 2 ).
The estimated coefficients for the treatment effects are obtained from the interactive model as follows:
Table 6 presents the regression results after resetting the double ML model, revealing that the sample split ratio, ML algorithm, and the model estimation form in double ML model did not affect the conclusion that the Made in China 2025 strategy promotes urban green economic growth, and only alters the magnitude of the policy effect, once again validating the robustness of our conclusions.
Difference-in-differences model
To further verify the robustness of the estimation results, we use traditional econometric models for regression. Based on the difference-in-differences (DID) model, a synthetic difference-in-differences (SDID) model is constructed by combining the synthetic control method 68 . It constructs a composite control group with a similar pre-trend to the treatment group by linearly combining several individuals in the control group, and compares it with the treatment group 69 . Table 7 presents the regression results of traditional DID model and SDID model. The estimated coefficient of the Made in China 2025 policy remains significantly positive at the 1% level, which once again verifies the robustness of the study’s findings.
Mechanism verification
This section conducts mechanism verification from four perspectives of green technology advancement, energy consumption structure, industrial structure, and environmental supervision. The positive impacts of the Made in China 2025 strategy on green technology advancement, energy consumption structure optimization, industrial structure upgrading, and strengthening environmental supervision are empirically examined using a dual ML model (see Table A.1 in the Online Appendix for details). Referencing Farbmacher et al. (2022) 39 for causal mediating effect analysis of double ML (see the Appendix for details), we test the transmission mechanism of the Made in China 2025 strategy on green economic growth based on the Lasso algorithm, presenting the results in Table 8 . The findings show that the total effects under different mediating paths are all significantly positive at the 1% level, verifying that the Made in China 2025 strategy positively promotes urban green economic growth.
Mechanism of green technology advancement
The indirect effect of green technological innovation is significantly positive for both the treatment and control groups. After stripping out the path of green technology advancement, the direct effects of the treatment and control groups remain significantly positive, indicating that the increase in the level of green technological innovation brought about by the Made in China 2025 strategy significantly promotes urban green economic growth. The Made in China 2025 strategy proposes to strengthen financial and tax policy support, intellectual property protection, and talent training systems. Through the implementation of policy incentives, pilot cities have fostered the concentration of high-technology enterprises and scientific and technological talent cultivation, exerting a knowledge spillover effect that further promotes green technology advancement. At the same time, policy preferences have stimulated the demand for innovation in energy conservation and emissions reduction, which raises enterprises’ motivation to engage in green innovation activities. Green technology advancement helps cities achieve an intensive development model, bringing multiple dividends such as lower resource consumption, reduced pollution emissions, and improved production efficiency, which subsequently promotes green economic growth.
Mechanism of energy consumption structure
The indirect effect of energy consumption structure is significantly positive for the treatment and control groups, while the direct effect of the Made in China 2025 pilot policy on green economic growth remains significantly positive, indicating that the policy promotes urban green economic growth through energy consumption structure optimization. The policy encourages the introduction of clean energy into production processes, reducing pressure on enterprise performance and the cost of clean energy use, which helps enterprises to reduce traditional energy consumption that is dominated by coal and optimize the energy structure to promote green urban development.
Mechanism of industrial structure
The indirect effects of industrial structure on the treatment and control groups are significantly positive. After stripping out the path of industrial structure upgrading, the direct effects remain significantly positive for both groups, indicating that the Made in China 2025 strategy promotes urban green economic growth through industrial structure optimization. Deepening the restructuring of the manufacturing industry is a strategic task specified in Made in China 2025. Pilot cities focus on transforming and guiding the traditional manufacturing industry toward high-end, intelligent equipment upgrades and digital transformation, driving the regional industrial structure toward rationalization and advancement to achieve rational allocation of resources. Upgrading industrial structure is a prerequisite for cities to advance intensive growth and sustainable development. By assuming the roles of “resource converter” and “pollutant controller,” industrial upgrading can continue to release the dividends of industrial structure, optimize resource allocation, and improve production efficiency, establishing strong support for green economic growth.
Mechanism of environmental supervision
The treatment and control groups of environmental supervision has a positive indirect effect in the process of the Made in China 2025 pilot policy affecting green economic growth that is significant at the 1% level, affirming the transmission path of environmental supervision. The Made in China 2025 strategy states that energy consumption, material consumption, and pollutant emissions per unit of industrial added value in key industries should reach the world’s advanced level by 2025. This requires pilot cities to consolidate and propagate the effectiveness of green development by strengthening environmental supervision while promoting the manufacturing sector’s green development. Strengthening environmental supervision promotes enterprises’ energy saving and emissions reduction through innovative compensation effects, while restraining enterprises’ emissions behaviors by tightening environmental protection policies, promoting environmental legislation, and increasing penalties to advance green urban development. Based on the above analysis, Hypothesis 2 is validated.
Heterogeneity analysis
Heterogeneity of manufacturing agglomeration.
To reduce production and transaction costs and realize economies of scale and scope, the manufacturing industry tends to accelerate its growth through agglomeration, exerting an “oasis effect” 70 . Cities with a high degree of manufacturing agglomeration are prone to scale and knowledge spillover effects, which amplify the agglomeration functions of talent, capital, and technology, strengthening the effectiveness of pilot policies. Based on this, we use the locational entropy of manufacturing employees to measure the degree of urban manufacturing agglomeration in the year (2015) before policy implementation, using the median to divide the full sample of cities into high and low agglomeration groups. Columns (1) and (2) in Table 9 reveal that the Made in China 2025 pilot policy has a stronger effect in promoting green economic growth in cities with high manufacturing concentration compared to those with low concentration. The rationale for this outcome may be that cities with a high concentration of manufacturing industries has large population and developed economy, which is conducive to leveraging agglomeration economies and knowledge spillover effects. Meanwhile, they are able to offer greater policy concessions by virtue of economic scale, public services, infrastructure, and other advantages. These benefits can attract the clustering of productive services and the influx of innovative elements such as R&D talent, accelerating the transformation and upgrading of the manufacturing industry and the integration and advancement of green technologies, empowering the green urban development.
Heterogeneity of industrial intelligence
As a landmark technology for the integration of the new scientific and technological revolution with manufacturing, industrial intelligence is a new approach for advancing the green transformation of manufacturing production methods. Based on this, we use the density of industrial robot installations to measure the level of industrial intelligence in cities in the year (2015) prior to policy implementation 71 , using the median to classify the full sample of cities into high and low level groups. Columns (3) and (4) in Table 9 reveals that the Made in China 2025 pilot policy has a stronger driving effect on the green economic growth of highly industrial intelligent cities. The rationale for this outcome may be that with the accumulation of smart factories, technologies, and equipment, a high degree of industrial intelligence is more likely to leverage the green development effects of pilot policies. For cities where the development of industrial intelligence is in its infancy or has not yet begun, the cost of information and knowledge required for enterprises to undertake technological R&D is higher, reducing the motivation and incentive to conduct innovative activities, diminishing the pilot policy’s contribution to green economic growth.
Heterogeneity of digital finance
As a fusion of traditional finance and information technology, digital finance has a positive impact on the development of the manufacturing industry by virtue of its advantages of low financing thresholds, fast mobile payments, and wide range of services 72 . Cities with a high degree of digital finance development have abundant financial resources and well-developed financial infrastructure that provide enterprises with more complete financial services, with subsequent influence on the effects of pilot policies. We use the Peking University Digital Inclusive Finance Index to measure the level of digital financial development in cities in the year (2015) prior to policy implementation, using the median to divide the full sample of cities into high and low level groups. Columns (5) and (6) in Table 9 reveal that the Made in China 2025 pilot policy has a stronger driving effect on the green economic growth of cities with highly developed digital finance. The rationale for this outcome may be that cities with a high degree of digital finance development can fully leverage the universality of financial resources, provide financial supply for environmentally friendly and technology-intensive enterprises, effectively alleviate the mismatch of financial capital supply, and provide financial security for enterprises to conduct green technology R&D. Digital finance also makes enterprises’ information more transparent through a rich array of data access channels, which strengthens government pollution regulation and public environmental supervision and compels enterprises to engage in green technological innovation to promote green economic growth.
Conclusion and policy recommendation
Conclusions.
This study examines the impact of the Made in China 2025 strategy on urban green economic growth using the double ML model based on panel data for 281 Chinese cities from 2006 to 2021. The relevant research results are threefold. First, the Made in China 2025 strategy significantly promotes urban green economic growth; a conclusion that is supported by a series of robustness tests. Second, regarding mechanisms, the Made in China 2025 strategy promotes urban green economic growth through green technology advancement, energy consumption structure optimization, industrial structure upgrading, and strengthening of environmental supervision. Third, the heterogeneity analysis reveals that the Made in China 2025 strategy has a stronger driving effect on green economic growth for cities with a high concentration of manufacturing and high degrees of industrial intelligence and digital finance.
policy recommendations
We next propose specific policy recommendations based on our findings. First, policymakers should summarize the experience of building pilot cities and create a strategic model to advance the transformation and upgrading of the manufacturing industry to drive green urban development. The Made in China 2025 pilot policy effectively promotes green economic growth and highlights the significance of the transformation and upgrading of the manufacturing industry to empower sustainable urban development. The government should strengthen the model and publicize summaries of successful cases of manufacturing development in pilot cities to promote the experience of manufacturing transformation and upgrading by producing typical samples to guide the transformation of the manufacturing industry to intelligence and greening. Policies should endeavor to optimize the industrial structure and production system of the manufacturing industry to create a solid real economy support for high-quality urban development.
Second, policymakers should explore the multidimensional driving paths of urban green economic growth and actively stimulate the green development dividend of pilot policies by increasing support for enterprise-specific technologies, subsidizing R&D in areas of energy conservation and emissions reduction, consumption reduction and efficiency, recycling and pollution prevention, and promoting the progress of green technologies. The elimination of outdated production capacity must be accelerated and the low-carbon transformation of traditional industries must be targeted, while guiding the clustering of high-tech industries, optimizing cities’ industrial structure, and driving industrial structure upgrading. Policymakers can regulate enterprises’ production practices and enhance the effectiveness of environmental supervision by improving the system of environmental information disclosure and mechanisms of rewards and penalties for pollution discharge. In addition, strategies should consider cities’ own resource endowment, promote large-scale production of new energy, encourage enterprises to increase the proportion of clean energy use, and optimize the structure of energy consumption.
Third, policymakers should engage a combination of urban development characteristics and strategic policy implementation to empower green urban development, actively promoting optimization of manufacturing industry structure, and accelerating the development of high-technology industries under the guidance of policies and the market to promote high-quality development and agglomeration of the manufacturing industry. At the same time, the government should strive to popularize the industrial internet, promote the construction of smart factories and the application of smart equipment, increase investment in R&D to advance industrial intelligence, and actively cultivate new modes and forms of industrial intelligence. In addition, new infrastructure construction must be accelerated, the application of information technology must be strengthened, and digital financial services must be deepened to ease the financing constraints for enterprises conducting R&D on green technologies and to help cities develop in a high-quality manner.
Data availability
The datasets used or analysed during the current study are available from the corresponding author on reasonable request.
Cheng, K. & Liu, S. Does urbanization promote the urban–rural equalization of basic public services? Evidence from prefectural cities in China. Appl. Econ. 56 (29), 3445–3459. https://doi.org/10.1080/00036846.2023.2206625 (2023).
Article Google Scholar
Yin, X. & Xu, Z. An empirical analysis of the coupling and coordinative development of China’s green finance and economic growth. Resour. Policy 75 , 102476. https://doi.org/10.1016/j.resourpol.2021.102476 (2022).
Fernandes, C. I., Veiga, P. M., Ferreira, J. J. M. & Hughes, M. Green growth versus economic growth: Do sustainable technology transfer and innovations lead to an imperfect choice?. Bus. Strateg. Environ. 30 (4), 2021–2037. https://doi.org/10.1002/bse.2730 (2021).
Orsatti, G., Quatraro, F. & Pezzoni, M. The antecedents of green technologies: The role of team-level recombinant capabilities. Res. Policy 49 (3), 103919. https://doi.org/10.1016/j.respol.2019.103919 (2020).
Lin, B. & Zhou, Y. Measuring the green economic growth in China: Influencing factors and policy perspectives. Energy 241 (15), 122518. https://doi.org/10.1016/j.energy.2021.122518 (2022).
Fang, M. & Chang, C. L. Nexus between fiscal imbalances, green fiscal spending, and green economic growth: Empirical findings from E-7 economies. Econ. Change Restruct. 55 , 2423–2443. https://doi.org/10.1007/s10644-022-09392-6 (2022).
Qian, Y., Liu, J. & Forrest, J. Y. L. Impact of financial agglomeration on regional green economic growth: Evidence from China. J. Environ. Plan. Manag. 65 (9), 1611–1636. https://doi.org/10.1080/09640568.2021.1941811 (2022).
Awais, M., Afzal, A., Firdousi, S. & Hasnaoui, A. Is fintech the new path to sustainable resource utilisation and economic development?. Resour. Policy 81 , 103309. https://doi.org/10.1016/j.resourpol.2023.103309 (2023).
Ahmed, E. M. & Elfaki, K. E. Green technological progress implications on long-run sustainable economic growth. J. Knowl. Econ. https://doi.org/10.1007/s13132-023-01268-y (2023).
Shen, F. et al. The effect of economic growth target constraints on green technology innovation. J. Environ. Manag. 292 (15), 112765. https://doi.org/10.1016/j.jenvman.2021.112765 (2021).
Zhao, L. et al. Enhancing green economic recovery through green bonds financing and energy efficiency investments. Econ. Anal. Policy 76 , 488–501. https://doi.org/10.1016/j.eap.2022.08.019 (2022).
Ferreira, J. J. et al. Diverging or converging to a green world? Impact of green growth measures on countries’ economic performance. Environ. Dev. Sustain. https://doi.org/10.1007/s10668-023-02991-x (2023).
Article PubMed PubMed Central Google Scholar
Song, X., Zhou, Y. & Jia, W. How do economic openness and R&D investment affect green economic growth?—Evidence from China. Resour. Conserv. Recycl. 149 , 405–415. https://doi.org/10.1016/j.resconrec.2019.03.050 (2019).
Xu, J., She, S., Gao, P. & Sun, Y. Role of green finance in resource efficiency and green economic growth. Resour. Policy 81 , 103349 (2023).
Zhou, Y., Tian, L. & Yang, X. Schumpeterian endogenous growth model under green innovation and its enculturation effect. Energy Econ. 127 , 107109. https://doi.org/10.1016/j.eneco.2023.107109 (2023).
Luukkanen, J. et al. Resource efficiency and green economic sustainability transition evaluation of green growth productivity gap and governance challenges in Cambodia. Sustain. Dev. 27 (3), 312–320. https://doi.org/10.1002/sd.1902 (2019).
Wang, K., Umar, M., Akram, R. & Caglar, E. Is technological innovation making world “Greener”? An evidence from changing growth story of China. Technol. Forecast. Soc. Change 165 , 120516. https://doi.org/10.1016/j.techfore.2020.120516 (2021).
Talebzadehhosseini, S. & Garibay, I. The interaction effects of technological innovation and path-dependent economic growth on countries overall green growth performance. J. Clean. Prod. 333 (20), 130134. https://doi.org/10.1016/j.jclepro.2021.130134 (2022).
Ge, T., Li, C., Li, J. & Hao, X. Does neighboring green development benefit or suffer from local economic growth targets? Evidence from China. Econ. Modell. 120 , 106149. https://doi.org/10.1016/j.econmod.2022.106149 (2023).
Lin, B. & Zhu, J. Fiscal spending and green economic growth: Evidence from China. Energy Econ. 83 , 264–271. https://doi.org/10.1016/j.eneco.2019.07.010 (2019).
Sohail, M. T., Ullah, S. & Majeed, M. T. Effect of policy uncertainty on green growth in high-polluting economies. J. Clean. Prod. 380 (20), 135043. https://doi.org/10.1016/j.jclepro.2022.135043 (2022).
Sarwar, S. Impact of energy intensity, green economy and blue economy to achieve sustainable economic growth in GCC countries: Does Saudi Vision 2030 matters to GCC countries. Renew. Energy 191 , 30–46. https://doi.org/10.1016/j.renene.2022.03.122 (2022).
Park, J. & Page, G. W. Innovative green economy, urban economic performance and urban environments: An empirical analysis of US cities. Eur. Plann. Stud. 25 (5), 772–789. https://doi.org/10.1080/09654313.2017.1282078 (2017).
Feng, Y., Chen, Z. & Nie, C. The effect of broadband infrastructure construction on urban green innovation: Evidence from a quasi-natural experiment in China. Econ. Anal. Policy 77 , 581–598. https://doi.org/10.1016/j.eap.2022.12.020 (2023).
Zhang, X. & Fan, D. Collaborative emission reduction research on dual-pilot policies of the low-carbon city and smart city from the perspective of multiple innovations. Urban Climate 47 , 101364. https://doi.org/10.1016/j.uclim.2022.101364 (2023).
Cheng, J., Yi, J., Dai, S. & Xiong, Y. Can low-carbon city construction facilitate green growth? Evidence from China’s pilot low-carbon city initiative. J. Clean. Prod. 231 (10), 1158–1170. https://doi.org/10.1016/j.jclepro.2019.05.327 (2019).
Li, L. China’s manufacturing locus in 2025: With a comparison of “Made-in-China 2025” and “Industry 4.0”. Technol. Forecast. Soc. Change 135 , 66–74. https://doi.org/10.1016/j.techfore.2017.05.028 (2018).
Wang, J., Wu, H. & Chen, Y. Made in China 2025 and manufacturing strategy decisions with reverse QFD. Int. J. Prod. Econ. 224 , 107539. https://doi.org/10.1016/j.ijpe.2019.107539 (2020).
Liu, X., Megginson, W. L. & Xia, J. Industrial policy and asset prices: Evidence from the Made in China 2025 policy. J. Bank. Finance 142 , 106554. https://doi.org/10.1016/j.jbankfin.2022.106554 (2022).
Chen, K. et al. How does industrial policy experimentation influence innovation performance? A case of Made in China 2025. Humanit. Soc. Sci. Commun. 11 , 40. https://doi.org/10.1057/s41599-023-02497-x (2024).
Article CAS Google Scholar
Xu, L. Towards green innovation by China’s industrial policy: Evidence from Made in China 2025. Front. Environ. Sci. 10 , 924250. https://doi.org/10.3389/fenvs.2022.924250 (2022).
Li, X., Han, H. & He, H. Advanced manufacturing firms’ digital transformation and exploratory innovation. Appl. Econ. Lett. https://doi.org/10.1080/13504851.2024.2305665 (2024).
Liu, G. & Liu, B. How digital technology improves the high-quality development of enterprises and capital markets: A liquidity perspective. Finance Res. Lett. 53 , 103683 (2023).
Chernozhukov, V. et al. Double/debiased machine learning for treatment and structural parameters. Econom. J. 21 (1), C1–C68. https://doi.org/10.1111/ectj.12097 (2018).
Article MathSciNet Google Scholar
Athey, S., Tibshirani, J. & Wager, S. Generalized random forests. Ann. Stat. 47 (2), 1148–1178. https://doi.org/10.1214/18-AOS1709 (2019).
Knittel, C. R. & Stolper, S. Machine learning about treatment effect heterogeneity: The case of household energy use. AEA Pap. Proc. 111 , 440–444 (2021).
Yang, J., Chuang, H. & Kuan, C. Double machine learning with gradient boosting and its application to the Big N audit quality effect. J. Econom. 216 (1), 268–283. https://doi.org/10.1016/j.jeconom.2020.01.018 (2020).
Zhang, Y., Li, H. & Ren, G. Quantifying the social impacts of the London Night Tube with a double/debiased machine learning based difference-in-differences approach. Transp. Res. Part A Policy Pract. 163 , 288–303. https://doi.org/10.1016/j.tra.2022.07.015 (2022).
Farbmacher, H., Huber, M., Lafférs, L., Langen, H. & Spindler, M. Causal mediation analysis with double machine learning. Econom. J. 25 (2), 277–300. https://doi.org/10.1093/ectj/utac003 (2022).
Chiang, H., Kato, K., Ma, Y. & Sasaki, Y. Multiway cluster robust double/debiased machine learning. J. Bus. Econ. Stat. 40 (3), 1046–1056. https://doi.org/10.1080/07350015.2021.1895815 (2022).
Bodory, H., Huber, M. & Lafférs, L. Evaluating (weighted) dynamic treatment effects by double machine learning. Econom. J. 25 (3), 628–648. https://doi.org/10.1093/ectj/utac018 (2022).
Waheed, R., Sarwar, S. & Alsaggaf, M. I. Relevance of energy, green and blue factors to achieve sustainable economic growth: Empirical study of Saudi Arabia. Technol. Forecast. Soc. Change 187 , 122184. https://doi.org/10.1016/j.techfore.2022.122184 (2023).
Taskin, D., Vardar, G. & Okan, B. Does renewable energy promote green economic growth in OECD countries?. Sustain. Account. Manag. Policy J. 11 (4), 771–798. https://doi.org/10.1108/SAMPJ-04-2019-0192 (2020).
Ding, X. & Liu, X. Renewable energy development and transportation infrastructure matters for green economic growth? Empirical evidence from China. Econ. Anal. Policy 79 , 634–646. https://doi.org/10.1016/j.eap.2023.06.042 (2023).
Ferguson, P. The green economy agenda: Business as usual or transformational discourse?. Environ. Polit. 24 (1), 17–37. https://doi.org/10.1080/09644016.2014.919748 (2015).
Pan, D., Yu, Y., Hong, W. & Chen, S. Does campaign-style environmental regulation induce green economic growth? Evidence from China’s central environmental protection inspection policy. Energy Environ. https://doi.org/10.1177/0958305X231152483 (2023).
Zhang, Q., Qu, Y. & Zhan, L. Great transition and new pattern: Agriculture and rural area green development and its coordinated relationship with economic growth in China. J. Environ. Manag. 344 , 118563. https://doi.org/10.1016/j.jenvman.2023.118563 (2023).
Li, J., Dong, K. & Dong, X. Green energy as a new determinant of green growth in China: The role of green technological innovation. Energy Econ. 114 , 106260. https://doi.org/10.1016/j.eneco.2022.106260 (2022).
Herman, K. S. et al. A critical review of green growth indicators in G7 economies from 1990 to 2019. Sustain. Sci. 18 , 2589–2604. https://doi.org/10.1007/s11625-023-01397-y (2023).
Mura, M., Longo, M., Zanni, S. & Toschi, L. Exploring socio-economic externalities of development scenarios. An analysis of EU regions from 2008 to 2016. J. Environ. Manag. 332 , 117327. https://doi.org/10.1016/j.jenvman.2023.117327 (2023).
Huang, S. Do green financing and industrial structure matter for green economic recovery? Fresh empirical insights from Vietnam. Econ. Anal. Policy 75 , 61–73. https://doi.org/10.1016/j.eap.2022.04.010 (2022).
Li, J., Dong, X. & Dong, K. Is China’s green growth possible? The roles of green trade and green energy. Econ. Res.-Ekonomska Istraživanja 35 (1), 7084–7108. https://doi.org/10.1080/1331677X.2022.2058978 (2022).
Zhang, H. et al. Promoting eco-tourism for the green economic recovery in ASEAN. Econ. Change Restruct. 56 , 2021–2036. https://doi.org/10.1007/s10644-023-09492-x (2023).
Article ADS Google Scholar
Ahmed, F., Kousar, S., Pervaiz, A. & Shabbir, A. Do institutional quality and financial development affect sustainable economic growth? Evidence from South Asian countries. Borsa Istanbul Rev. 22 (1), 189–196. https://doi.org/10.1016/j.bir.2021.03.005 (2022).
Yuan, S., Li, C., Wang, M., Wu, H. & Chang, L. A way toward green economic growth: Role of energy efficiency and fiscal incentive in China. Econ. Anal. Policy 79 , 599–609. https://doi.org/10.1016/j.eap.2023.06.004 (2023).
Capasso, M., Hansen, T., Heiberg, J., Klitkou, A. & Steen, M. Green growth – A synthesis of scientific findings. Technol. Forecast. Soc. Change 146 , 390–402. https://doi.org/10.1016/j.techfore.2019.06.013 (2019).
Wei, X., Ren, H., Ullah, S. & Bozkurt, C. Does environmental entrepreneurship play a role in sustainable green development? Evidence from emerging Asian economies. Econ. Res. Ekonomska Istraživanja 36 (1), 73–85. https://doi.org/10.1080/1331677X.2022.2067887 (2023).
Iqbal, K., Sarfraz, M. & Khurshid,. Exploring the role of information communication technology, trade, and foreign direct investment to promote sustainable economic growth: Evidence from Belt and Road Initiative economies. Sustain. Dev. 31 (3), 1526–1535. https://doi.org/10.1002/sd.2464 (2023).
Li, Y., Zhang, J. & Lyu, Y. Toward inclusive green growth for sustainable development: A new perspective of labor market distortion. Bus. Strategy Environ. 32 (6), 3927–3950. https://doi.org/10.1002/bse.3346 (2023).
Chernozhukov, V. et al. Double/Debiased/Neyman machine learning of treatment effects. Am. Econ. Rev. 107 (5), 261–265. https://doi.org/10.1257/aer.p20171038 (2017).
Chen, C. Super efficiencies or super inefficiencies? Insights from a joint computation model for slacks-based measures in DEA. Eur. J. Op. Res. 226 (2), 258–267. https://doi.org/10.1016/j.ejor.2012.10.031 (2013).
Article ADS MathSciNet Google Scholar
Tone, K., Chang, T. & Wu, C. Handling negative data in slacks-based measure data envelopment analysis models. Eur. J. Op. Res. 282 (3), 926–935 (2020).
Sarkodie, S. A., Owusu, P. A. & Taden, J. Comprehensive green growth indicators across countries and territories. Sci. Data 10 , 413. https://doi.org/10.1038/s41597-023-02319-4 (2023).
Jiang, Z., Wang, Z. & Lan, X. How environmental regulations affect corporate innovation? The coupling mechanism of mandatory rules and voluntary management. Technol. Soc. 65 , 101575 (2021).
Oh, D. H. & Heshmati, A. A sequential Malmquist-Luenberger productivity index: Environmentally sensitive productivity growth considering the progressive nature of technology. Energy Econ. 32 (6), 1345–1355. https://doi.org/10.1016/j.eneco.2010.09.003 (2010).
Tone, K. & Tsutsui, M. An epsilon-based measure of efficiency in DEA - A third pole of technical efficiency. Eur. J. Op. Res. 207 (3), 1554–1563. https://doi.org/10.1016/j.ejor.2010.07.014 (2010).
Lv, C., Song, J. & Lee, C. Can digital finance narrow the regional disparities in the quality of economic growth? Evidence from China. Econ. Anal. Policy 76 , 502–521. https://doi.org/10.1016/j.eap.2022.08.022 (2022).
Arkhangelsky, D., Athey, S., Hirshberg, D. A., Imbens, G. W. & Wager, S. Synthetic difference-in-differences. Am. Econ. Rev. 111 (12), 4088–4118 (2021).
Abadie, A., Diamond, A. & Hainmueller, J. Synthetic control methods for comparative case studies: Estimating the effect of California’s tobacco control program. J. Am. Stat. Assoc. 105 (490), 493–505 (2010).
Article MathSciNet CAS Google Scholar
Fang, J., Tang, X., Xie, R. & Han, F. The effect of manufacturing agglomerations on smog pollution. Struct. Change Econ. Dyn. 54 , 92–101. https://doi.org/10.1016/j.strueco.2020.04.003 (2020).
Yang, S. & Liu, F. Impact of industrial intelligence on green total factor productivity: The indispensability of the environmental system. Ecol. Econ. 216 , 108021. https://doi.org/10.1016/j.ecolecon.2023.108021 (2024).
Zhang, P., Wang, Y., Wang, R. & Wang, T. Digital finance and corporate innovation: Evidence from China. Appl. Econ. 56 (5), 615–638. https://doi.org/10.1080/00036846.2023.2169242 (2024).
Download references
Acknowledgements
This work was supported by the Major Program of National Fund of Philosophy and Social Science of China (20&ZD133).
Author information
Authors and affiliations.
School of Public Finance and Taxation, Zhejiang University of Finance and Economics, Hangzhou, 310018, China
School of Economics, Xiamen University, Xiamen, 361005, China
Shucheng Liu
You can also search for this author in PubMed Google Scholar
Contributions
J.Y.: Methodology, Validation. S.L.: Writing - Reviewing and Editing, Validation, Methodology. All authors have read and agreed to the published version of the manuscript.
Corresponding author
Correspondence to Shucheng Liu .
Ethics declarations
Competing interests.
The authors declare no competing interests.
Additional information
Publisher's note.
Springer Nature remains neutral with regard to jurisdictional claims in published maps and institutional affiliations.
Supplementary Information
Supplementary information., rights and permissions.
Open Access This article is licensed under a Creative Commons Attribution 4.0 International License, which permits use, sharing, adaptation, distribution and reproduction in any medium or format, as long as you give appropriate credit to the original author(s) and the source, provide a link to the Creative Commons licence, and indicate if changes were made. The images or other third party material in this article are included in the article's Creative Commons licence, unless indicated otherwise in a credit line to the material. If material is not included in the article's Creative Commons licence and your intended use is not permitted by statutory regulation or exceeds the permitted use, you will need to obtain permission directly from the copyright holder. To view a copy of this licence, visit http://creativecommons.org/licenses/by/4.0/ .
Reprints and permissions
About this article
Cite this article.
Yuan, J., Liu, S. A double machine learning model for measuring the impact of the Made in China 2025 strategy on green economic growth. Sci Rep 14 , 12026 (2024). https://doi.org/10.1038/s41598-024-62916-0
Download citation
Received : 05 March 2024
Accepted : 22 May 2024
Published : 26 May 2024
DOI : https://doi.org/10.1038/s41598-024-62916-0
Share this article
Anyone you share the following link with will be able to read this content:
Sorry, a shareable link is not currently available for this article.
Provided by the Springer Nature SharedIt content-sharing initiative
- Made in China 2025
- Industrial policy
- Double machine learning
- Causal inference
By submitting a comment you agree to abide by our Terms and Community Guidelines . If you find something abusive or that does not comply with our terms or guidelines please flag it as inappropriate.
Quick links
- Explore articles by subject
- Guide to authors
- Editorial policies
Sign up for the Nature Briefing: Anthropocene newsletter — what matters in anthropocene research, free to your inbox weekly.

- Open access
- Published: 04 June 2024
Sharpening the lens to evaluate interprofessional education and interprofessional collaboration by improving the conceptual framework: a critical discussion
- Florian B. Neubauer 1 ,
- Felicitas L. Wagner 1 ,
- Andrea Lörwald 1 &
- Sören Huwendiek 1
BMC Medical Education volume 24 , Article number: 615 ( 2024 ) Cite this article
182 Accesses
Metrics details
It has been difficult to demonstrate that interprofessional education (IPE) and interprofessional collaboration (IPC) have positive effects on patient care quality, cost effectiveness of patient care, and healthcare provider satisfaction. Here we propose a detailed explanation for this difficulty based on an adjusted theory about cause and effect in the field of IPE and IPC by asking: 1) What are the critical weaknesses of the causal models predominantly used which link IPE with IPC, and IPE and IPC with final outcomes? 2) What would a more precise causal model look like? 3) Can the proposed novel model help us better understand the challenges of IPE and IPC outcome evaluations? In the format of a critical theoretical discussion, based on a critical appraisal of the literature, we first reason that a monocausal, IPE-biased view on IPC and IPC outcomes does not form a sufficient foundation for proper IPE and IPC outcome evaluations; rather, interprofessional organization (IPO) has to be considered an additional necessary cause for IPC; and factors outside of IPC additional causes for final outcomes. Second, we present an adjusted model representing the “multi-stage multi-causality” of patient, healthcare provider, and system outcomes. Third, we demonstrate the model’s explanatory power by employing it to deduce why misuse of the modified Kirkpatrick classification as a causal model in IPE and IPC outcome evaluations might have led to inconclusive results in the past. We conclude by applying the derived theoretical clarification to formulate recommendations for enhancing future evaluations of IPE, IPO, and IPC. Our main recommendations: 1) Focus should be placed on a comprehensive evaluation of factual IPC as the fundamental metric and 2) A step-by-step approach should be used that separates the outcome evaluation of IPE from that of IPC in the overarching quest for proving the benefits of IPE, IPO and IPC for patients, healthcare providers, and health systems. With this critical discussion we hope to enable more effective evaluations of IPE, IPO and IPC in the future.
Peer Review reports
There is scant knowledge on the extent to which the quality of interprofessional education (IPE) and interprofessional collaboration (IPC) at healthcare institutions influences the patient care quality [ 1 , 2 , 3 ], the cost effectiveness of patient care, the job satisfaction of healthcare professionals [ 1 ] and, as a result, their retention [ 4 , 5 ]. Patients, people who organize and finance healthcare, policy makers, tax payers, and arguably societies as a whole have a reasonable interest in an answer to this question.
According to the peer-reviewed literature, relevant knowledge gaps persist about the benefits of IPE and IPC despite multiple studies on IPE and IPC outcomes covering a period of almost 50 years [ 2 , 3 , 6 , 7 , 8 , 9 , 10 , 11 , 12 , 13 ]. Several explanations as to how this can be possible are proposed: The number of evaluation studies is still too low [ 10 ]; the time periods typically covered by evaluations is too short to detect final outcomes of IPE/IPC interventions [ 2 , 8 , 11 , 14 , 15 ]; too much focus is placed on immediate results without including measures for final outcomes from the outset [ 10 ]; or, ultimately, positive effects of IPE and IPC simply might not exist [ 6 , 9 , 10 ]. Another frequent and non-contradictory explanation proposes that a lack of clarity in theory and terminology of IPE and IPC and an insufficient use of conceptual frameworks are major deficits which obscure evaluation results [ 8 , 12 , 13 , 16 , 17 , 18 , 19 , 20 , 21 ].
In this article, we argue the latter: That an insufficient use of conceptual frameworks has obscured evaluation results. We propose that the persistence of the knowledge gap relating to patient outcomes, satisfaction of healthcare professionals, and cost effectiveness of IPE and IPC activities (briefly, “patient, healthcare provider, and system outcomes”) is rooted in a lack of accuracy in the theoretical models used for mapping causes and effects in IPE and IPC. Our objective is to contribute to overcoming the inconclusiveness in IPE and IPC outcome evaluations by achieving the missing accuracy through the lens of a novel “multi-stage multi-causality” model. Specifically, our research questions are: 1) What are the critical weaknesses of the causal models predominantly used which link IPE with IPC, and IPE and IPC with final outcomes? 2) What would a more precise causal model look like? 3) Can the proposed novel model help us better understand the challenges of IPE and IPC outcome evaluations?
In answering these questions, we first show evidence from the literature that the existing causal models of IPE and IPC exhibit a crucial imprecision. Second, we present the “multi-stage multi-causality model of patient, healthcare provider, and system outcomes” which fixes this imprecision by making a small but important modification to the causal role of IPO. Third, we demonstrate the explanatory power of the multi-stage multi-causality model showing why evaluations using the modified Kirkpatrick classification of interprofessional outcomes (MKC) [ 11 , 22 , 23 ] — a tool commonly used to evaluate outcomes of IPE activities — have failed to substantiate positive outcomes of IPE and IPC; namely, we show how the misuse of MKC leads to inconclusiveness and difficulties in evaluating final patient, healthcare provider, and system outcomes. We conclude with recommendations for future evaluations in the field of IPE, IPO and IPC.
With this theoretical investigation, we hope to contribute to a deeper understanding of the causal factors in IPE, IPO and IPC and to enable more precise evaluations in the future.
Based on our research questions, we performed iterative literature searches (detailed below) followed by critical appraisal by the authors, and transformed the resulting insights into the critical discussion presented in the main section of the present article by applying the 6 quality criteria of the SANRA scale [ 24 ]:
Justification of the article's importance for the readership: Our target audience consists of researchers whose goal is to evaluate whether IPE, IPO or IPC improve patient, healthcare provider, and system outcomes. For our target audience the present study is meaningful because it advances the understanding of the theoretical foundations of evaluations in this field. Further, in local contexts where the potential of IPE, IPO, and IPC is still neglected, clear evidence demonstrating substantial benefits would help to foster programs aimed at implementing better IPE, IPO, or IPC.
Statement of concrete/specific aims or formulation of questions : We set out to explore the following questions: 1) What are the critical weaknesses of the causal models predominantly used which link IPE with IPC, and IPE and IPC with final outcomes? 2) What would a more precise causal model look like? 3) Can the proposed novel model help us better understand the challenges of IPE and IPC outcome evaluations?
Description of literature searches: We searched for existing definitions, causal models, relevant indicators, and evaluation instruments for IPE, IPO, and IPC using PubMed, Google and Google Scholar with the following search terms in different combinations: “interprofessional education”, “interprofessional collaboration”, “interprofessional organization”, “interprofessional team work”, “evaluation”, “outcome evaluation”, “process evaluation”, “modified Kirkpatrick”, “conceptual framework”, “theory”, “model”, “instrument”, “assessment scale”, “survey”, “review”. We conducted all searches in English, covering the time period from 1950 to 2023. We augmented the initial body of literature found by this strategy with citation tracking: for backward tracking, we followed the references provided in articles which we deemed relevant for our research questions; for forward searches, we used the "cited by" feature of PubMed and Google Scholar. The subchapter-specific literature search used in the development of our definition of IPC is described under “Definition of factual IPC”.
Referencing: We consistently back key statements by references.
Scientific reasoni ng: We enable the reader to easily follow our narrative by structuring the present article around the three research questions as stated above, following a logical flow of arguments.
Appropriate presentation of data: We present the data by distinguishing which findings were taken from the literature and which novel arguments for answering the research questions were derived by us.
Definitions
Definition of ipe.
Occasions when two or more healthcare/social care professions learn with, from and about each other to improve collaboration and the quality of care for patients/clients [ 2 ] (slightly refining the CAIPE definition [ 25 ]).
These occasions can happen formally or informally, in dedicated educational settings or at the workplace of healthcare/social care professions, and at any stage along the learning continuum, i.e. foundational education, graduate education, and post-licensure continuing professional development [ 8 , 26 ]. The central concept in IPE is learning [ 13 ], the gain of knowledge, skills, and attitudes, or — from a constructivist’s perspective — changes in the brains of individuals.
Definition of factual IPC
Presence of activities in the following 7 dimensions:
Patient-centered care, including a shared treatment plan and effective error management;
Shared creation of the treatment plan and coordination of its execution;
Mutual respect between professions;
Communication, including shared decision-making, sharing of information, appropriate communication tools, and accessibility of team members;
Shared definition and acceptance of roles and responsibilities;
Effective conflict management; and
Leadership, including outcome orientation.
How did we arrive at this definition? IPC has to be distinguished from traditional “multiprofessional collaboration”. In multiprofessional collaboration, patient care is organized in a discipline-oriented way, affecting its organization, leadership, communication, and decision-making. Different professions work separately, each with their own treatment goals; the physician delegates treatment options to the other healthcare professionals in one-way, mostly bilateral communication [ 27 , 28 ]. IPC, in contrast, is defined as the occasions “when multiple health workers from different professional backgrounds work together with patients, families, carers and communities to deliver the highest quality of care” [ 29 ]. This definition by the WHO remains in use today [ 30 ]. However, we found that, in order to talk about specific effects of IPE on IPC and to tailor evaluations towards less ambiguous results, an operationalized definition of IPC is required which provides a higher level of applicability. To create such a definition, we searched the literature to collect a comprehensive list of IPC dimensions which covers all possible settings of IPC. In an iterative process of content-based thematic clustering, reviews, original articles and preexisting questionnaires on the evaluation of IPE and IPC were added until there was agreement between the authors that saturation was reached with regard to all relevant IPC dimensions. This resulted in the following list of publications: [ 3 , 7 , 9 , 19 , 26 , 28 , 31 , 32 , 33 , 34 , 35 , 36 , 37 , 38 , 39 ]. Next, we clustered the terms for IPC dimensions found in this body of literature by consensus agreement on sufficient equivalence between three of the authors (FBN, FLW, SH). Clustering was required due to a lack of consistent terminology in the literature and resulted in the comprehensive set of 7 IPC dimensions used in our definition of IPC provided above. Finally, we needed to differentiate IPC from IPE and learning: At the workplace, informal learning happens all the time. As a result, interprofessional work processes can comprise both IPC and IPE at the same time; however, interprofessional learning is only a possible , not a necessary element of IPC and hence was not included in our definition of IPC. For example, a healthcare professional who is fully equipped with all competencies required for factual IPC could proficiently work in an established team in an interprofessional way without having to learn any additional IPC-related skills.
In order to stress that our definition of IPC includes all the healthcare-related interprofessional work processes actually taking place but excludes the activities required to create them (those fall in the domains of IPE or IPO), we use the term “factual IPC” throughout the present article. Factual IPC not only happens in formal interprofessional work processes like regular, scheduled meetings but also “on the fly”, i.e. during informal and low-threshold communication and collaboration.
Definition of IPO
All activities at a healthcare institution which create, improve, or maintain regular work processes of factual IPC or create, improve, or maintain institutional conditions supporting formal and informal parts of factual IPC, but excluding activities related to IPE.
There is no agreed upon definition of IPO in the literature, so we propose this refined one here that is broad enough to encompass the full variety of IPC-supporting activities at a healthcare institution while, at the same time, being narrow enough to exclude all manifestations of IPE.
According to this definition, IPO complements IPE within the set of jointly sufficient causes of factual IPC. IPO comprises all conditions required for the realization of factual IPC which are not related to interprofessional learning. It includes the actions of healthcare managers to implement work processes for IPC and to create supportive conditions for IPC (cf. the definitions of IPO in [ 6 , 8 , 13 , 17 , 23 , 26 , 30 , 31 , 33 , 40 , 41 , 42 ]). All interventions which establish or improve interprofessional work processes, i.e. which change how things are done in patient care, or which improve the conditions for factual IPC at an institution, belong in the domain of IPO. IPO is also the continued support for factual IPC by management like encouragement, clarification of areas of responsibility, incentives, staffing, room allocation, other resources, or funding. In contrast, established and regular interprofessional tasks themselves, after they have become part of the day-to-day work life of healthcare teams, without requiring further actions by management, would be categorized as factual IPC, not IPO.
Taken together, IPE is the umbrella term for planning, organizing, conducting, being subject to, and the results of interprofessional learning activities, whereas IPO is the umbrella term for all other activities that, in addition to individual competencies of team members, are necessary to cause factual IPC of high quality.
Critical discussion
What are the critical weaknesses of the causal models predominantly used which link ipe with ipc, and ipe and ipc with final outcomes.
We start by exploring the models in the literature that describe causes and effects of interprofessional activities in the context of patient care. We will derive evidence from the literature that the existing models exhibit a crucial imprecision regarding the causal role of IPO.
The causal model of IPC proposed by the WHO [ 29 , 43 ] (Fig. 1 ) was the model predominantly used in past evaluations of IPE and IPC. The WHO model suggests that IPE-related learning leads to IPC competence (knowledge, skills, and attitudes) in the “health workforce” that is “IPC-ready” post-IPE. This readiness “automatically” leads (as the long diagonal arrow in Fig. 1 suggests) to factual IPC. “The World Health Organization and its partners acknowledge that there is sufficient evidence to indicate that effective interprofessional education enables effective collaborative practice” [ 29 ]. Factual IPC, in turn “strengthens health systems and improves health outcomes” [ 29 ]. As a result, this model suggests a kind of “transitivity” between first causes and last effects: effective IPE activities are expected to ultimately yield positive patient, healthcare provider, and system outcomes on their own.
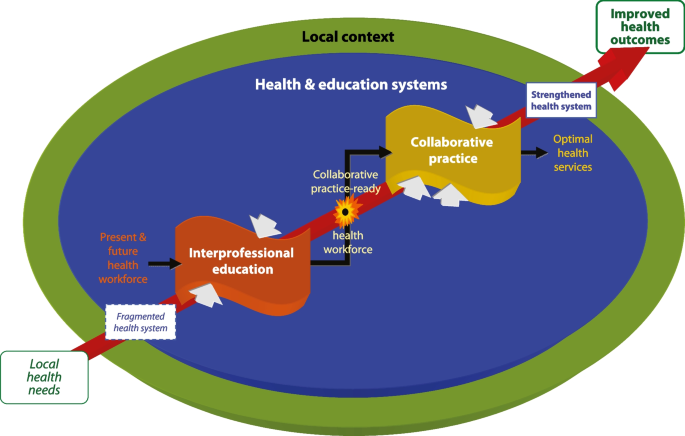
The WHO model of causes and effects in IPE and IPC (from [ 29 ], with permission)
After its publication, the WHO model was regularly cited and endorsed by IPE experts and continues to exert broad influence today. As of October 6, 2023, the “Google Scholar” search engine showed the original publication [ 29 ] to have 4393 citations, 367 of them in 2023 alone.
It is important to note that the WHO model is monocausal with respect to IPC, i.e. IPE is the sole necessary cause for factual IPC. While the model acknowledges that, next to IPE, there are further “mechanisms that shape how collaborative practice is introduced and executed”, it only ranks them as supportive: “ Once a collaborative practice-ready health workforce is in place [emphasis added], these [additional] mechanisms will help them [policy-makers] determine the actions they might take to support [emphasis added] collaborative practice” [ 29 ]. The following quotes by Reeves and colleagues further illustrate the strong emphasis causal models used to put on IPE: «It is commonly argued that IPE can promote the skills and behaviours required for effective IPC, which in turn can improve quality of health care and patient outcomes” [ 17 ] and “National organisations have created core competencies for interprofessional collaborative practice, positioning IPE as fundamental to practice improvement [emphasis added]” [ 10 ]. A couple of years later, Paradis and colleagues even state: “During this wave [of IPE; 1999–2015], advocates suggested IPE as the solution to nearly every health care problem that arose (…)” [ 6 ].
However, a scoping review by Reeves and colleagues aimed at improving “conceptualization of the interprofessional field” published soon after the WHO model, already acknowledged that the monocausal picture of factual IPC is incomplete [ 17 ]. Based on a broad analysis of the literature, their review offers a theoretical “Interprofessional framework” that includes the notion of IPO as an additional and different possible cause for desired interprofessional outcomes (Fig. 2 ). They define IPO interventions as “changes at the organizational level (e.g. space, staffing, policy) to enhance collaboration and the quality of care”. The explicit inclusion of IPO in this causal model of IPC was a very important step forward. The authors position IPO interventions parallel to IPE interventions, clearly indicating that IPO is an additional possible cause for desired interprofessional objectives and outcomes. However, in their framework, the capacity of IPO to be a second necessary cause in addition to IPE had not been clearly worked out yet.
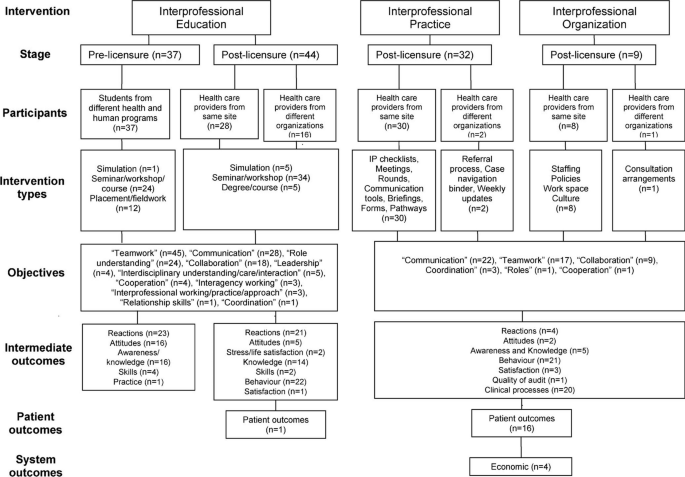
The Interprofessional Framework (from [ 17 ], reprinted by permission of the publisher (Taylor & Francis Ltd, [ 44 ]). Note that, next to IPE, IPO is listed as a different, additional cause for desired interprofessional objectives and outcomes, but the crucial concept that it also is a necessary cause has not yet been worked out here
Side note: This model and publications using it (e.g. [ 45 ]) specify “Interprofessional Practice” (IPP) as a fourth domain, different from IPO (Fig. 2 , middle column). However, the IPP elements describe interventions that support work processes of factual IPC, and support for work processes of factual IPC is fully included in our definition of IPO. As a result, we see no necessity to set IPP apart from IPO and do not include IPP as an additional domain in our model below.
For completeness’ sake, we want to mention another explicit model by D’amour and Oandasan [ 26 ] with a comparable level of causal clarity which similarly claims that “there are many factors that act as determinants for collaborative practice to be realized”. As this model does not alter our line of argument it is not shown here.
The ongoing imprecision about the causal role of IPO naturally led to the next iteration of models. The authors of a 2015 review, commissioned by the Institute of Medicine of the National Academy of Sciences (IOM), provide the most recent influential model of causes and effects in IPC which they call “Interprofessional learning continuum model” [ 8 ] (Fig. 3 ).
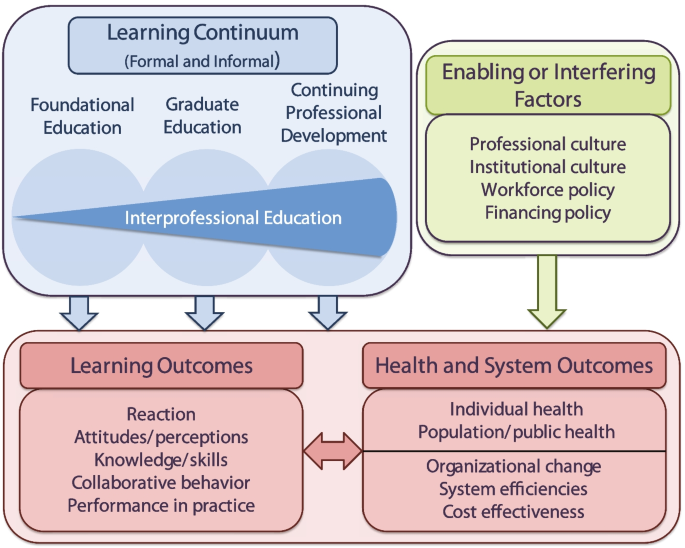
The Interprofessional learning continuum model (from [ 8 ], with permission). Under the labels of “Institutional culture”, “Workforce policy”, and “Financing policy” it not only comprises IPO but assigns to IPO the crucial property of being an “enabling” factor, i.e. being co-causal for factual IPC (here labeled as “Collaborative behavior” and “Performance in practice”, lower left row). Despite this important improvement, the hierarchy of causes and effects remains partially vague: a The green arrow seems to imply direct effects of IPO on health and system outcomes without acknowledging that if IPO is supposed to have an effect on those at all, it necessarily must improve factual IPC first. b The impression remains that factual IPC mainly belongs on the left-hand side, being primarily an effect of IPE. IPO seems less effective on IPC, depending on how one interprets the influence of the green arrow on the larger red box which groups learner, health and system outcomes. c The left tip of the red double arrow in the center, indicating an effect of health and system outcomes on learning outcomes, is not discussed in the publication
In comparison to the WHO model (Fig. 1 ) and the Interprofessional Framework (Fig. 2 ), this causal model acknowledges that IPO is not just an additional but also a necessary cause of IPC and thus provides the most elaborate description of the causal relationships between IPE, IPO and IPC in the literature so far. The authors state, “Diverse and often opaque payment structures and differences in professional and organizational cultures generate obstacles to innovative workforce arrangements, thereby impeding interprofessional work. On the other hand, positive changes in workforce and financing policies could enable [emphasis added] more effective collaboration (…)” [ 8 ]. The word “enable” implies causal necessity : if an enabling factor is absent, the effect is disabled, hence the enabling factor is necessary . The key insight that IPO is a further necessary cause of IPC next to IPE can be found in several other, partly less recent publications, with the only difference that these publications do not embed this insight in a formal model [ 6 , 7 , 13 , 23 , 31 , 33 , 41 , 42 ]. The causal necessity of IPO becomes evident if one considers the extreme case: imagine a healthcare team whose individual members have all learned through IPE the skill set necessary for high quality IPC, i.e. they are optimally trained for IPC. However, they work at an institution that does not support proper IPC work processes, e.g. there is no dedicated time for team discussions of treatment plans and no electronic tools that allow all team members equal access to patient data. Consequently, there effectively cannot be an optimal manifestation of factual IPC, and it is impossible to expect that the IPE that the team members experienced during their training will significantly affect the quality of patient care in this setting.
What would a more precise causal model look like?
As we have seen, the notion of IPO in causal models of interprofessionality in the literature progressed from “IPO supportive” (Fig. 1 ) to “IPO possible but optional” (Fig. 2 ) to “IPO enabling, i.e. necessary” (Fig. 3 ). The key result of our study is a refinement missing from the existing causal model of IPE/IPO/IPC. It is the explicit statement that IPO is an equally necessary factor next to IPE in the causation of factual IPC. Only jointly are IPE and IPO sufficient to cause factual IPC of high quality. We deem this small modification crucial to reach the conceptual resolution required to fully understand the causes of factual IPC. The fully adjusted causal model is presented in Fig. 4 . In this “multi-stage multi-causality model of patient, healthcare provider, and system outcomes”, IPO is now unequivocally labeled as co-necessary for factual IPC alongside IPE-caused individual competencies.
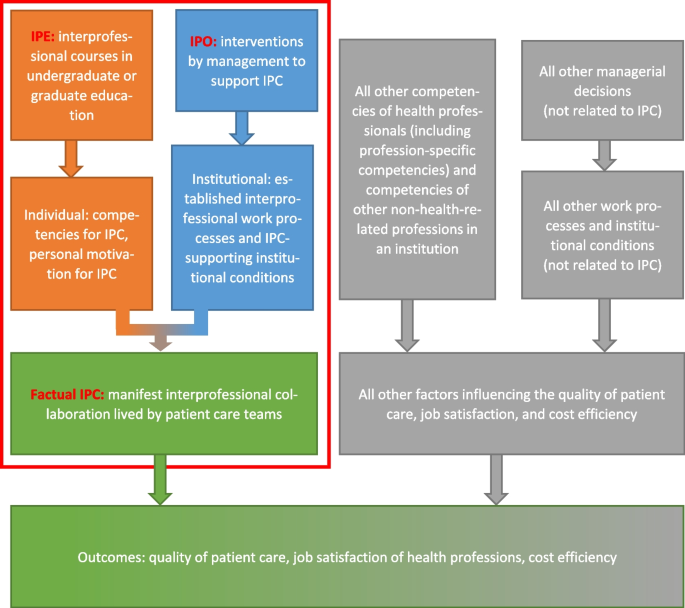
Multi-stage multi-causality model of patient, healthcare provider, and system outcomes. Key ideas: IPO is an equally necessary co-factor in the causation of high-quality factual IPC, in addition to IPE. And the entire realm of interprofessional activities (red-outlined box), of which factual IPC is the final and active ingredient, is in turn only one of several causes leading to final outcomes of interest. Orange boxes: Domain of IPE, the domain of acquisition of competencies for IPC by an individual person through learning. Blue boxes: Domain of IPO, defined as the institutional domain of implementation, improvement, and maintenance of work processes of factual IPC and of IPC-supportive institutional conditions. Green box: Domain of factual IPC at a healthcare institution. Green-gray box, bottom row: Final outcomes of interest, i.e. patient care quality, job satisfaction of healthcare professionals, and cost effectiveness of patient care
Much more explicitly than previous ones, the multi-stage multi-causality model further shows that there are additional necessary causes for beneficial patient, healthcare provider, and system outcomes that lie entirely outside of the realm of IPC-related activities (i.e. outside of IPE/IPO/IPC). It is important to understand that not only factual IPC, but also the final patient, healthcare provider, and system outcomes have more than only one necessary cause, as reflected in the concept of “multi-causality on multiple stages”. This means that optimizing factual IPC is necessary but still not sufficient to optimize patient, healthcare provider, and system outcomes. Examples for necessary co-factors on the same level as factual IPC but from outside the realm of IPE/IPO/IPC are a) profession-specific (“uniprofessional”) competencies for aspects of a task that can only be accomplished by members of a specific healthcare profession ( task work vs. team work in [ 41 ]), b) details of health insurance policies, which can affect the cost effectiveness of patient care [ 46 ], salaries paid to health professionals by a healthcare institution, a factor which can influence job satisfaction [ 47 ], or good management decisions at an institution of patient care in general which comprise much more than just full support for factual IPC [ 46 ].
It should be noted that the co-causality in this conceptual framework is not compatible with the transitivity of the WHO model, where IPE ultimately leads to patient and healthcare provider outcomes via a predefined chain of “self-sustaining” secondary effects.
In sum, the adjusted causal model proposes that patient, healthcare provider, and system outcomes depend on multi-stage multi-causality. Stage 1: IPE + IPO = factual IPC: competencies for IPC in the workforce, the final result of interprofessional learning (IPE), plus creating and maintaining IPC work processes and supportive institutional conditions (IPO) together cause factual IPC. Stage 2: Factual IPC + non-interprofessional factors = patient, healthcare provider, and system outcomes: Factual IPC of high quality plus additional necessary but interprofessionality-independent factors together cause the final outcomes of interest.
The intention of our notion of “multi-stage multi-causality” is not to devalue the arrow-less “causal halos” of contextual factors in other models but rather to emphasize that even in “complex” systems (systems with multiple interacting elements) the actual sequence of causes and effects should be understood as precisely as possible for optimizing evaluations.
Brandt and colleagues, after reviewing the impact of IPE and IPC, note in their outlook on IPE, “given the complexity of the healthcare world, training learners in effective team work may not ultimately lead to improved health outcomes or reduce the cost of care” [ 9 ]. We don’t share this degree of pessimism; above we have shown that a monocausal, IPE-biased view on IPC simply might be insufficient for proper outcome evaluation of IPE and IPC. There is hope that by considering IPO, evaluations will become more conclusive. Wei and colleagues state in a systematic meta-review of systematic reviews about IPC, “Effective IPC is not linear; it does not occur naturally when people come together but takes a whole system’s efforts, including organizations, teams, and individuals” [ 30 ]. As we have explained, IPO has to be factored in as an additional necessary cause for IPC, and factors from outside the realm of IPE/IPO/IPC contribute to the “hard” outcomes of interest as well. We presented an adjusted causal model which explicitly acknowledges this multi-stage multi-causality of patient, healthcare provider, and system outcomes.
Can the proposed novel model help us better understand the challenges of IPE and IPC outcome evaluations?
We claim that the multi-stage multi-causality model exhibits strong explanatory power with regards to the difficulties of showing positive consequences of IPE and IPC in outcome evaluations in the past. To illustrate this, we must first describe the prominent role the modified Kirkpatrick classification of interprofessional outcomes [ 11 , 22 , 23 ] plays in outcome evaluations of IPE and IPC.
The modified Kirkpatrick classification (MKC)
MKC is regularly used to classify outcomes of IPE learning activities, curricula and programs [ 2 , 8 , 14 , 20 , 42 , 45 , 48 , 49 ]. It is a derivative of the original Kirkpatrick model for evaluating training results, named after its author, Donald L. Kirkpatrick, which distinguishes four categories of learning outcomes (Level 1: Reaction, Level 2: Learning, Level 3: Behavior, Level 4: Results) [ 50 , 51 ]. Expanding the original model, MKC assigns outcomes of IPE activities to six categories [ 11 ]:
Level 1: Reaction
Level 2a: Modification of perceptions & attitudes
Level 2b: Acquisition of knowledge & skills
Level 3: Behavioural change
Level 4a: Change in organisational practice (wider changes in the organization and delivery of care)
Level 4b: Benefits to patients/clients
In 2007, the authors of MKC claimed, “We have used these categories since 2000. They have proved useful and, contrary to our initial expectations, sufficient to encompass all outcomes in the hundreds of studies reviewed to date” [ 11 ]. This completeness has made MKC a useful tool for authors of review articles as it allows a retrospective classification of IPE outcomes not labeled in the original literature. As a result, MKC was quickly adopted by IPE evaluators around the world to describe the effectiveness of IPE interventions. As Thistlethwaite and colleagues put it in 2015, “This (…) model is now ubiquitous for health professional education evaluation” [ 42 ].
At first glance, the existence of such a clear and simple classification of IPE outcomes which not only covers all possible IPE outcomes but also is widely embraced in the literature, seems to be good news. What exactly is the problem then? Why did the introduction of MKC more than twenty years ago, plus the conceptual clarification provided by it, not resolve the difficulty in demonstrating IPE-caused patient, healthcare provider, and system outcomes (i.e. effects on MKC levels 4a and 4b)? In the following, we unfold a detailed answer to this question after application of the multi-stage multi-causality model.
To achieve progress, IPE and IPC outcome evaluations need to be complemented with process evaluations
MKC classifies outcomes but is agnostic about how these outcomes come into existence. For an evaluator using MKC, the effects of IPE-related interventions unfold inside a black box. The input into the black box is the intervention, the output constitutes 6 different classes of outcomes, i.e. the 6 levels of MKC described above. Naturally, such solely outcome-focused evaluations cannot explain functional interdependencies between the elements of the system. As we have seen, the benefits of IPE and IPC do not unfold as trivially as initially thought. Therefore, after two decades of (overall rather) inconclusive results of applying MKC to the outcomes of interprofessional interventions, the “why” should have moved to the center of the IPE evaluation efforts. This question is posed variously under well-known labels: Authors aware of said stagnancy either call for “formative evaluation” [ 52 ], “process evaluation” [ 14 ], or “realist evaluation” [ 42 ] in order to understand why interventions work as intended or not. In the following, we use the term “process evaluation” because we focus on understanding the underlying mechanisms.
Process evaluations require a causal model
Process evaluations require a causal model for the system under study to be able to select relevant indicators from a potentially much larger number of conceivable indicators. Appropriately selected indicators, which reflect the inner mechanisms of the system, then replace the black box, reveal bottlenecks, and allow explanations as to why interventions did or did not have the expected or intended outcomes. To explicitly demand the use of a causal model in an evaluation is a core principle, for example, of the “realistic evaluation” approach [ 53 ]. By directly criticizing the (original) Kirkpatrick model, Holton similarly suggests that a “researchable evaluation model” is needed which should “account for the effects of intervening variables that affect outcomes, and indicate causal relationships” [ 54 ]. Specifically for the domain of IPE and IPC, Reeves and colleagues [ 20 ] recommend “the use of models which adopt a comprehensive approach to evaluation” and the IOM authors conclude, “Having a comprehensive conceptual model provides a taxonomy and framework for discussion of the evidence linking IPE with learning, health, and system outcomes. Without such a model, evaluating the impact of IPE on the health of patients and populations and on health system structure and function is difficult and perhaps impossible [emphasis added]” [ 8 ].
MKC is not a causal model
Aliger and Yanak note that when Donald Kirkpatrick first proposed his model, he did not assert that each level is caused by the previous level [ 55 ]. Similarly, the developers of MKC acknowledge that “Kirkpatrick did not see outcomes in these four areas as hierarchical.” Rather, most likely in an attempt to avoid indicating causality in MKC themselves, they talk about “categories” not “levels” throughout the majority of their abovementioned paper [ 11 ]. They even knew from the outset that besides IPE the domain which we now call IPO influences outcomes on MKC levels 4a and 4b (but did not include IPO in MKC): “(…) impact of one professional’s changes in behavior depend[s] on [a] number of organisational constraints such as individual’s freedom of action (…) and support for innovation within the organisation” [ 13 ]. This means that by design neither the original Kirkpatrick model nor MKC are intended to be or to include causal models. MKC simply doesn’t ask at all whether additional causes besides an IPE intervention might be required for creating the outcomes it classifies, especially those of levels 3, 4a and 4b. In case such additional causes exist, MKC neither detects nor reflects them. Yardley and Dornan conclude that Kirkpatrick’s levels “are unsuitable for (…) education interventions (…) in which process evaluation is as important as (perhaps even more important than) outcome evaluation” [ 14 ].
Nevertheless, MKC continues to be misunderstood as implying a causal model
The numbered levels in the original Kirkpatrick model have drawn criticism for implying causality [ 14 , 54 , 55 ]. Originally, Kirkpatrick had used the term “steps” not “levels” [ 15 , 42 , 55 ] whereas all current versions of the Kirkpatrick model, including MKC, now use the term “levels”. Bates [ 52 ] cites evidence that Kirkpatrick himself, in his later publications, started to imply causal relationships between the levels of his model. Bates bluntly declares: “Kirkpatrick’s model assumes that the levels of criteria represent a causal chain such that positive reactions lead to greater learning, which produces greater transfer and subsequently more positive organizational results” [ 52 ]. Alliger and Janak [ 55 ] provide other examples from the secondary literature which explicitly assume direct causal links between the levels and continue to show that this assumption is highly problematic. Most strikingly, the current (2023) version of the Kirkpatrick model [ 51 ], created by Donald Kirkpatrick’s successors, explicitly contains a causal model which uses the exact same causal logic Alliger and Janak had proposed as underlying it almost 3 decades earlier [ 55 ].
As a derivative of the Kirkpatrick model, MKC has inherited just that unfortunate property of implying causality between levels. While starting their above-mentioned publication with the carefully chosen term “categories”, the authors of MKC, in the same publication, later fall back on using “levels” [ 11 ]. In earlier publications, they even had explicitly assigned explanatory causal power to MKC: “Level 4b: Benefits to patients/clients. This final level covers any improvements in the health and well being of patients/clients as a direct result [emphasis added] of an education programme” [ 22 ]. Taken together, the authors of MKC themselves, while acknowledging that the original Kirkpatrick model didn’t imply a causal hierarchy, at times contradictorily fuel the notion that MKC provides a viable causal model for the mechanisms of IPE and IPC. As Roland observes, it became common in the literature in general to see the levels of MKC as building on each other, implying a linear causal chain from interprofessional learning to collaborative behavior to patient outcomes [ 15 ].
Why has the wrong attribution of being a causal model to MKC remained stable for so long?
Why has this misunderstanding of MKC as a causal model not drawn more criticism and why has it been so stable? We speculate that a formal parallelism between the transitive relations in the WHO causal model (Fig. 1 ) and the numbered levels of MKC, if wrongly understood as a linear chain of subsequent causes and effects, strengthens the erroneous attribution of a causal model to MKC (Fig. 5 ). Our reasoning: The continued use of the mono-causal WHO model, as opposed to switching to a model incorporating multiple causes for patient, healthcare provider, and system outcomes, stabilizes the misunderstanding of the monothematic (IPE-constricted) MKC as a causal model. (In defense of this mistake, one could say, if the transitivity assumption associated with the WHO causal model was true, i.e. if the causal chain actually was mono-linear, then MKC would be a valid causal model because intermediate outcomes would be the sole causes of subsequent outcomes, covering the entire, linear chain of causes. As a result, there would be no difference between outcome evaluation and process evaluation, and MKC would be an appropriate tool for process evaluations.) Conversely, we suspect that the wrong but established use of MKC as a conceptual framework in IPE and IPC outcome evaluations stabilizes the continued use of the mono-causal linear WHO model, reinforcing the wrong impression that IPE is the only cause of interprofessional outcomes. The “transitivity” of the WHO model strongly resonates with the observation that the (original) Kirkpatrick model implies the assumption that “all correlations among levels are positive” [ 55 ]. If the most upstream event (an IPE activity) is positively correlated with the most downstream elements (patient, healthcare provider, and system outcomes) anyway, why should one bother evaluating intermediate steps? The same fallacy holds true for MKC. When its authors state that “Level 4b (…) covers any improvements in the health and well being of patients/clients as a direct result of an education programme” [ 22 ], they not only assign causal explanatory power to MKC, but also neglect the “multi-causality on multiple stages” of outcomes. They assume the same causal transitivity for MKC as is present in the WHO model and thereby expect an “automatic” tertiary effect from an IPE intervention on patient outcomes without considering at all whether the quality of factual IPC – as a necessary intermediate link in the causal chain – has changed due to the intervention or not.
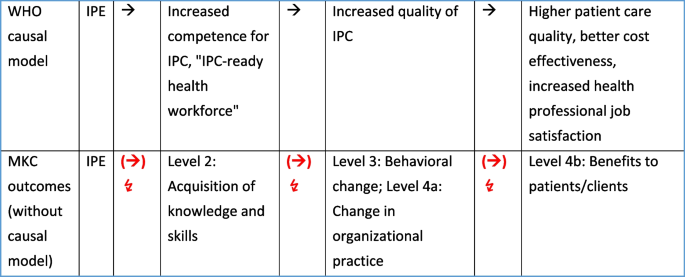
“Unhealthful alliance” between the WHO causal model and MKC. MKC as an outcome classification does not contain a causal model, but uses the term “level” and has numbers attached to each, suggesting causal hierarchy nonetheless. The “levels” of MKC resonate with the causal chain of the WHO model. We speculate that this formal similarity stabilizes the false assignment of a causal structure to MKC (red arrows in the lower row) and, at the same time, as MKC is widely used, perpetuates the use of the WHO model
If misused as a causal model, MKC does not function and can hinder progress in IPE and IPC evaluations
So far we have established that a) Pure outcome evaluations do not answer the question why it is so hard to detect patient, healthcare provider, and system outcomes of IPE and IPC interventions; b) Process evaluations are required to address this “why” question and to achieve progress in IPE and IPC evaluations; c) A theoretical causal model is required for such process evaluations; d) MKC is not such a causal model; e) Nevertheless, MKC falsely keeps being used as such a causal model; and f) The misuse of MKC has remained rather stable, possibly due to a formal parallelism between the WHO causal model and MKC.
The multi-staged multi-causality model of patient, healthcare provider, and system outcomes now makes it clear why evaluations which implicitly or explicitly treat MKC as a causal model are bound to fail in their process evaluation part: MKC, when used as a causal model, is crucially incomplete: In terms of the causes of factual IPC (cf. Figure 4 , orange and blue boxes), MKC sees IPE but is blind to IPO; and in terms of the direct causes of patient, healthcare provider, and system outcomes (cf. Figure 4 , green and grey boxes), MKC sees factual IPC but is blind to the complementary non-interprofessional causes because none of its levels covers them. MKC is a classification limited to detecting outcomes of IPE, and neither IPO nor non-interprofessional factors are such outcomes. When speaking about the original model (but with his statement being transferable to MKC), Bates notes that “Kirkpatrick’s model implicitly assumes that examination of (…) [contextual] factors is not essential for effective evaluation” [ 52 ]. Citing Goldstein and Ford [ 56 ], he continues, “when measurement is restricted to (…) the four (…) levels no formative data about why training was or was not effective is generated” [ 52 ]. Specifically targeting the MKC version, Thistlethwaite and colleagues imply that MKC lacks IPO: “When thinking of applying of Kirkpatrick’s framework to IPE, we must remember the importance of the clinical environment (…) and consider how conducive it is to, and facilitative of, any potential change in behaviour arising from interprofessional learning activities” [ 42 ].
Bordage calls conceptual frameworks “lenses” through which scientists see the subjects of their studies [ 57 ]. Following this metaphor, we conclude that the resolution of the “conceptual lens” of MKC, if misused as a causal model, is too low for process evaluations. In our perspective, this, in turn, is the most likely reason why outcome evaluations of the past have failed to reliably demonstrate terminal benefits of IPE and IPC.
It is important to note that MKC by design solely, agnostically and successfully measures outcomes of interprofessional education in different dimensions. Therefore, its failure to detect bottlenecks in IPE and IPC is not its own fault, but the fault of evaluators who continue to use it as a causal model while failing to acknowledge the multi-staged multi-causality of patient, healthcare provider, and system outcomes.
We next take a closer look at how exactly MKC fails. In the mono-linear, low-resolution view of MKC, if a study that evaluates the effects of an intervention fails to detect final outcomes, the only logical possible conclusion is to question the effectiveness of previous levels. If there are changes in interprofessional behavior (level 3) but there is no benefit to patients (level 4b), the conclusion is that changes in interprofessional behavior are not beneficial to patients; if there are interprofessional competencies acquired by learners (level 2) but no subsequent change in interprofessional behavior (level 3), then interprofessional competencies do not translate into behavior. Using MKC as the conceptual lens, the logical answer to “why” is that “the training program was not designed in ways that fostered effective transfer or (…) other input factors blocked skill application” [ 52 ], and a straightforward overall conclusion with regards to the knowledge gap about the benefits of IPE and IPC would be that IPE is not very effective in terms of patient, healthcare provider, and system outcomes. While this disappointing result has actually been considered as a possibility [ 6 , 9 , 10 ], more often alternative explanations are sought in an attempt to rescue IPE efforts and to avoid the conclusion that IPE is ineffective while sticking with MKC as the causal model.
One of these “escape routes” is to claim that it is methodologically too difficult to measure outcomes on MKC levels 3, 4a and 4b by using different variants of a temporal argument. Paraphrasing Belfield et al. [ 58 ], Roland [ 15 ] states that “patient outcomes may only become apparent over a protracted period of time due to the time needed for the learner to acquire and implement new skills [emphasis added by us, also in the following quotations]” whereas Hammick and colleagues state, “It is unsurprising that all but one of the studies (…) evaluated IPE for undergraduate students. The time gap between their interprofessional learning and qualification clearly presents a challenges [sic] associated with evaluating levels 3, 4a and 4b outcomes” [ 11 ]. Yardley and Dornan add, “early workplace experience (…) might take months or even years to have any demonstrable effect on learners, let alone patients” [ 14 ]. The IOM comments that “Efforts to generate this evidence are further hindered by the relatively long lag time between education interventions and patient, population, and system outcomes” [ 8 ] while Reeves and colleagues note that “ the time gap between undergraduates receiving their IPE and them qualifying as practitioners presents challenges with reporting outcomes at Levels 3, 4a, and 4b” [ 2 ]. The core argument here is always that undergraduate IPE happens in educational institutions whereas IPC happens at the workplace at healthcare institutions much later . By this logic, the causal chain assumed by MKC might be fully intact but the time lag between an IPE intervention and effects on levels 3, 4a and 4b constitutes an insurmountable methodological difficulty and renders comprehensive evaluations of IPE outcomes impossible.
Another “escape route” is to invoke “complexity” of IPE as the reason why its final outcomes are hard to detect. Thistlethwaite and colleagues [ 42 ] agree with Yardley and Dornan [ 14 ] that the MKC is not suited to evaluate “the complexity of health profession education and practice.” The authors from the IOM state that “The lack of a well-defined relationship between IPE and patient and population health and health care delivery system outcomes is due in part to the complexity of the learning and practice environments” [ 8 ]. The term “complexity” usually refers to systems which are cognitively difficult to understand because they have many elements or because science has not figured out yet how to model their interactions [ 59 ]. In our opinion, the term “complexity” in the context of IPE is ill-defined and a placeholder for saying that the set of causes of patient, healthcare provider, and system outcomes is not being understood well and that a more precise causal model is required to figure out what is going on.
Compare and contrast: “multi-stage multi-causality” as causal model
If we use “multi-stage multi-causality” as the conceptual lens instead of MKC we increase the available resolution and can see more elements of the system. If evaluations fail to show beneficial outcomes of IPE or IPC, we now can do much better asking the right sub-questions to find an answer to “why”. Viewed through the high-resolution lens of the multi-stage multi-causality model, the list of possible failure points on this trajectory significantly expands. The resulting high-resolution picture provides an exquisite set of novel testable hypotheses (Table 1 ). Collecting data on different levels, including the level of factual IPC, should enable decisions as to which of these scenarios were attributable to an IPE intervention having no multi-level effect.
Taken together, we argue that the answers to “why” allowed by the low resolution lens of MKC when misused as a causal model might sometimes be wrong and should be replaced with more detailed explanations.
It is premature to conclude that IPE has no effects on patient, healthcare provider, and system outcomes unless the presence or absence of all co-causes has been considered.
The deeper cause of the temporal argument might be to mistakenly use MKC as a causal model because the use of MKC masks any problems with IPO or other co-causes. Given the higher resolution of the multi-stage multi-causality model, it is now possible to conceptually distinguish between the known challenge arising from the passage of time (creating various confounders) and the case in which a lack of IPO blocks the effects of IPE. It should be possible, in principle, to assess at any later point in time, for example by means of a survey, how much and which types of IPE members of an interprofessional team had experienced earlier in their career and how much they remember; or even to assess their current competencies for IPC in a practical exam. Such measurements might reveal that individual competencies for IPC are present, no matter how much time has passed since their acquisition, and that IPO is the actual bottleneck.
Likewise, alleged methodological perplexity due to IPE “complexity” is de-emphasized if we swap the low-resolution lens of MKC for the high-resolution lens of the multi-staged multi-causality model. The high-resolution picture (Fig. 4 ; Table 1 ) replaces the fuzzy placeholder of “complexity” by adding missing elements of the system to the model.
In sum we have demonstrated that when MKC is misused as a causal model it neglects co-causing factors with essential influence on IPE outcomes, is therefore an insufficient tool to detect bottlenecks, and edges out any better-suited, viable causal model. This miscast hampers meaningful process evaluations, the subsequent improvement of indicators and interventions, and thereby ultimately the progress in proving beneficial patient, healthcare provider, and system outcomes of IPE and IPC.
Limitations
One limitation of our theoretical critical discussion is that we did not illuminate how hard it is to quantify patient, healthcare provider, and system outcomes from a methodological point of view (e.g. document-based patient data analysis). Neither did we address the extent to which this limits the meaningfulness of IPE/IPC outcome evaluations. However, we claim that the conceptual weakness of missing co-causalities is the deeper root of the evaluation problem, not particular methods, and that methodological issues are solvable as soon as relevant co-causalities are appropriately considered.
Another limitation is that a model is always a simplification. For example, the multi-stage multi-causality model does not include personality traits of team members, intra-personal abilities like self-regulation, or the harmony of personality types within a team, which also play a role in factual IPC. These traits would be difficult to incorporate into the model and gathering such information for evaluations might even be unethical. Similarly, the model does not reflect the influence which the behavior and health literacy of patients (and their families, caregivers, and communities) might have on factual IPC.
A third limitation is that we did not discuss a particular setting in which the use of MKC as mono-linear causal model could work, namely, if IPE champions themselves become IPO managers and subsequently establish factual IPC in their institutions through an appropriate combination of IPE and IPO. In this scenario, the roles of health professionals (as carriers of IPE-induced competence for factual IPC) and managers (as IPO decision makers) overlap – obviously potentially optimal to foster factual IPC. In a certain sense, in this particular case, IPE would lead to IPO and to factual IPC with the potential of “transitively” improving patient, healthcare provider, and system outcomes. However, as we believe that there is no fixed relationship between undergoing IPE and becoming a healthcare manager, we did not pursue this line of argument further, regarding it as an exception.
Conclusions
In our critical discussion we have analyzed previous models of causes and effects in IPC based on the existing literature, proposed a novel “multi-stage multi-causality” model, and demonstrated its explanatory power by establishing that MKC is not suited to foster progress in proving or disproving beneficial final outcomes of IPE and IPC. We conclude with 6 practical, applicable recommendations for future IPE, IPO, and IPC outcome evaluations.
Recommendation 1: stop (mis-)using MKC as a causal model
We have pointed out that the continued use of MKC as causal model seems to severely inhibit the scientific exploration of the co-necessity of IPO and non-interprofessional factors and therefore delays answering the important question whether IPE and IPC actually improve patient, healthcare provider, and system outcomes. As early as 1989, the use of the original Kirkpatrick model as a causal model was questioned [ 55 ]. In 2004, Bates took the position that the continued use of this model is unethical if beneficial results are missed by evaluations due to the narrow focus on outcomes [ 52 ]. Today, we conclude that using MKC as a causal model in IPE, IPO or IPC outcome evaluations should be discontinued.
Recommendation 2: state the causal model under which evaluations of IPE/IPO/IPC operate
Evaluators should make an explicit statement about the causal model under which they design interventions and interpret results, including their additional assumptions about the chain of causes and effects. Knowledge of these assumptions allows the reader to detect inconsistencies – an important element for causal clarification – and should prevent the field of IPE, IPO, and IPC outcome evaluations from getting mired down for even more decades.
Recommendation 3: always include some process evaluation
Even if the primary goal of a study is summative outcome evaluation, evaluators should always include some process evaluation to test the causal model they assume and under which they designed their evaluation, and do so at least until the topic of causality in IPE, IPO, and IPC is fully settled. For example, if an IPE intervention aims at improving factual IPC, evaluators who assume multi-causality would co-evaluate IPO to make sure that IPO is no bottleneck in the evaluated setting.
Recommendation 4: strive for specificity in IPE, IPO, or IPC interventions
If the only goal of an intervention is to improve a certain outcome metric like patient safety, one might initiate a broad, non-specific intervention using best-practice guidelines and all available resources. However, if a goal of the intervention is also to show the existence of specific benefits of IPE, IPO, or IPC in a scientific way, then the multi-causality of outcomes must be taken into account. Intervention designs that change both, interprofessional and non-interprofessional causes of outcomes, must be avoided. For example, if uniprofessional training (a cause outside the domain of IPE/IPO/IPC) is also part of an intervention (e.g. the re-design of the entire workflow in an emergency department in order to enhance patient safety), then this mix of causes obscures the contribution of IPE, IPO, or IPC to the desired effect. Reeves and colleagues euphemistically and aptly call measuring the particular influence of IPE on patient outcomes in such multifaceted interventions a “challenge” [ 10 ]. This example shows why theoretical clarity about the causal model is required to effectively evaluate beneficial outcomes of IPE, IPO, or IPC. Respecting the multi-stage multi-causality of patient, healthcare provider, and system outcomes means designing interventions that improve interprofessional elements only, or, if other components inevitably change as well, to control for those components through comprehensive measurements and/or by adding qualitative methods that allow final outcomes to be causally attributed to IPE, IPO, or IPC.
Recommendation 5: always quantify factual IPC
Recommendations 5 and 6 are our most important recommendations. It is self-explanatory that without the emergence of factual IPC there cannot be any final, globally desirable outcomes of upstream IPE or IPO activities; not until IPE or IPO activities improve factual IPC, does the attempt to evaluate their effects on patient, healthcare provider, and system outcomes start to make any sense. Further, if a positive correlation exists between the quality of factual IPC and patient, healthcare provider, and system outcomes, then correlating factual IPC with final outcomes is the most conclusive way to show it. While the notion that factual IPC is the minimum necessary condition for final outcomes of interprofessional efforts is not new [ 8 , 19 ], the realization that the attached transitivity assumption (that IPE automatically creates the necessary IPC) is wrong, certainly is. As shown above, dismissing transitivity is a cogent consequence of embracing the multi-stage multi-causality of final outcomes. In future evaluations, the quantification of IPE therefore should no longer serve as a surrogate for the quantification of factual IPC. Rather, factual IPC, as an intermediate necessary step towards final outcomes and their most direct cause within the realm of IPE/IPO/IPC, always needs to be evaluated on its own. The same holds true for future evaluations of IPO. IPO interventions do not automatically lead to factual IPC, but first must be shown to improve factual IPC before they can be expected to cause any changes in patient, healthcare provider, and system outcomes. Taken together, a comprehensive measurement of the quality of factual IPC needs to be the centerpiece of any meaningful evaluation of final outcomes achieved by IPE interventions, IPO interventions, combined IPE + IPO interventions, or of factual IPC itself.
From the large number of dimensions of factual IPC (see “Methods”) arises the necessity to evaluate it in detail. Such completeness in the evaluation of factual IPC is important for several reasons:
Obtaining a meaningful sum score: Evaluating factual IPC in a given setting against a hypothetical optimum requires integration of all of its subdimensions into one sum score.
Not missing correlations: If an IPC score does not cover all dimensions of factual IPC, correlations between factual IPC and its effects (or causes) might be missed, even if these relationships truly exist. Example: An evaluation which only includes the dimensions of “mutual respect” and “conflict management” might miss an actually existing correlation between factual IPC and cost effectiveness, mainly driven, say by the dimension of “shared creation of the treatment plan and coordination of its execution”. The result of this evaluation could cast substantial doubt on the existence of positive effects of factual IPC despite them actually being there. Similarly, only a complete set of IPC indicators is suited to reveal potentially diverging effects of different subdimensions of factual IPC on different final outcomes. For example, optimal interprofessional team behavior that maximizes patient safety, might, at the same time, turn out to be less cost effective than multiprofessional team behavior that compromises on patient safety.
Optimizing process evaluation: A complete IPC coverage further provides valuable information for process evaluations aimed at identifying weaknesses in factual IPC. Significant correlations between outcomes and specific subdimensions of IPC can suggest causal relationships and uncover crucial components for successful IPC in a given setting. Focusing on strengthening these subdimensions could help optimize patient, healthcare provider, and system outcomes.
Enabling setting independence and comparisons: Factual IPC is setting-specific [ 11 , 19 , 35 , 60 , 61 ], i.e. the needs of patients for specific medical services differ across different contexts of patient care (e.g. emergency care; acute care; rehabilitation; chronic care; multimorbid patients; palliative care). As a consequence, different subdimensions of factual IPC contribute to the outcomes of interest to a variable degree depending on the specific healthcare setting. Even within a specific setting, requirements and behaviors necessary for effective IPC can vary due to the specifics of the case, e.g. the particular rareness or severity of the patient’s condition. Assumptions made prior to an evaluation about which subdimensions of factual IPC are most important in a specific setting therefore should not preclude the exploratory evaluation of the other subdimensions. If an evaluation grid misses IPC subdimensions, it may work well in one setting but fail in others. Hence, the completeness of indicators for factual IPC in an evaluation instrument creates setting independence, eliminates the burden of adjusting the included IPC subdimensions every time a new healthcare setting is evaluated, and allows unchanged evaluation instruments to be re-used in subsequent studies (called for by e.g. [ 16 ]) as well as multi-center studies (called for by e.g. [ 2 ]). A starting point for the operationalization of factual IPC including all of its subdimensions is provided in our definition of factual IPC (see “Methods”; a validated evaluation toolbox based on this operationalization will be published elsewhere; a published tool which also covers all subdomains of factual IPC, with a focus on adaptive leadership, is the AITCS [ 39 ]).
Recommendation 6: use a step-by-step approach for proving benefits of IPE and IPO
The multi-stage multi-causality of patient, healthcare provider, and system outcomes naturally implies that the process of proving that IPE or IPO benefits final outcomes could be broken down into discrete steps. The key idea is to evaluate the impact of interprofessional activities on each of the subsequent levels in the causal chain while controlling for non-interprofessional factors. Showing the effects of IPE on IPC competencies, the effects of IPC competencies on factual IPC, and the effects of factual IPC on patient, healthcare provider, and system outcomes then becomes three different research agendas that can be processed independently. If it can be shown in the first of these research agendas that IPE leads to learning (by controlling for non-interprofessional learning-related factors), and in the second, independent research agenda, that learning leads to improved factual IPC (controlling for IPO), and in the third research agenda that factual IPC leads to desired final outcomes (controlling for co-conditions for final outcomes like uniprofessional competencies), then the benefit of IPE on patient, healthcare provider, and system outcomes is ultimately proved. If this approach fails, then at least it will be exactly revealed where the chain of effects breaks down. The same holds true for IPO: Show that IPO interventions lead to work processes and/or favorable institutional conditions which support factual IPC, separately show that these work processes and conditions lead to improved factual IPC (if co-conditions for factual IPC like IPE are present), and show that better factual IPC leads to an improvement of final outcomes; then the positive impact of IPO is verified.
By covering the entire process, this “step-by-step” approach could build a compelling case for how interprofessional interventions lead to desired final outcomes. It further could markedly simplify the agenda of interprofessional research because it takes the burden of showing the effect of one particular IPE or IPO intervention on one particular final outcome off the shoulders of evaluators. After breaking down the evaluation task into separate steps that prove the impact from link to link, researchers are free to work on one step at a time only.
The presented critical discussion advances the theoretical foundations of evaluations in the field of IPE, IPO and IPC. To improve patient-centered care by means of IPC, one needs to think bigger than just training of healthcare professionals in the competencies and mindsets required for effective IPC; work processes also have to be established and optimized in a setting-dependent manner to allow for factual IPC to happen. Besides IPC, factors like discipline-specific knowledge of health professionals or administrative aspects of patient management have to be optimized, too, to achieve optimal patient, healthcare provider, and system outcomes.
By sharing the multi-stage multi-causality model and its pertinent theoretical clarification we hope to contribute to a deeper understanding of causes and effects in interprofessional collaboration, to answer the repeated call in the research community for improved theory in this field, to explain difficulties faced by past evaluations, and to provide helpful guidance for future research studies. Our key recommendations for future evaluations of interprofessional outcomes are to focus on a comprehensive evaluation of factual IPC as the most fundamental metric and to deploy a step-by-step research agenda with the overarching goal of proving beneficial patient, healthcare provider, and system outcomes related to IPE, IPO, and IPC. With these contributions, we hope to help healthcare institutions improve their evaluations of IPE, IPO, and IPC, ultimately benefiting health, healthcare provider, and system outcomes.
Availability of data and materials
No datasets were generated or analysed during the current study.
Abbreviations
- Interprofessional collaboration
- Interprofessional education
- Interprofessional organization
- Modified Kirkpatrick classification
World Health Organization
Körner M, Bütof S, Müller C, Zimmermann L, Becker S, Bengel J. Interprofessional teamwork and team interventions in chronic care: A systematic review. J Interprof Care. 2016;30(1):15–28.
Article Google Scholar
Reeves S, Fletcher S, Barr H, Birch I, Boet S, Davies N, et al. A BEME systematic review of the effects of interprofessional education: BEME Guide No. 39. Med Teach. 2016;38(7):656–68.
Reeves S, Pelone F, Harrison R, Goldman J, Zwarenstein M. Interprofessional collaboration to improve professional practice and healthcare outcomes. Cochrane Database Syst Rev. 2017. https://www.cochranelibrary.com/cdsr/doi/10.1002/14651858.CD000072.pub3/full . Accessed 23 Feb 2024.
Sibbald B, Bojke C, Gravelle H. National survey of job satisfaction and retirement intentions among general practitioners in England. BMJ. 2003;326(7379):22.
Cowin LS, Johnson M, Craven RG, Marsh HW. Causal modeling of self-concept, job satisfaction, and retention of nurses. Int J Nurs Stud. 2008;45(10):1449–59.
Paradis E, Whitehead CR. Beyond the lamppost: a proposal for a fourth wave of education for collaboration. Acad Med. 2018;93(10):1457.
Holly C, Salmond S, Saimbert M. Comprehensive Systematic Review for Advanced Practice Nursing. 2nd ed. New York: Springer Publishing Company; 2016.
Google Scholar
IOM (Institute of Medicine). Measuring the impact of interprofessional education on collaborative practice and patient outcomes. Washington, DC: The National Academies Press; 2015.
Brandt B, Lutfiyya MN, King JA, Chioreso C. A scoping review of interprofessional collaborative practice and education using the lens of the Triple Aim. J Interprof Care. 2014;28(5):393–9.
Reeves S, Perrier L, Goldman J, Freeth D, Zwarenstein M. Interprofessional education: effects on professional practice and healthcare outcomes (Review) (Update). Cochrane Database Syst Rev. 2013. https://www.cochranelibrary.com/cdsr/doi/10.1002/14651858.CD002213.pub3/full . Accessed 23 Feb 2024.
Hammick M, Freeth D, Koppel I, Reeves S, Barr H. A best evidence systematic review of interprofessional education: BEME Guide no. 9. Med teach. 2007;29(8):735–51.
Zwarenstein M, Reeves S, Perrier L. Effectiveness of pre-licensure interprofessional education and post-licensure collaborative interventions. J Interprof Care. 2005;19(Suppl 1):148–65.
Barr H, Hammick M, Koppel I, Reeves S. Evaluating interprofessional education: two systematic reviews for health and social care. Br Edu Res J. 1999;25(4):533–44.
Yardley S, Dornan T. Kirkpatrick’s levels and education ‘evidence’. Med Educ. 2012;46(1):97–106.
Roland D. Proposal of a linear rather than hierarchical evaluation of educational initiatives: the 7Is framework. J Educ Eval Health Profess. 2015;12:35.
Thannhauser J, Russell-Mayhew S, Scott C. Measures of interprofessional education and collaboration. J Interprof Care. 2010;24(4):336–49.
Reeves S, Goldman J, Gilbert J, Tepper J, Silver I, Suter E, et al. A scoping review to improve conceptual clarity of interprofessional interventions. J Interprof Care. 2011;25(3):167–74.
Suter E, Goldman J, Martimianakis T, Chatalalsingh C, DeMatteo DJ, Reeves S. The use of systems and organizational theories in the interprofessional field: Findings from a scoping review. J Interprof Care. 2013;27(1):57–64.
Havyer RD, Wingo MT, Comfere NI, Nelson DR, Halvorsen AJ, McDonald FS, et al. Teamwork assessment in internal medicine: a systematic review of validity evidence and outcomes. J Gen Intern Med. 2014;29(6):894–910.
Reeves S, Boet S, Zierler B, Kitto S. Interprofessional education and practice guide No. 3: Evaluating interprofessional education. J Interprofess Care. 2015;29(4):305–12.
McNaughton SM, Flood B, Morgan CJ, Saravanakumar P. Existing models of interprofessional collaborative practice in primary healthcare: a scoping review. J Interprof Care. 2021;35(6):940–52.
Barr H, Freeth D, Hammick M, Koppel I, Reeves S. Evaluations of Interprofessional Education: A United Kingdom Review for Health and Social Care. Centre for the Advancement of Interprofessional Education and The British Educational Research Association. 2000. https://www.caipe.org/resources/publications/barr-h-freethd-hammick-m-koppel-i-reeves-s-2000-evaluations-of-interprofessional-education . Accessed 23 Feb 2024.
Barr H, Koppel I, Reeves S, Hammick M, Freeth D. Effective Interprofessional Education: Argument, assumption, and evidence. Wiley-Blackwell; 2005.
Baethge C, Goldbeck-Wood S, Mertens S. SANRA—a scale for the quality assessment of narrative review articles. Research integrity and peer review. 2019;4(1):1–7.
CAIPE (Centre for the Advancement of Interprofessional Education). About CAIPE, s. v. “Defining Interprofessional Education”. https://www.caipe.org/about . Accessed 23 Feb 2024.
D’amour D, Oandasan I. Interprofessionality as the field of interprofessional practice and interprofessional education: An emerging concept. J Interprof Care. 2005;19(Suppl 1):8–20.
Körner M, Wirtz MA. Development and psychometric properties of a scale for measuring internal participation from a patient and health care professional perspective. BMC Health Serv Res. 2013;13(1):374.
Körner M, Wirtz MA, Bengel J, Göritz AS. Relationship of organizational culture, teamwork and job satisfaction in interprofessional teams. BMC Health Serv Res. 2015;15(1):243.
World Health Organization. Framework for Action on Interprofessional Education & Collaborative Practice. 2010. https://www.who.int/publications/i/item/framework-for-action-on-interprofessional-education-collaborative-practice . Accessed 23 Feb 2024.
Wei H, Horns P, Sears SF, Huang K, Smith CM, Wei TL. A systematic meta-review of systematic reviews about interprofessional collaboration: facilitators, barriers, and outcomes. J Interprof Care. 2022;36(5):735–49.
Morey JC, Simon R, Jay GD, Wears RL, Salisbury M, Dukes KA, et al. Error reduction and performance improvement in the emergency department through formal teamwork training: evaluation results of the MedTeams project. Health Serv Res. 2002;37(6):1553–81.
D’Amour D, Ferrada-Videla M, San Martin Rodriguez L, Beaulieu M-D. The conceptual basis for interprofessional collaboration: core concepts and theoretical frameworks. J interprofess care. 2005;19(1):116–31.
San Martín-Rodríguez L, Beaulieu M-D, D’Amour D, Ferrada-Videla M. The determinants of successful collaboration: a review of theoretical and empirical studies. J Interprof Care. 2005;19(Suppl 1):132–47.
Guise J-M, Deering SH, Kanki BG, Osterweil P, Li H, Mori M, et al. Validation of a tool to measure and promote clinical teamwork. Simulation in Healthcare. 2008;3(4):217–23.
Orchard C, Bainbridge L, Bassendowski S.. A national interprofessional competency framework. Vancouver: Canadian Interprofessional Health Collaborative; University of British Columbia; 2010; Available online: http://ipcontherun.ca/wp-content/uploads/2014/06/National-Framework.pdf . Accessed 29 May 2024.
Oishi A, Murtagh FE. The challenges of uncertainty and interprofessional collaboration in palliative care for non-cancer patients in the community: a systematic review of views from patients, carers and health-care professionals. Palliat Med. 2014;28(9):1081–98.
Valentine MA, Nembhard IM, Edmondson AC. Measuring teamwork in health care settings: a review of survey instruments. Med Care. 2015;53(4):e16–30.
Lie DA, Richter-Lagha R, Forest CP, Walsh A, Lohenry K. When less is more: validating a brief scale to rate interprofessional team competencies. Med Educ Online. 2017;22(1):1314751.
Orchard C, Pederson LL, Read E, Mahler C, Laschinger H. Assessment of interprofessional team collaboration scale (AITCS): further testing and instrument revision. J Contin Educ Heal Prof. 2018;38(1):11–8.
Begun JW, White KR, Mosser G. Interprofessional care teams: the role of the healthcare administrator. J Interprof Care. 2011;25(2):119–23.
Weaver SJ, Salas E, King HB. Twelve best practices for team training evaluation in health care. The Joint Commission Journal on Quality and Patient Safety. 2011;37(8):341–9.
Thistlethwaite J, Kumar K, Moran M, Saunders R, Carr S. An exploratory review of pre-qualification interprofessional education evaluations. J Interprof Care. 2015;29(4):292–7.
Gilbert JH, Yan J, Hoffman SJ. A WHO report: framework for action on interprofessional education and collaborative practice. J Allied Health. 2010;39(3):196–7.
Taylor & Francis Online. http://www.tandfonline.com (2024). Accessed 23 Feb 2024.
Sockalingam S, Tan A, Hawa R, Pollex H, Abbey S, Hodges BD. Interprofessional education for delirium care: a systematic review. J Interprof Care. 2014;28(4):345–51.
Folland S, Goodman AC, Stano M. The Economics of Health and Health Care. 7th ed. New York, NY: Routledge; 2016.
Book Google Scholar
Hayes B, Bonner A, Pryor J. Factors contributing to nurse job satisfaction in the acute hospital setting: a review of recent literature. J Nurs Manag. 2010;18(7):804–14.
Curran V, Reid A, Reis P, Doucet S, Price S, Alcock L, et al. The use of information and communications technologies in the delivery of interprofessional education: A review of evaluation outcome levels. J Interprof Care. 2015;29(6):541–50.
Danielson J, Willgerodt M. Building a theoretically grounded curricular framework for successful interprofessional education. A J Pharmaceut Educ. 2018;82(10):1133–9.
Kirkpatrick D, Kirkpatrick J. Evaluating Training Programs: The Four Levels. 3rd ed. San Francisco, CA: Berrett-Koehler Publishers; 2006.
Kirkpatrick J, Kirkpatrick W. An Introduction to The New World Kirkpatrick Model. Kirkpatrick Partners. 2021. http://www.kirkpatrickpartners.com/wp-content/uploads/2021/11/Introduction-to-the-Kirkpatrick-New-World-Model.pdf . Accessed 30 Nov 2023.
Bates R. A critical analysis of evaluation practice: the Kirkpatrick model and the principle of beneficence. Eval Program Plann. 2004;27(3):341–7.
Pawson R, Tilley N. Realistic evaluation. 1st ed. London: Sage Publications Ltd; 1997.
Holton EF III. The flawed four-level evaluation model. Hum Resour Dev Q. 1996;7(1):5–21.
Alliger GM, Janak EA. Kirkpatrick’s levels of training criteria: Thirty years later. Pers Psychol. 1989;42(2):331–42.
Goldstein IL, Ford JK. Training in organisations: Needs assessment, development, and evaluation. 4th ed. Belmont, CA: Wadsworth; 2002.
Bordage G. Conceptual frameworks...: What lenses can they provide to medical education? Investigación en educación médica. 2012;1(4):167–9.
Belfield C, Thomas H, Bullock A, Eynon R, Wall D. Measuring effectiveness for best evidence medical education: a discussion. Med Teach. 2001;23(2):164–70.
Johnson N. Simply complexity: A clear guide to complexity theory. London: Oneworld Publications; 2009.
Retchin SM. A conceptual framework for interprofessional and co-managed care. Acad Med. 2008;83(10):929–33.
Schmitz C, Atzeni G, Berchtold P. Challenges in interprofessionalism in Swiss health care: the practice of successful interprofessional collaboration as experienced by professionals. Swiss Med Wkly. 2017;147: w14525.
Download references
Acknowledgements
Not applicable.
The Swiss Federal Office of Public Health partly funded this study with the contractual mandate “Bildung und Berufsausübung: Evaluationsinstrumente”.
Author information
Authors and affiliations.
Institute for Medical Education, Department for Assessment and Evaluation, University of Bern, Bern, Switzerland
Florian B. Neubauer, Felicitas L. Wagner, Andrea Lörwald & Sören Huwendiek
You can also search for this author in PubMed Google Scholar
Contributions
FBN, FLW, AL and SH made substantial contributions to the conception of the work and to the literature searches. FBN wrote the first full draft of the manuscript. All authors improved the draft. All authors read and approved the final manuscript.
Corresponding author
Correspondence to Florian B. Neubauer .
Ethics declarations
Ethics approval and consent to participate, consent for publication, competing interests.
The authors declare no competing interests.
Additional information
Publisher's note.
Springer Nature remains neutral with regard to jurisdictional claims in published maps and institutional affiliations.
Rights and permissions
Open Access This article is licensed under a Creative Commons Attribution 4.0 International License, which permits use, sharing, adaptation, distribution and reproduction in any medium or format, as long as you give appropriate credit to the original author(s) and the source, provide a link to the Creative Commons licence, and indicate if changes were made. The images or other third party material in this article are included in the article's Creative Commons licence, unless indicated otherwise in a credit line to the material. If material is not included in the article's Creative Commons licence and your intended use is not permitted by statutory regulation or exceeds the permitted use, you will need to obtain permission directly from the copyright holder. To view a copy of this licence, visit http://creativecommons.org/licenses/by/4.0/ . The Creative Commons Public Domain Dedication waiver ( http://creativecommons.org/publicdomain/zero/1.0/ ) applies to the data made available in this article, unless otherwise stated in a credit line to the data.
Reprints and permissions
About this article
Cite this article.
Neubauer, F.B., Wagner, F.L., Lörwald, A. et al. Sharpening the lens to evaluate interprofessional education and interprofessional collaboration by improving the conceptual framework: a critical discussion. BMC Med Educ 24 , 615 (2024). https://doi.org/10.1186/s12909-024-05590-0
Download citation
Received : 29 November 2023
Accepted : 22 May 2024
Published : 04 June 2024
DOI : https://doi.org/10.1186/s12909-024-05590-0
Share this article
Anyone you share the following link with will be able to read this content:
Sorry, a shareable link is not currently available for this article.
Provided by the Springer Nature SharedIt content-sharing initiative
- Outcome evaluation
- Process evaluation
- Causal model
- Conceptual framework
- Terminology
BMC Medical Education
ISSN: 1472-6920
- Submission enquiries: [email protected]
- General enquiries: [email protected]
- Open access
- Published: 03 June 2024
Understanding the integration of artificial intelligence in healthcare organisations and systems through the NASSS framework: a qualitative study in a leading Canadian academic centre
- Hassane Alami 1 , 2 , 3 , 4 ,
- Pascale Lehoux 1 , 2 ,
- Chrysanthi Papoutsi 4 ,
- Sara E. Shaw 4 ,
- Richard Fleet 5 , 6 &
- Jean-Paul Fortin 5 , 6
BMC Health Services Research volume 24 , Article number: 701 ( 2024 ) Cite this article
324 Accesses
1 Altmetric
Metrics details
Artificial intelligence (AI) technologies are expected to “revolutionise” healthcare. However, despite their promises, their integration within healthcare organisations and systems remains limited. The objective of this study is to explore and understand the systemic challenges and implications of their integration in a leading Canadian academic hospital.
Semi-structured interviews were conducted with 29 stakeholders concerned by the integration of a large set of AI technologies within the organisation (e.g., managers, clinicians, researchers, patients, technology providers). Data were collected and analysed using the Non-Adoption, Abandonment, Scale-up, Spread, Sustainability (NASSS) framework.
Among enabling factors and conditions, our findings highlight: a supportive organisational culture and leadership leading to a coherent organisational innovation narrative; mutual trust and transparent communication between senior management and frontline teams; the presence of champions, translators, and boundary spanners for AI able to build bridges and trust; and the capacity to attract technical and clinical talents and expertise.
Constraints and barriers include: contrasting definitions of the value of AI technologies and ways to measure such value; lack of real-life and context-based evidence; varying patients’ digital and health literacy capacities; misalignments between organisational dynamics, clinical and administrative processes, infrastructures, and AI technologies; lack of funding mechanisms covering the implementation, adaptation, and expertise required; challenges arising from practice change, new expertise development, and professional identities; lack of official professional, reimbursement, and insurance guidelines; lack of pre- and post-market approval legal and governance frameworks; diversity of the business and financing models for AI technologies; and misalignments between investors’ priorities and the needs and expectations of healthcare organisations and systems.
Thanks to the multidimensional NASSS framework, this study provides original insights and a detailed learning base for analysing AI technologies in healthcare from a thorough socio-technical perspective. Our findings highlight the importance of considering the complexity characterising healthcare organisations and systems in current efforts to introduce AI technologies within clinical routines. This study adds to the existing literature and can inform decision-making towards a judicious, responsible, and sustainable integration of these technologies in healthcare organisations and systems.
Peer Review reports
According to the Organisation for Economic Co-operation and Development (OECD), artificial intelligence (AI) refers to “a machine-based system that, for explicit or implicit objectives, infers, from the input it receives, how to generate outputs such as predictions, content, recommendations, or decisions that can influence physical or virtual environments. Different AI systems vary in their levels of autonomy and adaptiveness after deployment” [ 1 ]. Unlike conventional software, many AI systems indeed have learning capabilities and self-correcting error mechanisms that allow them to improve the accuracy of their results based on the feedback they receive [ 1 , 2 ].
There are many application areas for AI in healthcare, for example: diagnosis, treatment, monitoring (e.g., chronic diseases), and patient compliance [ 3 ]. In certain experimental settings, AI technologies have been shown to be more effective than clinicians (e.g., diagnostic accuracy, more personalised diagnostics) [ 4 , 5 , 6 , 7 ]. Several have already been approved for clinical use in real-world care and services [ 8 ]. These technologies are seen as a lever for evidence-based clinical decision-making and practice and for value-based care and services [ 9 , 10 , 11 ]. Research indicates their potential to contribute to better monitoring, detection, and diagnosis of diseases, to the reduction of clinical risk, and to the discovery of new drugs and treatments [ 4 , 9 , 12 , 13 , 14 ]. The use of AI technologies could help to reduce diagnostic and therapeutic errors [ 2 ], contribute to the optimisation of clinicians’ work, and help reduce waiting times by reorganising clinical and administrative tasks, and supporting coordination [ 10 , 14 ]. Many scholars also argue that AI technologies could contribute to reducing healthcare costs by decreasing hospital (re)admissions, medical visits, and treatments [ 14 , 15 ].
A predominant and enthusiastic discourse in the academic literature and media reports is that AI technologies will revolutionise and radically change healthcare in the coming years [ 2 , 16 , 17 , 18 ]. There is an explosion of AI offerings in the market [ 19 ]. In 2018, the global AI market in healthcare was valued at around US$1.4 billion and is expected to grow to US$17.8 billion by 2025 [ 14 ]. In North America, the market for AI in healthcare had exceeded US$1.15 billion by 2020 [ 14 ]. In this context, healthcare organisations and systems are increasingly being solicited (or even pressured) to integrate these technologies, even when evidence of real clinical added value is lacking and many social and ethical as well as adoption, routinisation, and practical issues remain to be clarified [ 16 , 18 ]. According to Topol (2019), who reviewed healthcare workforce readiness for a digital future: “Despite all the promises of AI technology, there are formidable obstacles and pitfalls. The state of AI hype has far exceeded the state of AI science, especially when it pertains to validation and readiness for implementation in patient care” [ 4 ]. Liu et al. (2019) reported that few published studies on AI had results from real-world healthcare contexts [ 20 ]. These findings were corroborated during the COVID-19 pandemic [ 21 , 22 , 23 ]. Wynants et al. (2020) identified 232 AI models for prediction or diagnosis of COVID-19, none of which were appropriate for clinical use and only two showing potential for future clinical use [ 24 ]. Roberts et al. (2021) analysed 415 AI models for COVID-19 detection and concluded similarly [ 25 ].
This gap between the promise and reality of AI technologies in healthcare could be explained by the fact that efforts have historically focused on technology development, market penetration, and commercialisation. Limited work has been done to look specifically at the conditions and factors necessary for the integration of AI technologies into routine clinical care [ 14 , 17 ]. While technical problems (e.g., performance, unreliability) have been regularly put forward as a reason for the difficulties of integrating these technologies into healthcare organisations and systems [ 26 ], they explain only a small part of the problem. Broader socio-technical conditions and factors rather explain many of these difficulties [ 18 , 26 ].
The social scientific literature on health innovations has shown that the introduction of technologies into healthcare organisations and systems is a complex phenomenon [ 27 ]. This is particularly true for many AI technologies, which are sometimes described in the medical literature as disruptive innovation due to their evolving and autonomous nature [ 28 , 29 , 30 ]. Their implementation and use may require rethinking and/or redesigning existing governance frameworks and care models as well as new clinical, organisational, regulatory, and technological processes, business models, capabilities, and skills [ 18 ]. These changes involve, and impact on, a variety of stakeholders who may have divergent or even antagonistic expectations, goals, and visions towards technology [ 31 , 32 , 33 , 34 , 35 , 36 ].
To contribute to addressing current knowledge gaps, the goal of this study is to explore and understand the challenges of integrating AI technologies within a large academic hospital in Canada (referred to as “the City hospital”). We aim to answer two questions:
How do multiple interacting influences facilitate and constrain the integration of AI technologies within the City hospital?
What learning can we derive for policy and practice for better integration of AI technologies in healthcare organisations and systems?
The study is not limited to a specific AI technology or clinical area but encompasses all 87 AI technology-based initiatives developed and used to varying extent in this hospital. Where relevant, we specify the type of AI involved to contextualise the factors, conditions, or challenges described.
Theoretical framework
To make sense of the complexity underpinning the AI integration efforts in the City hospital, we used an adapted version of the Nonadoption, Abandonment, and challenges to Scale-up, Spread, and Sustainability (NASSS) framework developed by Greenhalgh et al. [ 27 ], which supports an exhaustive sociotechnical approach to health innovation. Following this adapted version, we present the seven dimensions of the framework in a different order from the original version in order to make sense of the narrative within the organisation studied, thereby covering: 1) the organisation; 2) the condition(s) or illness; 3) the technology or technologies; 4) the value proposition; 5) the adopter system(s) (e.g., staff, patient, caregivers); 6) embedding and adaptation over time; and 7) the wider system [ 27 ]. See Fig. 1 for a description of the seven dimensions.
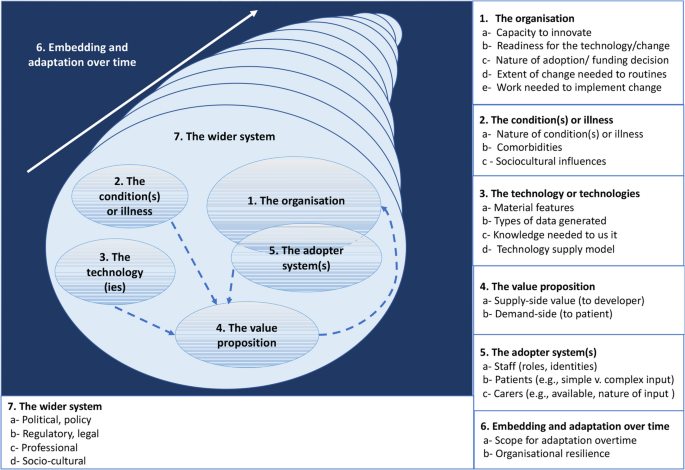
An adapted version of the NASSS framework (adapted from Greenhalgh et al. [ 27 ])
There were many reasons for adopting the NASSS framework over other frameworks. First, it stems from a hermeneutic systematic review, supported by empirical case studies of technology implementation in healthcare [ 27 , 37 ], and its key strength lies in its synthesis of 28 technology implementation frameworks, that is informed by several theoretical perspectives [ 27 , 37 ]. Second, it was developed to fill an important gap “on technology implementation—specifically, to address not just adoption but also nonadoption and abandonment of technologies and the challenges associated with moving from a local demonstration project to one that is fully mainstreamed and part of business as usual locally (scale-up), transferable to new settings (spread), and maintained long term through adaptation to context over time (sustainability)” [ 27 , 37 ]. Third, in contrast to the deterministic logic of many existing frameworks, the NASSS framework is characterised by its dynamic aspect, particularly in terms of interaction and adaptation over time. Indeed, a large part of the literature in the field has a tendency to “assume that the issues to be addressed [are] simple or complicated (hence knowable, predictable, and controllable) rather than complex (that is, inherently not knowable or predictable but dynamic and emergent)” [ 27 , 37 ]. Therefore, major failures of large and ambitious technology projects may be underestimated and their complexity for healthcare organisations and systems tossed away [ 27 , 37 ]. Fourth, whereas decision-makers and technology promoters as well as a part of the specialised literature often adopt a linear, predictable, and rational vision of change [ 38 ], the sociotechnical stance of the NASSS framework highlights the importance of examining how technology and the changes associated with it are perceived, interpreted, negotiated, and enacted by individuals and groups [ 33 , 39 , 40 ]. The same applies to AI technologies that may require transformation and/or redesign of services, a profound reconfiguration of clinical and organisational practices, and challenges to professional identities and practices [ 17 , 33 , 40 ]. Certain types of AI technologies also evolve autonomously over time – a particular characteristic that can be explicitly conceptualised through the NASSS framework [ 27 , 41 ]. Overall, the NASSS framework was developed to be used reflectively, to stimulate conversation and generate ideas, which is one of our study’s aspirations.
We conducted a qualitative study within the City hospital (Quebec, Canada) [ 42 ]. The latter had initiated several projects to integrate AI technologies in its care and service offer. Decision-makers and managers expressed a need for (independent) insights into the micro-, meso-, and macro-level systemic implications of the integration of these technologies within the organisation [ 43 ].
Presentation of the organisation
The City hospital is one of the largest academic hospitals in Canada. It offers specialised and sub-specialised services to adult patients. It treats around 500,000 patients annually. It employs over 14,000 people. It also houses one of the largest medical research centres in the country, with an academic mission to produce and disseminate knowledge and research results. It also presents itself as an organisation with state-of-the-art facilities and equipment. It has been ranked by the U.S-based magazine Newsweek as one of the world’s top 250 Best Smart Hospitals for 2021. It hosts one of the largest annual digital innovation events in Canada.
At the time of the study, the City hospital had over 115 digital health projects (Table 1 ), with 87 of these involving AI. Around 95% (≈82/87) of the AI technologies were in the development/experimentation or early implementation phase. Only four were integrated into services. Approximately 72% (≈62/87) of the AI technologies identified within the organisation were for the diagnosis, treatment and/or monitoring of complex chronic or acute conditions: cancers, neurological (e.g., epilepsy), and ocular conditions.
Recruitment
We identified a purposive sample of key stakeholders, with the aim of capturing diverse perspectives and experiences [ 44 ]. We conducted internet searches and consulted reports and documents produced by the City hospital to identify potential participants, who were drawn from distinct roles and varied levels of involvement in the development, implementation, and use of AI technologies.
A personalised invitation email was sent to each potential participant explaining the project and why they were invited to participate. Two reminders were sent in case of non-response. Respondents were invited to indicate other participants (i.e., snowballing) [ 45 ]. This resulted in a sample of senior and middle managers/decision-makers, clinicians (e.g., physicians, nurses), clinicians/informaticians/researchers, technology assessment specialists, procurement specialists, lawyers, patients, and technology providers. Patients were identified through patient partners (volunteers) collaborating with the City hospital. Of the 42 invitations sent, 29 people agreed to participate. Table 2 shows participant profiles, many of whom cumulated multiple professional and/or experiential backgrounds.
Data collection
Between March and July 2021, the first author (HA) conducted 29 interviews in French (27) and English (2), using the Zoom™ videoconferencing platform (interviews lasted 30–90 min). Prior to the interview, a consent form summarising the objectives of the project was shared. Interviews were audio recorded with the permission of the participant and transcribed verbatim by HA. The questions were formulated according to the dimensions of the NASSS framework and informed by documents shared by the City hospital (e.g., list of projects and technologies). HA first tested the qualitative interview guide with two respondents prior to the start of the study. No major revision of the initial version of the guide was required. He took notes during and after the interviews and subsequently used them to contextualise the analyses. The interview guide slightly evolved depending on the participants’ responses as new information emerged. By adapting the interview guide, we were able to capture both expected and unanticipated tensions and practical challenges, grounding the discussion in participant experiences to avoid vague or abstract responses. Given that the same person (HA) co-developed the guide and conducted the interviews in French and English, this minimised the risk of variability that could arise from having different people collecting data in different languages. Interview data and document analysis, alongside our knowledge of the context (team members have been involved in various research and evaluation projects on digital technologies and innovations in Quebec and Canadian healthcare organisations and systems for several years) guided triangulation of data sources [ 46 ].
Data analysis
Data were coded and analysed with Dedoose™ software. HA performed the first round of analysis and developed a preliminary coding scheme. In the second round, the scheme was refined, challenged, and discussed iteratively by the second author (PL) [ 43 ]. We conducted a deductive-inductive thematic analysis. The deductive analysis was guided by the NASSS framework (Fig. 1 ) [ 27 ]. Drawing on its seven dimensions, we created codes to capture the micro, meso and macro-level challenges and implications associated with the integration of AI technologies in the City hospital. The inductive analysis aimed to capture emerging themes not covered by the framework [ 44 , 47 ]. After agreeing on the different themes identified, we concluded that none required the addition of new dimensions, as all identified themes fitted within the NASSS framework. Data saturation was reached for the themes and observations reported in the findings. Given the importance of context in the NASSS framework, we sought to understand and clarify the contextual elements where respondents had different views or judgements. We decided not to disclose certain details either because the participants requested it or to ensure confidentiality. However, this information was useful to contextualise and better understand other findings and events. Our findings are illustrated with participant quotes organised around key themes of the NASSS framework (translated from French to English when needed) (Table 5 in Appendix ). The letter P used in quotes refers to “participant”, followed by numbers designating the order in which interviews were transcribed.
Findings are reported as a narrative account [ 48 ]. This is critical in allowing us to capture the complexity of the subject, the explanatory and interpretative dimensions, and the varied stories and perspectives gained from participants in making sense of the issues around the adoption of AI technologies.
We present the findings according to the seven dimensions of the adapted version of the NASSS framework (Fig. 1 ). To ensure fluidity in the presentation of the findings, the participant roles are used as a general category to help the reader identify certain tensions between the viewpoints and perspectives expressed. In this sense, there is no pretension of generalisation given the small number of respondents in each category. The analyses are intended primarily to provide high-level dynamics related to each dimension of the NASSS framework and not those specific to the types of AI discussed.
The organisation
For the technology providers we interviewed, the City hospital has several internationally renowned clinicians, both in the clinical field and in the use of AI. Several managers and clinicians also reported that senior management is known to value and encourage technological innovation, which has led to the creation of a “data lake” that allows the integration of data from different clinical systems (e.g., clinical records, laboratories, vital signs, imaging), which is a major asset for the development and/or validation of certain AI technologies. According to technology providers, access to the specialised expertise of clinicians who know the data is as important as access to the data itself. These clinicians play an important role as a trusted guarantor (or legitimising authority) for AI with other clinicians, decision-makers/managers, patients, and citizens. In the words of one clinician-manager, the relationship and communication between these clinicians and the City hospital’s senior management is generally perceived as positive. He pointed out that this synergy helps to mitigate some of the issues and conflicting visions and expectations of AI.
According to a technology provider, because of the characteristics of Quebec’s single-payer and universal health system, the City hospital allows for holistic management of patients suffering from several pathologies or requiring different care and treatments. He added that this unique advantage enables the development of AI technologies with a broad spectrum of action (i.e., compared to those developed in contexts where care is fragmented between different hospitals and/or clinics). Despite this asset, there is a broad agreement among the interviewees that the City hospital is characterised by significant complexity that has the potential to impact its ability to realise the value promise of AI technologies.
Use of AI technologies in the City hospital necessarily involves different departments, committees, and stakeholders (e.g., Information technology -IT- department, procurement department, project office, professional services department). According to several managers, clinicians, and industry providers, the roles and mandates for these different groups and stakeholders are not always clear. Coordination and communication between teams and/or departments are sometimes difficult or non-existent. According to a manager, this results in confusion and tension about expectations, visions, and responsibilities. He pointed out that difficulties experienced by some AI projects were due to a department or committee not being engaged at the right time (e.g., as a result of legal and/or procurement framework, Cloud storage space, professional services department). For managers and clinicians, a horizontal body should have been established to coordinate and ensure coherence and communication between the different initiatives and stakeholders, with the aim enabling mutual effort, coordination, and accountability. For another manager, by ensuring an initial screening of technologies proposed by industry, such a body would avoid the influx of useless technologies to clinical teams and associated time and resource costs.
Both industry and organisation respondents agree that the City hospital doesn’t always have the capacity to meet the initial and recurring costs and investments required for the successful integration of AI. To overcome this funding problem, at least partly, an interviewee told us that the organisation is obliged to open its doors to industry for co-development, or as a testing ground, of AI technologies. This sub-contracting allows the City hospital to benefit from a free user licence for a fixed period or for life. However, it was reported that this partnership contracts model (e.g., co-development or serving as a testing ground for the industry) is likely to lock the organisation into a technology-centric logic, with no real margin of manoeuvre to choose technologies that really meet its needs. There are multiple projects under this partnership model within the organisation. Several technologies could simply end up being only partially developed because the technology provider has withdrawn, or the technology was abandoned. Within such a context, several managers and clinicians recognise that it is difficult to create a real organising vision that supports and enables AI within the City hospital.
According to managers and clinicians, these partnerships with industry imply an over-solicitation of the clinical teams as, in addition to their clinical and administrative work, they must dedicate time to testing and experimenting with the various technologies presented by the technology providers. In this regard, several organisation and industry respondents pointed out that clinicians in the City hospital are not valued or remunerated for their contribution to the development and/or experimentation of technologies. It is not uncommon for some clinicians to feel that industry benefits from their clinical expertise without any real return on investment for them. Technology providers interviewed refuted this point. For them, the difficulties in integrating their technologies into the organisation are essentially due to the opposition of some influential clinician-researchers who are themselves developing in-house similar technologies. In the words of one industry respondent, this is a conflict of interest and unfair competition. Nonetheless, technology providers support the importance of creating incentives to encourage clinicians to collaborate with industry. On their part, several clinicians and managers consider that the organisation should value in-house initiatives more highly because they emerge from the needs and expectations of the field. However, there is agreement that the organisation does not have the financial and human resources to support these initiatives. In addition, according to one manager, as a public entity, the City hospital does not have a mandate to develop and/or commercialise technologies. At some point, a company would have to be involved to ensure commercialisation.
Managers, clinicians, and industry acknowledge that the nature and extent of the changes associated with the integration of AI within the organisation are still largely unknown. For example, it is very difficult to assess financial implications over time. Two managers reported that the City hospital paid an additional CA$20,000 to CA$30,000/year for the storage and management of its data. This cost was not initially budgeted but subsequently required by the Cloud service provider who had estimated the size of the data. According to the same respondents, such “little surprises” could lead to some technologies being abandoned along the way, even if they are clinically relevant, either because the organisation cannot afford the costs or the Quebec’s Ministry of Health and Social Services (known as MSSS) refuses to cover them.
Both industry and organisation respondents reported that many AI technologies require access, sometimes in quasi-real time and without human intervention, to large amounts of data of various types. Unanimously, interviewees acknowledge that the organisation’s rules and procedures do not currently allow this (or very barely). Technology providers are calling for easier access to data. However, on the organisational side, several managers consider that such rules and procedures need to be further strengthened. Some of them emphasised the importance of having a Specialist digital lawyer to ensure that these issues are addressed when contracts are signed. They also add that there should also be a Chief data officer to ensure adequate and coherent governance between the various initiatives that involve clinical-administrative data.
The condition(s) or illnesses
Most of the AI technologies identified (72% ≈62/87) within the City hospital are directed at the diagnosis, treatment and/or monitoring of complex chronic or acute conditions (e.g., cancers, neurological, ocular conditions) (Table 1 ). These conditions generally require ongoing or periodic support and monitoring over long periods of time with significant implications for patients and their families, and for the financial sustainability of the healthcare system. They also require complex, individualised, and evolving service models to continue to meet the needs of patients and their families. Several interviewees underscore that the use of AI could reduce waiting times and the costs of managing these pathologies. For a technology provider, these technologies are also expected to help identify new patterns and digital biomarkers that would facilitate the diagnosis and treatment of poorly characterised and/or unpredictable diseases.
For several respondents, this focus on specific diseases is partly due to the nature of the technologies available on the market. These technologies are addressing pathologies mainly through image analysis and/or signal quantification. This makes them more easily measurable, therefore more attractive to technology developers seeking rapid market access.
The technology or technologies
There are diverging perceptions between clinicians, managers, technology providers, and patients on what makes AI attractive, reliable, and mature enough for clinical use and/or interoperable with existing systems.
According to a manager, some of the technologies proposed to the City hospital under the label “AI” are, in fact, expert systems with advanced calculation software. Branding the products in this way is a strategy used by some companies to attract investment and/or obtain contracts. While an AI designation increases the market value of the technology, it does not necessarily increase the clinical value. For another manager, this labelling of AI products is also partly due to the organisation’s pressure on technology providers to integrate AI. This is a significant step for technology companies as, compared to traditional software, AI technologies require specific regulatory requirements, technical infrastructure, expertise, and resources.
Several participants raised emerging security issues specific to AI. This is not only about the security of the technology and infrastructure, but also about the security of the algorithm itself. The latter could be hacked and modified, which can have a direct clinical impact on the patient. According to a manager, being able to recombine data from different sources, AI technologies could easily re-identify individuals. On their side, technology providers pointed out that these security issues are mainly due to the City hospital’s obsolete systems and technology infrastructure. They underscore how their technologies conform to the best security and quality standards and norms on the market, and that unlike public organisations they have the best IT expertise. An industry respondent added that, since the customer is the guarantor of their added value on the market, they also regard data security as central to their reputation and brand image. If an incident occurs, the company could simply lose customers or even go bankrupt.
Some AI technologies need to run on an integrated technological platform or operating system (e.g., electronic health record -EHR-) that allows for optimal data flow and exchange between the different technological systems and organisational departments as well as across healthcare system organisations. Respondents agree that the City hospital’s departments generally have outdated and disparate systems and infrastructures that are frequently not interoperable. However, several managers, clinicians, and technology providers argue that this is a common problem for the whole healthcare system, as an integrated and interoperable EHR does not exist. In this regard, for a population of over 8 million people in Quebec, there are over 30 million patient identification cards. A patient may have several cards with a fragmented EHR in several organisations. Similarly, one interviewee stressed that the equipment used (e.g., scanner, magnetic resonance imaging -MRI) in the City hospital does not always meet the requirements for AI. In some situations, it is difficult to know where the data is, or how it is processed and collected by certain technologies or equipment. Problems with internet connection and data transmission via Wi-Fi are also reported.
There is a consensus that AI technologies need high-quality data. Both industry and organisation respondents highlighted that a significant amount of clinical-administrative data (e.g., handwritten clinical notes) and patient records are still scanned in portable document format (PDF), which is not usable for planned AI. For technology providers, the meaningful use of data, which raises the question of the purpose of the data collection, is missing within the organisation and should be given more consideration.
For its AI programme, the City hospital works with many specialised start-ups and small- and medium-sized enterprises (SU/SMEs). One such technology provider stresses that the survival of their company depends on their ability to seek liquidity in the financial market (e.g., venture capital). This means that they are necessarily accountable to their shareholders who may be looking for the fastest and most profitable exit events possible (i.e., when an investor sells his/her shares in a company to collect cash profits). This approach brings challenges for the City hospital in terms of working relationships, technology development, and continuity of care. For instance, SU/SMEs can be bought by multinationals or simply disappear (e.g., bankruptcy), or a company may stop a technology or cease to update it. According to a manager, the City hospital does not necessarily have the capacity to maintain these technologies on an ad hoc basis or replace them with others. Another interviewee added that sometimes the organisation has no guarantee of recovering data hosted or operated by these technology providers or their subcontractors (e.g., Cloud services).
The value proposition
Stakeholders interviewed have divergent definitions of what constitutes the perceived, anticipated and/or actual value of a technology and the parameters to be considered for measuring it (e.g., safety, efficacy, and effectiveness criteria). About 95% were still in development/experimentation or implementation.
Several technology providers mainly express the value of their technology in terms of its potential to improve healthcare and its efficiency. They pointed to significant consumption of resources by the healthcare system while at the same time being unable to meet the healthcare needs of the population. For these interviewees, AI can solve the problem whilst modernising the healthcare system. In this regard, for a supplier, to realise such value, the City hospital, and the healthcare system in general, must be willing to take some risks. He stressed that if the latter wait for AI to be perfect and risk-free before using it, the technology will never be integrated, and its value promise never delivered to the population.
A manager reported that many AI technologies in the City hospital were at a value promise stage (i.e., with anticipated, rather than actual value stage). Other interviewees consider that this value promise remains relatively speculative, based on vague projections and estimates. In this regard, from the organisation’s perspective, the perceived value of AI technologies is mainly about improved clinical quality and safety, and performance. The expectation to achieve this value is to have tailor-made AI technologies adapted to the setting, clinical contexts, and ways of working. However, focusing on tailored AI solutions can sometimes be a major constraint for technology providers. According to several interviewees, suppliers prefer to commercialise generic technologies that can be easily marketed elsewhere with minimum modification (plug-and-play). Several managers and clinicians added that the costs involved in implementing and adapting the technology to the local context are regularly underestimated by these suppliers. The latter often lack an understanding of the complexity of clinical practices. For example, one company stopped working with the City hospital because it considered that its clinical needs are too specific for the AI technology to be cost-effective.
Because of its status as a leading academic hospital, the City hospital is highly sought after by the AI industry. Several interviewees recognise that the organisation is used to showcase and legitimise the technology’s value proposition, hence its market value and potential for widespread commercialisation. A technology provider also reported that the organisation serves as a gateway to the healthcare systems of Quebec and other Canadian provinces. At the same time, according to organisation respondents, the City hospital benefits from media coverage, which gives it a competitive advantage in attracting talent and expertise. However, divergence over the actual added value of certain technologies may constitute a source of tension between senior management and clinical teams. Some AI technologies are likely to exacerbate workload and staff burnout (e.g., technologies intended for the optimisation of clinical-administrative processes). For a manager, since AI technologies are still considered over and above other priorities, their impact on the quality of work and clinicians’ satisfaction is not really taken into consideration in the organisation’s assessment of their value (e.g., flexibility, alignment with clinical-administrative workflows). He added that the City hospital has difficulty in moving the value of these innovations from the Triple Aim to the Quadruple Aim: “improving the patient experience, the population health and the quality of work and satisfaction of healthcare providers, and reducing costs” [ 49 ].
The organisation’s clinical-administrative data, which is used to develop and/or operate some AI technologies, may contain biases and may not be representative of the general population. For several interviewees, AI technologies may also not respond to the contextual realities and needs of some populations (e.g., indigenous, rural, or minority people). Patients and organisation respondents also pointed out that these populations are rarely involved in the design, development, and implementation of AI technologies within the City hospital. Several interviewees recognise that assessing the added value of AI technologies by population segment is essential, but very difficult to achieve.
The adopter system(s)
Interviewees overwhelmingly agree that certain AI technologies could have a direct impact on the patient-clinician relationship. Some progressive diseases require human care and support over time. For AI technologies designed to monitor chronic diseases, some patients fear being lost from sight by their healthcare providers. According to several patients, it is important to ensure that they always have the possibility of in-person meeting with their clinician. As a patient pointed out, technology could never understand their subjective experience with the disease better than the clinician. For this and another patient, listening and empathy are sometimes more important in a care pathway than medication and technology. They mentioned that the therapeutic relationship goes beyond the simple dimension of the disease.
According to a patient, some patients registered with the City hospital can have up to 5 technology applications, sometimes non-interoperable. Some of these technologies do not operate on older Apple- or Android-supported smartphones, making it hard for several patients to use them unless they upgrade their hardware. Technologies may also require access to patient-generated data at home. Patients, clinicians, and managers stressed that patients may not have the technology and equipment and/or a good internet connection, but also the social and cultural capital (e.g., literacy, family network) to fully benefit from the potential of these technologies. They recognise that these technologies could lead to additional costs and expenses for these patients. Even when they have the technology, they may need technical support at any time of the day (24/7) as the disease “has no working days”, as a patient notes. This support is not automatically provided by the organisation and not all patients have a family/friend network that can be mobilised when needed. Paradoxically, technology could exacerbate the disease burden for these patients.
Several respondents reported that the adoption and use of certain AI technologies typically requires a reorganisation, or even a redesign, of clinical practices, of the organisation of services, and of the modes of governance and control within the City hospital. According to clinicians and managers, these changes could be associated with a feeling of loss of professional autonomy, identities, values, and skills. In the words of a manager, AI technologies could cause an erosion of information asymmetry (in favour of the organisation and the MSSS) and challenge clinicians’ autonomy of practice. The erosion and reduction of the scope of expertise due to the replacement of part of the clinical activity by AI was also pointed. However, several respondents relativised these fears, stressing that it is rather the clinicians trained in AI (e.g., clinician-informatician, clinician-data scientist) who will replace the others. This new expertise will have to be institutionalised and valued. This could imply a revision of the boundaries of professional jurisdictions (e.g., reserved acts) and of certain negotiated orders and privileges, and therefore of powers (e.g., nurse vs. general practitioner; general practitioner vs. specialist physician). Managers and technology providers pointed out that a technology that provides real added value for patients will never be integrated into practice if clinicians perceive it as a threat to them.
It was reported that the effort to integrate AI within the City hospital is occurring in a context where clinicians are under great pressure with high workloads. Some emphasised that they have no time to waste on these technologies, particularly those imposed on them by senior management and/or industry. They also expressed a feeling of innovation fatigue. Managers and clinicians acknowledge that this lack of time, but also of engagement, has a negative impact on the success of technology training and promotion initiatives within the organisation, and therefore its subsequent adoption and use. In addition, clinicians involved in technology integration efforts are mainly volunteers (e.g., champions, super-users). As the contribution to innovation is not considered a clinical activity, it is not remunerated nor recognised in their performance indicators. According to several clinicians and managers, this point is a significant barrier to clinicians’ engagement, especially to embrace the necessary changes and adaptations, and to construct meaning and develop new identities with regards to AI.
There is agreement that the need for continuous monitoring and follow-up of some AI technologies in everyday clinical practice made the role of IT teams more critical to clinical practice. According to a manager, this is a major change as clinical and IT teams have historically evolved in silos. In this regard, it is difficult to align cultures and languages within the City hospital in the midst of developing AI technologies and services. For some clinicians, the increasing adoption of AI in their practice may make them dependent on IT teams (potentially conflicting with their autonomy of practice). To address this issue, an interviewee emphasised the importance of the presence of translators or boundary spanners with a hybrid clinical-IT profile to bridge and build a healthy collaborative space between clinical and IT teams. These translators could also act as a bridge between clinical teams and technology providers. The same respondent reported that such a role is already played by members of the City hospital’s Innovation Pole team and several clinicians.
Several managers and clinicians, acknowledge that the blind confidence and lack of critical distance could affect the use of certain AI technologies in clinical decision-making. In this regard, they see the problem of transparency and explainability of AI decisions (black box). According to an interviewee, the problems of data quality and bias are serious enough to be doubly vigilant on this point. A technology provider recognised the importance of clinicians being able to understand how the decision is made by the AI (e.g., parameters retained or excluded) and whether such a decision is right or wrong. To do so, clinicians may need technical support from AI experts, which the City hospital does not necessarily have. According to several respondents, it is difficult for public organisations to recruit AI experts, as the latter are more attracted by the private sector where working conditions and remuneration are very advantageous.
Embedding and adaptation over time
The City hospital’s IT systems are theoretically well secured for AI or associated technologies needed for its functioning. Indeed, any new technology for clinical-administrative use should meet strict criteria for safety and effectiveness. They should be licensed and/or authorised by the IT department or regulatory agencies. However, several managers and clinicians recognise that, once implemented, numerous technologies are not necessarily monitored and controlled over time. The result is a complex, fragmented, and non-interoperable technology environment that is difficult to manage and update, but also vulnerable to cyber-attacks. Some AI technologies are likely to dysfunction and/or operate and evolve awkwardly in such an environment, which could pose patient safety issues.
According to industry, clinicians, and managers, the lifecycle of AI technologies (i.e., the period during which they can function adequately without major upgrades and avoid replacement by new and better technologies) is often very short, and potentially only a few months. The City hospital should be able to upgrade its technology systems and equipment continuously. The costs can be significant. In this regard, equipment and devices (e.g., scanner, MRI) required for the functioning of certain AIs may be considered obsolete after only five years of use. The data they generate is no longer usable, which has a direct impact on their clinical reliability (e.g., ability to detect cancer). To remedy this problem, some technology providers offer to lease equipment. According to the latter, City hospital could then benefit from the latest equipment, with embedded AI, with no obligation to purchase. A technology provider explained that such a model involves the organisation to engage in service contracts over varying periods of time with the supplier. Such contracts usually include the implementation, maintenance, and upgrading of the equipment and associated technologies. The same respondent emphasised that this proximity model would also allow for a feedback process, necessary to adapt to the evolving needs and expectations of clinical teams. However, for several managers, this model raises concerns about the risk of locking the City hospital into a dependency relationship with a single supplier. They reported that this “chaining” could, among other things, increase the supplier’s control of the organisation’s data. To illustrate this point, an interviewee indicated that a technology provider has already “forced” the City hospital to pay for access to its own data (hosted/stored on the supplier’s servers). The same person reported that suppliers want to benefit from an annuity/rent, i.e., a continuous flow of money over time.
The wider system
A gap exists between those who call for a pragmatic approach (e.g., test-and-error, sandbox logic) and those who call for the consolidation of the precautionary principle (i.e., decision-makers adopt precautionary measures when scientific evidence about a human health or environmental hazard is uncertain and the stakes high) [ 50 ]. For several suppliers, the precautionary principle is a major obstacle to the integration of these technologies into the healthcare system. They stressed that regulation should be made more flexible, because zero risk does not exist in healthcare. An interviewee pointed out that the autonomous and evolving nature of some AI technologies will inevitably lead to failures and unforeseen incidents. Instead, lessons should be learned from these malfunctions and incidents to improve the technology. The Post-Market Approval/Post Market Surveillance model adopted in the USA was given as an example. This approach is rejected by other several managers and clinicians who consider that the lives and safety of patients cannot be subject to “hazardous test-and-error”.
Respondents are unanimous in stating that the authorisation, contracting, and financing process of AI technologies by the MSSS, which mainly focuses on the initial purchase price (capital equipment, which results in the procurement of technology with a fixed price, often the lowest, of which the organisation becomes the owner), is no longer adapted to the reality of AI technologies (Table 3 ). Firstly, many AIs operate with a “Software as a Service (SaaS)” business model. It is a monthly or yearly subscription for the organisation. According to technology providers, this model is justified by the fact that these technologies require continuous monitoring, control, and maintenance over time. Some respondents also called for the adoption of the “Value-Based Procurement (VBP)” business model. In this case, the suppliers are paid according to the value generated by their technology (e.g., 10% of the savings made over a patient’s entire care and service cycle). As these technologies are not cheap, there is a risk that they could be excluded from current tendering processes. According to several managers, the tender model does not consider the costs required for the implementation and adaptation of the technology to the local context. Examples where additional costs were required at the time of implementation, not initially foreseen, are relatively common. However, interviewees recognise that VBP is still difficult to implement. Because of the evolving nature of certain AIs, their value could change over time. Currently, it is difficult to ensure their continuous evaluation and monitoring due to the fragmentation of services and the lack of an integrated EHR, as well as trained and qualified human resources (e.g., collection, organisation, structuring, visualisation, and analysis of AI technology usage data), among other things.
According to several managers, the difficulty of acquiring certain AI technologies through the tendering process is another reason why the City hospital prioritises partnership contracts (e.g., co-development or serving as a testing ground) over service contracts (e.g., procurement of technology and/or associated services) with suppliers. In the words of a manager, as long as the organisation does not incur expenses (e.g., having the technology at no cost for a given period or forever) from its operating budgets, it does not have to justify its actions to the MSSS. This strategy also allows the City hospital to accelerate the integration of these technologies into its care and service offer by avoiding the complex bureaucratic process of the MSSS. However, some interviewees reported that partnership contracts do not always allow for the sustainable use of the technology beyond the free-of-charge period. In some situations, the organisation would have to incur expenses after this period and sign a service contract. It would then have to go through the tendering process again. If the latter is won by a different supplier, the initial technology should then be withdrawn, which condemns the City hospital to a kind of eternal restart.
Several technology providers argue that the tendering model is a barrier to entry into healthcare for SU/SMEs, although they could offer AI technologies with real added value. Unlike large companies, SU/SMEs do not have sufficient financial and marketing capacity to offer low prices.
Several respondents, both in the City hospital and industry, pointed out that the Act on the protection of personal information is also seen as a major obstacle to AI in the healthcare system. Typically, when a patient is treated in a public healthcare organisation, his/her consent does not include the secondary use of his/her data for research or other purposes. Legally, AI technologies developed or tested with this data cannot be used and/or commercialised, at least theoretically. According to an organisation interviewee, overcoming this barrier would entail considering that once a patient is treated in a public healthcare organisation, he/she automatically consents to the secondary use of his/her data for service improvement and research purposes. Several patients interviewed agree with this approach. However, they insisted that patients should always be able to withdraw their consent if they so want (opt-out).
Also concerning data, several interviewees highlighted the central role and necessity of Cloud services (e.g., data storage, exchange, and management) for optimal and effective use of AI technologies. According to a manager, Cloud services providers are mainly multi/transnational companies. The latter have servers and relay points all over the world, which means that data could travel across national borders. This challenges regulatory sovereignty. The same interviewee reported that Quebec legislation requires that data be hosted on servers located on its territory. However, the City hospital does not always have the levers to verify and ensure that the providers really respect this requirement. Nor does it always have the possibility of knowing whether an incident (e.g., security breach, data leakage) has occurred if the company does not communicate the information to it. In the words of another manager, “[The City hospital] does not always have the capacity to [ensure the security and reliability of the technologies], so it is forced to trust [the suppliers]”. In the same vein, it does not always have the levers and means to ensure that the technology provider has destroyed and/or deleted the dataset when requested to do so. In addition, according to another interviewee, the definition of responsibilities in the event of a patient harm incident is a not fully resolved issue yet. The latter highlighted that compensation could involve large sums of money that neither the supplier nor the City hospital would want to pay. In this regard, by simply being identified as a potential liable party in the event of an incident, the organisation or company could see the amount of its insurance contract increase considerably because of the risks involved.
Many AI technologies used in clinical decision making are considered as “Software as a Medical Device (SaMD)”. There is still no clear framework for their assessment and approval in Quebec and Canada. In addition, professional federations and colleges, and medical insurance bodies have not yet taken clear positions on their use in clinical practice. According to several interviewees, the absence of solid clinical practice guidelines, protocols, remuneration models, and professional responsibility frameworks limits the possibility of clinicians using these technologies. As an illustration, a manager pointed to the complexity of identifying responsibilities in the event of an AI error (e.g., misdiagnosis or mistreatment). Since certain technologies can decide autonomously, part of the responsibility of the clinician is transferred to them. For the same interviewee, numerous questions have yet to be answered: to what extent does the technology replace the clinician (totally or partially) or not? With the “black box” problem, AI does not always allow for tracing and understanding the decision-making process. Even when it is possible, technology providers might refuse to give access to their algorithm for commercial confidentiality and market competitiveness reasons. It is then difficult to know the nature and/or origin of the fault. Moreover, there is also the question of whether AI should imply an obligation of results, instead of the obligation of means to which clinicians are presently committed. According to another manager, technology providers prefer to classify their technologies outside the SaMD category. In this way, the clinician remains solely responsible in the event of harm. Then, the supplier avoids paying damages that may be substantial. Indeed, compared to a clinician’s error, which is usually limited to a single patient, an AI technology’s error could affect many patients. However, providers explained this choice by the fact that technology approval processes, such as SaMD, are time-consuming and very expensive.
Other regulatory constraints are pointed out by several interviewees. AI technologies never arrive ready for clinical use (plug-and-play). There is often adaptation and alignment work to be done. Some changes and/or adaptations are made informally (e.g., bricolage, workarounds) by clinicians. According to a clinician and a manager, these modifications are sometimes crucial in their decisions to use the technology or not. However, from a regulatory perspective, once licensed and authorised, a technology should not generally be modified, at least theoretically. Currently, any changes require the approval of the City hospital’s IT teams or of a governmental regulatory agency. Although justified in terms of financial and safety risks, there is a consensus among interviewees that this process is rigid, time consuming, and inadequate for the reality of AI. In this regard, updates to AI technologies should be quasi-automatic and continuous, in the spirit of how the iPhone works, often without human intervention. In the words of a clinician, any delay or blockage could have a direct impact on the diagnosis or treatment of patients.
According to a manager, aspects related to the organisations’ performance criteria and, therefore of their funding by the government are not yet fully defined for AI. In Quebec, the activity-based funding model is being deployed to complement the dominant historical budget model. This new model generally considers the activity of physicians (e.g., diagnosis, treatment, surgery), paid essentially on a fee-for-service basis, in the calculation of the budget the organisation will receive from the MSSS. The activity of other healthcare professionals, mainly salaried by the organisation (e.g., nurses), is not considered the same way (or only slightly) in these calculations. Numerous AI technologies intended for (or assisting in) diagnosis or treatment could be supervised by healthcare professionals other than physicians. The impact of this development on the funding of healthcare organisations remains unknown. In the same vein, the respondent highlighted the problem of the fragmentation of funding between medical, medico-social, and social services in Quebec. For example, some AI technologies have a clinical added value and are therefore covered by the MSSS. However, the latter does not cover other aspects such as the improvement of the patient’s quality of life (e.g., Quality-adjusted life year -QALY-). As a result, the City hospital could be required to solicit different departments, ministries and/or agencies to capture the different value components of the same AI technology.
According to several interviewees, funding from the federal government would have a direct impact on the integration of AI technologies into the City hospital. They report that federal programmes make it possible to fund expensive infrastructure projects, from several hundred thousand to several million CA$. However, implementation and sustainability are mainly under the responsibility of the Quebec MSSS because health falls under provincial authority in Canada. There is sometimes a gap between federal funding and provincial priorities. According to a manager, the Quebec MSSS does not automatically fund the implementation and sustainability of federally funded technologies. As a result, several technologies could eventually be abandoned. For another interviewee, one of the important limitations is that federal funding is often very targeted and specific to particular technologies and/or clinical areas. It does not provide sufficient flexibility for organisations to use it according to local needs and contingencies.
Lastly, several respondents recognise that inter-organisational collaboration for sharing expertise and experience is essential for AI. However, the fragmentation, lack of communication and coordination across public healthcare organisations make it difficult to establish such a collaborative environment. For example, according to a clinician, to develop AI technologies with real added value, it would be necessary to have access to large amounts of patient data. She explained that the way to do this, while competing with other technologies from other countries, is to pool the databases of different healthcare organisations in Quebec and Canada. Such an inter-organisational network is essential in the evaluation and approval process of AI technologies, as they are to be tested on data from different healthcare organisations (e.g., urban and rural hospitals, primary care clinics). For the same respondent, such multicentre testing would ensure reliability and effectiveness in different clinical and technological settings across the country.
Summary of key lessons
Our study aimed to generate a better understanding of the conditions that facilitate or constrain the integration of AI technologies in a large healthcare organisation in Canada. By analysing a rich corpus of data using the NASSS framework, the study highlights seven lessons:
Firstly, an organisational culture and leadership that creates favourable conditions for AI is essential as well as the presence of clinical champions who act as ambassadors for AI. This is a lever to attract clinical and/or technical talent and expertise, but also companies in the field. The strategic alignment of the organisation’s clinical-administrative processes and infrastructures with AI technologies remains a major challenge. A lack of alignment could lead to partial integration of technologies or their abandonment, resulting in innovation fatigue among clinical and administrative teams. In a context where clinicians are over-solicited, they should be given the time needed to integrate the change, but also develop the professional expertise and identities that AI could require. It is also important that the technologies proposed to them are supported by evidence of improvements in patient care and services as well as in their work conditions and quality. The integration of AI within a hospital also involves a multitude of stakeholders whose activities and actions should be coherent and synergistic. Communication is fundamental to clarify roles, responsibilities, and mandates and requires a horizontal structure capable of coordinating actions and shaping a consistent organisational story about AI. The technologies proposed by the industry should be filtered so that those that really meet the needs on the ground are prioritised.
Secondly, financial and other incentives are needed to encourage clinicians to experiment and adapt these technologies to their practices. Investments in the development of AI technologies have so far focused on specific complex pathologies that present a great burden to patients and their families as well as to the healthcare system. To address these pathologies, AI mainly exploits image analysis and/or signal quantification, which makes it easier for suppliers to develop technologies and introduce them more quickly to the market. Yet, the sensitivity of safety and data protection issues implies that the hospital hires a lawyer specialising in digital technologies (to ensure that contracts are properly made) and a Chief data officer (for adequate and consistent data governance). Upgrading IT systems and infrastructure and recruiting new expertise hence require planning for both initial and recurring investments and expenditures.
Thirdly, the interoperability of AI technologies and the organisation’s systems and infrastructure are major obstacles to their routine use. Some technologies need quasi-real time access to data, which requires an integrated platform to ensure optimal data circulation between different IT systems and departments of the organisation, or even other organisations involved in the patient’s treatment. The qualification of some advanced software as AI could have financial and legal implications for the organisation. In addition to traditional clinical safety issues, the AI algorithm itself could be hacked and modified, resulting in harm to patients. By recombining data from various sources, individuals could be easily re-identified. These technologies could also require high-tech equipment with very short lifecycles, which the organisation may not have. Furthermore, many AI technologies are driven by SU/SMEs that could disappear from the market at any time. Hence, organisations should have the capacity to maintain the technology on an ad hoc basis or find an alternative and be able to recover and/or ensure the deletion of data by the initial supplier.
Fourth, the definition of the value of AI technologies is far from consensual as well as the expectations regarding what they can or should do. The ability to measure this value is of considerable complexity given the great contrast between the value proposition stated by suppliers, and sometimes by managers, and the actual value to clinicians and patients. The value of AI is not self-evident. Indeed, even if it has shown great performance in a laboratory context, this may not materialise in the real-world context of care and services. The value of some AI technologies also contrasts with the risks they raise given their evolutionary and autonomous nature. There are trade-offs between the precautionary principle, the need for some risk tolerance, and its clinical potential. Moreover, clinical practice may require very specific AI technologies, whereas suppliers tend to prioritise plug-and-play technologies with a potential for widespread commercialisation. The global value of AI could vary widely depending on the balance of the changes and transformations it requires and what it actually provides. This value may also change over time. Evaluating and monitoring AI’s value on an ongoing basis requires resources and expertise the organisation may lack, especially in view of the (re)production of bias across sub-groups of the population.
Fifth, contrary to the rhetoric about their potential to humanise care, some AI technologies raise concerns about the patient-clinician relationship and, therefore, about quality of care. The risk of mechanisation of care and the difficulty of physically accessing healthcare providers is palpable. Digital literacy, technical support, and change management for clinicians and patients using these technologies are essential. For clinicians, AI technologies may imply redesigning clinical practice and service organisation, but also new governance and control strategies within the organisation. Although improbable, there is a real concern that AI could partially or totally replace the activity of clinicians. Hyper-dependence on technology raises concerns about the erosion of clinicians’ expertise and the risk of blind trust in the decisions made by AI. As a result, clinicians may worry about being subordinated to the IT teams that would play a central role in the production of care. This new reality highlights the central role of translators or boundary spanners in building bridges and trust between clinical and IT teams, but also with industry. On a larger scale, the technology-driven approach to AI could cause a deterioration in clinicians’ work conditions and quality.
Sixth, the evolving and self-learning nature of some AI technologies makes time critical, distinguishing them from previous licensed technologies that do not generally require a new approval review. IT teams should approve and validate any changes or adaptations, and this becomes difficult with some AI technologies that evolve autonomously and update themselves. Any delay or blockage could threaten the diagnostic or treatment quality of patients. Continuous monitoring and control over time is required to avoid malfunctions and incidents, but also to make the necessary improvements. In this regard, the increasingly short lifecycle of software and hardware challenges the technical and financial capacity of the organisation to adapt and evolve its systems, equipment, and infrastructure at the right pace. Evolutionary AI technologies create the need for close and sustainable relationships between the organisation and the technology providers, a new relationship that: 1) requires solid frameworks to identify and resolve conflicts of interest as they arise over time; and 2) must avoid lock-in and dependence upon a single provider.
Seventh, many socio-political, economic, and regulatory factors are decisive in the integration of AI technologies, which are mainly offered under SaaS and/or VBP business models. These models are in opposition to the current tender model in Quebec that emphasises the cheapest technology (capital equipment). The legal framework of the current model constitutes a barrier to entry for SU/SMEs, some with high value-added technologies. Established bureaucratic acquisition processes are inadequate for the very short lifecycle of AI technologies. Consent requirements for the use of patient data are misaligned with this new reality and are prompting consideration of an opt-out consent model. AI technologies increasing rely on Cloud services mainly offered by multinational companies with servers and relay points all over the world. Data governance is even more important as healthcare organisations and systems have limited resources and tools to ensure that data management and storage comply with applicable laws. Identifying liability in the event of harm could therefore be very complex. AI technologies classified as SaMD, on the other hand, have specific requirements for quality, efficiency, and clinical reliability. To date, the lack of reference technologies makes it difficult for regulatory agencies to assess and approve them. Established mechanisms and processes are not adapted to the complexity and very short lifecycle of AI. Ongoing evaluation and monitoring mechanisms in the real-world context seem necessary, but the high degree of uncertainty associated with them requires a balance between the precautionary principle and a laissez-faire integration in clinical routine. Beyond the lack of clear frameworks and directives from the MSSS and other regulatory bodies regarding the use of these technologies by clinicians, inter-organisational networks facilitating the sharing of expertise and experience are essential. The current context is characterised by fragmentation, and poor communication and coordination between organisations and government agencies, which hinders an integrated and coherent vision of AI at the healthcare system: provincial- and federal-level of governance.
Contribution to the existing literature
The results of this study contribute to knowledge in several ways. They shed a new and different light on the trend of recent years where the literature has mainly focused on the technical and promissory dimensions of AI. Our findings are consistent with those of Pumplun et al. (2021) and Petersson et al. (2022) who analysed implementation issues raised by AI technologies in healthcare in Germany and Sweden, respectively [ 3 , 51 ]. Studies on telehealth and EHR also reported results that corroborate ours on AI [ 26 , 31 , 32 , 34 , 52 , 53 , 54 , 55 , 56 , 57 , 58 ]. In this regard, several authors pointed out the major contrast between the techno-optimistic discourse on the performance and efficiency of technology and the reality of services that are difficult to transform [ 56 , 57 , 58 ]. These experiences have shown that the difficulties encountered in the deployment of digital technologies are mainly due to the historical lack of attention paid to the sociotechnical factors and conditions necessary for their integration into healthcare organisations and systems. Hence, our study adds to the growing literature that considers technology in a complex sociotechnical transformation perspective that requires not only technological but also human, clinical, professional, organisational, socio-political, economic, regulatory, legal, and cultural changes [ 27 , 40 , 41 , 56 , 59 , 60 , 61 ]. Very limited attention has been paid to this perspective in examining AI to date, whereas our study clarifies its contribution and indicates some avenues for future research (Table 4 ) [ 3 , 18 , 26 , 51 ].
From a theoretical standpoint, our study provides an original contribution to the literature on health innovations. It is one of the first to demonstrate that the NASSS framework is relevant for the analysis of the integration of AI technologies in healthcare organisations and systems [ 51 ]. The study contributes to the knowledge on the importance of a sociotechnical perspective to understand the complexity and unpredictability of transformations related to disruptive innovations such as AI [ 27 , 51 , 62 ].
Implication for practice and policy
Our study provides new insights for decision-making and practice on the conditions required but also on the pitfalls to be avoided to ensure successful integration of AI technologies into healthcare organisations and systems. It shows that the pitfalls of the technocentric vision of digital health of the last thirty years in Quebec (and elsewhere too) could easily be repeated with AI technologies, but this time with more profound repercussions [ 31 , 32 , 33 , 35 , 36 , 63 ]. As Matheny et al. (2020) highlighted: “Disconnects between reality and expectations have led to prior precipitous declines in use of the technology, termed AI winters, and another such event is possible, especially in health care” [ 64 ]. In this regard, the various stakeholders must be aware that AI is more an object of transformation at all levels of healthcare system governance, than a simple “intrinsically good/bad” tool. Its successful integration depends on several structural conditions, namely, appropriate: regulatory and governance frameworks; funding, business, and remuneration models; definition of the value proposition; management of conflicts of interest; governance of data; cybersecurity strategies; training and expertise, models of care and service delivery; inter-professional collaboration; and up-to-date infrastructure and equipment.
Specifically, AI highlights the importance of rethinking the collaboration between healthcare organisations and systems, on the one hand, and technology providers, on the other hand. Indeed, their interests sometimes represent competing financial and political objectives between which a difficult balance must be established [ 65 ]. Given their disruptive nature at all levels of the healthcare system, IA technologies could generate tensions and require trade-offs between perceptions, expectations, interests, and agendas that may be divergent or even antagonistic (ex. industry and venture capital, decision-makers, managers, clinicians, patients). These dynamics and power relations influence the trajectory of AI technologies in healthcare, either positively or negatively [ 59 , 66 ]. Thus, if healthcare organisations and systems are not sufficiently equipped and prepared, “the AI landscape risks being shaped by early established companies and decisions made with insufficient evaluations in place due to pressures to embrace technology” [ 67 ].
In addition, one of the fundamental issues remains the lack of digital literacy and culture, and AI technology skills among healthcare professionals [ 68 ]. Currently, initial and continuing training programmes do not sufficiently integrate these technologies into the expertise that trainees (e.g., physicians, nurses) need to achieve to be authorised to practice. As reported in our study, without appropriate training, clinicians are unlikely to adopt in an appropriate way these technologies. Indeed, training is required to adapt provider protocols, administrative workflows, pathways, and business processes [ 67 ]. According to Mistry (2019), for such change to take place, healthcare professionals will need:1) to have access to education content enabling them to learn new skills as AI users and work differently; 2) to be able to train AI systems themselves for setting them up to perform specified tasks, which implies knowing what data to select and its quality; 3) to develop abilities to interpret AI outputs, including a solid understanding of its limitations and bounds of function; and 4) to know “how the system learns and what constitutes appropriate use, so that ethical norms are upheld and any introduction of biases is avoided” [ 67 ].
Strengths and limitations
This study offers one of the first holistic and multilevel analyses of the complexity of the changes and transformations associated with the integration of AI technologies into clinical routine, beyond technical issues. It is also part of the few studies that go beyond looking at one single AI technology and delves into the organisational and systemic complexity of integrating multiple AI technologies concurrently.
However, the study has limitations. By its qualitative nature, it has a high level of internal validity, but the transferability (or generalisability) of its findings is limited to similar healthcare organisations and systems. In other contexts, it can increase the awareness of different stakeholders regarding the importance of taking better account of the sociotechnical dimension of AI. Healthcare organisations and systems can vary considerably, hence the importance of contextualising the results.
The number of interviewees ( n = 29) is relatively low in view of the large number of AI technologies covered in this study. Although we made great efforts to include a wide range of stakeholders, several people were unable to participate due to the COVID-19 context. This is the case for women heading technology companies, whereas decision-makers, managers, and clinicians were unable to participate because of their direct involvement in the management of the pandemic. However, the people who participated, through their expertise and experience, provided us with rich data, necessary for a detailed understanding of the challenges of integrating AI in healthcare organisations and systems. The application of a rigorous research approach, guided by best methodological practices and an exhaustive theoretical framework, has reinforced the reliability of our results.
Conclusions
AI in healthcare is still in its infancy. There are huge expectations that it will provide answers to major contemporary challenges in healthcare organisations and systems. This is reflected in the funding it receives from governments, but also in the interest of the financial and venture capital sector. The COVID-19 pandemic was a test case for AI, and it did not fully deliver. However, the pandemic has served as an accelerator for its experimentation, for example, through the relaxation of regulatory requirements and less resistance from some stakeholders. AI represents as much a logistical, psychological, cultural, and philosophical change, particularly in terms of what it could and should do in healthcare organisations and systems. It is a “new era” that requires a real critical examination to learn from the many past experiences with the digitalisation of healthcare organisations and systems. With AI, the nature, scale and complexity of the changes and transformations are at such a level and intensity that the implications could be profound for society. At present, little is known about how such an announced revolution may take shape and under what conditions. This study provides a unique learning base for analysing AI technologies in healthcare organisations and systems from a sociotechnical perspective using the NASSS framework. It adds to the existing literature and can better inform decision-making towards the judicious, responsible, and sustainable integration of these technologies in healthcare organisations and systems.
Availability of data and materials
The data that support the findings of this study are available from the corresponding author (HA) upon reasonable request. The data are not publicly available due to information that could compromise the privacy of the research participants.
Abbreviations
- Artificial intelligence
Canadian Dollar
Coronavirus Disease 2019
Quebec’s Ministry of Health and Social Services Information Technology Division
Electronic Health Record
International Organization for Standardization
Information Technology
Act on Contracting by Public Bodies
Magnetic Resonance Imaging
Quebec’s Ministry of Health and Social Services
Non-Adoption, Abandonment, Scale-up, Spread, Sustainability
Picture Archiving and Communication System
Portable Document Format
Quality-Adjusted Life Year
Software as a Service
Software as a Medical Device
Start-ups and Small- and Medium-sized Enterprises
United States Dollar
United States of America
Value-Based Procurement
Organisation for Economic Co-operation and Development (OECD). Recommendation of the Council on Artificial Intelligence. OECD; 2019. https://legalinstruments.oecd.org/en/instruments/OECD-LEGAL-0449#mainText .
Alloghani M, Al-Jumeily D, Aljaaf A, Tan S, Khalaf M, Mustafina J. The application of artificial intelligence technology in healthcare: a systematic review. CCIS. 2020;1174:248–61.
Google Scholar
Petersson L, Larsson I, Nygren JM, Nilsen P, Neher M, Reed JE, et al. Challenges to implementing artificial intelligence in healthcare: a qualitative interview study with healthcare leaders in Sweden. BMC Health Serv Res. 2022;22(1):1–16.
Article Google Scholar
Topol EJ. High-performance medicine: the convergence of human and artificial intelligence. Nat Med. 2019;25(1):44–56.
Article CAS PubMed Google Scholar
Abràmoff MD, Lavin PT, Birch M, Shah N, Folk JC. Pivotal trial of an autonomous AI-based diagnostic system for detection of diabetic retinopathy in primary care offices. NPJ Digital Med. 2018;1(1):39.
Ching T, Himmelstein DS, Beaulieu-Jones BK, Kalinin AA, Do BT, Way GP, et al. Opportunities and obstacles for deep learning in biology and medicine. J R Soc Interface. 2018;15(141):20170387.
Article PubMed PubMed Central Google Scholar
van Leeuwen KG, de Rooij M, Schalekamp S, van Ginneken B, Rutten MJ. How does artificial intelligence in radiology improve efficiency and health outcomes? Pediatric Radiol. 2022;52(11):2087–93.
Benjamens S, Dhunnoo P, Meskó B. The state of artificial intelligence-based FDA-approved medical devices and algorithms: an online database. NPJ Digital Med. 2020;3(1):1–8.
Hamet P, Tremblay J. Artificial intelligence in medicine. Metabolism. 2017;10(01):11.
Jiang F, Jiang Y, Zhi H, Dong Y, Li H, Ma S, et al. Artificial intelligence in healthcare: past, present and future. Stroke Vasc Neurol. 2017;2(4):230–43.
Chen M, Decary M. Artificial intelligence in healthcare: an essential guide for health leaders. Healthc Manage Forum. 2020;33(1):10–8. https://doi.org/10.1177/0840470419873123 .
Article PubMed Google Scholar
Rajkomar A, Dean J, Kohane I. Machine learning in medicine. N Engl J Med. 2019;380(14):1347–58.
Miller DD, Brown EW. Artificial intelligence in medical practice: The question to the answer? Am J Med. 2018;131(2):129–33.
Dicuonzo G, Donofrio F, Fusco A, Shini M. Healthcare system: moving forward with artificial intelligence. Technovation. 2023;120:102510.
Bohr A, Memarzadeh K. The rise of artificial intelligence in healthcare applications. Artif Intell Healthc. 2020;Chap2:25–60.
Alami H, Rivard L, Lehoux P, Hoffman SJ, Cadeddu SBM, Savoldelli M, et al. Artificial intelligence in health care: laying the foundation for responsible, sustainable, and inclusive innovation in low- and middle-income countries. Glob Health. 2020;16(1):52.
Alami H, Lehoux P, Denis J-L, Motulsky A, Petitgand C, Savoldelli M, et al. Organizational readiness for artificial intelligence in health care: insights for decision-making and practice. J Heal Organ Manag. 2020;35(1):106–14.
Alami H, Lehoux P, Auclair Y, de Guise M, Gagnon M-P, Shaw J, et al. Artificial intelligence and health technology assessment: anticipating a new level of complexity. J Med Internet Res. 2020;22(7):e17707.
Sharon T. When digital health meets digital capitalism, how many common goods are at stake? Big Data Soc. 2018;5(2):2053951718819032.
Liu X, Faes L, Kale AU, Wagner SK, Fu DJ, Bruynseels A, et al. A comparison of deep learning performance against health-care professionals in detecting diseases from medical imaging: a systematic review and meta-analysis. Lancet Digital Health. 2019;1(6):e271–97.
Bullock J, Luccioni A, Pham KH, Lam CS, Luengo-Oroz M. Mapping the landscape of artificial intelligence applications against COVID-19. J Artif Intell Res. 2020;19(69):807–45.
Naudé W. Artificial intelligence vs COVID-19: limitations, constraints and pitfalls. Ai Soc. 2020;35(3):761–5.
Heaven WD. Hundreds of AI tools have been built to catch covid. None of them helped. MIT Technology Review; 2021. https://www.technologyreview.com/2021/07/30/1030329/machine-learning-ai-failed-covid-hospital-diagnosis-pandemic/ .
Wynants L, Van Calster B, Collins GS, Riley RD, Heinze G, Schuit E, et al. Prediction models for diagnosis and prognosis of covid-19: systematic review and critical appraisal. Br Med J. 2020;7:369.
Roberts M, Driggs D, Thorpe M, Gilbey J, Yeung M, Ursprung S, et al. Common pitfalls and recommendations for using machine learning to detect and prognosticate for COVID-19 using chest radiographs and CT scans. Nat Machine Intell. 2021;3(3):199–217.
Lebcir R, Hill T, Atun R, Cubric M. Stakeholders’ views on the organisational factors affecting application of artificial intelligence in healthcare: a scoping review protocol. BMJ Open. 2021;11(3):e044074.
Greenhalgh T, Wherton J, Papoutsi C, Lynch J, Hughes G, A'Court C, et al. Beyond adoption: a new framework for theorizing and evaluating nonadoption, abandonment, and challenges to the scale-up, spread, and sustainability of health and care technologies. J Med Int Res. 2017;19(11):e8775.
Skaria R, Satam P, Khalpey Z. Opportunities and challenges of disruptive innovation in medicine using artificial intelligence. Am J Med. 2020;133(6):e215–7.
Thompson RF, Valdes G, Fuller CD, Carpenter CM, Morin O, Aneja S, et al. Artificial intelligence in radiation oncology: a specialty-wide disruptive transformation? Radiother Oncol. 2018;129(3):421–6.
Rubeis G. The disruptive power of artificial intelligence. Ethical aspects of gerontechnology in elderly care. Arch Gerontol Geriatr. 2020;91:104186.
Alami H, Gagnon M-P, Fortin J-P. Some multidimensional unintended consequences of telehealth utilization: a multi-project evaluation synthesis. Int J Health Policy Manag. 2019;8(6):337.
Alami H, Fortin J-P, Gagnon M-P, Pollender H, Têtu B, Tanguay F. The challenges of a complex and innovative telehealth project: a qualitative evaluation of the eastern Quebec Telepathology network. Int J Health Policy Manag. 2018;7(5):421.
Alami H, Fortin J-P, Gagnon M-P, Lamothe L, Ahmed MAA, Roy D. Cadre stratégique pour soutenir l’évaluation des projets complexes et innovants en santé numérique. Sante Publique. 2020;32(2):221–8.
Alami H, Gagnon M-P, Wootton R, Fortin J-P, Zanaboni P. Exploring factors associated with the uneven utilization of telemedicine in Norway: a mixed methods study. BMC Med Inform Decis Mak. 2017;17(1):180.
Article CAS PubMed PubMed Central Google Scholar
Alami H, Lamothe L, Fortin J-P, Gagnon M-P. L’implantation de la télésanté et la pérennité de son utilisation au Canada : quelques leçons à retenir. Eur Res Telemed. 2016;5(4):105–17.
Alami H, Shaw S-E, Fortin J-P, Savoldelli M, Fleet R, Têtu B. The ‘wrong pocket’problem as a barrier to the integration of telehealth in health organisations and systems. Digital Health. 2023;9:1–7.
Gremyr A, Gäre BA, Greenhalgh T, Malm U, Thor J, Andersson A-C. Using complexity assessment to inform the development and deployment of a digital dashboard for schizophrenia care: case study. J Med Internet Res. 2020;22(4):e15521.
Greenhalgh T, Maylor H, Shaw S, Wherton J, Papoutsi C, Betton V, et al. The NASSS-CAT tools for understanding, guiding, monitoring, and researching technology implementation projects in health and social care: protocol for an evaluation study in real-world settings. JMIR Res Protoc. 2020;9(5):e16861.
Berg M. Patient care information systems and health care work: a sociotechnical approach. Int J Med Inform. 1999;55(2):87–101.
Papoutsi C, Wherton J, Shaw S, Greenhalgh T. Explaining the mixed findings of a randomised controlled trial of telehealth with centralised remote support for heart failure: multi-site qualitative study using the NASSS framework. Trials. 2020;21(1):1–15.
Shaw J, Rudzicz F, Jamieson T, Goldfarb A. Artificial intelligence and the implementation challenge. J Med Internet Res. 2019;21(7):e13659.
Yin RK. Case study research and applications. Thousand Oaks CA: Sage; 2018.
Alami H, Rivard L, Lehoux P, Ahmed MAA, Fortin J-P, Fleet R. Integrating environmental considerations in digital health technology assessment and procurement: Stakeholders’ perspectives. Digital Health. 2023;9:1–17.
Miles MB, Huberman AM, Saldaña J. Qualitative data analysis: a methods sourcebook. 3rd: ed. Thousand Oaks, CA: Sage; 2014.
Morse JM. Designing funded qualitative research. Handbook of Qualitative Research. 1994.
Farmer T, Robinson K, Elliott SJ, Eyles J. Developing and implementing a triangulation protocol for qualitative health research. Qual Health Res. 2006;16(3):377–94.
De PP. l’analyse qualitative en général et de l’analyse thématique en particulier. Rec Qual. 1996;15:179–94.
Overcash JA. Narrative research: a review of methodology and relevance to clinical practice. Crit Rev Oncol Hematol. 2003;48(2):179–84.
Bodenheimer T, Sinsky C. From triple to quadruple aim: care of the patient requires care of the provider. Ann Fam Med. 2014;12(6):573–6.
Bourguignon D. The precautionary principle: definitions, applications and governance. Policy Commons. 2015. https://policycommons.net/artifacts/1334548/the-precautionary-principle/1940163/ .
Pumplun L, Fecho M, Wahl N, Peters F, Buxmann P. Adoption of machine learning systems for medical diagnostics in clinics: qualitative interview study. J Med Internet Res. 2021;23(10):e29301.
Alami H, Lehoux P, Gagnon M-P, Fortin J-P, Fleet R, Ahmed MAA. Rethinking the electronic health record through the quadruple aim: time to align its value with the health system. BMC Med Inform Decis Mak. 2020;20(1):1–5.
Alami H, Gagnon M-P, Fortin J-P, Kouri R. La télémédecine au Québec: état de la situation des considérations légales, juridiques et déontologiques. La Rec Eur Téléméd. 2015;4(2):33–43.
Alami H, Lehoux P, Attieh R, Fortin J-P, Fleet R, Niang M, et al. A “not so quiet” revolution: systemic benefits and challenges of telehealth in the context of COVID-19 in Quebec (Canada). Front Digit Health. 2021;10(3):721898.
Alami H, Gagnon MP, Fortin JP. Telehealth in light of cloud computing: clinical, technological, regulatory and policy issues. J Int Soc Telemed eHealth. 2016;4(e5):1–7.
Shaw S, Hughes G, Wherton J, Moore L, Rosen R, Papoutsi C, et al. Achieving spread, scale up and sustainability of video consulting services during the Covid-19 pandemic? Findings from a comparative case study of policy implementation in England, Wales, Scotland and Northern Ireland. Front Digital Health. 2021;3:754319.
Greenhalgh T, Shaw S, Wherton J, Vijayaraghavan S, Morris J, Bhattacharya S, et al. Real-world implementation of video outpatient consultations at macro, meso, and micro levels: mixed-method study. J Med Internet Res. 2018;20(4):e9897.
Shaw S, Wherton J, Vijayaraghavan S, Morris J, Bhattacharya S, Hanson P, et al. Advantages and limitations of virtual online consultations in a NHS acute trust: the VOCAL mixed-methods study. Health Serv Del Res. 2018;6(21):1–36.
Cresswell K, Hernández AD, Williams R, Sheikh A. Key challenges and opportunities for cloud technology in health care: semistructured interview study. JMIR Hum Factors. 2022;9(1):e31246.
Greenhalgh T, Rosen R, Shaw SE, Byng R, Faulkner S, Finlay T, et al. Planning and evaluating remote consultation services: a new conceptual framework incorporating complexity and practical ethics. Front Digital Health. 2021;103:726095.
James HM, Papoutsi C, Wherton J, Greenhalgh T, Shaw SE. Spread, scale-up, and sustainability of video consulting in health care: systematic review and synthesis guided by the NASSS framework. J Med Internet Res. 2021;23(1):e23775.
Papoutsi C, Wherton J, Shaw S, Morrison C, Greenhalgh T. Putting the social back into sociotechnical: Case studies of co-design in digital health. J Am Med Inform Assoc. 2021;28(2):284–93.
Alami H, Lehoux P, Shaw S-E, Papoutsi C, Rybczynska-Bunt S, Fortin J-P. Virtual care and the inverse care law: Implications for policy, practice, research, public and patients. Int J Environ Res Public Health. 2022;19(17):10591.
Matheny M-E, Whicher D, Israni STD. Artificial intelligence in health care: a report from the national academy of medicine. J Am Med Assoc. 2020;323(6):509–10.
Lehoux P, Daudelin G, Denis J-L, Miller F-A. A concurrent analysis of three institutions that transform health technology-based ventures: economic policy, capital investment, and market approval. Rev Policy Res. 2017;34(5):636–59.
Cennamo C, Santaló J. Generativity tension and value creation in platform ecosystems. Organ Sci. 2019;30(3):617–41.
Mistry P. Artificial intelligence in primary care. Br J Gen Pract. 2019;69(686):422–3.
Alami H, Gagnon M-P, Ahmed MAA, Fortin J-P. Digital health: cybersecurity is a value creation lever, not only a source of expenditure. Health Policy Technol. 2019;8(4):319–21.
Download references
Acknowledgements
We thank the interviewees and the City hospital personnel for their availability throughout the study, even in the midst of the COVID-19 pandemic. The findings and conclusions presented in the text are those of the authors. They do not necessarily reflect the position of their organisations.
HA was supported by the In Fieri research programme (led by P), the International Observatory on the Societal Impacts of Artificial Intelligence and Digital Technologies, and the Institute for Data Valorization (IVADO), (Canada).
Author information
Authors and affiliations.
Department of Health Management, Evaluation and Policy, School of Public Health, University of Montreal, P.O. Box 6128, Branch Centre-Ville, Montreal, QC, H3C 3J7, Canada
Hassane Alami & Pascale Lehoux
Center for Public Health Research of the University of Montreal, Montreal, QC, Canada
Institute for Data Valorization (IVADO), Montreal, QC, Canada
Hassane Alami
Nuffield Department of Primary Care Health Sciences, University of Oxford, Oxford, UK
Hassane Alami, Chrysanthi Papoutsi & Sara E. Shaw
Faculty of Medicine, Laval University, Quebec, QC, Canada
Richard Fleet & Jean-Paul Fortin
VITAM Research Centre on Sustainable Health, Faculty of Medicine, Laval University, Quebec, QC, Canada
You can also search for this author in PubMed Google Scholar
Contributions
HA and PL conceived and designed the study plan. HA and PL were responsible for data collection, analysis, and interpretation of results. HA, PL, CP, SES, RF and JPF were engaged in the drafting of the manuscript, and they all read and approved the final manuscript.
Corresponding author
Correspondence to Hassane Alami .
Ethics declarations
Ethics approval and consent to participate.
The study was approved by the City hospital Research Ethics Committee (Number: Comité d’éthique de la recherche- City hospital: 20.399). (Address is anonymised for confidentiality reasons). All methods were carried out in accordance with relevant guidelines and regulations. Informed consent was obtained from all subjects and/or their legal guardian(s).
Consent for publication
Not applicable.
Competing interests
The authors declare no competing interests.
Additional information
Publisher’s note.
Springer Nature remains neutral with regard to jurisdictional claims in published maps and institutional affiliations.
Rights and permissions
Open Access This article is licensed under a Creative Commons Attribution 4.0 International License, which permits use, sharing, adaptation, distribution and reproduction in any medium or format, as long as you give appropriate credit to the original author(s) and the source, provide a link to the Creative Commons licence, and indicate if changes were made. The images or other third party material in this article are included in the article's Creative Commons licence, unless indicated otherwise in a credit line to the material. If material is not included in the article's Creative Commons licence and your intended use is not permitted by statutory regulation or exceeds the permitted use, you will need to obtain permission directly from the copyright holder. To view a copy of this licence, visit http://creativecommons.org/licenses/by/4.0/ . The Creative Commons Public Domain Dedication waiver ( http://creativecommons.org/publicdomain/zero/1.0/ ) applies to the data made available in this article, unless otherwise stated in a credit line to the data.
Reprints and permissions
About this article
Cite this article.
Alami, H., Lehoux, P., Papoutsi, C. et al. Understanding the integration of artificial intelligence in healthcare organisations and systems through the NASSS framework: a qualitative study in a leading Canadian academic centre. BMC Health Serv Res 24 , 701 (2024). https://doi.org/10.1186/s12913-024-11112-x
Download citation
Received : 03 February 2023
Accepted : 14 May 2024
Published : 03 June 2024
DOI : https://doi.org/10.1186/s12913-024-11112-x
Share this article
Anyone you share the following link with will be able to read this content:
Sorry, a shareable link is not currently available for this article.
Provided by the Springer Nature SharedIt content-sharing initiative
- Digital health
- Health organisation
- Health system
- Business models
- Implementation
- Innovation adoption
BMC Health Services Research
ISSN: 1472-6963
- General enquiries: [email protected]
Theoretical background and literature review
Cite this chapter.
- Monika Mischke 2
462 Accesses
This chapter provides the theoretical backdrop of the study, giving an overview of existing approaches and describing empirical results in the literature. The first section briefly discusses the concept of institutions and describes insights from institutional theory. This section addresses the theoretical relationship between institutions and individuals and the question of how institutions impact on human behavior, preferences, and attitudes.
This is a preview of subscription content, log in via an institution to check access.
Access this chapter
- Available as PDF
- Read on any device
- Instant download
- Own it forever
- Available as EPUB and PDF
- Compact, lightweight edition
- Dispatched in 3 to 5 business days
- Free shipping worldwide - see info
Tax calculation will be finalised at checkout
Purchases are for personal use only
Institutional subscriptions
For critics and extensions made by other scholars, please refer to Leibfried (1992), Castles and Mitchell (1993), Ferrera (1996), Bonoli (1997), Arts and Gelissen (2002), and Scruggs and Allen (2006).
See Ferrarini (2006: Chpt. 3 ) for a thorough analysis of the political determinants of familypolicy development, such as class-political factors and women’s role in political decision making.
An exception might be the group of migrant families. However, the topic of migration and the social rights of migrants, e.g., in terms welfare state entitlements go beyond the scope of this study. For a discussion of these issues, see Alesina and Glaeser (2004) and Mau and Burkhardt (2009).
The idea of subsidiarity implies “that social services should be provided for at the lowest possible level in the community, public authorities playing a role only in the event that churches and families are unable to do so” (Fagnani 2007: 43).
I.e., “when a service is rendered as a matter of right, and when a person can maintain a livelihood without reliance on the market” (Esping-Andersen 1990: 21/22).
For a discussion of the distinctiveness of the Southern European countries, see also Ferrara (1996) and Karamessini (2008), who discussed the distinctiveness of this group of countries with respect to several fields of welfare-state intervention.
After the Second World War, Germany was divided into two countries. Between 1949 and 1989, the “German Democratic Republic” (East Germany) had a state socialist system with a centrally planned economy with socialist employment and family policies, whereas the “Federal Republic of Germany” (West Germany) had a multi-party parliament, a market economy, and a conservative-corporatist welfare state (Rosenfeld et al. 2004: 103).
Author information
Authors and affiliations.
Siegen, Deutschland
Monika Mischke
You can also search for this author in PubMed Google Scholar
Rights and permissions
Reprints and permissions
Copyright information
© 2014 Springer Fachmedien Wiesbaden
About this chapter
Mischke, M. (2014). Theoretical background and literature review. In: Public Attitudes towards Family Policies in Europe. Springer VS, Wiesbaden. https://doi.org/10.1007/978-3-658-03577-8_2
Download citation
DOI : https://doi.org/10.1007/978-3-658-03577-8_2
Publisher Name : Springer VS, Wiesbaden
Print ISBN : 978-3-658-03576-1
Online ISBN : 978-3-658-03577-8
eBook Packages : Humanities, Social Sciences and Law Social Sciences (R0)
Share this chapter
Anyone you share the following link with will be able to read this content:
Sorry, a shareable link is not currently available for this article.
Provided by the Springer Nature SharedIt content-sharing initiative
- Publish with us
Policies and ethics
- Find a journal
- Track your research

COMMENTS
Examples of literature reviews. Step 1 - Search for relevant literature. Step 2 - Evaluate and select sources. Step 3 - Identify themes, debates, and gaps. Step 4 - Outline your literature review's structure. Step 5 - Write your literature review.
Conducting a literature review, selecting a theoretical framework, and building a conceptual framework are some of the most difficult elements of a research study. It takes time to understand the relevant research, identify a theoretical framework that provides important insights into the study, and formulate a conceptual framework that ...
This is generally referred to as the "literature review," "theoretical framework," or "research background." However, for a literature review to become a proper research methodology, as with any other research, follow proper steps need to be followed and action taken to ensure the review is accurate, precise, and trustworthy.
A literature review and a theoretical framework are both important components of academic research. However, they serve different purposes and have distinct characteristics. In this article, we will examine the concepts of literature review and theoretical framework, explore their significance, and highlight the key differences between the two.
Understanding similarities and differences among the literature review, theoretical framework, and conceptual framework can help novice and experienced researchers in organizing, conceptualizing ...
Revised on 10 October 2022. A theoretical framework is a foundational review of existing theories that serves as a roadmap for developing the arguments you will use in your own work. Theories are developed by researchers to explain phenomena, draw connections, and make predictions. In a theoretical framework, you explain the existing theories ...
With this in mind, a complete theoretical framework will likely not emerge until after you have completed a thorough review of the literature. Just as a research problem in your paper requires contextualization and background information, a theory requires a framework for understanding its application to the topic being investigated.
A literature review is a survey of scholarly sources on a specific topic. It provides an overview of current knowledge, allowing you to identify relevant theories, methods, and gaps in the existing research. There are five key steps to writing a literature review: Search for relevant literature. Evaluate sources. Identify themes, debates and gaps.
It is important to mention that unlike in the theoretical background, the literature review uses peer-reviewed journal articles and conference proceedings more extensively than books and grey literature. This distinction between the background discussion and the literature review is vital to good research design.
Abstract. The theoretical background introduces and critically comments on definitions, theories and explanatory approaches in relation to problematic and non-habitual, controlled drug use; deficiency-oriented theories of drug use; characteristics of traditional samples used in drug and specifically heroin studies; qualitative drugs research ...
A literature review is defined as "a critical analysis of a segment of a published body of knowledge through summary, classification, and comparison of prior research studies, reviews of literature, and theoretical articles." (The Writing Center University of Winconsin-Madison 2022) A literature review is an integrated analysis, not just a summary of scholarly work on a specific topic.
A literature review may consist of simply a summary of key sources, but in the social sciences, a literature review usually has an organizational pattern and combines both summary and synthesis, often within specific conceptual categories.A summary is a recap of the important information of the source, but a synthesis is a re-organization, or a reshuffling, of that information in a way that ...
Literature Review, Research, Theoretical Framework. What is a Literature Review. A literature review is a vital component of a research study. A literature review is a discussion on the already existing material in the subject area. Thus, this will require a collection of published (in print or online) work concerning the selected research area.
Writing a literature review requires a range of skills to gather, sort, evaluate and summarise peer-reviewed published data into a relevant and informative unbiased narrative. Digital access to research papers, academic texts, review articles, reference databases and public data sets are all sources of information that are available to enrich ...
Introduction. Literature review is an essential feature of academic research. Fundamentally, knowledge advancement must be built on prior existing work. To push the knowledge frontier, we must know where the frontier is. By reviewing relevant literature, we understand the breadth and depth of the existing body of work and identify gaps to explore.
Theoretical Review The purpose of this form is to concretely examine the corpus of theory that has accumulated in regard to an issue, concept, theory, phenomenon. The theoretical literature review help establish what theories already exist, the relationships between them, to what degree the existing theories have been investigated, and to ...
A literature or narrative review is a comprehensive review and analysis of the published literature on a specific topic or research question. The literature that is reviewed contains: books, articles, academic articles, conference proceedings, association papers, and dissertations. It contains the most pertinent studies and points to important ...
Theoretical Framework Example for a Thesis or Dissertation. Published on October 14, 2015 by Sarah Vinz . Revised on July 18, 2023 by Tegan George. Your theoretical framework defines the key concepts in your research, suggests relationships between them, and discusses relevant theories based on your literature review.
This infographic lists 6 differences to help you distinguish between the background of a study and a literature review. Feel free to download a PDF version of this infographic and use it as a handy reference. How to write the background of your study. 8 Dos and 8 don'ts of writing an engaging study background.
Literature review and theoretical background. It has become imperative for both scholars and practitioners to understand the factors influencing telemedicine adoption. To address this, researchers have proposed several theoretical models to elucidate the complexities of telemedicine acceptance. Commonly utilized models include UTAUT, UTAUT2 ...
26 2 Theoretical background and literature review. 2.1 Institutional theory. Institutional theory has been used extensively to illuminate the impact of institutions on political and corporate actors (for an overview, see, Oliver and Mossialos 2005), whereas studies looking at institutions' effects on individuals are rare (Wendt et al. 2011 ...
Section "Literature review" presents a literature review. Section "Policy background and theoretical analysis" details our theoretical analysis and research hypotheses.
In this study, the concourse was developed from the informational qualities via (i) a literature review on students' academic well-being, which identified a framework with the dimensions of internal and external sources of academic well-being, and (ii) a quantitative study that validated a 37-item instrument on academic well-being (Chen, Du, et ...
Based on a broad analysis of the literature, their review offers a theoretical "Interprofessional framework" that includes the notion of IPO as an additional and different possible cause for desired interprofessional outcomes (Fig. 2). They define IPO interventions as "changes at the organizational level (e.g. space, staffing, policy) to ...
Background Artificial intelligence (AI) technologies are expected to "revolutionise" healthcare. However, despite their promises, their integration within healthcare organisations and systems remains limited. The objective of this study is to explore and understand the systemic challenges and implications of their integration in a leading Canadian academic hospital. Methods Semi-structured ...
Abstract. This chapter provides the theoretical backdrop of the study, giving an overview of existing approaches and describing empirical results in the literature. The first section briefly discusses the concept of institutions and describes insights from institutional theory. This section addresses the theoretical relationship between ...