- About ClinChoice
- Partnerships
- Our SEG/DEI Commitment
- Solutions Overview
- Biostatistics
- Statistical Programming
- Data Management
- CDISC eSubmission
- Project Management
- Clinical Monitoring
- Site Startup
- Site Service
- Case Processing
- Aggregate Report Development and Submission
- Signal Management
- Literature Monitoring
- Risk Evaluation & Mitigation
- Safety Data Exchange Agreements
- Pharmacovigilance System Master File (PSMF) Management
- Medical Information Call Center (MICC) Solutions
- Local Safety Officer (LSO) Services
- Medical Device Call Center
- Incident Reporting
- Medical Writing
- Market Intelligence and Strategy
- Electronic Submissions Publishing
- SPL Conversion Services
- Global Regulatory CMC Services
- Company Core Data Sheet Management Services
- Labeling and Artwork Management
- Legal Entity Alignment
- Regulatory Information Management
- Document Management
- Product Registration Support
- Regulatory Intelligence
- Regulatory Compliance
- Regulatory Strategy
- Registration Support
- Technical Document Submission & Approval Preparation
- Artwork & Labeling Services
- Copy Review Development
- Change Control Management
- EUDAMED Support
- Medical Affairs
- Pharmacology & Toxicology
- Quality & Compliance
- Scientific & Strategic Consulting

Therapeutic Areas
- Therapeutic Areas Overview
- Anti-Infective
- Autoimmune Diseases
- Musculoskeletal
- Ophthalmology
- Rare Disease
- Respiratory
- Early Development
- Phase II-IV Clinical Trials
- Real-World Evidence
- Medical Devices
- Consumer Products
- Natural Products
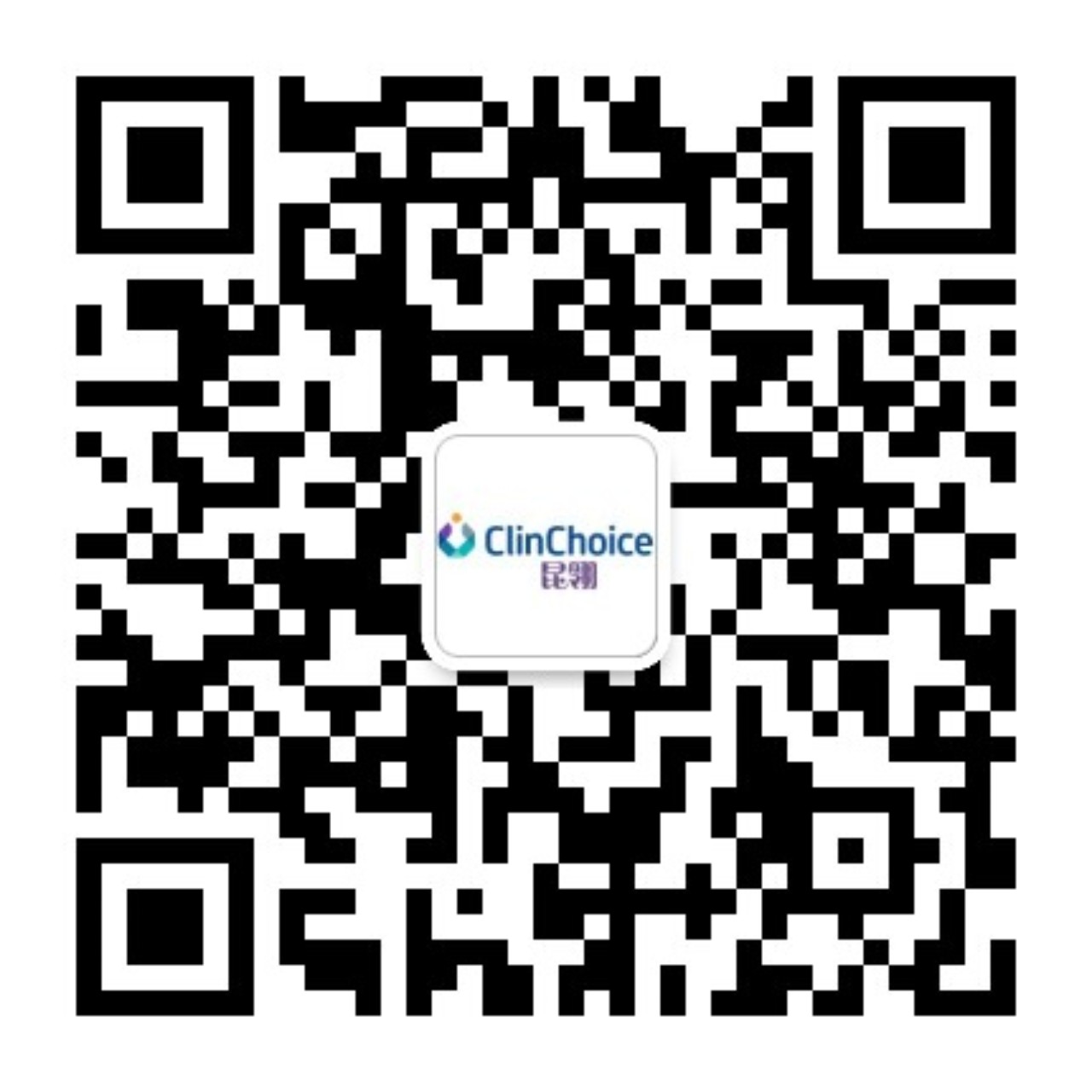
Home » Pharmacovigilance: Literature Monitoring Best Practices
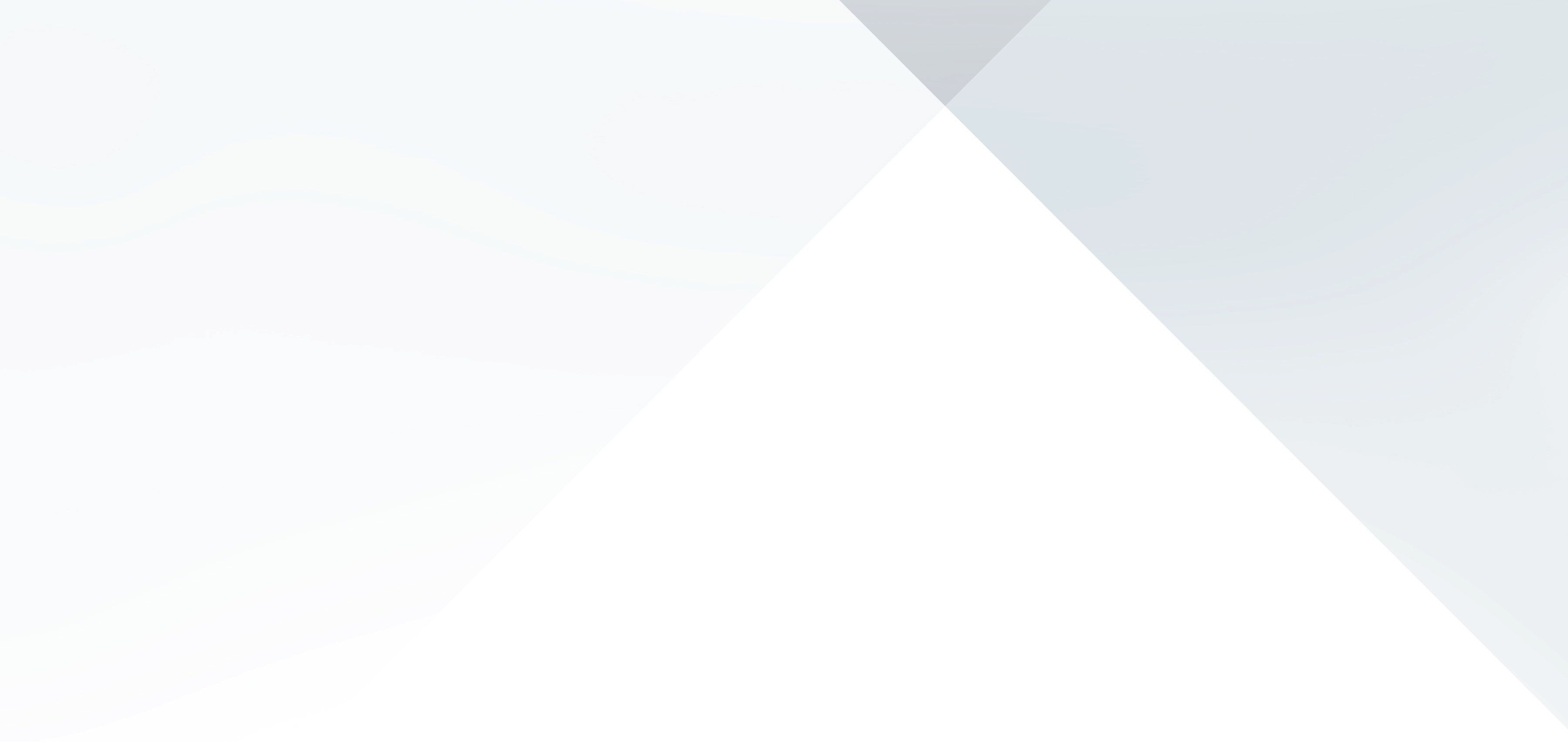
Pharmacovigilance: Literature Monitoring Best Practices
Your download is now available.
By ClinChoice
Safe and effective use of health products is a key objective of pharmacovigilance. Information is provided about the safety of these substances to patients, healthcare providers, and the general public as soon as possible. Pharmacovigilance includes reviewing the development, management, and introduction of pharmaceuticals. It is probably the most tightly regulated part of the pharmaceutical industry. Pharmacovigilance aims to identify, detect, assess, and report any adverse drug reaction (ADR) related to pharmaceutical products. In the United States, the European Union, and other parts of the world, regulatory requirements have emerged that have grown in diversity and nuance. These requirements include systematic monitoring and review of medical literature, including comprehensive screening of medical journals for adverse drug reactions, which remain on the rise. Having a robust pharmacovigilance system is paramount for a manufacturer, and any deficiencies can have an adverse effect on patient safety.
Literature monitoring includes published articles, articles, and reviews in indexed or non-indexed journals, any content posted anywhere online, posters and conference abstracts, etc. Holders of a Marketing Authorization (MA) must monitor global and local literature throughout the duration of that authorization, regardless of the availability of the product on the market1. Regulatory reports, clinical trial reports, literature reports, license partner reports, and spontaneous reports all serve as sources of data for deeper analysis of regulatory reporting, signal detection, and aggregate reporting. The individual safety report (ICSR) is valuable for developing risk assessments. It is incumbent upon holders of marketing authorizations to stay up-to-date on potential publications (including ahead of print articles) by reviewing widely used reference databases (e.g., Medline, Embase, Excerpta Medica) every week 2 . Adverse events that meet the criteria for the ICSR are handled per regulatory guidelines on handling and reporting adverse events. When a relevant article has been identified, it will be further screened to determine if it meets the four essential criteria for consideration for Individual case safety report (ICSR) and adverse event reporting: 1) identified source, 2) company product, 3) patient, 4) adverse event 3 . Any analysis regarding the safety profile of a product should be based on scientific and medical publications. Literature searches and monitoring are primarily intended to identify single case reports of adverse effects and to track any changes in benefit-risk profiles associated with the drug, particularly when new safety signals safety concerns arise 4 .
Literature Monitoring: An Overview of Best Practices
When the foundation is compromised, a process can result in a cascade of unintended repercussions. Therefore, an unbiased search is vital for monitoring medical literature accurately and efficiently. The growing volume of data has made it more critical to get the best results without introducing unwanted data. The literature monitoring process is usually characterized by two major challenges, which can be overcome. The first challenge is to come up with the right search strategy, and the second is to deal with duplication. Drug manufacturers must often track hundreds of drugs at once. So how can literature monitoring be accurate and valid?
Optimal Search Strategy Design and Database Selection
Regulatory authorities require marketing authorization holders to conduct medical literature surveillance at least weekly according to the GVP module VI and based on the required frequency as described by the local regulatory authorities, both for globally indexed literature databases and locally (non-indexed) literature journals 5 . When developing search strategies, it is important to consider ICSRs, aggregate reports, and any potential safety-related information. Therefore, it is essential to develop and progressively improve search strategies to limit the risk of overlooking relevant ADR information. Specifically, to retrieve all relevant records, query terms must be highly recallable and carefully crafted to retrieve maximum publications reporting any safety concerns about the product in question.
The database must be comprehensive and meet minimum standards to ensure that safety-critical signals are not missed. Pharmacovigilance searchers typically utilize at least two databases, usually three or more, because having access to multiple databases increases their recall-finding capabilities, ensuring more coverage.
Implement a search approach that balances the need for accuracy and precision. For example, 1) use several Boolean operators, 2) browse a thesaurus of terms, 3) perform proximity search, and 4) incorporate abbreviations to recall results. Using the most recent thesaurus update will ensure accuracy and compliance 6 .
As part of the local literature review, it is recommended to identify the non-indexed journals published locally and to screen those in either an online or print format depending on their availability. There are a few local regulatory agencies that recommend performing local literature searches in a few databases that are locally approved. The MAH handles any publications identified as containing information in local languages in accordance with the translation process established within the institution.
Industry best practice calls for constant review of search terms and updating them based on safety-related updates pertaining to the products. A GOLD standard data set of records is used to validate the modified search strategies. It is recommended to review your search strategy annually and make amendments as necessary 7 .
EMA hosts a robust system for medical literature monitoring. Thousands of records are added daily. It is generally the responsibility of marketing authorization holders to monitor medical literature and report individual cases of suspected adverse reactions into EudraVigilance and national safety databases 8 . They are not required to monitor or report suspected adverse reactions for active substances to EudraVigilance for substances covered by EMA’s service 9 .
Duplicate Data Management
Scientific publications and medical literature are abundant with sources and references, so it’s likely that the same publication could be indexed in multiple formats across a variety of journals, which results in duplicate findings. This creates a whole series of redundant tasks and false signals regarding drug safety. It leads to erroneous evaluations and, ultimately, compliance problems. Duplicate management processes, however, can solve this issue. Even though this is the best way of dealing with articles, it comes with a few challenges. There may be limitations to duplicate identification within the tool due to the presence of special characters, or it may be the case that the same study has been published across different journals or conference abstracts, making the process cumbersome.
It is important to search multiple databases to capture multiple publications across different journals. Keeping track of previous searches will also facilitate the identification of duplicates. To identify duplicate publications, there should not be just a focus on the article title but also the author’s name and, in some cases, the name of the study cited in the article.
According to Article 107(3) of Directive 2001/83/EC, to avoid duplicate submissions of ICSRs, the holder of a marketing authorization must submit the ICSRs that are not already assessed or monitored by EMA through the Medical Literature Monitoring (MLM) services 10 .
Service providers should use a standardized and well-established deduplication system, enabling them to confirm that they are not missing relevant references or creating duplicates inadvertently.
Along with routine literature surveillance, MAH also conducts targeted literature searches, which are searches specifically designed to answer a specific research question. When conducting signal analysis, these searches are conducted to confirm or disprove the association between the adverse event and the product.
Pharmacovigilance involves a substantial amount of literature monitoring . The process of devising a solid search strategy could be challenging but is essential. A professional with the required skills, experience, and training will ensure adverse event-related safety information is never missed. It is necessary to develop and maintain search strategies, elicit ideas from different stakeholders, and develop approved and suitable strategies for the purpose at hand. It is critical to set up a thorough process to handle and manage duplicate articles. Regularly review search strategies, and ensure the documentation is robust to ensure the finest quality results. The following points can be considered to check whether the MAH’s literature monitoring systems meet quality standards;
- A drug safety expert with experience researching literature is needed.
- Conduct risk assessments to ensure that the search criteria are robust and relevant to the objective of the literature search.
- Conduct literature searches and evaluate the results for literature per regional requirements (Global and Local).
- Monitoring and reviewing the Eudravigilance Medical Literature Monitoring (MLM) system, managed by EMA, to identify ICSRs in the literature if your product is included in the active ingredient screened by EMA.
- The search string is reviewed and updated annually to optimize results.
About the Author
Dr. Poonam Wagle; Associate Manager, Pharmacovigilance
Poonam brings expertise in literature management as a pharmacovigilance and safety expert with over eleven years of experience in the areas of ICSR, Literature, Aggregate Reports, and Signal Reviews. She has established and led several projects and programs in the field, including ICSRs, and literature monitoring, in both global and local surveillance.
ClinChoice is a leading global Contract Research Organization (CRO), with over 3400 clinical research professionals across North America, Asia, and Europe. For more than 27 years, ClinChoice has been providing high-quality contract research services to pharmaceutical, biotechnology, medical device, and consumer products clients, encompassing a broad range of services and therapeutic areas. ClinChoice offers cutting-edge, full-service solutions for Clinical Trials, Regulatory Affairs, Medical Device Safety, Toxicology, and Medical Affairs.
- 1) https://www.ema.europa.eu/en/human-regulatory/post-authorisation/pharmacovigilance/medical-literature-monitoring
- 2) https://www.ema.europa.eu/en/documents/scientific-guideline/guideline-good-pharmacovigilance-practices-module-vi-management-reporting-adverse-reactions_en-0.pdf
- 3) https://www.ema.europa.eu/en/documents/scientific-guideline/guideline-good-pharmacovigilance-practices-gvp-module-vi-collection-management-submission-reports_en.pdf
- 4) https://database.ich.org/sites/default/files/E2D_Guideline.pdf
- 5) https://europa.eu/en/documents/scientific-guideline/guideline-good-pharmacovigilance-practices-gvp-module-iv-pharmacovigilance-audits-rev-1_en.pdf
- 6) https://www.ema.europa.eu/en/documents/other/monitoring-medical-literature-entry-relevant-information-eudravigilance-database-european-medicines_en.pdf
- 7) https://www.elsevier.com/solutions/embase-biomedical-research/coverage-and-content
- 8) https://www.ema.europa.eu/en/human-regulatory/post-authorisation/pharmacovigilance/medical-literature-monitoring
- 9) https://www.ema.europa.eu/en/human-regulatory/post-authorisation/pharmacovigilance/medical-literature-monitoring
- 10) https://www.ema.europa.eu/en/human-regulatory/post-authorisation/pharmacovigilance/medical-literature-monitoring
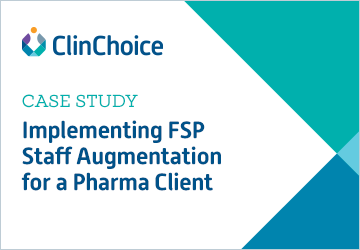
This case study shows a real-world example of ClinChoice implementing FSP...
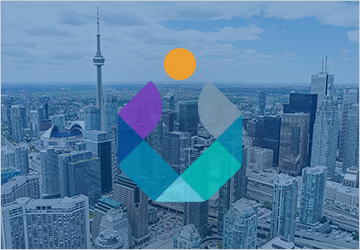
ClinChoice Opens Canadian Development Center - Expanding its North American...
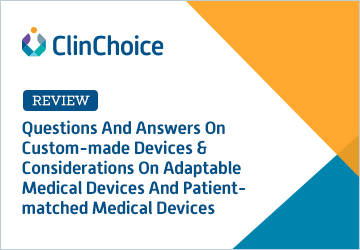
This review explores questions and considerations around custom-made, adaptable...
Want to be the first to know about new blogs and resources? Sign up for our mailing list.
Please fill out this form to unlock your download.
- First Name *
- Last Name *
- Company Name *
- Accept ClinChoice Privacy Policy* * Accept ClinChoice Privacy Policy*
This site uses cookies to ensure you get the best experience. Read our Privacy Policy
Privacy settings
Privacy Settings
This site uses functional cookies and external scripts to improve your experience. Which cookies and scripts are used and how they impact your visit is specified on the left. You may change your settings at any time. Your choices will not impact your visit.
NOTE: These settings will only apply to the browser and device you are currently using.
Consent Bar
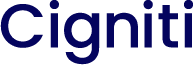
- Communications
- Energy and Utilities (E&U)
- Financial Services
- Healthcare & Life Sciences
- Manufacturing
- Media & Entertainment
- Retail and Ecommerce
- Travel & Hospitality
- Artificial Intelligence Testing
- Big Data & Analytics Testing
- Blockchain Testing
- Cloud Migration Assurance
- Security Assurance
- Internet of Things (IoT) Testing
- Mobile Testing
- Robotic Process Automation (RPA)
- 5G Assurance Services
- DevOps Testing
- Compatibility Testing
- Functional Testing
- Performance Testing
- Regression Testing
- Security Testing
- Test Automation
- Crowdsourced Testing
- Quality Engineering
- ERP Testing
- Salesforce Testing
- Medical Devices Testing
- Agile Testing
- Test Data Management
- Service Virtualization
- DevOps Transformation
- Testing Center of Excellence
- Test Advisory & Transformation Services
- Build Operate Transfer (BOT) Model
- Programmatic Innovation
- Agile Transformation
- Software Product Engineering
- Application Modernization
- Blockchain Engineering
- Enterprise Application Development
- User Experience Engineering
- Site Reliability Engineering
- Data Insights
- AI & ML
- Verita – Quality Engineering Dashboard
- Velocita – Test Automation Accelerators
- Praxia – Process Accelerator
- MAP – Model Assurance Platform
- CLAP – Cloud Migration Assurance Platform
- iNSta – Intelligent Scriptless Test Automation
- Cesta – Integrated Migration Solutions
- Incight – App Experience Analyzer Tool
- Zastra.ai – Active Learning Driven Annotation Platform
- Performance Engineering Lab
- Robotics Lab
- IOT and Smart Meters Lab
- Case Studies
- Digital ARMER
- Digital Dialogues – Podcast
- Digital Dialogues – Webinars
- herDIGITALstory TM
- Newsletter – Cignizine
- Tech Briefs
- Testimonials
- White Papers
- Infrastructure
- Cigniti in the News
- Press Releases
- Board of Directors
- Board Code of Conduct
- Annual Reports
- Corporate Presentation
- Quarterly Results
- Shareholding Pattern
- Announcements
- Analyst Recognitions
- Cigniti Careers
- Campus Connect
- Alumni Relations
- Life @ Cigniti
- Diversity, Equity, and Inclusion
- Corporate Social Responsibility
- Sustainability
Literature Search in Pharmacovigilance & Drug Safety: A Guide
Pharmacovigilance is a domain that works entirely on drug safety through the collection, detection, assessment, monitoring, and prevention of adverse effects associated with pharmaceutical products. It is a science that needs no introduction these days, thanks to increased vigilance in pharmaceutical drug discovery and development, as well as the introduction of new products to the market.
For Drug safety or pharmacovigilance, data will be collected from different sources to understand and assess the drug, either in its development stage while doing clinical trials or after releasing it into the larger population to understand its safety and efficacy in a wide range of populations.
Spontaneous reports, clinical trial reports, literature reports, license partner reports, and regulatory reports are major reports a company receives and processes for further analysis on regulatory reporting, signal detection, and aggregate reporting. Out of different source data, literature case reporting is one of the essential inputs, as the literature reports sometimes come with a combination of information from other report types.
This makes it more critical for companies to collect the literature information about the cases or articles involved with their drug. Literature article collection is required to assess a drug’s benefit-risk profile and comply with the regulatory requirement of not missing any reportable case within the required timelines.
Role of EMA
The EU health authority, EMA, is also responsible for monitoring several substances and selecting medical literature to help identify suspected adverse reactions to medicines authorized in the European Union. EMA’s Medical Literature Monitoring (MLM) services started on September 1, 2015. EMA maintains this information in its database called EudraVigilance. However, EMA can only monitor a certain number of substances based on information submitted to EMA’s Article 57 database by marketing authorization holders. So, the companies are responsible for monitoring their products in the global and local literature databases.
As per the EMA, marketing authorization holders are usually responsible for monitoring the medical literature on their medicines and reporting individual cases of suspected adverse reactions into EudraVigilance and national safety databases, which are not covered under the EMA’s services for that marketing authorization holder.
It was also declared that all products having marketing authorization, regardless of commercial status, should undergo literature searches. As a result, it’s reasonable to expect that literature searches begin with submitting a marketing permission application and continue throughout the authorization.
Where to search for literature articles?
Medline is a well-known database with the latest medicinal product information. Along with Medline, the other databases with the newest information on published medical literature articles or journals are Embase and Excerpta Medica. The published articles or journals and relevant abstracts from meetings and draught manuscripts should be reviewed for valid ICSRs and included in periodic safety update reports.
Literature search, monitoring, and screening
As we discussed above, the goal of literature search and monitoring is to identify individual case safety reports and any possible changes to the benefit-risk profile of the substance being monitored, particularly about detecting new safety signals or emerging safety issues.
Marketing authorization holders should perform medical literature monitoring on their products from day one of product authorization until they are active, whether the product is in the market or not. All the medical literature published globally and locally should be monitored and screened for all the adverse events reported in that literature for ICSR processing, as the medical literature is an essential source of information for identifying suspected adverse reactions to authorized medicines.
Once an article is identified as relevant to the MAH’s product, it will be screened further to determine whether it meets its four minimum criteria (identified reporter, company product, patient, and an adverse event) to consider it for further case processing and adverse event reporting. If there are any signals to consider for aggregate reporting and benefit-risk assessment, they will be shared for further review by the MAH.
How can Cigniti help you with this?
From this article, we got an idea of how important it is to monitor and screen the published literature articles and abstracts about an authorized medicinal product for an MAH. We at Cigniti have our experts to help you with literature monitoring and screening for your timely reporting of ICSR.
Our experts work with you to understand the requirements and develop a search strategy based on your global and local literature data collection requirements. We will work with you to understand the purpose of your requirement, whether it is for ICSR reporting, signal detection, or aggregate reporting, and provide you with a solution that meets the regulatory requirements while being compliant with timelines.
Schedule a discussion with our healthcare and life sciences experts to learn more about the criticality of literature search in Drug Safety and Pharmacovigilance.
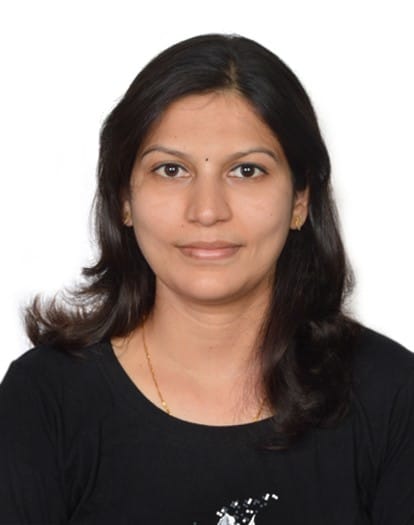
Anusha Chowdary is a Senior Business Analyst and Domain expert for Healthcare with 10 years of experience in working with Healthcare and Healthcare IT. She is an experienced professional and subject matter expert in handling Drug safety applications. Her expertise includes thorough knowledge of health care and pharmaceutical regulations, with an educational background in Pharmacy.
View all posts
Leave a Reply
Your email address will not be published. Required fields are marked *
Related Posts
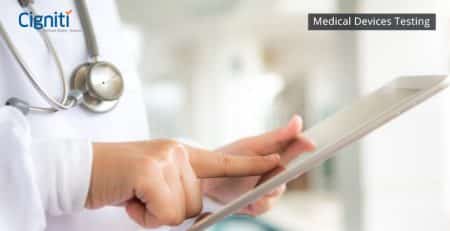
Remote Monitoring Devices – The digital Healthcare strategy to combat a pandemic
By Cigniti Technologies
Listen on the go! With the positive corona virus cases crossing over 1 million globally, we are at a risk of overwhelming our... read more
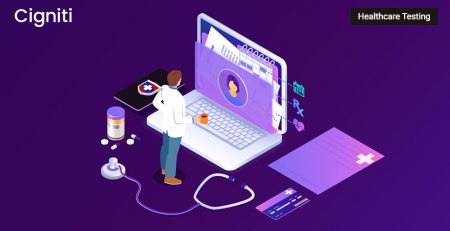
An Overview of HIPAA Compliance Testing in Software Applications
By Anusha Chowdary
Listen on the go! Are you using any software that is related to an individual’s information? Anything that deals with... read more
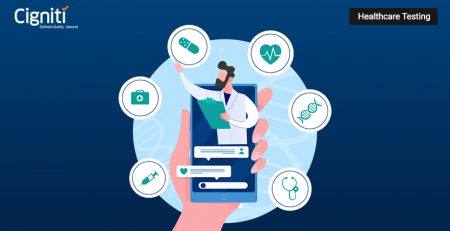
Validation and its Approach to Healthcare and Life Science Applications
Listen on the go! Validation is the most used and common term to verify the GxP systems, whether they are... read more
- Skip to main content
- Skip to FDA Search
- Skip to in this section menu
- Skip to footer links

The .gov means it’s official. Federal government websites often end in .gov or .mil. Before sharing sensitive information, make sure you're on a federal government site.
The site is secure. The https:// ensures that you are connecting to the official website and that any information you provide is encrypted and transmitted securely.
U.S. Food and Drug Administration
- Search
- Menu
- Regulatory Information
- Search for FDA Guidance Documents
- Good Pharmacovigilance Practices and Pharmacoepidemiologic Assessment
GUIDANCE DOCUMENT
Good Pharmacovigilance Practices and Pharmacoepidemiologic Assessment Guidance for Industry March 2005
This document provides guidance to industry on good pharmacovigilance practices and pharmacoepidemiologic assessment of observational data regarding drugs, including biological drug products (excluding blood and blood components). Specifically, this document provides guidance on (1) safety signal identification, (2) pharmacoepidemiologic assessment and safety signal interpretation, and (3) pharmacovigilance plan development.
Submit Comments
You can submit online or written comments on any guidance at any time (see 21 CFR 10.115(g)(5))
If unable to submit comments online, please mail written comments to:
Dockets Management Food and Drug Administration 5630 Fishers Lane, Rm 1061 Rockville, MD 20852
All written comments should be identified with this document's docket number: FDA-2004-D-0041 .
LiSA: an assisted literature search pipeline for detecting serious adverse drug events with deep learning
Affiliations.
- 1 Quinten, 8 rue Vernier, 75017, Paris, France. [email protected].
- 2 Quinten, 8 rue Vernier, 75017, Paris, France.
- 3 Swiss Agency for Therapeutic Products, Swissmedic, Hallerstrasse 7, 3012, Bern, Switzerland.
- 4 UMMISCO, Sorbonne University, IRD, Bondy, France. [email protected].
- PMID: 36550485
- PMCID: PMC9773506
- DOI: 10.1186/s12911-022-02085-0
Introduction: Detecting safety signals attributed to a drug in scientific literature is a fundamental issue in pharmacovigilance. The constant increase in the volume of publications requires the automation of this tedious task, in order to find and extract relevant articles from the pack. This task is critical, as serious Adverse Drug Reactions (ADRs) still account for a large number of hospital admissions each year.
Objectives: The aim of this study is to develop an augmented intelligence methodology for automatically identifying relevant publications mentioning an established link between a Drug and a Serious Adverse Event, according to the European Medicines Agency (EMA) definition of seriousness.
Methods: The proposed pipeline, called LiSA (for Literature Search Application), is based on three independent deep learning models supporting a precise detection of safety signals in the biomedical literature. By combining a Bidirectional Encoder Representations from Transformers (BERT) algorithms and a modular architecture, the pipeline achieves a precision of 0.81 and a recall of 0.89 at sentences level in articles extracted from PubMed (either abstract or full-text). We also measured that by using LiSA, a medical reviewer increases by a factor of 2.5 the number of relevant documents it can collect and evaluate compared to a simple keyword search. In the interest of re-usability, emphasis was placed on building a modular pipeline allowing the insertion of other NLP modules to enrich the results provided by the system, and extend it to other use cases. In addition, a lightweight visualization tool was developed to analyze and monitor safety signal results.
Conclusions: Overall, the generic pipeline and the visualization tool proposed in this article allows for efficient and accurate monitoring of serious adverse drug reactions from the literature and can easily be adapted to similar pharmacovigilance use cases. To facilitate reproducibility and benefit other research studies, we also shared a first benchmark dataset for Serious Adverse Drug Events detection.
Keywords: Adverse drug events; Assisted literature review; Deep Learning; NLP.
© 2022. The Author(s).
Publication types
- Research Support, Non-U.S. Gov't
- Adverse Drug Reaction Reporting Systems
- Deep Learning*
- Drug-Related Side Effects and Adverse Reactions* / diagnosis
- Drug-Related Side Effects and Adverse Reactions* / epidemiology
- Reproducibility of Results
- Open access
- Published: 22 December 2022
LiSA: an assisted literature search pipeline for detecting serious adverse drug events with deep learning
- Vincent Martenot 1 ,
- Valentin Masdeu 1 ,
- Jean Cupe 1 ,
- Faustine Gehin 1 ,
- Margot Blanchon 1 ,
- Julien Dauriat 1 ,
- Alexander Horst 2 ,
- Michael Renaudin 2 ,
- Philippe Girard 2 &
- Jean-Daniel Zucker 3
BMC Medical Informatics and Decision Making volume 22 , Article number: 338 ( 2022 ) Cite this article
2795 Accesses
3 Citations
Metrics details
Introduction
Detecting safety signals attributed to a drug in scientific literature is a fundamental issue in pharmacovigilance. The constant increase in the volume of publications requires the automation of this tedious task, in order to find and extract relevant articles from the pack. This task is critical, as serious Adverse Drug Reactions (ADRs) still account for a large number of hospital admissions each year.
The aim of this study is to develop an augmented intelligence methodology for automatically identifying relevant publications mentioning an established link between a Drug and a Serious Adverse Event, according to the European Medicines Agency (EMA) definition of seriousness.
The proposed pipeline, called LiSA (for Literature Search Application), is based on three independent deep learning models supporting a precise detection of safety signals in the biomedical literature. By combining a Bidirectional Encoder Representations from Transformers (BERT) algorithms and a modular architecture, the pipeline achieves a precision of 0.81 and a recall of 0.89 at sentences level in articles extracted from PubMed (either abstract or full-text). We also measured that by using LiSA, a medical reviewer increases by a factor of 2.5 the number of relevant documents it can collect and evaluate compared to a simple keyword search. In the interest of re-usability, emphasis was placed on building a modular pipeline allowing the insertion of other NLP modules to enrich the results provided by the system, and extend it to other use cases. In addition, a lightweight visualization tool was developed to analyze and monitor safety signal results.
Conclusions
Overall, the generic pipeline and the visualization tool proposed in this article allows for efficient and accurate monitoring of serious adverse drug reactions from the literature and can easily be adapted to similar pharmacovigilance use cases. To facilitate reproducibility and benefit other research studies, we also shared a first benchmark dataset for Serious Adverse Drug Events detection.
Peer Review reports
The development of a drug is a long road that can take several years. This journey involves several requests for approval with regulatory authorities, whether to start clinical trials, to actually market the drug or to modify some of the claims. Throughout these approval processes, the regulator, that carries out a public safety mission, must ensure that no prior safety signal about the drug is known at the time or after the authorization is granted. This task requires the regulator to review and monitor both biomedical literature and surveillance reports. More specifically, medical reviewers have to identify portions of text mentioning an explicit association between a drug and a serious ADR. According to the EMA, a serious adverse event is “any untoward medical occurrence that at any dose:
results in death,
is life-threatening,
requires inpatient hospitalisation or prolongation of existing hospitalisation,
results in persistent or significant disability/incapacity, or
is a congenital anomaly/birth defect.”
It should be distinguished from what is called an Important Medical Event (IME) where the outcome might not fall into one of these 5 categories. For example, in the sentence There was one treatment-related death due to myositis in the pembrolizumab group. , the serious outcome (death) is clearly associated with the drug (pembrolizumab) through the expression (treatment-related). Conversely, in We observed Rivaroxaban-induced rash in \(60\%\) of the patients , the side effect mentioned cannot be qualified as serious. As such, it would be regarded as a safety issue by the regulator. Meanwhile, the tremendous increase of publication volume, and the number of treatments that require authorization in a limited time frame make it practically impossible for medical reviewers to review all documents exhaustively. Consequently, critical safety-related information can be missed when applying a human-only process.
Even though many publications have focused on literature review assistance [ 1 , 2 , 3 ] or on the detection of relationship between drug and ADR [ 4 , 5 , 6 , 7 , 8 ], only two have proposed approaches to tackle the detection of seriousness [ 9 , 10 ]. Meanwhile, in the first publication, the targeted documents are FAERS reports which differ from biomedical literature in terms of syntax and vocabulary. The second one, thus tested on biomedical corpus, does not provide any kind of relationship between a drug and an adverse event.
In this paper, we present LiSA (Literature Search Application), an AI-based system designed to assist medical reviewers in their market surveillance by automatically screening the biomedical literature to detect safety signals.
LiSA was designed to enable medical reviewers to monitor the publication of articles related to potential safety signals on medical treatments or medicines. More specifically, it is able to identify, filter and rank publications mentioning an established relationship between a specified drug and one or several serious Adverse Events (SAE), i.e. severe Adverse Drug Reactions (SADR). To meet these goals, we propose 4 contributions to the problem of pharmacovigilance information retrieval from open data literature:
A deep learning pipeline for the identification of serious adverse events within biomedical literature based on Pub-Med. The performance achieved is respectively of 81.1 \(\%\) in precision and 88.6 \(\%\) in recall.
A visualization tool designed to allow biomedical expert to review and monitor the results provided by the pipeline for specific drugs.
A modular pipeline built on pre-existing and independent open source models (transformers) allowing flexibility of usage for related use-cases in pharmacovigilance. This approach also provides more explainability compared to a lone neural network algorithm. The pipeline, instead of creating a new neural network algorithm with very specific outputs, is composed of independent algorithms providing intermediate outputs. These outputs are then combined to build an efficient and performing system aiming at qualifying and extracting the information corresponding to the following questions:
What are the monitored drugs and indication mentioned in the document?
What are the sentences that mention an established relationship between a drug and an AE?
What are the entities recognized as Drug or Adverse Event?
The identification of relevant documents regarding seriousness drug adverse reaction signals is then performed on the basis of this information and meta data available in the data source (Ex: date, journal, type of publication, etc...).
A benchmark dataset for seriousness classification task based on PubMed literature sentences.
After a review of related work, we describe the LiSA pipeline architecture and provide a high-level performance analysis of the proposed solution.
Related work
In most of the papers mentioned in this section, the focus is on Adverse Events (AE) detection and not on Adverse Drug Reaction (ADR), meaning that there is no specific detection of a drug associated with an adverse event. For the sake of clarity we will use, only in this part, the terms Adverse Drug Events (ADR) to indifferently designate AE or ADR.
Adverse Drug Reaction detection plays a key role in drug-safety surveillance and has motivated the creation of various monitoring systems or databases. The FAERS [ 11 ] reporting system and Medwatch [ 12 ], a medical product for safety reporting, are the current official solutions provided by the FDA. Meanwhile, these tools are only based on declarative reports and not on systematic analysis of the biomedical literature or any web-based source to identify potential ADRs. Several solutions have been proposed to perform biomedical literature monitoring in order to identify, filter and rank papers related to a specific domain or medical concept. For example, ASE [ 13 ] demonstrates the value of reference management, statistics, natural language summarizing to interactively select key papers. STELLAR [ 3 ] leverages data mining techniques to help researcher to identify, rank and recommend reference papers for a specific literature review. More recently, [ 1 ] proposed ASReview, an efficient active learning based-tool to perform systematic literature review and meta-analysis.
As per today, only a small number of literature review systems relate to adverse drug reactions detection. Among them, the PV-OWL tool [ 2 ] was built to link different databases to obtain novel safety indicators (FAERS, PubMed, social media...). The semi-automated pipeline published by [ 14 ] supports extracting ADR pairs from adverse events databases using statistical BPCNN algorithm for Natural Language Processing. Among other classical approaches commonly used in NLP, distributional semantics based on patterns of ADR co-reporting [ 15 ], Hidden Markov Models [ 16 ] or disproportionality analysis (DPA) [ 17 ] were already attempted to perform ADR detection. In 2012, Gurulingappa, Harsha et al. published an open-source reference dataset and developed a dictionary-based algorithm for extraction of adverse drug events in PubMed literature [ 18 , 19 ]. Following the significant advances in natural language processing with deep learning, more recent publications have exploited these technologies to improve safety signal detection. Several works perform ADR detection and extraction on social networks (e.g. Twitter) or on drugs review platforms like Drugs . com using deep learning techniques [ 4 , 5 , 6 , 7 , 8 ].
However, there is a lack of studies aimed at predicting the seriousness of adverse events or any other type of qualification. The seriousness of an adverse event is nevertheless critical since it will decide whether or not to trigger actions from the safety surveillance agencies. We only found two publications related to this specific topic. The first one from [ 9 ] is based on FAERS report and does not treat biomedical literature. On the contrary, the second provides a robust approach to detect, extract and categorize serious adverse events [ 10 ]. The study relies on three different deep learning algorithms for seriousness classification, seriousness categorization and seriousness annotation. Performance is evaluated on three datasets among which one is built on biomedical literature. Like the latter study from [ 10 ], which will also be used as the primary basis for performance evaluation, LiSA is capable of qualifying potential severity but differs in its ability to detect and extract adverse drug reaction entities and classify documents for display in a literature search tool interface.
The LiSA pipeline description
The architecture described in this section is the final result of a sequence of iterations aimed at improving the overall performance to maintain a satisfactory balance between precision and recall (more details are available in the “ Results and discussion ” sect.). The objective of the following steps is to identify and extract relevant information in documents (drug names, Adverse Events, association between drug and AE, seriousness,...) to be used for the final ranking and filtering of articles. The document processing pipeline is described in Fig. 1 below.
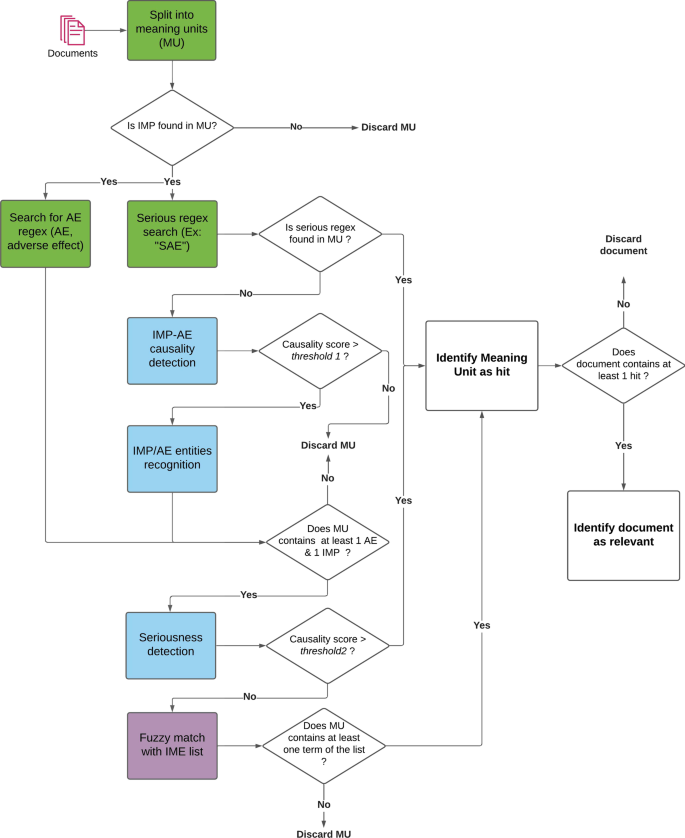
Decision diagram of the document processing pipeline. Green color boxes represent regex-based algorithms, blue color boxes represent deep learning based algorithms and purple color boxes fuzzy-matching based algorithms
Query definition and document collection
Most of the medical publications that mention adverse drug reaction are published and available through PubMed, a free archive of biomedical and life sciences journal literature and considered as a reference for biomedical publications. Some publications require a licensed access, but still provide a free version of the corresponding abstract. Therefore, PubMed was used as the main data source for literature monitoring to build the system. By construction, documents collection should be associated to a “query” which is composed of a combination search terms. A query contains a main drug, an optional second drug and optional indication. Indication should be only approved indication to avoid the case where indication and adverse events are confused. Consequently, LiSA collects all articles available on PubMed published on the last six years Footnote 1 associated to a query through the PubMed API. This timeframe was chosen as a good tradeoff between actuality of information and being sure not to miss a relevant signal that might have been reported a while ago. Only the main drug serves as keyword search to trigger the API. Other query terms (optional drug and indication) are only searched to tag the document if they are mentioned in it.
Drug and indication could be expressed under various synonyms in biomedical literature. To ensure the comprehensiveness of data collection, every drug and indication term is conjointly searched with all its synonyms based on open-source molecule and disease classification. For drug, we used the Chembl database [ 20 ], and for indications the MedDRA [ 21 ] hierarchy. Table 1 gives an example of a query definition.
Document preprocessing
This section describes the methodology applied to preprocess documents into a suitable format for deep learning algorithms described below.
Data preparation
To structure the documents, we propose a standard architecture able to accommodate any type of collected documents or data sources and adapted to natural language processing algorithms. As a matter of fact, raw documents cannot be processed directly by transformers and achieve a satisfying performance [ 22 ]. They should be split into meaning units of limited number of tokens like sentence or short paragraphs. This process, called sentence tokenization, is performed with a pre-built algorithm (on common English language) from the package nltk and adapted with specific cases found in biomedical literature.
Structured data is then formatted into 3 different tables:
Documents table: This table stores all the metadata and the full content of a document. This table contains one line per document.
Contents table: This table stores only the content of a document but split in different sections or paragraphs based on the pre-defined structuration already available in the document (e.g.: abstract, methods, results, conclusions...). The contents available in figures captions or tables was not collected.
Meaning units table: This table stores information at sentence level and is built from the contents table. A section or paragraph is split in different sentences and each sentence represents one line in this table. During the split, if a sentence is too short (between 4 to 10 words), it is concatenated with either the previous or the next one (only in case it is less than 20 words long) to reduce the risk of missing an AE-Drug relationship. These choices were applied for two reasons:
Concerning the maximum length of a meaning unit: BERT input size is limited to 512 tokens, which makes it impossible to use a whole article as input for prediction. Furthermore, it has been shown in the literature that BERT performs better on a limited number of tokens, therefore sentence as in input will be better than paragraph as in input.
Concerning the minimum length of a meaning unit: this decision was motivated by the empirical observation that in case of very short sentences, one information was actually present in the adjacent sentence. The threshold number of tokens was selected empirically and could be optimized in further work.
This generic structure has been designed to fit any type of document and serves as a basis for the visualisation tool presented in the “ Visualisation interface ” section.
Drug and indication search
The first filter applies to all meaning units found in collected documents and is based on a simple keyword search method. We use the Aho-corasick algorithm [ 23 ], an efficient dictionary-matching algorithm, to search for a drug term and associated synonyms in every meaning unit. Aho-corasick was used for its computation efficiency and because drug names have an invariant spelling in biomedical literature, there is thus no need to perform fuzzy-matching at this step. This association is then stored in the meaning units table. This step has a double objective:
First, to isolate the meaning units associated with the drug of interest (since LiSA is built to monitor serious adverse events associated with a defined drug).
Second, to reduce the number of meaning units to be used as input for the downstream deep learning modules that are more computationally intensive.
At the same time, a second keyword search is applied to identify mentions of therapeutic indications in historical documents. Unlike drug search, indication search is only performed at document level and is used to provide a clue of whether the document discusses about a drug aiming at treating a specific indication. The detection of an established relationship between a molecule and a disease is not performed in this pipeline. This task would be part of a possible improvement. The indication of interest are defined by biomedical reviewers and enriched with associated synonyms using the MedDRA hierarchy. Meanwhile, unlike drug names, indication terms are frequently composed of multiple tokens, which are not always expressed with the exact same form in the literature. For example the MedDRA indication “B-cell chronic lymphocytic leukaemia” could be found as “B-cell lymphocytic leukaemia” or “lymphocytic leukaemia of B-cell” in published articles. Therefore a simple expression search will most likely miss some expressions associated to the same indication. To overcome this problem, we built a fuzzy-matching algorithm allowing permuted and incomplete expression of an indication to be found in the text, which creates a list of expressions on the basis of a root indication. This list is composed of all permutations of the tokens contained in the root indication, with a random suppression of some of them to keep at least 2 tokens. All the expression of that list are then searched in the document, with the same Aho-corasick algorithm but allowing the presence of 20 characters between 2 consecutive tokens of the list. For example when searching for “B-cell lymphocytic leukaemia”, the expression “B-cell and C-cell lymphocytic leukaemia” will be accepted by this algorithm.
Deep learning
The three main AI modules presented in this section are the core of LiSA. They correspond to 3 different NLP tasks which are computed in parallel for all sentences containing a monitored drug (as described in the next section). Once calculated and stored in the database the different information are used to filter and qualify the hit sentences and relevant documents as depicted in Fig. 2 . Details about the different pre-trained algorithms and their respective performance are provided in Table 3 .
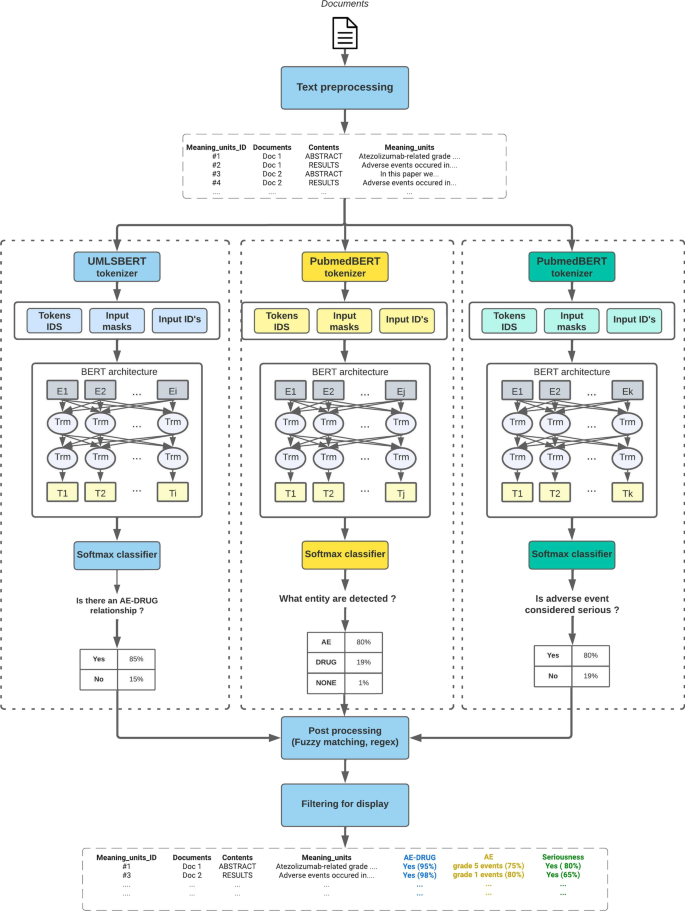
Schematized machine learning architecture of the LiSA pipeline and its three main modules. Unlike other post processing tasks, the serious regex search task is performed before deep learning inference and is not represented on the schema
Drug-AE relationship classification
To assess the association between a drug and an AE, we chose to rely on state of the art deep learning algorithms with attention-based mechanism (BERT). This family of algorithms is trained on very large corpora to build contextual embeddings and has been shown to perform extremely well in highly context-dependent prediction tasks, such as AE detection. The presence of a drug-AE causality relationship within a sentence was predicted with a two class (“has causality”, “has no causality”) sentence classifier, as defined in the ADE-Corpus-V2 dataset [ 18 ] used for training. This dataset contains more than 20 000 sentences extracted from PubMed and pre-labelled for drug-AE causality classification. In particular, the two classes are defined without prior knowledge of the entities corresponding to drug and ADRs. The ADE-Corpus-V2 dataset was split into training, validation, and testing sets with the ratio of 8:1:1 and used to fine-tune several pre-trained algorithms and to select the most accurate one.
In order to further increase prediction performance, we performed manual data augmentation based on badly predicted observations of ADE-corpus-V2. Typical treated case are sentences including a negation form, containing an unspecified adverse effect (“AEs”, “TRAEs”, “Serious adverse effects”) or related to specific lexical fields. The score threshold to predict a sentence as positive was chosen at 0.2. This value offers the highest possible recall and keep precision higher than 0.9 (threshold determination was manually performed based on a precision-recall curve) In the production version of LiSA, every meaning units predicted class and score are stored in the meaning units table.
Named Entity Recognition (NER)
LiSA is also supposed to identify the different entities found in a relevant document corresponding to a drug or an Adverse Drug Reaction. For this task, we used Named Entity Recognition (NER) pre-trained algorithms within the same family of algorithms built on BERT architecture. Using the same open source corpus, we fine-tuned and bench-marked several models for the task of identifying two different entities: drug and ADR.
The NER task was built as defined in the \(ADE-corpus-V2\) dataset [ 19 ]: find spans associated to 2 types of entities: DRUG and AE. No distinction was made between beginning, inside and outside tokens of a selected entity.
In the final pipeline, the entity detection is only applied on meaning units that successfully passed the drug-AE causality prediction with a score higher than the defined threshold (the standard threshold value 0.5 was used). This pre-filtering step was made to reduce the inference computation time. As for the previous step, detected entities and associated scores are stored in the meaning units database. The NER step was also applied after Drug-AE relationship classification since it reduces the computation time without major change in terms of performance. Inference time remains the most time-consuming task in the LiSA pipeline, which is critical for the system to be used in production.
Seriousness score prediction
According to the European Medicines Agency [ 24 ], an adverse event can be qualified of serious of the consecutive reaction to a treatment:
results in death
is life-threatening
requires inpatient hospitalisation or prolongation of existing hospitalisation
results in persistent or significant disability/incapacity
is a congenital anomaly/birth defect.
This definition clearly underlines the fact that the seriousness of an ADR is measured according to the outcome that it produces, whose expression in a document, is here again, highly context-dependent. BERT-like architecture based on contextual embeddings is once more a very promising solution. The same training framework applied in the two previous NLP tasks was applied here. We fine-tuned several pre-trained models on a sentence classification task. Unlike common ADR detection, we did not found an open access dataset to train the seriousness detection algorithm. This problem was overcome by labelling 7776 sentences extracted from PubMed in three categories: “serious”, “important medical event”, “none” (a “serious” sentence being an “important medical event” sentence with a serious outcome). The labelling process was performed by medical reviewers and based on examples extracted from positive examples of the \(ADE-corpus-V2\) dataset [ 18 ]. The third class “important medical event” was only added to have a more detailed labelled dataset for possible additional application in ADR detection. The ADR entities were not provided to the expert during annotation to force the annotator to take into account the full sentence and not only part of it (like extacted ADR) to make his decision. In addition, we performed data augmentation by semi-automatically building sentence examples to address some weaknesses of the algorithm in specific contexts or syntax (negation, cancer, etc..), that were also annotated by medical experts before being included in the training set. 917 sentences were used as a testing test and allowed to reach a performance at the state of the art. More concretely, this models yields a class and a score and is only calculated on meaning units that contains at least one drug entity and one ADR entity from the NER module.
Post-processing for performance improvement
Although the performances obtained by the previous pipeline on average matches the level reached in recent publications [ 10 , 19 ] (more details in the “ Results ” Sect.), it appeared that some specific cases were relatively badly predicted. A typical encountered issue was a random detection of non specific adverse events corresponding to expressions like “AE”, “adverse effects”, “TRAEs”,... To address those issues, different strategies were implemented in addition to the improvement of the three previous deep learning algorithms by data augmentation.
The first strategy implemented was the use of regular expressions that by themselves indicate the presence of an adverse event in sentence. A few example of these are “side/adverse event(s)/effect(s)/reaction(s)” or “(TR)AE(s)”. The same method is applied to the case of non specific serious adverse events with regular expressions such as “serious adverse event(s)/effect(s)/reaction(s)”, “grade 4/5 reaction(s)” or “SAE(s)”. This double search is applied on all meaning units containing an drug of interest since they are computationally light.
The second strategy used is specifically designed to catch serious adverse outcomes based on a list of terms built together with biomedical experts. That list contains expressions of diseases or reactions that are always associated with a serious outcome (death, hospitalization, infirmity, congenital, life-threatening). This is for example the case for “pneumonia”, “ventricular fibrillation”, “intracranial bleeding”,“teratogenic effects”. The same fuzzy-matching approaches as the one described in the previous section is applied in this case, since we are considering multiple-tokens expressions. Unlike regular expressions search, the fuzzy-matching is only applied to meaning units that were rejected by the seriousness score algorithm to optimize the computation time.
Document filtering and ranking
LiSA is built to provide a curated list of documents to the user, as well as the sentences where safety signals (called “hits”) are detected, and the recognized entities (drug and ADR). The decision process depicted in Fig. 1 is used to select and filter the documents to be finally displayed to the final user. It can appear counter-intuitive that the AE-drug relationship classification results are used before the entity recognition. This order showed the best performance and was selected after different experiments that are not detailed in this paper.
A rule-based system was also implemented to calculate a ranking score based on some information extracted from documents (sentence hit scores, number of hits per document,...). This score is then used by the user to rank the relevant papers in the visualisation interface.
Visualisation interface
Visualizing and exploring the results is key to ensure user adoption. Depending of the query definition, the pipeline can return a relatively large number of documents (volume of some example queries are provided on Fig. 5 ) indeed. In order to prevent users from being overwhelmed by a mass of articles to review, and in order for them to monitor results over time, we propose a simple exploration interface built with PowerBI, a powerful and cost-effective data visualisation tool. Captures of the two main interfaces are presented on Fig. 3 , 4 . First the QUERY DEFINITION interface allows a user to create or join search queries containing one or several search criteria, as defined above. Second, the RESULTS interface displays documents found in the literature, with at least one hit mentioning a serious drug adverse reaction. On the left side, a series of filtering options (publication date, indication found, AR Frequency, Route of administration, etc...) are available to help the user refine displayed results. These filters are fed by information already extracted by the pipeline, and by results from keyword searches performed by powerQuery (PowerBI’s data preparation engine). The results can be explored at a document/sentence level (high level results) showing only information down to the sentence and document, and at a more detailed level (detailed results) which includes ADR entities detected in the text.
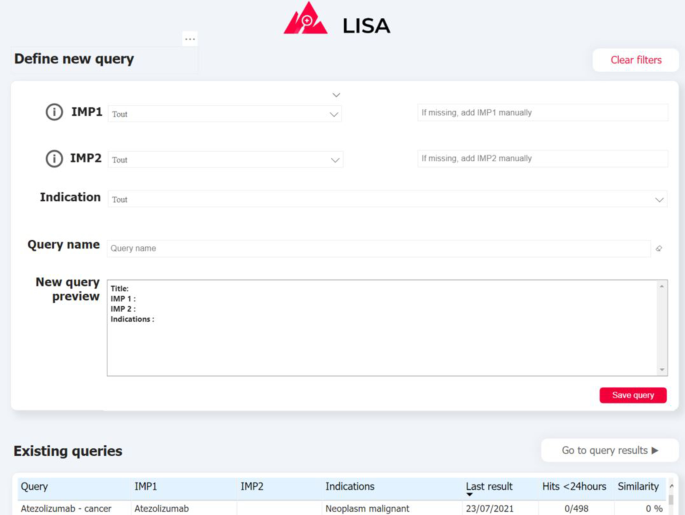
Screenshot of the “QUERY DEFINITION” tab of the interface. (1) Drug and indication dictionaries drop-down lists (2) Query preview (3) Summary of previously-created queries
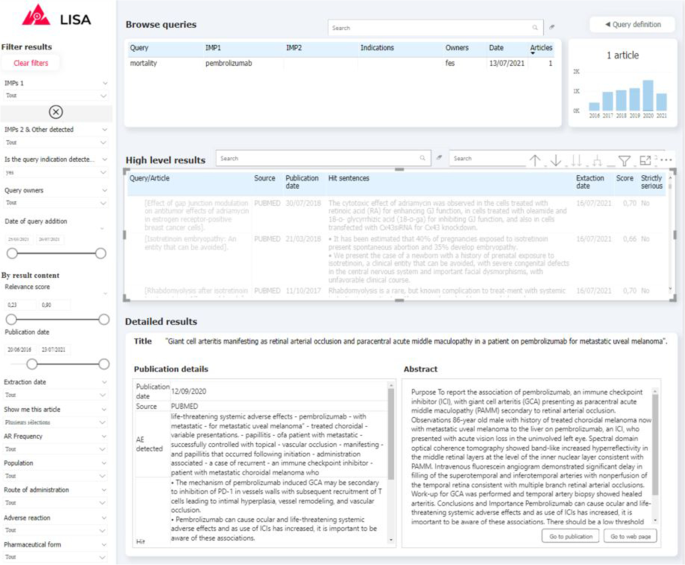
Screenshot of the “RESULTS” tab of the visualisation interface. (1) Query browser to select a set of results (2) Filtering tab to refine query results (3) High-level results table containing general information about results associated to the selected queries (4) Detailed results which provide additional information for every article selected in the high-level results table (5) Histogram of results volume of publication by year for the selected queries
Results and discussion
The following section is dedicated to:
Describing the obtained results and justify the need for the use of a new benchmark dataset for evaluating the task of serious ADR detection.
Discussing the limits of the current pipeline and pave the way for future work.
Performance assessment was performed with two strategies:
Evaluate the results based on a train/test approach on different datasets for different tasks. The performance of the tested models is displayed in Table 3 .
Evaluate the performance of LiSA from the perspective of medical reviewers (end users).
Implementation details
For individual NLP tasks evaluation, we used a specific test dataset for each task. This test set was created by selecting 10 \(\%\) of available labeled data that remained unseen by the algorithm. For AE-drug relationship classification as well as NER, we used the ADE-corpus-v2 dataset. For seriousness classification, the test set was carved out of the manually labelled dataset mentioned in subsection. Training was systematically performed with a learning rate of 3E-5, using the Adam optimizer and a batch size equal to 16. The pre-trained language model used in the evaluation are detailed in Table 2 .
Evaluation metrics
We choose to first evaluate the performance separately at task level and select the best performing algorithm according to results displayed in Table 3 . Meanwhile, a good performance of each independent algorithm does not necessary imply a good performance of the whole pipeline. This could especially be the case if the decision process that narrows down the scope of relevant sentences with successive filters becomes too restrictive. In addition to that, the performance of each independent algorithm is calculated at the meaning units level and not at the document level, which is a more representative metric for the intended use-case of LiSA. Nonetheless, performance evaluation at document level is difficult since it requires to find a sample corpus of relevant publications in the literature. That sample should have the same ratio of relevant and irrelevant documents available in PubMed. However it is almost impossible to estimate that ratio unless going through hundreds of articles for every single drug.
Instead, we propose two methods to measure the global performance of the pipeline. First, we calculate the precision and recall at sentence level only, with a sample dataset extracted from PubMed. Second, we propose to evaluate LiSA with a simple keyword search-based method to perform safety monitoring literature review.
Dataset-based performance evaluation
Sentence level evaluation of LiSA
To assess the performance at sentence level, we chose to use the classic performance metric for binary classification: precision, recall and f1-score. To calculate those metrics, we retrieved all documents associated with a list of drugs, as described in “ The LiSA pipeline description ” section. The list of selected drugs was selected to demonstrate how LiSA performs with new preparations, named with labcodes, and with established tradenames and comprise compounds for which certain signals were known to the experts in order to check whether they had been found accordingly (the list is available in “Appendix”). All documents were then fed into the LiSA pipeline to detect all positives sentences (hits) and their parent articles. The volume of documents and meaning units after every successive filter is available in Fig. 5 .
In the absence of a benchmark dataset to evaluate the performance of serious ADR detection, we created the SADR dataset with the help of medical reviewers with the following procedure. We first collected all documents freely available on Pubmed that contains a drug in the list available in “Appendix ”, and only kept the sentences that explicitly contains one of the drugs (since its absence would inevitably make the sentence irrelevant). These sentences were passed to the pipeline to get a prediction regarding the presence of a serious ADR. Then we asked medical experts to review the sentences and check whether the prediction was correct or not. In total, 1231 sentences from 988 unique documents were analyzed, among which 275 are abstracts only and 713 also provide main text. Tables and figures were not analyzed, as well as references. In that sample, LiSA reached a performance of 88.6 \(\%\) in recall, 81.1 \(\%\) in precision and 84,7 \(\%\) in F1-score. We observed better results on abstracts sentences with 89.7 \(\%\) in recall, 81.4 \(\%\) in precision and 85.3 \(\%\) in F1-score than on documents other parts (TITLE, INTRO, METHODS, RESULTS, DISCUSS, CASE, CONCL). More details is provided in Table 4 .
The achieved performance makes LiSA a state of the art system in terms of safety signal detection for the use-case considered in as much as it is closed to the performance obtained in [ 10 ]. Meanwhile, the task evaluated in this paper differs from the case of LiSA. Especially, there is no mention of a drug-AE relationship classification task. In addition there is no code available neither benchmark dataset from [ 10 ] that could have been used for direct comparison. For benchmark purpose, we provide the test dataset used to assess LiSA’s performance at sentence level, in supplementary materials.
The performance is higher for recall than for precision. This was designed on purpose, since there is a stronger need to not miss safety signals publication than achieving a higher precision. This optimization towards recall was especially enabled by the additional post processing modules described previously.
As far as the total number of collected documents and meaning units is concerned, as displayed in Fig. 5 , LiSA is able to perform a very imbalanced prediction task with a high precision. Indeed with more than 53k documents and 3.8 millions meaning units to filter, there are only \(0.2\%\) of meaning units that should be considered as relevant, for about \(10\%\) of all collected documents.
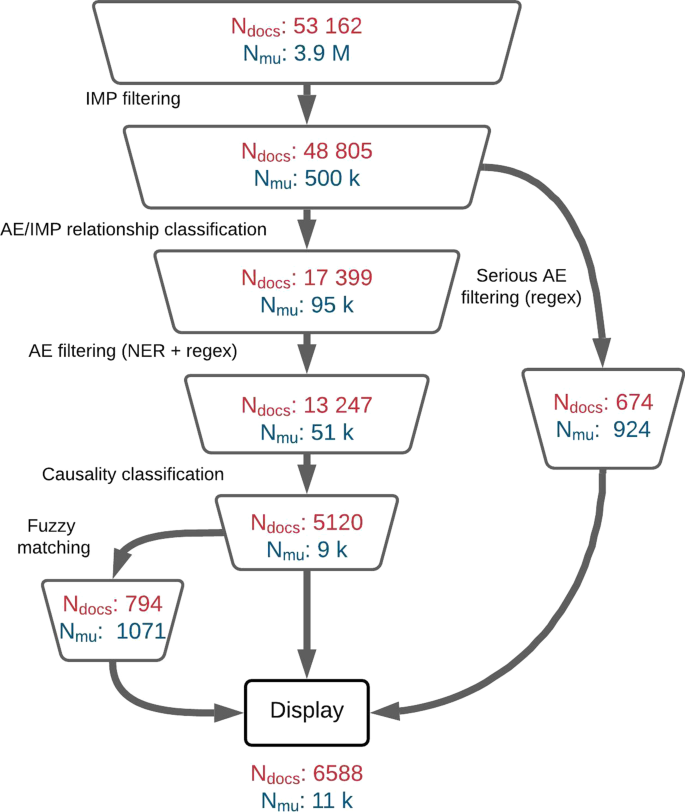
Volume of documents ( \(N_{docs}\) ) and meaning units ( \(N_{mu}\) ) after all decision steps in the LiSA pipeline. The volume corresponds to the documents collected with the drug list available in “Appendix”
Document level evaluation of LiSA
As mentioned before, evaluating the performance at document level is quite challenging. We can calculate the precision using the benchmark dataset available in “Appendix”. Over the 988 documents contained in the benchmark dataset, we found a precision of \(78.5\%\) .
Meanwhile, we are not in capacity to provide a good estimation of LiSA document recall. For that purpose, we should be able to measure to which extent the system is able to avoid missing relevant articles in the literature, which would require to label a corpus of at least a few thousand documents (which corresponds to about 80 000 sentences in total). This is an extremely time consuming task and is not immune to potential bias during the document selection phase to build the sample corpus.
User-based performance evaluation
To further assess the ability of LiSA to perform an efficient and comprehensive literature review on safety issues, we compare the results obtained by an expert medical reviewer using LiSA and using a simple keyword based search on PubMed. This type of evaluation is common in other systems for assisted literature [ 32 ].
For that purpose, we selected one drug, chosen for its relatively low number of associated papers found in the literature, making an exhaustive safety survey difficult. The goal is to compare the number of relevant articles that a manual search would yield to a LiSA-assisted search. On the one hand, a medical reviewer was asked to perform keyword search on PubMed with the expression “drug” + “serious adverse events” to review as much papers as possible within 2 h and retrieve the relevant papers and sentence hits only relatively to the presence of a serious adverse event. Some examples of queries used for this work are “sildenafil adverse events”, “emtricitacine serious adverse effects”. On the other hand, a second medical reviewer was asked to do the same literature review based on LiSA interface, within the same time frame. We also performed the same work for a drug notoriously known for its serious adverse drug effects: Azetolizumab. Due to the large number of papers mentioning serious ADRs in the literature (a few hundreds), the comparative performance between LiSA and a manual search is not significant. Time frame was limited because LiSA aims at speeding up drug monitoring process. Providing unlimited time to medical reviewer is not realistic regarding their daily work. In addition, the two reviews were performed by a different reviewers in order to ensure that the results of the second review will not be influenced by the first one if the same reviewer was doing both of them. Inter-rater Reliability between reviewers was measured on other molecules and was superior to 95
For a survey based on the drugs “Emtricitabine” and “Aflibercept”, the results achieved were as follows:
Emtricitabine:
7 articles were found with the keyword-based search
18 articles were found with the LiSA-assisted search.
Aflibercept:
8 articles were found with the keyword-based search
17 articles were found with the LiSA-assisted search.
The use of LiSA therefore makes it possible to largely increase the volume of relevant papers found during a defined search time (by a factor 2.5), especially when serious ADRs mentions are rare in the literature.
Comparison with state of the art models
The comparative analysis of pre-trained language models has shown different behaviors depending on the task:
for AE-Drug relationship, no major differences were observed between the 7 selected models. This is most probably linked to the nature itself of the task which consists in detecting an association/causality relationship. This will not depends on specific biomedical vocabulary but rather on grammatical forms used to link a drug to an adverse event. This is probably why non-biomedical models like BERT and sciBERT also obtained good results. UMLSBERT provided the best baseline in terms of F1-score and was then selected.
For Named Entity Recognition, the ability of a model to properly identify entities highly depends on the vocabulary learned by the model. On Table 3 , the F1-score levels largely hide subtle differences in performance for specific biomedical sub-domain. Especially, we observed that UMLSBERT and PubMedBERT performed better on text related to oncology where there is a subtle difference between Adverse Events and drug effects related to drug mechanisms (that could be destructive). The specific pre-training of these algorithms might explain their superiority over other models used in the benchmark. We choose PubMedBERT as the best performing model.
For seriousness classification, the vocabulary mastered by the model also highly matters. Indeed, many serious adverse events expressed with technical terms are by essence considered as serious (Stevens Johnson Syndrom, Rhabdomyolysis, Agranulocytosis...) and are better captured with specialized models like PubMedBERT, BioBERT and UMLSBERT. PubMedBERT was selected in this case.
The lack of extensive work on seriousness detection of Adverse Drug Reactions in the literature makes the comparison difficult to perform. In addition to that, the only publication [ 10 ] that tackles the problem does not provide any code implementation. Thus, apart from re-implementing the solution, there is no possibility to compare our algorithm with the one of this publication. Meanwhile, on a corpus extracted from Medical Literature, our pipeline reached a higher performance up to 0.81 in precision and 0.88 in recall (respectively compared to 0.83 and 0.82 [ 10 ]. Even if the dataset are not strictly comparable, we can conclude that our pipeline reached a state of the art performance on the specific task of seriousness classification.
Besides, the calculated overall performance of the pipeline at document level relies on a reduced number of documents (988). The statistical significance of the conclusion might be arguable since we cannot cover all the variety of semantic fields available in PubMed. Meanwhile, we believe that the global performance remains valid, especially since it is added to the already good performance achieved at sentence level, and calculated over a larger volume of examples.
Pipeline flexibility and portability
One important objective of the study was to build a system with a flexible architecture to enable the use of the pipeline on related use cases. For example, we could replace the seriousness classification by seriousness categorization (Death, Hospitalization, IME, Disability, Congenital anomaly [ 10 ]) or adverse events grade classification (Grade 1 to 5). This adjustment would of course require to train a new algorithm (for seriousness categories or adverse events grades classification) but with no impact of the 2 other modules. This is made possible by the independence of the three algorithms, them not being chained. They can then perform inference on the same type of input (a sentence containing at least one monitored drug). This approach is likely to introduce an overlap between the 3 NLP tasks that could be criticized, but allows a full flexibility in the combination of their outputs to build the required decision process.
limitations of the proposed system
A first type of limitation of our systel is related to relation extraction. Indeed, the proposed pipeline does not predict a direct relationship between an adverse event and a drug as defined, for example, in relation extraction tasks in NLP. As a matter of fact, the AE-drug relationship classifier is only trained to categorize meaning units into 2 categories “states a relationship” or “does not state a relationship”. Therefore, if two AEs and two drugs are coexisting in the same meaning unit, the pipeline is not able to separate and identify the possible multiple AE-drug relationships. Meanwhile, due to the relatively reduced length of meaning units (25 tokens on average and max 80–100 tokens) this situation remains very rare and has low impact on the performance.
Another limitation is related to the very assessment of the recall. Indeed, one of the main difficulty in assessing the performance of such systems lies in evaluating the proportion of documents existing in the literature, that are actually missed by the system. As mentioned during the results presentation, this would require the extraction of a test sample with the same distribution of relevant documents available in the literature. Unfortunately, except with a comprehensive work consisting of reviewing hundreds of articles and a strict control of bias during article selection, it is very difficult to get a correct and unbiased estimation of the recall. Instead, we chose to evaluate the recall only within relevant documents at sentence level.
In this paper, we presented the LiSA approach, a deep learning based pipeline for Adverse Drug Reaction monitoring in the biomedical literature. To our knowledge, our work is the first one to rely on a modular architecture of open-source fine-tuned models and providing access to multilevel outputs (AE/Drug relationship, AE and Drug entities, ADR Seriousness monitoring). We evaluated the performance of the system at two levels a) predictive performance based on a benchmark dataset labeled by medical reviewer and made available for future research and b) user-based performance where ADR monitoring with LiSA is compared with a semi-manual work based on keyword search on PubMed search engine. We have shown that based on LiSA user interface, a medical reviewer is able to retrieve 2.5 times more relevant documents than with a simple semi-manual search. Assisted literature monitoring with deep learning has proved to be a viable an extremely efficient approach to address the current challenges in pharmacovigilance. Future research could move toward assessing relationships across the boundaries of single units of meaning, attempting to combine the benefits of the deep learning described here with traditional language models, which would expand the application areas of the pipeline described here for other pharmacovigilance tasks.
Data Availability
The data that support the findings of this study are available from the excel file that our research group created as a supplementary material.
This number was chosen by experts as a good compromise between timeliness of information and the certainty of not missing a relevant signal that might have been reported some time ago.
Abbreviations
Natural language processing
Named entity recognition
Adverse drug reaction
Adverse reaction
Severe adverse drug reaction
Adverse effect
Adverse drug effect
Serious adverse effect
van de Schoot R, de Bruin J, Schram R, Zahedi P, de Boer J, Weijdema F, Kramer B, Huijts M, Hoogerwerf M, Ferdinands G, Harkema A, Willemsen J, Ma Y, Fang Q, Hindriks S, Tummers L, Oberski DL. An open source machine learning framework for efficient and transparent systematic reviews. Nature Machine Intelligence 2021;3(2):125–133. https://doi.org/10.1038/s42256-020-00287-7 . Bandiera_abtest: a Cc_license_type: cc_by Cg_type: Nature Research Journals Number: 2 Primary_atype: Research Publisher: Nature Publishing Group Subject_term: Computational biology and bioinformatics;Computer science;Medical research;SARS-CoV-2 Subject_term_id: computational-biology-and-bioinformatics;computer-science;medical-research;sars-cov-2
Piccinni C, Poluzzi E, Orsini M, Bergamaschi S. PV-OWL – Pharmacovigilance surveillance through semantic web-based platform for continuous and integrated monitoring of drug-related adverse effects in open data sources and social media. 2017 IEEE 3rd International Forum on Research and Technologies for Society and Industry (RTSI) (2017). https://doi.org/10.1109/RTSI.2017.8065931 .
Brisebois R, Abran A, Nadembega A, N’techobo P. An Assisted Literature Review using Machine Learning Models to Recommend a Relevant Reference Papers List, 2017;24.
Alimova I, Tutubalina E. Detecting Adverse Drug Reactions from Biomedical Texts with Neural Networks. In: Proceedings of the 57th Annual Meeting of the Association for Computational Linguistics: Student Research Workshop, 2019;415–421. Association for Computational Linguistics, Florence, Italy. https://doi.org/10.18653/v1/P19-2058 . https://www.aclweb.org/anthology/P19-2058
Fan B, Fan W, Smith C, Garner H. Adverse drug event detection and extraction from open data: a deep learning approach. Inf Process Manage. 2020;57(1): 102131. https://doi.org/10.1016/j.ipm.2019.102131 .
Article Google Scholar
Eberts M, Ulges A. Span-based Joint Entity and Relation Extraction with Transformer Pre-training. arXiv:1909.07755 [cs] 2021. https://doi.org/10.3233/FAIA200321
Ding P, Zhou X, Zhang X, Wang J, Lei Z. An attentive neural sequence labeling model for adverse drug reactions mentions extraction. IEEE Access. 2018;PP:1–1. https://doi.org/10.1109/ACCESS.2018.2882443 .
Zhang S, Dev S, Voyles J, Rao AS. Attention-Based Multi-Task Learning in Pharmacovigilance. In: 2018 IEEE International Conference on Bioinformatics and Biomedicine (BIBM), 2018, pp. 2324–22328. https://doi.org/10.1109/BIBM.2018.8621286 .
Yuwen L, Chen S, Zhang H. Detecting Potential Serious Adverse Drug Reactions Using Sequential Pattern Mining Method. In: 2018 IEEE 9th International Conference on Software Engineering and Service Science (ICSESS), 2018, pp. 56–59. https://doi.org/10.1109/ICSESS.2018.8663856
Routray R, Tetarenko N, Abu-Assal C, Mockute R, Assuncao B, Chen H, Bao S, Danysz K, Desai S, Cicirello S, Willis V, Alford SH, Krishnamurthy V, Mingle E. Application of augmented intelligence for pharmacovigilance case seriousness determination. Drug Saf. 2020;43(1):57–66. https://doi.org/10.1007/s40264-019-00869-4 .
Article CAS Google Scholar
FDA: FAERS (FDA Adverse Event Reporting System). [Online; Accessed 11 Dec 2021]. https://open.fda.gov/data/faers/ .
FDA: MedWatch: The FDA Safety Information and Adverse Event Reporting Program. [Online; Accessed 11 Dec 2021]. https://www.fda.gov/safety/medwatch-fda-safety-information-and-adverse-event-reporting-program .
Dunne C, Shneiderman B, Gove R, Klavans J, Dorr B. Rapid understanding of scientific paper collections: integrating statistics, text analysis, and visualization (2011).
Yeleswarapu S, Rao A, Joseph T, Saipradeep VG, Srinivasan R. A pipeline to extract drug-adverse event pairs from multiple data sources. BMC Med Inform Decis Mak. 2014;14:13. https://doi.org/10.1186/1472-6947-14-13 .
Gattepaille LM. Using the WHO database of spontaneous reports to build joint vector representations of drugs and adverse drug reactions, a promising avenue for pharmacovigilance. In: 2019 IEEE International Conference on Healthcare Informatics (ICHI), 2019:1–6. https://doi.org/10.1109/ICHI.2019.8904551 .
Sampathkumar H, Chen X-W, Luo B. Mining Adverse Drug Reactions from online healthcare forums using Hidden Markov Model. BMC Med Inform Decis Mak. 2014;14(1):91. https://doi.org/10.1186/1472-6947-14-91 .
Harpaz R, Vilar S, Dumouchel W, Salmasian H, Haerian K, Shah NH, Chase HS, Friedman C. Combing signals from spontaneous reports and electronic health records for detection of adverse drug reactions. JAMIA. 2013;20(3):413–9. https://doi.org/10.1136/amiajnl-2012-000930 .
Gurulingappa H, Rajput AM, Roberts A, Fluck J, Hofmann-Apitius M, Toldo L. Development of a benchmark corpus to support the automatic extraction of drug-related adverse effects from medical case reports. J Biomed Inform. 2012;45(5):885–92. https://doi.org/10.1016/j.jbi.2012.04.008 .
Gurulingappa H, Mateen-Rajput A, Toldo L. Extraction of potential adverse drug events from medical case reports. J Biomed Semant. 2012;3:15. https://doi.org/10.1186/2041-1480-3-15 .
ChEMBL: ChEMBL Is a Manually curated database of bioactive molecules with drug-like properties. [Online; Accessed 11 Dec 2021]. https://www.ebi.ac.uk/chembl/ .
MedDRA: Medical Dictionary for Regulatory Activities. [Online; Accessed 11 Dec 2021]. https://www.meddra.org/ .
Pappagari R, Żelasko P, Villalba J, Carmiel Y, Dehak N. Hierarchical Transformers for Long Document Classification. arXiv:1910.10781 [cs, stat] 2019. arXiv: 1910.10781
Aho AV, Corasick MJ. Efficient string matching: an aid to bibliographic search. Commun ACM. 1975;18(6):333–40. https://doi.org/10.1145/360825.360855 .
EMA: ICH E2A Clinical Safety Data Management: Definitions and Standards for Expedited Reporting. [Online; Accessed 11 Dec 2021]. https://www.fda.gov/safety/reporting-serious-problems-fda/what-serious-adverse-event .
Yuan Z, Zhao Z, Sun H, Li J, Wang F, Yu S. Coder: Knowledge-infused cross-lingual medical term embedding for term normalization. J Biomed Inf. 2022. https://doi.org/10.1016/j.jbi.2021.103983 .
Lee J, Yoon W, Kim S, Kim D, Kim S, So CH, Kang J. BioBERT: a pre-trained biomedical language representation model for biomedical text mining. Bioinformatics. 2019;36(4):1234–40. https://doi.org/10.1093/bioinformatics/btz682 . arxiv:1901.08746 .
Peng Y, Yan S, Lu Z. Transfer learning in biomedical natural language processing: An evaluation of bert and elmo on ten benchmarking datasets. In: Proceedings of the 2019 Workshop on Biomedical Natural Language Processing (BioNLP 2019), (2019), pp. 58–65.
Beltagy I, Lo K, Cohan A. Scibert: A pretrained language model for scientific text. In: EMNLP. Association for Computational Linguistics? (2019). https://www.aclweb.org/anthology/D19-1371 .
Alsentzer E, Murphy JR, Boag W, Weng W, Jin D, Naumann T, McDermott MBA. Publicly available clinical BERT embeddings. CoRR abs/1904.03323 2019. arxiv:1904.03323 .
Devlin J, Chang M, Lee K, Toutanova K. BERT: pre-training of deep bidirectional transformers for language understanding. CoRR abs/1810.04805 (2018). arxiv:1810.04805 .
Gu Y, Tinn R, Cheng H, Lucas M, Usuyama N, Liu X, Naumann T, Gao J, Poon H. Domain-Specific Language Model Pretraining for Biomedical Natural Language Processing 2020. arxiv:2007.15779 .
Marshall IJ, Kuiper J, Wallace BC. RobotReviewer: evaluation of a system for automatically assessing bias in clinical trials. J Am Med Inform Assoc. 2016;23(1):193–201.
Download references
Acknowledgements
We would like to thank Mayra Latorre Martinez (Swissmedic) for her valuable contribution throughout the project.
The results presented here were obtained within a project funded by Swissmedic; however, this study was conducted independently without any Swissmedic funding.
Author information
Authors and affiliations.
Quinten, 8 rue Vernier, 75017, Paris, France
Vincent Martenot, Valentin Masdeu, Jean Cupe, Faustine Gehin, Margot Blanchon & Julien Dauriat
Swiss Agency for Therapeutic Products, Swissmedic, Hallerstrasse 7, 3012, Bern, Switzerland
Alexander Horst, Michael Renaudin & Philippe Girard
UMMISCO, Sorbonne University, IRD, Bondy, France
Jean-Daniel Zucker
You can also search for this author in PubMed Google Scholar
Contributions
Conception and design: All authors, Collection and assembly of data: Vi. M., J. D., M. B., Data analysis and interpretation: Vi. M., Va. M., J. C., M. B., A. H., and J. D., Manuscript writing: Vi. M., Va. M., J. C., F. G. and J-D. Z., Manuscript correcting: all authors, Final approval of manuscript: All authors. All authors read and approved the final manuscript.
Corresponding authors
Correspondence to Vincent Martenot or Jean-Daniel Zucker .
Ethics declarations
Ethics approval and consent to participate.
Not applicable.
Consent for publication
Competing interests.
The authors declare that they have no competing interests.
Additional information
Publisher's note.
Springer Nature remains neutral with regard to jurisdictional claims in published maps and institutional affiliations.
Rights and permissions
Open Access This article is licensed under a Creative Commons Attribution 4.0 International License, which permits use, sharing, adaptation, distribution and reproduction in any medium or format, as long as you give appropriate credit to the original author(s) and the source, provide a link to the Creative Commons licence, and indicate if changes were made. The images or other third party material in this article are included in the article's Creative Commons licence, unless indicated otherwise in a credit line to the material. If material is not included in the article's Creative Commons licence and your intended use is not permitted by statutory regulation or exceeds the permitted use, you will need to obtain permission directly from the copyright holder. To view a copy of this licence, visit http://creativecommons.org/licenses/by/4.0/ . The Creative Commons Public Domain Dedication waiver ( http://creativecommons.org/publicdomain/zero/1.0/ ) applies to the data made available in this article, unless otherwise stated in a credit line to the data.
Reprints and permissions
About this article
Cite this article.
Martenot, V., Masdeu, V., Cupe, J. et al. LiSA: an assisted literature search pipeline for detecting serious adverse drug events with deep learning. BMC Med Inform Decis Mak 22 , 338 (2022). https://doi.org/10.1186/s12911-022-02085-0
Download citation
Received : 28 June 2022
Accepted : 13 December 2022
Published : 22 December 2022
DOI : https://doi.org/10.1186/s12911-022-02085-0
Share this article
Anyone you share the following link with will be able to read this content:
Sorry, a shareable link is not currently available for this article.
Provided by the Springer Nature SharedIt content-sharing initiative
- Adverse drug events
- Assisted literature review
- Deep Learning
BMC Medical Informatics and Decision Making
ISSN: 1472-6947
- General enquiries: [email protected]
- All services
- +34 91 277 10 76

- November 06, 2023
- How to perform a good Pharmacovigilance literature search strategy
The search of scientific literature in Pharmacovigilance (PV) is an activity of great importance within pharmacovigilance, as it allows the collection of valuable information on the safety and efficacy of drugs, in addition to complying with the applicable legal requirements (in Spain it includes Royal Decree 577/2013 and the European directive 2010/84/EU ) and good pharmacovigilance practices ( Module VI GVP “Guideline on good pharmacovigilance practices (GVP) Module VI- Collection, management and submission of reports of suspected adverse reactions to medicinal products ).
The first action to be taken when defining the PV search strategy is the selection of sources, which will determine which materials will be used, depending on the type of search to be performed, such as publications in local journals in each region or country, and global databases and other international publications, in the case of international searches. The differentiation between these 2 types of searches is due to the need to address drug safety from multiple perspectives and to adapt to the type of monitoring and, as we have seen above, to comply with local and global regulations.
Another important consideration is to have an updated list of the products and active ingredients of the project on which the pharmacovigilance search will be performed, creating procedures that allow the inclusion of new entries, as well as the elimination of drugs that become obsolete for bibliographic searches. Both the list of products and active ingredients, as well as the list of journals chosen for monitoring, should be reviewed annually to maintain an updated list and to ensure that the searches are relevant. In the case of journals, it is important to bear in mind that the publications should be appropriate to the objective and therapeutic area.

For pharmacovigilance searches in databases, it will be necessary to define a strategy that takes into account the creation of periodic alerts, day zero and algorithms that allow retrieving the most appropriate and relevant information. For the construction of an algorithm, it will be necessary to take into account that it must be built in a robust way, giving the expected and consistent results, i.e., reproducible and easy to update. These parameters not only guarantee the quality of the service, but also provide a fully auditable activity.
Finally, in order to ensure the quality of the searches, and again to allow traceability and quality, a “Quality Control” should be included as part of the strategy to check both the relevance and suitability of the results and the formal aspects of their recording. This is where the training of technicians, both in pharmacovigilance and in the project, becomes essential to guarantee the best service.
In short, in order to obtain valuable information on drug safety in the search for scientific literature, it is first necessary to establish an adapted strategy for PV searches that defines the needs and methods to be used to retrieve the information, and to guarantee the quality of the service through internal control systems and adequate staff training.
From Azierta part of QbD Group, we are experts in Medical Information and in Pharmacovigilance. We can help you with our solutions. Do not hesitate to contact us!
Share this post with your friends.


The search of scientific literature in Pharmacovigilance (PV) ...

- Animal Health
- Audit & Certification
- Clinical Trials
- Food Supplements
- GxP Compliance & Safety
- Landing in Europe
- Medical Devices
- medical information
- Pharmacovigilance
- Regulatory Affairs
- Sin categoría
Latest posts
- Bibliometric analysis in scientific publications
- First publications of electronic medicines information (ePI)
- Life Sciences Management Program at IE Business School and Azierta part of QbD Group
- Current and Future Perspectives on Environmental Impact Assessment for Medicines: Regulation, Implementation and Pharmaceutical Sustainability
- Submit RFI/RFP
Literature Monitoring in Pharmacovigilance
Incorporating data from medical and scientific literature is a paramount aspect of patient safety. This wealth of knowledge often constitutes a substantial part of the safety profile of medicinal products. From providing insights on potential side effects to shedding light on different patient experiences, this literature contributes significantly to how we understand and navigate the safety of medical products.
Regulatory bodies hold medicinal products’ marketing authorization holders (MAHs) to a high standard. One key aspect of their responsibility is performing regular scientific literature searches, including unpublished manuscripts and abstracts presented at medical or scientific conferences.
To underscore the importance of literature monitoring in pharmacovigilance, consider that properly conducted searches can lead to important discoveries, timely interventions, and overall improved product safety. On the other hand, inadequate searches can result in missed opportunities, overlooked risks, and in some cases, significant patient harm.
Types of Literature Searches
Literature searches generally fall into two categories. First, exhaustive searches cast a wide net across various databases and resources, capturing a broad spectrum of available literature on a given subject. Second, selective searches focus on specific databases or key publications, often targeting high-impact literature.
Exhaustive Searches
Exhaustive searches, or systematic reviews, aim to include all available evidence on a specific research question. This involves extensive searching of several databases, websites, and sometimes even manual searching of journals and books to ensure no relevant study is overlooked.
The exhaustive search is labor-intensive and requires a clear understanding of the topic and complex search strategies. However, the end result is a comprehensive overview of the literature, including studies of all levels of quality and size. This approach is often used when performing meta-analyses, where the results of several studies are combined to provide a more definitive answer to a research question.
Selective Searches
On the other hand, selective searches are more focused and usually target specific databases or key publications. Instead of casting a wide net, selective searches aim at catching ‘big fish’, which refers to high-impact literature that has the potential to change practices or policies.
This method requires knowledge about the topic and the ability to discern the importance of different pieces of literature. The targeted databases or journals often have high impact factors, meaning their published works are frequently cited in other research. While less comprehensive than exhaustive searches, selective searches are quicker and more feasible in certain circumstances, especially when time is limited.
The choice between the two depends on the research question, the project’s scope, and the available resources.
Global vs. Local Literature Searches
When conducting research, you might come across the terms ‘global literature search’ and ‘local literature search.’ Understanding the differences between these two methods can significantly influence the depth and breadth of the findings of a research project. Let’s dive into the characteristics that distinguish global and local literature searches.
Global Literature Searches
Global literature searches are broad and comprehensive. They seek to capture the full scope of existing research on a topic, regardless of the geographical origin of the data or the research institution. Global searches often employ multiple databases and resources, not limited to any specific country or region.
A global literature search intends to gather information from diverse sources, giving researchers an extensive understanding of the subject matter. This approach offers several benefits, including a broad perspective on the topic, a wide range of data, and the inclusion of different cultural, geographical, and socioeconomic contexts. However, it may require more time and resources due to the vast amount of information that needs to be reviewed and analyzed.
Local Literature Searches
Local literature searches, on the other hand, are more focused and region-specific. They center on information from a particular area, country, or group of countries. Researchers may use region-specific databases, local libraries, government reports, and documents from local institutions in a local literature search.
This search type is beneficial when the research question pertains to a particular region or population. Considering local conditions, policies, and cultural practices can provide more context-specific information. However, a local literature search may offer a more limited perspective and overlook globally relevant findings.
In conclusion, choosing between a global or local literature search depends on your research objectives. Both have merits and can be used effectively to gather relevant information. Sometimes, combining both may be the best approach to ensure a comprehensive literature review.
Literature Search Strategies
One does not simply dive into literature searches; it requires a strategy. An effective literature search strategy is one that is constructed to ensure the optimum retrieval of the most relevant articles. This includes identifying the right keywords, knowing the databases to target, and being able to discern the value and relevance of different pieces of literature.
Embarking on a literature search is a bit like setting off on a treasure hunt. In this case, the treasure is the relevant, high-quality literature on your subject of interest. And, like any good treasure hunt, success lies in having a solid strategy. Here’s a closer look at the components of an effective literature search strategy.
Identifying the Right Keywords
Your search for literature starts with the right keywords. Keywords are the terms that best represent the core concepts of your topic. Brainstorming a comprehensive list of keywords covering various aspects of your subject is essential. Using a combination of broad and narrow terms can be beneficial. Moreover, consider synonyms, alternative spellings, acronyms, and both your keywords’ singular and plural forms.
Using Boolean Operators
Boolean operators—AND, OR, and NOT—are the glue that holds your search together. They allow you to combine or exclude keywords in a way that focuses your search. For example, using AND narrows your search (e.g., “diabetes AND children”), using OR broadens it (e.g., “children OR adolescents”), and using NOT helps eliminate irrelevant results (e.g., “diabetes NOT type 2”).
Knowing the Databases to Target
Different databases host different types of literature. For example, PubMed is excellent for biomedical literature, while IEEE Xplore is better suited for engineering and technology literature. Knowing the appropriate databases to target for your subject matter can significantly increase the relevancy of your search results.
Understanding Search Filters
Most databases provide options to filter your search results. Filters can be applied based on the publication date, language, study type, and more. Utilizing these filters can help streamline your search results, making finding the most relevant literature easier.
Appraising the Value and Relevance of Literature
Finally, having retrieved a list of literature, it’s important to appraise each article for its value and relevance to your research question. Look at the credibility of the authors, the methodology used, and whether the article’s conclusions are supported by the data presented.
In summary, a well-constructed literature search strategy is like a roadmap guiding you to the most relevant and valuable literature for your research. Developing can take time and patience, but the rewards are well worth the effort.
Implications of Inadequate Literature Searches
The importance of thorough literature searches becomes clear when we consider the implications of inadequate ones. Inadequate searches can lead to a lack of crucial information, causing potential risks to patient safety, regulatory repercussions, and even damaging the reputation of the MAH.
When to Start Literature Searches
The ideal time to start literature searches is early in product development. This allows for the early identification and management of potential risks. However, these searches must continue post-launch to stay abreast of the latest research and findings related to the product.
Regulatory Requirements for Literature Monitoring In Pharmacovigilance
In the realm of patient safety and medicinal products, regulatory requirements play a vital role. MAHs are expected to abide by specific protocols, including regularly and thoroughly searching the scientific literature. Compliance with these requirements is not only necessary for maintaining their licensing but also for ensuring patient safety and the overall integrity of the medical product.
Guidance from FDA and EMA on Literature Monitoring In Pharmacovigilance
Both the FDA (Food and Drug Administration) in the United States and the EMA (European Medicines Agency) provide guidance on literature monitoring in the realm of pharmacovigilance.
The FDA’s guidance on the subject can be found in various documents provided by the agency. These documents provide comprehensive information on how to conduct effective and thorough literature searches. A crucial resource is the FDA’s Guidance for Industry, which provides detailed instructions and recommendations on good pharmacovigilance practices, including literature monitoring.
FDA Guidance for Industry
Meanwhile, the EMA also provides extensive guidance on literature monitoring. Their Good Pharmacovigilance Practices (GVP) guidelines, particularly Module VI on the management and reporting of adverse reactions to medicinal products, outlines the responsibilities of MAHs in regards to conducting literature searches. This module addresses the scope and frequency of literature searches, the criteria for selecting data sources, and how to report findings.
EMA Good Pharmacovigilance Practices (GVP)
These guidelines provided by regulatory bodies are not just recommendations; they form a part of the regulatory requirements for MAHs. Therefore, understanding and adhering to them is crucial in ensuring compliance and maintaining the safety profile of medicinal products.
Always remember to review these documents regularly, as the regulatory landscape evolves, and guidelines can be updated. This helps to ensure that your literature monitoring activities align with the most recent regulatory requirements and best practices.
Effective and comprehensive literature searches form a cornerstone of patient safety data. They allow MAHs and regulatory bodies to gather crucial information about medicinal products, offering valuable insights that contribute to their safety profiles. The importance of conducting these searches early and regularly, and the potential consequences of inadequate searches, underscore the need for rigorous and strategic literature review processes.
Start A Conversation
Are you considering pharmacovigilance outsourcing for your organization? Navigating the pharmacovigilance can be daunting, but you don’t have to do it alone. Our team of experienced professionals is ready to provide the support and expertise necessary to ensure your pharmacovigilance operations are efficient, compliant, and effective.
Don’t hesitate – reach out today for a personalized pharmacovigilance consultation. We look forward to partnering with you to enhance patient safety and streamline your operations.
Literature monitoring of Pharmacovigilance in realities of war in Ukraine
Feb 6, 2024 | Pharmacovigilance
It is fascinating to observe how the realities of war have affected the way in which the literature monitoring of Pharmacovigilance is carried out in Ukraine. The need for continuous monitoring of drug safety in a country at war, and the ability to adapt to the...
Pharmacovigilance & CSV: System Upgrades
Oct 9, 2023 | Pharmacovigilance
Explore the intersection of Pharmacovigilance & CSV during system upgrades. Ensure drug safety while optimizing computer system validation.
CSV & Risk Management in Pharmacovigilance
Sep 1, 2023 | Pharmacovigilance
CSV & Risk Management Pharmacovigilance stands as a guardian in drug development and monitoring, ensuring that medicines remain safe and effective throughout their lifecycle. But behind this sentinel lies a complex web of systems, processes, and regulations, each...
Big Enough To Cover All Your Needs. Small Enough To Care.
Clinical research, regulatory affairs, pharmacovigilance, medical information, clinical trials, pv system & qppv, post-authorization, we value your privacy., privacy overview.
Pharmacovigilance
9. Literature reports
“Scientific & medical literature is a significant source of information for the monitoring of the safety profile and of the risk benefit balance of medicinal products, particularly in relation to the detection of new safety signals or emerging safety issues.”
Literature report is any adverse drug reactions reported in
1. Published abstracts or
2. Articles in medical/scientific journals
3. Unpublished manuscripts involving case reports
4. Important safety findings or clinical studies including posters, letters to the editors, and associated communication from scientific meetings.
Current regulatory guidance:
- Per the European Medicines Agency (EMA), MAHs are required to monitor local scientific and medical publications in countries where they have a marketing authorisation, irrespective of commercial status of products.
- The US Food and Drug Administration (FDA) requires submission of reports of serious, unexpected adverse drug reactions (ADRs) described in the scientific literature for products with the same active moiety as products marketed in the US, even though excipient, dosage forms, strengths, routes of administration and indications may vary.
- The “literature” section of the periodic benefit– risk evaluation report (PBRER) requires a summary of new and significant safety findings for approved products, obtained from published peer-reviewed scientific literature or unpublished manuscripts during the reporting interval.
- EMA guidelines also require inclusion of relevant applicable safety information for other active substances of the same class as the marketed drug. Consequently, any potentially relevant event identified in the literature may be considered an emerging safety issue requiring prompt immediate analysis and, if needed, corrective and preventive action.
- Marketing authorisation holders are therefore expected to maintain awareness of possible publications through a systematic literature review of widely used reference databases (e.g. Medline, Excerpta Medica or Embase, Eudravigilance) no less frequently than once a week.
Summary of MLM guidelines:
- MAHs should perform a systematic literature review of widely used reference databases no less frequently than once a week, unless the active substances of their products are present in the list of publications monitored by the European Medicines Agency (EMA) pursuant to Article 27 of Regulation (EC) No 726/2004.
- However, the MAHs need to continue to monitor all other medical literature not covered by the literature reference databases applied for the service by the EMA.
- The MLM services of EMA started on September 1st, 2015. The full monitoring list contains more than 400 active substance groups. The EMA is responsible for monitoring selected medical literature and for entering identified reports of suspected adverse reactions in EudraVigilance.
- The clock for reporting starts (Day 0) with awareness of a publication containing the minimum information for reporting.
- All the suspect adverse reactions found by the EMA in the listed medical literature, both serious (EU and non-EU) and non-serious (EU only), are not transmitted to MAHs, indeed they are transmitted to EudraVigilance and National Competent Authorities (NCAs) and made available to MAHs via EudraVigilance.
- The MAHs, however, should download and include these ICSRs in their safety database. They can provide an assessment of the case, describe a disagreement with, and/or alternatives to the diagnoses given by the primary source and/or indicate the degree of suspected relatedness of each medicinal product to the adverse reactions.
- Where the MAH identifies a literature case entered by EMA to be a duplicate of the company’s individual case, which was previously submitted to EudraVigilance, there is a need to send a follow-up with the world-wide unique case identifier to EudraVigilance.
- In addition to the activities above, MAHs should also monitor the scientific and medical publications in local journals in countries where medicinal products have a MA.
- Reports of suspected adverse reactions from the scientific and medical literature, including relevant published abstracts from meetings and draft manuscripts, should be reviewed and assessed by MAHs to identify and record possible ICSRs.
How the companies search relevant cases in database:
- It is important that, in addition to searching for adverse events/reactions, the search is constructed to retrieve any special situation reports (e.g. pregnancy and breastfeeding, overdose, abuse, misuse, medication errors, occupational exposure) and, if relevant, use in specific patient populations (e.g. paediatrics).
- The search strategy should also be able to retrieve reports of off-label and compassionate use.
- As a general rule, searches should be performed using the active substance.
- For combination products, all active substances need to be included in the search strategy.
- Searches should not be routinely conducted that exclude unbranded products.
- It is common for authors of literature articles to refer to a generic medicinal product and the MAH should assume ownership of the product if it can not, with absolute certainty, confirm that the product is not its own.
- In addition, where the formulation is not specified, ownership of the product should be assumed.
- For some regulators, reports should not be excluded based on the formulation.
- Articles referring to a class of drugs that describe a class effect, whilst not appropriate for ICSRs may be relevant for inclusion in periodic reports.
Safety information: Below safety information is collected from literature reports.
- New, unexpected serious and non-serious ICSR reports with a reasonable causal association with the product.
- Pregnancy outcomes (including termination) with no adverse outcomes
- Use in paediatric populations
- Compassionate supply, named patient use
- Lack of efficacy
- Asymptomatic overdose, abuse or misuse
- Medication error where no adverse events occurred
- Important non-clinical safety results
Processing of Confirmed ICSRs
1. For literature reports of confirmed cases which can generate ICSRs, a full text of the citation is obtained and, if not in English, translated into English though it is not specified how/who does this or how long this takes).
2. The article is reviewed and the number of valid ICSRs is determined and seriousness/non-seriousness is noted.
3. An ICSR is then created along with a case narrative for serious cases. No narrative is prepared for non-serious ICSRs.
4. Causality assessment and relatedness also performed.
A regulatory reporting form with relevant medical information should be provided for each identifiable patient. The regulatory reporting time clock starts as soon as the MAH has knowledge that the case meets minimum criteria for reportability.
Examples of new events identified through literature review:
Nifedipine and Fatal Aplastic Anemia (1998): Article described a case- control study linking six cases of fatal aplastic anemia with nifedipine Report identified a Type B ADR (bizarre or idiosyncratic, dose independent and unpredictable reaction) Reference: Laporte JR, Ibanez L, Ballarin E, Perez E, Vidal X. Fatal Aplastic anemia associated with nifedipine. Lancet. 1998;352: 619-20
Tamsulosin and ‘Floppy Iris Syndrome” (2005): 15 cases were described in the literature in April, 2005 At the time of publication, none had been reported to the Regulatory Authorities! Reference: Chang DF, Campbell JR,. Intraoperative floppy iris syndrome associated with tamsulosin. J Cataract Refract Surg. 2005;3: 664-73
4 responses to “9. Literature reports”
👍🏼👍🏼 Good information
Indetailed information about literature cases. Thans for your fabulous work.
Great information Author. Can you also provide information on how MLM is actually conducted and where companies can go wrong?
Great Information. It is very informative for all PV profession specifically freshers self understandable. Great efforts. Thank you
Leave a Reply Cancel reply

Pharmacovigilance Literature Screening
– recognized also as medical literature monitoring (mlm), surveillance or search –
Now, fully automated for global and local literature searches with Tepsivo Literature >

Why tepsivo?
Tepsivo Platform with its literature monitoring software module manages global and local literature review around all world the world from one place. Major benefit is in removing all unnecessary admin overhead heavily pushing down total costs, all in line with our value-based philosophy.
Reach out to us to learn more >
✓ automated reporting, ✓ full audit trail of actions, ✓ no admin overhead, ✓ lowest cost possible, ✓ 100% legally compliant.
For simplicity, we follow the usual split between global and literature screening. Global literature monitoring meaning the weekly search in databases such as PubMed or Embase and Local literature screenin g to mean the searches in non-indexed journals published locally around the world.
Global Literature Screening
Global literature screening is one of the key pharmacovigilance obligations you have. Depending on what your portfolio is, it can either be one of the easiest tasks or one of the most time-consuming ones. Either way, Tepsivo literature monitoring service can fully cover your regulatory duties.
Do you have one or a handful of products on the market?
First things first. Let us check if your substance isn’t already monitored by EMA’s Medical Literature Monitoring service. If yes, then there is no reason to duplicate the effort. To its credit, EMA has gone in the right direction by centralizing the screening in one place for many substances.
If your substance isn’t monitored centrally by EMA, you will need someone to screen the databases weekly and track any relevant results. That’s what we can easily do. Thanks to our automated Tepsivo Platform, it’s very easy for us to track and report any outputs of the screening, further dropping down the total price.
Do you have a large portfolio of products?
If you represent a generic company with a large portfolio, the global literature screening may in fact become a colossal cost for you. Here is where you need to automate.
We’ve spent a lot of time going through the best possible options how to optimize literature monitoring and decrease the associated time effort and cost. Reach out to us to discuss your situation and we’ll prepare the most fitting solution for you. Most likely a combination of an AI-powered search tool and our inhouse Tepsivo Platform, automating both the screening time as well as the reporting side of the process.
Let’s talk!
Are you all caught up with your reading all over the world?
With Tepsivo global PV network covering over 150 countries , you can be assured to comply with the literature monitoring requirements from EMA and regulatory authorities around the world. Based on your drug and commercial status in your respective markets, we will prepare your literature screening strategy for each country individually.
Our literature screeners will use their PV and relevant language knowledge to define the right keywords and, especially, to select the most relevant journals. To ensure you don’t miss any potentially relevant information, the journal selection is crucial for a good literature monitoring strategy, and it’s best done by specialists with thorough knowledge of the medical environment in their relevant countries.
What makes us special?
Our pragmatism and belief in efficiency in contrast to greed.
It is our view that local literature screening in non-indexed journals is an outdated requirement, and it shouldn’t really exist in today’s world , at least not in the form it is required now. That is, if we believe that pharmacovigilance process effectiveness should be a measurement of quality.
If you’re present globally, local literature screening will constitute a large part of your PV budget. We’ve been making arguments to the EMA to remove this requirement but to no avail. It’s here to stay and local literature screening will remain expensive for pharmaceutical companies.
So if EMA and legislators are not taking action, what can we do?

We can automate the process and heavily bring down your total costs.

Our unique Tepsivo Literature searches through thousands of sources based on keywords and sends auto-translated abstracts to one central place that is Tepsivo Platform. All with 0 people trapped doing mundane activities.
Plus, thanks to Tepsivo Platform , we removed the traditionally heavy work in reporting/tracking/reconciling that usually adds so much extra time effort to an already time intense activity. Especially in global operations.
For traditional pharmacovigilance providers and CROs, local literature monitoring is a financially lucrative activity. But we’re not greedy, we don’t believe that it belongs, in its current form, to the field and it certainly doesn’t meet the criteria of what we see as value-based healthcare. That is what we developed Tepsivo Literature, saving time and money on meaningless tasks
Want to learn more?
List of countries
Sub-saharan africa, let’s have a chat.
Whatever you needs are, we look forward to getting in touch with you. Feel free to drop us a message and we will contact you right away.
Tepsivo Oy | Urho Kekkosen katu, 4-6 E, 00 100, Helsinki, Finland | VAT number FI31367614 | [email protected] | +358 402 204 698 | Privacy policy
- Privacy Overview
- Strictly Necessary Cookies
This website uses cookies so that we can provide you with the best user experience possible. Cookie information is stored in your browser and performs functions such as recognising you when you return to our website and helping our team to understand which sections of the website you find most interesting and useful.
Strictly Necessary Cookie should be enabled at all times so that we can save your preferences for cookie settings.
If you disable this cookie, we will not be able to save your preferences. This means that every time you visit this website you will need to enable or disable cookies again.
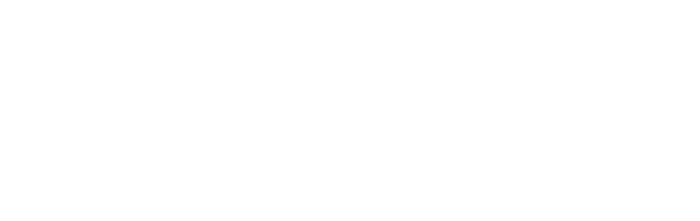
- ICSR Case Processing
Literature Search
- Aggregate Reporting
- Signal Management
- Risk Management
Global and local literature monitoring for adverse event identification across the drug life cycle
Marketing Authorization Holders (MAH) are required to monitor the scientific literature of their products on a periodic basis. Global and local literature search is performed to identify the adverse reactions and for reporting to health authorities.
APCER conducts the literature searches for products under investigational stage (in clinical trial phase), in pre-authorization stages, and post-marketing phase to meet the regulatory requirements of its clients.
Our Literature Search services portfolio includes:
- Global literature screening
- End-to-end literature search and review
- Defining search strategy
- Expertise in handling various literature search databases such as Embase, PubMed, and customer-specified databases
- Medical literature monitoring
- Local literature screening as per local regulations
- Procurement and review of full text articles where applicable
- Translation of literature articles by certified translators
How can we work together for better health?
Explore more.
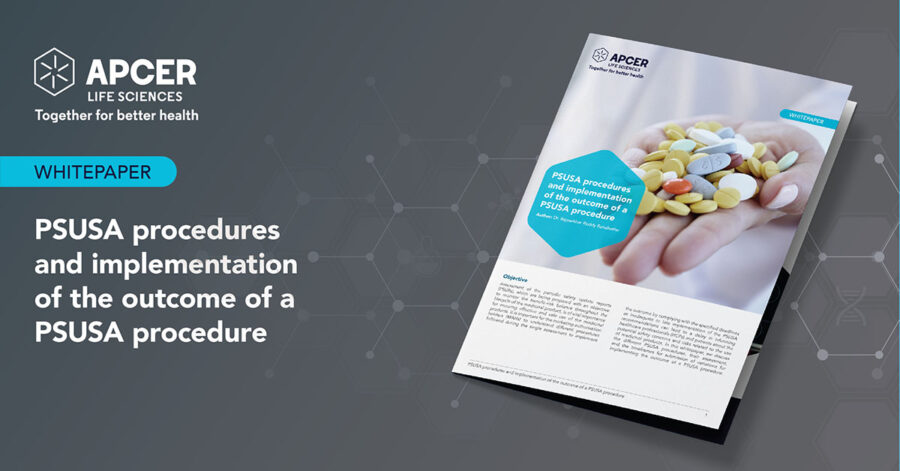
PSUSA procedures and implementation of the outcome of a PSUSA procedure
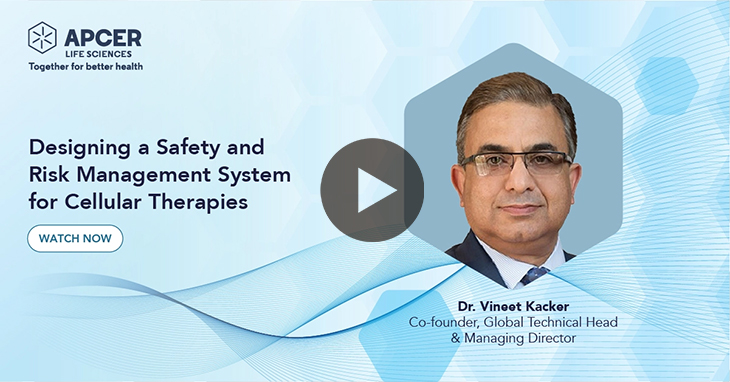
Designing a Safety and Risk Management System for Cellular Therapies
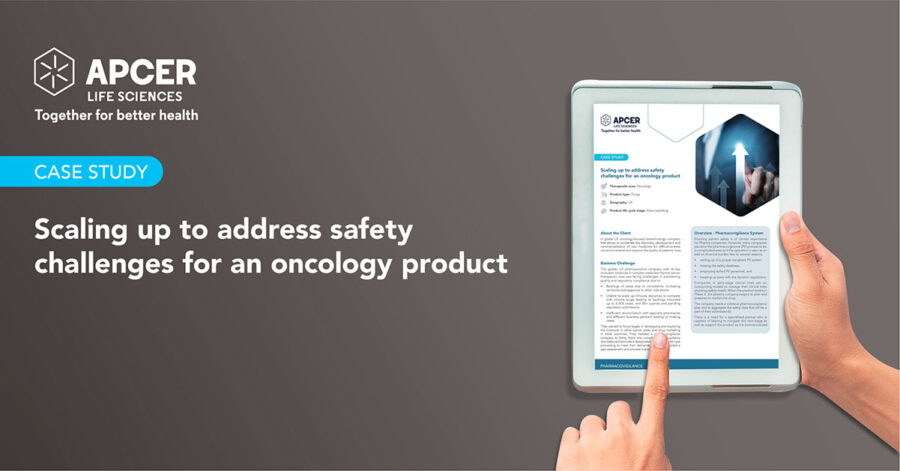
Scaling up to address safety challenges for an Oncology product
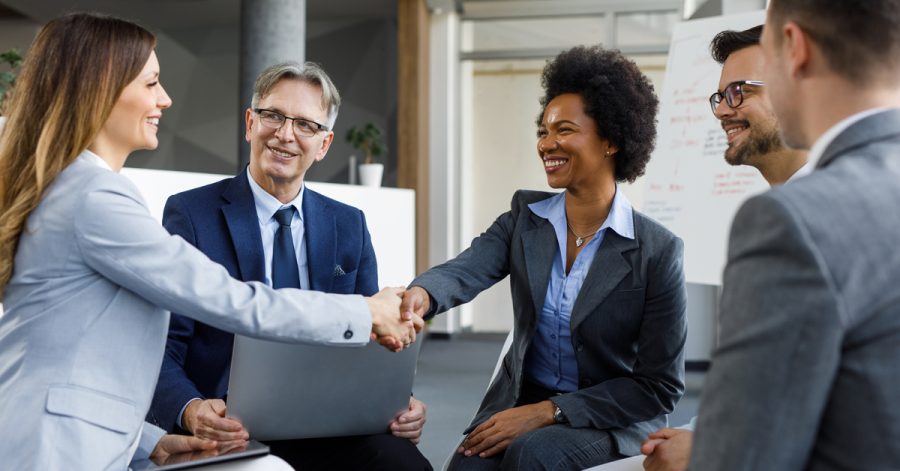
Outsourcing Pharmacovigilance: Benefits of a Specialized Safety Partner
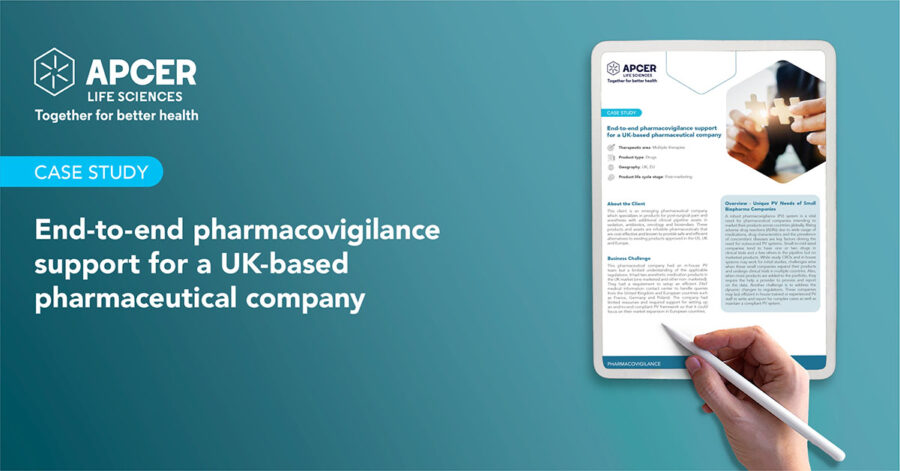
End-to-end pharmacovigilance support for a UK-based pharmaceutical company
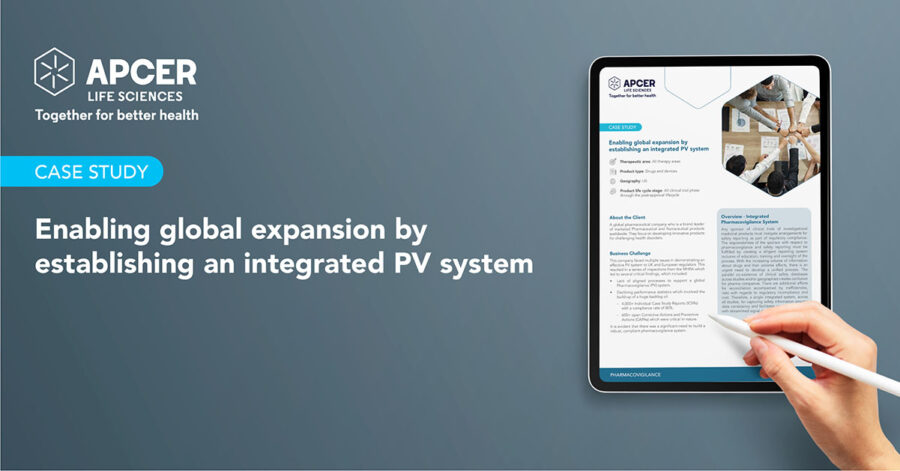
Enabling global expansion by establishing an integrated PV system
Importance of engaging a safety partner early in the drug life cycle.
Upload Document ( Optional ) (Format Supported : PDF, DOC or DOCX | Max File Size : 1 MB)
Please check here to indicate your consent to receive catalogues, service offerings and marketing related emails from APCER Life Sciences.
Please note that by submitting this form, you consent to your personal data being processed in alignment with our Privacy Policy . APCER is committed to ensuring the security and protection of your personal information. Your personal data will be processed for purpose of facilitating your request and may be used for sending you additional marketing and business development-related information about APCER Life Sciences, its affiliates and our services. You can withdraw your consent by writing to us at [email protected] .
- Have any questions?
- (+1) 609 455 1600
- [email protected]
- Conference Coverage
- CRO/Sponsor
- 2023 Salary Survey

- Publications
- Conferences
Pharmacovigilance: Literature Monitoring Best Practices
Safe and effective use of health products is a key objective of pharmacovigilance. Information is provided about the safety of these substances to patients, healthcare providers, and the general public as soon as possible. Pharmacovigilance includes reviewing the development, management, and introduction of pharmaceuticals. It is probably the most tightly regulated part of the pharmaceutical industry. Pharmacovigilance aims to identify, detect, assess, and report any adverse drug reaction (ADR) related to pharmaceutical products. In the United States, the European Union, and other parts of the world, regulatory requirements have emerged that have grown in diversity and nuance. These requirements include systematic monitoring and review of medical literature, including comprehensive screening of medical journals for adverse drug reactions, which remain on the rise. Having a robust pharmacovigilance system is paramount for a manufacturer, and any deficiencies can have an adverse effect on patient safety.
Literature monitoring includes published articles, articles, and reviews in indexed or non-indexed journals, any content posted anywhere online, posters and conference abstracts, etc. Holders of a Marketing Authorization (MA) must monitor global and local literature throughout the duration of that authorization, regardless of the availability of the product on the market 1 . Regulatory reports, clinical trial reports, literature reports, license partner reports, and spontaneous reports all serve as sources of data for deeper analysis of regulatory reporting, signal detection, and aggregate reporting. The individual safety report (ICSR) is valuable for developing risk assessments. It is incumbent upon holders of marketing authorizations to stay up-to-date on potential publications (including ahead of print articles) by reviewing widely used reference databases (e.g., Medline, Embase, Excerpta Medica) every week 2 . Adverse events that meet the criteria for the ICSR are handled per regulatory guidelines on handling and reporting adverse events. When a relevant article has been identified, it will be further screened to determine if it meets the four essential criteria for consideration for Individual case safety report (ICSR) and adverse event reporting: 1) identified source, 2) company product, 3) patient, 4) adverse event 3 . Any analysis regarding the safety profile of a product should be based on scientific and medical publications. Literature searches and monitoring are primarily intended to identify single case reports of adverse effects and to track any changes in benefit-risk profiles associated with the drug, particularly when new safety signals safety concerns arise 4 .
Literature Monitoring: An Overview of Best Practices
When the foundation is compromised, a process can result in a cascade of unintended repercussions. Therefore, an unbiased search is vital for monitoring medical literature accurately and efficiently. The growing volume of data has made it more critical to get the best results without introducing unwanted data. The literature monitoring process is usually characterized by two major challenges, which can be overcome. The first challenge is to come up with the right search strategy, and the second is to deal with duplication. Drug manufacturers must often track hundreds of drugs at once. So how can literature monitoring be accurate and valid?
Optimal Search Strategy Design and Database Selection
Regulatory authorities require marketing authorization holders to conduct medical literature surveillance at least weekly according to the GVP module VI and based on the required frequency as described by the local regulatory authorities, both for globally indexed literature databases and locally (non-indexed) literature journals5. When developing search strategies, it is important to consider ICSRs, aggregate reports, and any potential safety-related information. Therefore, it is essential to develop and progressively improve search strategies to limit the risk of overlooking relevant ADR information. Specifically, to retrieve all relevant records, query terms must be highly recallable and carefully crafted to retrieve maximum publications reporting any safety concerns about the product in question.
The database must be comprehensive and meet minimum standards to ensure that safety-critical signals are not missed. Pharmacovigilance searchers typically utilize at least two databases, usually three or more, because having access to multiple databases increases their recall-finding capabilities, ensuring more coverage.
Implement a search approach that balances the need for accuracy and precision. For example, 1) use several Boolean operators, 2) browse a thesaurus of terms, 3) perform proximity search, and 4) incorporate abbreviations to recall results. Using the most recent thesaurus update will ensure accuracy and compliance 6 .
As part of the local literature review, it is recommended to identify the non-indexed journals published locally and to screen those in either an online or print format depending on their availability. There are a few local regulatory agencies that recommend performing local literature searches in a few databases that are locally approved. The MAH handles any publications identified as containing information in local languages in accordance with the translation process established within the institution.
Industry best practice calls for constant review of search terms and updating them based on safety-related updates pertaining to the products. A GOLD standard data set of records is used to validate the modified search strategies. It is recommended to review your search strategy annually and make amendments as necessary 7 .
EMA hosts a robust system for medical literature monitoring. Thousands of records are added daily. It is generally the responsibility of marketing authorization holders to monitor medical literature and report individual cases of suspected adverse reactions into EudraVigilance and national safety databases 8 . They are not required to monitor or report suspected adverse reactions for active substances to EudraVigilance for substances covered by EMA’s service 9 .
Duplicate Data Management
Scientific publications and medical literature are abundant with sources and references, so it’s likely that the same publication could be indexed in multiple formats across a variety of journals, which results in duplicate findings. This creates a whole series of redundant tasks and false signals regarding drug safety. It leads to erroneous evaluations and, ultimately, compliance problems. Duplicate management processes, however, can solve this issue. Even though this is the best way of dealing with articles, it comes with a few challenges. There may be limitations to duplicate identification within the tool due to the presence of special characters, or it may be the case that the same study has been published across different journals or conference abstracts, making the process cumbersome.
It is important to search multiple databases to capture multiple publications across different journals. Keeping track of previous searches will also facilitate the identification of duplicates. To identify duplicate publications, there should not be just a focus on the article title but also the author’s name and, in some cases, the name of the study cited in the article.
According to Article 107(3) of Directive 2001/83/EC, to avoid duplicate submissions of ICSRs, the holder of a marketing authorization must submit the ICSRs that are not already assessed or monitored by EMA through the Medical Literature Monitoring (MLM) services 10 .
Service providers should use a standardized and well-established deduplication system, enabling them to confirm that they are not missing relevant references or creating duplicates inadvertently.
Along with routine literature surveillance, MAH also conducts targeted literature searches, which are searches specifically designed to answer a specific research question. When conducting signal analysis, these searches are conducted to confirm or disprove the association between the adverse event and the product.
Pharmacovigilance involves a substantial amount of literature monitoring. The process of devising a solid search strategy could be challenging but is essential. A professional with the required skills, experience, and training will ensure adverse event-related safety information is never missed. It is necessary to develop and maintain search strategies, elicit ideas from different stakeholders, and develop approved and suitable strategies for the purpose at hand. It is critical to set up a thorough process to handle and manage duplicate articles. Regularly review search strategies, and ensure the documentation is robust to ensure the finest quality results. The following points can be considered to check whether the MAH’s literature monitoring systems meet quality standards;
- A drug safety expert with experience researching literature is needed.
- Conduct risk assessments to ensure that the search criteria are robust and relevant to the objective of the literature search.
- Conduct literature searches and evaluate the results for literature per regional requirements (Global and Local).
- Monitoring and reviewing the Eudravigilance Medical Literature Monitoring (MLM) system, managed by EMA, to identify ICSRs in the literature if your product is included in the active ingredient screened by EMA.
- The search string is reviewed and updated annually to optimize results.
ClinChoice is a leading global Contract Research Organization (CRO), with over 3400 clinical research professionals across North America, Asia, and Europe. For more than 27 years, ClinChoice has been providing high-quality contract research services to pharmaceutical, biotechnology, medical device, and consumer products clients, encompassing a broad range of services and therapeutic areas. ClinChoice offers cutting-edge, full-service solutions for Clinical Trials, Regulatory Affairs, Medical Device Safety, Toxicology, and Medical Affairs.
- https://www.ema.europa.eu/en/human-regulatory/post-authorisation/pharmacovigilance/medical-literature-monitoring
- https://www.ema.europa.eu/en/documents/scientific-guideline/guideline-good-pharmacovigilance-practices-module-vi-management-reporting-adverse-reactions_en-0.pdf
- https://www.ema.europa.eu/en/documents/scientific-guideline/guideline-good-pharmacovigilance-practices-gvp-module-vi-collection-management-submission-reports_en.pdf
- https://database.ich.org/sites/default/files/E2D_Guideline.pdf
- https://europa.eu/en/documents/scientific-guideline/guideline-good-pharmacovigilance-practices-gvp-module-iv-pharmacovigilance-audits-rev-1_en.pdf
- https://www.ema.europa.eu/en/documents/other/monitoring-medical-literature-entry-relevant-information-eudravigilance-database-european-medicines_en.pdf
- https://www.elsevier.com/solutions/embase-biomedical-research/coverage-and-content
2 Commerce Drive Cranbury, NJ 08512
609-716-7777

Good pharmacovigilance practices (GVP)
Guideline on gvp.
The guideline on GVP was a key deliverable of the 2010 pharmacovigilance legislation .
Each chapter and revisions are developed by a team consisting of experts from EMA and from EU Member States.
The guideline on GVP is divided into chapters that fall into two categories:
- modules covering major pharmacovigilance processes;
- product- or population-specific considerations.
Modules covering major pharmacovigilance processes
GVP modules I to XVI cover major pharmacovigilance processes and the development of this set of guidance is concluded. The module numbers XI, XII, XIII and XIV stay void, as their planned topics have been addressed by other guidance documents on the Agency's website (see bullet points below Final GVP modules table).
Product- or population-specific considerations
The chapters on product- or population-specific considerations are available for vaccines, biological medicinal products and the paediatric population. EMA plans one more consideration chapter as follows:
GVP modules and considerations are regularly reviewed for revision needs and schedules.
Archives of documents
- Archive of development of GVP
- Superseded pharmacovigilance guidance documents
Also on this topic
- Archive of development of good pharmacovigilance practices
Introduction
Guidelines on good pharmacovigilance practices (GVP): Introductory cover note, last updated with release of Addendum III of Module XVI on pregnancy prevention programmes for public consultation
English (EN) (201.43 KB - PDF)
Final GVP modules
Guideline on good pharmacovigilance practices: Module I – Pharmacovigilance systems and their quality systems
English (EN) (213.14 KB - PDF)
Guideline on good pharmacovigilance practices: Module II – Pharmacovigilance system master file (Rev. 2)
English (EN) (340.71 KB - PDF)
Guideline on good pharmacovigilance practices: Module III – Pharmacovigilance inspections
This Module contains guidance on the planning, conduct, reporting and follow-up of pharmacovigilance inspections in the EU and outlines the role of the different parties involved. General guidance is provided under III.B., while III.C. covers the overall operation of pharmacovigilance inspections in the EU.
English (EN) (189.47 KB - PDF)
Guideline on good pharmacovigilance practices (GVP) - Module IV – Pharmacovigilance audits (Rev. 1)
English (EN) (154.02 KB - PDF)
Guideline on good pharmacovigilance practices: Module V – Risk management systems (Rev. 2)
English (EN) (568.8 KB - PDF)
Guideline on good pharmacovigilance practices (GVP) - Module VI – Collection, management and submission of reports of suspected adverse reactions to medicinal products (Rev. 2)
GVP Module VI – Management and reporting of adverse reactions to
medicinal products (EMA/873138/2011 Rev. 2)
English (EN) (2.02 MB - PDF)
Guideline on good pharmacovigilance practices (GVP) - Module VI Addendum I – Duplicate management of suspected adverse reaction reports
Module VI Addendum I – Duplicate management of suspected adverse reaction reports
English (EN) (359.92 KB - PDF)
Guideline on good pharmacovigilance practices (GVP): Module VII – Periodic safety update report
English (EN) (1.45 MB - PDF)
Guideline on good pharmacovigilance practices (GVP) - Module VIII – Post-authorisation safety studies (Rev. 3)
English (EN) (235.42 KB - PDF)
Guideline on good pharmacovigilance practices (GVP): Module VIII Addendum I - Requirements and recommendations for the submission of information on non-interventional post-authorisation safety studies (Rev. 3)
English (EN) (86.49 KB - PDF)
Guideline on good pharmacovigilance practices (GVP): Module IX – Signal management (Rev. 1)
Revision 1 is a major revision with modifications throughout and contains the following:
- Revised definition and process for emerging safety issues, previously addressed in GVP Module VI (IX.A.1.1. and IX.C.2.);
- Streamlined information on scientific aspects of signal management (IX.B.2. to IX.B.4.), statistical aspects now addressed in Addendum I;
English (EN) (283.21 KB - PDF)
Guideline on good pharmacovigilance practices (GVP): Module IX Addendum I – Methodological aspects of signal detection from spontaneous reports of suspected adverse reactions
This guidance extends and updates some of the information given in the Guideline on the Use of Statistical Signal Detection Methods in the EudraVigilance Data Analysis System (EMEA/106464/2006 rev. 1) and supersedes the previous advice in the areas addressed by this new guidance.
English (EN) (133.95 KB - PDF)
Guideline on good pharmacovigilance practices: Module X – Additional monitoring
English (EN) (271.91 KB - PDF)
Guideline on good pharmacovigilance practices: Module XV – Safety communication (Rev. 1)
English (EN) (188.52 KB - PDF)
Guideline on good pharmacovigilance practices: Module XVI – Risk minimisation measures: selection of tools and effectiveness indicators (Rev. 2)
English (EN) (375.58 KB - PDF)
Guideline on good pharmacovigilance practices (GVP): Module XVI Addendum I – Educational materials
Module XVI Addendum I – Educational materials
English (EN) (81.66 KB - PDF)
- Where GVP chapters refer to Modules XI or XIV, consult the Agency's page on partners and networks .
- Where GVP chapters refer to Module XII, consult the Agency's page on post-marketing authorisation: regulatory and procedural guidance for human medicinal products.
- Where GVP chapters refer to Module XIII, consult the Agency's page on the incident management plan .

- Where GVP modules refer to the European Medicines Agency's and the Heads of Medicines Agencies' procedural advice on referral procedures for safety reasons, consult referral procedures page.
Final GVP product- or population-specific considerations
Guideline on good pharmacovigilance practices (GVP): Product- or population-specific considerations I: Vaccines for prophylaxis against infectious diseases
English (EN) (460.19 KB - PDF)
Guideline on good pharmacovigilance practices (GVP): Product- or population-specific considerations II: Biological medicinal products
English (EN) (377.05 KB - PDF)
Guideline on good pharmacovigilance practices (GVP): Product- or Population-Specific Considerations IV: Paediatric population
English (EN) (206.02 KB - PDF)
Final GVP annex I - Definitions
Guideline on good pharmacovigilance practices: Annex I - Definitions (Rev. 4)
English (EN) (295.69 KB - PDF)
Final GVP annex II - Templates
Guideline on good pharmacovigilance practices: Annex II – Templates: Cover page of periodic safety update report (PSUR)
English (EN) (176.08 KB - PDF)
Guideline on good pharmacovigilance practices: Annex II – Templates: Direct Healthcare Professional Communication (DHPC) (Rev. 1)
English (EN) (61.21 KB - PDF)
Guideline on good pharmacovigilance practices (GVP): Annex II – Templates: Communication Plan for Direct Healthcare Professional Communication (CP DHPC)
English (EN) (80.24 KB - PDF)
For other templates developed outside the GVP process, see:
- Risk-management plans
- Pharmacovigilance: regulatory and procedural guidance
Final GVP annex III - Other pharmacovigilance guidance
These pharmacovigilance guidance documents were developed under the previous legal framework but are still valid in conjunction with GVP.
Guideline on the exposure to medicinal products during pregnancy: Need for post-authorisation data
English (EN) (277.32 KB - PDF)
Note for guidance: EudraVigilance Human – Processing of safety messages and individual case safety reports (ICSRs) (historical)
English (EN) (840.18 KB - PDF)
Overview of comments received on draft note for guidance: EudraVigilance version 7.1 - Processing of safety messages and individual case safety reports (ICSRs)
English (EN) (215.05 KB - PDF)
Draft note for guidance: EudraVigilance Human version 7.1 - Processing of safety messages and individual case safety reports (ICSRs)
English (EN) (720.45 KB - PDF)
For other pharmacovigilance guidance developed outside the GVP process, see:
- Consideration on core requirements for RMPs of COVID-19 vaccines
- Signal management
- EudraVigilance
- European Medicines Agency policy on access to EudraVigilance data for medicinal products for human use - Revision 3
- Union reference dates and submission of periodic safety update reports
- Guideline on registry-based studies
- Standards and guidances of the European Network of Centres for Pharmacoepidemiology and Pharmacovigilance (ENCePP)
Guideline on specific adverse reaction follow-up questionnaires (Specific AR FUQ)
- Providing general guidance when to request, when and how to use Specific AR FUQs to Marketing authorisation holders (MAHs) and competent authorities in Member States (NCAs); Pharmacovigilance risk assessment committee (PRAC), pharmacovigilance and clinical assessors, pharmacovigilance (GVP) inspectors, and the European Medicines Agency (EMA)
- Developing guidance that can be used by MAHs and recommend if and how approved Specific AR FUQs could be published
- Considerations on discontinuation and removal of Specific AR FUQs
English (EN) (295.46 KB - PDF)
Final GVP annex IV - International Conference on Harmonisation of Technical Requirements for Registration of Pharmaceuticals for Human Use (ICH) guidelines for pharmacovigilance
International Conference on Harmonisation of Technical Requirements for Registration of Pharmaceuticals for Human Use topic E 2 A: Clinical safety data management: Definitions and standards for expedited reporting - Step...
English (EN) (177.91 KB - PDF)
International Conference on Harmonisation of Technical Requirements for Registration of Pharmaceuticals for Human Use guideline E2B (R3): Electronic transmission of individual case safety reports (ICSRs) - data elements ...
English (EN) (1.49 MB - PDF)
International Conference on Harmonisation of Technical Requirements for Registration of Pharmaceuticals for Human Use topic E 2 B (R3): Questions and answers: Data elements for transmission of individual case safety repo...
English (EN) (480.36 KB - PDF)
International Conference on Harmonisation of Technical Requirements for Registration of Pharmaceuticals for Human Use guideline E2C (R2) on periodic benefit-risk evaluation report - Step 5
The Periodic Benefit-Risk Evaluation Report (PBRER) described in this guideline is intended to be a common standard for periodic benefit-risk evaluation reporting on marketed products (including approved drugs that are under further study) among the ICH regions.
This guideline defines the recommended format and content of a PBRER and provides an outline of points to be considered in its preparation and submission.
English (EN) (476.27 KB - PDF)
International Conference on Harmonisation of Technical Requirements for Registration of Pharmaceuticals for Human Use topic E 2 D: Postapproval safety data management - Step 5
English (EN) (181.92 KB - PDF)
International Conference on Harmonisation of Technical Requirements for Registration of Pharmaceuticals for Human Use topic E 2 E: Pharmacovigilance planning - Step 5
English (EN) (225.43 KB - PDF)
ICH guideline E2F on development safety update report - Step 5
The DSUR proposed in this guideline is intended to be a common standard for periodic reporting on drugs under development among the ICH regions. US and EU regulators consider that the DSUR, submitted annually, would meet national and regional requirements currently met by the US IND Annual Report and the EU Annual Safety Report, respectively, and can therefore take the place of these existing reports. This guideline defines the recommended content and format of a DSUR and provides an outline of points to be considered in its preparation and submission.
English (EN) (352.87 KB - PDF)
- E2C(R2)Implementation working group ICH E2C(R2) Guideline: Periodic benefit-risk evaluation report questions & answers
- ICH M1 Medical Dictionary for Regulatory Activites (MedDRA)
- MedDRA support documentation
- ICH M2 electronic standards for the transfer of regulatory information (ESTRI) is available on multidisciplinary guidelines .
Final GVP annex V - Abbreviations
Guideline on good pharmacovigilance practices: Annex V – Abbreviations (Rev. 1)
English (EN) (84.58 KB - PDF)
Public consultations
Draft guideline on good pharmacovigilance practices (GVP): Module XVI Addendum III – Pregnancy prevention programme and other pregnancy-specific risk minimisation measures
This new guidance defines the elements of a pregnancy prevention programme and provides for deciding when such programme is needed or other risk minimisation measures are considered appropriate to avoid adverse pregnancy outcomes due to use of medicines and to preserve health of both the mother and the child.
Those participating in the public consultation are asked to submit comments via the EU survey tool linked here .
English (EN) (212.27 KB - PDF)
Draft guideline on good pharmacovigilance practices: Product- or population-specific considerations III: Pregnant and breastfeeding women
This product- and population- specific considerations chapter P.III of the good pharmacovigilance practices (GVP) aims to provide guidance to marketing authorisation applicants/holders, competent authorities of Member States and the Agency for facilitating appropriate pharmacovigilance for medicinal products that may be used in pregnant or breastfeeding women.
Comments should be provided using .
English (EN) (475.52 KB - PDF)
Guideline on good pharmacovigilance practices (GVP): Module XVI - Risk minimisation measures: selection of tools and effectiveness indicators (Rev 3)
Risk management includes the identification, characterisation (including quantification), prevention and minimisation of risks. Risk management systems consist of pharmacovigilance activities and interventions relating to individual medicinal products for this purpose, including the assessment of the effectiveness of those activities and interventions, in accordance with Article 1(28b) of Directive 2001/83/EC. The objectives of risk minimisation are achieved through the implementation of risk minimisation measures (RMM) required by the competent authorities and generation of evidence that these measures are effective.
English (EN) (422.3 KB - PDF)
Guideline on good pharmacovigilance practices (GVP): Module XVI Addendum II - Methods for effectiveness evaluation
This Addendum to GVP Module XVI provides additional guidance for marketing authorisation holders and competent authorities on data sources and methodologies for monitoring outcomes of risk minimisation measures (RMM) in line with the principles for RMM effectiveness evaluation laid down in GVP Module XVI. Depending on the risk minimisation objective, studies evaluating RMM effectiveness may integrate different quantitative measurements and qualitative research approaches to evaluate risk minimisation outcomes for individual tools or sets of RMM described in GVP Module XVI.
English (EN) (335.45 KB - PDF)
- Privacy statement
- Regulatory and procedural guidance
- Pharmacovigilance
Share this page
How useful do you find this page.

IMAGES
VIDEO
COMMENTS
The European Medicines Agency (EMA) is responsible for monitoring a number of substances and selected medical literature, to help identify suspected adverse reactions to medicines authorised in the European Union (EU). EMA also enters relevant information into the EudraVigilance database. Human Regulatory and procedural guidance Pharmacovigilance.
QVigilance's Pharmacovigilance Specialists are experienced with performing literature searches. Working with the customer, we can develop the search strategy and undertake sensitivity testing to ensure that search criteria are robust and appropriate for the purpose of the literature search. We can run the literature search
Pharmacovigilance in the Center for Drug Evaluation and Research (CDER) March 26, 2019 Kim Swank, PharmD. Division of Pharmacovigilance. ... literature search. Identify a safety signal.
The search string is reviewed and updated annually to optimize results. About the Author. Dr. Poonam Wagle; Associate Manager, Pharmacovigilance. Poonam brings expertise in literature management as a pharmacovigilance and safety expert with over eleven years of experience in the areas of ICSR, Literature, Aggregate Reports, and Signal Reviews.
As we discussed above, the goal of literature search and monitoring is to identify individual case safety reports and any possible changes to the benefit-risk profile of the substance being monitored, particularly about detecting new safety signals or emerging safety issues. Marketing authorization holders should perform medical literature ...
Docket Number: FDA-2004-D-0041. Issued by: Center for Drug Evaluation and Research. Center for Biologics Evaluation and Research. Guidance for Industry - Good Pharmacovigilance Practices and ...
Keywords: Pharmacovigilance, software design, user-computer interface, data mining, translational research. Go to: 1. Background and Significance. Literature reports are highly relevant to the detection of drug-adverse event (ADE) safety 'signals' for drugs and biologic products [ 1 ]. The published literature complements the safety ...
Overall, the generic pipeline and the visualization tool proposed in this article allows for efficient and accurate monitoring of serious adverse drug reactions from the literature and can easily be adapted to similar pharmacovigilance use cases. To facilitate reproducibility and benefit other resea …
Detecting safety signals attributed to a drug in scientific literature is a fundamental issue in pharmacovigilance. The constant increase in the volume of publications requires the automation of this tedious task, in order to find and extract relevant articles from the pack. This task is critical, as serious Adverse Drug Reactions (ADRs) still account for a large number of hospital admissions ...
The search of scientific literature in Pharmacovigilance (PV) is an activity of great importance within pharmacovigilance, as it allows the collection of valuable information on the safety and efficacy of drugs, in addition to complying with the applicable legal requirements (in Spain it includes Royal Decree 577/2013 and the European directive 2010/84/EU) and good pharmacovigilance practices ...
Literature Monitoring in Pharmacovigilance. Incorporating data from medical and scientific literature is a paramount aspect of patient safety. This wealth of knowledge often constitutes a substantial part of the safety profile of medicinal products. From providing insights on potential side effects to shedding light on different patient ...
Methods. A systematic literature search was conducted in PubMed, CINAHL, Journals@Ovid and the Cochrane Library. Studies were included if they contained: (i) reviews about patient reporting; (ii) evaluation of patient reports to national or supranational pharmacovigilance authorities; (iii) a comparison between patient and healthcare professional (HCP) reports submitted to pharmacovigilance ...
Pharmacovigilance is a science that involves the ongoing monitoring of adverse drug reactions to existing medicines. Traditional approaches in this field can be expensive and time-consuming. ... In this systematic review, a broad and multi-disciplinary literature search was conducted involving four databases. A total of 5318 publications were ...
How the medical literature plays a crucial role in pharmacovigilance is discussed, to improve systematic reviews of adverse drug reactions and to develop a prototype that first reproduces and standardizes search strategies to have a better information retrieval. Pharmacovigilance is to track and detect new adverse drug reactions mainly due to drugs or due to any other chemical substance or ...
Literature report is any adverse drug reactions reported in. 1. Published abstracts or. 2. Articles in medical/scientific journals. 3. Unpublished manuscripts involving case reports. 4. Important safety findings or clinical studies including posters, letters to the editors, and associated communication from scientific meetings.
As the Good pharmacovigilance practices (GVP) module VI states "medical literature is a significant source of information" for marketing authorization holders and has to be taken into consideration. Marketing authorization holders are expected to perform international and local literature searches to identify pharmacovigilance (PV) cases ...
Global literature screening is one of the key pharmacovigilance obligations you have. Depending on what your portfolio is, it can either be one of the easiest tasks or one of the most time-consuming ones. Either way, Tepsivo literature monitoring service can fully cover your regulatory duties. Do you have one or a handful of products on the market?
Global and local literature search is performed to identify the adverse reactions and for reporting to health authorities. APCER conducts the literature searches for products under investigational stage (in clinical trial phase), in pre-authorization stages, and post-marketing phase to meet the regulatory requirements of its clients.
The search was initiated using the term 'pharmacovigilance' and its synonyms in combination with other groups of keywords that covered 'evaluation'. ... Segura-Bedmar I, Martinez P. Special issue on mining the pharmacovigilance literature. J Biomed Inform. 2014; 49:1-2. doi: 10.1016/j.jbi.2014.04.007.
A Whitepaper on pharmacovigilance literature searches and optmizing search strategies for patient safety +44 (0)1462 440 084 [email protected] Contact Us ... Literature search strategy and how these should be constructed in such a way to ensure the optimum retrieval of the most appropriate articles;
When constructing a literature search strategy, it is important to define the purpose of the search. For example, a literature search designed purely to identify Individual Case Safety Reports (ICSRs) may not be suitable or broad enough to identify any articles that would be required for aggregate reports, such as the Development Safety Update ...
Pharmacovigilance involves a substantial amount of literature monitoring. The process of devising a solid search strategy could be challenging but is essential. A professional with the required skills, experience, and training will ensure adverse event-related safety information is never missed.
HCPs' awareness of pharmacovigilance and ADR reporting varies among countries. Some studies have documented lower awareness regarding the ADR reporting and monitoring system. 5-7 Few studies have revealed a lack of understanding about how, where, and when to report an ADR. 8-10 In contrast, studies have also documented good knowledge of ADR reporting. 1,11 Furthermore, many studies have ...
Good pharmacovigilance practices (GVP) are a set of measures drawn up to facilitate the performance of pharmacovigilance in the European Union (EU). GVP apply to marketing-authorisation holders, the European Medicines Agency (EMA) and medicines regulatory authorities in EU Member States. They cover medicines authorised centrally via the Agency ...