- En español – ExME
- Em português – EME

Case-control and Cohort studies: A brief overview
Posted on 6th December 2017 by Saul Crandon
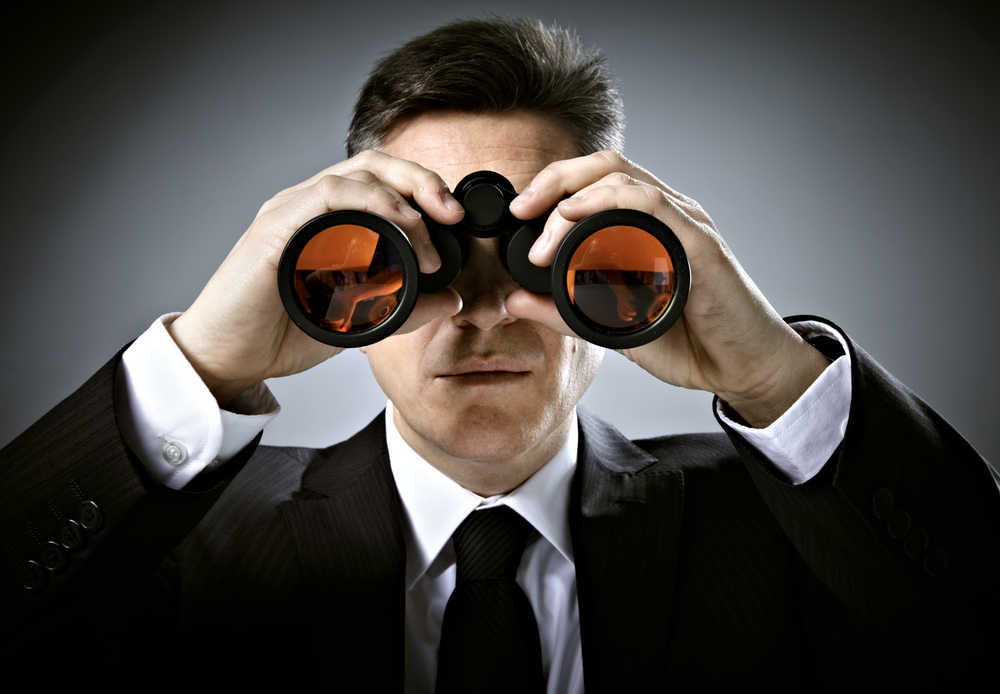
Introduction
Case-control and cohort studies are observational studies that lie near the middle of the hierarchy of evidence . These types of studies, along with randomised controlled trials, constitute analytical studies, whereas case reports and case series define descriptive studies (1). Although these studies are not ranked as highly as randomised controlled trials, they can provide strong evidence if designed appropriately.
Case-control studies
Case-control studies are retrospective. They clearly define two groups at the start: one with the outcome/disease and one without the outcome/disease. They look back to assess whether there is a statistically significant difference in the rates of exposure to a defined risk factor between the groups. See Figure 1 for a pictorial representation of a case-control study design. This can suggest associations between the risk factor and development of the disease in question, although no definitive causality can be drawn. The main outcome measure in case-control studies is odds ratio (OR) .
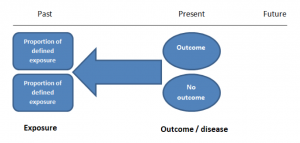
Figure 1. Case-control study design.
Cases should be selected based on objective inclusion and exclusion criteria from a reliable source such as a disease registry. An inherent issue with selecting cases is that a certain proportion of those with the disease would not have a formal diagnosis, may not present for medical care, may be misdiagnosed or may have died before getting a diagnosis. Regardless of how the cases are selected, they should be representative of the broader disease population that you are investigating to ensure generalisability.
Case-control studies should include two groups that are identical EXCEPT for their outcome / disease status.
As such, controls should also be selected carefully. It is possible to match controls to the cases selected on the basis of various factors (e.g. age, sex) to ensure these do not confound the study results. It may even increase statistical power and study precision by choosing up to three or four controls per case (2).
Case-controls can provide fast results and they are cheaper to perform than most other studies. The fact that the analysis is retrospective, allows rare diseases or diseases with long latency periods to be investigated. Furthermore, you can assess multiple exposures to get a better understanding of possible risk factors for the defined outcome / disease.
Nevertheless, as case-controls are retrospective, they are more prone to bias. One of the main examples is recall bias. Often case-control studies require the participants to self-report their exposure to a certain factor. Recall bias is the systematic difference in how the two groups may recall past events e.g. in a study investigating stillbirth, a mother who experienced this may recall the possible contributing factors a lot more vividly than a mother who had a healthy birth.
A summary of the pros and cons of case-control studies are provided in Table 1.
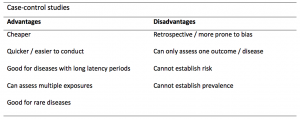
Table 1. Advantages and disadvantages of case-control studies.
Cohort studies
Cohort studies can be retrospective or prospective. Retrospective cohort studies are NOT the same as case-control studies.
In retrospective cohort studies, the exposure and outcomes have already happened. They are usually conducted on data that already exists (from prospective studies) and the exposures are defined before looking at the existing outcome data to see whether exposure to a risk factor is associated with a statistically significant difference in the outcome development rate.
Prospective cohort studies are more common. People are recruited into cohort studies regardless of their exposure or outcome status. This is one of their important strengths. People are often recruited because of their geographical area or occupation, for example, and researchers can then measure and analyse a range of exposures and outcomes.
The study then follows these participants for a defined period to assess the proportion that develop the outcome/disease of interest. See Figure 2 for a pictorial representation of a cohort study design. Therefore, cohort studies are good for assessing prognosis, risk factors and harm. The outcome measure in cohort studies is usually a risk ratio / relative risk (RR).
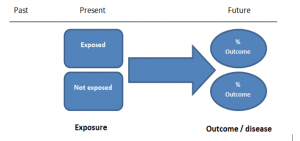
Figure 2. Cohort study design.
Cohort studies should include two groups that are identical EXCEPT for their exposure status.
As a result, both exposed and unexposed groups should be recruited from the same source population. Another important consideration is attrition. If a significant number of participants are not followed up (lost, death, dropped out) then this may impact the validity of the study. Not only does it decrease the study’s power, but there may be attrition bias – a significant difference between the groups of those that did not complete the study.
Cohort studies can assess a range of outcomes allowing an exposure to be rigorously assessed for its impact in developing disease. Additionally, they are good for rare exposures, e.g. contact with a chemical radiation blast.
Whilst cohort studies are useful, they can be expensive and time-consuming, especially if a long follow-up period is chosen or the disease itself is rare or has a long latency.
A summary of the pros and cons of cohort studies are provided in Table 2.
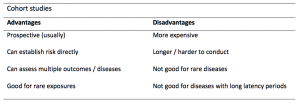
The Strengthening of Reporting of Observational Studies in Epidemiology Statement (STROBE)
STROBE provides a checklist of important steps for conducting these types of studies, as well as acting as best-practice reporting guidelines (3). Both case-control and cohort studies are observational, with varying advantages and disadvantages. However, the most important factor to the quality of evidence these studies provide, is their methodological quality.
- Song, J. and Chung, K. Observational Studies: Cohort and Case-Control Studies . Plastic and Reconstructive Surgery.  2010 Dec;126(6):2234-2242.
- Ury HK. Efficiency of case-control studies with multiple controls per case: Continuous or dichotomous data . Biometrics . 1975 Sep;31(3):643–649.
- von Elm E, Altman DG, Egger M, Pocock SJ, Gøtzsche PC, Vandenbroucke JP; STROBE Initiative. The Strengthening the Reporting of Observational Studies in Epidemiology (STROBE) statement: guidelines for reporting observational studies.  Lancet 2007 Oct;370(9596):1453-14577. PMID: 18064739.
Saul Crandon
Leave a reply cancel reply.
Your email address will not be published. Required fields are marked *
Save my name, email, and website in this browser for the next time I comment.
No Comments on Case-control and Cohort studies: A brief overview
Very well presented, excellent clarifications. Has put me right back into class, literally!
Very clear and informative! Thank you.
very informative article.
Thank you for the easy to understand blog in cohort studies. I want to follow a group of people with and without a disease to see what health outcomes occurs to them in future such as hospitalisations, diagnoses, procedures etc, as I have many health outcomes to consider, my questions is how to make sure these outcomes has not occurred before the “exposure disease”. As, in cohort studies we are looking at incidence (new) cases, so if an outcome have occurred before the exposure, I can leave them out of the analysis. But because I am not looking at a single outcome which can be checked easily and if happened before exposure can be left out. I have EHR data, so all the exposure and outcome have occurred. my aim is to check the rates of different health outcomes between the exposed)dementia) and unexposed(non-dementia) individuals.
Very helpful information
Thanks for making this subject student friendly and easier to understand. A great help.
Thanks a lot. It really helped me to understand the topic. I am taking epidemiology class this winter, and your paper really saved me.
Happy new year.
Wow its amazing n simple way of briefing ,which i was enjoyed to learn this.its very easy n quick to pick ideas .. Thanks n stay connected
Saul you absolute melt! Really good work man
am a student of public health. This information is simple and well presented to the point. Thank you so much.
very helpful information provided here
really thanks for wonderful information because i doing my bachelor degree research by survival model
Quite informative thank you so much for the info please continue posting. An mph student with Africa university Zimbabwe.
Thank you this was so helpful amazing
Apreciated the information provided above.
So clear and perfect. The language is simple and superb.I am recommending this to all budding epidemiology students. Thanks a lot.
Great to hear, thank you AJ!
I have recently completed an investigational study where evidence of phlebitis was determined in a control cohort by data mining from electronic medical records. We then introduced an intervention in an attempt to reduce incidence of phlebitis in a second cohort. Again, results were determined by data mining. This was an expedited study, so there subjects were enrolled in a specific cohort based on date(s) of the drug infused. How do I define this study? Thanks so much.
thanks for the information and knowledge about observational studies. am a masters student in public health/epidemilogy of the faculty of medicines and pharmaceutical sciences , University of Dschang. this information is very explicit and straight to the point
Very much helpful
Subscribe to our newsletter
You will receive our monthly newsletter and free access to Trip Premium.
Related Articles
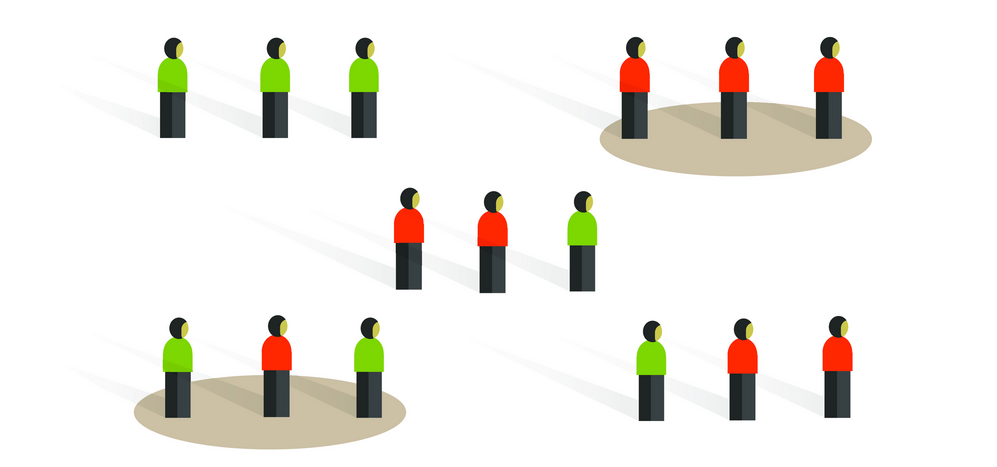
Cluster Randomized Trials: Concepts
This blog summarizes the concepts of cluster randomization, and the logistical and statistical considerations while designing a cluster randomized controlled trial.
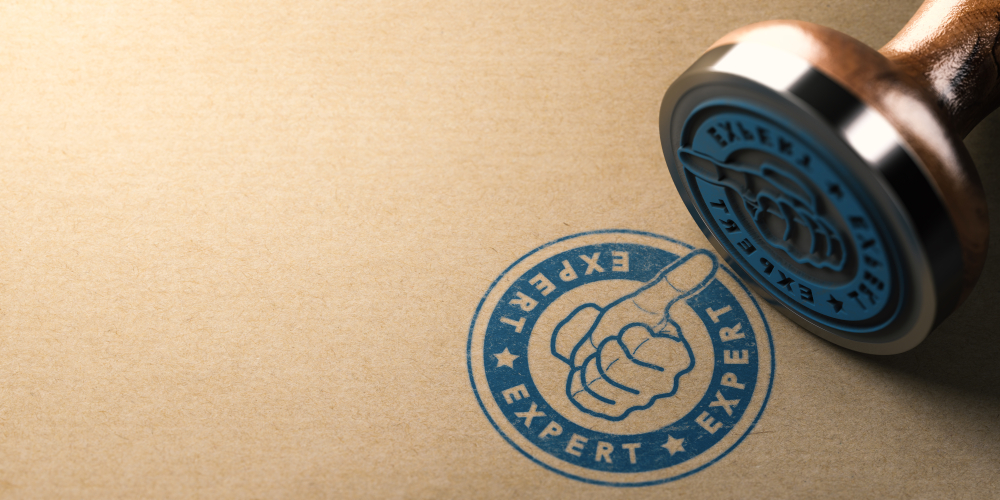
Expertise-based Randomized Controlled Trials
This blog summarizes the concepts of Expertise-based randomized controlled trials with a focus on the advantages and challenges associated with this type of study.
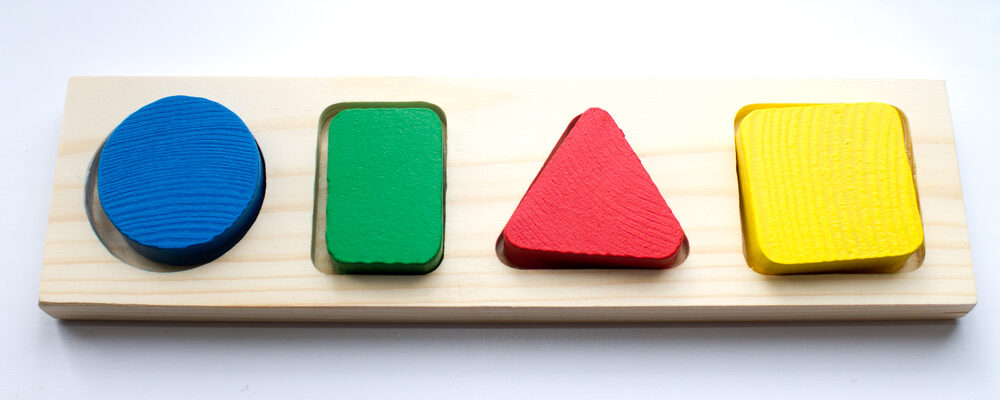
An introduction to different types of study design
Conducting successful research requires choosing the appropriate study design. This article describes the most common types of designs conducted by researchers.
What Is A Case Control Study?
Julia Simkus
Editor at Simply Psychology
BA (Hons) Psychology, Princeton University
Julia Simkus is a graduate of Princeton University with a Bachelor of Arts in Psychology. She is currently studying for a Master's Degree in Counseling for Mental Health and Wellness in September 2023. Julia's research has been published in peer reviewed journals.
Learn about our Editorial Process
Saul Mcleod, PhD
Editor-in-Chief for Simply Psychology
BSc (Hons) Psychology, MRes, PhD, University of Manchester
Saul Mcleod, PhD., is a qualified psychology teacher with over 18 years of experience in further and higher education. He has been published in peer-reviewed journals, including the Journal of Clinical Psychology.
Olivia Guy-Evans, MSc
Associate Editor for Simply Psychology
BSc (Hons) Psychology, MSc Psychology of Education
Olivia Guy-Evans is a writer and associate editor for Simply Psychology. She has previously worked in healthcare and educational sectors.
On This Page:
A case-control study is a research method where two groups of people are compared – those with the condition (cases) and those without (controls). By looking at their past, researchers try to identify what factors might have contributed to the condition in the ‘case’ group.
Explanation
A case-control study looks at people who already have a certain condition (cases) and people who don’t (controls). By comparing these two groups, researchers try to figure out what might have caused the condition. They look into the past to find clues, like habits or experiences, that are different between the two groups.
The “cases” are the individuals with the disease or condition under study, and the “controls” are similar individuals without the disease or condition of interest.
The controls should have similar characteristics (i.e., age, sex, demographic, health status) to the cases to mitigate the effects of confounding variables .
Case-control studies identify any associations between an exposure and an outcome and help researchers form hypotheses about a particular population.
Researchers will first identify the two groups, and then look back in time to investigate which subjects in each group were exposed to the condition.
If the exposure is found more commonly in the cases than the controls, the researcher can hypothesize that the exposure may be linked to the outcome of interest.
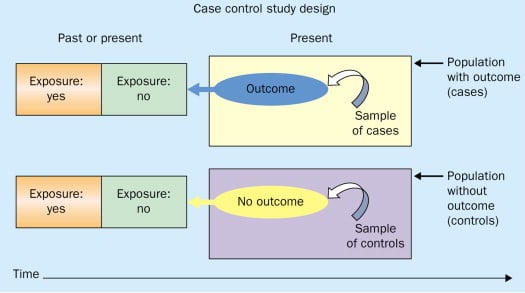
Figure: Schematic diagram of case-control study design. Kenneth F. Schulz and David A. Grimes (2002) Case-control studies: research in reverse . The Lancet Volume 359, Issue 9304, 431 – 434
Quick, inexpensive, and simple
Because these studies use already existing data and do not require any follow-up with subjects, they tend to be quicker and cheaper than other types of research. Case-control studies also do not require large sample sizes.
Beneficial for studying rare diseases
Researchers in case-control studies start with a population of people known to have the target disease instead of following a population and waiting to see who develops it. This enables researchers to identify current cases and enroll a sufficient number of patients with a particular rare disease.
Useful for preliminary research
Case-control studies are beneficial for an initial investigation of a suspected risk factor for a condition. The information obtained from cross-sectional studies then enables researchers to conduct further data analyses to explore any relationships in more depth.
Limitations
Subject to recall bias.
Participants might be unable to remember when they were exposed or omit other details that are important for the study. In addition, those with the outcome are more likely to recall and report exposures more clearly than those without the outcome.
Difficulty finding a suitable control group
It is important that the case group and the control group have almost the same characteristics, such as age, gender, demographics, and health status.
Forming an accurate control group can be challenging, so sometimes researchers enroll multiple control groups to bolster the strength of the case-control study.
Do not demonstrate causation
Case-control studies may prove an association between exposures and outcomes, but they can not demonstrate causation.
A case-control study is an observational study where researchers analyzed two groups of people (cases and controls) to look at factors associated with particular diseases or outcomes.
Below are some examples of case-control studies:
- Investigating the impact of exposure to daylight on the health of office workers (Boubekri et al., 2014).
- Comparing serum vitamin D levels in individuals who experience migraine headaches with their matched controls (Togha et al., 2018).
- Analyzing correlations between parental smoking and childhood asthma (Strachan and Cook, 1998).
- Studying the relationship between elevated concentrations of homocysteine and an increased risk of vascular diseases (Ford et al., 2002).
- Assessing the magnitude of the association between Helicobacter pylori and the incidence of gastric cancer (Helicobacter and Cancer Collaborative Group, 2001).
- Evaluating the association between breast cancer risk and saturated fat intake in postmenopausal women (Howe et al., 1990).
Frequently asked questions
1. what’s the difference between a case-control study and a cross-sectional study.
Case-control studies are different from cross-sectional studies in that case-control studies compare groups retrospectively while cross-sectional studies analyze information about a population at a specific point in time.
In cross-sectional studies , researchers are simply examining a group of participants and depicting what already exists in the population.
2. What’s the difference between a case-control study and a longitudinal study?
Case-control studies compare groups retrospectively, while longitudinal studies can compare groups either retrospectively or prospectively.
In a longitudinal study , researchers monitor a population over an extended period of time, and they can be used to study developmental shifts and understand how certain things change as we age.
In addition, case-control studies look at a single subject or a single case, whereas longitudinal studies can be conducted on a large group of subjects.
3. What’s the difference between a case-control study and a retrospective cohort study?
Case-control studies are retrospective as researchers begin with an outcome and trace backward to investigate exposure; however, they differ from retrospective cohort studies.
In a retrospective cohort study , researchers examine a group before any of the subjects have developed the disease, then examine any factors that differed between the individuals who developed the condition and those who did not.
Thus, the outcome is measured after exposure in retrospective cohort studies, whereas the outcome is measured before the exposure in case-control studies.
Boubekri, M., Cheung, I., Reid, K., Wang, C., & Zee, P. (2014). Impact of windows and daylight exposure on overall health and sleep quality of office workers: a case-control pilot study. Journal of Clinical Sleep Medicine: JCSM: Official Publication of the American Academy of Sleep Medicine, 10 (6), 603-611.
Ford, E. S., Smith, S. J., Stroup, D. F., Steinberg, K. K., Mueller, P. W., & Thacker, S. B. (2002). Homocyst (e) ine and cardiovascular disease: a systematic review of the evidence with special emphasis on case-control studies and nested case-control studies. International journal of epidemiology, 31 (1), 59-70.
Helicobacter and Cancer Collaborative Group. (2001). Gastric cancer and Helicobacter pylori: a combined analysis of 12 case control studies nested within prospective cohorts. Gut, 49 (3), 347-353.
Howe, G. R., Hirohata, T., Hislop, T. G., Iscovich, J. M., Yuan, J. M., Katsouyanni, K., … & Shunzhang, Y. (1990). Dietary factors and risk of breast cancer: combined analysis of 12 case—control studies. JNCI: Journal of the National Cancer Institute, 82 (7), 561-569.
Lewallen, S., & Courtright, P. (1998). Epidemiology in practice: case-control studies. Community eye health, 11 (28), 57–58.
Strachan, D. P., & Cook, D. G. (1998). Parental smoking and childhood asthma: longitudinal and case-control studies. Thorax, 53 (3), 204-212.
Tenny, S., Kerndt, C. C., & Hoffman, M. R. (2021). Case Control Studies. In StatPearls . StatPearls Publishing.
Togha, M., Razeghi Jahromi, S., Ghorbani, Z., Martami, F., & Seifishahpar, M. (2018). Serum Vitamin D Status in a Group of Migraine Patients Compared With Healthy Controls: A Case-Control Study. Headache, 58 (10), 1530-1540.
Further Information
- Schulz, K. F., & Grimes, D. A. (2002). Case-control studies: research in reverse. The Lancet, 359(9304), 431-434.
- What is a case-control study?

Related Articles
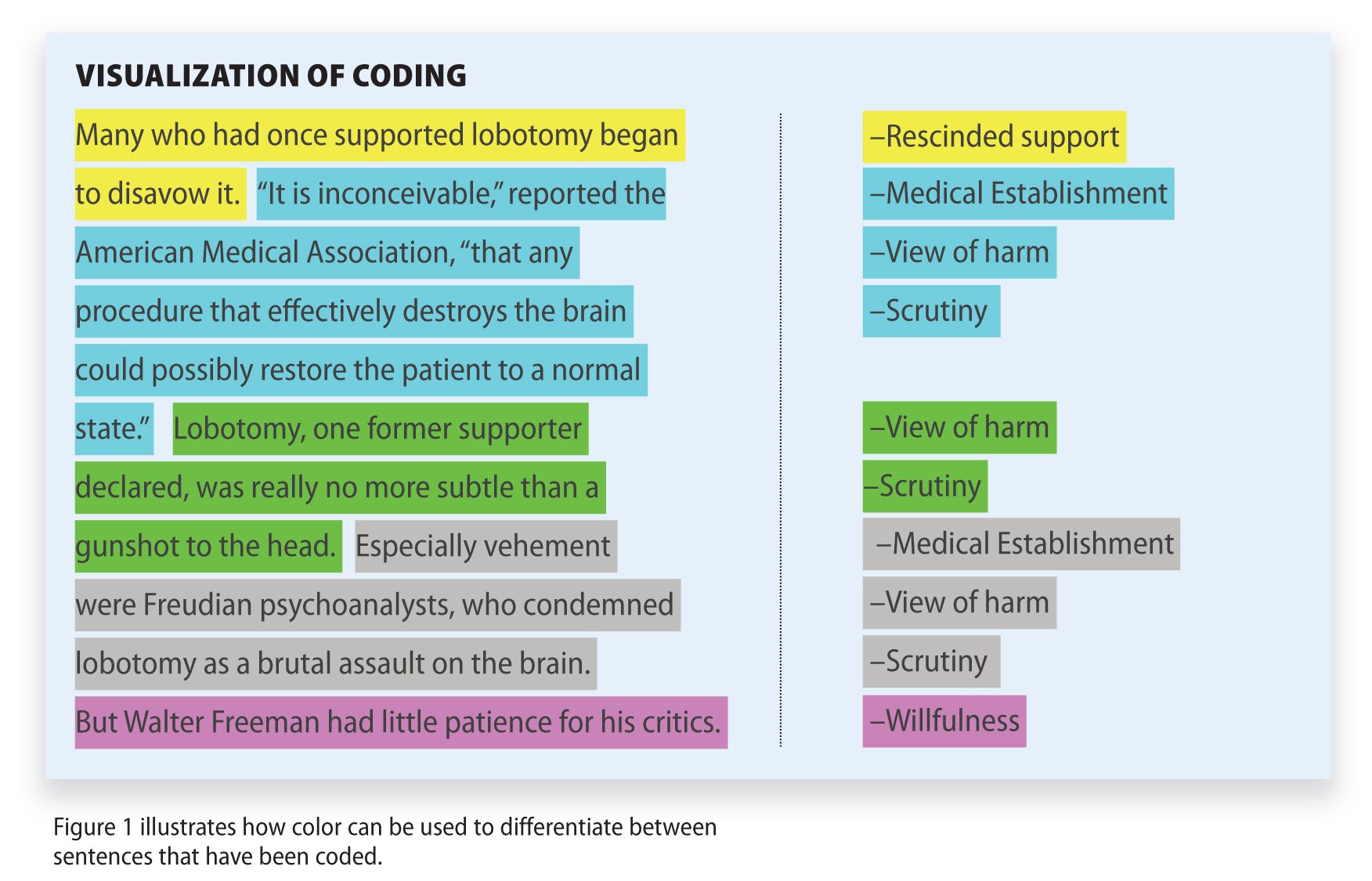
Research Methodology
Qualitative Data Coding
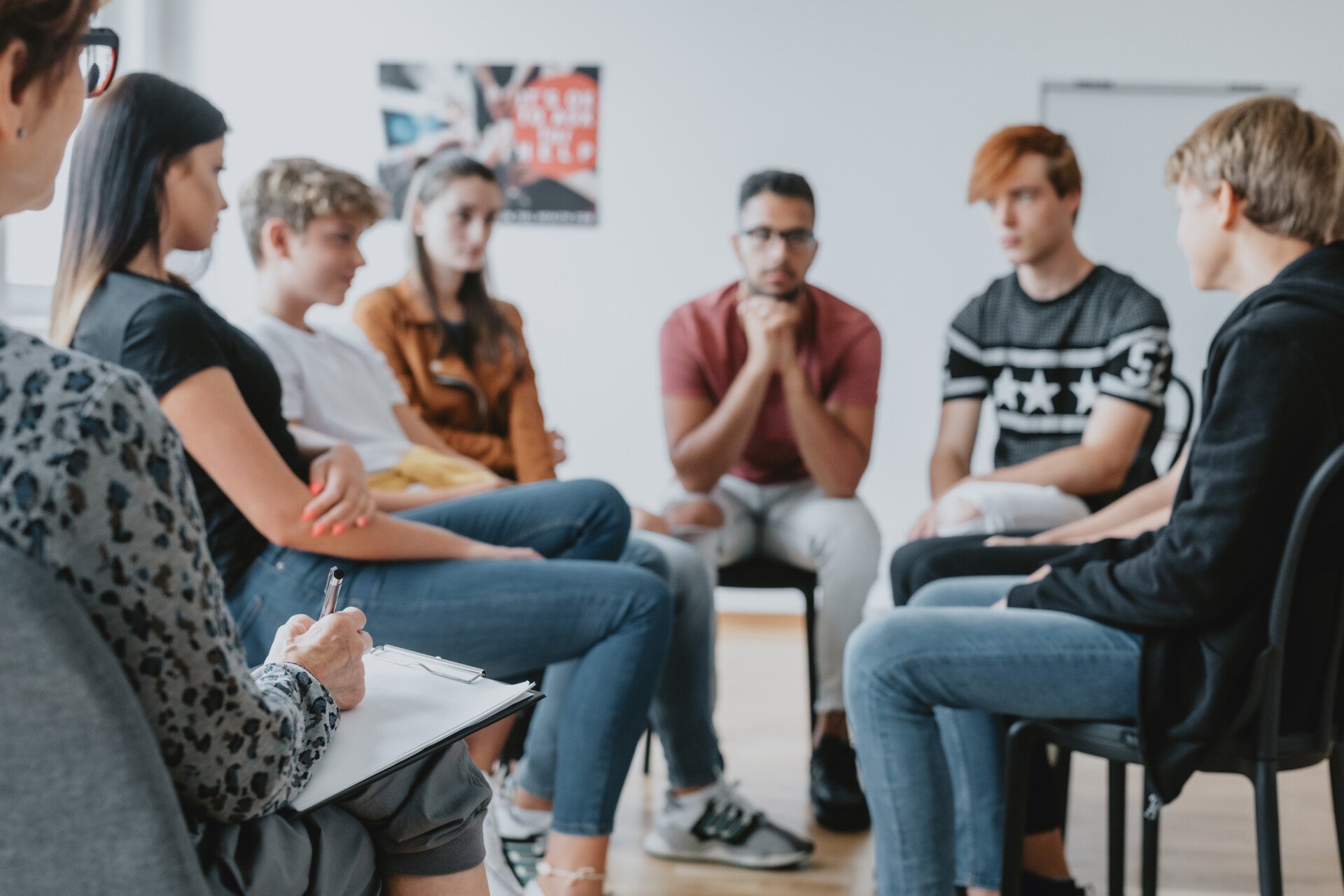
What Is a Focus Group?
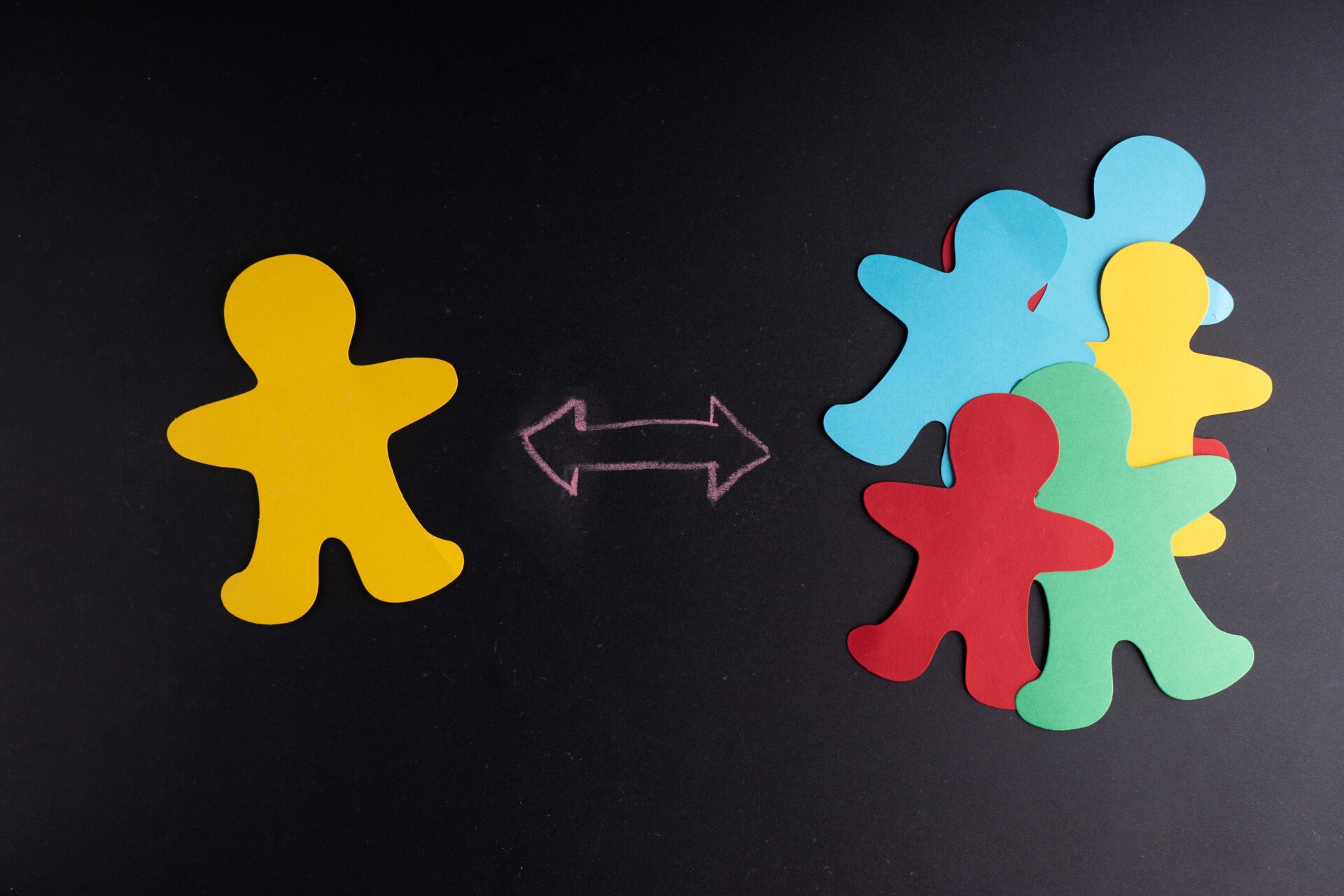
Cross-Cultural Research Methodology In Psychology
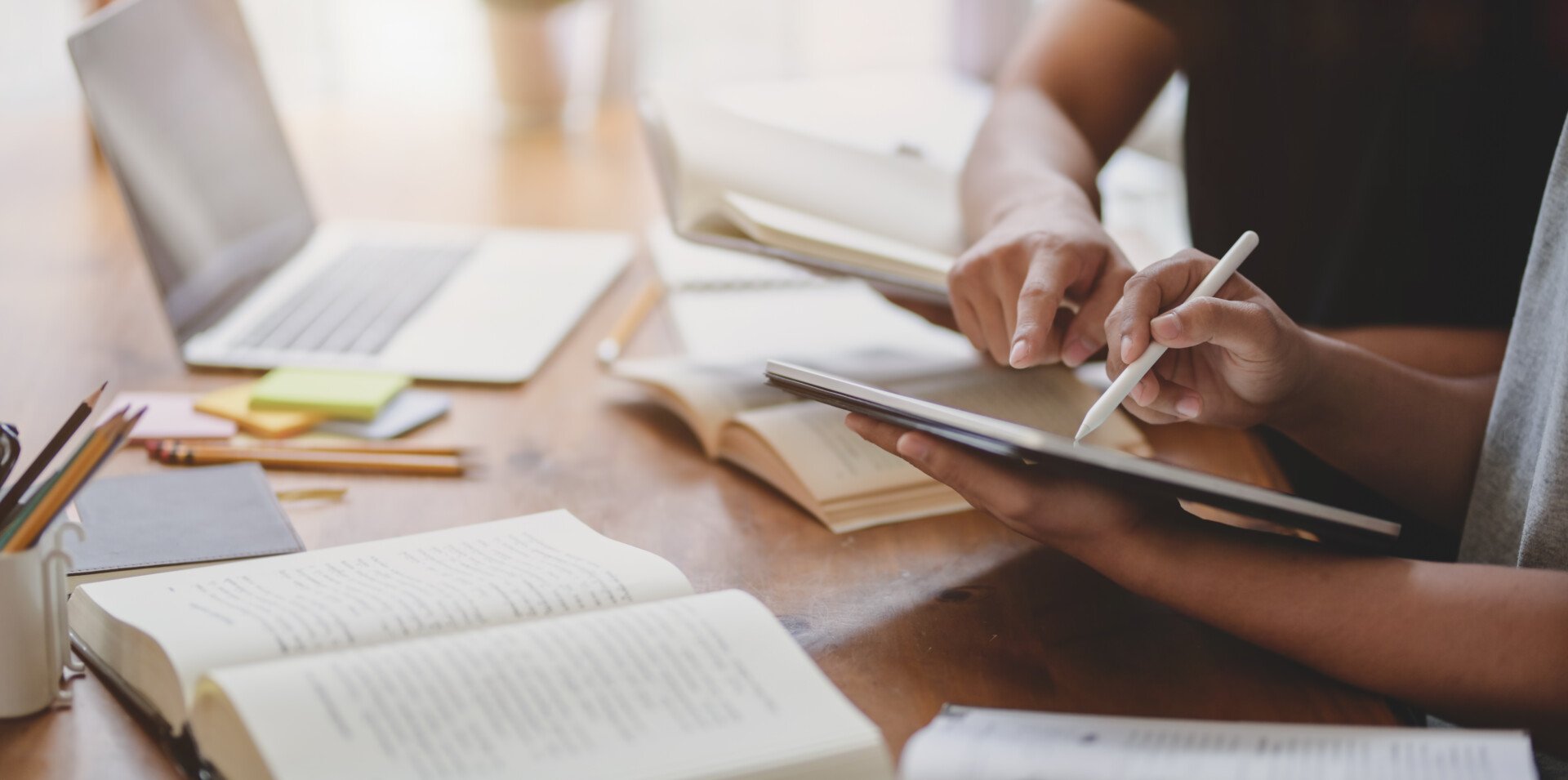
What Is Internal Validity In Research?
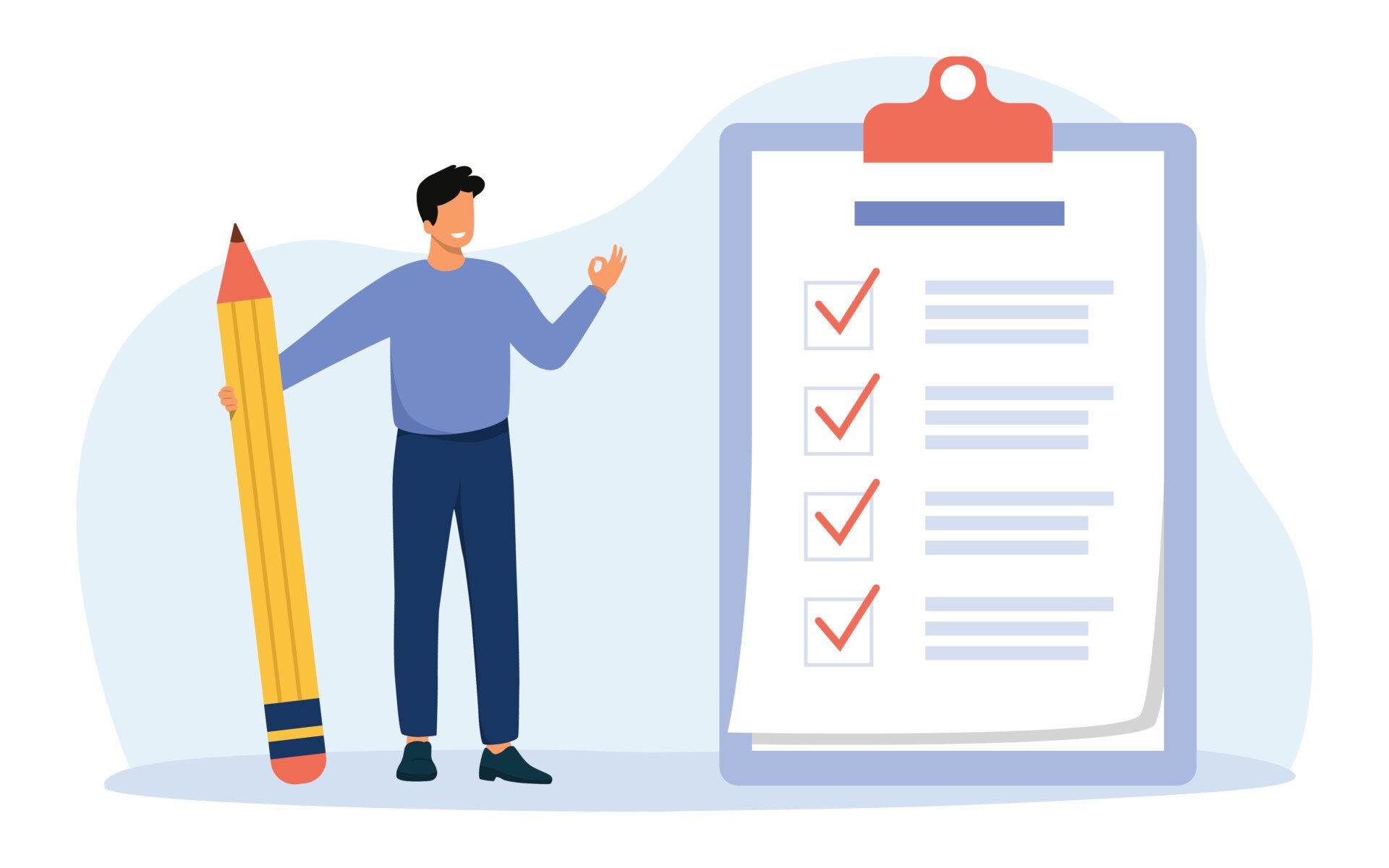
Research Methodology , Statistics
What Is Face Validity In Research? Importance & How To Measure
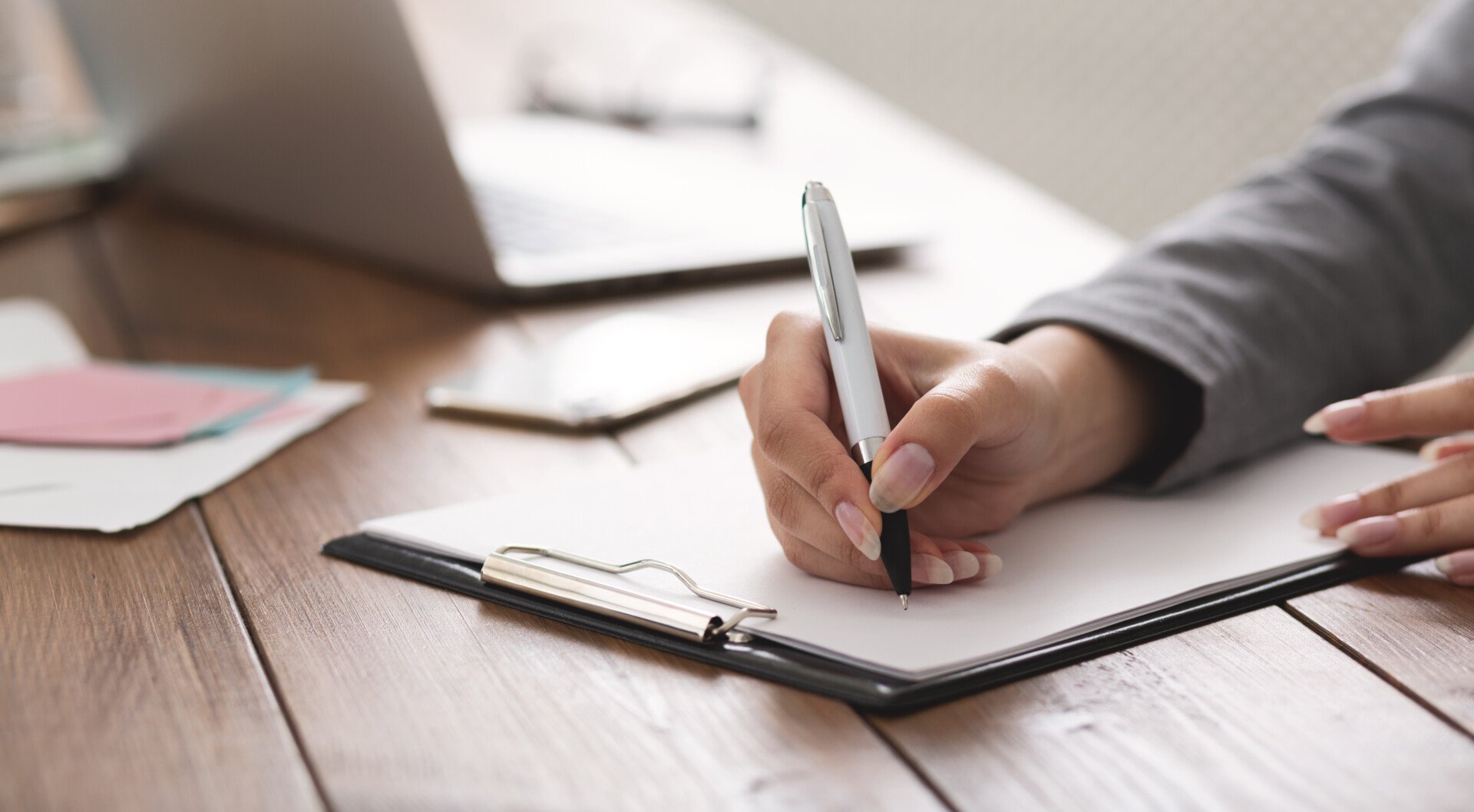
Criterion Validity: Definition & Examples

Overview of Analytic Studies
- 1
- | 2
- | 3
- | 4
- | 5
- | 6

Epi_Tools.XLSX
All Modules
Case-Control Studies
Cohort studies have an intuitive logic to them, but they can be very problematic when:
- The outcomes being investigated are rare;
- There is a long time period between the exposure of interest and the development of the disease; or
- It is expensive or very difficult to obtain exposure information from a cohort.
In the first case, the rarity of the disease requires enrollment of very large numbers of people. In the second case, the long period of follow-up requires efforts to keep contact with and collect outcome information from individuals. In all three situations, cost and feasibility become an important concern.
A case-control design offers an alternative that is much more efficient. The goal of a case-control study is the same as that of cohort studies, i.e. to estimate the magnitude of association between an exposure and an outcome. However, case-control studies employ a different sampling strategy that gives them greater efficiency. As with a cohort study, a case-control study attempts to identify all people who have developed the disease of interest in the defined population. This is not because they are inherently more important to estimating an association, but because they are almost always rarer than non-diseased individuals, and one of the requirements of accurate estimation of the association is that there are reasonable numbers of people in both the numerators (cases) and denominators (people or person-time) in the measures of disease frequency for both exposed and reference groups. However, because most of the denominator is made up of people who do not develop disease, the case-control design avoids the need to collect information on the entire population by selecting a sample of the underlying population.
To illustrate this consider the following hypothetical scenario in which the source population is Plymouth County in Massachusetts, which has a total population of 6,647 (hypothetical). Thirteen people in the county have been diagnosed with an unusual disease and seven of them have a particular exposure that is suspected of being an important contributing factor. The chief problem here is that the disease is quite rare.

If I somehow had exposure and outcome information on all of the subjects in the source population and looked at the association using a cohort design, it might look like this:
Therefore, the incidence in the exposed individuals would be 7/1,007 = 0.70%, and the incidence in the non-exposed individuals would be 6/5,640 = 0.11%. Consequently, the risk ratio would be 0.70/0.11=6.52, suggesting that those who had the risk factor (exposure) had 6.5 times the risk of getting the disease compared to those without the risk factor. This is a strong association.
In this hypothetical example, I had data on all 6,647 people in the source population, and I could compute the probability of disease (i.e., the risk or incidence) in both the exposed group and the non-exposed group, because I had the denominators for both the exposed and non-exposed groups.
The problem , of course, is that I usually don't have the resources to get the data on all subjects in the population. If I took a random sample of even 5-10% of the population, I might not have any diseased people in my sample.
An alternative approach would be to use surveillance databases or administrative databases to find most or all 13 of the cases in the source population and determine their exposure status. However, instead of enrolling all of the other 5,634 residents, suppose I were to just take a sample of the non-diseased population. In fact, suppose I only took a sample of 1% of the non-diseased people and I then determined their exposure status. The data might look something like this:
With this sampling approach I can no longer compute the probability of disease in each exposure group, because I no longer have the denominators in the last column. In other words, I don't know the exposure distribution for the entire source population. However, the small control sample of non-diseased subjects gives me a way to estimate the exposure distribution in the source population. So, I can't compute the probability of disease in each exposure group, but I can compute the odds of disease in the case-control sample.
The Odds Ratio
The odds of disease among the exposed sample are 7/10, and the odds of disease in the non-exposed sample are 6/56. If I compute the odds ratio, I get (7/10) / (5/56) = 6.56, very close to the risk ratio that I computed from data for the entire population. We will consider odds ratios and case-control studies in much greater depth in a later module. However, for the time being the key things to remember are that:
- The sampling strategy for a case-control study is very different from that of cohort studies, despite the fact that both have the goal of estimating the magnitude of association between the exposure and the outcome.
- In a case-control study there is no "follow-up" period. One starts by identifying diseased subjects and determines their exposure distribution; one then takes a sample of the source population that produced those cases in order to estimate the exposure distribution in the overall source population that produced the cases. [In cohort studies none of the subjects have the outcome at the beginning of the follow-up period.]
- In a case-control study, you cannot measure incidence, because you start with diseased people and non-diseased people, so you cannot calculate relative risk.
- The case-control design is very efficient. In the example above the case-control study of only 79 subjects produced an odds ratio (6.56) that was a very close approximation to the risk ratio (6.52) that was obtained from the data in the entire population.
- Case-control studies are particularly useful when the outcome is rare is uncommon in both exposed and non-exposed people.
The Difference Between "Probability" and "Odds"?

- The odds are defined as the probability that the event will occur divided by the probability that the event will not occur.
If the probability of an event occurring is Y, then the probability of the event not occurring is 1-Y. (Example: If the probability of an event is 0.80 (80%), then the probability that the event will not occur is 1-0.80 = 0.20, or 20%.
The odds of an event represent the ratio of the (probability that the event will occur) / (probability that the event will not occur). This could be expressed as follows:
Odds of event = Y / (1-Y)
So, in this example, if the probability of the event occurring = 0.80, then the odds are 0.80 / (1-0.80) = 0.80/0.20 = 4 (i.e., 4 to 1).
- If a race horse runs 100 races and wins 25 times and loses the other 75 times, the probability of winning is 25/100 = 0.25 or 25%, but the odds of the horse winning are 25/75 = 0.333 or 1 win to 3 loses.
- If the horse runs 100 races and wins 5 and loses the other 95 times, the probability of winning is 0.05 or 5%, and the odds of the horse winning are 5/95 = 0.0526.
- If the horse runs 100 races and wins 50, the probability of winning is 50/100 = 0.50 or 50%, and the odds of winning are 50/50 = 1 (even odds).
- If the horse runs 100 races and wins 80, the probability of winning is 80/100 = 0.80 or 80%, and the odds of winning are 80/20 = 4 to 1.
NOTE that when the probability is low, the odds and the probability are very similar.
On Sept. 8, 2011 the New York Times ran an article on the economy in which the writer began by saying "If history is a guide, the odds that the American economy is falling into a double-dip recession have risen sharply in recent weeks and may even have reached 50 percent." Further down in the article the author quoted the economist who had been interviewed for the story. What the economist had actually said was, "Whether we reach the technical definition [of a double-dip recession] I think is probably close to 50-50."
Question: Was the author correct in saying that the "odds" of a double-dip recession may have reached 50 percent?
return to top | previous page | next page
Content ©2023. All Rights Reserved. Date last modified: August 15, 2023. Wayne W. LaMorte, MD, PhD, MPH
- Chapter 8. Case-control and cross sectional studies
Case-control studies
Selection of cases, selection of controls, ascertainment of exposure, cross sectional studies.
- Chapter 1. What is epidemiology?
- Chapter 2. Quantifying disease in populations
- Chapter 3. Comparing disease rates
- Chapter 4. Measurement error and bias
- Chapter 5. Planning and conducting a survey
- Chapter 6. Ecological studies
- Chapter 7. Longitudinal studies
- Chapter 9. Experimental studies
- Chapter 10. Screening
- Chapter 11. Outbreaks of disease
- Chapter 12. Reading epidemiological reports
- Chapter 13. Further reading
Follow us on
Content links.
- Collections
- Health in South Asia
- Women’s, children’s & adolescents’ health
- News and views
- BMJ Opinion
- Rapid responses
- Editorial staff
- BMJ in the USA
- BMJ in South Asia
- Submit your paper
- BMA members
- Subscribers
- Advertisers and sponsors
Explore BMJ
- Our company
- BMJ Careers
- BMJ Learning
- BMJ Masterclasses
- BMJ Journals
- BMJ Student
- Academic edition of The BMJ
- BMJ Best Practice
- The BMJ Awards
- Email alerts
- Activate subscription
Information

Official websites use .gov
A .gov website belongs to an official government organization in the United States.
Secure .gov websites use HTTPS
A lock ( ) or https:// means you've safely connected to the .gov website. Share sensitive information only on official, secure websites.

Designing and Conducting Analytic Studies in the Field
Brendan R. Jackson And Patricia M. Griffin
Analytic studies can be a key component of field investigations, but beware of an impulse to begin one too quickly. Studies can be time- and resource-intensive, and a hastily constructed study might not answer the correct questions. For example, in a foodborne disease outbreak investigation, if the culprit food is not on your study’s questionnaire, you probably will not be able to implicate it. Analytic studies typically should be used to test hypotheses, not generate them. However, in certain situations, collecting data quickly about patients and a comparison group can be a way to explore multiple hypotheses. In almost all situations, generating hypotheses before designing a study will help you clarify your study objectives and ask better questions.
- Generating Hypotheses
- Study Designs for Testing Hypotheses
- Types of Observational Studies for Testing Hypotheses
- Selection of Controls in Case–Control Studies
- Matching in Case–Control Studies
- Example: Using an Analytic Study to Solve an Outbreak at a Church Potluck Dinner (But Not That Church Potluck)
- Outbreaks with Universal Exposure
The initial steps of an investigation, described in previous chapters, are some of your best sources of hypotheses. Key activities include the following:
- By examining the sex distribution among persons in outbreaks, US enteric disease investigators have learned to suspect a vegetable as the source when most patients are women. (Of course, generalizations do not always hold true!)
- In an outbreak of bloodstream infections caused by Serratia marcescens among patients receiving parenteral nutrition (food administered through an intravenous catheter), investigators had a difficult time finding the source until they noted that none of the 19 cases were among children. Further investigation of the parenteral nutrition administered to adults but not children in that hospital identified contaminated amino acid solution as the source ( 1 ).
- Focus on outliers. Give extra attention to the earliest and latest cases on an epidemic curve and to persons who recently visited the neighborhood where the outbreak is occurring. Interviews with these patients can yield important clues (e.g., by identifying the index case, secondary case, or a narrowed list of common exposures).
- Determine sources of similar outbreaks. Consult health department records, review the literature, and consult experts to learn about previous sources. Be mindful that new sources frequently occur, given ever-changing social, behavioral, and commercial trends.
- Conduct a small number of in-depth, open-ended interviews. When a likely source is not quickly evident, conducting in-depth (often >1 hour), open-ended interviews with a subset of patients (usually 5 to 10) or their caregivers can be the best way to identify possible sources. It helps to begin with a semistructured list of questions designed to help the patient recall the events and exposures of every day during the incubation period. The interview can end with a “shotgun” questionnaire (see activity 6) ( Box 7.1 ). A key component of this technique is that one investigator ideally conducts, or at least participates in, as many interviews as possible (five or more) because reading notes from others’ interviews is no substitute for soliciting and hearing the information first-hand. For example, in a 2009 Escherichia coli O157 outbreak, investigators were initially unable to find the source through general and targeted questionnaires. During open-ended interviews with five patients, the interviewer noted that most reported having eaten strawberries, a particular type of candy, and uncooked prepackaged cookie dough. An analytic study was then conducted that included questions about these exposures; it confirmed cookie dough as the source ( 3 ).
- Ask patients what they think. Patients can have helpful thoughts about the source of their illness. However, be aware that patients often associate their most recent food exposure (e.g., a meal) with illness, whereas the inciting exposure might have been long before.
- Consider administering a shotgun questionnaire. Such questionnaires, which typically ask about hundreds of possible exposures, are best used on a limited number of patients as part of hypothesis-generating interviews. After generating hypotheses, investigators can create a questionnaire targeted to that investigation. Although not an ideal method, shotgun questionnaires can be used by multiple interviewers to obtain data about large numbers of patients ( Box 7.1 ).
In November 2014, a US surveillance system for foodborne diseases (PulseNet) detected a cluster (i.e., a possible outbreak) of listeriosis cases based on similar-appearing Listeria monocytogenes isolates by pulsed-field gel electrophoresis of the isolates. No suspected foods were identified through routine patient interviews by using a Listeria -specific questionnaire with approximately 40 common food sources of listeriosis (e.g., soft cheese and deli meat). The outbreak’s descriptive epidemiology offered no clear leads: the sex distribution was nearly even, the age spectrum was wide, and the case-fatality rate of approximately 20% was typical. Notably, however, 3 of the 35 cases occurred among previously healthy school-aged children, which is highly unusual for listeriosis. Most cases occurred during late October and early November.
Investigators began reinterviewing patients by using a hypothesis-generating shotgun questionnaire with more than 500 foods, but it did not include caramel apples. By comparing the first nine patient responses with data from a published survey of food consumption, strawberries and ice cream emerged as hypotheses. However, several interviewed patients denied having eaten these foods during the month before illness. An investigator then conducted lengthy, open-ended interviews with patients and their family members. During one interview, he asked about special foods eaten during recent holidays, and the patient’s wife replied that her husband had eaten prepackaged caramel apples around Halloween. Although produce items had been implicated in past listeriosis outbreaks, caramel apples seemed an unlikely source. However, the interviewer took note of this connection because he had previously interviewed another patient who reported having eaten caramel apples. This event underscores the importance of one person conducting multiple interviews because that person might make subtle mental connections that may be missed when reviewing other interviewers’ notes. In fact, several other investigators listening to the interview noted this exposure—among hundreds of others—but thought little of it.
In this investigation, the finding of high strawberry and ice cream consumption among patients, coupled with the timing of the outbreak during a holiday period, helped make a sweet food (i.e., caramel apples) seem more plausible as the possible source.
To explore the caramel apple hypothesis, investigators asked five other patients about this exposure, and four reported having eaten them. On the basis of these initial results, investigators designed and administered a targeted questionnaire to patients involved in the outbreak, as well as to patients infected with unrelated strains of L. monocytogenes (i.e., a case–case study). This study, combined with testing of apples and the apple packing facility, confirmed that caramel apples were the source (2). Had a single interviewer performed multiple open-ended interviews to generate hypotheses before the shotgun questionnaire, the outbreak might have been solved sooner.
As evident in public health and clinical guidelines, randomized controlled trials (e.g., trials of drugs, vaccines, and community-level interventions) are the reference standard for epidemiology, providing the highest level of evidence. However, such studies are not possible in certain situations, including outbreak investigations. Instead, investigators must rely on observational studies, which can provide sufficient evidence for public health action. In observational studies, the epidemiologist documents rather than determines the exposures, quantifying the statistical association between exposure and disease. Here again, the key when designing such studies is to obtain a relevant comparison group for the patients ( Box 7.2 ).
Because field analytic studies are used to quantify the association between exposure and disease, defining what is meant by exposure and disease is essential. Exposure is used broadly, meaning demographic characteristics, genetic or immunologic makeup, behaviors, environmental exposures, and other factors that might influence a person’s risk for disease. Because precise information can help accurately estimate an exposure’s effect on disease, exposure measures should be as objective and standard as possible. Developing a measure of exposure can be conceptually straightforward for an exposure that is a relatively discrete event or characteristic—for example, whether a person received a spinal injection with steroid medication compounded at a specific pharmacy or whether a person received a typhoid vaccination during the year before international travel. Although these exposures might be straightforward in theory, they can be subject to interpretation in practice. Should a patient injected with a medication from an unknown pharmacy be considered exposed? Whatever decision is made should be documented and applied consistently.
Additionally, exposures often are subject to the whims of memory. Memory aids (e.g., restaurant menus, vaccination cards, credit card receipts, and shopper cards) can be helpful. More than just a binary yes or no, the dose of an exposure can also be enlightening. For example, in an outbreak of fungal bloodstream infections linked to contaminated intravenous saline flushes administered at an oncology clinic, affected patients had received a greater number of flushes than unaffected patients ( 4 ). Similarly, in an outbreak of Listeria monocytogenes infections, the association with deli meat became apparent only when the exposure evaluated was consumption of deli meat more than twice a week ( 5 ).
Defining disease (e.g., does a person have botulism?) might sound simple, but often it is not; read more about making and applying disease case definitions in Chapter 3 .
Three types of observational studies are commonly used in the field. All are best performed by using a standard questionnaire specific for that investigation, developed on the basis of hypothesis-generating interviews.
Observational Study Type 1: Cohort
In concept, a cohort study, like an experimental study, begins with a group of persons without the disease under study, but with different exposure experiences, and follows them over time to find out whether they experience the disease or health condition of interest. However, in a cohort study, each person’s exposure is merely recorded rather than assigned randomly by the investigator. Then the occurrence of disease among persons with different exposures is compared to assess whether the exposures are associated with increased risk for disease. Cohort studies can be prospective or retrospective.
Prospective Cohort Studies
A prospective cohort study enrolls participants before they experience the disease or condition of interest. The enrollees are then followed over time for occurrence of the disease or condition. The unexposed or lowest exposure group serves as the comparison group, providing an estimate of the baseline or expected amount of disease. An example of a prospective cohort study is the Framingham Heart Study. By assessing the exposures of an original cohort of more than 5,000 adults without cardiovascular disease (CVD), beginning in 1948 and following them over time, the study was the first to identify common CVD risk factors ( 6 ). Each case of CVD identified after enrollment was counted as an incident case. Incidence was then quantified as the number of cases divided by the sum of time that each person was followed (incidence rate) or as the number of cases divided by the number of participants being followed (attack rate or risk or i ncidence proportion). In field epidemiology, prospective cohort studies also often involve a group of persons who have had a known exposure (e.g., survived the World Trade Center attack on September 11, 2001 [ 7 ]) and who are then followed to examine the risk for subsequent illnesses with long incubation or latency periods.
Retrospective Cohort Studies
A retrospective cohort study enrolls a defined participant group after the disease or condition of interest has occurred. In field epidemiology, these studies are more common than prospective studies. The population affected is often well-defined (e.g., banquet attendees, a particular school’s students, or workers in a certain industry). Investigators elicit exposure histories and compare disease incidence among persons with different exposures or exposure levels.
Observational Study Type 2: Case–Control
In a case–control study, the investigator must identify a comparison group of control persons who have had similar opportunities for exposure as the case-patients. Case–control studies are commonly performed in field epidemiology when a cohort study is impractical (e.g., no defined cohort or too many non-ill persons in the group to interview). Whereas a cohort study proceeds conceptually from exposure to disease or condition, a case–control study begins conceptually with the disease or condition and looks backward at exposures. Excluding controls by symptoms alone might not guarantee that they do not have mild cases of the illness under investigation. Table 7.1 presents selected key differences between a case–control and retrospective cohort study.
Observational Study Type 3: Case–Case
In case–case studies, a group of patients with the same or similar disease serve as a comparison group (8). This method might require molecular subtyping of the suspected pathogen to distinguish outbreak-associated cases from other cases and is especially useful when relevant controls are difficult to identify. For example, controls for an investigation of Listeria illnesses typically are patients with immunocompromising conditions (e.g., cancer or corticosteroid use) who might be difficult to identify among the general population. Patients with Listeria isolates of a different subtype than the outbreak strain can serve as comparisons to help reduce bias when comparing food exposures. However, patients with similar illnesses can have similar exposures, which can introduce a bias, making identifying the source more difficult. Moreover, other considerations should influence the choice of a comparison group. If most outbreak-associated case-patients are from a single neighborhood or are of a certain race/ethnicity, other patients with listeriosis from across the country will serve as an inadequate comparison group.
Considerations for Selecting Controls
Selecting relevant controls is one of the most important considerations when designing a case–control study. Several key considerations are presented here; consult other resources for in-depth discussion ( 9,10 ). Ideally, controls should
- Thoroughly reflect the source population from which case-patients arose, and
- Provide a good estimate of the level of exposure one would expect from that population. Sometimes the source population is not so obvious, and a case–control study using controls from the general population might be needed to implicate a general exposure (e.g., visiting a specific clinic, restaurant, or fair). The investigation can then focus on specific exposures among persons with the general exposure (see also next section).
Controls should be chosen independently of any specific exposure under evaluation. If you select controls on the basis of lack of exposure, you are likely to find an association between illness and that exposure regardless of whether one exists. Also important is selecting controls from a source population in a way that minimizes confounding (see Chapter 8 ), which is the existence of a factor (e.g., annual income) that, by being associated with both exposure and disease, can affect the associations you are trying to examine.
When trying to enroll controls who reflect the source population, try to avoid overmatching (i.e., enrolling controls who are too similar to case-patients, resulting in fewer differences among case-patients and controls than ought to exist and decreased ability to identify exposure–disease associations). When conducting case–control studies in hospitals and other healthcare settings, ensure that controls do not have other diseases linked to the exposure under study.
Commonly Used Control Selection Methods
When an outbreak does not affect a defined population (e.g., potluck dinner attendees) but rather the community at large, a range of options can be used to determine how to select controls from a large group of persons.
- Random-digit dialing . This method, which involves selecting controls by using a system that randomly selects telephone numbers from a directory, has been a staple of US outbreak investigations. In recent years, however, declining response rates because of increasing use of caller identification and cellular phones and lack of readily available directory listings of cellular phone numbers by geographic area have made this method increasingly difficult. Even when this method was most useful, often 50 or more numbers needed to be dialed to reach one household or person who both answered and provided a usable match for the case-patient. Commercial databases that include cellular phone numbers have been used successfully to partially address this problem, but the method remains time-consuming ( 11 ).
- Random or systematic sampling from a list . For investigations in settings where a roster is available (e.g., attendees at a resort on certain dates), controls can be selected by either random or systematic sampling. Government records (e.g., motor vehicle, voter, or tax records) can provide lists of possible controls, but they might not be representative of the population being studied ( 11 ). For random sampling, a table or computer-generated list of random numbers can be used to select every n th persons to contact (e.g., every 12th or 13th).
- Neighborhood . Recruiting controls from the same neighborhood as case-patients (i.e., neighborhood matching) has commonly been used during case–control studies, particularly in low-and middle-income countries. For example, during an outbreak of typhoid fever in Tajikistan ( 12 ), investigators recruited controls by going door-to-door down a street, starting at a case-patient’s house; a study of cholera in Haiti used a similar method ( 13 ). Typically, the immediately neighboring households are skipped to prevent overmatching.
- Patients’ friends or relatives . Using friends and relatives as controls can be an effective technique when the characteristics of case-patients (e.g., very young children) make finding controls by a random method difficult. Typically, the investigator interviews a patient or his or her parent, then asks for the names and contact information for more friends or relatives who are needed as controls. One advantage is that the friends of an ill person are usually willing to participate, knowing their cooperation can help solve the puzzle. However, because they can have similar personal habits and preferences as patients, their exposures might be similar. Such overmatching can decrease the likelihood of finding the source of the illness or condition.
- Databases of persons with exposure information . Sources of data on persons with exposure information include survey data (e.g., FoodNet Population Survey [ 14 ]), public health databases of patients with other illnesses or a different subtype of the same illness, and previous studies. ( Chapter 4 describes additional sources.)
When considering outside data sources, investigators must determine whether those data provide an appropriate comparison group. For example, persons in surveys might differ from case-patients in ways that are impossible to determine. Other patients might be so similar to case-patients that risky exposures are unidentifiable, or they might be so different that exposures identified as risks are not true risks.
To help control for confounding, controls can be matched to case-patients on characteristics specified by investigators, including age group, sex, race/ethnicity, and neighborhood. Such matching does not itself reduce confounding, but it enables greater efficiency when matched analyses are performed that do ( 15 ). When deciding to match, however, be judicious. Matching on too many characteristics can make controls difficult to find (making a tough process even harder). Imagine calling hundreds of random telephone numbers trying to find a man of a particular ethnicity aged 50–54 years who is then willing to answer your questions. Also, remember not to match on the exposure of interest or on any other characteristic you wish to examine. Matched case–control study data typically necessitate a matched analysis (e.g., conditional logistic regression) ( 15 ).
Matching Types
The two main types of matching are pair matching and frequency matching.
Pair Matching
In pair matching, each control is matched to a specific case-patient. This method can be helpful logistically because it allows matching by friends or relatives, neighborhood, or telephone exchange, but finding controls who meet specific criteria can be burdensome.
Frequency Matching
In frequency matching, also called category matching , controls are matched to case-patients in proportion to the distribution of a characteristic among case-patients. For example, if 20% of case-patients are children aged 5–18 years, 50% are adults aged 19–49 years, and 30% are adults 50 years or older, controls should be enrolled in similar proportions. This method works best when most case-patients have been identified before control selection begins. It is more efficient than pair matching because a person identified as a possible control who might not meet the criteria for matching a particular case-patient might meet criteria for one of the case-patient groups.
Number of Controls
Most field case–control studies use control-to-case-patient ratios of 1:1, 2:1, or 3:1. Enrolling more than one control per case-patient can increase study power, which might be needed to detect a statistically significant difference in exposure between case-patients and controls, particularly when an outbreak involves a limited number of cases. The incremental gain of adding more controls beyond three or four is small because study power begins to plateau. Note that not all case-patients need to have the same number of controls. Sample size calculations can help in estimating a target number of controls to enroll, although sample sizes in certain field investigations are limited more by time and resource constraints. Still, estimating study power under a range of scenarios is wise because an analytic study might not be worth doing if you have little chance of detecting a statistically significant association. Sample size calculators for unmatched case–control studies are available at http://www.openepi.com and in the StatCalc function of Epi Info ( https://www.cdc.gov/epiinfo ).
More than One Control Group
Sometimes the choice of a control group is so vexing that investigators decide to use more than one type of control group (e.g., a hospital-based group and a community group). If the two control groups provide similar results and conclusions about risk factors for disease, the credibility of the findings is increased. In contrast, if the two control groups yield conflicting results, interpretation becomes more difficult.
Since the 1940s, field epidemiology students have studied a classic outbreak of gastrointestinal illness at a church potluck dinner in Oswego, New York ( 16 ). However, the case study presented here, used to illustrate study designs, is a different potluck dinner.
In April 2015, an astute neurologist in Lancaster, Ohio, contacted the local health department about a patient in the emergency department with a suspected case of botulism. Within 2 hours, four more patients arrived with similar symptoms, including blurred vision and shortness of breath. Health officials immediately recognized this as a botulism outbreak.
- If the source is a widely distributed commercial product, then the population to study is persons across the United States and possibly abroad.
- If the source is airborne, then the population to study is residents of a single city or area.
- If the source is food from a restaurant, then the population to study is predominantly local residents and some travelers.
- If the source is a meal at a workplace or social setting, then the population to study is meal attendees.
- If the source is a meal at home, then the population to study is household members and any guests.
Descriptive epidemiology and questioning of the case-patients revealed that all had eaten at the same church potluck dinner and had no other common exposures, making the potluck the likely exposure site and attendees the likely source population. Thus, an analytic study would be targeted at potluck attendees, although investigators must remain alert to case-patients among nonattendees. As initial interviews were conducted, more cases of botulism were being diagnosed, quickly increasing to more than 25. The source of the outbreak needed to be identified rapidly to halt further exposure and illness.
- List of foods served at the potluck.
- Approximate number of attendees.
- A case definition.
- Information from 5–10 hypothesis-generating interviews with a few case-patients or their family members.
- A cohort study would be a reasonable option because a defined group exists (i.e., a cohort) of exposed persons who could be interviewed in a reasonable amount of time. The study would be retrospective because the outcome (i.e., botulism) has already occurred, and investigators could assess exposures retrospectively (i.e., foods eaten at the potluck) by interviewing attendees.
- In a cohort study, investigators can calculate the attack rate for botulism among potluck attendees who reported having eaten each food and for those who had not. For example, if 20 of the 30 attendees who had eaten a particular food (e.g., potato salad) had botulism, you would calculate the attack rate by dividing 20 (corresponding to cell a in Handout 7.1 ) by 30 (total exposed, or a + b), yielding approximately 67%. If 5 of the 45 attendees who had not eaten potato salad had botulism, the attack rate among the unexposed—5 / 45, corresponding to c/ (c + d)—would be approximately 11%. The risk ratio would be 6, which is calculated by dividing the attack rate among the exposed (67%) by the attack rate among the unexposed (11%).
- A case–control study would be the most feasible option because the entire cohort could not be identified and because the large number of attendees could make interviewing them all difficult. Rather than interview all non-ill persons, a subset could be interviewed as control subjects.
- The method of control subject selection should be considered carefully. If all attendees are not interviewed, determining the risk for botulism among the exposed and unexposed is impossible because investigators would not know the exposures for all non-ill attendees. Instead of risk, investigators calculate the odds of exposure, which can approximate risk. For example, if 20 (80%) of 25 case-patients had eaten potato salad, the odds of potato salad exposure among case-patients would be 20/ 5 = 4 (exposed/ unexposed, or a/ c in Handout 7.2 ). If 10 (20%) of 50 selected controls had eaten potato salad, the odds of exposure among control subjects would be 10/ 40 = 0.25 (or b/ d in Handout 7.2). Dividing the odds of exposure among the case-patients (a/ c) by the odds of exposure among control subjects (b / d) yields an odds ratio of 16 (4/ 0.25). The odds ratio is not a true measure of risk, but it can be used to implicate a food. An odds ratio can approximate a risk ratio when the outcome or disease is rare (e.g., roughly <5% of a population). In such cases, a/ b is similar to a/ (a + b). The odds ratio is typically higher than the risk ratio when >5% of exposed persons in the analysis have the illness.
In the actual outbreak, 29 (38%) of 77 potluck attendees had botulism. The investigators performed a cohort study, interviewing 75 of the 77 attendees about 52 foods served ( 17 ). The attack rate among persons who had eaten potato salad was significantly and substantially higher than the attack rate among those who had not, with a risk ratio of 14 (95% confidence interval 5–42). One of the potato salads served was made with incorrectly home-canned potatoes (a known source of botulinum toxin), and samples of discarded potato salad tested positive for botulinum toxin, supporting the findings of the analytic study. (Of note, persons often blame potato salad for causing illness when, in fact, it rarely is a source. This outbreak was a notable exception.)
In field epidemiology, the link between exposure and illness is often so strong that it is evident despite such inherent study limitations as small sample size and exposure misclassification. In this outbreak, a few of the patients with botulism reported not having eaten potato salad, and some of the attendees without botulism reported having eaten it. In epidemiologic studies, you rarely find 100% concordance between exposure and outcome for various reasons, including incomplete or erroneous recall because remembering everything eaten is difficult. Here, cross-contamination of potato salad with other foods might have helped explain cases among patients who had not eaten potato salad because only a small amount of botulinum toxin is needed to produce illness.
Two-by-Two Table to Calculate the Relative Risk, or Risk Ratio, in Cohort Studies
Two- by- two tables are covered in more detail in Chapter 8 .
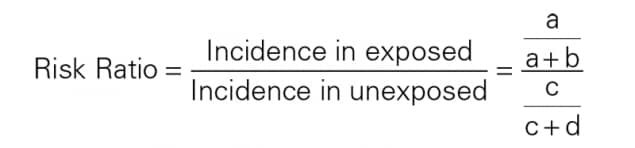
Two-by-Two Table to Calculate the Odds Ratio in Case–Control Studies
A risk ratio cannot be calculated from a case–control study because true attack rates cannot be calculated.

What kind of study would you design if your hypothesis-generating interviews lead you to believe that everyone, or nearly everyone, was exposed to the same suspected infection source? How would you test hypotheses if all barbecue attendees, ill and non-ill, had eaten the chicken or if all town residents had drunk municipal tap water, and no unexposed group exists for comparison? A few factors that might be of help are the exposure timing (e.g., a particularly undercooked batch of barbeque), the exposure place (e.g., a section of the water system more contaminated than others), and the exposure dose (e.g., number of chicken pieces eaten or glasses of water drunk). Including questions about the time, place, and frequency of highly suspected exposures in a questionnaire can improve the chances of detecting a difference ( 18 ).
Cohort, case–control, and case–case studies are the types of analytic studies that field epidemiologists use most often. They are best used as mechanisms for evaluating—quantifying and testing—hypotheses identified in earlier phases of the investigation. Cohort studies, which are oriented conceptually from exposure to disease, are appropriate in settings in which an entire population is well-defined and available for enrollment (e.g., guests at a wedding reception). Cohort studies are also appropriate when well-defined groups can be enrolled by exposure status (e.g., employees working in different parts of a manufacturing plant). Case–control studies, in contrast, are useful when the population is less clearly defined. Case–control studies, oriented from disease to exposure, identify persons with disease and a comparable group of persons without disease (controls). Then the exposure experiences of the two groups are compared. Case–case studies are similar to case–control studies, except that controls have an illness not linked to the outbreak. Case–control studies are probably the type most often appropriate for field investigations. Although conceptually straightforward, the design of an effective epidemiologic study requires many careful decisions. Taking the time needed to develop good hypotheses can result in a questionnaire that is useful for identifying risk factors. The choice of an appropriate comparison group, how many controls per case-patient to enroll, whether to match, and how best to avoid potential biases are all crucial decisions for a successful study.
This chapter relies heavily on the work of Richard C. Dicker, who authored this chapter in the previous edition.
- Gupta N, Hocevar SN, Moulton-Meissner HA, et al. Outbreak of Serratia marcescens bloodstream infections in patients receiving parenteral nutrition prepared by a compounding pharmacy. Clin Infect Dis. 2014;59:1–8.
- Angelo K, Conrad A, Saupe A, et al. Multistate outbreak of Listeria monocytogenes infections linked to whole apples used in commercially produced, prepackaged caramel apples: United States, 2014–2015. Epidemiol Infect. 2017;145:848–56.
- Neil KP, Biggerstaff G, MacDonald JK, et al. A novel vehicle for transmission of Escherichia coli O157: H7 to humans: multistate outbreak of E. coli O157: H7 infections associated with consumption of ready-to-bake commercial prepackaged cookie dough—United States, 2009. Clin Infect Dis. 2012;54:511–8.
- Vasquez AM, Lake J, Ngai S, et al. Notes from the field: fungal bloodstream infections associated with a compounded intravenous medication at an outpatient oncology clinic—New York City, 2016. MMWR. 2016;65:1274–5.
- Gottlieb SL, Newbern EC, Griffin PM, et al. Multistate outbreak of listeriosis linked to turkey deli meat and subsequent changes in US regulatory policy. Clin Infect Dis. 2006;42:29–36.
- Framingham Heart Study: A Project of the National Heart, Lung, and Blood Institute and Boston University. Framingham, MA: Framingham Heart Study; 2017. https://www.framinghamheartstudy.org/
- Jordan HT, Brackbill RM, Cone JE, et al. Mortality among survivors of the Sept 11, 2001, World Trade Center disaster: results from the World Trade Center Health Registry cohort. Lancet. 2011;378:879–87.
- McCarthy N, Giesecke J. Case– case comparisons to study causation of common infectious diseases. Int J Epidemiol. 1999;28:764–8.
- Rothman KJ, Greenland S. Modern epidemiology . 3rd ed. Philadelphia: Lippincott Williams & Wilkins; 2008.
- Wacholder S, McLaughlin JK, Silverman DT, Mandel JS. Selection of controls in case–control studies. I. Principles. Am J Epidemiol. 1992;135:1019–28.
- Chintapalli S, Goodman M, Allen M, et al. Assessment of a commercial searchable population directory as a means of selecting controls for case–control studies. Public Health Rep. 2009;124:378–83.
- Centers for Disease Control and Prevention. Epidemiologic case studies: typhoid in Tajikistan. http://www.cdc.gov/epicasestudies/classroom_typhoid.html
- Dunkle SE, Mba-Jonas A, Loharikar A, Fouche B, Peck M, Ayers T. Epidemic cholera in a crowded urban environment, Port-au-Prince, Haiti. Emerg Infect Dis. 2011;17:2143–6.
- Centers for Disease Control and Prevention. Foodborne Diseases Active Surveillance Network (FoodNet): population survey. http://www.cdc.gov/foodnet/surveys/population.html
- Pearce N. Analysis of matched case–control studies. BMJ. 2016;352:1969.
- Centers for Disease Control and Prevention. Case studies in applied epidemiology: Oswego: an outbreak of gastrointestinal illness following a church supper. http://www.cdc.gov/eis/casestudies.html
- McCarty CL, Angelo K, Beer KD, et al. Notes from the field.: large outbreak of botulism associated with a church potluck meal—Ohio, 2015. MMWR. 2015;64:802–3.
- Tostmann A, Bousema JT, Oliver I. Investigation of outbreaks complicated by universal exposure. Emerg Infect Dis. 2012;18:1717–22.
< Previous Chapter 6: Describing Epidemiologic Data
Next Chapter 8: Analayzing and Interpreting Data >
The fellowship application period will be open March 1-June 5, 2024.
The host site application period is closed.
For questions about the EIS program, please contact us directly at [email protected] .
- Laboratory Leadership Service (LLS)
- Fellowships and Training Opportunities
- Division of Workforce Development
Exit Notification / Disclaimer Policy
- The Centers for Disease Control and Prevention (CDC) cannot attest to the accuracy of a non-federal website.
- Linking to a non-federal website does not constitute an endorsement by CDC or any of its employees of the sponsors or the information and products presented on the website.
- You will be subject to the destination website's privacy policy when you follow the link.
- CDC is not responsible for Section 508 compliance (accessibility) on other federal or private website.
Thank you for visiting nature.com. You are using a browser version with limited support for CSS. To obtain the best experience, we recommend you use a more up to date browser (or turn off compatibility mode in Internet Explorer). In the meantime, to ensure continued support, we are displaying the site without styles and JavaScript.
- View all journals
- My Account Login
- Explore content
- About the journal
- Publish with us
- Sign up for alerts
- Open access
- Published: 29 May 2024
A case–control study on pre-, peri-, and neonatal risk factors associated with autism spectrum disorder among Armenian children
- Meri Mkhitaryan 1 ,
- Tamara Avetisyan 2 , 3 ,
- Anna Mkhoyan 4 ,
- Larisa Avetisyan 2 , 5 &
- Konstantin Yenkoyan 1
Scientific Reports volume 14 , Article number: 12308 ( 2024 ) Cite this article
Metrics details
- Autism spectrum disorders
- Public health
- Risk factors
We aimed to investigate the role of pre-, peri- and neonatal risk factors in the development of autism spectrum disorder (ASD) among Armenian children with the goal of detecting and addressing modifiable risk factors to reduce ASD incidence. For this purpose a retrospective case–control study using a random proportional sample of Armenian children with ASD to assess associations between various factors and ASD was conducted. The study was approved by the local ethical committee, and parental written consent was obtained. A total of 168 children with ASD and 329 controls were included in the analysis. Multivariable logistic regression analysis revealed that male gender, maternal weight gain, use of MgB6, self-reported stress during the pregnancy, pregnancy with complications, as well as use of labor-inducing drugs were associated with a significant increase in the odds of ASD, whereas Duphaston use during pregnancy, the longer interpregnancy interval and birth height were associated with decreased odds of ASD. These findings are pertinent as many identified factors may be preventable or modifiable, underscoring the importance of timely and appropriate public health strategies aimed at disease prevention in pregnant women to reduce ASD incidence.
Introduction
Autism spectrum disorder is a neurodevelopmental disorder by the Diagnostic and Statistical Manual of Mental Disorders, the 5th Edition (DSM-5). It is identified by limited repeating patterns of behavior, activities, and interests, as well as impaired social interaction and communication 1 . A systematic review of research articles spanning from 2012 to 2021 indicates that the worldwide median prevalence of ASD in children stands at 1% 2 . Nevertheless, this reported percentage may not fully capture the actual prevalence of ASD in low- and middle-income nations, potentially leading to underestimations. In 2016, data compiled by the CDC's Autism and Developmental Disabilities Monitoring (ADDM) Network revealed that approximately one out of 54 children in the United States (one out of 34 boys and one out of 144 girls) received a diagnosis of ASD. This marks a ten percent increase from the reported rate of one out of 59 in 2014, a 105 percent increase from one out of 110 in 2006, and a 176 percent increase from one out of 150 in 2000 3 . According to the most recent update from the CDC’s ADDM Network, one out of 36 (2.8%) 8-year-old children has been diagnosed with ASD. These latest statistics exceed the 2018 findings, which indicated a rate of 1 in 44 (2.3%) 4 . To our understanding, there is no existing registry for ASD in the Republic of Armenia (RA). Additionally, there is no available data concerning the incidence and prevalence of ASD in the country.
The etiology of ASD remains unclear despite substantial research on the disorder; yet, important advances have been made in identifying some of the disorder's genetic and neurobiological underpinnings. It has been discovered that ASD is heritable, with environmental variables also being involved 5 , 6 , 7 . According to certain research, ASD is associated with both hereditary and environmental factors 5 , 8 , 9 . It is especially important to identify environmental risk factors because, unlike genetic risk factors, they can be prevented.
There are more than 20 pre-, peri- and neonatal risk factors associated with ASD 10 , 11 , 12 . Prenatal risk factors that have been associated with ASD involve parental age 13 , interpregnancy interval 14 , 15 , immune factors (such as autoimmune diseases, both viral and bacterial infections during pregnancy) 16 , 17 , medication use (especially antidepressants, anti-asthmatics, and anti-epileptics) 18 , 19 , 20 , maternal metabolic conditions (such as diabetes, gestational weight gain, and hypertension) 21 , 22 , 23 , and maternal dietary factors (such as folic acid and other supplement use, maternal iron (Fe) intake, as well as maternal vitamin D levels) 24 , 25 , 26 , 27 , 28 , 29 .
Numerous studies indicate that an increased risk of ASD is linked to several perinatal and neonatal factors. These factors include small gestational age or preterm birth, gestational small or large size, the use of labor and delivery drugs 30 , 31 , 32 , 33 . The risk of ASD associated with cesarean delivery is also a subject of continuous discussion 34 , 35 , 36 . Overall, there is no apparent link between assisted conception and a notably higher risk of ASD, however some particular therapies might make ASD more likely.
This study aimed to determine main pre-, peri- and neonatal risk factors linked to ASD among Armenian children. The following research questions were derived to address the objectives of the study:
What are the primary prenatal risk factors associated with the development of ASD among Armenian children?
How do perinatal factors such as maternal complications during childbirth, labor mode, labor interventions, use of labor-inducing drugs, contribute to the risk of ASD in Armenian children?
What neonatal factors, such as birth weight and gestational age, are linked to the likelihood of ASD diagnosis among Armenian children?
How do socio-demographic factors, such as parental education, gender of the child, number of kids in the family, sequence of the kid, influence the relationship between pre-, peri-, and neonatal risk factors and risk of ASD among Armenian children?
To the best of our knowledge, this was the first study of its kind conducted in Armenia that focused on a variety of factors linked to ASD.
The analysis encompassed a total sample of 497 participants, consisting of 168 children diagnosed with ASD and 329 children without ASD. The descriptive analysis revealed significant differences between the cases and controls on several socio demographic variables as well as prenatal, peri- and neonatal risk factors (see Tables 1 , 2 , 3 and 4 ).
The summary of socio demographic characteristics (Table 1 ). Among the cases (the ASD group), the distribution of gender of the child was significantly different to that in the control group. More specifically, while the distribution of male and female were balanced in the control group (52.89% and 47.11% respectively), the proportion of male children was significantly higher in the ASD group (82.14% and 17.86% respectively, p < 0.01). Furthermore, the number of children in the families of cases and controls were slightly different. While the proportion of cases and controls who had two children were similar, families with one child were slightly higher in the ASD group compared to the control group (29.94% and 17.02% respectively, p < 0.01). This picture is reversed with respect to the number of families with more than two children (16.17% and 29.79% respectively). A higher percentage of ASD cases are the first child in the family compared to controls (67.86% vs. 49.54%, p < 0.01). The proportion of non-married families (those that reported to be single, widowed, divorced etc.) were higher in the ASD group compared to the control group (10.24% and 4.28% respectively, p < 0.05). The distribution of the level of educational attainment of the parents were also different between the groups. More specifically, the prevalence of university degree among the cases were somewhat lower compared to that in the control group.
The summary of prenatal risk factors (Table 2 ). With respect to prenatal risk factors, there were significant differences between the cases and controls in interpregnancy intervals, self-reported complications and diseases, medication use, vitamin D levels, maternal weight gain, and the self-reported stress during pregnancy. More specifically, the cases had on average lower interpregnancy intervals compared to the controls (M = 12.9 and M = 23.7 months respectively, p < 0.01). The cases more frequently reported to have had complications during the pregnancy compared to the controls (42.86% and 8.54% respectively, p < 0.01). The prevalence of reported infectious diseases, other diseases and anemia during the pregnancy were also somewhat higher among the cases compared to the control group. The use of medications was higher among the cases compared to the control group (41.67% and 17.74% respectively, p < 0.01). Various medications including vitamins, anticoagulants, Paracetamol, MgB6, Duphaston, iron preparation, No-spa, calcium preparation, antibiotics, and Utrogestan showed significant differences in usage between cases and controls (all p < 0.05) (Table 3 ). The maternal weight gain among the cases was on average higher among the cases compared to the control group (M = 15.4 and M = 13.9 kg respectively, p < 0.05). The self-reported stress was also more frequent among the cases compared to the controls (56.02 and 10.98% respectively, p < 0.01). Specifically, comparing data on self-reported stress during different pregnancy periods, it was obvious that 47.06% of mothers of cases and 91.25% of mothers in the control group reported no stress experienced during pregnancy. During the first trimester, 14.38% of mothers with cases of autism reported stress, whereas only 0.94% of mothers in the control group reported stress. In the second trimester, 11.11% of mothers with cases of autism reported stress, compared to 4.06% of mothers in the control group. During the third trimester, 8.50% of mothers with cases of autism reported stress, while 1.88% of mothers in the control group reported stress. Across the entire pregnancy, 18.95% of mothers with cases of autism reported stress, compared to 1.88% of mothers in the control group. The differences in stress levels between the two groups were statistically significant, indicating a potential link between maternal stress during pregnancy and the odds of autism spectrum disorder in offspring.
The summary of perinatal and neonatal risk factors (Table 4 ). The interpretation of the data comparing various peri- and neonatal risk factors between cases (individuals with ASD) and controls (individuals without ASD) are shown below. 83.33% of cases and 91.77% of controls were born within 37–42 weeks of gestation, with a statistically significant difference ( p < 0.05), whereas 16.67% of cases and 8.23% of controls were born either preterm (before 37 weeks) or post-term (after 42 weeks), also showing a significant difference. There was no statistically significant difference in birth weight between cases and controls (M = 3137.8 and M = 3176.9 g respectively, p > 0.05). The mean birth height was slightly lower for cases compared to controls (M = 50.4 and M = 50.9 cm), with a statistically significant difference ( p < 0.05). No statistically significant difference was reported regarding mode of labor. According to the data interventions during labor were reported more in ASD group compared to controls (39.76% and 17.23% respectively, p < 0.01). Also, labor-inducing drugs were administered more in cases compared to the controls (39.76% and 21.04%, p < 0.01).
The results of multivariable logistic regression
The multivariable logistic regression analysis indicated significant associations between sociodemographic, prenatal, perinatal and neonatal risk factors. More specifically, male children have 4 times higher odds of having ASD compared to female children (OR = 4.21, CI 2.33–7.63). Among prenatal factors, the maternal weight gain, use of MgB6, the self-reported stress during the pregnancy, as well as pregnancy with complications were associated with a significant increase in the odds of ASD, whereas use of Duphaston was associated with decreased odds of ASD (see Table 5 ). Additionally, the longer interpregnancy interval was associated with decreased odds of ASD diagnosis (OR = 0.708, CI 0.52–0.97). Among peri- and neonatal factors, use of labor-inducing drugs was associated with increase in the odds of ASD diagnosis (OR = 2.295, CI 1.3–4.1), while birth height showed association with decrease in odds (OR = 0.788, CI 0.6–1.0).
Our study provides comprehensive insights into the multifaceted nature of ASD, elucidating the intricate relationships between sociodemographic, prenatal, perinatal, and neonatal factors and ASD risk.
Our findings highlight significant gender disparities in ASD prevalence, with a notably higher proportion (4:1) of male children in the ASD group. This aligns with existing literature demonstrating a male predominance in ASD diagnosis 37 . Meanwhile, Loomes et al. reported 3:1 male-to-female ratio referring to a diagnostic gender bias, where girls meeting the criteria for ASD are at an elevated risk of not receiving a clinical diagnosis 38 . Furthermore, our study highlights the potential impact of family structure on the likelihood of ASD occurrence, indicating higher ASD rates among first-born children and in households where the parents are non-married (divorced, widowed, separated, etc.). A study conducted by Ugur et al. yielded comparable findings, suggesting that the prevalence of being the eldest child was higher in the ASD group compared to the control group 39 . Contrary to this, research conducted in the United States found no evidence to suggest that children diagnosed with ASD are more likely to live in households not composed of both their biological or adoptive parents compared to children without ASD 40 .
The association between prenatal risk factors and ASD risk underscores the importance of maternal health during pregnancy. Our findings suggest that factors such as lower IPIs, maternal complications and diseases during pregnancy, medication use, vitamin D levels, maternal weight gain and maternal self-reported stress during pregnancy may increase the odds of ASD in offspring. It is crucial to note that the higher number of firstborn children among cases compared to the control group could introduce bias when accurately estimating the association between IPI and ASD. Therefore, the coefficients of IPI should be interpreted cautiously. Despite this, we opted not to remove this variable from the model, as IPI is recognized as an important factor in existing literature. Several studies report different results regarding long and short IPIs and ASD risk 14 , 15 , 41 , 42 . The underlying reasons for the link between ASD and short and long IPIs may differ. Short IPIs could be associated with maternal nutrient depletion, stress, infertility, and inflammation, whereas long IPIs may be linked to infertility and related complications. According to our results the frequency of self-reported complications during pregnancy was notably higher among children with ASD compared to the controls. Additionally, there was a somewhat higher prevalence of reported infectious diseases, other illnesses, and anemia during pregnancy among the cases compared to the control group. Several previous investigations have associated maternal hospitalization resulting from infection during pregnancy with an elevated risk of ASD. This includes a substantial study involving over two million individuals, which indicated an increased risk associated with viral and bacterial infections during the prenatal period 12 , 17 . Furthermore, our study results indicate that medication usage during pregnancy was more common among the mothers of cases than the controls. Notably, various medications, including vitamins, anticoagulants, Paracetamol, MgB6, Duphaston, iron preparation, No-spa, calcium preparation, antibiotics, and Utrogestan (micronized progesterone), exhibited significant differences in usage between the cases and controls. According to the results of multiple logistic regression analysis use of MgB6 was associated with a significant increase in the odds of ASD, whereas use of Duphaston (Dydrogesterone), a progestin medication, was associated with decreased odds of ASD. Emphasizing the potential impact of additional variables in evaluating the link between Duphaston and ASD is essential. There is a possibility of factors overlooked in our study. However, after analyzing the included variables, no significant confounding effects were detected. This assessment involved scrutinizing the Cramer’s V value between Duphaston and other variables, and sequentially introducing new variables into the model to evaluate changes in the coefficients of Duphaston. In both cases, no significant confounding effects emerged. In contrast to our results certain researchers have shown that the use of supplements during pregnancy is linked to a decreased risk of ASD in offsprings compared to those whose mothers did not take supplements during pregnancy 43 , 44 . The results of an epidemiology study conducted by Li et al. have showed that prenatal progestin exposure was strongly associated with ASD prevalence, and the experiments in rats showed that prenatal consumption of progestin-contaminated seafood induced autism-like behavior 45 . On the other hand other authors suggest that insufficient maternal progesterone levels might contribute to both obstetrical complications and ASD development 46 . The observed association regarding MgB6 use could potentially be influenced by an unmeasured confounding variable in our study. This warrants further investigation and consideration in future research. Additionally, our study highlighted another modifiable risk factor for ASD that was significantly associated with higher odds of ASD: maternal gestational weight gain. This factor retained its significance even in the multivariable analysis. This finding is consistent with the results of several studies which have shown that maternal metabolic conditions like diabetes, gestational weight gain, and hypertension have been associated with mechanisms pertinent to ASD, such as oxidative stress, fetal hypoxia, and chronic inflammation 23 , 47 . These conditions can induce prolonged or acute hypoxia in the fetus, which might pose a substantial risk factor for neurodevelopmental disturbances.
Furthermore, our findings suggest that the self-reported stress during pregnancy was associated with a significant increase in the odds of ASD. When comparing self-reported stress levels during different pregnancy periods, a notable disparity emerged. The statistically significant differences in stress levels between the two groups were reported in all trimesters of pregnancy suggesting a potential correlation between maternal stress during pregnancy and the odds of autism spectrum disorder in offspring. Several authors report comparable findings suggesting that prenatal maternal stress show significant association with both autistic traits and Attention Deficit Hyperactivity Disorder (ADHD) behaviors 48 , 49 , 50 , 51 . Various mechanisms could be suggested to explain the link between prenatal stress and likelihood of ASD. For instance, stress during pregnancy can trigger physiological changes in the mother's body, such as increased cortisol levels and alterations in immune function, which may impact fetal development and contribute to the risk of ASD. Also, stress may affect placental function, leading to adverse changes in the transfer of nutrients and oxygen to the fetus. Furthermore, prenatal stress can influence gene expression in both the mother and the developing fetus. Certain genes involved in brain development and the stress response system may be affected, potentially increasing the risk of ASD. Additionally, maternal stress may influence parenting behaviors and interactions with the child after birth. High levels of maternal stress may affect the quality of caregiving, which in turn can impact the child's social and emotional development, potentially contributing to ASD risk. Lastly, stress during pregnancy could induce epigenetic modifications, which are alterations in gene expression that occur without changes in DNA sequence. These modifications might affect neurodevelopmental processes, making individuals more susceptible to ASD.
Our study reports notable statistical difference among cases and controls regarding gestational age. According to the results 16.67% of cases were born either preterm (before 37 weeks) or post-term (after 42 weeks), highlighting another significant distinction. Early gestational age is linked with unfavorable health consequences, such as developmental delays and subsequent intellectual impairments throughout childhood and adolescence. Similar results are reported by several authors as well 30 , 31 , 52 . According to our study results birth height was associated with decrease in odds of ASD, however there was no statistically significant difference in birth weight between cases and controls. Some authors demonstrated that infants with birth weights of < 2.5 kg were associated with ADHD and ASD 53 , 54 .
Other than the previously mentioned factors the results of our study have demonstrated that use of labor-inducing drugs was associated with increase in the odds of ASD. The study participants did not report or specify the type of used labor-inducing drugs. Recent investigations have indicated a potential correlation between the utilization of drugs during labor and delivery and the emergence of ASD 55 , 56 , especially given the increased usage of epidurals and labor-inducing medications in the past 30 years. However, conflicting findings exist, with some studies suggesting no link between the administration of labor-inducing drugs and the risk of ASD development 57 , 58 . Recent findings from Qiu et al. propose a potential link between maternal labor epidural analgesia and the risk of ASD in children, particularly when oxytocin was concurrently administered. However, oxytocin exposure in the absence of labor epidural analgesia did not show an association with ASD risk in children 59 . The potential link between the use of labor-inducing drugs and the risk of ASD is complex and not yet fully understood. However, several mechanisms have been proposed to explain this association: for example, labor-inducing drugs, such as oxytocin and prostaglandins, can affect hormonal levels in both the mother and the fetus. These hormonal changes may impact brain development and neural connectivity, potentially increasing the risk of ASD. In addition, labor induction may increase the risk of oxygen deprivation (hypoxia) during labor. Prolonged hypoxia during birth has been linked to adverse neurological outcomes, including an increased risk of neurodevelopmental disorders like ASD. Furthermore, labor induction can lead to an inflammatory response in both the mother and the fetus. This immune system activation may affect neurodevelopmental processes and contribute to the development of ASD. Overall, the relationship between labor-inducing drugs and ASD risk is multifactorial and likely involves interactions between genetic, environmental, and biological factors. Further research is needed to elucidate the specific mechanisms underlying this association.
To our knowledge this is the first study identifying potential pre-, peri- and neonatal risk factors associated with ASD in Armenia.
Limitations and strength
While our study possesses a retrospective design, a notable limitation, it depended on parental recall for details dating back several years. Additionally, the sample was not balanced in terms of gender, with more male children included, potentially introducing selection bias. Despite the relatively small sample size multivariable analysis using the presence or absence of ASD as the dependent variable was implemented to address potential confounding factors.
Nevertheless, our study included a representative sample of the Armenian ASD population, evaluating various factors in comparison with a randomly selected control group matched for age. All ASD diagnoses were made according to DSM-5 by professionals (psychiatrists, pediatricians, neurologists, speech therapists and developmental psychologists), and face-to-face interviews with parents at the time of the interview minimized the risk of information bias.
Our findings indicated that male gender, maternal weight gain, MgB6 usage, self-reported stress during pregnancy, pregnancy complications, and labor-inducing drugs were linked to a significant rise in ASD odds (Fig. 1 ). Conversely, the use of Duphaston during pregnancy, longer interpregnancy intervals, and higher birth height were associated with reduced odds of ASD (Fig. 1 ). These observations underpin the significance of regional investigations to uncover the unique environmental factors contributing to ASD. The implications are profound, as several identified factors may be preventable or adjustable, highlighting the urgency of implementing evidence-based practices and public health interventions. Emphasizing a culture of health promotion, screenings, timely diagnosis, and disease prevention strategies, particularly among pregnant women, holds promise for reducing ASD and related disorders. Moreover, further prospective and focused research is imperative to discern the interplay between various factors and gene-environment interactions that may serve as potential ASD risk factors. Enhanced understanding in this area could lead to earlier detection and improved ASD management. Future studies incorporating analyses of biological samples for genetic, epigenetic, and inflammatory markers will be pivotal in elucidating underlying mechanisms and ushering in a new phase of research focusing on modifiable risk factors for developmental disorders.
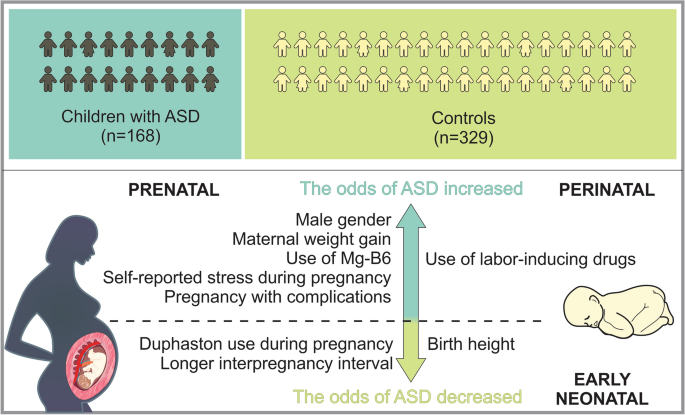
Sum up scheme showing prenatal, perinatal and neonatal factors which increase, as well as decrease the odds of ASD.
The study population comprised of 497 participants, of which 168 were children with ASD and 329 were typical development controls. The subject recruitment was done during 2021 to 2022. The controls and children with ASD were age matched (3–18 years). The subjects were formally diagnosed with ASD according to DSM-5 by professionals (pediatricians, neurologists, speech therapists and developmental psychologists). The children with ASD were recruited at MY WAY Educational and Rehabilitation Center in Yerevan. Inclusion criteria for cases were age between 3 and 18 years with ASD with diagnosis confirmed using DSM-V. Exclusion criteria for cases were other neurodevelopment disorders other than ASD. The control participants were randomly selected in the same period at Muratsan Hospital Complex. They were not known to have any neurodevelopmental or behavioral disruptions that might be related to ASD. The control group consisted exclusively of individuals diagnosed with simple conditions like flu or a simple routine physical examination.
Questionnaire and data collection
The self-reported questionnaire was completed via a face-to-face interview with the child’s parent. The questionnaire comprised three sections: various aspects of sociodemographic characteristics, prenatal risk factors, and perinatal/neonatal risk factors. Each section addressed specific questions related to these factors for comprehensive data collection. On average, the questionnaire was completed in about 15 to 20 min. The parent had the choice of accepting or refusing to complete the questionnaire. At the end of the process, the completed questionnaires were collected and sent for data entry by using SPSS 21 statistical software. The questionnaire was designed to acquire the information regarding risk factors of ASD. It consisted of different sections: sociodemographic characteristics (e.g., age, sex, family history of ASD, etc.), data on prenatal risk factors (e.g., pregnancy process, complications during pregnancy, infections during pregnancy, other diseases, stress, medication and supplement use during pregnancy, vitamin D level, etc.), questions related to maternal lifestyle risk factors (e.g., smoking, alcohol consumption, gestational weight gain). Respondents were also asked about peri- and neonatal risk factors, including gestational age, gestational size, the use of labor and delivery drugs, mode of delivery, etc.
Ethics disclosure
The study protocol was approved by the Ethics Committee (N 8–2/20; 27.11.2020) of Yerevan State Medical University in line with the principles set forth in the Declaration of Helsinki. Written informed consent was obtained from all the parents of the participants prior to data collection.
Statistical analysis
The data was processed and modelled in Python (version 3.11.6), an open- source software often used for data processing and modelling. The statistical analysis was performed by using the Statsmodels package (version 0.14.1) 60 .
Descriptive statistics were employed to summarize the characteristics of the study variables. Continuous variables were described using means and standard deviations, while categorical variables were presented as proportions. Prior to statistical analysis, numeric variables underwent standardization through the computation of z scores. To evaluate potential multicollinearity among predictor variables, the Variable Inflation Index (VIF) was computed. A predetermined threshold of 5 was established, and variables exceeding this threshold were considered indicative of multicollinearity. Bivariate analyses were conducted to explore associations between predictor variables and the outcome variable (presence or absence of autism diagnosis). T-tests were employed for continuous variables, while chi-squared tests were utilized for nominal and categorical variables. A multivariable logistic regression model was constructed to estimate the relationship between predictor variables and the outcome variable (autism diagnosis). Initially, a comprehensive strategy was employed by first fitting a null model and then iteratively introducing blocks of variables (e.g., socio demographic factors prenatal, peri- and neonatal factors). After introducing the new block of factors, a likelihood ratio test was conducted to evaluate the contribution of the added variables. If the p -value associated with the likelihood ratio test was insignificant ( p > 0.05), the preference was given to a less complex model. In our analysis all added blocks had significant contribution to the overall fit of the model.
Subsequently, the significance of each variable was systematically assessed by applying a stepwise elimination technique whereby insignificant variables were progressively removed from the model. Similar to the above-mentioned process, at each step, a likelihood ratio test to evaluate the significance of the excluded variable was conducted. If the p -value associated with this test was found to be insignificant ( p > 0.05), the adoption of a simpler model was favored. This iterative process allowed us to identify the most parsimonious model that retained statistically significant predictors while minimizing unnecessary complexity. Odds ratios (ORs) were computed, accompanied by 95% confidence intervals (CIs), to quantify the strength and direction of these associations.
Data availability
Data can be made available by the corresponding author upon reasonable request.
Diagnostic and Statistical Manual of Mental Disorders: DSM-5 TM , 5th Ed . xliv, 947 (American Psychiatric Publishing, Inc., Arlington, VA, US, 2013). https://doi.org/10.1176/appi.books.9780890425596 .
Zeidan, J. et al. Global prevalence of autism: A systematic review update. Autism Res. 15 , 778–790 (2022).
Article PubMed PubMed Central Google Scholar
CDC. Autism and Developmental Disabilities Monitoring (ADDM) Network. Centers for Disease Control and Prevention https://www.cdc.gov/ncbddd/autism/addm.html (2023).
CDC. Data and Statistics on Autism Spectrum Disorder | CDC. Centers for Disease Control and Prevention https://www.cdc.gov/ncbddd/autism/data.html (2024).
Tick, B., Bolton, P., Happé, F., Rutter, M. & Rijsdijk, F. Heritability of autism spectrum disorders: A meta-analysis of twin studies. J. Child Psychol. Psychiatry 57 , 585–595 (2016).
Article PubMed Google Scholar
Lord, C., Elsabbagh, M., Baird, G. & Veenstra-Vanderweele, J. Autism spectrum disorder. Lancet 392 , 508–520 (2018).
Muhle, R. A., Reed, H. E., Stratigos, K. A. & Veenstra-VanderWeele, J. The emerging clinical neuroscience of autism spectrum disorder: A review. JAMA Psychiatry 75 , 514–523 (2018).
Bai, D. et al. Association of genetic and environmental factors with autism in a 5-country cohort. JAMA Psychiatry 76 , 1035–1043 (2019).
Jutla, A., Reed, H. & Veenstra-VanderWeele, J. The architecture of autism spectrum disorder risk: What do we know, and where do we go from here?. JAMA Psychiatry 76 , 1005–1006 (2019).
Gardener, H., Spiegelman, D. & Buka, S. L. Perinatal and neonatal risk factors for autism: A comprehensive meta-analysis. Pediatrics 128 , 344–355 (2011).
Guinchat, V. et al. Pre-, peri- and neonatal risk factors for autism. Acta Obstet. Gynecol. Scand. 91 , 287–300 (2012).
Yenkoyan, K., Mkhitaryan, M. & Bjørklund, G. Environmental risk factors in autism spectrum disorder: A narrative review. Curr. Med. Chem. https://doi.org/10.2174/0109298673252471231121045529 (2024).
Lyall, K. et al. The association between parental age and autism-related outcomes in children at high familial risk for autism. Autism. Res. 13 , 998–1010 (2020).
Cheslack-Postava, K. et al. Increased risk of autism spectrum disorders at short and long interpregnancy intervals in Finland. J. Am. Acad. Child Adolesc. Psychiatry 53 , 1074-1081.e4 (2014).
Zerbo, O., Yoshida, C., Gunderson, E. P., Dorward, K. & Croen, L. A. Interpregnancy interval and risk of autism spectrum disorders. Pediatrics 136 , 651–657 (2015).
Lee, B. K. et al. Maternal hospitalization with infection during pregnancy and risk of autism spectrum disorders. Brain Behav. Immun. 44 , 100–105 (2015).
Zerbo, O. et al. Maternal infection during pregnancy and autism spectrum disorders. J. Autism Dev. Disord. 45 , 4015–4025 (2015).
Christensen, J. et al. Prenatal valproate exposure and risk of autism spectrum disorders and childhood autism. JAMA 309 , 1696–1703 (2013).
Article CAS PubMed PubMed Central Google Scholar
Bromley, R. L. et al. The prevalence of neurodevelopmental disorders in children prenatally exposed to antiepileptic drugs. J. Neurol. Neurosurg. Psychiatry 84 , 637–643 (2013).
Gidaya, N. B. et al. In utero exposure to β-2-adrenergic receptor agonist drugs and risk for autism spectrum disorders. Pediatrics 137 , e20151316 (2016).
Walker, C. K. et al. Preeclampsia, placental insufficiency, and autism spectrum disorder or developmental delay. JAMA Pediatr 169 , 154–162 (2015).
Krakowiak, P. et al. Maternal metabolic conditions and risk for autism and other neurodevelopmental disorders. Pediatrics 129 , e1121-1128 (2012).
Li, M. et al. The association of maternal obesity and diabetes with autism and other developmental disabilities. Pediatrics 137 , e20152206 (2016).
Black, M. M. Effects of vitamin B12 and folate deficiency on brain development in children. Food Nutr. Bull. 29 , S126-131 (2008).
Surén, P. et al. Association between maternal use of folic acid supplements and risk of autism spectrum disorders in children. JAMA 309 , 570–577 (2013).
Skalny, A. V. et al. Magnesium status in children with attention-deficit/hyperactivity disorder and/or autism spectrum disorder. Soa Chongsonyon Chongsin Uihak 31 , 41–45 (2020).
PubMed PubMed Central Google Scholar
Virk, J. et al. Preconceptional and prenatal supplementary folic acid and multivitamin intake and autism spectrum disorders. Autism 20 , 710–718 (2016).
Whitehouse, A. J. O. et al. Maternal vitamin D levels and the autism phenotype among offspring. J. Autism Dev. Disord. 43 , 1495–1504 (2013).
Zhong, C., Tessing, J., Lee, B. & Lyall, K. Maternal dietary factors and the risk of autism spectrum disorders: A systematic review of existing evidence. Autism Res. 13 , 1634–1658 (2020).
Moore, G. S., Kneitel, A. W., Walker, C. K., Gilbert, W. M. & Xing, G. Autism risk in small- and large-for-gestational-age infants. Am. J. Obstet. Gynecol. 206 (314), e1-9 (2012).
Google Scholar
Leavey, A., Zwaigenbaum, L., Heavner, K. & Burstyn, I. Gestational age at birth and risk of autism spectrum disorders in Alberta, Canada. J. Pediatr. 162 , 361–368 (2013).
Smallwood, M. et al. Increased risk of autism development in children whose mothers experienced birth complications or received labor and delivery drugs. ASN Neuro. 8 , 1759091416659742 (2016).
Qiu, C. et al. Association between epidural analgesia during labor and risk of autism spectrum disorders in offspring. JAMA Pediatr. 174 , 1168–1175 (2020).
Curran, E. A. et al. Association between obstetric mode of delivery and autism spectrum disorder: A population-based sibling design study. JAMA Psychiatry 72 , 935–942 (2015).
Curran, E. A. et al. Research review: Birth by caesarean section and development of autism spectrum disorder and attention-deficit/hyperactivity disorder: A systematic review and meta-analysis. J. Child Psychol. Psychiatry 56 , 500–508 (2015).
Nagano, M. et al. Cesarean section delivery is a risk factor of autism-related behaviors in mice. Sci. Rep. 11 , 8883 (2021).
Article ADS CAS PubMed PubMed Central Google Scholar
Werling, D. M. & Geschwind, D. H. Sex differences in autism spectrum disorders. Curr. Opin. Neurol. 26 , 146–153 (2013).
Loomes, R., Hull, L. & Mandy, W. P. L. What is the male-to-female ratio in autism spectrum disorder? A systematic review and meta-analysis. J. Am. Acad. Child Adolesc. Psychiatry 56 , 466–474 (2017).
Ugur, C., Tonyali, A., Goker, Z. & Uneri, O. S. Birth order and reproductive stoppage in families of children with autism spectrum disorder. Psychiatry Clin. Psychopharmacol. 29 , 509–514 (2019).
Article Google Scholar
Freedman, B. H., Kalb, L. G., Zablotsky, B. & Stuart, E. A. Relationship status among parents of children with autism spectrum disorders: A population-based study. J. Autism. Dev. Disord. 42 , 539–548 (2012).
Gunnes, N. et al. Interpregnancy interval and risk of autistic disorder. Epidemiology 24 , 906–912 (2013).
Durkin, M. S., Allerton, L. & Maenner, M. J. Inter-pregnancy intervals and the risk of autism spectrum disorder: Results of a population-based study. J. Autism. Dev. Disord. 45 , 2056–2066 (2015).
Levine, S. Z. et al. Association of maternal use of folic acid and multivitamin supplements in the periods before and during pregnancy with the risk of autism spectrum disorder in offspring. JAMA Psychiatry 75 , 176–184 (2018).
Article ADS PubMed PubMed Central Google Scholar
Schmidt, R. J., Iosif, A.-M., Guerrero Angel, E. & Ozonoff, S. Association of maternal prenatal vitamin use with risk for autism spectrum disorder recurrence in young siblings. JAMA Psychiatry 76 , 391–398 (2019).
Li, L. et al. Prenatal progestin exposure is associated with autism spectrum disorders. Front Psychiatry 9 , 611 (2018).
Whitaker-Azmitia, P. M., Lobel, M. & Moyer, A. Low maternal progesterone may contribute to both obstetrical complications and autism. Med Hypotheses 82 , 313–318 (2014).
Article CAS PubMed Google Scholar
Vecchione, R. et al. Maternal dietary patterns during pregnancy and child autism-related traits: Results from two US cohorts. Nutrients 14 , 2729 (2022).
Ronald, A., Pennell, C. & Whitehouse, A. Prenatal maternal stress associated with ADHD and autistic traits in early childhood. Front. Psychol. https://doi.org/10.3389/fpsyg.2010.00223 (2011).
Caparros-Gonzalez, R. A. et al. Stress during pregnancy and the development of diseases in the offspring: A systematic-review and meta-analysis. Midwifery 97 , 102939 (2021).
Manzari, N., Matvienko-Sikar, K., Baldoni, F., O’Keeffe, G. W. & Khashan, A. S. Prenatal maternal stress and risk of neurodevelopmental disorders in the offspring: A systematic review and meta-analysis. Soc. Psychiatry Psychiatr. Epidemiol. 54 , 1299–1309 (2019).
Say, G. N., Karabekiroğlu, K., Babadağı, Z. & Yüce, M. Maternal stress and perinatal features in autism and attention deficit/hyperactivity disorder. Pediatr. Int. 58 , 265–269 (2016).
Abel, K. M. et al. Deviance in fetal growth and risk of autism spectrum disorder. Am. J. Psychiatry 170 , 391–398 (2013).
Song, I. G. et al. Association between birth weight and neurodevelopmental disorders assessed using the Korean National Health Insurance Service claims data. Sci. Rep. 12 , 2080 (2022).
Lampi, K. M. et al. Risk of autism spectrum disorders in low birth weight and small for gestational age infants. J. Pediatr. 161 , 830–836 (2012).
Bashirian, S., Seyedi, M., Razjouyan, K. & Jenabi, E. The association between labor induction and autism spectrum disorders among children: A meta-analysis. Curr. Pediatr. Rev. 17 , 238–243 (2021).
Oberg, A. S. et al. Association of labor induction with offspring risk of autism spectrum disorders. JAMA Pediatr. 170 , e160965 (2016).
Karim, J. L. et al. Exogenous oxytocin administration during labor and autism spectrum disorder. Am. J. Obstet. Gynecol. MFM 5 , 101010 (2023).
Qiu, C. et al. Association of labor epidural analgesia, oxytocin exposure, and risk of autism spectrum disorders in children. JAMA Netw. Open 6 , e2324630 (2023).
Seabold, S. & Perktold, J. Statsmodels: Econometric and Statistical Modeling with Python. Proceedings of the 9th Python in Science Conference 92–96 (2010) https://doi.org/10.25080/Majora-92bf1922-011 .
Download references
Acknowledgements
We express our gratitude to all parents participated in the study.
This work was supported by Higher Education and Science Committee, Ministry of Education, Science, Culture and Sports of RA (24YSMU-CON-I-3AN and 23LCG-3A020), and YSMU.

Author information
Authors and affiliations.
Neuroscience Laboratory, Cobrain Center, Yerevan State Medical University Named After M. Heratsi, 0025, Yerevan, Armenia
Meri Mkhitaryan & Konstantin Yenkoyan
Cobrain Center, Yerevan State Medical University Named After M. Heratsi, 0025, Yerevan, Armenia
Tamara Avetisyan & Larisa Avetisyan
Muratsan University Hospital Complex, Yerevan State Medical University Named After M. Heratsi, 0075, Yerevan, Armenia
Tamara Avetisyan
Department of Infectious Diseases, Yerevan State Medical University Named After M. Heratsi, 0025, Yerevan, Armenia
Anna Mkhoyan
Department of Hygiene, Yerevan State Medical University Named After M. Heratsi, 0025, Yerevan, Armenia
Larisa Avetisyan
You can also search for this author in PubMed Google Scholar
Contributions
M.M. and K.Y. conceived the project; M.M., T.A., A.M., L.A. and K.Y. designed experiments, collected and analyzed data; M.M., T.A., A.M., L.A., and K.Y. wrote the draft; M.M., A.M., and K.Y. edited the manuscript. K.Y. obtained funding and supervised the study. All authors have read and agreed to the published version of the manuscript.
Corresponding author
Correspondence to Konstantin Yenkoyan .
Ethics declarations
Competing interests.
The authors declare no competing interests.
Additional information
Publisher's note.
Springer Nature remains neutral with regard to jurisdictional claims in published maps and institutional affiliations.
Rights and permissions
Open Access This article is licensed under a Creative Commons Attribution 4.0 International License, which permits use, sharing, adaptation, distribution and reproduction in any medium or format, as long as you give appropriate credit to the original author(s) and the source, provide a link to the Creative Commons licence, and indicate if changes were made. The images or other third party material in this article are included in the article's Creative Commons licence, unless indicated otherwise in a credit line to the material. If material is not included in the article's Creative Commons licence and your intended use is not permitted by statutory regulation or exceeds the permitted use, you will need to obtain permission directly from the copyright holder. To view a copy of this licence, visit http://creativecommons.org/licenses/by/4.0/ .
Reprints and permissions
About this article
Cite this article.
Mkhitaryan, M., Avetisyan, T., Mkhoyan, A. et al. A case–control study on pre-, peri-, and neonatal risk factors associated with autism spectrum disorder among Armenian children. Sci Rep 14 , 12308 (2024). https://doi.org/10.1038/s41598-024-63240-3
Download citation
Received : 02 March 2024
Accepted : 27 May 2024
Published : 29 May 2024
DOI : https://doi.org/10.1038/s41598-024-63240-3
Share this article
Anyone you share the following link with will be able to read this content:
Sorry, a shareable link is not currently available for this article.
Provided by the Springer Nature SharedIt content-sharing initiative
- Autism spectrum disorder
- Case–control study
By submitting a comment you agree to abide by our Terms and Community Guidelines . If you find something abusive or that does not comply with our terms or guidelines please flag it as inappropriate.
Quick links
- Explore articles by subject
- Guide to authors
- Editorial policies
Sign up for the Nature Briefing newsletter — what matters in science, free to your inbox daily.

Candidate Genes from an FDA-Approved Algorithm Fail to Predict Opioid Use Disorder Risk in Over 450,000 Veterans
- PMID: 38798430
- PMCID: PMC11118646
- DOI: 10.1101/2024.05.16.24307486
Importance: Recently, the Food and Drug Administration gave pre-marketing approval to algorithm based on its purported ability to identify genetic risk for opioid use disorder. However, the clinical utility of the candidate genes comprising the algorithm has not been independently demonstrated.
Objective: To assess the utility of 15 variants in candidate genes from an algorithm intended to predict opioid use disorder risk.
Design: This case-control study examined the association of 15 candidate genetic variants with risk of opioid use disorder using available electronic health record data from December 20, 1992 to September 30, 2022.
Setting: Electronic health record data, including pharmacy records, from Million Veteran Program participants across the United States.
Participants: Participants were opioid-exposed individuals enrolled in the Million Veteran Program (n = 452,664). Opioid use disorder cases were identified using International Classification of Disease diagnostic codes, and controls were individuals with no opioid use disorder diagnosis.
Exposures: Number of risk alleles present across 15 candidate genetic variants.
Main outcome and measures: Predictive performance of 15 genetic variants for opioid use disorder risk assessed via logistic regression and machine learning models.
Results: Opioid exposed individuals (n=33,669 cases) were on average 61.15 (SD = 13.37) years old, 90.46% male, and had varied genetic similarity to global reference panels. Collectively, the 15 candidate genetic variants accounted for 0.4% of variation in opioid use disorder risk. The accuracy of the ensemble machine learning model using the 15 genes as predictors was 52.8% (95% CI = 52.1 - 53.6%) in an independent testing sample.
Conclusions and relevance: Candidate genes that comprise the approved algorithm do not meet reasonable standards of efficacy in predicting opioid use disorder risk. Given the algorithm's limited predictive accuracy, its use in clinical care would lead to high rates of false positive and negative findings. More clinically useful models are needed to identify individuals at risk of developing opioid use disorder.
Key points: Question: How well do candidate genes from an algorithm designed to predict risk of opioid use disorder, which recently received pre-marketing approval by the Food and Drug Administration, perform in a large, independent sample? Findings: In a case-control study of over 450,000 individuals, the 15 genetic variants from candidate genes collectively accounted for 0.4% of the variation in opioid use disorder risk. In this independent sample, the SNPs predicted risk at a level of accuracy near random chance (52.8%). Meaning: Candidate genes from the approved genetic risk algorithm do not meet standards of reasonable clinical efficacy in assessing risk of opioid use disorder.
Publication types
Case-control study to identify management practices associated with morbidity or mortality due to bovine anaplasmosis in Mississippi cow-calf herds
- W. Isaac Jumper Department of Pathobiology and Population Medicine, College of Veterinary Medicine, Mississippi State University, MS 39762
- Carla L. Huston Department of Pathobiology and Population Medicine, College of Veterinary Medicine, Mississippi State University, MS 39762
- David R. Smith Department of Pathobiology and Population Medicine, College of Veterinary Medicine, Mississippi State University, MS 39762
Bovine anaplasmosis (BA) is a costly disease affecting the U.S. beef cattle industry. Chlortetracycline (CTC) in feed or mineral supplements is often used to control clinical signs of BA. The objective of this study was to determine if management practices, such as feeding CTC, are associated with illness or death from BA in Mississippi cow-calf herds. Case and control herds were solicited from veterinary practices across Mississippi. Cases were herds with clinical BA diagnosed by a veterinarian within the previous calendar year. Controls were herds under the care of the same practice with no clinical BA diagnosed in the previous year. Blinded interviewers conducted telephone surveys of case and control herd owners. Management and biosecurity factors were tested for association with herd status using a logistic regression generalized linear mixed model with veterinary practice as a random variable. Twenty-two case and 25 control herds from 6 veterinary practices across Mississippi were interviewed, representing 22 counties. The average herd size was 132 for case herds, and 136 for control herds. Twenty case herds and 13 control herds fed CTC medicated mineral or feed. Providing CTC was associated with case herd status (OR = 9.2, 95% C.I. = 1.7-50.7). The association observed between case herds and feeding CTC might be because: 1) herds that had experienced previous BA morbidity and mortality subsequently began feeding CTC, or 2) some individual cattle consume enough CTC to achieve clearance of the persistent carrier state, thereby increasing risk of reinfection and clinical BA.
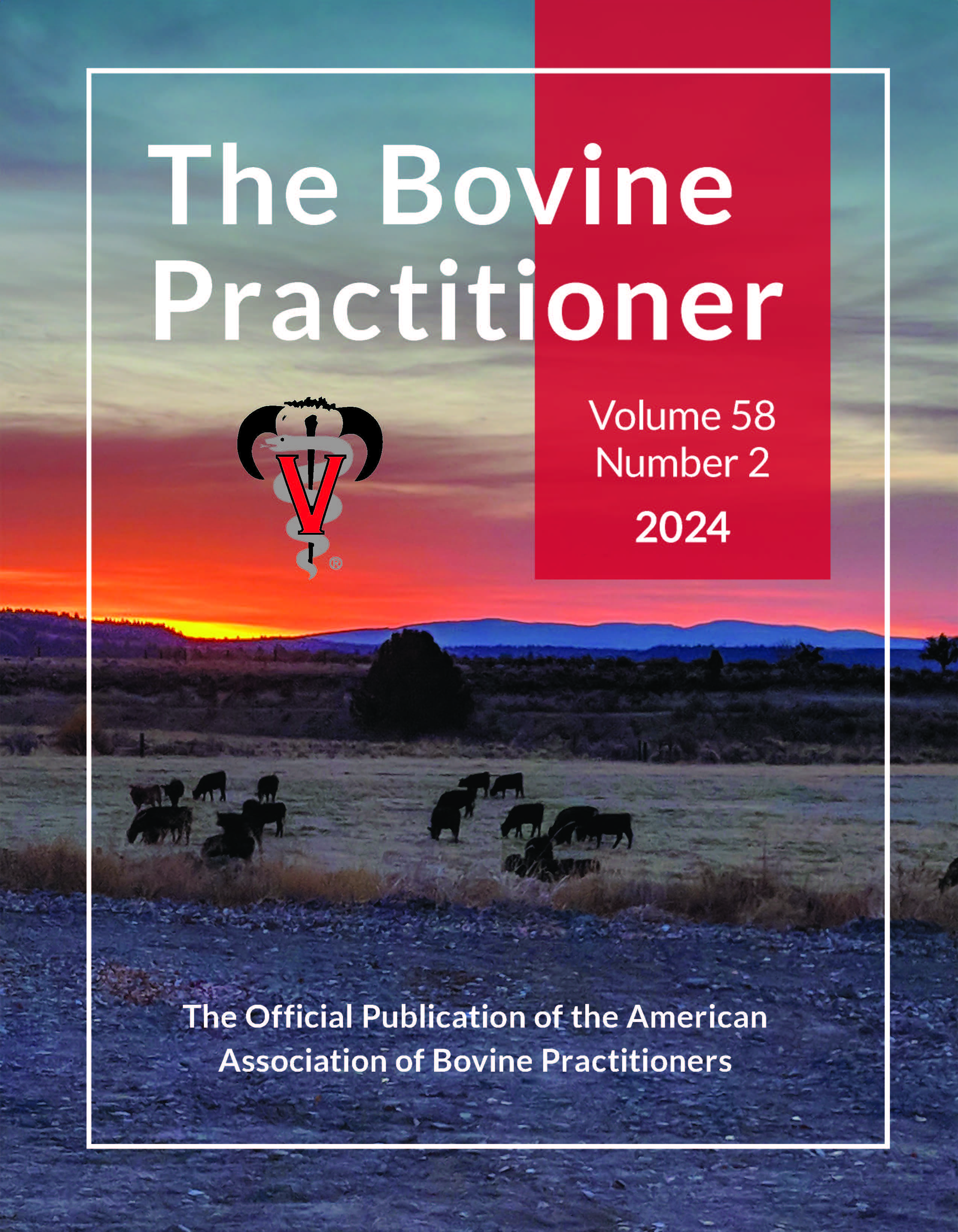
How to Cite
- Endnote/Zotero/Mendeley (RIS)
Most read articles by the same author(s)
- Alexis C. Thompson, David R. Smith, Failed transfer of passive immunity is a component cause of pre-weaning disease in beef and dairy calves: A systematic review and meta-analysis , The Bovine Practitioner: Vol. 56 No. 2 (2022)
- W. Isaac Jumper, Carla L. Huston, Robert W. Willis, David R. Smith, Survey of U.S. cow-calf producer methods and opinions of cattle health and production record-keeping , The Bovine Practitioner: Vol. 56 No. 2 (2022)
- W. Isaac Jumper, Carla L. Huston, Robert W. Wills, David R. Smith, Survey of the cattle health and production record-keeping methods and opinions of cow-calf producers in Mississippi , The Bovine Practitioner: Vol. 55, No. 1 (2021)
- Kristina J. Hubbard, Amelia R. Woolums, Brandi B. Karisch, John R. Blanton, William B. Epperson, David R. Smith, Case report , The Bovine Practitioner: Vol. 52, No. 2 (2018 Summer)
- W. Isaac Jumper, Carla L. Huston, Robert L. Willis, David R. Smith, Survey of veterinary involvement in cattle health and production record-keeping on U.S. cow-calf operations , The Bovine Practitioner: Vol. 56 No. 2 (2022)
- David R. Smith, Dale M. Grotelueschen, Tim R. Knott, Sharon L. Clowser, Gail R. Nason, Population Dynamics of Undifferentiated Neonatal Calf Diarrhea among Ranch Beef Calves , The Bovine Practitioner: Vol. 42, No. 1 (2008 Spring)
- Clayton L. Kelling, Dale M. Grotelueschen, David R. Smith, Bruce W. Brodersen, Testing and Management Strategies for Effective Beef and Dairy Herd BVDV Biosecurity Programs , The Bovine Practitioner: Vol. 34, No. 1 (2000 January)
- W. Isaac Jumper, Carla L. Huston, Robert W. Wills, David R. Wills, Survey of veterinary involvement in management decisions on Mississippi cow-calf operations , The Bovine Practitioner: Vol. 55, No. 1 (2021)
- W. Isaac Jumper, Carla L. Huston, Robert W. Willis, David R. Smith, Survey of U.S. cow-calf producer access to and use of technology for cattle health and production record-keeping purposes , The Bovine Practitioner: Vol. 56 No. 2 (2022)
- Amelia R. Woolums, Roy D. Berghaus, David R. Smith, Brad J. White, Terry J. Engelken, Max B. Irsik, Darin K. Matlik, A. Lee Jones, Isaiah J. Smith, A Survey of veterinarians in 6 US states regarding their experience with nursing beef calf respiratory disease , The Bovine Practitioner: Vol. 48, No. 1 (2014 Spring)
Print ISSN: 0524-1685
americanabp

proceedings

Make a Submission
Information.
- For Readers
- For Authors
- For Librarians
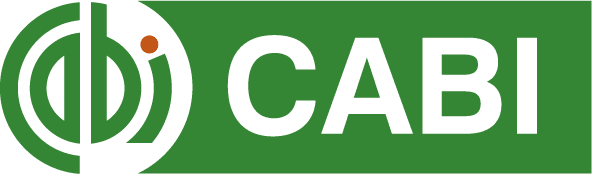
Background image is "Cows on pasture in the morning light" by Dietmar Rabich
Dietmar Rabich , Dülmen, Weide am Borgplackenweg -- 2015 -- 8742 , CC BY-SA 4.0
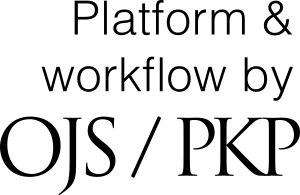

An official website of the United States government
The .gov means it’s official. Federal government websites often end in .gov or .mil. Before sharing sensitive information, make sure you’re on a federal government site.
The site is secure. The https:// ensures that you are connecting to the official website and that any information you provide is encrypted and transmitted securely.
- Publications
- Account settings
Preview improvements coming to the PMC website in October 2024. Learn More or Try it out now .
- Advanced Search
- Journal List
- Ann Indian Acad Neurol
- v.16(4); Oct-Dec 2013
Design and data analysis case-controlled study in clinical research
Sanjeev v. thomas.
Department of Neurology, Sree Chitra Tirunal Institute for Medical Sciences and Technology, Trivandrum, Kerala, India
Karthik Suresh
1 Department of Pulmonary and Critical Care Medicine, Johns Hopkins University School of Medicine, Louiseville, USA
Geetha Suresh
2 Department of Justice Administration, University of Louisville, Louiseville, USA
Clinicians during their training period and practice are often called upon to conduct studies to explore the association between certain exposures and disease states or interventions and outcomes. More often they need to interpret the results of research data published in the medical literature. Case-control studies are one of the most frequently used study designs for these purposes. This paper explains basic features of case control studies, rationality behind applying case control design with appropriate examples and limitations of this design. Analysis of sensitivity and specificity along with template to calculate various ratios are explained with user friendly tables and calculations in this article. The interpretation of some of the laboratory results requires sound knowledge of the various risk ratios and positive or negative predictive values for correct identification for unbiased analysis. A major advantage of case-control study is that they are small and retrospective and so they are economical than cohort studies and randomized controlled trials.
Introduction
Clinicians think of case-control study when they want to ascertain association between one clinical condition and an exposure or when a researcher wants to compare patients with disease exposed to the risk factors to non-exposed control group. In other words, case-control study compares subjects who have disease or outcome (cases) with subjects who do not have the disease or outcome (controls). Historically, case control studies came into fashion in the early 20 th century, when great interest arose in the role of environmental factors (such as pipe smoke) in the pathogenesis of disease. In the 1950s, case control studies were used to link cigarette smoke and lung cancer. Case-control studies look back in time to compare “what happened” in each group to determine the relationship between the risk factor and disease. The case-control study has important advantages, including cost and ease of deployment. However, it is important to note that a positive relationship between exposure and disease does not imply causality.
At the center of the case-control study is a collection of cases. [ Figure 1 ] This explains why this type of study is often used to study rare diseases, where the prevalence of the disease may not be high enough to permit for a cohort study. A cohort study identifies patients with and without an exposure and then “looks forward” to see whether or not greater numbers of patients with an exposure develop disease.

Comparison of cohort and case control studies
For instance, Yang et al . studied antiepileptic drug (AED) associated rashes in Asians in a case-control study.[ 1 ] They collected cases of confirmed anti-epileptic induced severe cutaneous reactions (such as Stevens Johnson syndrome) and then, using appropriate controls, analyzed various exposures (including type of [AED] used) to look for risk factors to developing AED induced skin disease.
Choosing controls is very important aspect of case-control study design. The investigator must weigh the need for the controls to be relevant against the tendency to over match controls such that potential differences may become muted. In general, one may consider three populations: Cases, the relevant control population and the population at large. For the study above, the cases include patients with AED skin disease. In this case, the relevant control population is a group of Asian patients without skin disease. It is important for controls to be relevant: In the anti-epileptic study, it would not be appropriate to choose a population across ethnicities since one of the premises of the paper revolves around particularly susceptibility to AED drug rashes in Asian populations.
One popular method of choosing controls is to choose patients from a geographic population at large. In studying the relationship between non-steroidal anti-inflammatory drugs and Parkinson's disease (PD), Wahner et al . chose a control population from several rural California counties.[ 2 ] There are other methods of choosing controls (using patients without disease admitted to the hospital during the time of study, neighbors of disease positive cases, using mail routes to identify disease negative cases). However, one must be careful not to introduce bias into control selection. For instance, a study that enrolls cases from a clinic population should not use a hospital population as control. Studies looking at geography specific population (e.g., Neurocysticercosis in India) cannot use controls from large studies done in other populations (registries of patients from countries where disease prevalence may be drastically different than in India). In general, geographic clustering is probably the easiest way to choose controls for case-control studies.
Two popular ways of choosing controls include hospitalized patients and patients from the general population. Choosing hospitalized, disease negative patients offers several advantages, including good rates of response (patients admitted to the hospital are generally already being examined and evaluated and often tend to be available to further questioning for a study, compared with the general population, where rates of response may be much lower) and possibly less amnestic bias (patients who are already in the hospital are, by default, being asked to remember details of their presenting illnesses and as such, may more reliably remember details of exposures). However, using hospitalized patients has one large disadvantage; these patients have higher severity of disease since they required hospitalization in the first place. In addition, patients may be hospitalized for disease processes that may share features with diseases under study, thus confounding results.
Using a general population offers the advantage of being a true control group, random in its choosing and without any common features that may confound associations. However, disadvantages include poor response rates and biasing based on geography. Administering long histories and questions regarding exposures are often hard to accomplish in the general population due to the number of people willing (or rather, not willing) to undergo testing. In addition, choosing cases from the general population from particular geographic areas may bias the population toward certain characteristics (such as a socio-economic status) of that geographic population. Consider a study that uses cases from a referral clinic population that draws patients from across socio-economic strata. Using a control group selected from a population from a very affluent or very impoverished area may be problematic unless the socio-economic status is included in the final analysis.
In case-controls studies, cases are usually available before controls. When studying specific diseases, cases are often collected from specialty clinics that see large numbers of patients with a specific disease. Consider for example, the study by Garwood et al .[ 3 ] which looked at patients with established PD and looked for associations between prior amphetamine use and subsequent development various neurologic disorders. Patients in this study were chosen from specialty clinics that see large numbers of patients with certain neurologic disorders. Case definitions are very important when planning to choose cases. For instance, in a hypothetical study aiming to study cases of peripheral neuropathy, will all patients who carry a diagnosis of peripheral neuropathy be included? Or, will only patients with definite electromyography evidence of neuropathy be included? If a disease process with known histopathology is being studied, will tissue diagnosis be required for all cases? More stringent case definitions that require multiple pieces of data to be present may limit the number of cases that can be used in the study. Less stringent criteria (for instance, counting all patients with the diagnosis of “peripheral neuropathy” listed in the chart) may inadvertently choose a group of cases that are too heterogeneous.
The disease history status of the chosen cases must also be decided. Will the cases being chosen have newly diagnosed disease, or will cases of ongoing/longstanding disease also be included? Will decedent cases be included? This is important when looking at exposures in the following fashion: Consider exposure X that is associated with disease Y. Suppose that exposure X negatively affects disease Y such that patients that are X + have more severe disease. Now, a case-control study that used only patients with long-standing or ongoing disease might miss a potential association between X and Y because X + patients, due to their more aggressive course of disease, are no longer alive and therefore were not included in the analysis. If this particular confounding effect is of concern, it can be circumvented by using incident cases only.
Selection bias occurs when the exposure of interest results in more careful screening of a population, thus mimicking an association. The classic example of this phenomenon was noted in the 70s, when certain studies noted a relationship between estrogen use and endometrial cancer. However, on close analysis, it was noted that patients who used estrogen were more likely to experience vaginal bleeding, which in turn is often a cause for close examination by physicians to rule out endometrial cancer. This is often seen with certain drug exposures as well. A drug may produce various symptoms, which lead to closer physician evaluation, thus leading to more disease positive cases. Thus, when analyzed in a retrospective fashion, more of the cases may have a particular exposure only insofar as that particular exposure led to evaluations that resulted in a diagnosis, but without any direct association or causality between the exposure and disease.
One advantage of case-control studies is the ability to study multiple exposures and other risk factors within one study. In addition, the “exposure” being studied can be biochemical in nature. Consider the study, which looked at a genetic variant of a kinase enzyme as a risk factor for development of Alzheimer's disease.[ 4 ] Compare this with the study mentioned earlier by Garwood et al .,[ 3 ] where exposure data was collected by surveys and questionnaires. In this study, the authors drew blood work on cases and controls in order to assess their polymorphism status. Indeed, more than one exposure can be assessed in the same study and with planning, a researcher may look at several variables, including biochemical ones, in single case-control study.
Matching is one of three ways (along with exclusion and statistical adjustment) to adjust for differences. Matching attempts to make sure that the control group is sufficiently similar to the cases group, with respects to variables such as age, sex, etc., Cases and controls should not be matched on variables that will be analyzed for possible associations to disease. Not only should exposure variables not be included, but neither should variables that are closely related to these variables. Lastly, overmatching should be avoided. If the control group is too similar to the cases group, the study may fail to detect the difference even if one exists. In addition, adding matching categories increases expense of the study.
One measure of association derived from case control studies are sensitivity and specificity ratios. These measures are important to a researcher, to understand the correct classification. A good understanding of sensitivity and specificity is essential to understand receiver operating characteristic curve and in distinguishing correct classification of positive exposure and disease with negative exposure and no disease. Table 1 explains a hypothetical example and method of calculation of specificity and sensitivity analysis.
Hypothetical example of sensitivity, specificity and predictive values

Interpretation of sensitivity, specificity and predictive values
Sensitivity and specificity are statistical measures of the performance of a two by two classification of cases and controls (sick or healthy) against positives and negatives (exposed or non-exposed).[ 5 ] Sensitivity measures or identifies the proportion of actual positives identified as the percentage of sick people who are correctly identified as sick. Specificity measures or identifies the proportion of negatives identified as the percentage of healthy people who are correctly identified as healthy. Theoretically, optimum prediction aims at 100% sensitivity and specificity with a minimum of margin of error. Table 1 also shows false positive rate, which is referred to as Type I error commonly stated as α “Alpha” is calculated using the following formula: 100 − specificity, which is equal to 100 − 90.80 = 9.20% for Table 1 example. Type 1 error is also known as false positive error is referred to as a false alarm, indicates that a condition is present when it is actually not present. In the above mentioned example, a false positive error indicates the percent falsely identified healthy as sick. The reason why we want Type 1 error to be as minimum as possible is because healthy should not get treatment.
The false negative rate, which is referred to as Type II error commonly stated as β “Beta” is calculated using the following formula: 100 − sensitivity which is equal to 100 − 73.30 = 26.70% for Table 1 example. Type II error is also known as false negative error indicates that a condition is not present when it should have been present. In the above mentioned example, a false negative error indicates percent falsely identified sick as healthy. A Type 1 error unnecessarily treats a healthy, which in turn increases the budget and Type II error would risk the sick, which would act against study objectives. A researcher wants to minimize both errors, which not a simple issue because an effort to decrease one type of error increases the other type of error. The only way to minimize both type of error statistically is by increasing sample size, which may be difficult sometimes not feasible or expensive. If the sample size is too low it lacks precision and it is too large, time and resources will be wasted. Hence, the question is what should be the sample size so that the study has the power to generalize the result obtained from the study. The researcher has to decide whether, the study has enough power to make a judgment of the population from their sample. The researcher has to decide this issue in the process of designing an experiment, how large a sample is needed to enable reliable judgment.
Statistical power is same as sensitivity (73.30%). In this example, large number of false positives and few false negatives indicate the test conducted alone is not the best test to confirm the disease. Higher statistical power increase statistical significance by reducing Type 1 error which increases confidence interval. In other words, larger the power more accurately the study can mirror the behavior of the study population.
The positive predictive values (PPV) or the precision rate is referred to as the proportion of positive test results, which means correct diagnoses. If the test correctly identifies all positive conditions then the PPV would be 100% and negative predictive value (NPV) would be 0. The calculative PPV in Table 1 is 11.8%, which is not large enough to predict cases with test conducted alone. However, the NPV 99.9% indicates the test correctly identifies negative conditions.
Clinical interpretation of a test
In a sample, there are two groups those who have the disease and those who do not have the disease. A test designed to detect that disease can have two results a positive result that states that the disease is present and a negative result that states that the disease is absent. In an ideal situation, we would want the test to be positive for all persons who have the disease and test to be negative for all persons who do not have the disease. Unfortunately, reality is often far from ideal. The clinician who had ordered the test has the result as positive or negative. What conclusion can he or she make about the disease status for his patient? The first step would be to examine the reliability of the test in statistical terms. (1) What is the sensitivity of the test? (2) What is the specificity of the test? The second step is to examine it applicability to his patient. (3) What is the PPV of the test? (4) What is the NPV of the test?
Suppose the test result had come as positive. In this example the test has a sensitivity of 73.3% and specificity of 90.8%. This test is capable of detecting the disease status in 73% of cases only. It has a false positivity of 9.2%. The PPV of the test is 11.8%. In other words, there is a good possibility that the test result is false positive and the person does not have the disease. We need to look at other test results and the clinical situation. Suppose the PPV of this test was close to 80 or 90%, one could conclude that most likely the person has the disease state if the test result is positive.
Suppose the test result had come as negative. The NPV of this test is 99.9%, which means this test gave a negative result in a patient with the disease only very rarely. Hence, there is only 0.1% possibility that the person who tested negative has in fact the disease. Probably no further tests are required unless the clinical suspicion is very high.
It is very important how the clinician interprets the result of a test. The usefulness of a positive result or negative result depends upon the PPV or NPV of the test respectively. A screening test should have high sensitivity and high PPV. A confirmatory test should have high specificity and high NPV.
Case control method is most efficient, for the study of rare diseases and most common diseases. Other measures of association from case control studies are calculation of odds ratio (OR) and risk ratio which is presented in Table 2 .
Different ratio calculation templates with sample calculation

Absolute risk means the probability of an event occurring and are not compared with any other type of risk. Absolute risk is expressed as a ratio or percent. In the example, absolute risk reduction indicates 27.37% decline in risk. Relative risk (RR) on the other hand compares the risk among exposed and non-exposed. In the example provided in Table 2 , the non-exposed control group is 69.93% less likely compared to exposed cases. Reader should keep in mind that RR does not mean increase in risk. This means that while a 100% likely risk among those exposed cases, unexposed control is less likely by 69.93%. RR does not explain actual risk but is expressed as relative increase or decrease in risk of exposed compared to non-exposed.
OR help the researcher to conclude whether the odds of a certain event or outcome are same for two groups. It calculates the odds of a health outcome when exposed compared to non-exposed. In our example an OR of. 207 can be interpreted as the non-exposed group is less likely to experience the event compared to the exposed group. If the OR is greater than 1 (example 1.11) means that the exposed are 1.11 times more likely to be riskier than the non-exposed.
Event rate for cases (E) and controls (C) in biostatistics explains how event ratio is a measure of how often a particular statistical exposure results in occurrence of disease within the experimental group (cases) of an experiment. This value in our example is 11.76%. This value or percent explains the extent of risk to patients exposed, compared with the non-exposed.
The statistical tests that can be used for ascertain an association depends upon the variable characteristics also. If the researcher wants to find the association between two categorical variables (e.g., a positive versus negative test result and disease state expressed as present or absent), Cochran-Armitage test, which is same as Pearson Chi-squared test can be used. When the objective is to find the association between two interval or ratio level (continuous) variables, correlation and regression analysis can be performed. In order to evaluate statistical significant difference between the means of cases and control, a test of group difference can be performed. If the researcher wants to find statically significant difference among means of more than two groups, analysis of variance can be performed. A detailed explanation and how to calculate various statistical tests will be published in later issues. The success of the research directly and indirectly depends on how the following biases or systematic errors, are controlled.
When selecting cases and controls, based on exposed or not-exposed factors, the ability of subjects to recall information on exposure is collected retrospectively and often forms the basis for recall bias. Recall bias is a methodological issue. Problems of recall method are: Limitations in human ability to recall and cases may remember their exposure with more accuracy than the controls. Other possible bias is the selection bias. In case-control studies, the cases and controls are selected from the same inherited characteristics. For instance, cases collected from referral clinics often exposed to selection bias cases. If selection bias is not controlled, the findings of association, most likely may be due to of chance resulting from the study design. Another possible bias is information bias, which arises because of misclassification of the level of exposure or misclassification of disease or other symptoms of outcome itself.
Case control studies are good for studying rare diseases, but they are not generally used to study rare exposures. As Kaelin and Bayona explains[ 6 ] if a researcher want to study the risk of asthma from working in a nuclear submarine shipyard, a case control study may not be a best option because a very small proportion of people with asthma might be exposed. Similarly, case-control studies cannot be the best option to study multiple diseases or conditions because the selection of the control group may not be comparable for multiple disease or conditions selected. The major advantage of case-control study is that they are small and retrospective and so they are economical than cohort studies and randomized controlled trials.
Source of Support: Nil
Conflict of Interest: Nil
This paper is in the following e-collection/theme issue:
Published on 28.5.2024 in Vol 26 (2024)
The Effect of Artificial Intelligence on Patient-Physician Trust: Cross-Sectional Vignette Study
Authors of this article:

Original Paper
- Anna G M Zondag 1 , MSc ;
- Raoul Rozestraten 2 , MSc ;
- Stephan G Grimmelikhuijsen 2 , PhD ;
- Karin R Jongsma 3 , PhD ;
- Wouter W van Solinge 1 , PhD ;
- Michiel L Bots 3 , MD, PhD ;
- Robin W M Vernooij 3, 4 , PhD ;
- Saskia Haitjema 1 , MD, PhD
1 Central Diagnostic Laboratory, University Medical Center Utrecht, Utrecht University, Utrecht, Netherlands
2 Utrecht University School of Governance, Utrecht University, Utrecht, Netherlands
3 Julius Center for Health Sciences and Primary Care, University Medical Center Utrecht, Utrecht University, Utrecht, Netherlands
4 Department of Nephrology and Hypertension, University Medical Center Utrecht, Utrecht, Netherlands
Corresponding Author:
Anna G M Zondag, MSc
Central Diagnostic Laboratory
University Medical Center Utrecht
Utrecht University
Heidelberglaan 100
Utrecht, 3584 CX
Netherlands
Phone: 31 631117922
Email: [email protected]
Background: Clinical decision support systems (CDSSs) based on routine care data, using artificial intelligence (AI), are increasingly being developed. Previous studies focused largely on the technical aspects of using AI, but the acceptability of these technologies by patients remains unclear.
Objective: We aimed to investigate whether patient-physician trust is affected when medical decision-making is supported by a CDSS.
Methods: We conducted a vignette study among the patient panel (N=860) of the University Medical Center Utrecht, the Netherlands. Patients were randomly assigned into 4 groups—either the intervention or control groups of the high-risk or low-risk cases. In both the high-risk and low-risk case groups, a physician made a treatment decision with (intervention groups) or without (control groups) the support of a CDSS. Using a questionnaire with a 7-point Likert scale, with 1 indicating “strongly disagree” and 7 indicating “strongly agree,” we collected data on patient-physician trust in 3 dimensions: competence, integrity, and benevolence. We assessed differences in patient-physician trust between the control and intervention groups per case using Mann-Whitney U tests and potential effect modification by the participant’s sex, age, education level, general trust in health care, and general trust in technology using multivariate analyses of (co)variance.
Results: In total, 398 patients participated. In the high-risk case, median perceived competence and integrity were lower in the intervention group compared to the control group but not statistically significant (5.8 vs 5.6; P =.16 and 6.3 vs 6.0; P =.06, respectively). However, the effect of a CDSS application on the perceived competence of the physician depended on the participant’s sex ( P =.03). Although no between-group differences were found in men, in women, the perception of the physician’s competence and integrity was significantly lower in the intervention compared to the control group ( P =.009 and P =.01, respectively). In the low-risk case, no differences in trust between the groups were found. However, increased trust in technology positively influenced the perceived benevolence and integrity in the low-risk case ( P =.009 and P =.04, respectively).
Conclusions: We found that, in general, patient-physician trust was high. However, our findings indicate a potentially negative effect of AI applications on the patient-physician relationship, especially among women and in high-risk situations. Trust in technology, in general, might increase the likelihood of embracing the use of CDSSs by treating professionals.
Introduction
It was John McCarthy who coined the term “artificial intelligence” (AI) at the Dartmouth conference in 1956 and defined it as “the science and engineering of making intelligent machines, especially intelligent computer programs” [ 1 ]. However, it was only in the 1990s, after the first so-called “AI winter,” that interest in AI began to increase again [ 2 ]. Since then, AI applications have been on the rise, ranging from self-driving cars to AI-powered web search [ 3 ]. Development and implementation of AI in health care is similarly increasing. It is believed that AI has the potential to improve every facet of health care—screening, diagnosis, prognosis, and treatment [ 4 ]. Although the use of AI in routine clinical care is still in the early stages, it has already shown promise in specific medical fields, such as radiology, for the recognition of complex patterns in imaging data [ 3 , 5 ]. Hospital-wide strategic programs have been initiated to develop predictive AI algorithms based on routine clinical care data in various hospitals [ 6 ]. The goal of these projects is, among others, to integrate AI algorithms in clinical decision support systems (CDSSs). CDSSs are often classified as either knowledge-based or non–knowledge-based systems. Knowledge-based CDSSs provide an output by evaluating a certain rule, which is programmed based on evidence or practice. Non–knowledge-based CDSSs use AI techniques, such as machine learning, for decision support and prediction [ 7 ]. AI-based CDSSs are often developed when dealing with complex, high-dimensional, and large amounts of data (ie, big data), such as routine care data. By linking patient information to evidence-based knowledge, a CDSS can provide case-specific information, which may support physicians in developing more personalized judgments and recommendations [ 8 ]. Despite these efforts, however, to date, only a fraction of all developed AI-based algorithms have been implemented in clinical care [ 9 ].
The debate on AI-based CDSSs in health care has mainly focused on the technical aspects of the technology [ 10 , 11 ], including questions like “How well does the predictive algorithm perform in terms of, for example, recall and precision?” or “What is the importance of its features?” To date, less attention has been given to the acceptability of using AI-based CDSSs in terms of patients’ or physicians’ trust in CDSSs even though these are crucial for the implementation and acceptance of these systems [ 12 , 13 ]. Some concerns revolve around how the patient-physician relationship is directly affected by the integration of AI applications into clinical practice. This concern arises due to all the new possibilities that AI offers, such as decision support, patient dashboards, and eHealth [ 14 - 16 ]. Figure 1 illustrates how the relationship between a patient and physician could be influenced by AI [ 17 , 18 ]. Studies have shown that algorithms developed to diagnose or predict a disease often perform as well as, and sometimes even better than, a physician [ 19 ]. As a result, physicians may be able to make more informed decisions and subsequently improve patient care, which was the incentive to start the Applied Data Analytics in Medicine (ADAM) project at the University Medical Center (UMC) Utrecht [ 6 ]. On the other hand, to build and maintain interpersonal trust, patient involvement in the decision-making process is important [ 20 - 23 ]. However, these algorithms, especially those that include AI, are sometimes perceived as “black boxes,” which could potentially lead to a reduction in trust in the physician, even though these algorithms can be beneficial to the patient’s care process. In medicine, trust is considered a central aspect of the patient-physician relationship. Without trust, treatments have proven to be less effective, and patients are more prone to ask for a second opinion [ 24 ].

To the best of our knowledge, we are among the first to study the impact of AI-based CDSSs on patient-physician trust in both a clinical high-risk and low-risk setting. Trust is a complex and multidimensional concept [ 25 ]. Over the past decades, a lot of research has been conducted on this notion and various dimensions of trust have been described in the academic literature [ 26 ]. The most commonly named dimensions of trust in a physician are privacy and confidentiality [ 27 , 28 ], compassion [ 27 , 29 ], reliability and dependence [ 28 ], competence [ 27 - 31 ], communication [ 32 ], and honesty [ 33 ]. However, these dimensions of trust are often studied separately. With so many described trust dimensions, it is difficult to develop a framework specifically for patient-physician trust. Therefore, a framework that integrates these components of trust in a physician has not been established yet. In social sciences, trust is commonly studied in 3 dimensions: benevolence, competence, and integrity [ 34 - 37 ]. In a clinical context, benevolence is the extent to which a patient perceives a physician as caring about the patient’s personal and health interests. Competence is the extent to which a patient perceives a physician as competent, capable, effective, and professional. Integrity concerns the extent to which a patient sees a physician as honest and truthful, handling the patient’s sensitive information with care and confidentially.
We aim to study the extent to which these aspects of patient-physician trust are affected by AI-based CDSSs.
We followed the Strengthening the Reporting of Observational Studies in Epidemiology (STROBE) guidelines for observational (cross-sectional) studies.
Study Setting
We conducted a cross-sectional vignette study at the UMC Utrecht, the Netherlands. We reached out to all members of the UMC Utrecht patient panel (N=860) as part of the ADAM program (2017-2020; currently known as the Digital Health Department within the UMC Utrecht) [ 6 ]. In brief, the ADAM program consisted of 11 projects all aiming to answer clinical questions to personalize health care using routine care data and innovative methodology, such as AI. The overall goal of the ADAM program was to investigate the need to apply this health care innovation on a large scale at the UMC Utrecht to establish a learning health care system [ 6 ].
For our study, we simulated possible future clinical decision support applications of 2 of the ADAM projects. The Neonatal Intensive Care Unit (NICU) application concerned data of babies between 24 and 32 weeks old admitted in the NICU using an algorithm that was trained on a data set from the Wilhelmina Children’s Hospital of the UMC Utrecht. This CDSS tool aimed to predict late-onset sepsis using the algorithm to prevent unnecessary antibiotic use and enable timely treatment [ 6 ]. The rheumatoid arthritis (RA) CDSS worked on the premise that disease flares can be predicted based on data about the course of the disease, patient characteristics, and information about current treatment. The aim of the RA application was to predict flares to support data-driven reduction of high preventative doses of RA medication [ 6 , 38 ]. This algorithm was trained on data of patients with RA of the UMC Utrecht [ 38 ].
Study Population
Members of the patient panel (N=860) were all current and former patients of the UMC Utrecht willing to participate in research from the UMC Utrecht. The majority (693/860, 80.6%) of the panel was between 45 and 80 years of age, 57.3% (493/860) had higher education levels, and 52.8% (454/860) of the patient panel were women.
Questionnaire
In our study, we focused on interpersonal trust. We used the definition from Mayer et al [ 34 ] to define interpersonal trust as “the willingness of a party to be vulnerable to the actions of another party based on the expectation that the other will perform a particular action important to the trustor, irrespective of the ability to monitor or control that other party.”
We studied 3 dimensions of trust: competence, integrity, and benevolence. We used an adapted version of the “Trust in Physician Scale” to assess patient-physician trust. The “Trust in Physician Scale” is a questionnaire consisting of 11 items and is commonly used to measure patient-physician trust [ 28 , 39 ]. Table 1 illustrates how the 11 questions of the Trust in Physician Scale were adapted and divided into the 3 trust dimensions.
In addition to the questions of the Trust in Physician Scale, we added several self-constructed questions to study these aspects of trust more comprehensively. We added the following 3 statements in the integrity dimension:
- I have the feeling that this physician is not holding anything back from me (scale 1-7).
- I have the feeling that this physician is being honest with me (scale 1-7).
- I trust this physician is handling my medical data with care (scale 1-7).
One additional statement was added in the benevolence dimension, as follows: “This physician’s recommendation is in my personal best interest (scale 1-7).”
Besides trust in the physician, we added 2 additional questions about trust in health care and technology in general on a 7-point Likert scale (1: “no trust at all”; 7: “fully trust”), as follows:
- Please indicate your level of trust in health care in general (scale 1-7).
- Please indicate your level of trust in technology in general (scale 1-7).
Additionally, we asked the panel members their age, sex, and education level.
Subsequently, we performed a factor analysis on the questionnaire, including the Trust in Physician Scale and the self-constructed questions, to check whether the predefined dimensions were present in the questionnaire. The factor analysis revealed the correlations between the items of the questionnaire and subdivided them into factors. Thereafter, we calculated Cronbach α to measure the degree of coherence between the 3 trust dimensions [ 40 ]. A Cronbach α of more than 0.75 was considered high, and thus, acceptable, meaning that all items of the dimension measured the same concept [ 41 ].
Design of Data Collection
Data were collected in April 2019. The questionnaire included 1 of the 2 hypothetical cases in the form of a so-called “vignette.” These vignettes were developed together with physicians from the neonatology and rheumatology department as well as the ADAM program staff to make them as realistic as possible (eg, to assess whether the physician’s communication style in the vignette reflected clinical practice) and to ensure that the AI-based CDSS applications of both projects were accurately described. The vignettes were tested on master’s degree students of Public Management from Utrecht University and employees of the ADAM program (N=36) first to study whether they could empathize well with the situation presented to them. This test resulted in a mean score of 4.6 for the NICU and 5.7 for the RA vignette on a 7-point Likert scale (the higher the score, the better they could empathize with the situation presented). We processed the feedback we received from the test participants to further improve the vignettes. After successful testing, we randomly divided the panel members into 4 groups ( Multimedia Appendix 1 ). The groups were presented with either a life-threatening (high-risk) vignette in the NICU (from now on referred to as the “high-risk case”), or a non–life-threatening (low-risk) vignette regarding RA (referred to as the “low-risk case”). Details of the vignettes are described in Multimedia Appendix 2 . The high-risk case described a baby in the NICU that possibly had sepsis. In this case, we asked the panel members to empathize with the role of the baby’s parents. The low-risk case described a patient with RA, and panel members were asked to empathize with the role of the patient. In both situations, the physician made a treatment recommendation. This recommendation was made by a physician only in the control group and by a physician supported by a CDSS application in the intervention group. After reading the vignette, the panel members were asked to fill in the questionnaire. Possible answers were given using a 7-point Likert scale, with 1 indicating “strongly disagree” and 7 indicating “strongly agree.”
We tracked the time it took panel members to read the vignettes. To minimize the potential loss of statistical power due to panel members not being diligent and motivated to complete the survey, we excluded the 2.5% slowest and fastest readers from all analyses. Figure 2 illustrates the patient inclusion and exclusion procedure in a flow diagram.

Data Analyses
We presented the results of all groups in medians (IQRs), stratified by case. We compared perceived trust between the intervention and control group using the Mann-Whitney U test (Wilcoxon rank sum test).
We assessed the presence or absence of effect modification using a two-way multivariate analysis of variance (MANOVA) or multivariate analysis of covariance (MANCOVA), as appropriate. The participant’s sex, age, education level, general trust in health care, and general trust in technology were considered potential effect modifiers in the relation between the use of a CDSS and patient-physician trust. We further stratified the results by sex in case of effect modification to examine this potential effect.
All analyses were performed using SPSS edition 26 (IBM SPSS Inc) [ 42 ]. Differences with a 2-sided P value of <.05 were considered statistically significant.
Ethical Considerations
All participants provided informed consent digitally for this study. The panel members received an introductory text with information about this study. At the end of the introductory text, the privacy statement informed the panel members that by clicking the “Continue” button, they agreed to the use of their data in this study. Data were anonymized and IP addresses were not stored.
The questionnaires were administered in Qualtrics in accordance with Utrecht University guidelines and stored on the Yoda server, which is a research data management service from the Utrecht University, among others, enabling secure storage of the research data [ 43 , 44 ]. The methods have been performed based on relevant guidelines and regulations. The institutional review board of UMC Utrecht waived ethical approval because it ruled that this study did not concern a medical research question.
Demographics of the Study Population
In total, 398/860 (46.3%) panel members of the UMC Utrecht patient panel participated in this study. Characteristics of the study population can be seen in Table 2 . Participants were older in the intervention group of both cases. In the high-risk case, the intervention group consisted of more men (52/92, 56.5%), whereas the control group consisted of more women (54/93, 58.1%). In the low-risk case, both the control and intervention groups consisted of more women (55/109, 50.5% and 56/104, 53.8%, respectively). The education level was similar across the groups. In all groups, the majority of participants (62/93, 66.7%; 60/92, 65.9%; 69/109, 63.3%; and 68/104, 66.0%) had a high education level, that is, a degree from a university of applied sciences or higher.
Measured Trust in the Physician
Based on the results of the factor analysis and Cronbach α calculation, we assessed trust on both a multidimensional and a unidimensional scale. The reason to use a unidimensional scale was that the factor analysis initially showed 2 factors (Table S1 in Multimedia Appendix 3 ); however, after removing the 2 items that loaded strongly on both factors and 1 item that primarily loaded on the second factor, only 1 factor remained. The reason for using a multidimensional scale was that the Cronbach α of the 3 dimensions was considered high enough (>0.75) to measure trust on a multidimensional scale (Table S2 in Multimedia Appendix 3 ).
Overall, trust in the physician was high, with a median of 5.8 (control group IQR 5.0-6.5; intervention group IQR 4.7-6.2) in the high-risk case and 6.0 (control group IQR 5.3-6.5; intervention group IQR 5.1-6.5) in the low-risk case on a 7-point Likert scale ( Multimedia Appendix 4 ). The high-risk case showed a lower median for the integrity of the physician using a CDSS (6.3, IQR 5.3-6.8 vs 6.0, IQR 5.0-6.7; U =3590.0; P =.06; Table 3 ) compared to the physician who did not use a CDSS, but these results were not statistically significant. Similarly, perceived competence was lower in the intervention group compared to the control group (median 5.8, IQR 4.8-6.5 vs 5.6, IQR 4.3-6.3; U =3771.5; P =.16). We observed no between-group differences in perceived competence, integrity, and benevolence of the low-risk group.
In the analyses exploring whether the results were different among subgroups, in the high-risk case, the effect of a CDSS application on the perceived competence of the physician depended on the participant’s sex ( F 1,181 =4.694; P =.03; Multimedia Appendix 5 ). In women, perceived competence and integrity were significantly lower in physicians who used a CDSS compared to physicians who did not ( U =740.5; P =.009 and U =756.0; P =.01, respectively; Table 4 ), whereas no such statistically significant differences were found in men.
In the low-risk case, results showed that the effect of the CDSS application on the perceived benevolence and integrity depended on the participant’s trust in technology in general ( F 1,209 =6.943; P =.009 and F 1,209 =4.119; P =.04, respectively; Multimedia Appendix 5 ). An increase in the participant’s trust in technology, in general, led to an increased perceived integrity and benevolence of physicians using a CDSS. This increase was more significant in the intervention group compared to the control group.
a U test: Wilcoxon test statistic.
b The P values in the table indicate the difference between the control and intervention groups.
a U : Wilcoxon test statistic.
b P values indicate the difference between the control and intervention groups.
Principal Findings
We aimed to assess the extent to which patient-physician trust was affected by using a CDSS application. We found that, in general, trust in physicians was high. Nonetheless, in the high-risk case, we observed that trust, in terms of competence and integrity, was lower in physicians using a CDSS compared to physicians who did not, and that the differences were larger in women than in men. No differences were found in the low-risk case. However, we found that perceived benevolence and integrity in the low-risk case depended on the participant’s trust in technology in general.
Comparison With Prior Literature
To our knowledge, this study is among the first to examine the impact of AI-based CDSSs on patient-physician trust in both a clinical high-risk and low-risk setting. There are several possible explanations for our results. First, prior to our study, we asked several physicians of the UMC Utrecht about their expectations regarding CDSS applications. Some anticipated that the use of CDSS applications in clinical practice could evoke a critical response from patients, believing that CDSS applications “first must prove their value before being accepted by patients.” Regarding competence, physicians mentioned that the use of a CDSS application could raise doubts about their professionalism, as it could come across as “being dependent on such an application.” This dependence and the associated loss of competence, known as “deskilling,” is a known concern of the introduction of clinical decision support applications in health care [ 45 ]. Some of these concerns seem reasonable, as our study indicates that patients’ trust in their physicians can decrease when a CDSS is used. Moreover, the decrease found in trust in terms of the integrity of a physician who uses a CDSS could be explained by concerns about data protection. Patients may feel they have no control over what happens with their personal data. However, some studies indicated that privacy was not an issue for some patients, as long as they trusted their physician [ 46 , 47 ]. Yakar et al [ 48 ] studied the general population’s view on the use of AI in health care through a web-based survey in the Netherlands and found less trust in AI than initially hypothesized. They, and some others, also found that women were less trusting of AI than men [ 48 , 49 ]. A reason for this could be that the women participating in this study were aware of the potential presence of gender bias in AI, as this issue has often been raised in previous research and media [ 50 - 52 ]. Additionally, women generally are considered to have more risk aversion than men [ 53 ]. Both these reasons combined could explain why the effect of using a CDSS on the perceived trust in the physician in the high-risk case depended on the participant’s sex and why this was not the case in the low-risk case.
In the low-risk case, our results showed that a patient’s general trust in technology played a significant role in the effect that CDSS applications could have on the perceived integrity and benevolence of the physician. An increase in the perceived integrity and benevolence of the physician seemed to be more likely in patients who had more trust in technology in general. This is in line with previous research investigating the association between trust in technology in general and trust in the implementation of AI in medicine [ 48 , 54 ].
Implications
Results from this study underline that mainly communicating about the technological aspects of AI algorithms in health care may ultimately hamper the successful implementation and acceptance of these algorithms in clinical practice. Personalized health care starts with putting the patient’s values first. Therefore, we believe that the development of AI-based CDSSs in health care should be transparent in terms of methods, alternatives, and understandability. In addition, patients should be involved early in the developmental phase of the CDSS, and the use of AI should be explained well during the visit with the health care professional. Furthermore, AI applications should not only be developed to predict the prognosis of a certain disease (precision medicine) but also to improve and facilitate discussions between patients and physicians about the patient’s illness and personal care needs (shared decision-making). The discussion between the patient and the physician may be improved by being transparent about the CDSS application. This potentially leads to more trust in the application, which, as shown in this study, could lead to an increase in the perceived integrity and benevolence of the physician. Moreover, it seems sensible to distinguish between the types of situations or patient populations in the development of AI algorithms for CDSS in health care; the results from our study indicate that less favorable reactions occur when AI is used in more life-threatening situations, which could also be due to the different patient populations, for example, babies in an acute situation versus adults in a chronic situation. By implementing AI-based CDSS in less risky situations first, patients could get acquainted with such CDSSs before implementing them in high-risk situations. Further study is warranted to establish the best implementation strategy regarding AI-based CDSSs in clinical care.
We recommend replicating this study while providing a clear explanation of the AI application. For example, by explaining how it works, how patient data are handled, and the benefits of such AI-based CDSSs for the patients. In addition, further research with a larger sample size and in different preventive care and cure settings, and across several age groups, should be conducted to confirm the results found in this study. External validation of our results will inform us whether our results are similar in, for example, patients in other health care settings or areas. Additionally, qualitative research methods should be considered to gain more insights into the reasons patient-physician trust might decline when using a CDSS from a patient perspective.
Strengths and Limitations
Vignette studies have been criticized in the past for their limitations. First, a frequently heard criticism of vignette studies is the lack of reality due to their hypothetical nature [ 55 ]. However, we minimized this by creating both hypothetical cases in collaboration with physicians working in the involved departments—the neonatology and rheumatology departments—and tested whether the participants could empathize well with the situation during a pilot study. Second, the participants in this study were (or still are) patients of the UMC Utrecht. This made it easier for the participants to empathize with the vignettes because they may have been in similar situations themselves, and they indicated they could (median 6.0, IQR 5.0-7.0 in all 4 groups). Third, it has been shown that participants of vignette studies are not always diligent and motivated to complete surveys or take these kinds of experiments seriously, which may reduce statistical power [ 56 ]. Participants could, for example, have put the questionnaire aside for a while, or might not have read the described situations properly [ 57 ]. We reduced this effect by tracking the time the participants took to read the vignettes and by excluding the fastest and the slowest 2.5% of readers from the analyses. Therefore, participants spending a remarkably long or short time reading the vignette were not included.
Conclusions
Trust in physicians is generally high among patients. However, our findings point toward a negative effect that AI applications can have on the patient-physician relationship, especially among women. We, therefore, believe that, for a successful adoption of AI applications in clinical practice, patients should be involved in both the development and implementation of such applications. Moreover, a broader societal discussion needs to take place about humane values and AI to gain insights into how we want AI to influence our lives when we encounter health care. In addition, clear communication to patients and society about the functions of the AI applications and what personal data they use seems equally important.
Acknowledgments
AGMZ was supported by the European Union’s Horizon 2020 research and innovation program under grant agreement number 101017331 (ODIN).
The funding source was not involved in the study design, analysis, and interpretation of the data nor the writing and in the decision to submit the manuscript for publication.
Authors' Contributions
AGMZ, RR, SGG, WWvS, MLB, RWMV, and SH contributed to the conceptualization and methodology of the project. AGMZ and RR analyzed the data. AGMZ wrote the draft manuscript. All authors contributed to the interpretation of the results and critically reviewed the manuscript. All authors read and approved the final version of the manuscript.
Conflicts of Interest
None declared.
Study design.
Details of the high-risk and low-risk vignettes.
Questionnaire factor analysis and Cronbach α.
Unidimensional trust scores, stratified by case.
Multivariate regression analyses to test potential effect modification.
- What is AI? Basic questions. Stanford University. URL: http://jmc.stanford.edu/artificial-intelligence/what-is-ai/index.html [accessed 2022-01-06]
- Ahuja AS. The impact of artificial intelligence in medicine on the future role of the physician. PeerJ. 2019;7:e7702. [ FREE Full text ] [ CrossRef ] [ Medline ]
- Hosny A, Parmar C, Quackenbush J, Schwartz LH, Aerts HJWL. Artificial intelligence in radiology. Nat Rev Cancer. Dec 2018;18(8):500-510. [ FREE Full text ] [ CrossRef ] [ Medline ]
- Tran AQ, Nguyen LH, Nguyen HSA, Nguyen CT, Vu LG, Zhang M, et al. Determinants of intention to use artificial intelligence-based diagnosis support system among prospective physicians. Front Public Health. 2021;9:755644. [ FREE Full text ] [ CrossRef ] [ Medline ]
- Thrall JH, Li X, Li Q, Cruz C, Do S, Dreyer K, et al. Artificial intelligence and machine learning in radiology: opportunities, challenges, pitfalls, and criteria for success. J Am Coll Radiol. Mar 2018;15(3 Pt B):504-508. [ CrossRef ] [ Medline ]
- Haitjema S, Prescott TR, van Solinge WW. The applied data analytics in medicine program: lessons learned from four years' experience with personalizing health care in an academic teaching hospital. JMIR Form Res. Jan 28, 2022;6(1):e29333. [ CrossRef ] [ Medline ]
- Sutton RT, Pincock D, Baumgart DC, Sadowski DC, Fedorak RN, Kroeker KI. An overview of clinical decision support systems: benefits, risks, and strategies for success. NPJ Digit Med. 2020;3:17. [ FREE Full text ] [ CrossRef ] [ Medline ]
- Melton GB, McDonald CJ, Tang PC, Hripcsak G. Electronic health records. In: Shortliffe EH, Cimino JJ, editors. Biomedical Informatics. 5th Edition. Cham, Switzerland. Springer Nature; 2021:467-509.
- Abbasgholizadeh Rahimi S, Cwintal M, Huang Y, Ghadiri P, Grad R, Poenaru D, et al. Application of artificial intelligence in shared decision making: scoping review. JMIR Med Inform. Aug 09, 2022;10(8):e36199. [ FREE Full text ] [ CrossRef ] [ Medline ]
- Stai B, Heller N, McSweeney S, Rickman J, Blake P, Vasdev R, et al. Public perceptions of artificial intelligence and robotics in medicine. J Endourol. Oct 01, 2020;34(10):1041-1048. [ FREE Full text ] [ CrossRef ] [ Medline ]
- Asan O, Bayrak AE, Choudhury A. Artificial intelligence and human trust in healthcare: focus on clinicians. J Med Internet Res. Jun 19, 2020;22(6):e15154. [ FREE Full text ] [ CrossRef ] [ Medline ]
- Morley J, Machado CCV, Burr C, Cowls J, Joshi I, Taddeo M, et al. The ethics of AI in health care: a mapping review. Soc Sci Med. Sep 2020;260:113172. [ CrossRef ] [ Medline ]
- Gerke S, Minssen T, Cohen IG. Ethical and legal challenges of artificial intelligence-driven health care. SSRN Journal. 2020:295-336. [ CrossRef ]
- Lucivero F, Jongsma KR. A mobile revolution for healthcare? Setting the agenda for bioethics. J Med Ethics. Oct 2018;44(10):685-689. [ FREE Full text ] [ CrossRef ] [ Medline ]
- Schermer M. Telecare and self-management: opportunity to change the paradigm? J Med Ethics. Nov 2009;35(11):688-691. [ CrossRef ] [ Medline ]
- Fiske A, Buyx A, Prainsack B. The double-edged sword of digital self-care: physician perspectives from Northern Germany. Soc Sci Med. Sep 2020;260:113174. [ FREE Full text ] [ CrossRef ] [ Medline ]
- Groenhof TKJ. General discussion: how to make a learning healthcare system work? In: Towards a Learning Healthcare System. Enschede, The Netherlands. Gildeprint B.V; 2020:215-232.
- Attribution 4.0 International (CC BY 4.0). Creative Commons. URL: https://creativecommons.org/licenses/by/4.0/ [accessed 2024-05-16]
- Tucker I. AI cancer detectors. The Guardian. Jun 10, 2018. URL: https://www.theguardian.com/technology/2018/jun/10/artificial-intelligence-cancer-detectors-the-five [accessed 2024-03-06]
- Bardes CL. Defining "patient-centered medicine". N Engl J Med. Mar 01, 2012;366(9):782-783. [ CrossRef ] [ Medline ]
- Weston WW. Informed and shared decision-making: the crux of patient-centered care. CMAJ. Aug 21, 2001;165(4):438-439. [ FREE Full text ] [ Medline ]
- Reuben DB, Tinetti ME. Goal-oriented patient care--an alternative health outcomes paradigm. N Engl J Med. Mar 01, 2012;366(9):777-779. [ CrossRef ] [ Medline ]
- Kitson A, Marshall A, Bassett K, Zeitz K. What are the core elements of patient-centred care? A narrative review and synthesis of the literature from health policy, medicine and nursing. J Adv Nurs. Jan 2013;69(1):4-15. [ CrossRef ] [ Medline ]
- LaVeist TA, Isaac LA, Williams KP. Mistrust of health care organizations is associated with underutilization of health services. Health Serv Res. Dec 13, 2009;44(6):2093-2105. [ FREE Full text ] [ CrossRef ] [ Medline ]
- Jones GR, George JM. The experience and evolution of trust: implications for cooperation and teamwork. AMR. Jul 1998;23(3):531-546. [ CrossRef ]
- Gill H, Boies K, Finegan JE, McNally J. Antecedents of trust: establishing a boundary condition for the relation between propensity to trust and intention to trust. J Bus Psychol. Apr 2005;19(3):287-302. [ CrossRef ]
- Mechanic D, Schlesinger M. The impact of managed care on patients' trust in medical care and their physicians. JAMA. Jun 05, 1996;275(21):1693-1697. [ Medline ]
- Anderson LA, Dedrick RF. Development of the Trust in Physician scale: a measure to assess interpersonal trust in patient-physician relationships. Psychol Rep. Dec 1990;67(3 Pt 2):1091-1100. [ CrossRef ] [ Medline ]
- Emanuel EJ, Dubler NN. Preserving the physician-patient relationship in the era of managed care. JAMA. Jan 25, 1995;273(4):323-329. [ Medline ]
- Gray BH. Trust and trustworthy care in the managed care era. Health Aff (Millwood). Jan 1997;16(1):34-49. [ CrossRef ] [ Medline ]
- Caterinicchio RP. Testing plausible path models of interpersonal trust in patient-physician treatment relationships. Soc Sci Med. Part A: Med Psychol Med Sociol. Jan 1979;13:81-99. [ CrossRef ]
- Mechanic D. The functions and limitations of trust in the provision of medical care. J Health Polit Policy Law. Aug 1998;23(4):661-686. [ CrossRef ] [ Medline ]
- Thom DH, Campbell B. Patient-physician trust: an exploratory study. J Fam Pract. Feb 1997;44(2):169-176. [ Medline ]
- Mayer RC, Davis JH, Schoorman FD. An integrative model of organizational trust. AMR. Jul 1995;20(3):709. [ CrossRef ]
- Grimmelikhuijsen S, Knies E. Validating a scale for citizen trust in government organizations. Int Rev Adm Sci. Sep 03, 2015;83(3):583-601. [ CrossRef ]
- McEvily B, Tortoriello M. Measuring trust in organisational research: review and recommendations. J Trust Res. Apr 2011;1(1):23-63. [ CrossRef ]
- McKnight DH, Choudhury V, Kacmar C. Developing and validating trust measures for e-commerce: an integrative typology. ISR. Sep 2002;13(3):334-359. [ CrossRef ]
- van der Leeuw MS, Messelink MA, Tekstra J, Medina O, van Laar JM, Haitjema S, et al. Using real-world data to dynamically predict flares during tapering of biological DMARDs in rheumatoid arthritis: development, validation, and potential impact of prediction-aided decisions. Arthritis Res Ther. Mar 23, 2022;24(1):74. [ FREE Full text ] [ CrossRef ] [ Medline ]
- Pearson SD, Raeke LH. Patients' trust in physicians: many theories, few measures, and little data. J Gen Intern Med. Jul 2000;15(7):509-513. [ FREE Full text ] [ CrossRef ] [ Medline ]
- Kimberlin CL, Winterstein AG. Validity and reliability of measurement instruments used in research. Am J Health Syst Pharm. Dec 01, 2008;65(23):2276-2284. [ CrossRef ] [ Medline ]
- Gottems LBD, Carvalho EMPD, Guilhem D, Pires MRGM. Good practices in normal childbirth: reliability analysis of an instrument by Cronbach's Alpha. Rev Lat Am Enfermagem. May 17, 2018;26:e3000. [ FREE Full text ] [ CrossRef ] [ Medline ]
- IBM SPSS Statistics for Windows. IBM. URL: https://www.ibm.com/support/pages/downloading-ibm-spss-statistics-26 [accessed 2024-03-21]
- Qualtrics - een enquêtetool. Utrecht University. URL: https://students.uu.nl/qualtrics-een-enquetetool [accessed 2022-05-02]
- Yoda - a research data management service. Utrecht University. URL: https://www.uu.nl/en/research/yoda/about-yoda/why-yoda [accessed 2022-05-02]
- Cabitza F, Rasoini R, Gensini GF. Unintended consequences of machine learning in medicine. JAMA. Aug 08, 2017;318(6):517-518. [ CrossRef ] [ Medline ]
- Belfrage S, Helgesson G, Lynøe N. Trust and digital privacy in healthcare: a cross-sectional descriptive study of trust and attitudes towards uses of electronic health data among the general public in Sweden. BMC Med Ethics. Mar 04, 2022;23(1):19. [ FREE Full text ] [ CrossRef ] [ Medline ]
- Haselager DR, Boers SN, Jongsma KR, Vinkers CH, Broekman ML, Bredenoord AL. Breeding brains? Patients' and laymen's perspectives on cerebral organoids. Regen Med. Dec 2020;15(12):2351-2360. [ CrossRef ] [ Medline ]
- Yakar D, Ongena YP, Kwee TC, Haan M. Do people favor artificial intelligence over physicians? A survey among the general population and their view on artificial intelligence in medicine. Value Health. Mar 2022;25(3):374-381. [ FREE Full text ] [ CrossRef ] [ Medline ]
- Kaplan AD, Kessler TT, Brill JC, Hancock PA. Trust in artificial intelligence: meta-analytic findings. Hum Factors. Mar 28, 2023;65(2):337-359. [ FREE Full text ] [ CrossRef ] [ Medline ]
- Paul K. Disastrous lack of diversity in AI industry perpetuates bias, study finds. The Guardian. URL: https://www.theguardian.com/technology/2019/apr/16/artificial-intelligence-lack-diversity-new-york-university-study [accessed 2022-09-08]
- Cirillo D, Catuara-Solarz S, Morey C, Guney E, Subirats L, Mellino S, et al. Sex and gender differences and biases in artificial intelligence for biomedicine and healthcare. NPJ Digit Med. 2020;3:81. [ FREE Full text ] [ CrossRef ] [ Medline ]
- Parikh RB, Teeple S, Navathe AS. Addressing bias in artificial intelligence in health care. JAMA. Dec 24, 2019;322(24):2377-2378. [ CrossRef ] [ Medline ]
- van Diemen J, Verdonk P, Chieffo A, Regar E, Mauri F, Kunadian V, et al. The importance of achieving sex- and gender-based equity in clinical trials: a call to action. Eur Heart J. Aug 17, 2021;42(31):2990-2994. [ FREE Full text ] [ CrossRef ] [ Medline ]
- Gille F, Jobin A, Ienca M. What we talk about when we talk about trust: theory of trust for AI in healthcare. Intell -Based Med. Nov 2020;1-2:100001. [ CrossRef ]
- Krupnikov Y, Levine A. Expressing versus revealing preferences in experimental research. In: Sourcebook for Political Communication Research. 1st Edition. London, UK. Routledge; 2010:9780203938669.
- Blom-Hansen J, Morton R, Serritzlew S. Experiments in public management research. IPMJ. Mar 11, 2015;18(2):151-170. [ CrossRef ]
- Oppenheimer DM, Meyvis T, Davidenko N. Instructional manipulation checks: detecting satisficing to increase statistical power. J Exp Soc Psychol. Jul 2009;45(4):867-872. [ CrossRef ]
Abbreviations
Edited by Y Zhuang; submitted 17.07.23; peer-reviewed by Z Su, E Vashishtha, PH Liao, P Velmovitsky; comments to author 26.01.24; revised version received 21.03.24; accepted 16.04.24; published 28.05.24.
©Anna G M Zondag, Raoul Rozestraten, Stephan G Grimmelikhuijsen, Karin R Jongsma, Wouter W van Solinge, Michiel L Bots, Robin W M Vernooij, Saskia Haitjema. Originally published in the Journal of Medical Internet Research (https://www.jmir.org), 28.05.2024.
This is an open-access article distributed under the terms of the Creative Commons Attribution License (https://creativecommons.org/licenses/by/4.0/), which permits unrestricted use, distribution, and reproduction in any medium, provided the original work, first published in the Journal of Medical Internet Research, is properly cited. The complete bibliographic information, a link to the original publication on https://www.jmir.org/, as well as this copyright and license information must be included.

IMAGES
VIDEO
COMMENTS
A case-control study is a type of observational study commonly used to look at factors associated with diseases or outcomes.[1] The case-control study starts with a group of cases, which are the individuals who have the outcome of interest. The researcher then tries to construct a second group of individuals called the controls, who are similar to the case individuals but do not have the ...
Case-control studies are a type of observational study often used in fields like medical research, environmental health, or epidemiology. While most observational studies are qualitative in nature, case-control studies can also be quantitative, and they often are in healthcare settings. Case-control studies can be used for both exploratory and ...
A case-control study is a type of observational study commonly used to look at factors associated with diseases or outcomes. The case-control study starts with a group of cases, which are the individuals who have the outcome of interest. The researcher then tries to construct a second group of individuals called the controls, who are similar to ...
Case-control studies are one of the major observational study designs for performing clinical research. The advantages of these study designs over other study designs are that they are relatively quick to perform, economical, and easy to design and implement. Case-control studies are particularly appropriate for studying disease outbreaks, rare diseases, or outcomes of interest. This article ...
A case-control study cannot estimate incidence or prevalence rates for the disease. The data from these studies do not allow you to calculate the probability of a new person contracting the condition in a given period nor how common it is in the population. This limitation occurs because case-control studies do not use a representative sample.
A case-control study (also known as case-referent study) is a type of observational study in which two existing groups differing in outcome are identified and compared on the basis of some supposed causal attribute. Case-control studies are often used to identify factors that may contribute to a medical condition by comparing subjects who have the condition with patients who do not have ...
Characteristics of Case-Control Studies. How do case-control studies fit into classifications of research design described in an earlier article? 1 Case-control studies are empirical studies that are based on samples, not individual cases or case series. They are cross-sectional because cases and controls are identified and evaluated for caseness, historical exposures, and confounding ...
A case-control study is a type of medical research investigation often used to help determine the cause of a disease, particularly when investigating a disease outbreak or rare condition.
General Overview of Case-Control Studies. In observational studies, also called epidemiologic studies, the primary objective is to discover and quantify an association between exposures and the outcome of interest, in hopes of drawing causal inference. Observational studies can have a retrospective study design, a prospective design, a cross ...
A case-control study is designed to help determine if an exposure is associated with an outcome (i.e., disease or condition of interest). In theory, the case-control study can be described simply. First, identify the cases (a group known to have the outcome) and the controls (a group known to be free of the outcome).
Introduction. Case-control and cohort studies are observational studies that lie near the middle of the hierarchy of evidence. These types of studies, along with randomised controlled trials, constitute analytical studies, whereas case reports and case series define descriptive studies (1). Although these studies are not ranked as highly as ...
Case-control studies compare groups retrospectively, while longitudinal studies can compare groups either retrospectively or prospectively. In a longitudinal study, researchers monitor a population over an extended period of time, and they can be used to study developmental shifts and understand how certain things change as we age. In addition, case-control studies look at a single subject or ...
Matching is often used in case-control control studies to ensure that the cases and controls are similar in certain characteristics. For example, in the smoking and lung cancer study, the authors selected controls that were similar in age and sex to carcinoma cases. Matching is a useful technique to increase the efficiency of study.
Since case-control studies are often used for uncommon outcomes, investigators often have a limited number of cases but a plentiful supply of potential controls. In this situation the statistical power of the study can be increased somewhat by enrolling more controls than cases. However, the additional power that is achieved diminishes as the ...
The goal of a case-control study is the same as that of cohort studies, i.e. to estimate the magnitude of association between an exposure and an outcome. However, case-control studies employ a different sampling strategy that gives them greater efficiency. As with a cohort study, a case-control study attempts to identify all people who have ...
Case-control studies are one of the major observational study designs for performing clinical research. The advantages of these study designs over other study designs are that they are relatively quick to perform, economical, and easy to design and implement. Case-control studies are particularly appropriate for studying disease outbreaks, rare ...
Case-Control Studies The case-control approach is a powerful method for investigating factors that may explain a particular event. It is extensively used in epidemiology to study disease incidence, one of the best-known examples being the investigation by Bradford Hill and Doll of the possible connection between cigarette smoking and lung ...
Some case-control studies to test this have taken referents from the general population, whereas others have used patients with other types of cancer. Studies using controls from the general population will tend to overestimate risk because of differential recall, whereas studies using patients with other types of cancers as controls will ...
Case-control studies are used to determine if there is an association between an exposure and a specific health outcome. These studies proceed from effect (e.g. health outcome, condition, disease) to cause (exposure). Case-control studies assess whether exposure is
Case-control studies are particularly useful for studying the cause of an outcome that is rare and for studying the effects of prolonged exposure. For example, a case-control study could be used ...
The case-control study can be subcategorized into four different subtypes based on how the control group is selected and when the cases develop the disease of interest as described in the following sections. Nested Case-Control Study. When a case-control study is performed within a cohort study, it is called a nested case-control study.
Most field case-control studies use control-to-case-patient ratios of 1:1, 2:1, or 3:1. Enrolling more than one control per case-patient can increase study power, which might be needed to detect a statistically significant difference in exposure between case-patients and controls, particularly when an outbreak involves a limited number of cases.
For this purpose a retrospective case-control study using a random proportional sample of Armenian children with ASD to assess associations between various factors and ASD was conducted. The ...
A case-control study is an observational study design commonly used in epidemiology to identify factors associated with a particular disease or outcome. The key elements include: Retrospective Design: This design involves looking backward from the outcome (disease) to the exposure. Researchers start by identifying individuals who have the ...
Findings: In a case-control study of over 450,000 individuals, the 15 genetic variants from candidate genes collectively accounted for 0.4% of the variation in opioid use disorder risk. In this independent sample, the SNPs predicted risk at a level of accuracy near random chance (52.8%).
In a case-control study, a number of cases and noncases (controls) are identified, and the occurrence of one or more prior exposures is compared between groups to evaluate drug-outcome associations ( Figure 1 ). A case-control study runs in reverse relative to a cohort study. 21 As such, study inception occurs when a patient experiences ...
Bovine anaplasmosis (BA) is a costly disease affecting the U.S. beef cattle industry. Chlortetracycline (CTC) in feed or mineral supplements is often used to control clinical signs of BA. The objective of this study was to determine if management practices, such as feeding CTC, are associated with illness or death from BA in Mississippi cow-calf herds.
Case-control studies are one of the most frequently used study designs for these purposes. This paper explains basic features of case control studies, rationality behind applying case control design with appropriate examples and limitations of this design. Analysis of sensitivity and specificity along with template to calculate various ratios ...
Background: Clinical decision support systems (CDSSs) based on routine care data, using artificial intelligence (AI), are increasingly being developed. Previous studies focused largely on the technical aspects of using AI, but the acceptability of these technologies by patients remains unclear. Objective: We aimed to investigate whether patient-physician trust is affected when medical decision ...